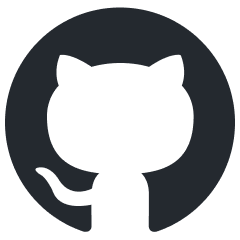
LLaMa2lang
Convenience scripts to finetune (chat-)LLaMa3 and other models for any language
Stars: 217
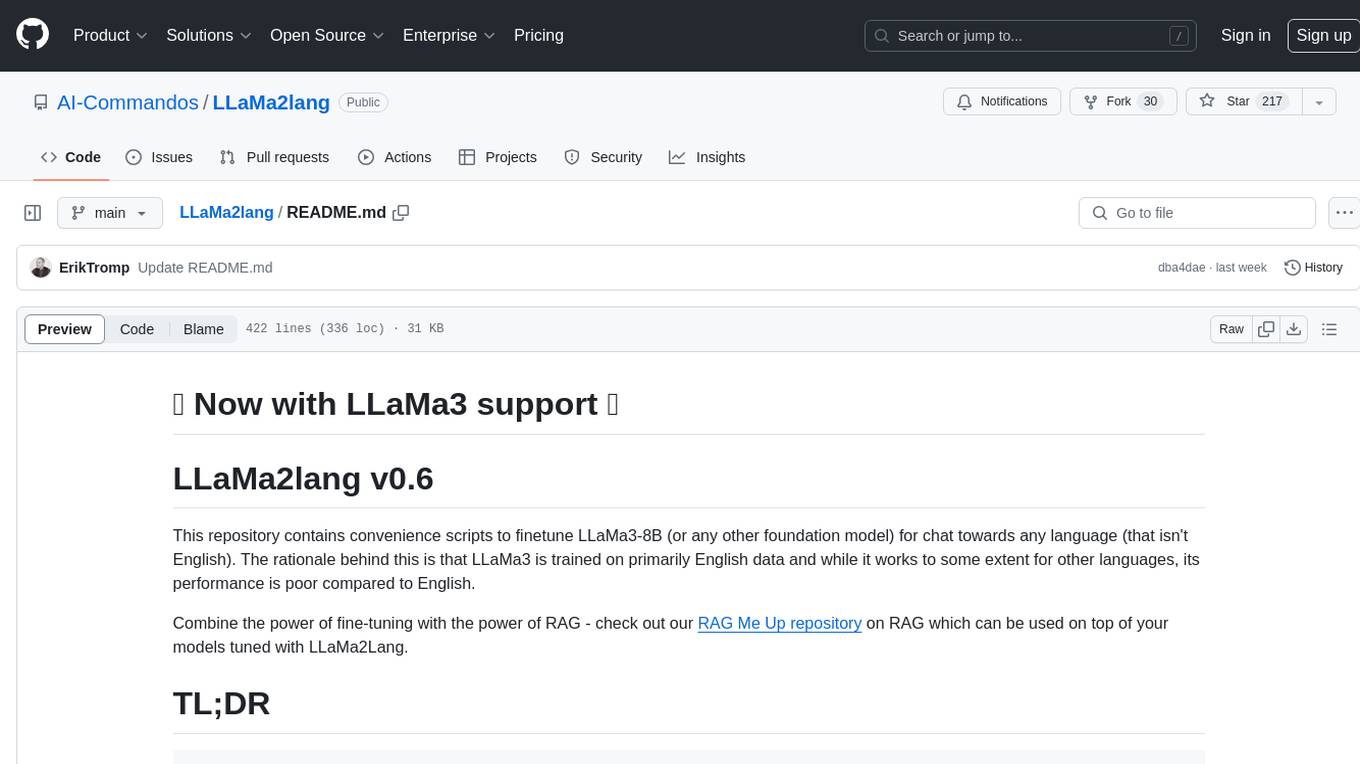
LLaMa2lang is a repository containing convenience scripts to finetune LLaMa3-8B (or any other foundation model) for chat towards any language that isn't English. The repository aims to improve the performance of LLaMa3 for non-English languages by combining fine-tuning with RAG. Users can translate datasets, extract threads, turn threads into prompts, and finetune models using QLoRA and PEFT. Additionally, the repository supports translation models like OPUS, M2M, MADLAD, and base datasets like OASST1 and OASST2. The process involves loading datasets, translating them, combining checkpoints, and running inference using the newly trained model. The repository also provides benchmarking scripts to choose the right translation model for a target language.
README:
This repository contains convenience scripts to finetune LLaMa3-8B (or any other foundation model) for chat towards any language (that isn't English). The rationale behind this is that LLaMa3 is trained on primarily English data and while it works to some extent for other languages, its performance is poor compared to English.
Combine the power of fine-tuning with the power of RAG - check out our RAG Me Up repository on RAG which can be used on top of your models tuned with LLaMa2Lang.
pip install -r requirements.txt
# Translate OASST1 to target language
python translate.py m2m target_lang checkpoint_location
# Combine the checkpoint files into a dataset
python combine_checkpoints.py input_folder output_location
# Finetune
python finetune.py tuned_model dataset_name instruction_prompt
# Optionally finetune with DPO (RLHF)
python finetune_dpo.py tuned_model dataset_name instruction_prompt
# Run inference
python run_inference.py model_name instruction_prompt input
The process we follow to tune a foundation model such as LLaMa3 for a specific language is as follows:
- Load a dataset that contains Q&A/instruction pairs.
- Translate the entire dataset to a given target language.
- Load the translated dataset and extract threads by recursively selecting prompts with their respective answers with the highest rank only, through to subsequent prompts, etc.
- Turn the threads into prompts following a given template (customizable).
- Use QLoRA and PEFT to finetune a base foundation model's instruct finetune on this dataset.
- Run inference using the newly trained model.
- OPUS
- M2M
- MADLAD
- mBART
- NLLB
- Seamless (Large only)
- Tower Instruct (Can correct spelling mistakes)
The following have been tested but potentially more will work
- OASST1
- OASST2
- LLaMa3
- LLaMa2
- Mistral
- (Unofficial) Mixtral 8x7B
- [L2L-6] Investigate interoperability with other libraries (Axolotl, llamacpp, unsloth)
- [L2L-7] Allow for different quantizations next to QLoRA (GGUF, GPTQ, AWQ)
- [L2L-10] Support extending the tokenizer and vocabulary
The above process can be fully run on a free Google Colab T4 GPU. The last step however, can only be successfully run with short enough context windows and a batch of at most 2. In addition, the translation in step 2 takes about 36 hours in total for any given language so should be run in multiple steps if you want to stick with a free Google Colab GPU.
Our fine-tuned models for step 5 were performed using an A40 on vast.ai and cost us less than a dollar for each model, completing in about 1.5 hours.
-
Make sure pytorch is installed and working for your environment (use of CUDA preferable): https://pytorch.org/get-started/locally/
-
Clone the repo and install the requirements.
pip install -r requirements.txt
- Translate your base dataset to your designated target language.
usage: translate.py [-h] [--quant8] [--quant4] [--base_dataset BASE_DATASET] [--base_dataset_text_field BASE_DATASET_TEXT_FIELD] [--base_dataset_lang_field BASE_DATASET_LANG_FIELD]
[--checkpoint_n CHECKPOINT_N] [--batch_size BATCH_SIZE] [--max_length MAX_LENGTH] [--cpu] [--source_lang SOURCE_LANG]
{opus,mbart,madlad,m2m,nllb,seamless_m4t_v2,towerinstruct} ... target_lang checkpoint_location
Translate an instruct/RLHF dataset to a given target language using a variety of translation models
positional arguments:
{opus,mbart,madlad,m2m,nllb,seamless_m4t_v2,towerinstruct}
The model/architecture used for translation.
opus Translate the dataset using HelsinkiNLP OPUS models.
mbart Translate the dataset using mBART.
madlad Translate the dataset using Google's MADLAD models.
m2m Translate the dataset using Facebook's M2M models.
nllb Translate the dataset using Facebook's NLLB models.
seamless_m4t_v2 Translate the dataset using Facebook's SeamlessM4T-v2 multimodal models.
towerinstruct Translate the dataset using Unbabel's Tower Instruct. Make sure your target language is in the 10 languages supported by the model.
target_lang The target language. Make sure you use language codes defined by the translation model you are using.
checkpoint_location The folder the script will write (JSONized) checkpoint files to. Folder will be created if it doesn't exist.
options:
-h, --help show this help message and exit
--quant8 Optional flag to load the translation model in 8 bits. Decreases memory usage, increases running time
--quant4 Optional flag to load the translation model in 4 bits. Decreases memory usage, increases running time
--base_dataset BASE_DATASET
The base dataset to translate, defaults to OpenAssistant/oasst1
--base_dataset_text_field BASE_DATASET_TEXT_FIELD
The base dataset's column name containing the actual text to translate. Defaults to text
--base_dataset_lang_field BASE_DATASET_LANG_FIELD
The base dataset's column name containing the language the source text was written in. Defaults to lang
--checkpoint_n CHECKPOINT_N
An integer representing how often a checkpoint file will be written out. To start off, 400 is a reasonable number.
--batch_size BATCH_SIZE
The batch size for a single translation model. Adjust based on your GPU capacity. Default is 10.
--max_length MAX_LENGTH
How much tokens to generate at most. More tokens might be more accurate for lengthy input but creates a risk of running out of memory. Default is unlimited.
--cpu Forces usage of CPU. By default GPU is taken if available.
--source_lang SOURCE_LANG
Source language to select from OASST based on lang property of dataset
If you want more parameters for the different translation models, run:
python translate.py [MODEL] -h
Be sure to specify model-specific parameters first before you specify common parameters from the list above. Example calls:
# Using M2M with 4bit quantization and differen batch sizes to translate Dutch
python translate.py m2m nl ./output_nl --quant4 --batch_size 20
# Using madlad 7B with 8bit quantization for German with different max_length
python translate.py madlad --model_size 7b de ./output_de --quant8 --batch_size 5 --max_length 512
# Be sure to use target language codes that the model you use understands
python translate.py mbart xh_ZA ./output_xhosa
python translate.py nllb nld_Latn ./output_nl
- Combine the JSON arrays from the checkpoints' files into a Huggingface Dataset and then either write it to disk or publish it to Huggingface. The script will try to write to disk by default and fall back to publishing to Huggingface if the folder doesn't exist on disk. For publishing to Huggingface, make sure you have your
HF_TOKEN
environment variable set up as per the documentation.
usage: combine_checkpoints.py [-h] input_folder output_location
Combine checkpoint files from translation.
positional arguments:
input_folder The checkpoint folder used in translation, with the target language appended.
Example: "./output_nl".
output_location Where to write the Huggingface Dataset. Can be a disk location or a Huggingface
Dataset repository.
options:
-h, --help show this help message and exit
- Turn the translated messages into chat/instruct/prompt threads and finetune a foundate model's instruct using LoRA and PEFT.
usage: finetune.py [-h] [--base_model BASE_MODEL] [--base_dataset_text_field BASE_DATASET_TEXT_FIELD] [--base_dataset_rank_field BASE_DATASET_RANK_FIELD] [--base_dataset_id_field BASE_DATASET_ID_FIELD] [--base_dataset_parent_field BASE_DATASET_PARENT_FIELD]
[--base_dataset_role_field BASE_DATASET_ROLE_FIELD] [--quant8] [--noquant] [--max_seq_length MAX_SEQ_LENGTH] [--num_train_epochs NUM_TRAIN_EPOCHS] [--batch_size BATCH_SIZE] [--threads_output_name THREADS_OUTPUT_NAME] [--thread_template THREAD_TEMPLATE]
[--padding PADDING]
tuned_model dataset_name instruction_prompt
Finetune a base instruct/chat model using (Q)LoRA and PEFT
positional arguments:
tuned_model The name of the resulting tuned model.
dataset_name The name of the dataset to use for fine-tuning. This should be the output of the combine_checkpoints script.
instruction_prompt An instruction message added to every prompt given to the chatbot to force it to answer in the target language. Example: "You are a generic chatbot that always answers in English."
options:
-h, --help show this help message and exit
--base_model BASE_MODEL
The base foundation model. Default is "NousResearch/Meta-Llama-3-8B-Instruct".
--base_dataset_text_field BASE_DATASET_TEXT_FIELD
The dataset's column name containing the actual text to translate. Defaults to text
--base_dataset_rank_field BASE_DATASET_RANK_FIELD
The dataset's column name containing the rank of an answer given to a prompt. Defaults to rank
--base_dataset_id_field BASE_DATASET_ID_FIELD
The dataset's column name containing the id of a text. Defaults to message_id
--base_dataset_parent_field BASE_DATASET_PARENT_FIELD
The dataset's column name containing the parent id of a text. Defaults to parent_id
--base_dataset_role_field BASE_DATASET_ROLE_FIELD
The dataset's column name containing the role of the author of the text (eg. prompter, assistant). Defaults to role
--quant8 Finetunes the model in 8 bits. Requires more memory than the default 4 bit.
--noquant Do not quantize the finetuning. Requires more memory than the default 4 bit and optional 8 bit.
--max_seq_length MAX_SEQ_LENGTH
The maximum sequence length to use in finetuning. Should most likely line up with your base model's default max_seq_length. Default is 512.
--num_train_epochs NUM_TRAIN_EPOCHS
Number of epochs to use. 2 is default and has been shown to work well.
--batch_size BATCH_SIZE
The batch size to use in finetuning. Adjust to fit in your GPU vRAM. Default is 4
--threads_output_name THREADS_OUTPUT_NAME
If specified, the threads created in this script for finetuning will also be saved to disk or HuggingFace Hub.
--thread_template THREAD_TEMPLATE
A file containing the thread template to use. Default is threads/template_fefault.txt
--padding PADDING What padding to use, can be either left or right.
6.1 [OPTIONAL] Finetune using DPO (similar to RLHF)
usage: finetune_dpo.py [-h] [--base_model BASE_MODEL] [--base_dataset_text_field BASE_DATASET_TEXT_FIELD] [--base_dataset_rank_field BASE_DATASET_RANK_FIELD] [--base_dataset_id_field BASE_DATASET_ID_FIELD] [--base_dataset_parent_field BASE_DATASET_PARENT_FIELD] [--quant8]
[--noquant] [--max_seq_length MAX_SEQ_LENGTH] [--max_prompt_length MAX_PROMPT_LENGTH] [--num_train_epochs NUM_TRAIN_EPOCHS] [--batch_size BATCH_SIZE] [--threads_output_name THREADS_OUTPUT_NAME] [--thread_template THREAD_TEMPLATE] [--max_steps MAX_STEPS]
[--padding PADDING]
tuned_model dataset_name instruction_prompt
Finetune a base instruct/chat model using (Q)LoRA and PEFT using DPO (RLHF)
positional arguments:
tuned_model The name of the resulting tuned model.
dataset_name The name of the dataset to use for fine-tuning. This should be the output of the combine_checkpoints script.
instruction_prompt An instruction message added to every prompt given to the chatbot to force it to answer in the target language. Example: "You are a generic chatbot that always answers in English."
options:
-h, --help show this help message and exit
--base_model BASE_MODEL
The base foundation model. Default is "NousResearch/Meta-Llama-3-8B-Instruct".
--base_dataset_text_field BASE_DATASET_TEXT_FIELD
The dataset's column name containing the actual text to translate. Defaults to text
--base_dataset_rank_field BASE_DATASET_RANK_FIELD
The dataset's column name containing the rank of an answer given to a prompt. Defaults to rank
--base_dataset_id_field BASE_DATASET_ID_FIELD
The dataset's column name containing the id of a text. Defaults to message_id
--base_dataset_parent_field BASE_DATASET_PARENT_FIELD
The dataset's column name containing the parent id of a text. Defaults to parent_id
--quant8 Finetunes the model in 8 bits. Requires more memory than the default 4 bit.
--noquant Do not quantize the finetuning. Requires more memory than the default 4 bit and optional 8 bit.
--max_seq_length MAX_SEQ_LENGTH
The maximum sequence length to use in finetuning. Should most likely line up with your base model's default max_seq_length. Default is 512.
--max_prompt_length MAX_PROMPT_LENGTH
The maximum length of the prompts to use. Default is 512.
--num_train_epochs NUM_TRAIN_EPOCHS
Number of epochs to use. 2 is default and has been shown to work well.
--batch_size BATCH_SIZE
The batch size to use in finetuning. Adjust to fit in your GPU vRAM. Default is 4
--threads_output_name THREADS_OUTPUT_NAME
If specified, the threads created in this script for finetuning will also be saved to disk or HuggingFace Hub.
--thread_template THREAD_TEMPLATE
A file containing the thread template to use. Default is threads/template_fefault.txt
--max_steps MAX_STEPS
The maximum number of steps to run DPO for. Default is -1 which will run the data through fully for the number of epochs but this will be very time-consuming.
--padding PADDING What padding to use, can be either left or right.
6.1 [OPTIONAL] Finetune using ORPO (similar to RLHF)
usage: finetune_orpo.py [-h] [--base_model BASE_MODEL] [--base_dataset_text_field BASE_DATASET_TEXT_FIELD] [--base_dataset_rank_field BASE_DATASET_RANK_FIELD] [--base_dataset_id_field BASE_DATASET_ID_FIELD] [--base_dataset_parent_field BASE_DATASET_PARENT_FIELD] [--quant8]
[--noquant] [--max_seq_length MAX_SEQ_LENGTH] [--max_prompt_length MAX_PROMPT_LENGTH] [--num_train_epochs NUM_TRAIN_EPOCHS] [--batch_size BATCH_SIZE] [--threads_output_name THREADS_OUTPUT_NAME] [--thread_template THREAD_TEMPLATE] [--max_steps MAX_STEPS]
[--padding PADDING]
tuned_model dataset_name instruction_prompt
Finetune a base instruct/chat model using (Q)LoRA and PEFT using ORPO (RLHF)
positional arguments:
tuned_model The name of the resulting tuned model.
dataset_name The name of the dataset to use for fine-tuning. This should be the output of the combine_checkpoints script.
instruction_prompt An instruction message added to every prompt given to the chatbot to force it to answer in the target language. Example: "You are a generic chatbot that always answers in English."
options:
-h, --help show this help message and exit
--base_model BASE_MODEL
The base foundation model. Default is "NousResearch/Meta-Llama-3-8B-Instruct".
--base_dataset_text_field BASE_DATASET_TEXT_FIELD
The dataset's column name containing the actual text to translate. Defaults to text
--base_dataset_rank_field BASE_DATASET_RANK_FIELD
The dataset's column name containing the rank of an answer given to a prompt. Defaults to rank
--base_dataset_id_field BASE_DATASET_ID_FIELD
The dataset's column name containing the id of a text. Defaults to message_id
--base_dataset_parent_field BASE_DATASET_PARENT_FIELD
The dataset's column name containing the parent id of a text. Defaults to parent_id
--quant8 Finetunes the model in 8 bits. Requires more memory than the default 4 bit.
--noquant Do not quantize the finetuning. Requires more memory than the default 4 bit and optional 8 bit.
--max_seq_length MAX_SEQ_LENGTH
The maximum sequence length to use in finetuning. Should most likely line up with your base model's default max_seq_length. Default is 512.
--max_prompt_length MAX_PROMPT_LENGTH
The maximum length of the prompts to use. Default is 512.
--num_train_epochs NUM_TRAIN_EPOCHS
Number of epochs to use. 2 is default and has been shown to work well.
--batch_size BATCH_SIZE
The batch size to use in finetuning. Adjust to fit in your GPU vRAM. Default is 4
--threads_output_name THREADS_OUTPUT_NAME
If specified, the threads created in this script for finetuning will also be saved to disk or HuggingFace Hub.
--thread_template THREAD_TEMPLATE
A file containing the thread template to use. Default is threads/template_fefault.txt
--max_steps MAX_STEPS
The maximum number of steps to run ORPO for. Default is -1 which will run the data through fully for the number of epochs but this will be very time-consuming.
--padding PADDING What padding to use, can be either left or right.
- Run inference using the newly created QLoRA model.
usage: run_inference.py [-h] model_name instruction_prompt input
Script to run inference on a tuned model.
positional arguments:
model_name The name of the tuned model that you pushed to Huggingface in the previous
step.
instruction_prompt An instruction message added to every prompt given to the chatbot to force
it to answer in the target language.
input The actual chat input prompt. The script is only meant for testing purposes
and exits after answering.
options:
-h, --help show this help message and exit
How do I know which translation model to choose for my target language?
We got you covered with out benchmark.py
script that helps make somewhat of a good guess (the dataset we use is the same as the OPUS models are trained on so the outcomes are always favorable towards OPUS). For usage, see the help of this script below. Models are loaded in 4-bit quantization and run on a small sample of the OPUS books subset.
Be sure to use the most commonly occurring languages in your base dataset as source_language and your target translation language as target_language. For OASST1 for example, be sure to at least run en
and es
as source languages.
usage: benchmark.py [-h] [--cpu] [--start START] [--n N] [--max_length MAX_LENGTH] source_language target_language included_models
Benchmark all the different translation models for a specific source and target language to find out which performs best. This uses 4bit quantization to limit GPU usage. Note:
the outcomes are indicative - you cannot assume corretness of the BLEU and CHRF scores but you can compare models against each other relatively.
positional arguments:
source_language The source language you want to test for. Check your dataset to see which occur most prevalent or use English as a good start.
target_language The source language you want to test for. This should be the language you want to apply the translate script on. Note: in benchmark, we use 2-character
language codes, in constrast to translate.py where you need to specify whatever your model expects.
included_models Comma-separated list of models to include. Allowed values are: opus, m2m_418m, m2m_1.2b, madlad_3b, madlad_7b, madlad_10b, madlad_7bbt, mbart,
nllb_distilled600m, nllb_1.3b, nllb_distilled1.3b, nllb_3.3b, seamless
options:
-h, --help show this help message and exit
--cpu Forces usage of CPU. By default GPU is taken if available.
--start START The starting offset to include sentences from the OPUS books dataset from. Defaults to 0.
--n N The number of sentences to benchmark on. Defaults to 100.
--max_length MAX_LENGTH
How much tokens to generate at most. More tokens might be more accurate for lengthy input but creates a risk of running out of memory. Default is 512.
We have created and will continue to create numerous datasets and models already. Want to help democratize LLMs? Clone the repo and create datasets and models for other languages, then create a PR.
Dutch UnderstandLing/oasst1_nl | Spanish UnderstandLing/oasst1_es | French UnderstandLing/oasst1_fr | German UnderstandLing/oasst1_de |
Catalan xaviviro/oasst1_ca | Portuguese UnderstandLing/oasst1_pt | Arabic HeshamHaroon/oasst-arabic | Italian UnderstandLing/oasst1_it |
Russian UnderstandLing/oasst1_ru | Hindi UnderstandLing/oasst1_hi | Chinese UnderstandLing/oasst1_zh | Polish chrystians/oasst1_pl |
Japanese UnderstandLing/oasst1_jap | Basque xezpeleta/oasst1_eu | Bengali UnderstandLing/oasst1_bn | Turkish UnderstandLing/oasst1_tr |
Make sure you have access to Meta's LLaMa3-8B model and set your HF_TOKEN before using these models.
Dutch UnderstandLing/oasst1_nl_threads | Spanish UnderstandLing/oasst1_es_threads | French UnderstandLing/oasst1_fr_threads | German UnderstandLing/oasst1_de_threads |
Catalan xaviviro/oasst1_ca_threads | Portuguese UnderstandLing/oasst1_pt_threads | Arabic HeshamHaroon/oasst-arabic_threads | Italian UnderstandLing/oasst1_it_threads |
Russian UnderstandLing/oasst1_ru_threads | Hindi UnderstandLing/oasst1_hi_threads | Chinese UnderstandLing/oasst1_zh_threads | Polish chrystians/oasst1_pl_threads |
Japanese UnderstandLing/oasst1_jap_threads | Basque xezpeleta/oasst1_eu_threads | Bengali UnderstandLing/oasst1_bn_threads | Turkish UnderstandLing/oasst1_tr_threads |
UnderstandLing/llama-2-7b-chat-nl Dutch | UnderstandLing/llama-2-7b-chat-es Spanish | UnderstandLing/llama-2-7b-chat-fr French | UnderstandLing/llama-2-7b-chat-de German |
xaviviro/llama-2-7b-chat-ca Catalan | UnderstandLing/llama-2-7b-chat-pt Portuguese | HeshamHaroon/llama-2-7b-chat-ar Arabic | UnderstandLing/llama-2-7b-chat-it Italian |
UnderstandLing/llama-2-7b-chat-ru Russian | UnderstandLing/llama-2-7b-chat-hi Hindi | UnderstandLing/llama-2-7b-chat-zh Chinese | chrystians/llama-2-7b-chat-pl-polish-polski Polish |
xezpeleta/llama-2-7b-chat-eu Basque | UnderstandLing/llama-2-7b-chat-bn Bengali | UnderstandLing/llama-2-7b-chat-tr Turkish |
UnderstandLing/Mistral-7B-Instruct-v0.2-nl Dutch | UnderstandLing/Mistral-7B-Instruct-v0.2-es Spanish | UnderstandLing/Mistral-7B-Instruct-v0.2-de German |
UnderstandLing/llama-2-13b-chat-nl Dutch | UnderstandLing/llama-2-13b-chat-es Spanish | UnderstandLing/llama-2-13b-chat-fr French |
UnderstandLing/Mixtral-8x7B-Instruct-nl Dutch |
<s>[INST] <<SYS>> Je bent een generieke chatbot die altijd in het Nederlands antwoord geeft. <</SYS>> Wat is de hoofdstad van Nederland? [/INST] Amsterdam</s>
<s>[INST] <<SYS>> Je bent een generieke chatbot die altijd in het Nederlands antwoord geeft. <</SYS>> Wat is de hoofdstad van Nederland? [/INST] Amsterdam</s><s>[INST] Hoeveel inwoners heeft die stad? [/INST] 850 duizend inwoners (2023)</s>
<s>[INST] <<SYS>> Je bent een generieke chatbot die altijd in het Nederlands antwoord geeft. <</SYS>> Wat is de hoofdstad van Nederland? [/INST] Amsterdam</s><s>[INST] Hoeveel inwoners heeft die stad? [/INST] 850 duizend inwoners (2023)</s><s>[INST] In welke provincie ligt die stad? [/INST] In de provincie Noord-Holland</s>
<s>[INST] <<SYS>> Je bent een generieke chatbot die altijd in het Nederlands antwoord geeft. <</SYS>> Wie is de minister-president van Nederland? [/INST] Mark Rutte is sinds 2010 minister-president van Nederland. Hij is meerdere keren herkozen.</s>
-
Q: Why do you translate the full OASST1/2 dataset first? Wouldn't it be faster to only translate highest ranked threads?
-
A: While you can gain quite a lot in terms of throughput time by first creating the threads and then translating them, we provide full OASST1/2 translations to the community as we believe they can be useful on their own.
-
Q: How well do the fine-tunes perform compared to vanilla LLaMa3?
-
A: While we do not have formal benchmarks, getting LLaMa3 to consistently speak another language than English to begin with is challenging if not impossible. The non-English language it does produce is often grammatically broken. Our fine-tunes do not show this behavior.
-
Q: Can I use other frameworks for fine-tuning?
-
A: Yes you can, we use Axolotl for training on multi-GPU setups.
-
Q: Can I mix different translation models?
-
A: Absolutely, we think it might even increase performance to have translation done by multiple models. You can achieve this by early-stopping a translation and continuing from the checkpoints by reruning the translate script with a different translation model.
We are actively looking for funding to democratize AI and advance its applications. Contact us at [email protected] if you want to invest.
For Tasks:
Click tags to check more tools for each tasksFor Jobs:
Alternative AI tools for LLaMa2lang
Similar Open Source Tools
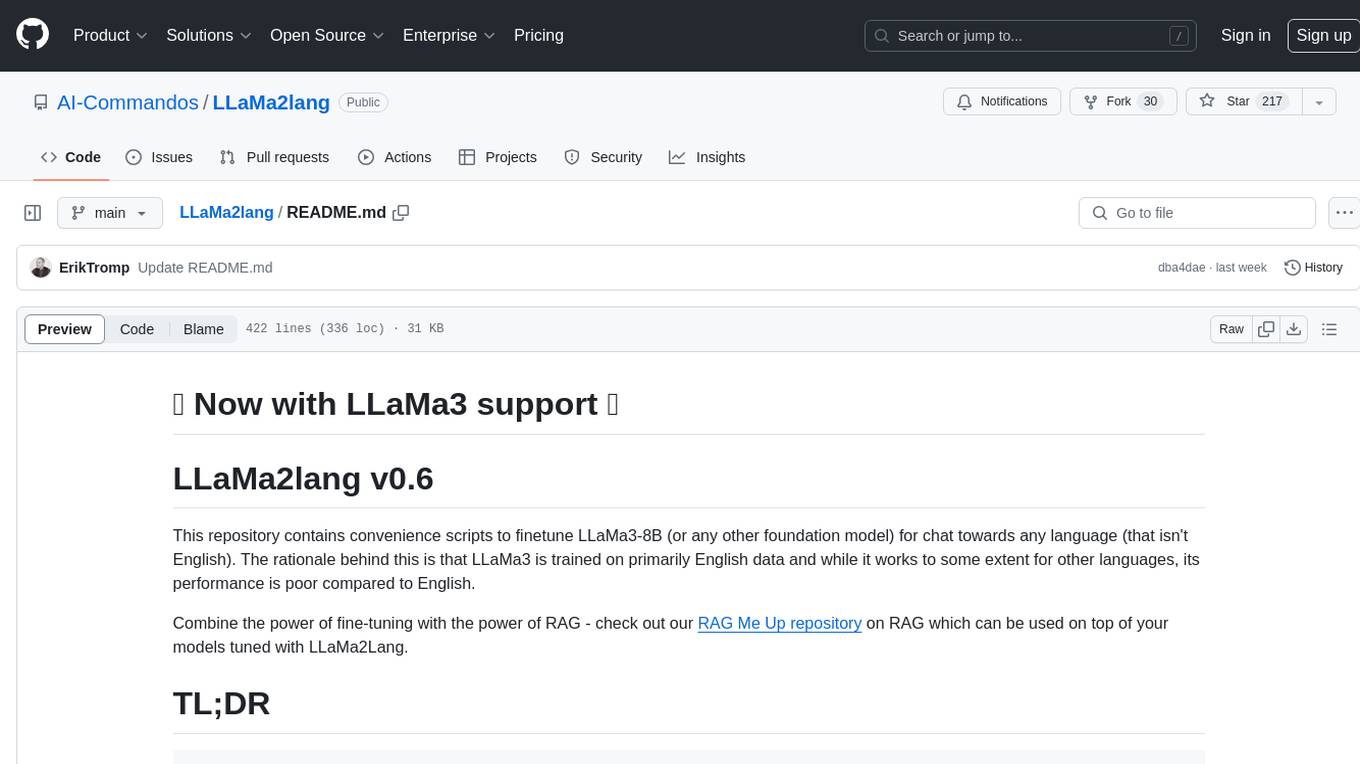
LLaMa2lang
LLaMa2lang is a repository containing convenience scripts to finetune LLaMa3-8B (or any other foundation model) for chat towards any language that isn't English. The repository aims to improve the performance of LLaMa3 for non-English languages by combining fine-tuning with RAG. Users can translate datasets, extract threads, turn threads into prompts, and finetune models using QLoRA and PEFT. Additionally, the repository supports translation models like OPUS, M2M, MADLAD, and base datasets like OASST1 and OASST2. The process involves loading datasets, translating them, combining checkpoints, and running inference using the newly trained model. The repository also provides benchmarking scripts to choose the right translation model for a target language.
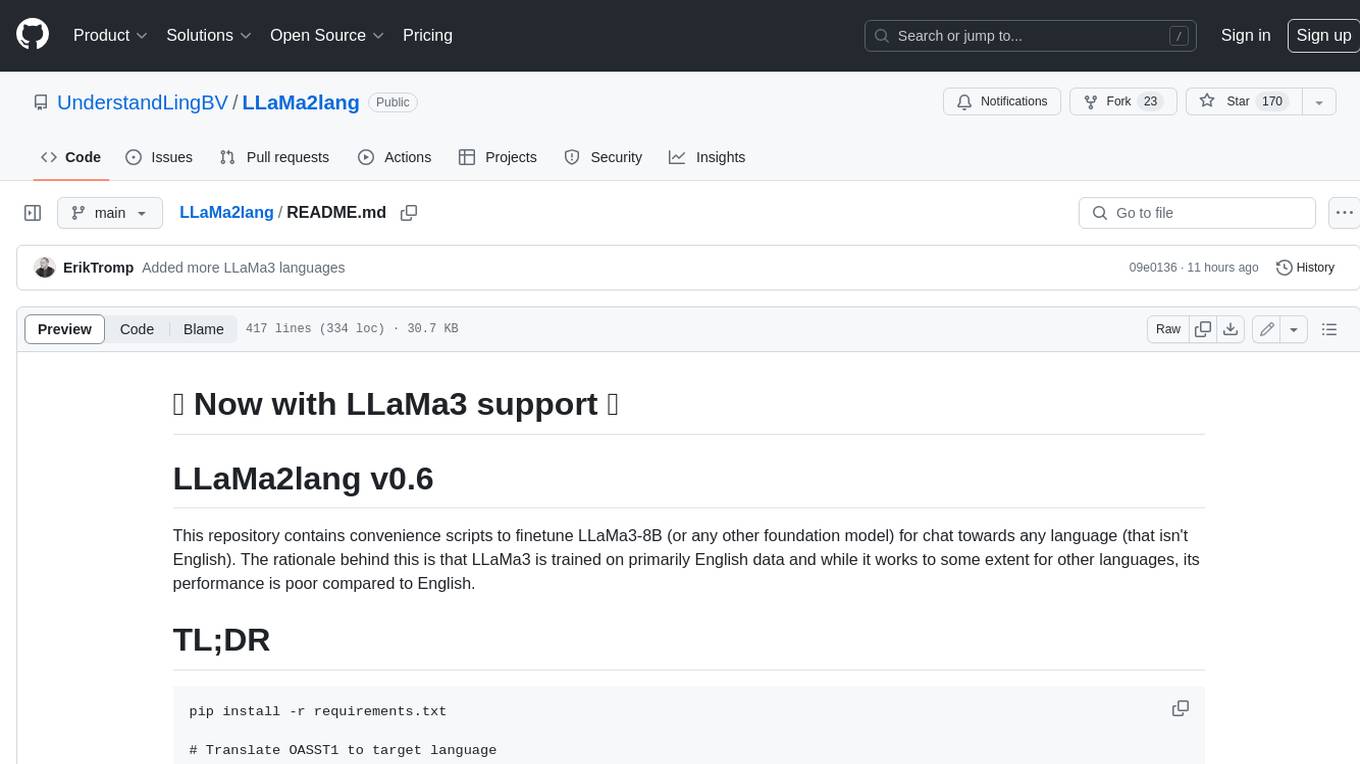
LLaMa2lang
This repository contains convenience scripts to finetune LLaMa3-8B (or any other foundation model) for chat towards any language (that isn't English). The rationale behind this is that LLaMa3 is trained on primarily English data and while it works to some extent for other languages, its performance is poor compared to English.
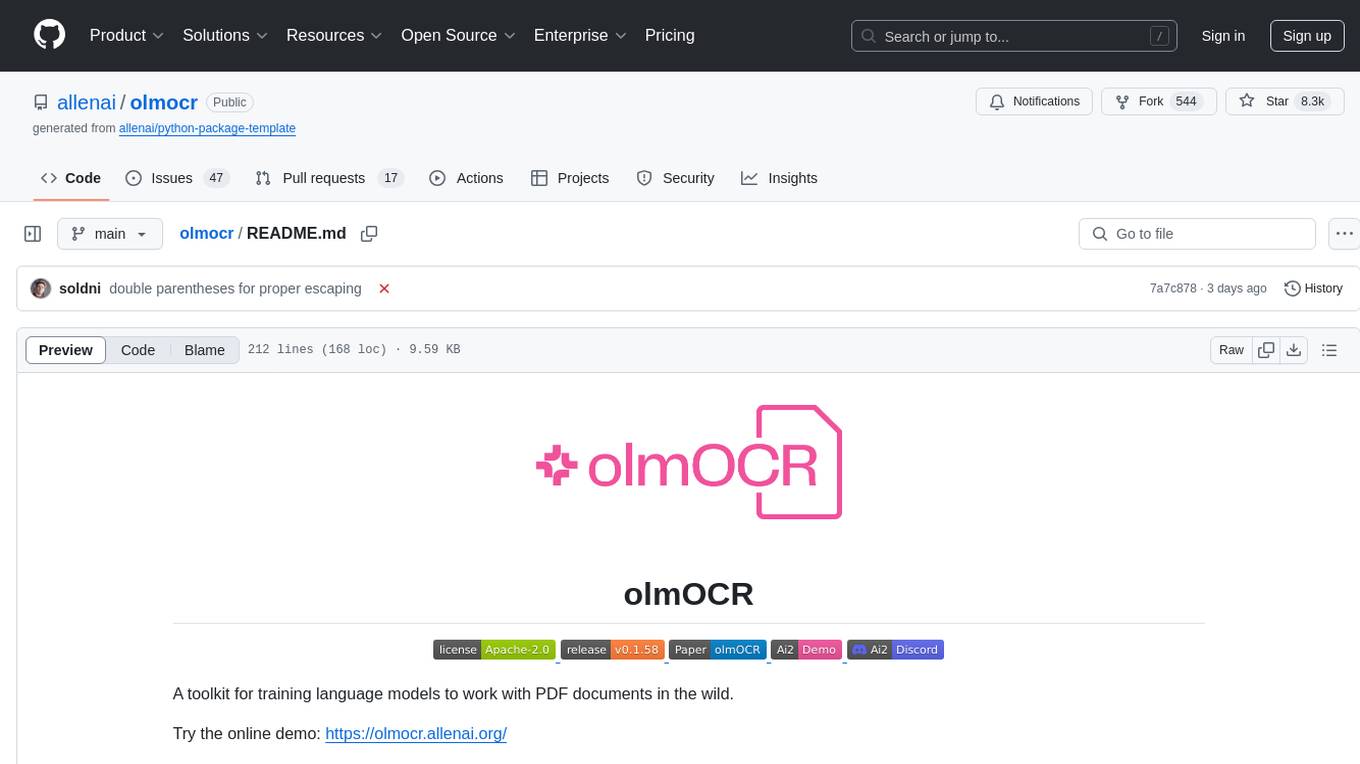
olmocr
olmOCR is a toolkit designed for training language models to work with PDF documents in real-world scenarios. It includes various components such as a prompting strategy for natural text parsing, an evaluation toolkit for comparing pipeline versions, filtering by language and SEO spam removal, finetuning code for specific models, processing PDFs through a finetuned model, and viewing documents created from PDFs. The toolkit requires a recent NVIDIA GPU with at least 20 GB of RAM and 30GB of free disk space. Users can install dependencies, set up a conda environment, and utilize olmOCR for tasks like converting single or multiple PDFs, viewing extracted text, and running batch inference pipelines.
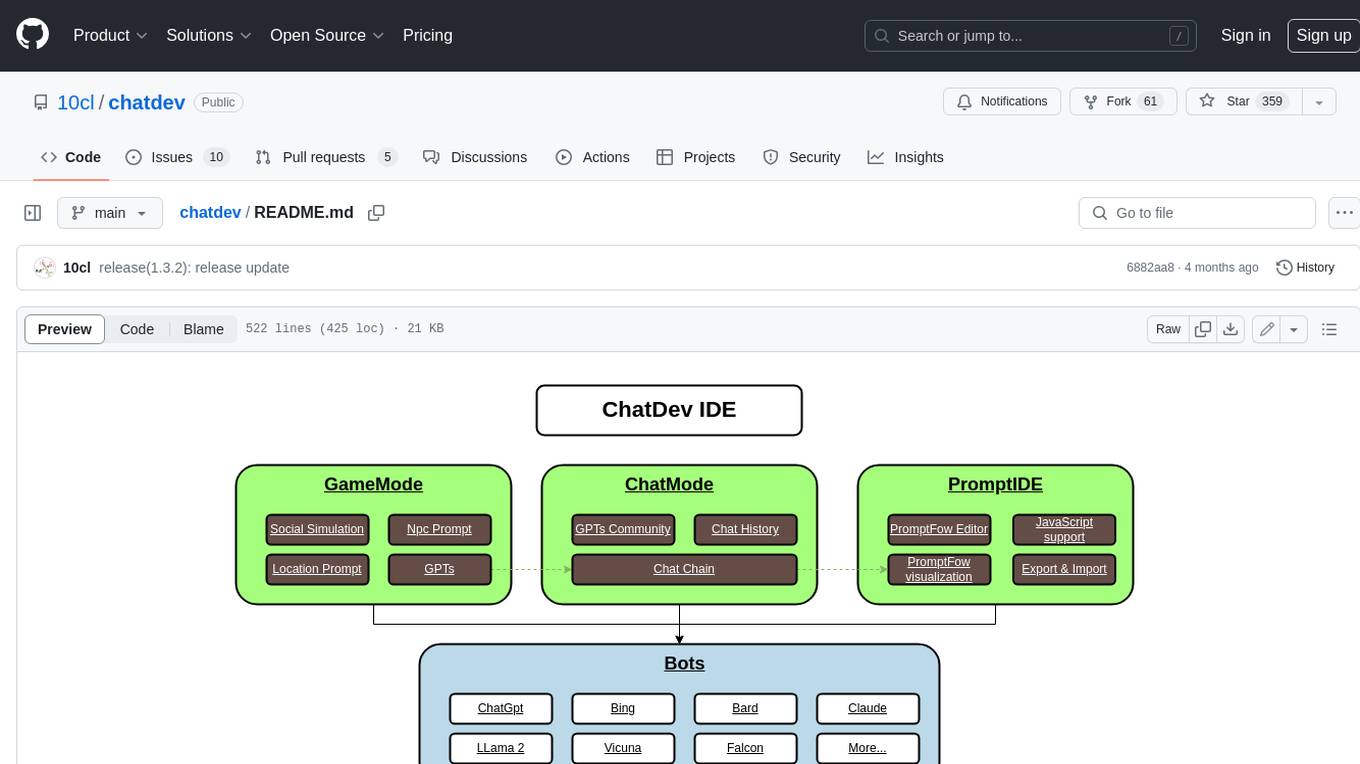
chatdev
ChatDev IDE is a tool for building your AI agent, Whether it's NPCs in games or powerful agent tools, you can design what you want for this platform. It accelerates prompt engineering through **JavaScript Support** that allows implementing complex prompting techniques.
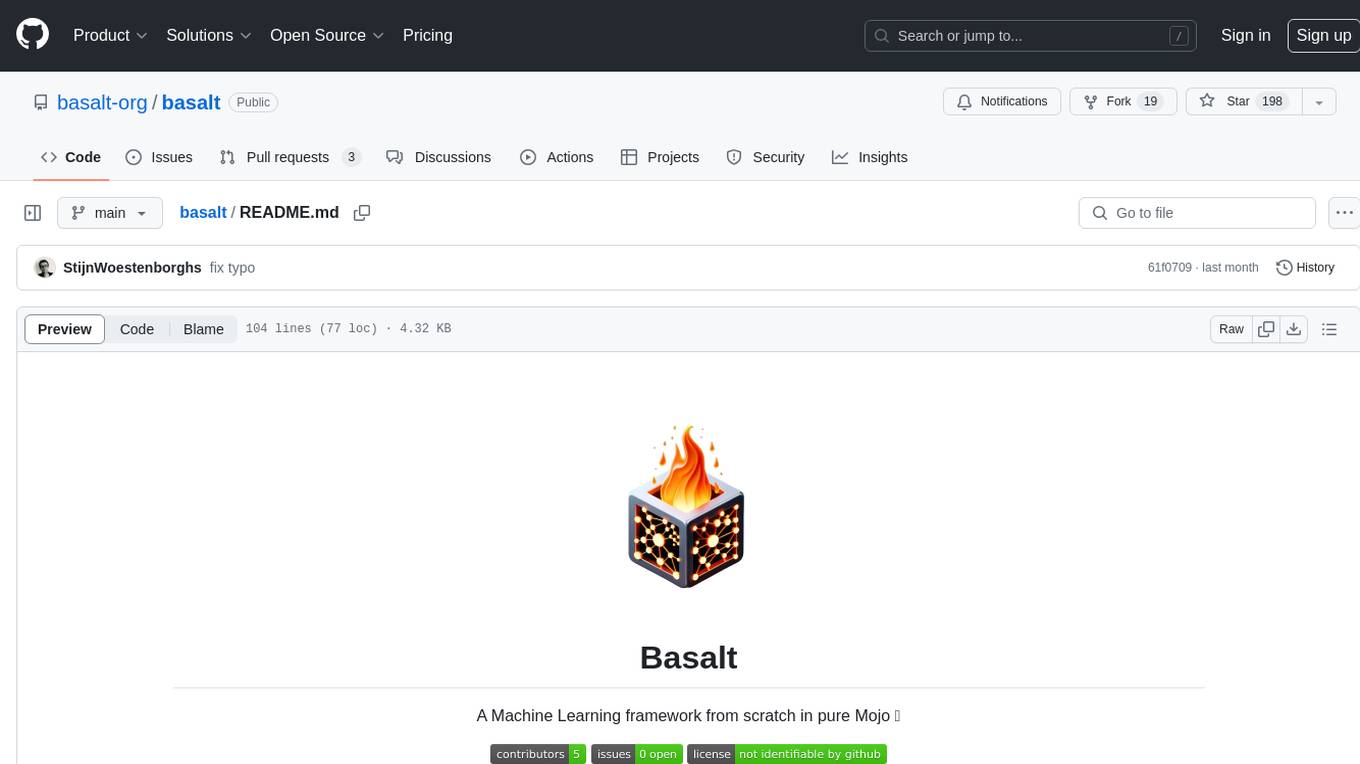
basalt
Basalt is a lightweight and flexible CSS framework designed to help developers quickly build responsive and modern websites. It provides a set of pre-designed components and utilities that can be easily customized to create unique and visually appealing web interfaces. With Basalt, developers can save time and effort by leveraging its modular structure and responsive design principles to create professional-looking websites with ease.
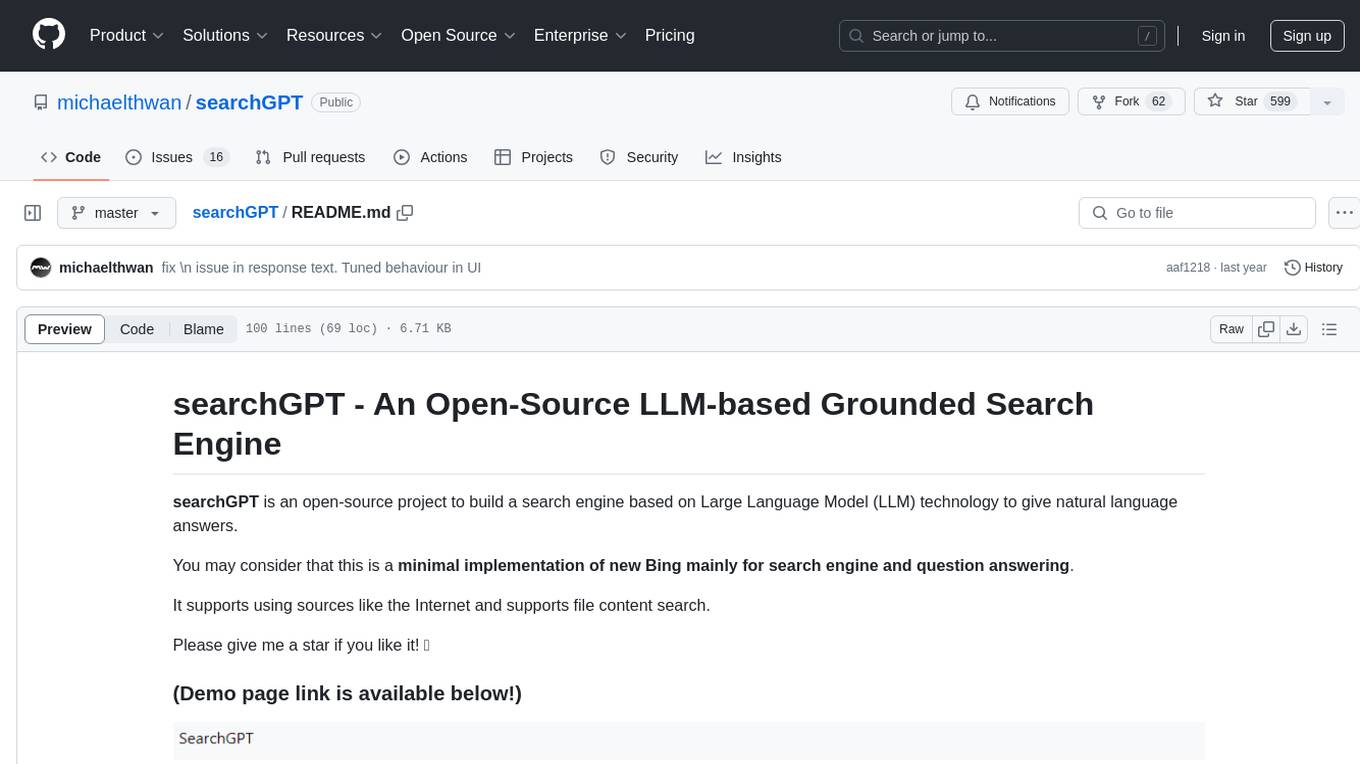
searchGPT
searchGPT is an open-source project that aims to build a search engine based on Large Language Model (LLM) technology to provide natural language answers. It supports web search with real-time results, file content search, and semantic search from sources like the Internet. The tool integrates LLM technologies such as OpenAI and GooseAI, and offers an easy-to-use frontend user interface. The project is designed to provide grounded answers by referencing real-time factual information, addressing the limitations of LLM's training data. Contributions, especially from frontend developers, are welcome under the MIT License.
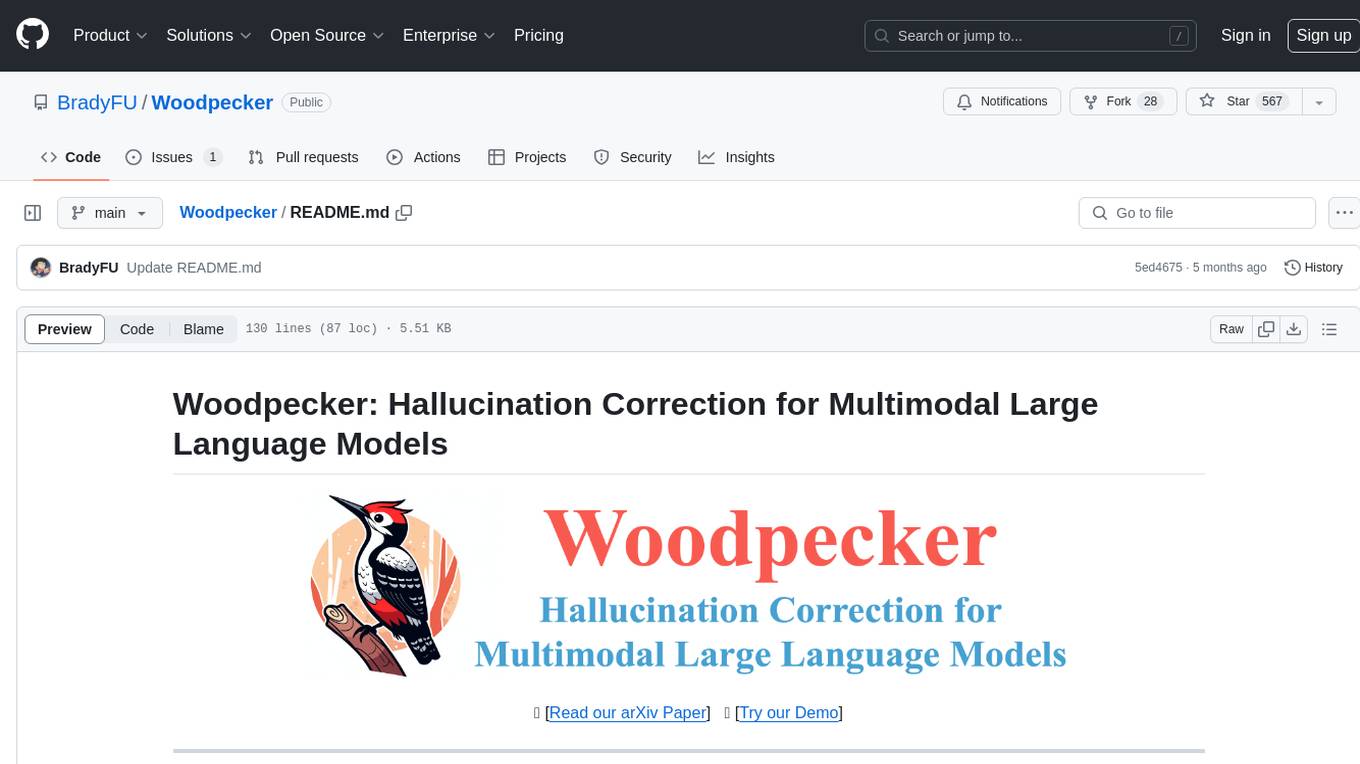
Woodpecker
Woodpecker is a tool designed to correct hallucinations in Multimodal Large Language Models (MLLMs) by introducing a training-free method that picks out and corrects inconsistencies between generated text and image content. It consists of five stages: key concept extraction, question formulation, visual knowledge validation, visual claim generation, and hallucination correction. Woodpecker can be easily integrated with different MLLMs and provides interpretable results by accessing intermediate outputs of the stages. The tool has shown significant improvements in accuracy over baseline models like MiniGPT-4 and mPLUG-Owl.
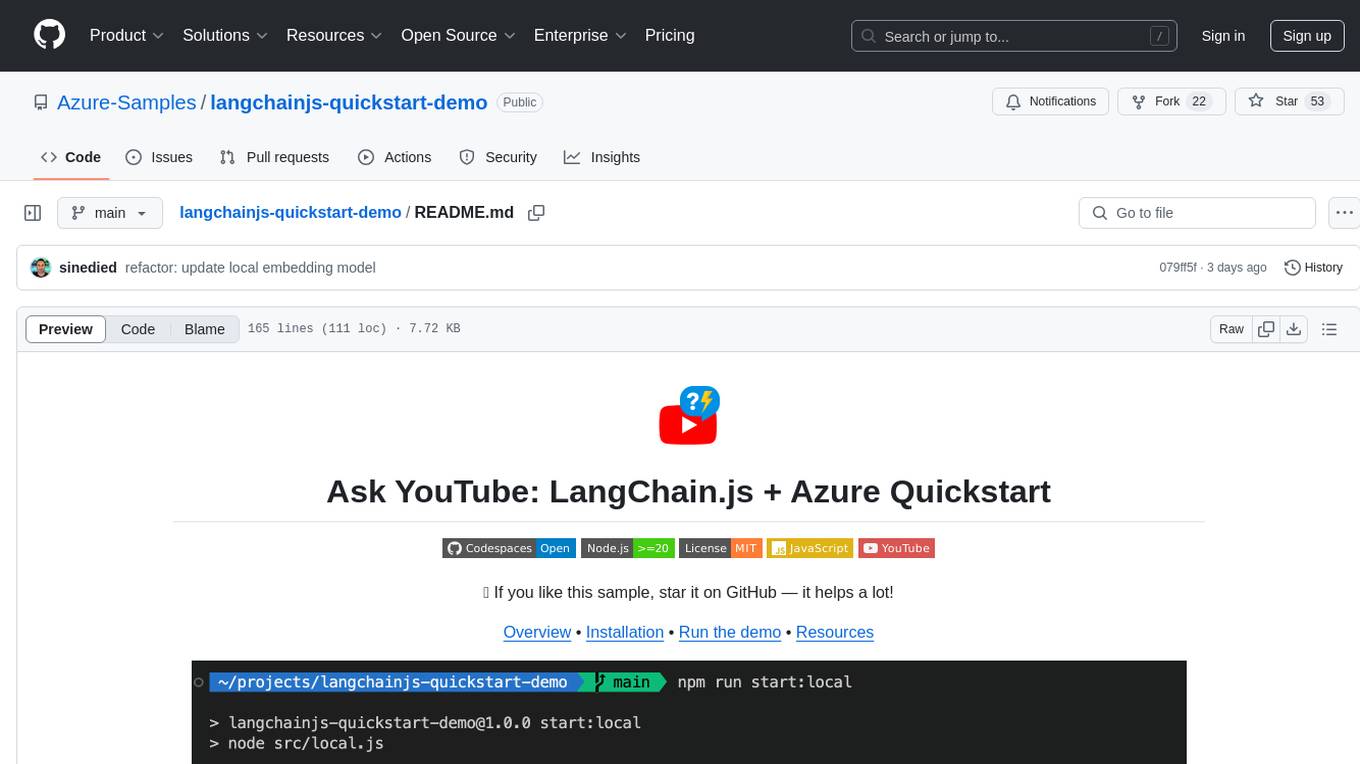
langchainjs-quickstart-demo
Discover the journey of building a generative AI application using LangChain.js and Azure. This demo explores the development process from idea to production, using a RAG-based approach for a Q&A system based on YouTube video transcripts. The application allows to ask text-based questions about a YouTube video and uses the transcript of the video to generate responses. The code comes in two versions: local prototype using FAISS and Ollama with LLaMa3 model for completion and all-minilm-l6-v2 for embeddings, and Azure cloud version using Azure AI Search and GPT-4 Turbo model for completion and text-embedding-3-large for embeddings. Either version can be run as an API using the Azure Functions runtime.
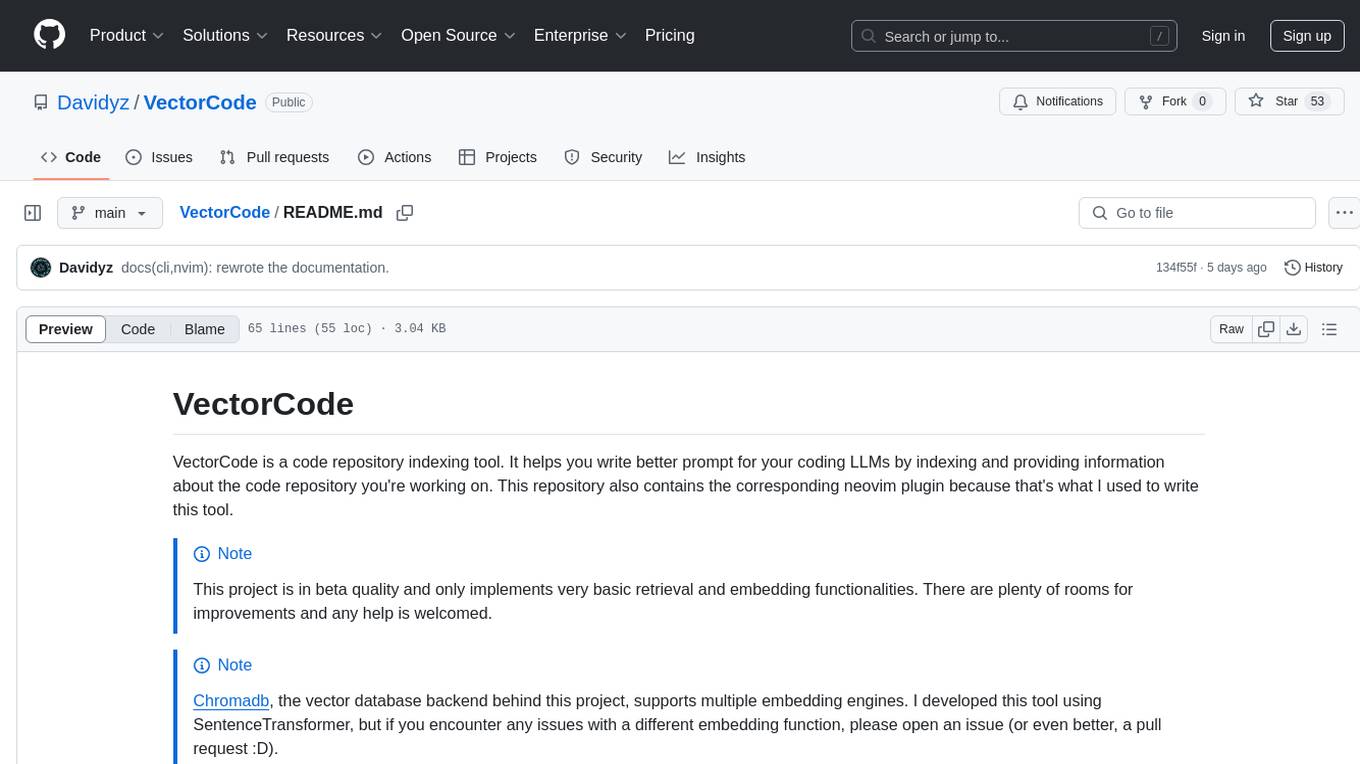
VectorCode
VectorCode is a code repository indexing tool that helps users write better prompts for coding LLMs by providing information about the code repository being worked on. It includes a neovim plugin and supports multiple embedding engines. The tool enhances completion results by providing project context and improves understanding of close-source or cutting edge projects.
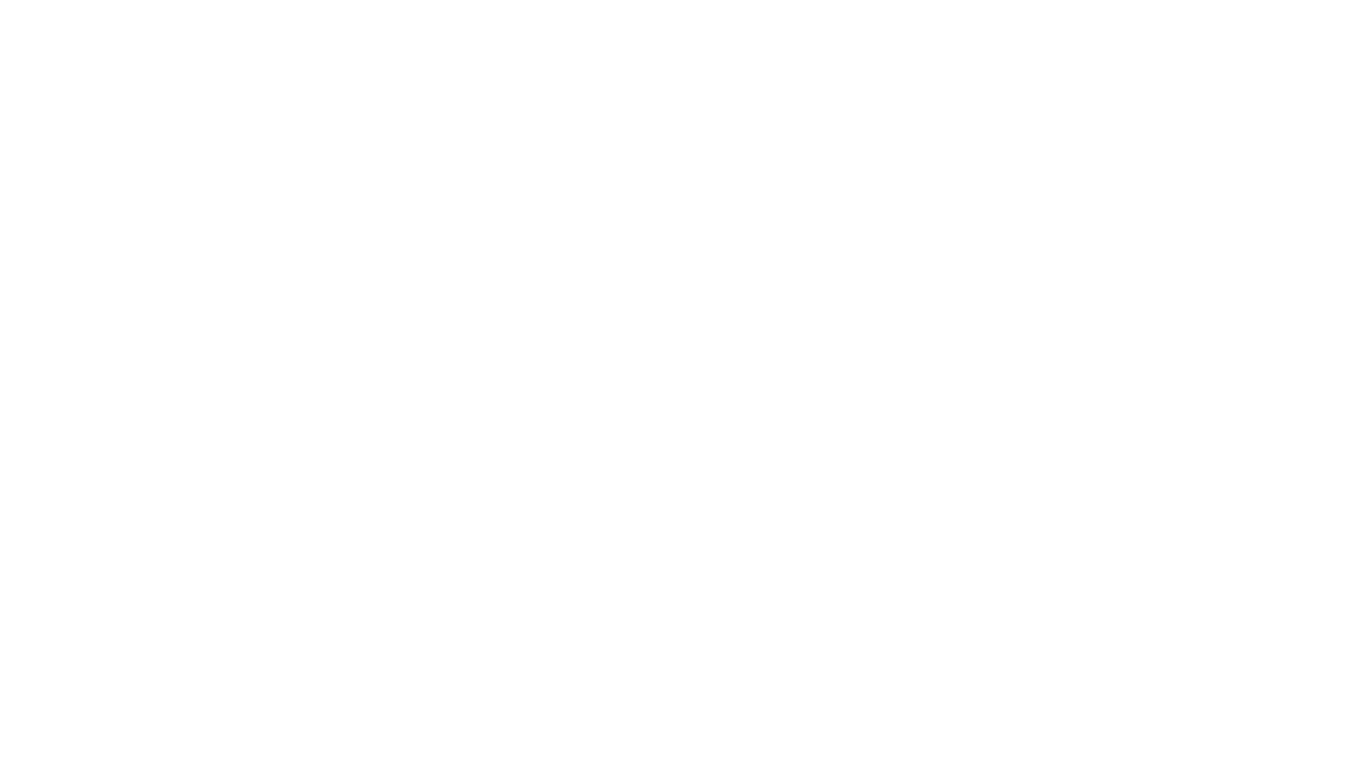
project_alice
Alice is an agentic workflow framework that integrates task execution and intelligent chat capabilities. It provides a flexible environment for creating, managing, and deploying AI agents for various purposes, leveraging a microservices architecture with MongoDB for data persistence. The framework consists of components like APIs, agents, tasks, and chats that interact to produce outputs through files, messages, task results, and URL references. Users can create, test, and deploy agentic solutions in a human-language framework, making it easy to engage with by both users and agents. The tool offers an open-source option, user management, flexible model deployment, and programmatic access to tasks and chats.
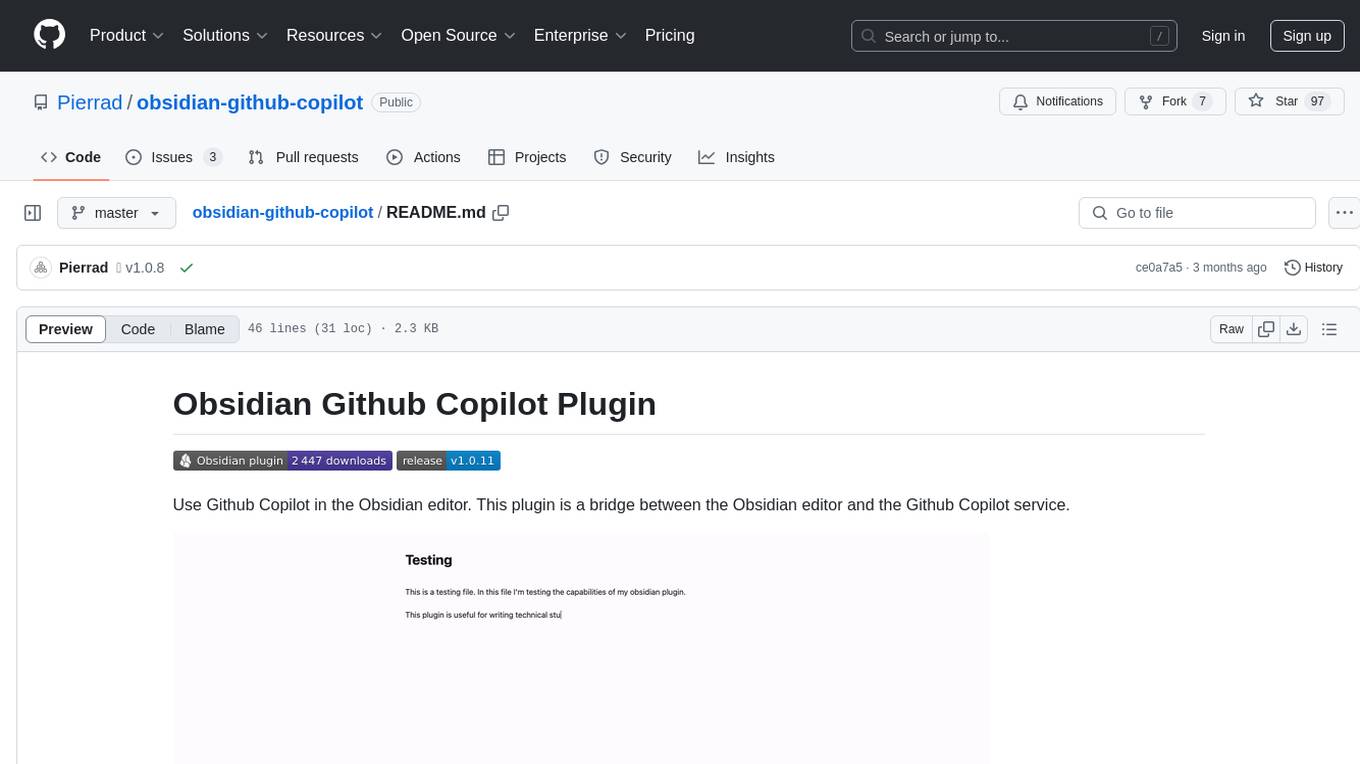
obsidian-github-copilot
Obsidian Github Copilot Plugin is a tool that enables users to utilize Github Copilot within the Obsidian editor. It acts as a bridge between Obsidian and the Github Copilot service, allowing for enhanced code completion and suggestion features. Users can configure various settings such as suggestion generation delay, key bindings, and visibility of suggestions. The plugin requires a Github Copilot subscription, Node.js 18 or later, and a network connection to interact with the Copilot service. It simplifies the process of writing code by providing helpful completions and suggestions directly within the Obsidian editor.
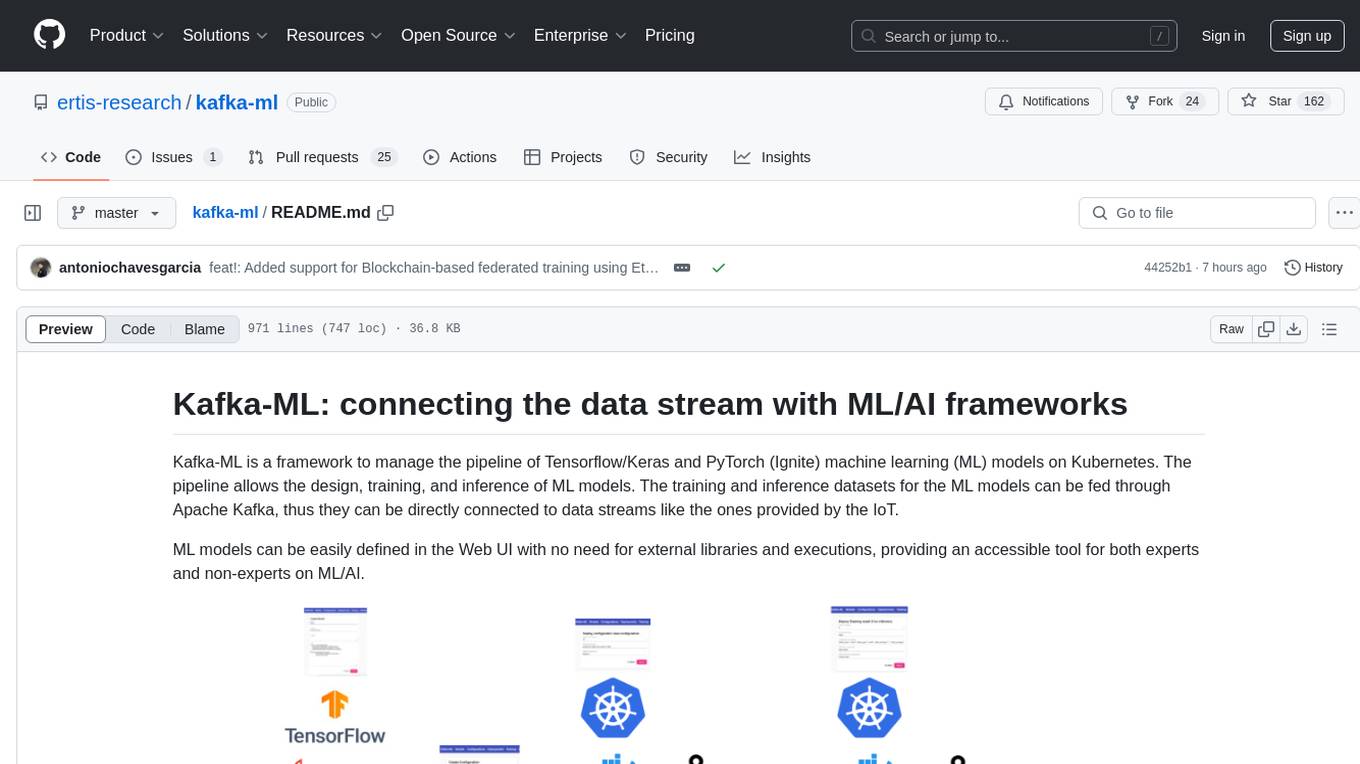
kafka-ml
Kafka-ML is a framework designed to manage the pipeline of Tensorflow/Keras and PyTorch machine learning models on Kubernetes. It enables the design, training, and inference of ML models with datasets fed through Apache Kafka, connecting them directly to data streams like those from IoT devices. The Web UI allows easy definition of ML models without external libraries, catering to both experts and non-experts in ML/AI.
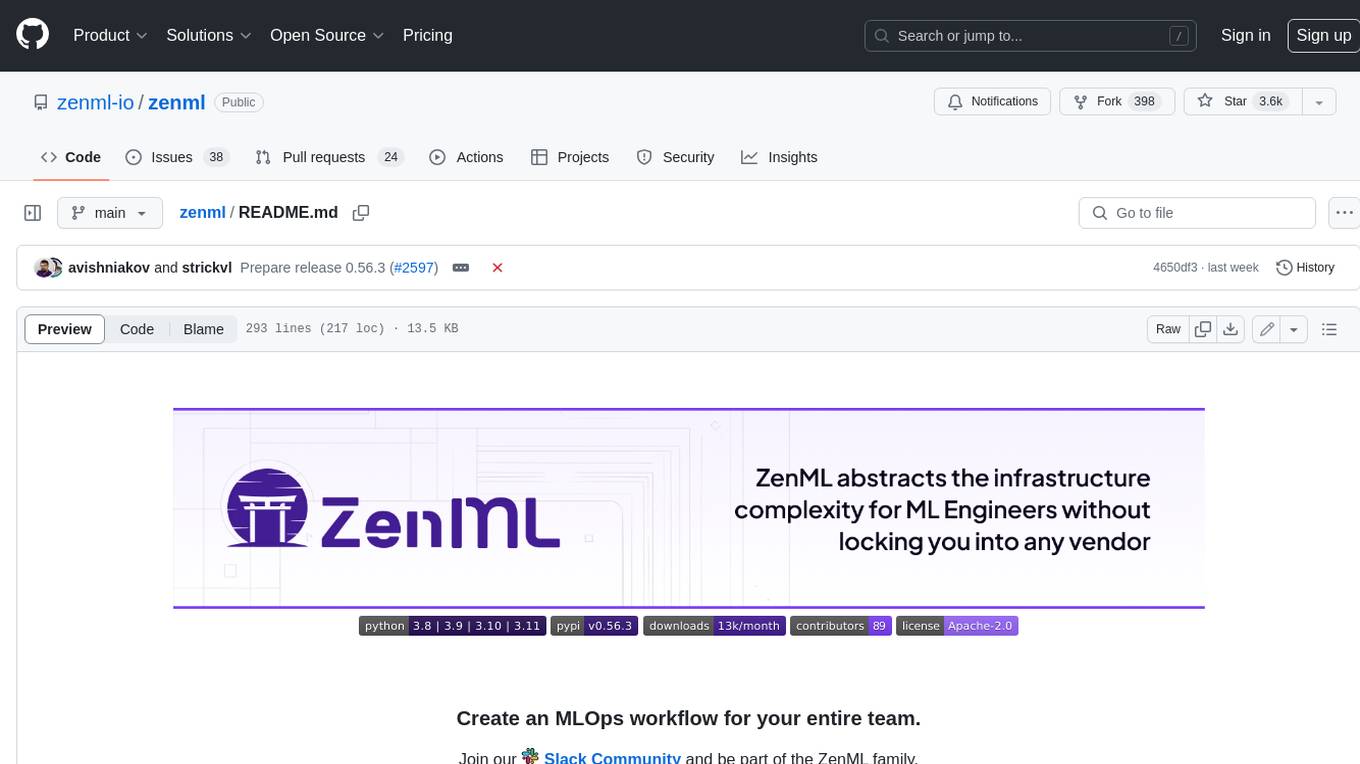
zenml
ZenML is an extensible, open-source MLOps framework for creating portable, production-ready machine learning pipelines. By decoupling infrastructure from code, ZenML enables developers across your organization to collaborate more effectively as they develop to production.
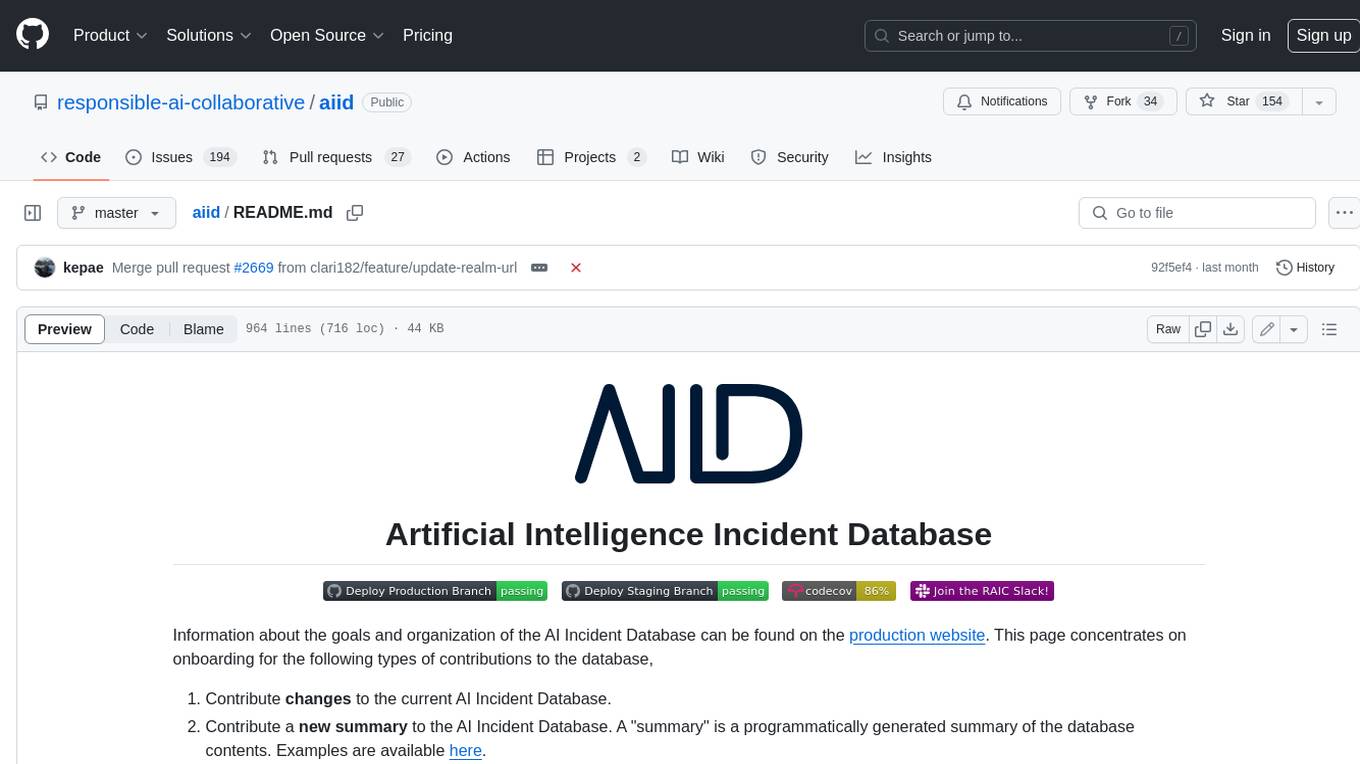
aiid
The Artificial Intelligence Incident Database (AIID) is a collection of incidents involving the development and use of artificial intelligence (AI). The database is designed to help researchers, policymakers, and the public understand the potential risks and benefits of AI, and to inform the development of policies and practices to mitigate the risks and promote the benefits of AI. The AIID is a collaborative project involving researchers from the University of California, Berkeley, the University of Washington, and the University of Toronto.
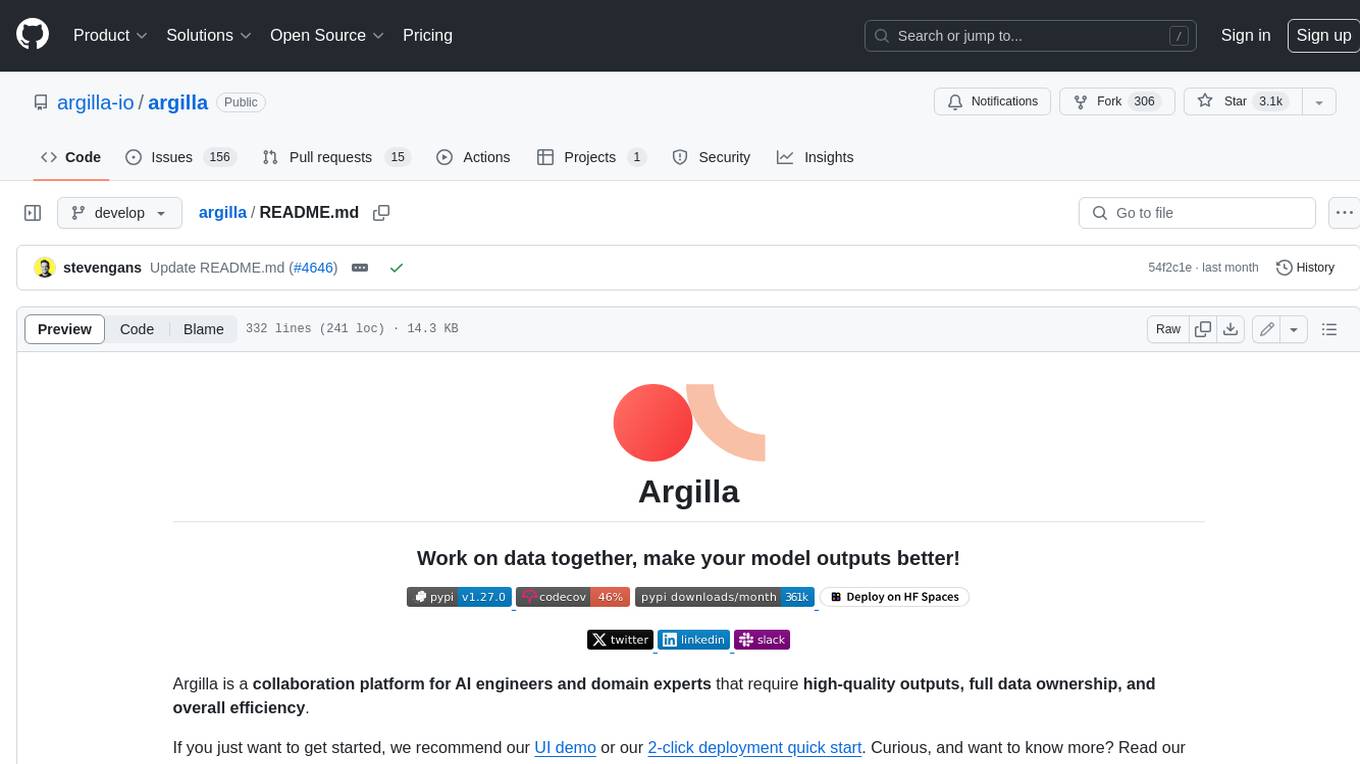
argilla
Argilla is a collaboration platform for AI engineers and domain experts that require high-quality outputs, full data ownership, and overall efficiency. It helps users improve AI output quality through data quality, take control of their data and models, and improve efficiency by quickly iterating on the right data and models. Argilla is an open-source community-driven project that provides tools for achieving and maintaining high-quality data standards, with a focus on NLP and LLMs. It is used by AI teams from companies like the Red Cross, Loris.ai, and Prolific to improve the quality and efficiency of AI projects.
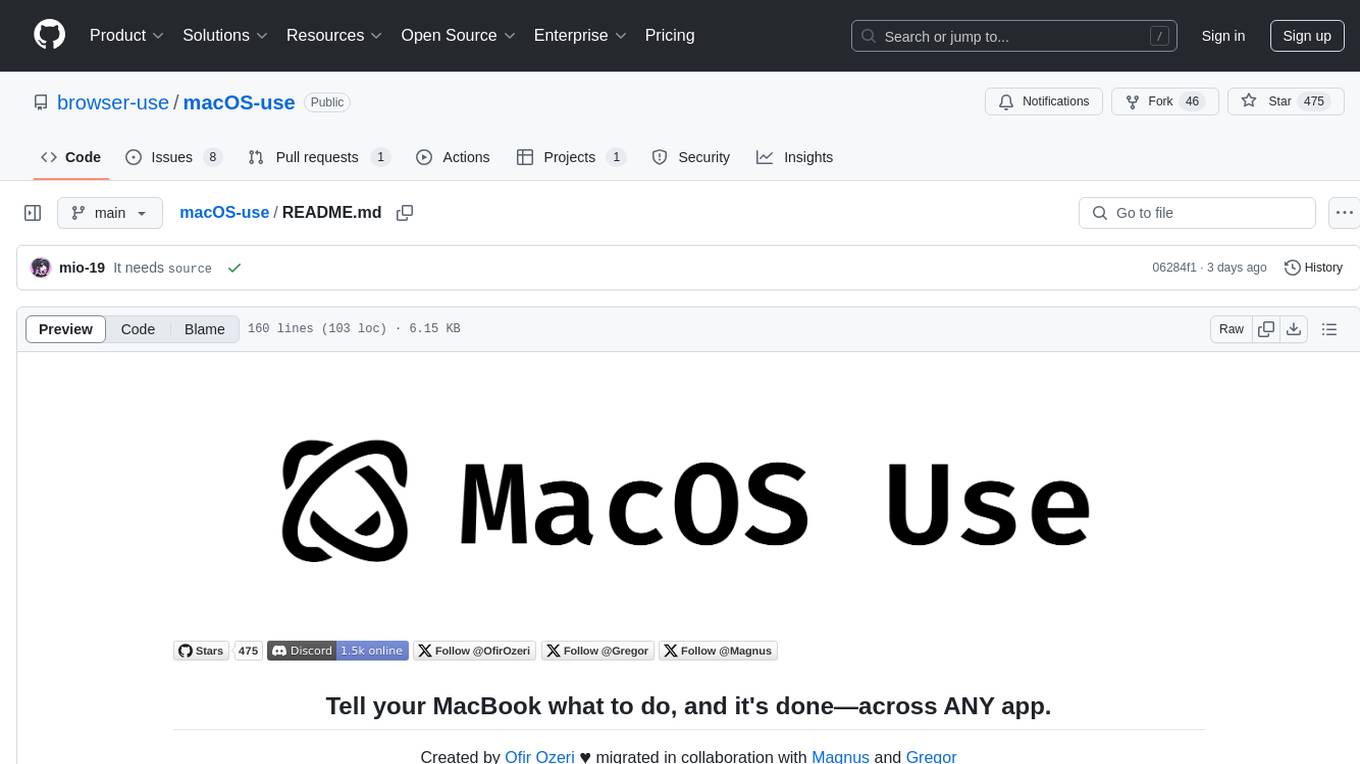
macOS-use
macOS-use is a project that enables AI agents to interact with a MacBook across any app. It aims to build an AI agent for the MLX by Apple framework to perform actions on Apple devices. The project is under active development and allows users to prompt the agent to perform various tasks on their MacBook. Users need to be cautious as the tool can interact with apps, UI components, and use private credentials. The project is open source and welcomes contributions from the community.
For similar tasks
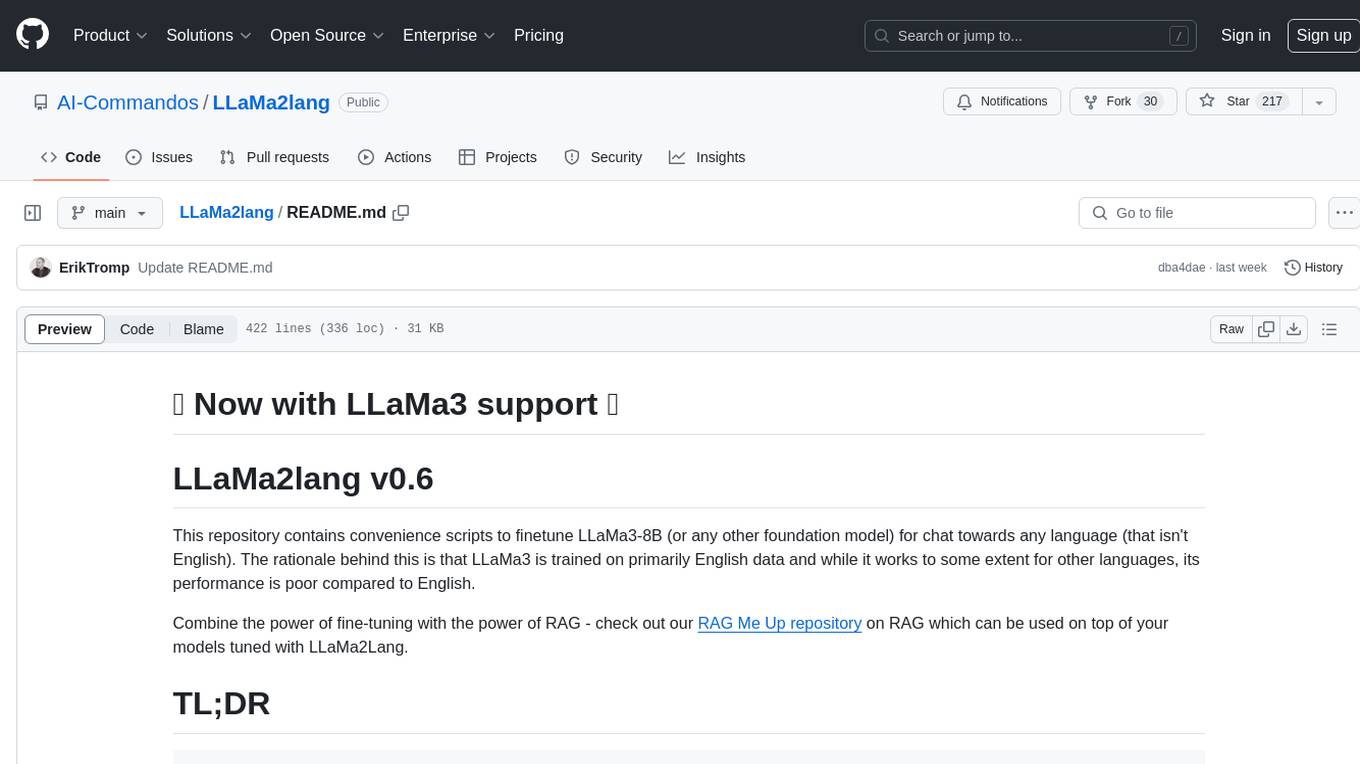
LLaMa2lang
LLaMa2lang is a repository containing convenience scripts to finetune LLaMa3-8B (or any other foundation model) for chat towards any language that isn't English. The repository aims to improve the performance of LLaMa3 for non-English languages by combining fine-tuning with RAG. Users can translate datasets, extract threads, turn threads into prompts, and finetune models using QLoRA and PEFT. Additionally, the repository supports translation models like OPUS, M2M, MADLAD, and base datasets like OASST1 and OASST2. The process involves loading datasets, translating them, combining checkpoints, and running inference using the newly trained model. The repository also provides benchmarking scripts to choose the right translation model for a target language.
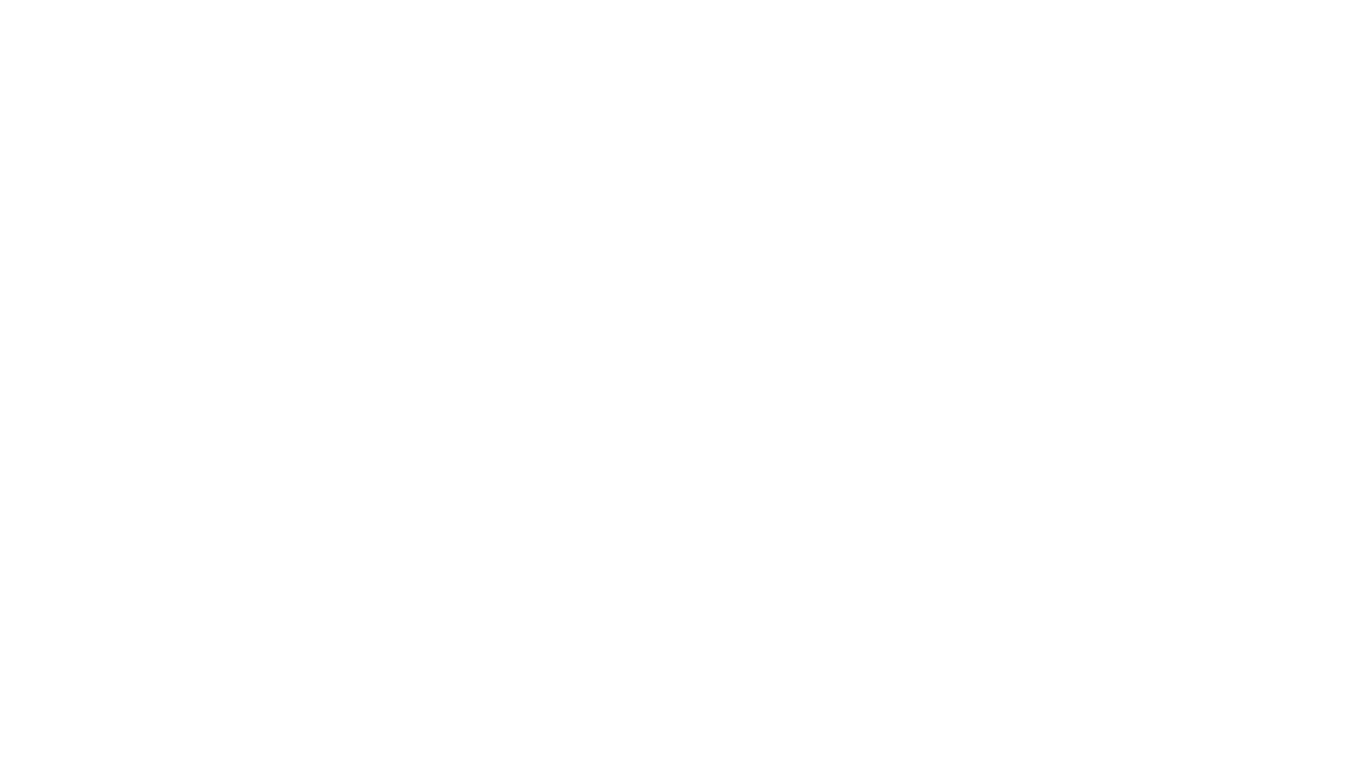
Raspberry
Raspberry is an open source project aimed at creating a toy dataset for finetuning Large Language Models (LLMs) with reasoning abilities. The project involves synthesizing complex user queries across various domains, generating CoT and Self-Critique data, cleaning and rectifying samples, finetuning an LLM with the dataset, and seeking funding for scalability. The ultimate goal is to develop a dataset that challenges models with tasks requiring math, coding, logic, reasoning, and planning skills, spanning different sectors like medicine, science, and software development.
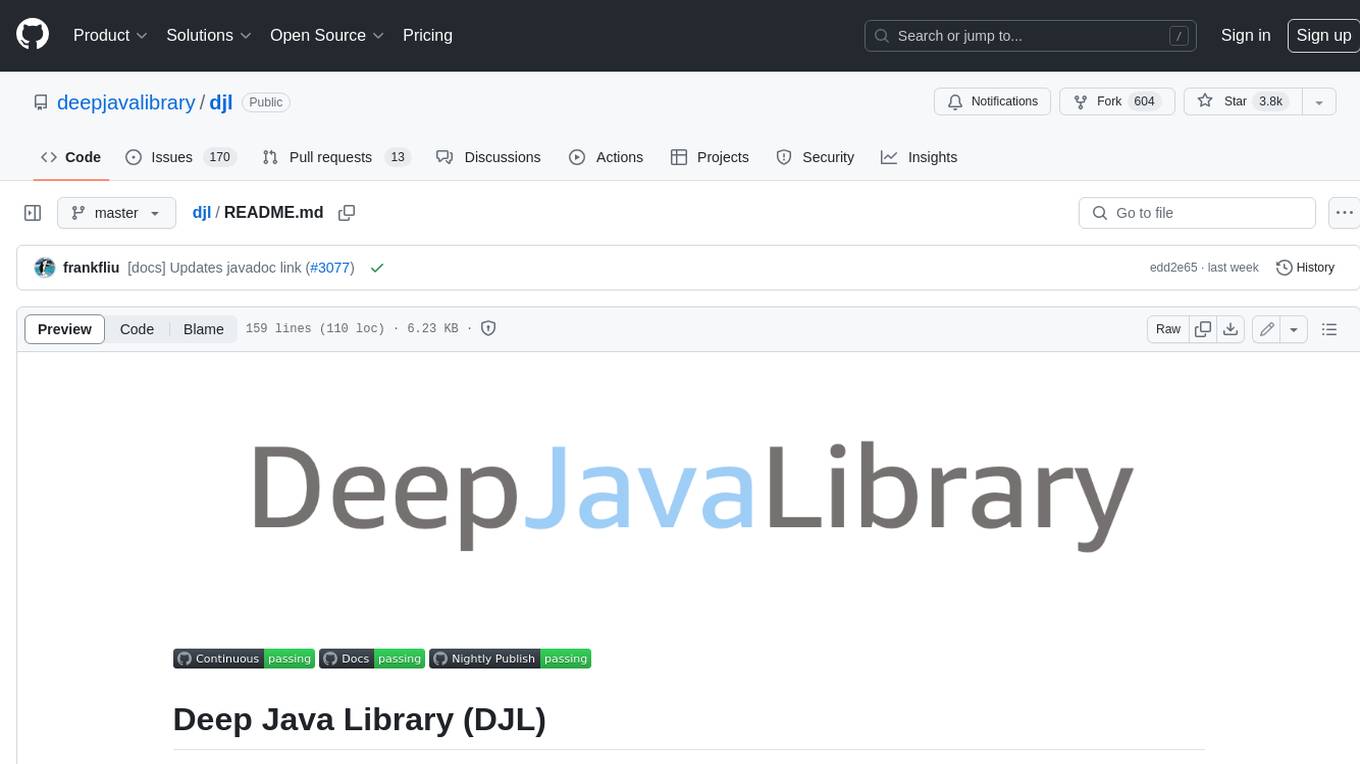
djl
Deep Java Library (DJL) is an open-source, high-level, engine-agnostic Java framework for deep learning. It is designed to be easy to get started with and simple to use for Java developers. DJL provides a native Java development experience and allows users to integrate machine learning and deep learning models with their Java applications. The framework is deep learning engine agnostic, enabling users to switch engines at any point for optimal performance. DJL's ergonomic API interface guides users with best practices to accomplish deep learning tasks, such as running inference and training neural networks.
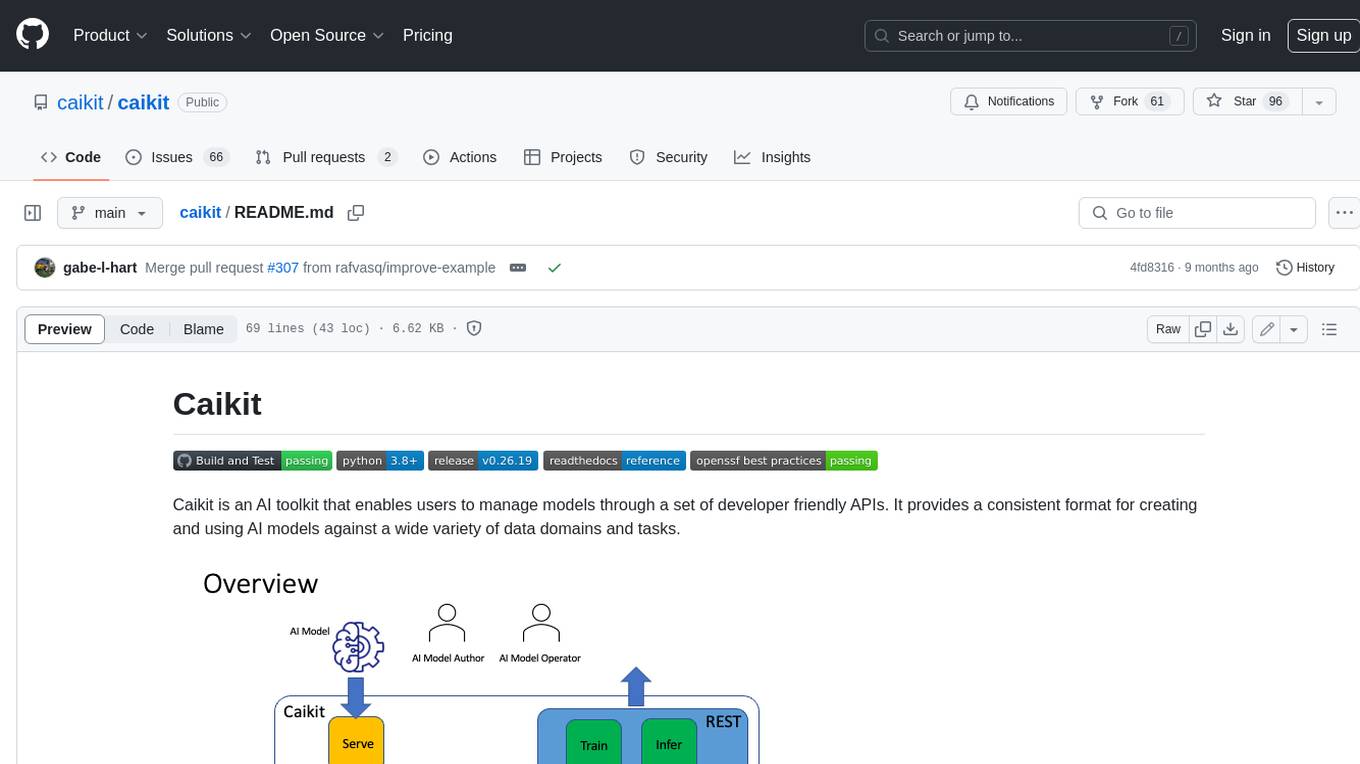
caikit
Caikit is an AI toolkit that enables users to manage models through a set of developer friendly APIs. It provides a consistent format for creating and using AI models against a wide variety of data domains and tasks.
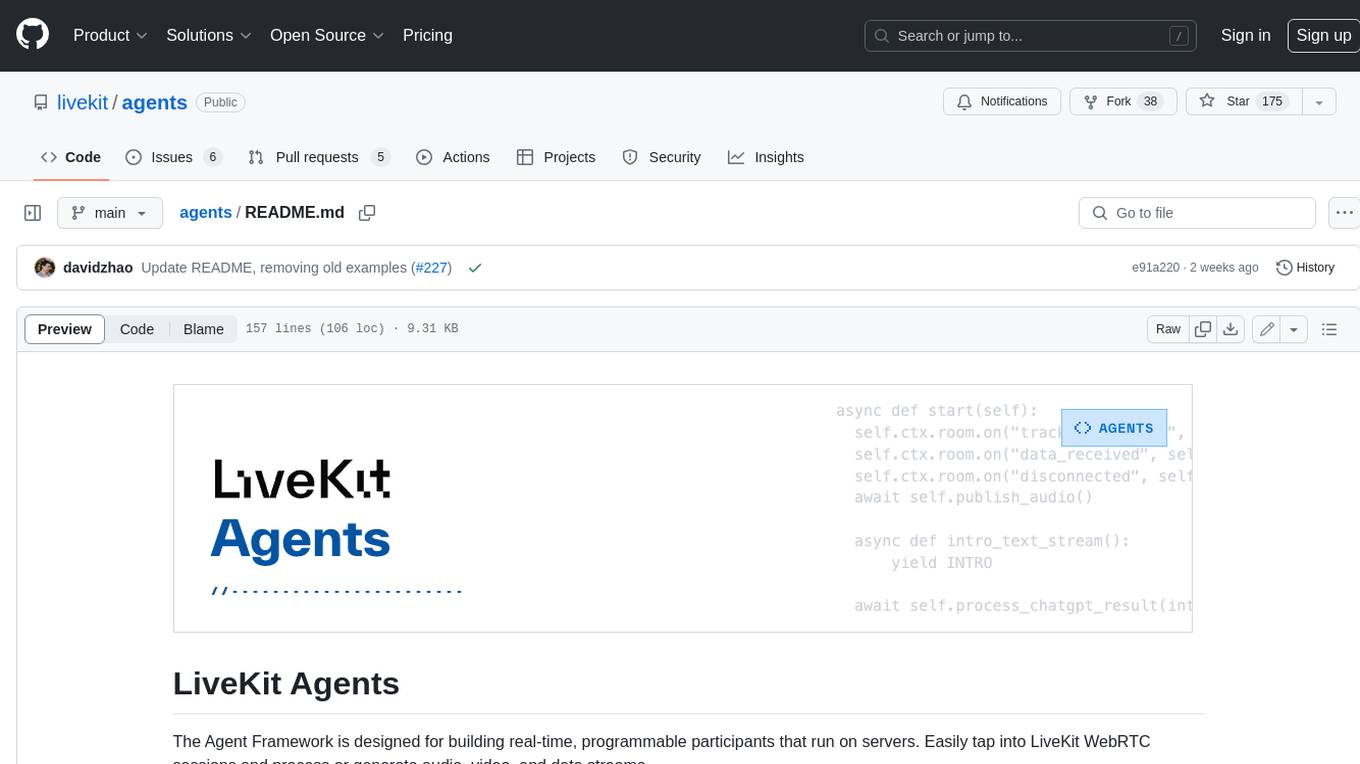
agents
The LiveKit Agent Framework is designed for building real-time, programmable participants that run on servers. Easily tap into LiveKit WebRTC sessions and process or generate audio, video, and data streams. The framework includes plugins for common workflows, such as voice activity detection and speech-to-text. Agents integrates seamlessly with LiveKit server, offloading job queuing and scheduling responsibilities to it. This eliminates the need for additional queuing infrastructure. Agent code developed on your local machine can scale to support thousands of concurrent sessions when deployed to a server in production.
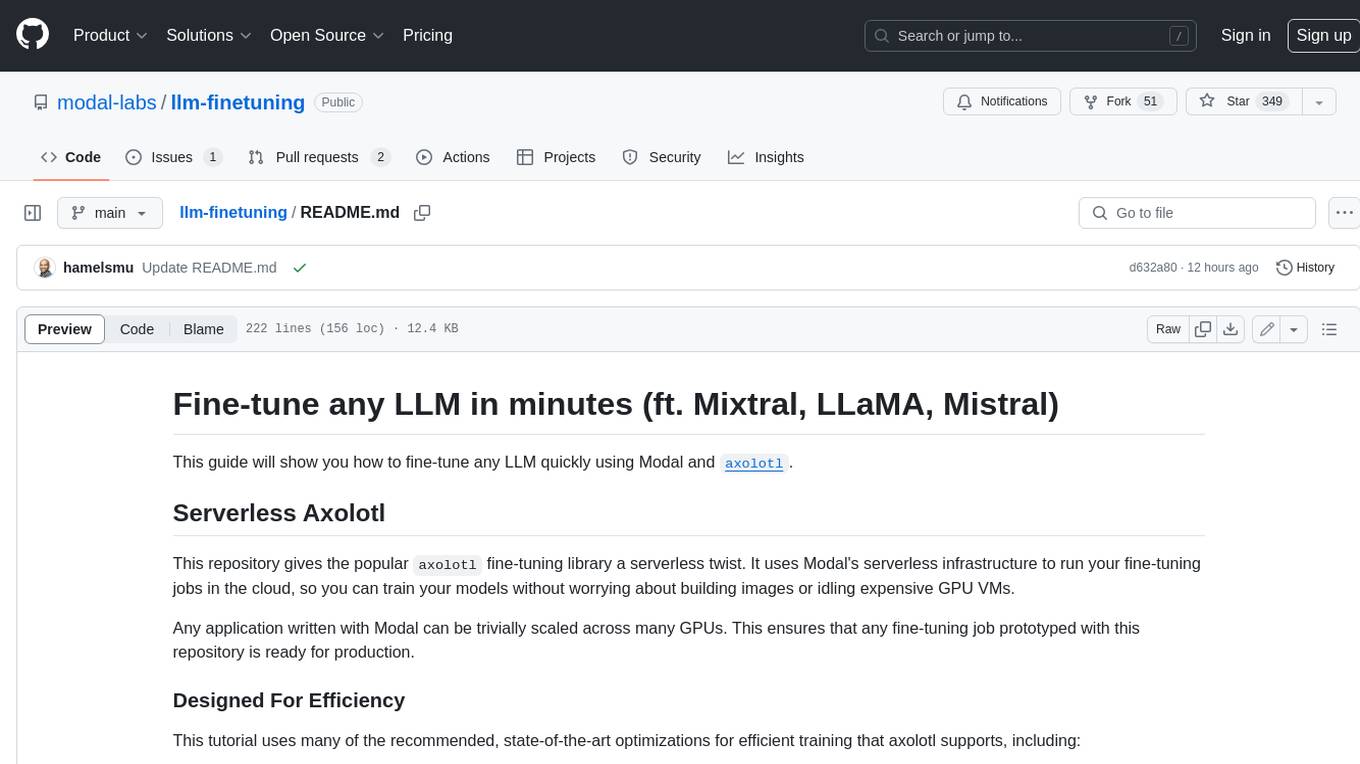
llm-finetuning
llm-finetuning is a repository that provides a serverless twist to the popular axolotl fine-tuning library using Modal's serverless infrastructure. It allows users to quickly fine-tune any LLM model with state-of-the-art optimizations like Deepspeed ZeRO, LoRA adapters, Flash attention, and Gradient checkpointing. The repository simplifies the fine-tuning process by not exposing all CLI arguments, instead allowing users to specify options in a config file. It supports efficient training and scaling across multiple GPUs, making it suitable for production-ready fine-tuning jobs.
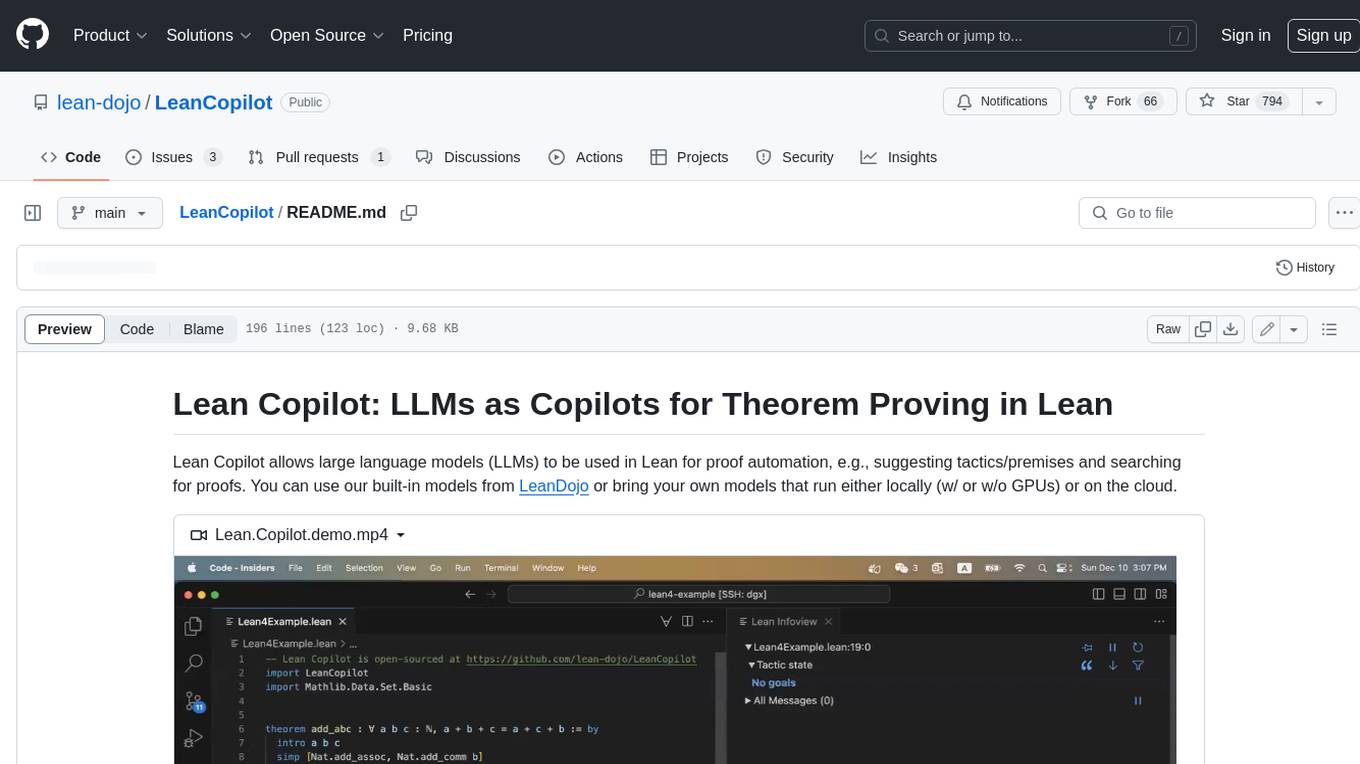
LeanCopilot
Lean Copilot is a tool that enables the use of large language models (LLMs) in Lean for proof automation. It provides features such as suggesting tactics/premises, searching for proofs, and running inference of LLMs. Users can utilize built-in models from LeanDojo or bring their own models to run locally or on the cloud. The tool supports platforms like Linux, macOS, and Windows WSL, with optional CUDA and cuDNN for GPU acceleration. Advanced users can customize behavior using Tactic APIs and Model APIs. Lean Copilot also allows users to bring their own models through ExternalGenerator or ExternalEncoder. The tool comes with caveats such as occasional crashes and issues with premise selection and proof search. Users can get in touch through GitHub Discussions for questions, bug reports, feature requests, and suggestions. The tool is designed to enhance theorem proving in Lean using LLMs.
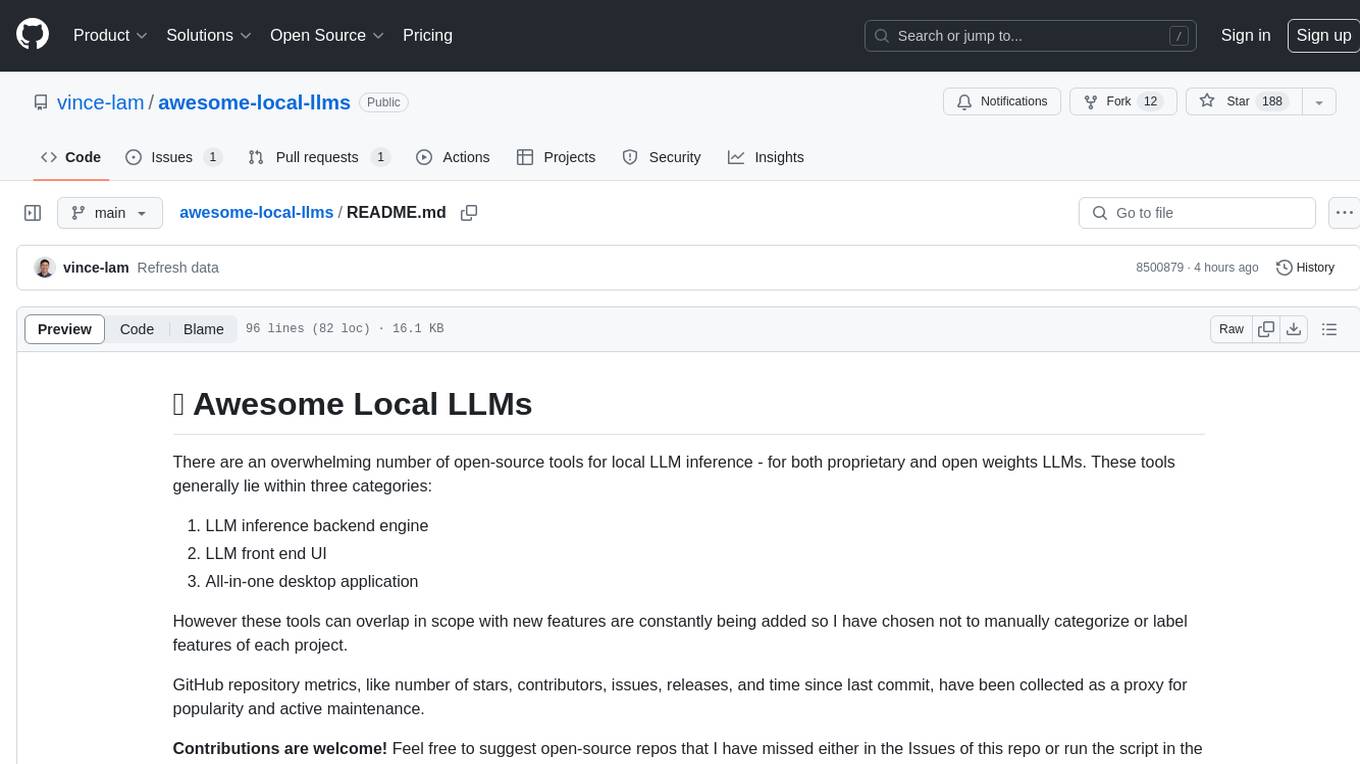
awesome-local-llms
The 'awesome-local-llms' repository is a curated list of open-source tools for local Large Language Model (LLM) inference, covering both proprietary and open weights LLMs. The repository categorizes these tools into LLM inference backend engines, LLM front end UIs, and all-in-one desktop applications. It collects GitHub repository metrics as proxies for popularity and active maintenance. Contributions are encouraged, and users can suggest additional open-source repositories through the Issues section or by running a provided script to update the README and make a pull request. The repository aims to provide a comprehensive resource for exploring and utilizing local LLM tools.
For similar jobs
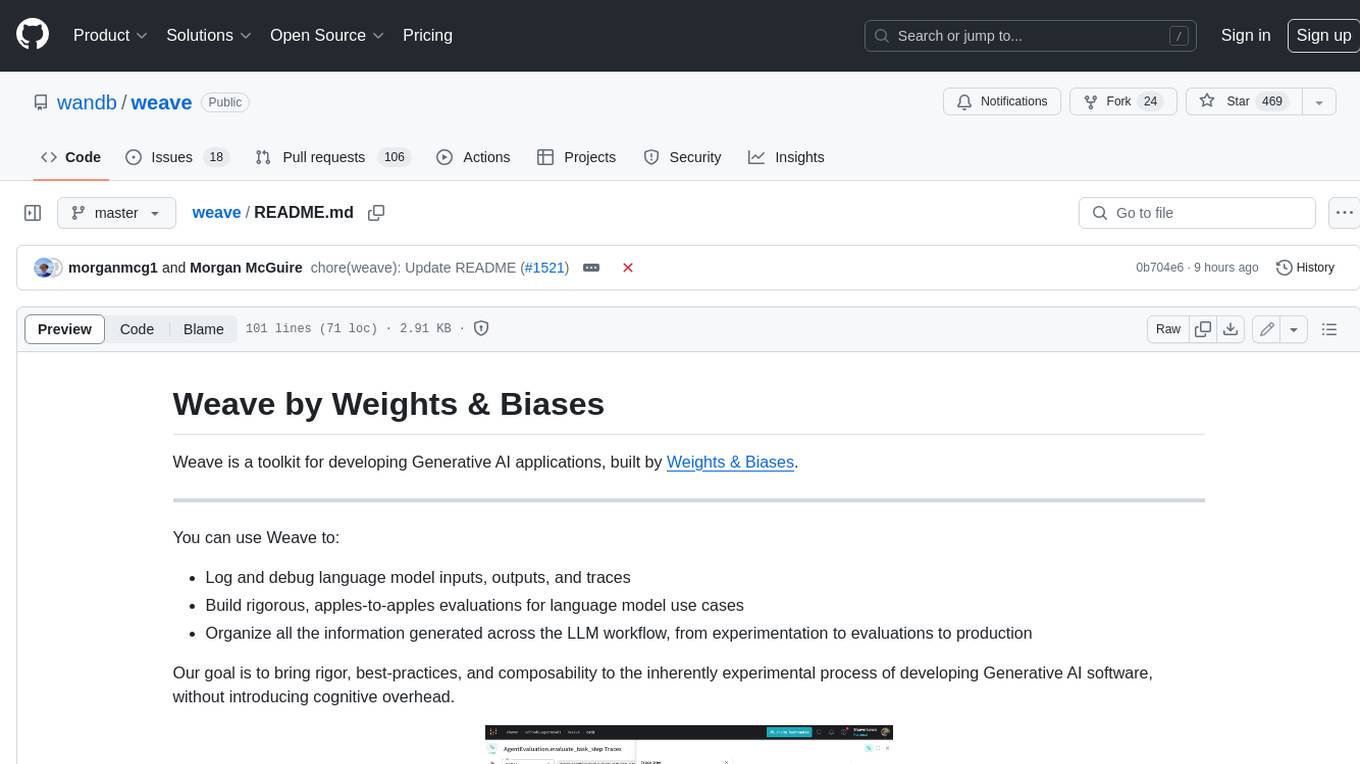
weave
Weave is a toolkit for developing Generative AI applications, built by Weights & Biases. With Weave, you can log and debug language model inputs, outputs, and traces; build rigorous, apples-to-apples evaluations for language model use cases; and organize all the information generated across the LLM workflow, from experimentation to evaluations to production. Weave aims to bring rigor, best-practices, and composability to the inherently experimental process of developing Generative AI software, without introducing cognitive overhead.
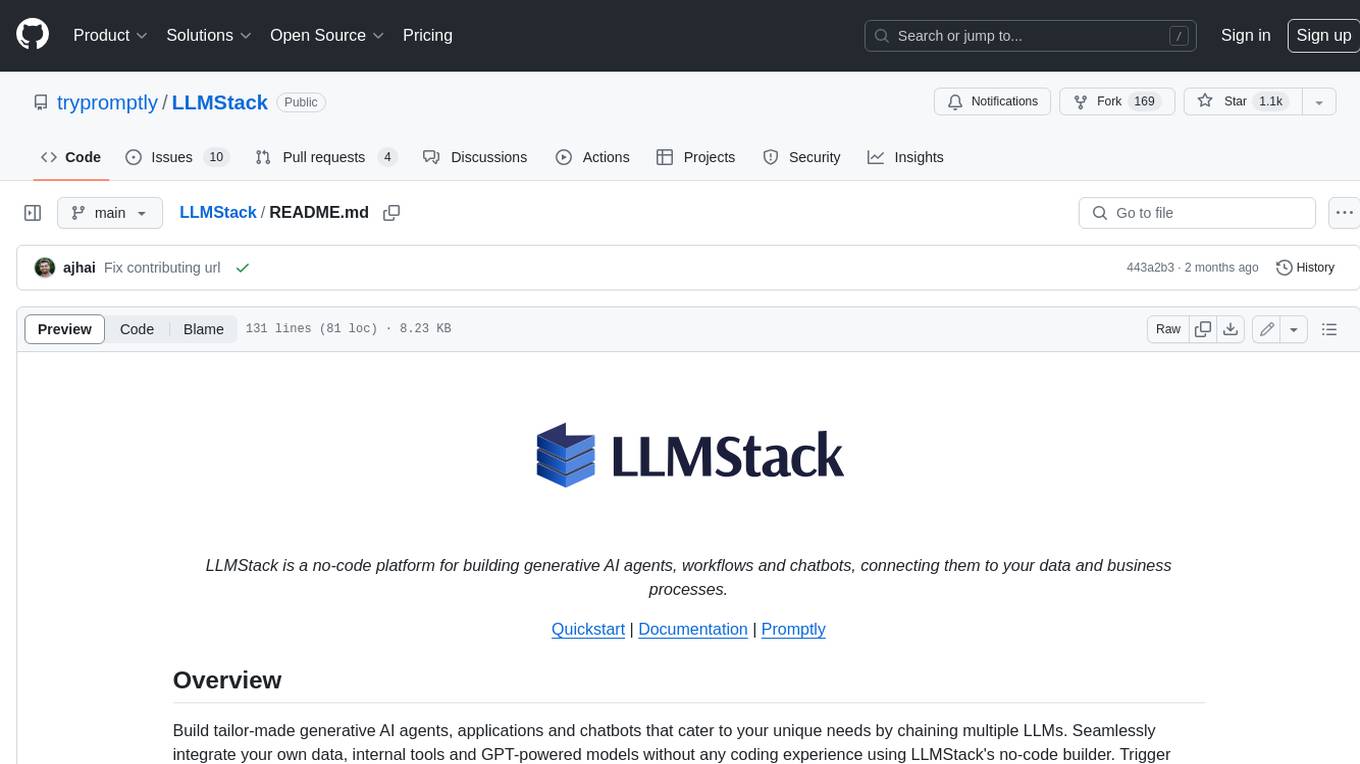
LLMStack
LLMStack is a no-code platform for building generative AI agents, workflows, and chatbots. It allows users to connect their own data, internal tools, and GPT-powered models without any coding experience. LLMStack can be deployed to the cloud or on-premise and can be accessed via HTTP API or triggered from Slack or Discord.
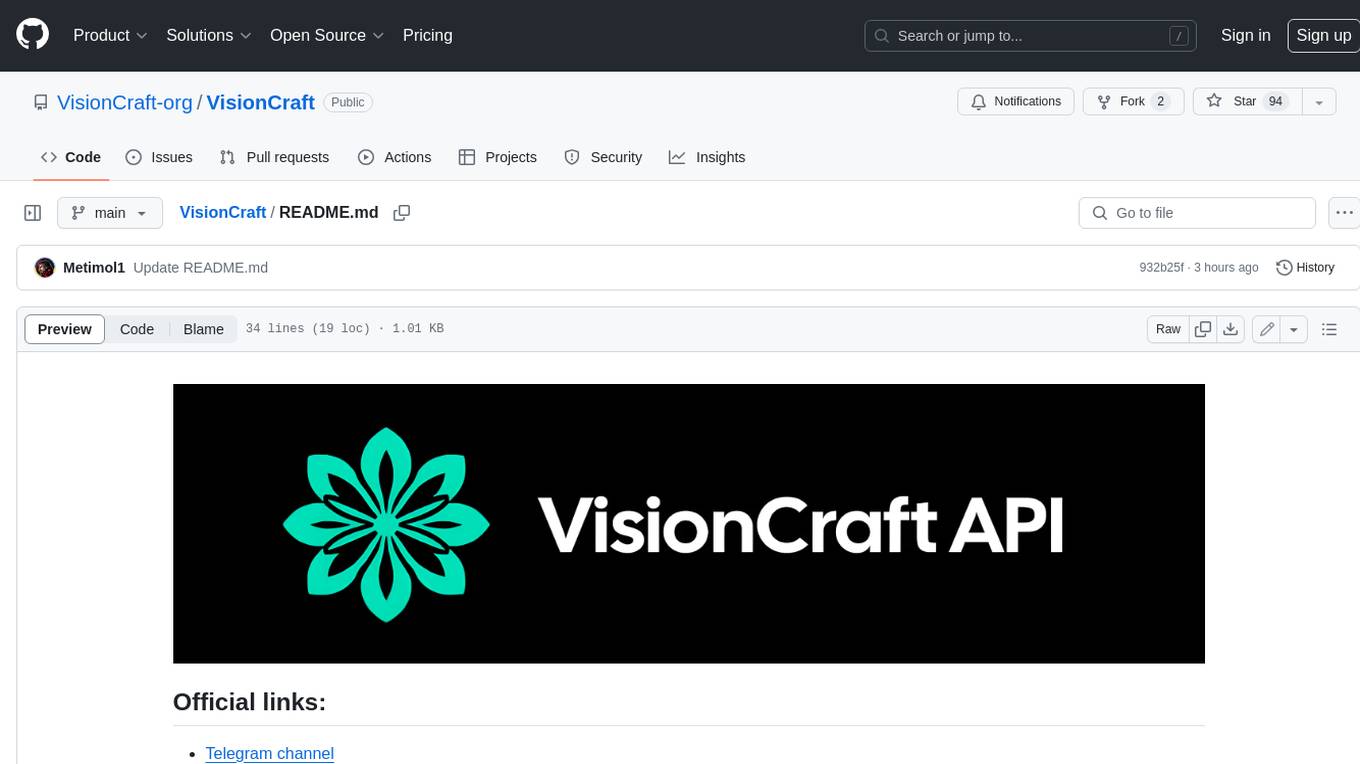
VisionCraft
The VisionCraft API is a free API for using over 100 different AI models. From images to sound.
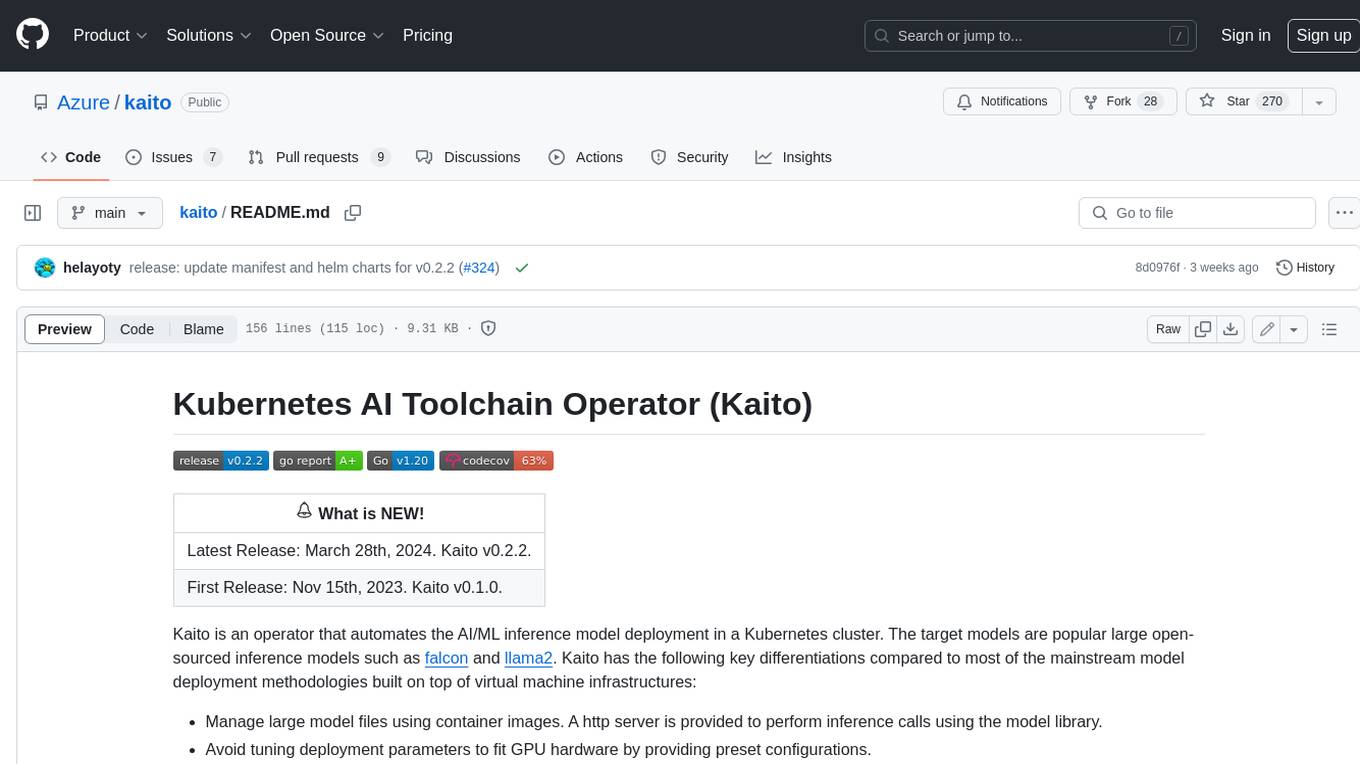
kaito
Kaito is an operator that automates the AI/ML inference model deployment in a Kubernetes cluster. It manages large model files using container images, avoids tuning deployment parameters to fit GPU hardware by providing preset configurations, auto-provisions GPU nodes based on model requirements, and hosts large model images in the public Microsoft Container Registry (MCR) if the license allows. Using Kaito, the workflow of onboarding large AI inference models in Kubernetes is largely simplified.
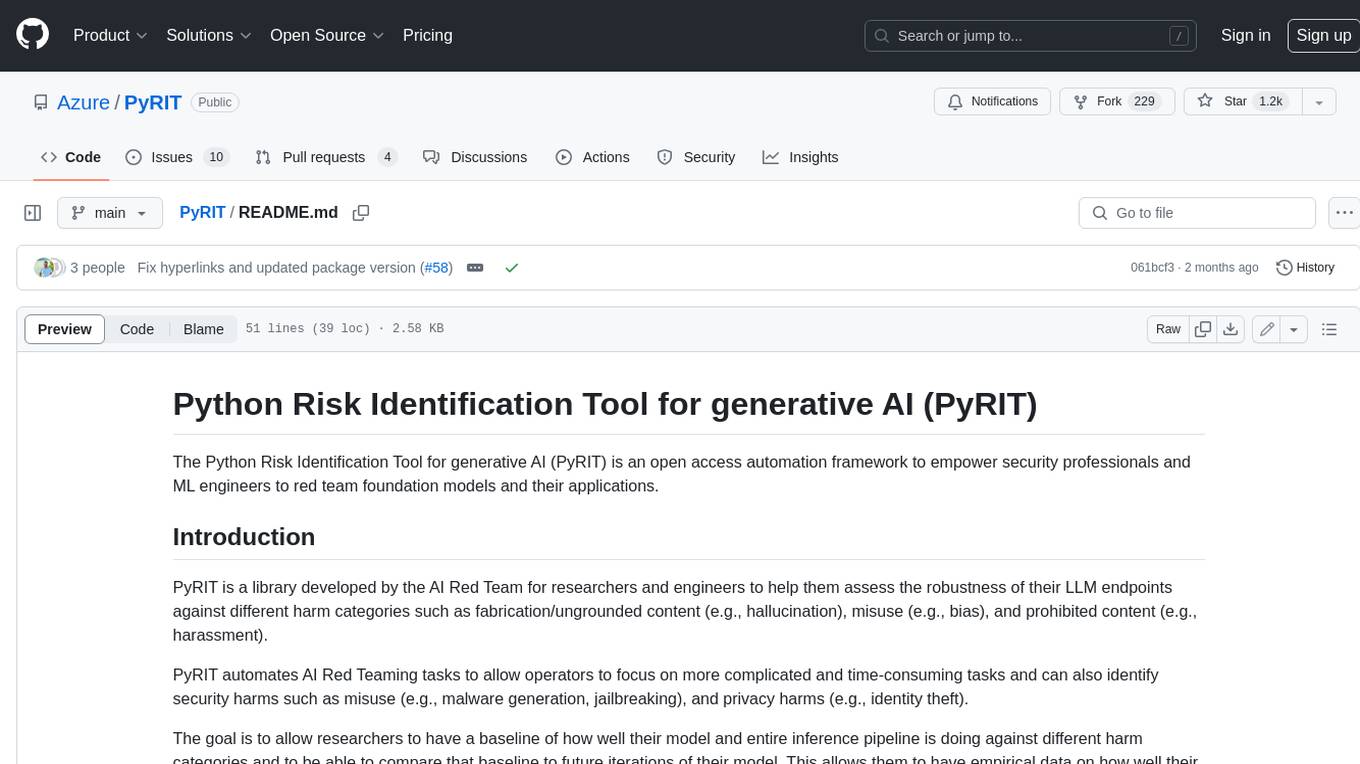
PyRIT
PyRIT is an open access automation framework designed to empower security professionals and ML engineers to red team foundation models and their applications. It automates AI Red Teaming tasks to allow operators to focus on more complicated and time-consuming tasks and can also identify security harms such as misuse (e.g., malware generation, jailbreaking), and privacy harms (e.g., identity theft). The goal is to allow researchers to have a baseline of how well their model and entire inference pipeline is doing against different harm categories and to be able to compare that baseline to future iterations of their model. This allows them to have empirical data on how well their model is doing today, and detect any degradation of performance based on future improvements.
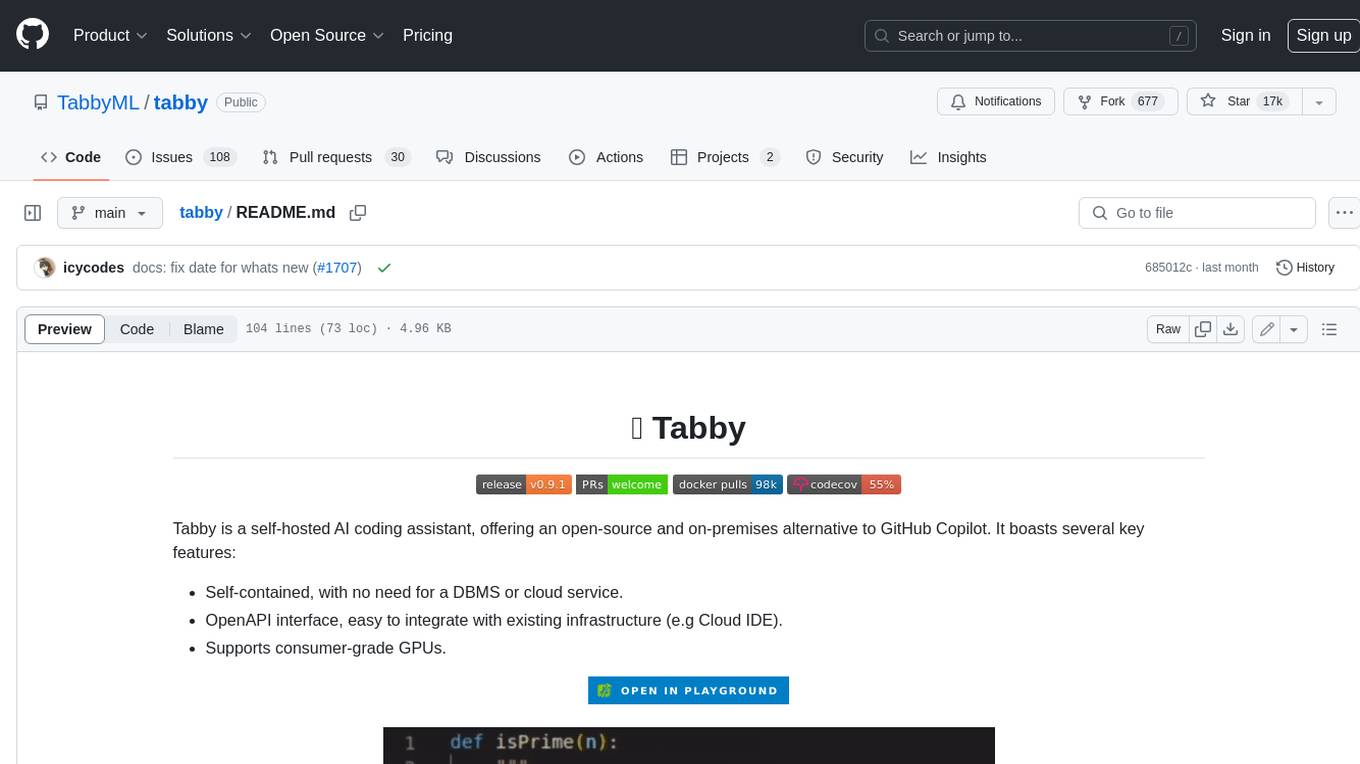
tabby
Tabby is a self-hosted AI coding assistant, offering an open-source and on-premises alternative to GitHub Copilot. It boasts several key features: * Self-contained, with no need for a DBMS or cloud service. * OpenAPI interface, easy to integrate with existing infrastructure (e.g Cloud IDE). * Supports consumer-grade GPUs.
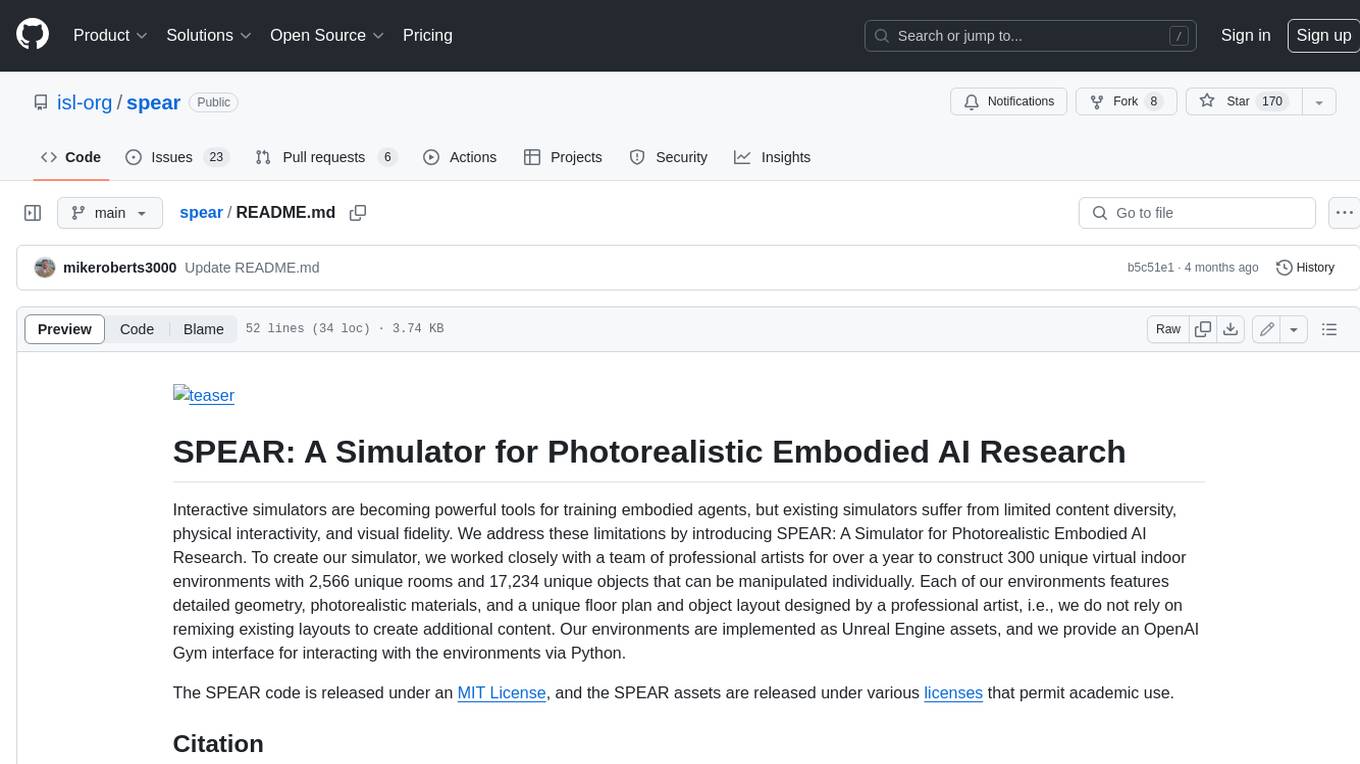
spear
SPEAR (Simulator for Photorealistic Embodied AI Research) is a powerful tool for training embodied agents. It features 300 unique virtual indoor environments with 2,566 unique rooms and 17,234 unique objects that can be manipulated individually. Each environment is designed by a professional artist and features detailed geometry, photorealistic materials, and a unique floor plan and object layout. SPEAR is implemented as Unreal Engine assets and provides an OpenAI Gym interface for interacting with the environments via Python.
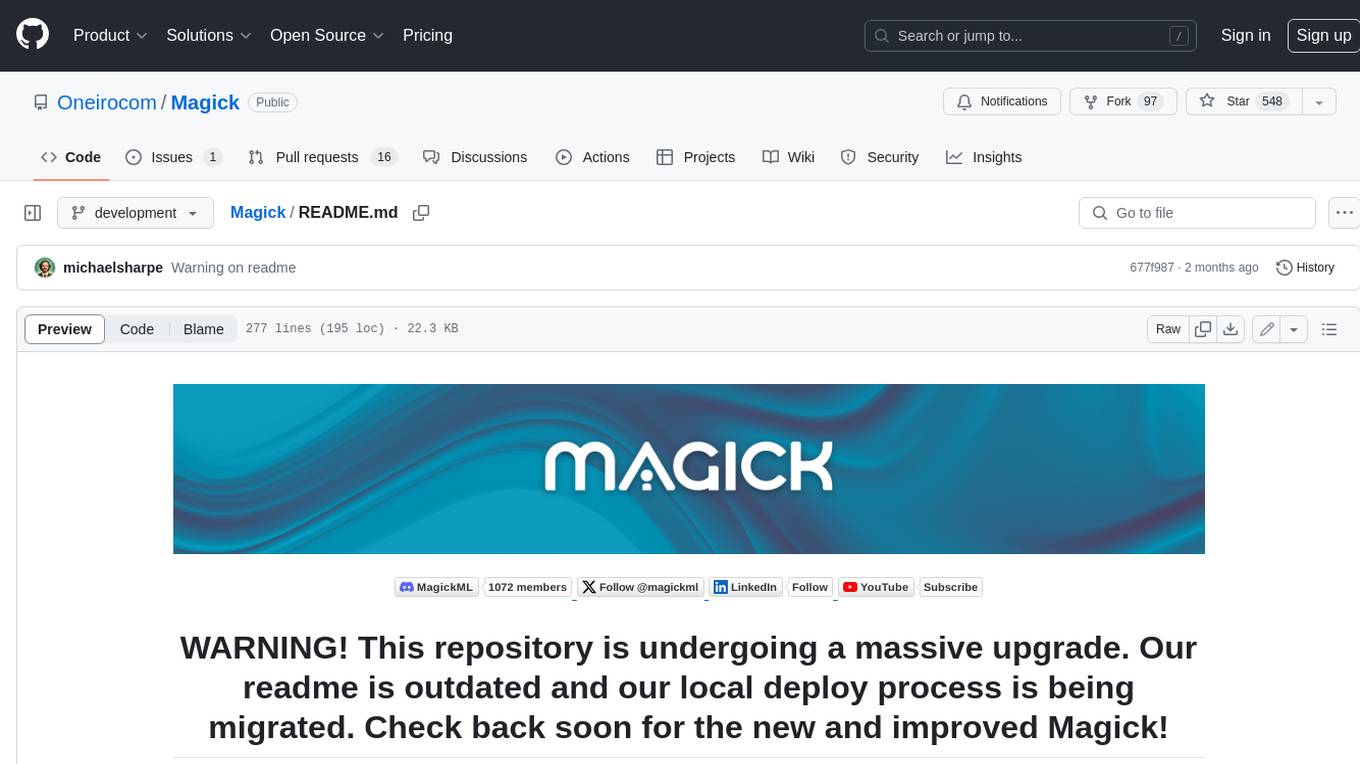
Magick
Magick is a groundbreaking visual AIDE (Artificial Intelligence Development Environment) for no-code data pipelines and multimodal agents. Magick can connect to other services and comes with nodes and templates well-suited for intelligent agents, chatbots, complex reasoning systems and realistic characters.