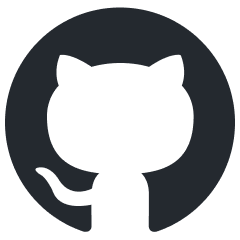
generative-ai
Sample code and notebooks for Generative AI on Google Cloud, with Gemini on Vertex AI
Stars: 10015
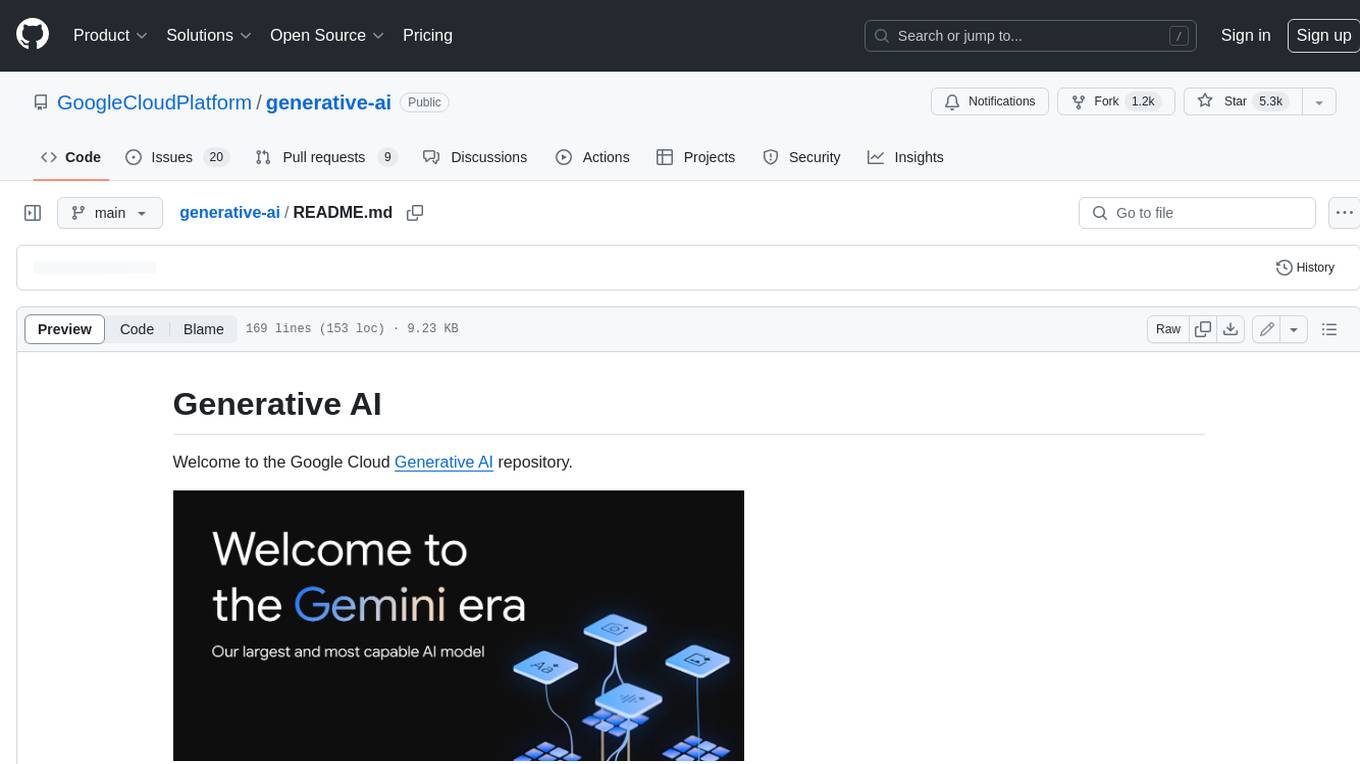
This repository contains notebooks, code samples, sample apps, and other resources that demonstrate how to use, develop and manage generative AI workflows using Generative AI on Google Cloud, powered by Vertex AI. For more Vertex AI samples, please visit the Vertex AI samples Github repository.
README:
NOTE: Gemini 2.5 Pro have been released!
Here are the latest notebooks and demos using the new models:
This repository contains notebooks, code samples, sample apps, and other resources that demonstrate how to use, develop and manage generative AI workflows using Generative AI on Google Cloud with Vertex AI.
Description | |
---|---|
gemini/
|
Discover Gemini through starter notebooks, use cases, function calling, sample apps, and more. |
search/
|
Use this folder if you're interested in using Vertex AI Search, a Google-managed solution to help you rapidly build search engines for websites and across enterprise data. (Formerly known as Enterprise Search on Generative AI App Builder). |
rag-grounding/
|
Use this folder for information on Retrieval Augmented Generation (RAG) and Grounding with Vertex AI. This is an index of notebooks and samples across other directories focused on this topic. |
vision/
|
Use this folder if you're interested in building your own solutions from scratch using features from Imagen on Vertex AI (Vertex AI Imagen API).
These are the features that Imagen on Vertex AI offers:
|
audio/
|
Use this folder if you're interested in building your own solutions from scratch using features from Chirp, a version of Google's Universal Speech Model (USM) on Vertex AI (Vertex AI Chirp API). |
setup-env/
|
Instructions on how to set up Google Cloud, the Vertex AI Python SDK, and notebook environments on Google Colab and Vertex AI Workbench. |
RESOURCES.md
|
Learning resources (e.g. blogs, YouTube playlists) about Generative AI on Google Cloud. |
-
🚀 Agent Starter Pack
- A collection of production-ready Generative AI Agent templates built for Google Cloud.
- It accelerates development by providing a holistic, production-ready solution, addressing common challenges (Deployment & Operations, Evaluation, Customization, Observability) in building and deploying Gen AI agents.
- Gemini Cookbook
- Google Cloud Applied AI Engineering
- Generative AI for Marketing using Google Cloud
- Generative AI for Developer Productivity
- Vertex AI Core
- Conversational AI
- Document AI
- Gemini in Google Cloud
- Cloud Databases
- Other
- ai-on-gke
- ai-infra-cluster-provisioning
- solutions-genai-llm-workshop
- terraform-genai-doc-summarization
- terraform-genai-knowledge-base
- genai-product-catalog
- solutionbuilder-terraform-genai-doc-summarization
- solutions-viai-edge-provisioning-configuration
- mis-ai-accelerator
- dataflow-opinion-analysis
- genai-beyond-basics
Contributions welcome! See the Contributing Guide.
Please use the issues page to provide suggestions, feedback or submit a bug report.
This repository itself is not an officially supported Google product. The code in this repository is for demonstrative purposes only.
For Tasks:
Click tags to check more tools for each tasksFor Jobs:
Alternative AI tools for generative-ai
Similar Open Source Tools
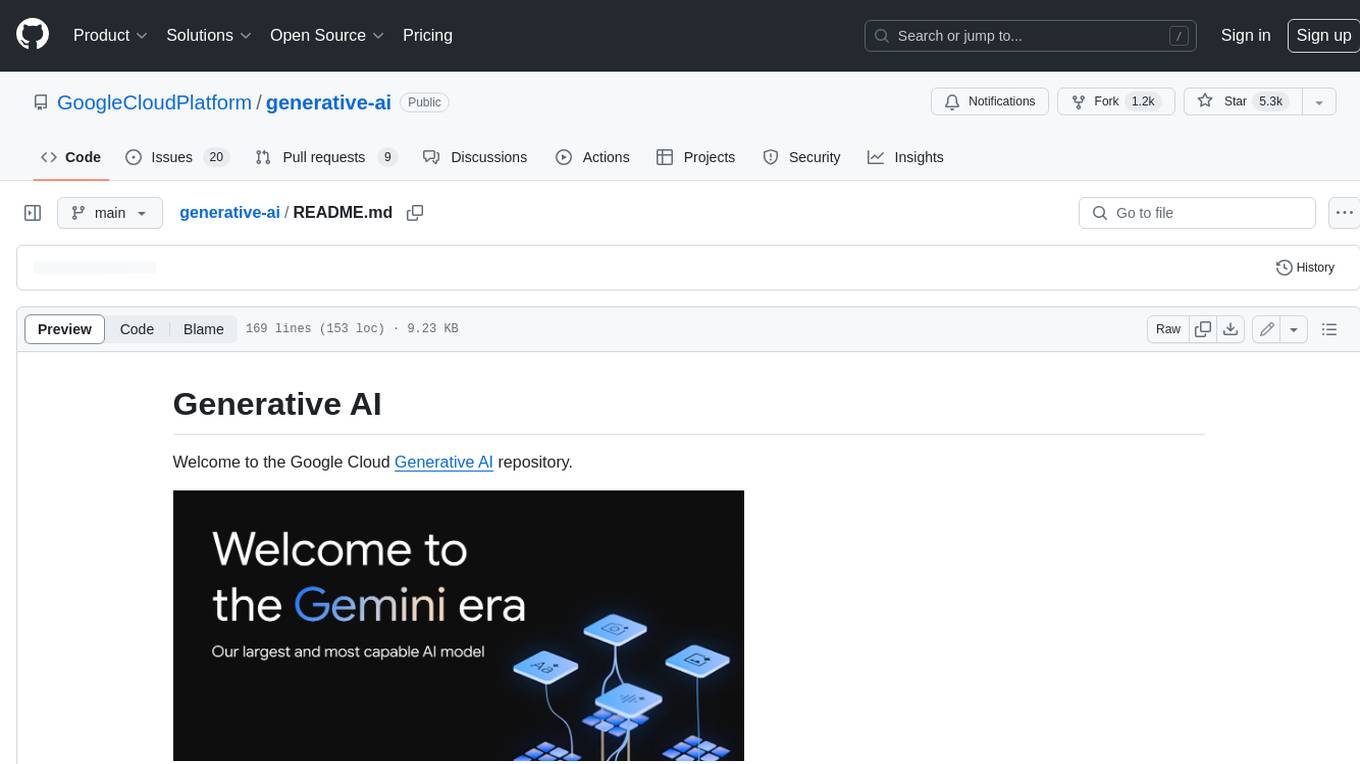
generative-ai
This repository contains notebooks, code samples, sample apps, and other resources that demonstrate how to use, develop and manage generative AI workflows using Generative AI on Google Cloud, powered by Vertex AI. For more Vertex AI samples, please visit the Vertex AI samples Github repository.
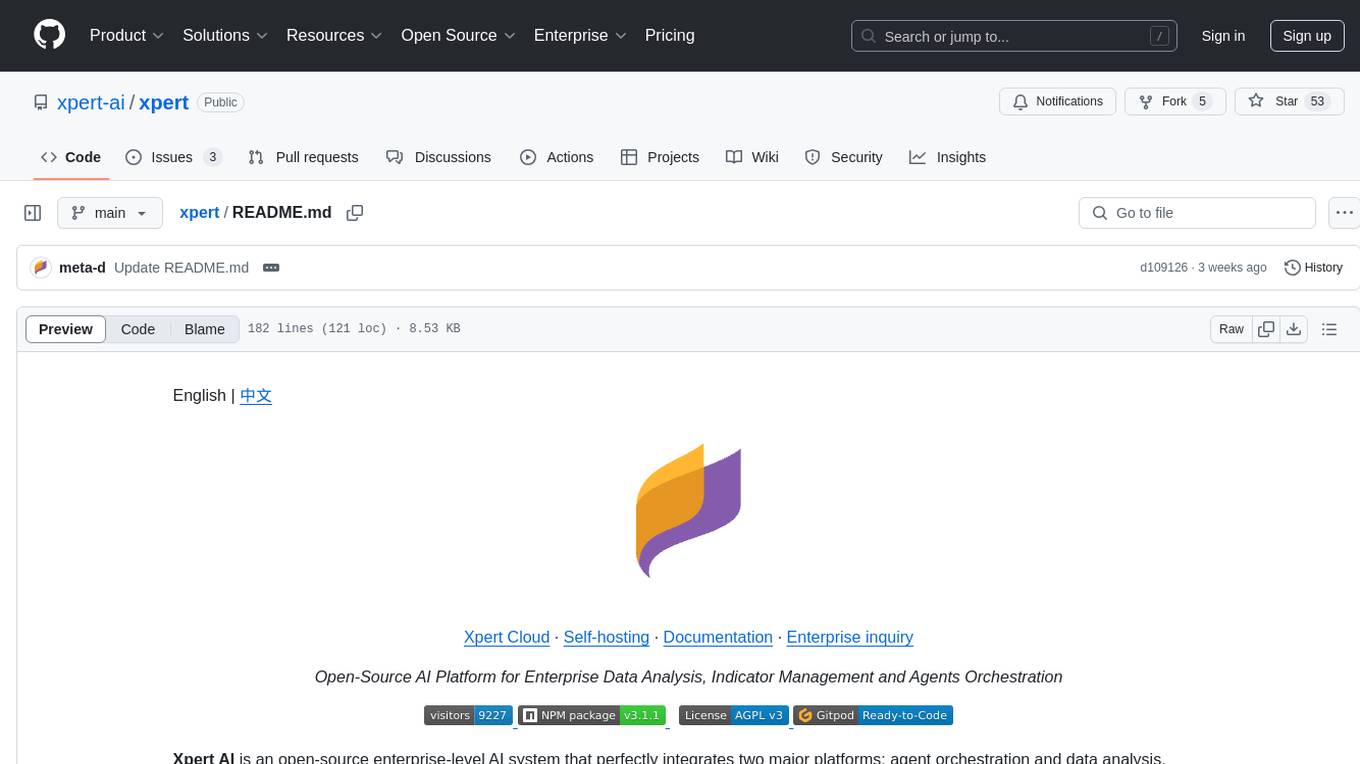
xpert
Xpert is a powerful tool for data analysis and visualization. It provides a user-friendly interface to explore and manipulate datasets, perform statistical analysis, and create insightful visualizations. With Xpert, users can easily import data from various sources, clean and preprocess data, analyze trends and patterns, and generate interactive charts and graphs. Whether you are a data scientist, analyst, researcher, or student, Xpert simplifies the process of data analysis and visualization, making it accessible to users with varying levels of expertise.
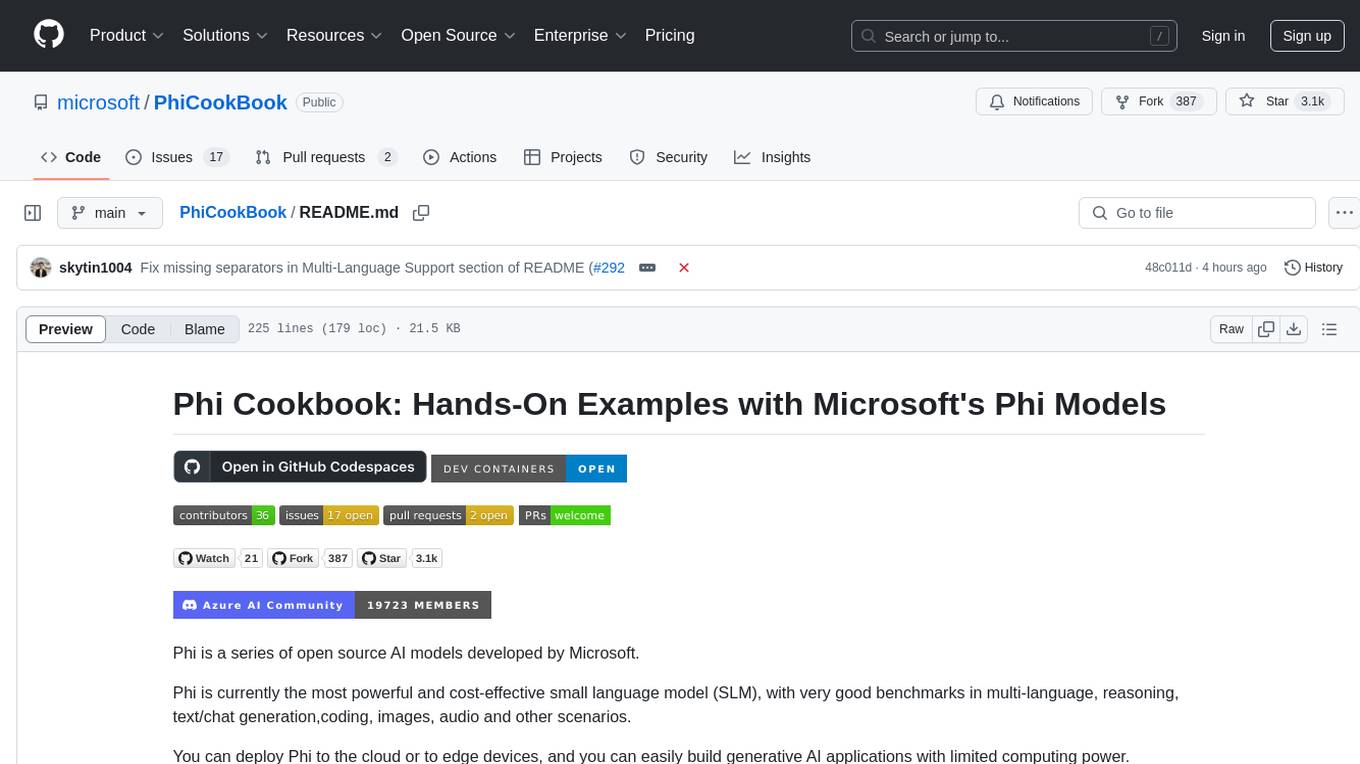
PhiCookBook
Phi Cookbook is a repository containing hands-on examples with Microsoft's Phi models, which are a series of open source AI models developed by Microsoft. Phi is currently the most powerful and cost-effective small language model with benchmarks in various scenarios like multi-language, reasoning, text/chat generation, coding, images, audio, and more. Users can deploy Phi to the cloud or edge devices to build generative AI applications with limited computing power.
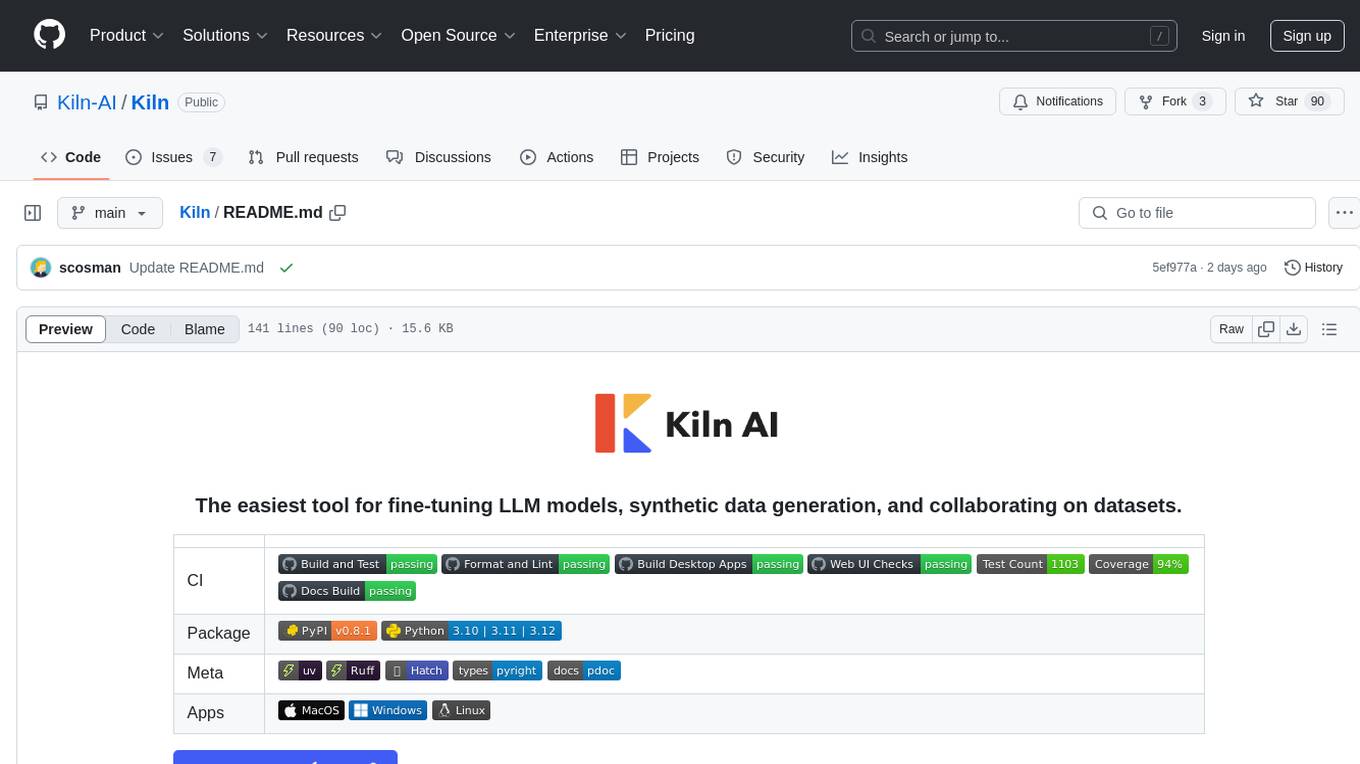
Kiln
Kiln is an intuitive tool for fine-tuning LLM models, generating synthetic data, and collaborating on datasets. It offers desktop apps for Windows, MacOS, and Linux, zero-code fine-tuning for various models, interactive data generation, and Git-based version control. Users can easily collaborate with QA, PM, and subject matter experts, generate auto-prompts, and work with a wide range of models and providers. The tool is open-source, privacy-first, and supports structured data tasks in JSON format. Kiln is free to use and helps build high-quality AI products with datasets, facilitates collaboration between technical and non-technical teams, allows comparison of models and techniques without code, ensures structured data integrity, and prioritizes user privacy.
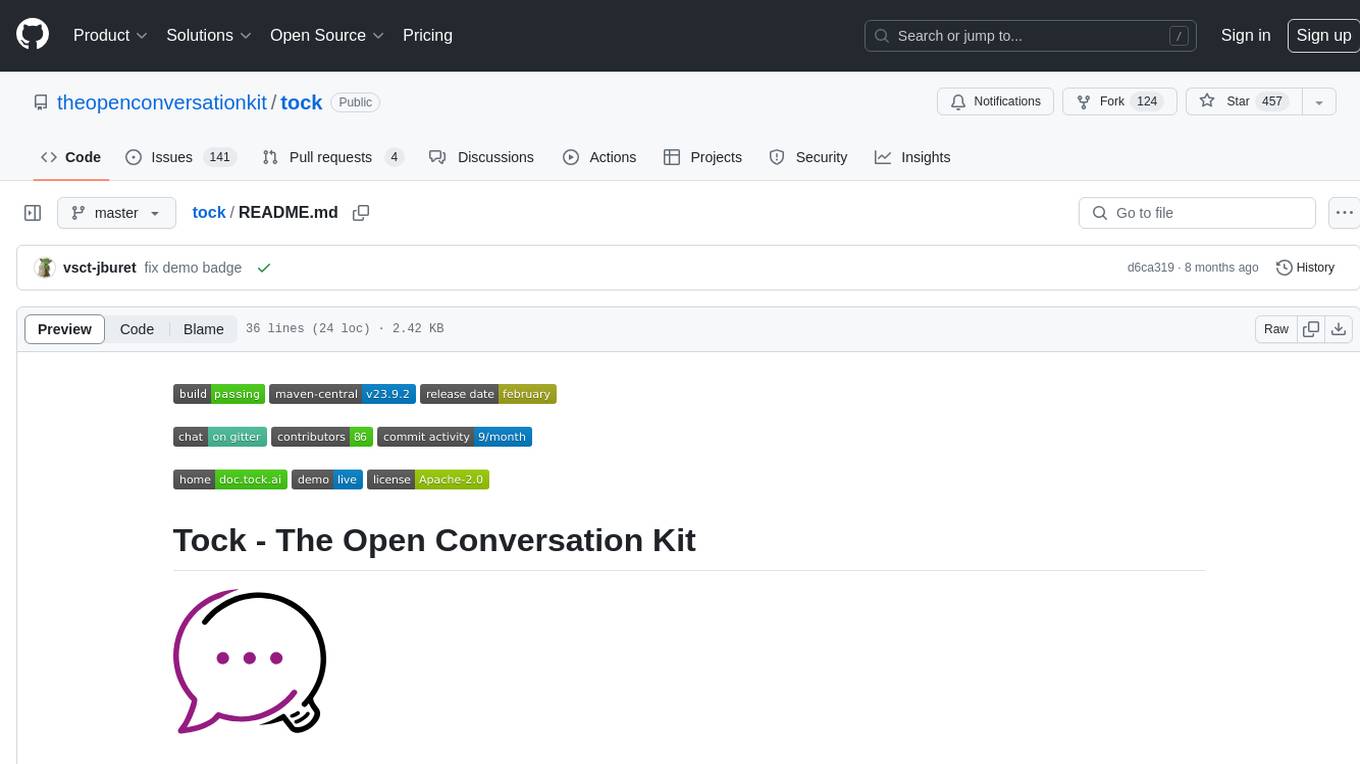
tock
Tock is an open conversational AI platform for building bots. It offers a natural language processing open source stack compatible with various tools, a user interface for building stories and analytics, a conversational DSL for different programming languages, built-in connectors for text/voice channels, toolkits for custom web/mobile integration, and the ability to deploy anywhere in the cloud or on-premise with Docker.
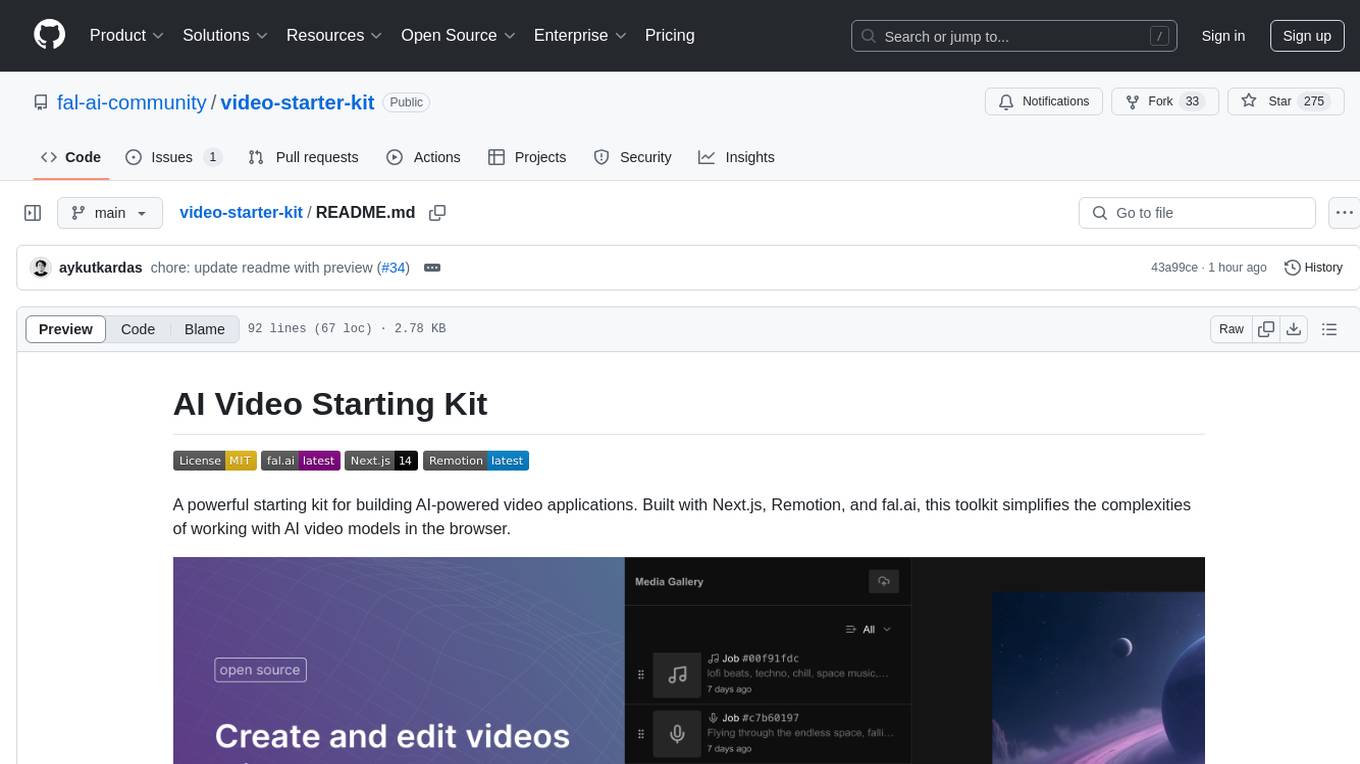
video-starter-kit
A powerful starting kit for building AI-powered video applications. This toolkit simplifies the complexities of working with AI video models in the browser. It offers browser-native video processing, AI model integration, advanced media capabilities, and developer utilities. The tech stack includes fal.ai for AI model infrastructure, Next.js for React framework, Remotion for video processing, IndexedDB for browser-based storage, Vercel for deployment platform, and UploadThing for file upload. The kit provides features like seamless video handling, multi-clip composition, audio track integration, voiceover support, metadata encoding, and ready-to-use UI components.
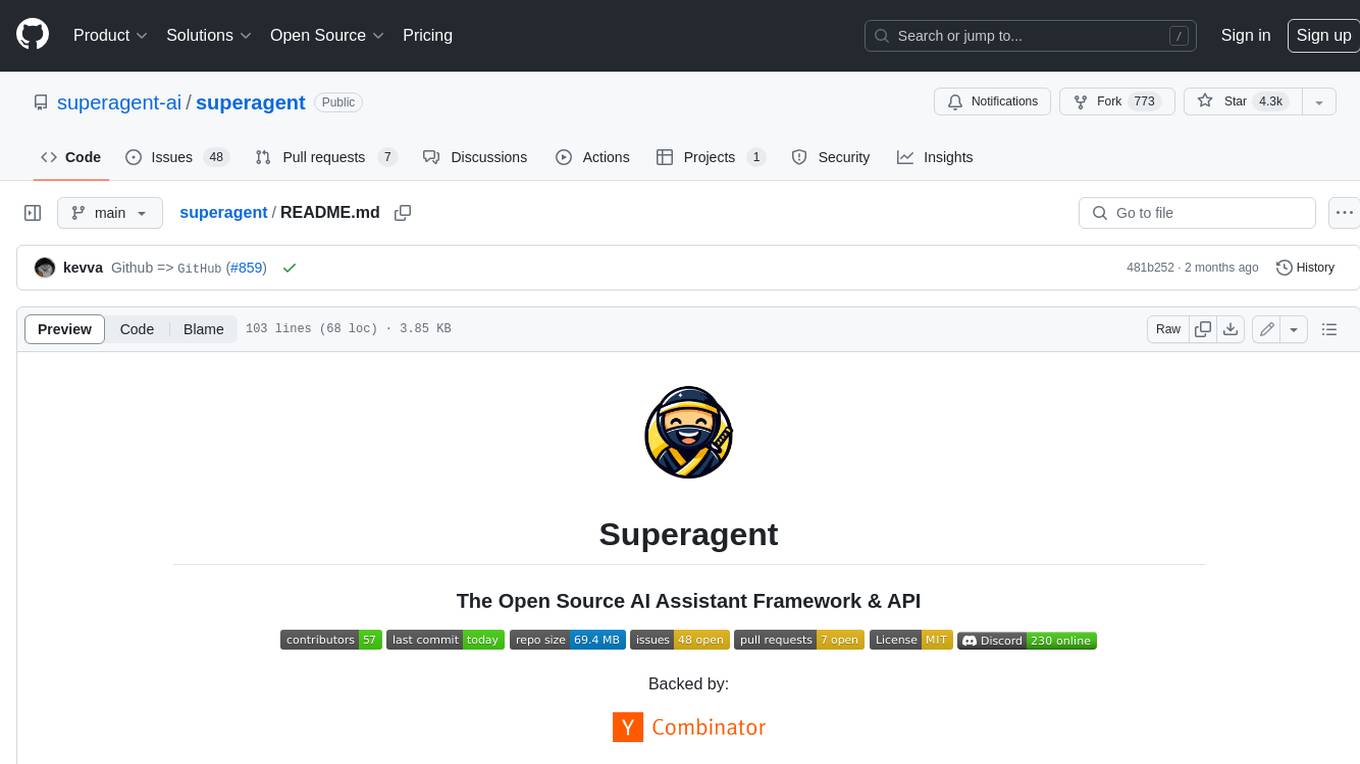
superagent
Superagent is an open-source AI assistant framework and API that allows developers to add powerful AI assistants to their applications. These assistants use large language models (LLMs), retrieval augmented generation (RAG), and generative AI to help users with a variety of tasks, including question answering, chatbot development, content generation, data aggregation, and workflow automation. Superagent is backed by Y Combinator and is part of YC W24.
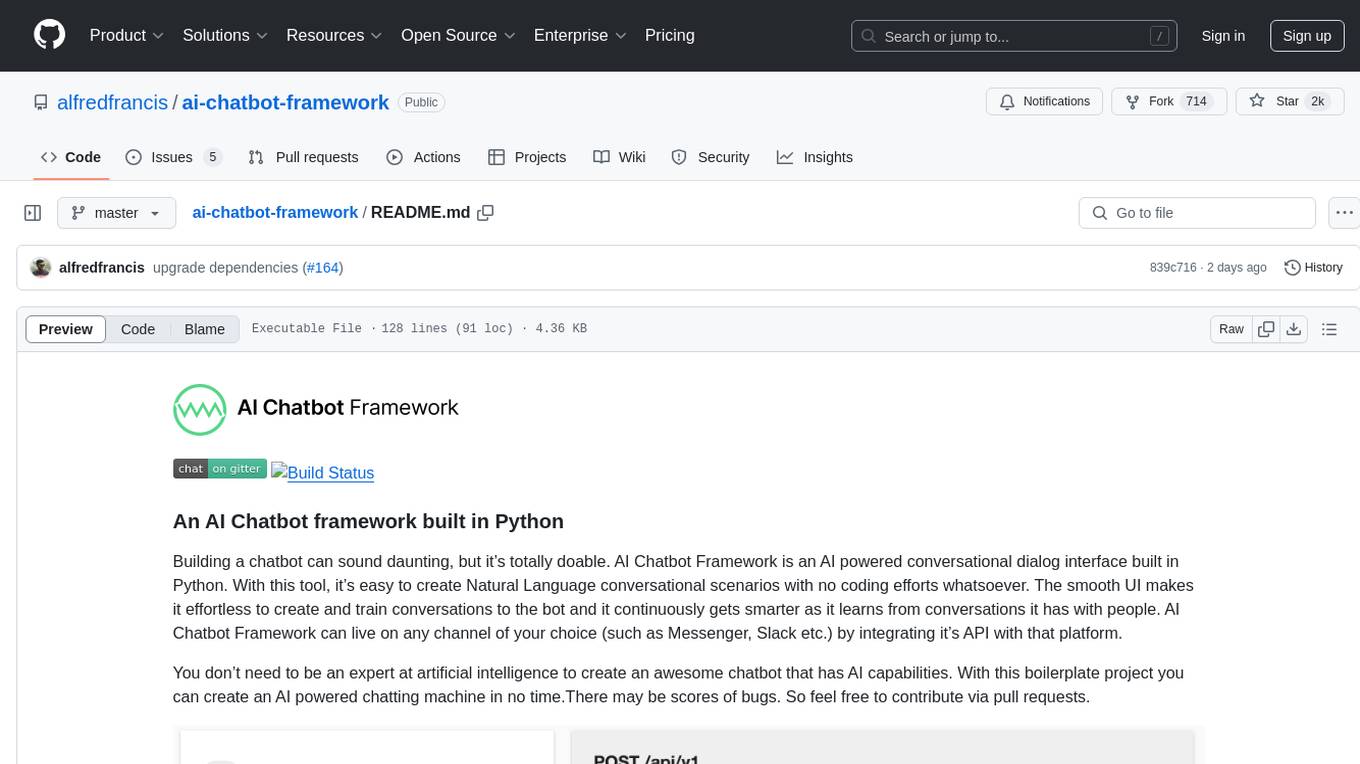
ai-chatbot-framework
An AI Chatbot framework built in Python. It allows users to easily create Natural Language conversational scenarios with no coding efforts. The tool continuously learns from conversations to improve its capabilities. It can be integrated with various channels like Messenger and Slack. Users can create AI-powered chatbots without expertise in artificial intelligence.
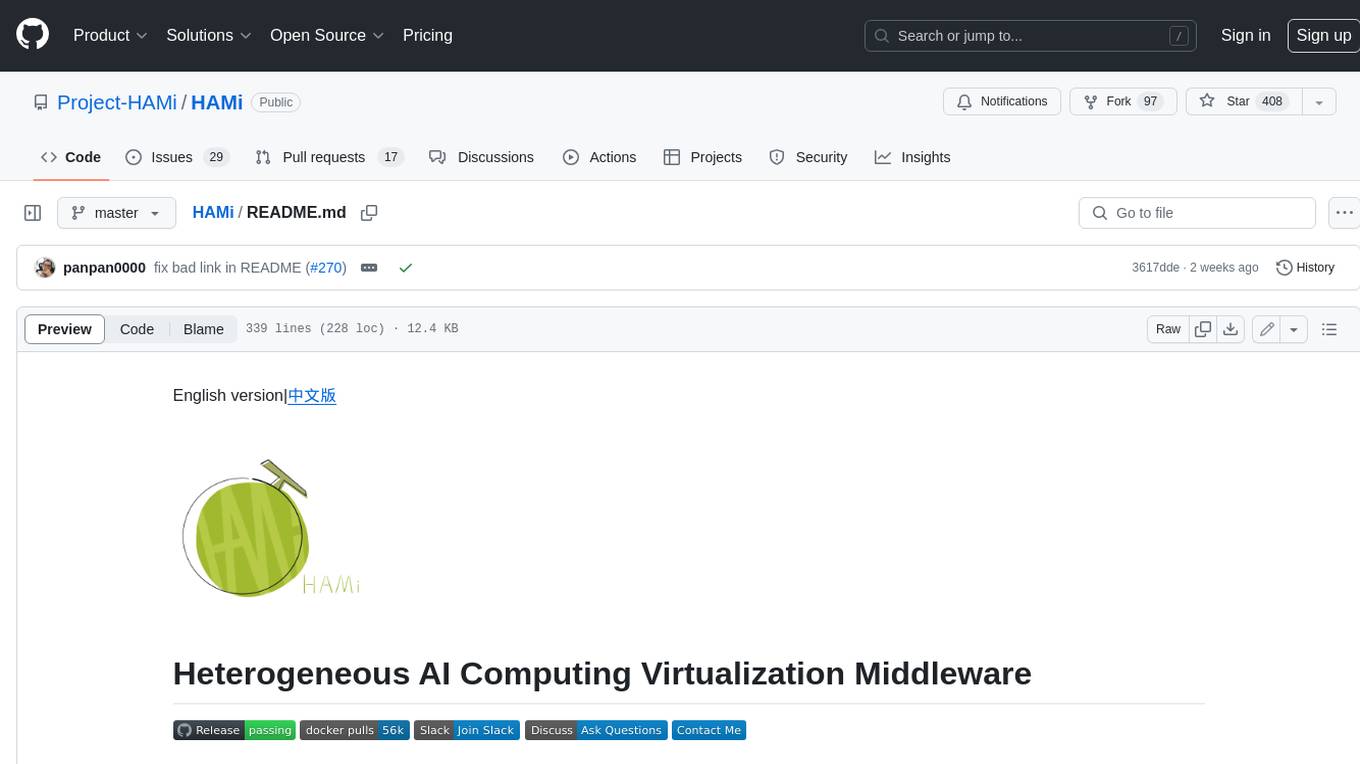
HAMi
HAMi is a Heterogeneous AI Computing Virtualization Middleware designed to manage Heterogeneous AI Computing Devices in a Kubernetes cluster. It allows for device sharing, device memory control, device type specification, and device UUID specification. The tool is easy to use and does not require modifying task YAML files. It includes features like hard limits on device memory, partial device allocation, streaming multiprocessor limits, and core usage specification. HAMi consists of components like a mutating webhook, scheduler extender, device plugins, and in-container virtualization techniques. It is suitable for scenarios requiring device sharing, specific device memory allocation, GPU balancing, low utilization optimization, and scenarios needing multiple small GPUs. The tool requires prerequisites like NVIDIA drivers, CUDA version, nvidia-docker, Kubernetes version, glibc version, and helm. Users can install, upgrade, and uninstall HAMi, submit tasks, and monitor cluster information. The tool's roadmap includes supporting additional AI computing devices, video codec processing, and Multi-Instance GPUs (MIG).
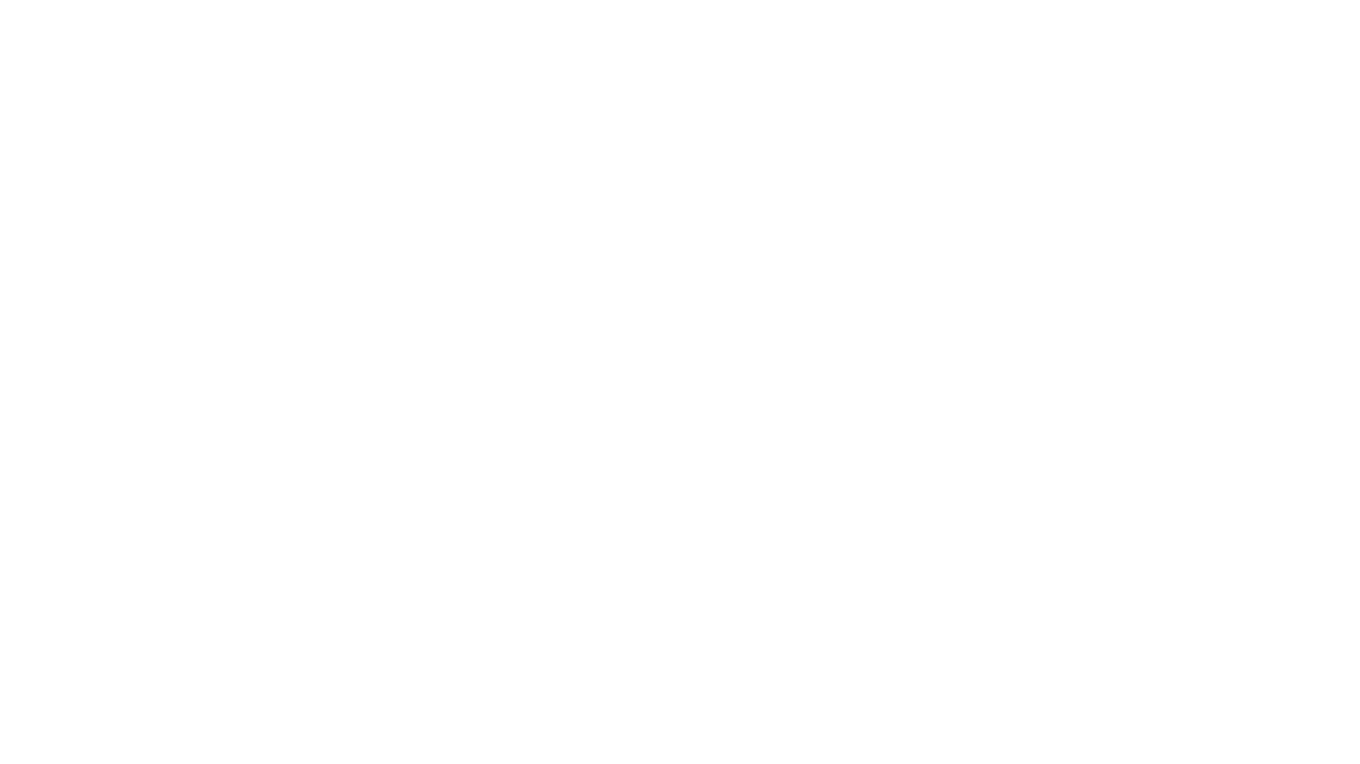
macai
Macai is a native macOS client for interacting with modern AI tools, such as ChatGPT and Ollama. It features organized chats with custom system messages, system-defined light/dark themes, backup and restore functionality, customizable context size, support for any model with a compatible API, formatted code blocks and tables, multiple chat tabs, CoreData data storage, streamed responses, and automatic chat name generation. Macai is in active development, with contributions welcome.
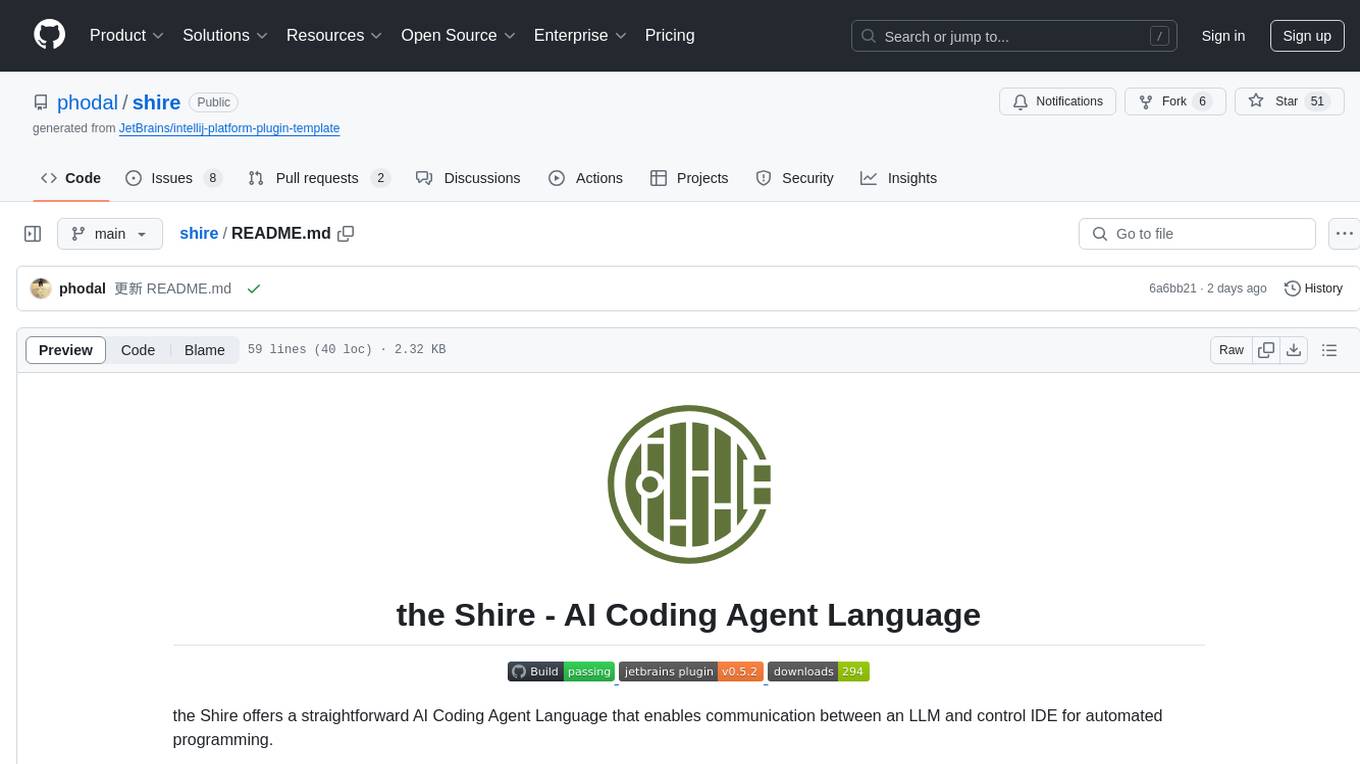
shire
The Shire is an AI Coding Agent Language that facilitates communication between an LLM and control IDE for automated programming. It offers a straightforward approach to creating AI agents tailored to individual IDEs, enabling users to build customized AI-driven development environments. The concept of Shire originated from AutoDev, a subproject of UnitMesh, with DevIns as its precursor. The tool provides documentation and resources for implementing AI in software engineering projects.
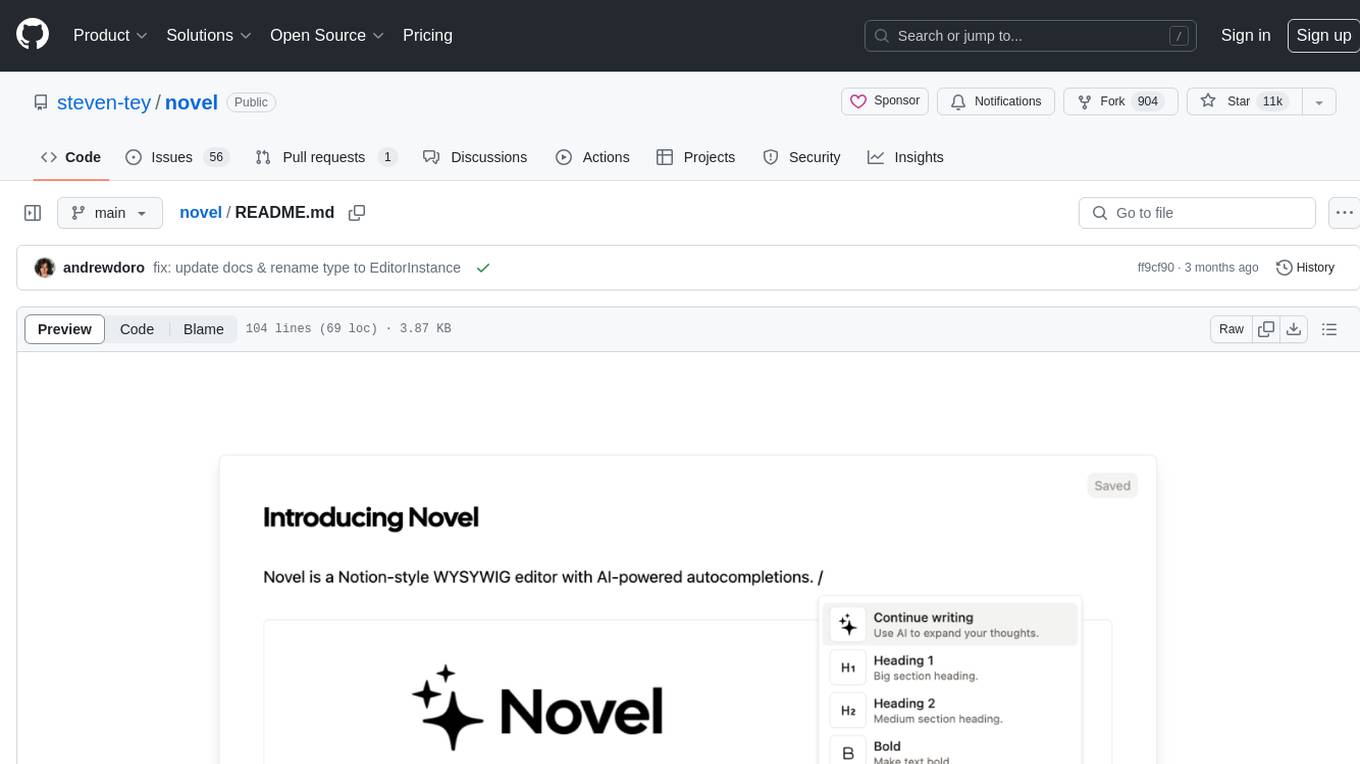
novel
Novel is an open-source Notion-style WYSIWYG editor with AI-powered autocompletions. It allows users to easily create and edit content with the help of AI suggestions. The tool is built on a modern tech stack and supports cross-framework development. Users can deploy their own version of Novel to Vercel with one click and contribute to the project by reporting bugs or making feature enhancements through pull requests.
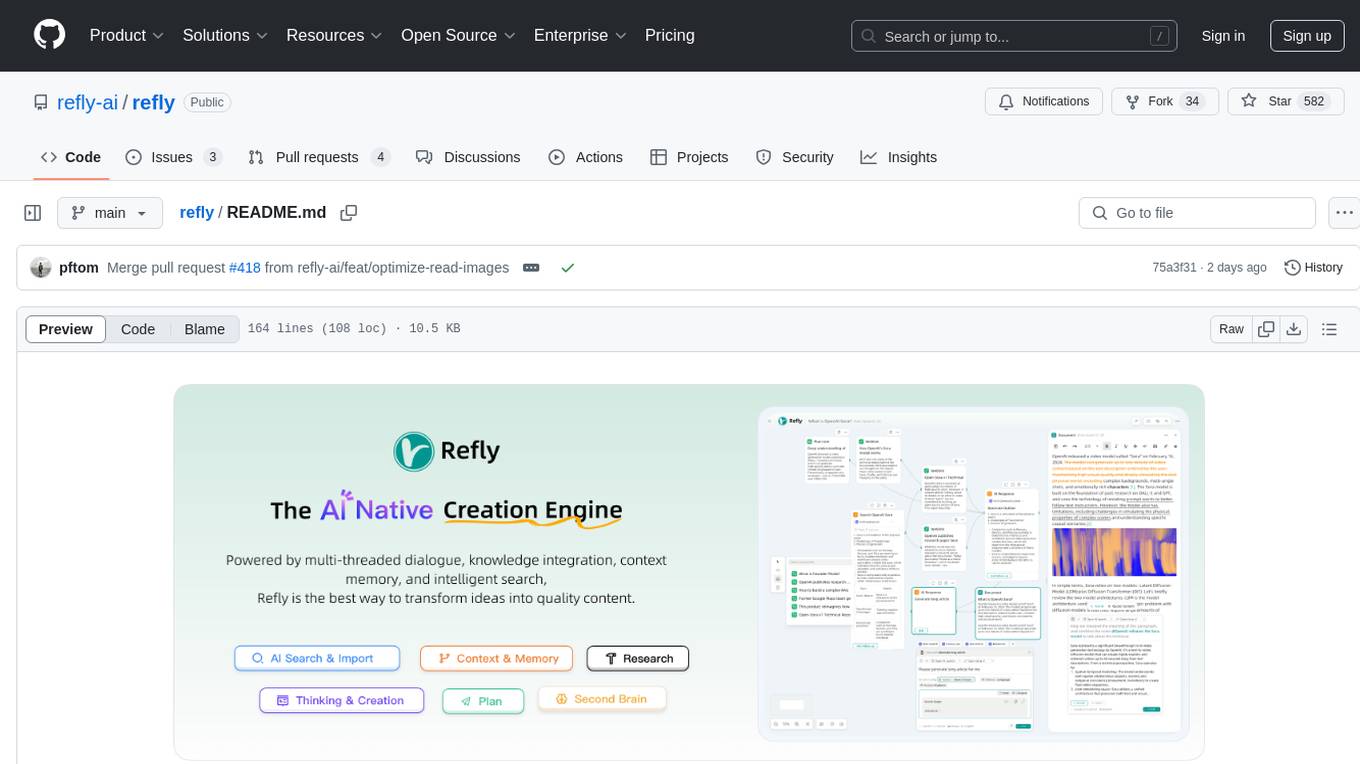
refly
Refly.AI is an open-source AI-native creation engine that empowers users to transform ideas into production-ready content. It features a free-form canvas interface with multi-threaded conversations, knowledge base integration, contextual memory, intelligent search, WYSIWYG AI editor, and more. Users can leverage AI-powered capabilities, context memory, knowledge base integration, quotes, and AI document editing to enhance their content creation process. Refly offers both cloud and self-hosting options, making it suitable for individuals, enterprises, and organizations. The tool is designed to facilitate human-AI collaboration and streamline content creation workflows.
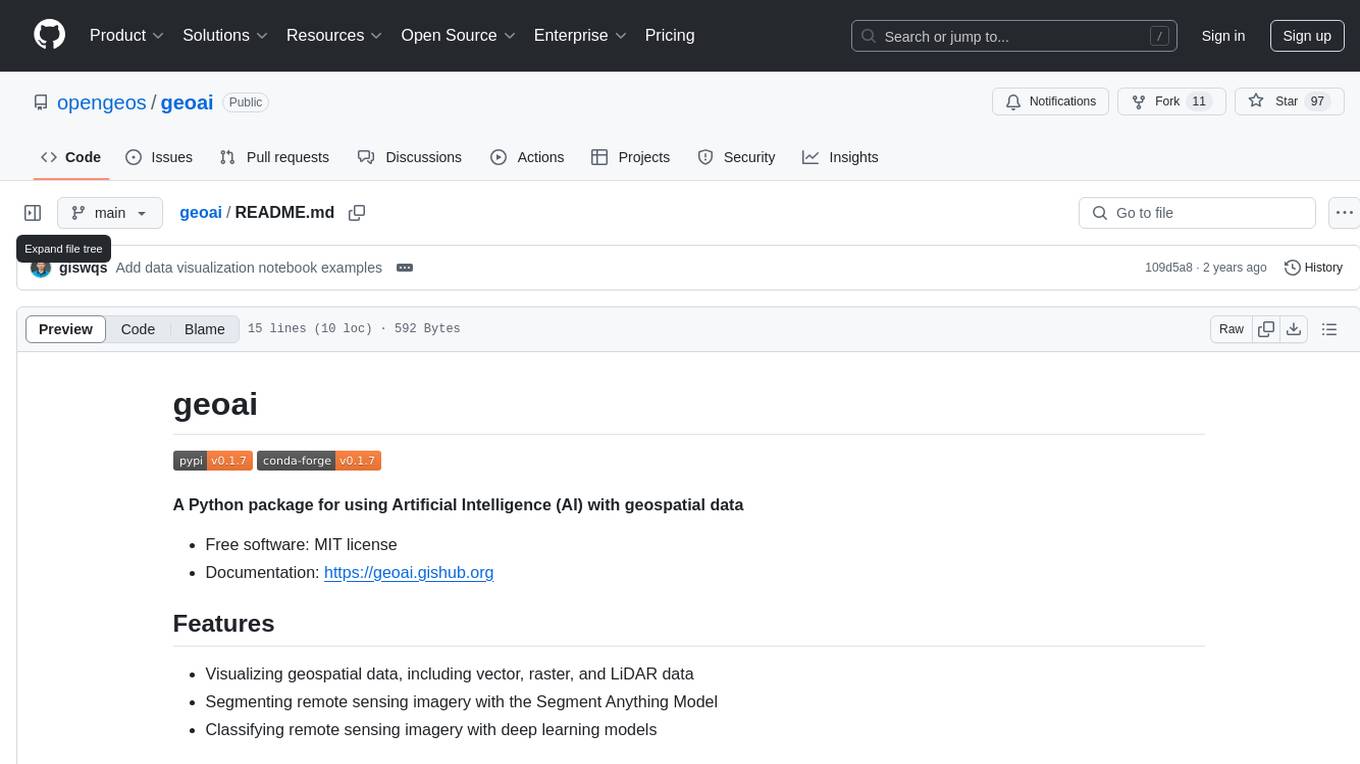
geoai
geoai is a Python package designed for utilizing Artificial Intelligence (AI) in the context of geospatial data. It allows users to visualize various types of geospatial data such as vector, raster, and LiDAR data. Additionally, the package offers functionalities for segmenting remote sensing imagery using the Segment Anything Model and classifying remote sensing imagery with deep learning models. With a focus on geospatial AI applications, geoai provides a versatile tool for processing and analyzing spatial data with the power of AI.
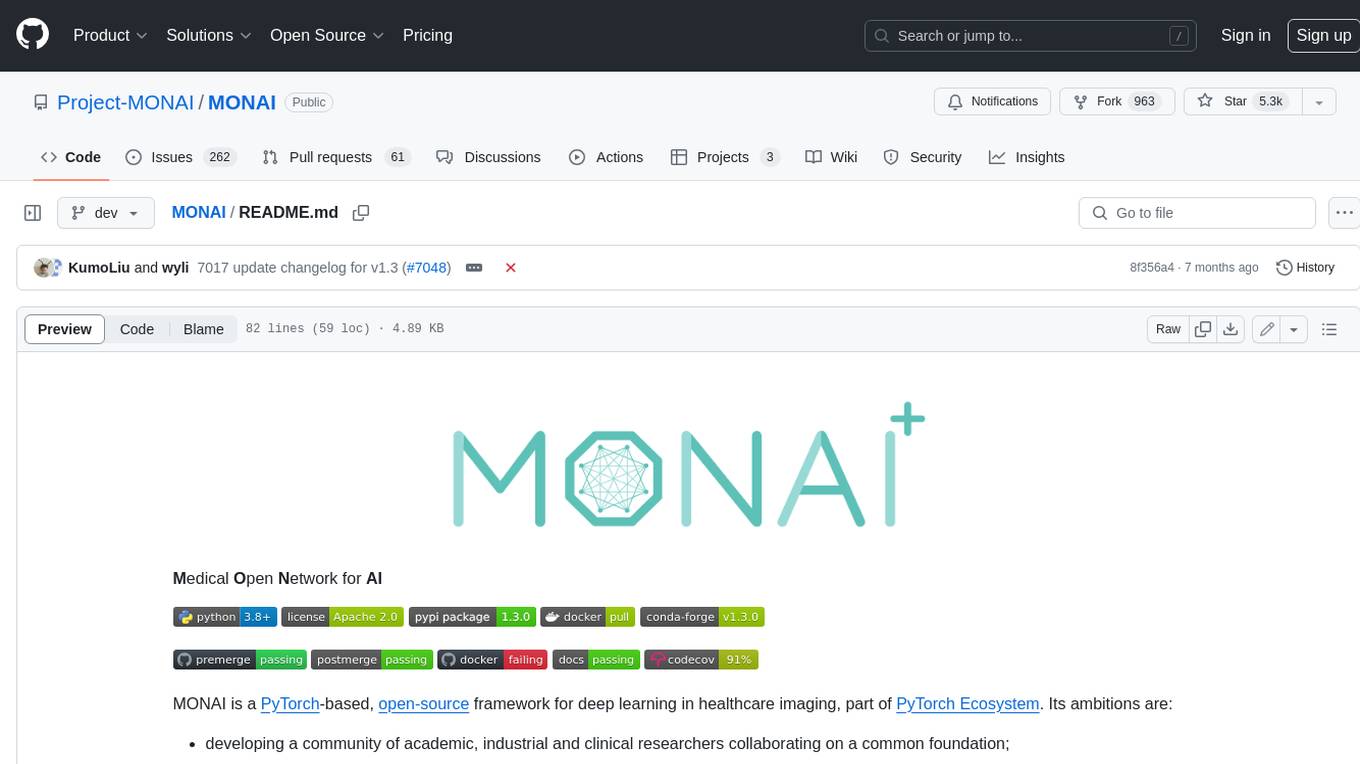
MONAI
MONAI is a PyTorch-based, open-source framework for deep learning in healthcare imaging. It provides a comprehensive set of tools for medical image analysis, including data preprocessing, model training, and evaluation. MONAI is designed to be flexible and easy to use, making it a valuable resource for researchers and developers in the field of medical imaging.
For similar tasks
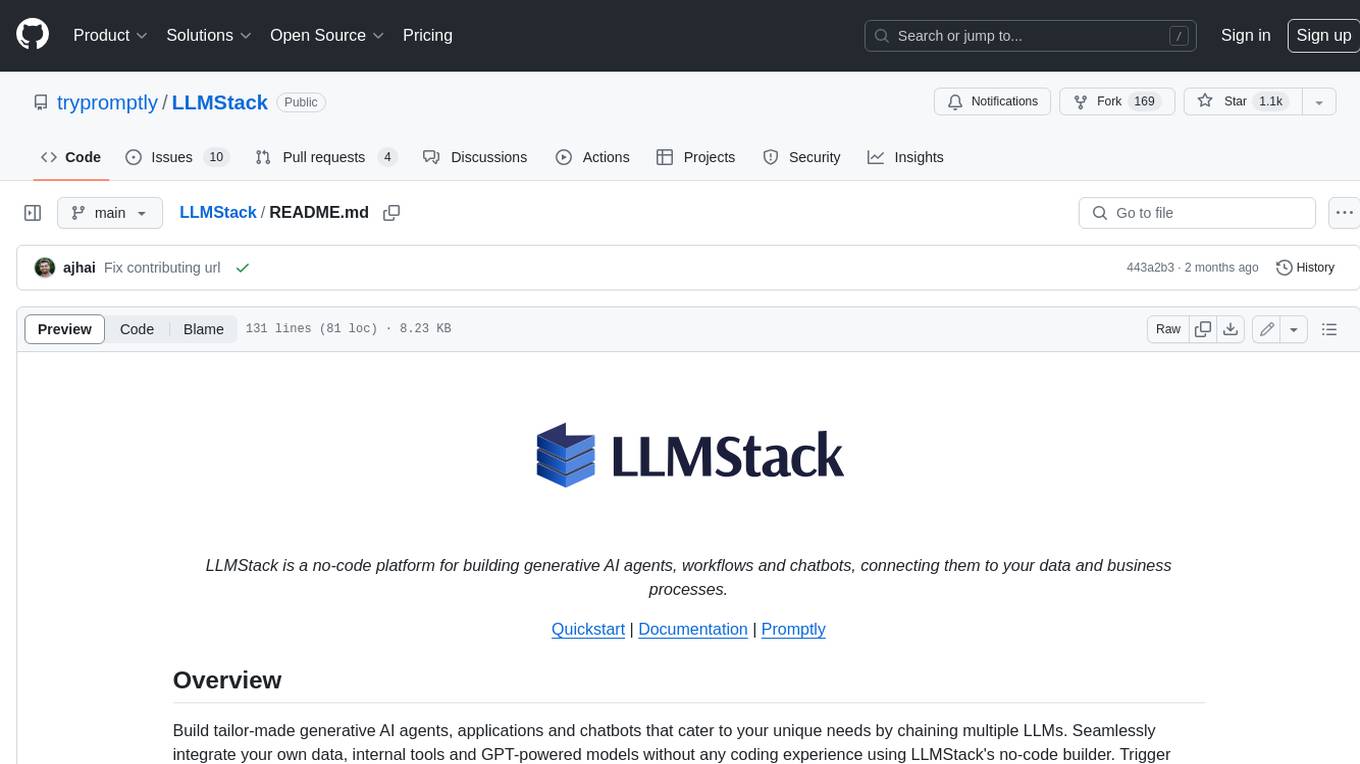
LLMStack
LLMStack is a no-code platform for building generative AI agents, workflows, and chatbots. It allows users to connect their own data, internal tools, and GPT-powered models without any coding experience. LLMStack can be deployed to the cloud or on-premise and can be accessed via HTTP API or triggered from Slack or Discord.
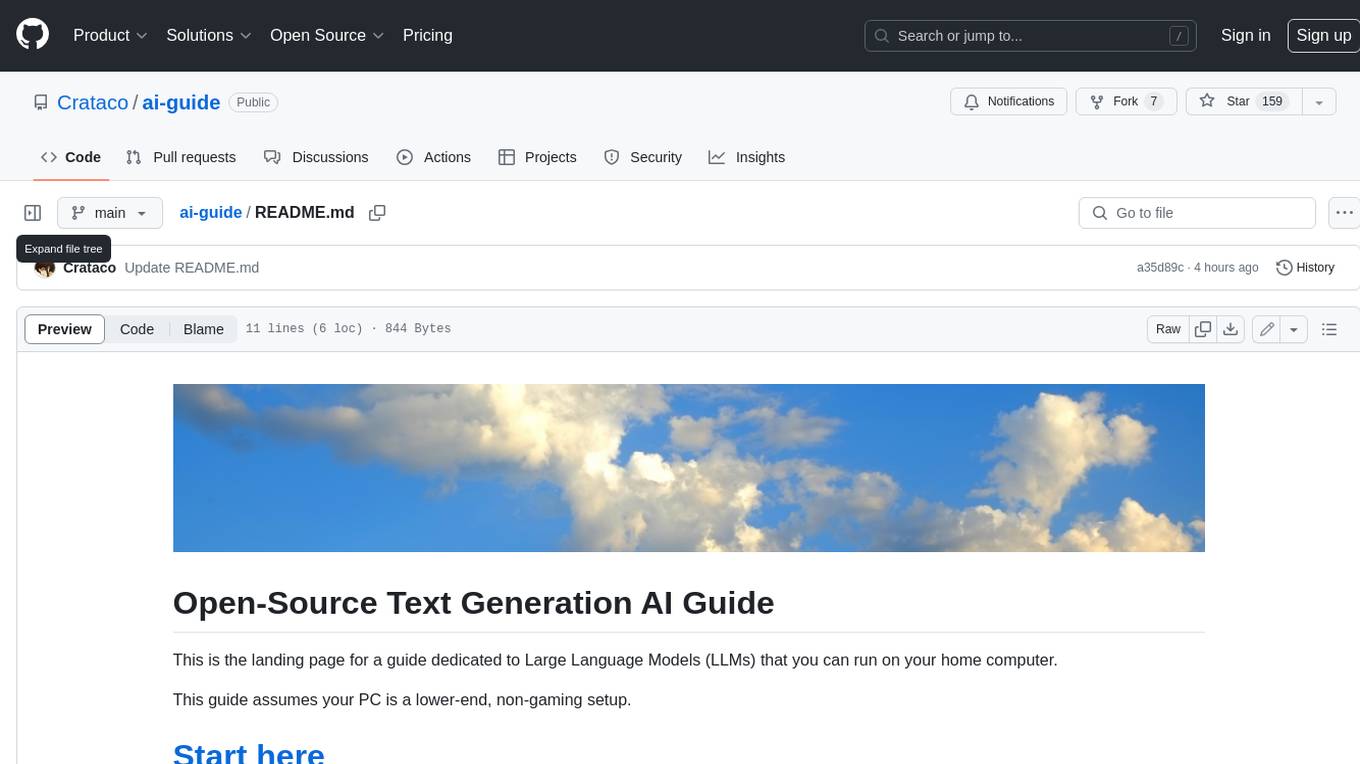
ai-guide
This guide is dedicated to Large Language Models (LLMs) that you can run on your home computer. It assumes your PC is a lower-end, non-gaming setup.
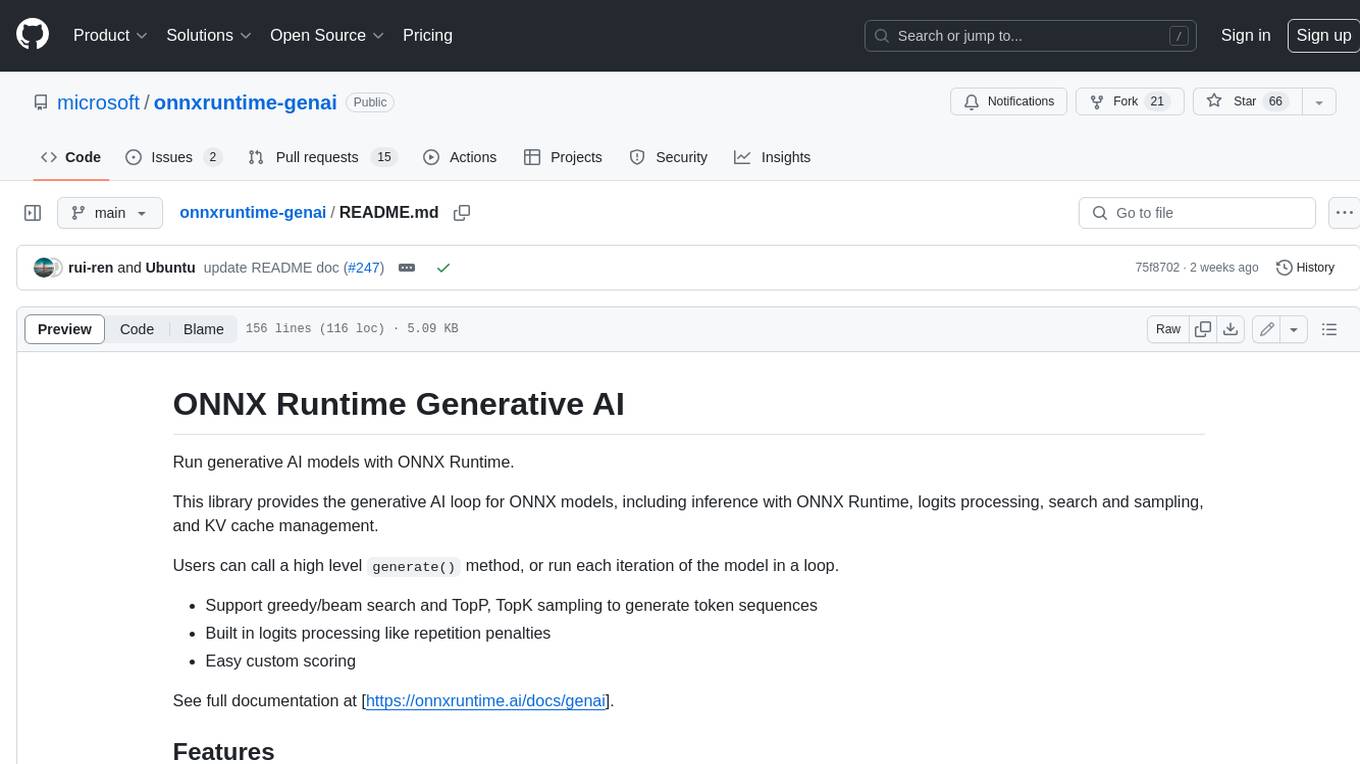
onnxruntime-genai
ONNX Runtime Generative AI is a library that provides the generative AI loop for ONNX models, including inference with ONNX Runtime, logits processing, search and sampling, and KV cache management. Users can call a high level `generate()` method, or run each iteration of the model in a loop. It supports greedy/beam search and TopP, TopK sampling to generate token sequences, has built in logits processing like repetition penalties, and allows for easy custom scoring.
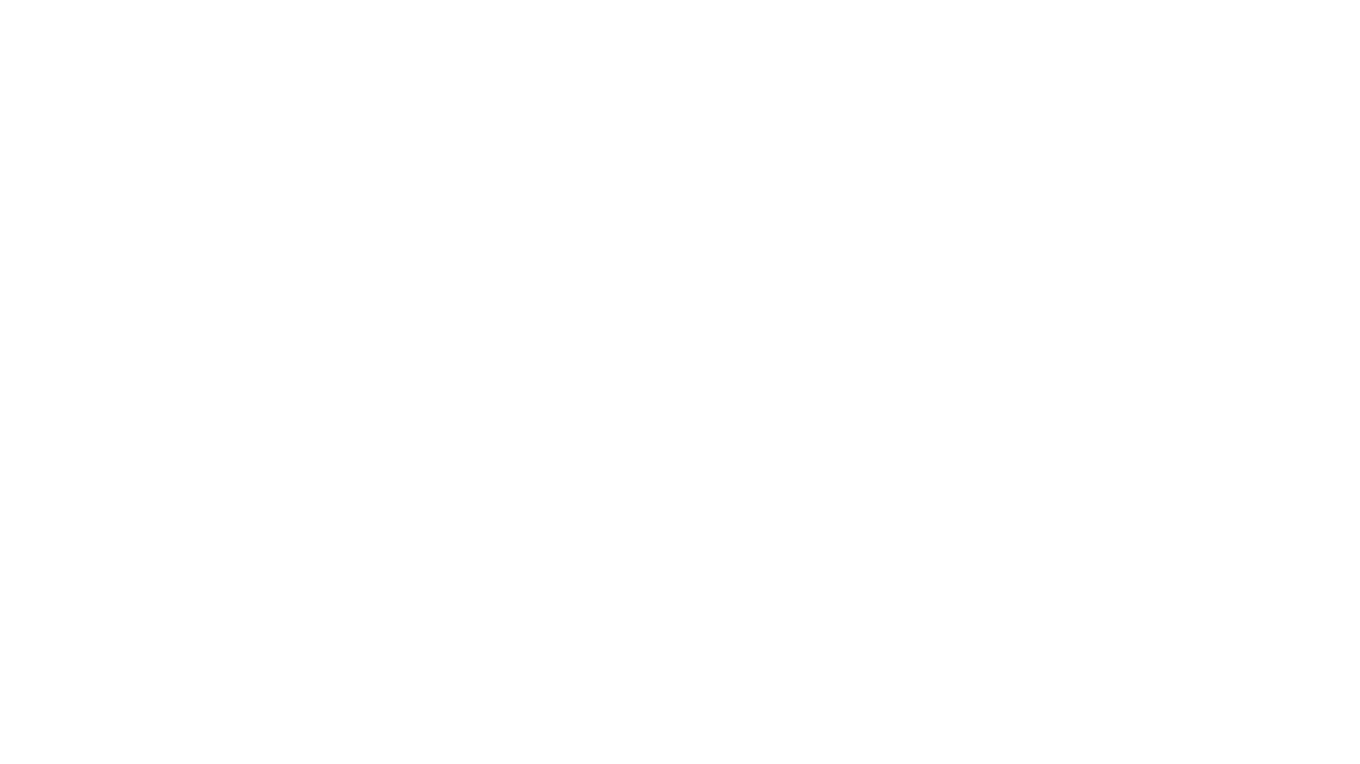
jupyter-ai
Jupyter AI connects generative AI with Jupyter notebooks. It provides a user-friendly and powerful way to explore generative AI models in notebooks and improve your productivity in JupyterLab and the Jupyter Notebook. Specifically, Jupyter AI offers: * An `%%ai` magic that turns the Jupyter notebook into a reproducible generative AI playground. This works anywhere the IPython kernel runs (JupyterLab, Jupyter Notebook, Google Colab, Kaggle, VSCode, etc.). * A native chat UI in JupyterLab that enables you to work with generative AI as a conversational assistant. * Support for a wide range of generative model providers, including AI21, Anthropic, AWS, Cohere, Gemini, Hugging Face, NVIDIA, and OpenAI. * Local model support through GPT4All, enabling use of generative AI models on consumer grade machines with ease and privacy.
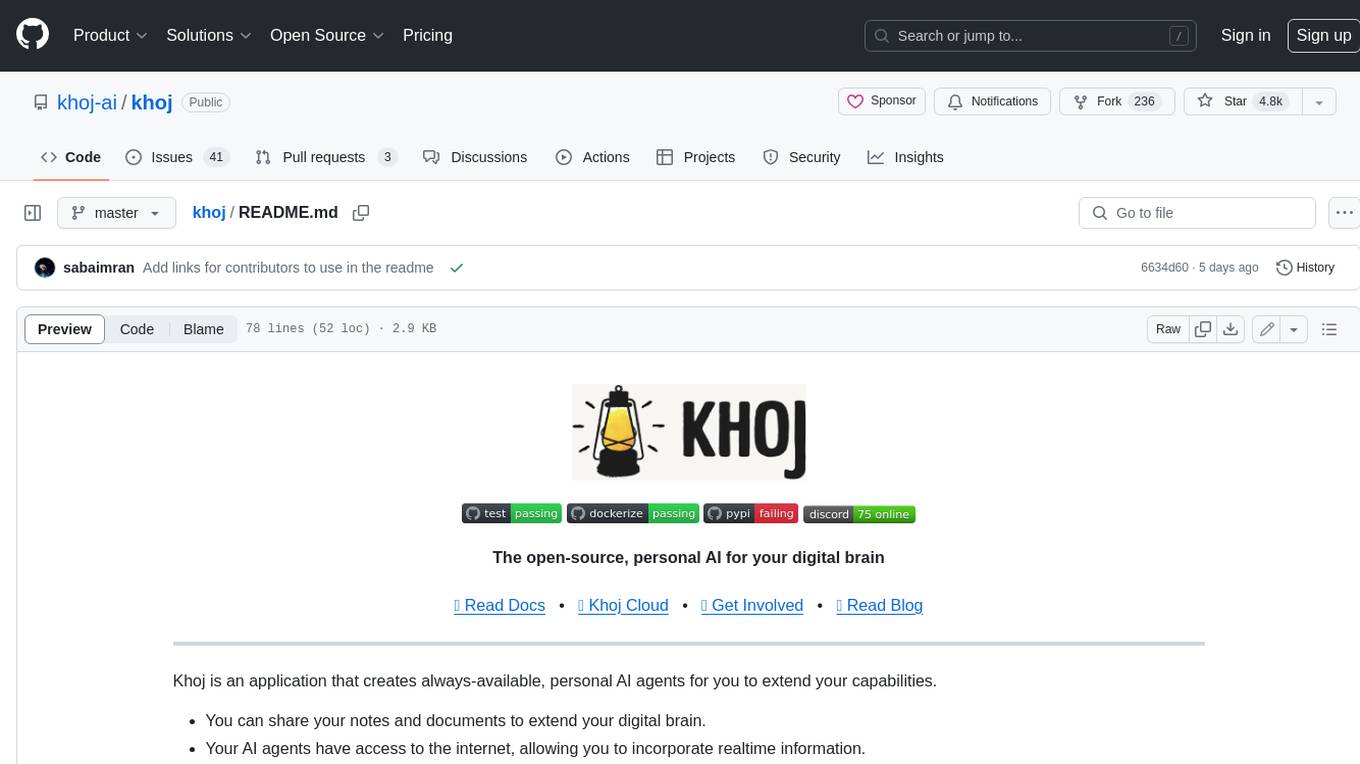
khoj
Khoj is an open-source, personal AI assistant that extends your capabilities by creating always-available AI agents. You can share your notes and documents to extend your digital brain, and your AI agents have access to the internet, allowing you to incorporate real-time information. Khoj is accessible on Desktop, Emacs, Obsidian, Web, and Whatsapp, and you can share PDF, markdown, org-mode, notion files, and GitHub repositories. You'll get fast, accurate semantic search on top of your docs, and your agents can create deeply personal images and understand your speech. Khoj is self-hostable and always will be.
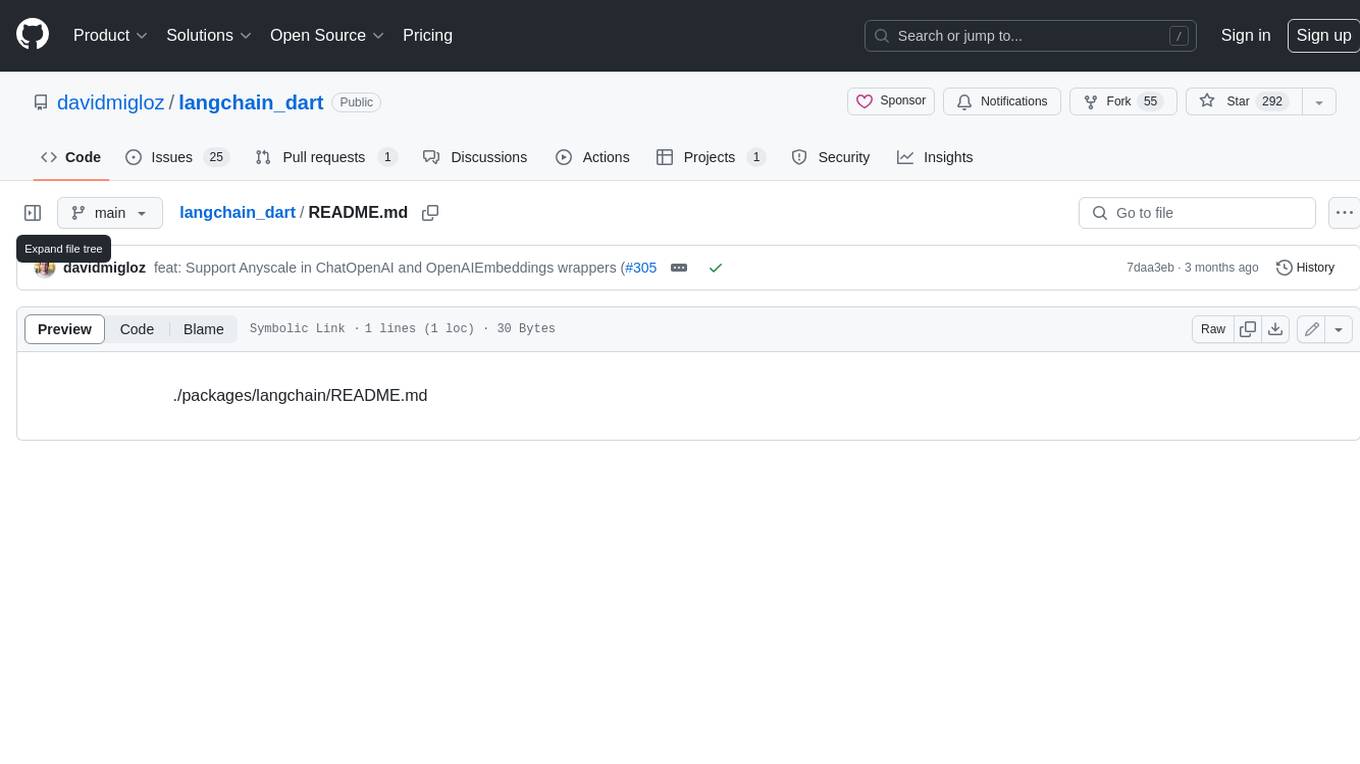
langchain_dart
LangChain.dart is a Dart port of the popular LangChain Python framework created by Harrison Chase. LangChain provides a set of ready-to-use components for working with language models and a standard interface for chaining them together to formulate more advanced use cases (e.g. chatbots, Q&A with RAG, agents, summarization, extraction, etc.). The components can be grouped into a few core modules: * **Model I/O:** LangChain offers a unified API for interacting with various LLM providers (e.g. OpenAI, Google, Mistral, Ollama, etc.), allowing developers to switch between them with ease. Additionally, it provides tools for managing model inputs (prompt templates and example selectors) and parsing the resulting model outputs (output parsers). * **Retrieval:** assists in loading user data (via document loaders), transforming it (with text splitters), extracting its meaning (using embedding models), storing (in vector stores) and retrieving it (through retrievers) so that it can be used to ground the model's responses (i.e. Retrieval-Augmented Generation or RAG). * **Agents:** "bots" that leverage LLMs to make informed decisions about which available tools (such as web search, calculators, database lookup, etc.) to use to accomplish the designated task. The different components can be composed together using the LangChain Expression Language (LCEL).
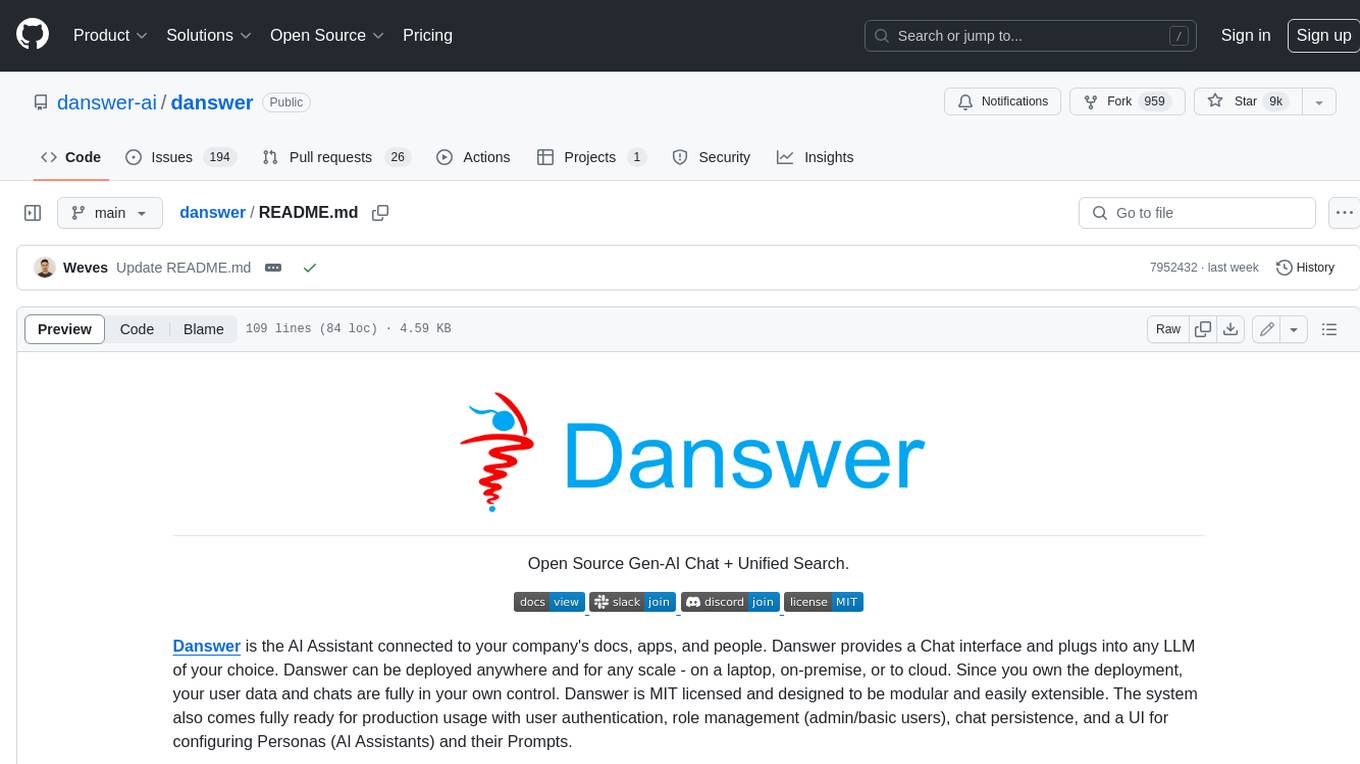
danswer
Danswer is an open-source Gen-AI Chat and Unified Search tool that connects to your company's docs, apps, and people. It provides a Chat interface and plugs into any LLM of your choice. Danswer can be deployed anywhere and for any scale - on a laptop, on-premise, or to cloud. Since you own the deployment, your user data and chats are fully in your own control. Danswer is MIT licensed and designed to be modular and easily extensible. The system also comes fully ready for production usage with user authentication, role management (admin/basic users), chat persistence, and a UI for configuring Personas (AI Assistants) and their Prompts. Danswer also serves as a Unified Search across all common workplace tools such as Slack, Google Drive, Confluence, etc. By combining LLMs and team specific knowledge, Danswer becomes a subject matter expert for the team. Imagine ChatGPT if it had access to your team's unique knowledge! It enables questions such as "A customer wants feature X, is this already supported?" or "Where's the pull request for feature Y?"
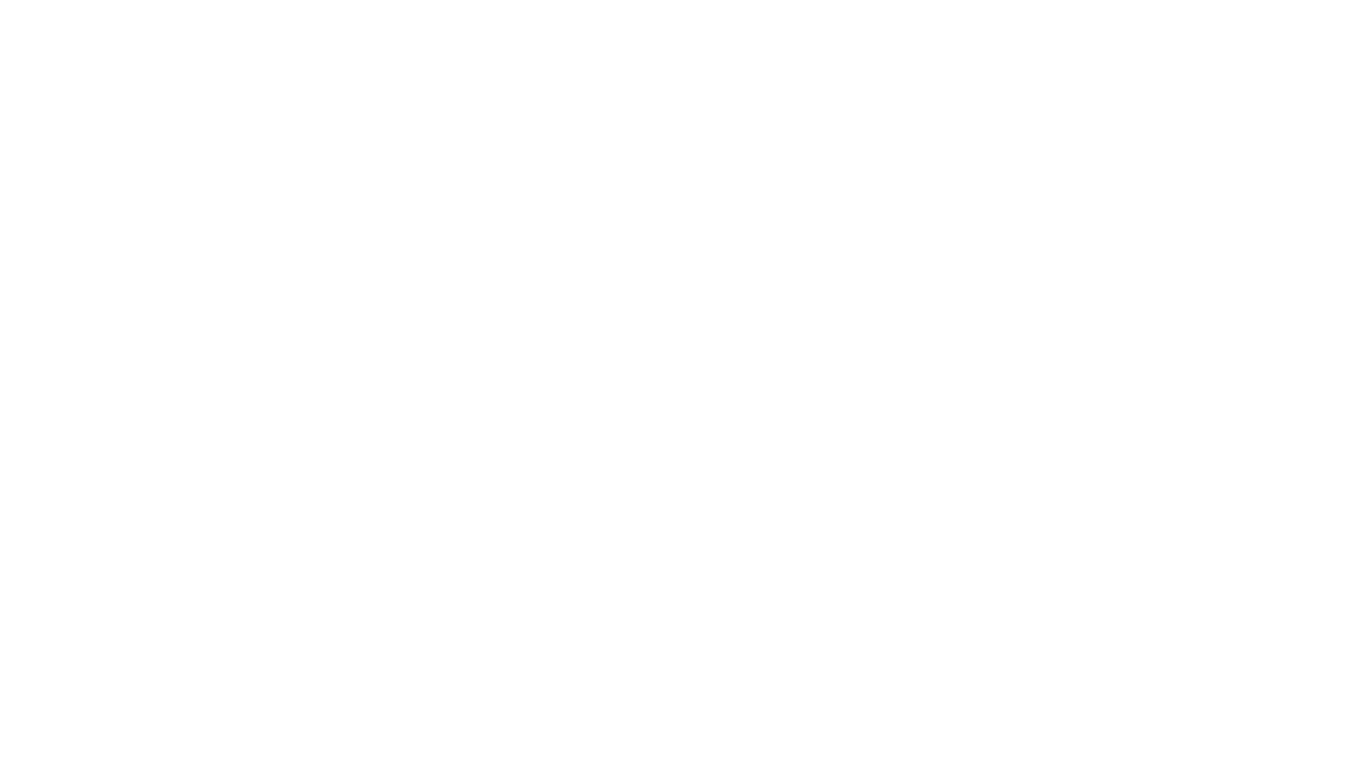
infinity
Infinity is an AI-native database designed for LLM applications, providing incredibly fast full-text and vector search capabilities. It supports a wide range of data types, including vectors, full-text, and structured data, and offers a fused search feature that combines multiple embeddings and full text. Infinity is easy to use, with an intuitive Python API and a single-binary architecture that simplifies deployment. It achieves high performance, with 0.1 milliseconds query latency on million-scale vector datasets and up to 15K QPS.
For similar jobs
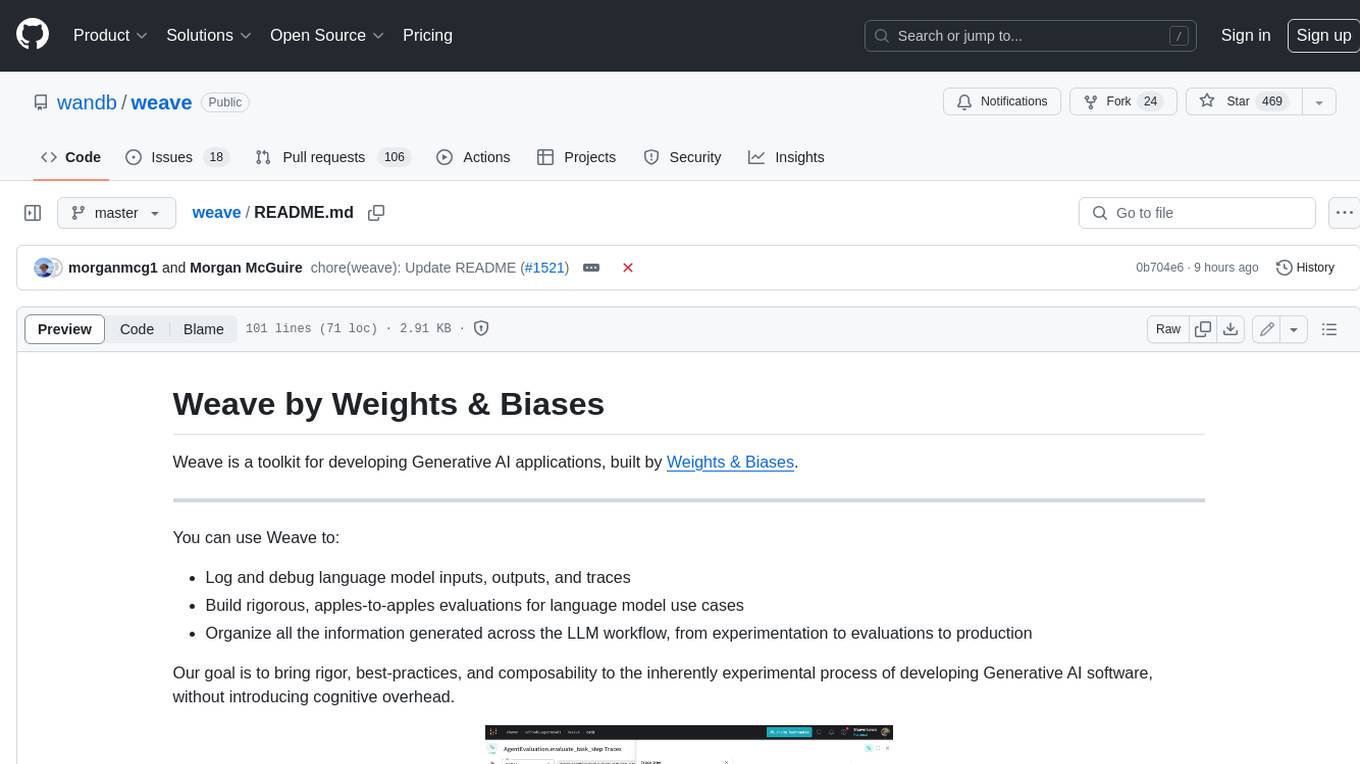
weave
Weave is a toolkit for developing Generative AI applications, built by Weights & Biases. With Weave, you can log and debug language model inputs, outputs, and traces; build rigorous, apples-to-apples evaluations for language model use cases; and organize all the information generated across the LLM workflow, from experimentation to evaluations to production. Weave aims to bring rigor, best-practices, and composability to the inherently experimental process of developing Generative AI software, without introducing cognitive overhead.
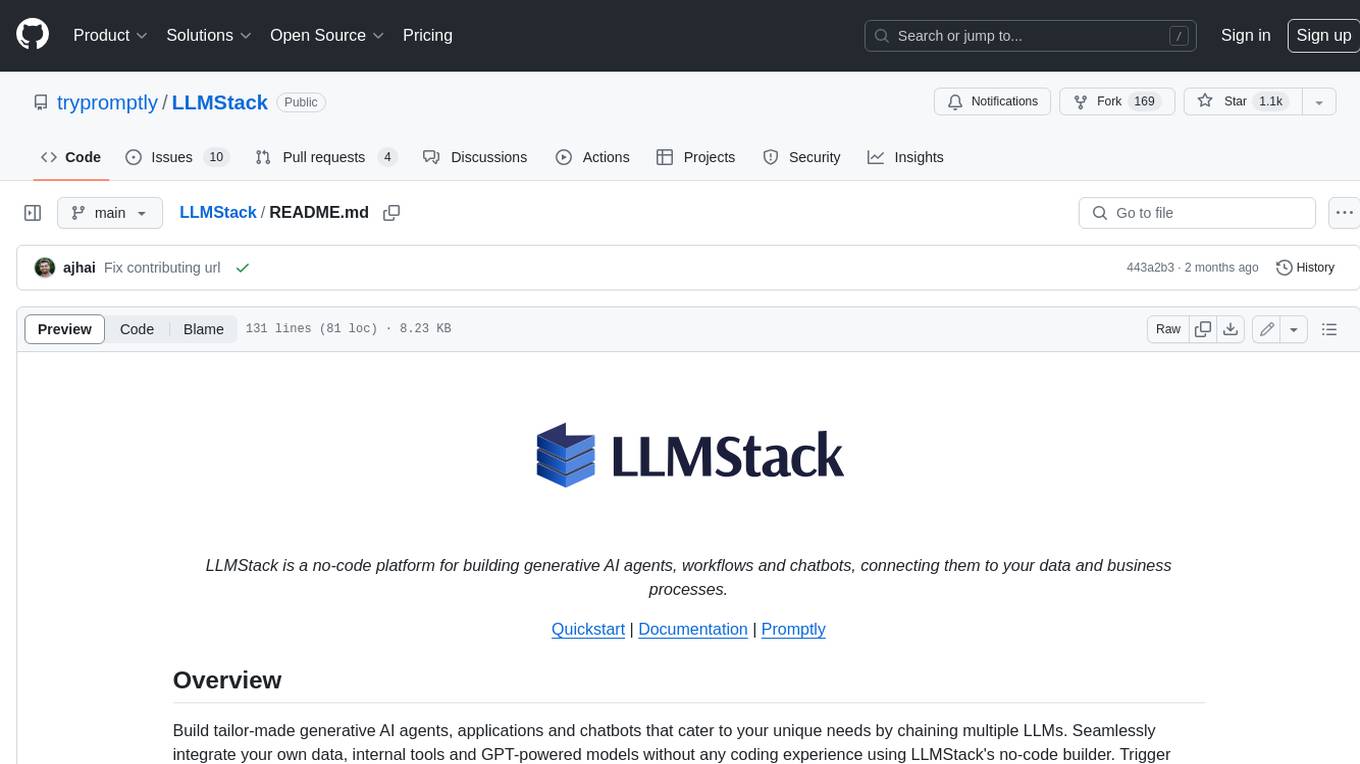
LLMStack
LLMStack is a no-code platform for building generative AI agents, workflows, and chatbots. It allows users to connect their own data, internal tools, and GPT-powered models without any coding experience. LLMStack can be deployed to the cloud or on-premise and can be accessed via HTTP API or triggered from Slack or Discord.
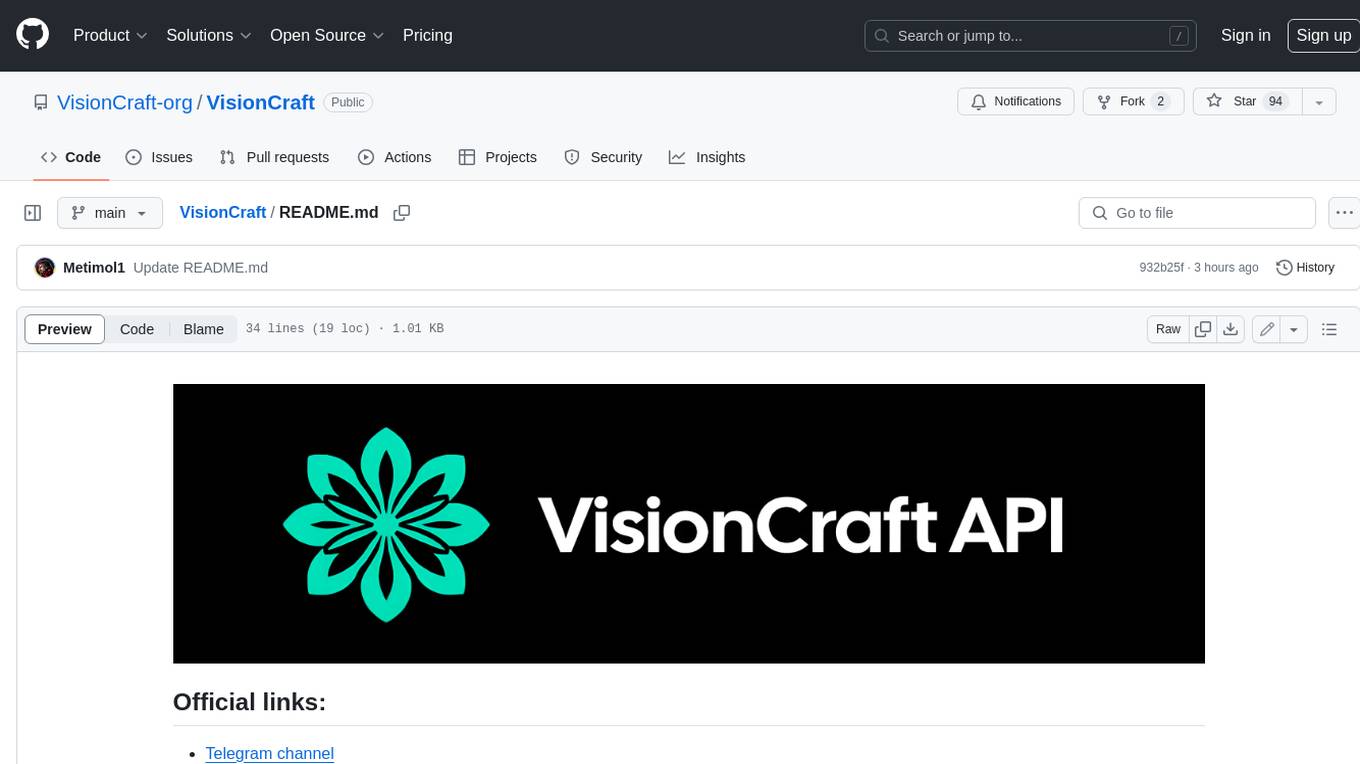
VisionCraft
The VisionCraft API is a free API for using over 100 different AI models. From images to sound.
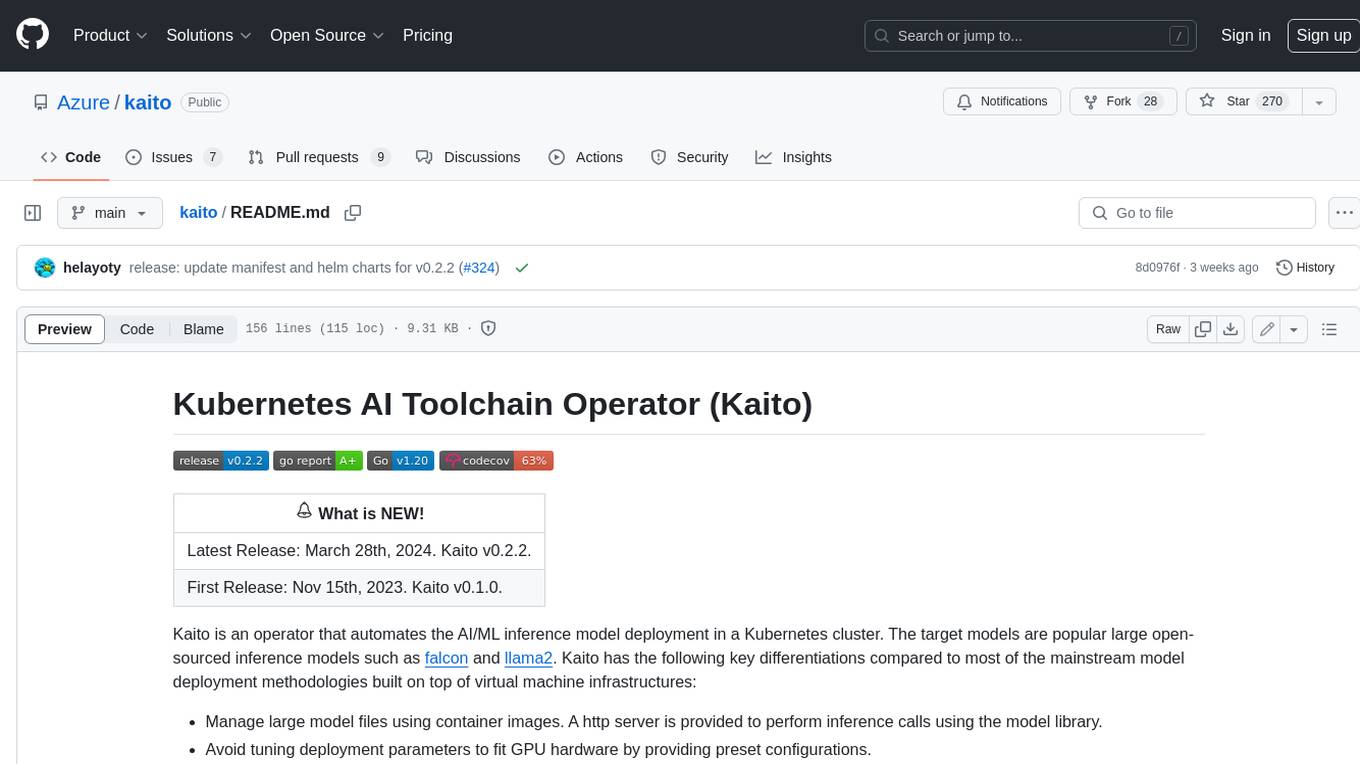
kaito
Kaito is an operator that automates the AI/ML inference model deployment in a Kubernetes cluster. It manages large model files using container images, avoids tuning deployment parameters to fit GPU hardware by providing preset configurations, auto-provisions GPU nodes based on model requirements, and hosts large model images in the public Microsoft Container Registry (MCR) if the license allows. Using Kaito, the workflow of onboarding large AI inference models in Kubernetes is largely simplified.
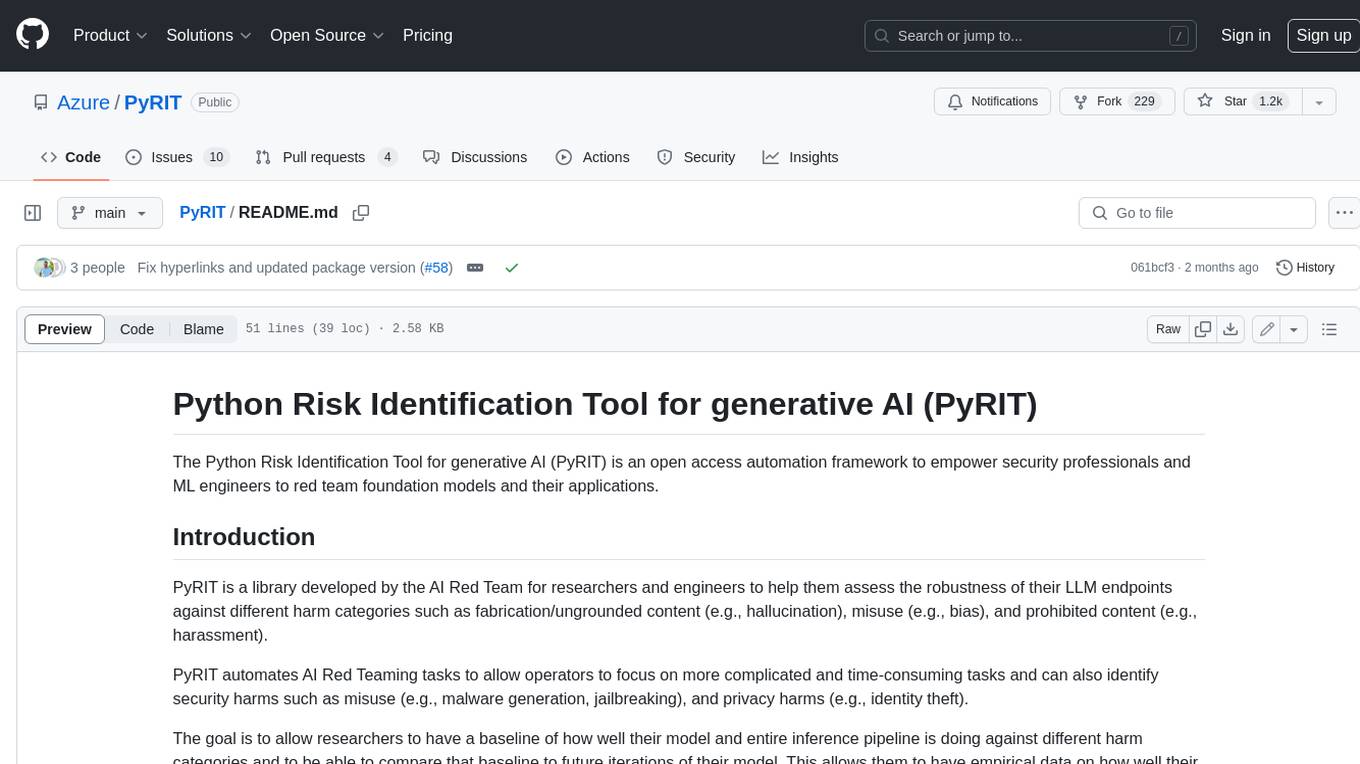
PyRIT
PyRIT is an open access automation framework designed to empower security professionals and ML engineers to red team foundation models and their applications. It automates AI Red Teaming tasks to allow operators to focus on more complicated and time-consuming tasks and can also identify security harms such as misuse (e.g., malware generation, jailbreaking), and privacy harms (e.g., identity theft). The goal is to allow researchers to have a baseline of how well their model and entire inference pipeline is doing against different harm categories and to be able to compare that baseline to future iterations of their model. This allows them to have empirical data on how well their model is doing today, and detect any degradation of performance based on future improvements.
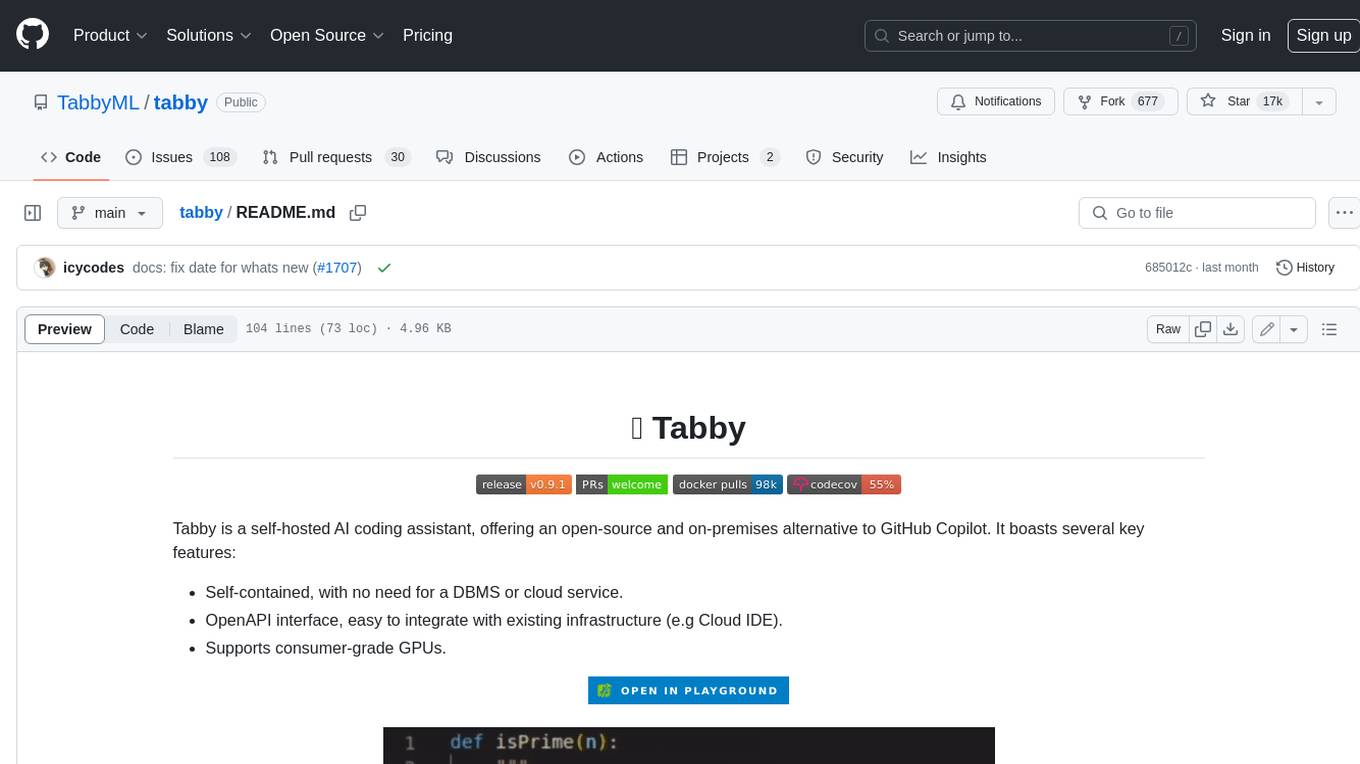
tabby
Tabby is a self-hosted AI coding assistant, offering an open-source and on-premises alternative to GitHub Copilot. It boasts several key features: * Self-contained, with no need for a DBMS or cloud service. * OpenAPI interface, easy to integrate with existing infrastructure (e.g Cloud IDE). * Supports consumer-grade GPUs.
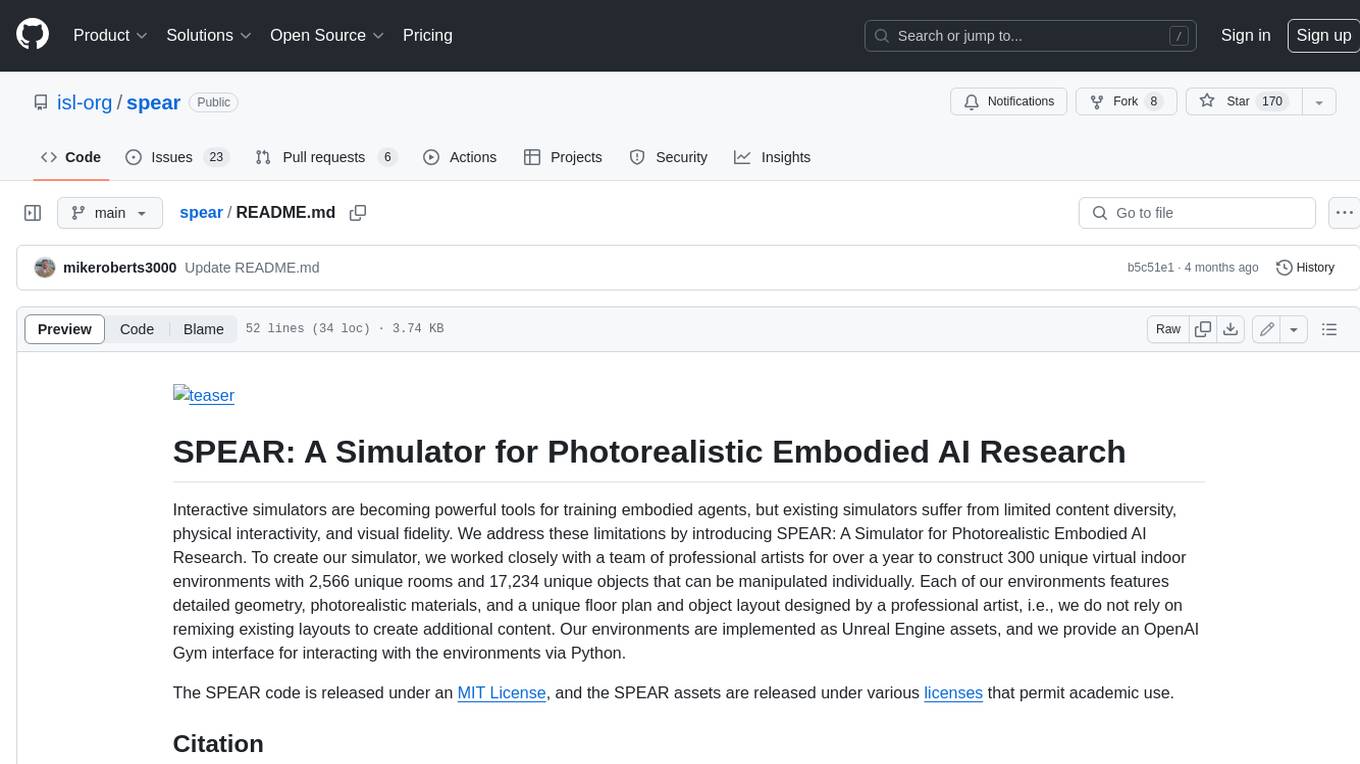
spear
SPEAR (Simulator for Photorealistic Embodied AI Research) is a powerful tool for training embodied agents. It features 300 unique virtual indoor environments with 2,566 unique rooms and 17,234 unique objects that can be manipulated individually. Each environment is designed by a professional artist and features detailed geometry, photorealistic materials, and a unique floor plan and object layout. SPEAR is implemented as Unreal Engine assets and provides an OpenAI Gym interface for interacting with the environments via Python.
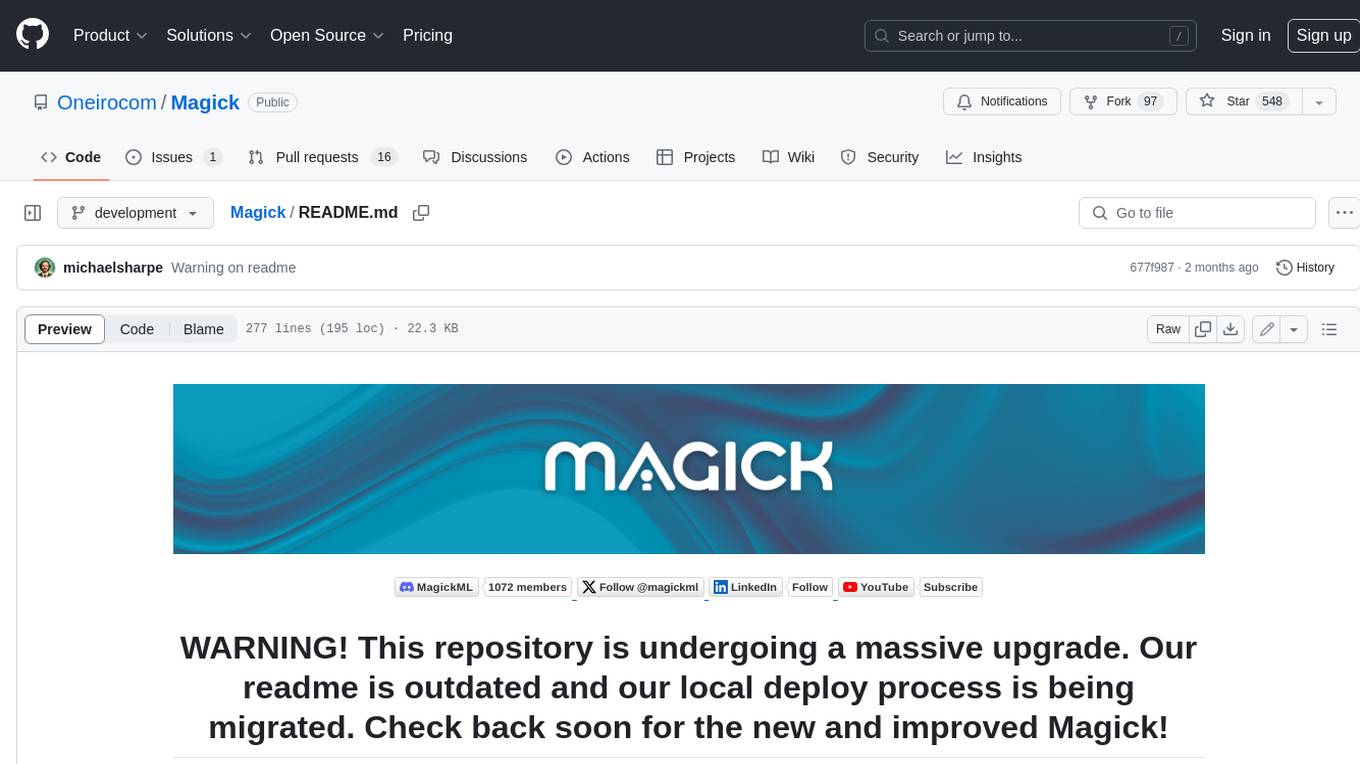
Magick
Magick is a groundbreaking visual AIDE (Artificial Intelligence Development Environment) for no-code data pipelines and multimodal agents. Magick can connect to other services and comes with nodes and templates well-suited for intelligent agents, chatbots, complex reasoning systems and realistic characters.