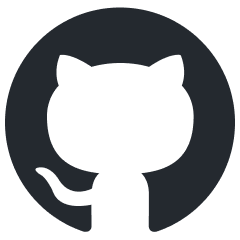
nexa-sdk
Nexa SDK is a comprehensive toolkit for supporting GGML and ONNX models. It supports text generation, image generation, vision-language models (VLM), Audio Language Model, auto-speech-recognition (ASR), and text-to-speech (TTS) capabilities.
Stars: 5474
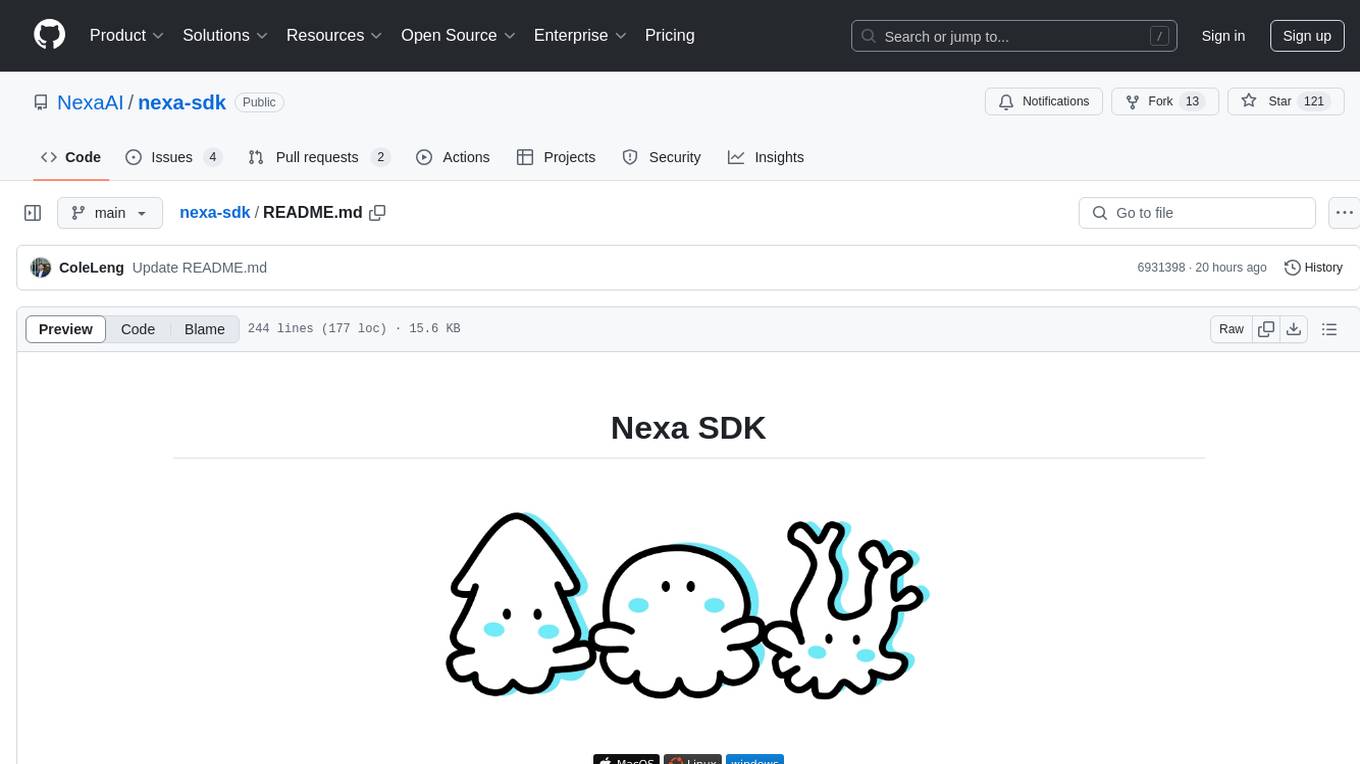
Nexa SDK is a comprehensive toolkit supporting ONNX and GGML models for text generation, image generation, vision-language models (VLM), and text-to-speech (TTS) capabilities. It offers an OpenAI-compatible API server with JSON schema mode and streaming support, along with a user-friendly Streamlit UI. Users can run Nexa SDK on any device with Python environment, with GPU acceleration supported. The toolkit provides model support, conversion engine, inference engine for various tasks, and differentiating features from other tools.
README:
On-Device Model Hub | Documentation | Discord | Blogs | X (Twitter)
Nexa SDK is a local on-device inference framework for ONNX and GGML models, supporting text generation, image generation, vision-language models (VLM), audio-language models, speech-to-text (ASR), and text-to-speech (TTS) capabilities. Installable via Python Package or Executable Installer.
- Device Support: CPU, GPU (CUDA, Metal, ROCm), iOS
- Server: OpenAI-compatible API, JSON schema for function calling and streaming support
- Local UI: Streamlit for interactive model deployment and testing
- Support Nexa AI's own vision language model (0.9B parameters):
nexa run omniVLM
and audio language model (2.9B parameters):nexa run omniaudio
- Support audio language model:
nexa run qwen2audio
, we are the first open-source toolkit to support audio language model with GGML tensor library. - Support iOS Swift binding for local inference on iOS mobile devices.
- Support embedding model:
nexa embed <model_path> <prompt>
- Support pull and run supported Computer Vision models in GGUF format from HuggingFace or ModelScope:
nexa run -hf <hf_model_id> -mt COMPUTER_VISION
ornexa run -ms <ms_model_id> -mt COMPUTER_VISION
- Support pull and run NLP models in GGUF format from HuggingFace or ModelScope:
nexa run -hf <hf_model_id> -mt NLP
ornexa run -ms <ms_model_id> -mt NLP
Welcome to submit your requests through issues, we ship weekly.
curl -fsSL https://public-storage.nexa4ai.com/install.sh | sh
FAQ: cannot use executable with nexaai python package already installed
Try using nexa-exe
instead:
nexa-exe <command>
We have released pre-built wheels for various Python versions, platforms, and backends for convenient installation on our index page.
CPU
pip install nexaai --prefer-binary --index-url https://github.nexa.ai/whl/cpu --extra-index-url https://pypi.org/simple --no-cache-dir
Apple GPU (Metal)
For the GPU version supporting Metal (macOS):
CMAKE_ARGS="-DGGML_METAL=ON -DSD_METAL=ON" pip install nexaai --prefer-binary --index-url https://github.nexa.ai/whl/metal --extra-index-url https://pypi.org/simple --no-cache-dir
FAQ: cannot use Metal/GPU on M1
Try the following command:
wget https://github.com/conda-forge/miniforge/releases/latest/download/Miniforge3-MacOSX-arm64.sh
bash Miniforge3-MacOSX-arm64.sh
conda create -n nexasdk python=3.10
conda activate nexasdk
CMAKE_ARGS="-DGGML_METAL=ON -DSD_METAL=ON" pip install nexaai --prefer-binary --index-url https://github.nexa.ai/whl/metal --extra-index-url https://pypi.org/simple --no-cache-dir
Nvidia GPU (CUDA)
To install with CUDA support, make sure you have CUDA Toolkit 12.0 or later installed.
For Linux:
CMAKE_ARGS="-DGGML_CUDA=ON -DSD_CUBLAS=ON" pip install nexaai --prefer-binary --index-url https://github.nexa.ai/whl/cu124 --extra-index-url https://pypi.org/simple --no-cache-dir
For Windows PowerShell:
$env:CMAKE_ARGS="-DGGML_CUDA=ON -DSD_CUBLAS=ON"; pip install nexaai --prefer-binary --index-url https://github.nexa.ai/whl/cu124 --extra-index-url https://pypi.org/simple --no-cache-dir
For Windows Command Prompt:
set CMAKE_ARGS="-DGGML_CUDA=ON -DSD_CUBLAS=ON" & pip install nexaai --prefer-binary --index-url https://github.nexa.ai/whl/cu124 --extra-index-url https://pypi.org/simple --no-cache-dir
For Windows Git Bash:
CMAKE_ARGS="-DGGML_CUDA=ON -DSD_CUBLAS=ON" pip install nexaai --prefer-binary --index-url https://github.nexa.ai/whl/cu124 --extra-index-url https://pypi.org/simple --no-cache-dir
AMD GPU (ROCm)
To install with ROCm support, make sure you have ROCm 6.2.1 or later installed.
For Linux:
CMAKE_ARGS="-DGGML_HIPBLAS=on" pip install nexaai --prefer-binary --index-url https://github.nexa.ai/whl/rocm621 --extra-index-url https://pypi.org/simple --no-cache-dir
GPU (Vulkan)
To install with Vulkan support, make sure you have Vulkan SDK 1.3.261.1 or later installed.
For Windows PowerShell:
$env:CMAKE_ARGS="-DGGML_VULKAN=on"; pip install nexaai --prefer-binary --index-url https://github.nexa.ai/whl/vulkan --extra-index-url https://pypi.org/simple --no-cache-dir
For Windows Command Prompt:
set CMAKE_ARGS="-DGGML_VULKAN=on" & pip install nexaai --prefer-binary --index-url https://github.nexa.ai/whl/vulkan --extra-index-url https://pypi.org/simple --no-cache-dir
For Windows Git Bash:
CMAKE_ARGS="-DGGML_VULKAN=on" pip install nexaai --prefer-binary --index-url https://github.nexa.ai/whl/vulkan --extra-index-url https://pypi.org/simple --no-cache-dir
Local Build
How to clone this repo
git clone --recursive https://github.com/NexaAI/nexa-sdk
If you forget to use --recursive
, you can use below command to add submodule
git submodule update --init --recursive
Then you can build and install the package
pip install -e .
Below is our differentiation from other similar tools:
Feature | Nexa SDK | ollama | Optimum | LM Studio |
---|---|---|---|---|
GGML Support | â | â | â | â |
ONNX Support | â | â | â | â |
Text Generation | â | â | â | â |
Image Generation | â | â | â | â |
Vision-Language Models | â | â | â | â |
Audio-Language Models | â | â | â | â |
Text-to-Speech | â | â | â | â |
Server Capability | â | â | â | â |
User Interface | â | â | â | â |
Executable Installation | â | â | â | â |
Our on-device model hub offers all types of quantized models (text, image, audio, multimodal) with filters for RAM, file size, Tasks, etc. to help you easily explore models with UI. Explore on-device models at On-device Model Hub
Supported model examples (full list at Model Hub):
Model | Type | Format | Command |
---|---|---|---|
omniaudio | AudioLM | GGUF | nexa run omniaudio |
qwen2audio | AudioLM | GGUF | nexa run qwen2audio |
octopus-v2 | Function Call | GGUF | nexa run octopus-v2 |
octo-net | Text | GGUF | nexa run octo-net |
omniVLM | Multimodal | GGUF | nexa run omniVLM |
nanollava | Multimodal | GGUF | nexa run nanollava |
llava-phi3 | Multimodal | GGUF | nexa run llava-phi3 |
llava-llama3 | Multimodal | GGUF | nexa run llava-llama3 |
llava1.6-mistral | Multimodal | GGUF | nexa run llava1.6-mistral |
llava1.6-vicuna | Multimodal | GGUF | nexa run llava1.6-vicuna |
llama3.2 | Text | GGUF | nexa run llama3.2 |
llama3-uncensored | Text | GGUF | nexa run llama3-uncensored |
gemma2 | Text | GGUF | nexa run gemma2 |
qwen2.5 | Text | GGUF | nexa run qwen2.5 |
mathqwen | Text | GGUF | nexa run mathqwen |
codeqwen | Text | GGUF | nexa run codeqwen |
mistral | Text | GGUF/ONNX | nexa run mistral |
deepseek-coder | Text | GGUF | nexa run deepseek-coder |
phi3.5 | Text | GGUF | nexa run phi3.5 |
openelm | Text | GGUF | nexa run openelm |
stable-diffusion-v2-1 | Image Generation | GGUF | nexa run sd2-1 |
stable-diffusion-3-medium | Image Generation | GGUF | nexa run sd3 |
FLUX.1-schnell | Image Generation | GGUF | nexa run flux |
lcm-dreamshaper | Image Generation | GGUF/ONNX | nexa run lcm-dreamshaper |
whisper-large-v3-turbo | Speech-to-Text | BIN | nexa run faster-whisper-large-turbo |
whisper-tiny.en | Speech-to-Text | ONNX | nexa run whisper-tiny.en |
mxbai-embed-large-v1 | Embedding | GGUF | nexa embed mxbai |
nomic-embed-text-v1.5 | Embedding | GGUF | nexa embed nomic |
all-MiniLM-L12-v2 | Embedding | GGUF | nexa embed all-MiniLM-L12-v2:fp16 |
bark-small | Text-to-Speech | GGUF | nexa run bark-small:fp16 |
You can pull, convert (to .gguf), quantize and run llama.cpp supported text generation models from HF or MS with Nexa SDK.
Use nexa run -hf <hf-model-id>
or nexa run -ms <ms-model-id>
to run models with provided .gguf files:
nexa run -hf Qwen/Qwen2.5-Coder-7B-Instruct-GGUF
nexa run -ms Qwen/Qwen2.5-Coder-7B-Instruct-GGUF
Note: You will be prompted to select a single .gguf file. If your desired quantization version has multiple split files (like fp16-00001-of-00004), please use Nexa's conversion tool (see below) to convert and quantize the model locally.
Install Nexa Python package, and install Nexa conversion tool with pip install "nexaai[convert]"
, then convert models from huggingface with nexa convert <hf-model-id>
:
nexa convert HuggingFaceTB/SmolLM2-135M-Instruct
Or you can convert models from ModelScope with nexa convert -ms <ms-model-id>
:
nexa convert -ms Qwen/Qwen2.5-7B-Instruct
Note: Check our leaderboard for performance benchmarks of different quantized versions of mainstream language models and HuggingFace docs to learn about quantization options.
đ You can view downloaded and converted models with nexa list
[!NOTE]
- If you want to use ONNX model, just replace
pip install nexaai
withpip install "nexaai[onnx]"
in provided commands.- If you want to run benchmark evaluation, just replace
pip install nexaai
withpip install "nexaai[eval]"
in provided commands.- If you want to convert and quantize huggingface models to GGUF models, just replace
pip install nexaai
withpip install "nexaai[convert]"
in provided commands.- For Chinese developers, we recommend you to use Tsinghua Open Source Mirror as extra index url, just replace
--extra-index-url https://pypi.org/simple
with--extra-index-url https://pypi.tuna.tsinghua.edu.cn/simple
in provided commands.
Here's a brief overview of the main CLI commands:
-
nexa run
: Run inference for various tasks using GGUF models. -
nexa onnx
: Run inference for various tasks using ONNX models. -
nexa convert
: Convert and quantize huggingface models to GGUF models. -
nexa server
: Run the Nexa AI Text Generation Service. -
nexa eval
: Run the Nexa AI Evaluation Tasks. -
nexa pull
: Pull a model from official or hub. -
nexa remove
: Remove a model from local machine. -
nexa clean
: Clean up all model files. -
nexa list
: List all models in the local machine. -
nexa login
: Login to Nexa API. -
nexa whoami
: Show current user information. -
nexa logout
: Logout from Nexa API.
For detailed information on CLI commands and usage, please refer to the CLI Reference document.
To start a local server using models on your local computer, you can use the nexa server
command.
For detailed information on server setup, API endpoints, and usage examples, please refer to the Server Reference document.
Swift SDK: Provides a Swifty API, allowing Swift developers to easily integrate and use llama.cpp models in their projects.
We would like to thank the following projects:
For Tasks:
Click tags to check more tools for each tasksFor Jobs:
Alternative AI tools for nexa-sdk
Similar Open Source Tools
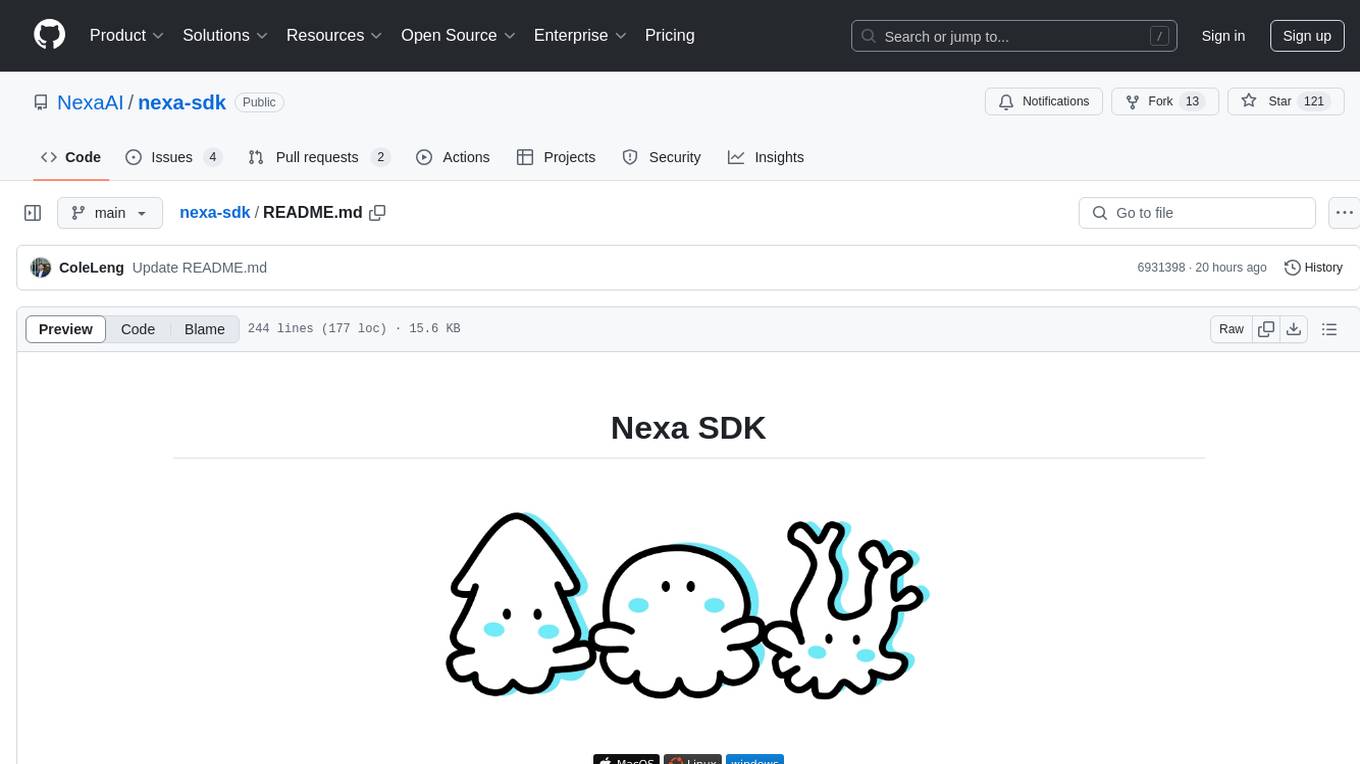
nexa-sdk
Nexa SDK is a comprehensive toolkit supporting ONNX and GGML models for text generation, image generation, vision-language models (VLM), and text-to-speech (TTS) capabilities. It offers an OpenAI-compatible API server with JSON schema mode and streaming support, along with a user-friendly Streamlit UI. Users can run Nexa SDK on any device with Python environment, with GPU acceleration supported. The toolkit provides model support, conversion engine, inference engine for various tasks, and differentiating features from other tools.
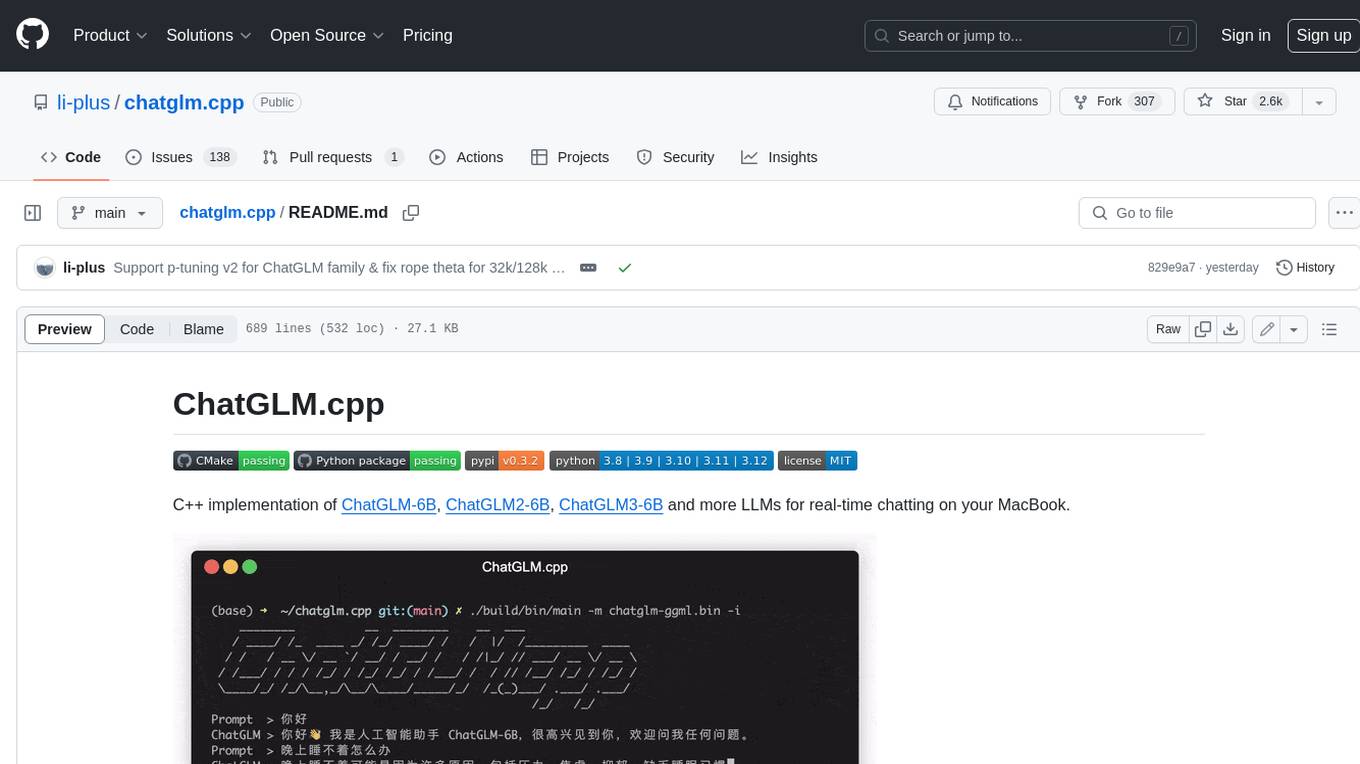
chatglm.cpp
ChatGLM.cpp is a C++ implementation of ChatGLM-6B, ChatGLM2-6B, ChatGLM3-6B and more LLMs for real-time chatting on your MacBook. It is based on ggml, working in the same way as llama.cpp. ChatGLM.cpp features accelerated memory-efficient CPU inference with int4/int8 quantization, optimized KV cache and parallel computing. It also supports P-Tuning v2 and LoRA finetuned models, streaming generation with typewriter effect, Python binding, web demo, api servers and more possibilities.
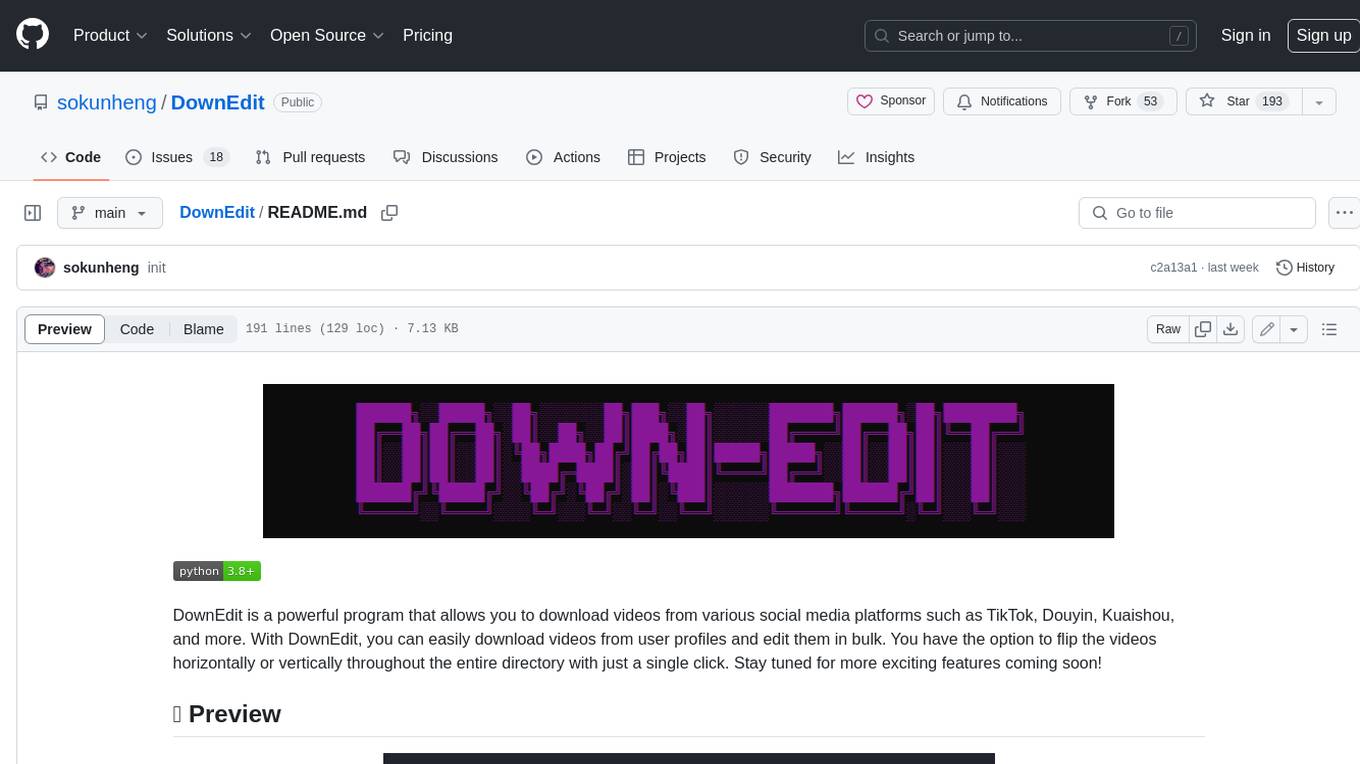
DownEdit
DownEdit is a powerful program that allows you to download videos from various social media platforms such as TikTok, Douyin, Kuaishou, and more. With DownEdit, you can easily download videos from user profiles and edit them in bulk. You have the option to flip the videos horizontally or vertically throughout the entire directory with just a single click. Stay tuned for more exciting features coming soon!
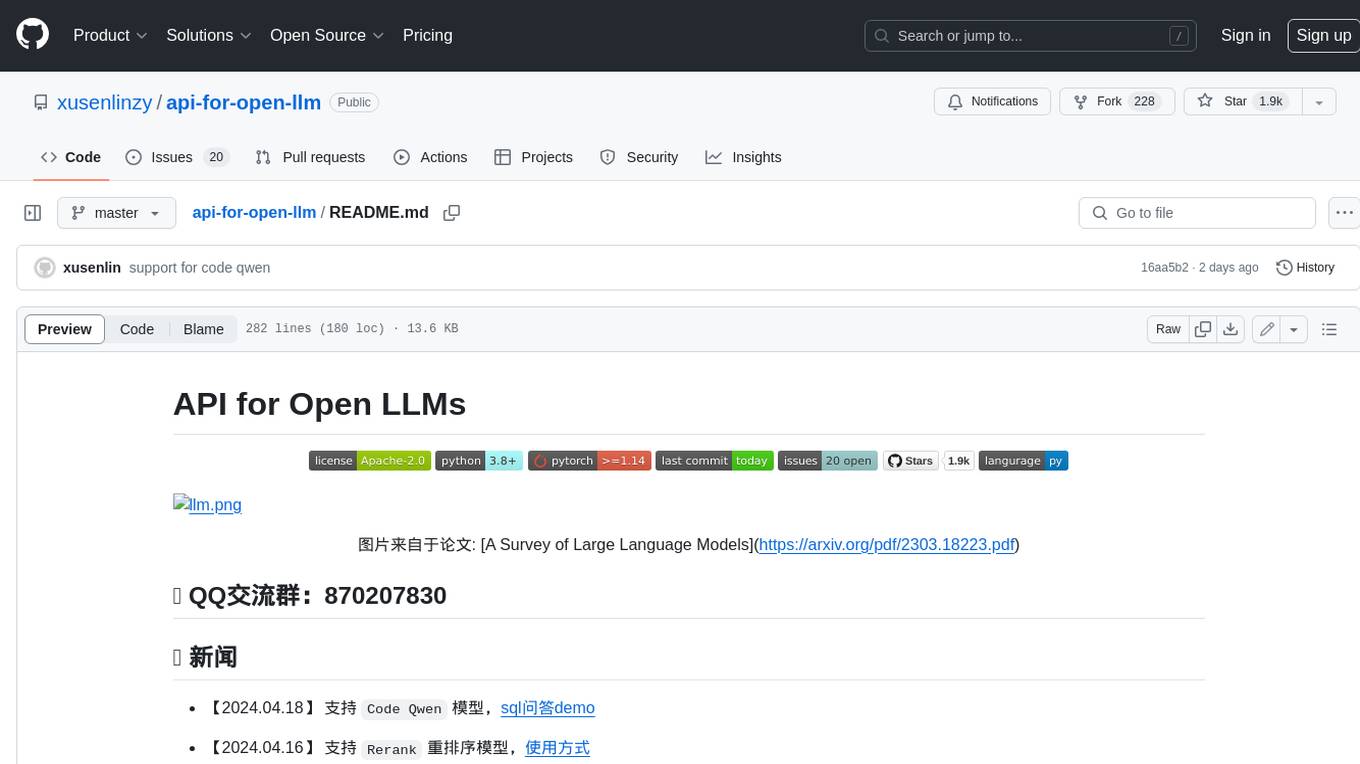
api-for-open-llm
This project provides a unified backend interface for open large language models (LLMs), offering a consistent experience with OpenAI's ChatGPT API. It supports various open-source LLMs, enabling developers to seamlessly integrate them into their applications. The interface features streaming responses, text embedding capabilities, and support for LangChain, a tool for developing LLM-based applications. By modifying environment variables, developers can easily use open-source models as alternatives to ChatGPT, providing a cost-effective and customizable solution for various use cases.
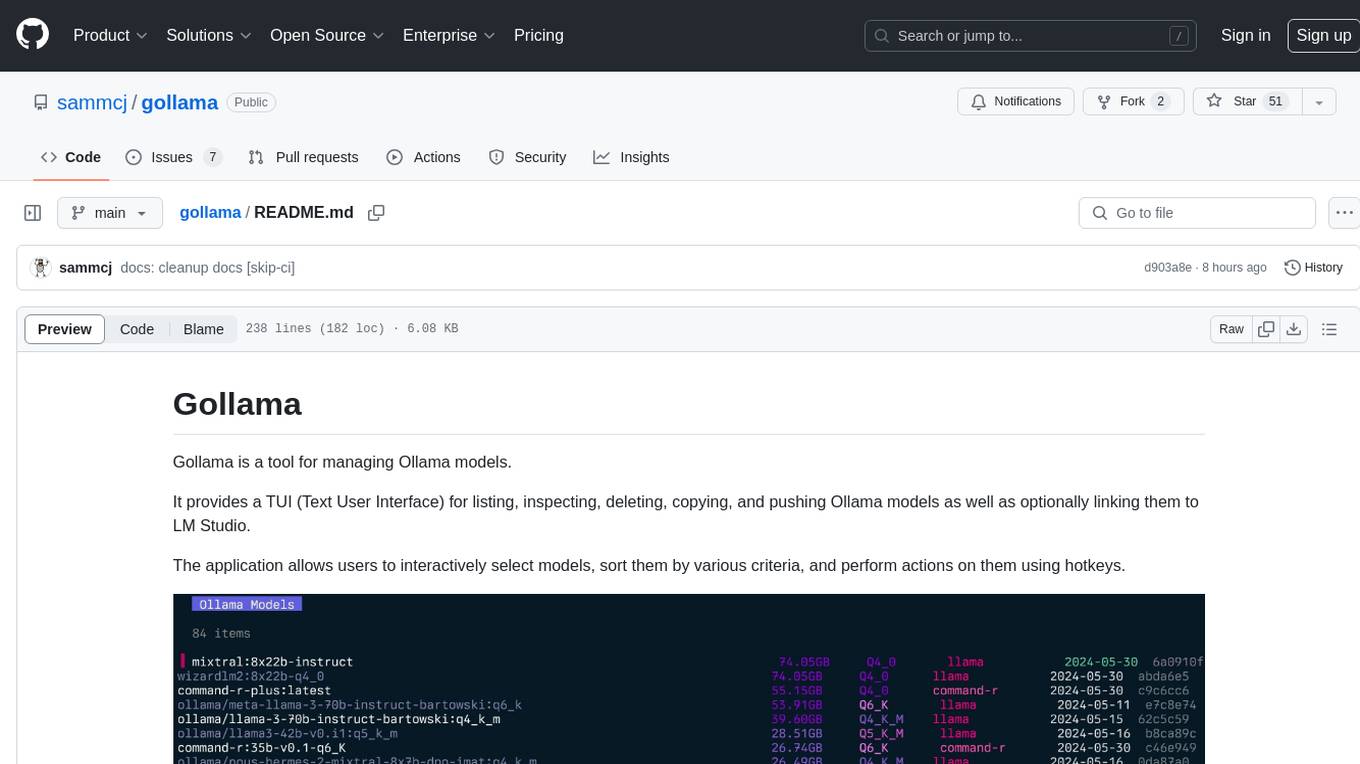
gollama
Gollama is a tool designed for managing Ollama models through a Text User Interface (TUI). Users can list, inspect, delete, copy, and push Ollama models, as well as link them to LM Studio. The application offers interactive model selection, sorting by various criteria, and actions using hotkeys. It provides features like sorting and filtering capabilities, displaying model metadata, model linking, copying, pushing, and more. Gollama aims to be user-friendly and useful for managing models, especially for cleaning up old models.
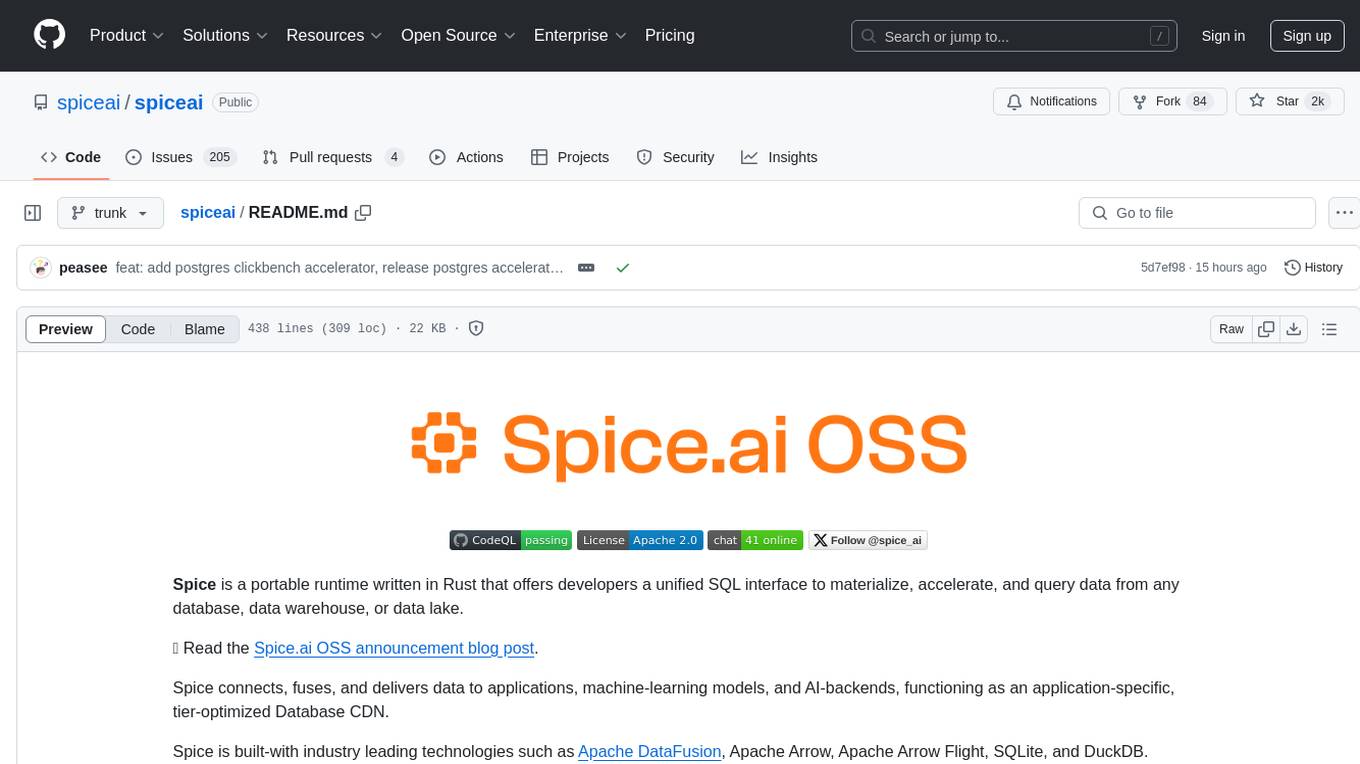
spiceai
Spice is a portable runtime written in Rust that offers developers a unified SQL interface to materialize, accelerate, and query data from any database, data warehouse, or data lake. It connects, fuses, and delivers data to applications, machine-learning models, and AI-backends, functioning as an application-specific, tier-optimized Database CDN. Built with industry-leading technologies such as Apache DataFusion, Apache Arrow, Apache Arrow Flight, SQLite, and DuckDB. Spice makes it fast and easy to query data from one or more sources using SQL, co-locating a managed dataset with applications or machine learning models, and accelerating it with Arrow in-memory, SQLite/DuckDB, or attached PostgreSQL for fast, high-concurrency, low-latency queries.
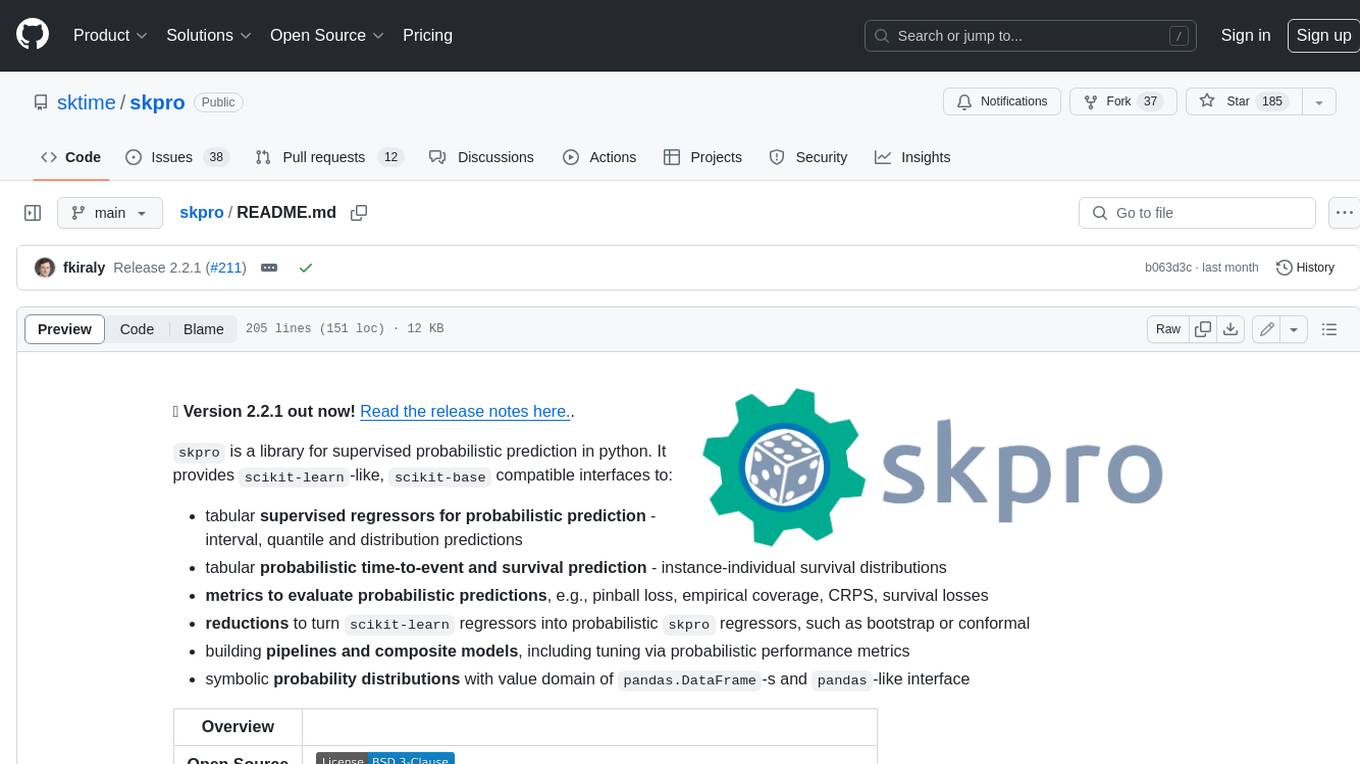
skpro
skpro is a library for supervised probabilistic prediction in python. It provides `scikit-learn`-like, `scikit-base` compatible interfaces to: * tabular **supervised regressors for probabilistic prediction** \- interval, quantile and distribution predictions * tabular **probabilistic time-to-event and survival prediction** \- instance-individual survival distributions * **metrics to evaluate probabilistic predictions** , e.g., pinball loss, empirical coverage, CRPS, survival losses * **reductions** to turn `scikit-learn` regressors into probabilistic `skpro` regressors, such as bootstrap or conformal * building **pipelines and composite models** , including tuning via probabilistic performance metrics * symbolic **probability distributions** with value domain of `pandas.DataFrame`-s and `pandas`-like interface
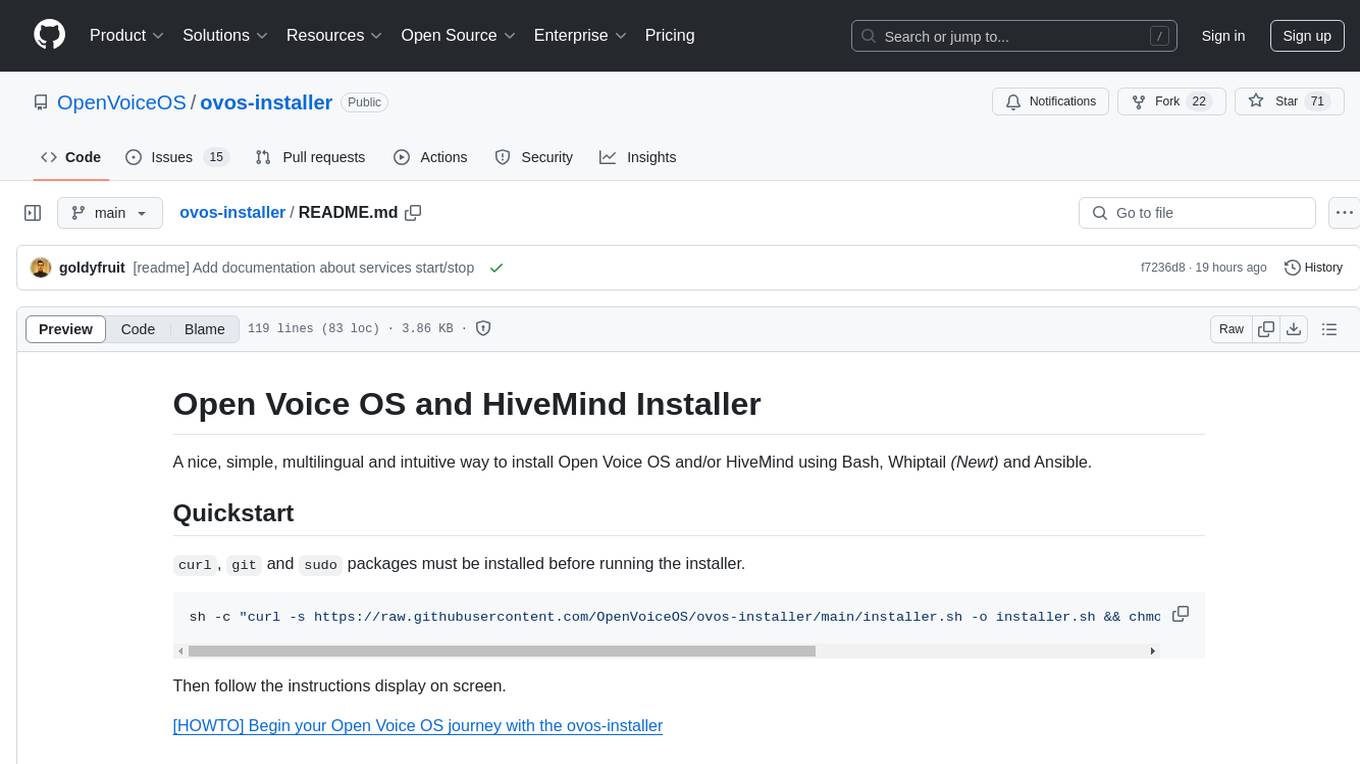
ovos-installer
The ovos-installer is a simple and multilingual tool designed to install Open Voice OS and HiveMind using Bash, Whiptail, and Ansible. It supports various Linux distributions and provides an automated installation process. Users can easily start and stop services, update their Open Voice OS instance, and uninstall the tool if needed. The installer also allows for non-interactive installation through scenario files. It offers a user-friendly way to set up Open Voice OS on different systems.
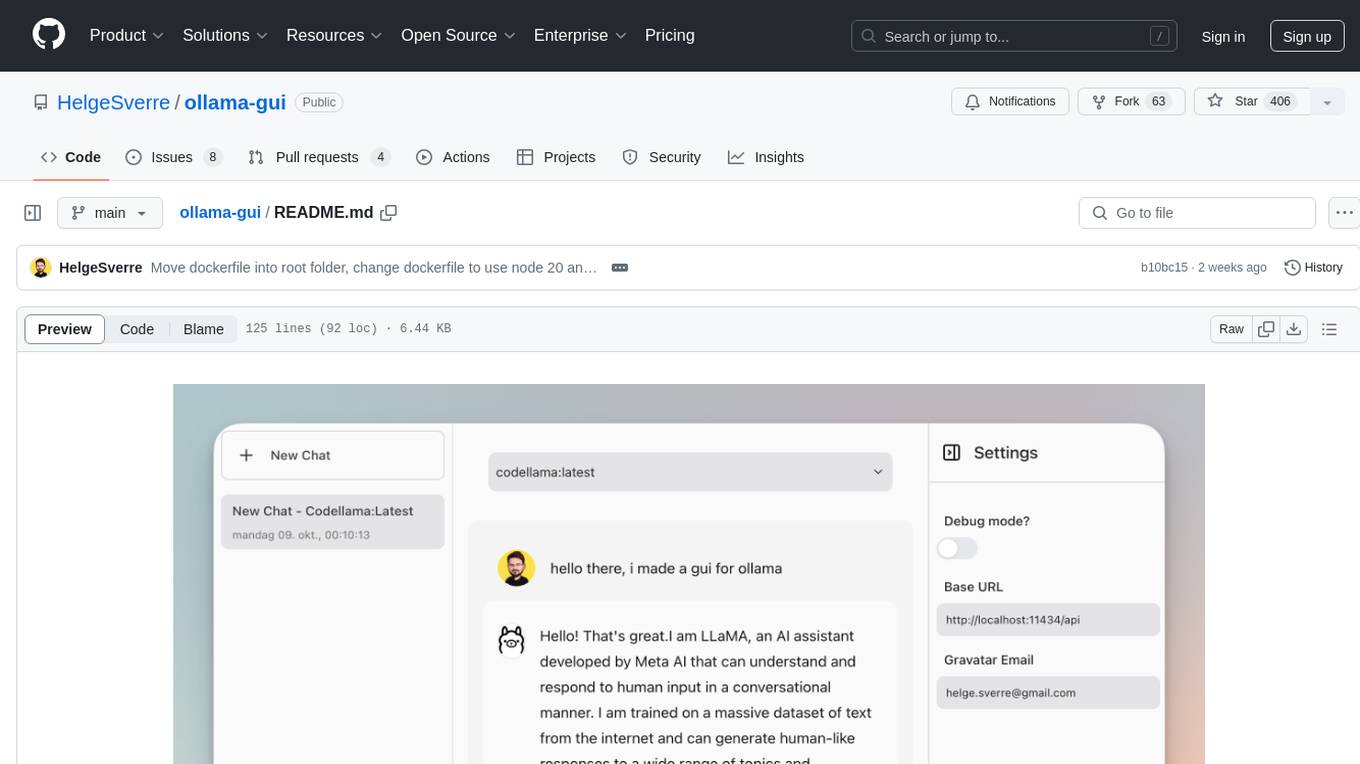
ollama-gui
Ollama GUI is a web interface for ollama.ai, a tool that enables running Large Language Models (LLMs) on your local machine. It provides a user-friendly platform for chatting with LLMs and accessing various models for text generation. Users can easily interact with different models, manage chat history, and explore available models through the web interface. The tool is built with Vue.js, Vite, and Tailwind CSS, offering a modern and responsive design for seamless user experience.
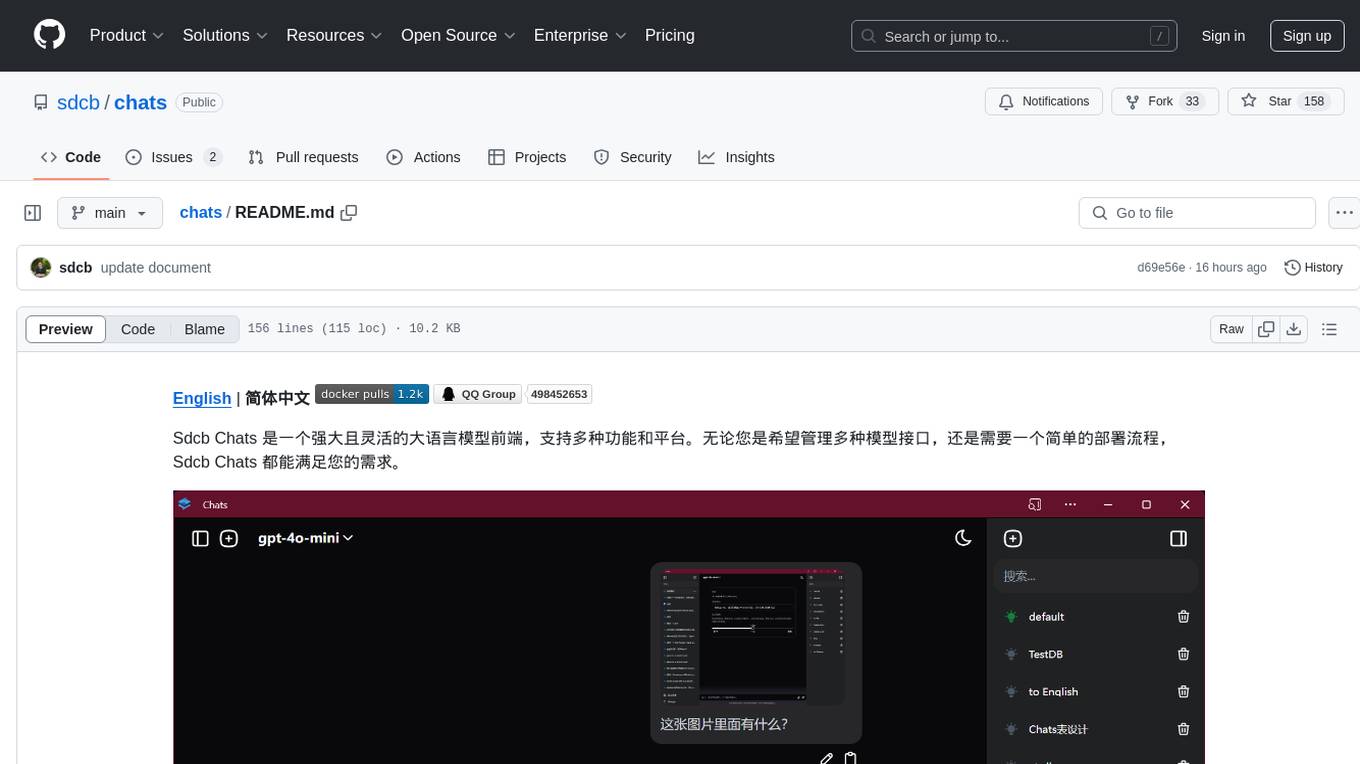
chats
Sdcb Chats is a powerful and flexible frontend for large language models, supporting multiple functions and platforms. Whether you want to manage multiple model interfaces or need a simple deployment process, Sdcb Chats can meet your needs. It supports dynamic management of multiple large language model interfaces, integrates visual models to enhance user interaction experience, provides fine-grained user permission settings for security, real-time tracking and management of user account balances, easy addition, deletion, and configuration of models, transparently forwards user chat requests based on the OpenAI protocol, supports multiple databases including SQLite, SQL Server, and PostgreSQL, compatible with various file services such as local files, AWS S3, Minio, Aliyun OSS, Azure Blob Storage, and supports multiple login methods including Keycloak SSO and phone SMS verification.
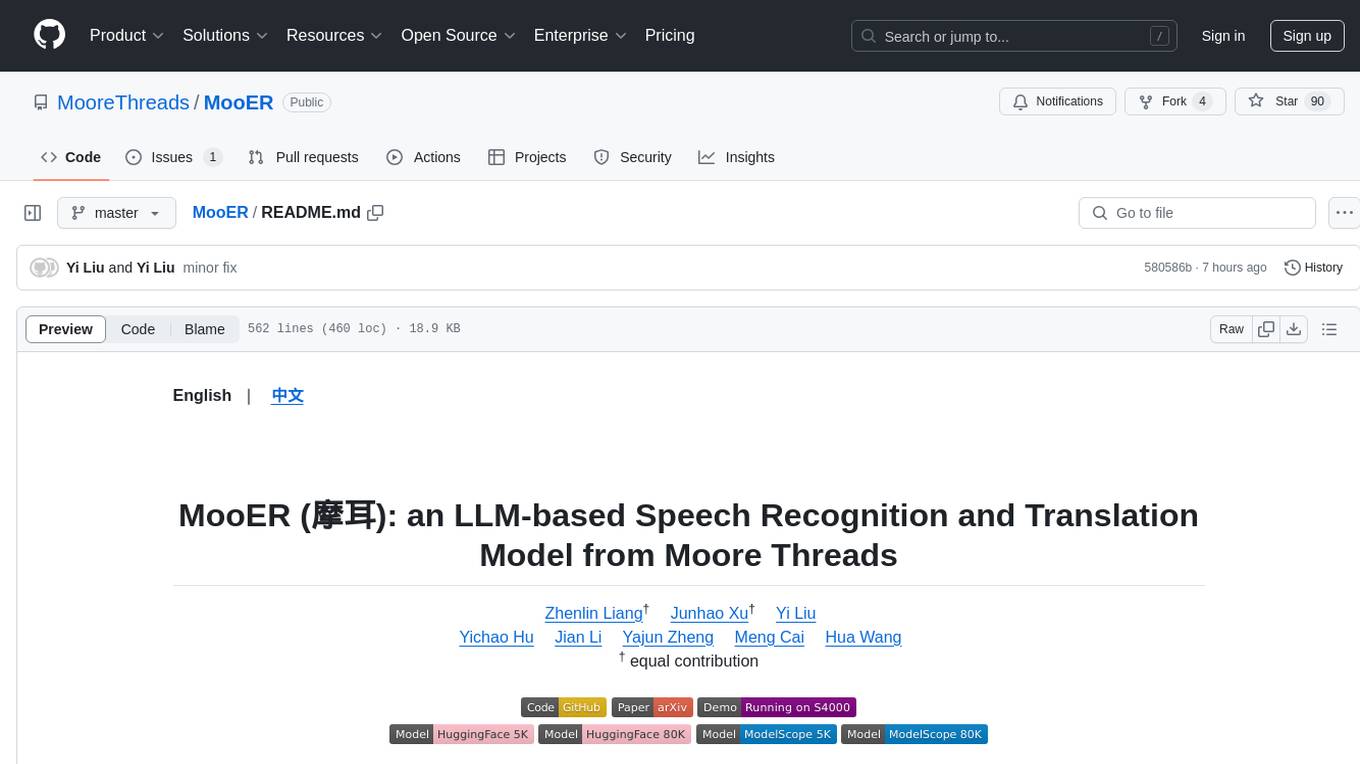
MooER
MooER (æŠčŗ) is an LLM-based speech recognition and translation model developed by Moore Threads. It allows users to transcribe speech into text (ASR) and translate speech into other languages (AST) in an end-to-end manner. The model was trained using 5K hours of data and is now also available with an 80K hours version. MooER is the first LLM-based speech model trained and inferred using domestic GPUs. The repository includes pretrained models, inference code, and a Gradio demo for a better user experience.
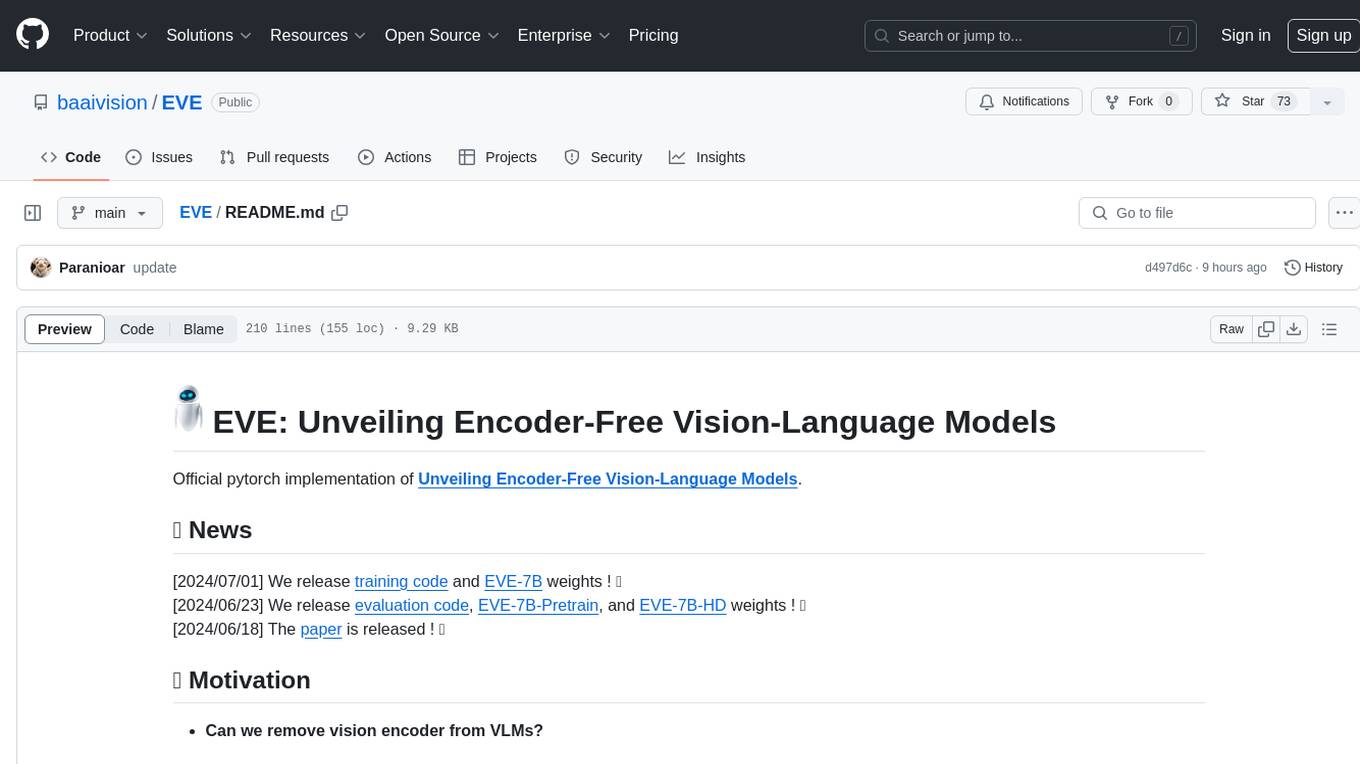
EVE
EVE is an official PyTorch implementation of Unveiling Encoder-Free Vision-Language Models. The project aims to explore the removal of vision encoders from Vision-Language Models (VLMs) and transfer LLMs to encoder-free VLMs efficiently. It also focuses on bridging the performance gap between encoder-free and encoder-based VLMs. EVE offers a superior capability with arbitrary image aspect ratio, data efficiency by utilizing publicly available data for pre-training, and training efficiency with a transparent and practical strategy for developing a pure decoder-only architecture across modalities.
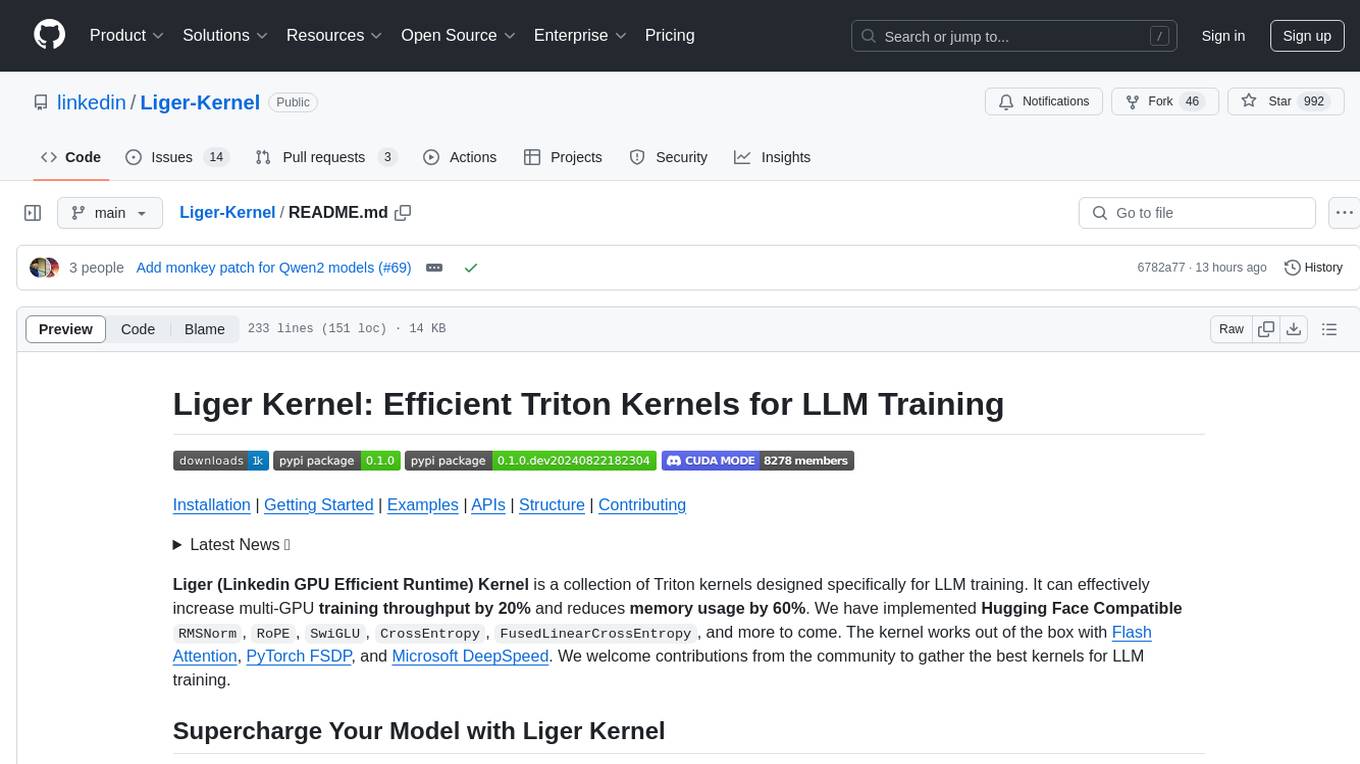
Liger-Kernel
Liger Kernel is a collection of Triton kernels designed for LLM training, increasing training throughput by 20% and reducing memory usage by 60%. It includes Hugging Face Compatible modules like RMSNorm, RoPE, SwiGLU, CrossEntropy, and FusedLinearCrossEntropy. The tool works with Flash Attention, PyTorch FSDP, and Microsoft DeepSpeed, aiming to enhance model efficiency and performance for researchers, ML practitioners, and curious novices.
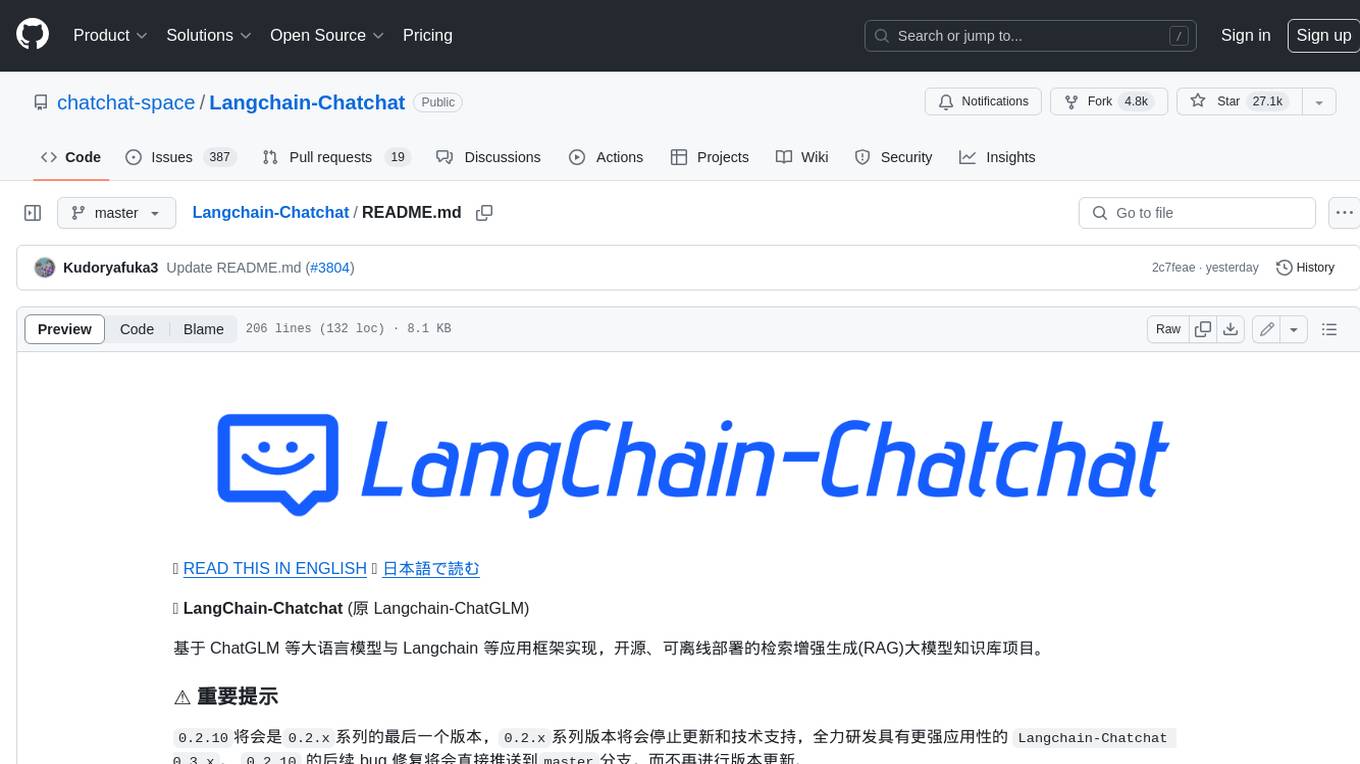
Langchain-Chatchat
LangChain-Chatchat is an open-source, offline-deployable retrieval-enhanced generation (RAG) large model knowledge base project based on large language models such as ChatGLM and application frameworks such as Langchain. It aims to establish a knowledge base Q&A solution that is friendly to Chinese scenarios, supports open-source models, and can run offline.
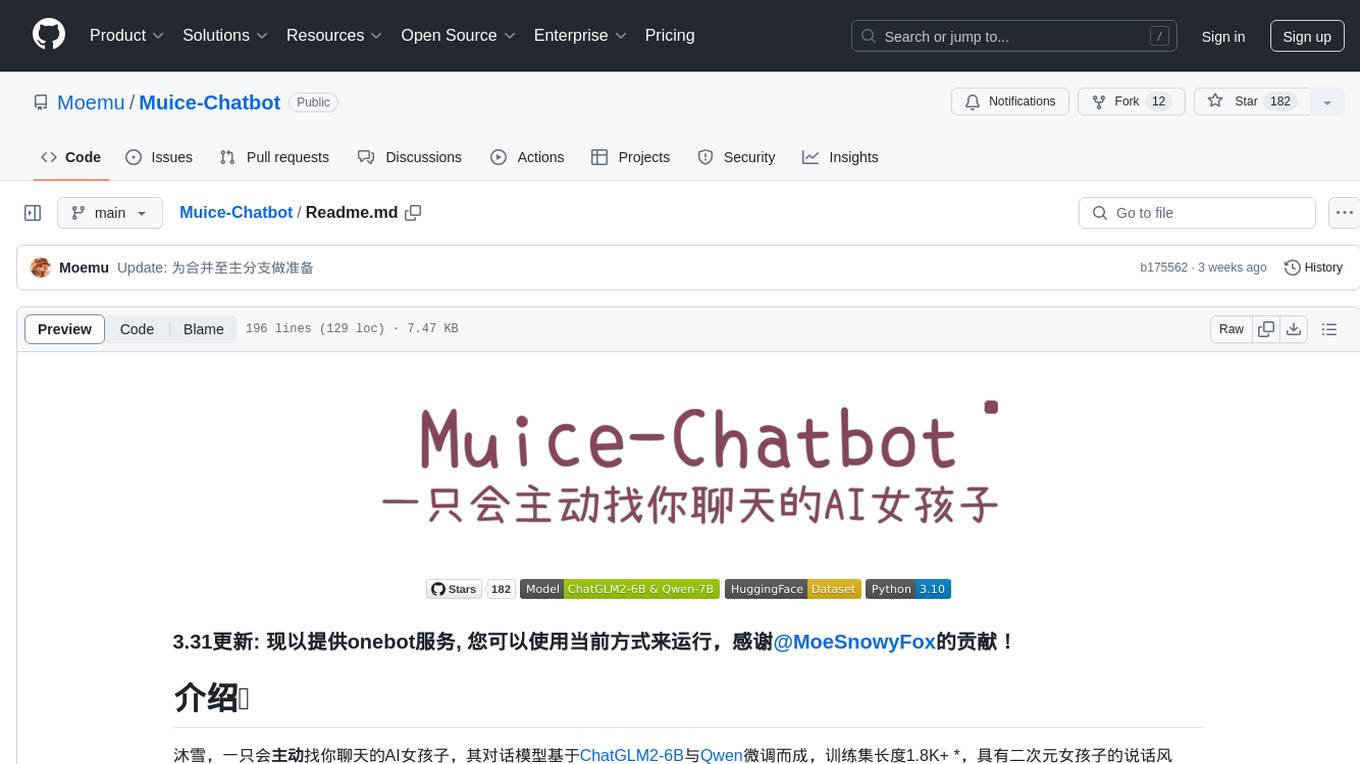
Muice-Chatbot
Muice-Chatbot is an AI chatbot designed to proactively engage in conversations with users. It is based on the ChatGLM2-6B and Qwen-7B models, with a training dataset of 1.8K+ dialogues. The chatbot has a speaking style similar to a 2D girl, being somewhat tsundere but willing to share daily life details and greet users differently every day. It provides various functionalities, including initiating chats and offering 5 available commands. The project supports model loading through different methods and provides onebot service support for QQ users. Users can interact with the chatbot by running the main.py file in the project directory.
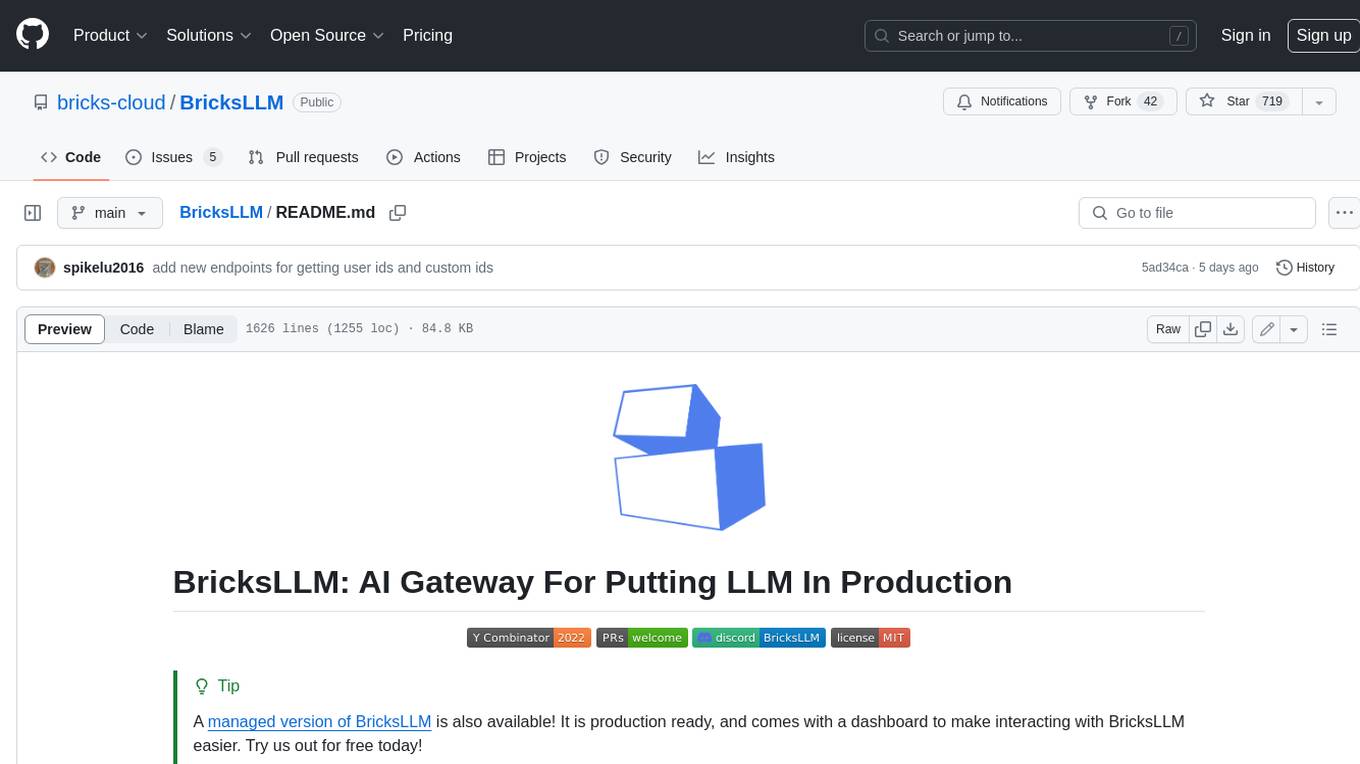
BricksLLM
BricksLLM is a cloud native AI gateway written in Go. Currently, it provides native support for OpenAI, Anthropic, Azure OpenAI and vLLM. BricksLLM aims to provide enterprise level infrastructure that can power any LLM production use cases. Here are some use cases for BricksLLM: * Set LLM usage limits for users on different pricing tiers * Track LLM usage on a per user and per organization basis * Block or redact requests containing PIIs * Improve LLM reliability with failovers, retries and caching * Distribute API keys with rate limits and cost limits for internal development/production use cases * Distribute API keys with rate limits and cost limits for students
For similar tasks
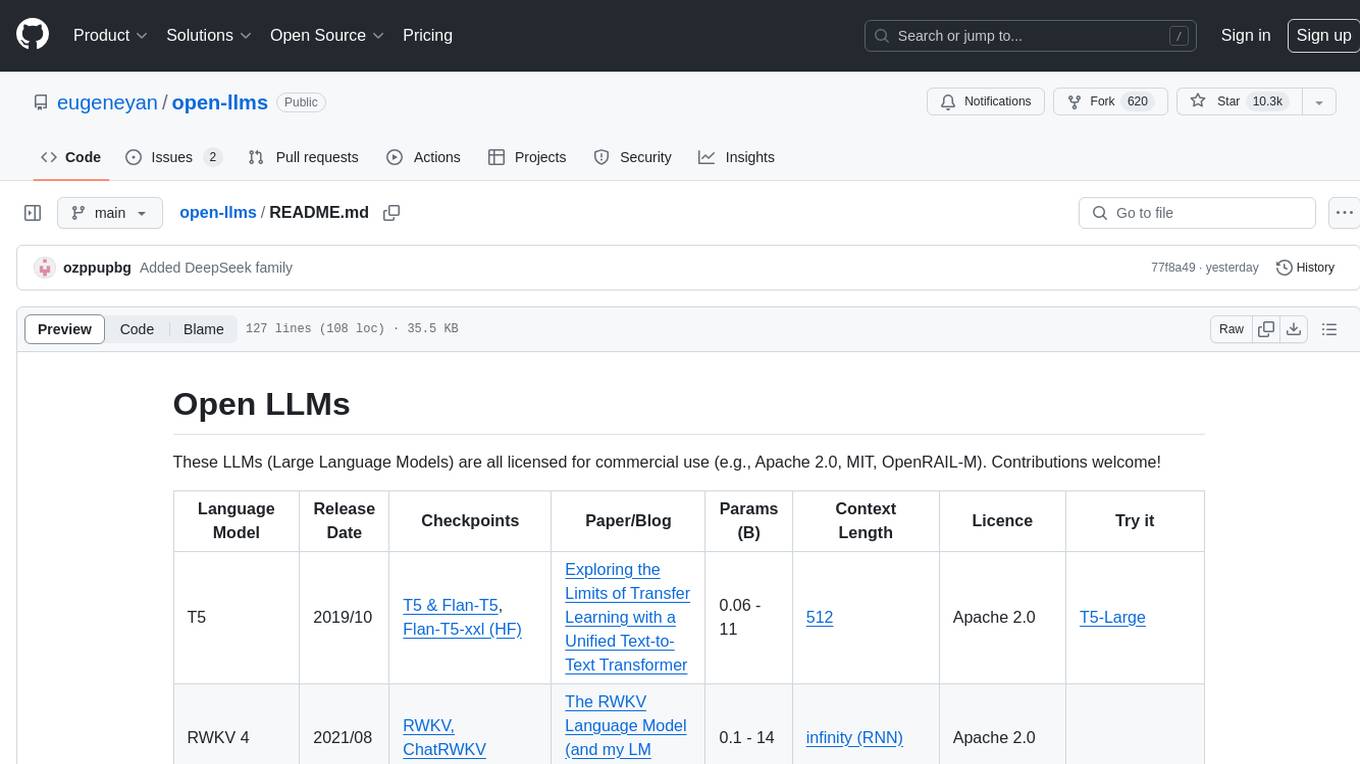
open-llms
Open LLMs is a repository containing various Large Language Models licensed for commercial use. It includes models like T5, GPT-NeoX, UL2, Bloom, Cerebras-GPT, Pythia, Dolly, and more. These models are designed for tasks such as transfer learning, language understanding, chatbot development, code generation, and more. The repository provides information on release dates, checkpoints, papers/blogs, parameters, context length, and licenses for each model. Contributions to the repository are welcome, and it serves as a resource for exploring the capabilities of different language models.
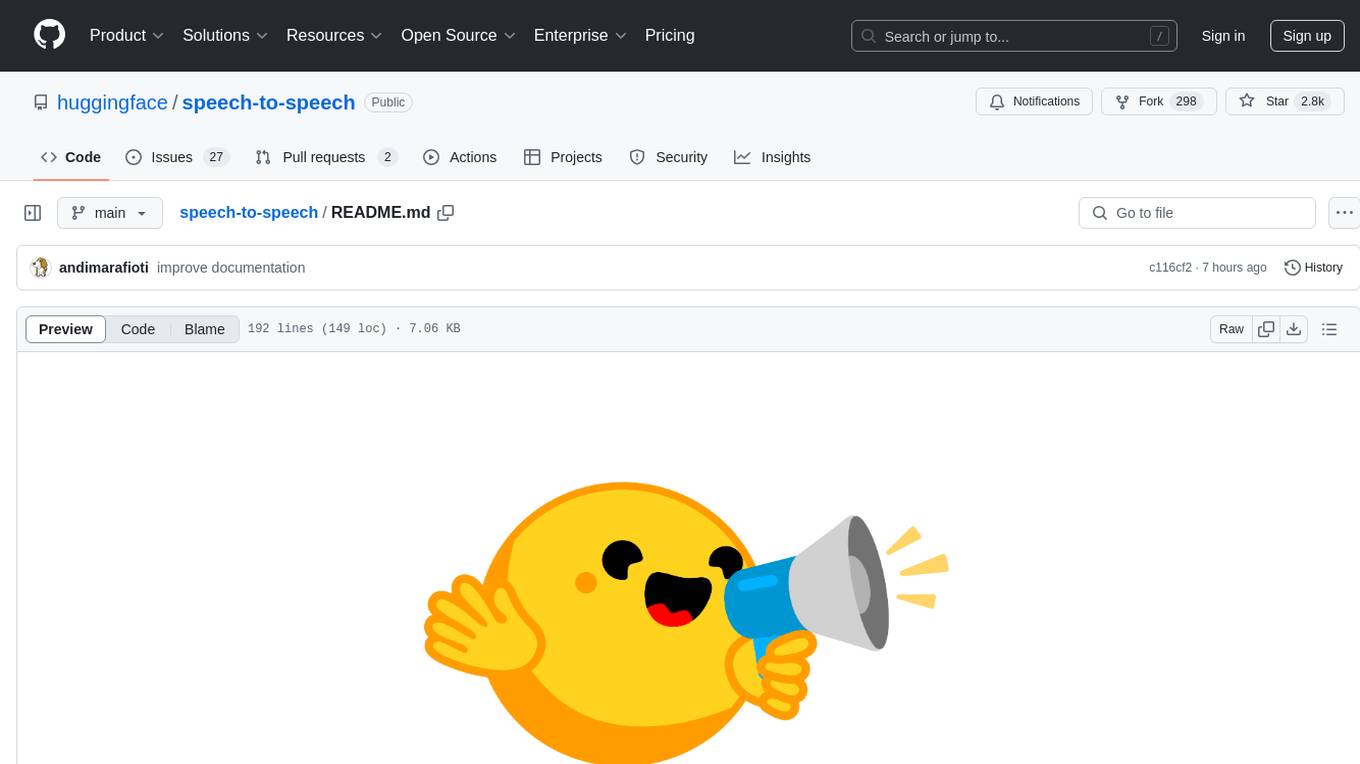
speech-to-speech
This repository implements a speech-to-speech cascaded pipeline with consecutive parts including Voice Activity Detection (VAD), Speech to Text (STT), Language Model (LM), and Text to Speech (TTS). It aims to provide a fully open and modular approach by leveraging models available on the Transformers library via the Hugging Face hub. The code is designed for easy modification, with each component implemented as a class. Users can run the pipeline either on a server/client approach or locally, with detailed setup and usage instructions provided in the readme.
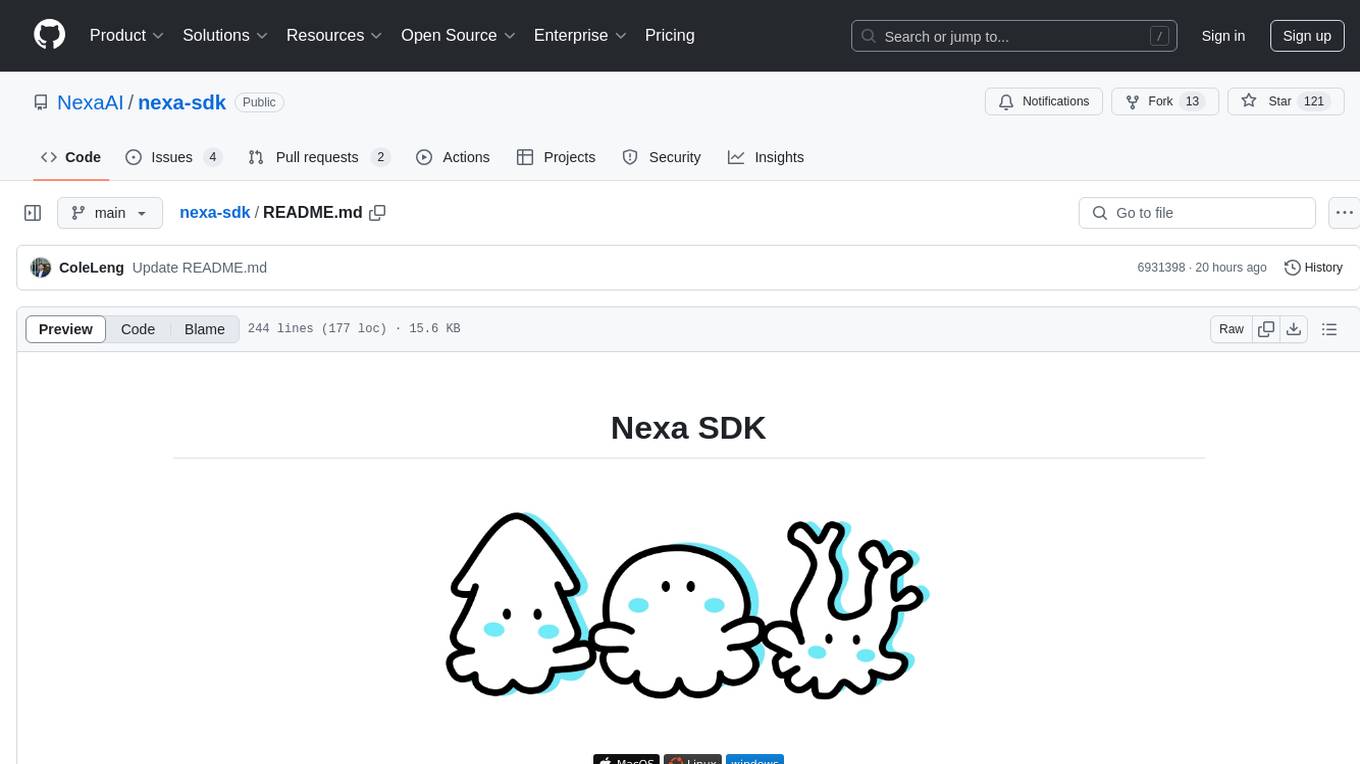
nexa-sdk
Nexa SDK is a comprehensive toolkit supporting ONNX and GGML models for text generation, image generation, vision-language models (VLM), and text-to-speech (TTS) capabilities. It offers an OpenAI-compatible API server with JSON schema mode and streaming support, along with a user-friendly Streamlit UI. Users can run Nexa SDK on any device with Python environment, with GPU acceleration supported. The toolkit provides model support, conversion engine, inference engine for various tasks, and differentiating features from other tools.
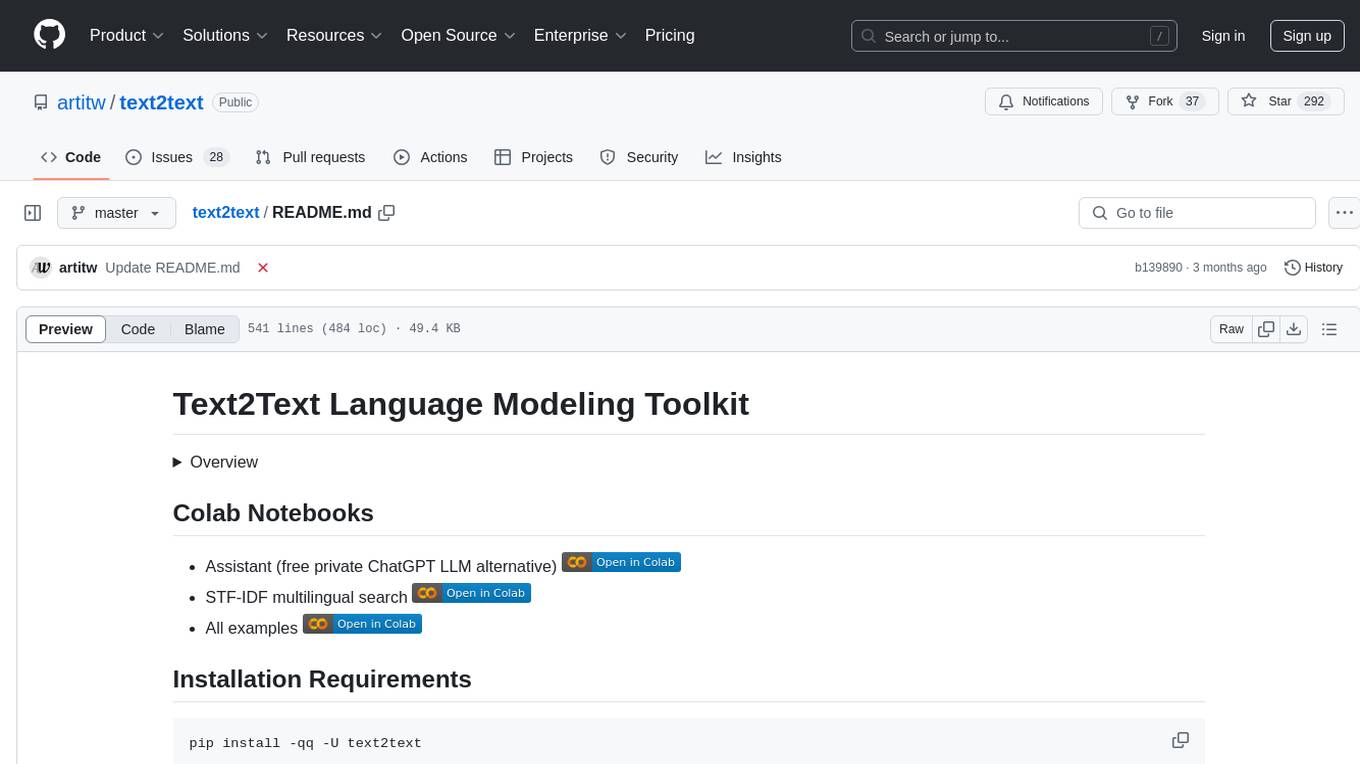
text2text
Text2Text is a comprehensive language modeling toolkit that offers a wide range of functionalities for text processing and generation. It provides tools for tokenization, embedding, TF-IDF calculations, BM25 scoring, indexing, translation, data augmentation, distance measurement, training/finetuning models, language identification, and serving models via a web server. The toolkit is designed to be user-friendly and efficient, offering a variety of features for natural language processing tasks.
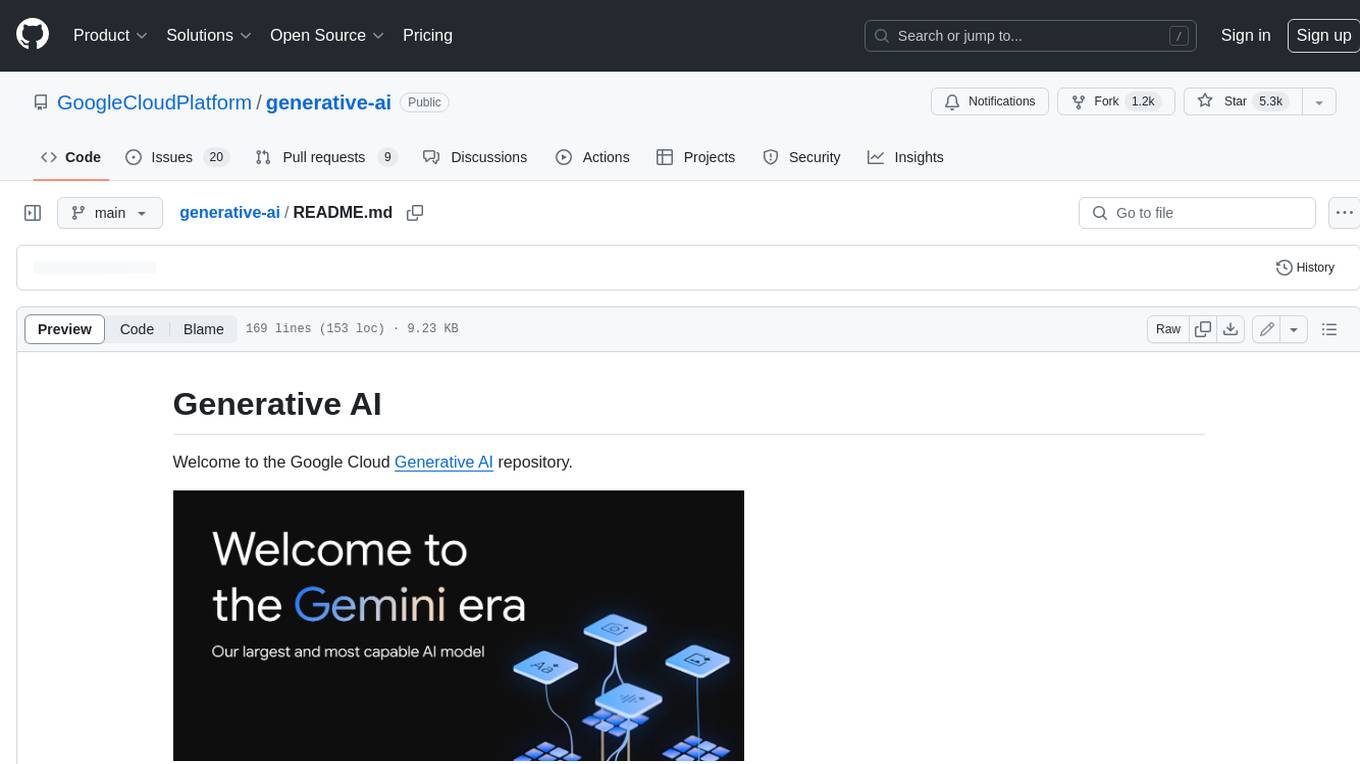
generative-ai
This repository contains notebooks, code samples, sample apps, and other resources that demonstrate how to use, develop and manage generative AI workflows using Generative AI on Google Cloud, powered by Vertex AI. For more Vertex AI samples, please visit the Vertex AI samples Github repository.
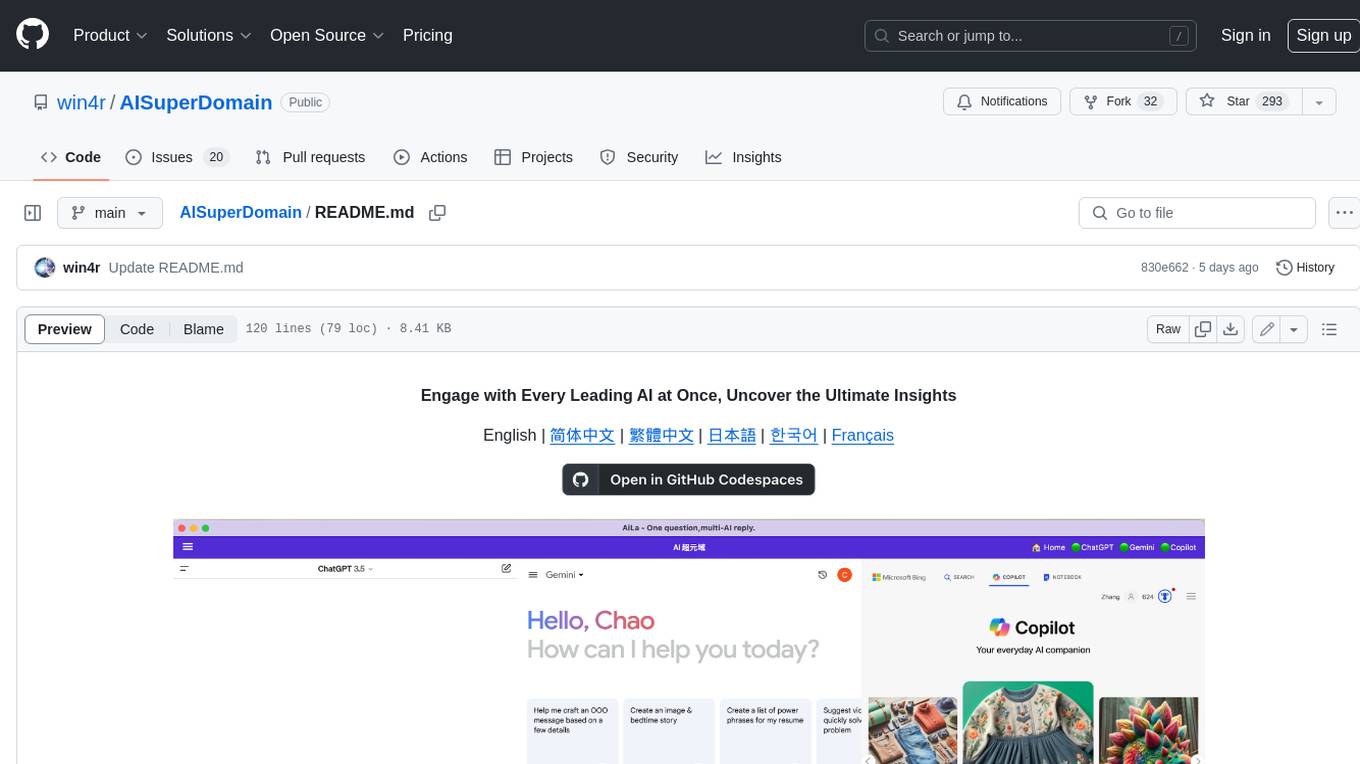
AISuperDomain
Aila Desktop Application is a powerful tool that integrates multiple leading AI models into a single desktop application. It allows users to interact with various AI models simultaneously, providing diverse responses and insights to their inquiries. With its user-friendly interface and customizable features, Aila empowers users to engage with AI seamlessly and efficiently. Whether you're a researcher, student, or professional, Aila can enhance your AI interactions and streamline your workflow.
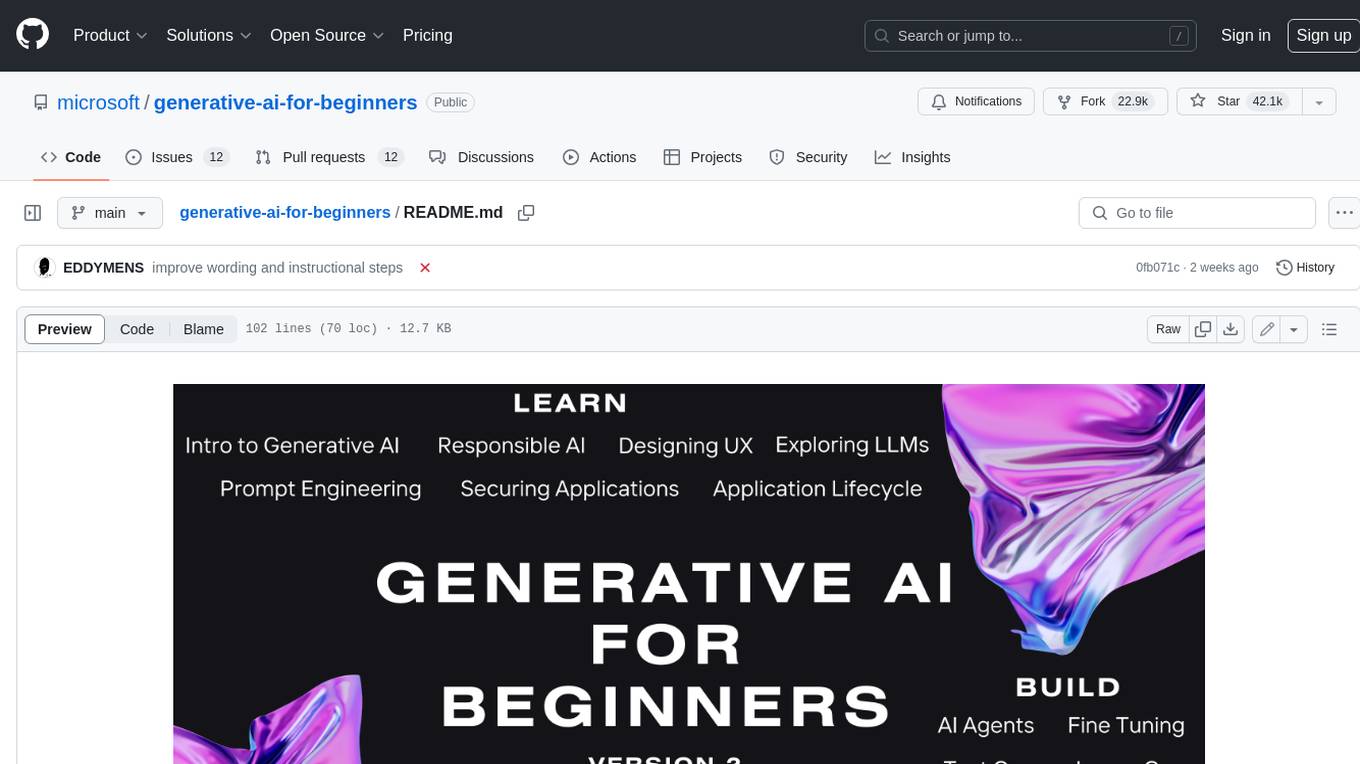
generative-ai-for-beginners
This course has 18 lessons. Each lesson covers its own topic so start wherever you like! Lessons are labeled either "Learn" lessons explaining a Generative AI concept or "Build" lessons that explain a concept and code examples in both **Python** and **TypeScript** when possible. Each lesson also includes a "Keep Learning" section with additional learning tools. **What You Need** * Access to the Azure OpenAI Service **OR** OpenAI API - _Only required to complete coding lessons_ * Basic knowledge of Python or Typescript is helpful - *For absolute beginners check out these Python and TypeScript courses. * A Github account to fork this entire repo to your own GitHub account We have created a **Course Setup** lesson to help you with setting up your development environment. Don't forget to star (đ) this repo to find it easier later. ## đ§ Ready to Deploy? If you are looking for more advanced code samples, check out our collection of Generative AI Code Samples in both **Python** and **TypeScript**. ## đŖī¸ Meet Other Learners, Get Support Join our official AI Discord server to meet and network with other learners taking this course and get support. ## đ Building a Startup? Sign up for Microsoft for Startups Founders Hub to receive **free OpenAI credits** and up to **$150k towards Azure credits to access OpenAI models through Azure OpenAI Services**. ## đ Want to help? Do you have suggestions or found spelling or code errors? Raise an issue or Create a pull request ## đ Each lesson includes: * A short video introduction to the topic * A written lesson located in the README * Python and TypeScript code samples supporting Azure OpenAI and OpenAI API * Links to extra resources to continue your learning ## đī¸ Lessons | | Lesson Link | Description | Additional Learning | | :-: | :------------------------------------------------------------------------------------------------------------------------------------------: | :---------------------------------------------------------------------------------------------: | ------------------------------------------------------------------------------ | | 00 | Course Setup | **Learn:** How to Setup Your Development Environment | Learn More | | 01 | Introduction to Generative AI and LLMs | **Learn:** Understanding what Generative AI is and how Large Language Models (LLMs) work. | Learn More | | 02 | Exploring and comparing different LLMs | **Learn:** How to select the right model for your use case | Learn More | | 03 | Using Generative AI Responsibly | **Learn:** How to build Generative AI Applications responsibly | Learn More | | 04 | Understanding Prompt Engineering Fundamentals | **Learn:** Hands-on Prompt Engineering Best Practices | Learn More | | 05 | Creating Advanced Prompts | **Learn:** How to apply prompt engineering techniques that improve the outcome of your prompts. | Learn More | | 06 | Building Text Generation Applications | **Build:** A text generation app using Azure OpenAI | Learn More | | 07 | Building Chat Applications | **Build:** Techniques for efficiently building and integrating chat applications. | Learn More | | 08 | Building Search Apps Vector Databases | **Build:** A search application that uses Embeddings to search for data. | Learn More | | 09 | Building Image Generation Applications | **Build:** A image generation application | Learn More | | 10 | Building Low Code AI Applications | **Build:** A Generative AI application using Low Code tools | Learn More | | 11 | Integrating External Applications with Function Calling | **Build:** What is function calling and its use cases for applications | Learn More | | 12 | Designing UX for AI Applications | **Learn:** How to apply UX design principles when developing Generative AI Applications | Learn More | | 13 | Securing Your Generative AI Applications | **Learn:** The threats and risks to AI systems and methods to secure these systems. | Learn More | | 14 | The Generative AI Application Lifecycle | **Learn:** The tools and metrics to manage the LLM Lifecycle and LLMOps | Learn More | | 15 | Retrieval Augmented Generation (RAG) and Vector Databases | **Build:** An application using a RAG Framework to retrieve embeddings from a Vector Databases | Learn More | | 16 | Open Source Models and Hugging Face | **Build:** An application using open source models available on Hugging Face | Learn More | | 17 | AI Agents | **Build:** An application using an AI Agent Framework | Learn More | | 18 | Fine-Tuning LLMs | **Learn:** The what, why and how of fine-tuning LLMs | Learn More |
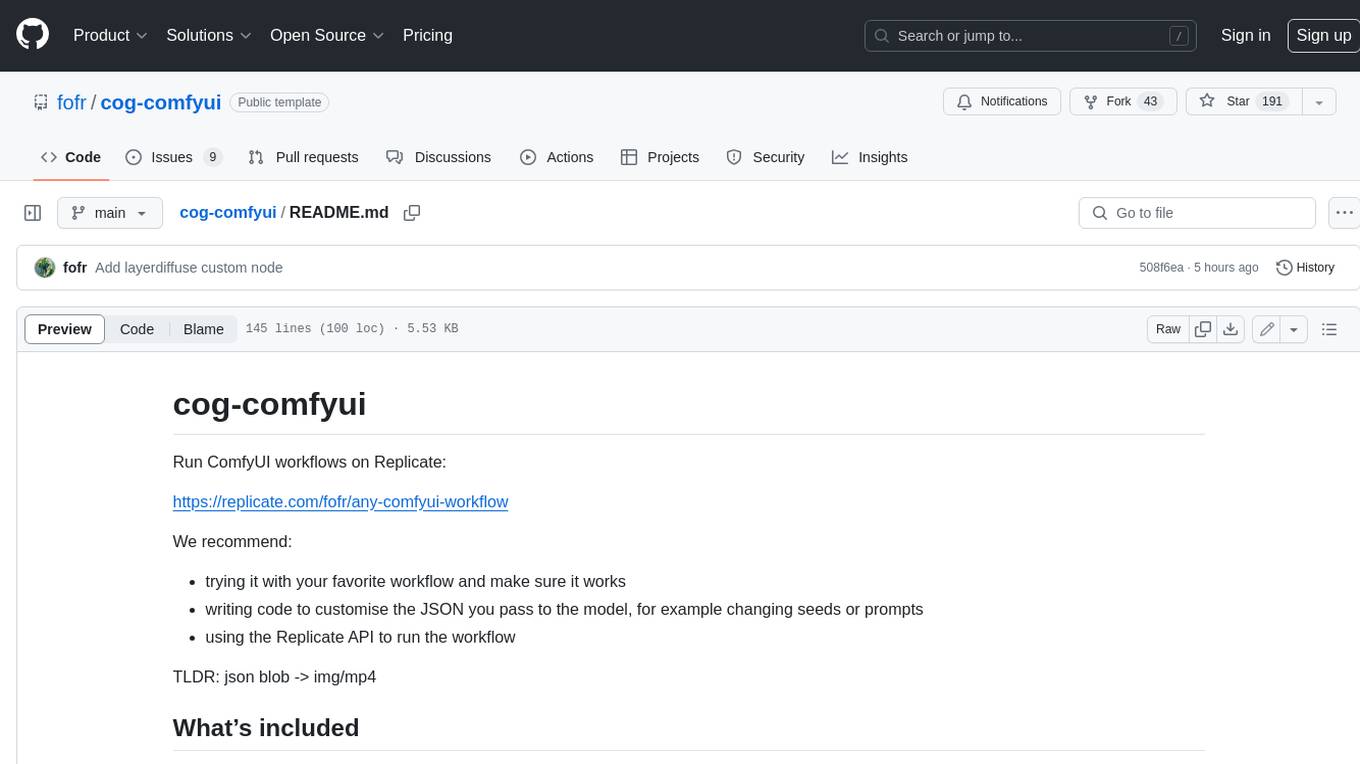
cog-comfyui
Cog-comfyui allows users to run ComfyUI workflows on Replicate. ComfyUI is a visual programming tool for creating and sharing generative art workflows. With cog-comfyui, users can access a variety of pre-trained models and custom nodes to create their own unique artworks. The tool is easy to use and does not require any coding experience. Users simply need to upload their API JSON file and any necessary input files, and then click the "Run" button. Cog-comfyui will then generate the output image or video file.
For similar jobs
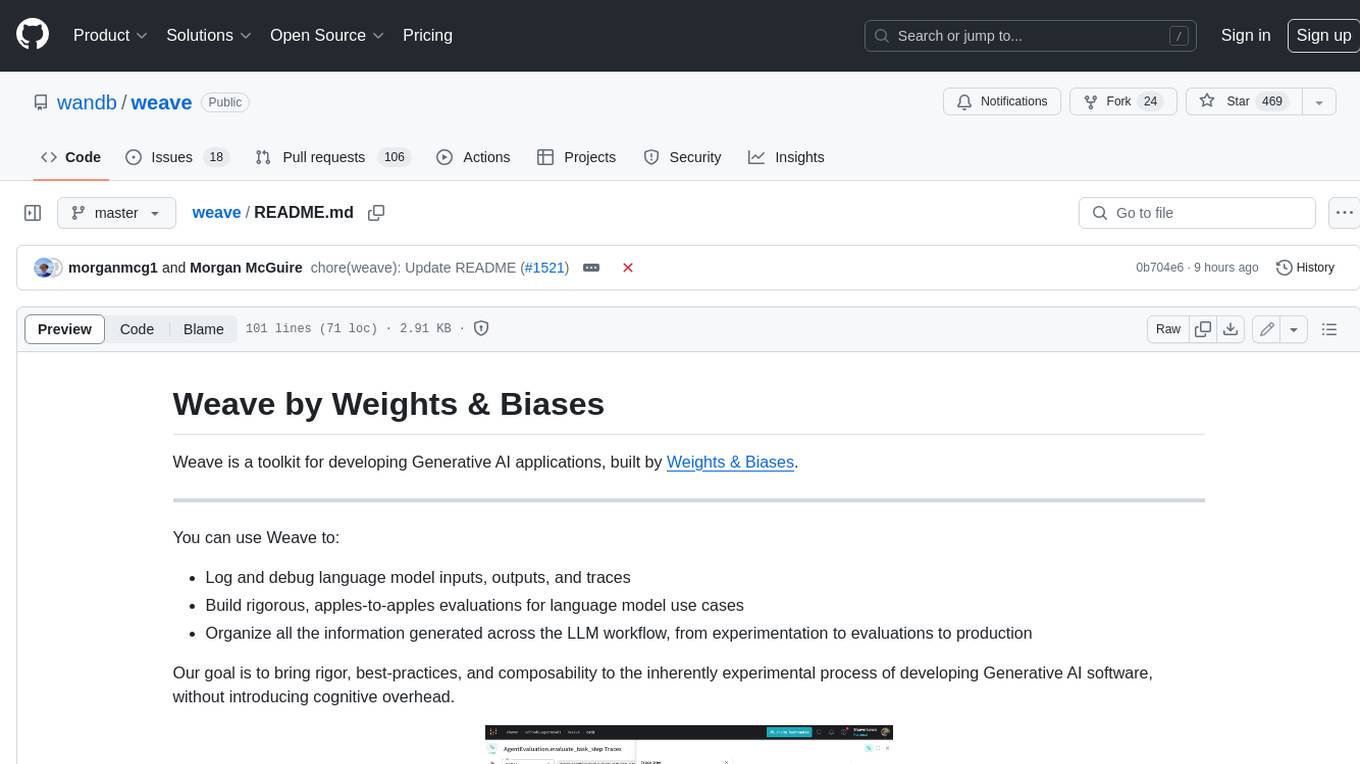
weave
Weave is a toolkit for developing Generative AI applications, built by Weights & Biases. With Weave, you can log and debug language model inputs, outputs, and traces; build rigorous, apples-to-apples evaluations for language model use cases; and organize all the information generated across the LLM workflow, from experimentation to evaluations to production. Weave aims to bring rigor, best-practices, and composability to the inherently experimental process of developing Generative AI software, without introducing cognitive overhead.
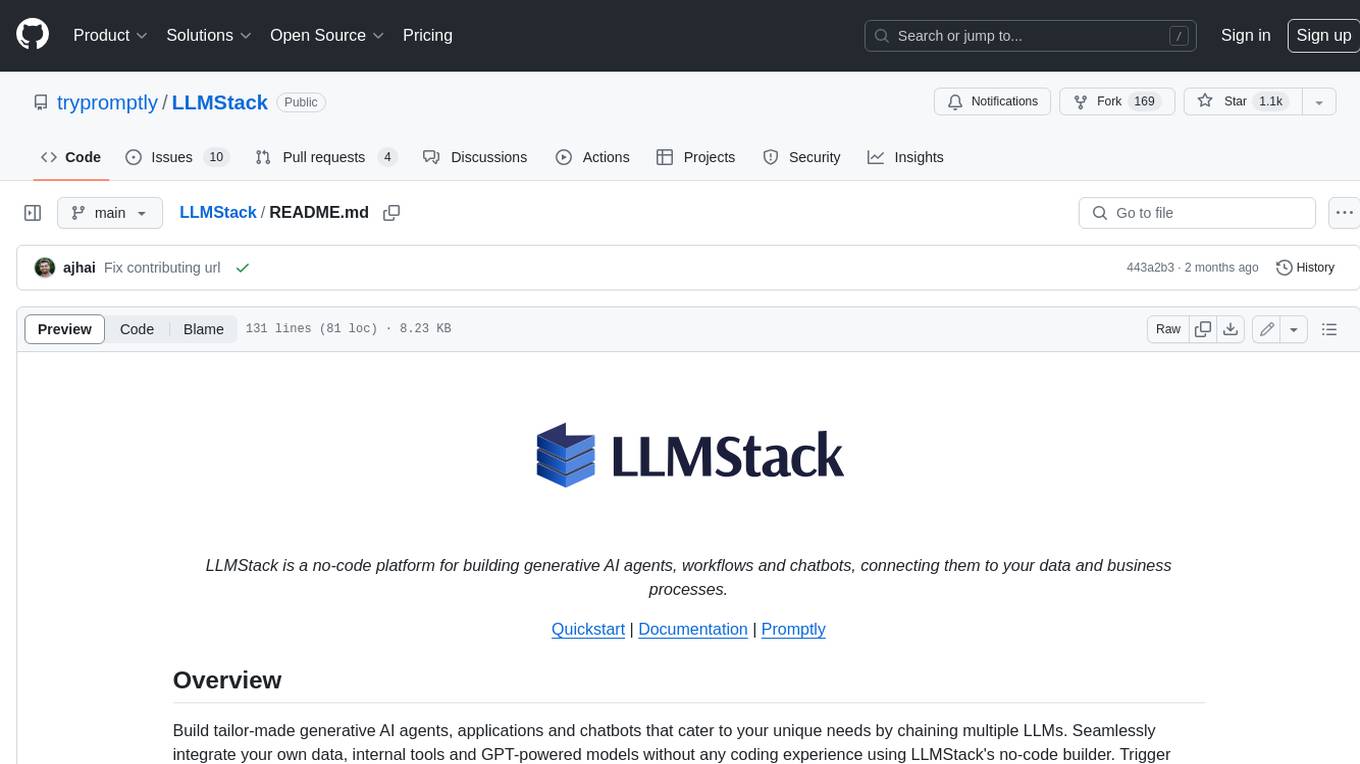
LLMStack
LLMStack is a no-code platform for building generative AI agents, workflows, and chatbots. It allows users to connect their own data, internal tools, and GPT-powered models without any coding experience. LLMStack can be deployed to the cloud or on-premise and can be accessed via HTTP API or triggered from Slack or Discord.
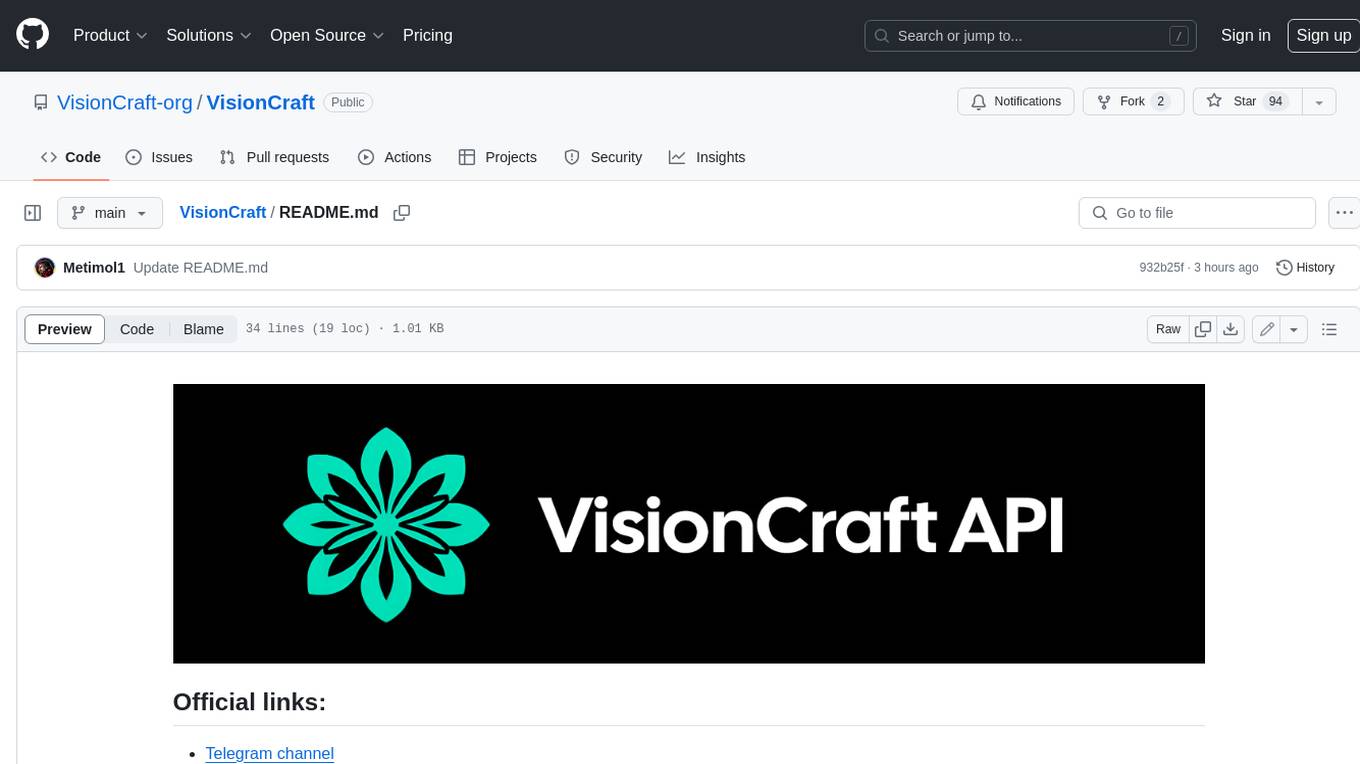
VisionCraft
The VisionCraft API is a free API for using over 100 different AI models. From images to sound.
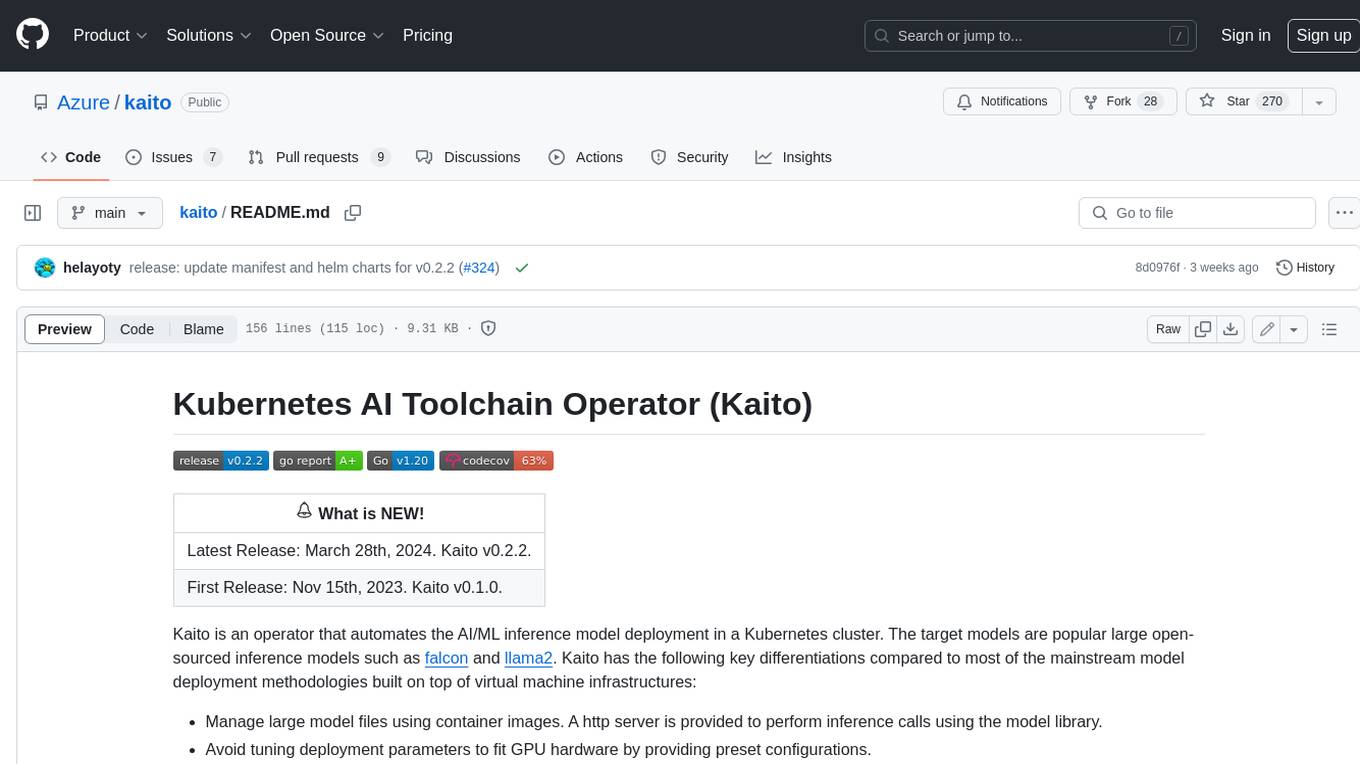
kaito
Kaito is an operator that automates the AI/ML inference model deployment in a Kubernetes cluster. It manages large model files using container images, avoids tuning deployment parameters to fit GPU hardware by providing preset configurations, auto-provisions GPU nodes based on model requirements, and hosts large model images in the public Microsoft Container Registry (MCR) if the license allows. Using Kaito, the workflow of onboarding large AI inference models in Kubernetes is largely simplified.
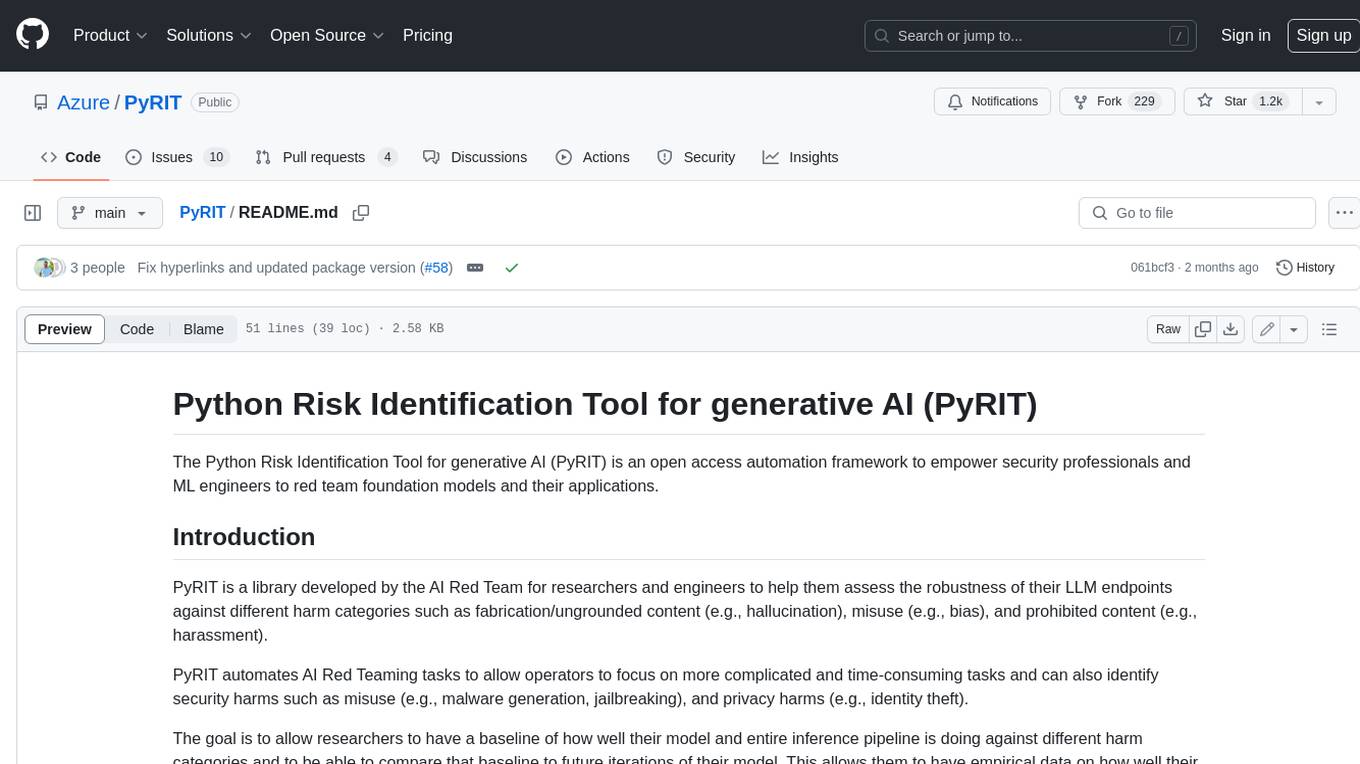
PyRIT
PyRIT is an open access automation framework designed to empower security professionals and ML engineers to red team foundation models and their applications. It automates AI Red Teaming tasks to allow operators to focus on more complicated and time-consuming tasks and can also identify security harms such as misuse (e.g., malware generation, jailbreaking), and privacy harms (e.g., identity theft). The goal is to allow researchers to have a baseline of how well their model and entire inference pipeline is doing against different harm categories and to be able to compare that baseline to future iterations of their model. This allows them to have empirical data on how well their model is doing today, and detect any degradation of performance based on future improvements.
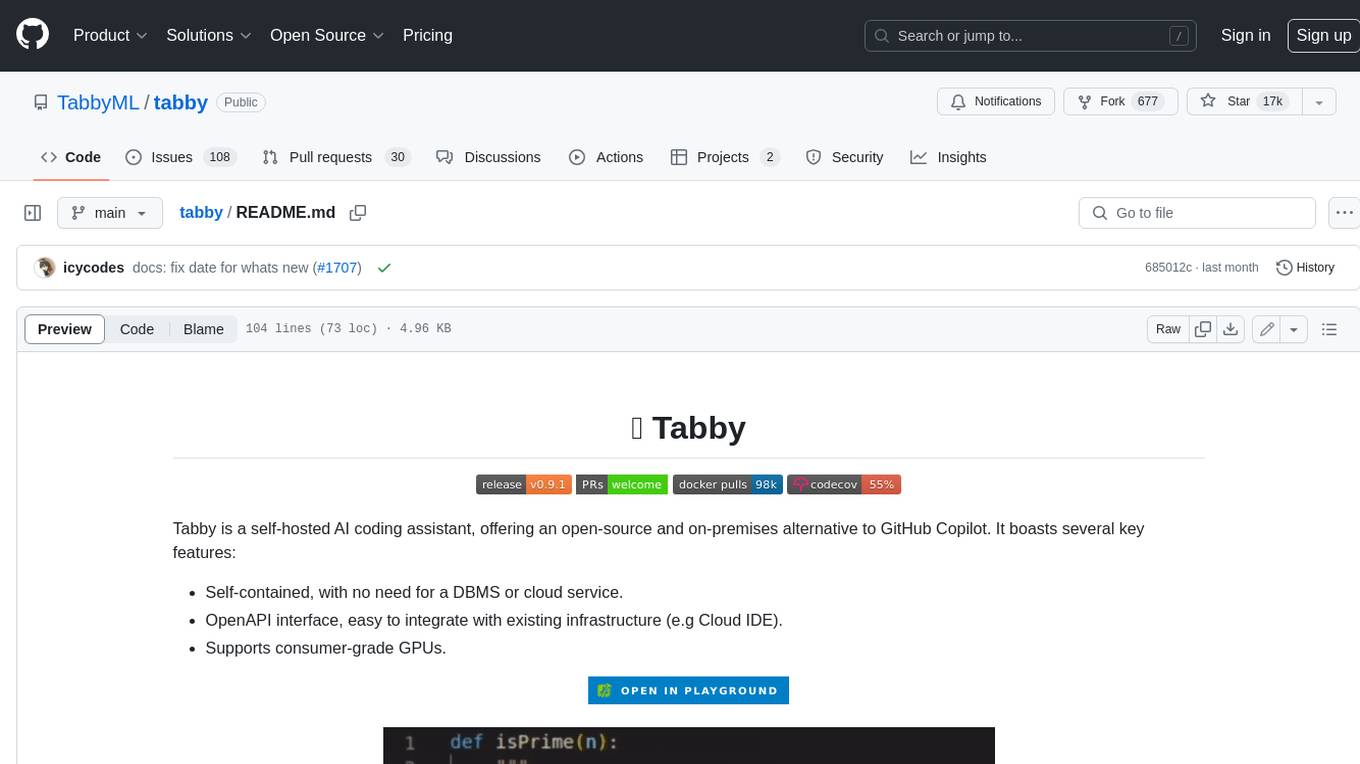
tabby
Tabby is a self-hosted AI coding assistant, offering an open-source and on-premises alternative to GitHub Copilot. It boasts several key features: * Self-contained, with no need for a DBMS or cloud service. * OpenAPI interface, easy to integrate with existing infrastructure (e.g Cloud IDE). * Supports consumer-grade GPUs.
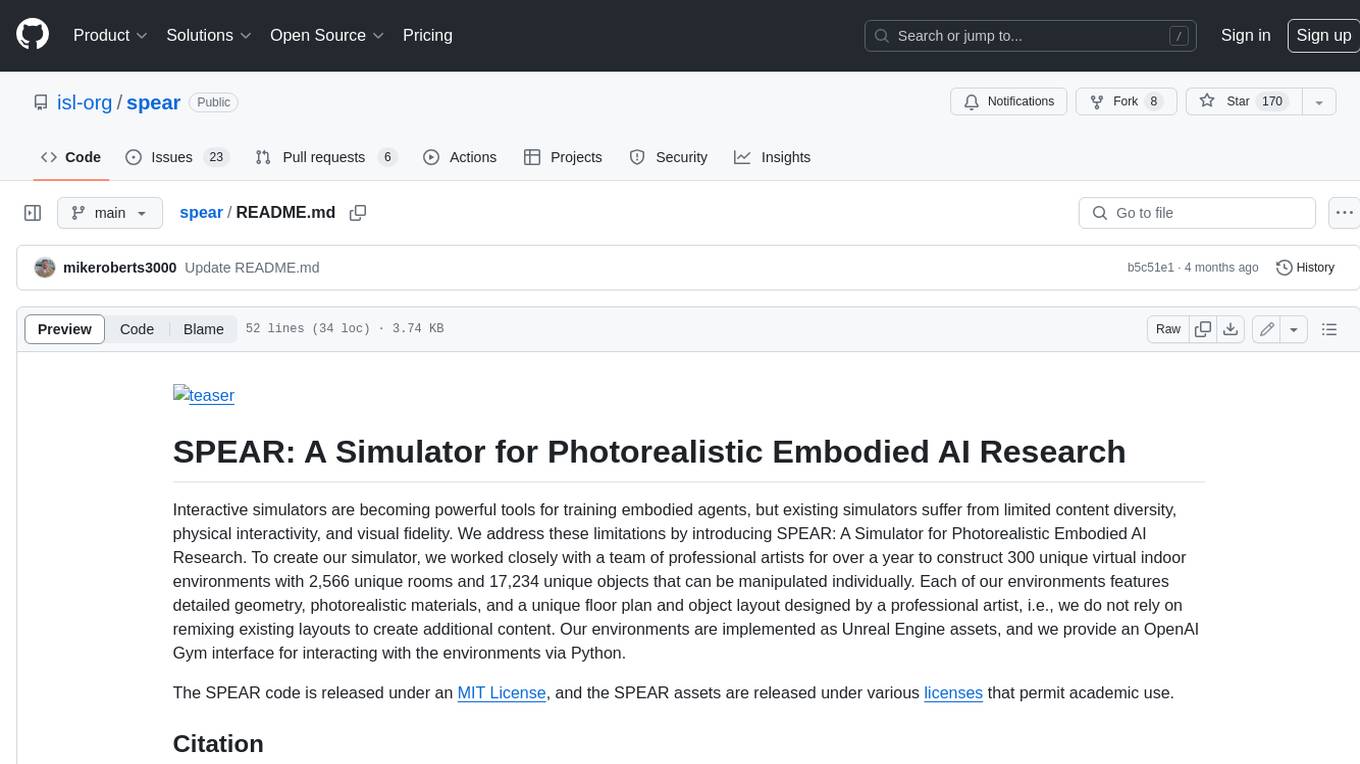
spear
SPEAR (Simulator for Photorealistic Embodied AI Research) is a powerful tool for training embodied agents. It features 300 unique virtual indoor environments with 2,566 unique rooms and 17,234 unique objects that can be manipulated individually. Each environment is designed by a professional artist and features detailed geometry, photorealistic materials, and a unique floor plan and object layout. SPEAR is implemented as Unreal Engine assets and provides an OpenAI Gym interface for interacting with the environments via Python.
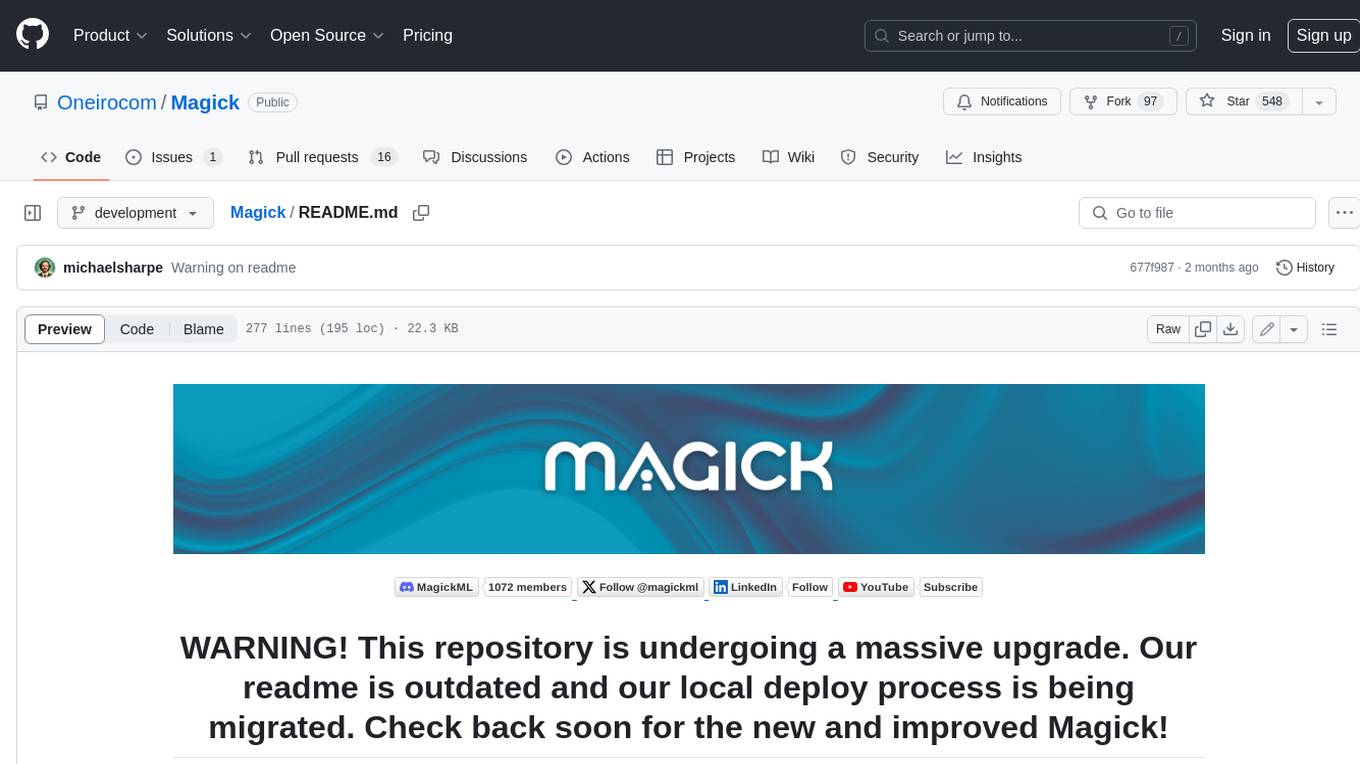
Magick
Magick is a groundbreaking visual AIDE (Artificial Intelligence Development Environment) for no-code data pipelines and multimodal agents. Magick can connect to other services and comes with nodes and templates well-suited for intelligent agents, chatbots, complex reasoning systems and realistic characters.