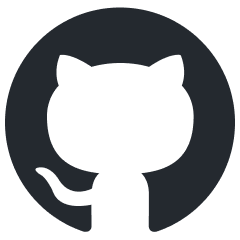
ComfyBench
Implementation for the paper "ComfyBench: Benchmarking LLM-based Agents in ComfyUI for Autonomously Designing Collaborative AI Systems".
Stars: 135
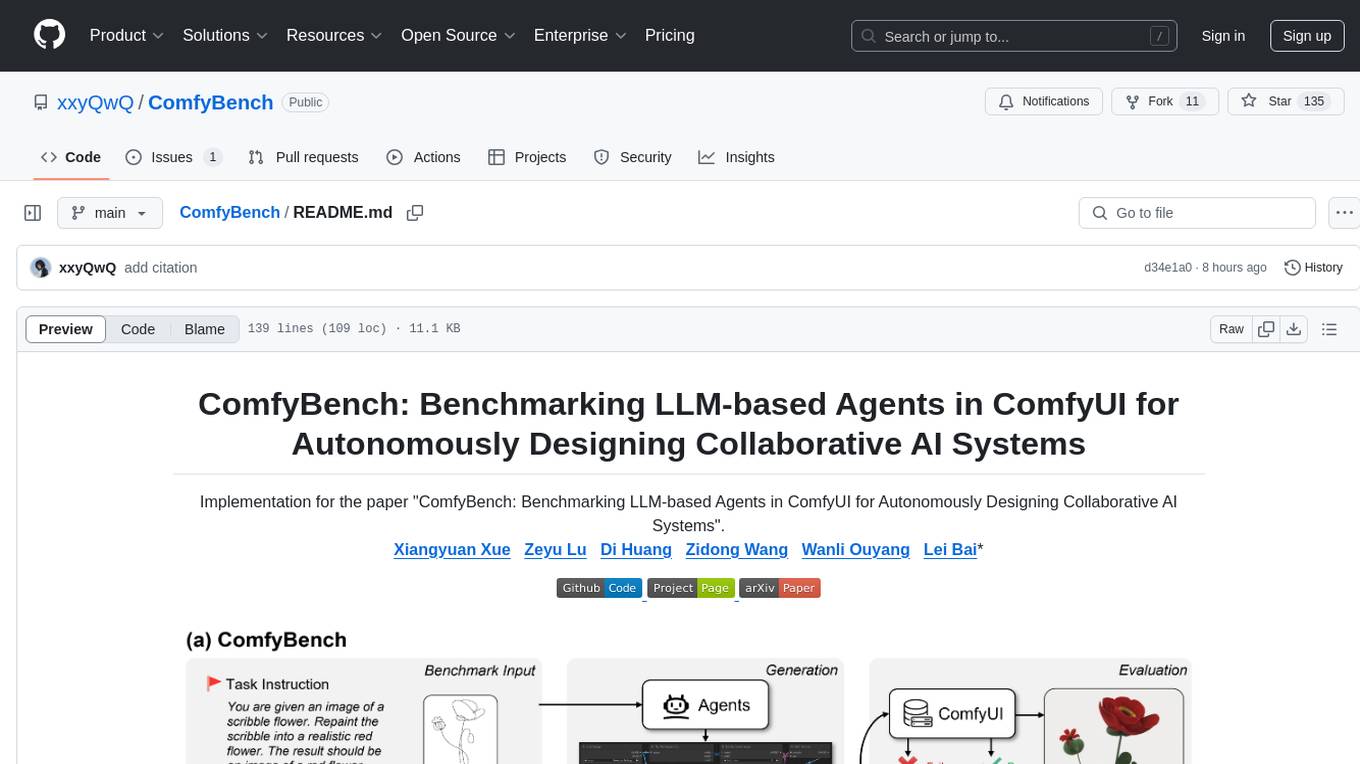
ComfyBench is a comprehensive benchmark tool designed to evaluate agents' ability to design collaborative AI systems in ComfyUI. It provides tasks for agents to learn from documents and create workflows, which are then converted into code for better understanding by LLMs. The tool measures performance based on pass rate and resolve rate, reflecting the correctness of workflow execution and task realization. ComfyAgent, a component of ComfyBench, autonomously designs new workflows by learning from existing ones, interpreting them as collaborative AI systems to complete given tasks.
README:
ComfyBench: Benchmarking LLM-based Agents in ComfyUI for Autonomously Designing Collaborative AI Systems
Implementation for the paper "ComfyBench: Benchmarking LLM-based Agents in ComfyUI for Autonomously Designing Collaborative AI Systems".
Xiangyuan Xue
Zeyu Lu
Di Huang
Zidong Wang
Wanli Ouyang
Lei Bai*
(a) ComfyBench is a comprehensive benchmark to evaluate agents's ability to design collaborative AI systems in ComfyUI. Given the task instruction, agents are required to learn from documents and create workflows to describe collaborative AI systems. The performance is measured by pass rate and resolve rate, reflecting whether the workflow can be correctly executed and whether the task requirements are realized. (b) ComfyAgent builds collaborative Al systems in ComfyUI by generating workflows. The workflows are converted into equivalent code so that LLMs can better understand them. ComfyAgent can learn from existing workflows and autonomously design new ones. The generated workflows can be interpreted as collaborative AI systems to complete given tasks.
- [2024/11/20] The latest version of our code is updated.
- [2024/11/14] The work is further extended and renamed as ComfyBench.
- [2024/09/04] Our code implementation is released on GitHub.
- [2024/09/02] The initial version of our paper is submitted to arXiv.
First, clone the repository and navigate to the project directory:
git clone https://github.com/xxyQwQ/ComfyBench
cd ComfyBench
Then, create a new conda environment and install the dependencies:
conda create -n comfybench python=3.12
conda activate comfybench
pip install -r requirements.txt
Finally, modify config.yaml
to set your ComfyUI server and API key. Feel free to change proxies and models if necessary.
Despite some models can be automatically installed, other models need to be manually downloaded and placed in the specific directory. You may find them by yourself on Hugging Face or directly download from our Cloud Drive. Besides, we provide a list of extensions in assets/extension.md
so that you can install them manually. You can verify the completeness with the workflows in dataset/benchmark/workflow
.
Run the following commands to execute the ComfyAgent pipeline:
# activate the conda environment
conda activate comfybench
# execute the main script
# see `main.py` for more parameter settings
python main.py \
--instruction "task-instruction" \
--agent_name "comfy" \
--save_path "path/to/save/result"
The log file together with the workflow will be saved in the specified path. If your ComfyUI server is working properly, the workflow will be executed automatically to produce the result.
ComfyBench is provided under dataset/benchmark
. The document
folder contains documentation for 3205 nodes, where meta.json
records the metadata of each node. The workflow
folder contains 20 curriculum workflows for agents to learn from. The instruction
provides all the tasks in ComfyBench, where complete.json
contains 200 task instructions and sample.json
contains a subset of 10 task instructions for validation.
Before evaluating on ComfyBench, you should copy the resource files in dataset/benchmark/resource
into the input folder of ComfyUI, so that ComfyUI can load them during the workflow execution. Then you can evaluate the specific agent by running the following commands. Here we take ComfyAgent as an example.
# activate the conda environment and set up environment variables
conda activate comfybench
export PYTHONPATH=./
# execute the inference script
# see `script/inference.py` for more parameter settings
python script/inference.py \
--agent_name "comfy" \
--save_path "cache/benchmark/comfy"
# execute the evaluation script
# see `script/evaluation.py` for more parameter settings
python script/evaluation.py \
--submit_folder "cache/benchmark/comfy/workflow" \
--cache_path "cache/benchmark/comfy/outcome"
In this example, the log files and generated workflows will be saved in cache/benchmark/comfy/logging
and cache/benchmark/comfy/workflow
, respectively. The produced results will be saved in cache/benchmark/comfy/outcome
, together with a result.json
recording whether each task is passed and resolved, and a summary.txt
summarizing the overall metrics.
Here are some examples of the results produced by ComfyAgent on ComfyBench. Visit our Project Page for more details.
If you find our work helpful, please consider citing our paper:
@article{xue2024comfybench,
title={ComfyBench: Benchmarking LLM-based Agents in ComfyUI for Autonomously Designing Collaborative AI Systems},
author={Xue, Xiangyuan and Lu, Zeyu and Huang, Di and Wang, Zidong and Ouyang, Wanli and Bai, Lei},
journal={arXiv preprint arXiv:2409.01392},
year={2024}
}
For Tasks:
Click tags to check more tools for each tasksFor Jobs:
Alternative AI tools for ComfyBench
Similar Open Source Tools
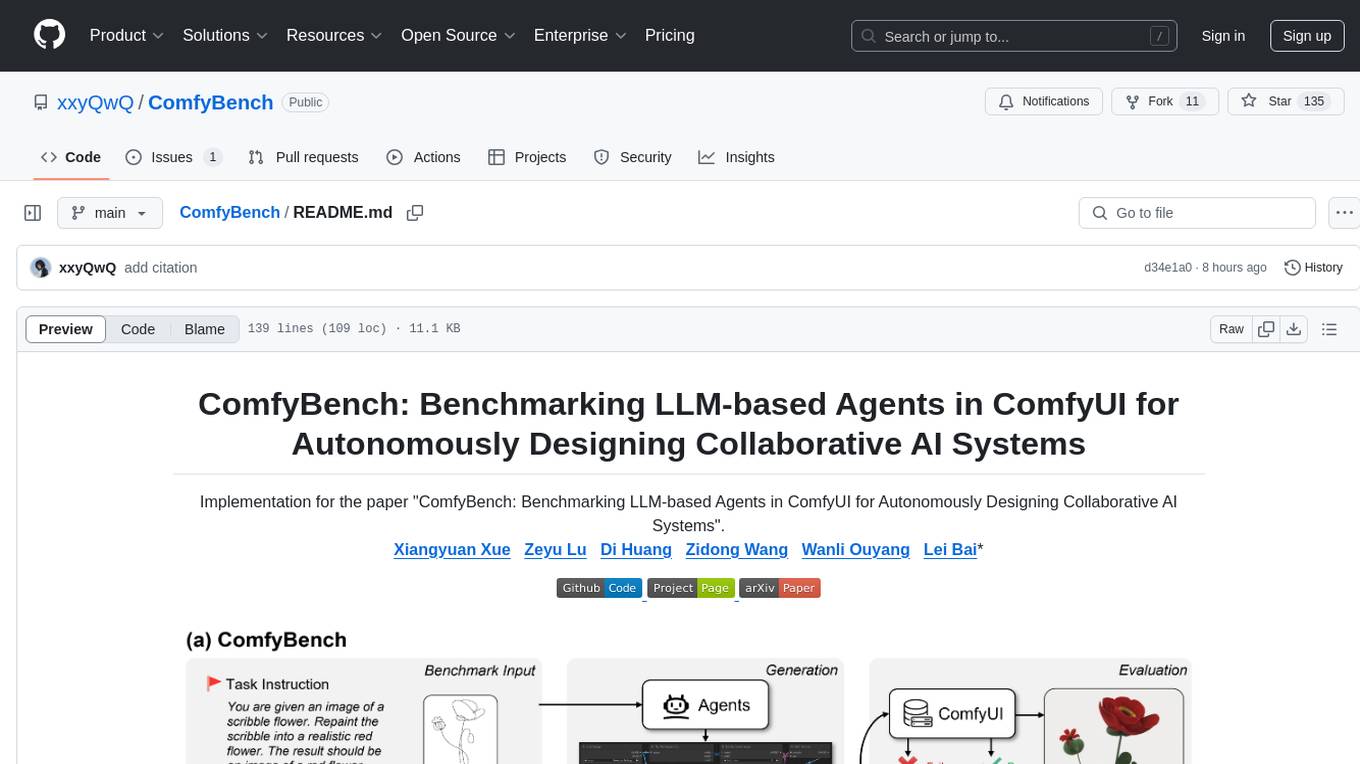
ComfyBench
ComfyBench is a comprehensive benchmark tool designed to evaluate agents' ability to design collaborative AI systems in ComfyUI. It provides tasks for agents to learn from documents and create workflows, which are then converted into code for better understanding by LLMs. The tool measures performance based on pass rate and resolve rate, reflecting the correctness of workflow execution and task realization. ComfyAgent, a component of ComfyBench, autonomously designs new workflows by learning from existing ones, interpreting them as collaborative AI systems to complete given tasks.
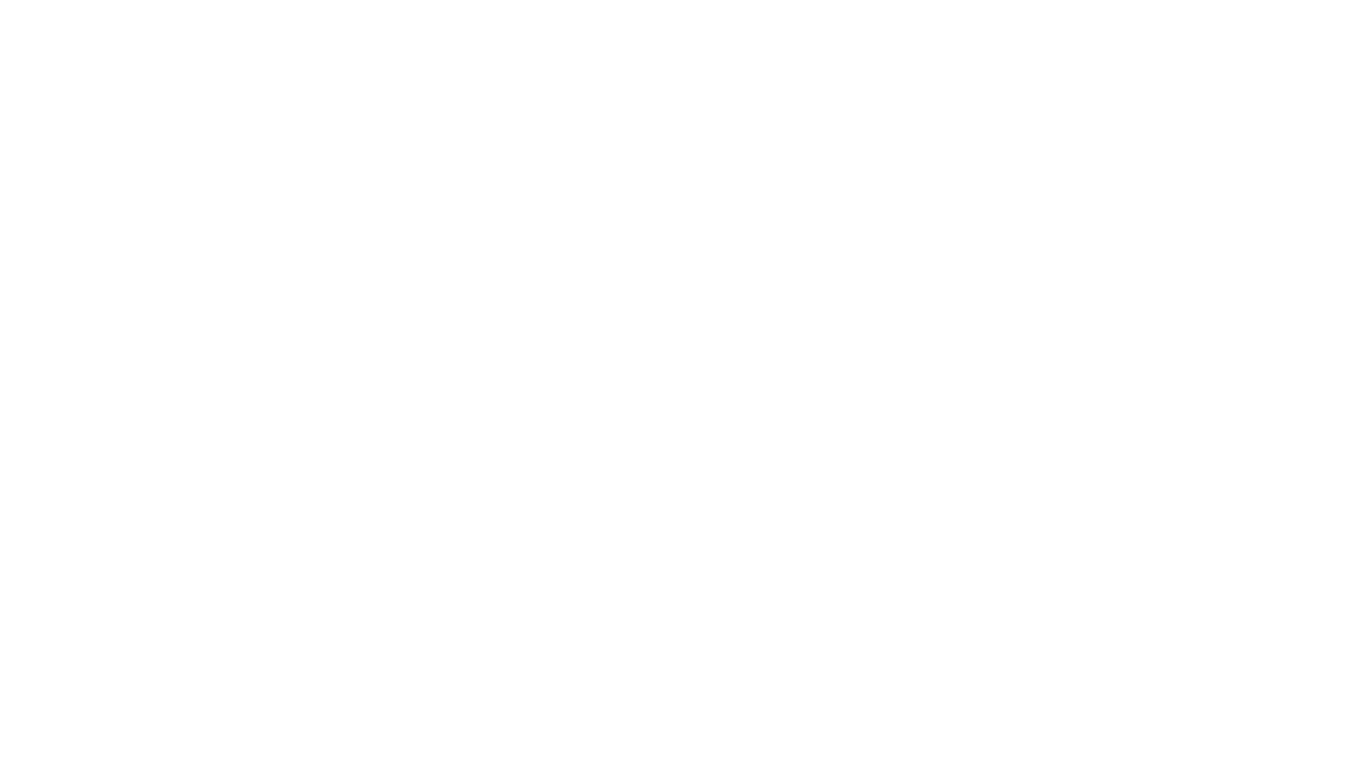
PanelCleaner
Panel Cleaner is a tool that uses machine learning to find text in images and generate masks to cover it up with high accuracy. It is designed to clean text bubbles without leaving artifacts, avoiding painting over non-text parts, and inpainting bubbles that can't be masked out. The tool offers various customization options, detailed analytics on the cleaning process, supports batch processing, and can run OCR on pages. It supports CUDA acceleration, multiple themes, and can handle bubbles on any solid grayscale background color. Panel Cleaner is aimed at saving time for cleaners by automating monotonous work and providing precise cleaning of text bubbles.
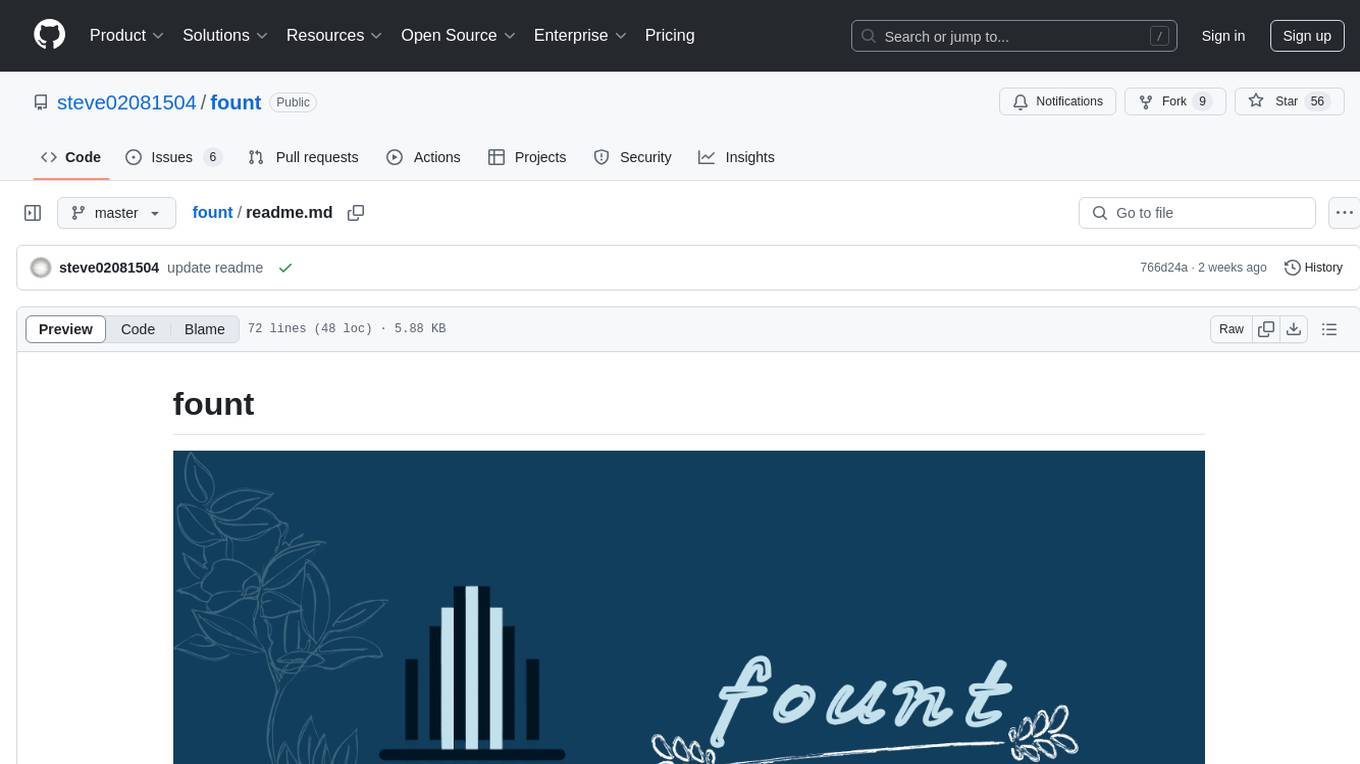
fount
fount is a character card frontend page that decouples AI sources, AI characters, user personas, dialogue environments, and AI plugins, allowing them to be freely combined to spark infinite possibilities. It serves as a bridge connecting imagination and reality, a lighthouse guiding characters and stories, and a free garden for AI sources, characters, personas, dialogue environments, and plugins to grow and bloom. It integrates AI sources without the need for reverse proxy servers, improves web experience with features like multi-device synchronization and unfiltered HTML rendering, and extends companionship beyond the web by connecting characters to Discord groups and providing gentle reminders through fount-pwsh. For character creators, fount offers infinite possibilities with JavaScript or TypeScript code customization, execution of code without filtering, loading npm packages, and creating custom HTML pages. It encourages extension through modularization and community contributions.
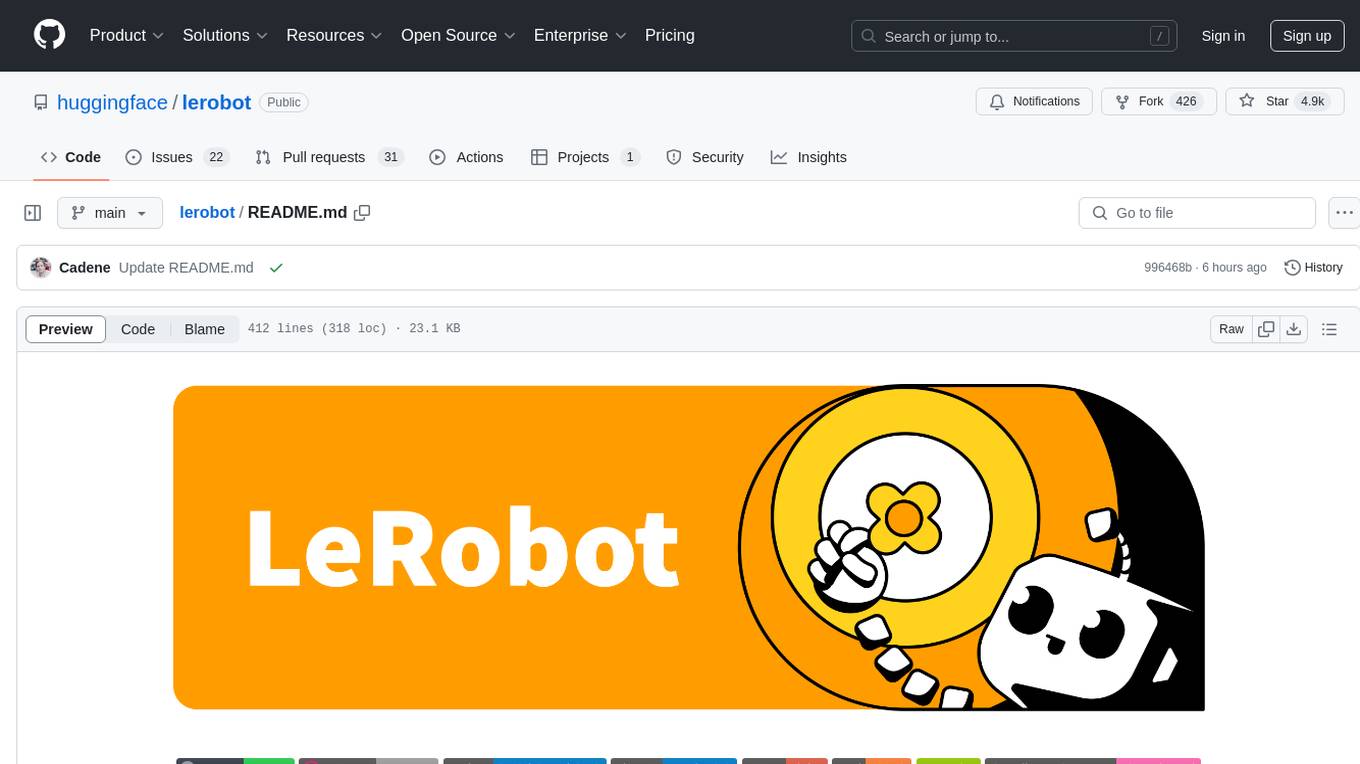
lerobot
LeRobot is a state-of-the-art AI library for real-world robotics in PyTorch. It aims to provide models, datasets, and tools to lower the barrier to entry to robotics, focusing on imitation learning and reinforcement learning. LeRobot offers pretrained models, datasets with human-collected demonstrations, and simulation environments. It plans to support real-world robotics on affordable and capable robots. The library hosts pretrained models and datasets on the Hugging Face community page.
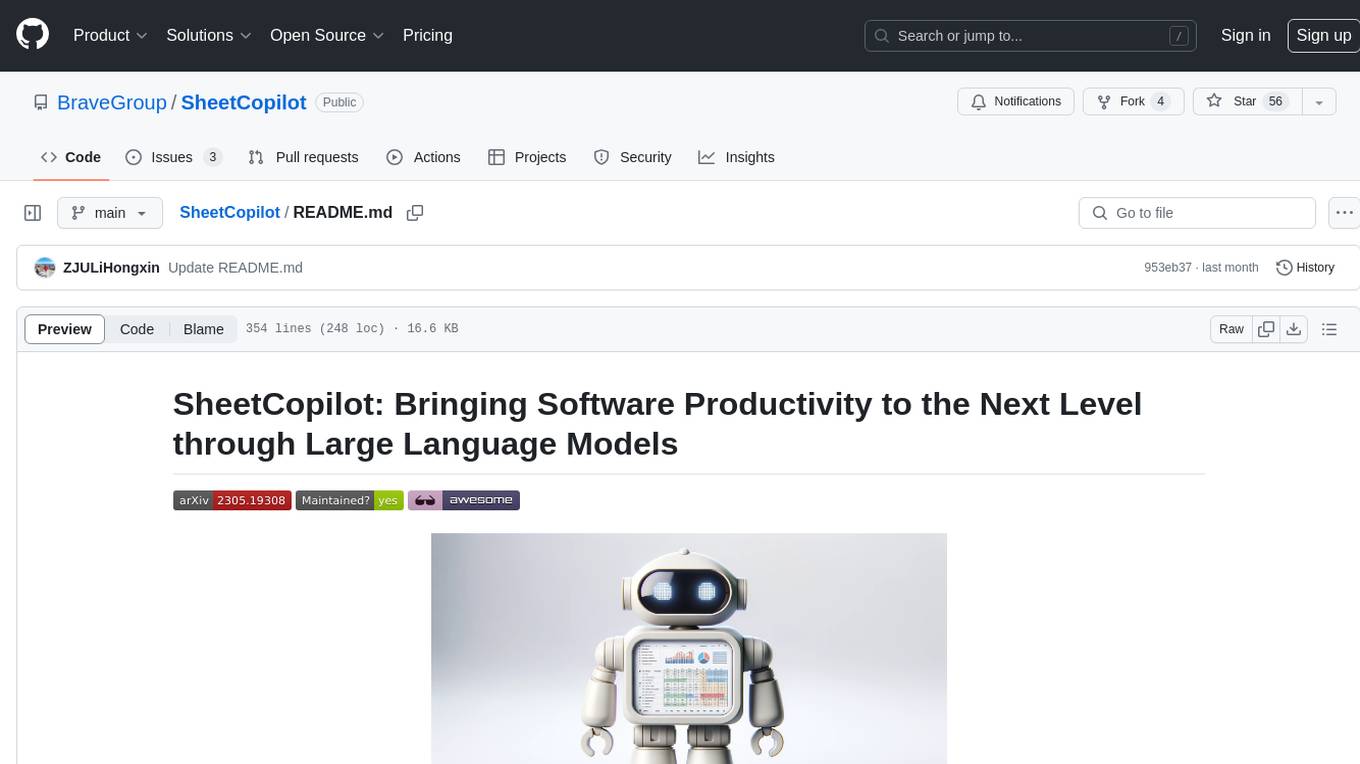
SheetCopilot
SheetCopilot is an assistant agent that manipulates spreadsheets by following user commands. It leverages Large Language Models (LLMs) to interact with spreadsheets like a human expert, enabling non-expert users to complete tasks on complex software such as Google Sheets and Excel via a language interface. The tool observes spreadsheet states, polishes generated solutions based on external action documents and error feedback, and aims to improve success rate and efficiency. SheetCopilot offers a dataset with diverse task categories and operations, supporting operations like entry & manipulation, management, formatting, charts, and pivot tables. Users can interact with SheetCopilot in Excel or Google Sheets, executing tasks like calculating revenue, creating pivot tables, and plotting charts. The tool's evaluation includes performance comparisons with leading LLMs and VBA-based methods on specific datasets, showcasing its capabilities in controlling various aspects of a spreadsheet.
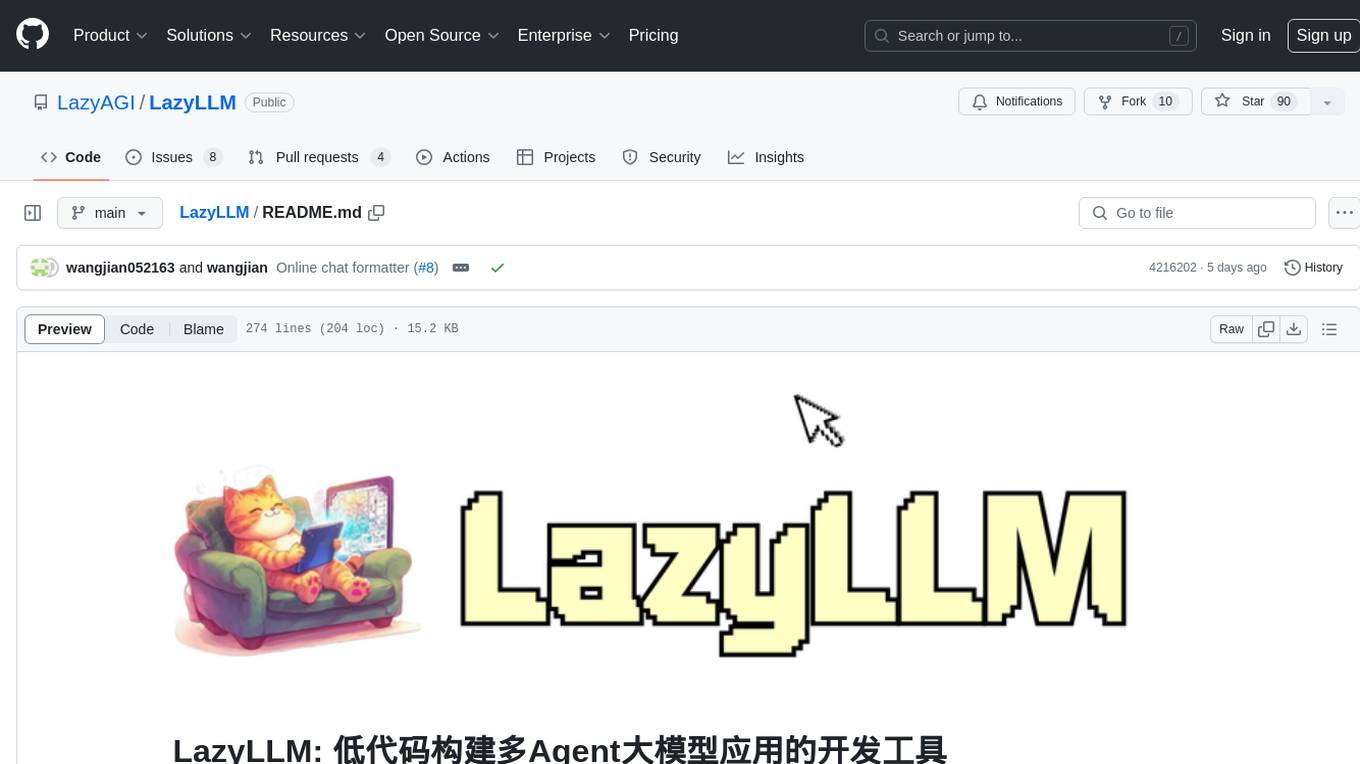
LazyLLM
LazyLLM is a low-code development tool for building complex AI applications with multiple agents. It assists developers in building AI applications at a low cost and continuously optimizing their performance. The tool provides a convenient workflow for application development and offers standard processes and tools for various stages of application development. Users can quickly prototype applications with LazyLLM, analyze bad cases with scenario task data, and iteratively optimize key components to enhance the overall application performance. LazyLLM aims to simplify the AI application development process and provide flexibility for both beginners and experts to create high-quality applications.
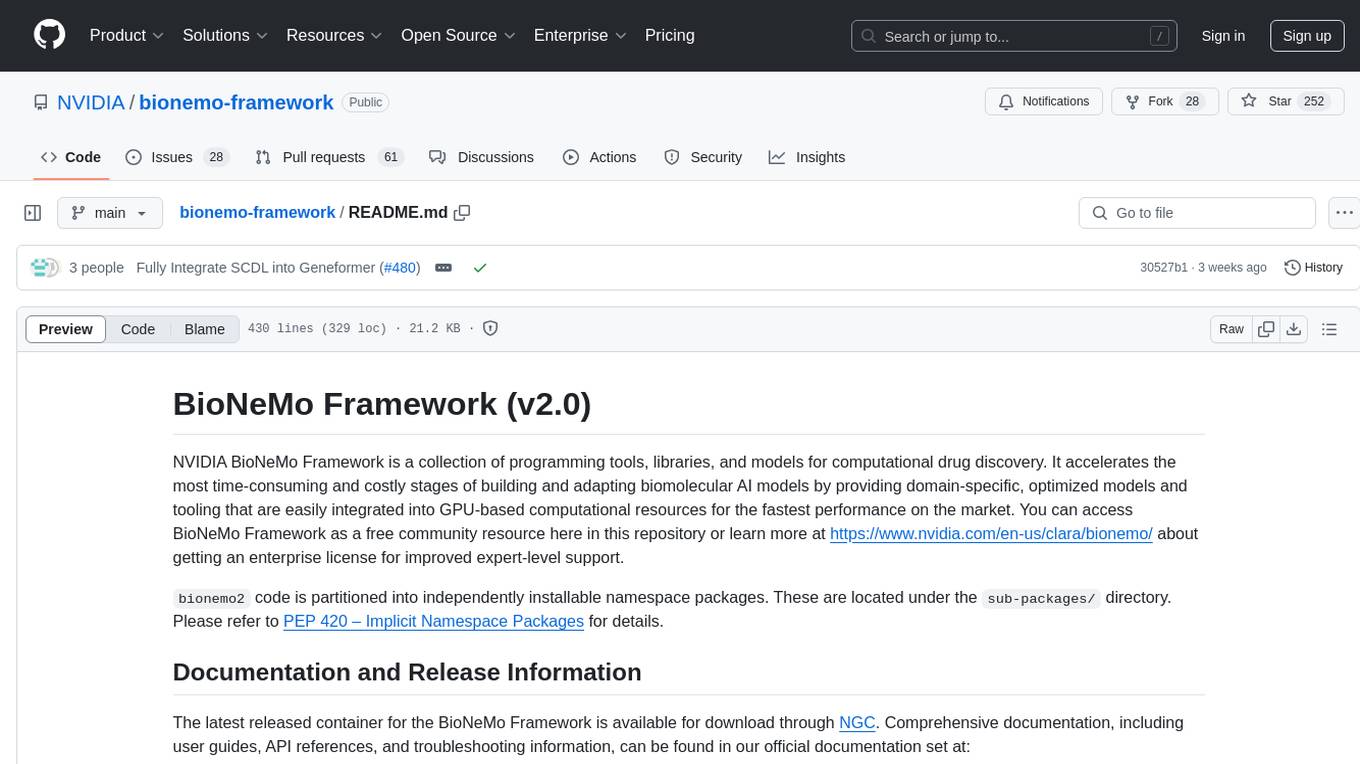
bionemo-framework
NVIDIA BioNeMo Framework is a collection of programming tools, libraries, and models for computational drug discovery. It accelerates building and adapting biomolecular AI models by providing domain-specific, optimized models and tooling for GPU-based computational resources. The framework offers comprehensive documentation and support for both community and enterprise users.
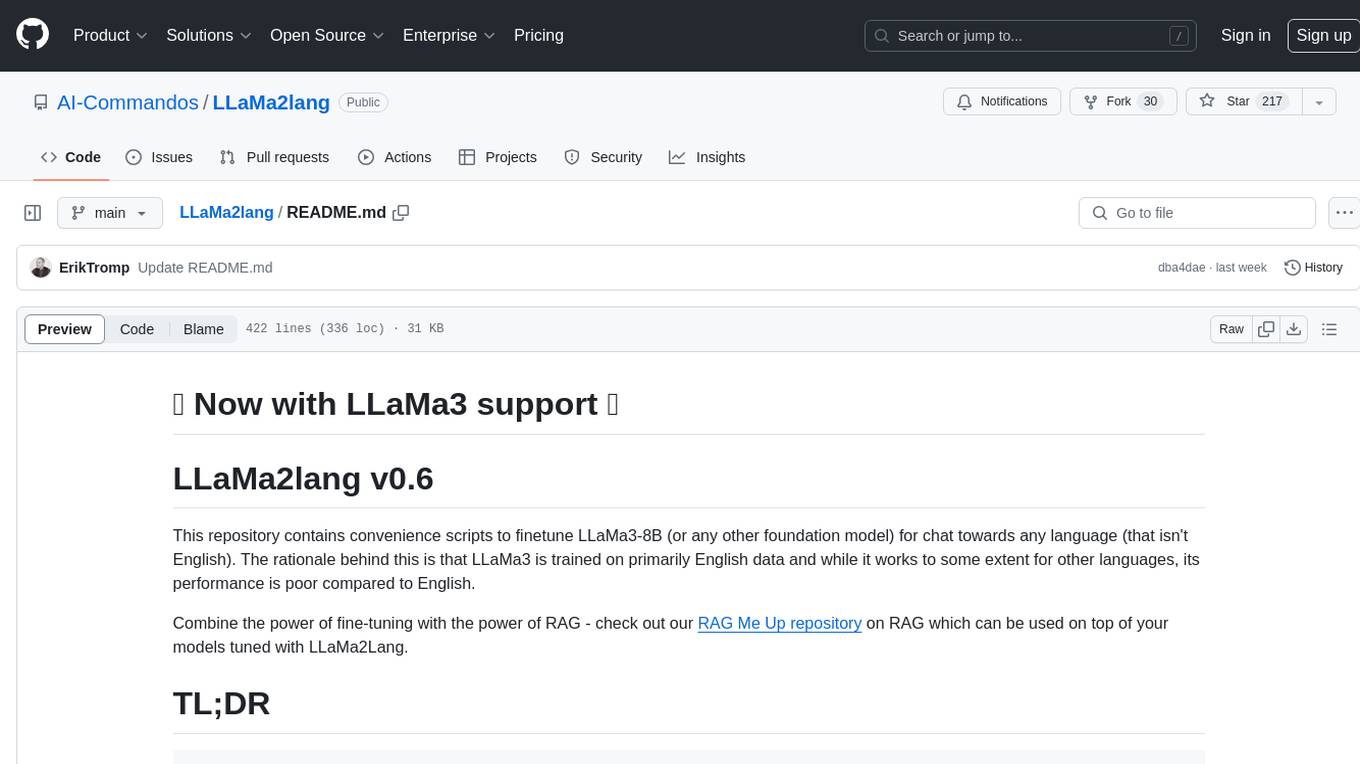
LLaMa2lang
LLaMa2lang is a repository containing convenience scripts to finetune LLaMa3-8B (or any other foundation model) for chat towards any language that isn't English. The repository aims to improve the performance of LLaMa3 for non-English languages by combining fine-tuning with RAG. Users can translate datasets, extract threads, turn threads into prompts, and finetune models using QLoRA and PEFT. Additionally, the repository supports translation models like OPUS, M2M, MADLAD, and base datasets like OASST1 and OASST2. The process involves loading datasets, translating them, combining checkpoints, and running inference using the newly trained model. The repository also provides benchmarking scripts to choose the right translation model for a target language.
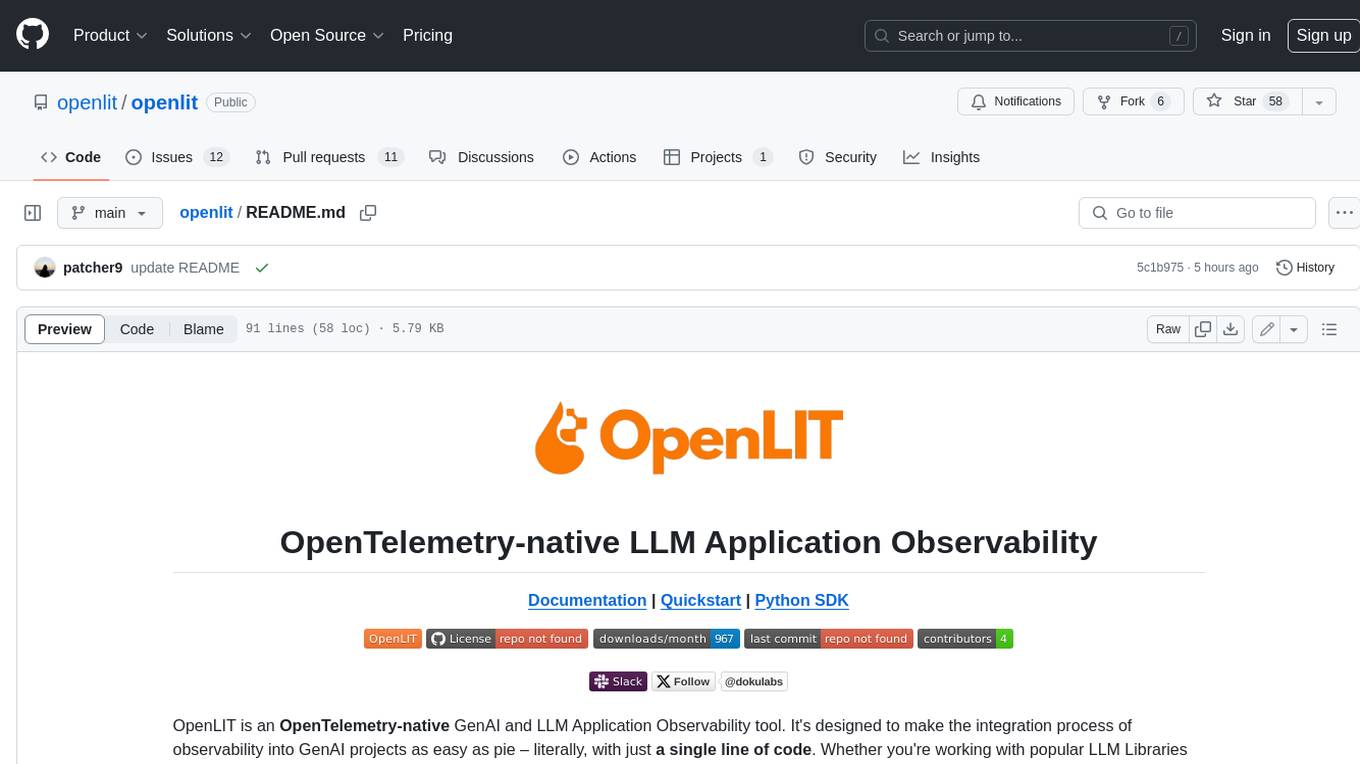
doku
OpenLIT is an OpenTelemetry-native GenAI and LLM Application Observability tool. It's designed to make the integration process of observability into GenAI projects as easy as pie – literally, with just a single line of code. Whether you're working with popular LLM Libraries such as OpenAI and HuggingFace or leveraging vector databases like ChromaDB, OpenLIT ensures your applications are monitored seamlessly, providing critical insights to improve performance and reliability.
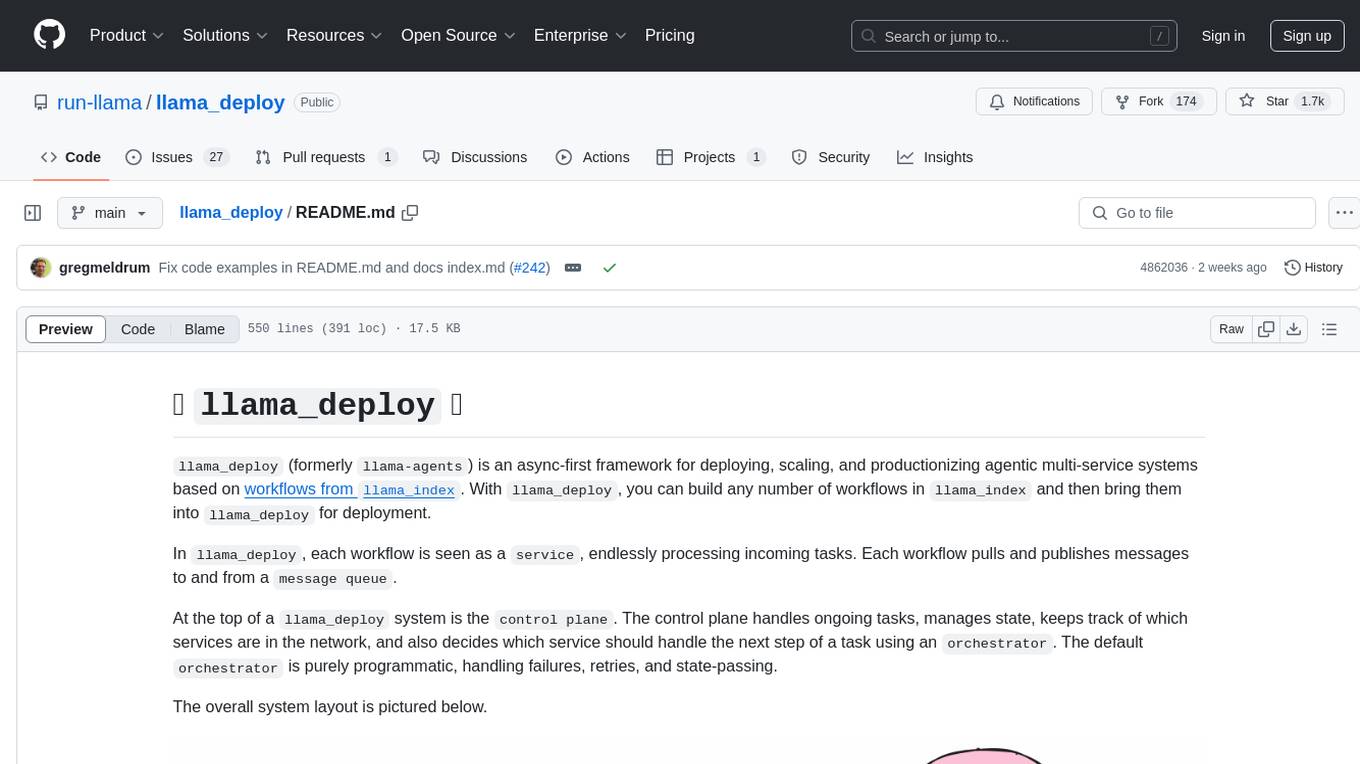
llama_deploy
llama_deploy is an async-first framework for deploying, scaling, and productionizing agentic multi-service systems based on workflows from llama_index. It allows building workflows in llama_index and deploying them seamlessly with minimal changes to code. The system includes services endlessly processing tasks, a control plane managing state and services, an orchestrator deciding task handling, and fault tolerance mechanisms. It is designed for high-concurrency scenarios, enabling real-time and high-throughput applications.
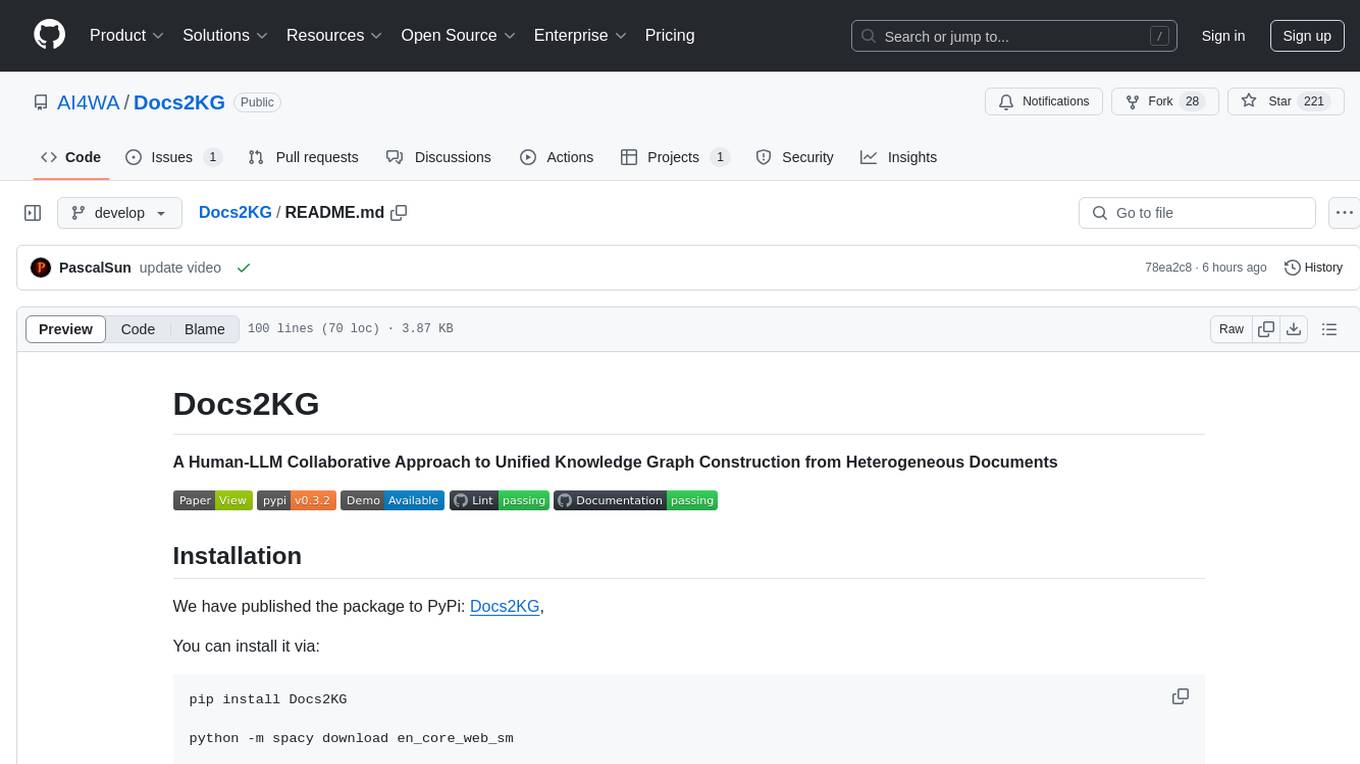
Docs2KG
Docs2KG is a tool designed for constructing a unified knowledge graph from heterogeneous documents. It addresses the challenges of digitizing diverse unstructured documents and constructing a high-quality knowledge graph with less effort. The tool combines bottom-up and top-down approaches, utilizing a human-LLM collaborative interface to enhance the generated knowledge graph. It organizes the knowledge graph into MetaKG, LayoutKG, and SemanticKG, providing a comprehensive view of document content. Docs2KG aims to streamline the process of knowledge graph construction and offers metrics for evaluating the quality of automatic construction.
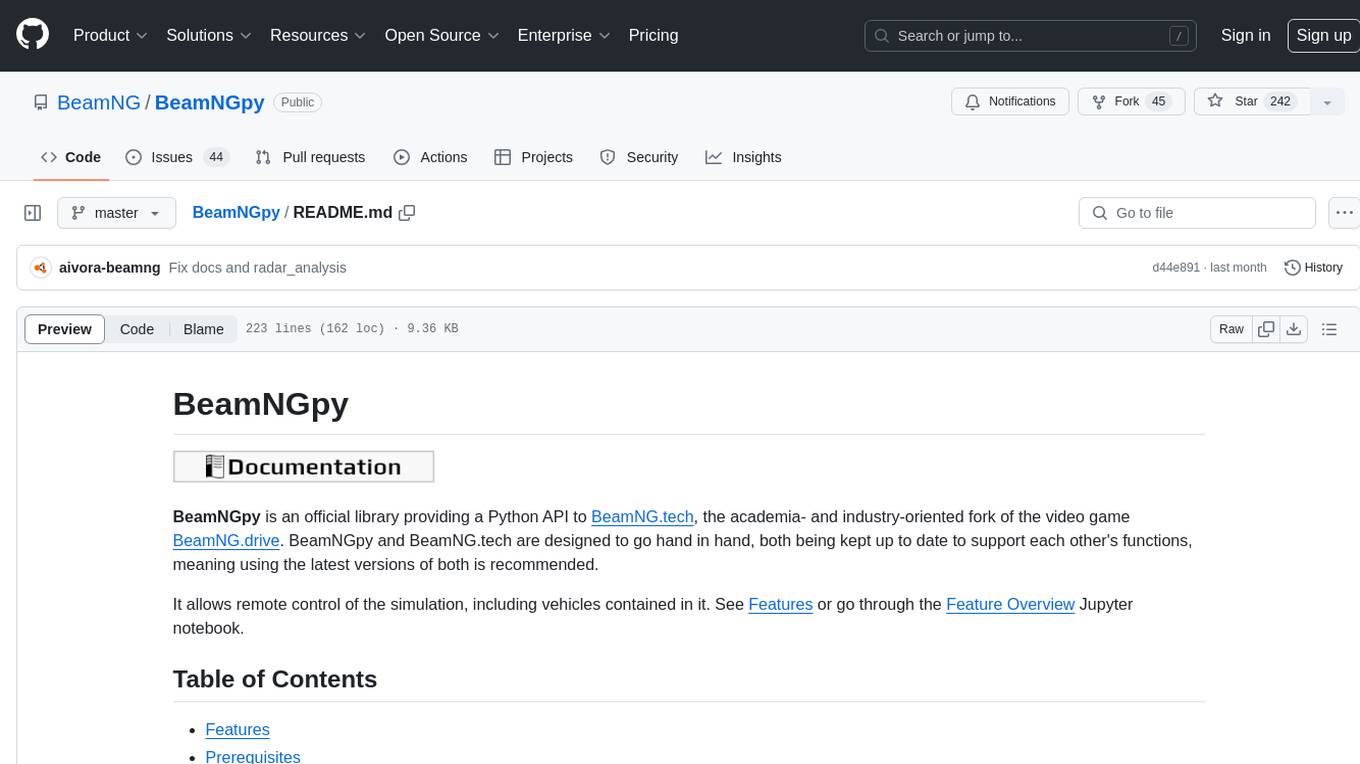
BeamNGpy
BeamNGpy is an official Python library providing an API to interact with BeamNG.tech, a video game focused on academia and industry. It allows remote control of vehicles, AI-controlled vehicles, dynamic sensor models, access to road network and scenario objects, and multiple clients. The library comes with low-level functions and higher-level interfaces for complex actions. BeamNGpy requires BeamNG.tech for usage and offers compatibility information for different versions. It also provides troubleshooting tips and encourages user contributions.
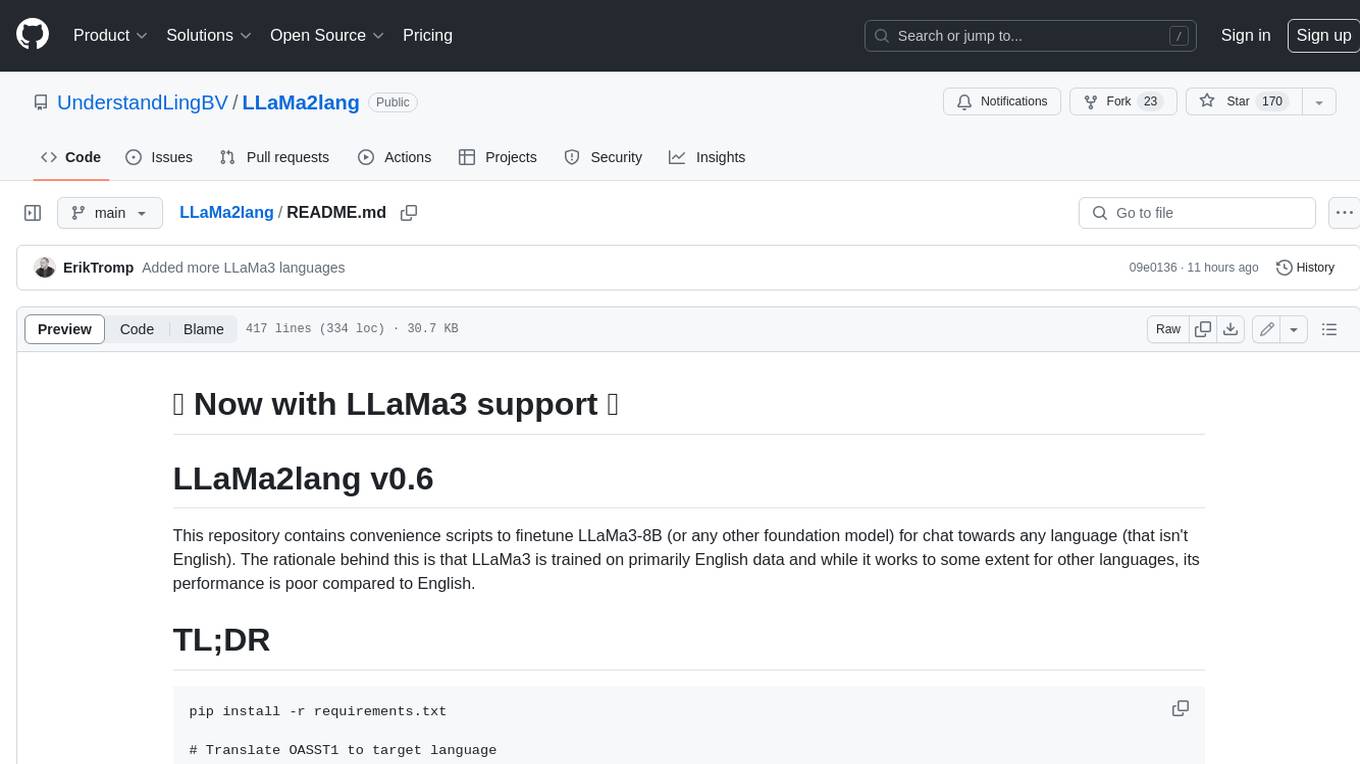
LLaMa2lang
This repository contains convenience scripts to finetune LLaMa3-8B (or any other foundation model) for chat towards any language (that isn't English). The rationale behind this is that LLaMa3 is trained on primarily English data and while it works to some extent for other languages, its performance is poor compared to English.
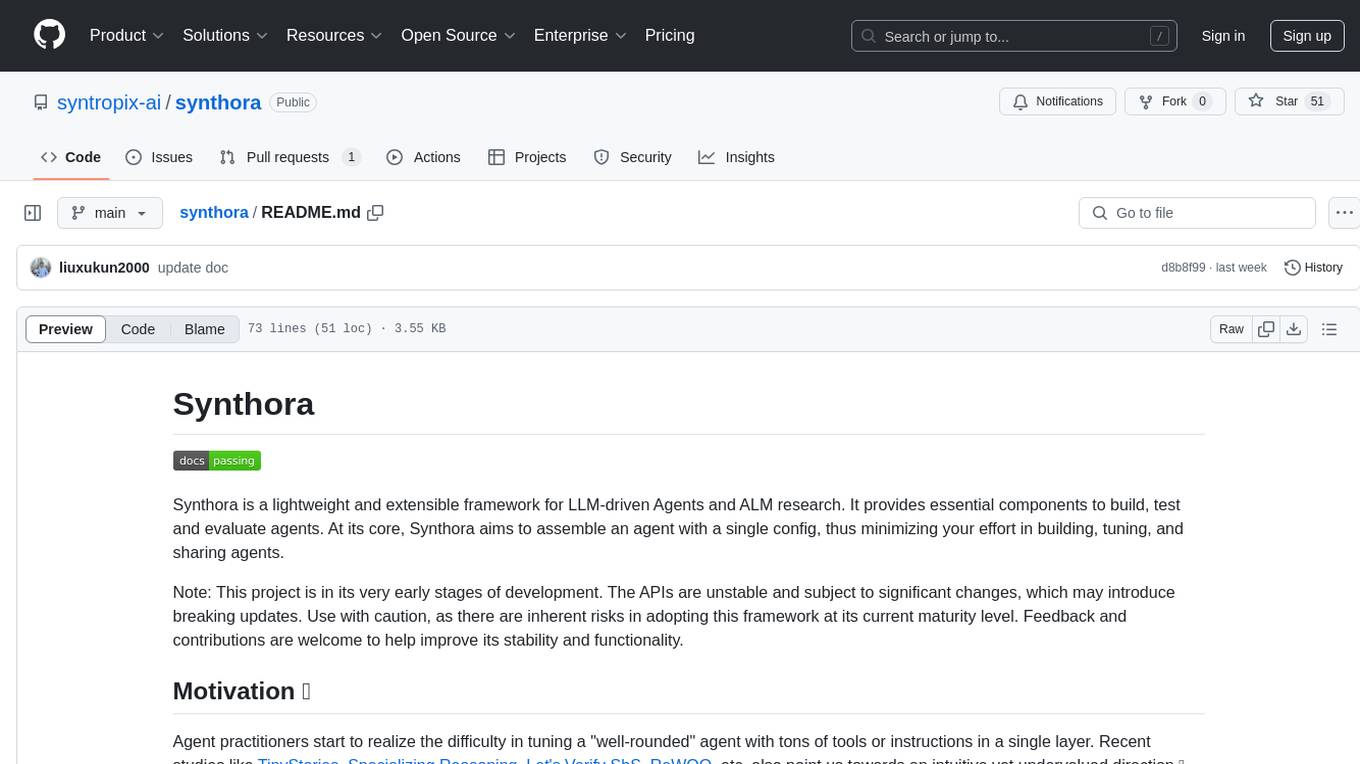
synthora
Synthora is a lightweight and extensible framework for LLM-driven Agents and ALM research. It aims to simplify the process of building, testing, and evaluating agents by providing essential components. The framework allows for easy agent assembly with a single config, reducing the effort required for tuning and sharing agents. Although in early development stages with unstable APIs, Synthora welcomes feedback and contributions to enhance its stability and functionality.
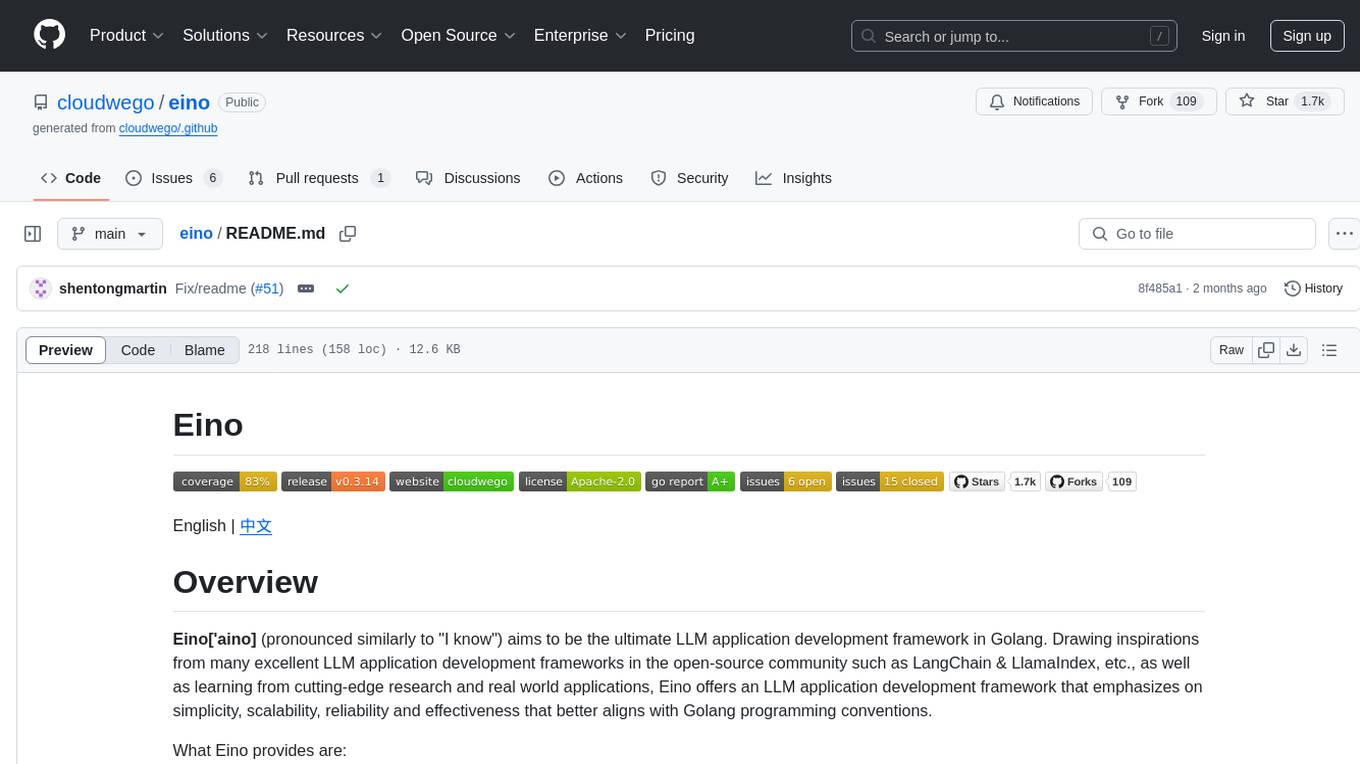
eino
Eino is an ultimate LLM application development framework in Golang, emphasizing simplicity, scalability, reliability, and effectiveness. It provides a curated list of component abstractions, a powerful composition framework, meticulously designed APIs, best practices, and tools covering the entire development cycle. Eino standardizes and improves efficiency in AI application development by offering rich components, powerful orchestration, complete stream processing, highly extensible aspects, and a comprehensive framework structure.
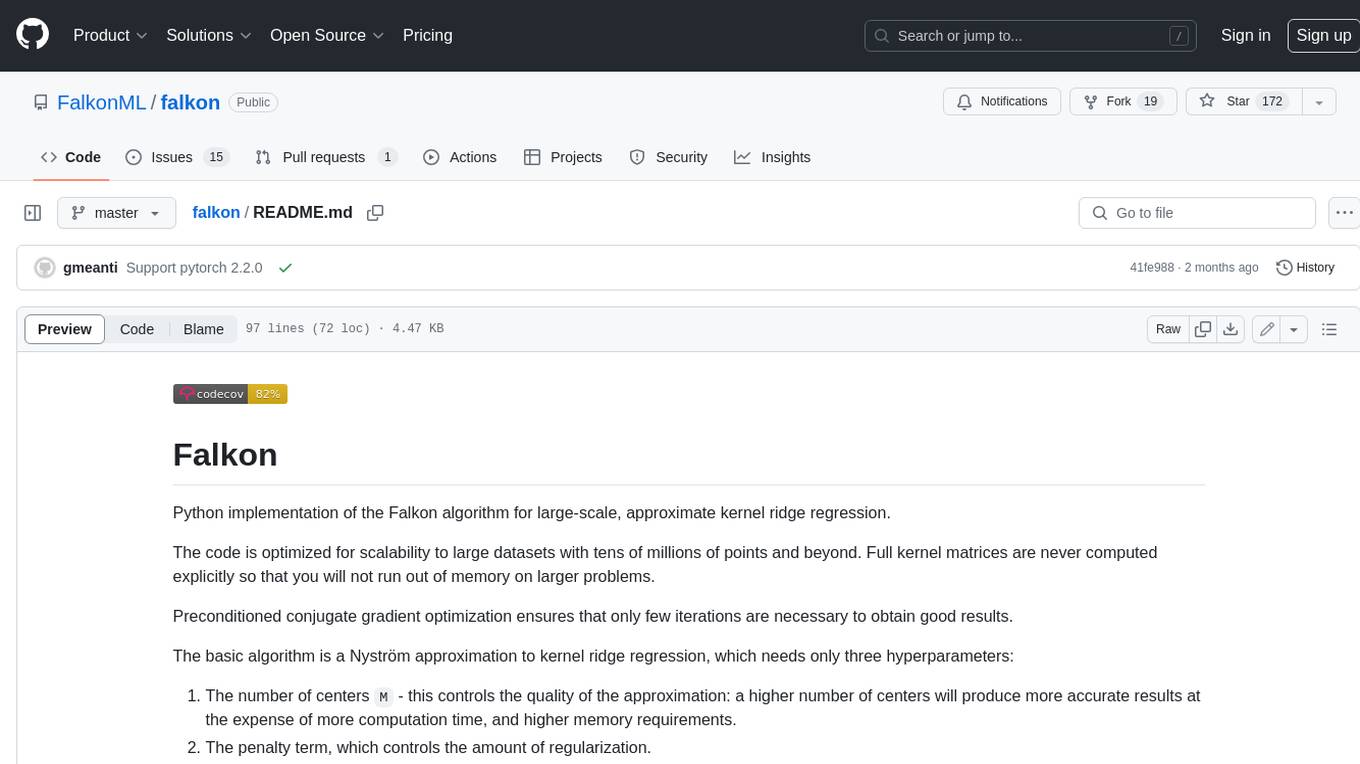
falkon
Falkon is a Python implementation of the Falkon algorithm for large-scale, approximate kernel ridge regression. The code is optimized for scalability to large datasets with tens of millions of points and beyond. Full kernel matrices are never computed explicitly so that you will not run out of memory on larger problems. Preconditioned conjugate gradient optimization ensures that only few iterations are necessary to obtain good results. The basic algorithm is a Nyström approximation to kernel ridge regression, which needs only three hyperparameters: 1. The number of centers M - this controls the quality of the approximation: a higher number of centers will produce more accurate results at the expense of more computation time, and higher memory requirements. 2. The penalty term, which controls the amount of regularization. 3. The kernel function. A good default is always the Gaussian (RBF) kernel (`falkon.kernels.GaussianKernel`).
For similar tasks
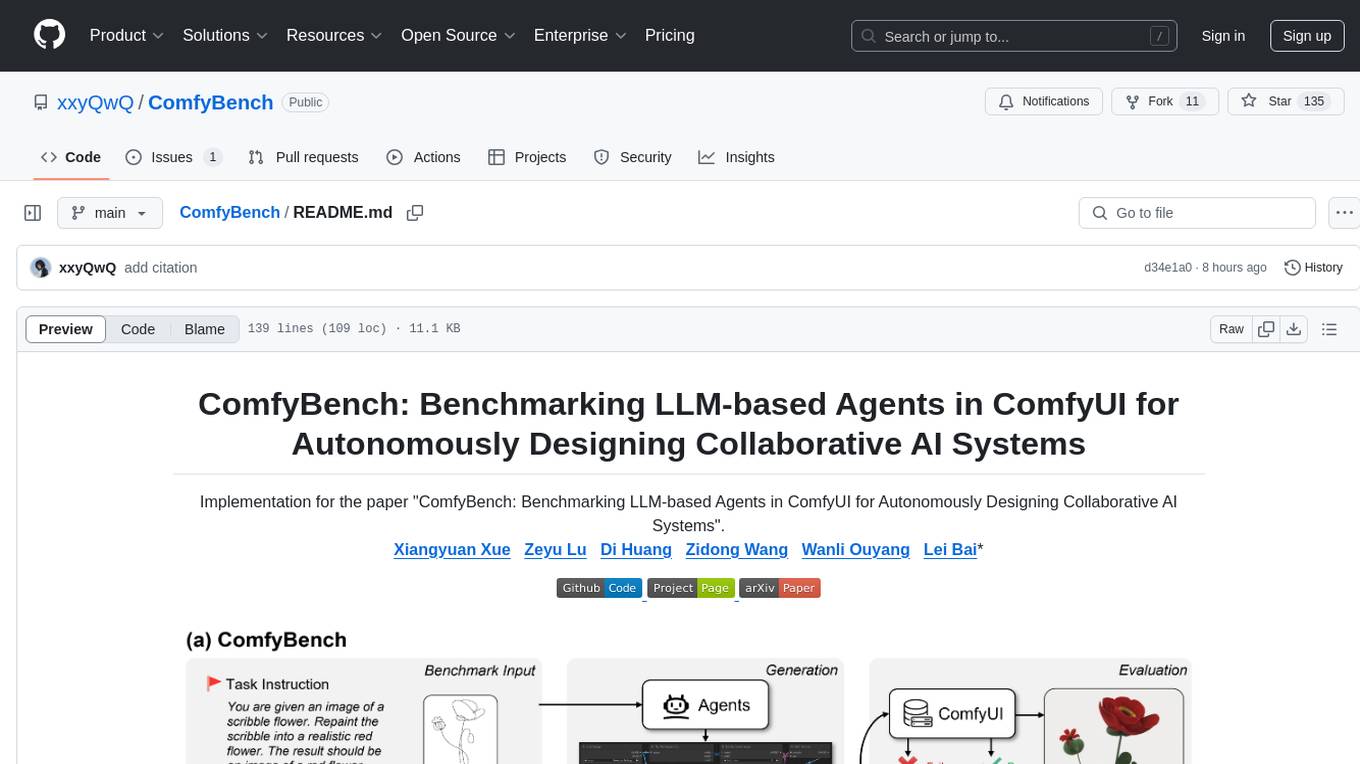
ComfyBench
ComfyBench is a comprehensive benchmark tool designed to evaluate agents' ability to design collaborative AI systems in ComfyUI. It provides tasks for agents to learn from documents and create workflows, which are then converted into code for better understanding by LLMs. The tool measures performance based on pass rate and resolve rate, reflecting the correctness of workflow execution and task realization. ComfyAgent, a component of ComfyBench, autonomously designs new workflows by learning from existing ones, interpreting them as collaborative AI systems to complete given tasks.
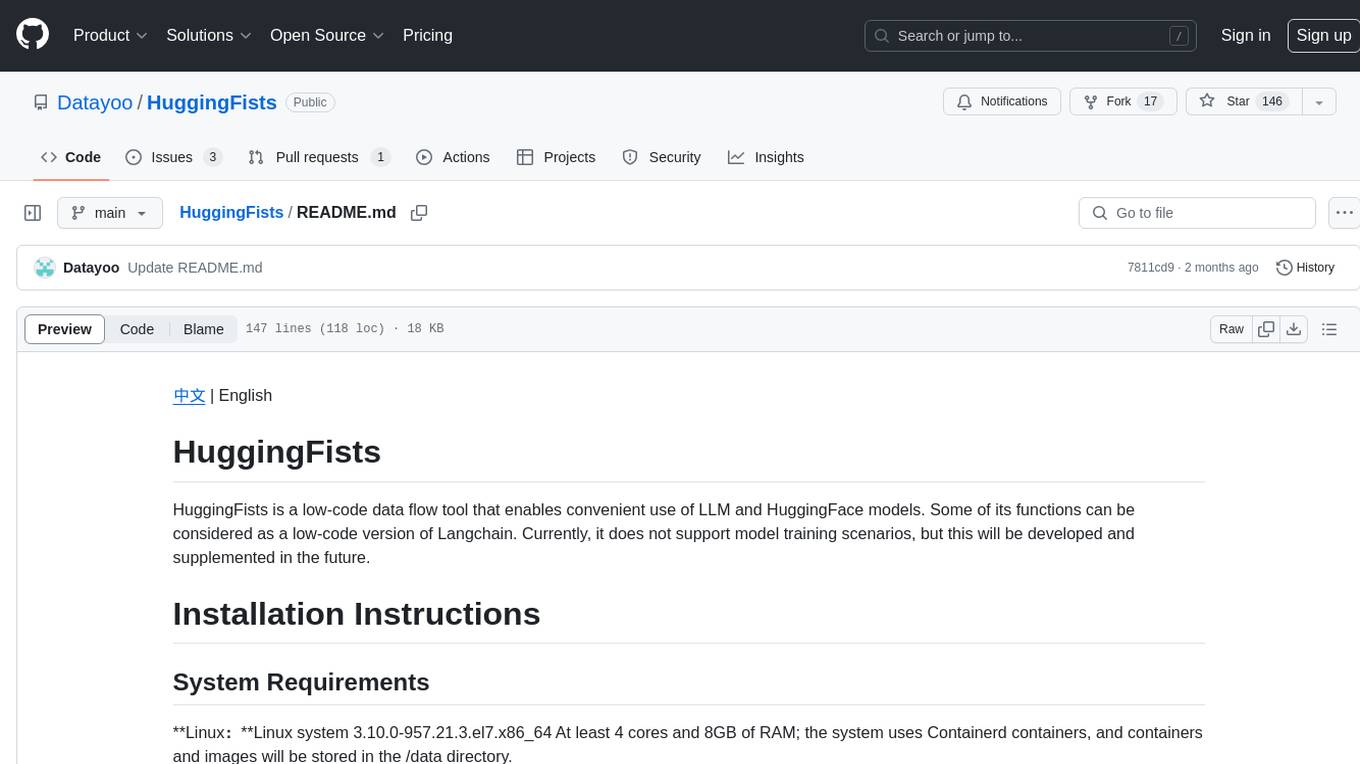
HuggingFists
HuggingFists is a low-code data flow tool that enables convenient use of LLM and HuggingFace models. It provides functionalities similar to Langchain, allowing users to design, debug, and manage data processing workflows, create and schedule workflow jobs, manage resources environment, and handle various data artifact resources. The tool also offers account management for users, allowing centralized management of data source accounts and API accounts. Users can access Hugging Face models through the Inference API or locally deployed models, as well as datasets on Hugging Face. HuggingFists supports breakpoint debugging, branch selection, function calls, workflow variables, and more to assist users in developing complex data processing workflows.
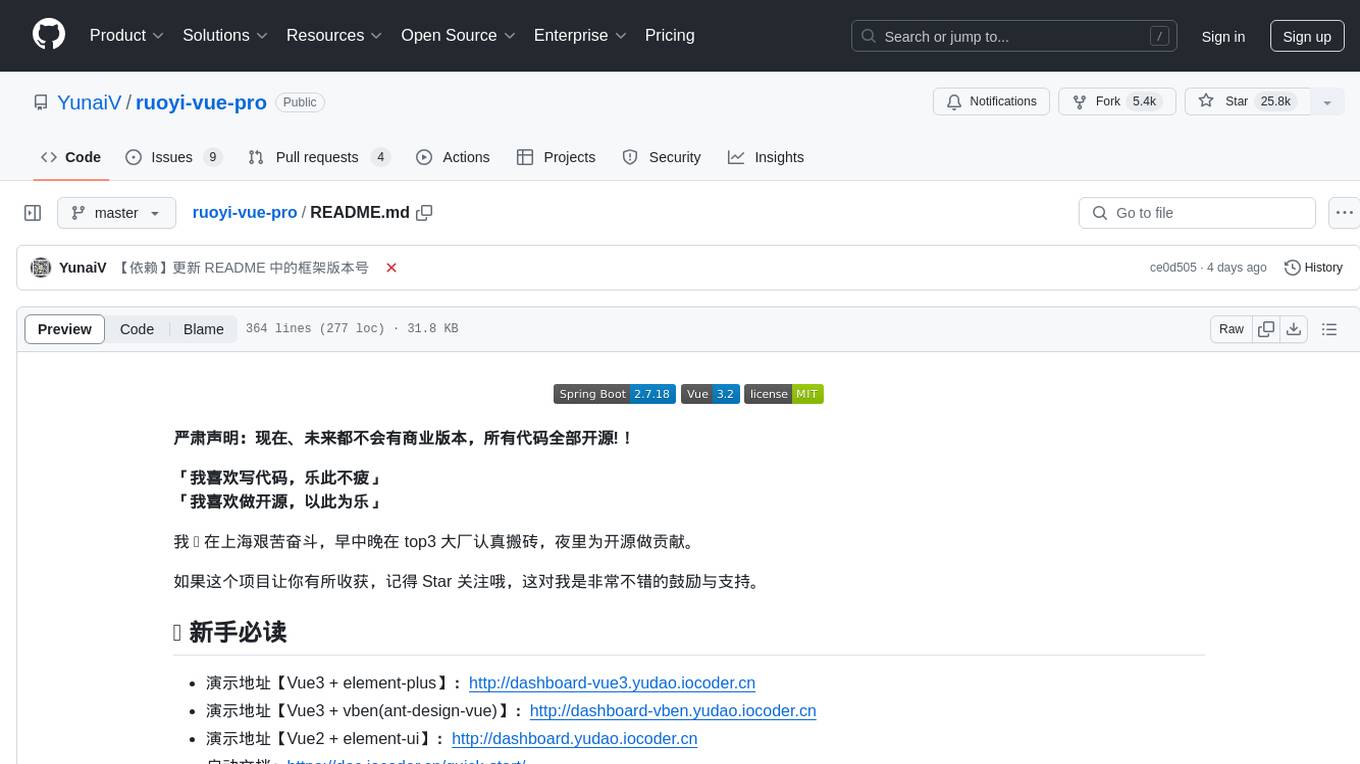
ruoyi-vue-pro
The ruoyi-vue-pro repository is an open-source project that provides a comprehensive development platform with various functionalities such as system features, infrastructure, member center, data reports, workflow, payment system, mall system, ERP system, CRM system, and AI big model. It is built using Java backend with Spring Boot framework and Vue frontend with different versions like Vue3 with element-plus, Vue3 with vben(ant-design-vue), and Vue2 with element-ui. The project aims to offer a fast development platform for developers and enterprises, supporting features like dynamic menu loading, button-level access control, SaaS multi-tenancy, code generator, real-time communication, integration with third-party services like WeChat, Alipay, and cloud services, and more.
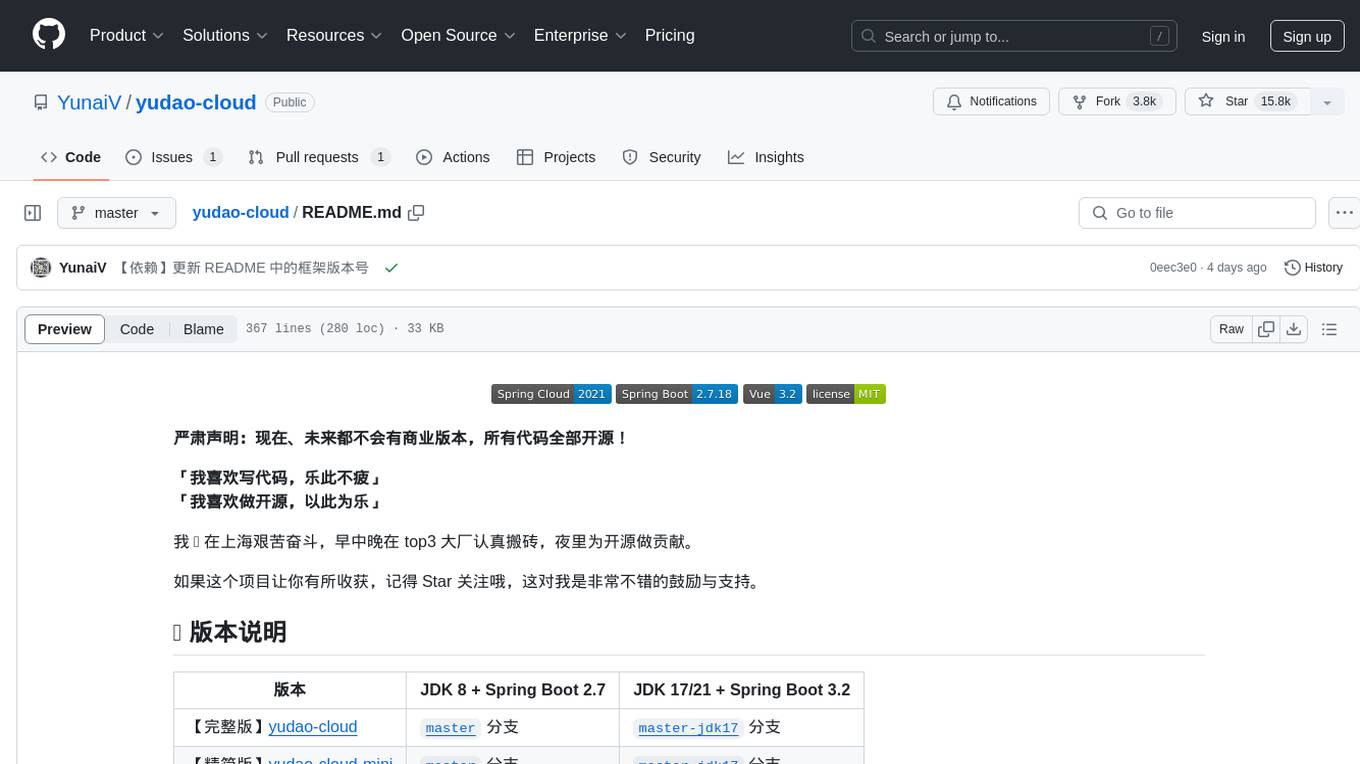
yudao-cloud
Yudao-cloud is an open-source project designed to provide a fast development platform for developers in China. It includes various system functions, infrastructure, member center, data reports, workflow, mall system, WeChat public account, CRM, ERP, etc. The project is based on Java backend with Spring Boot and Spring Cloud Alibaba microservices architecture. It supports multiple databases, message queues, authentication systems, dynamic menu loading, SaaS multi-tenant system, code generator, real-time communication, integration with third-party services like WeChat, Alipay, and more. The project is well-documented and follows the Alibaba Java development guidelines, ensuring clean code and architecture.
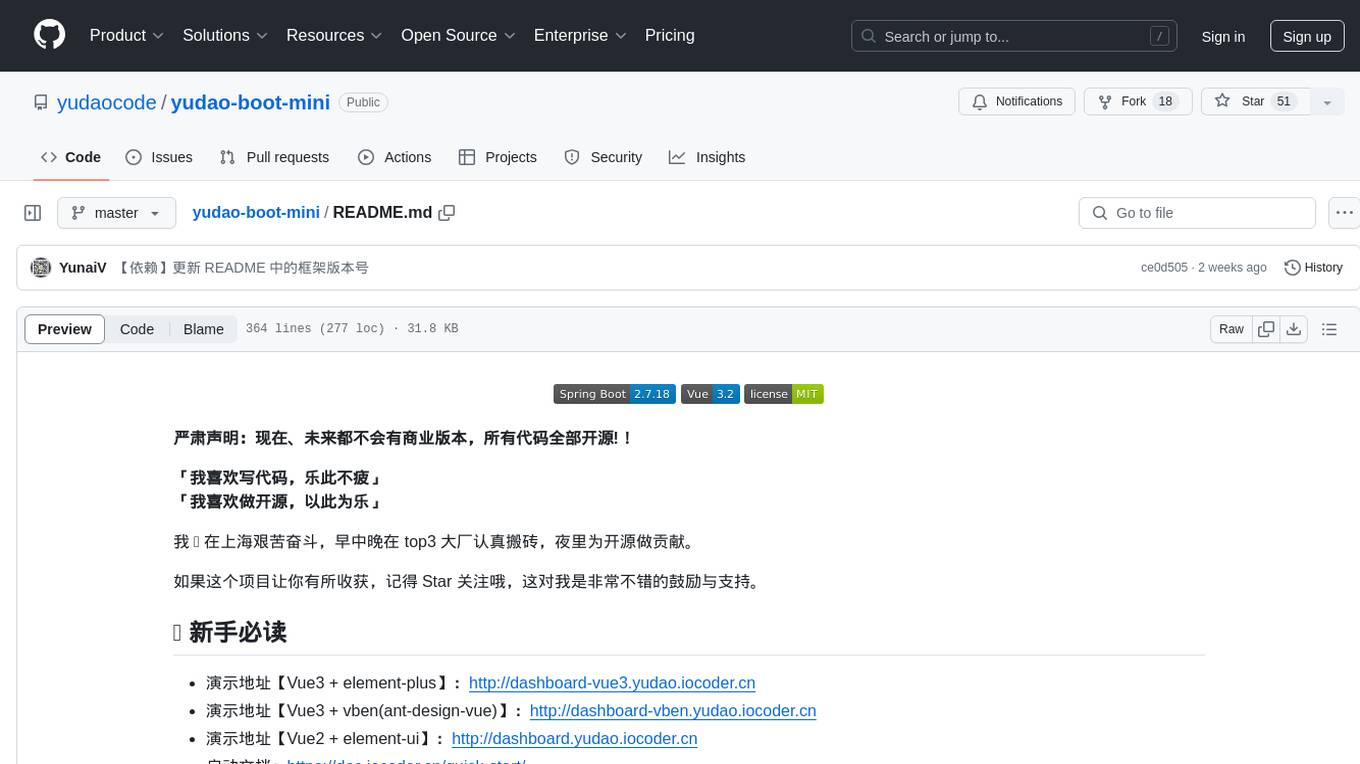
yudao-boot-mini
yudao-boot-mini is an open-source project focused on developing a rapid development platform for developers in China. It includes features like system functions, infrastructure, member center, data reports, workflow, mall system, WeChat official account, CRM, ERP, etc. The project is based on Spring Boot with Java backend and Vue for frontend. It offers various functionalities such as user management, role management, menu management, department management, workflow management, payment system, code generation, API documentation, database documentation, file service, WebSocket integration, message queue, Java monitoring, and more. The project is licensed under the MIT License, allowing both individuals and enterprises to use it freely without restrictions.
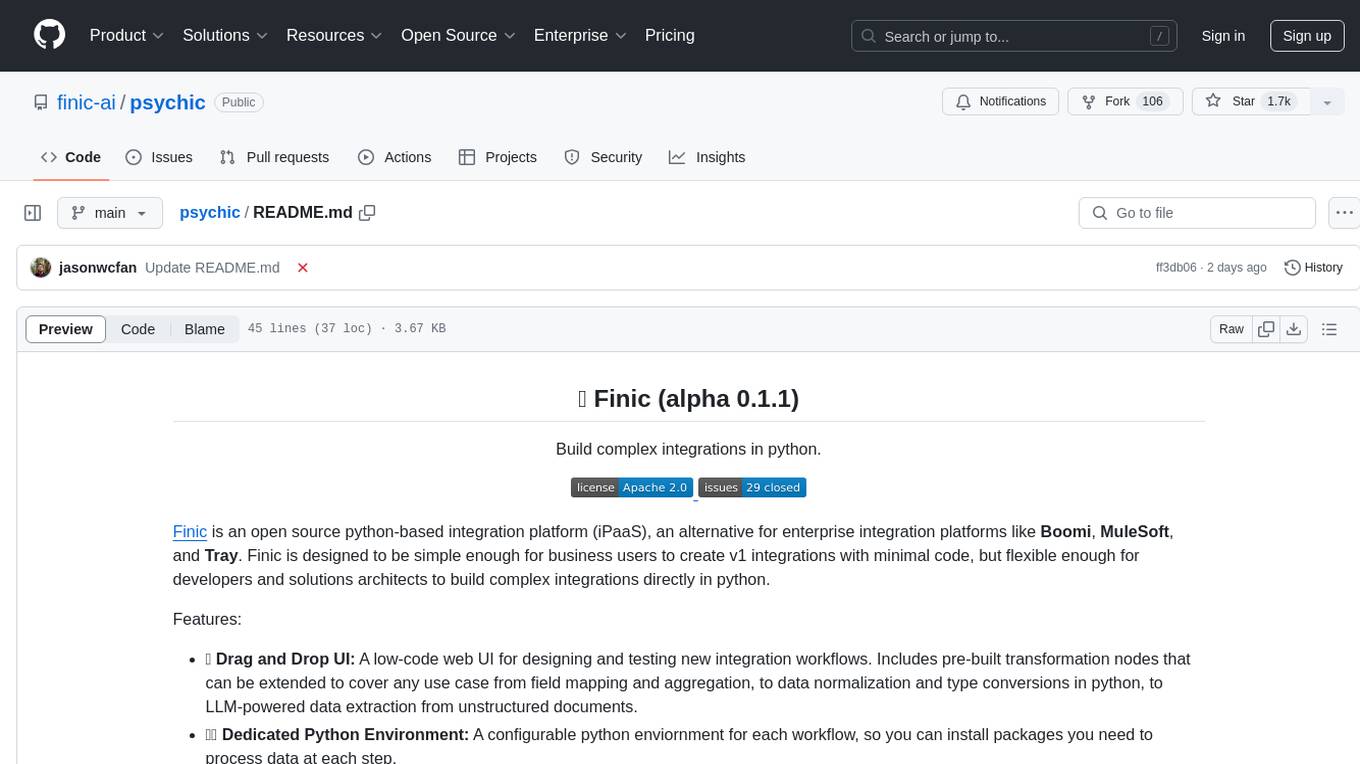
psychic
Finic is an open source python-based integration platform designed to simplify integration workflows for both business users and developers. It offers a drag-and-drop UI, a dedicated Python environment for each workflow, and generative AI features to streamline transformation tasks. With a focus on decoupling integration from product code, Finic aims to provide faster and more flexible integrations by supporting custom connectors. The tool is open source and allows deployment to users' own cloud environments with minimal legal friction.
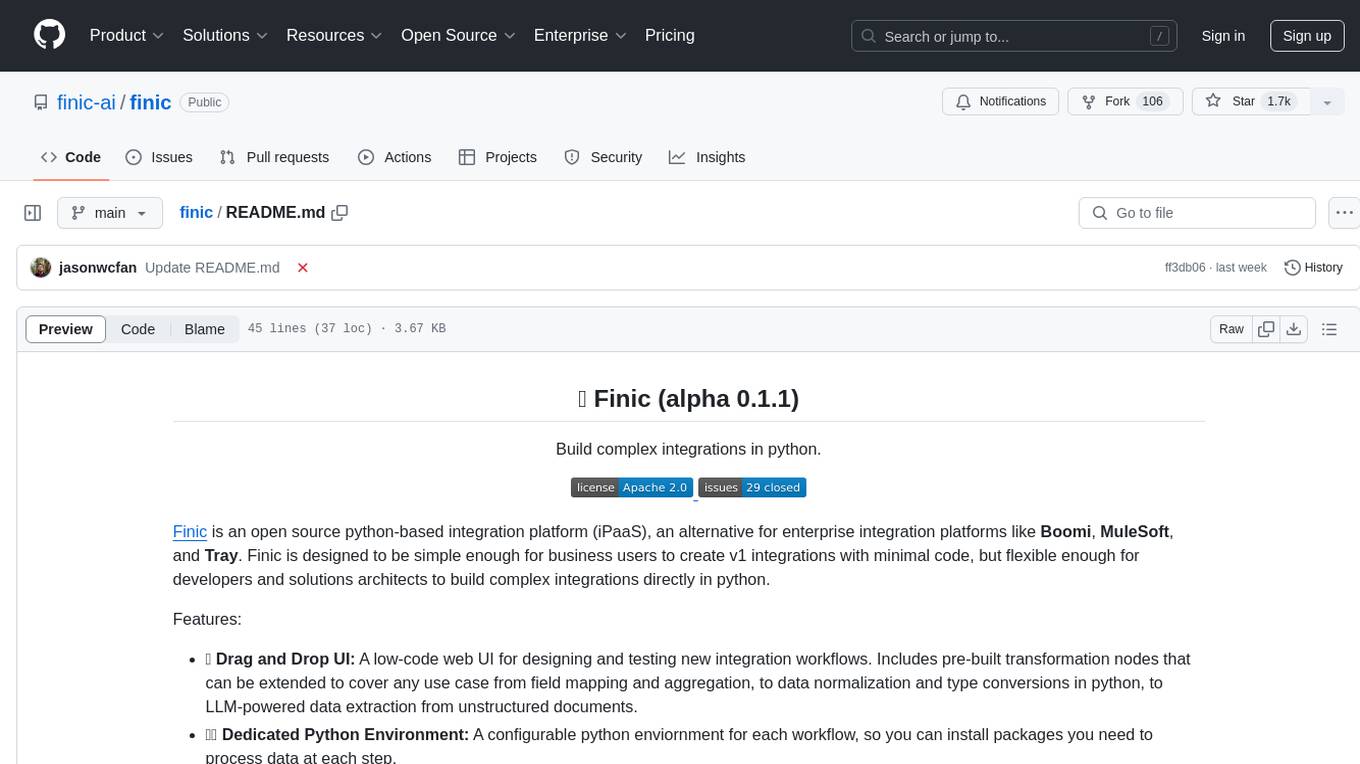
finic
Finic is an open source python-based integration platform designed for business users to create v1 integrations with minimal code, while also being flexible for developers to build complex integrations directly in python. It offers a low-code web UI, a dedicated Python environment for each workflow, and generative AI features. Finic decouples integration from product code, supports custom connectors, and is open source. It is not an ETL tool but focuses on integrating functionality between applications via APIs or SFTP, and it is not a workflow automation tool optimized for complex use cases.
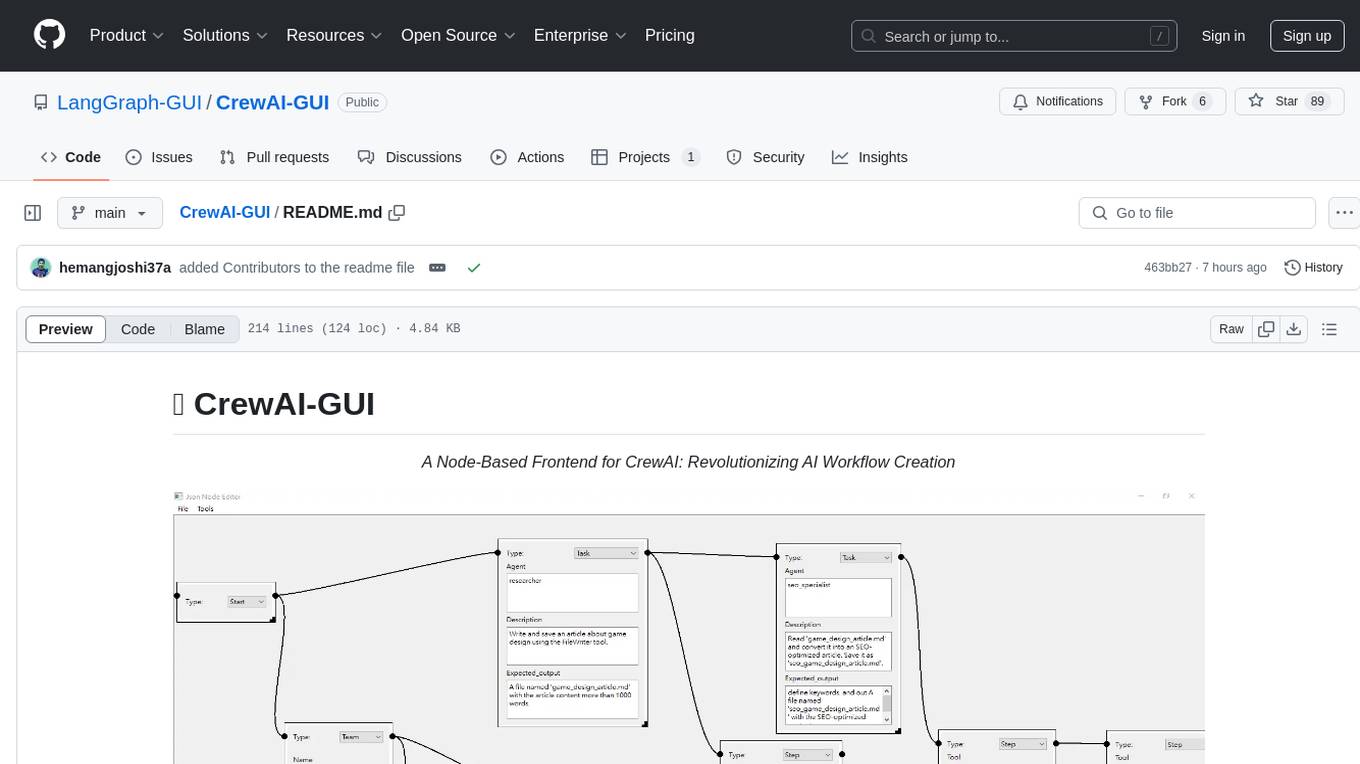
CrewAI-GUI
CrewAI-GUI is a Node-Based Frontend tool designed to revolutionize AI workflow creation. It empowers users to design complex AI agent interactions through an intuitive drag-and-drop interface, export designs to JSON for modularity and reusability, and supports both GPT-4 API and Ollama for flexible AI backend. The tool ensures cross-platform compatibility, allowing users to create AI workflows on Windows, Linux, or macOS efficiently.
For similar jobs
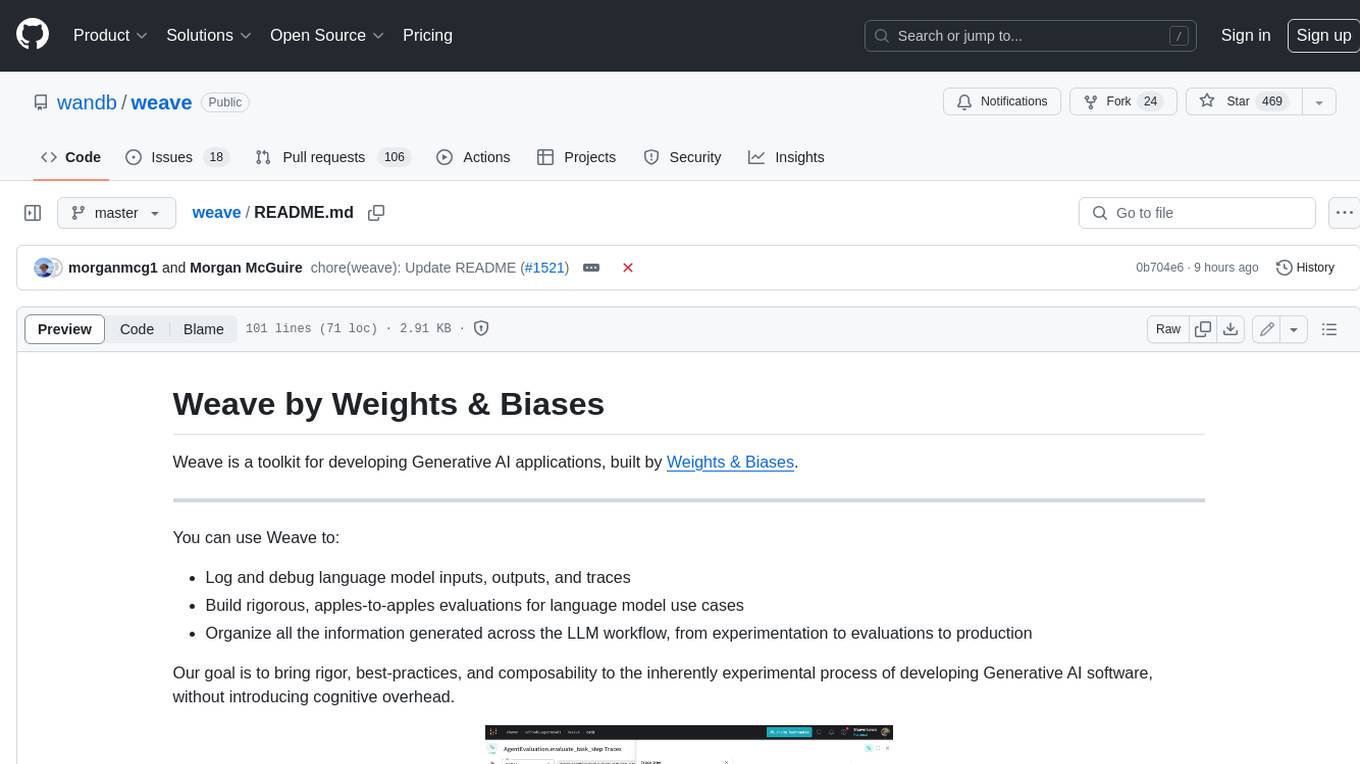
weave
Weave is a toolkit for developing Generative AI applications, built by Weights & Biases. With Weave, you can log and debug language model inputs, outputs, and traces; build rigorous, apples-to-apples evaluations for language model use cases; and organize all the information generated across the LLM workflow, from experimentation to evaluations to production. Weave aims to bring rigor, best-practices, and composability to the inherently experimental process of developing Generative AI software, without introducing cognitive overhead.
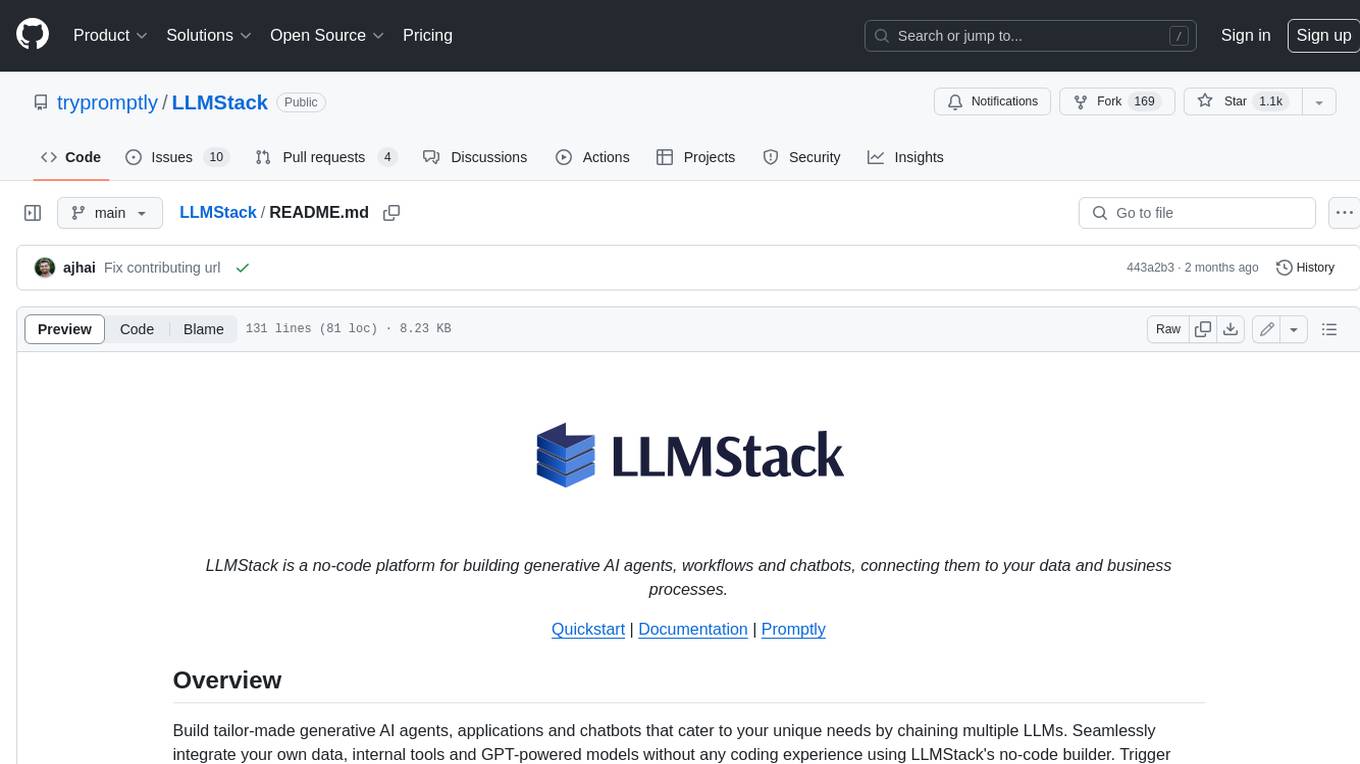
LLMStack
LLMStack is a no-code platform for building generative AI agents, workflows, and chatbots. It allows users to connect their own data, internal tools, and GPT-powered models without any coding experience. LLMStack can be deployed to the cloud or on-premise and can be accessed via HTTP API or triggered from Slack or Discord.
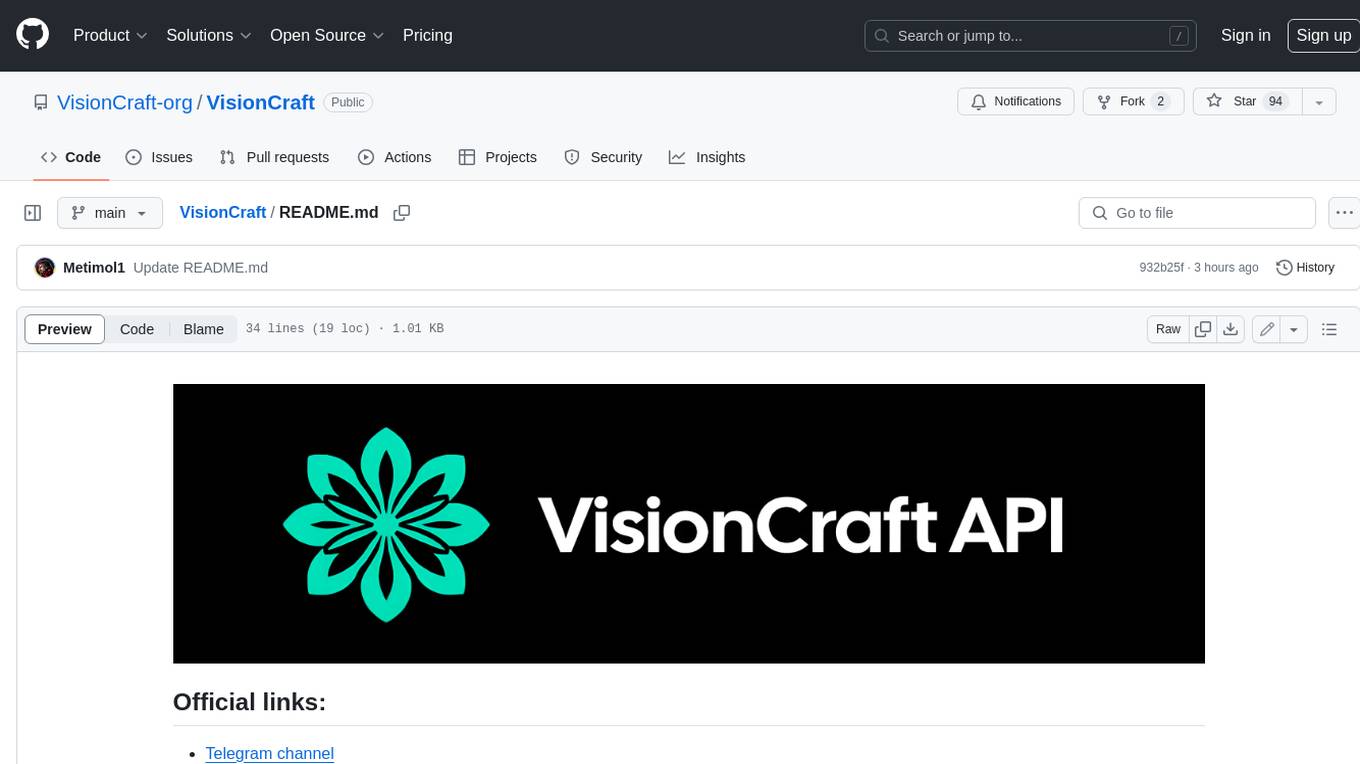
VisionCraft
The VisionCraft API is a free API for using over 100 different AI models. From images to sound.
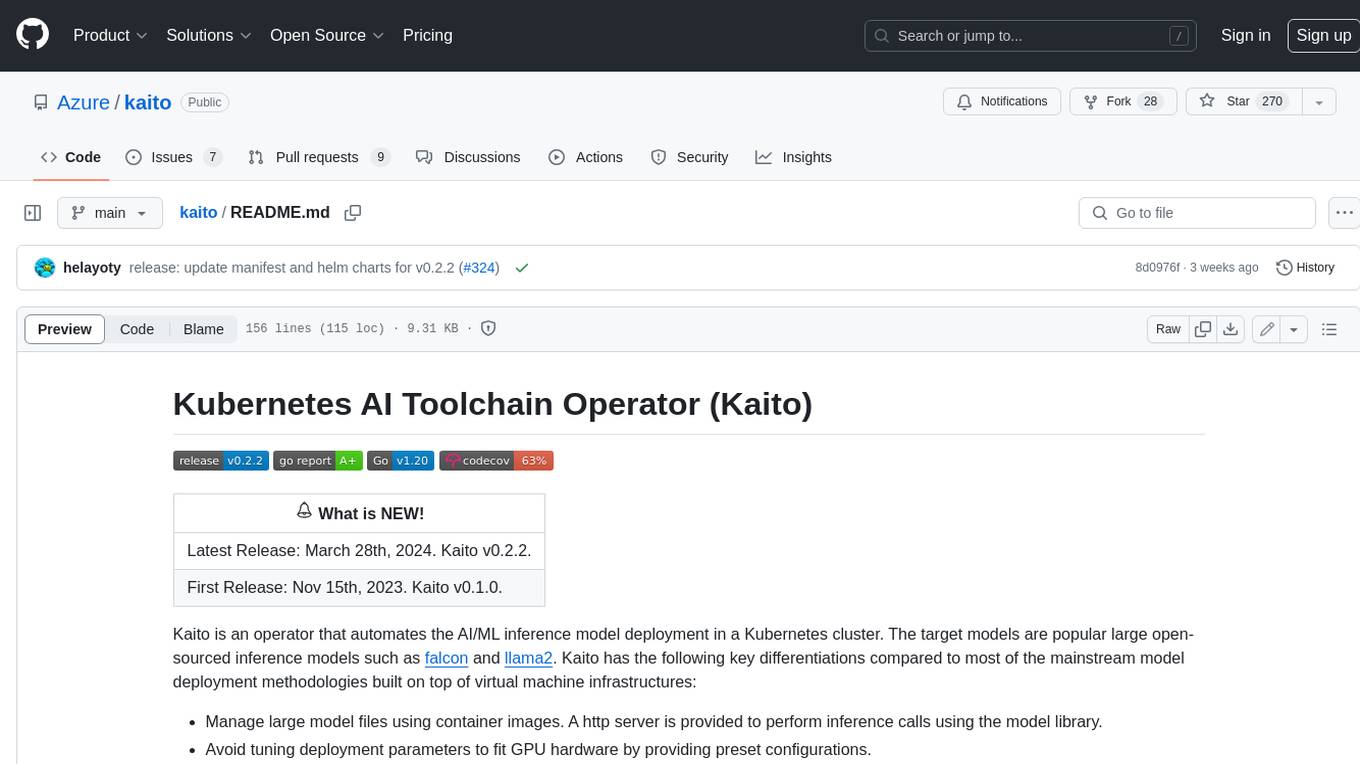
kaito
Kaito is an operator that automates the AI/ML inference model deployment in a Kubernetes cluster. It manages large model files using container images, avoids tuning deployment parameters to fit GPU hardware by providing preset configurations, auto-provisions GPU nodes based on model requirements, and hosts large model images in the public Microsoft Container Registry (MCR) if the license allows. Using Kaito, the workflow of onboarding large AI inference models in Kubernetes is largely simplified.
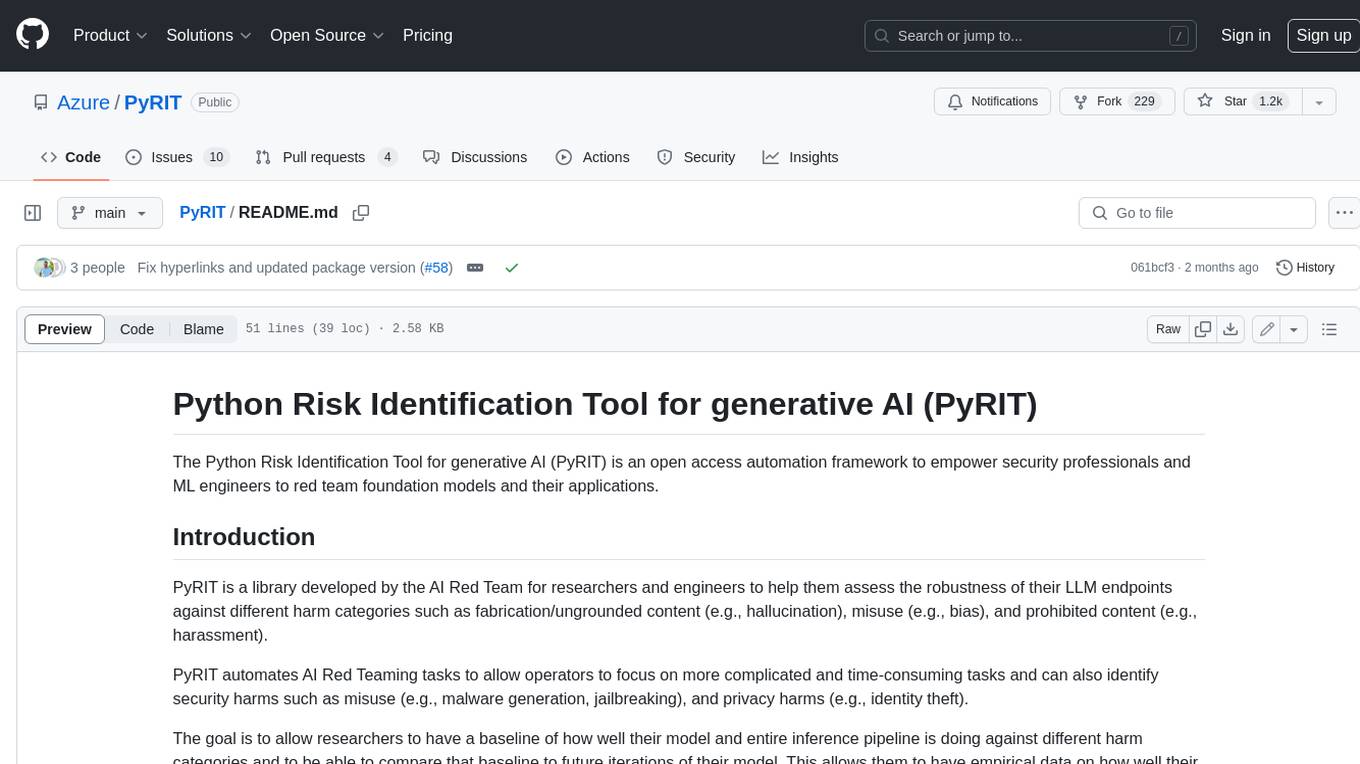
PyRIT
PyRIT is an open access automation framework designed to empower security professionals and ML engineers to red team foundation models and their applications. It automates AI Red Teaming tasks to allow operators to focus on more complicated and time-consuming tasks and can also identify security harms such as misuse (e.g., malware generation, jailbreaking), and privacy harms (e.g., identity theft). The goal is to allow researchers to have a baseline of how well their model and entire inference pipeline is doing against different harm categories and to be able to compare that baseline to future iterations of their model. This allows them to have empirical data on how well their model is doing today, and detect any degradation of performance based on future improvements.
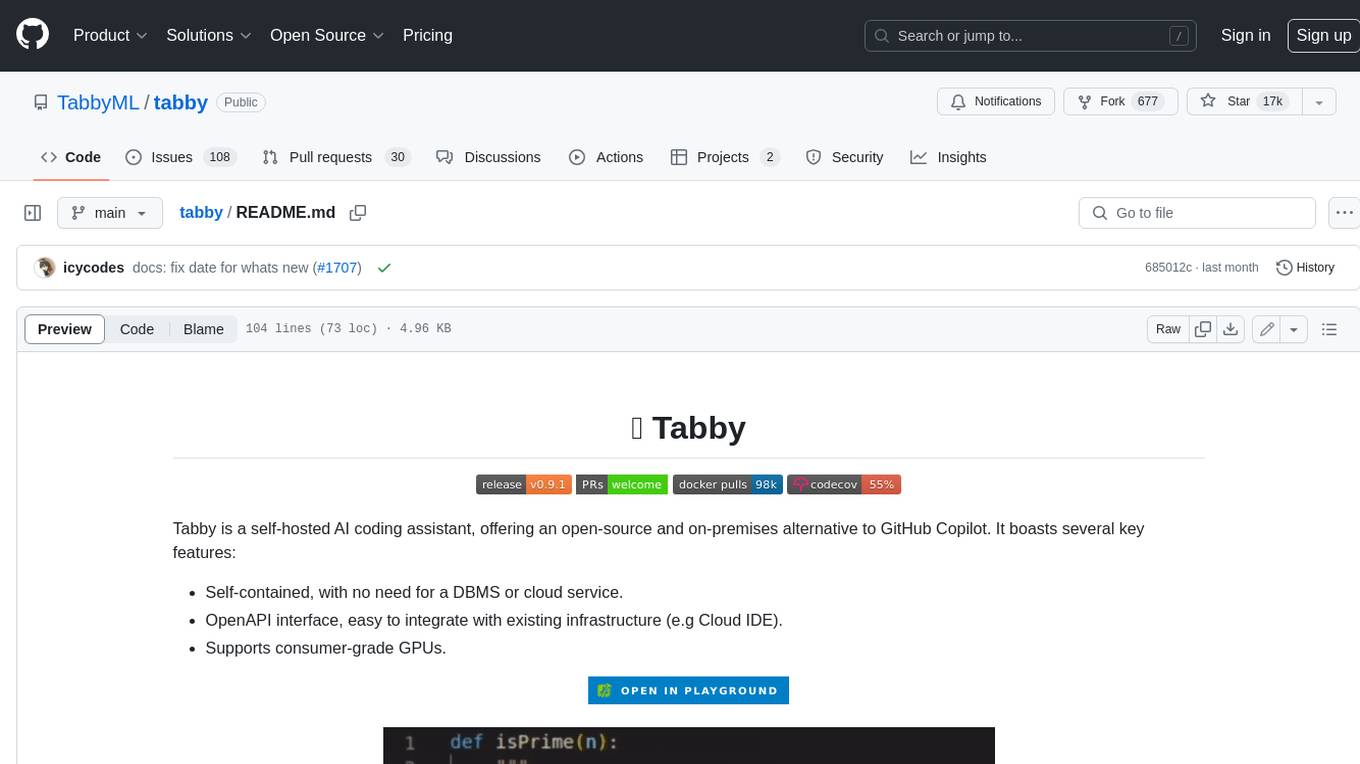
tabby
Tabby is a self-hosted AI coding assistant, offering an open-source and on-premises alternative to GitHub Copilot. It boasts several key features: * Self-contained, with no need for a DBMS or cloud service. * OpenAPI interface, easy to integrate with existing infrastructure (e.g Cloud IDE). * Supports consumer-grade GPUs.
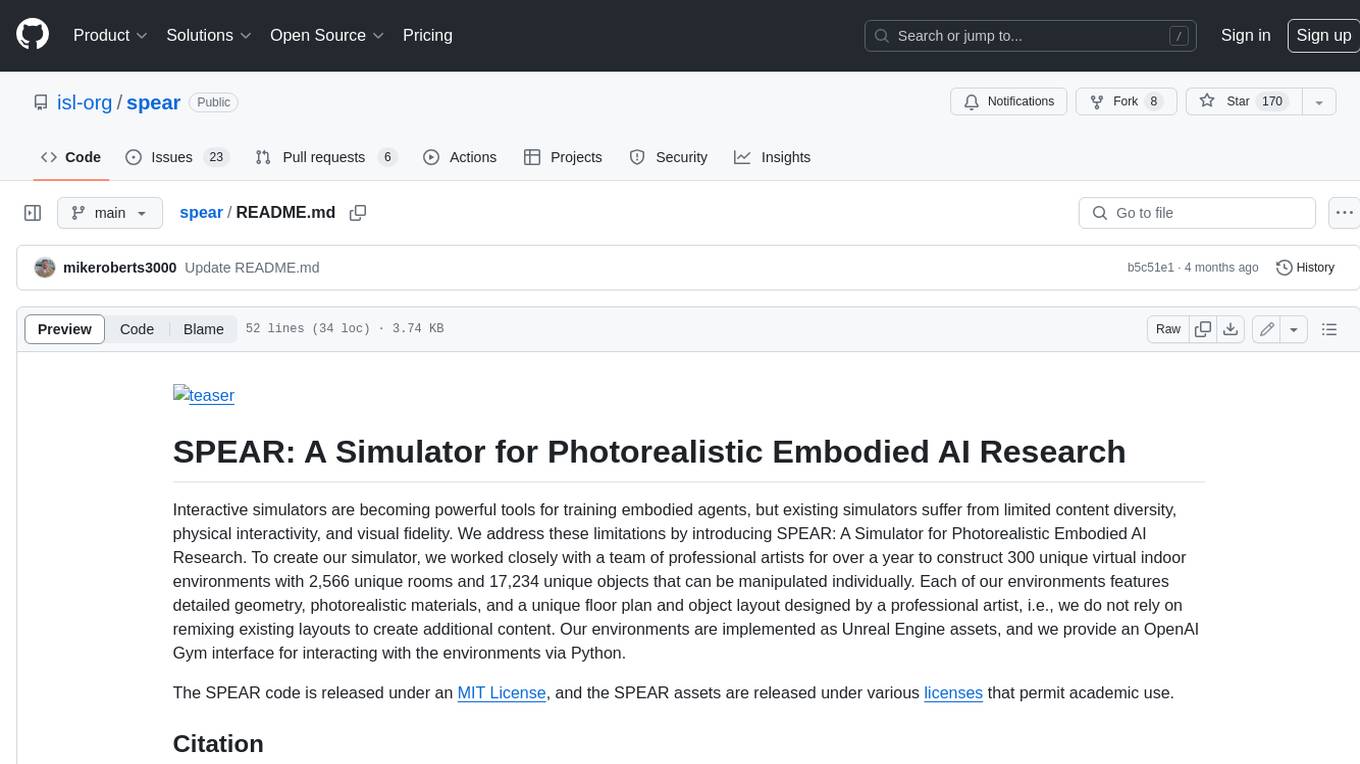
spear
SPEAR (Simulator for Photorealistic Embodied AI Research) is a powerful tool for training embodied agents. It features 300 unique virtual indoor environments with 2,566 unique rooms and 17,234 unique objects that can be manipulated individually. Each environment is designed by a professional artist and features detailed geometry, photorealistic materials, and a unique floor plan and object layout. SPEAR is implemented as Unreal Engine assets and provides an OpenAI Gym interface for interacting with the environments via Python.
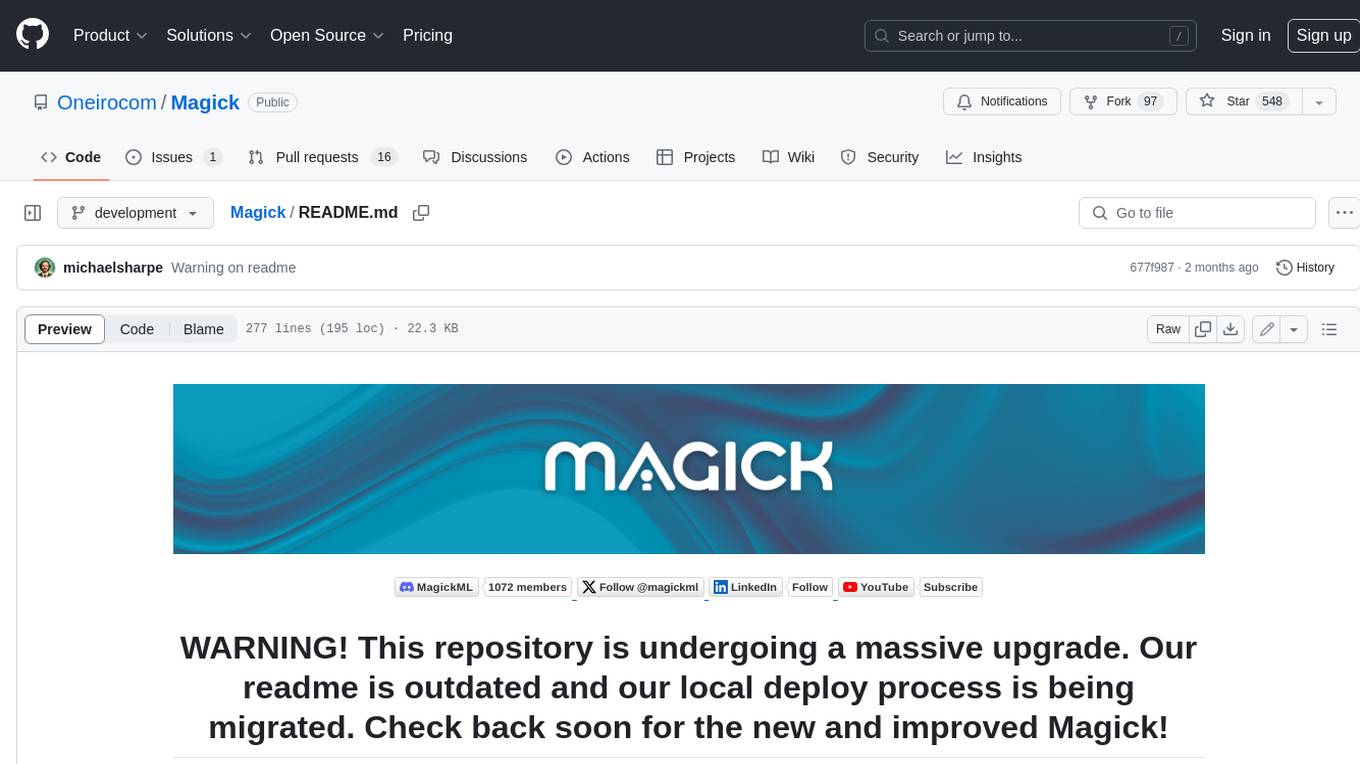
Magick
Magick is a groundbreaking visual AIDE (Artificial Intelligence Development Environment) for no-code data pipelines and multimodal agents. Magick can connect to other services and comes with nodes and templates well-suited for intelligent agents, chatbots, complex reasoning systems and realistic characters.