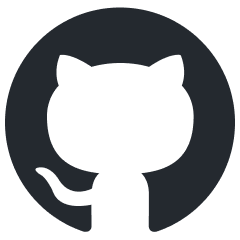
intel-extension-for-tensorflow
Intel® Extension for TensorFlow*
Stars: 305
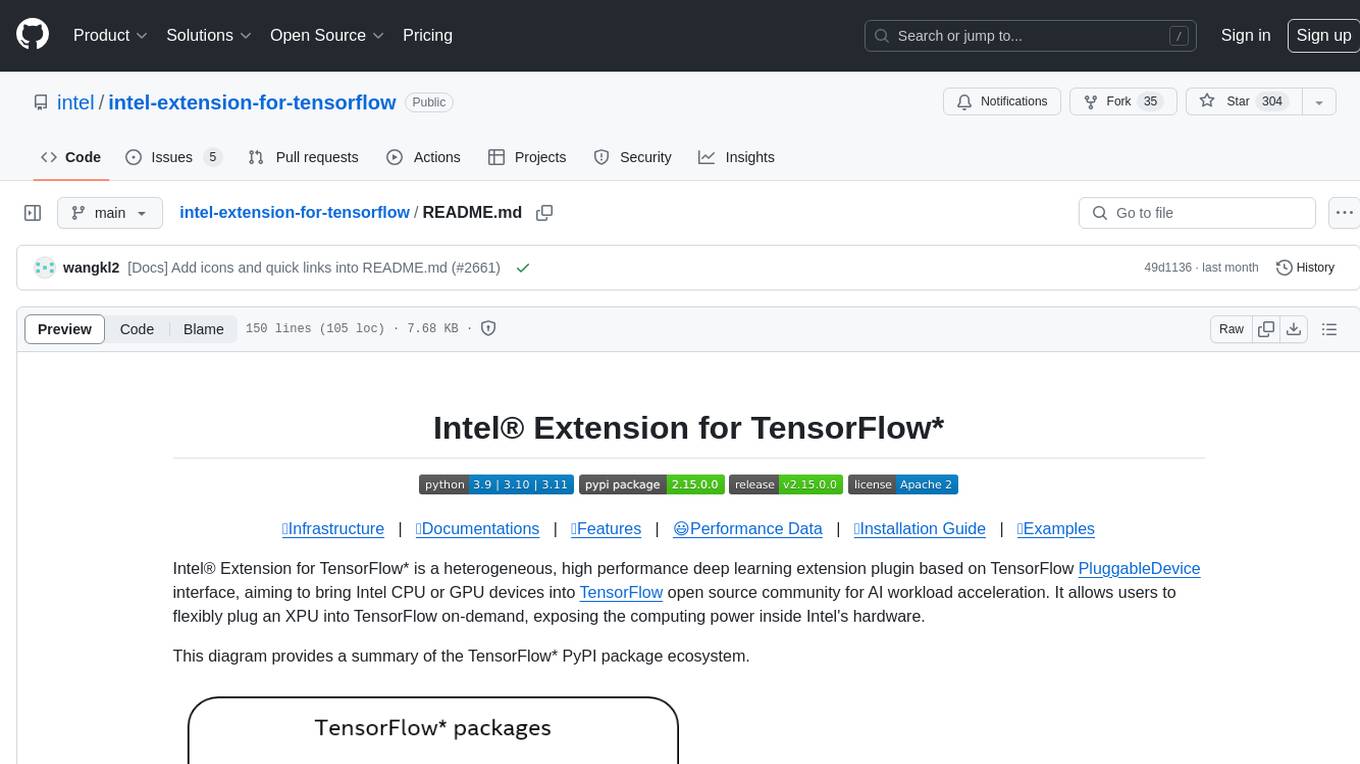
Intel® Extension for TensorFlow* is a high performance deep learning extension plugin based on TensorFlow PluggableDevice interface. It aims to accelerate AI workloads by allowing users to plug Intel CPU or GPU devices into TensorFlow on-demand, exposing the computing power inside Intel's hardware. The extension provides XPU specific implementation, kernels & operators, graph optimizer, device runtime, XPU configuration management, XPU backend selection, and options for turning on/off advanced features.
README:
Intel® Extension for TensorFlow* is a heterogeneous, high performance deep learning extension plugin based on TensorFlow PluggableDevice interface, aiming to bring Intel CPU or GPU devices into TensorFlow open source community for AI workload acceleration. It allows users to flexibly plug an XPU into TensorFlow on-demand, exposing the computing power inside Intel's hardware.
This diagram provides a summary of the TensorFlow* PyPI package ecosystem.
-
TensorFlow PyPI packages: estimator, keras, tensorboard, tensorflow-base
-
Intel® Extension for TensorFlow* package:
intel_extension_for_tensorflow
contains:- XPU specific implementation
- Kernels & operators
- Graph optimizer
- Device runtime
- XPU configuration management
- XPU backend selection
- Options turning on/off advanced features
- XPU specific implementation
Intel® Extension for TensorFlow* provides Intel XPU and Intel CPU support.
Package | CPU | XPU | Installation |
---|---|---|---|
Intel GPU driver | Y | Install Intel GPU driver | |
Intel® oneAPI Base Toolkit | Y | Install Intel® oneAPI Base Toolkit | |
TensorFlow | Y | Y | Install TensorFlow 2.15.0 |
Intel® Extension for TensorFlow* can be installed through the following channels:
- PyPI: XPU \ CPU
- DockerHub: XPU Container \ CPU Container
- Source: Build from source
Intel® Extension for TensorFlow* | Stock TensorFlow |
---|---|
latest build from source | 2.15 |
v2.14.0.1 & v2.14.0.2 | 2.14 |
v2.13.0.0 | 2.13 |
v1.2.0 | 2.12 |
v1.1.0 | 2.10 & 2.11 |
v1.0.0 | 2.10 |
pip install --upgrade intel-extension-for-tensorflow[xpu]
Environment check instructions for XPU:
export path_to_site_packages=`python -c "import site; print(site.getsitepackages()[0])"`
bash ${path_to_site_packages}/intel_extension_for_tensorflow/tools/env_check.sh
Refer to XPU installation for details.
pip install --upgrade intel-extension-for-tensorflow[cpu]
Sanity check instructions:
python -c "import intel_extension_for_tensorflow as itex; print(itex.__version__)"
pip install --upgrade intel-extension-for-tensorflow-weekly[xpu] -f https://developer.intel.com/itex-whl-weekly
Environment check instructions for GPU weekly:
export path_to_site_packages=`python -c "import site; print(site.getsitepackages()[0])"`
bash ${path_to_site_packages}/intel_extension_for_tensorflow/tools/env_check.sh
pip install --upgrade intel-extension-for-tensorflow-weekly[cpu] -f https://developer.intel.com/itex-whl-weekly
Sanity check instructions:
python -c "import intel_extension_for_tensorflow as itex; print(itex.__version__)"
Visit the online document website, and then get started with a tour of Intel® Extension for TensorFlow* examples.
We welcome community contributions to Intel® Extension for TensorFlow*.
This project is intended to be a safe, welcoming space for collaboration, and contributors are expected to adhere to the Contributor Covenant. Please see contribution guidelines for additional details.
- TensorFlow GPU device plugins
- Accelerating TensorFlow on Intel® Data Center GPU Flex Series
- Meet the Innovation of Intel AI Software: Intel® Extension for TensorFlow*
- Efficient TensorFlow Distributed Training on Intel Data Center GPU Max Series
- Accelerate JAX models on Intel GPUs via PJRT
- Running TensorFlow Stable Diffusion on Intel Arc GPUs
- AI workload Acceleration with Intel® Extension for TensorFlow* | Intel Software
Submit your questions, feature requests, and bug reports on the GitHub issues page.
See Intel's Security Center for information on how to report a potential security issue or vulnerability.
See also: Security Policy
This distribution includes third party software governed by separate license terms. This third party software, even if included with the distribution of the Intel software, may be governed by separate license terms, including without limitation, third party license terms, other Intel software license terms, and open source software license terms. These separate license terms govern your use of the third party programs as set forth in the "THIRD-PARTY-PROGRAMS" file.
For Tasks:
Click tags to check more tools for each tasksFor Jobs:
Alternative AI tools for intel-extension-for-tensorflow
Similar Open Source Tools
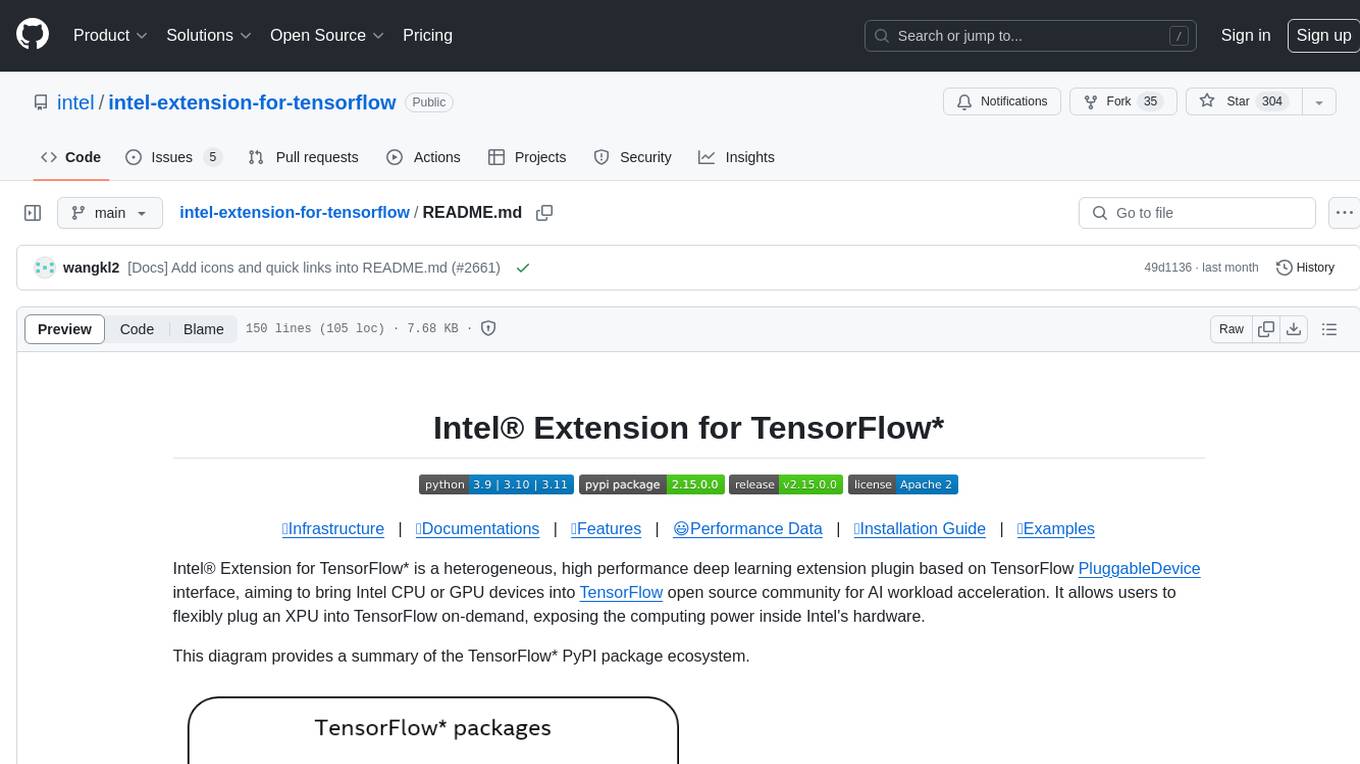
intel-extension-for-tensorflow
Intel® Extension for TensorFlow* is a high performance deep learning extension plugin based on TensorFlow PluggableDevice interface. It aims to accelerate AI workloads by allowing users to plug Intel CPU or GPU devices into TensorFlow on-demand, exposing the computing power inside Intel's hardware. The extension provides XPU specific implementation, kernels & operators, graph optimizer, device runtime, XPU configuration management, XPU backend selection, and options for turning on/off advanced features.
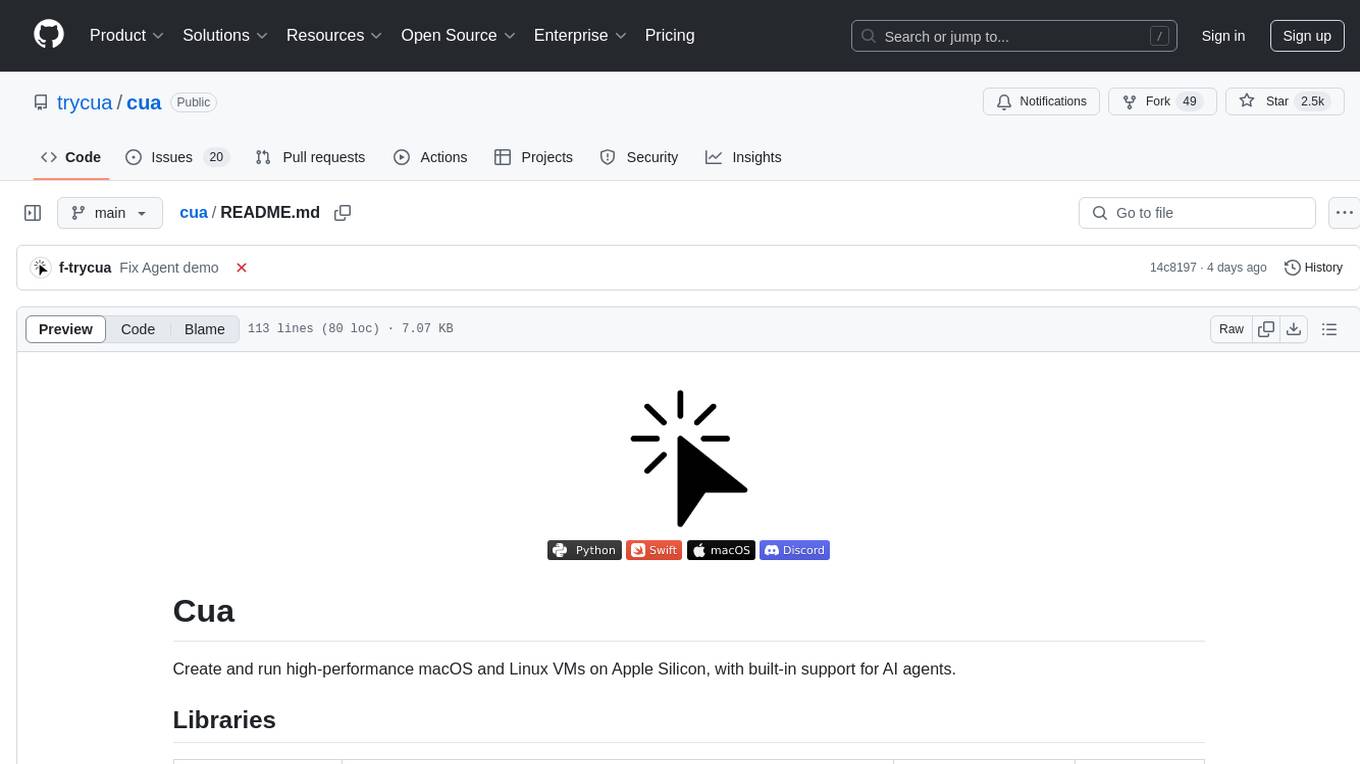
cua
Cua is a tool for creating and running high-performance macOS and Linux virtual machines on Apple Silicon, with built-in support for AI agents. It provides libraries like Lume for running VMs with near-native performance, Computer for interacting with sandboxes, and Agent for running agentic workflows. Users can refer to the documentation for onboarding, explore demos showcasing AI-Gradio and GitHub issue fixing, and utilize accessory libraries like Core, PyLume, Computer Server, and SOM. Contributions are welcome, and the tool is open-sourced under the MIT License.
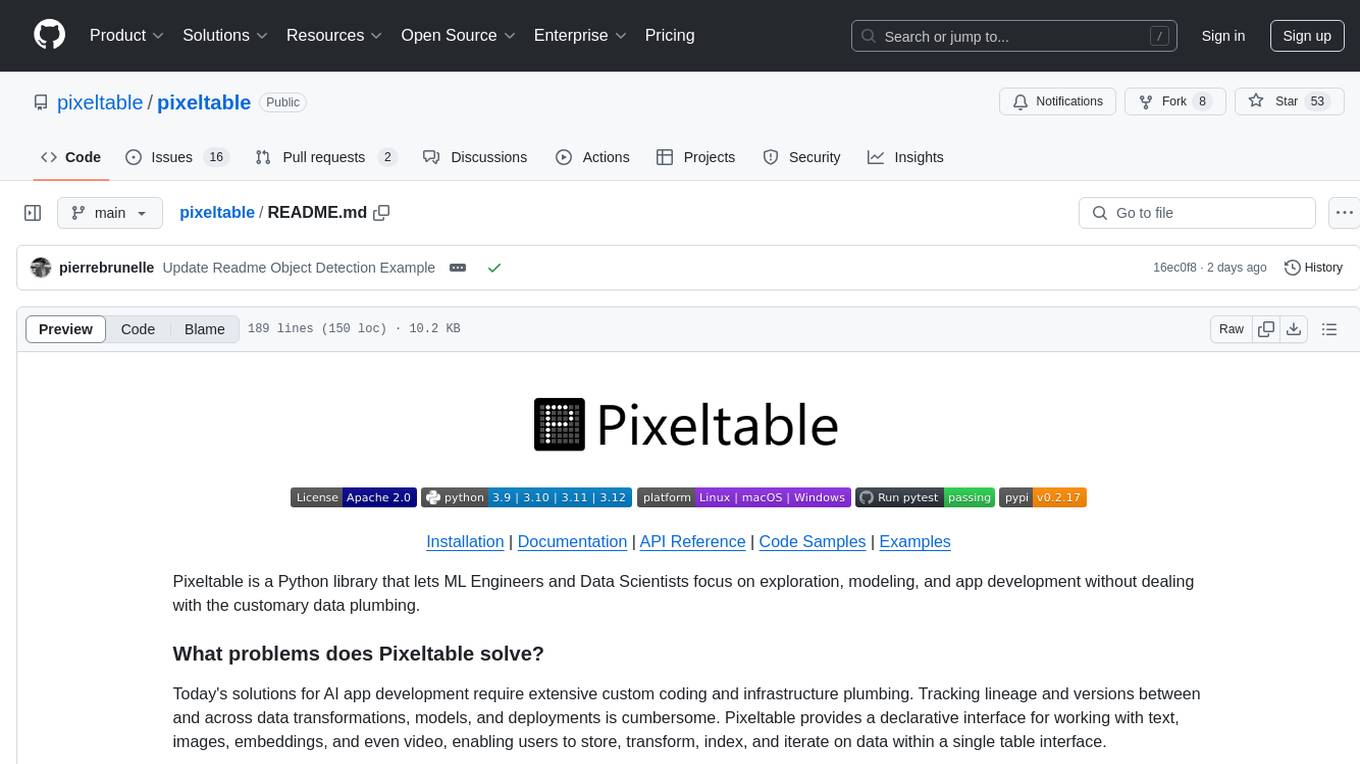
pixeltable
Pixeltable is a Python library designed for ML Engineers and Data Scientists to focus on exploration, modeling, and app development without the need to handle data plumbing. It provides a declarative interface for working with text, images, embeddings, and video, enabling users to store, transform, index, and iterate on data within a single table interface. Pixeltable is persistent, acting as a database unlike in-memory Python libraries such as Pandas. It offers features like data storage and versioning, combined data and model lineage, indexing, orchestration of multimodal workloads, incremental updates, and automatic production-ready code generation. The tool emphasizes transparency, reproducibility, cost-saving through incremental data changes, and seamless integration with existing Python code and libraries.
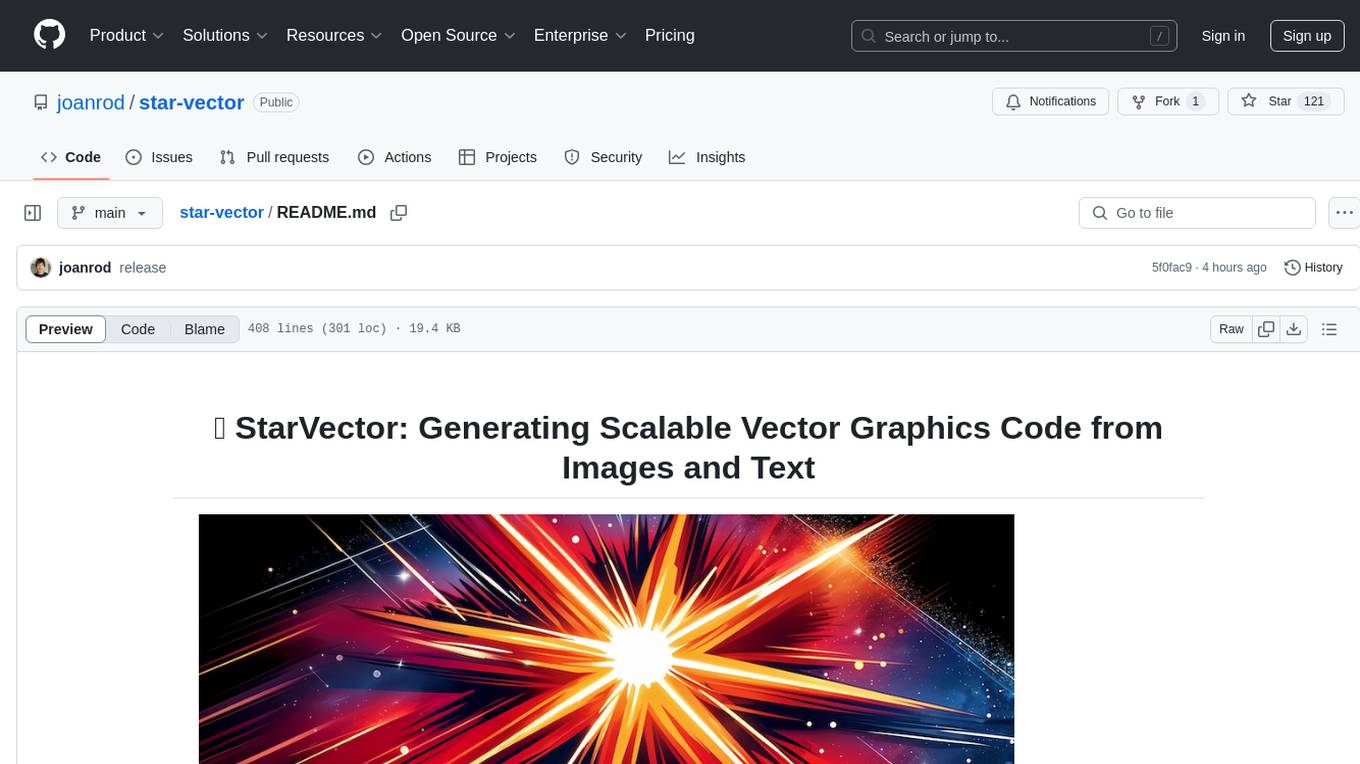
star-vector
StarVector is a multimodal vision-language model for Scalable Vector Graphics (SVG) generation. It can be used to perform image2SVG and text2SVG generation. StarVector works directly in the SVG code space, leveraging visual understanding to apply accurate SVG primitives. It achieves state-of-the-art performance in producing compact and semantically rich SVGs. The tool provides Hugging Face model checkpoints for image2SVG vectorization, with models like StarVector-8B and StarVector-1B. It also offers datasets like SVG-Stack, SVG-Fonts, SVG-Icons, SVG-Emoji, and SVG-Diagrams for evaluation. StarVector can be trained using Deepspeed or FSDP for tasks like Image2SVG and Text2SVG generation. The tool provides a demo with options for HuggingFace generation or VLLM backend for faster generation speed.
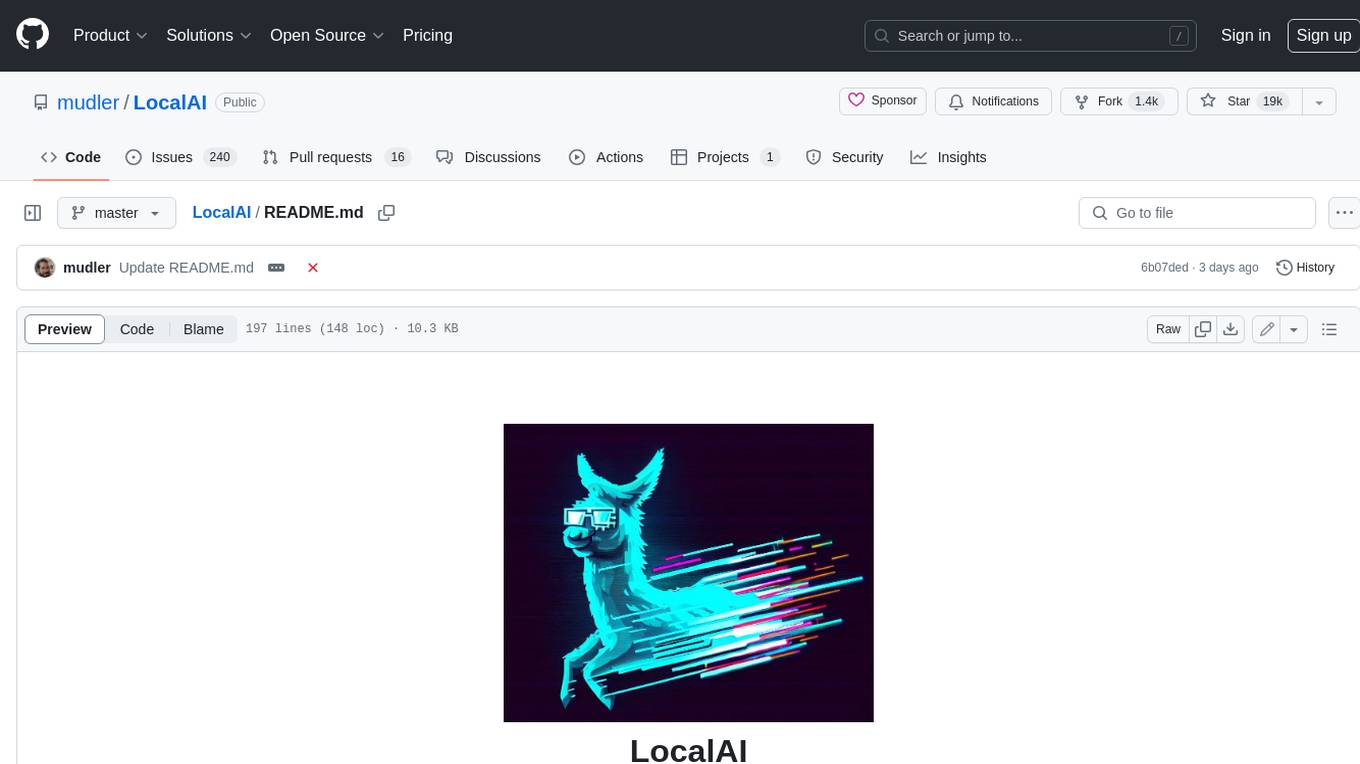
LocalAI
LocalAI is a free and open-source OpenAI alternative that acts as a drop-in replacement REST API compatible with OpenAI (Elevenlabs, Anthropic, etc.) API specifications for local AI inferencing. It allows users to run LLMs, generate images, audio, and more locally or on-premises with consumer-grade hardware, supporting multiple model families and not requiring a GPU. LocalAI offers features such as text generation with GPTs, text-to-audio, audio-to-text transcription, image generation with stable diffusion, OpenAI functions, embeddings generation for vector databases, constrained grammars, downloading models directly from Huggingface, and a Vision API. It provides a detailed step-by-step introduction in its Getting Started guide and supports community integrations such as custom containers, WebUIs, model galleries, and various bots for Discord, Slack, and Telegram. LocalAI also offers resources like an LLM fine-tuning guide, instructions for local building and Kubernetes installation, projects integrating LocalAI, and a how-tos section curated by the community. It encourages users to cite the repository when utilizing it in downstream projects and acknowledges the contributions of various software from the community.
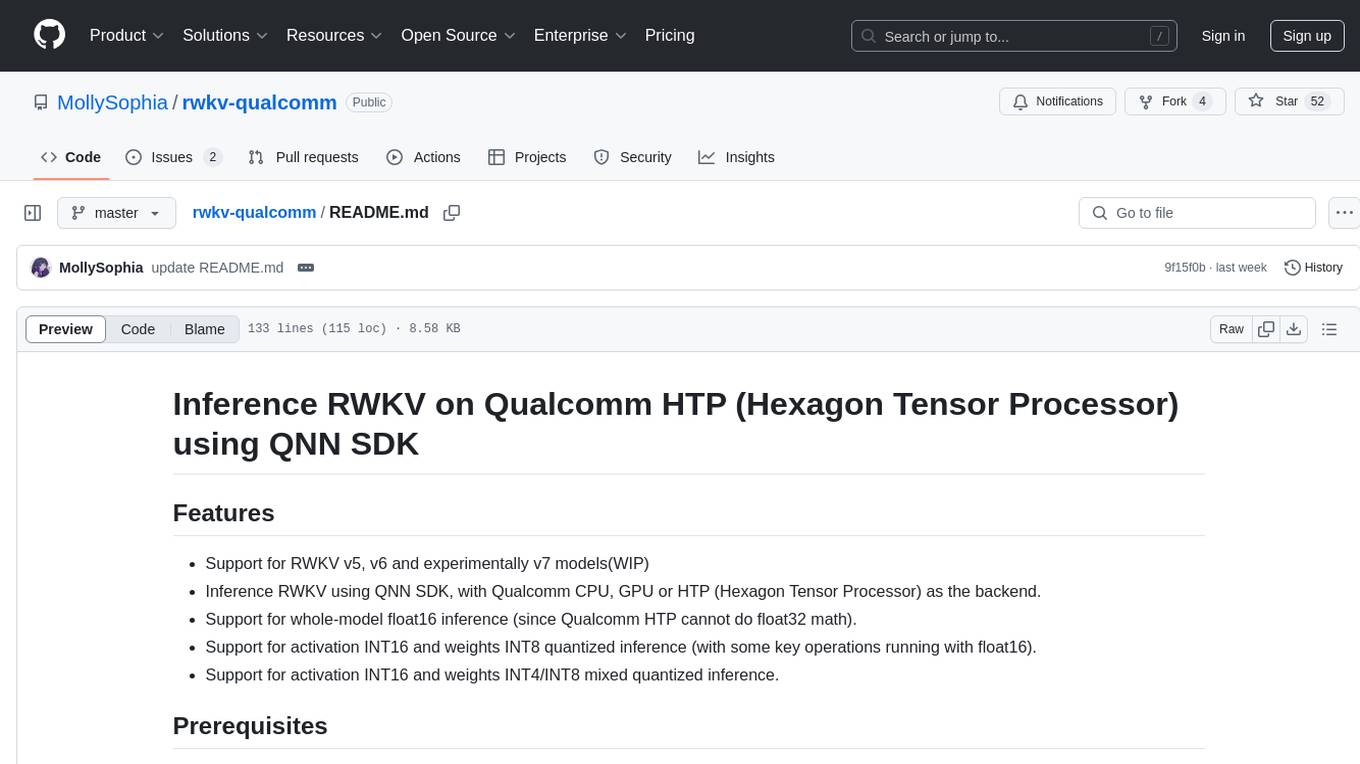
rwkv-qualcomm
This repository provides support for inference RWKV models on Qualcomm HTP (Hexagon Tensor Processor) using QNN SDK. It supports RWKV v5, v6, and experimentally v7 models, inference using Qualcomm CPU, GPU, or HTP as the backend, whole-model float16 inference, activation INT16 and weights INT8 quantized inference, and activation INT16 and weights INT4/INT8 mixed quantized inference. Users can convert model weights to QNN model library files, generate HTP context cache, and run inference on Qualcomm Snapdragon SM8650 with HTP v75. The project requires QNN SDK, AIMET toolkit, and specific hardware for verification.
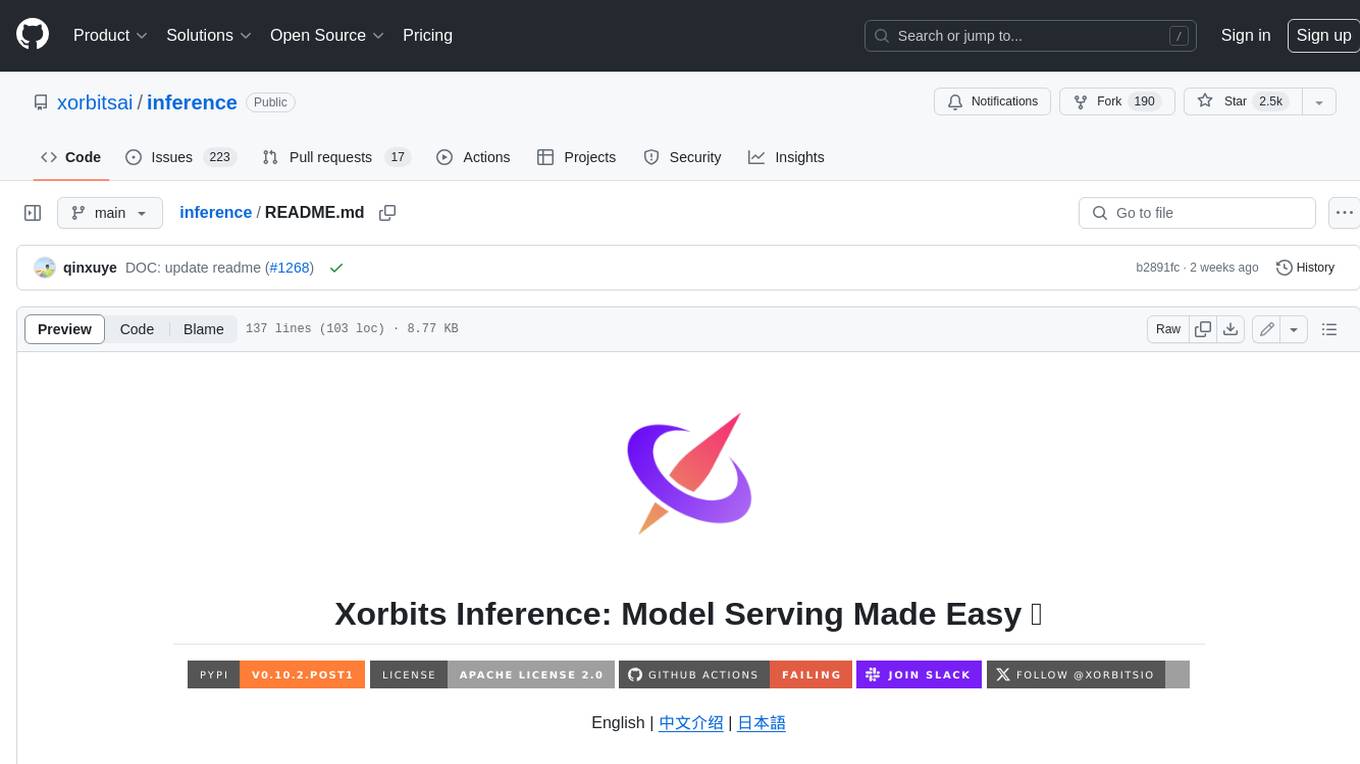
inference
Xorbits Inference (Xinference) is a powerful and versatile library designed to serve language, speech recognition, and multimodal models. With Xorbits Inference, you can effortlessly deploy and serve your or state-of-the-art built-in models using just a single command. Whether you are a researcher, developer, or data scientist, Xorbits Inference empowers you to unleash the full potential of cutting-edge AI models.
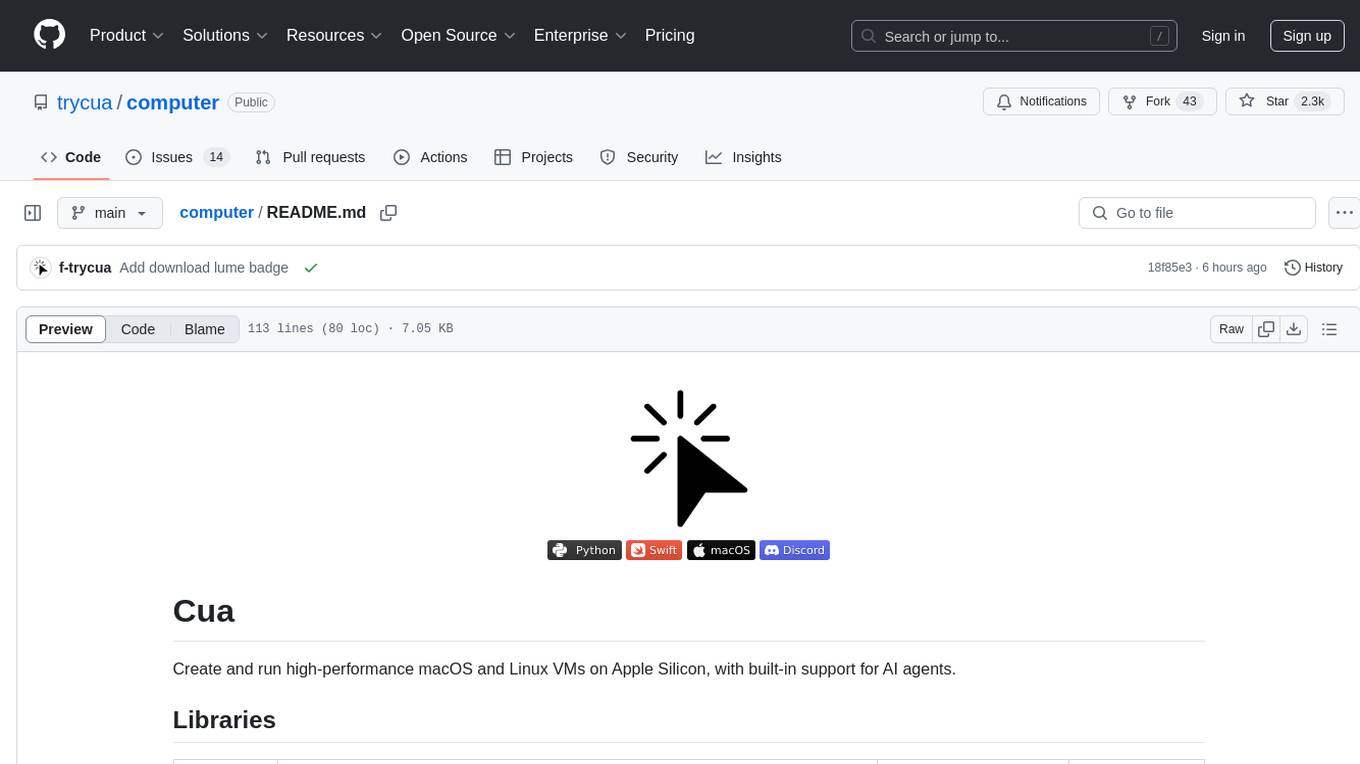
computer
Cua is a tool for creating and running high-performance macOS and Linux VMs on Apple Silicon, with built-in support for AI agents. It provides libraries like Lume for running VMs with near-native performance, Computer for interacting with sandboxes, and Agent for running agentic workflows. Users can refer to the documentation for onboarding and explore demos showcasing the tool's capabilities. Additionally, accessory libraries like Core, PyLume, Computer Server, and SOM offer additional functionality. Contributions to Cua are welcome, and the tool is open-sourced under the MIT License.
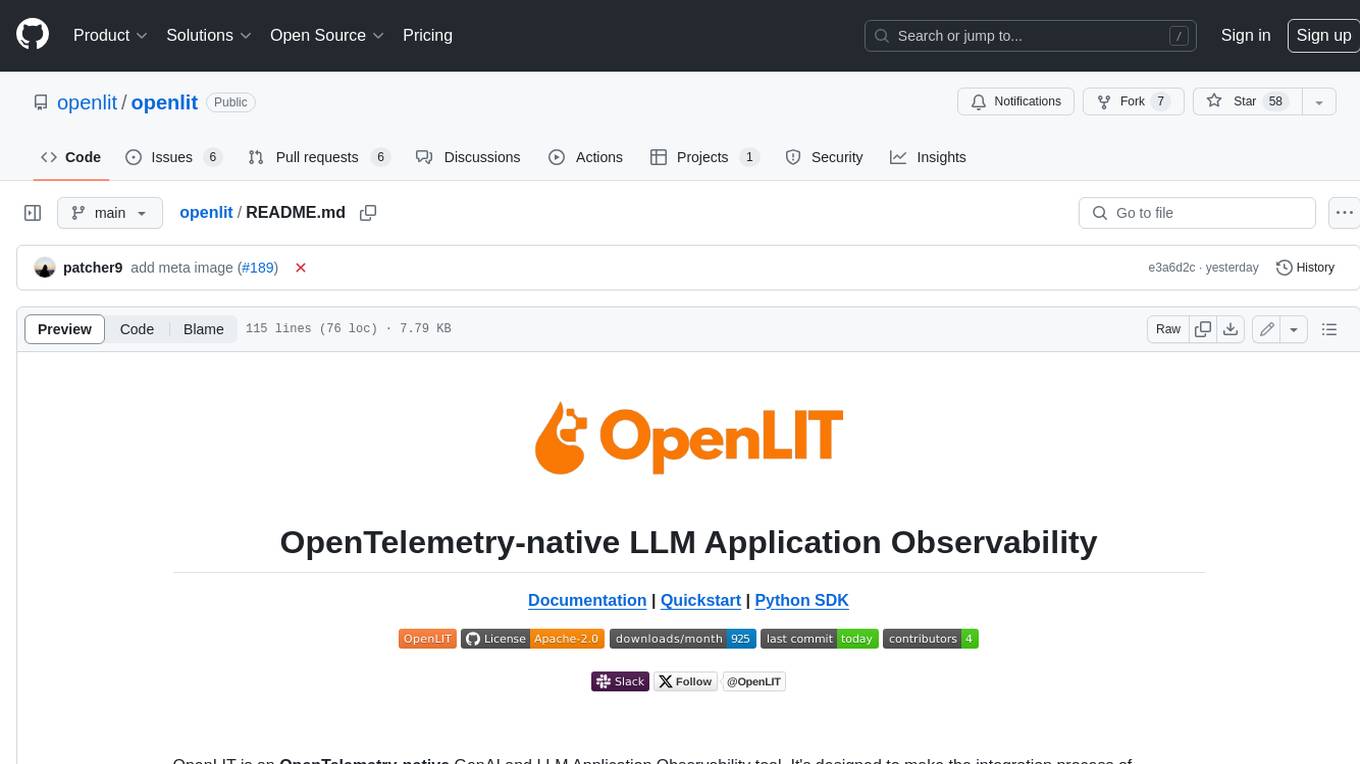
openlit
OpenLIT is an OpenTelemetry-native GenAI and LLM Application Observability tool. It's designed to make the integration process of observability into GenAI projects as easy as pie – literally, with just **a single line of code**. Whether you're working with popular LLM Libraries such as OpenAI and HuggingFace or leveraging vector databases like ChromaDB, OpenLIT ensures your applications are monitored seamlessly, providing critical insights to improve performance and reliability.
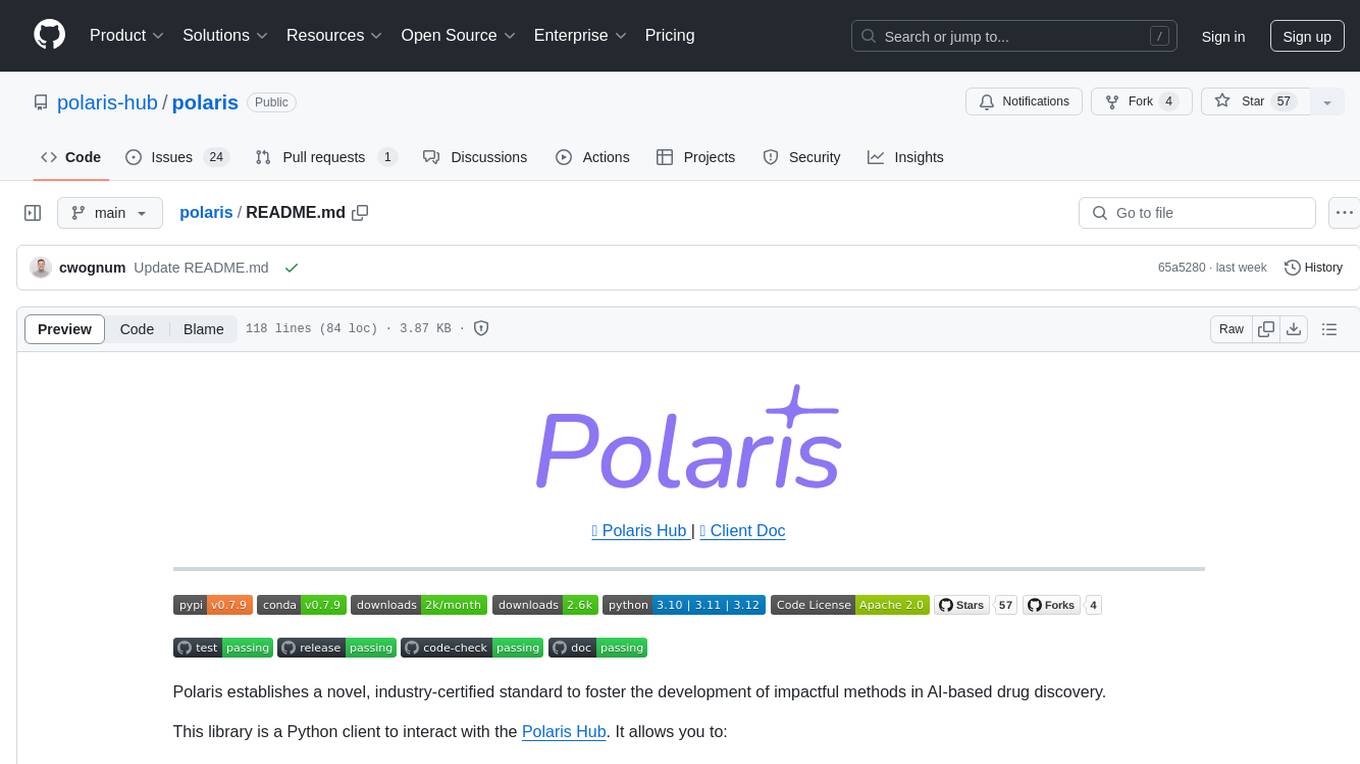
polaris
Polaris establishes a novel, industry‑certified standard to foster the development of impactful methods in AI-based drug discovery. This library is a Python client to interact with the Polaris Hub. It allows you to download Polaris datasets and benchmarks, evaluate a custom method against a Polaris benchmark, and create and upload new datasets and benchmarks.
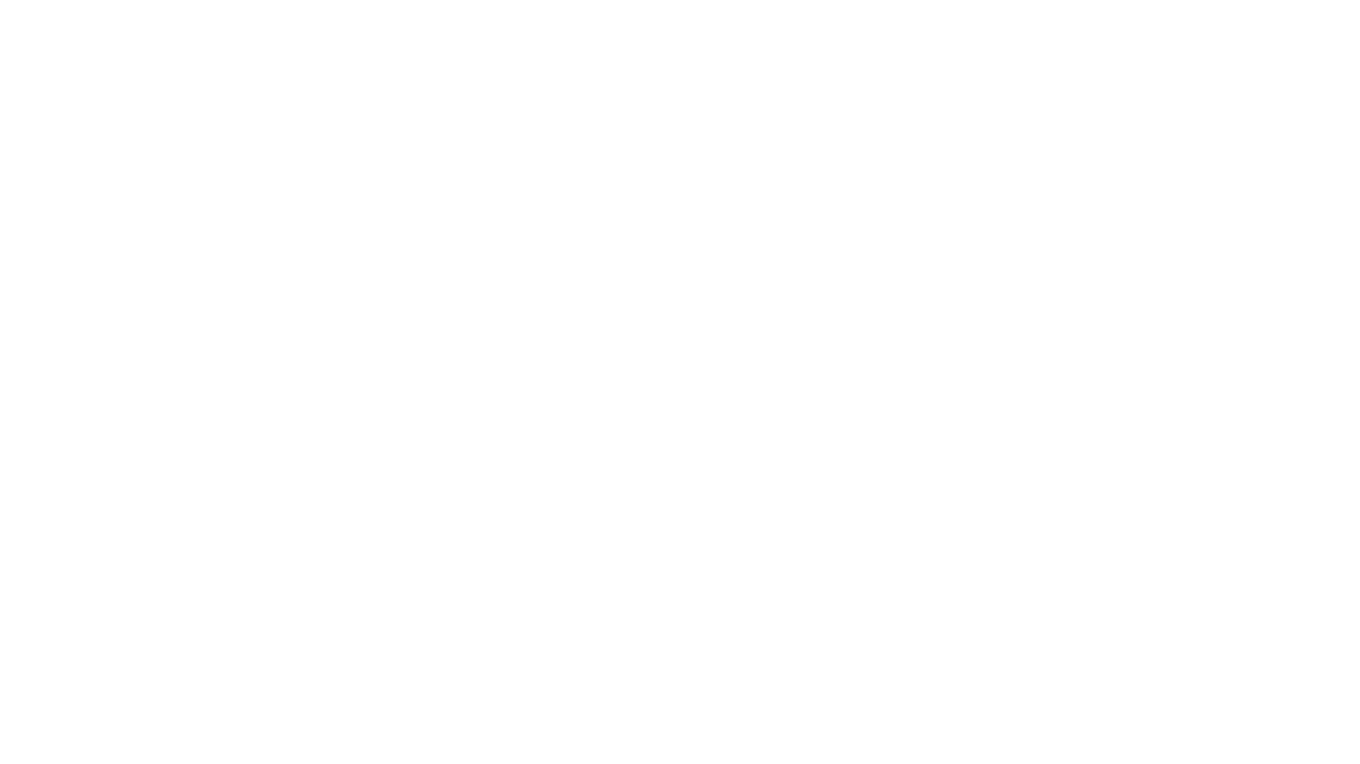
auto-news
Auto-News is an automatic news aggregator tool that utilizes Large Language Models (LLM) to pull information from various sources such as Tweets, RSS feeds, YouTube videos, web articles, Reddit, and journal notes. The tool aims to help users efficiently read and filter content based on personal interests, providing a unified reading experience and organizing information effectively. It features feed aggregation with summarization, transcript generation for videos and articles, noise reduction, task organization, and deep dive topic exploration. The tool supports multiple LLM backends, offers weekly top-k aggregations, and can be deployed on Linux/MacOS using docker-compose or Kubernetes.
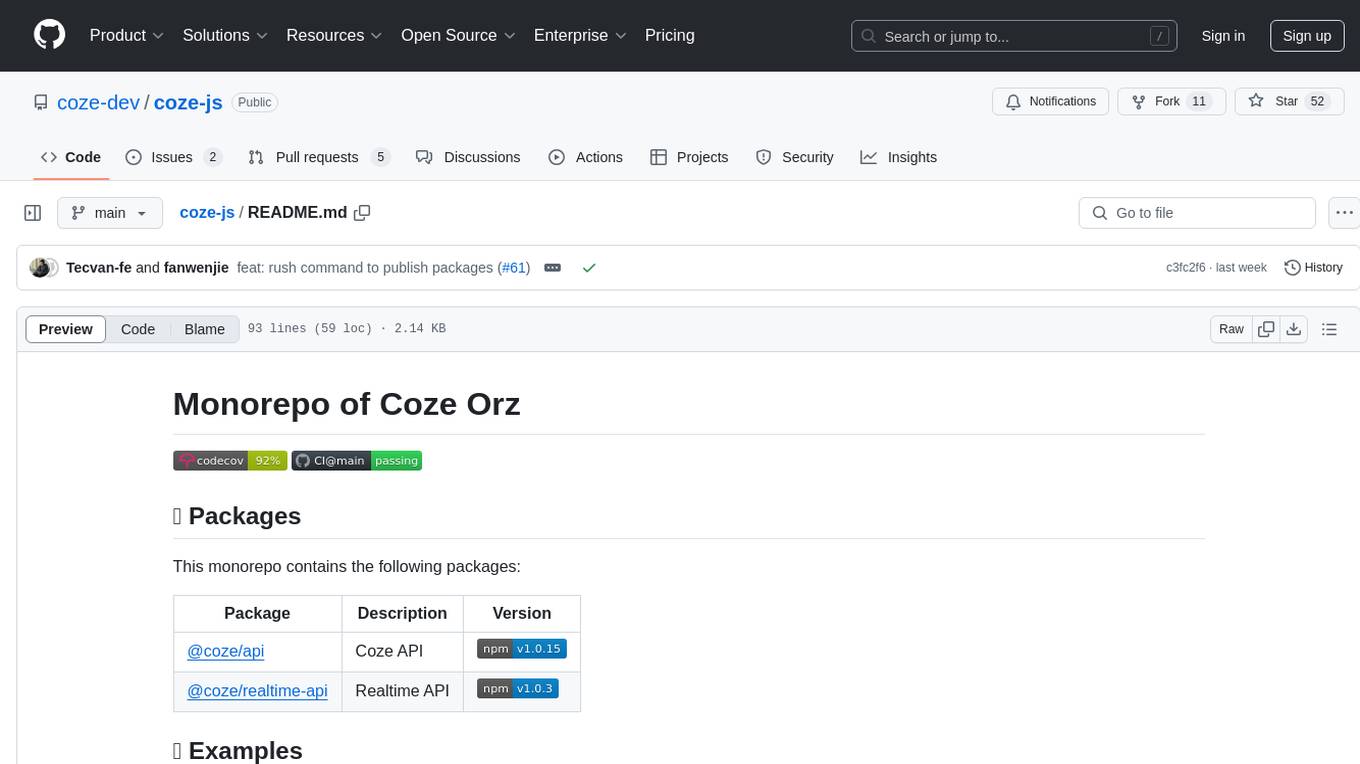
coze-js
Coze-js is a monorepo containing packages for Coze API and Realtime API. It provides usage examples for Node.js and React Web, as well as full console and sample call up demos. The tool requires Node.js 18+, pnpm 9.12.0, and Rush 5.140.0 for installation. Developers can start developing projects within the repository by following the provided steps. Each package in the monorepo can be developed and published independently, with documentation on contributing guidelines and publishing. The tool is licensed under MIT.
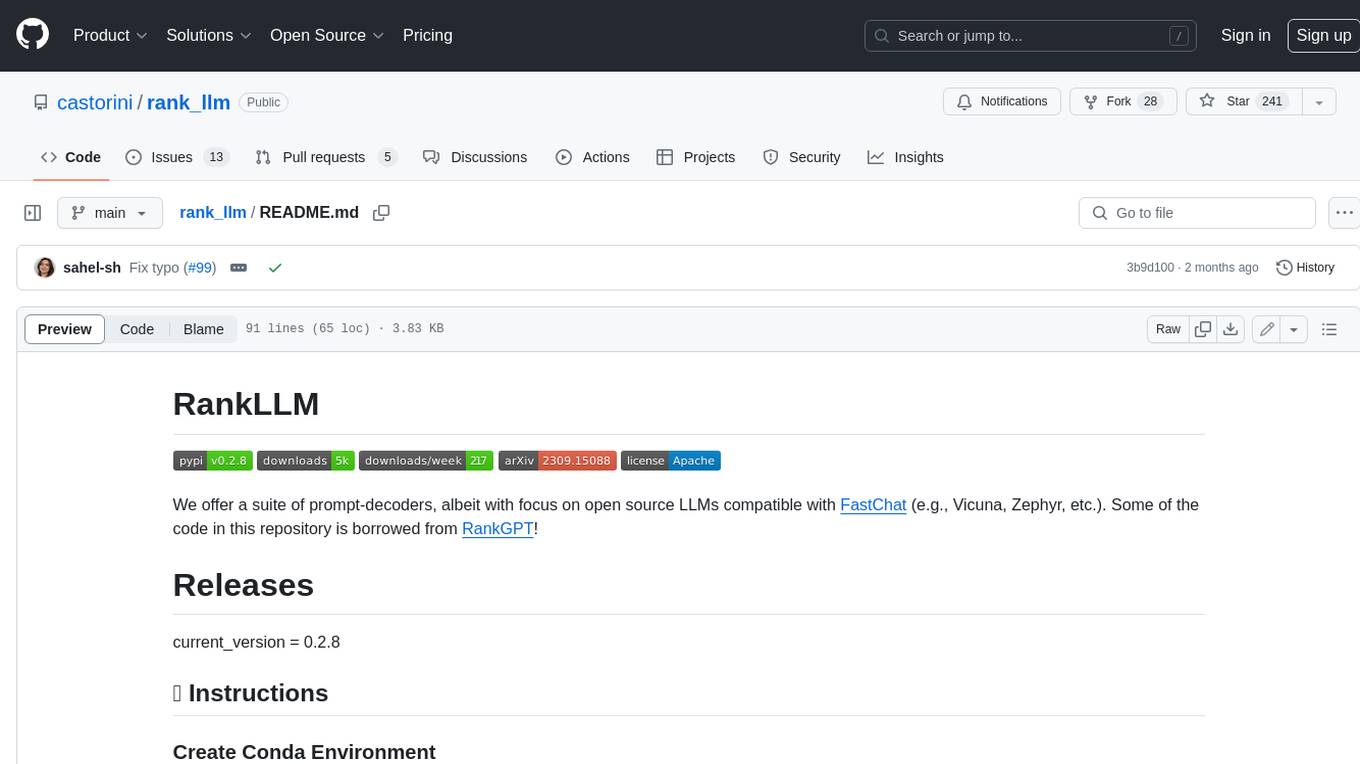
rank_llm
RankLLM is a suite of prompt-decoders compatible with open source LLMs like Vicuna and Zephyr. It allows users to create custom ranking models for various NLP tasks, such as document reranking, question answering, and summarization. The tool offers a variety of features, including the ability to fine-tune models on custom datasets, use different retrieval methods, and control the context size and variable passages. RankLLM is easy to use and can be integrated into existing NLP pipelines.
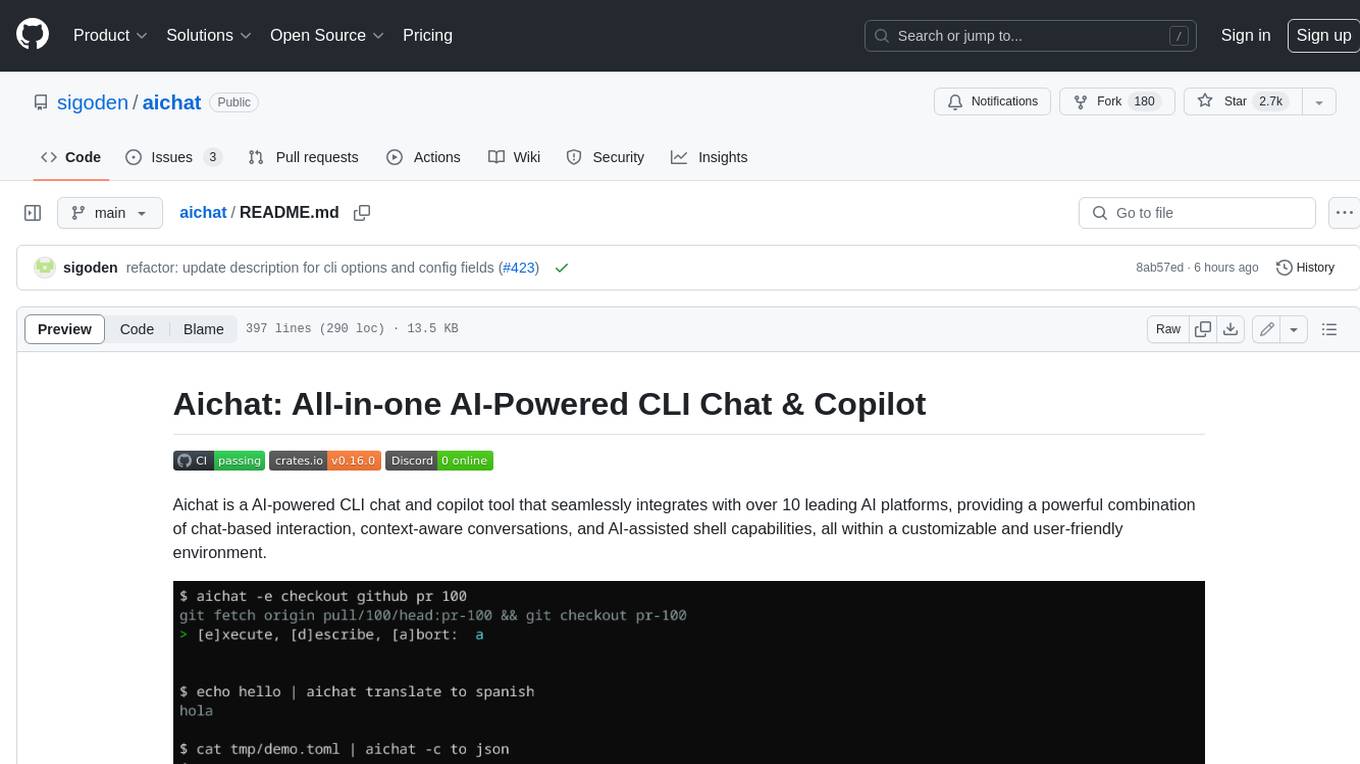
aichat
Aichat is an AI-powered CLI chat and copilot tool that seamlessly integrates with over 10 leading AI platforms, providing a powerful combination of chat-based interaction, context-aware conversations, and AI-assisted shell capabilities, all within a customizable and user-friendly environment.
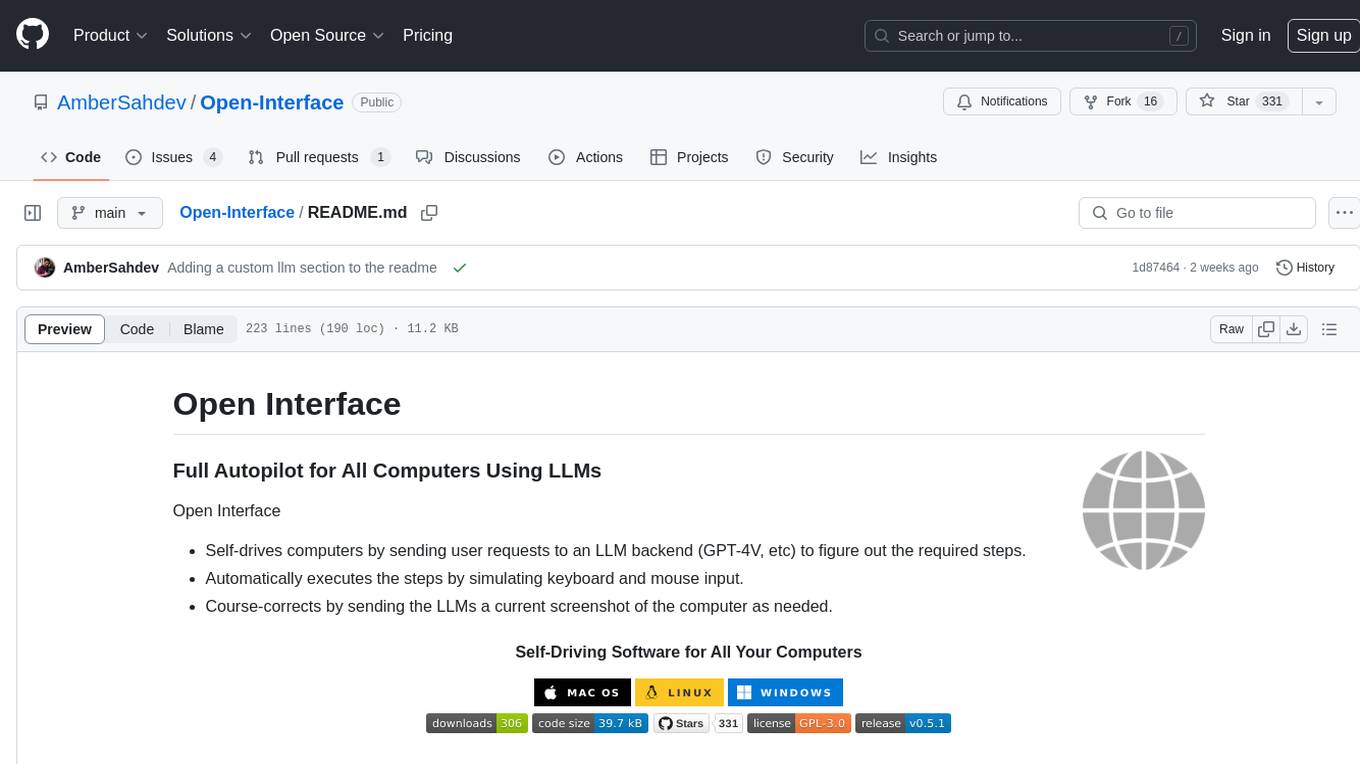
Open-Interface
Open Interface is a self-driving software that automates computer tasks by sending user requests to a language model backend (e.g., GPT-4V) and simulating keyboard and mouse inputs to execute the steps. It course-corrects by sending current screenshots to the language models. The tool supports MacOS, Linux, and Windows, and requires setting up the OpenAI API key for access to GPT-4V. It can automate tasks like creating meal plans, setting up custom language model backends, and more. Open Interface is currently not efficient in accurate spatial reasoning, tracking itself in tabular contexts, and navigating complex GUI-rich applications. Future improvements aim to enhance the tool's capabilities with better models trained on video walkthroughs. The tool is cost-effective, with user requests priced between $0.05 - $0.20, and offers features like interrupting the app and primary display visibility in multi-monitor setups.
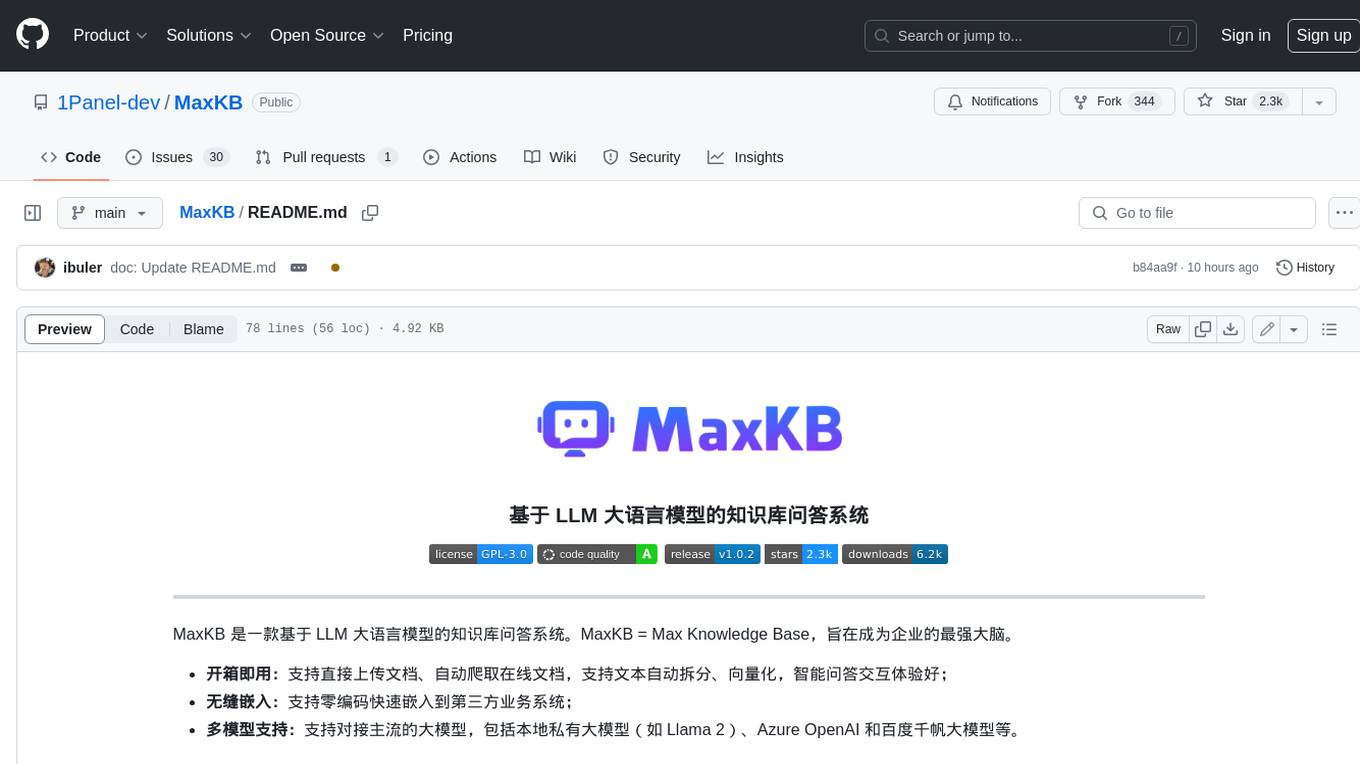
MaxKB
MaxKB is a knowledge base Q&A system based on the LLM large language model. MaxKB = Max Knowledge Base, which aims to become the most powerful brain of the enterprise.
For similar tasks
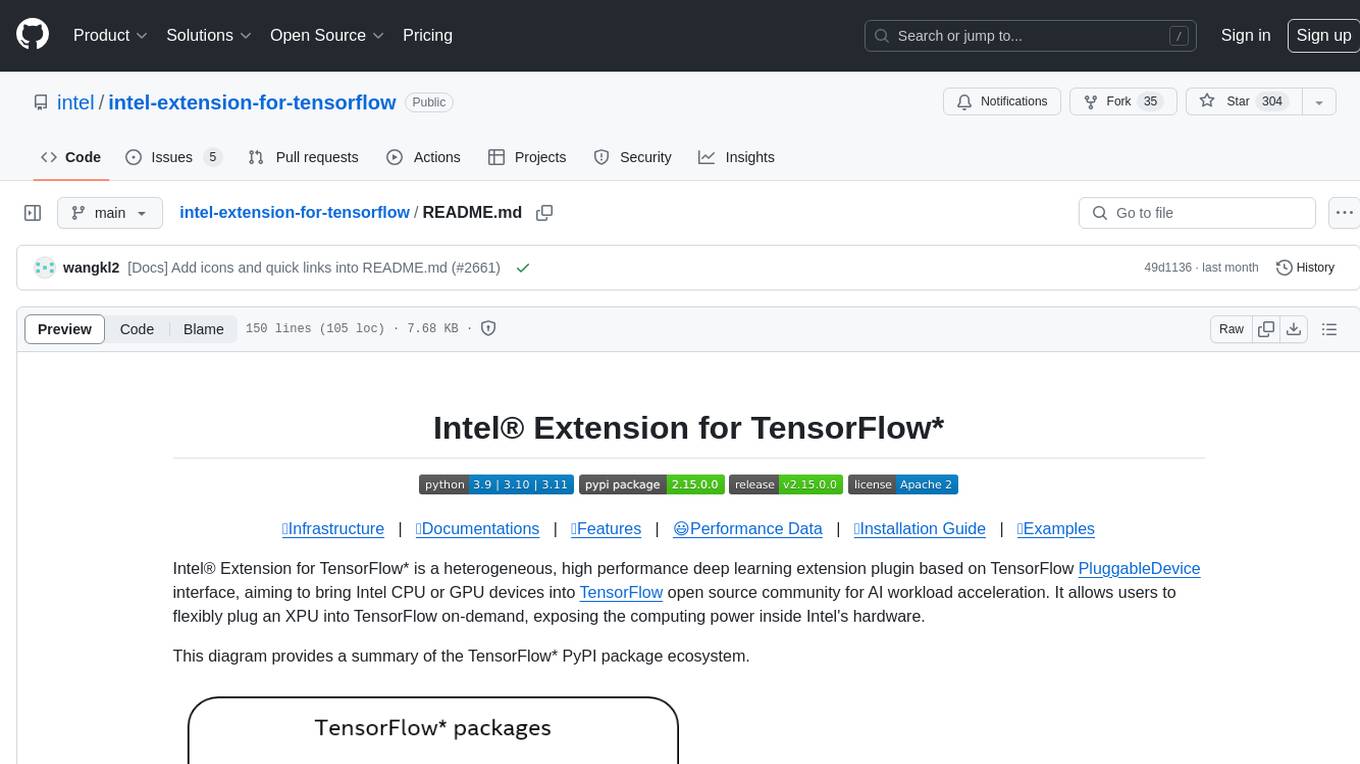
intel-extension-for-tensorflow
Intel® Extension for TensorFlow* is a high performance deep learning extension plugin based on TensorFlow PluggableDevice interface. It aims to accelerate AI workloads by allowing users to plug Intel CPU or GPU devices into TensorFlow on-demand, exposing the computing power inside Intel's hardware. The extension provides XPU specific implementation, kernels & operators, graph optimizer, device runtime, XPU configuration management, XPU backend selection, and options for turning on/off advanced features.
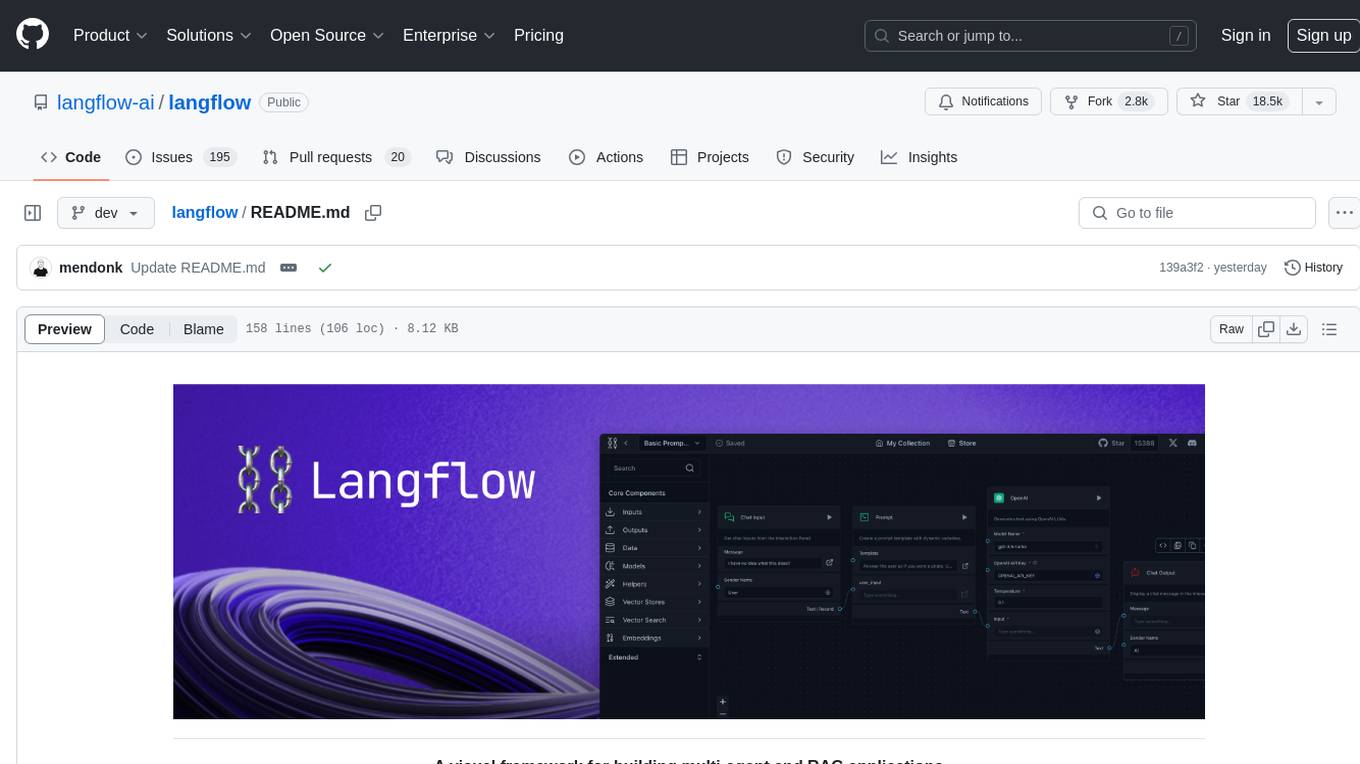
langflow
Langflow is an open-source Python-powered visual framework designed for building multi-agent and RAG applications. It is fully customizable, language model agnostic, and vector store agnostic. Users can easily create flows by dragging components onto the canvas, connect them, and export the flow as a JSON file. Langflow also provides a command-line interface (CLI) for easy management and configuration, allowing users to customize the behavior of Langflow for development or specialized deployment scenarios. The tool can be deployed on various platforms such as Google Cloud Platform, Railway, and Render. Contributors are welcome to enhance the project on GitHub by following the contributing guidelines.
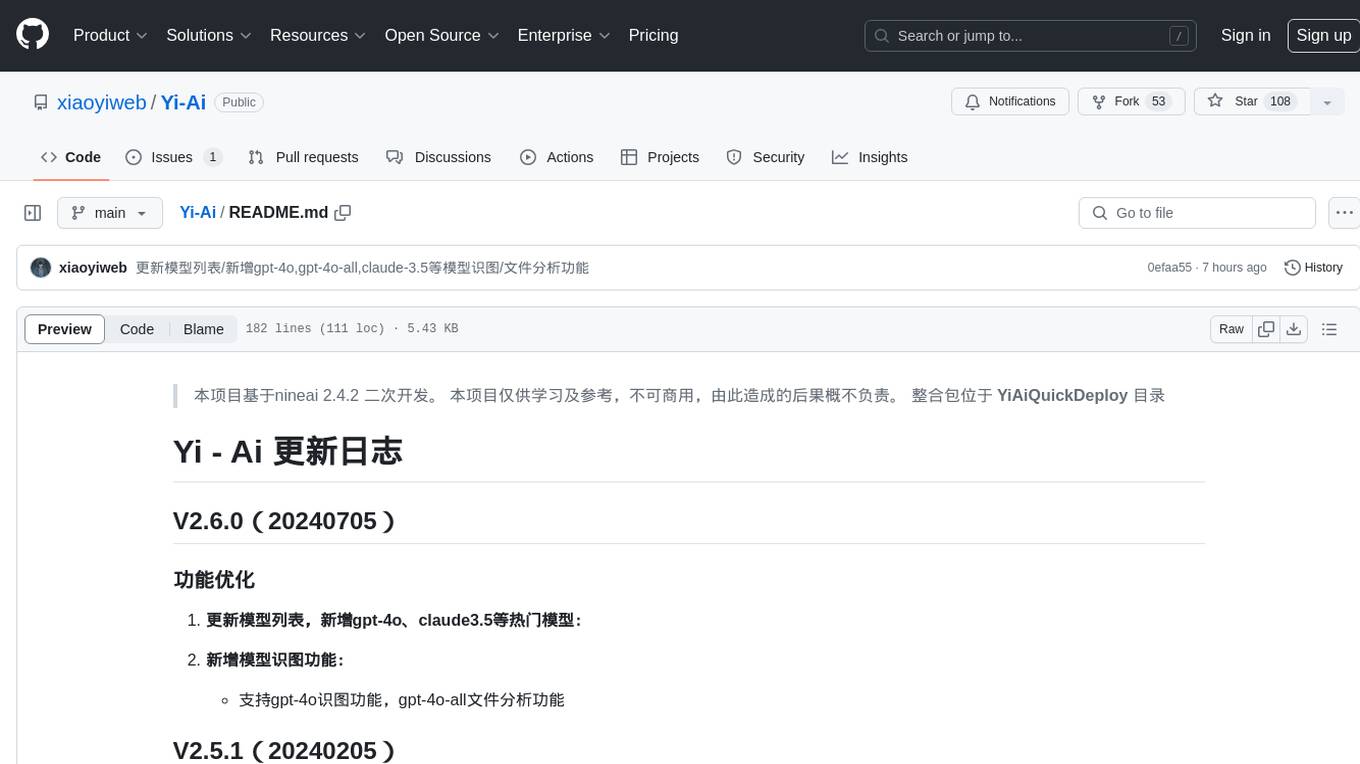
Yi-Ai
Yi-Ai is a project based on the development of nineai 2.4.2. It is for learning and reference purposes only, not for commercial use. The project includes updates to popular models like gpt-4o and claude3.5, as well as new features such as model image recognition. It also supports various functionalities like model sorting, file type extensions, and bug fixes. The project provides deployment tutorials for both integrated and compiled packages, with instructions for environment setup, configuration, dependency installation, and project startup. Additionally, it offers a management platform with different access levels and emphasizes the importance of following the steps for proper system operation.
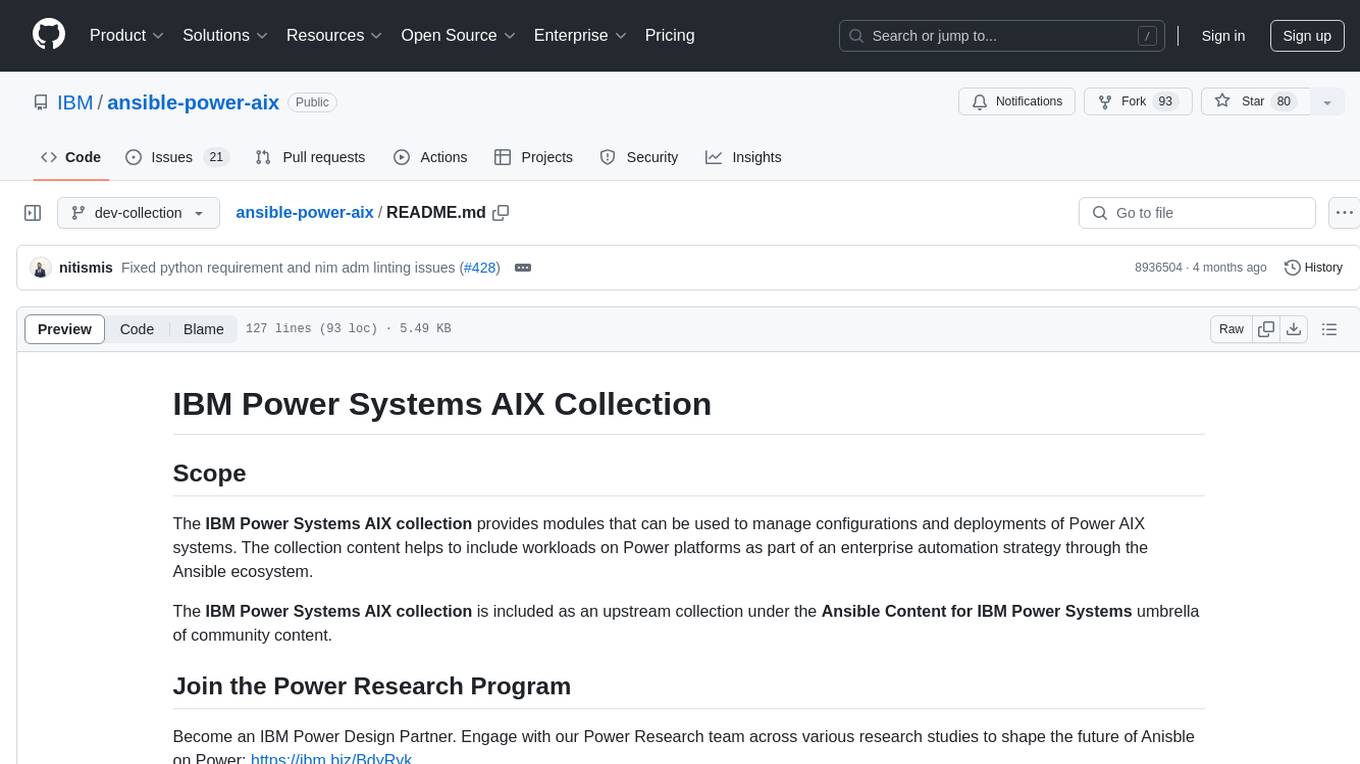
ansible-power-aix
The IBM Power Systems AIX Collection provides modules to manage configurations and deployments of Power AIX systems, enabling workloads on Power platforms as part of an enterprise automation strategy through the Ansible ecosystem. It includes example best practices, requirements for AIX versions, Ansible, and Python, along with resources for documentation and contribution.
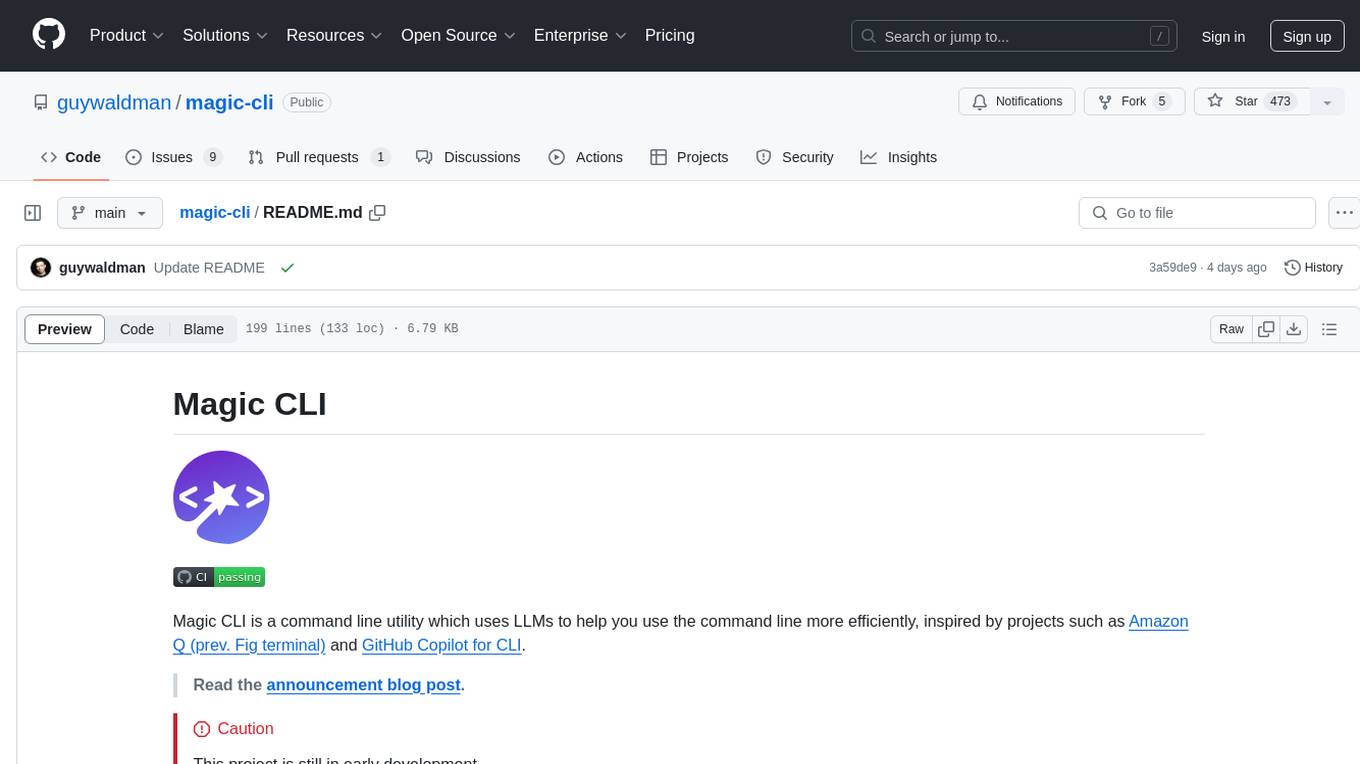
magic-cli
Magic CLI is a command line utility that leverages Large Language Models (LLMs) to enhance command line efficiency. It is inspired by projects like Amazon Q and GitHub Copilot for CLI. The tool allows users to suggest commands, search across command history, and generate commands for specific tasks using local or remote LLM providers. Magic CLI also provides configuration options for LLM selection and response generation. The project is still in early development, so users should expect breaking changes and bugs.
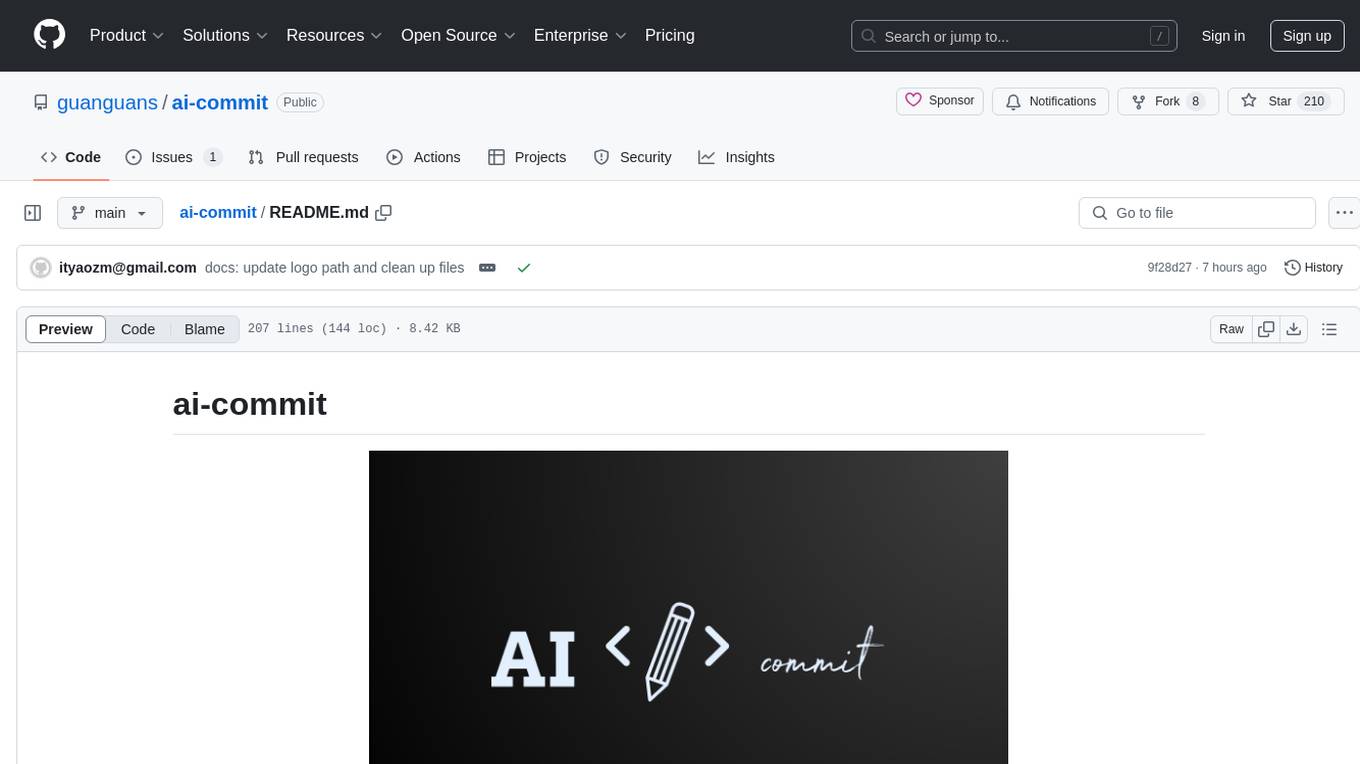
ai-commit
ai-commit is a tool that automagically generates conventional git commit messages using AI. It supports various generators like Bito Cli, ERNIE-Bot-turbo, ERNIE-Bot, Moonshot, and OpenAI Chat. The tool requires PHP version 7.3 or higher for installation. Users can configure generators, set API keys, and easily generate and commit messages with customizable options. Additionally, ai-commit provides commands for managing configurations, self-updating, and shell completion scripts.
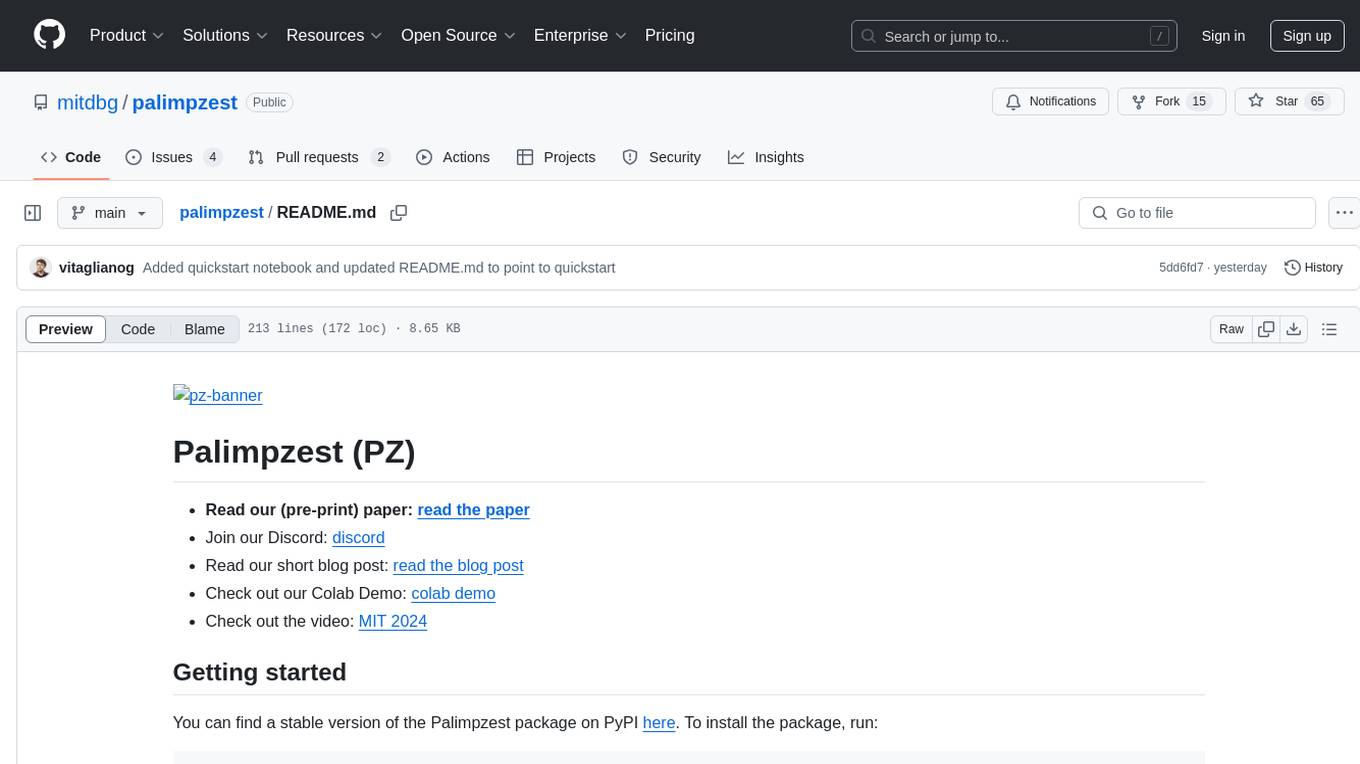
palimpzest
Palimpzest (PZ) is a tool for managing and optimizing workloads, particularly for data processing tasks. It provides a CLI tool and Python demos for users to register datasets, run workloads, and access results. Users can easily initialize their system, register datasets, and manage configurations using the CLI commands provided. Palimpzest also supports caching intermediate results and configuring for parallel execution with remote services like OpenAI and together.ai. The tool aims to streamline the workflow of working with datasets and optimizing performance for data extraction tasks.
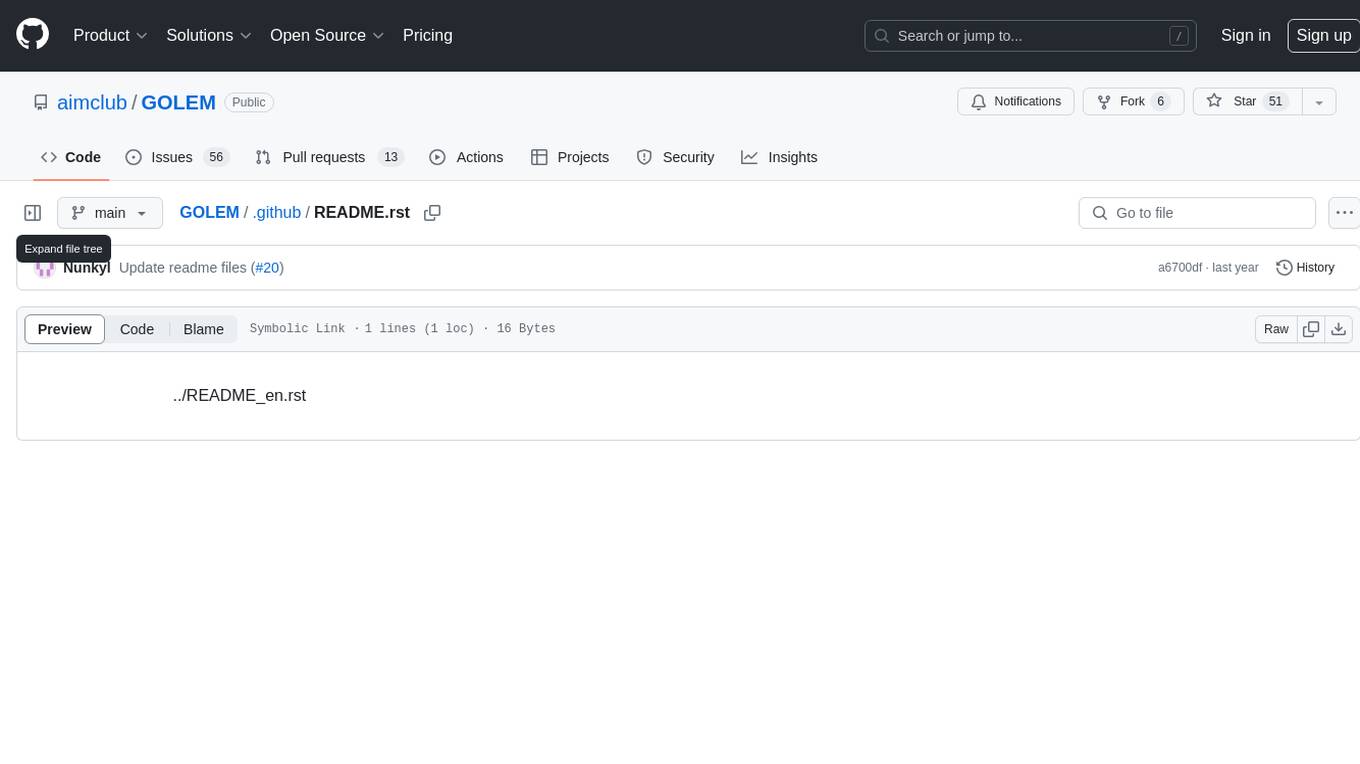
GOLEM
GOLEM is an open-source AI framework focused on optimization and learning of structured graph-based models using meta-heuristic methods. It emphasizes the potential of meta-heuristics in complex problem spaces where gradient-based methods are not suitable, and the importance of structured models in various problem domains. The framework offers features like structured model optimization, metaheuristic methods, multi-objective optimization, constrained optimization, extensibility, interpretability, and reproducibility. It can be applied to optimization problems represented as directed graphs with defined fitness functions. GOLEM has applications in areas like AutoML, Bayesian network structure search, differential equation discovery, geometric design, and neural architecture search. The project structure includes packages for core functionalities, adapters, graph representation, optimizers, genetic algorithms, utilities, serialization, visualization, examples, and testing. Contributions are welcome, and the project is supported by ITMO University's Research Center Strong Artificial Intelligence in Industry.
For similar jobs
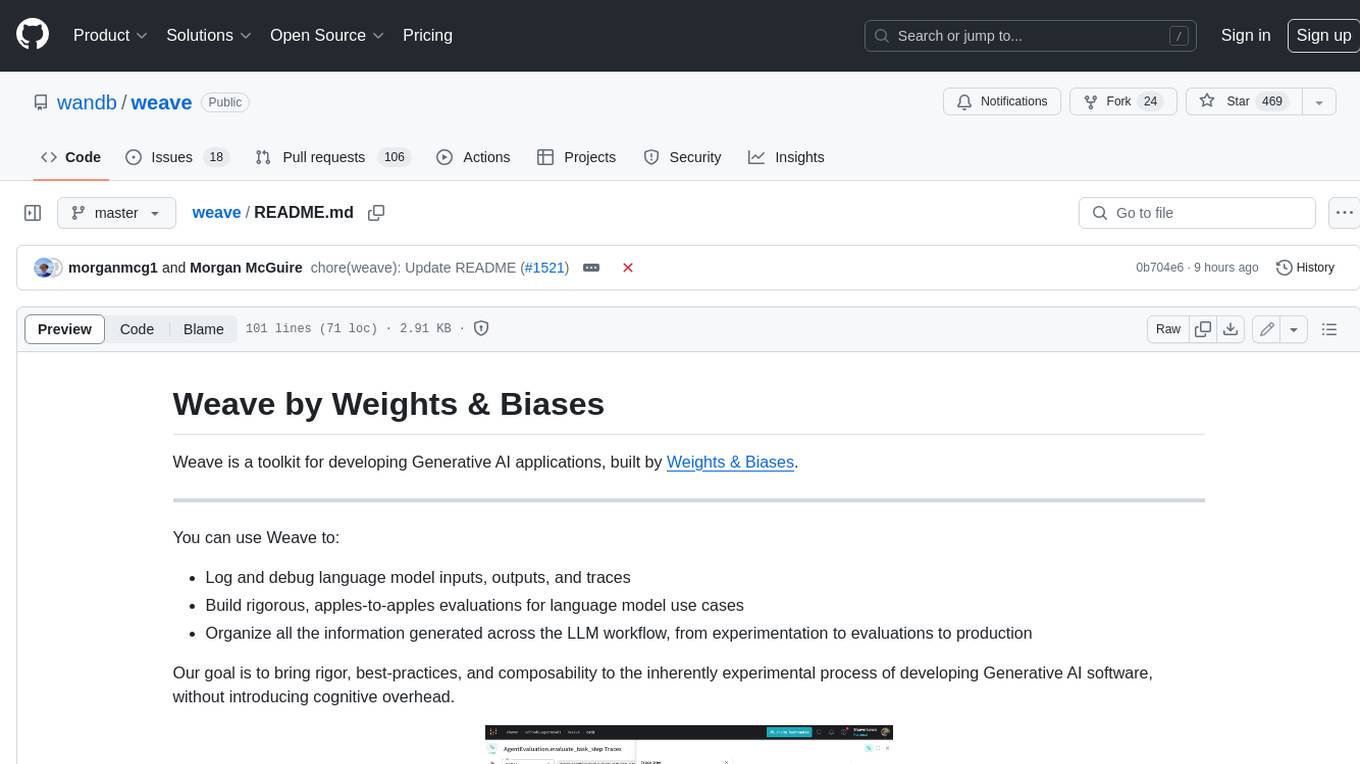
weave
Weave is a toolkit for developing Generative AI applications, built by Weights & Biases. With Weave, you can log and debug language model inputs, outputs, and traces; build rigorous, apples-to-apples evaluations for language model use cases; and organize all the information generated across the LLM workflow, from experimentation to evaluations to production. Weave aims to bring rigor, best-practices, and composability to the inherently experimental process of developing Generative AI software, without introducing cognitive overhead.
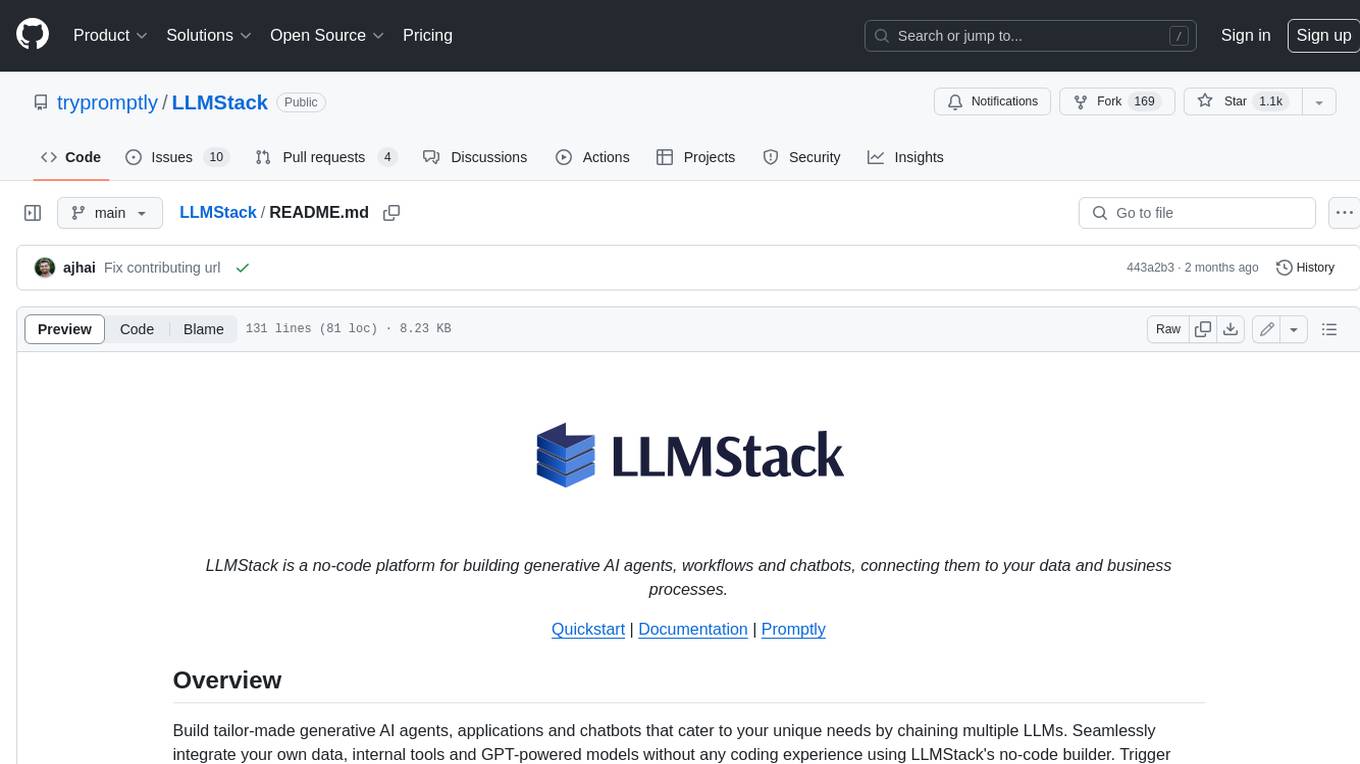
LLMStack
LLMStack is a no-code platform for building generative AI agents, workflows, and chatbots. It allows users to connect their own data, internal tools, and GPT-powered models without any coding experience. LLMStack can be deployed to the cloud or on-premise and can be accessed via HTTP API or triggered from Slack or Discord.
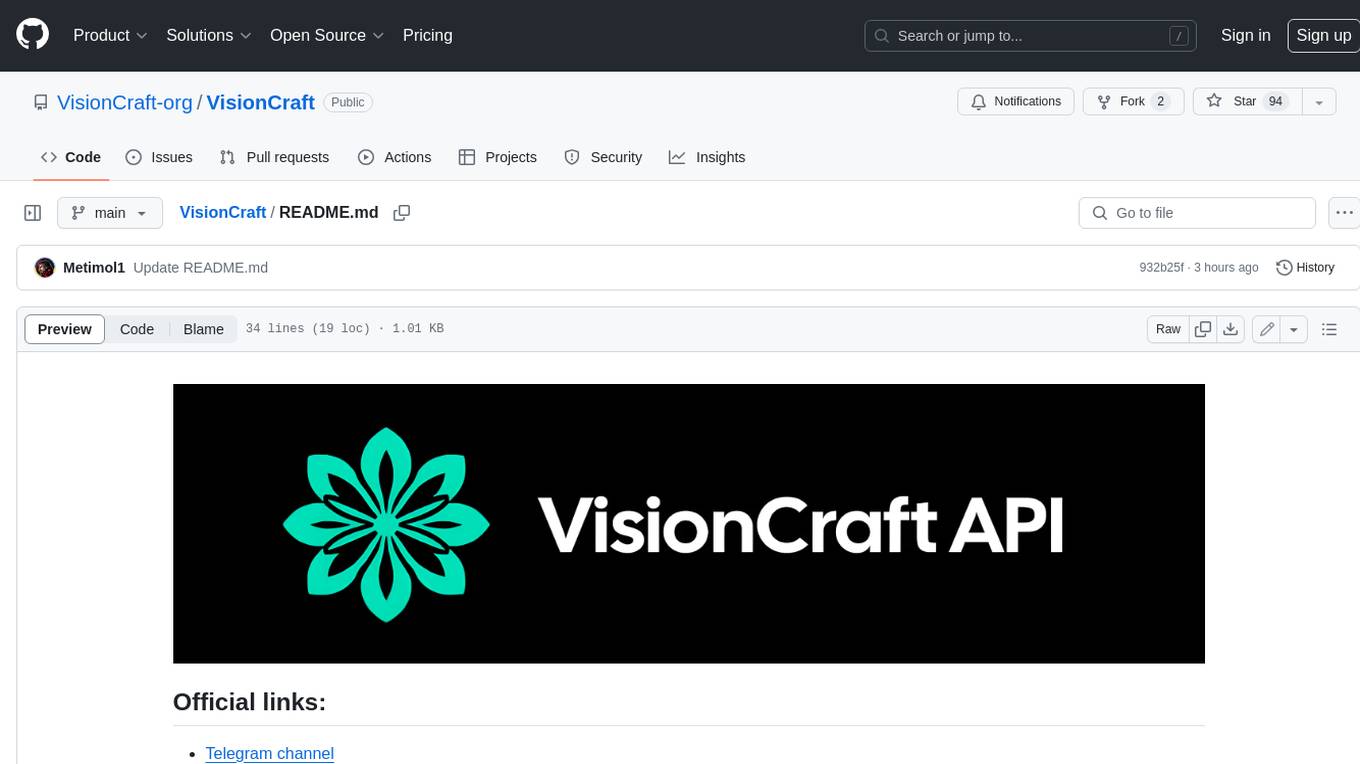
VisionCraft
The VisionCraft API is a free API for using over 100 different AI models. From images to sound.
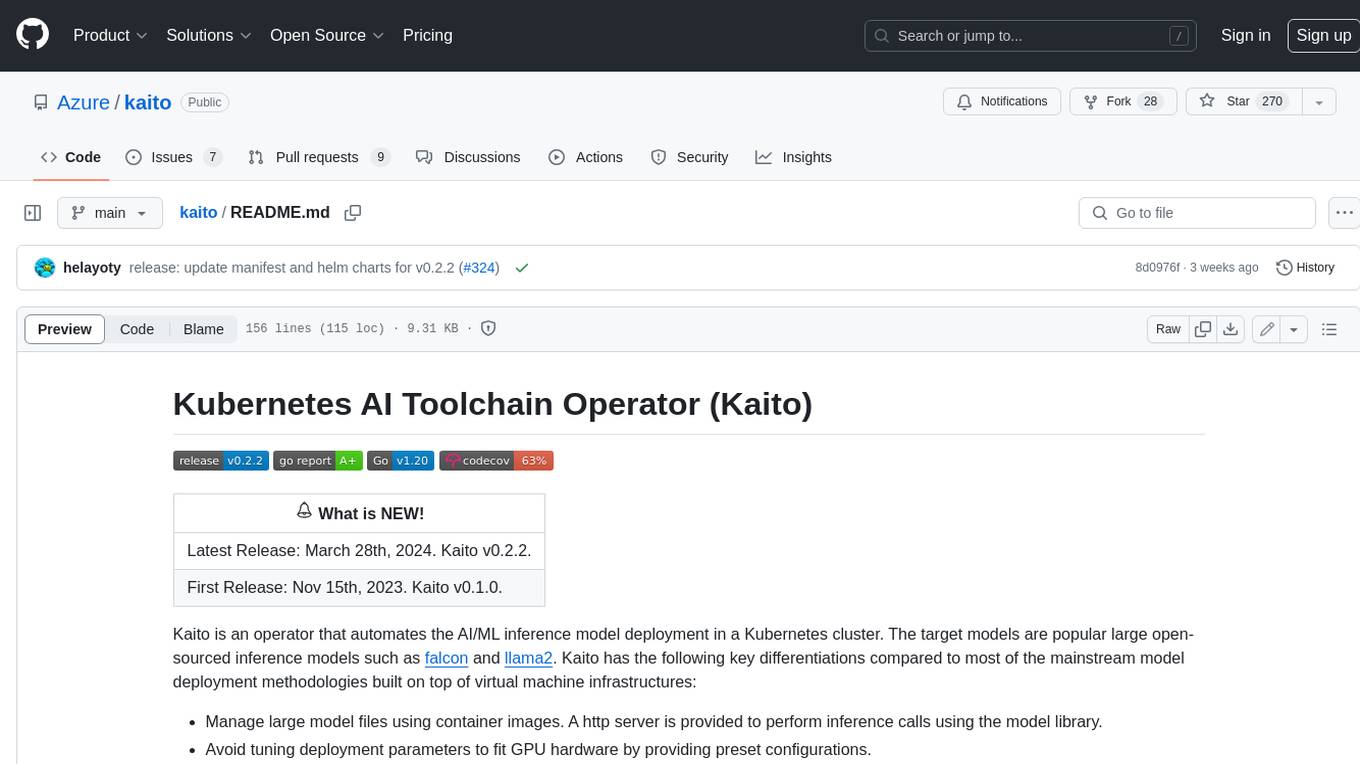
kaito
Kaito is an operator that automates the AI/ML inference model deployment in a Kubernetes cluster. It manages large model files using container images, avoids tuning deployment parameters to fit GPU hardware by providing preset configurations, auto-provisions GPU nodes based on model requirements, and hosts large model images in the public Microsoft Container Registry (MCR) if the license allows. Using Kaito, the workflow of onboarding large AI inference models in Kubernetes is largely simplified.
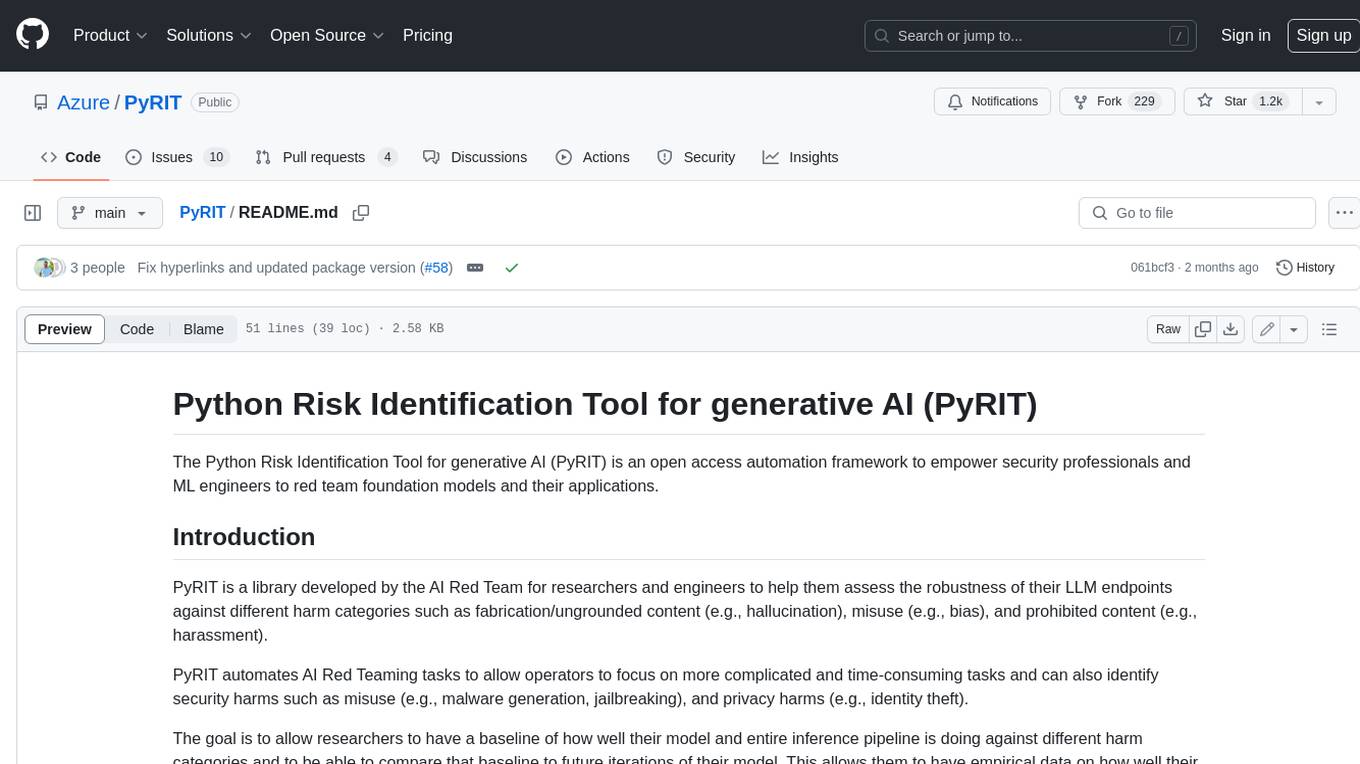
PyRIT
PyRIT is an open access automation framework designed to empower security professionals and ML engineers to red team foundation models and their applications. It automates AI Red Teaming tasks to allow operators to focus on more complicated and time-consuming tasks and can also identify security harms such as misuse (e.g., malware generation, jailbreaking), and privacy harms (e.g., identity theft). The goal is to allow researchers to have a baseline of how well their model and entire inference pipeline is doing against different harm categories and to be able to compare that baseline to future iterations of their model. This allows them to have empirical data on how well their model is doing today, and detect any degradation of performance based on future improvements.
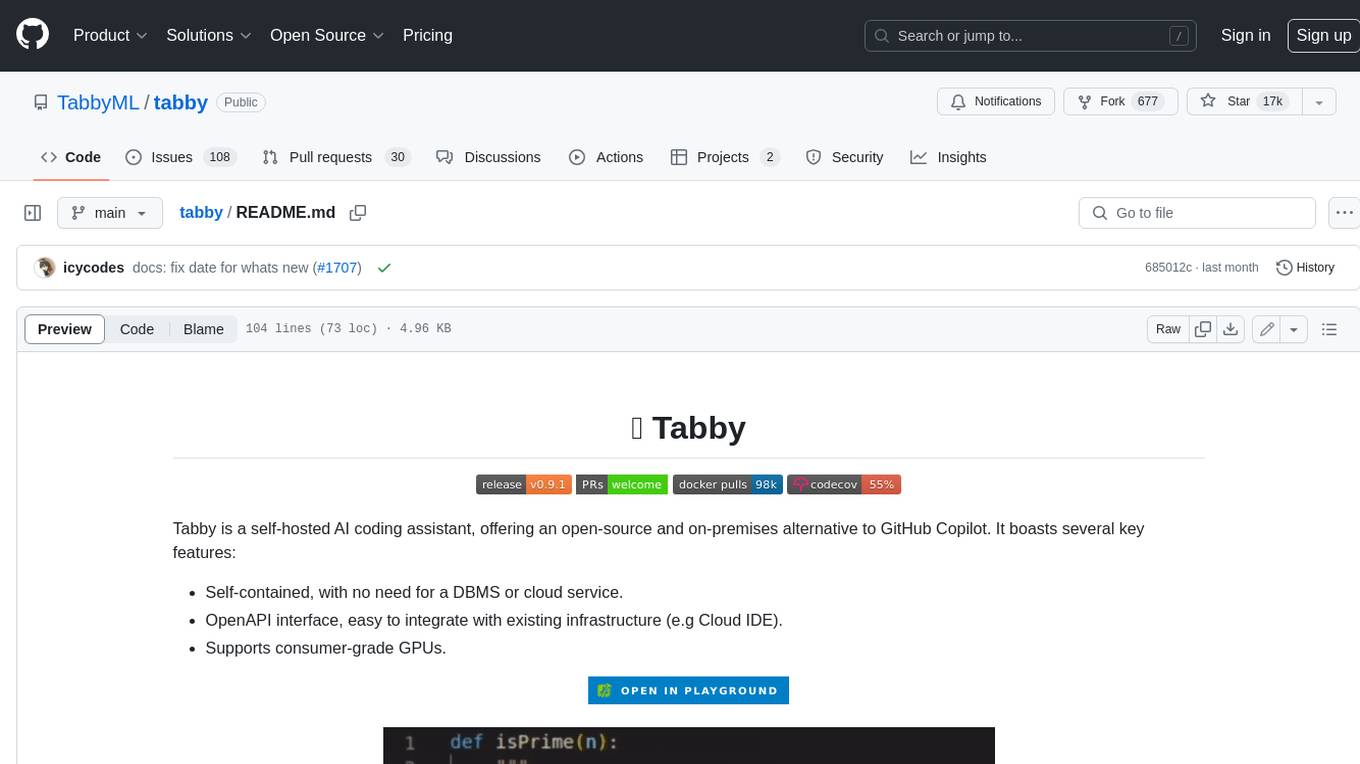
tabby
Tabby is a self-hosted AI coding assistant, offering an open-source and on-premises alternative to GitHub Copilot. It boasts several key features: * Self-contained, with no need for a DBMS or cloud service. * OpenAPI interface, easy to integrate with existing infrastructure (e.g Cloud IDE). * Supports consumer-grade GPUs.
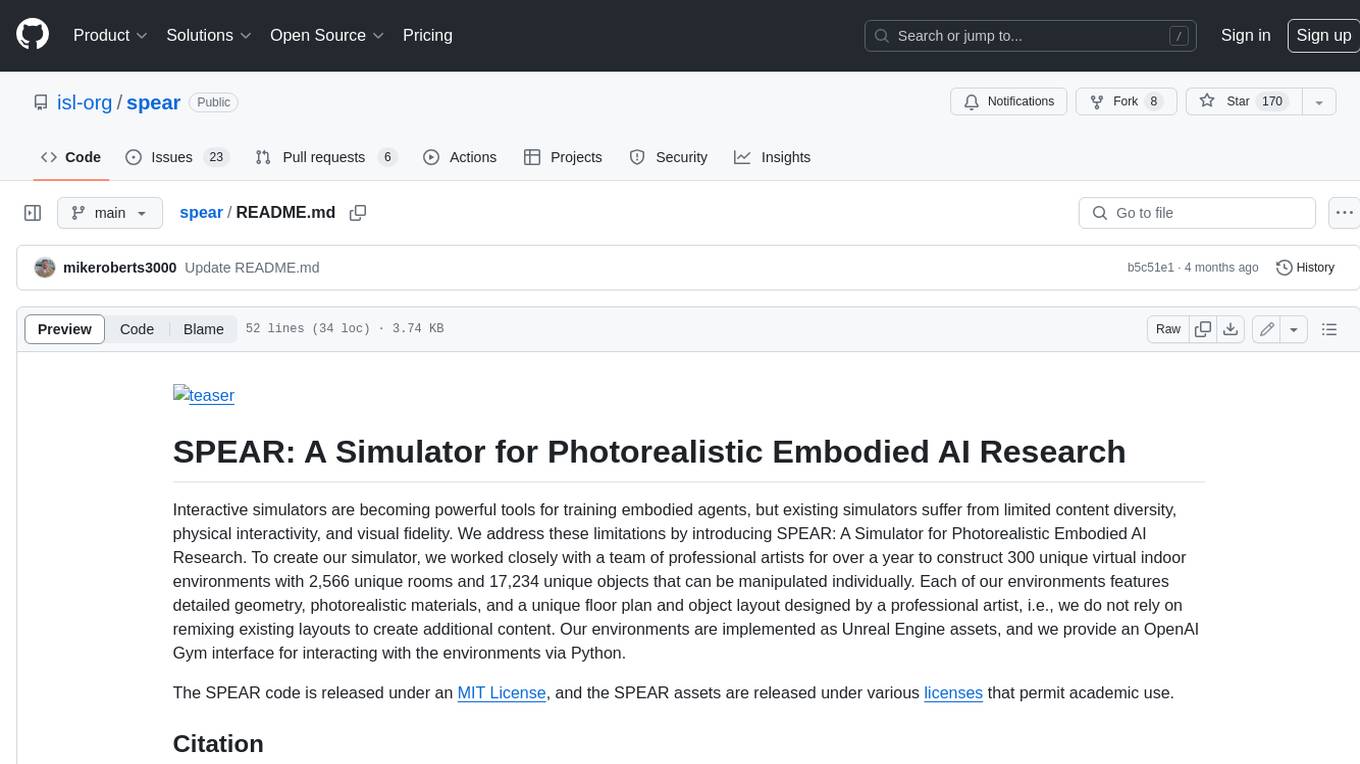
spear
SPEAR (Simulator for Photorealistic Embodied AI Research) is a powerful tool for training embodied agents. It features 300 unique virtual indoor environments with 2,566 unique rooms and 17,234 unique objects that can be manipulated individually. Each environment is designed by a professional artist and features detailed geometry, photorealistic materials, and a unique floor plan and object layout. SPEAR is implemented as Unreal Engine assets and provides an OpenAI Gym interface for interacting with the environments via Python.
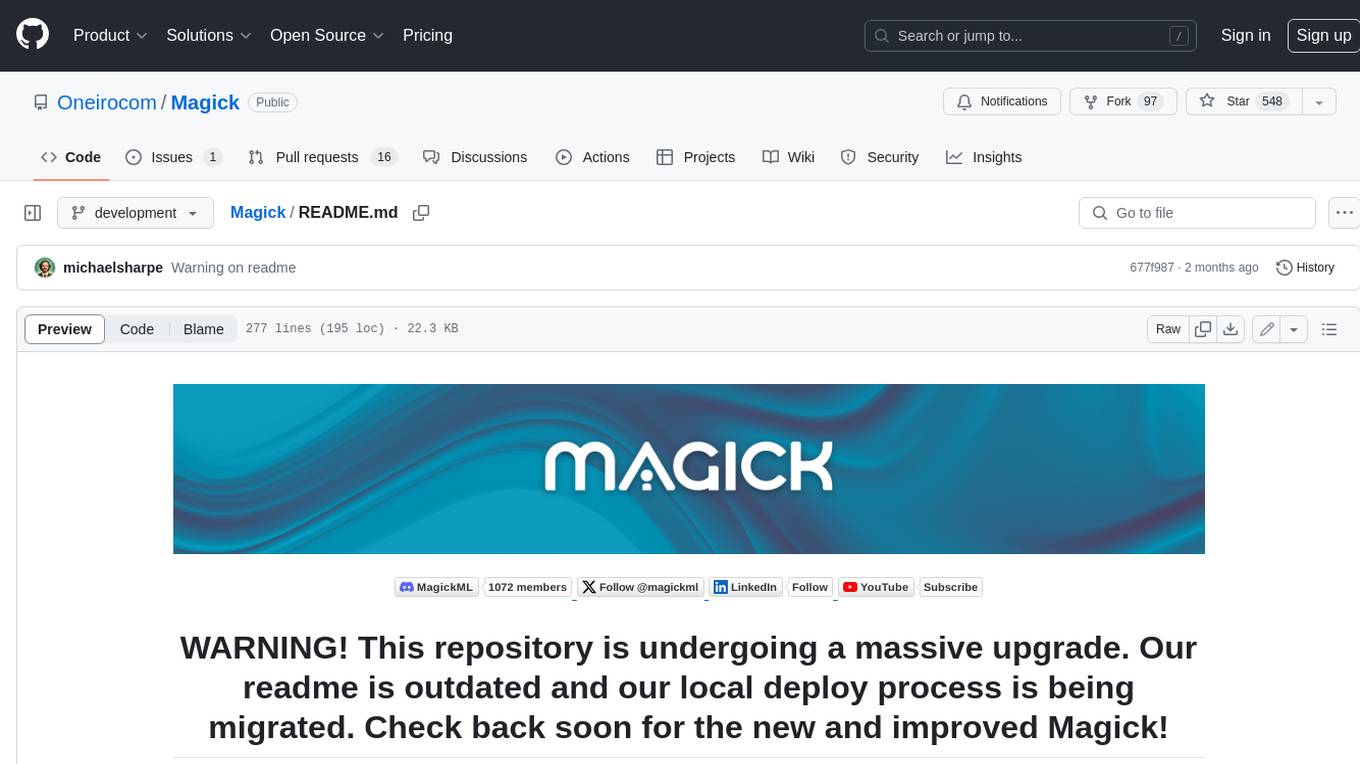
Magick
Magick is a groundbreaking visual AIDE (Artificial Intelligence Development Environment) for no-code data pipelines and multimodal agents. Magick can connect to other services and comes with nodes and templates well-suited for intelligent agents, chatbots, complex reasoning systems and realistic characters.