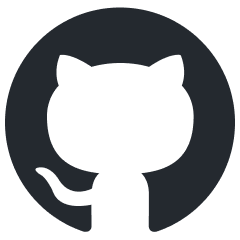
npcsh
The AI toolkit for the AI developer
Stars: 272
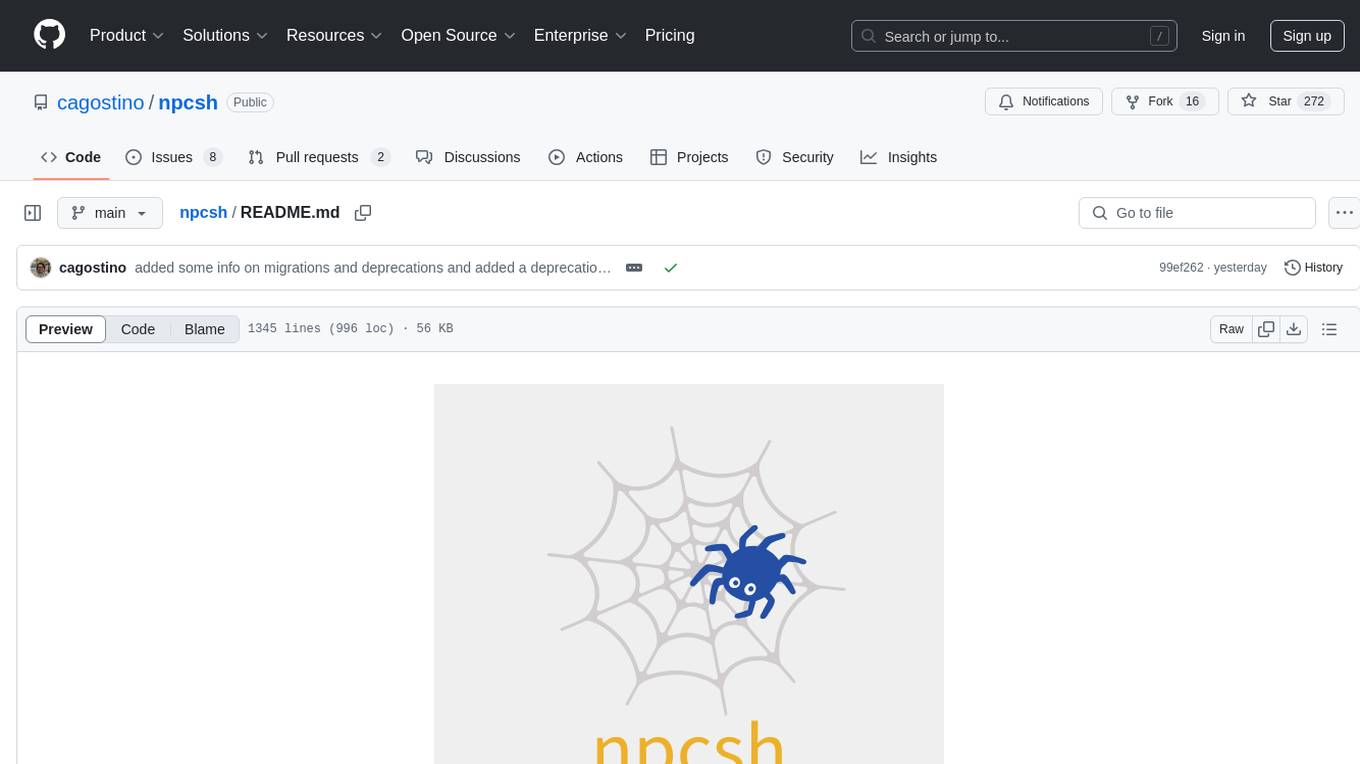
`npcsh` is a python-based command-line tool designed to integrate Large Language Models (LLMs) and Agents into one's daily workflow by making them available and easily configurable through the command line shell. It leverages the power of LLMs to understand natural language commands and questions, execute tasks, answer queries, and provide relevant information from local files and the web. Users can also build their own tools and call them like macros from the shell. `npcsh` allows users to take advantage of agents (i.e. NPCs) through a managed system, tailoring NPCs to specific tasks and workflows. The tool is extensible with Python, providing useful functions for interacting with LLMs, including explicit coverage for popular providers like ollama, anthropic, openai, gemini, deepseek, and openai-like providers. Users can set up a flask server to expose their NPC team for use as a backend service, run SQL models defined in their project, execute assembly lines, and verify the integrity of their NPC team's interrelations. Users can execute bash commands directly, use favorite command-line tools like VIM, Emacs, ipython, sqlite3, git, pipe the output of these commands to LLMs, or pass LLM results to bash commands.
README:
-
npcsh
is a python-based command-line tool designed to integrate Large Language Models (LLMs) and Agents into one's daily workflow by making them available and easily configurable through the command line shell. -
Smart Interpreter:
npcsh
leverages the power of LLMs to understand your natural language commands and questions, executing tasks, answering queries, and providing relevant information from local files and the web. -
Macros:
npcsh
provides macros to accomplish common tasks with LLMs like voice control (/whisper
), image generation (/vixynt
), screenshot capture and analysis (/ots
), one-shot questions (/sample
), computer use (/plonk
), retrieval augmented generation (/rag
), search (/search
) and more. Users can also build their own tools and call them like macros from the shell. -
NPC-Driven Interactions:
npcsh
allows users to take advantage of agents (i.e. NPCs) through a managed system. Users build a directory of NPCs and associated tools that can be used to accomplish complex tasks and workflows. NPCs can be tailored to specific tasks and have unique personalities, directives, and tools. Users can combine NPCs and tools in assembly line like workflows or use them in SQL-style models.
-
Extensible with Python:
npcsh
's python package provides useful functions for interacting with LLMs, including explicit coverage for popular providers like ollama, anthropic, openai, gemini, deepseek, and openai-like providers. Each macro has a corresponding function and these can be used in python scripts.npcsh
's functions are purpose-built to simplify NPC interactions but NPCs are not required for them to work if you don't see the need. -
Simple, Powerful CLI: Use the
npc
CLI commands to set up a flask server so you can expose your NPC team for use as a backend service. You can also use thenpc
CLI to run SQL models defined in your project, execute assembly lines, and verify the integrity of your NPC team's interrelations.npcsh
's NPCs take advantage of jinja templating to reference other NPCs and tools in their properties, and thenpc
CLI can be used to verify these references. -
Shell Strengths: Execute bash commands directly. Use your favorite command-line tools like VIM, Emacs, ipython, sqlite3, git. Pipe the output of these commands to LLMs or pass LLM results to bash commands.
Interested to stay in the loop and to hear the latest and greatest about npcsh
? Be sure to sign up for the npcsh newsletter!
npcsh
is available on PyPI and can be installed using pip. Before installing, make sure you have the necessary dependencies installed on your system. Below are the instructions for installing such dependencies on Linux, Mac, and Windows. If you find any other dependencies that are needed, please let us know so we can update the installation instructions to be more accommodating.
sudo apt-get install espeak
sudo apt-get install portaudio19-dev python3-pyaudio
sudo apt-get install alsa-base alsa-utils
sudo apt-get install libcairo2-dev
sudo apt-get install libgirepository1.0-dev
sudo apt-get install ffmpeg
#And if you don't have ollama installed, use this:
curl -fsSL https://ollama.com/install.sh | sh
ollama pull llama3.2
ollama pull llava:7b
ollama pull nomic-embed-text
pip install npcsh
brew install portaudio
brew install ffmpeg
brew install ollama
brew services start ollama
brew install pygobject3
ollama pull llama3.2
ollama pull llava:7b
ollama pull nomic-embed-text
pip install npcsh
Download and install ollama exe.
Then, in a powershell. Download and install ffmpeg.
ollama pull llama3.2
ollama pull llava:7b
ollama pull nomic-embed-text
pip install npcsh
As of now, npcsh appears to work well with some of the core functionalities like /ots and /whisper.
python3-dev (fixes hnswlib issues with chroma db) xhost + (pyautogui) python-tkinter (pyautogui)
After it has been pip installed, npcsh
can be used as a command line tool. Start it by typing:
npcsh
When initialized, npcsh
will generate a .npcshrc file in your home directory that stores your npcsh settings.
Here is an example of what the .npcshrc file might look like after this has been run.
# NPCSH Configuration File
export NPCSH_INITIALIZED=1
export NPCSH_CHAT_PROVIDER='ollama'
export NPCSH_CHAT_MODEL='llama3.2'
export NPCSH_DB_PATH='~/npcsh_history.db'
npcsh
also comes with a set of tools and NPCs that are used in processing. It will generate a folder at ~/.npcsh/ that contains the tools and NPCs that are used in the shell and these will be used in the absence of other project-specific ones. Additionally, npcsh
records interactions and compiled information about npcs within a local SQLite database at the path specified in the .npcshrc file. This will default to ~/npcsh_history.db if not specified. When the data mode is used to load or analyze data in CSVs or PDFs, these data will be stored in the same database for future reference.
The installer will automatically add this file to your shell config, but if it does not do so successfully for whatever reason you can add the following to your .bashrc or .zshrc:
# Source NPCSH configuration
if [ -f ~/.npcshrc ]; then
. ~/.npcshrc
fi
We support inference via openai
, anthropic
, ollama
,gemini
, deepseek
, and openai-like
APIs. The default provider must be one of ['openai','anthropic','ollama', 'gemini', 'deepseek', 'openai-like']
and the model must be one available from those providers.
To use tools that require API keys, create an .env
file up in the folder where you are working or place relevant API keys as env variables in your ~/.npcshrc. If you already have these API keys set in a ~/.bashrc or a ~/.zshrc or similar files, you need not additionally add them to ~/.npcshrc or to an .env
file. Here is an example of what an .env
file might look like:
export OPENAI_API_KEY="your_openai_key"
export ANTHROPIC_API_KEY="your_anthropic_key"
export DEEPSEEK_API_KEY='your_deepseek_key'
export GEMINI_API_KEY='your_gemini_key'
export PERPLEXITY_API_KEY='your_perplexity_key'
Individual npcs can also be set to use different models and providers by setting the model
and provider
keys in the npc files.
Once initialized and set up, you will find the following in your ~/.npcsh directory:
~/.npcsh/
├── npc_team/ # Global NPCs
│ ├── tools/ # Global tools
│ └── assembly_lines/ # Workflow pipelines
For cases where you wish to set up a project specific set of NPCs, tools, and assembly lines, add a npc_team
directory to your project and npcsh
should be able to pick up on its presence, like so:
./npc_team/ # Project-specific NPCs
├── tools/ # Project tools
└── assembly_lines/ # Project workflows
-In v0.3.4, the structure for tools was adjusted. If you have made custom tools please refer to the structure within npc_compiler to ensure that they are in the correct format. Otherwise, do the following
rm ~/.npcsh/npc_team/tools/*.tool
and then
npcsh
and the updated tools will be copied over into the correct location.
-Version 0.3.5 included a complete overhaul and refactoring of the llm_funcs module. This was done to make it not as horribly long and to make it easier to add new models and providers
-in version 0.3.5, a change was introduced to the database schema for messages to add npcs, models, providers, and associated attachments to data. If you have used npcsh
before this version, you will need to run this migration script to update your database schema: migrate_conversation_history_v0.3.5.py
-additionally, NPCSH_MODEL and NPCSH_PROVIDER have been renamed to NPCSH_CHAT_MODEL and NPCSH_CHAT_PROVIDER
to provide a more consistent naming scheme now that we have additionally introduced NPCSH_VISION_MODEL
and NPCSH_VISION_PROVIDER
, NPCSH_EMBEDDING_MODEL
, NPCSH_EMBEDDING_PROVIDER
, NPCSH_REASONING_MODEL
, NPCSH_REASONING_PROVIDER
, NPCSH_IMAGE_GEN_MODEL
, and NPCSH_IMAGE_GEN_PROVIDER
.
- In addition, we have added NPCSH_API_URL to better accommodate openai-like apis that require a specific url to be set as well as
NPCSH_STREAM_OUTPUT
to indicate whether or not to use streaming in one's responses. It will be set to 0 (false) by default as it has only been tested and verified for a small subset of the models and providers we have available (openai, anthropic, and ollama). If you try it and run into issues, please post them here so we can correct them as soon as possible !
In the npcsh
shell, users can ask LLMs questions, have LLMLs execute commands or use tools, or utilize macros that provide additional functionality. When a user does not invoke a specific macro, the shell will automatically decide which tool to use based on the user's input. Here are some examples of things one might ask the npcsh shell.
Here are some examples of how you can use npcsh
npcsh>Who was Simon Bolivar?
Simón Bolívar, often referred to as "El Libertador," was a Venezuelan military and political leader who played a key role in the Latin American wars of independence against Spanish
colonial rule in the early 19th century. He was born on July 24, 1783, in Caracas, Venezuela, into a wealthy Creole family.
Bolívar's vision extended beyond merely liberating territories; he aspired to unify the newly independent nations of South America into a single federation, which he called "Gran
Colombia," consisting of present-day Colombia, Venezuela, Ecuador, and Panama. He was known for his exceptional military strategies and leadership, which led to successful campaigns in
various regions, including the battles of Boyacá, Carabobo, and Ayacucho.
He faced numerous challenges, including political fragmentation, regional rivalries, and personal betrayals. Despite his initial successes, the unity he sought for Latin America proved
difficult to achieve, and Gran Colombia eventually disintegrated in the early 1830s.
Bolívar's influence and legacy extend far beyond his lifetime. He is celebrated in various countries across Latin America as a national hero and symbol of independence. His thoughts on
liberty, governance, and social issues continue to inspire movements for democracy and social justice in the region. Simón Bolívar died on December 17, 1830, but remains a pivotal figure
in the history of Latin America.
npcsh> What is the capital of France?
The capital of France is Paris. It is the largest city in the country and is known for its rich history, art, culture, and architecture, including famous landmarks such as the Eiffel Tower, Notre-Dame Cathedral, and the Louvre Museum.
npcsh> What's the weather in Tokyo?
handle_tool_call invoked with tool_name: generic_search_tool
The weather in Tokyo, Japan, is expected to be mixed with sun and clouds. Here are some details from the recent forecasts:
• Highs: Around 53°F to 58°F with a few variations depending on the day.
• Lows: Approximately 35°F to 40°F.
• Winds: Generally from the northwest at 5 to 10 mph.
• Condition: Mainly sunny, but there may be periods of clouds and some overcast conditions throughout the week.
For more detailed information, you can refer to sources like The Weather Channel or AccuWeather.
/home/caug/npcww/npcsh:npcsh>
In the below example, the code that was open was the screen capture analysis tool itself.
npcsh> Can you explain what the code does in the currently open VS code window?
handle_tool_call invoked with tool_name: screen_capture_analysis_tool
Screenshot saved as screenshot_20241223_225815.png
The code in the visible section of your VS Code window appears to be a script for capturing and analyzing screenshots. Here's a breakdown of what the code does:
1 Import Necessary Libraries: It imports required libraries like system, datetime, and pyautogui, which are essential for capturing screenshots and handling date-time operations.
2 Capture the Screen: The code captures the current screen using pyautogui.screenshot(), taking a screenshot of the entire screen.
3 File Management: It generates a unique filename for the screenshot using the current date and time. This ensures that each screenshot file is distinct.
4 Save the Screenshot: The captured screenshot is saved to a file with the generated filename.
5 Image Analysis: It likely imports a functionality (analyze_image_base) to analyze the screenshot for specific content or information.
6 Run the Analysis: While not explicitly shown, it appears to call this analysis function to process the saved screenshot.
In summary, this code automates the process of capturing a screenshot, saving it with a unique filename, and analyzing that image for specific content or patterns.
npcsh> What is the biggest file in my current folder?
LLM suggests the following bash command: ls -S | head -n 1
Running command: ls -S | head -n 1
Command executed with output: image_20241111_000033.png
I ran the command ls -S | head -n 1 in your current folder. This command sorts all files by size in descending order and then selects the first entry, which represents the largest file. The result of this operation shows that the biggest file in your current folder is image_20241111_000033.png.
npcsh>What is the best way to implement a linked list in Python?
The best way to implement a linked list in Python is to define a Node class for the individual elements and a LinkedList class to manage the nodes. Here's a basic implementation:
class Node:
def __init__(self, data):
self.data = data # Store the data
self.next = None # Initialize the next node as None
class LinkedList:
def __init__(self):
self.head = None # Initialize the head of the list
def insert(self, data):
"""Inserts a new node at the end of the linked list."""
new_node = Node(data)
if not self.head: # If list is empty, set new node as head
self.head = new_node
return
last_node = self.head
while last_node.next: # Traverse to the last node
last_node = last_node.next
last_node.next = new_node # Link the new node
def display(self):
"""Prints the linked list."""
current = self.head
while current:
print(current.data, end=" -> ")
current = current.next
print("None")
# Example usage:
llist = LinkedList()
llist.insert(1)
llist.insert(2)
llist.insert(3)
llist.display() # Output: 1 -> 2 -> 3 -> None
Explanation:
1 Node Class: Represents each node in the linked list. It has two attributes: data (to store the value) and next (to point to the next node).
2 LinkedList Class: Manages the linked list. It has a head attribute pointing to the first node.
3 Insert Method: Adds a new node to the end of the list.
4 Display Method: Prints the elements of the list in order.
This implementation is simple and can be extended with more methods (e.g., deletion, searching, etc.) as needed.
npcsh> In which of the files in the current directory is "Simon Bolivar" mentioned?
The user's query was about "Simon Bolivar." Based on the relevant context from the codebase, I found specific mentions and relevant information regarding Simon Bolívar in the documentation provided
in the README.md file.
From the relevant context, specifically in the section of README.md, it shared a code example that demonstrates how to load an NPC (Non-Player Character) named "Simon Bolivar" using SQLite and
answer a question about project updates. This code snippet indicates that "Simon Bolivar" is represented in the NPC system, allowing for interaction through questions to retrieve information.
Additionally, there is another passage that directly answers the query. It reads:
▌ "Simón Bolívar, often referred to as 'El Libertador,' was a Venezuelan military and political leader who played a key role in the Latin American wars of independence against Spanish colonial
▌ rule in the early 19th century."
This information highlights Bolívar's significant historical role and achievements, answering the query regarding who Simon Bolivar was, thus making it clear that he was a prominent leader in Latin
America's fight for independence.
To summarize, I arrived at this answer by examining the provided context in the README.md file that includes both the coding example that initializes an NPC named "Simon Bolivar" and a historical
overview of his contributions, which directly addresses the user's inquiry.
npcsh>what process is using the most ram on my computer right now?
LLM suggests the following bash command: ps aux --sort=-%mem | head -n 10
Running command: ps aux --sort=-%mem | head -n 10
Command executed with output: USER PID %CPU %MEM VSZ RSS TTY STAT START TIME COMMAND
caug 1040046 1.1 2.7 1226193464 1598980 ? SNl Dec22 23:30 /opt/vivaldi/vivaldi-bin --type=renderer --crashpad-handler-pid=4529 --enable-crash-reporter=,stable --change-stack-guard-on-fork=
caug 683148 0.0 1.9 2047464 1137232 ? SNl Dec13 10:11 io.elementary.appcenter
ollama 1884 0.0 1.8 3683920 1051020 ? SNsl Dec02 0:30 /usr/local/bin/ollama serve
caug 1191836 5.3 1.6 7346052 964988 pts/17 Sl+ 22:57 0:22 /home/caug/.pyenv/versions/npcsh/bin/python /home/caug/.pyenv/versions/3.11.0/envs/npcsh/bin/npcsh
caug 1145749 0.0 1.5 7068128 892500 pts/13 Sl+ Dec22 0:15 /home/caug/.pyenv/versions/3.11.0/bin/python3.11 /home/caug/.pyenv/versions/3.11.0/bin/npcsh
caug 493912 0.0 1.5 7050968 890012 pts/11 Sl+ Dec10 0:14 /home/caug/.pyenv/versions/npcsh/bin/python /home/caug/.pyenv/versions/npcsh/bin/npcsh
caug 4522 0.9 1.5 34391236 874756 ? SNLl Dec02 295:06 /opt/vivaldi/vivaldi-bin
caug 3995 0.7 1.1 6051812 683916 ? R<sl Dec02 221:19 /usr/bin/gnome-shell
caug 501721 0.3 1.0 1212584660 626248 ? SNl Dec10 60:22 /opt/vivaldi/vivaldi-bin --type=renderer --crashpad-handler-pid=4529 --enable-crash-reporter=,stable --change-stack-guard-on-fork=
To determine which processes are using the most RAM on your computer, I executed the command ps aux --sort=-%mem | head -n 10.
This command lists all running processes (ps aux), sorts them by memory usage in descending order (--sort=-%mem), and then retrieves the top 10 entries (head -n 10).
The output shows the processes currently consuming the most memory. In the results, the process using the most RAM is Vivaldi's renderer, followed by other applications such as the Elementary App Center, Ollama server, and several instances of Python related to your NPC shell.
npcsh> can you generate an image of a calico cat and an orange tabby cat in the style of a japanese woodblock print where the two cats are engaged in a samurai battle but instead of samurai swords they are holding cat toys that are like wands with felt birds on the ends of them on strings
handle_tool_call invoked with tool_name: image_generation_tool
In addition to its ability to execute one-off tasks or tool calls, npcsh also has the ability to generate tool call sequences when asked:
npcsh> can you use a tool sequence to find the gdp of russia in 2024 and then to use that information to generate an image?
handle_tool_call invoked with tool_name: generic_search_tool
Tool found: generic_search_tool
handle_tool_call invoked with tool_name: image_generation_tool
Tool found: image_generation_tool
The Gross Domestic Product (GDP) of Russia is estimated to be $8.311 trillion by the end of 2024, according to World Economics.
This figure is significantly larger than the official estimate of $5.804 trillion published by the World Bank for the end of 2023.
It seems that you've generated an image. If you have any questions or need assistance related to this image, please let me know how I can help!
and then the associated image :
An important facet that makes npcsh
so powerful is the ability to pipe outputs from one tool call to another. This allows for the chaining of commands and the creation of complex workflows. For example, you can use the output of a search to generate an image, or you can use the output of an image analysis to generate a report. Here is an example of how this might look in practice:
npcsh> what is the gdp of russia in 2024? | /vixynt 'generate an image that contains {0}'
### Executing Bash Commands
You can execute bash commands directly within npcsh. The LLM can also generate and execute bash commands based on your natural language requests.
For example:
```npcsh
npcsh> ls -l
npcsh> cp file1.txt file2.txt
npcsh> mv file1.txt file2.txt
npcsh> mkdir new_directory
npcsh> git status
npcsh> vim file.txt
While npcsh can decide the best option to use based on the user's input, the user can also execute certain actions with a macro. Macros are commands that start with a forward slash (/) and are followed (in some cases) by the relevant arguments for those macros.
To learn about them from within the shell, type:
npcsh> /help
To exit the shell:
npcsh> /exit
Otherwise, here are some more detailed examples of macros that can be used in npcsh:
Use the /conjure
macro to generate an NPC, a NPC tool, an assembly line, a job, or an SQL model
Use the /debate
macro to have two or more NPCs debate a topic, problem, or question.
For example:
npcsh> /debate Should humans colonize Mars? npcs = ['sibiji', 'mark', 'ted']
Jot down notes and store them within the npcsh database and in the current directory as a text file.
npcsh> /notes
Use the /ots macro to take a screenshot and write a prompt for an LLM to answer about the screenshot.
npcsh> /ots
Screenshot saved to: /home/caug/.npcsh/screenshots/screenshot_1735015011.png
Enter a prompt for the LLM about this image (or press Enter to skip): describe whats in this image
The image displays a source control graph, likely from a version control system like Git. It features a series of commits represented by colored dots connected by lines, illustrating the project's development history. Each commit message provides a brief description of the changes made, including tasks like fixing issues, merging pull requests, updating README files, and adjusting code or documentation. Notably, several commits mention specific users, particularly "Chris Agostino," indicating collaboration and contributions to the project. The graph visually represents the branching and merging of code changes.
Alternatively, pass an existing image in like :
npcsh> /ots test_data/catfight.PNG
Enter a prompt for the LLM about this image (or press Enter to skip): whats in this ?
The image features two cats, one calico and one orange tabby, playing with traditional Japanese-style toys. They are each holding sticks attached to colorful pom-pom balls, which resemble birds. The background includes stylized waves and a red sun, accentuating a vibrant, artistic style reminiscent of classic Japanese art. The playful interaction between the cats evokes a lively, whimsical scene.
Use the /plan macro to schedule tasks to be run at regular intervals.
npcsh> /plan run a rag search on the files in the current directory every 5 minutes
Use the /plonk macro to allow the LLM to control your computer.
npcsh> /plonk go to a web browser and go to wikipedia and find out information about simon bolivar
Use the /rag macro to perform a local rag search.
If you pass a -f
flag with a filename or list of filenames (e.g. *.py) then it will embed the documents and perform the cosine similarity scoring.
npcsh> /rag -f *.py what is the best way to implement a linked list in Python?
Alternatively , if you want to perform rag on your past conversations, you can do so like this:
npcsh> /rag what is the best way to implement a linked list in Python?
and it will automatically look through the recorded conversations in the ~/npcsh_history.db
Use the /rehash macro to re-send the last message to the LLM.
npcsh> /rehash
Send a one-shot question to the LLM.
npcsh> /sample What is the capital of France?
Search can be accomplished through the /search
macro. You can specify the provider as being "perplexity" or "duckduckgo". For the former,
you must set a perplexity api key as an environment variable as described above. The default provider is duckduckgo.
NOTE: while google is an available search engine, they recently implemented changes (early 2025) that make the python google search package no longer as reliable. For now, we will use duckduckgo and revisit this issue when other more critical aspects are handled.
npcsh!> /search -p duckduckgo who is the current us president
President Donald J. Trump entered office on January 20, 2025. News, issues, and photos of the President Footer Disclaimer This is the official website of the U.S. Mission to the United Nations. External links to other Internet sites should not be construed as an endorsement of the views or privacy policies contained therein.
Citation: https://usun.usmission.gov/our-leaders/the-president-of-the-united-states/
45th & 47th President of the United States After a landslide election victory in 2024, President Donald J. Trump is returning to the White House to build upon his previous successes and use his mandate to reject the extremist policies of the radical left while providing tangible quality of life improvements for the American people. Vice President of the United States In 2024, President Donald J. Trump extended JD the incredible honor of asking him to serve as the Vice-Presidential Nominee for th...
Citation: https://www.whitehouse.gov/administration/
Citation: https://www.instagram.com/potus/?hl=en
The president of the United States (POTUS)[B] is the head of state and head of government of the United States. The president directs the executive branch of the federal government and is the commander-in-chief of the United States Armed Forces. The power of the presidency has grown substantially[12] since the first president, George Washington, took office in 1789.[6] While presidential power has ebbed and flowed over time, the presidency has played an increasingly significant role in American ...
Citation: https://en.wikipedia.org/wiki/President_of_the_United_States
Citation Links: https://usun.usmission.gov/our-leaders/the-president-of-the-united-states/
https://www.whitehouse.gov/administration/
https://www.instagram.com/potus/?hl=en
https://en.wikipedia.org/wiki/President_of_the_United_States
```npcsh
npcsh> /search -p perplexity who is the current us president
The current President of the United States is Donald Trump, who assumed office on January 20, 2025, for his second non-consecutive term as the 47th president[1].
Citation Links: ['https://en.wikipedia.org/wiki/List_of_presidents_of_the_United_States',
'https://en.wikipedia.org/wiki/Joe_Biden',
'https://www.britannica.com/topic/Presidents-of-the-United-States-1846696',
'https://news.gallup.com/poll/329384/presidential-approval-ratings-joe-biden.aspx',
'https://www.usa.gov/presidents']
Users can change the default model and provider from within npcsh by using the following commands:
npcsh> /set model ollama
npcsh> /set provider llama3.2
Use the /sleep
macro to create or update a knowledge graph. A knowledge graph is a structured representation of facts about you as a user that the NPCs can determine based on the conversations you have had with it.
npcsh> /sleep
breathe: a method for condensing context on a regular cadence (# messages, len(context), etc) (under construction)
-every 10 messages/7500 characters, condense the conversation into lessons learned. write the lessons learned down by the np for the day, then the npc will see the lessons they have learned that day in that folder as part of the context.
Spool mode allows one to enter into a conversation with a specific LLM or a specific NPC. This is used for having distinct interactions from those in the base shell and these will be separately contained.
Start the spool mode:
npcsh> /spool
Start the spool mode with a specific npc
npcsh> /spool npc=foreman
Start the spool mode with specific files in context that will be referenced through RAG searches when relevant.
npcsh> /spool files=[*.py,*.md] # Load specific files for context
Have a conversation and switch between text and voice mode by invoking /whisper
mode from within spool mode.
spool> what can you tell me about green bull from one piece?
Green Bull, also known as Ryokugyu, is a character from the popular anime and manga series One Piece. He is one of the Marine Admirals and was introduced during the Wano Country arc. Here are some key points about Green
Bull:
1 Real Name: His real name is Aramaki, but he is primarily known by his title, Green Bull (Ryokugyu in Japanese).
2 Appearance: Green Bull has a distinct look characterized by a green military uniform, a long cape, and a
noticeable plant-based theme, which aligns with his powers.
3 Devil Fruit: Green Bull is a user of the Mori Mori no Mi (Forest Forest Fruit), a Logia-type Devil Fruit that
allows him to generate and manipulate plant matter. This ability can be seen in his combat style, which utilizes
plants to entrap and attack opponents.
4 Role as an Admiral: As an Admiral, Green Bull holds a high-ranking position in the Marines and is
known for his strength and authority. He is fiercely loyal to the Marine organization and its goals.
5 Personality: Green Bull displays a laid-back attitude and often appears more relaxed compared to other Admirals.
His personality can be somewhat ambiguous, which adds depth to his character.
6 Involvement in the Story: Green Bull's introduction brings anticipation regarding his potential confrontations with
key characters in the series. His role in major arcs like Wano and his interactions with the Straw Hat Pirates and
other significant figures are crucial for the unfolding storyline.
Overall, Green Bull is an intriguing character with unique abilities and a significant role within the One Piece universe.
spool> /whisper
Calibrating silence level. Please remain quiet...
Silence threshold set to: 5679.193548387097
Listening... (speak now)
...............
Max duration reached.
Processing...
You said: What do you think his greatest weakness is?
Based on what we know about Green Bull from the One Piece series, I can identify a few potential weaknesses:
1. **Extreme Laziness**:
- Green Bull is known for being incredibly lazy, often refusing to move or exert himself unnecessarily.
- This laziness could be exploited by opponents who understand how to manipulate or provoke him into action.
2. **Dependency on External Nutrition**:
- His Devil Fruit power allows him to absorb nutrients from the environment, which suggests he might become weakened in environments with limited plant life or nutrients.
- In extremely barren or non-vegetative settings, his regenerative and plant-based abilities might be significantly reduced.
3. **Pride and Marine Ideology**:
- Like many Marines, he likely has a rigid sense of justice that could be used against him strategically.
- His commitment to Marine principles might make him predictable in certain confrontational scenarios.
4. **Potential Overconfidence**:
- As an Admiral, he might underestimate opponents, especially pirates, due to his high-ranking status.
- His laid-back nature might lead him to not take threats seriously until it's too late.
The most pronounced weakness seems to be his extreme laziness, which could potentially be exploited tactically by skilled opponents who understand how to force him into action or create scenarios that challenge his passive nature.
Here are some additional details about Green Bull (Aramaki) in "One Piece":
1 Devil Fruit Name:
• Green Bull's Devil Fruit has been confirmed to be the Mori Mori no Mi, which is a mythical Zoan-type fruit. This allows him to utilize various forms of plant life in combat and has regenerative
capabilities.
2 Combat Abilities:
• His ability to control vegetation gives him significant advantages in battle. He can generate plants to attack or defend and possibly use them for tactical advantages, such as creating barriers
or entangling enemies.
3 Reputation:
• As an Admiral, Green Bull holds a position of great power and authority within the Marines. His reputation is likely formidable among pirates and other opponents.
4 Interactions with Other Characters:
• Green Bull's character dynamics with others in the series, particularly with fellow Marines and pirates, can provide insight into his personality and values. His interactions during missions or
discussions about justice will reveal more about his character.
5 Appearance and Style:
• He has a rather unique aesthetic, characterized by his green clothing that symbolizes his connection to nature. His overall appearance contributes to his identity as a plant-based fighter.
6 Backstory:
• As of now, specific details about his backstory and how he rose to the rank of Admiral are not extensively explored in the series. This leaves room for further character development and
background to be unveiled in future chapters.
7 Ambiguous Personality:
• While his laziness and laid-back demeanor are evident, it is possible that there are deeper layers to his character that might be revealed through his actions and motivations within the
overarching narrative of "One Piece."
8 Role in the Marine Organization:
• His position as Admiral places him in direct opposition to the main pirate characters, particularly the Straw Hat crew, making him a significant figure in the ongoing conflict between pirates
and the Marines.
As the story continues to develop, Green Bull's character may evolve and reveal more complexities, weaknesses, and relationships within the world of "One Piece."
Start the spool with a specific llm model:
#note this is not yet implemented
npcsh> /spool model=llama3.3
Image generation can be done with the /vixynt macro. Use /vixynt like so where you can also specify the model to use with an @ reference. This @ reference will override the default model in ~/.npcshrc.
npcsh> /vixynt A futuristic cityscape @dall-e-3
npcsh> /vixynt A peaceful landscape @runwayml/stable-diffusion-v1-5
Enter into a voice-controlled mode to interact with the LLM. This mode can executet commands and use tools just like the basic npcsh shell.
npcsh> /whisper
Compile a specified NPC profile. This will make it available for use in npcsh interactions.
npcsh> /compile <npc_file>
You can also use /com
as an alias for /compile
. If no NPC file is specified, all NPCs in the npc_team directory will be compiled.
Begin a conversations with a specified NPC by referencing their name
npcsh> /<npc_name>:
NPCs are defined in YAML files within the npc_team directory. Each NPC has a name, primary directive, and optionally, a list of tools. See the examples in the npc_profiles directory for guidance.
Here is a typical NPC file:
name: sibiji
primary_directive: You are a foundational AI assistant. Your role is to provide basic support and information. Respond to queries concisely and accurately.
tools:
- simple data retrieval
model: llama3.2
provider: ollama
Tools are defined as YAMLs with .tool
extension within the npc_team/tools directory. Each tool has a name, inputs, and consists of three distinct steps: preprocess, prompt, and postprocess. The idea here is that a tool consists of a stage where information is preprocessed and then passed to a prompt for some kind of analysis and then can be passed to another stage for postprocessing. In each of these three cases, the engine must be specified. The engine can be either "natural" for natural language processing or "python" for Python code. The code is the actual code that will be executed.
Here is an example of a tool file:
tool_name: "screen_capture_analysis_tool"
inputs:
- "prompt"
preprocess:
- engine: "python"
code: |
# Capture the screen
import pyautogui
import datetime
import os
from PIL import Image
from npcsh.image import analyze_image_base
# Generate filename
filename = f"screenshot_{datetime.datetime.now().strftime('%Y%m%d_%H%M%S')}.png"
screenshot = pyautogui.screenshot()
screenshot.save(filename)
print(f"Screenshot saved as {filename}")
# Load image
image = Image.open(filename)
# Full file path
file_path = os.path.abspath('./'+filename)
# Analyze the image
llm_output = analyze_image_base(inputs['prompt']+ '\n\n attached is a screenshot of my screen currently.', file_path, filename, npc=npc)
prompt:
engine: "natural"
code: ""
postprocess:
- engine: "natural"
code: |
Screenshot captured and saved as {{ filename }}.
Analysis Result: {{ llm_output }}
When you have created a tool, it will be surfaced as a potential option to be used when you ask a question in the base npcsh shell. The LLM will decide if it is the best tool to use based on the user's input. Alternatively, if you'd like, you can call the tools directly, without needing to let the AI decide if it's the right one to use.
npcsh> /screen_cap_tool <prompt>
or
npcsh> /sql_executor select * from conversation_history limit 1
or
npcsh> /calculator 5+6
Let's say you want to create a pipeline of steps where NPCs are used along the way. Let's initialize with a pipeline file we'll call morning_routine.pipe
:
steps:
- step_name: "review_email"
npc: "{{ ref('email_assistant') }}"
task: "Get me up to speed on my recent emails: {{source('emails')}}."
- step_name: "market_update"
npc: "{{ ref('market_analyst') }}"
task: "Give me an update on the latest events in the market: {{source('market_events')}}."
- step_name: "summarize"
npc: "{{ ref('sibiji') }}"
model: llama3.2
provider: ollama
task: "Review the outputs from the {{review_email}} and {{market_update}} and provide me with a summary."
Now youll see that we reference NPCs in the pipeline file. We'll need to make sure we have each of those NPCs available. Here is an example for the email assistant:
name: email_assistant
primary_directive: You are an AI assistant specialized in managing and summarizing emails. You should present the information in a clear and concise manner.
model: gpt-4o-mini
provider: openai
Now for the marketing analyst:
name: market_analyst
primary_directive: You are an AI assistant focused on monitoring and analyzing market trends. Provide de
model: llama3.2
provider: ollama
and then here is our trusty friend sibiji:
name: sibiji
primary_directive: You are a foundational AI assistant. Your role is to provide basic support and information. Respond to queries concisely and accurately.
suggested_tools_to_use:
- simple data retrieval
model: claude-3-5-sonnet-latest
provider: anthropic
Now that we have our pipeline and NPCs defined, we also need to ensure that the source data we are referencing will be there. When we use source('market_events') and source('emails') we are asking npcsh to pull those data directly from tables in our npcsh database. For simplicity we will just make these in python to insert them for this demo:
import pandas as pd
from sqlalchemy import create_engine
import os
# Sample market events data
market_events_data = {
"datetime": [
"2023-10-15 09:00:00",
"2023-10-16 10:30:00",
"2023-10-17 11:45:00",
"2023-10-18 13:15:00",
"2023-10-19 14:30:00",
],
"headline": [
"Stock Market Rallies Amid Positive Economic Data",
"Tech Giant Announces New Product Line",
"Federal Reserve Hints at Interest Rate Pause",
"Oil Prices Surge Following Supply Concerns",
"Retail Sector Reports Record Q3 Earnings",
],
}
# Create a DataFrame
market_events_df = pd.DataFrame(market_events_data)
# Define database path relative to user's home directory
db_path = os.path.expanduser("~/npcsh_history.db")
# Create a connection to the SQLite database
engine = create_engine(f"sqlite:///{db_path}")
with engine.connect() as connection:
# Write the data to a new table 'market_events', replacing existing data
market_events_df.to_sql(
"market_events", con=connection, if_exists="replace", index=False
)
print("Market events have been added to the database.")
email_data = {
"datetime": [
"2023-10-10 10:00:00",
"2023-10-11 11:00:00",
"2023-10-12 12:00:00",
"2023-10-13 13:00:00",
"2023-10-14 14:00:00",
],
"subject": [
"Meeting Reminder",
"Project Update",
"Invoice Attached",
"Weekly Report",
"Holiday Notice",
],
"sender": [
"[email protected]",
"[email protected]",
"[email protected]",
"[email protected]",
"[email protected]",
],
"recipient": [
"[email protected]",
"[email protected]",
"[email protected]",
"[email protected]",
"[email protected]",
],
"body": [
"Don't forget the meeting tomorrow at 10 AM.",
"The project is progressing well, see attached update.",
"Please find your invoice attached.",
"Here is the weekly report.",
"The office will be closed on holidays, have a great time!",
],
}
# Create a DataFrame
emails_df = pd.DataFrame(email_data)
# Define database path relative to user's home directory
db_path = os.path.expanduser("~/npcsh_history.db")
# Create a connection to the SQLite database
engine = create_engine(f"sqlite:///{db_path}")
with engine.connect() as connection:
# Write the data to a new table 'emails', replacing existing data
emails_df.to_sql("emails", con=connection, if_exists="replace", index=False)
print("Sample emails have been added to the database.")
With these data now in place, we can proceed with running the pipeline. We can do this in npcsh by using the /compile command.
npcsh> /compile morning_routine.pipe
Alternatively we can run a pipeline like so in Python:
from npcsh.npc_compiler import PipelineRunner
import os
pipeline_runner = PipelineRunner(
pipeline_file="morning_routine.pipe",
npc_root_dir=os.path.abspath("./"),
db_path="~/npcsh_history.db",
)
pipeline_runner.execute_pipeline(inputs)
What if you wanted to run operations on each row and some operations on all the data at once? We can do this with the pipelines as well. Here we will build a pipeline for news article analysis. First we make the data for the pipeline that well use:
import pandas as pd
from sqlalchemy import create_engine
import os
# Sample data generation for news articles
news_articles_data = {
"news_article_id": list(range(1, 21)),
"headline": [
"Economy sees unexpected growth in Q4",
"New tech gadget takes the world by storm",
"Political debate heats up over new policy",
"Health concerns rise amid new disease outbreak",
"Sports team secures victory in last minute",
"New economic policy introduced by government",
"Breakthrough in AI technology announced",
"Political leader delivers speech on reforms",
"Healthcare systems pushed to limits",
"Celebrated athlete breaks world record",
"Controversial economic measures spark debate",
"Innovative tech startup gains traction",
"Political scandal shakes administration",
"Healthcare workers protest for better pay",
"Major sports event postponed due to weather",
"Trade tensions impact global economy",
"Tech company accused of data breach",
"Election results lead to political upheaval",
"Vaccine developments offer hope amid pandemic",
"Sports league announces return to action",
],
"content": ["Article content here..." for _ in range(20)],
"publication_date": pd.date_range(start="1/1/2023", periods=20, freq="D"),
}
Then we will create the pipeline file:
# news_analysis.pipe
steps:
- step_name: "classify_news"
npc: "{{ ref('news_assistant') }}"
task: |
Classify the following news articles into one of the categories:
["Politics", "Economy", "Technology", "Sports", "Health"].
{{ source('news_articles') }}
- step_name: "analyze_news"
npc: "{{ ref('news_assistant') }}"
batch_mode: true # Process articles with knowledge of their tags
task: |
Based on the category assigned in {{classify_news}}, provide an in-depth
analysis and perspectives on the article. Consider these aspects:
["Impacts", "Market Reaction", "Cultural Significance", "Predictions"].
{{ source('news_articles') }}
Then we can run the pipeline like so:
/compile ./npc_team/news_analysis.pipe
or in python like:
from npcsh.npc_compiler import PipelineRunner
import os
runner = PipelineRunner(
"./news_analysis.pipe",
db_path=os.path.expanduser("~/npcsh_history.db"),
npc_root_dir=os.path.abspath("."),
)
results = runner.execute_pipeline()
Alternatively, if youd like to use a mixture of agents in your pipeline, set one up like this:
steps:
- step_name: "classify_news"
npc: "news_assistant"
mixa: true
mixa_agents:
- "{{ ref('news_assistant') }}"
- "{{ ref('journalist_npc') }}"
- "{{ ref('data_scientist_npc') }}"
mixa_voters:
- "{{ ref('critic_npc') }}"
- "{{ ref('editor_npc') }}"
- "{{ ref('researcher_npc') }}"
mixa_voter_count: 5
mixa_turns: 3
mixa_strategy: "vote"
task: |
Classify the following news articles...
{{ source('news_articles') }}
You'll have to make npcs for these references to work, here are versions that should work with the above:
name: news_assistant
Then, we can run the mixture of agents method like:
/compile ./npc_team/news_analysis_mixa.pipe
or in python like:
from npcsh.npc_compiler import PipelineRunner
import os
runner = PipelineRunner(
"./news_analysis_mixa.pipe",
db_path=os.path.expanduser("~/npcsh_history.db"),
npc_root_dir=os.path.abspath("."),
)
results = runner.execute_pipeline()
Note, in the future we will aim to separate compilation and running so that we will have a compilation step that is more like a jinja rendering of the relevant information so that it can be more easily audited.
When npcsh is installed, it comes with the npc
cli as well. The npc
cli has various command to make initializing and serving NPC projects easier.
To serve an NPC project, first install redis-server and start it
on Ubuntu:
sudo apt update && sudo apt install redis-server
redis-server
on macOS:
brew install redis
redis-server
Then navigate to the project directory and run:
npc serve
If you want to specify a certain port, you can do so with the -p
flag:
npc serve -p 5337
or with the --port
flag:
npc serve --port 5337
Once the server is up and running, you can access the API endpoints at http://localhost:5337/api/
. Here are some example curl commands to test the endpoints:
echo "Testing health endpoint..."
curl -s http://localhost:5337/api/health | jq '.'
echo -e "\nTesting execute endpoint..."
curl -s -X POST http://localhost:5337/api/execute \
-H "Content-Type: application/json" \
-d '{"commandstr": "hello world", "currentPath": "/media/caug/extradrive1/npcww/npcsh", "conversationId": "test124"}' | jq '.'
echo -e "\nTesting conversations endpoint..."
curl -s "http://localhost:5337/api/conversations?path=/tmp" | jq '.'
echo -e "\nTesting conversation messages endpoint..."
curl -s http://localhost:5337/api/conversation/test123/messages | jq '.'
- Planned: -npc scripts -npc run select +sql_model -npc run select +sql_model+ -npc run select sql_model+ -npc run line <assembly_line>
Integrate npcsh into your Python projects for additional flexibility. Below are a few examples of how to use the library programmatically.
This example shows how to create and initialize an NPC and use it to answer a question.
import sqlite3
from npcsh.npc_compiler import NPC
# Set up database connection
db_path = '~/npcsh_history.db'
conn = sqlite3.connect(db_path)
# Load NPC from a file
npc = NPC(db_conn=conn,
name='Simon Bolivar',
primary_directive='Liberate South America from the Spanish Royalists.',
model='gpt-4o-mini',
provider='openai',
)
response = npc.get_llm_response("What is the most important territory to retain in the Andes mountains?")
print(response['response'])
'The most important territory to retain in the Andes mountains for the cause of liberation in South America would be the region of Quito in present-day Ecuador. This area is strategically significant due to its location and access to key trade routes. It also acts as a vital link between the northern and southern parts of the continent, influencing both military movements and the morale of the independence struggle. Retaining control over Quito would bolster efforts to unite various factions in the fight against Spanish colonial rule across the Andean states.'
This example shows how to use an NPC to perform data analysis on a DataFrame using LLM commands.
from npcsh.npc_compiler import NPC
import sqlite3
import os
# Set up database connection
db_path = '~/npcsh_history.db'
conn = sqlite3.connect(os.path.expanduser(db_path))
# make a table to put into npcsh_history.db or change this example to use an existing table in a database you have
import pandas as pd
data = {
'customer_feedback': ['The product is great!', 'The service was terrible.', 'I love the new feature.'],
'customer_id': [1, 2, 3],
'customer_rating': [5, 1, 3],
'timestamp': ['2022-01-01', '2022-01-02', '2022-01-03']
}
df = pd.DataFrame(data)
df.to_sql('customer_feedback', conn, if_exists='replace', index=False)
npc = NPC(db_conn=conn,
name='Felix',
primary_directive='Analyze customer feedback for sentiment.',
model='llama3.2',
provider='ollama',
)
response = npc.analyze_db_data('Provide a detailed report on the data contained in the `customer_feedback` table?')
You can define a tool and execute it from within your Python script. Here we'll create a tool that will take in a pdf file, extract the text, and then answer a user request about the text.
from npcsh.npc_compiler import Tool, NPC
import sqlite3
import os
tool_data = {
"tool_name": "pdf_analyzer",
"inputs": ["request", "file"],
"preprocess": [{ # Make this a list with one dict inside
"engine": "python",
"code": """
try:
import fitz # PyMuPDF
shared_context = {}
shared_context['inputs'] = inputs
pdf_path = inputs['file']
print(f"Processing PDF file: {pdf_path}")
# Open the PDF
doc = fitz.open(pdf_path)
text = ""
# Extract text from each page
for page_num in range(len(doc)):
page = doc[page_num]
text += page.get_text()
# Close the document
doc.close()
print(f"Extracted text length: {len(text)}")
if len(text) > 100:
print(f"First 100 characters: {text[:100]}...")
shared_context['extracted_text'] = text
print("Text extraction completed successfully")
except Exception as e:
error_msg = f"Error processing PDF: {str(e)}"
print(error_msg)
shared_context['extracted_text'] = f"Error: {error_msg}"
"""
}],
"prompt": {
"engine": "natural",
"code": """
{% if shared_context and shared_context.extracted_text %}
{% if shared_context.extracted_text.startswith('Error:') %}
{{ shared_context.extracted_text }}
{% else %}
Here is the text extracted from the PDF:
{{ shared_context.extracted_text }}
Please provide a response to user request: {{ inputs.request }} using the information extracted above.
{% endif %}
{% else %}
Error: No text was extracted from the PDF.
{% endif %}
"""
},
"postprocess": [] # Empty list instead of empty dict
}
# Instantiate the tool
tool = Tool(tool_data)
# Create an NPC instance
npc = NPC(
name='starlana',
primary_directive='Analyze text from Astrophysics papers with a keen attention to theoretical machinations and mechanisms.',
db_conn=sqlite3.connect(os.path.expanduser('~/npcsh_database.db'))
)
# Define input values dictionary
input_values = {
"request": "what is the point of the yuan and narayanan work?",
"file": os.path.abspath("test_data/yuan2004.pdf")
}
print(f"Attempting to read file: {input_values['file']}")
print(f"File exists: {os.path.exists(input_values['file'])}")
# Execute the tool
output = tool.execute(input_values, npc.tools_dict, None, 'Sample Command', npc)
print('Tool Output:', output)
In addition to NPCs being used in npcsh
and through the python package, users may wish to take advantage of agentic interactions in SQL-like pipelines.
npcsh
contains a pseudo-SQL interpreter that processes SQL models which lets users write queries containing LLM-function calls that reference specific NPCs. npcsh
interprets these queries, renders any jinja template references through its python implementation, and then executes them accordingly.
Here is an example of a SQL-like query that uses NPCs to analyze data:
SELECT debate(['logician','magician'], 'Analyze the sentiment of the customer feedback.') AS sentiment_analysis
squish is an aggregate NPC LLM function that compresses information contained in whole columns or grouped chunks of data based on the SQL aggregation.
Splat is a row-wise NPC LLM function that allows for the application of an LLM function on each row of a dataset or a re-sampling
Contributions are welcome! Please submit issues and pull requests on the GitHub repository.
If you appreciate the work here, consider supporting NPC Worldwide. If you'd like to explore how to use npcsh
to help your business, please reach out to [email protected] .
Coming soon! NPC Studio will be a desktop application for managing chats and agents on your own machine. Be sure to sign up for the npcsh newsletter to hear updates!
This project is licensed under the MIT License.
For Tasks:
Click tags to check more tools for each tasksFor Jobs:
Alternative AI tools for npcsh
Similar Open Source Tools
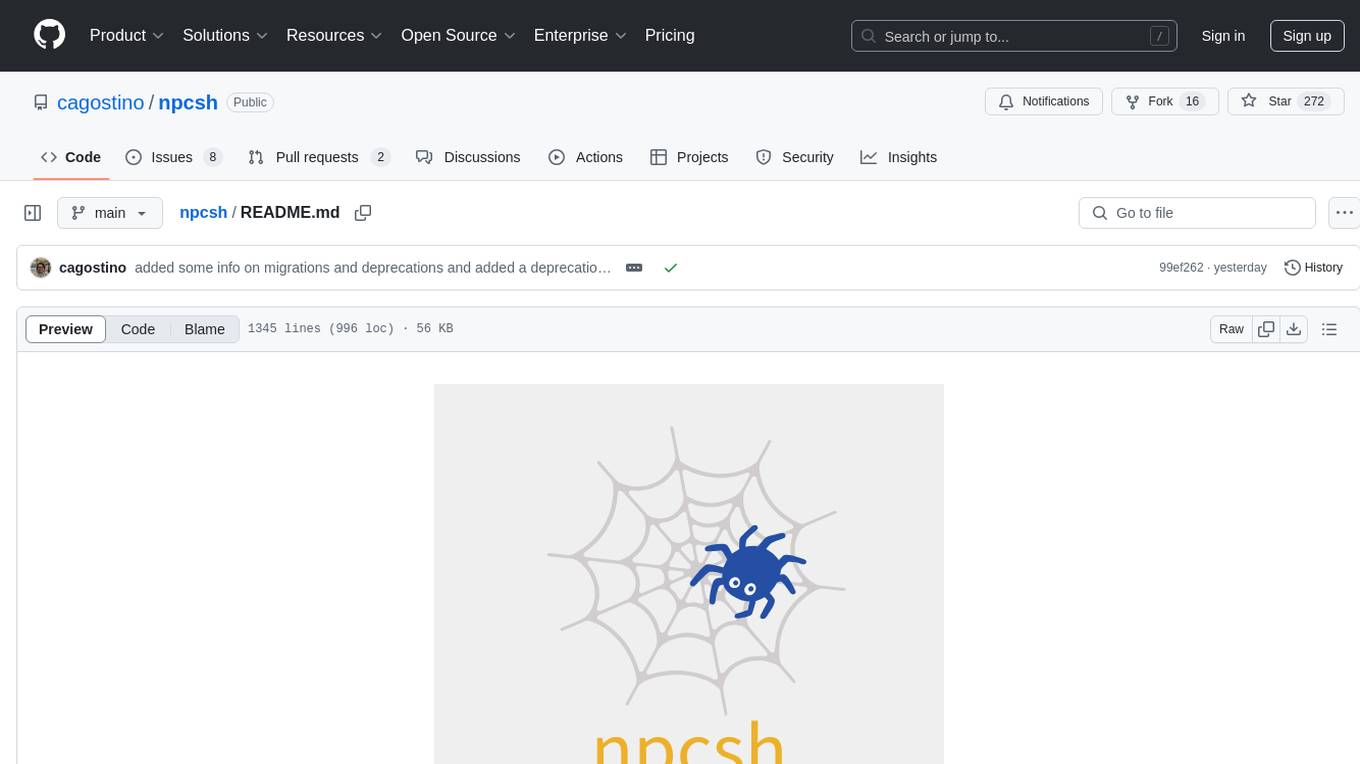
npcsh
`npcsh` is a python-based command-line tool designed to integrate Large Language Models (LLMs) and Agents into one's daily workflow by making them available and easily configurable through the command line shell. It leverages the power of LLMs to understand natural language commands and questions, execute tasks, answer queries, and provide relevant information from local files and the web. Users can also build their own tools and call them like macros from the shell. `npcsh` allows users to take advantage of agents (i.e. NPCs) through a managed system, tailoring NPCs to specific tasks and workflows. The tool is extensible with Python, providing useful functions for interacting with LLMs, including explicit coverage for popular providers like ollama, anthropic, openai, gemini, deepseek, and openai-like providers. Users can set up a flask server to expose their NPC team for use as a backend service, run SQL models defined in their project, execute assembly lines, and verify the integrity of their NPC team's interrelations. Users can execute bash commands directly, use favorite command-line tools like VIM, Emacs, ipython, sqlite3, git, pipe the output of these commands to LLMs, or pass LLM results to bash commands.
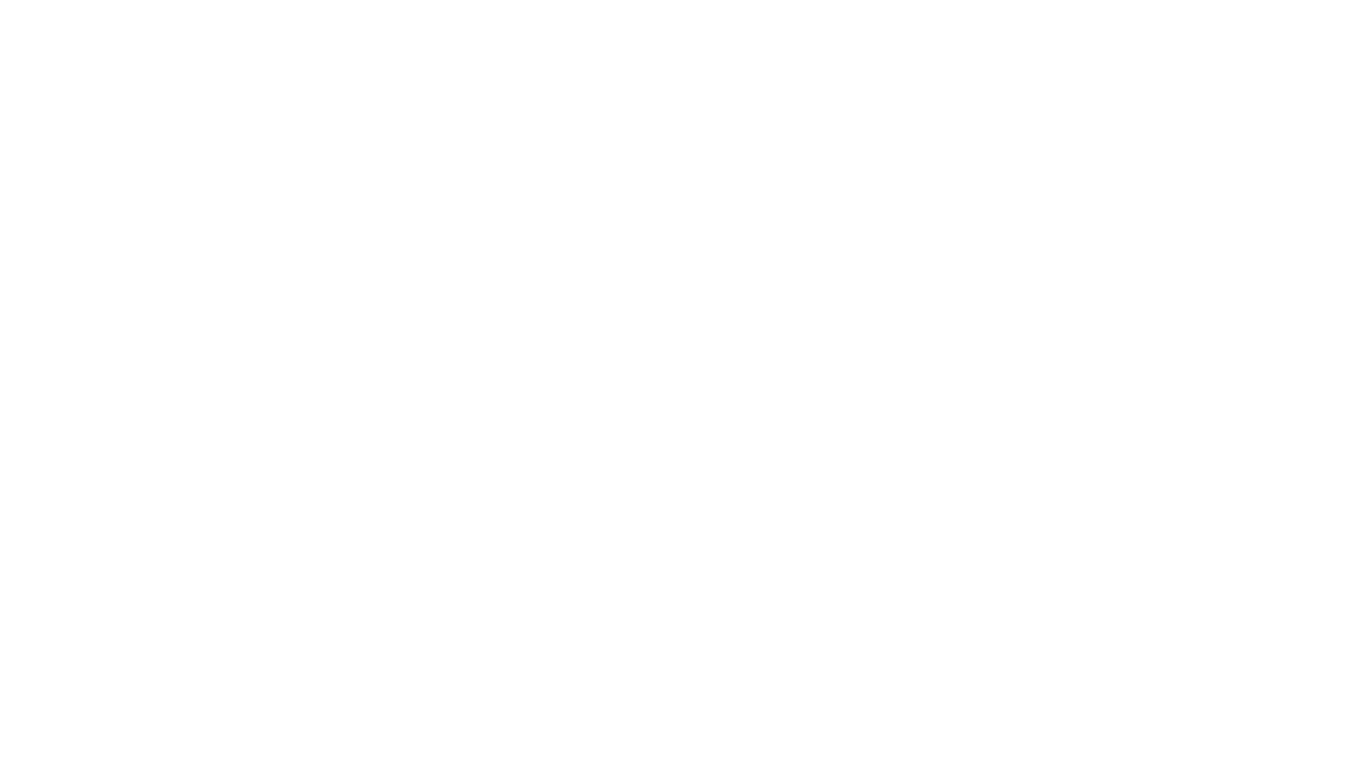
rag-experiment-accelerator
The RAG Experiment Accelerator is a versatile tool that helps you conduct experiments and evaluations using Azure AI Search and RAG pattern. It offers a rich set of features, including experiment setup, integration with Azure AI Search, Azure Machine Learning, MLFlow, and Azure OpenAI, multiple document chunking strategies, query generation, multiple search types, sub-querying, re-ranking, metrics and evaluation, report generation, and multi-lingual support. The tool is designed to make it easier and faster to run experiments and evaluations of search queries and quality of response from OpenAI, and is useful for researchers, data scientists, and developers who want to test the performance of different search and OpenAI related hyperparameters, compare the effectiveness of various search strategies, fine-tune and optimize parameters, find the best combination of hyperparameters, and generate detailed reports and visualizations from experiment results.
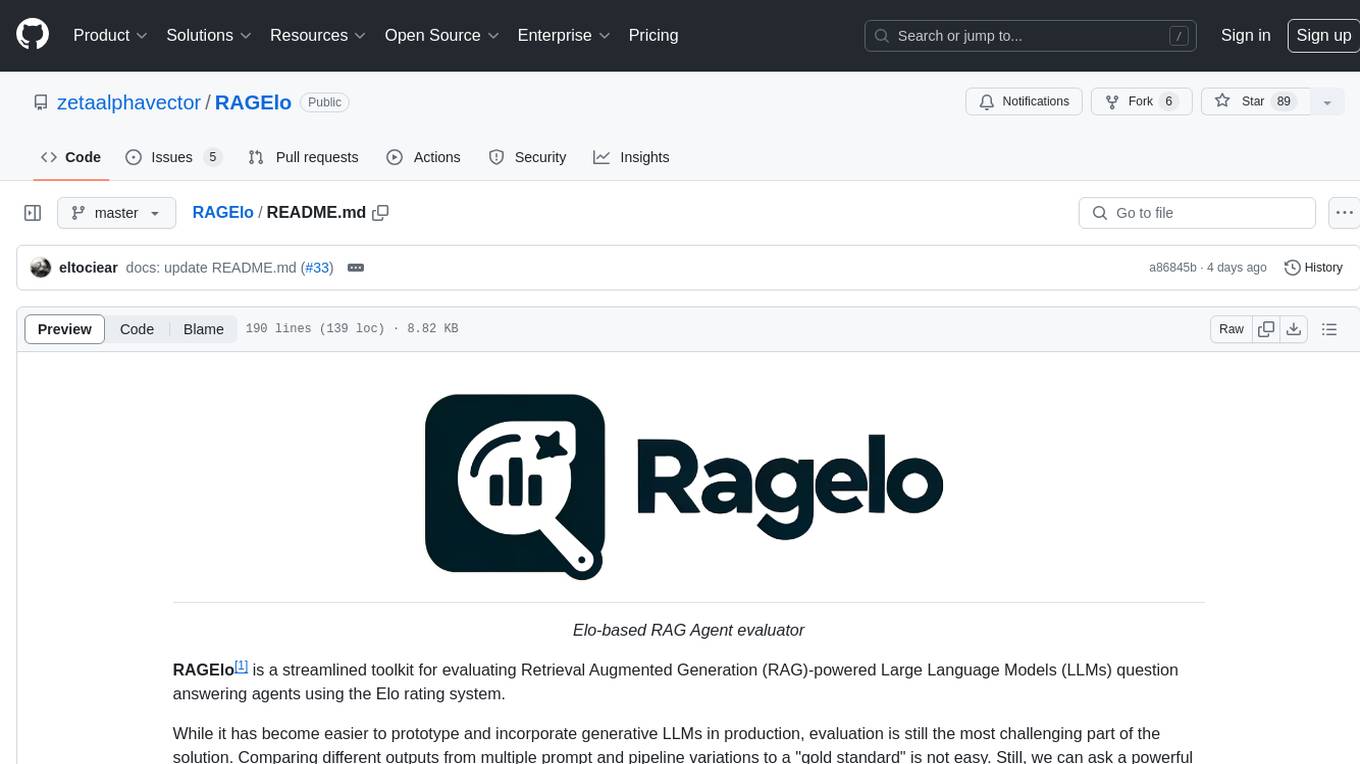
RAGElo
RAGElo is a streamlined toolkit for evaluating Retrieval Augmented Generation (RAG)-powered Large Language Models (LLMs) question answering agents using the Elo rating system. It simplifies the process of comparing different outputs from multiple prompt and pipeline variations to a 'gold standard' by allowing a powerful LLM to judge between pairs of answers and questions. RAGElo conducts tournament-style Elo ranking of LLM outputs, providing insights into the effectiveness of different settings.
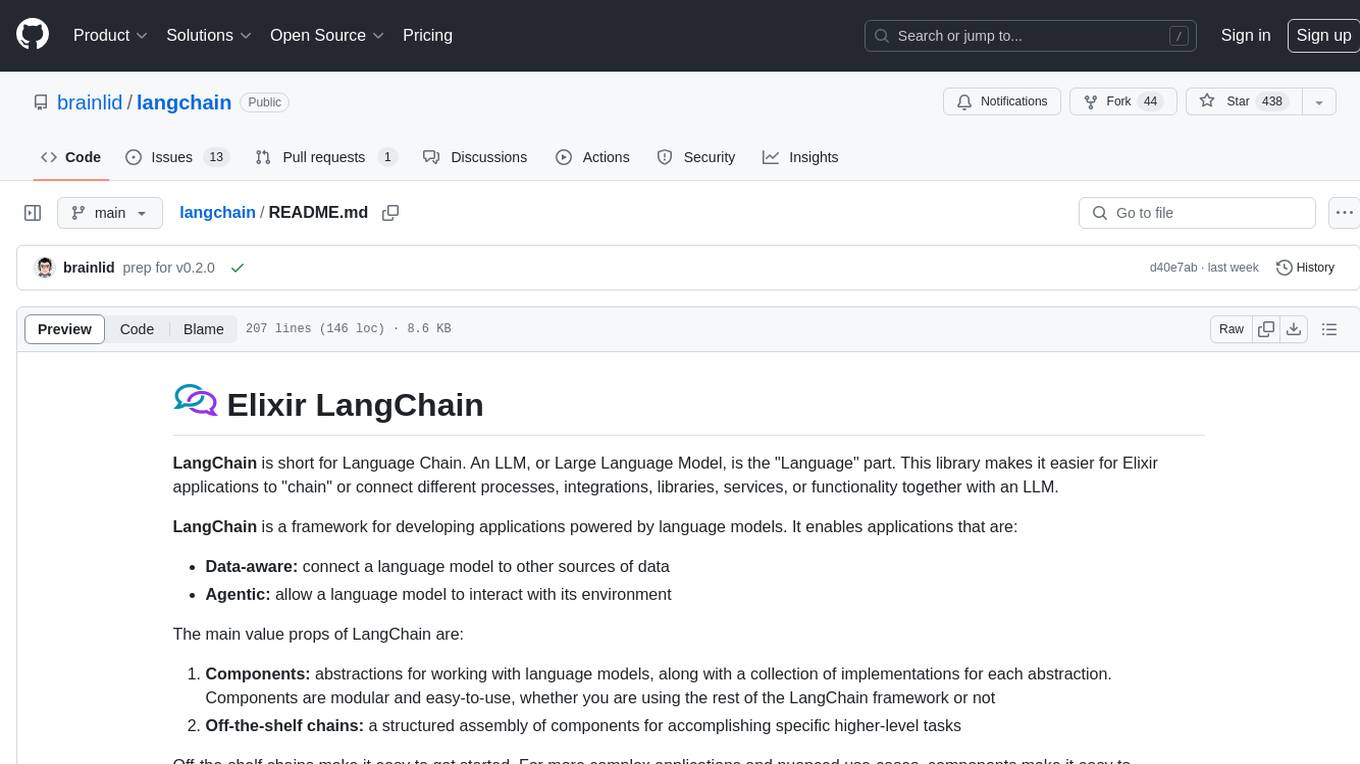
langchain
LangChain is a framework for developing Elixir applications powered by language models. It enables applications to connect language models to other data sources and interact with the environment. The library provides components for working with language models and off-the-shelf chains for specific tasks. It aims to assist in building applications that combine large language models with other sources of computation or knowledge. LangChain is written in Elixir and is not aimed for parity with the JavaScript and Python versions due to differences in programming paradigms and design choices. The library is designed to make it easy to integrate language models into applications and expose features, data, and functionality to the models.
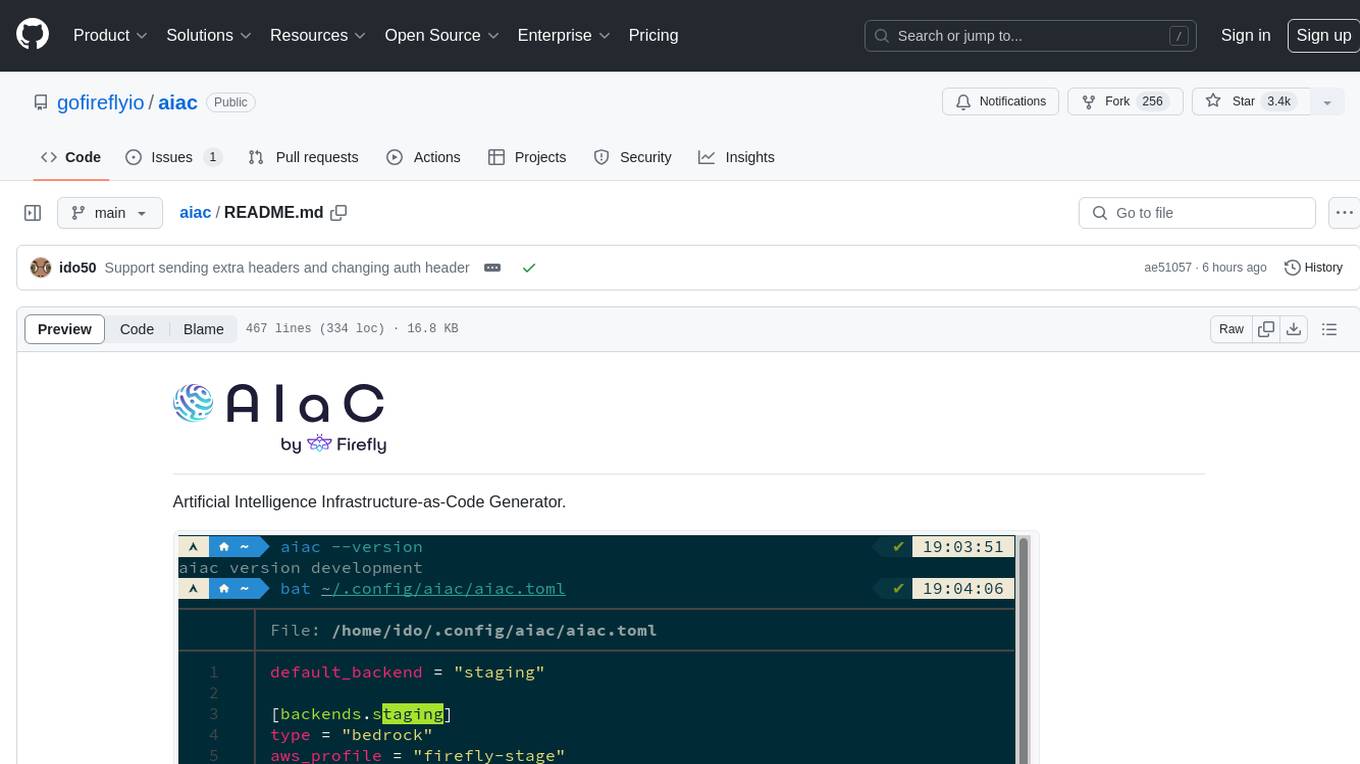
aiac
AIAC is a library and command line tool to generate Infrastructure as Code (IaC) templates, configurations, utilities, queries, and more via LLM providers such as OpenAI, Amazon Bedrock, and Ollama. Users can define multiple 'backends' targeting different LLM providers and environments using a simple configuration file. The tool allows users to ask a model to generate templates for different scenarios and composes an appropriate request to the selected provider, storing the resulting code to a file and/or printing it to standard output.
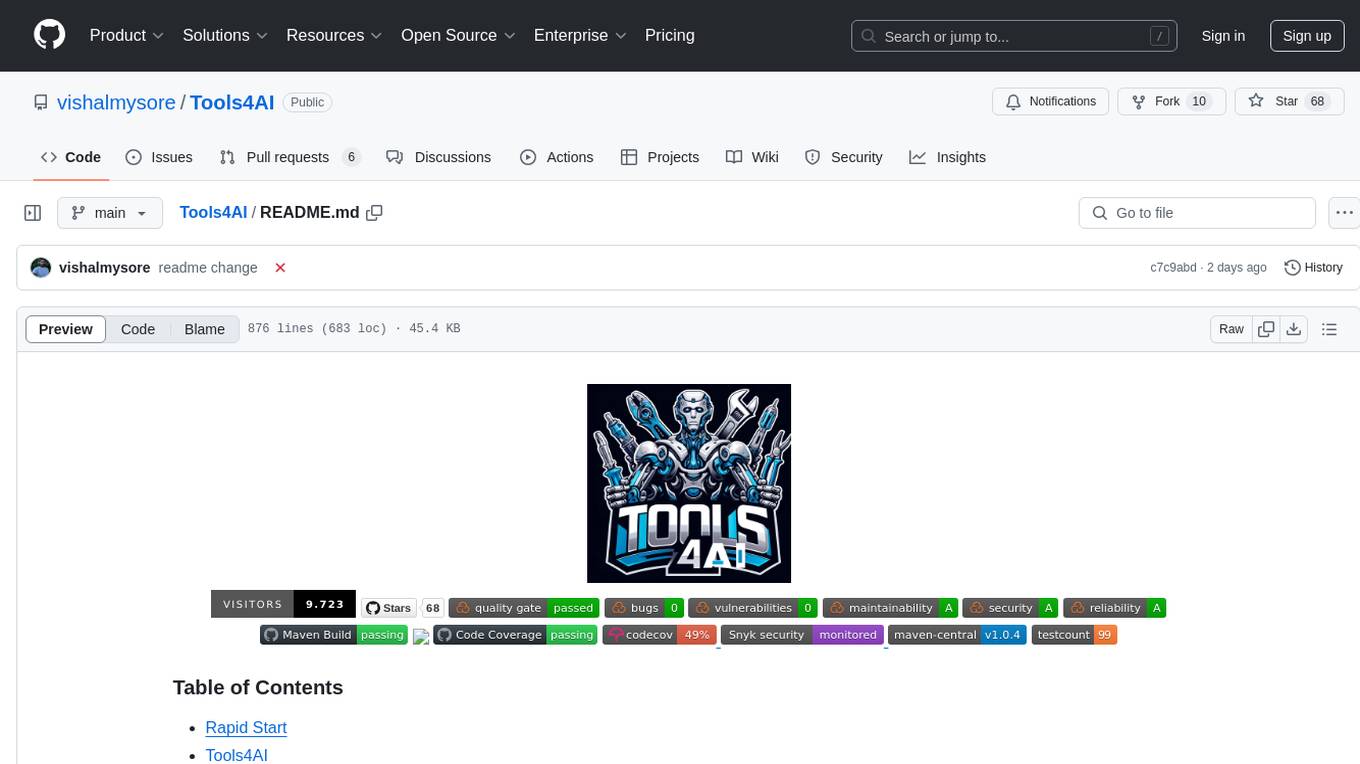
Tools4AI
Tools4AI is a Java-based Agentic Framework for building AI agents to integrate with enterprise Java applications. It enables the conversion of natural language prompts into actionable behaviors, streamlining user interactions with complex systems. By leveraging AI capabilities, it enhances productivity and innovation across diverse applications. The framework allows for seamless integration of AI with various systems, such as customer service applications, to interpret user requests, trigger actions, and streamline workflows. Prompt prediction anticipates user actions based on input prompts, enhancing user experience by proactively suggesting relevant actions or services based on context.
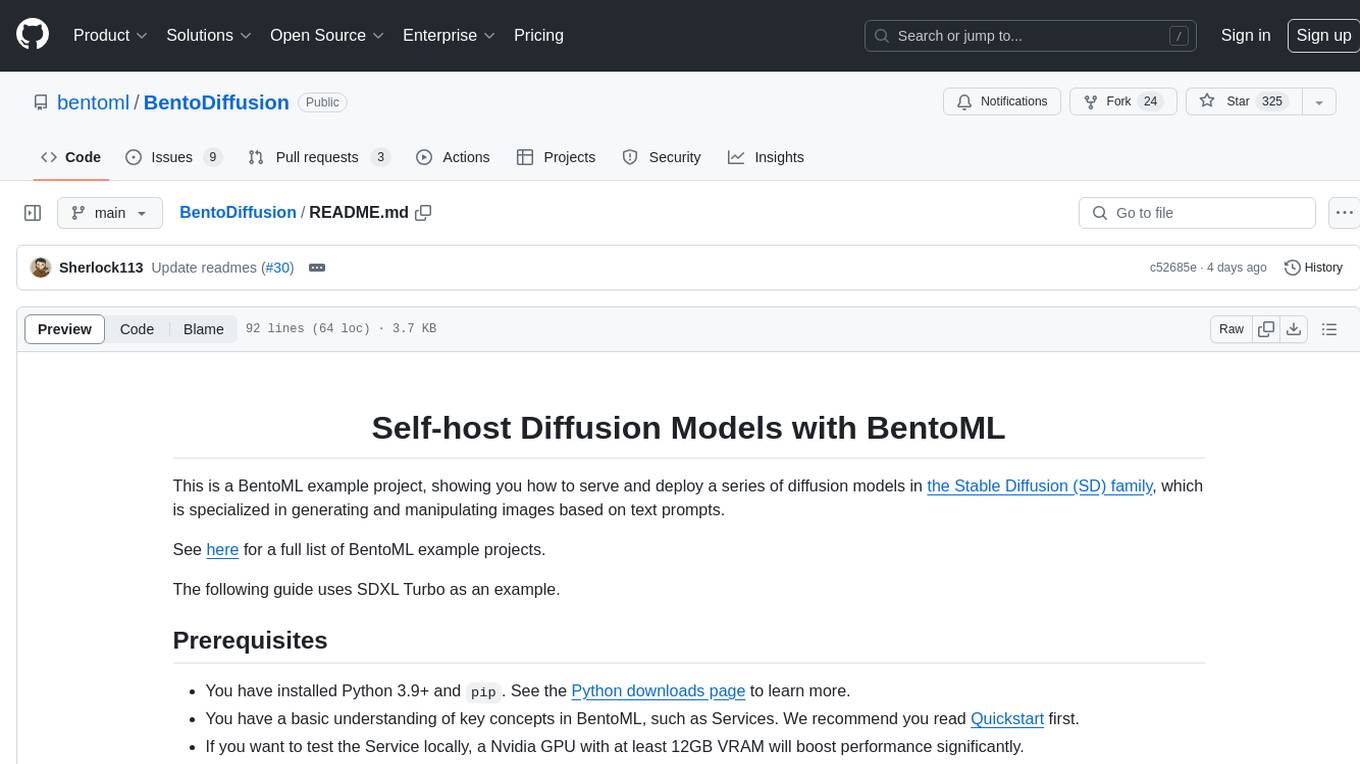
BentoDiffusion
BentoDiffusion is a BentoML example project that demonstrates how to serve and deploy diffusion models in the Stable Diffusion (SD) family. These models are specialized in generating and manipulating images based on text prompts. The project provides a guide on using SDXL Turbo as an example, along with instructions on prerequisites, installing dependencies, running the BentoML service, and deploying to BentoCloud. Users can interact with the deployed service using Swagger UI or other methods. Additionally, the project offers the option to choose from various diffusion models available in the repository for deployment.
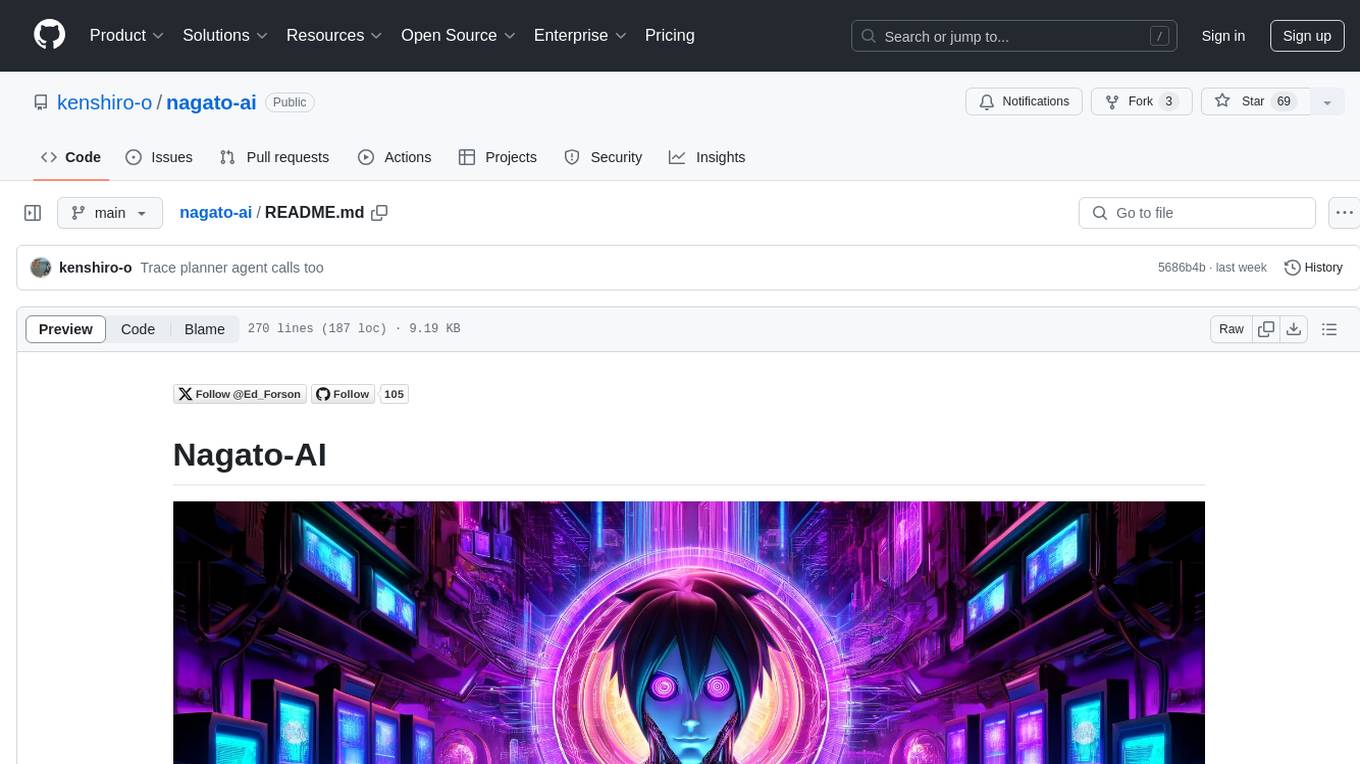
nagato-ai
Nagato-AI is an intuitive AI Agent library that supports multiple LLMs including OpenAI's GPT, Anthropic's Claude, Google's Gemini, and Groq LLMs. Users can create agents from these models and combine them to build an effective AI Agent system. The library is named after the powerful ninja Nagato from the anime Naruto, who can control multiple bodies with different abilities. Nagato-AI acts as a linchpin to summon and coordinate AI Agents for specific missions. It provides flexibility in programming and supports tools like Coordinator, Researcher, Critic agents, and HumanConfirmInputTool.
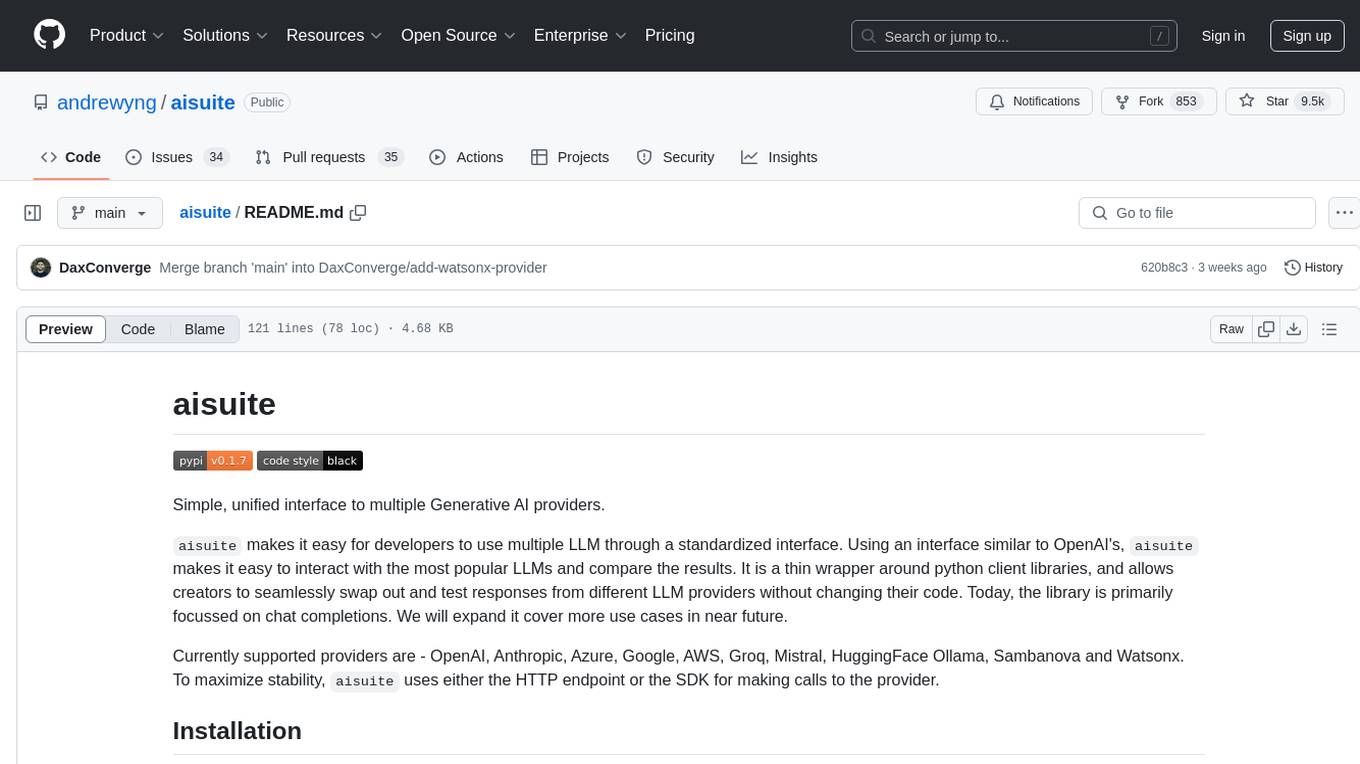
aisuite
Aisuite is a simple, unified interface to multiple Generative AI providers. It allows developers to easily interact with various Language Model (LLM) providers like OpenAI, Anthropic, Azure, Google, AWS, and more through a standardized interface. The library focuses on chat completions and provides a thin wrapper around python client libraries, enabling creators to test responses from different LLM providers without changing their code. Aisuite maximizes stability by using HTTP endpoints or SDKs for making calls to the providers. Users can install the base package or specific provider packages, set up API keys, and utilize the library to generate chat completion responses from different models.
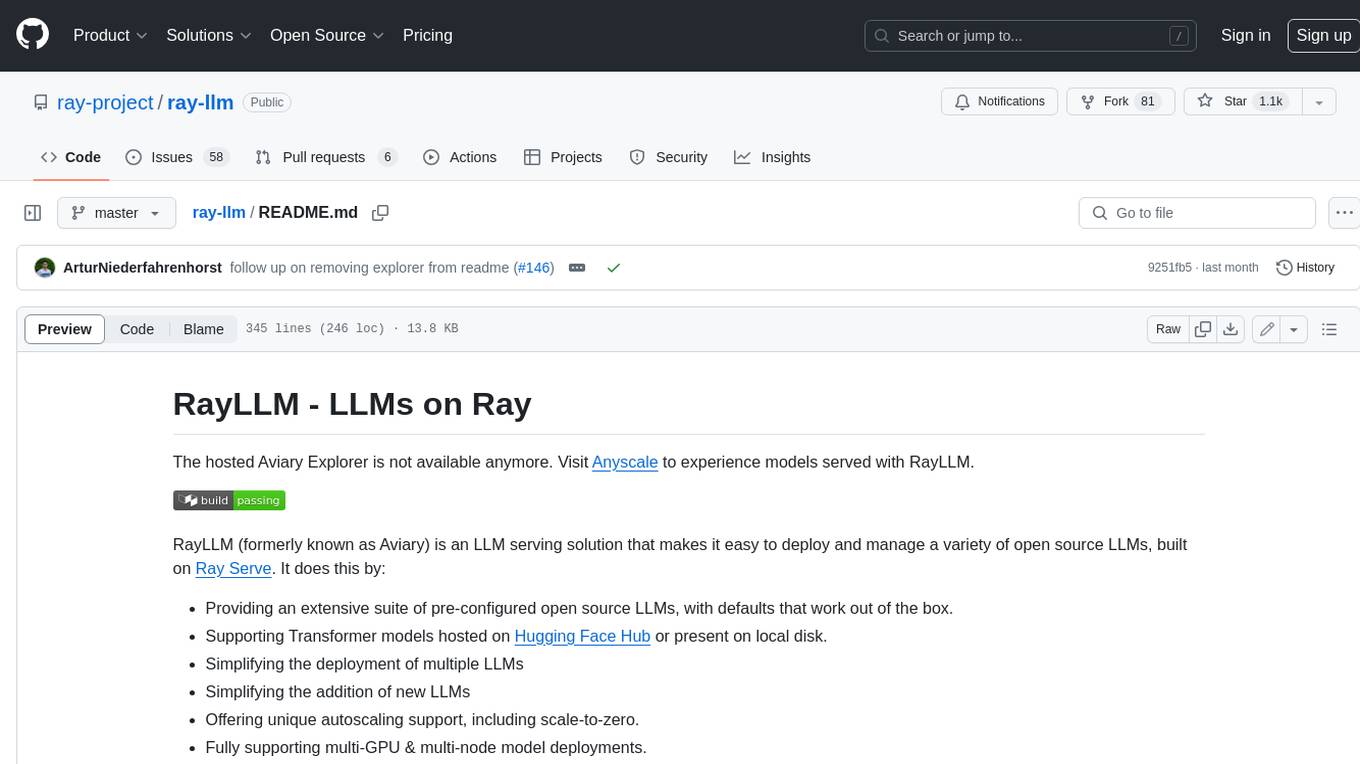
ray-llm
RayLLM (formerly known as Aviary) is an LLM serving solution that makes it easy to deploy and manage a variety of open source LLMs, built on Ray Serve. It provides an extensive suite of pre-configured open source LLMs, with defaults that work out of the box. RayLLM supports Transformer models hosted on Hugging Face Hub or present on local disk. It simplifies the deployment of multiple LLMs, the addition of new LLMs, and offers unique autoscaling support, including scale-to-zero. RayLLM fully supports multi-GPU & multi-node model deployments and offers high performance features like continuous batching, quantization and streaming. It provides a REST API that is similar to OpenAI's to make it easy to migrate and cross test them. RayLLM supports multiple LLM backends out of the box, including vLLM and TensorRT-LLM.
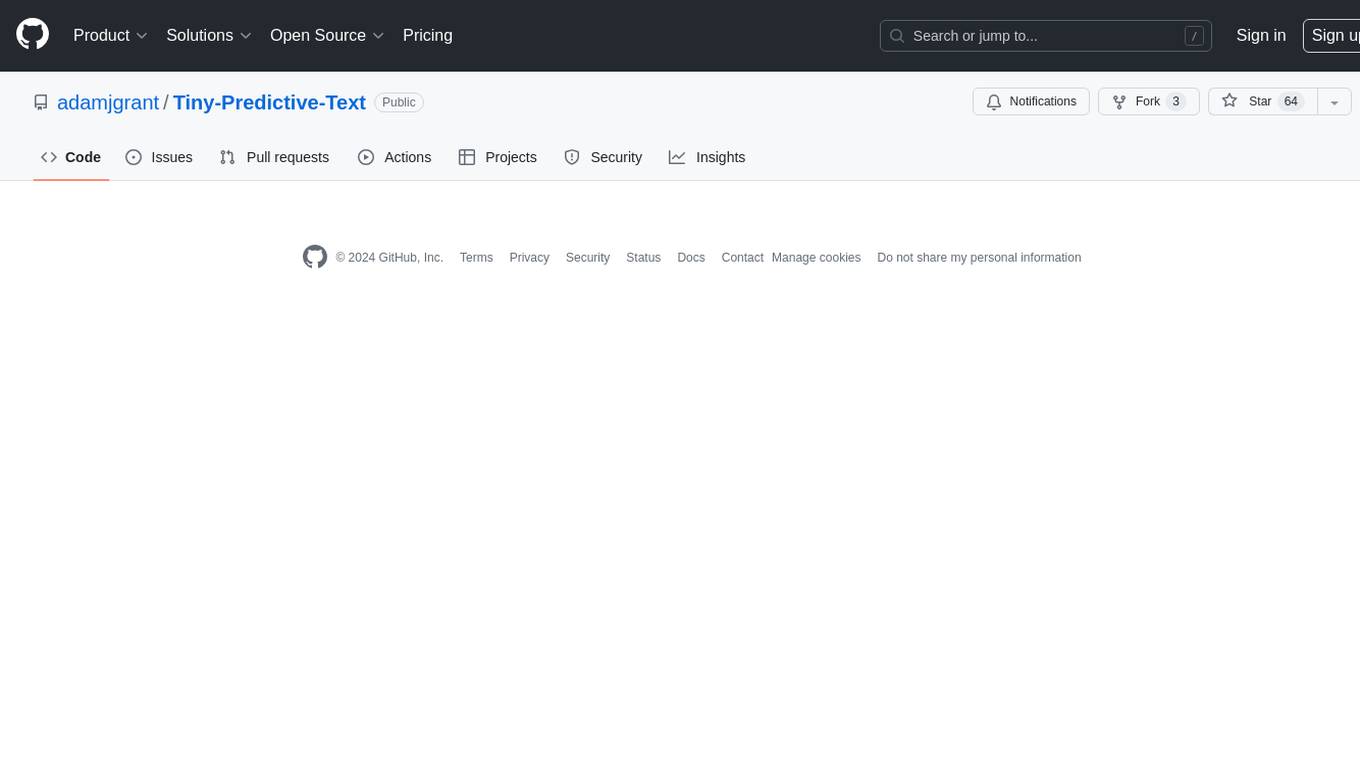
Tiny-Predictive-Text
Tiny-Predictive-Text is a demonstration of predictive text without an LLM, using permy.link. It provides a detailed description of the tool, including its features, benefits, and how to use it. The tool is suitable for a variety of jobs, including content writers, editors, and researchers. It can be used to perform a variety of tasks, such as generating text, completing sentences, and correcting errors.
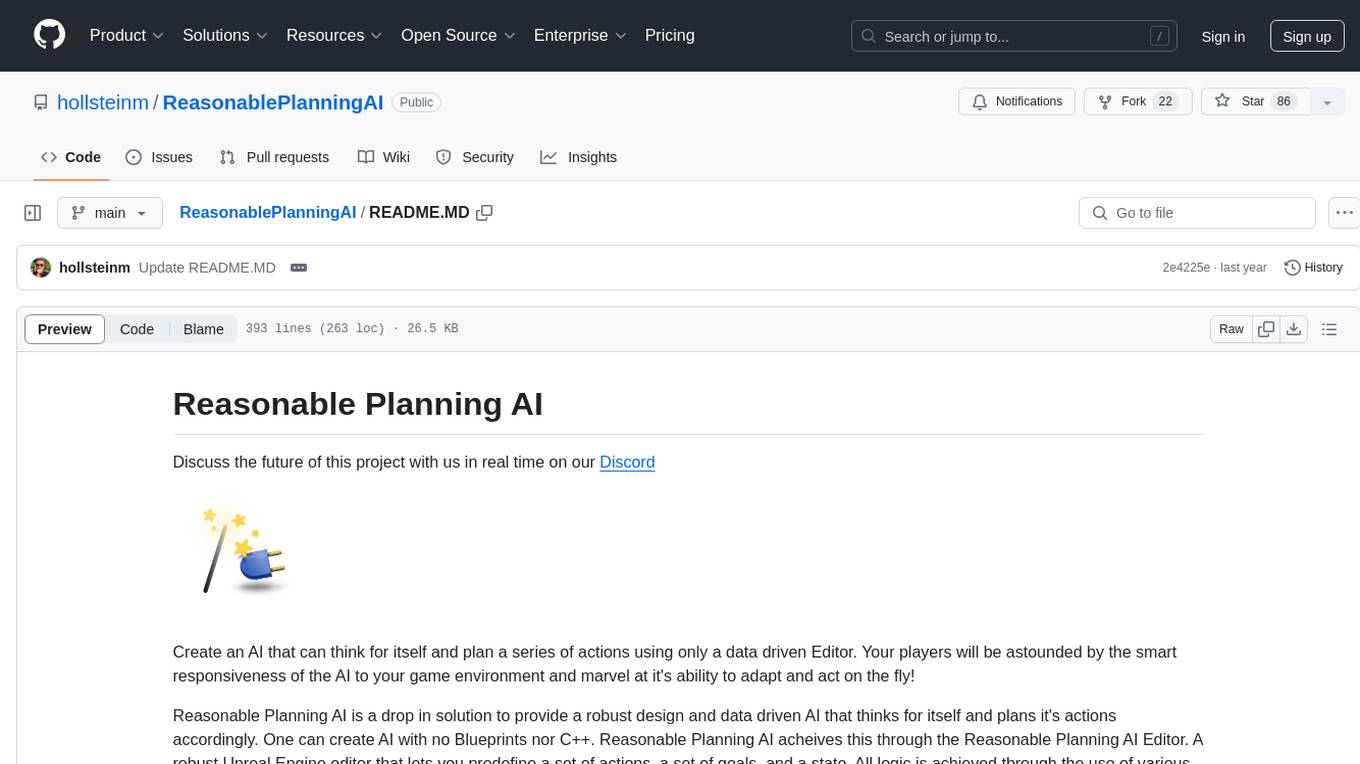
ReasonablePlanningAI
Reasonable Planning AI is a robust design and data-driven AI solution for game developers. It provides an AI Editor that allows creating AI without Blueprints or C++. The AI can think for itself, plan actions, adapt to the game environment, and act dynamically. It consists of Core components like RpaiGoalBase, RpaiActionBase, RpaiPlannerBase, RpaiReasonerBase, and RpaiBrainComponent, as well as Composer components for easier integration by Game Designers. The tool is extensible, cross-compatible with Behavior Trees, and offers debugging features like visual logging and heuristics testing. It follows a simple path of execution and supports versioning for stability and compatibility with Unreal Engine versions.
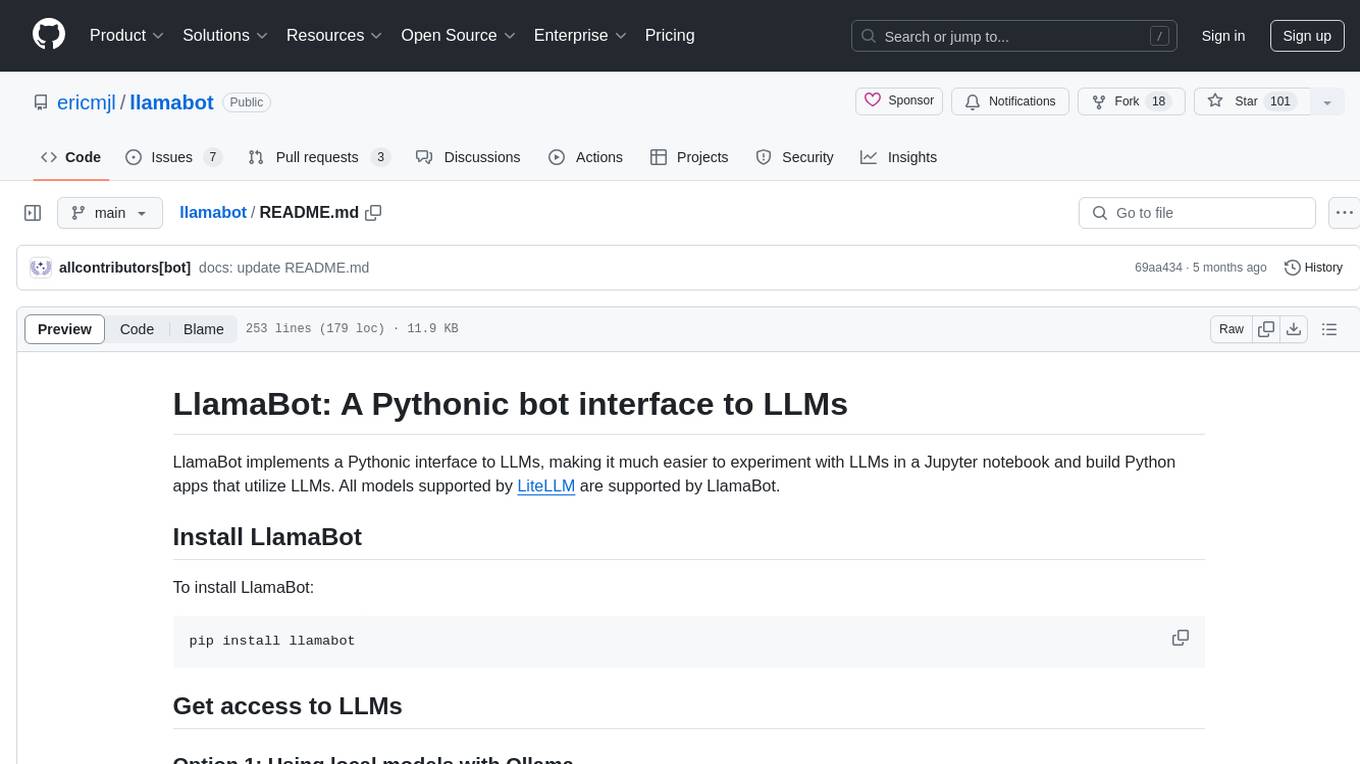
llamabot
LlamaBot is a Pythonic bot interface to Large Language Models (LLMs), providing an easy way to experiment with LLMs in Jupyter notebooks and build Python apps utilizing LLMs. It supports all models available in LiteLLM. Users can access LLMs either through local models with Ollama or by using API providers like OpenAI and Mistral. LlamaBot offers different bot interfaces like SimpleBot, ChatBot, QueryBot, and ImageBot for various tasks such as rephrasing text, maintaining chat history, querying documents, and generating images. The tool also includes CLI demos showcasing its capabilities and supports contributions for new features and bug reports from the community.
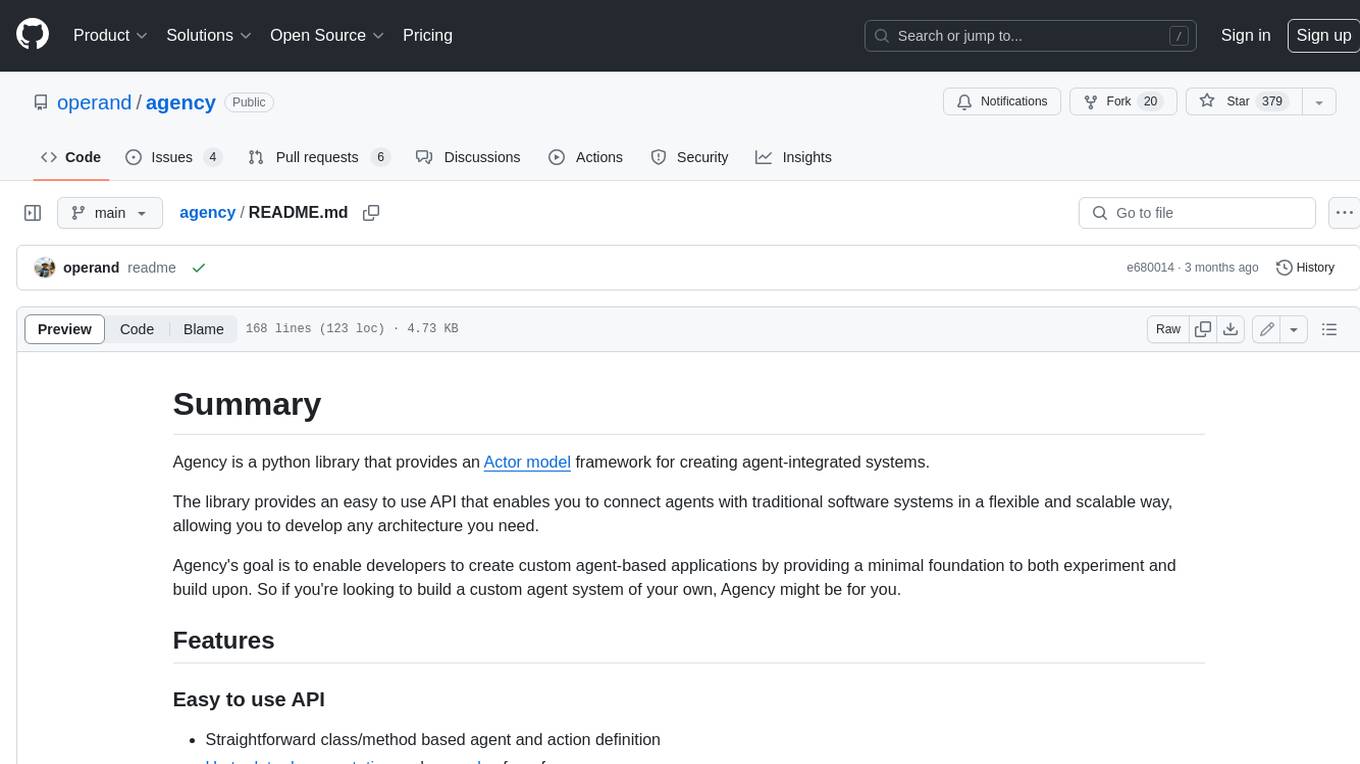
agency
Agency is a python library that provides an Actor model framework for creating agent-integrated systems. It offers an easy-to-use API for connecting agents with traditional software systems, enabling flexible and scalable architectures. Agency aims to empower developers in creating custom agent-based applications by providing a foundation for experimentation and development. Key features include an intuitive API, performance and scalability through multiprocessing and AMQP support, observability and control with action and lifecycle callbacks, access policies, and detailed logging. The library also includes a demo application with multiple agent examples, OpenAI agent examples, HuggingFace transformers agent example, operating system access, Gradio UI, and Docker configuration for reference and development.
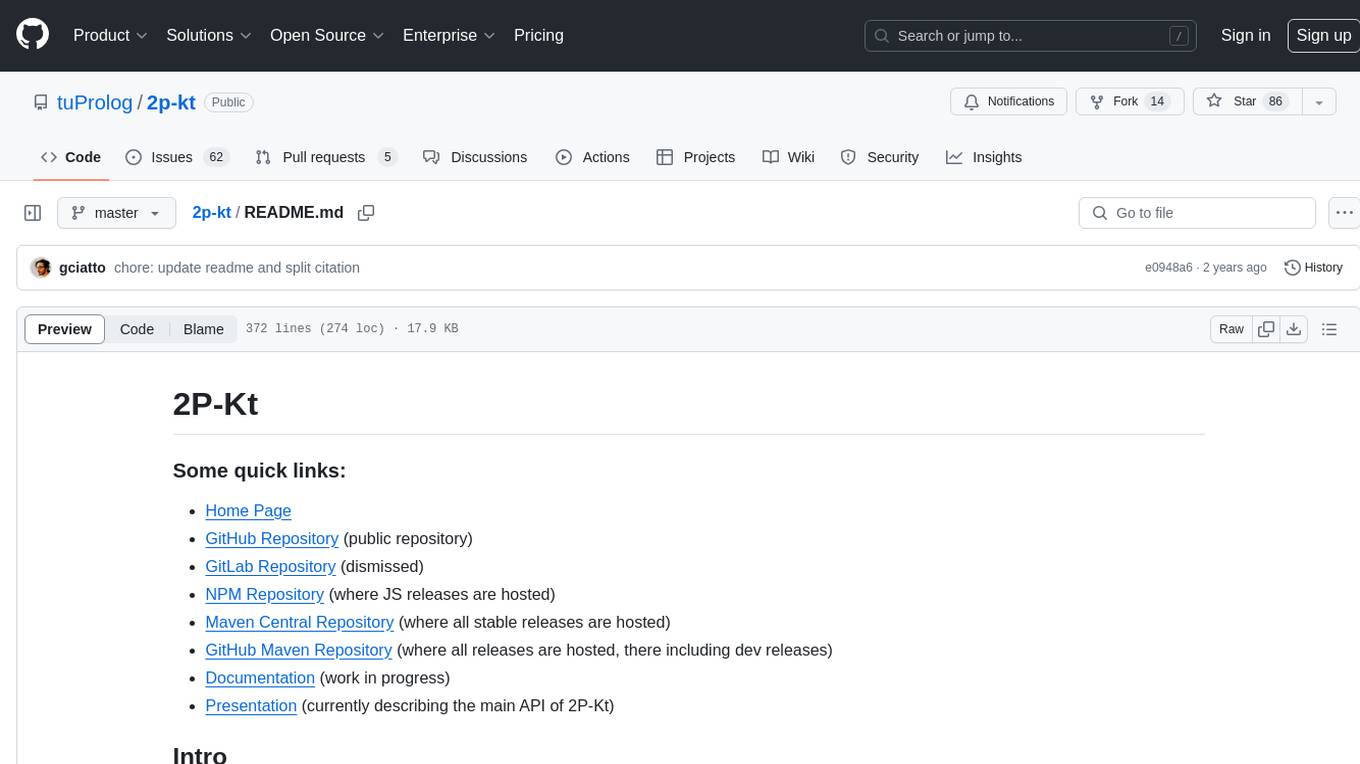
2p-kt
2P-Kt is a Kotlin-based and multi-platform reboot of tuProlog (2P), a multi-paradigm logic programming framework written in Java. It consists of an open ecosystem for Symbolic Artificial Intelligence (AI) with modules supporting logic terms, unification, indexing, resolution of logic queries, probabilistic logic programming, binary decision diagrams, OR-concurrent resolution, DSL for logic programming, parsing modules, serialisation modules, command-line interface, and graphical user interface. The tool is designed to support knowledge representation and automatic reasoning through logic programming in an extensible and flexible way, encouraging extensions towards other symbolic AI systems than Prolog. It is a pure, multi-platform Kotlin project supporting JVM, JS, Android, and Native platforms, with a lightweight library leveraging the Kotlin common library.
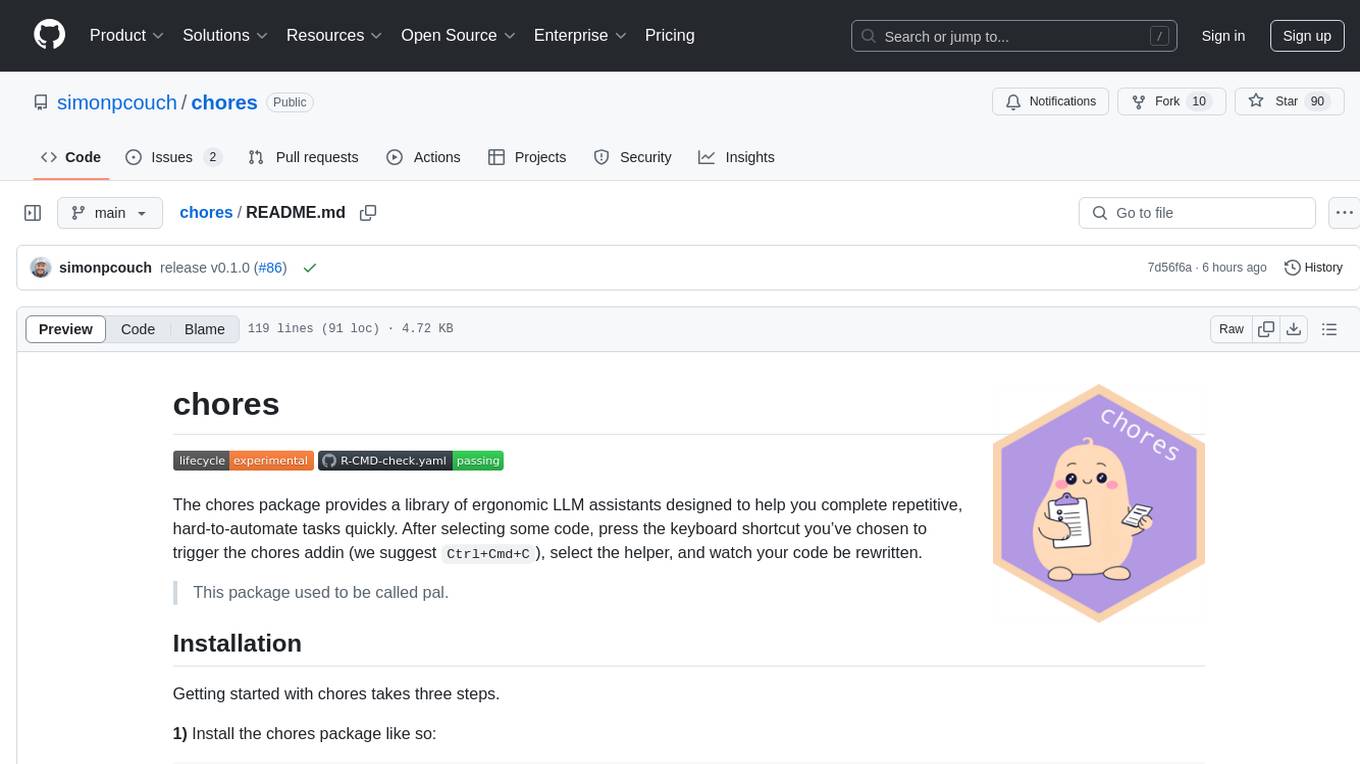
chores
The chores package provides a library of ergonomic LLM assistants designed to help users complete repetitive, hard-to-automate tasks quickly. Users can select code, trigger the chores addin, choose a helper, and watch their code be rewritten. The package offers chore helpers for tasks like converting to cli, testthat, and documenting functions with roxygen. Users can also create their own chore helpers by providing instructions in a markdown file. The cost of using helpers depends on the length of the prompt and the model chosen.
For similar tasks
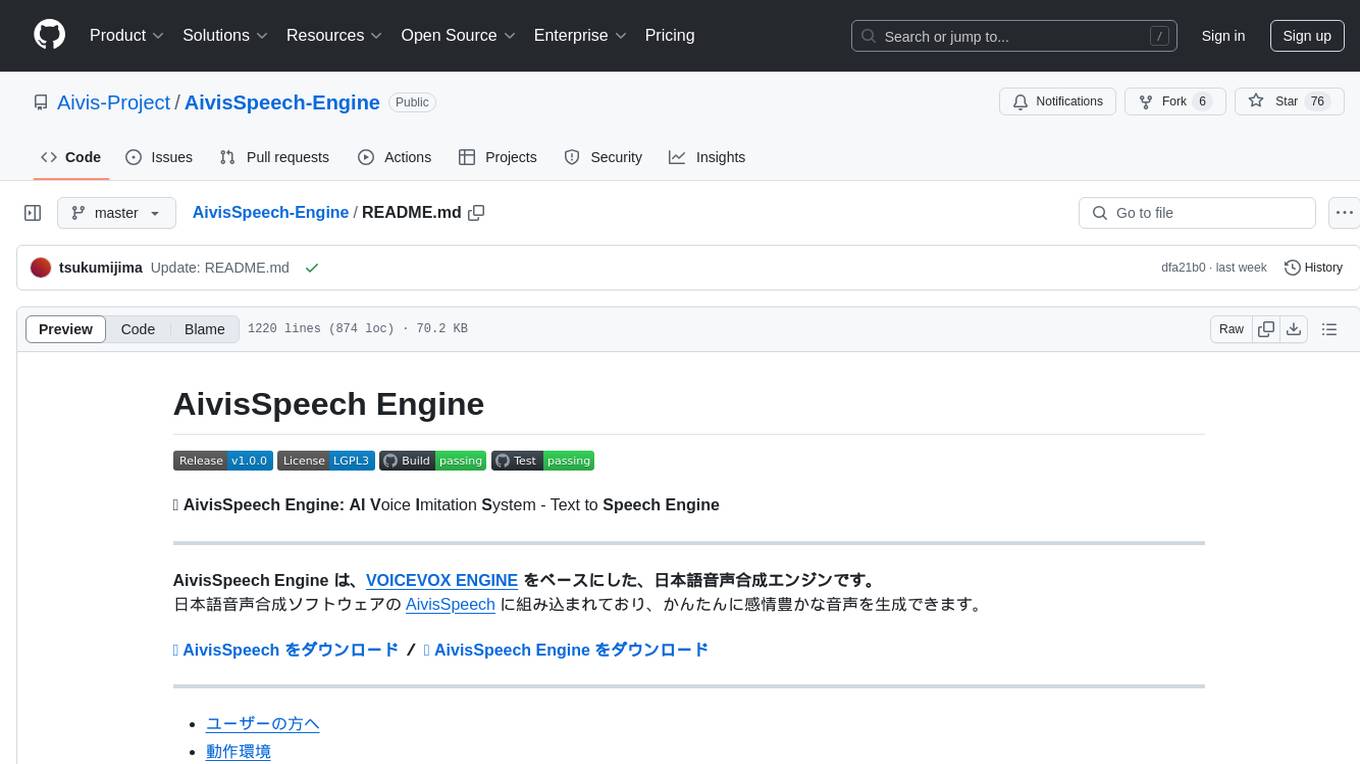
AivisSpeech-Engine
AivisSpeech-Engine is a powerful open-source tool for speech recognition and synthesis. It provides state-of-the-art algorithms for converting speech to text and text to speech. The tool is designed to be user-friendly and customizable, allowing developers to easily integrate speech capabilities into their applications. With AivisSpeech-Engine, users can transcribe audio recordings, create voice-controlled interfaces, and generate natural-sounding speech output. Whether you are building a virtual assistant, developing a speech-to-text application, or experimenting with voice technology, AivisSpeech-Engine offers a comprehensive solution for all your speech processing needs.
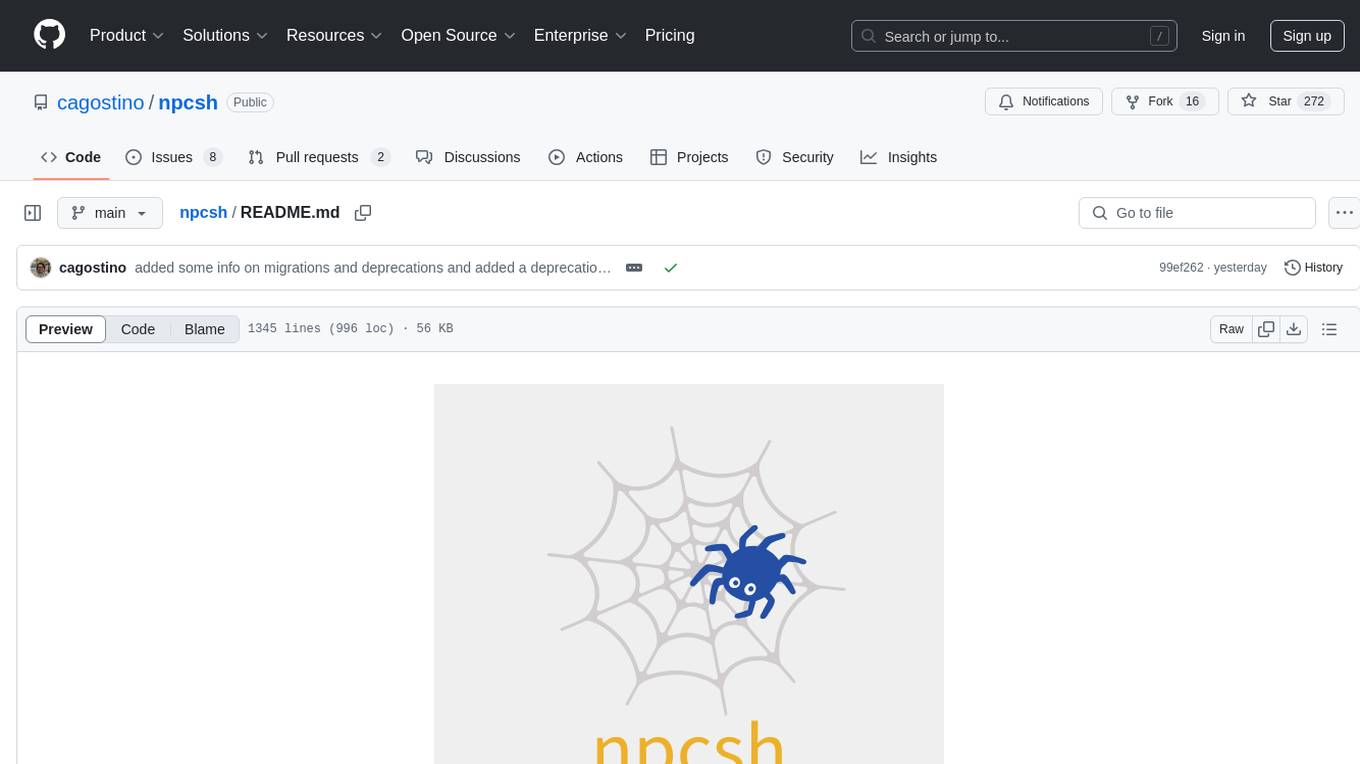
npcsh
`npcsh` is a python-based command-line tool designed to integrate Large Language Models (LLMs) and Agents into one's daily workflow by making them available and easily configurable through the command line shell. It leverages the power of LLMs to understand natural language commands and questions, execute tasks, answer queries, and provide relevant information from local files and the web. Users can also build their own tools and call them like macros from the shell. `npcsh` allows users to take advantage of agents (i.e. NPCs) through a managed system, tailoring NPCs to specific tasks and workflows. The tool is extensible with Python, providing useful functions for interacting with LLMs, including explicit coverage for popular providers like ollama, anthropic, openai, gemini, deepseek, and openai-like providers. Users can set up a flask server to expose their NPC team for use as a backend service, run SQL models defined in their project, execute assembly lines, and verify the integrity of their NPC team's interrelations. Users can execute bash commands directly, use favorite command-line tools like VIM, Emacs, ipython, sqlite3, git, pipe the output of these commands to LLMs, or pass LLM results to bash commands.
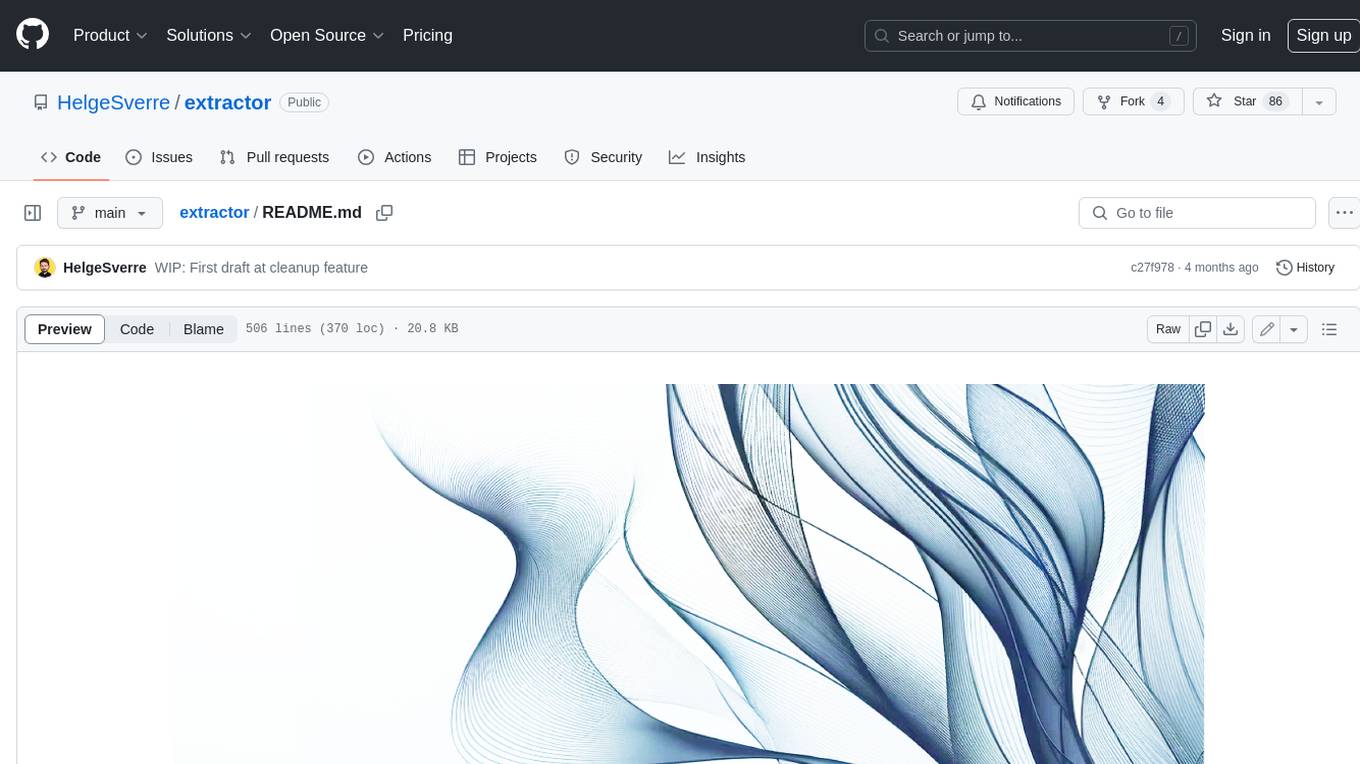
extractor
Extractor is an AI-powered data extraction library for Laravel that leverages OpenAI's capabilities to effortlessly extract structured data from various sources, including images, PDFs, and emails. It features a convenient wrapper around OpenAI Chat and Completion endpoints, supports multiple input formats, includes a flexible Field Extractor for arbitrary data extraction, and integrates with Textract for OCR functionality. Extractor utilizes JSON Mode from the latest GPT-3.5 and GPT-4 models, providing accurate and efficient data extraction.
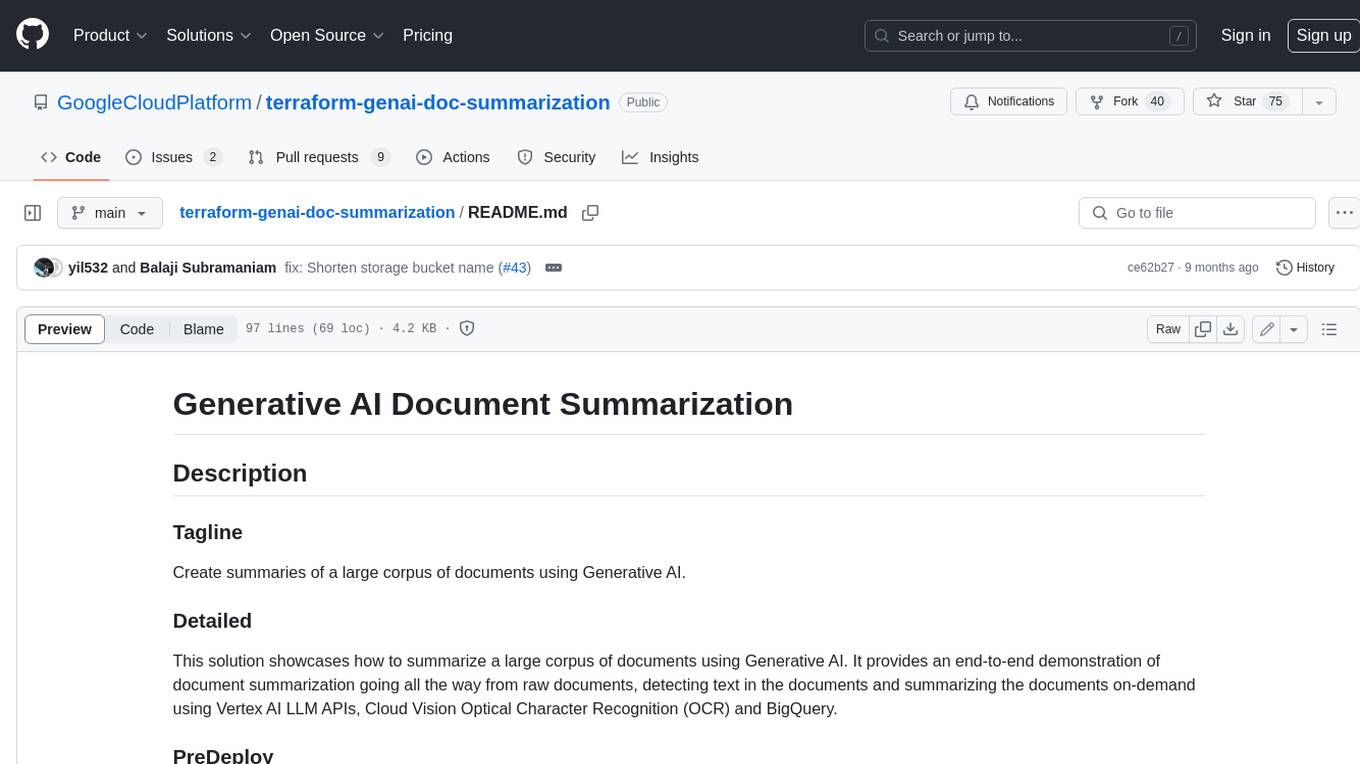
terraform-genai-doc-summarization
This solution showcases how to summarize a large corpus of documents using Generative AI. It provides an end-to-end demonstration of document summarization going all the way from raw documents, detecting text in the documents and summarizing the documents on-demand using Vertex AI LLM APIs, Cloud Vision Optical Character Recognition (OCR) and BigQuery.
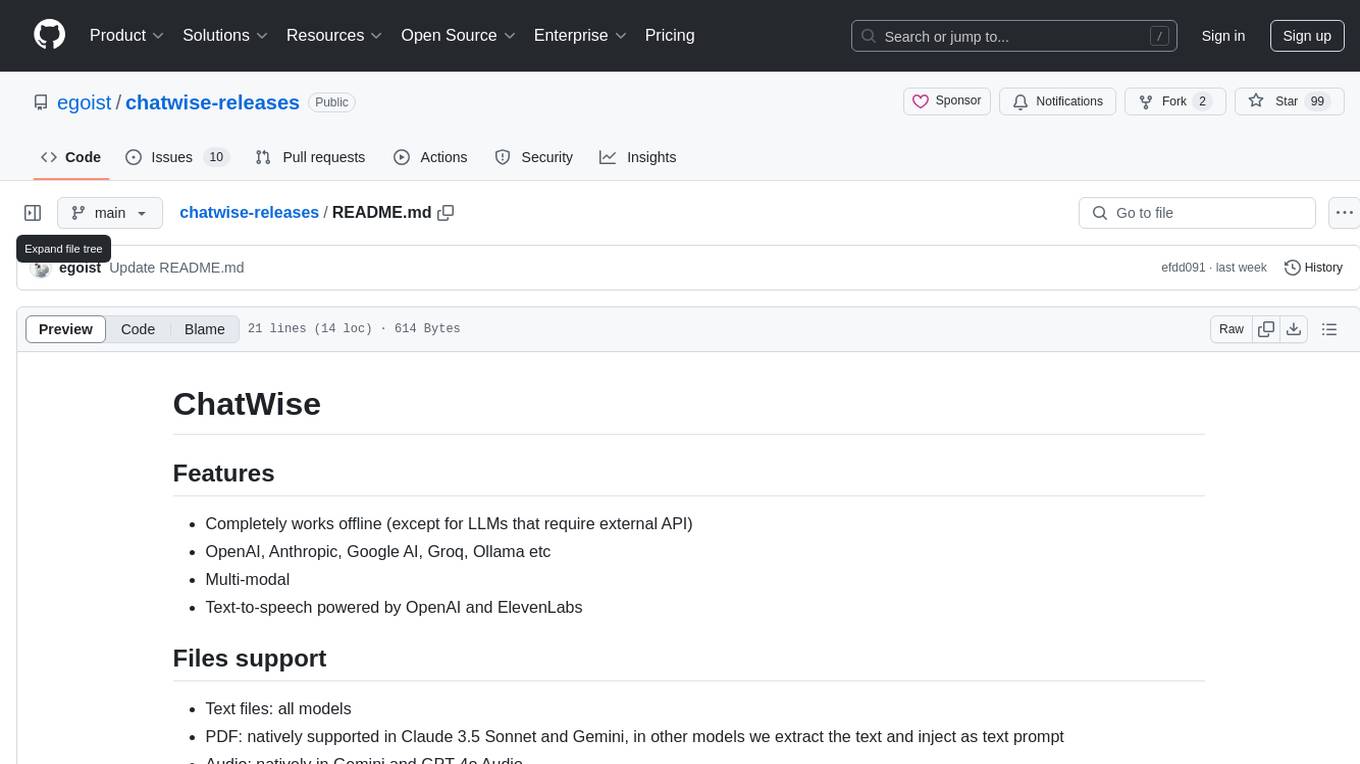
chatwise-releases
ChatWise is an offline tool that supports various AI models such as OpenAI, Anthropic, Google AI, Groq, and Ollama. It is multi-modal, allowing text-to-speech powered by OpenAI and ElevenLabs. The tool supports text files, PDFs, audio, and images across different models. ChatWise is currently available for macOS (Apple Silicon & Intel) with Windows support coming soon.
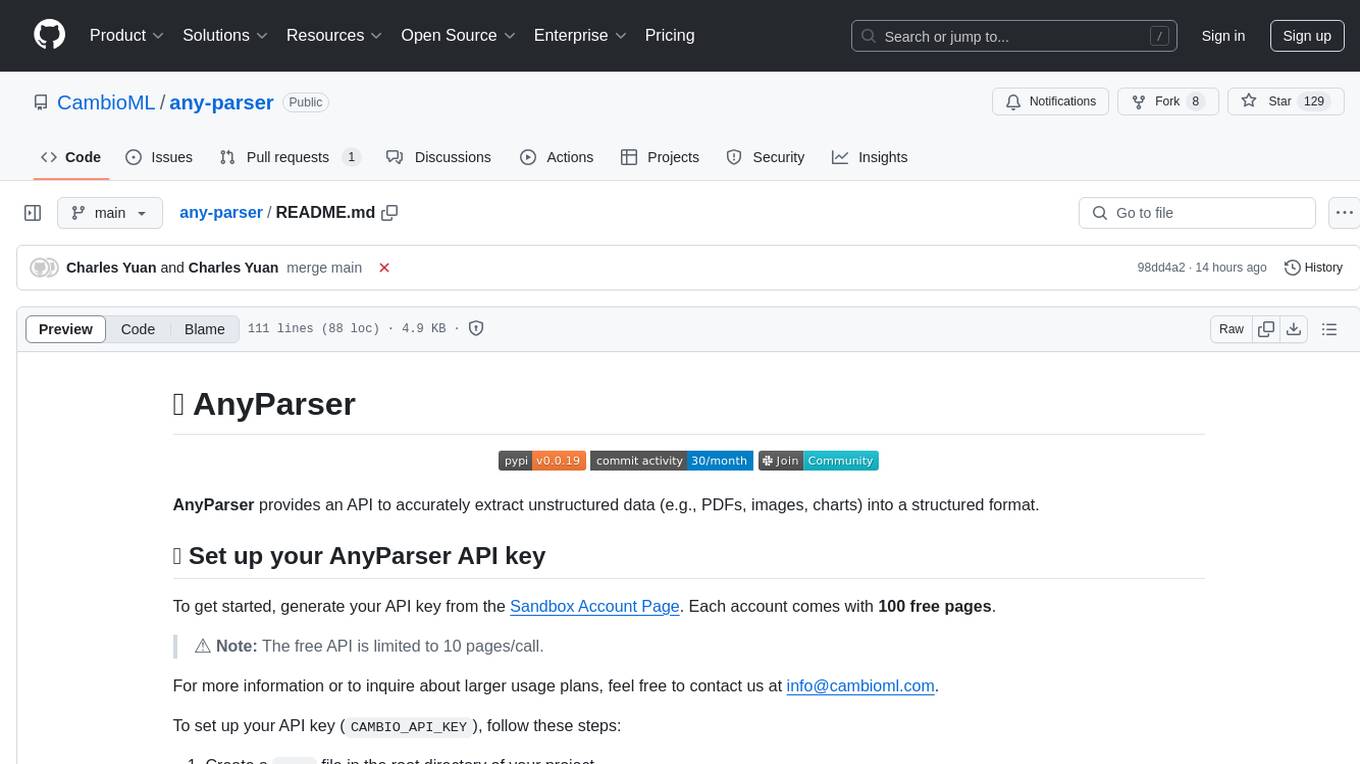
any-parser
AnyParser provides an API to accurately extract unstructured data (e.g., PDFs, images, charts) into a structured format. Users can set up their API key, run synchronous and asynchronous extractions, and perform batch extraction. The tool is useful for extracting text, numbers, and symbols from various sources like PDFs and images. It offers flexibility in processing data and provides immediate results for synchronous extraction while allowing users to fetch results later for asynchronous and batch extraction. AnyParser is designed to simplify data extraction tasks and enhance data processing efficiency.
For similar jobs
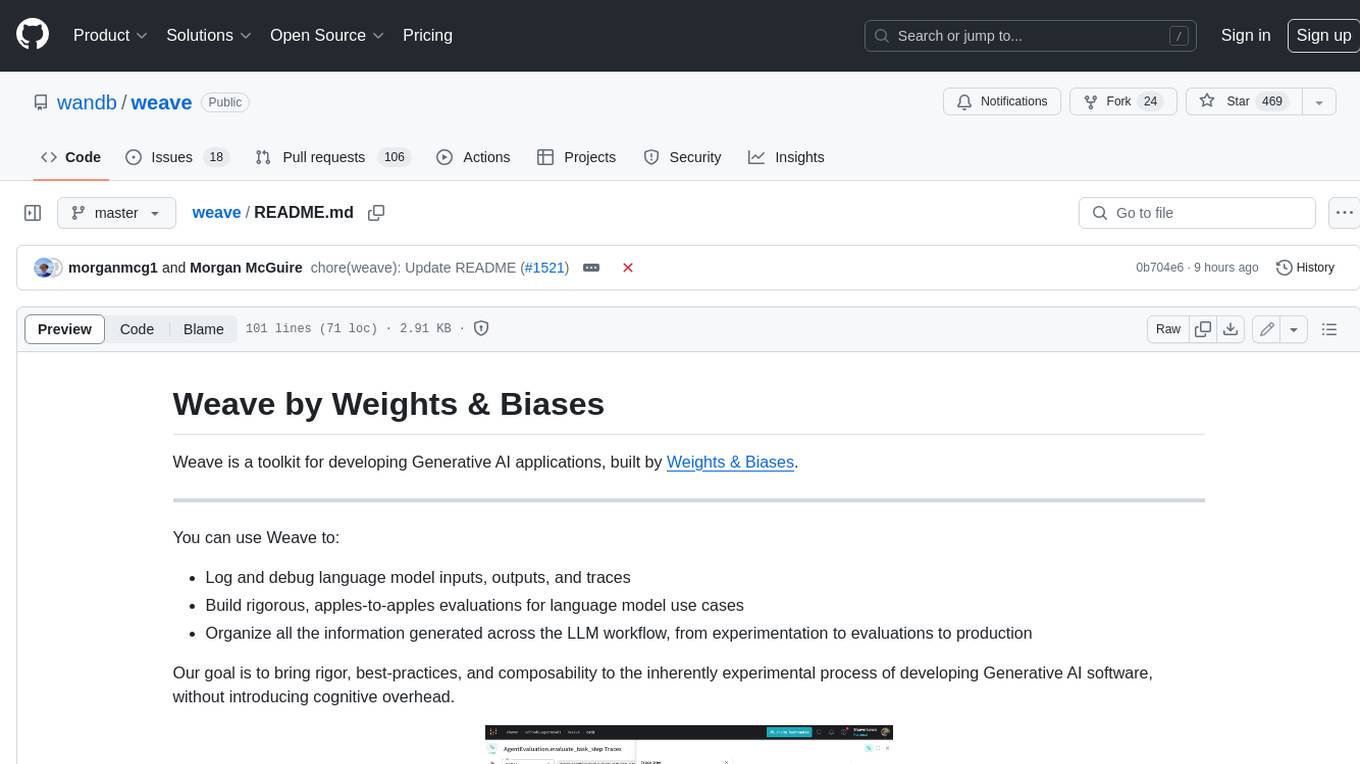
weave
Weave is a toolkit for developing Generative AI applications, built by Weights & Biases. With Weave, you can log and debug language model inputs, outputs, and traces; build rigorous, apples-to-apples evaluations for language model use cases; and organize all the information generated across the LLM workflow, from experimentation to evaluations to production. Weave aims to bring rigor, best-practices, and composability to the inherently experimental process of developing Generative AI software, without introducing cognitive overhead.
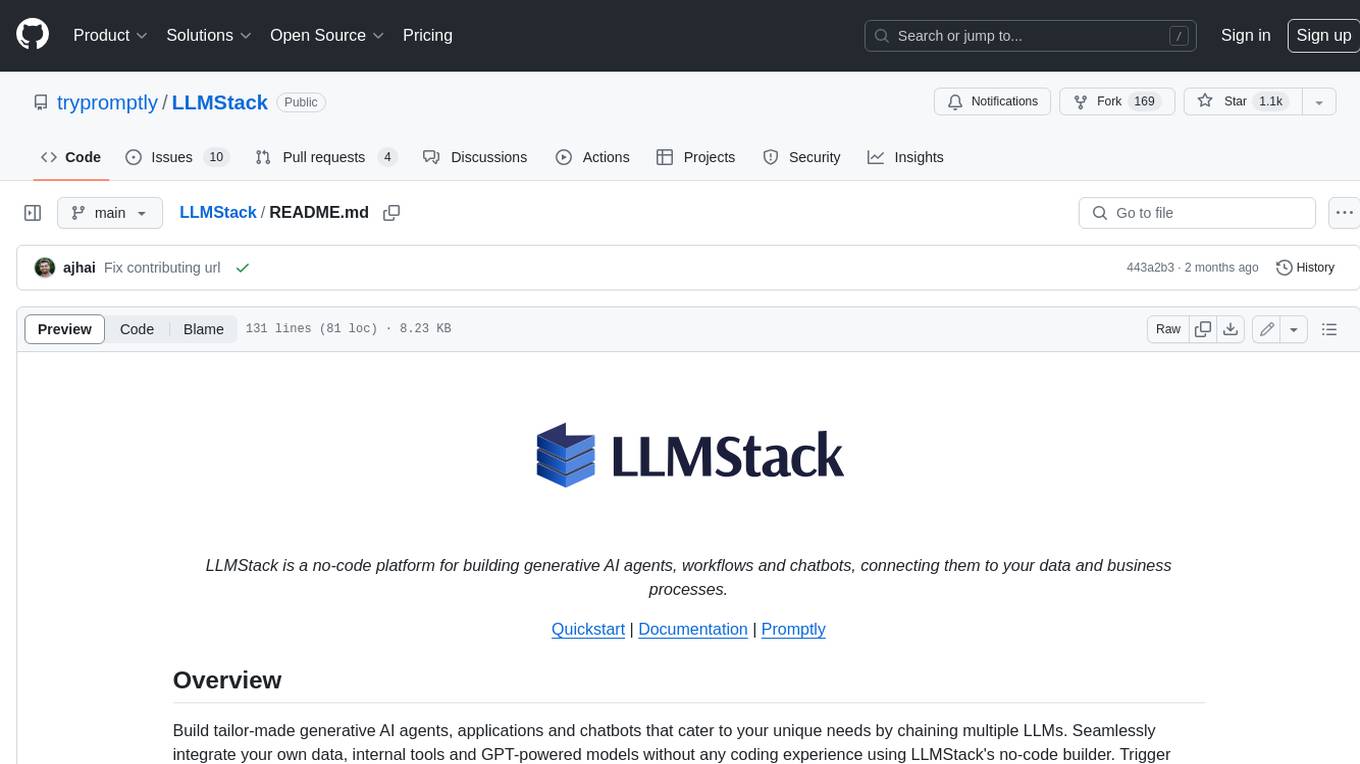
LLMStack
LLMStack is a no-code platform for building generative AI agents, workflows, and chatbots. It allows users to connect their own data, internal tools, and GPT-powered models without any coding experience. LLMStack can be deployed to the cloud or on-premise and can be accessed via HTTP API or triggered from Slack or Discord.
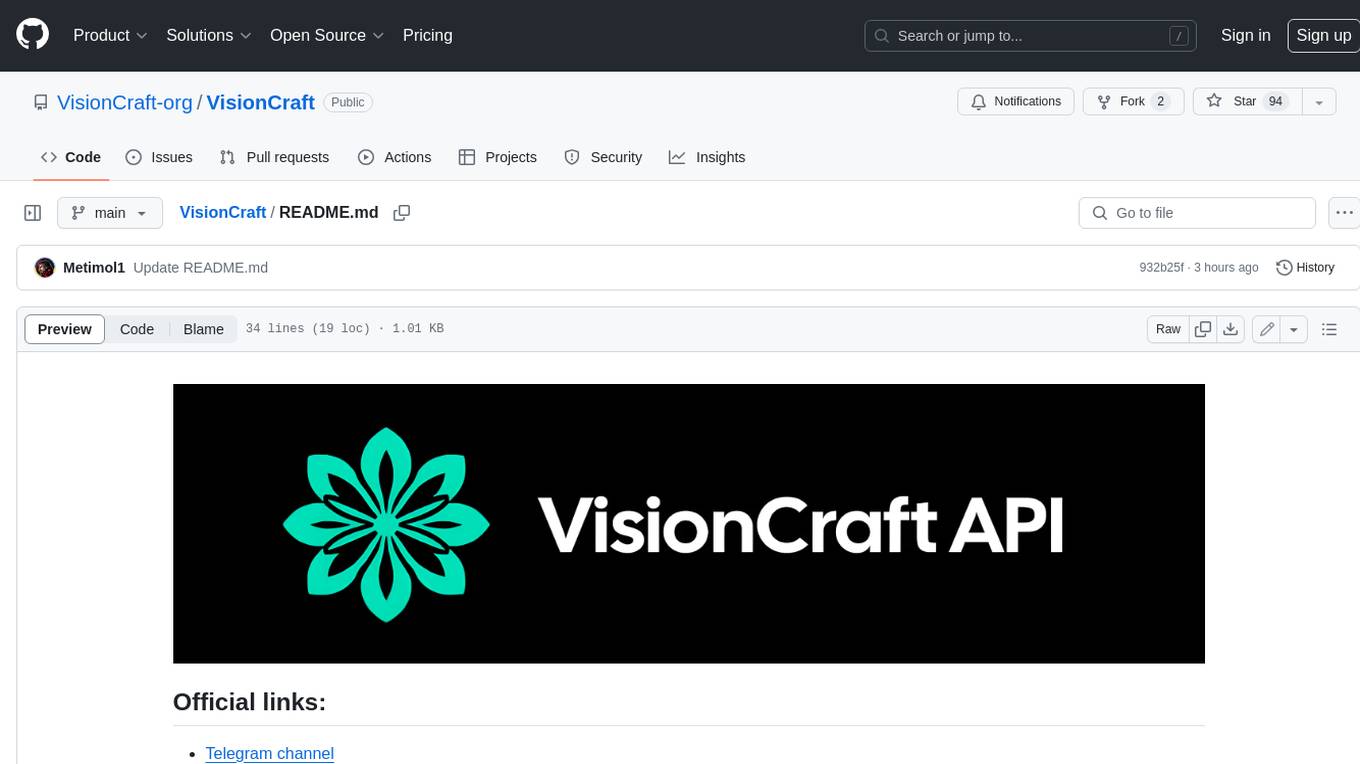
VisionCraft
The VisionCraft API is a free API for using over 100 different AI models. From images to sound.
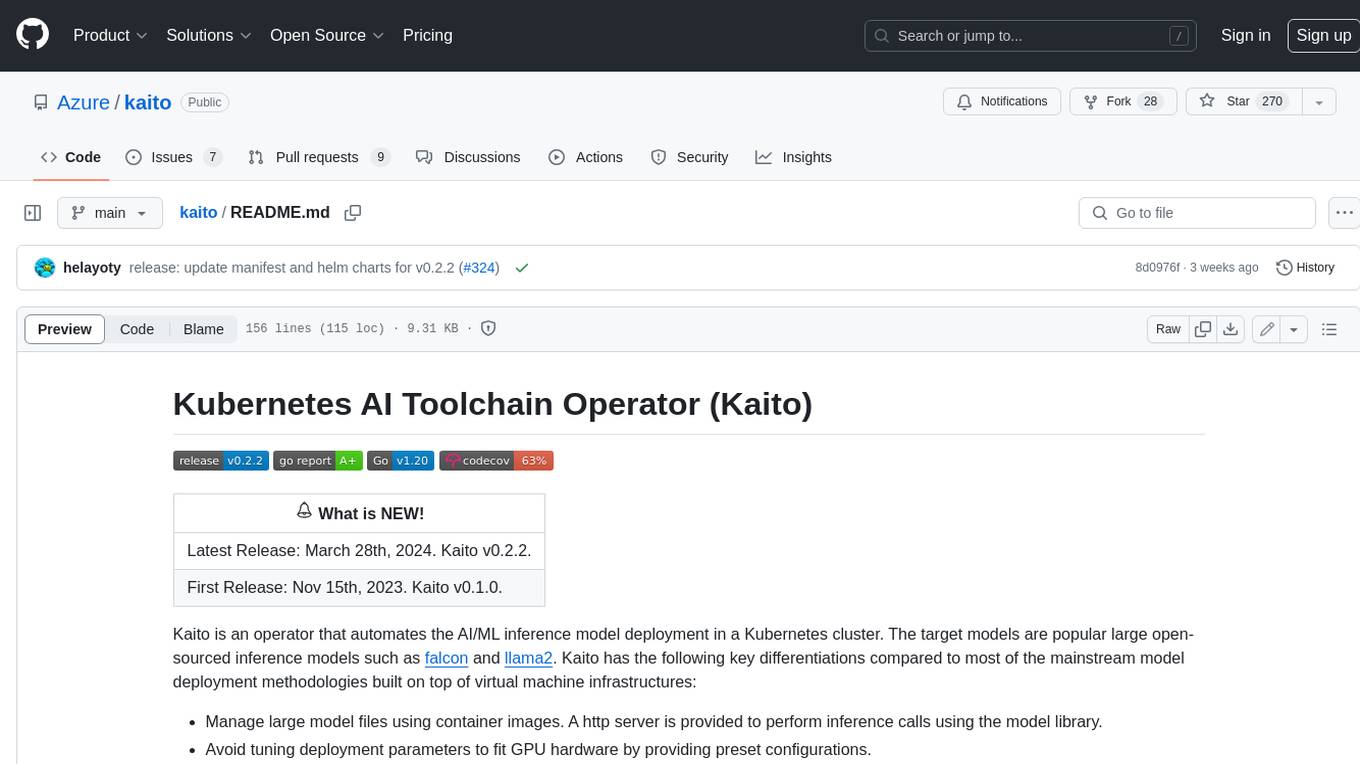
kaito
Kaito is an operator that automates the AI/ML inference model deployment in a Kubernetes cluster. It manages large model files using container images, avoids tuning deployment parameters to fit GPU hardware by providing preset configurations, auto-provisions GPU nodes based on model requirements, and hosts large model images in the public Microsoft Container Registry (MCR) if the license allows. Using Kaito, the workflow of onboarding large AI inference models in Kubernetes is largely simplified.
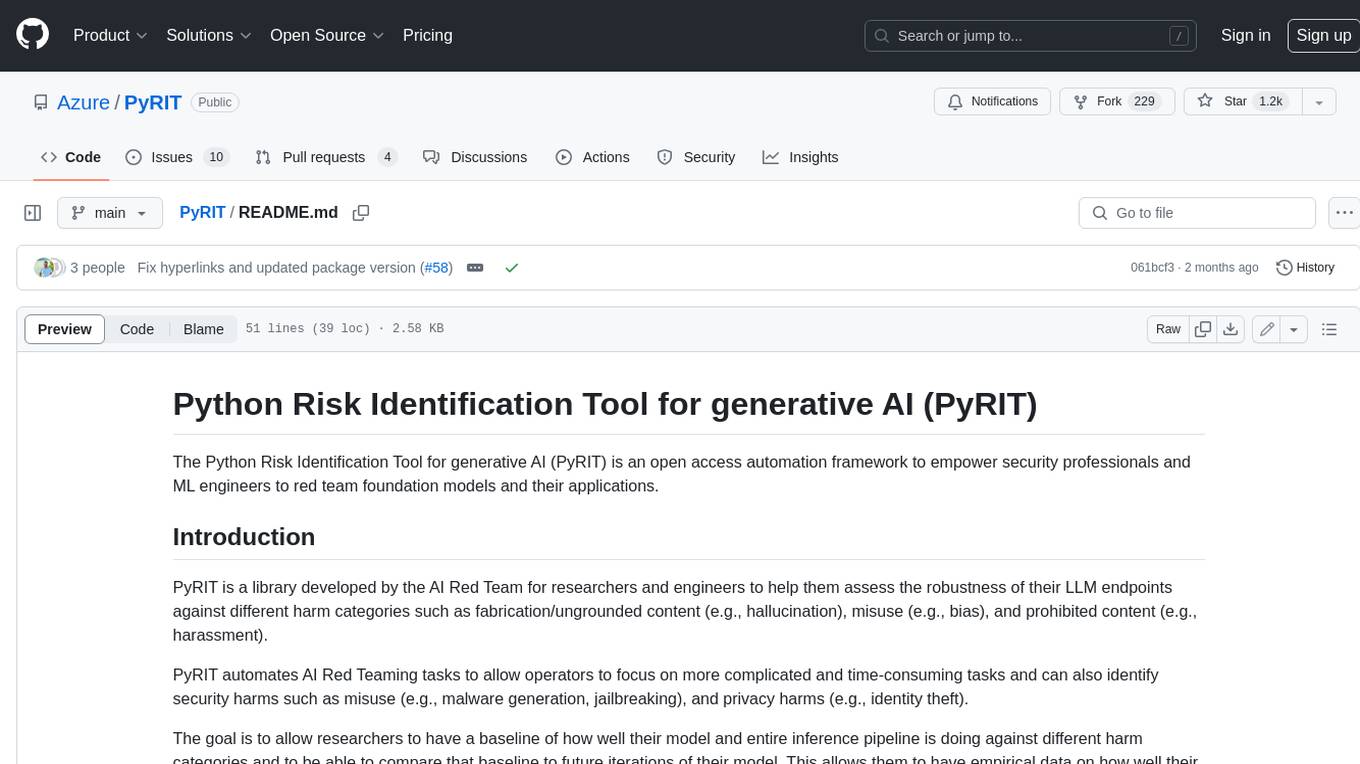
PyRIT
PyRIT is an open access automation framework designed to empower security professionals and ML engineers to red team foundation models and their applications. It automates AI Red Teaming tasks to allow operators to focus on more complicated and time-consuming tasks and can also identify security harms such as misuse (e.g., malware generation, jailbreaking), and privacy harms (e.g., identity theft). The goal is to allow researchers to have a baseline of how well their model and entire inference pipeline is doing against different harm categories and to be able to compare that baseline to future iterations of their model. This allows them to have empirical data on how well their model is doing today, and detect any degradation of performance based on future improvements.
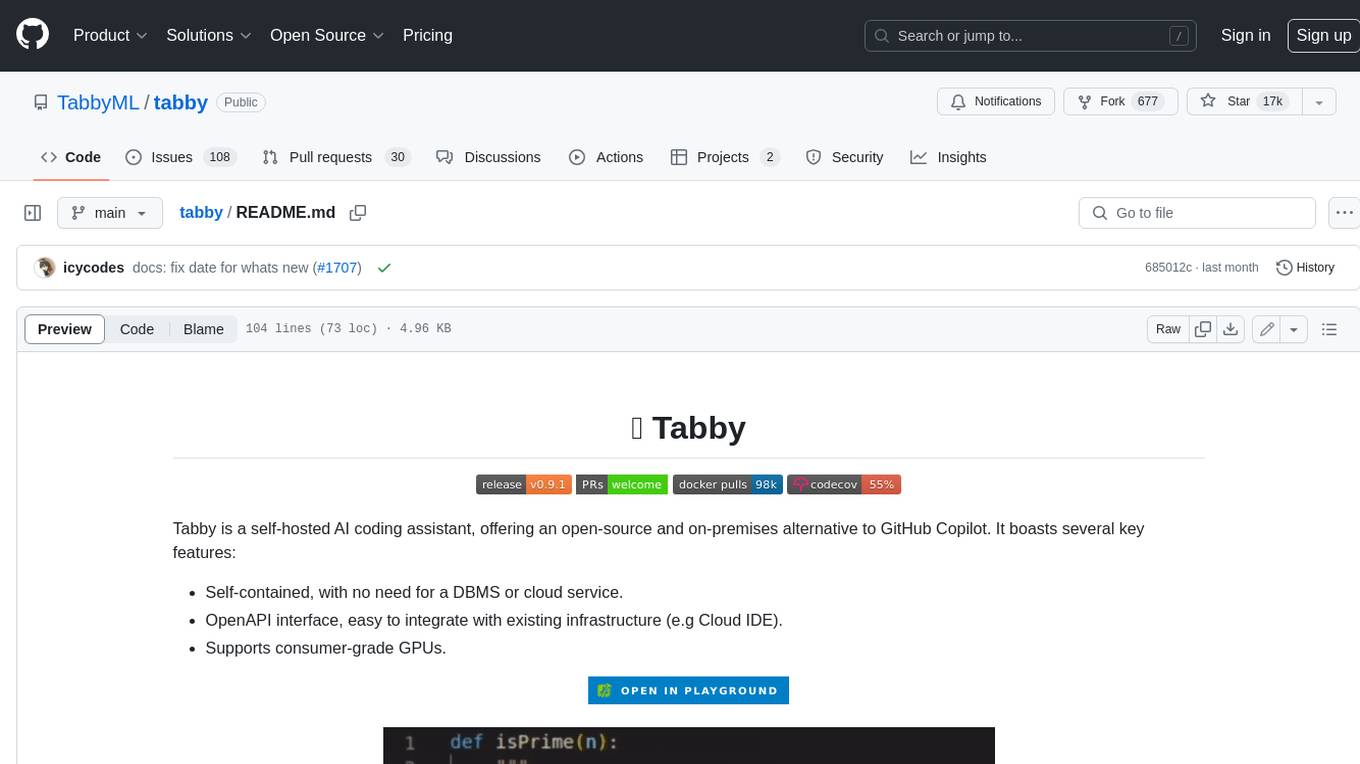
tabby
Tabby is a self-hosted AI coding assistant, offering an open-source and on-premises alternative to GitHub Copilot. It boasts several key features: * Self-contained, with no need for a DBMS or cloud service. * OpenAPI interface, easy to integrate with existing infrastructure (e.g Cloud IDE). * Supports consumer-grade GPUs.
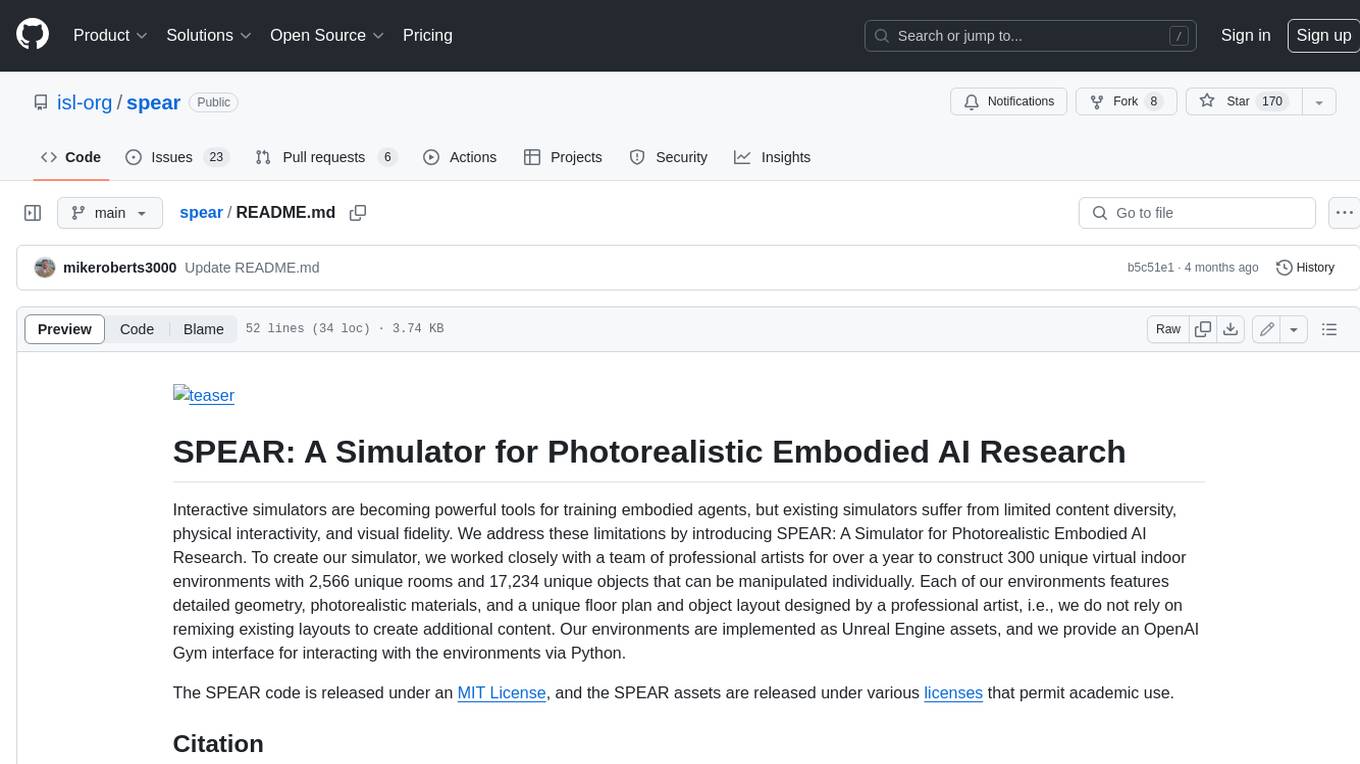
spear
SPEAR (Simulator for Photorealistic Embodied AI Research) is a powerful tool for training embodied agents. It features 300 unique virtual indoor environments with 2,566 unique rooms and 17,234 unique objects that can be manipulated individually. Each environment is designed by a professional artist and features detailed geometry, photorealistic materials, and a unique floor plan and object layout. SPEAR is implemented as Unreal Engine assets and provides an OpenAI Gym interface for interacting with the environments via Python.
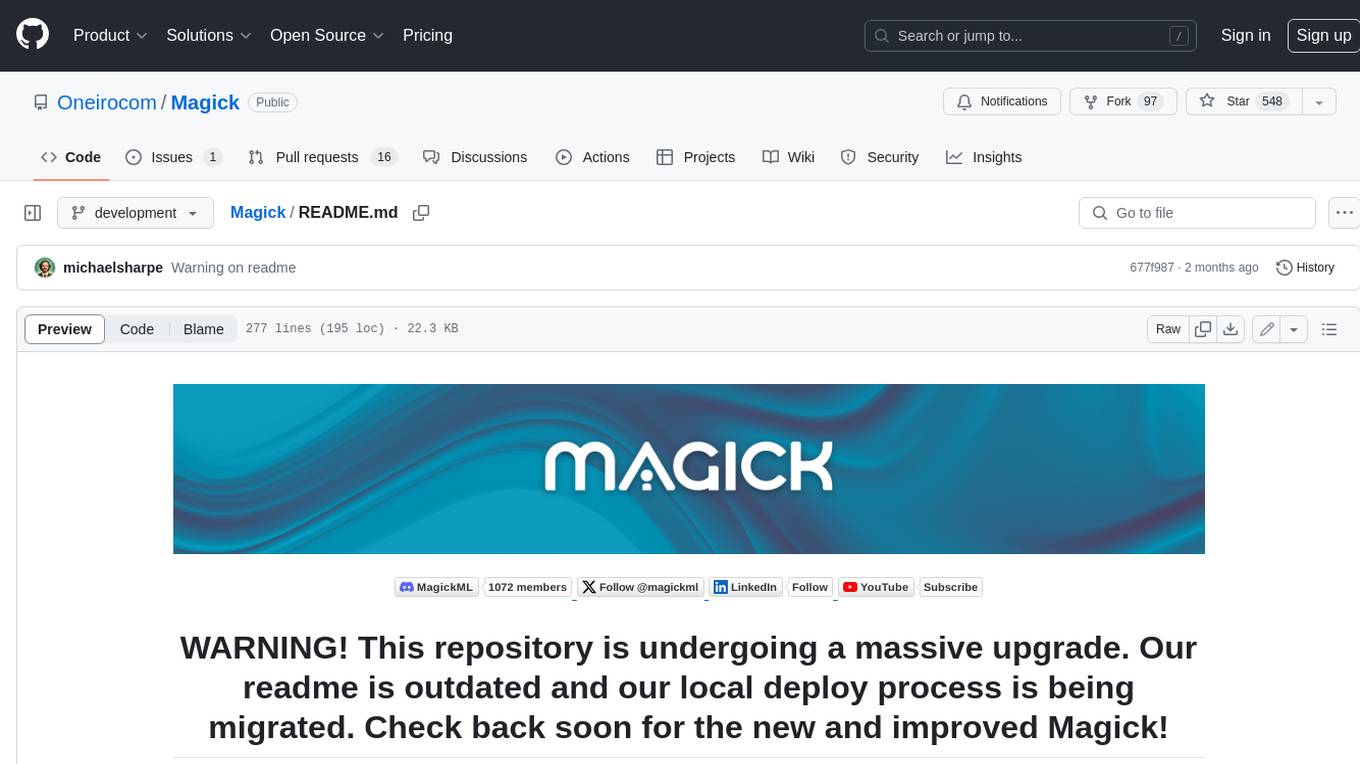
Magick
Magick is a groundbreaking visual AIDE (Artificial Intelligence Development Environment) for no-code data pipelines and multimodal agents. Magick can connect to other services and comes with nodes and templates well-suited for intelligent agents, chatbots, complex reasoning systems and realistic characters.