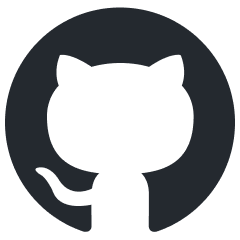
ai21-python
AI21 Python SDK
Stars: 55
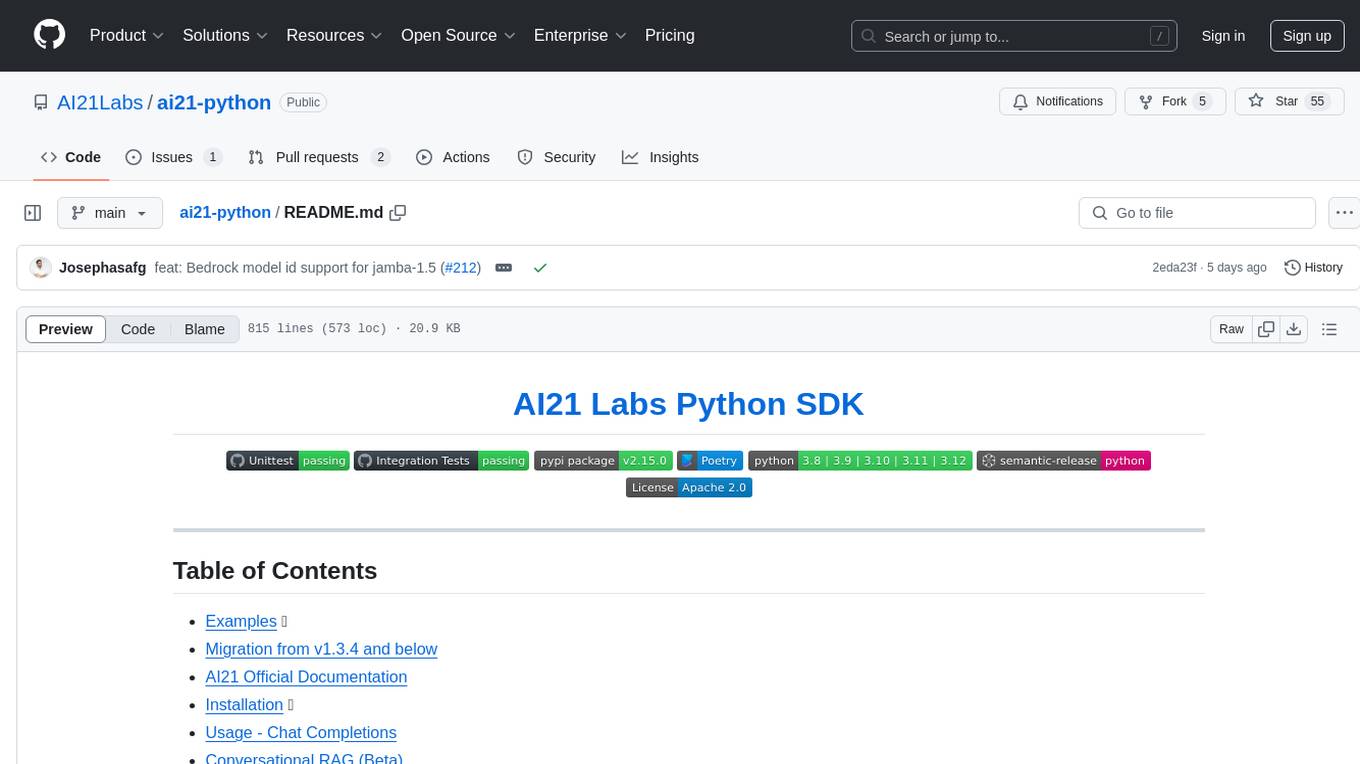
The AI21 Labs Python SDK is a comprehensive tool for interacting with the AI21 API. It provides functionalities for chat completions, conversational RAG, token counting, error handling, and support for various cloud providers like AWS, Azure, and Vertex. The SDK offers both synchronous and asynchronous usage, along with detailed examples and documentation. Users can quickly get started with the SDK to leverage AI21's powerful models for various natural language processing tasks.
README:
- Examples 🗂️
- Migration from v1.3.4 and below
- AI21 Official Documentation
- Installation 💿
- Usage - Chat Completions
- Conversational RAG (Beta)
- Older Models Support Usage
- More Models
- Token Counting
- Environment Variables
- Error Handling
- Cloud Providers ☁️
If you want to quickly get a glance how to use the AI21 Python SDK and jump straight to business, you can check out the examples. Take a look at our models and see them in action! Several examples and demonstrations have been put together to show our models' functionality and capabilities.
Feel free to dive in, experiment, and adapt these examples to suit your needs. We believe they'll help you get up and running quickly.
In v2.0.0
we introduced a new SDK that is not backwards compatible with the previous version.
This version allows for non-static instances of the client, defined parameters to each resource, modelized responses and
more.
Migration Examples
from ai21 import AI21Client
client = AI21Client(api_key='my_api_key')
# or set api_key in environment variable - AI21_API_KEY and then
client = AI21Client()
We No longer support static methods for each resource, instead we have a client instance that has a method for each allowing for more flexibility and better control.
prompt = "some prompt"
- import ai21
- response = ai21.Completion.execute(model="j2-light", prompt=prompt, maxTokens=2)
+ from ai21 import AI21Client
+ client = ai21.AI21Client()
+ response = client.completion.create(model="j2-light", prompt=prompt, max_tokens=2)
This applies to all resources. You would now need to create a client instance and use it to call the resource method.
- response = ai21.Tokenization.execute(text=prompt)
- print(len(response)) # number of tokens
+ from ai21 import AI21Client
+ client = AI21Client()
+ token_count = client.count_tokens(text=prompt)
It is no longer possible to access the response object as a dictionary. Instead, you can access the response object as an object with attributes.
- import ai21
- response = ai21.Summarize.execute(source="some text", sourceType="TEXT")
- response["summary"]
+ from ai21 import AI21Client
+ from ai21.models import DocumentType
+ client = AI21Client()
+ response = client.summarize.create(source="some text", source_type=DocumentType.TEXT)
+ response.summary
- import ai21
- destination = ai21.BedrockDestination(model_id=ai21.BedrockModelID.J2_MID_V1)
- response = ai21.Completion.execute(prompt=prompt, maxTokens=1000, destination=destination)
+ from ai21 import AI21BedrockClient, BedrockModelID
+ client = AI21BedrockClient()
+ response = client.completion.create(prompt=prompt, max_tokens=1000, model_id=BedrockModelID.J2_MID_V1)
- import ai21
- destination = ai21.SageMakerDestination("j2-mid-test-endpoint")
- response = ai21.Completion.execute(prompt=prompt, maxTokens=1000, destination=destination)
+ from ai21 import AI21SageMakerClient
+ client = AI21SageMakerClient(endpoint_name="j2-mid-test-endpoint")
+ response = client.completion.create(prompt=prompt, max_tokens=1000)
The full documentation for the REST API can be found on docs.ai21.com.
pip install ai21
from ai21 import AI21Client
from ai21.models.chat import ChatMessage
client = AI21Client(
# defaults to os.enviorn.get('AI21_API_KEY')
api_key='my_api_key',
)
system = "You're a support engineer in a SaaS company"
messages = [
ChatMessage(content=system, role="system"),
ChatMessage(content="Hello, I need help with a signup process.", role="user"),
]
chat_completions = client.chat.completions.create(
messages=messages,
model="jamba-1.5-mini",
)
You can use the AsyncAI21Client
to make asynchronous requests.
There is no difference between the sync and the async client in terms of usage.
import asyncio
from ai21 import AsyncAI21Client
from ai21.models.chat import ChatMessage
system = "You're a support engineer in a SaaS company"
messages = [
ChatMessage(content=system, role="system"),
ChatMessage(content="Hello, I need help with a signup process.", role="user"),
]
client = AsyncAI21Client(
# defaults to os.enviorn.get('AI21_API_KEY')
api_key='my_api_key',
)
async def main():
response = await client.chat.completions.create(
messages=messages,
model="jamba-1.5-mini",
)
print(response)
asyncio.run(main())
A more detailed example can be found here.
Examples
- j2-light
- j2-ultra
- j2-mid
- jamba-instruct
you can read more about the models here.
from ai21 import AI21Client
from ai21.models import RoleType
from ai21.models import ChatMessage
system = "You're a support engineer in a SaaS company"
messages = [
ChatMessage(text="Hello, I need help with a signup process.", role=RoleType.USER),
ChatMessage(text="Hi Alice, I can help you with that. What seems to be the problem?", role=RoleType.ASSISTANT),
ChatMessage(text="I am having trouble signing up for your product with my Google account.", role=RoleType.USER),
]
client = AI21Client()
chat_response = client.chat.create(
system=system,
messages=messages,
model="j2-ultra",
)
For a more detailed example, see the chat examples.
from ai21 import AI21Client
client = AI21Client()
completion_response = client.completion.create(
prompt="This is a test prompt",
model="j2-mid",
)
from ai21 import AI21Client
from ai21.models.chat import ChatMessage
system = "You're a support engineer in a SaaS company"
messages = [
ChatMessage(content=system, role="system"),
ChatMessage(content="Hello, I need help with a signup process.", role="user"),
ChatMessage(content="Hi Alice, I can help you with that. What seems to be the problem?", role="assistant"),
ChatMessage(content="I am having trouble signing up for your product with my Google account.", role="user"),
]
client = AI21Client()
response = client.chat.completions.create(
messages=messages,
model="jamba-instruct",
max_tokens=100,
temperature=0.7,
top_p=1.0,
stop=["\n"],
)
print(response)
Note that jamba-instruct supports async and streaming as well.
For a more detailed example, see the completion examples.
We currently support streaming for the Chat Completions API in Jamba.
from ai21 import AI21Client
from ai21.models.chat import ChatMessage
messages = [ChatMessage(content="What is the meaning of life?", role="user")]
client = AI21Client()
response = client.chat.completions.create(
messages=messages,
model="jamba-instruct",
stream=True,
)
for chunk in response:
print(chunk.choices[0].delta.content, end="")
import asyncio
from ai21 import AsyncAI21Client
from ai21.models.chat import ChatMessage
messages = [ChatMessage(content="What is the meaning of life?", role="user")]
client = AsyncAI21Client()
async def main():
response = await client.chat.completions.create(
messages=messages,
model="jamba-1.5-mini",
stream=True,
)
async for chunk in response:
print(chunk.choices[0].delta.content, end="")
asyncio.run(main())
Like chat, but with the ability to retrieve information from your Studio library.
from ai21 import AI21Client
from ai21.models.chat import ChatMessage
messages = [
ChatMessage(content="Ask a question about your files", role="user"),
]
client = AI21Client()
client.library.files.create(
file_path="path/to/file",
path="path/to/file/in/library",
labels=["my_file_label"],
)
chat_response = client.beta.conversational_rag.create(
messages=messages,
labels=["my_file_label"],
)
For a more detailed example, see the chat sync and async examples.
from ai21 import AI21Client
client = AI21Client()
file_id = client.library.files.create(
file_path="path/to/file",
path="path/to/file/in/library",
labels=["label1", "label2"],
public_url="www.example.com",
)
uploaded_file = client.library.files.get(file_id)
By using the count_tokens
method, you can estimate the billing for a given request.
from ai21.tokenizers import get_tokenizer
tokenizer = get_tokenizer(name="jamba-tokenizer")
total_tokens = tokenizer.count_tokens(text="some text") # returns int
print(total_tokens)
from ai21.tokenizers import get_async_tokenizer
## Your async function code
#...
tokenizer = await get_async_tokenizer(name="jamba-tokenizer")
total_tokens = await tokenizer.count_tokens(text="some text") # returns int
print(total_tokens)
Available tokenizers are:
jamba-tokenizer
j2-tokenizer
For more information on AI21 Tokenizers, see the documentation.
You can set several environment variables to configure the client.
We use the standard library logging
module.
To enable logging, set the AI21_LOG_LEVEL
environment variable.
$ export AI21_LOG_LEVEL=debug
-
AI21_API_KEY
- Your API key. If not set, you must pass it to the client constructor. -
AI21_API_VERSION
- The API version. Defaults tov1
. -
AI21_API_HOST
- The API host. Defaults tohttps://api.ai21.com/v1/
. -
AI21_TIMEOUT_SEC
- The timeout for API requests. -
AI21_NUM_RETRIES
- The maximum number of retries for API requests. Defaults to3
retries. -
AI21_AWS_REGION
- The AWS region to use for AWS clients. Defaults tous-east-1
.
from ai21 import errors as ai21_errors
from ai21 import AI21Client, AI21APIError
from ai21.models import ChatMessage
client = AI21Client()
system = "You're a support engineer in a SaaS company"
messages = [
# Notice the given role does not exist and will be the reason for the raised error
ChatMessage(text="Hello, I need help with a signup process.", role="Non-Existent-Role"),
]
try:
chat_completion = client.chat.create(
messages=messages,
model="j2-ultra",
system=system
)
except ai21_errors.AI21ServerError as e:
print("Server error and could not be reached")
print(e.details)
except ai21_errors.TooManyRequestsError as e:
print("A 429 status code was returned. Slow down on the requests")
except AI21APIError as e:
print("A non 200 status code error. For more error types see ai21.errors")
AI21 Library provides convenient ways to interact with two AWS clients for use with AWS Bedrock and AWS SageMaker.
pip install -U "ai21[AWS]"
This will make sure you have the required dependencies installed, including boto3 >= 1.28.82
.
from ai21 import AI21BedrockClient, BedrockModelID
from ai21.models.chat import ChatMessage
client = AI21BedrockClient(region='us-east-1') # region is optional, as you can use the env variable instead
messages = [
ChatMessage(content="You are a helpful assistant", role="system"),
ChatMessage(content="What is the meaning of life?", role="user")
]
response = client.chat.completions.create(
messages=messages,
model_id=BedrockModelID.JAMBA_1_5_LARGE,
)
from ai21 import AI21BedrockClient, BedrockModelID
from ai21.models.chat import ChatMessage
system = "You're a support engineer in a SaaS company"
messages = [
ChatMessage(content=system, role="system"),
ChatMessage(content="Hello, I need help with a signup process.", role="user"),
ChatMessage(content="Hi Alice, I can help you with that. What seems to be the problem?", role="assistant"),
ChatMessage(content="I am having trouble signing up for your product with my Google account.", role="user"),
]
client = AI21BedrockClient()
response = client.chat.completions.create(
messages=messages,
model=BedrockModelID.JAMBA_1_5_LARGE,
stream=True,
)
for chunk in response:
print(chunk.choices[0].message.content, end="")
import asyncio
from ai21 import AsyncAI21BedrockClient, BedrockModelID
from ai21.models.chat import ChatMessage
client = AsyncAI21BedrockClient(region='us-east-1') # region is optional, as you can use the env variable instead
messages = [
ChatMessage(content="You are a helpful assistant", role="system"),
ChatMessage(content="What is the meaning of life?", role="user")
]
async def main():
response = await client.chat.completions.create(
messages=messages,
model_id=BedrockModelID.JAMBA_1_5_LARGE,
)
asyncio.run(main())
import boto3
from ai21 import AI21BedrockClient, BedrockModelID
from ai21.models.chat import ChatMessage
boto_session = boto3.Session(region_name="us-east-1")
client = AI21BedrockClient(session=boto_session)
messages = [
ChatMessage(content="You are a helpful assistant", role="system"),
ChatMessage(content="What is the meaning of life?", role="user")
]
response = client.chat.completions.create(
messages=messages,
model_id=BedrockModelID.JAMBA_1_5_LARGE,
)
import boto3
import asyncio
from ai21 import AsyncAI21BedrockClient, BedrockModelID
from ai21.models.chat import ChatMessage
boto_session = boto3.Session(region_name="us-east-1")
client = AsyncAI21BedrockClient(session=boto_session)
messages = [
ChatMessage(content="You are a helpful assistant", role="system"),
ChatMessage(content="What is the meaning of life?", role="user")
]
async def main():
response = await client.chat.completions.create(
messages=messages,
model_id=BedrockModelID.JAMBA_1_5_LARGE,
)
asyncio.run(main())
from ai21 import AI21SageMakerClient
client = AI21SageMakerClient(endpoint_name="j2-endpoint-name")
response = client.summarize.create(
source="Text to summarize",
source_type="TEXT",
)
print(response.summary)
import asyncio
from ai21 import AsyncAI21SageMakerClient
client = AsyncAI21SageMakerClient(endpoint_name="j2-endpoint-name")
async def main():
response = await client.summarize.create(
source="Text to summarize",
source_type="TEXT",
)
print(response.summary)
asyncio.run(main())
from ai21 import AI21SageMakerClient
import boto3
boto_session = boto3.Session(region_name="us-east-1")
client = AI21SageMakerClient(
session=boto_session,
endpoint_name="j2-endpoint-name",
)
If you wish to interact with your Azure endpoint on Azure AI Studio, use the AI21AzureClient
and AsyncAI21AzureClient
clients.
The following models are supported on Azure:
jamba-instruct
from ai21 import AI21AzureClient
from ai21.models.chat import ChatMessage
client = AI21AzureClient(
base_url="https://<YOUR-ENDPOINT>.inference.ai.azure.com",
api_key="<your Azure api key>",
)
messages = [
ChatMessage(content="You are a helpful assistant", role="system"),
ChatMessage(content="What is the meaning of life?", role="user")
]
response = client.chat.completions.create(
model="jamba-1.5-mini",
messages=messages,
)
import asyncio
from ai21 import AsyncAI21AzureClient
from ai21.models.chat import ChatMessage
client = AsyncAI21AzureClient(
base_url="https://<YOUR-ENDPOINT>.inference.ai.azure.com/v1/chat/completions",
api_key="<your Azure api key>",
)
messages = [
ChatMessage(content="You are a helpful assistant", role="system"),
ChatMessage(content="What is the meaning of life?", role="user")
]
async def main():
response = await client.chat.completions.create(
model="jamba-instruct",
messages=messages,
)
asyncio.run(main())
If you wish to interact with your Vertex AI endpoint on GCP, use the AI21VertexClient
and AsyncAI21VertexClient
clients.
The following models are supported on Vertex:
jamba-1.5-mini
jamba-1.5-large
from ai21 import AI21VertexClient
from ai21.models.chat import ChatMessage
# You can also set the project_id, region, access_token and Google credentials in the constructor
client = AI21VertexClient()
messages = ChatMessage(content="What is the meaning of life?", role="user")
response = client.chat.completions.create(
model="jamba-1.5-mini",
messages=[messages],
)
import asyncio
from ai21 import AsyncAI21VertexClient
from ai21.models.chat import ChatMessage
# You can also set the project_id, region, access_token and Google credentials in the constructor
client = AsyncAI21VertexClient()
async def main():
messages = ChatMessage(content="What is the meaning of life?", role="user")
response = await client.chat.completions.create(
model="jamba-1.5-mini",
messages=[messages],
)
asyncio.run(main())
Happy prompting! 🚀
For Tasks:
Click tags to check more tools for each tasksFor Jobs:
Alternative AI tools for ai21-python
Similar Open Source Tools
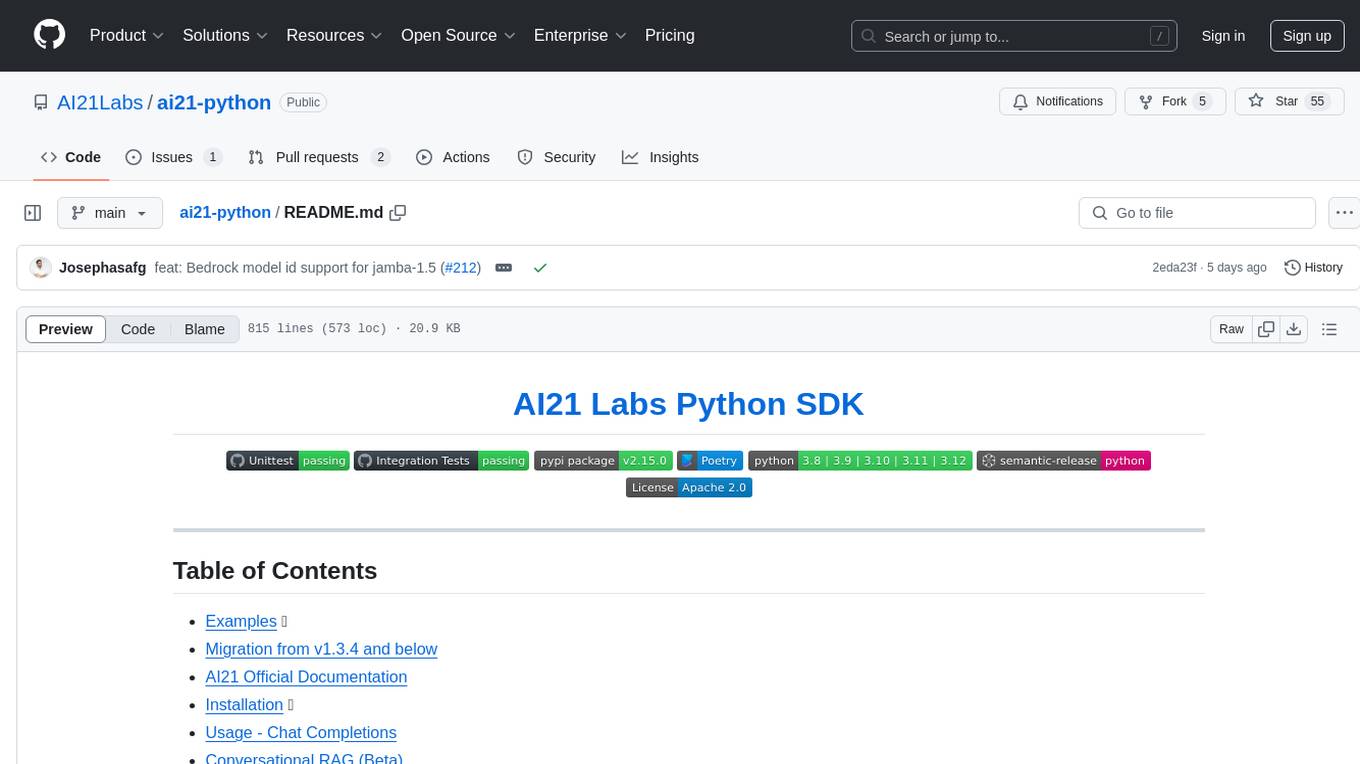
ai21-python
The AI21 Labs Python SDK is a comprehensive tool for interacting with the AI21 API. It provides functionalities for chat completions, conversational RAG, token counting, error handling, and support for various cloud providers like AWS, Azure, and Vertex. The SDK offers both synchronous and asynchronous usage, along with detailed examples and documentation. Users can quickly get started with the SDK to leverage AI21's powerful models for various natural language processing tasks.
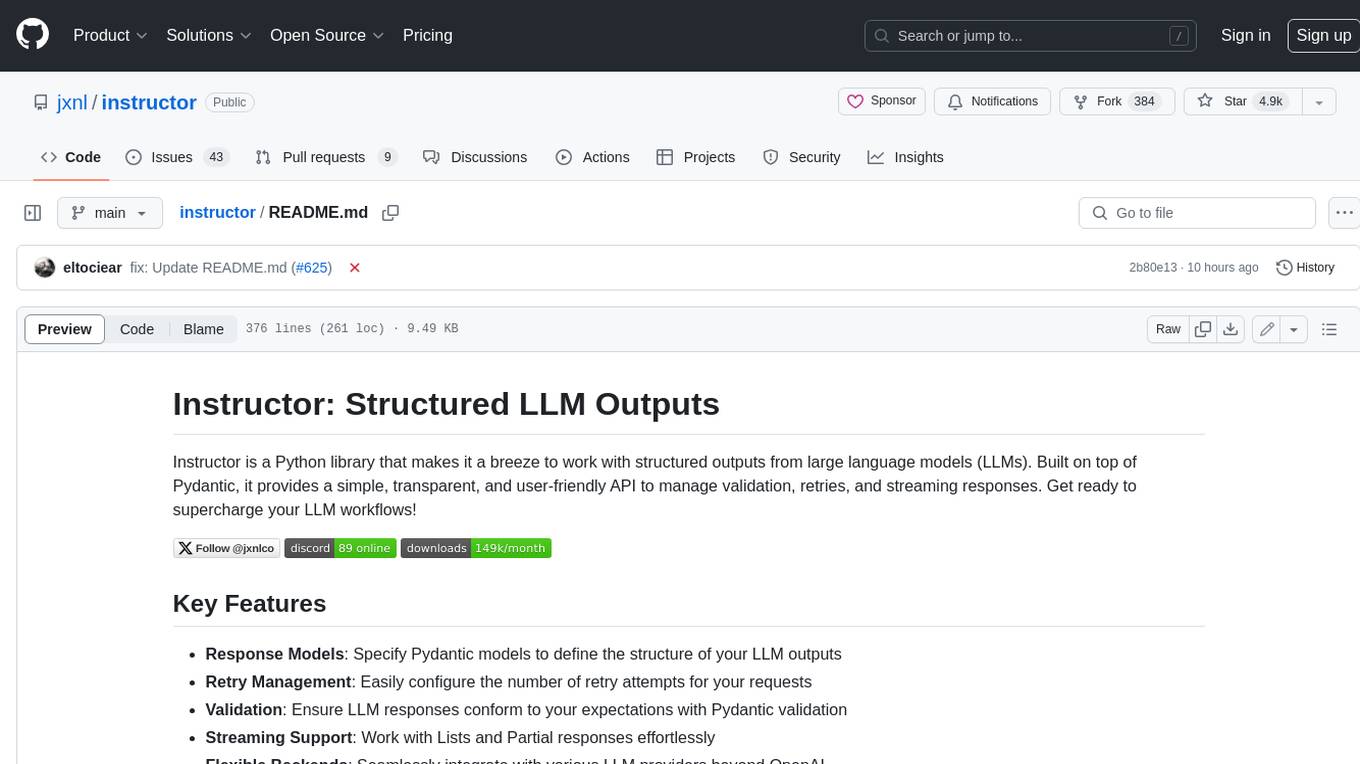
instructor
Instructor is a Python library that makes it a breeze to work with structured outputs from large language models (LLMs). Built on top of Pydantic, it provides a simple, transparent, and user-friendly API to manage validation, retries, and streaming responses. Get ready to supercharge your LLM workflows!
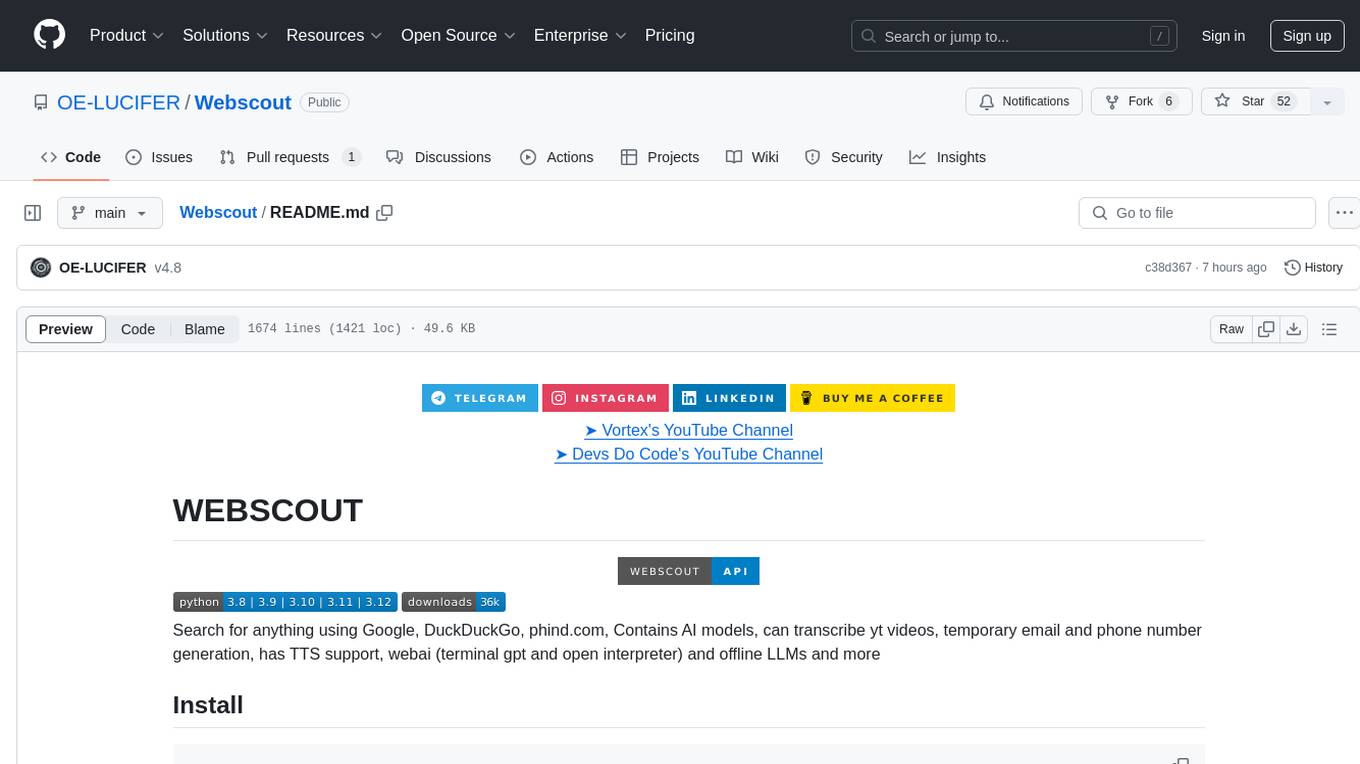
Webscout
WebScout is a versatile tool that allows users to search for anything using Google, DuckDuckGo, and phind.com. It contains AI models, can transcribe YouTube videos, generate temporary email and phone numbers, has TTS support, webai (terminal GPT and open interpreter), and offline LLMs. It also supports features like weather forecasting, YT video downloading, temp mail and number generation, text-to-speech, advanced web searches, and more.
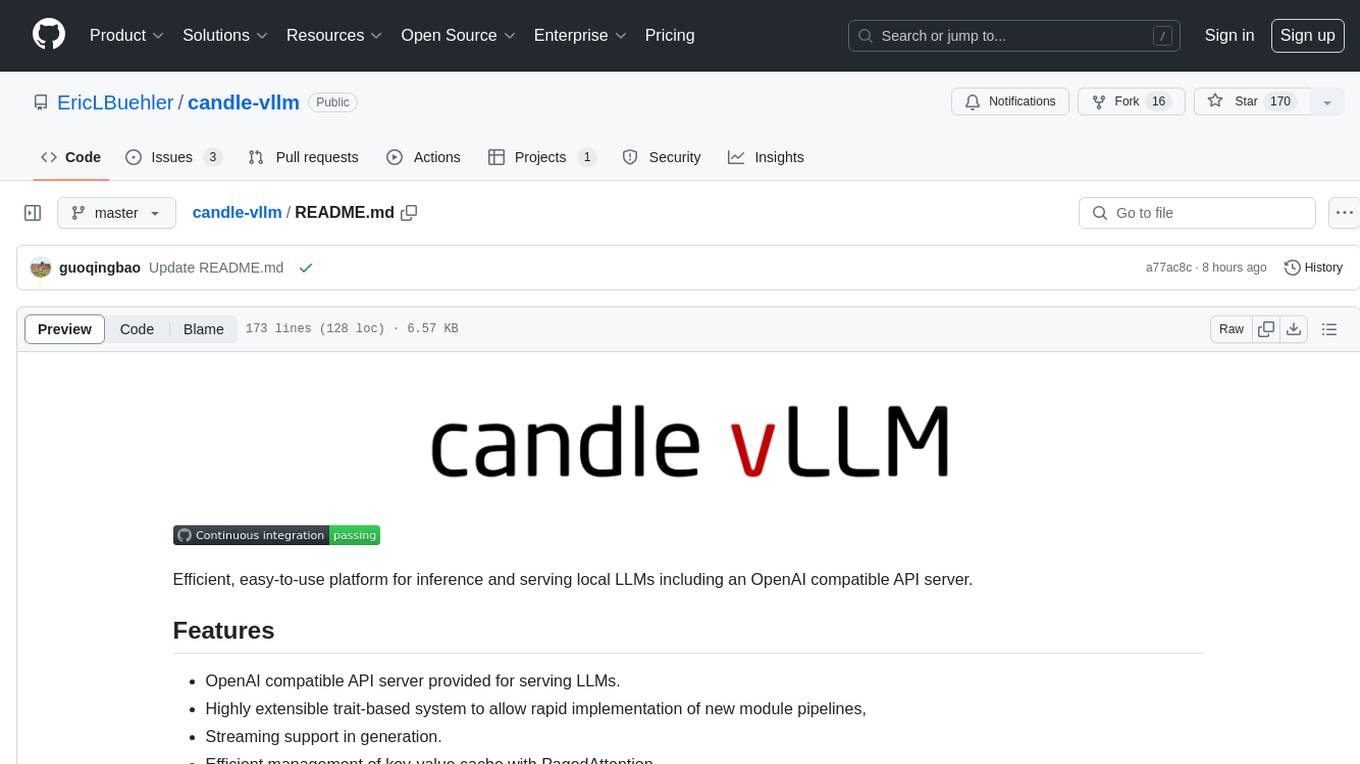
candle-vllm
Candle-vllm is an efficient and easy-to-use platform designed for inference and serving local LLMs, featuring an OpenAI compatible API server. It offers a highly extensible trait-based system for rapid implementation of new module pipelines, streaming support in generation, efficient management of key-value cache with PagedAttention, and continuous batching. The tool supports chat serving for various models and provides a seamless experience for users to interact with LLMs through different interfaces.
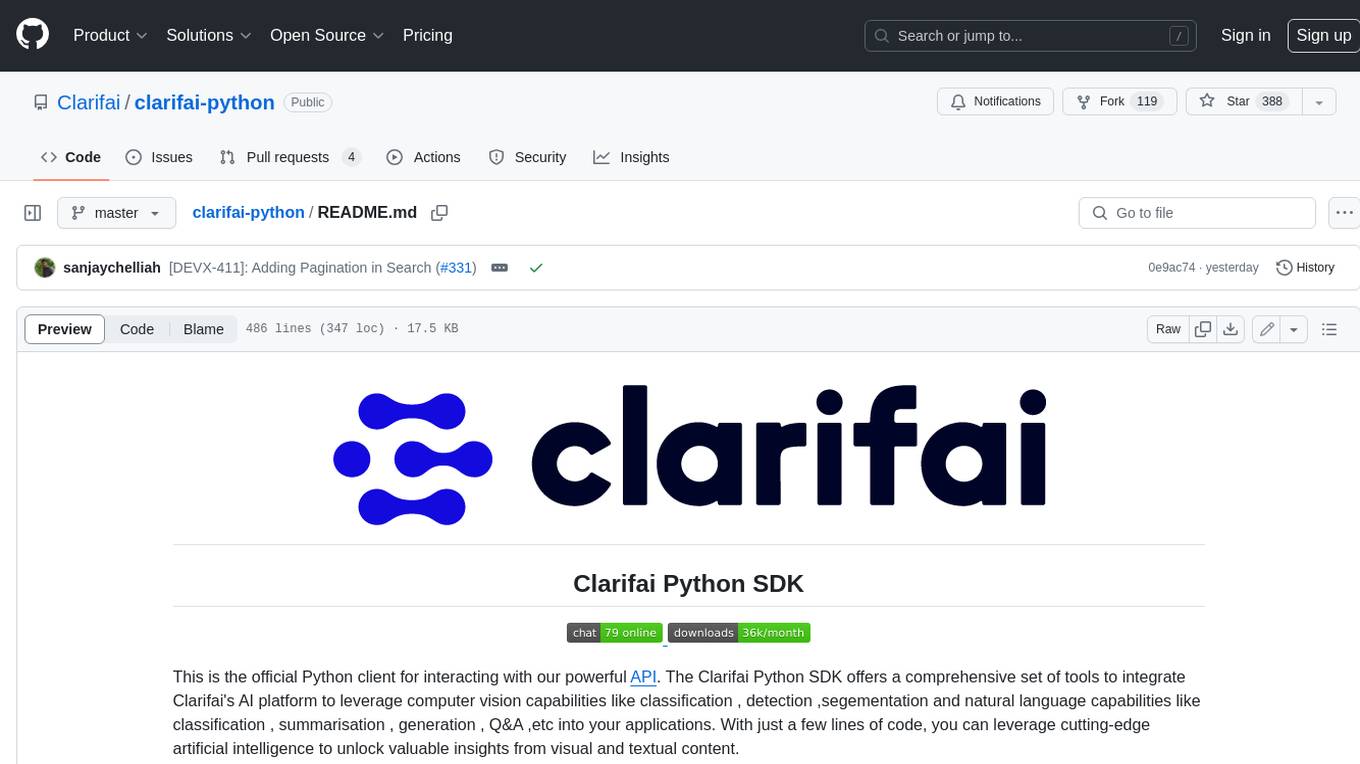
clarifai-python
The Clarifai Python SDK offers a comprehensive set of tools to integrate Clarifai's AI platform to leverage computer vision capabilities like classification , detection ,segementation and natural language capabilities like classification , summarisation , generation , Q&A ,etc into your applications. With just a few lines of code, you can leverage cutting-edge artificial intelligence to unlock valuable insights from visual and textual content.
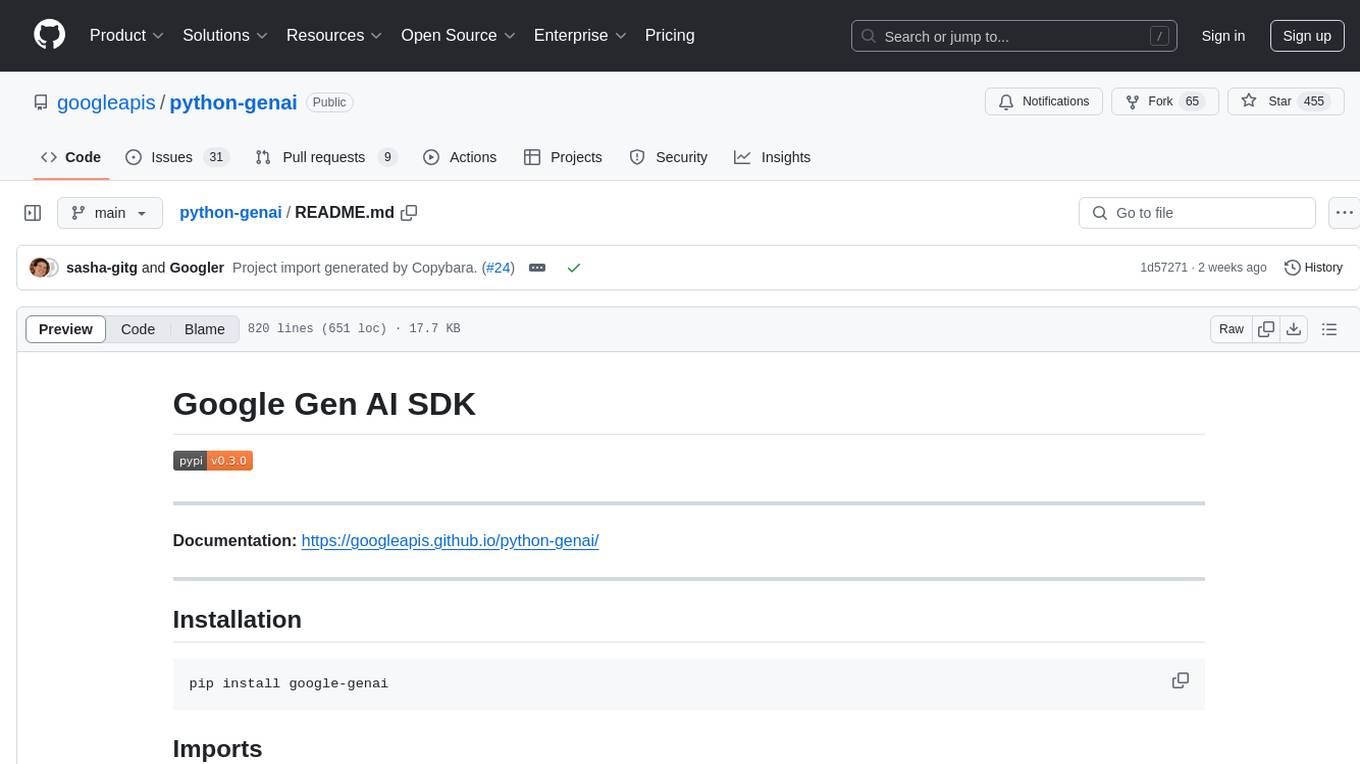
python-genai
The Google Gen AI SDK is a Python library that provides access to Google AI and Vertex AI services. It allows users to create clients for different services, work with parameter types, models, generate content, call functions, handle JSON response schemas, stream text and image content, perform async operations, count and compute tokens, embed content, generate and upscale images, edit images, work with files, create and get cached content, tune models, distill models, perform batch predictions, and more. The SDK supports various features like automatic function support, manual function declaration, JSON response schema support, streaming for text and image content, async methods, tuning job APIs, distillation, batch prediction, and more.
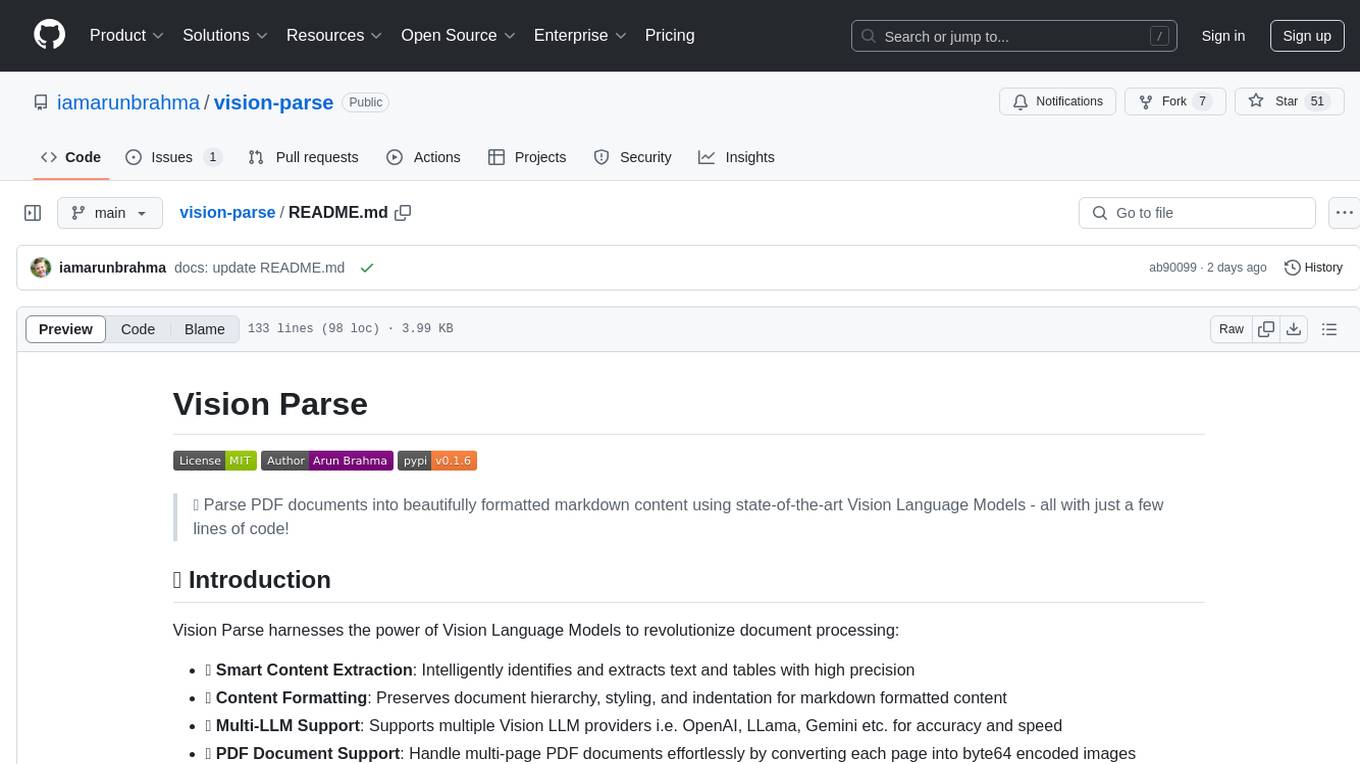
vision-parse
Vision Parse is a tool that leverages Vision Language Models to parse PDF documents into beautifully formatted markdown content. It offers smart content extraction, content formatting, multi-LLM support, PDF document support, and local model hosting using Ollama. Users can easily convert PDFs to markdown with high precision and preserve document hierarchy and styling. The tool supports multiple Vision LLM providers like OpenAI, LLama, and Gemini for accuracy and speed, making document processing efficient and effortless.
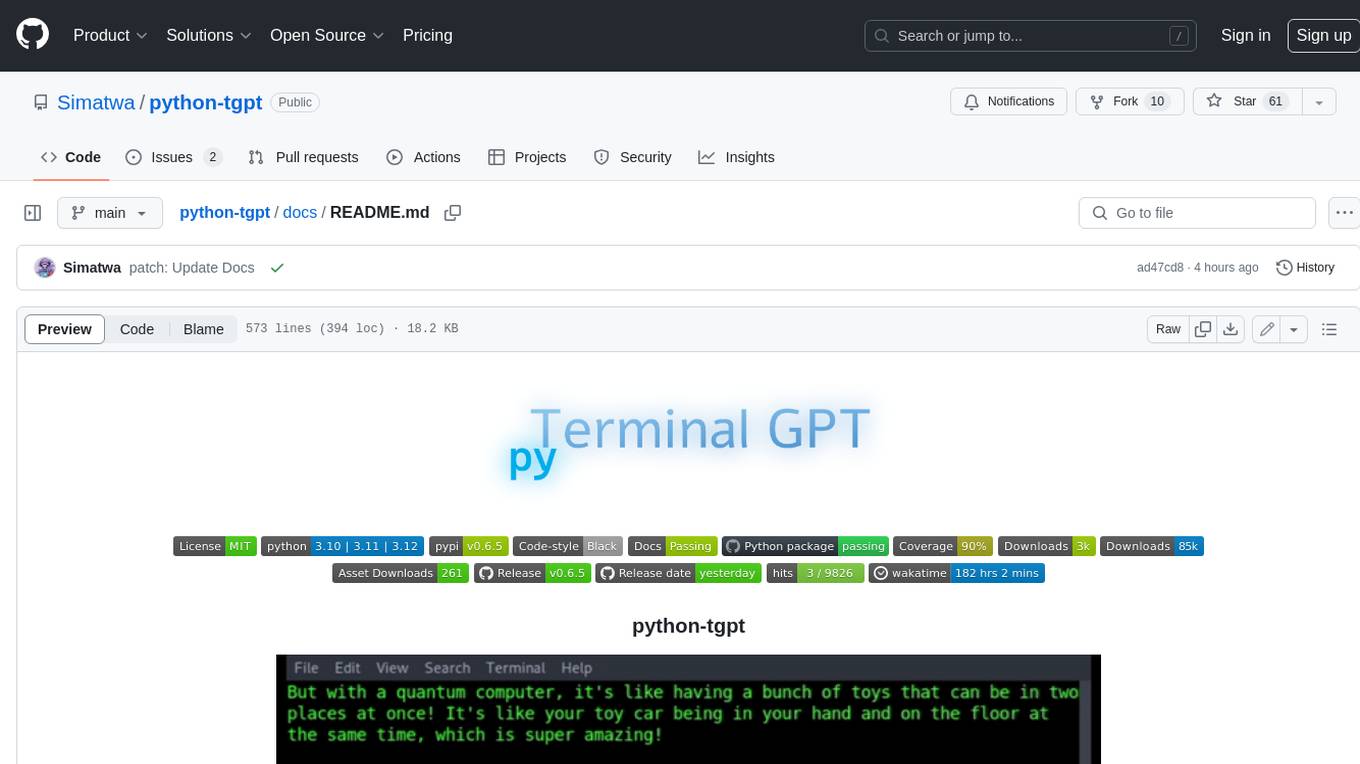
python-tgpt
Python-tgpt is a Python package that enables seamless interaction with over 45 free LLM providers without requiring an API key. It also provides image generation capabilities. The name _python-tgpt_ draws inspiration from its parent project tgpt, which operates on Golang. Through this Python adaptation, users can effortlessly engage with a number of free LLMs available, fostering a smoother AI interaction experience.
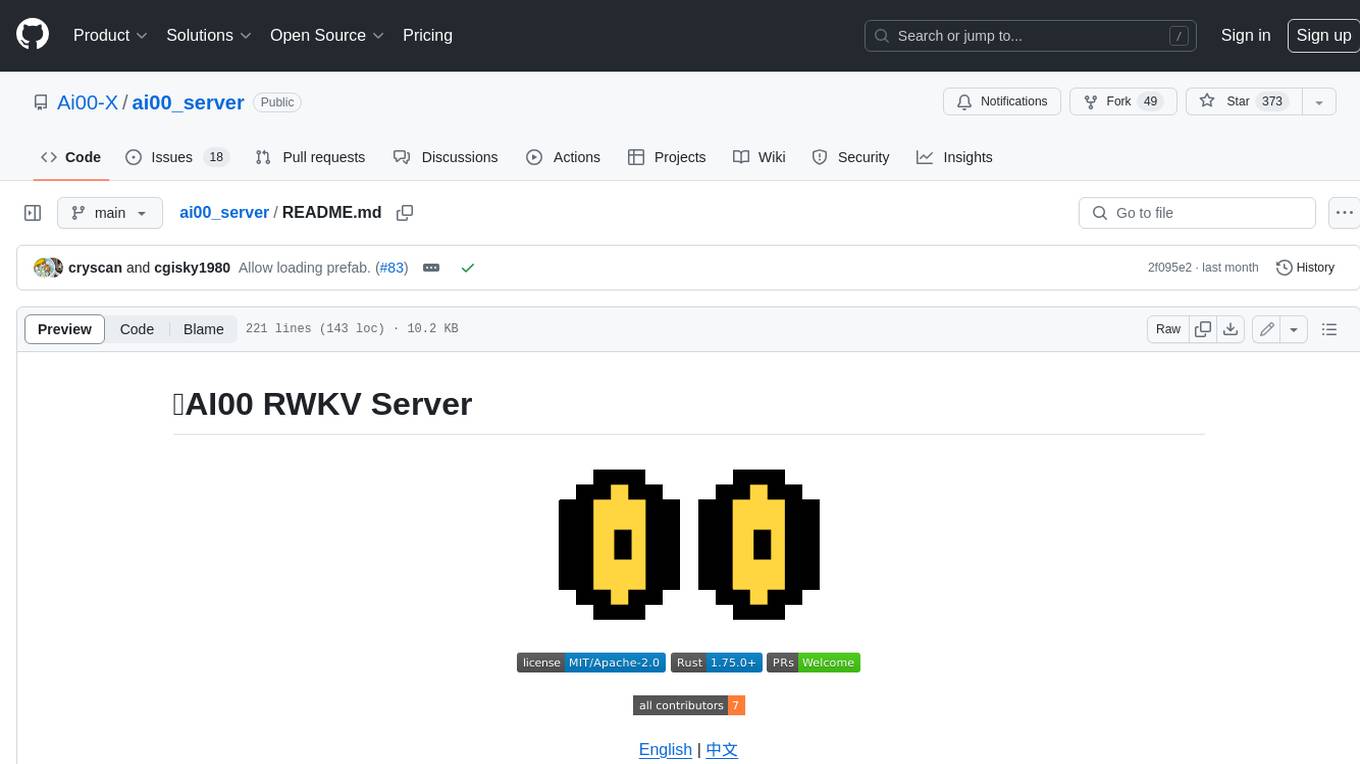
ai00_server
AI00 RWKV Server is an inference API server for the RWKV language model based upon the web-rwkv inference engine. It supports VULKAN parallel and concurrent batched inference and can run on all GPUs that support VULKAN. No need for Nvidia cards!!! AMD cards and even integrated graphics can be accelerated!!! No need for bulky pytorch, CUDA and other runtime environments, it's compact and ready to use out of the box! Compatible with OpenAI's ChatGPT API interface. 100% open source and commercially usable, under the MIT license. If you are looking for a fast, efficient, and easy-to-use LLM API server, then AI00 RWKV Server is your best choice. It can be used for various tasks, including chatbots, text generation, translation, and Q&A.
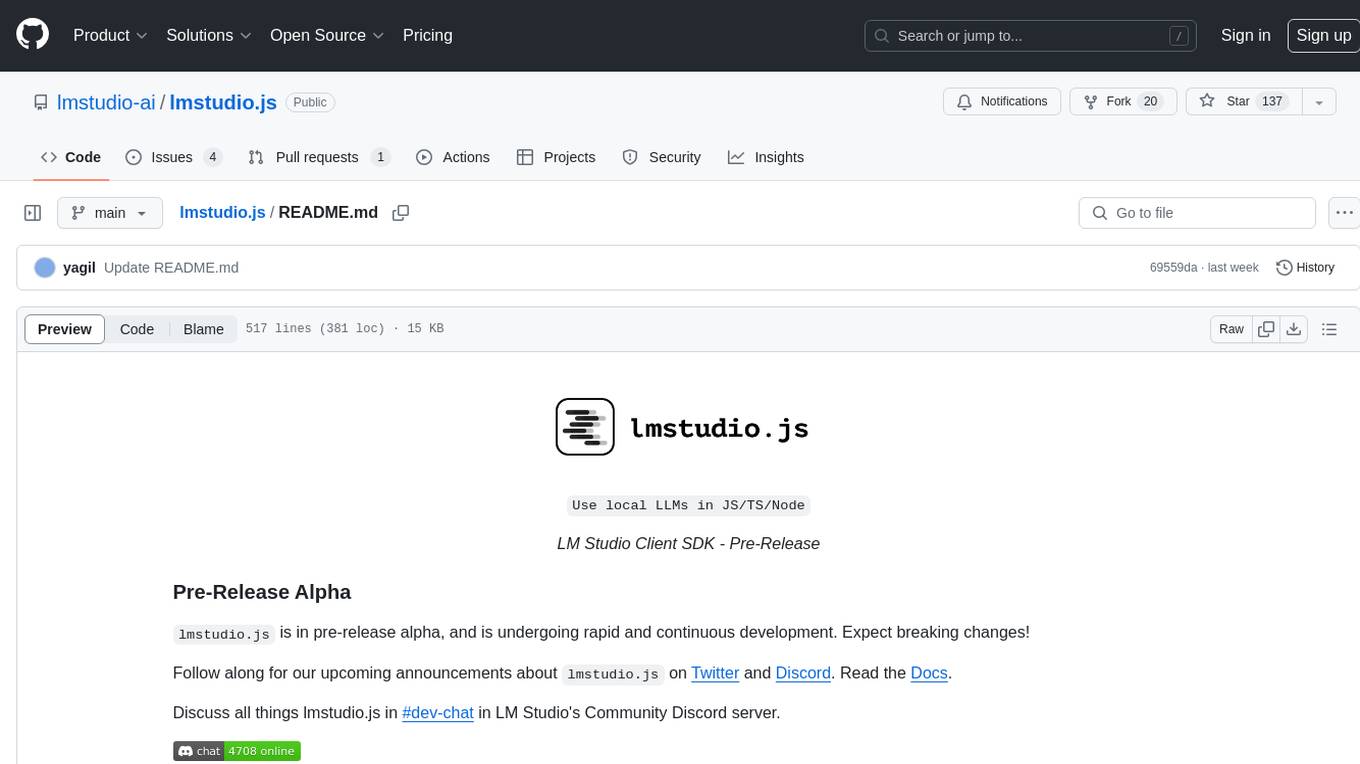
lmstudio.js
lmstudio.js is a pre-release alpha client SDK for LM Studio, allowing users to use local LLMs in JS/TS/Node. It is currently undergoing rapid development with breaking changes expected. Users can follow LM Studio's announcements on Twitter and Discord. The SDK provides API usage for loading models, predicting text, setting up the local LLM server, and more. It supports features like custom loading progress tracking, model unloading, structured output prediction, and cancellation of predictions. Users can interact with LM Studio through the CLI tool 'lms' and perform tasks like text completion, conversation, and getting prediction statistics.
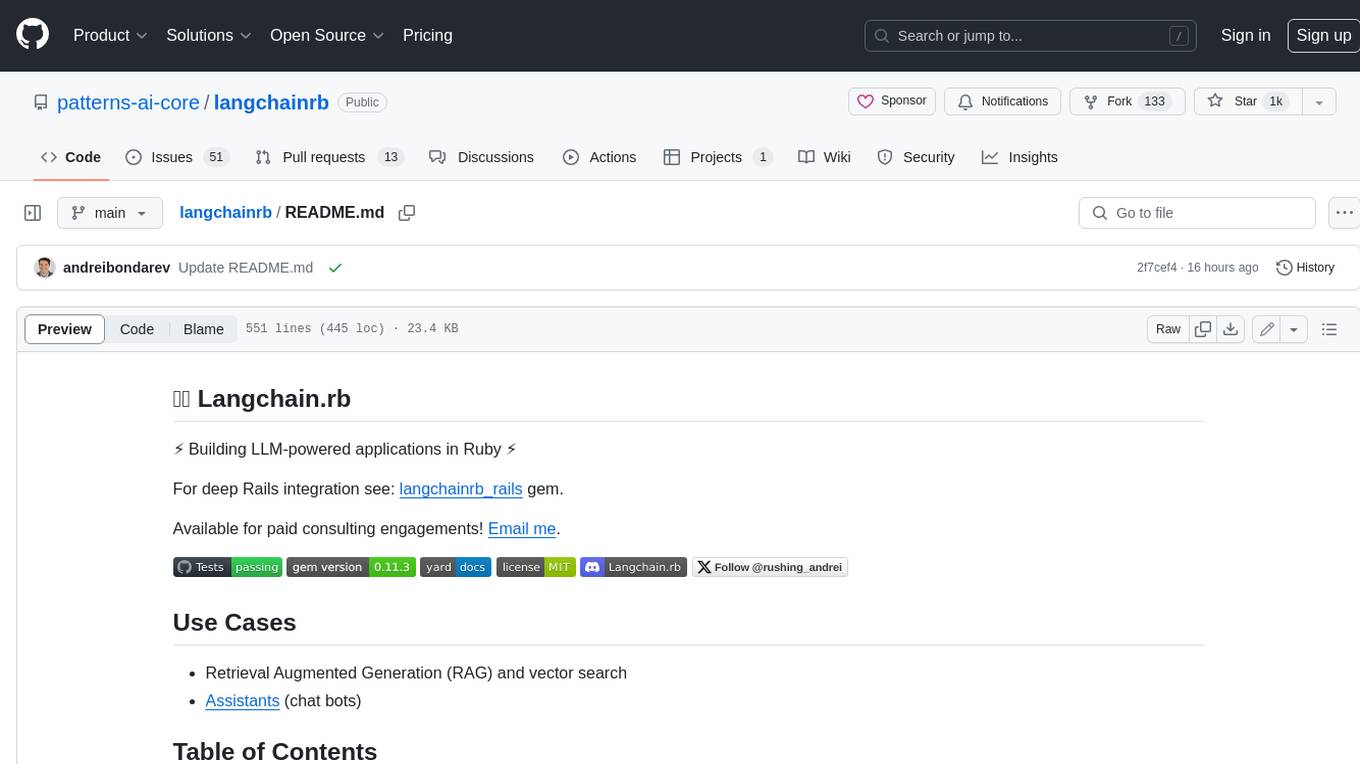
langchainrb
Langchain.rb is a Ruby library that makes it easy to build LLM-powered applications. It provides a unified interface to a variety of LLMs, vector search databases, and other tools, making it easy to build and deploy RAG (Retrieval Augmented Generation) systems and assistants. Langchain.rb is open source and available under the MIT License.
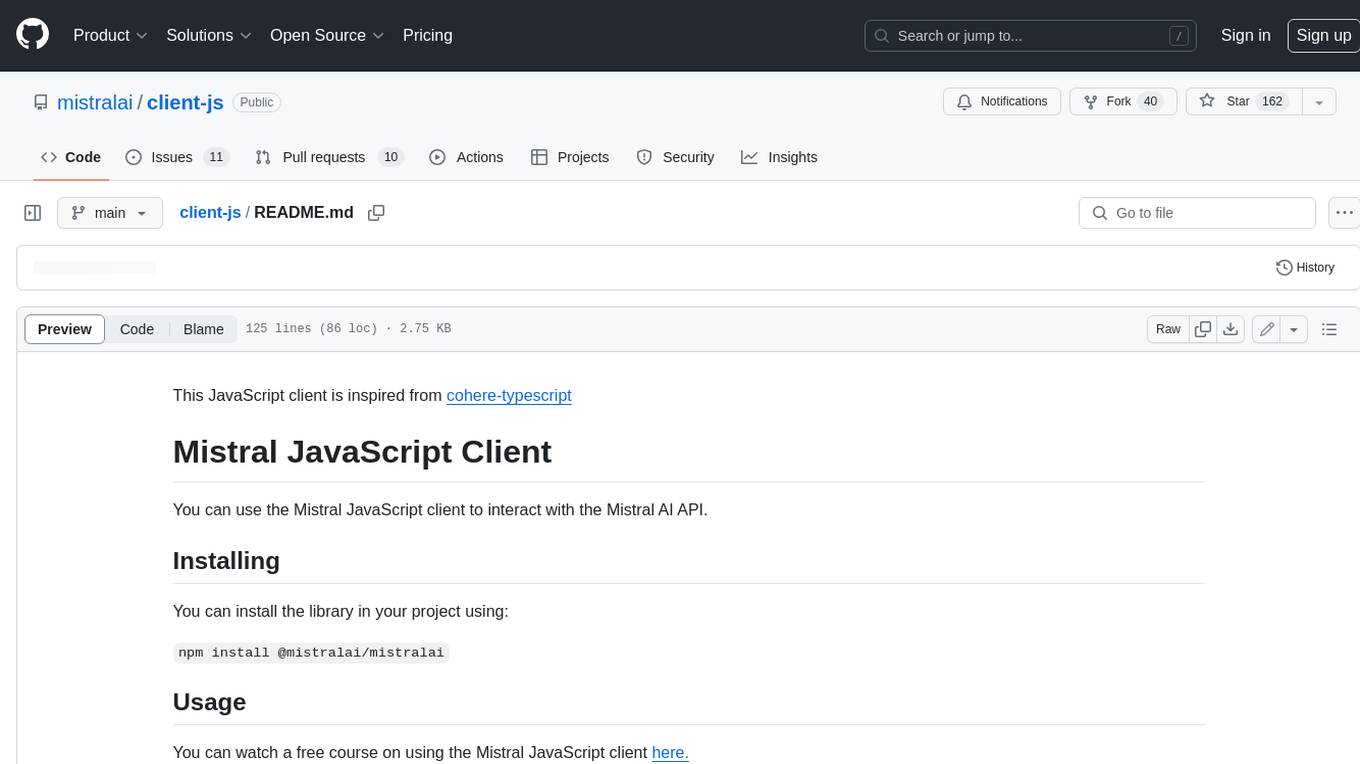
client-js
The Mistral JavaScript client is a library that allows you to interact with the Mistral AI API. With this client, you can perform various tasks such as listing models, chatting with streaming, chatting without streaming, and generating embeddings. To use the client, you can install it in your project using npm and then set up the client with your API key. Once the client is set up, you can use it to perform the desired tasks. For example, you can use the client to chat with a model by providing a list of messages. The client will then return the response from the model. You can also use the client to generate embeddings for a given input. The embeddings can then be used for various downstream tasks such as clustering or classification.
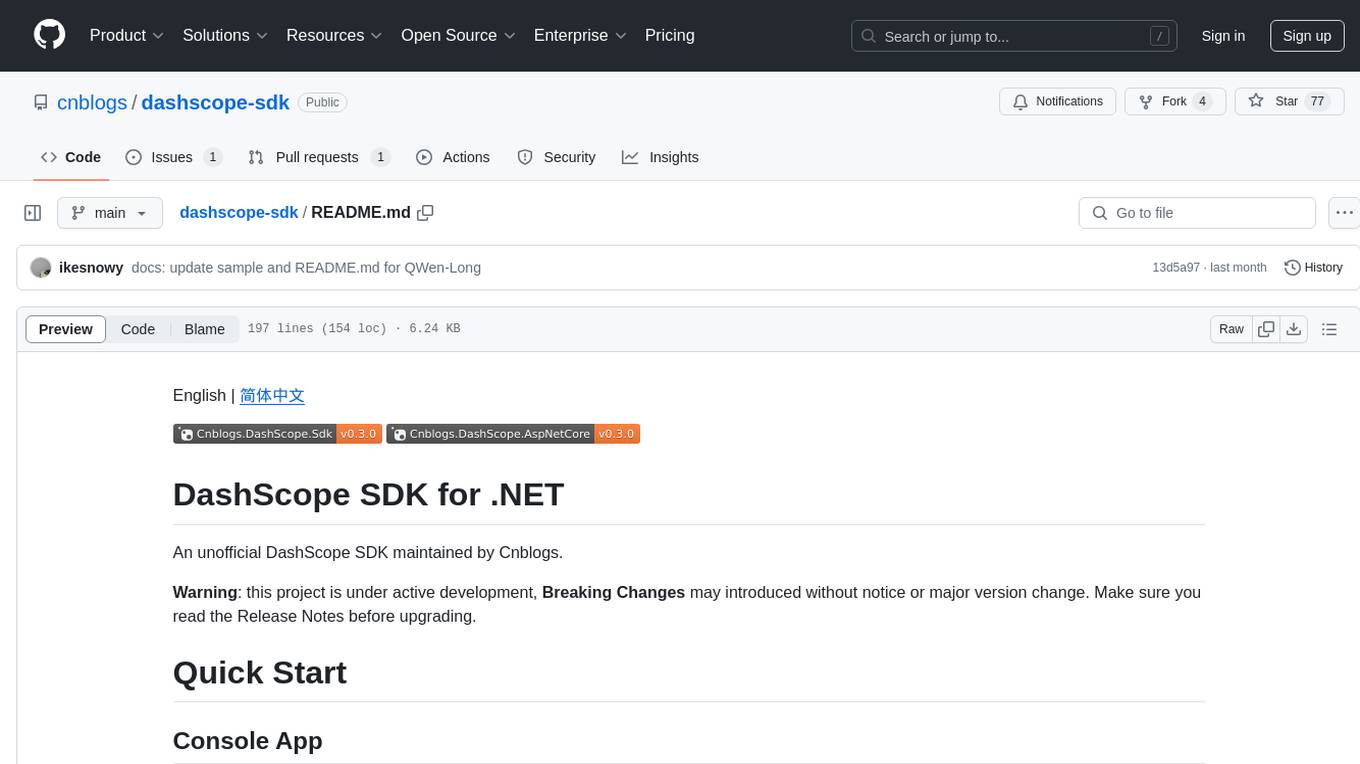
dashscope-sdk
DashScope SDK for .NET is an unofficial SDK maintained by Cnblogs, providing various APIs for text embedding, generation, multimodal generation, image synthesis, and more. Users can interact with the SDK to perform tasks such as text completion, chat generation, function calls, file operations, and more. The project is under active development, and users are advised to check the Release Notes before upgrading.
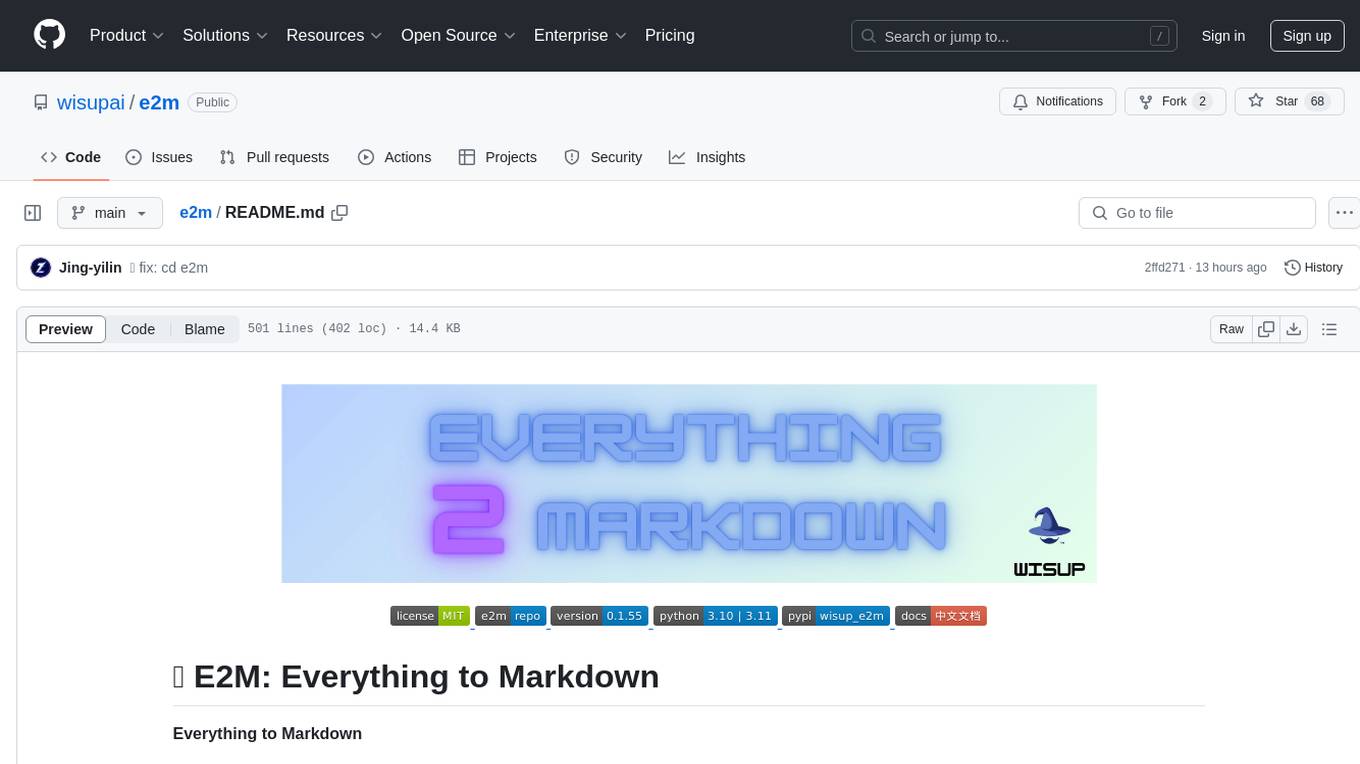
e2m
E2M is a Python library that can parse and convert various file types into Markdown format. It supports the conversion of multiple file formats, including doc, docx, epub, html, htm, url, pdf, ppt, pptx, mp3, and m4a. The ultimate goal of the E2M project is to provide high-quality data for Retrieval-Augmented Generation (RAG) and model training or fine-tuning. The core architecture consists of a Parser responsible for parsing various file types into text or image data, and a Converter responsible for converting text or image data into Markdown format.
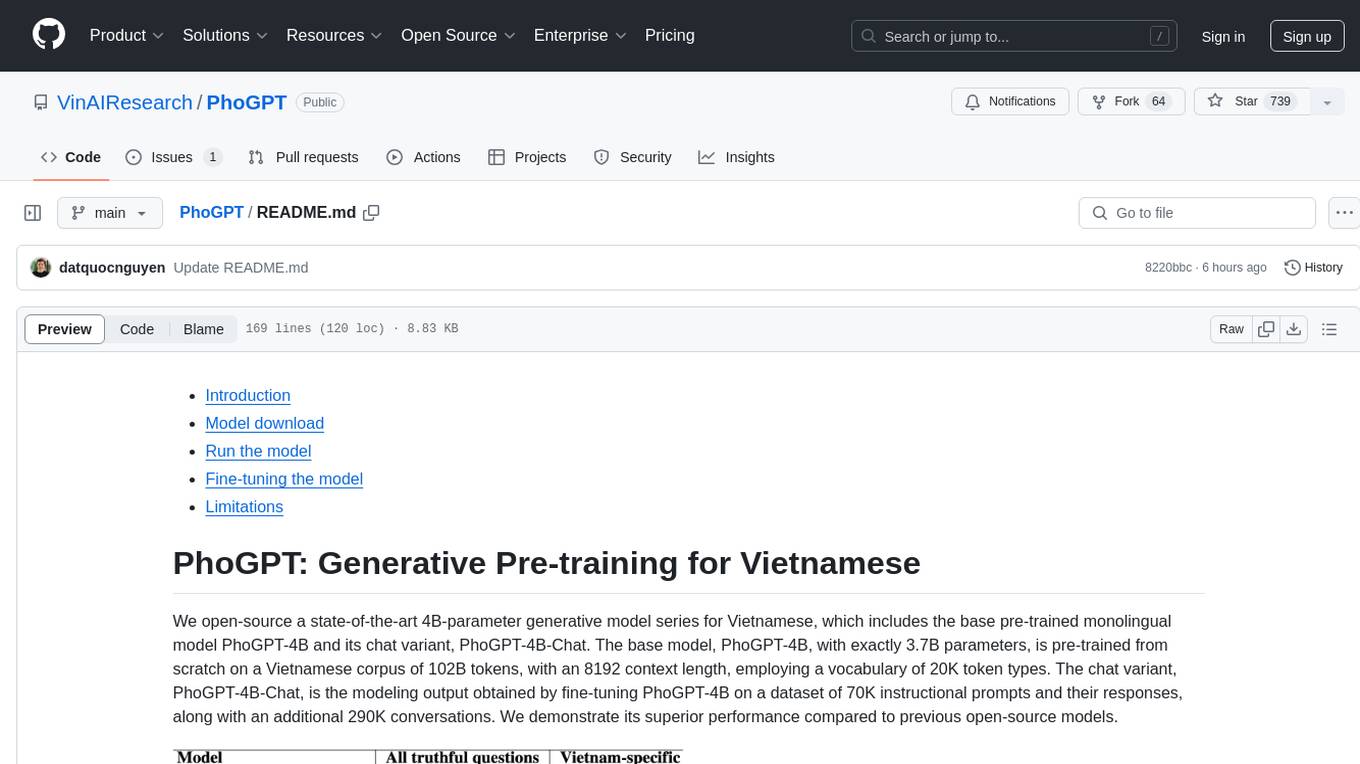
PhoGPT
PhoGPT is an open-source 4B-parameter generative model series for Vietnamese, including the base pre-trained monolingual model PhoGPT-4B and its chat variant, PhoGPT-4B-Chat. PhoGPT-4B is pre-trained from scratch on a Vietnamese corpus of 102B tokens, with an 8192 context length and a vocabulary of 20K token types. PhoGPT-4B-Chat is fine-tuned on instructional prompts and conversations, demonstrating superior performance. Users can run the model with inference engines like vLLM and Text Generation Inference, and fine-tune it using llm-foundry. However, PhoGPT has limitations in reasoning, coding, and mathematics tasks, and may generate harmful or biased responses.
For similar tasks
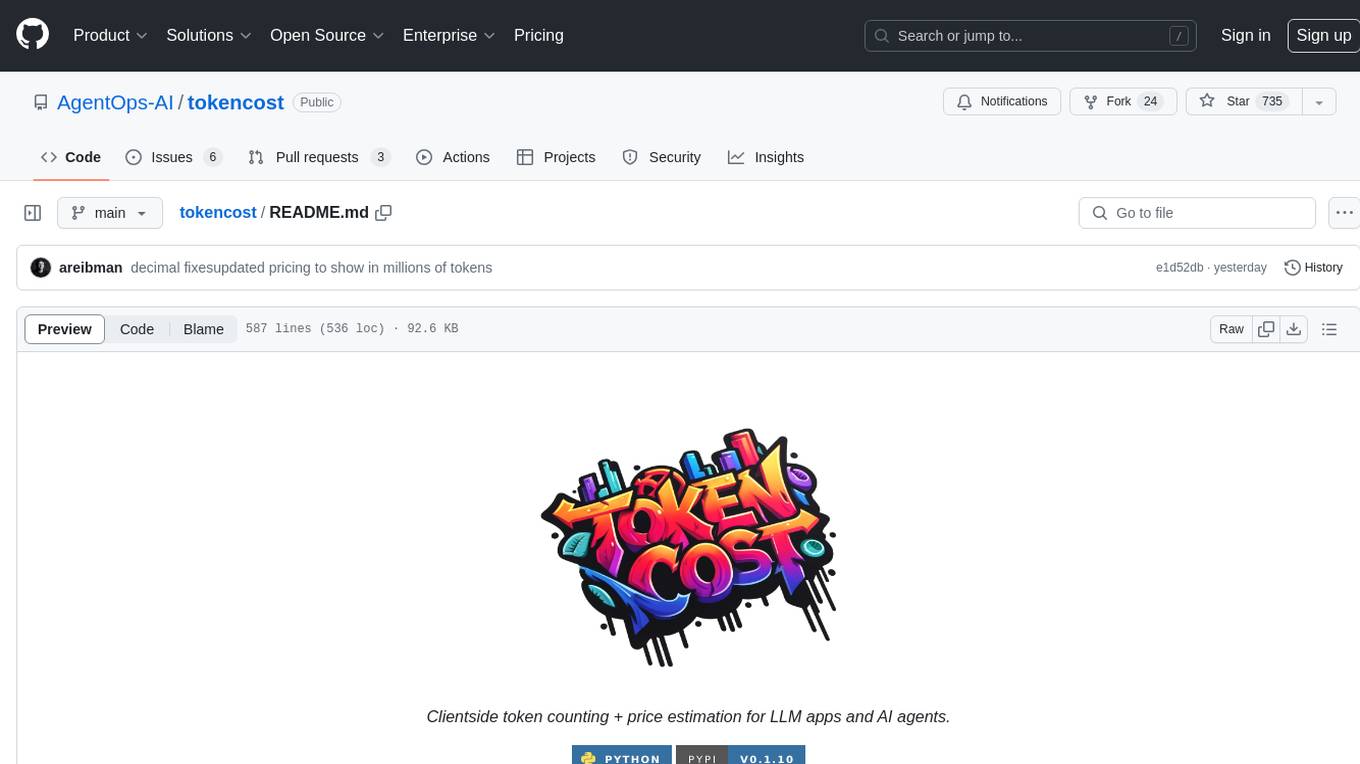
tokencost
Tokencost is a clientside tool for calculating the USD cost of using major Large Language Model (LLMs) APIs by estimating the cost of prompts and completions. It helps track the latest price changes of major LLM providers, accurately count prompt tokens before sending OpenAI requests, and easily integrate to get the cost of a prompt or completion with a single function. Users can calculate prompt and completion costs using OpenAI requests, count tokens in prompts formatted as message lists or string prompts, and refer to a cost table with updated prices for various LLM models. The tool also supports callback handlers for LLM wrapper/framework libraries like LlamaIndex and Langchain.
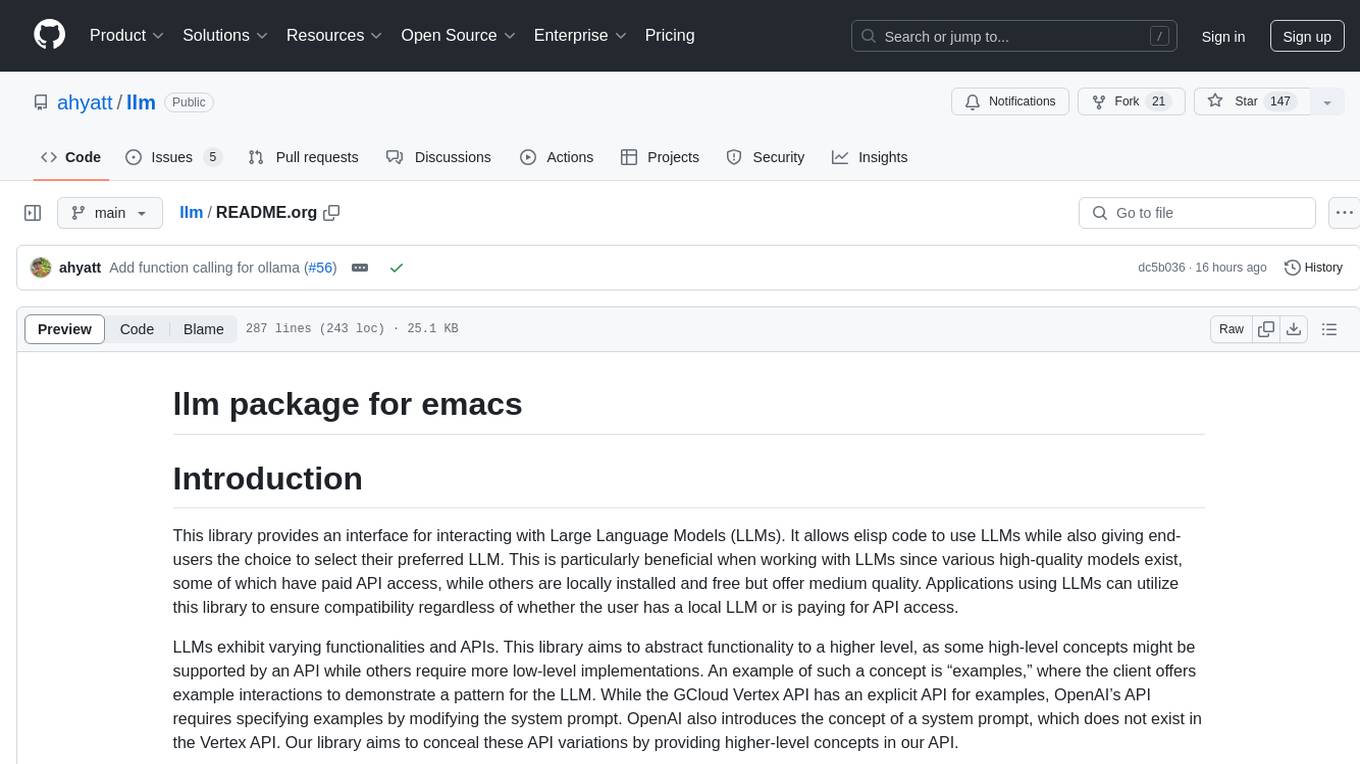
llm
The 'llm' package for Emacs provides an interface for interacting with Large Language Models (LLMs). It abstracts functionality to a higher level, concealing API variations and ensuring compatibility with various LLMs. Users can set up providers like OpenAI, Gemini, Vertex, Claude, Ollama, GPT4All, and a fake client for testing. The package allows for chat interactions, embeddings, token counting, and function calling. It also offers advanced prompt creation and logging capabilities. Users can handle conversations, create prompts with placeholders, and contribute by creating providers.
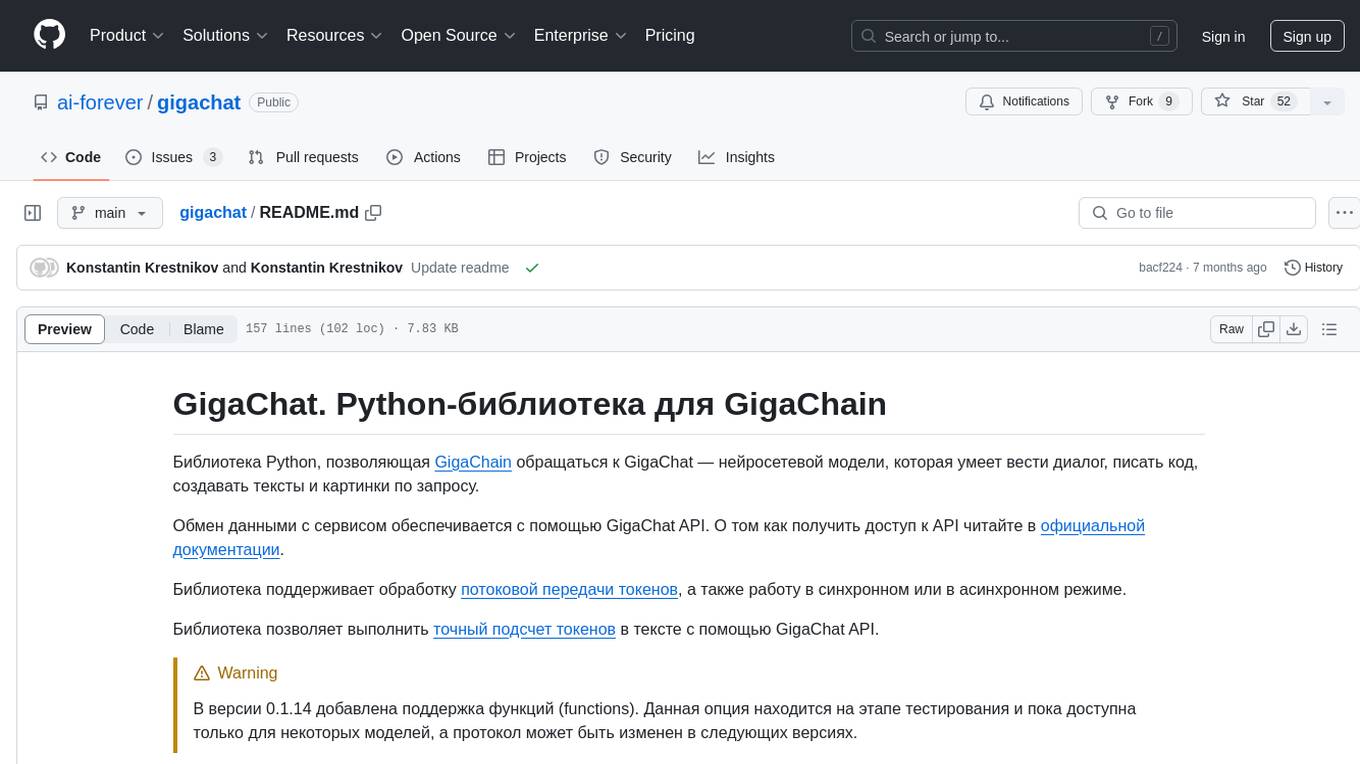
gigachat
GigaChat is a Python library that allows GigaChain to interact with GigaChat, a neural network model capable of engaging in dialogue, writing code, creating texts, and images on demand. Data exchange with the service is facilitated through the GigaChat API. The library supports processing token streaming, as well as working in synchronous or asynchronous mode. It enables precise token counting in text using the GigaChat API.
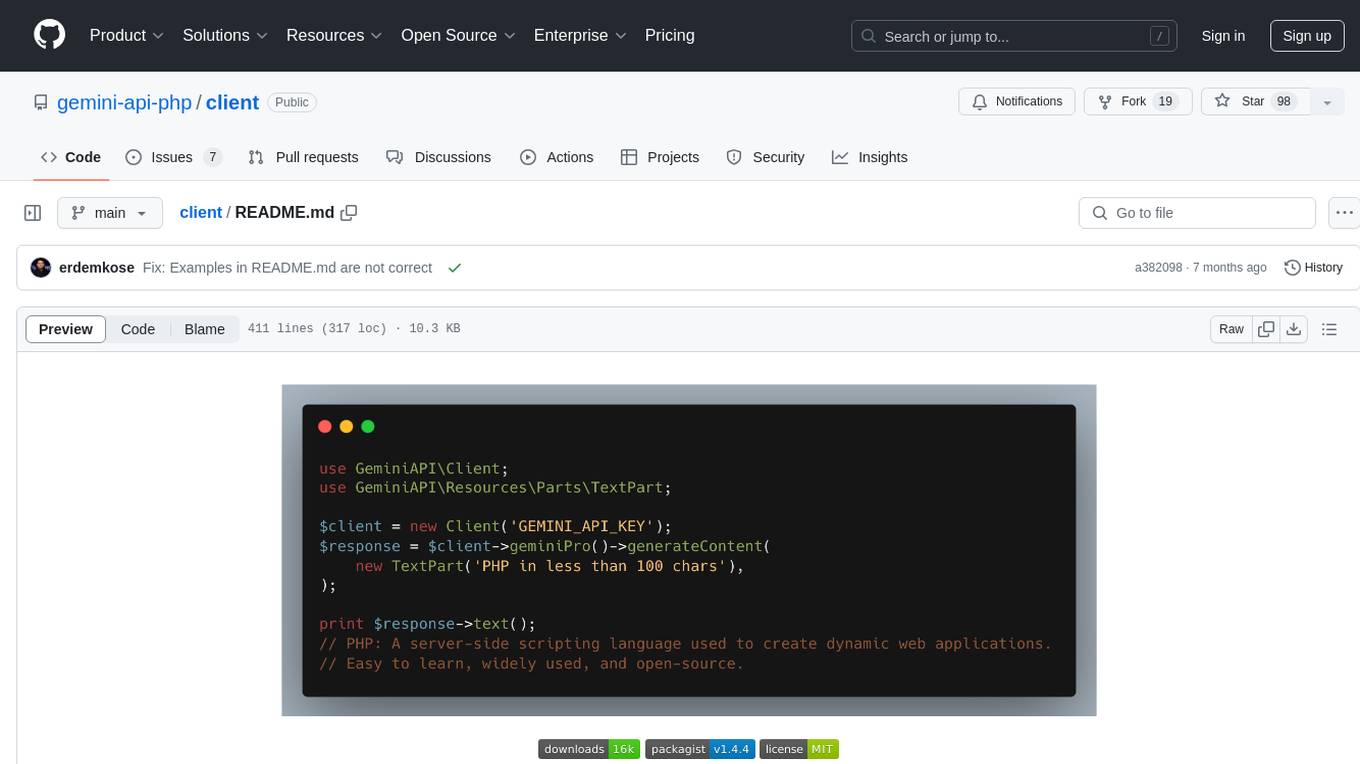
client
Gemini API PHP Client is a library that allows you to interact with Google's generative AI models, such as Gemini Pro and Gemini Pro Vision. It provides functionalities for basic text generation, multimodal input, chat sessions, streaming responses, tokens counting, listing models, and advanced usages like safety settings and custom HTTP client usage. The library requires an API key to access Google's Gemini API and can be installed using Composer. It supports various features like generating content, starting chat sessions, embedding content, counting tokens, and listing available models.
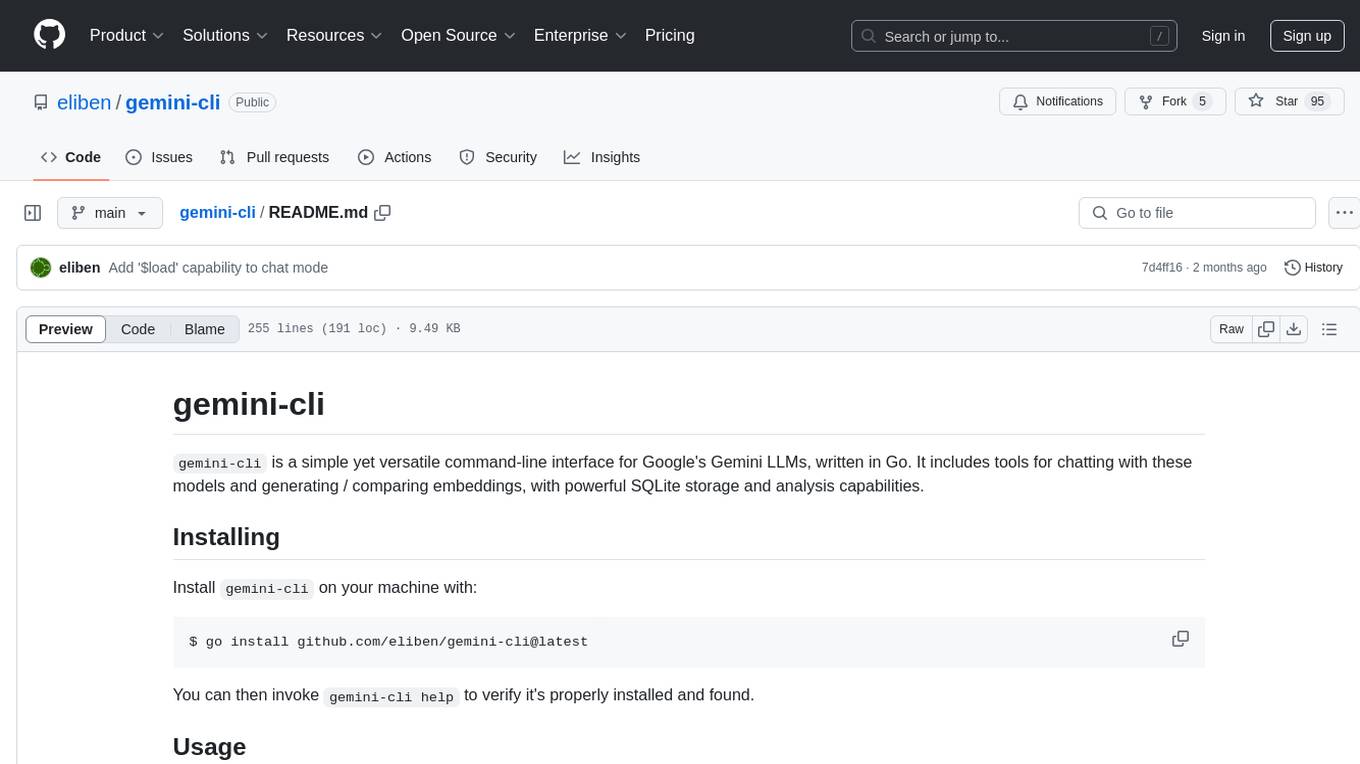
gemini-cli
gemini-cli is a versatile command-line interface for Google's Gemini LLMs, written in Go. It includes tools for chatting with models, generating/comparing embeddings, and storing data in SQLite for analysis. Users can interact with Gemini models through various subcommands like prompt, chat, counttok, embed content, embed db, and embed similar.
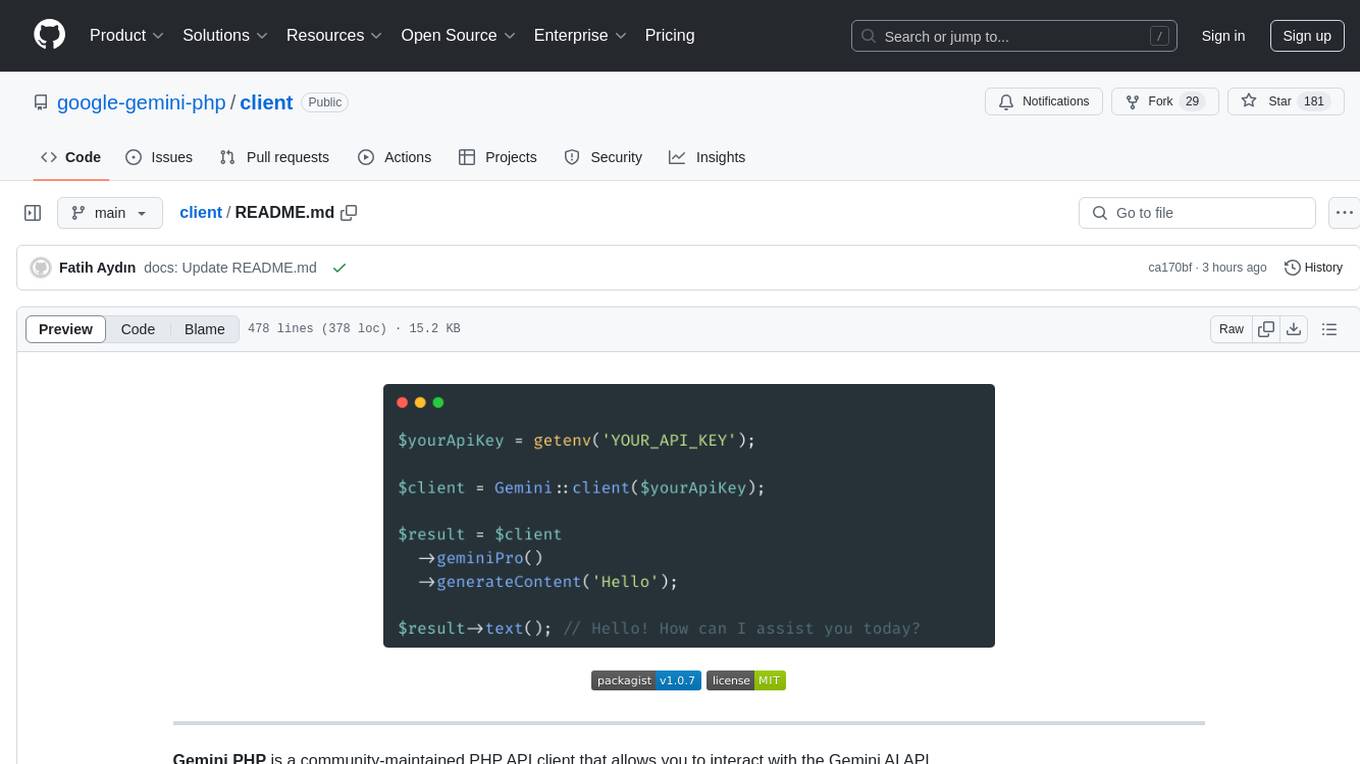
client
Gemini PHP is a PHP API client for interacting with the Gemini AI API. It allows users to generate content, chat, count tokens, configure models, embed resources, list models, get model information, troubleshoot timeouts, and test API responses. The client supports various features such as text-only input, text-and-image input, multi-turn conversations, streaming content generation, token counting, model configuration, and embedding techniques. Users can interact with Gemini's API to perform tasks related to natural language generation and text analysis.
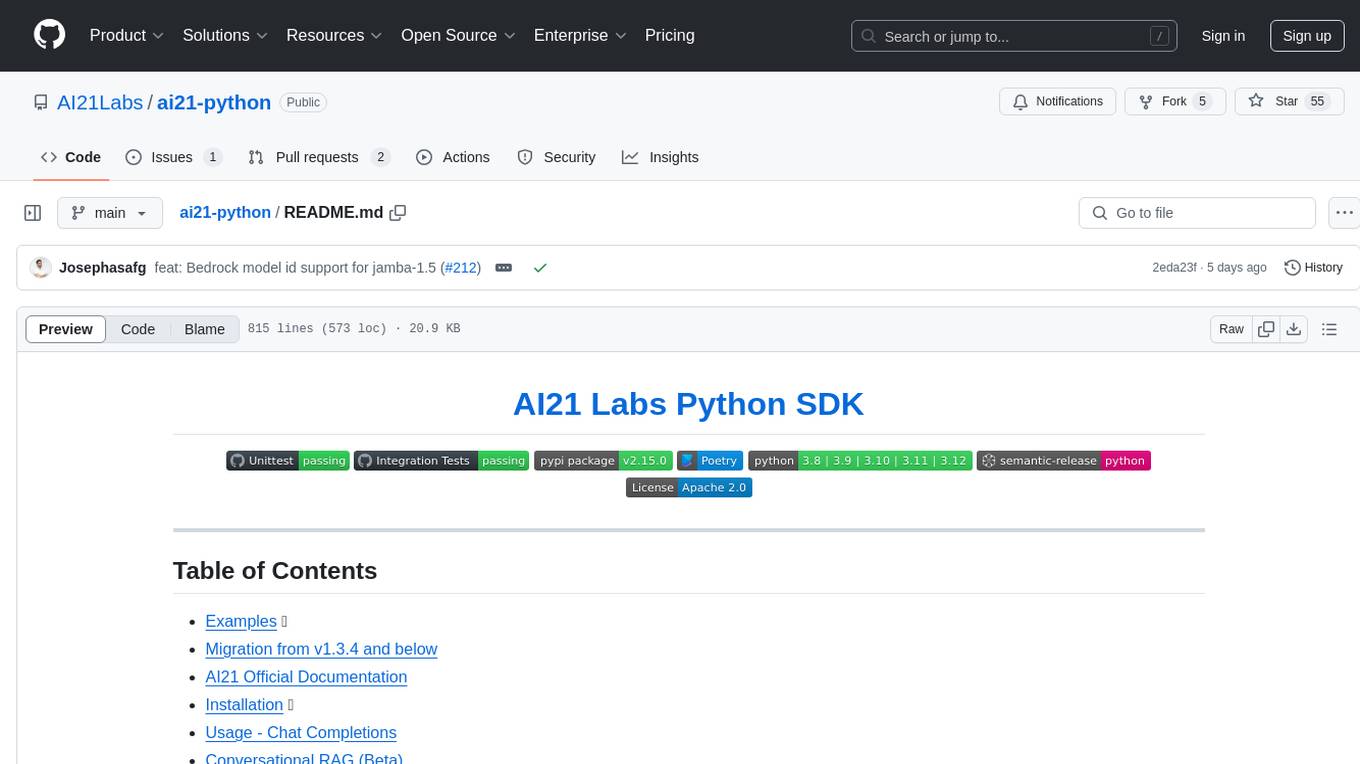
ai21-python
The AI21 Labs Python SDK is a comprehensive tool for interacting with the AI21 API. It provides functionalities for chat completions, conversational RAG, token counting, error handling, and support for various cloud providers like AWS, Azure, and Vertex. The SDK offers both synchronous and asynchronous usage, along with detailed examples and documentation. Users can quickly get started with the SDK to leverage AI21's powerful models for various natural language processing tasks.
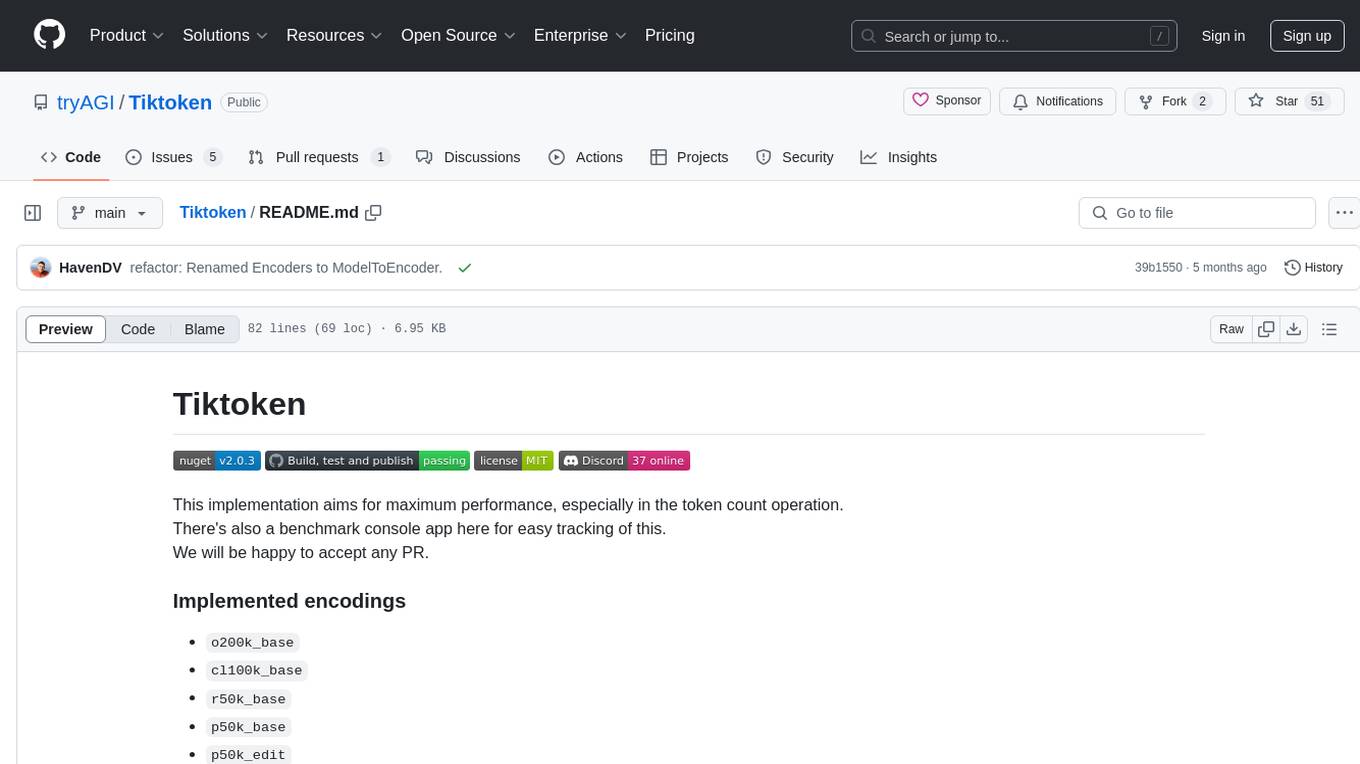
Tiktoken
Tiktoken is a high-performance implementation focused on token count operations. It provides various encodings like o200k_base, cl100k_base, r50k_base, p50k_base, and p50k_edit. Users can easily encode and decode text using the provided API. The repository also includes a benchmark console app for performance tracking. Contributions in the form of PRs are welcome.
For similar jobs
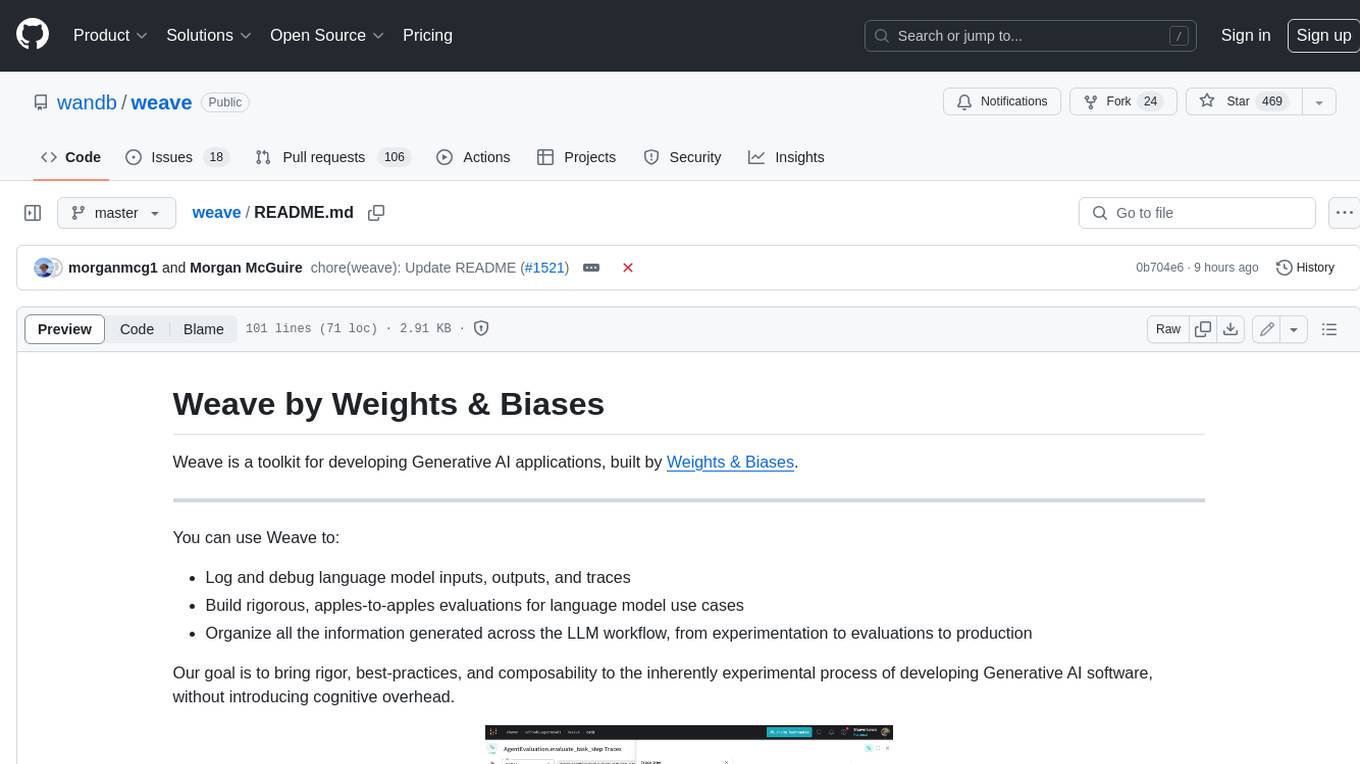
weave
Weave is a toolkit for developing Generative AI applications, built by Weights & Biases. With Weave, you can log and debug language model inputs, outputs, and traces; build rigorous, apples-to-apples evaluations for language model use cases; and organize all the information generated across the LLM workflow, from experimentation to evaluations to production. Weave aims to bring rigor, best-practices, and composability to the inherently experimental process of developing Generative AI software, without introducing cognitive overhead.
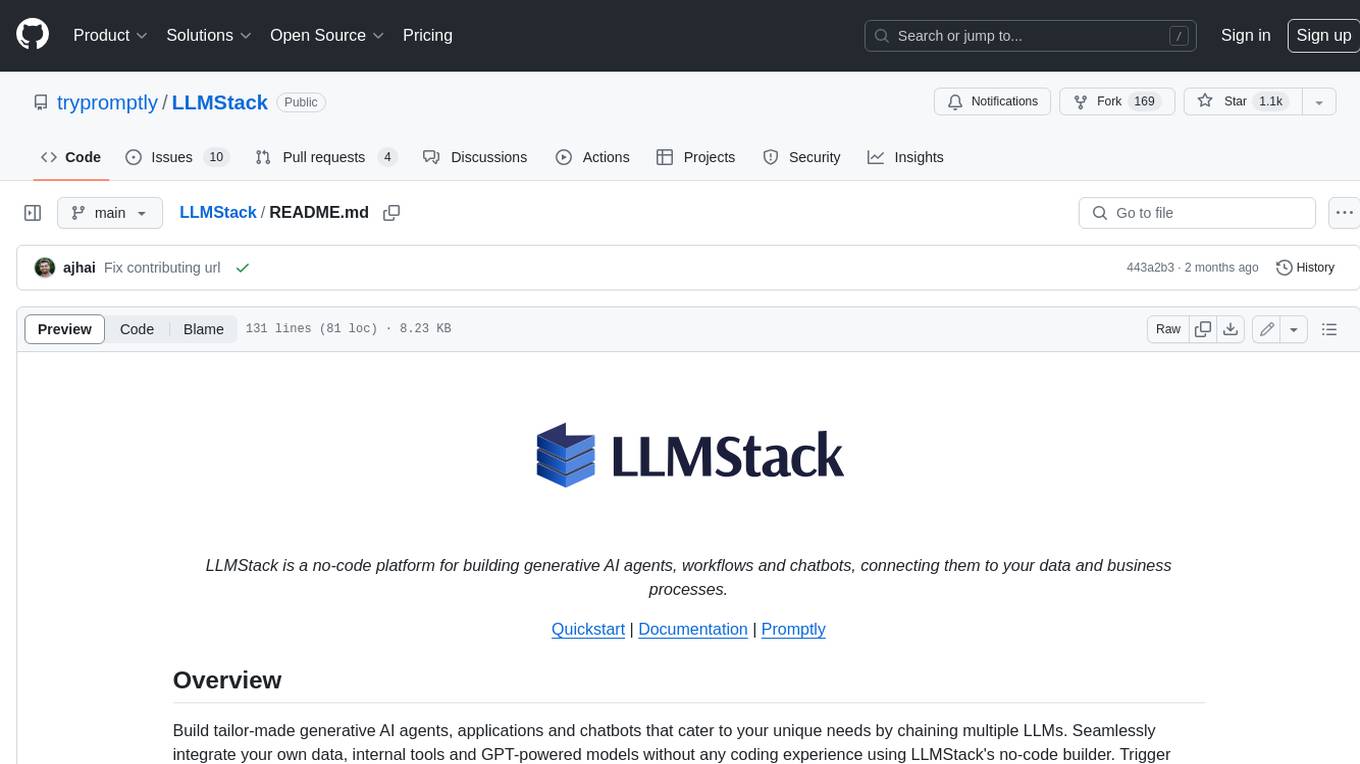
LLMStack
LLMStack is a no-code platform for building generative AI agents, workflows, and chatbots. It allows users to connect their own data, internal tools, and GPT-powered models without any coding experience. LLMStack can be deployed to the cloud or on-premise and can be accessed via HTTP API or triggered from Slack or Discord.
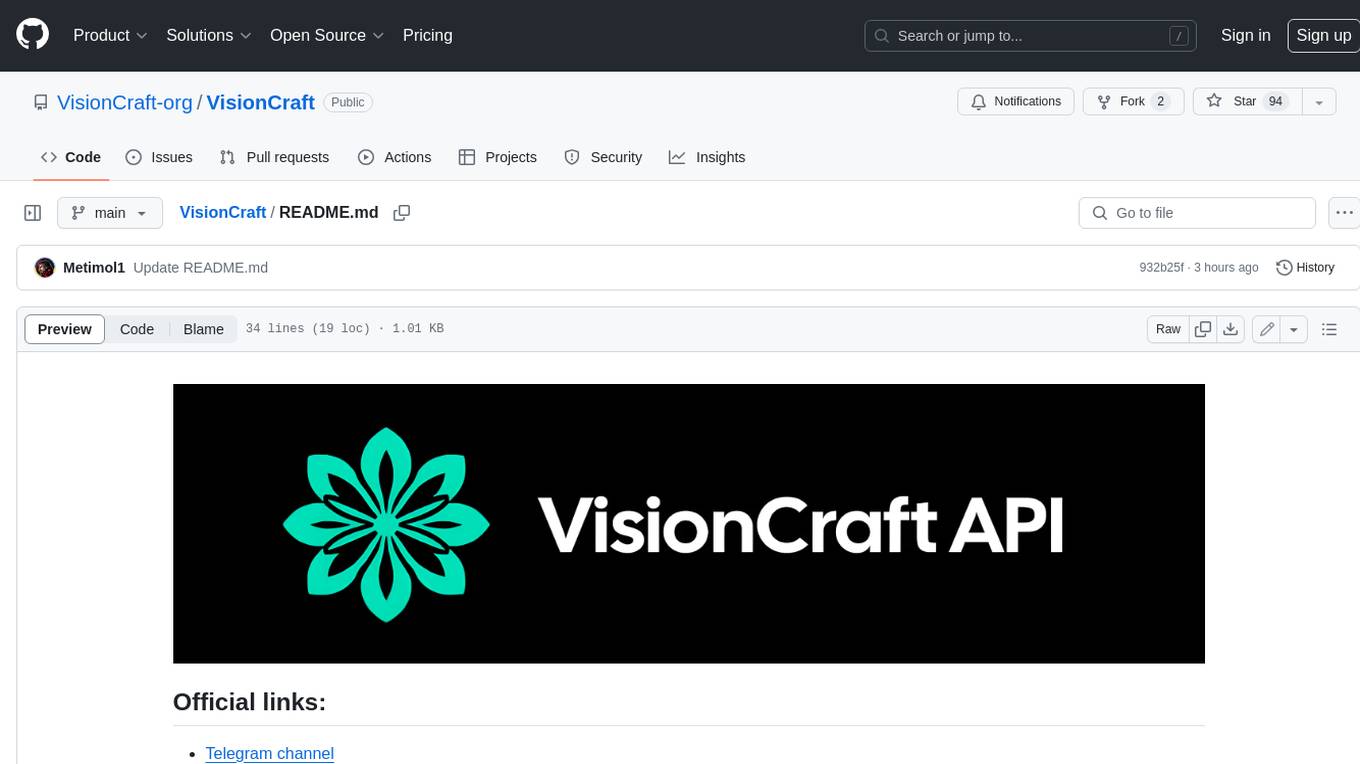
VisionCraft
The VisionCraft API is a free API for using over 100 different AI models. From images to sound.
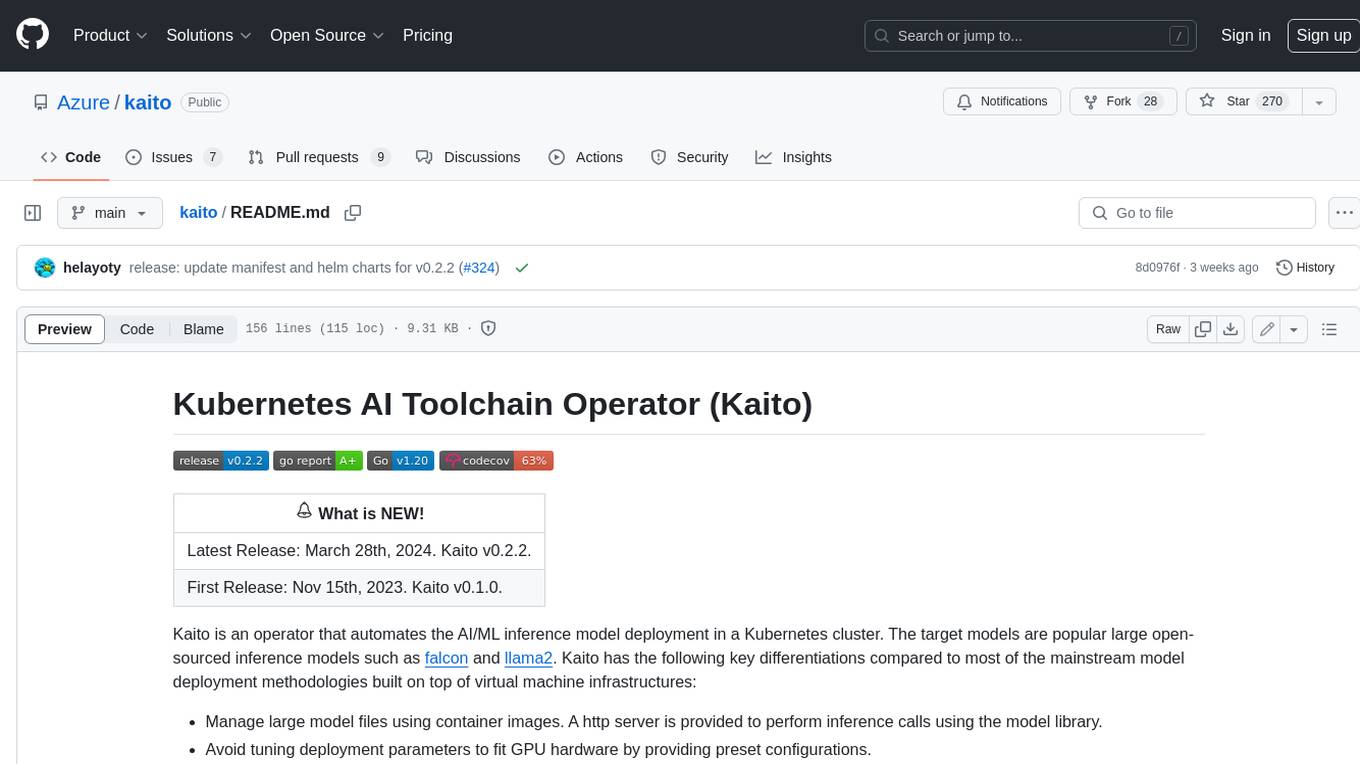
kaito
Kaito is an operator that automates the AI/ML inference model deployment in a Kubernetes cluster. It manages large model files using container images, avoids tuning deployment parameters to fit GPU hardware by providing preset configurations, auto-provisions GPU nodes based on model requirements, and hosts large model images in the public Microsoft Container Registry (MCR) if the license allows. Using Kaito, the workflow of onboarding large AI inference models in Kubernetes is largely simplified.
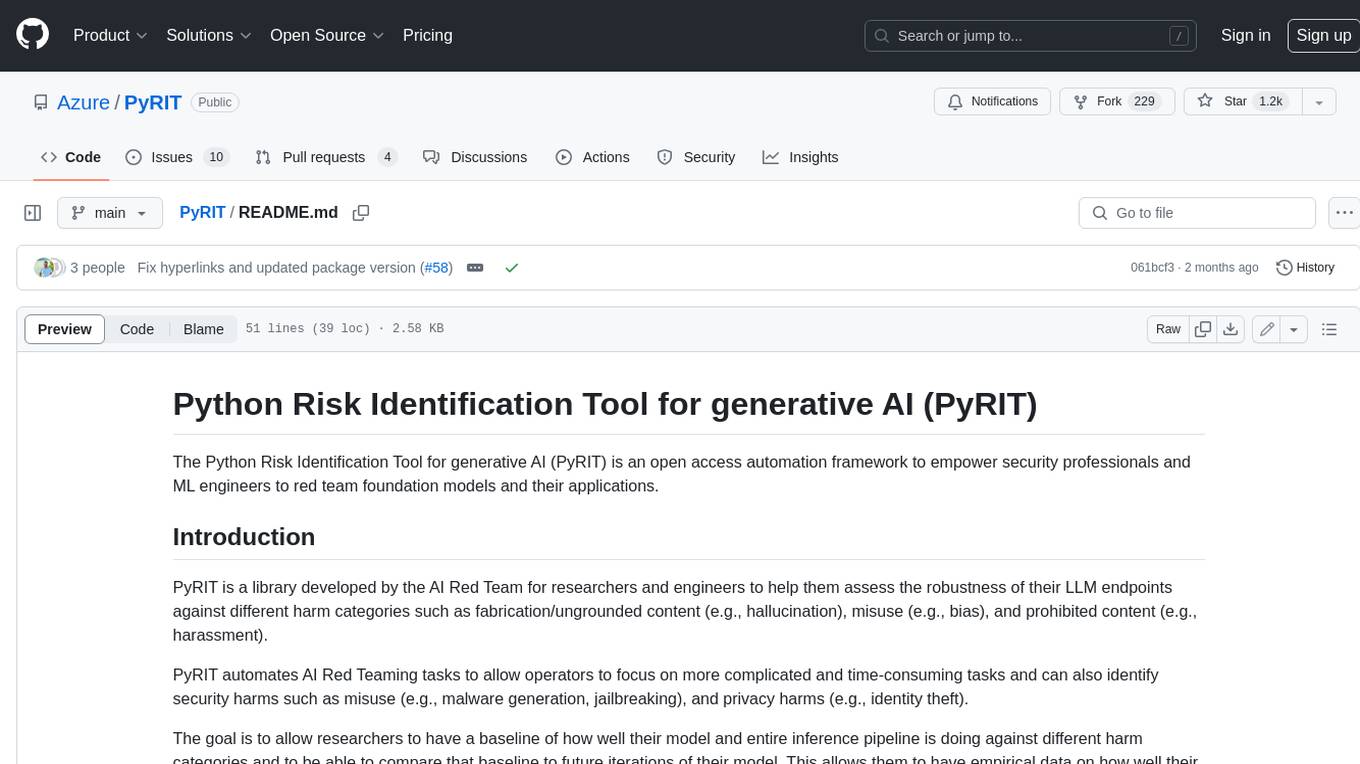
PyRIT
PyRIT is an open access automation framework designed to empower security professionals and ML engineers to red team foundation models and their applications. It automates AI Red Teaming tasks to allow operators to focus on more complicated and time-consuming tasks and can also identify security harms such as misuse (e.g., malware generation, jailbreaking), and privacy harms (e.g., identity theft). The goal is to allow researchers to have a baseline of how well their model and entire inference pipeline is doing against different harm categories and to be able to compare that baseline to future iterations of their model. This allows them to have empirical data on how well their model is doing today, and detect any degradation of performance based on future improvements.
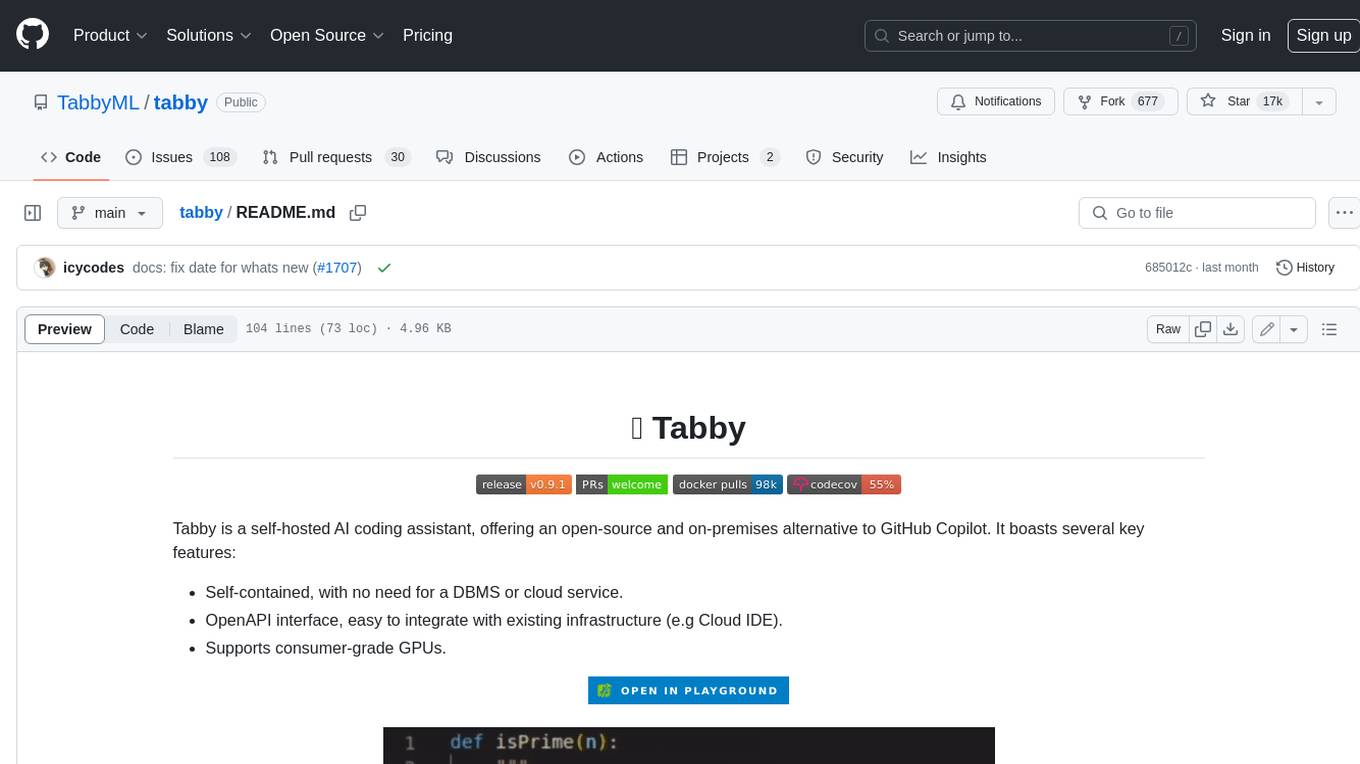
tabby
Tabby is a self-hosted AI coding assistant, offering an open-source and on-premises alternative to GitHub Copilot. It boasts several key features: * Self-contained, with no need for a DBMS or cloud service. * OpenAPI interface, easy to integrate with existing infrastructure (e.g Cloud IDE). * Supports consumer-grade GPUs.
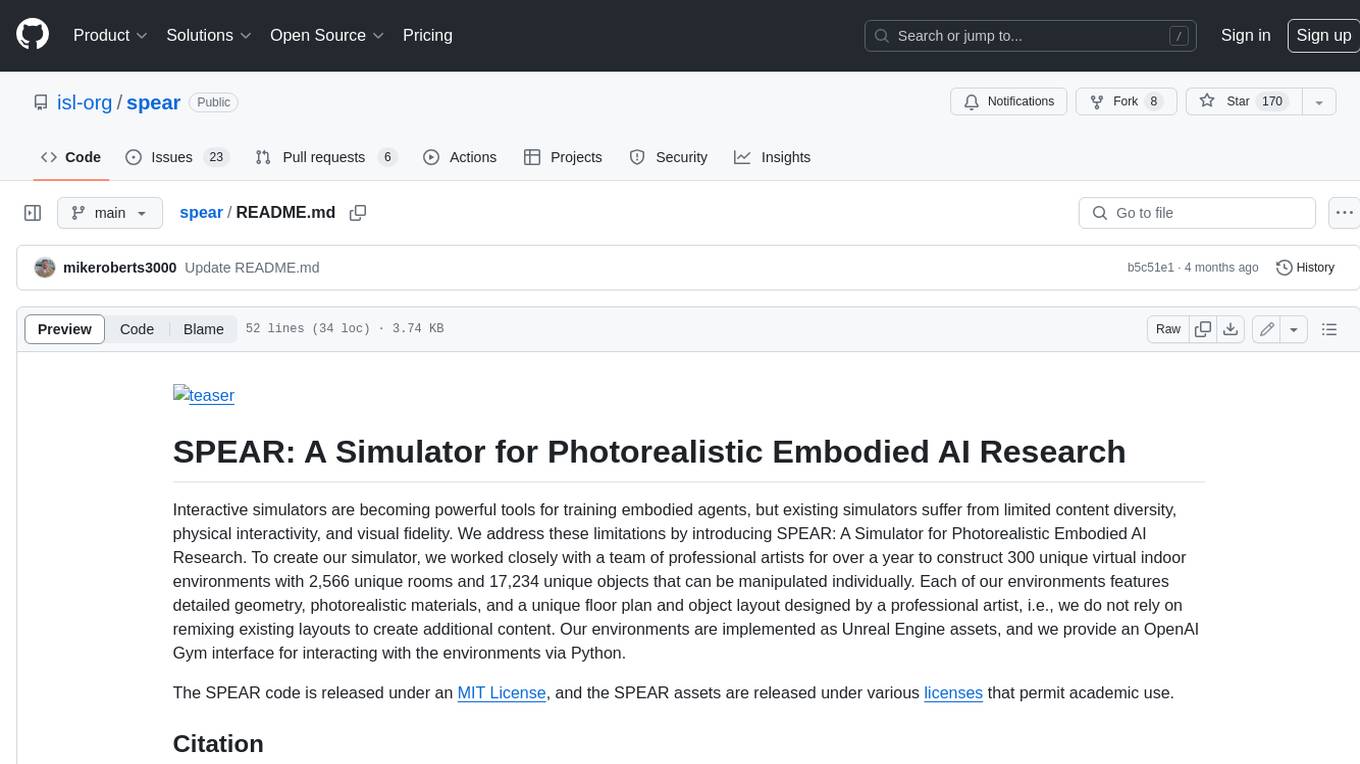
spear
SPEAR (Simulator for Photorealistic Embodied AI Research) is a powerful tool for training embodied agents. It features 300 unique virtual indoor environments with 2,566 unique rooms and 17,234 unique objects that can be manipulated individually. Each environment is designed by a professional artist and features detailed geometry, photorealistic materials, and a unique floor plan and object layout. SPEAR is implemented as Unreal Engine assets and provides an OpenAI Gym interface for interacting with the environments via Python.
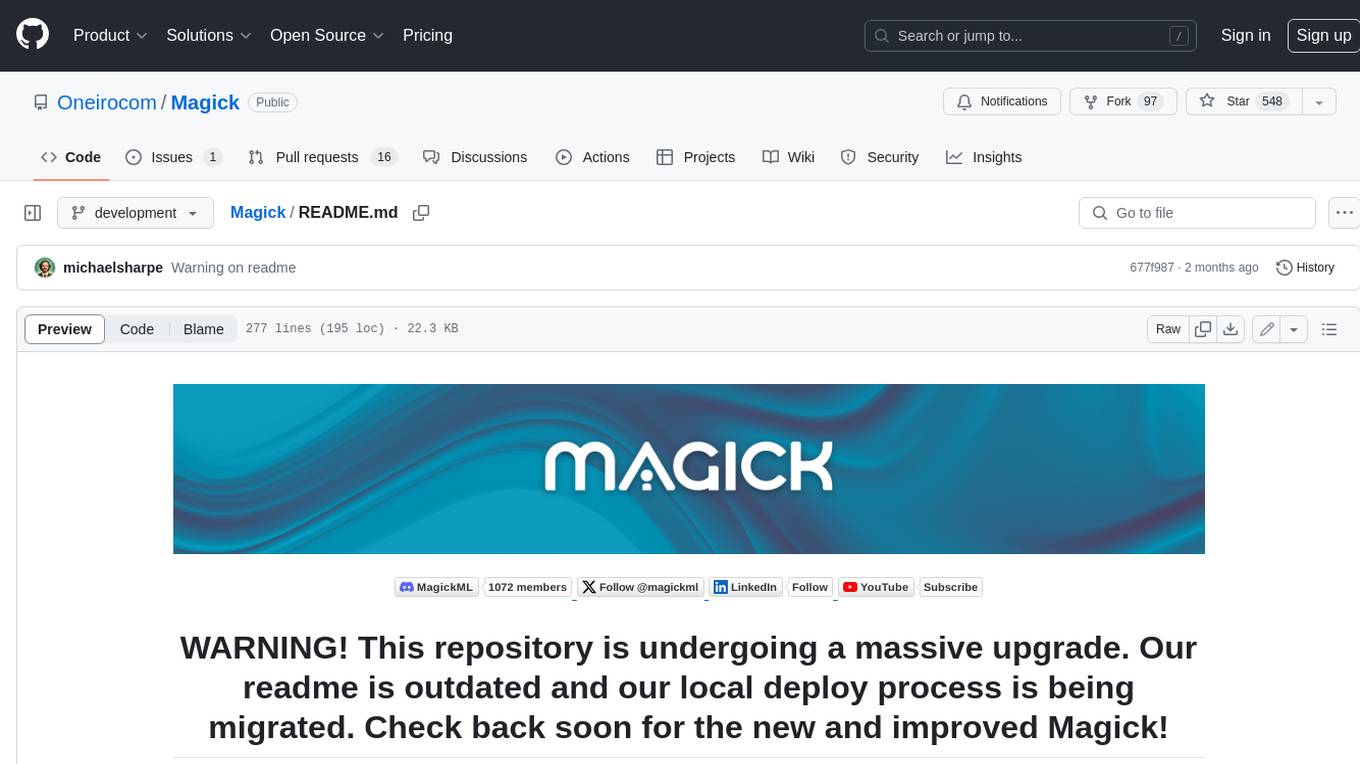
Magick
Magick is a groundbreaking visual AIDE (Artificial Intelligence Development Environment) for no-code data pipelines and multimodal agents. Magick can connect to other services and comes with nodes and templates well-suited for intelligent agents, chatbots, complex reasoning systems and realistic characters.