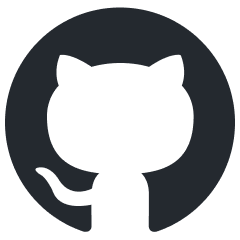
olmocr
Toolkit for linearizing PDFs for LLM datasets/training
Stars: 8237
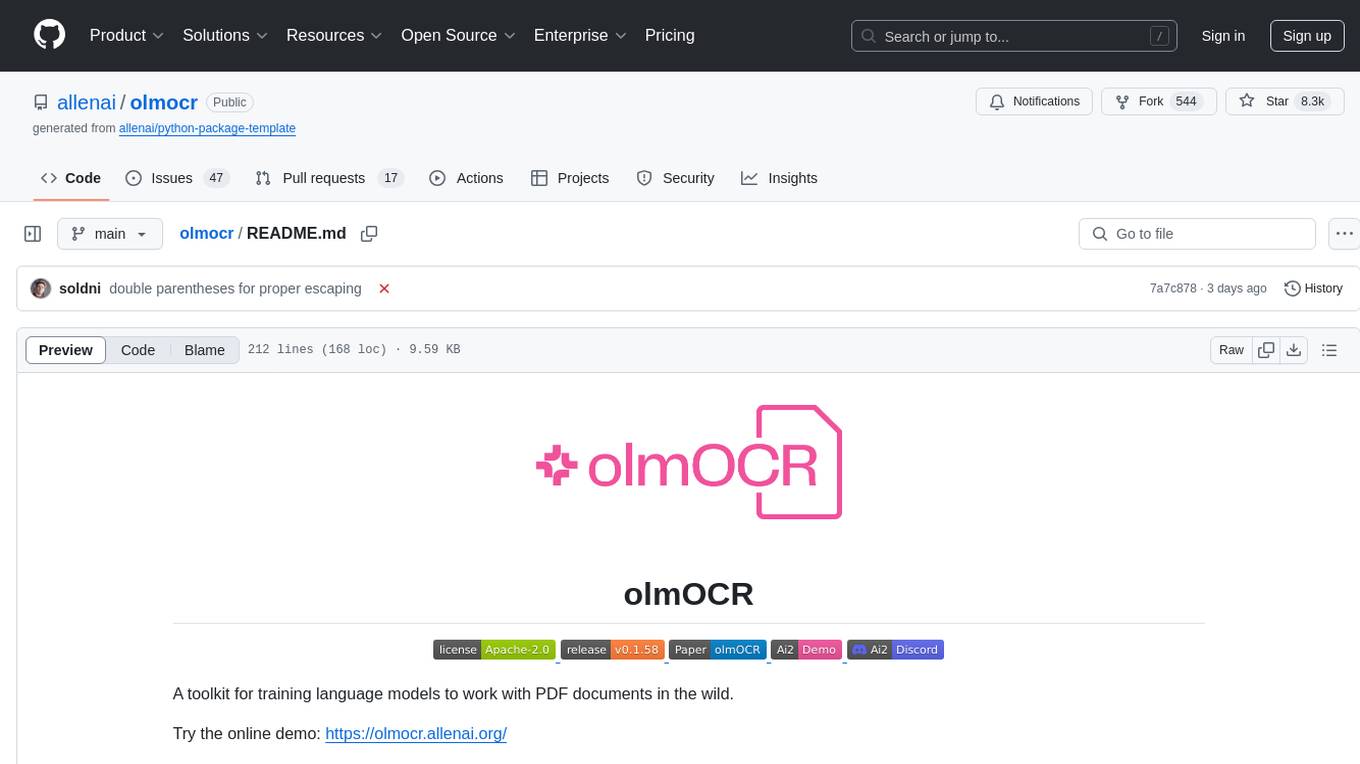
olmOCR is a toolkit designed for training language models to work with PDF documents in real-world scenarios. It includes various components such as a prompting strategy for natural text parsing, an evaluation toolkit for comparing pipeline versions, filtering by language and SEO spam removal, finetuning code for specific models, processing PDFs through a finetuned model, and viewing documents created from PDFs. The toolkit requires a recent NVIDIA GPU with at least 20 GB of RAM and 30GB of free disk space. Users can install dependencies, set up a conda environment, and utilize olmOCR for tasks like converting single or multiple PDFs, viewing extracted text, and running batch inference pipelines.
README:
A toolkit for training language models to work with PDF documents in the wild.
Try the online demo: https://olmocr.allenai.org/
What is included:
- A prompting strategy to get really good natural text parsing using ChatGPT 4o - buildsilver.py
- An side-by-side eval toolkit for comparing different pipeline versions - runeval.py
- Basic filtering by language and SEO spam removal - filter.py
- Finetuning code for Qwen2-VL and Molmo-O - train.py
- Processing millions of PDFs through a finetuned model using Sglang - pipeline.py
- Viewing Dolma docs created from PDFs - dolmaviewer.py
Requirements:
- Recent NVIDIA GPU (tested on RTX 4090, L40S, A100, H100) with at least 20 GB of GPU RAM
- 30GB of free disk space
You will need to install poppler-utils and additional fonts for rendering PDF images.
Install dependencies (Ubuntu/Debian)
sudo apt-get update
sudo apt-get install poppler-utils ttf-mscorefonts-installer msttcorefonts fonts-crosextra-caladea fonts-crosextra-carlito gsfonts lcdf-typetools
Set up a conda environment and install olmocr
conda create -n olmocr python=3.11
conda activate olmocr
git clone https://github.com/allenai/olmocr.git
cd olmocr
pip install -e .
Install sglang with flashinfer if you want to run inference on GPU.
pip install sgl-kernel==0.0.3.post1 --force-reinstall --no-deps
pip install "sglang[all]==0.4.2" --find-links https://flashinfer.ai/whl/cu124/torch2.4/flashinfer/
For quick testing, try the web demo. To run locally, a GPU is required, as inference is powered by sglang under the hood. Convert a Single PDF:
python -m olmocr.pipeline ./localworkspace --pdfs tests/gnarly_pdfs/horribleocr.pdf
Convert Multiple PDFs:
python -m olmocr.pipeline ./localworkspace --pdfs tests/gnarly_pdfs/*.pdf
Results will be stored as JSON in ./localworkspace
.
Extracted text is stored as Dolma-style JSONL inside of the ./localworkspace/results
directory.
cat localworkspace/results/output_*.jsonl
View results side-by-side with the original PDFs (uses dolmaviewer
command):
python -m olmocr.viewer.dolmaviewer localworkspace/results/output_*.jsonl
Now open ./dolma_previews/tests_gnarly_pdfs_horribleocr_pdf.html
in your favorite browser.
If you want to convert millions of PDFs, using multiple nodes running in parallel, then olmOCR supports reading your PDFs from AWS S3, and coordinating work using an AWS S3 output bucket.
For example, you can start this command on your first worker node, and it will set up a simple work queue in your AWS bucket and start converting PDFs.
python -m olmocr.pipeline s3://my_s3_bucket/pdfworkspaces/exampleworkspace --pdfs s3://my_s3_bucket/jakep/gnarly_pdfs/*.pdf
Now on any subsequent nodes, just run this and they will start grabbing items from the same workspace queue.
python -m olmocr.pipeline s3://my_s3_bucket/pdfworkspaces/exampleworkspace
If you are at Ai2 and want to linearize millions of PDFs efficiently using beaker, just add the --beaker
flag. This will prepare the workspace on your local machine, and then launch N GPU workers in the cluster to start
converting PDFs.
For example:
python -m olmocr.pipeline s3://my_s3_bucket/pdfworkspaces/exampleworkspace --pdfs s3://my_s3_bucket/jakep/gnarly_pdfs/*.pdf --beaker --beaker_gpus 4
python -m olmocr.pipeline --help
usage: pipeline.py [-h] [--pdfs PDFS] [--workspace_profile WORKSPACE_PROFILE] [--pdf_profile PDF_PROFILE] [--pages_per_group PAGES_PER_GROUP]
[--max_page_retries MAX_PAGE_RETRIES] [--max_page_error_rate MAX_PAGE_ERROR_RATE] [--workers WORKERS] [--apply_filter] [--stats] [--model MODEL]
[--model_max_context MODEL_MAX_CONTEXT] [--model_chat_template MODEL_CHAT_TEMPLATE] [--target_longest_image_dim TARGET_LONGEST_IMAGE_DIM]
[--target_anchor_text_len TARGET_ANCHOR_TEXT_LEN] [--beaker] [--beaker_workspace BEAKER_WORKSPACE] [--beaker_cluster BEAKER_CLUSTER]
[--beaker_gpus BEAKER_GPUS] [--beaker_priority BEAKER_PRIORITY]
workspace
Manager for running millions of PDFs through a batch inference pipeline
positional arguments:
workspace The filesystem path where work will be stored, can be a local folder, or an s3 path if coordinating work with many workers, s3://bucket/prefix/
options:
-h, --help show this help message and exit
--pdfs PDFS Path to add pdfs stored in s3 to the workspace, can be a glob path s3://bucket/prefix/*.pdf or path to file containing list of pdf paths
--workspace_profile WORKSPACE_PROFILE
S3 configuration profile for accessing the workspace
--pdf_profile PDF_PROFILE
S3 configuration profile for accessing the raw pdf documents
--pages_per_group PAGES_PER_GROUP
Aiming for this many pdf pages per work item group
--max_page_retries MAX_PAGE_RETRIES
Max number of times we will retry rendering a page
--max_page_error_rate MAX_PAGE_ERROR_RATE
Rate of allowable failed pages in a document, 1/250 by default
--workers WORKERS Number of workers to run at a time
--apply_filter Apply basic filtering to English pdfs which are not forms, and not likely seo spam
--stats Instead of running any job, reports some statistics about the current workspace
--model MODEL List of paths where you can find the model to convert this pdf. You can specify several different paths here, and the script will try to use the
one which is fastest to access
--model_max_context MODEL_MAX_CONTEXT
Maximum context length that the model was fine tuned under
--model_chat_template MODEL_CHAT_TEMPLATE
Chat template to pass to sglang server
--target_longest_image_dim TARGET_LONGEST_IMAGE_DIM
Dimension on longest side to use for rendering the pdf pages
--target_anchor_text_len TARGET_ANCHOR_TEXT_LEN
Maximum amount of anchor text to use (characters)
--beaker Submit this job to beaker instead of running locally
--beaker_workspace BEAKER_WORKSPACE
Beaker workspace to submit to
--beaker_cluster BEAKER_CLUSTER
Beaker clusters you want to run on
--beaker_gpus BEAKER_GPUS
Number of gpu replicas to run
--beaker_priority BEAKER_PRIORITY
Beaker priority level for the job
olmOCR is developed and maintained by the AllenNLP team, backed by the Allen Institute for Artificial Intelligence (AI2). AI2 is a non-profit institute with the mission to contribute to humanity through high-impact AI research and engineering. To learn more about who specifically contributed to this codebase, see our contributors page.
olmOCR is licensed under Apache 2.0. A full copy of the license can be found on GitHub.
@misc{olmocr,
title={{olmOCR: Unlocking Trillions of Tokens in PDFs with Vision Language Models}},
author={Jake Poznanski and Jon Borchardt and Jason Dunkelberger and Regan Huff and Daniel Lin and Aman Rangapur and Christopher Wilhelm and Kyle Lo and Luca Soldaini},
year={2025},
eprint={2502.18443},
archivePrefix={arXiv},
primaryClass={cs.CL},
url={https://arxiv.org/abs/2502.18443},
}
For Tasks:
Click tags to check more tools for each tasksFor Jobs:
Alternative AI tools for olmocr
Similar Open Source Tools
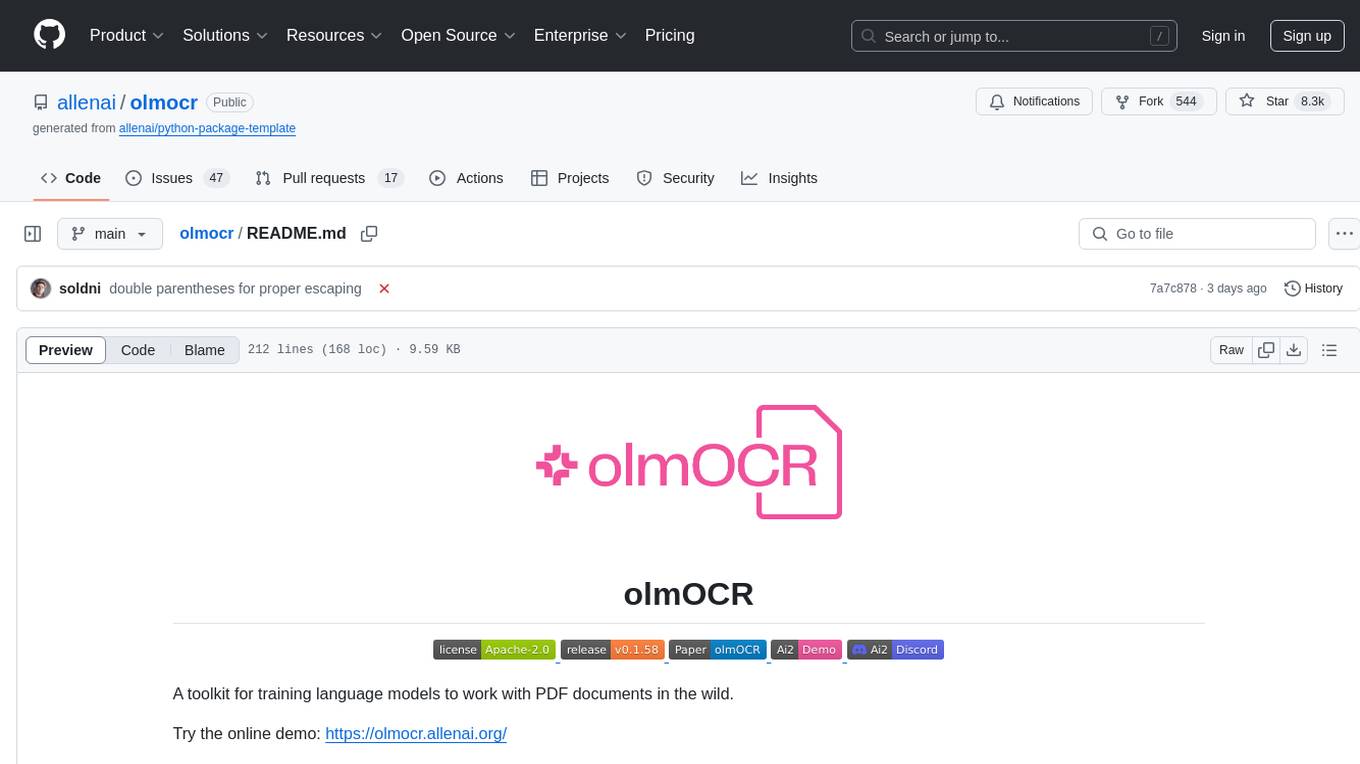
olmocr
olmOCR is a toolkit designed for training language models to work with PDF documents in real-world scenarios. It includes various components such as a prompting strategy for natural text parsing, an evaluation toolkit for comparing pipeline versions, filtering by language and SEO spam removal, finetuning code for specific models, processing PDFs through a finetuned model, and viewing documents created from PDFs. The toolkit requires a recent NVIDIA GPU with at least 20 GB of RAM and 30GB of free disk space. Users can install dependencies, set up a conda environment, and utilize olmOCR for tasks like converting single or multiple PDFs, viewing extracted text, and running batch inference pipelines.
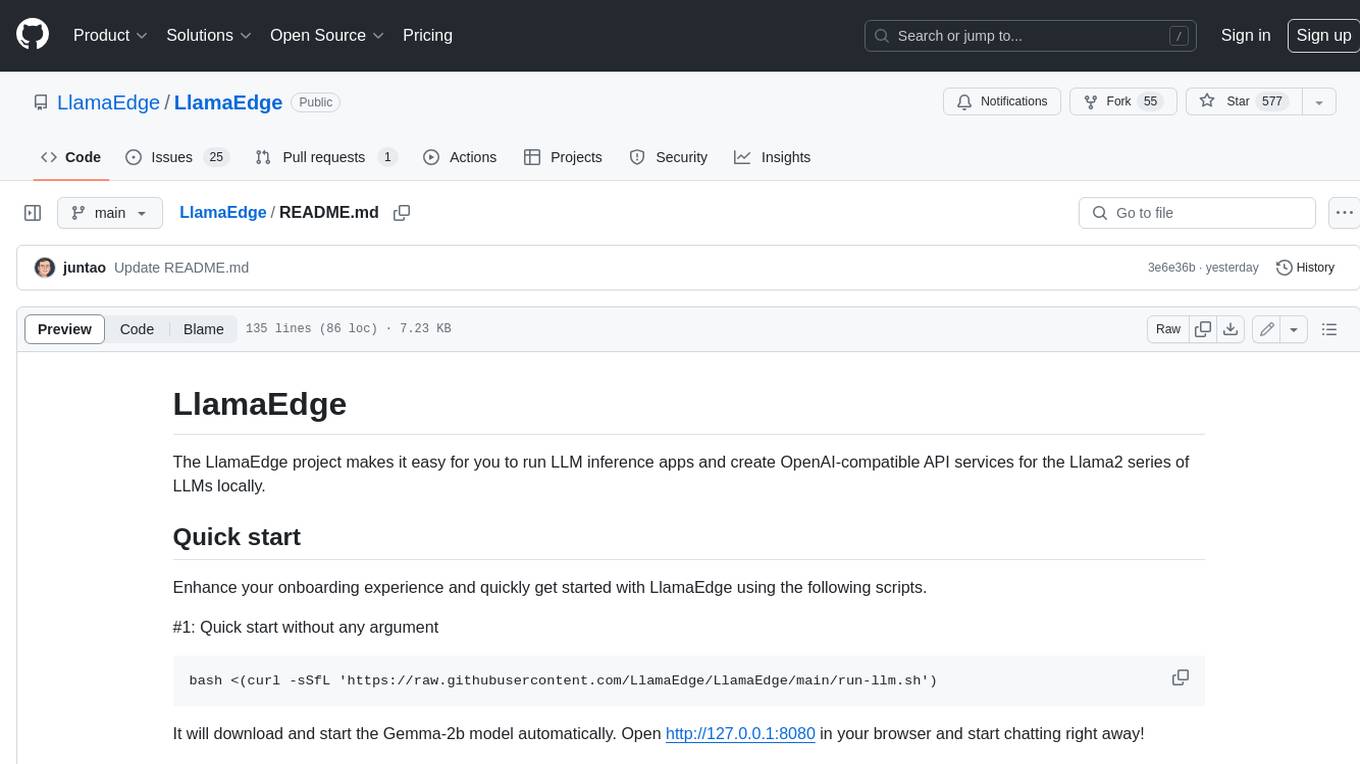
LlamaEdge
The LlamaEdge project makes it easy to run LLM inference apps and create OpenAI-compatible API services for the Llama2 series of LLMs locally. It provides a Rust+Wasm stack for fast, portable, and secure LLM inference on heterogeneous edge devices. The project includes source code for text generation, chatbot, and API server applications, supporting all LLMs based on the llama2 framework in the GGUF format. LlamaEdge is committed to continuously testing and validating new open-source models and offers a list of supported models with download links and startup commands. It is cross-platform, supporting various OSes, CPUs, and GPUs, and provides troubleshooting tips for common errors.
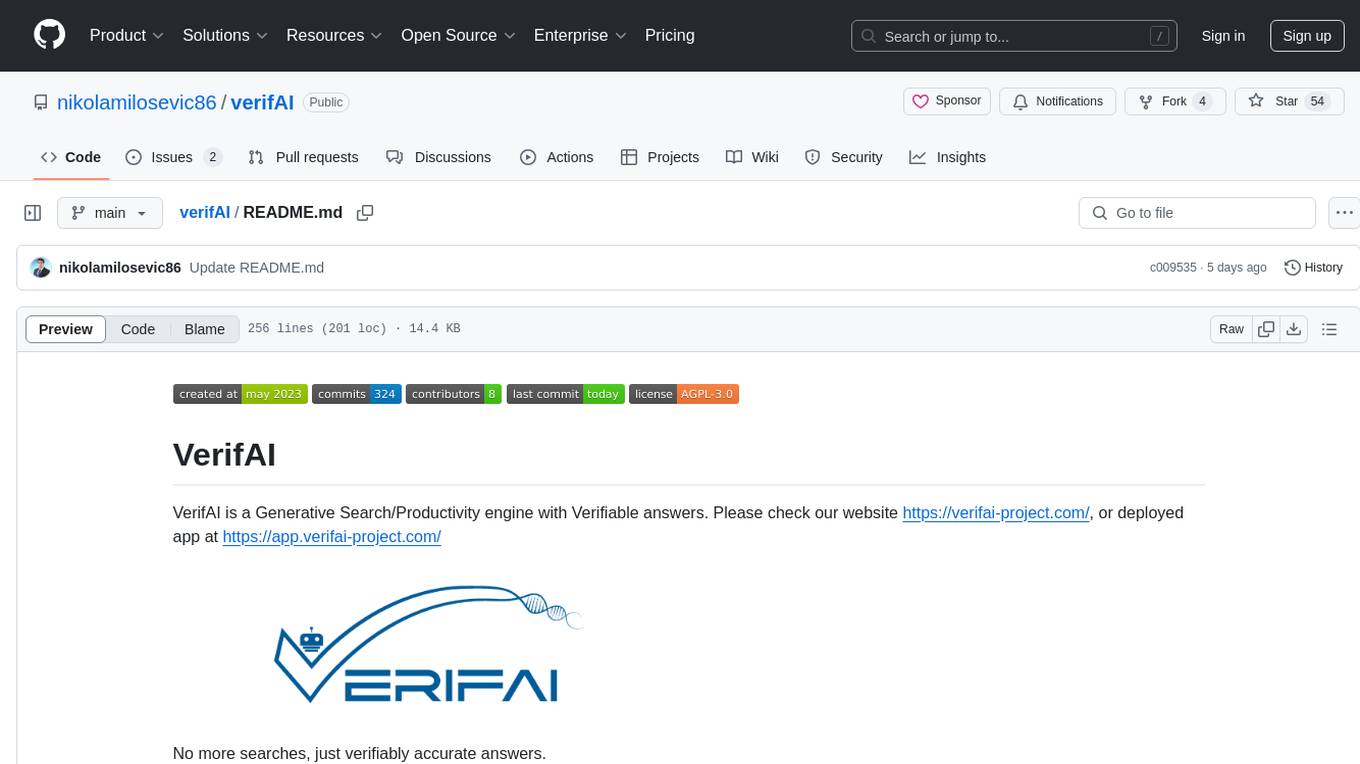
verifAI
VerifAI is a document-based question-answering system that addresses hallucinations in generative large language models and search engines. It retrieves relevant documents, generates answers with references, and verifies answers for accuracy. The engine uses generative search technology and a verification model to ensure no misinformation. VerifAI supports various document formats and offers user registration with a React.js interface. It is open-source and designed to be user-friendly, making it accessible for anyone to use.
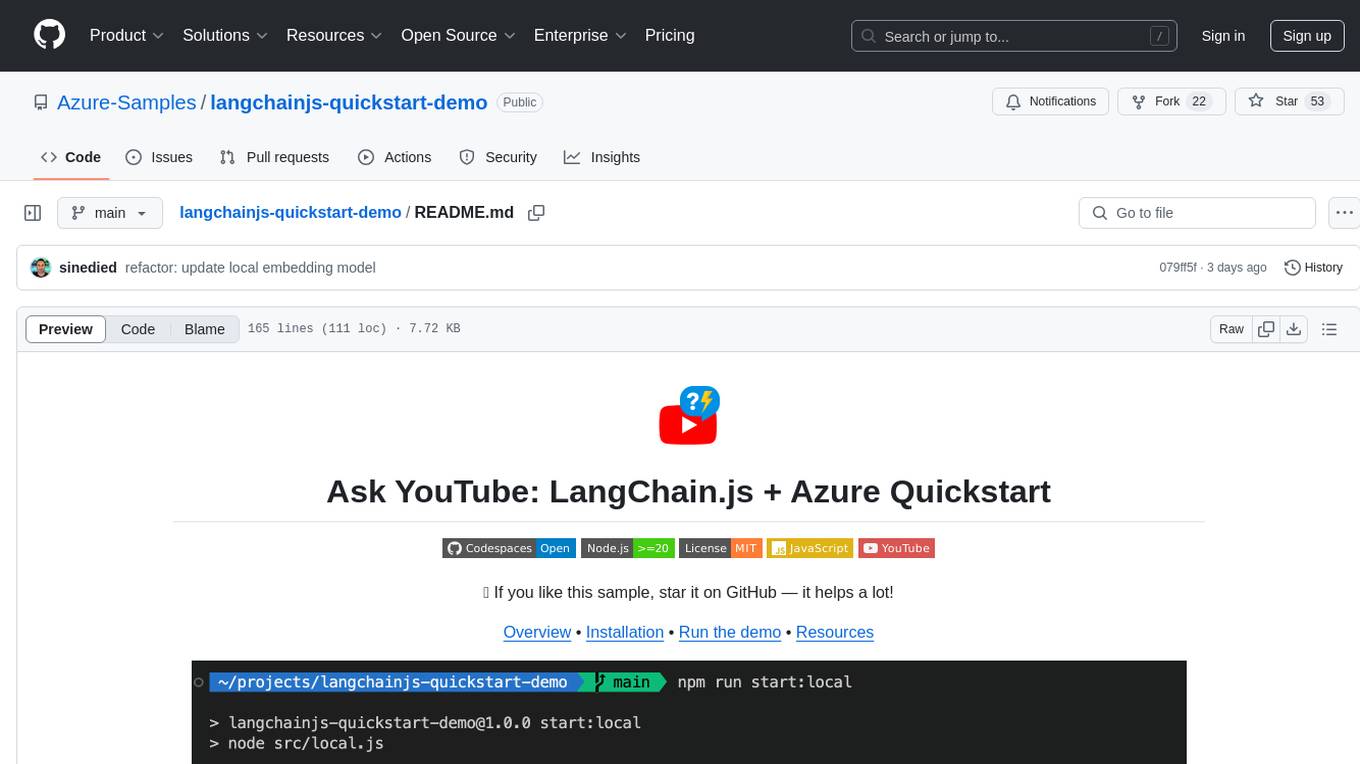
langchainjs-quickstart-demo
Discover the journey of building a generative AI application using LangChain.js and Azure. This demo explores the development process from idea to production, using a RAG-based approach for a Q&A system based on YouTube video transcripts. The application allows to ask text-based questions about a YouTube video and uses the transcript of the video to generate responses. The code comes in two versions: local prototype using FAISS and Ollama with LLaMa3 model for completion and all-minilm-l6-v2 for embeddings, and Azure cloud version using Azure AI Search and GPT-4 Turbo model for completion and text-embedding-3-large for embeddings. Either version can be run as an API using the Azure Functions runtime.
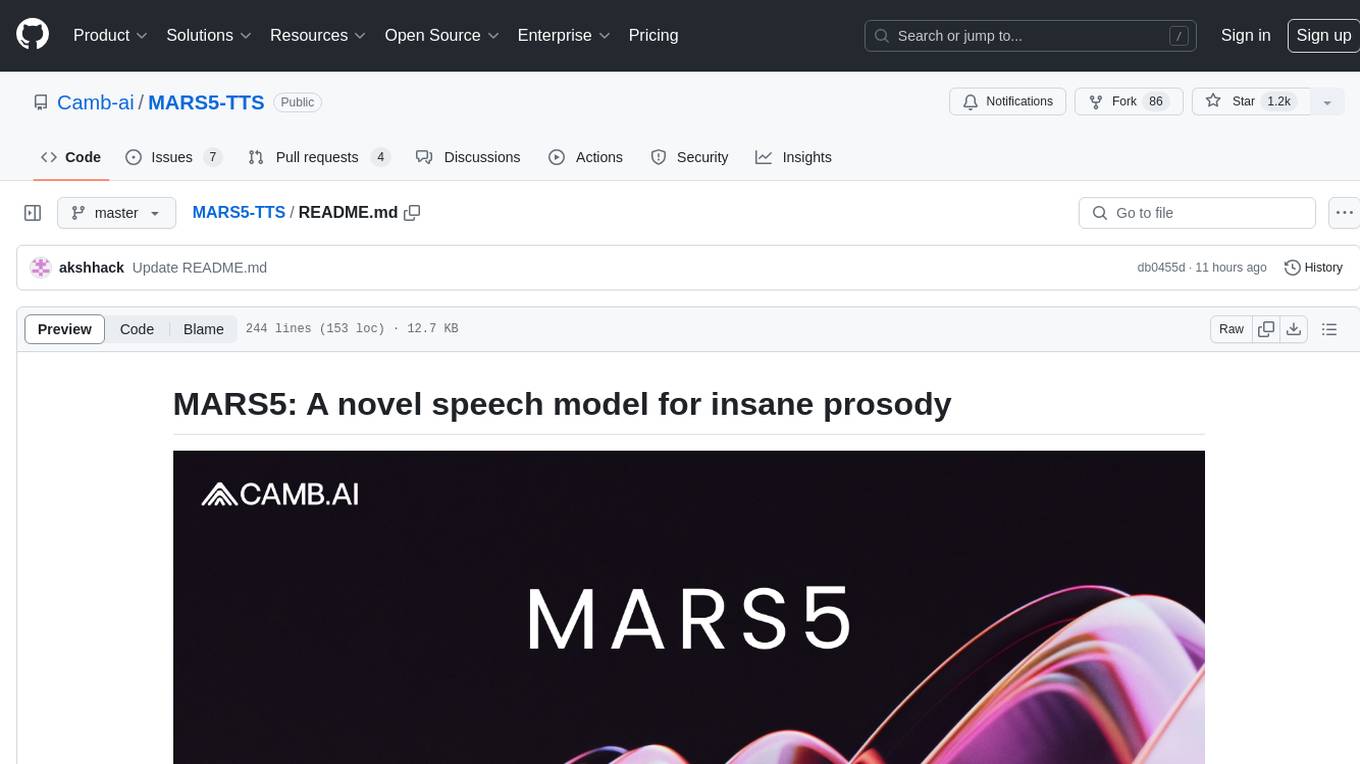
MARS5-TTS
MARS5 is a novel English speech model (TTS) developed by CAMB.AI, featuring a two-stage AR-NAR pipeline with a unique NAR component. The model can generate speech for various scenarios like sports commentary and anime with just 5 seconds of audio and a text snippet. It allows steering prosody using punctuation and capitalization in the transcript. Speaker identity is specified using an audio reference file, enabling 'deep clone' for improved quality. The model can be used via torch.hub or HuggingFace, supporting both shallow and deep cloning for inference. Checkpoints are provided for AR and NAR models, with hardware requirements of 750M+450M params on GPU. Contributions to improve model stability, performance, and reference audio selection are welcome.
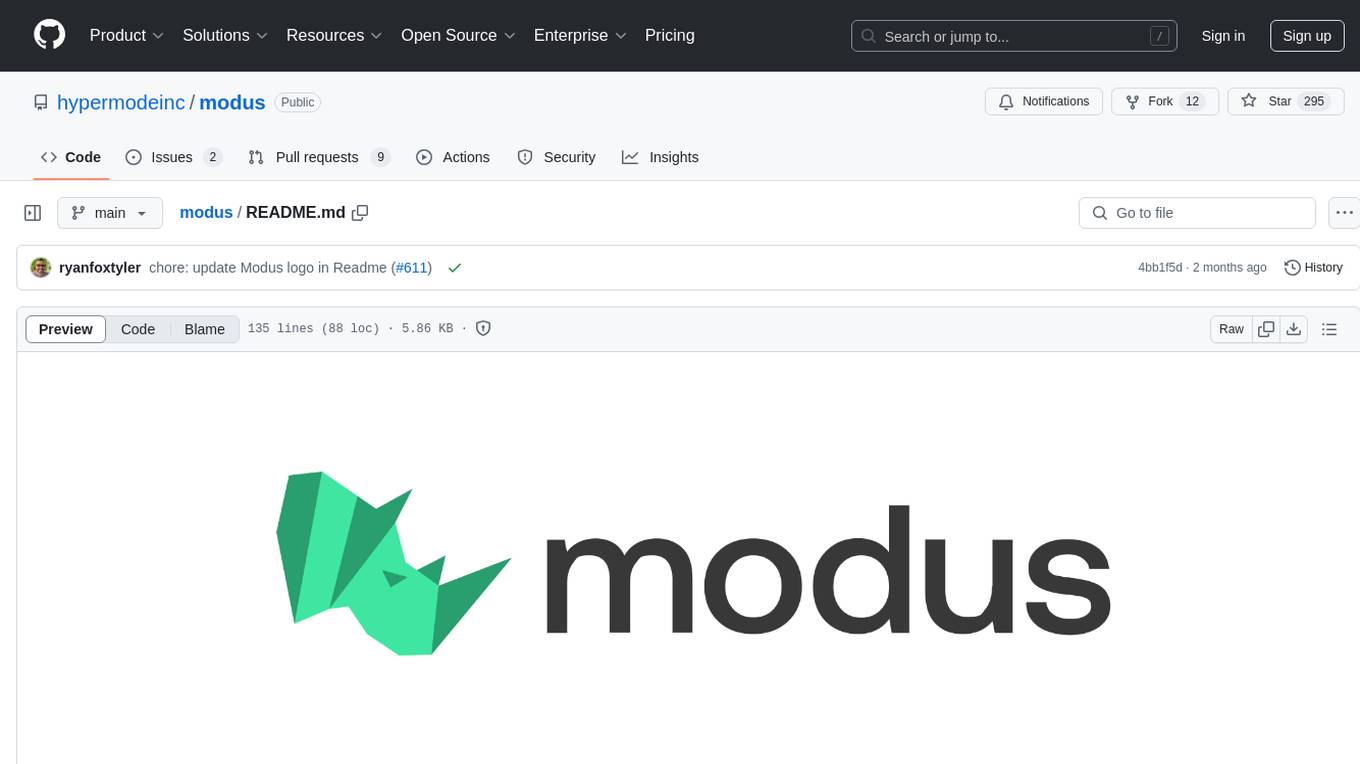
modus
Modus is an open-source, serverless framework for building APIs powered by WebAssembly. It simplifies integrating AI models, data, and business logic with sandboxed execution. Modus extracts metadata, compiles code with optimizations, caches compiled modules, prepares invocation plans, generates API schema, and activates endpoints. Users query the endpoint, and Modus loads compiled code into a sandboxed environment, runs code securely, queries data and AI models, and responds via API. It provides a production-ready scalable endpoint for AI-enabled apps, optimized for sub-second response times. Modus supports programming languages like AssemblyScript and Go, and can be hosted on Hypermode or any platform. Developed by Hypermode as an open-source project, Modus welcomes external contributions.
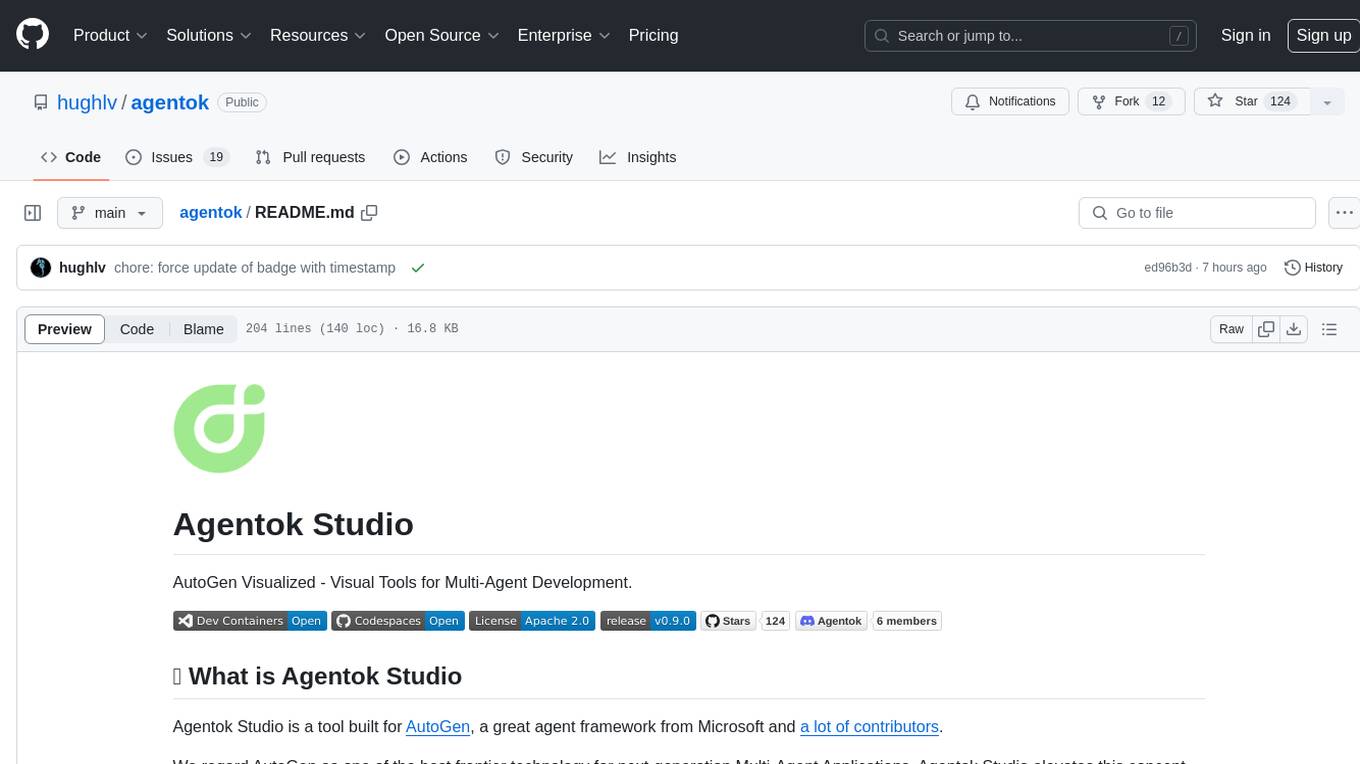
agentok
Agentok Studio is a visual tool built for AutoGen, a cutting-edge agent framework from Microsoft and various contributors. It offers intuitive visual tools to simplify the construction and management of complex agent-based workflows. Users can create workflows visually as graphs, chat with agents, and share flow templates. The tool is designed to streamline the development process for creators and developers working on next-generation Multi-Agent Applications.
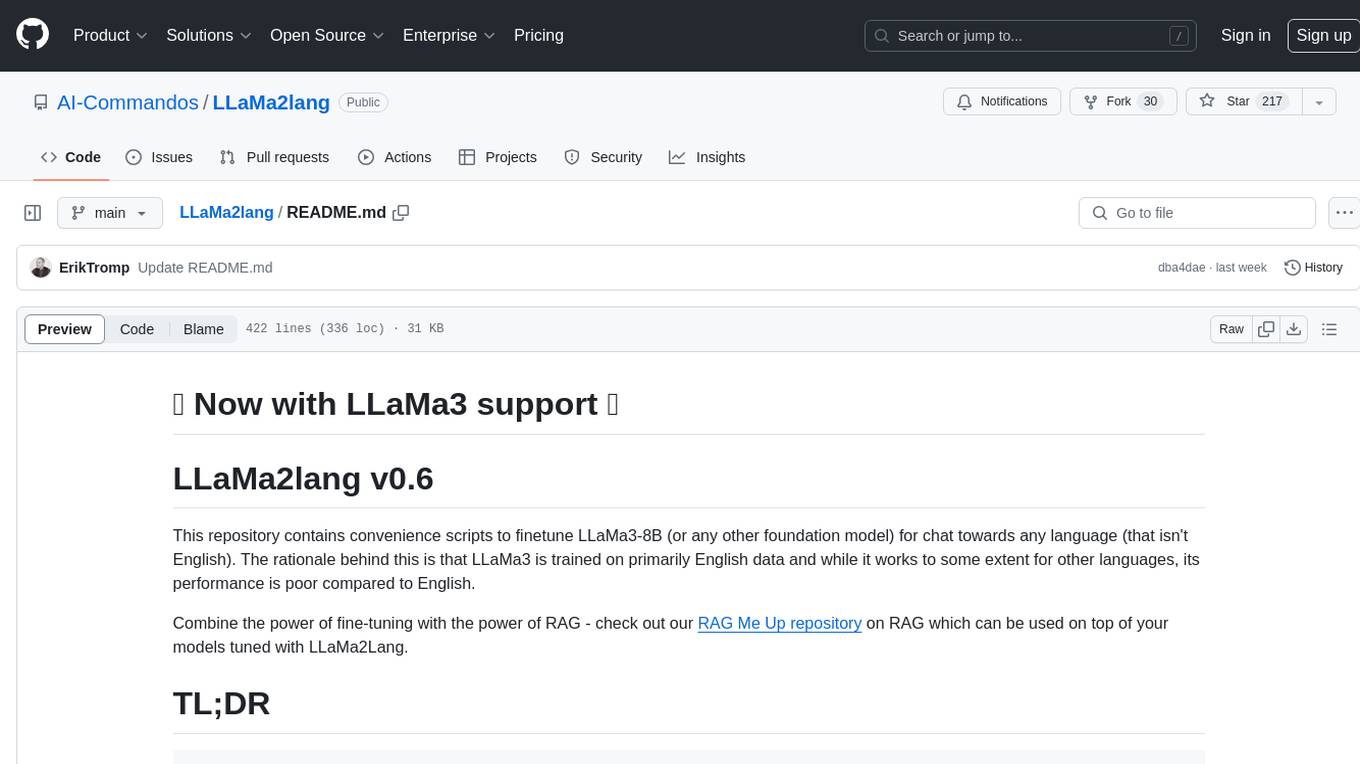
LLaMa2lang
LLaMa2lang is a repository containing convenience scripts to finetune LLaMa3-8B (or any other foundation model) for chat towards any language that isn't English. The repository aims to improve the performance of LLaMa3 for non-English languages by combining fine-tuning with RAG. Users can translate datasets, extract threads, turn threads into prompts, and finetune models using QLoRA and PEFT. Additionally, the repository supports translation models like OPUS, M2M, MADLAD, and base datasets like OASST1 and OASST2. The process involves loading datasets, translating them, combining checkpoints, and running inference using the newly trained model. The repository also provides benchmarking scripts to choose the right translation model for a target language.
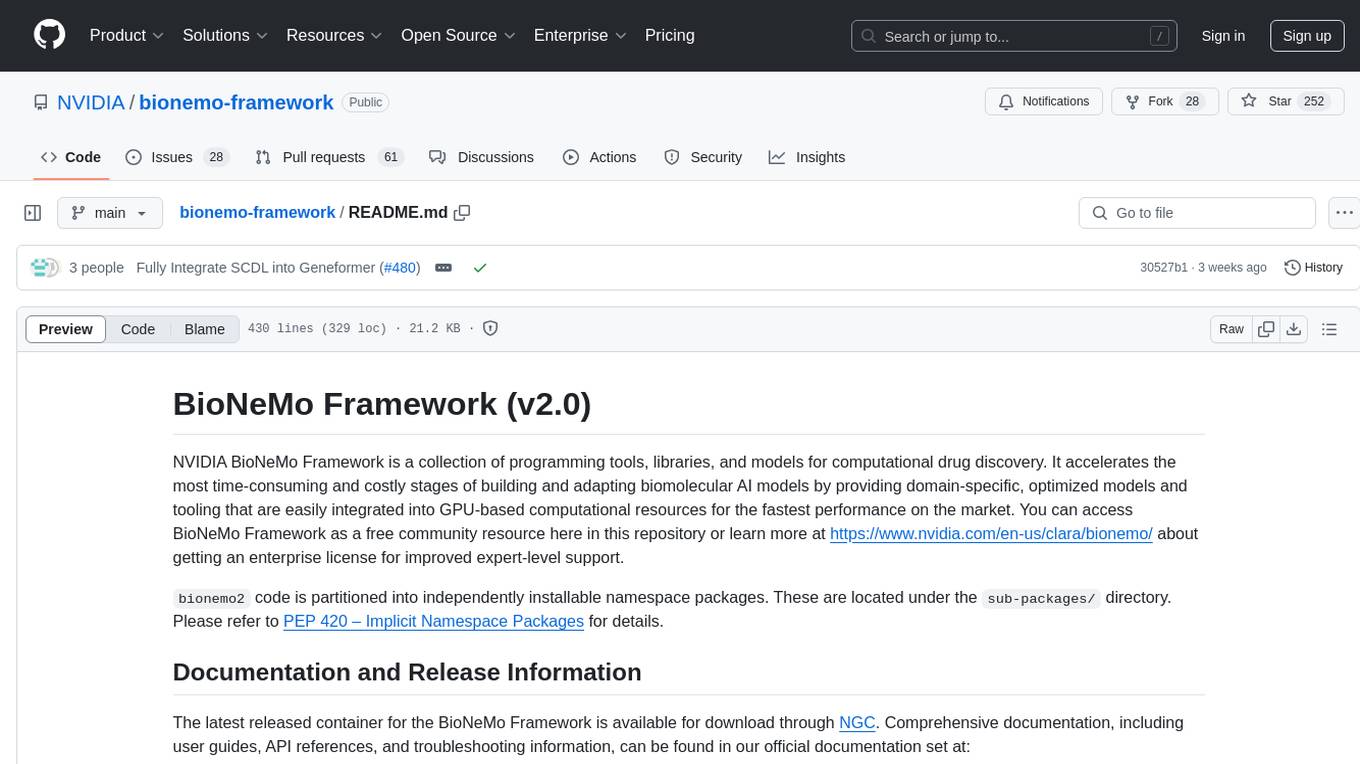
bionemo-framework
NVIDIA BioNeMo Framework is a collection of programming tools, libraries, and models for computational drug discovery. It accelerates building and adapting biomolecular AI models by providing domain-specific, optimized models and tooling for GPU-based computational resources. The framework offers comprehensive documentation and support for both community and enterprise users.
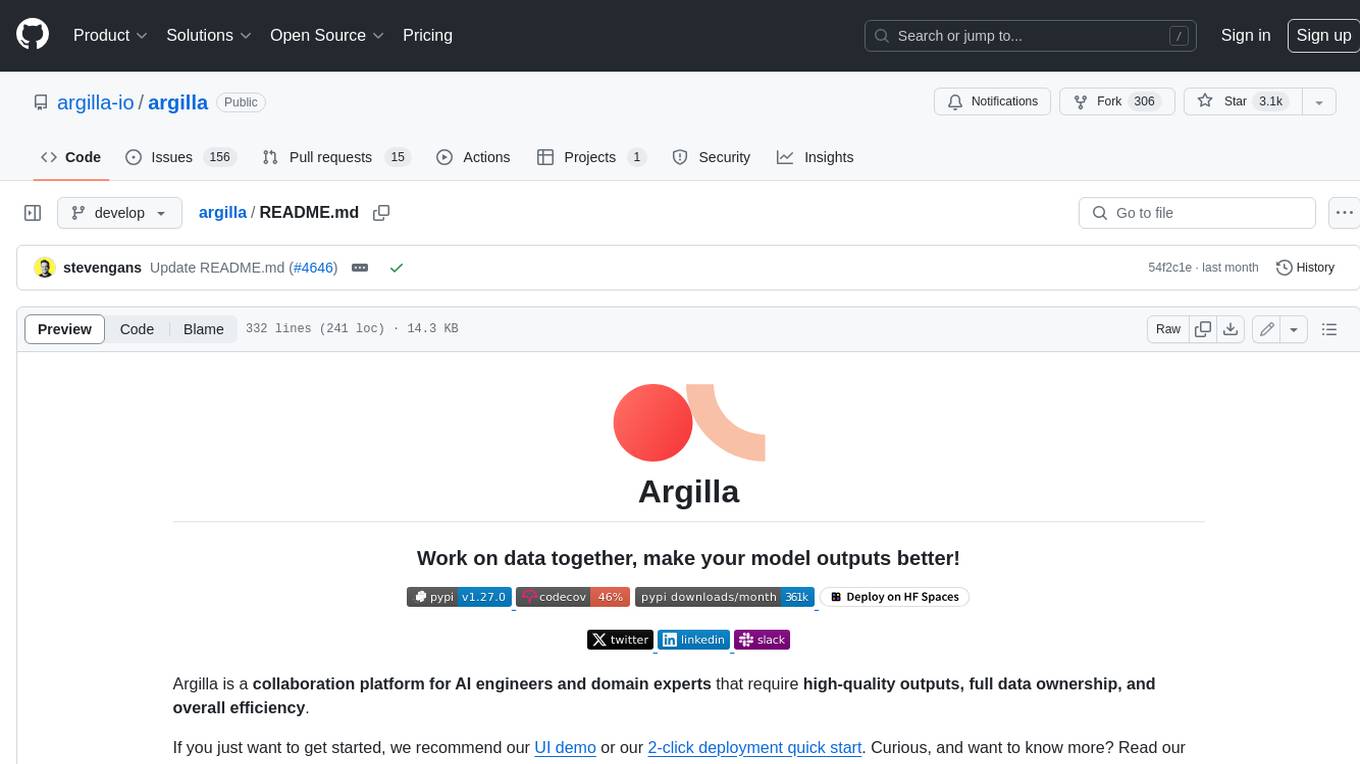
argilla
Argilla is a collaboration platform for AI engineers and domain experts that require high-quality outputs, full data ownership, and overall efficiency. It helps users improve AI output quality through data quality, take control of their data and models, and improve efficiency by quickly iterating on the right data and models. Argilla is an open-source community-driven project that provides tools for achieving and maintaining high-quality data standards, with a focus on NLP and LLMs. It is used by AI teams from companies like the Red Cross, Loris.ai, and Prolific to improve the quality and efficiency of AI projects.
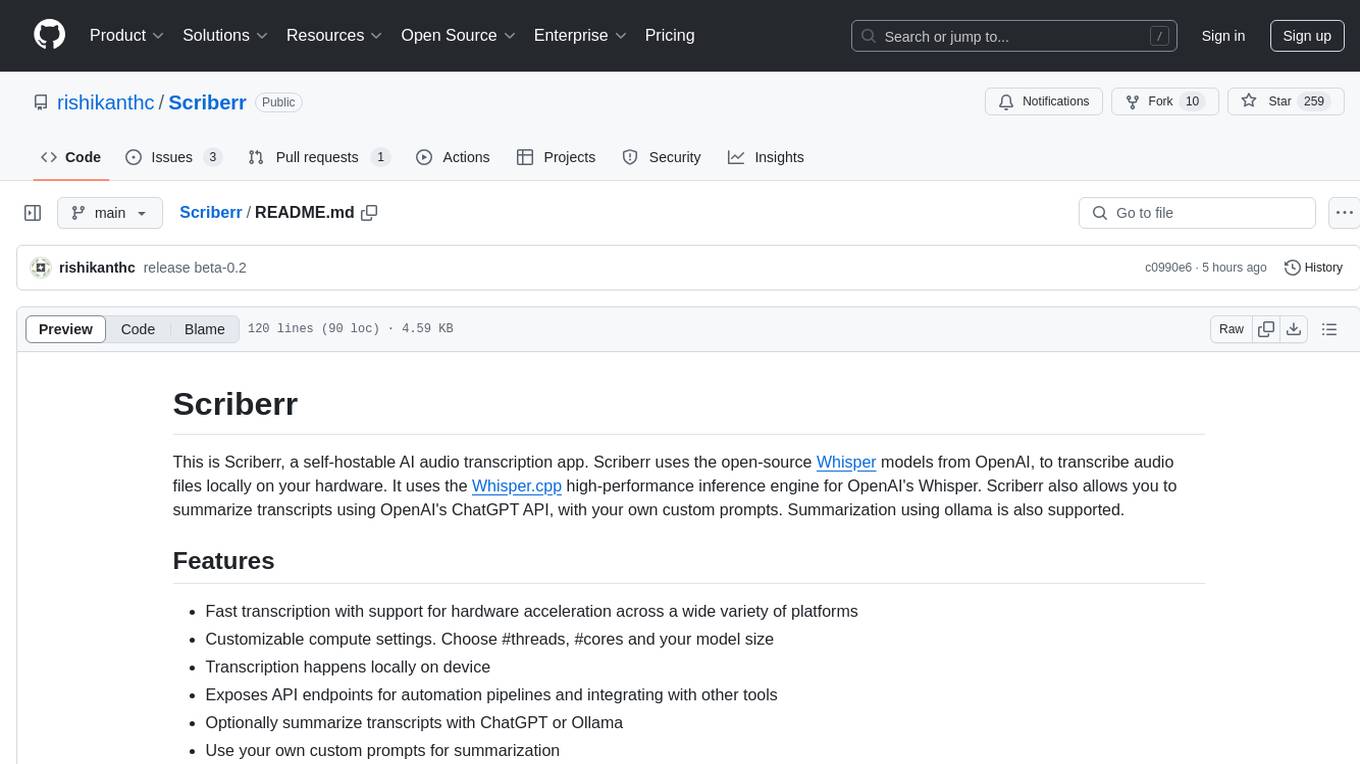
Scriberr
Scriberr is a self-hostable AI audio transcription app that utilizes open-source Whisper models from OpenAI for transcribing audio files locally on user's hardware. It offers fast transcription with customizable compute settings, local transcription on device, API endpoints for automation, and integration with other tools. Users can optionally summarize transcripts using ChatGPT or Ollama, with support for custom prompts. The app is mobile-ready, simple, and easy to use, with planned features including speaker diarization, audio recording, file actions, full text fuzzy search, tag-based organization, follow-along text with playback, edit summaries, export options, and support for other languages. Despite being in beta, Scriberr is functional and usable, albeit with some rough edges and minor bugs.
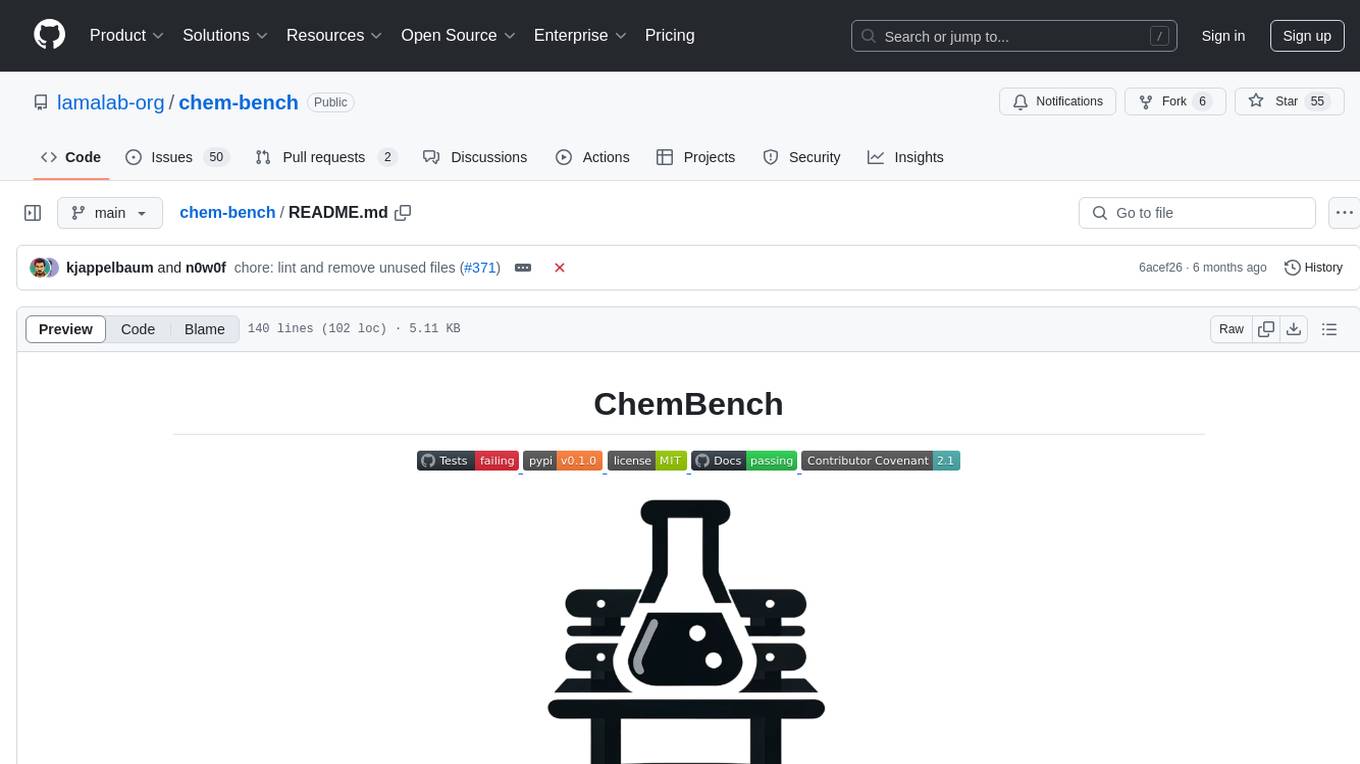
chem-bench
ChemBench is a project aimed at expanding chemistry benchmark tasks in a BIG-bench compatible way, providing a pipeline to benchmark frontier and open models. It allows users to run benchmarking tasks on models with existing presets, offering predefined parameters and processing steps. The library facilitates benchmarking models on the entire suite, addressing challenges such as prompt structure, parsing, and scoring methods. Users can contribute to the project by following the developer notes.
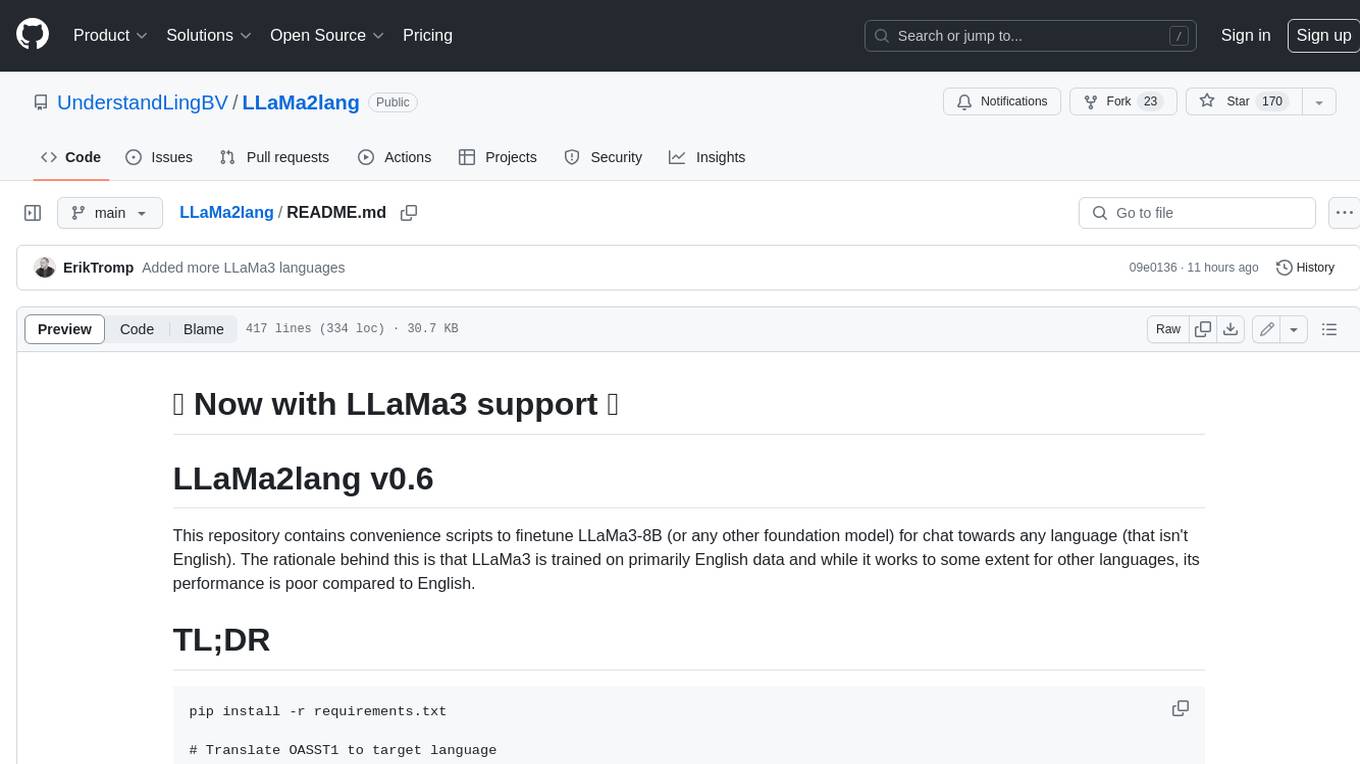
LLaMa2lang
This repository contains convenience scripts to finetune LLaMa3-8B (or any other foundation model) for chat towards any language (that isn't English). The rationale behind this is that LLaMa3 is trained on primarily English data and while it works to some extent for other languages, its performance is poor compared to English.
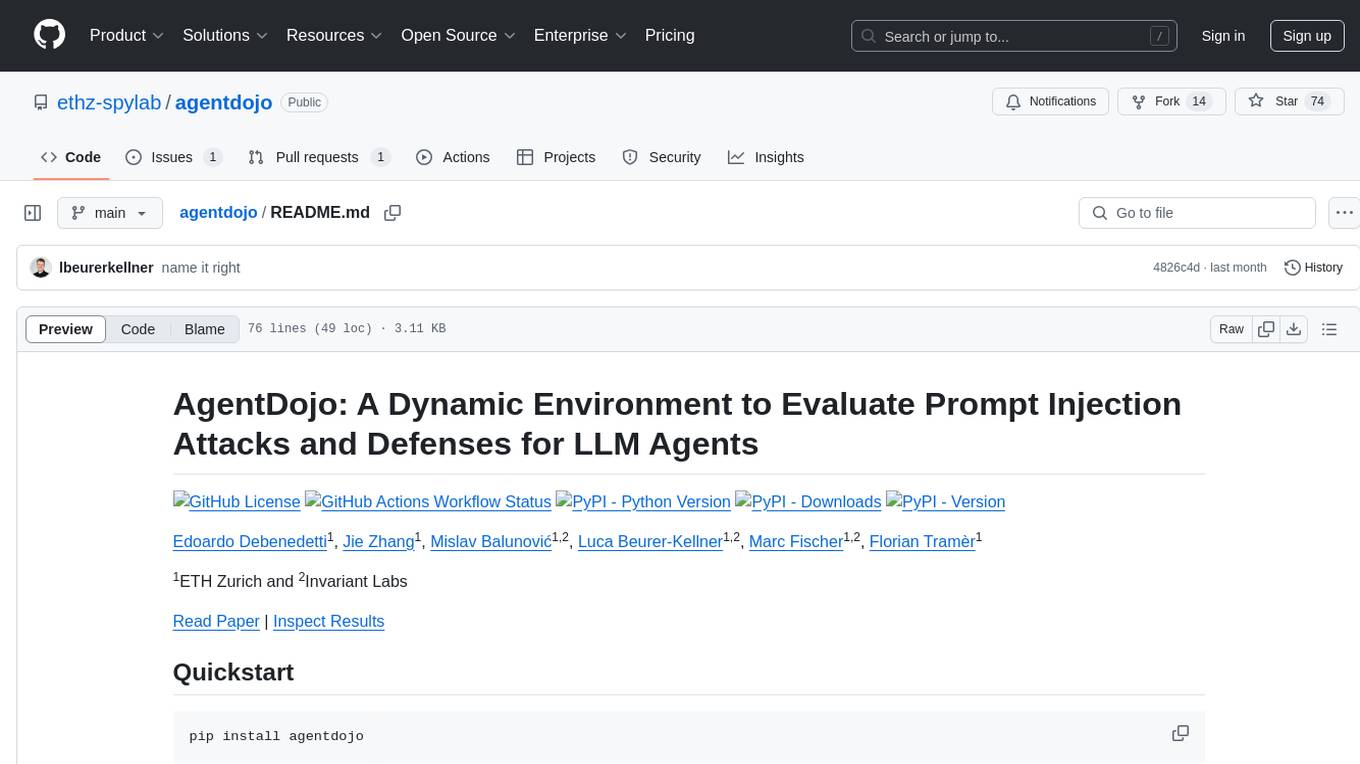
agentdojo
AgentDojo is a dynamic environment designed to evaluate prompt injection attacks and defenses for large language models (LLM) agents. It provides a benchmark script to run different suites and tasks with specified LLM models, defenses, and attacks. The tool is under active development, and users can inspect the results through dedicated documentation pages and the Invariant Benchmark Registry.
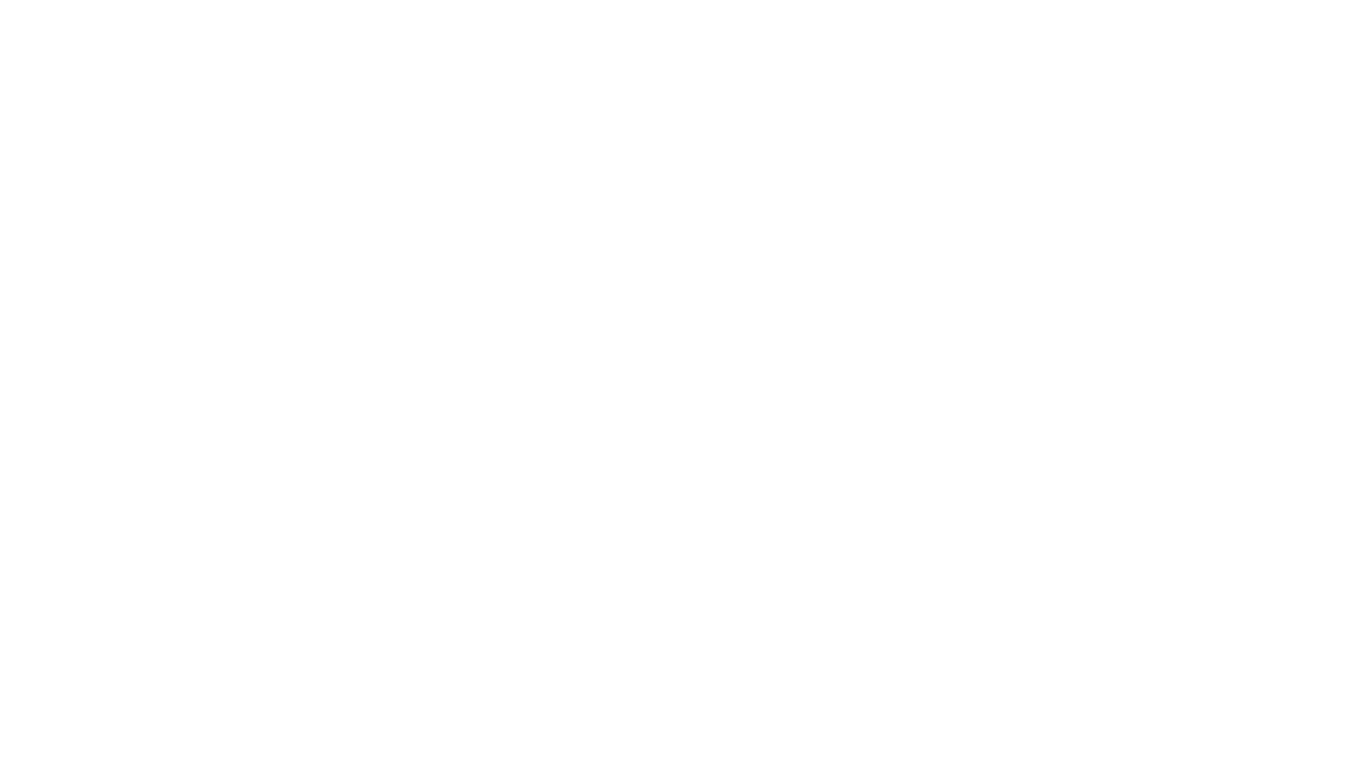
PanelCleaner
Panel Cleaner is a tool that uses machine learning to find text in images and generate masks to cover it up with high accuracy. It is designed to clean text bubbles without leaving artifacts, avoiding painting over non-text parts, and inpainting bubbles that can't be masked out. The tool offers various customization options, detailed analytics on the cleaning process, supports batch processing, and can run OCR on pages. It supports CUDA acceleration, multiple themes, and can handle bubbles on any solid grayscale background color. Panel Cleaner is aimed at saving time for cleaners by automating monotonous work and providing precise cleaning of text bubbles.
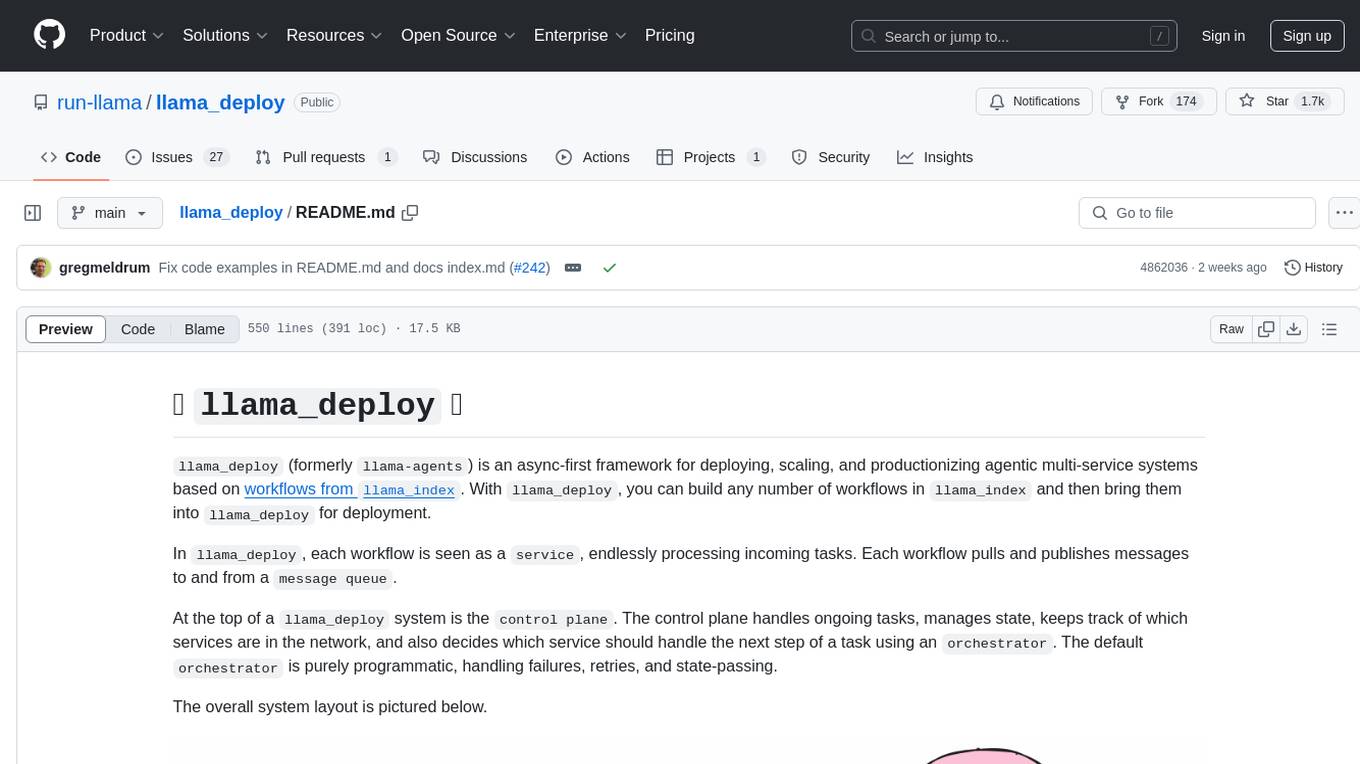
llama_deploy
llama_deploy is an async-first framework for deploying, scaling, and productionizing agentic multi-service systems based on workflows from llama_index. It allows building workflows in llama_index and deploying them seamlessly with minimal changes to code. The system includes services endlessly processing tasks, a control plane managing state and services, an orchestrator deciding task handling, and fault tolerance mechanisms. It is designed for high-concurrency scenarios, enabling real-time and high-throughput applications.
For similar tasks
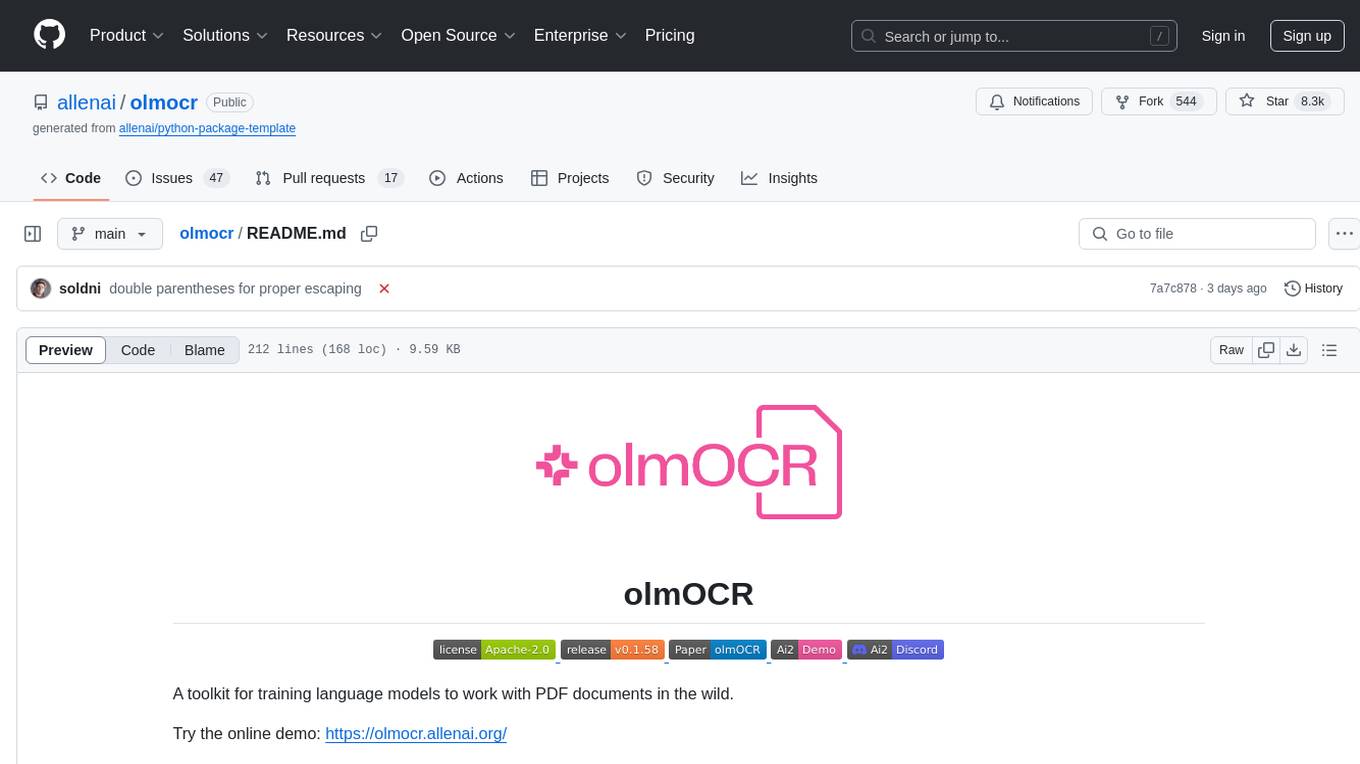
olmocr
olmOCR is a toolkit designed for training language models to work with PDF documents in real-world scenarios. It includes various components such as a prompting strategy for natural text parsing, an evaluation toolkit for comparing pipeline versions, filtering by language and SEO spam removal, finetuning code for specific models, processing PDFs through a finetuned model, and viewing documents created from PDFs. The toolkit requires a recent NVIDIA GPU with at least 20 GB of RAM and 30GB of free disk space. Users can install dependencies, set up a conda environment, and utilize olmOCR for tasks like converting single or multiple PDFs, viewing extracted text, and running batch inference pipelines.
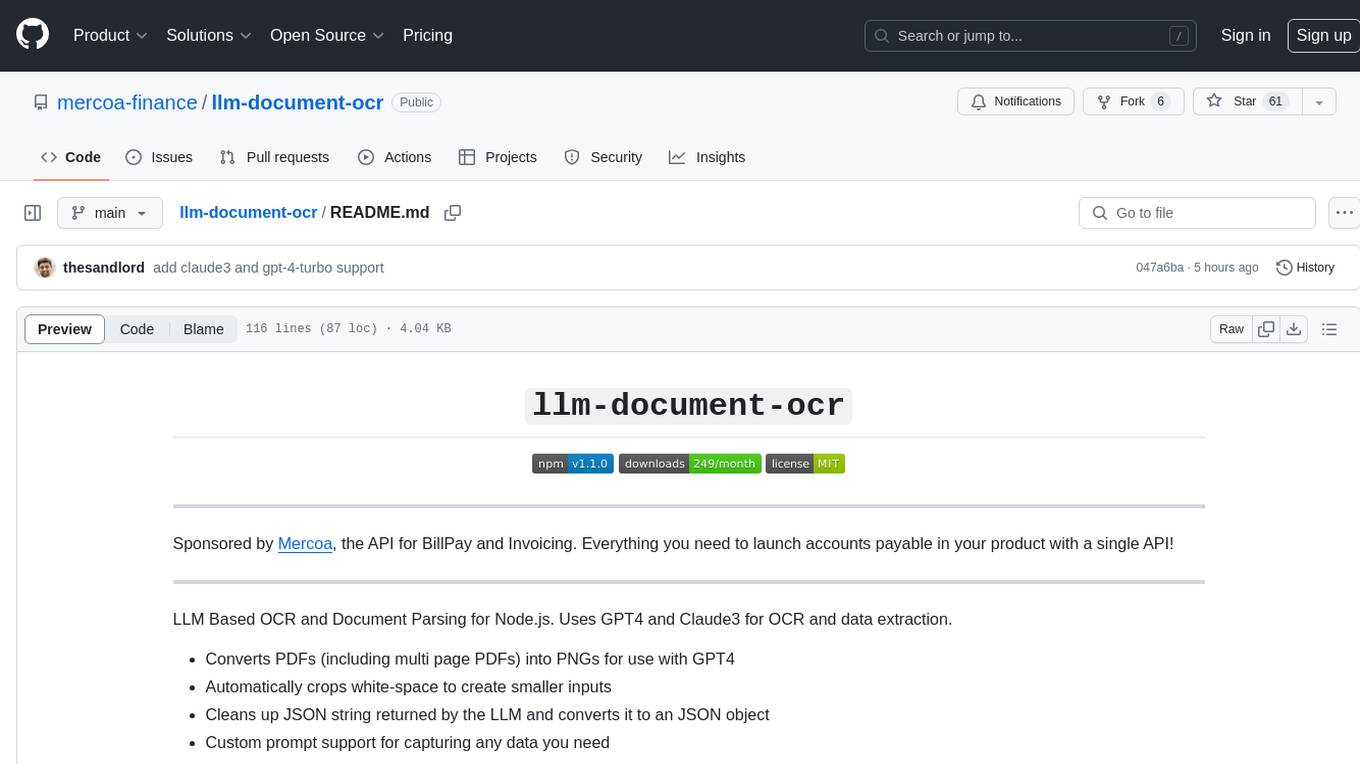
llm-document-ocr
LLM Document OCR is a Node.js tool that utilizes GPT4 and Claude3 for OCR and data extraction. It converts PDFs into PNGs, crops white-space, cleans up JSON strings, and supports various image formats. Users can customize prompts for data extraction. The tool is sponsored by Mercoa, offering API for BillPay and Invoicing.
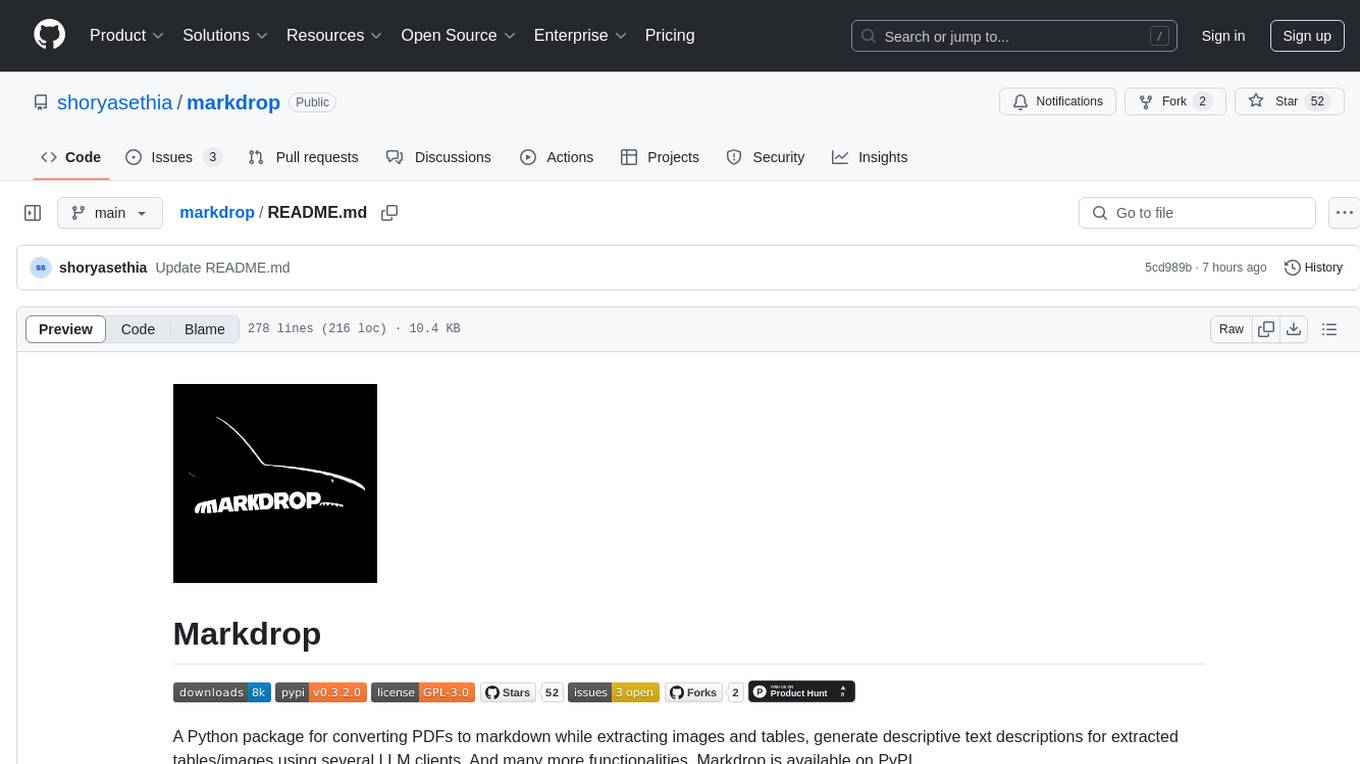
markdrop
Markdrop is a Python package that facilitates the conversion of PDFs to markdown format while extracting images and tables. It also generates descriptive text descriptions for extracted tables and images using various LLM clients. The tool offers additional functionalities such as PDF URL support, AI-powered image and table descriptions, interactive HTML output with downloadable Excel tables, customizable image resolution and UI elements, and a comprehensive logging system. Markdrop aims to simplify the process of handling PDF documents and enhancing their content with AI-generated descriptions.
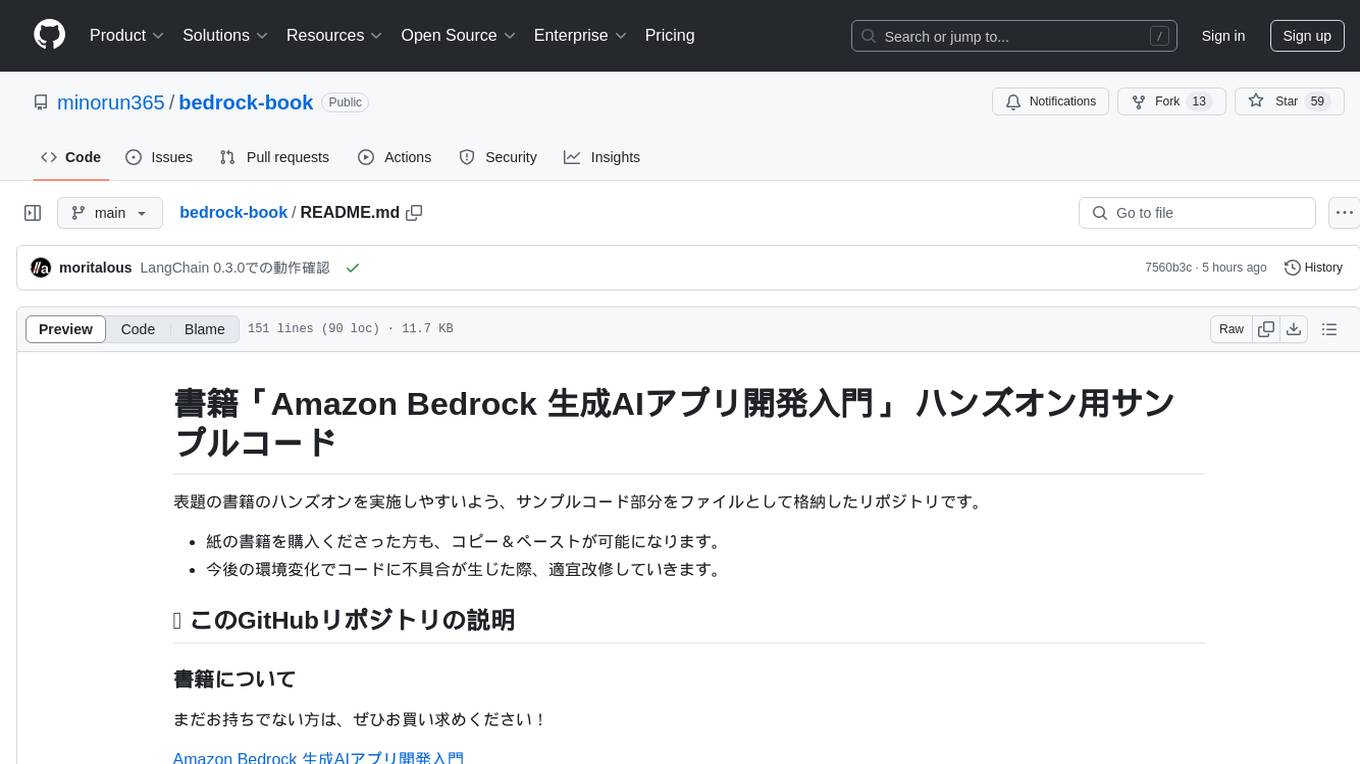
bedrock-book
This repository contains sample code for hands-on exercises related to the book 'Amazon Bedrock 生成AIアプリ開発入門'. It allows readers to easily access and copy the code. The repository also includes directories for each chapter's hands-on code, settings, and a 'requirements.txt' file listing necessary Python libraries. Updates and error fixes will be provided as needed. Users can report issues in the repository's 'Issues' section, and errata will be published on the SB Creative official website.
For similar jobs
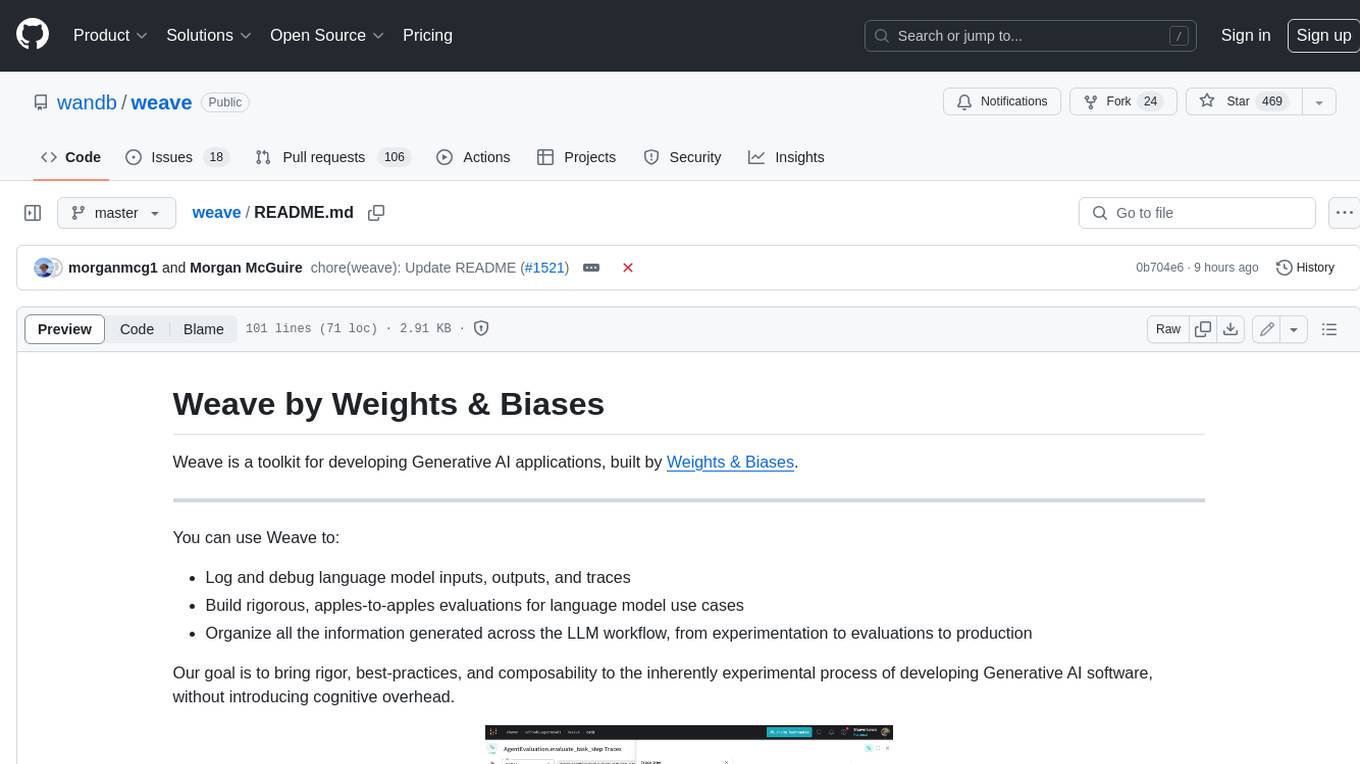
weave
Weave is a toolkit for developing Generative AI applications, built by Weights & Biases. With Weave, you can log and debug language model inputs, outputs, and traces; build rigorous, apples-to-apples evaluations for language model use cases; and organize all the information generated across the LLM workflow, from experimentation to evaluations to production. Weave aims to bring rigor, best-practices, and composability to the inherently experimental process of developing Generative AI software, without introducing cognitive overhead.
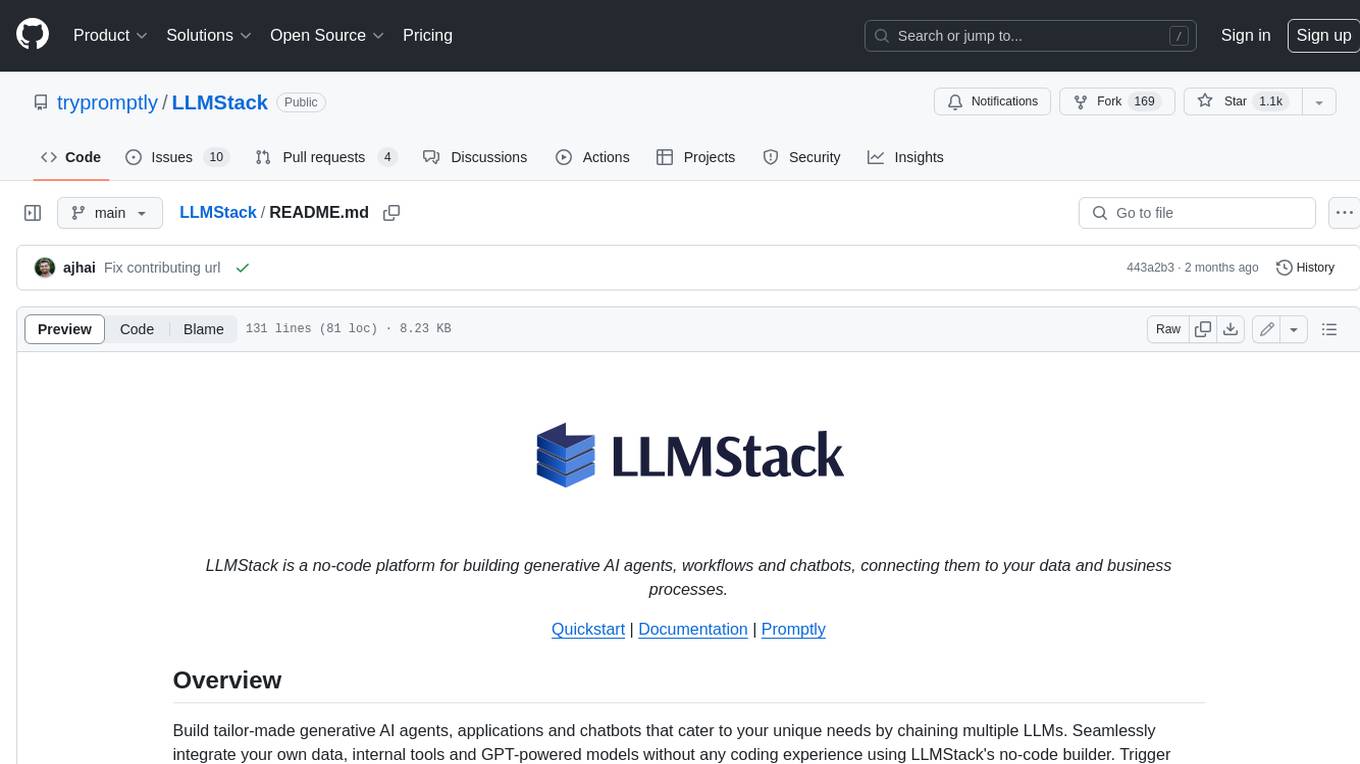
LLMStack
LLMStack is a no-code platform for building generative AI agents, workflows, and chatbots. It allows users to connect their own data, internal tools, and GPT-powered models without any coding experience. LLMStack can be deployed to the cloud or on-premise and can be accessed via HTTP API or triggered from Slack or Discord.
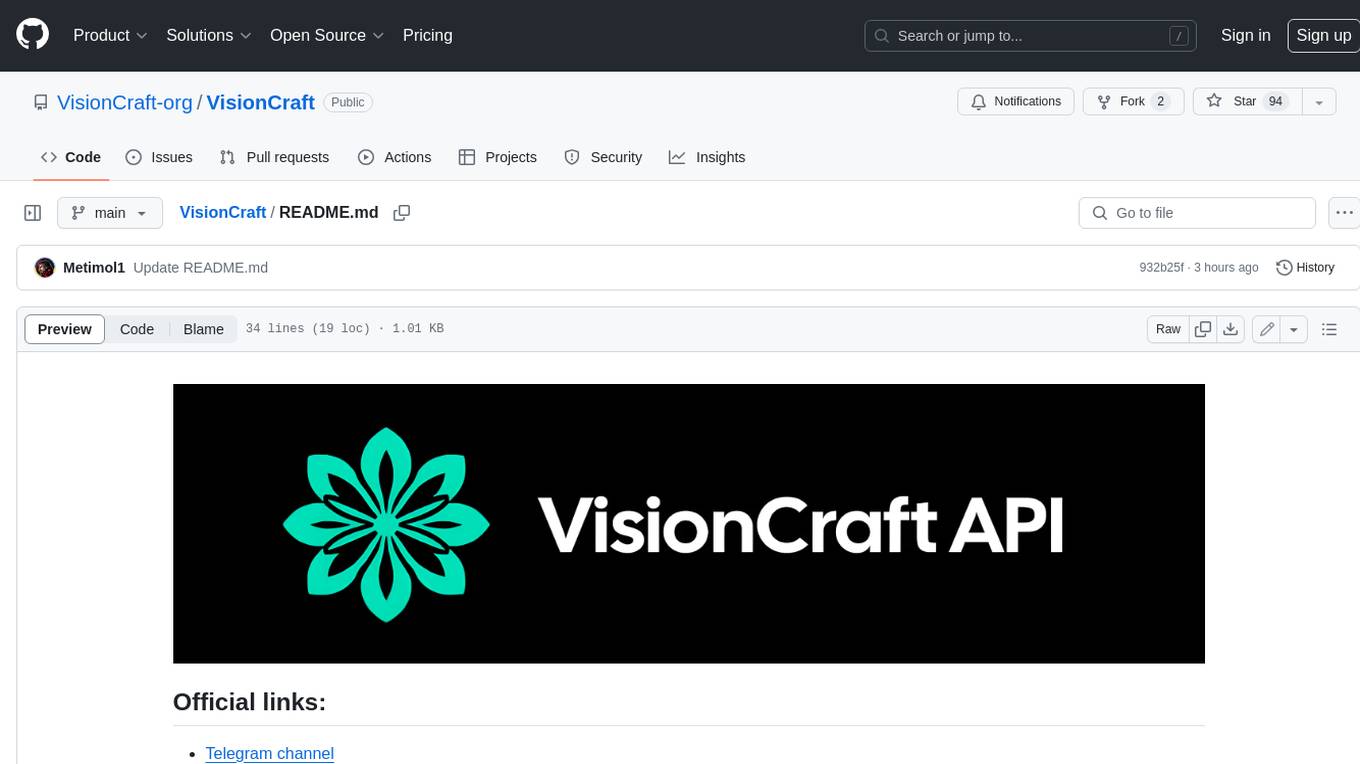
VisionCraft
The VisionCraft API is a free API for using over 100 different AI models. From images to sound.
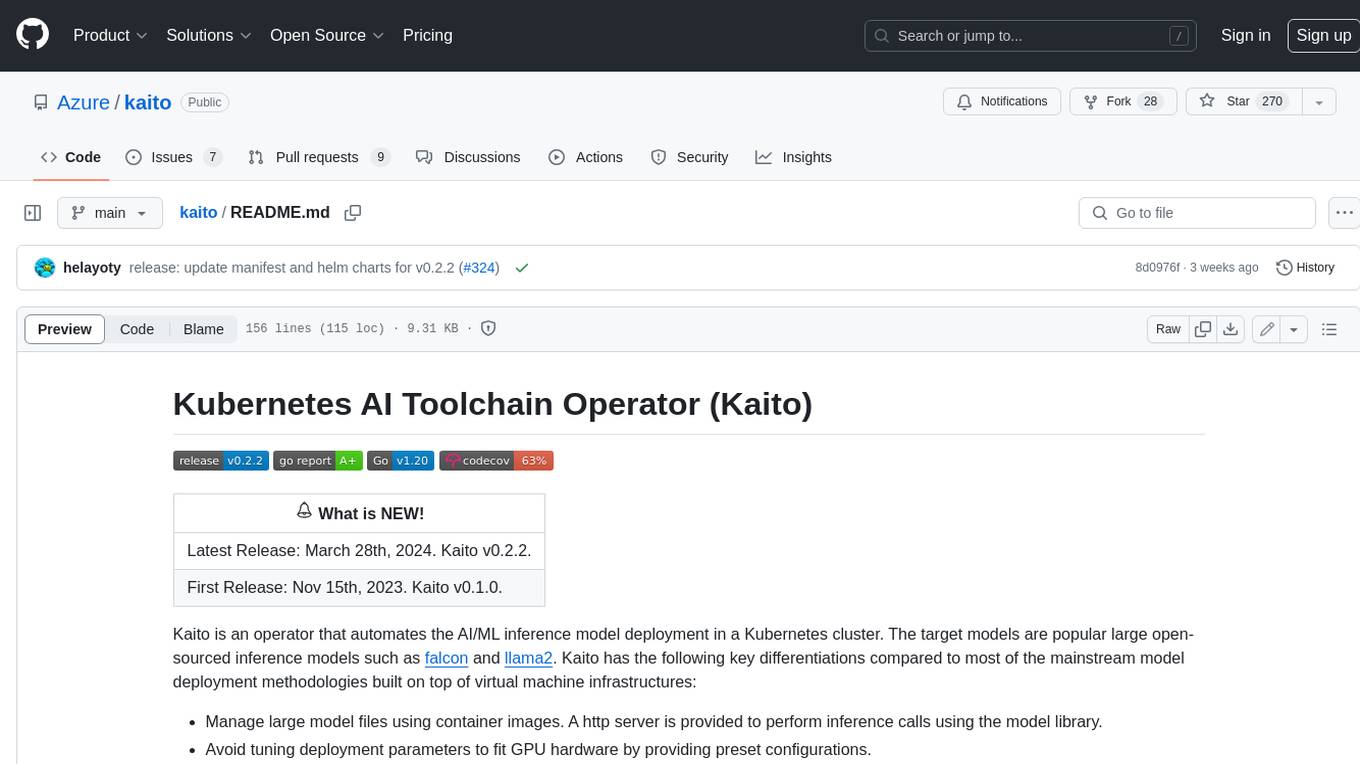
kaito
Kaito is an operator that automates the AI/ML inference model deployment in a Kubernetes cluster. It manages large model files using container images, avoids tuning deployment parameters to fit GPU hardware by providing preset configurations, auto-provisions GPU nodes based on model requirements, and hosts large model images in the public Microsoft Container Registry (MCR) if the license allows. Using Kaito, the workflow of onboarding large AI inference models in Kubernetes is largely simplified.
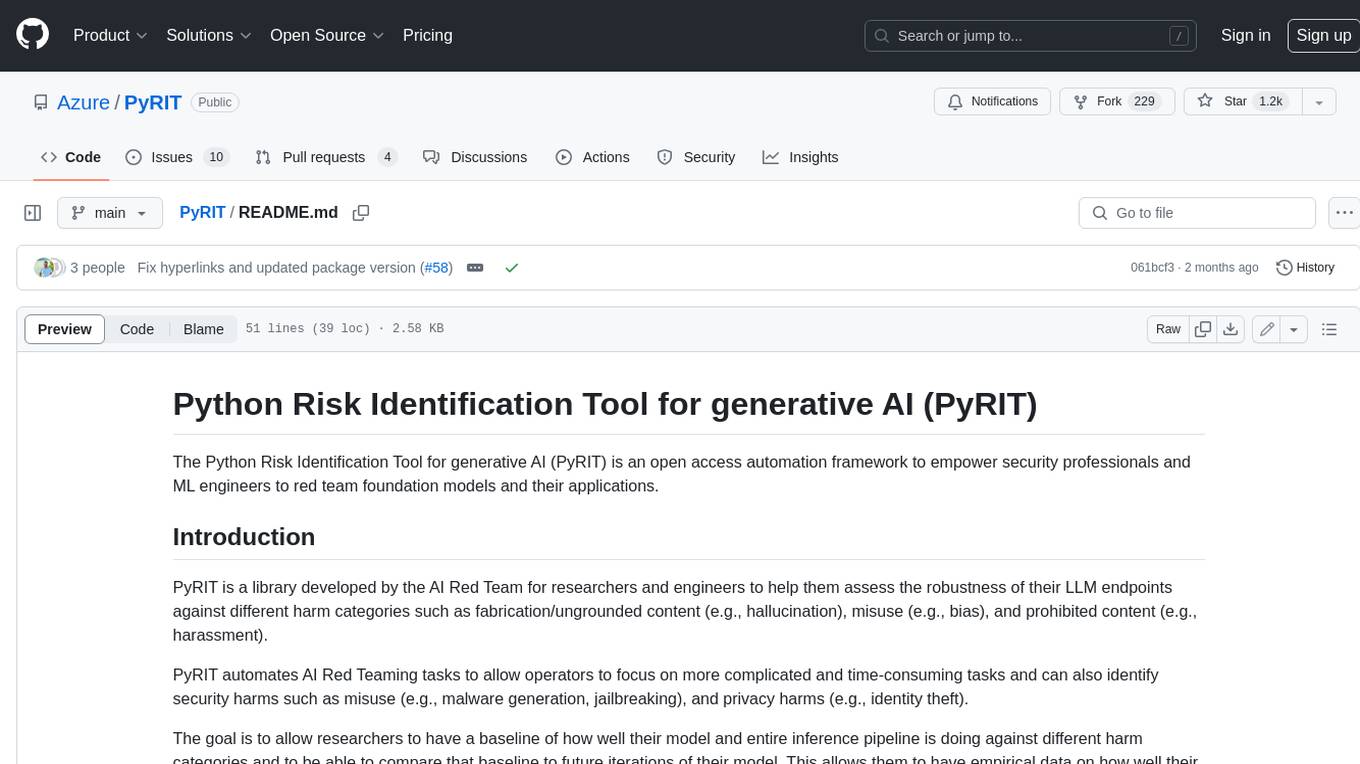
PyRIT
PyRIT is an open access automation framework designed to empower security professionals and ML engineers to red team foundation models and their applications. It automates AI Red Teaming tasks to allow operators to focus on more complicated and time-consuming tasks and can also identify security harms such as misuse (e.g., malware generation, jailbreaking), and privacy harms (e.g., identity theft). The goal is to allow researchers to have a baseline of how well their model and entire inference pipeline is doing against different harm categories and to be able to compare that baseline to future iterations of their model. This allows them to have empirical data on how well their model is doing today, and detect any degradation of performance based on future improvements.
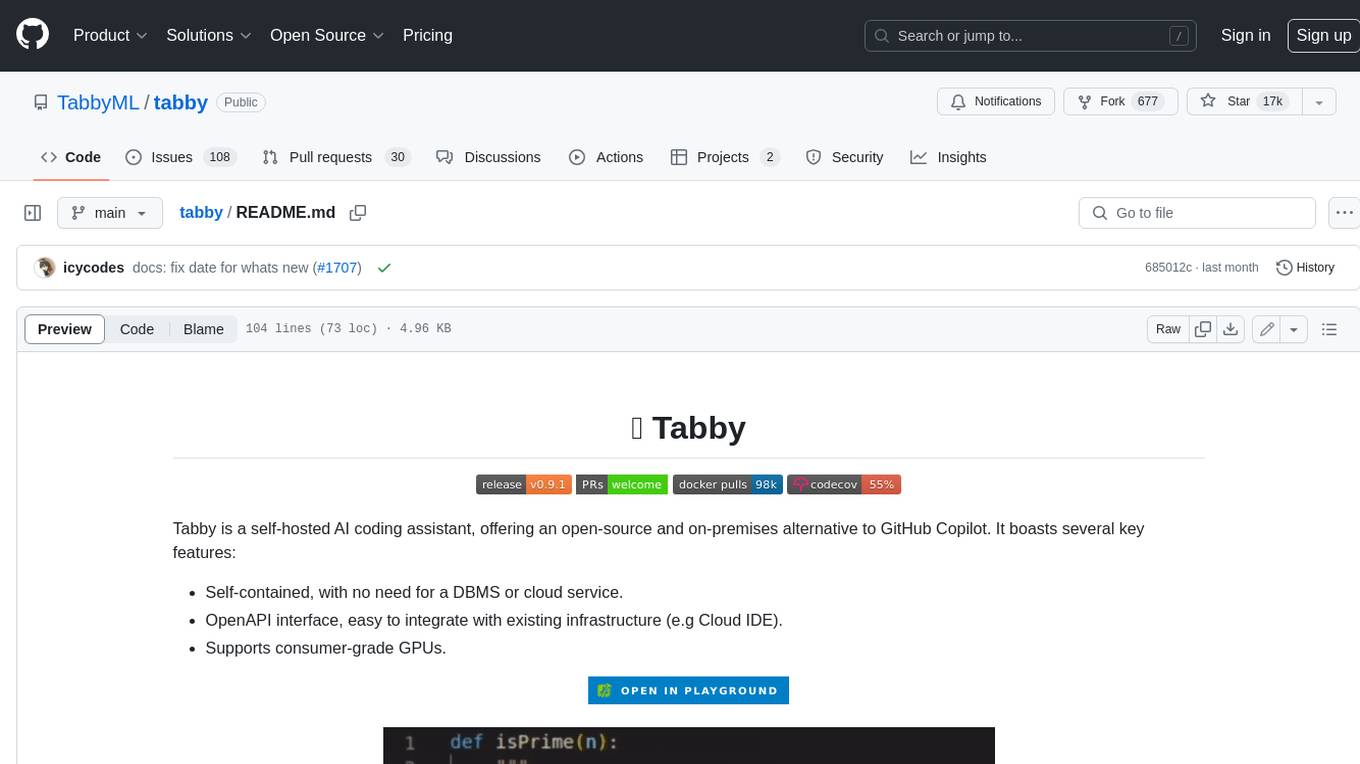
tabby
Tabby is a self-hosted AI coding assistant, offering an open-source and on-premises alternative to GitHub Copilot. It boasts several key features: * Self-contained, with no need for a DBMS or cloud service. * OpenAPI interface, easy to integrate with existing infrastructure (e.g Cloud IDE). * Supports consumer-grade GPUs.
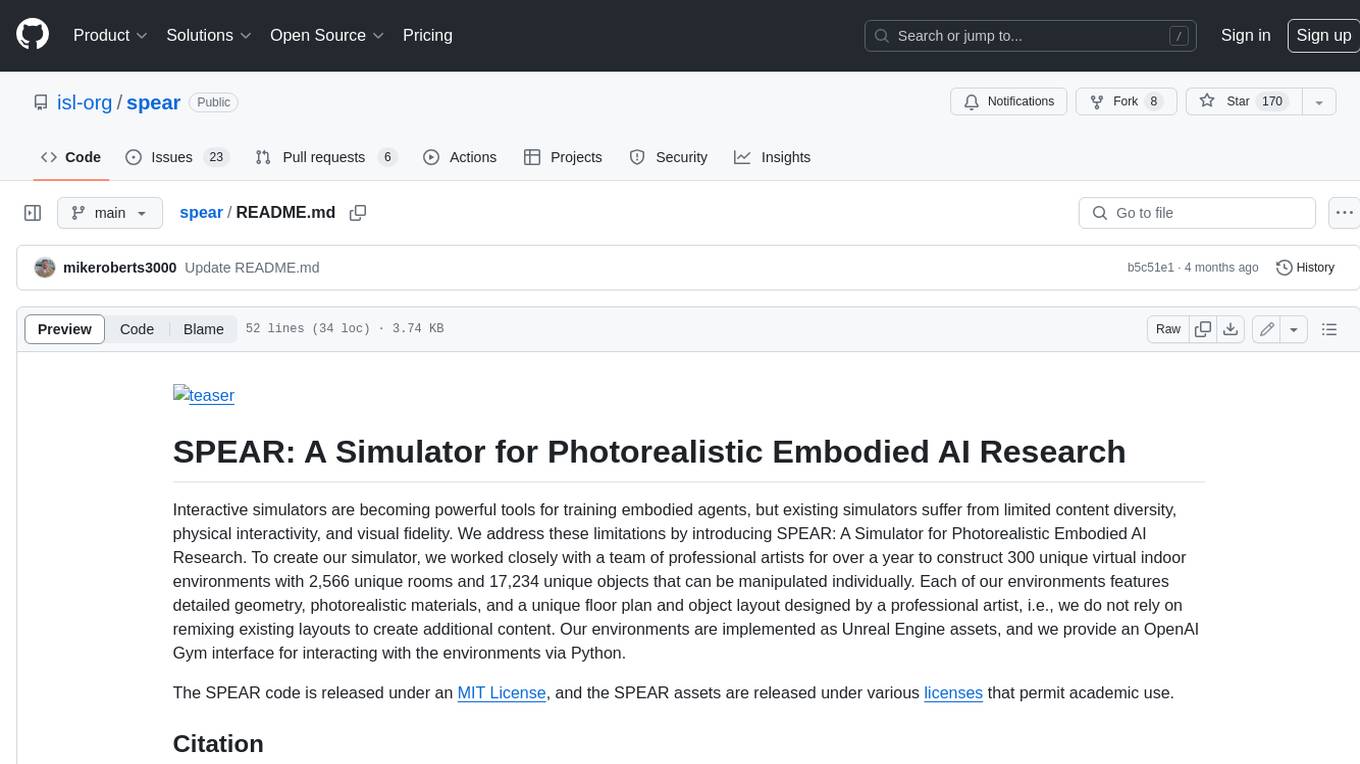
spear
SPEAR (Simulator for Photorealistic Embodied AI Research) is a powerful tool for training embodied agents. It features 300 unique virtual indoor environments with 2,566 unique rooms and 17,234 unique objects that can be manipulated individually. Each environment is designed by a professional artist and features detailed geometry, photorealistic materials, and a unique floor plan and object layout. SPEAR is implemented as Unreal Engine assets and provides an OpenAI Gym interface for interacting with the environments via Python.
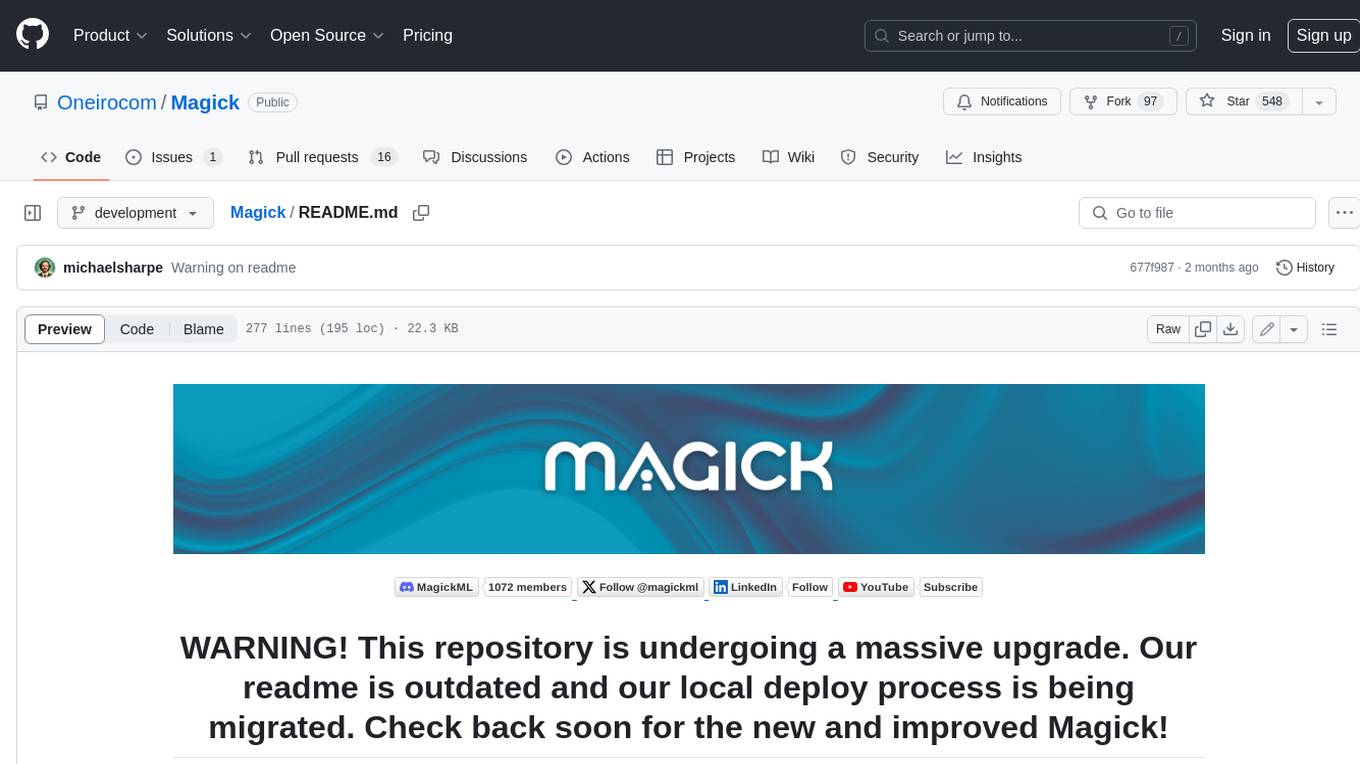
Magick
Magick is a groundbreaking visual AIDE (Artificial Intelligence Development Environment) for no-code data pipelines and multimodal agents. Magick can connect to other services and comes with nodes and templates well-suited for intelligent agents, chatbots, complex reasoning systems and realistic characters.