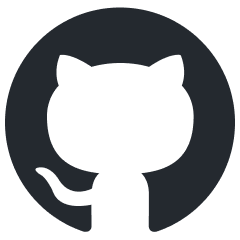
llm
A package abstracting llm capabilities for emacs.
Stars: 235
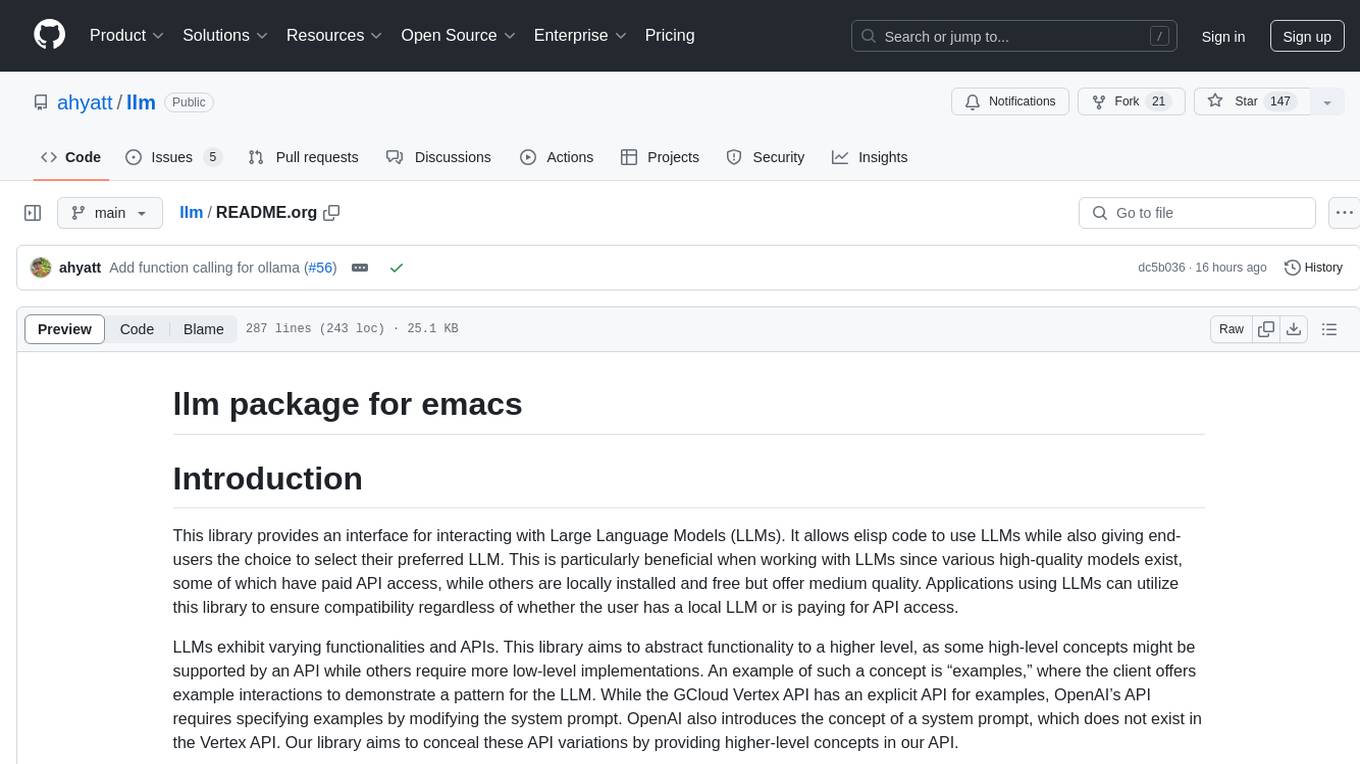
The 'llm' package for Emacs provides an interface for interacting with Large Language Models (LLMs). It abstracts functionality to a higher level, concealing API variations and ensuring compatibility with various LLMs. Users can set up providers like OpenAI, Gemini, Vertex, Claude, Ollama, GPT4All, and a fake client for testing. The package allows for chat interactions, embeddings, token counting, and function calling. It also offers advanced prompt creation and logging capabilities. Users can handle conversations, create prompts with placeholders, and contribute by creating providers.
README:
#+TITLE: llm package for emacs
- Introduction This library provides an interface for interacting with Large Language Models (LLMs). It allows elisp code to use LLMs while also giving end-users the choice to select their preferred LLM. This is particularly beneficial when working with LLMs since various high-quality models exist, some of which have paid API access, while others are locally installed and free but offer medium quality. Applications using LLMs can utilize this library to ensure compatibility regardless of whether the user has a local LLM or is paying for API access.
This library abstracts several kinds of features:
- Chat functionality: the ability to query the LLM and get a response, and continue to take turns writing to the LLM and receiving responses. The library supports both synchronous, asynchronous, and streaming responses.
- Chat with image and other kinda of media inputs are also supported, so that the user can input images and discuss them with the LLM.
- Function calling (aka "tool use") is supported, for having the LLM call elisp functions that it chooses, with arguments it provides.
- Embeddings: Send text and receive a vector that encodes the semantic meaning of the underlying text. Can be used in a search system to find similar passages.
- Prompt construction: Create a prompt to give to an LLM from one more sources of data.
Certain functionalities might not be available in some LLMs. Any such unsupported functionality will raise a 'not-implemented signal, or it may fail in some other way. Clients are recommended to check =llm-capabilities= when trying to do something beyond basic text chat.
- Setting up providers
Users of an application that uses this package should not need to install it themselves. The llm package should be installed as a dependency when you install the package that uses it. However, you do need to require the llm module and set up the provider you will be using. Typically, applications will have a variable you can set. For example, let's say there's a package called "llm-refactoring", which has a variable
llm-refactoring-provider. You would set it up like so:
#+begin_src emacs-lisp (use-package llm-refactoring :init (require 'llm-openai) (setq llm-refactoring-provider (make-llm-openai :key my-openai-key)) #+end_src
Here my-openai-key would be a variable you set up before with your OpenAI key. Or, just substitute the key itself as a string. It's important to remember never to check your key into a public repository such as GitHub, because your key must be kept private. Anyone with your key can use the API, and you will be charged.
You can also use a function as a key, so you can store your key in a secure place and retrieve it via a function. For example, you could add a line to =~/.authinfo.gpg=:
#+begin_example machine llm.openai password #+end_example
And then set up your provider like: #+begin_src emacs-lisp (setq llm-refactoring-provider (make-llm-openai :key (plist-get (car (auth-source-search :host "llm.openai")) :secret))) #+end_src
All of the providers (except for =llm-fake=), can also take default parameters that will be used if they are not specified in the prompt. These are the same parameters as appear in the prompt, but prefixed with =default-chat-=. So, for example, if you find that you like Ollama to be less creative than the default, you can create your provider like:
#+begin_src emacs-lisp (make-llm-ollama :embedding-model "mistral:latest" :chat-model "mistral:latest" :default-chat-temperature 0.1) #+end_src
For embedding users. if you store the embeddings, you must set the embedding model. Even though there's no way for the llm package to tell whether you are storing it, if the default model changes, you may find yourself storing incompatible embeddings.
** Open AI
You can set up with make-llm-openai, with the following parameters:
-
:key, the Open AI key that you get when you sign up to use Open AI's APIs. Remember to keep this private. This is non-optional. -
:chat-model: A model name from the [[https://platform.openai.com/docs/models/gpt-4][list of Open AI's model names.]] Keep in mind some of these are not available to everyone. This is optional, and will default to a reasonable model. -
:embedding-model: A model name from [[https://platform.openai.com/docs/guides/embeddings/embedding-models][list of Open AI's embedding model names.]] This is optional, and will default to a reasonable model. ** Open AI Compatible There are many Open AI compatible APIs and proxies of Open AI. You can set up one withmake-llm-openai-compatible, with the following parameter: -
:url, the URL of leading up to the command ("embeddings" or "chat/completions"). So, for example, "https://api.openai.com/v1/" is the URL to use Open AI (although if you wanted to do that, just usemake-llm-openaiinstead. ** Azure's Open AI Microsoft Azure has an Open AI integration, although it doesn't support everything Open AI does, such as function calling. You can set it up withmake-llm-azure, with the following parameter: -
:url, the endpoint URL, such as "https://docs-test-001.openai.azure.com/". -
:key, the Azure key for Azure OpenAI service. -
:chat-model, the chat model, which must be deployed in Azure. -
embedding-model, the embedding model which must be deployed in Azure. ** Gemini (not via Google Cloud) This is Google's AI model. You can get an API key via their [[https://makersuite.google.com/app/apikey][page on Google AI Studio]]. Set this up withmake-llm-gemini, with the following parameters: -
:key, the Google AI key that you get from Google AI Studio. -
:chat-model, the model name, from the [[https://ai.google.dev/models][list]] of models. This is optional and will default to the text Gemini model. -
:embedding-model: the model name, currently must be "embedding-001". This is optional and will default to "embedding-001". ** Vertex (Gemini via Google Cloud) This is mostly for those who want to use Google Cloud specifically, most users should use Gemini instead, which is easier to set up.
You can set up with make-llm-vertex, with the following parameters:
-
:project: Your project number from Google Cloud that has Vertex API enabled. -
:chat-model: A model name from the [[https://cloud.google.com/vertex-ai/docs/generative-ai/chat/chat-prompts#supported_model][list of Vertex's model names.]] This is optional, and will default to a reasonable model. -
:embedding-model: A model name from the [[https://cloud.google.com/vertex-ai/docs/generative-ai/embeddings/get-text-embeddings#supported_models][list of Vertex's embedding model names.]] This is optional, and will default to a reasonable model.
In addition to the provider, which you may want multiple of (for example, to charge against different projects), there are customizable variables:
-
llm-vertex-gcloud-binary: The binary to use for generating the API key. -
llm-vertex-gcloud-region: The gcloud region to use. It's good to set this to a region near where you are for best latency. Defaults to "us-central1".If you haven't already, you must run the following command before using this: #+begin_src sh gcloud beta services identity create --service=aiplatform.googleapis.com --project=PROJECT_ID #+end_src ** Claude [[https://docs.anthropic.com/claude/docs/intro-to-claude][Claude]] is Anthropic's large language model. It does not support embeddings. It does support function calling, but currently not in streaming. You can set it up with the following parameters:
=:key=: The API key you get from [[https://console.anthropic.com/settings/keys][Claude's settings page]]. This is required. =:chat-model=: One of the [[https://docs.anthropic.com/claude/docs/models-overview][Claude models]]. Defaults to "claude-3-opus-20240229", the most powerful model. ** Ollama [[https://ollama.ai/][Ollama]] is a way to run large language models locally. There are [[https://ollama.ai/library][many different models]] you can use with it, and some of them support function calling. You set it up with the following parameters:
-
:scheme: The scheme (http/https) for the connection to ollama. This default to "http". -
:host: The host that ollama is run on. This is optional and will default to localhost. -
:port: The port that ollama is run on. This is optional and will default to the default ollama port. -
:chat-model: The model name to use for chat. This is not optional for chat use, since there is no default. -
:embedding-model: The model name to use for embeddings (only some models can be used for embeddings. This is not optional for embedding use, since there is no default. ** GPT4All [[https://gpt4all.io/index.html][GPT4All]] is a way to run large language models locally. To use it with =llm= package, you must click "Enable API Server" in the settings. It does not offer embeddings or streaming functionality, though, so Ollama might be a better fit for users who are not already set up with local models. You can set it up with the following parameters: -
:host: The host that GPT4All is run on. This is optional and will default to localhost. -
:port: The port that GPT4All is run on. This is optional and will default to the default ollama port. -
:chat-model: The model name to use for chat. This is not optional for chat use, since there is no default. ** llama.cpp [[https://github.com/ggerganov/llama.cpp][llama.cpp]] is a way to run large language models locally. To use it with the =llm= package, you need to start the server (with the "--embedding" flag if you plan on using embeddings). The server must be started with a model, so it is not possible to switch models until the server is restarted to use the new model. As such, model is not a parameter to the provider, since the model choice is already set once the server starts.
There is a deprecated provider, however it is no longer needed. Instead, llama cpp is Open AI compatible, so the Open AI Compatible provider should work. ** Fake This is a client that makes no call, but it just there for testing and debugging. Mostly this is of use to programmatic clients of the llm package, but end users can also use it to understand what will be sent to the LLMs. It has the following parameters:
-
:output-to-buffer: if non-nil, the buffer or buffer name to append the request sent to the LLM to. -
:chat-action-func: a function that will be called to provide a string or symbol and message cons which are used to raise an error. -
:embedding-action-func: a function that will be called to provide a vector or symbol and message cons which are used to raise an error.
- Models
When picking a chat or embedding model, anything can be used, as long as the service thinks it is valid. However, models vary on context size and capabilities. The =llm-prompt= module, and any client, can depend on the context size of the model via
llm-chat-token-limit. Similarly, some models have different capabilities, exposed inllm-capabilities. The =llm-models= module defines a list of popular models, but this isn't a comprehensive list. If you want to add a model, it is fairly easy to do, for example here is adding the Mistral model (which is already included, though):
#+begin_src emacs-lisp (require 'llm-models) (llm-models-add :name "Mistral" :symbol 'mistral :capabilities '(generation tool-use free-software) :context-length 8192 :regex "mistral")) #+end_src
The =:regex= needs to uniquely identify the model passed in from a provider's chat or embedding model.
Once this is done, the model will be recognized to have the given context length and capabilities.
- =llm= and the use of non-free LLMs
The =llm= package is part of GNU Emacs by being part of GNU ELPA. Unfortunately, the most popular LLMs in use are non-free, which is not what GNU software should be promoting by inclusion. On the other hand, by use of the =llm= package, the user can make sure that any client that codes against it will work with free models that come along. It's likely that sophisticated free LLMs will, emerge, although it's unclear right now what free software means with respect to LLMs. Because of this tradeoff, we have decided to warn the user when using non-free LLMs (which is every LLM supported right now except the fake one). You can turn this off the same way you turn off any other warning, by clicking on the left arrow next to the warning when it comes up. Alternatively, you can set
llm-warn-on-nonfreetonil. This can be set via customization as well.
To build upon the example from before: #+begin_src emacs-lisp (use-package llm-refactoring :init (require 'llm-openai) (setq llm-refactoring-provider (make-llm-openai :key my-openai-key) llm-warn-on-nonfree nil) #+end_src
- Programmatic use
Client applications should require the =llm= package, and code against it. Most functions are generic, and take a struct representing a provider as the first argument. The client code, or the user themselves can then require the specific module, such as =llm-openai=, and create a provider with a function such as
(make-llm-openai :key user-api-key). The client application will use this provider to call all the generic functions.
For all callbacks, the callback will be executed in the buffer the function was first called from. If the buffer has been killed, it will be executed in a temporary buffer instead. ** Main functions
-
llm-chat provider prompt: With user-chosenprovider, and allm-chat-promptstructure (created byllm-make-chat-prompt), send that prompt to the LLM and wait for the string output. -
llm-chat-async provider prompt response-callback error-callback: Same asllm-chat, but executes in the background. Takes aresponse-callbackwhich will be called with the text response. Theerror-callbackwill be called in case of error, with the error symbol and an error message. -
llm-chat-streaming provider prompt partial-callback response-callback error-callback: Similar tollm-chat-async, but request a streaming response. As the response is built up,partial-callbackis called with the all the text retrieved up to the current point. Finally,reponse-callbackis called with the complete text. -
llm-embedding provider string: With the user-chosenprovider, send a string and get an embedding, which is a large vector of floating point values. The embedding represents the semantic meaning of the string, and the vector can be compared against other vectors, where smaller distances between the vectors represent greater semantic similarity. -
llm-embedding-async provider string vector-callback error-callback: Same asllm-embeddingbut this is processed asynchronously.vector-callbackis called with the vector embedding, and, in case of error,error-callbackis called with the same arguments as inllm-chat-async. -
llm-batch-embedding provider strings: same asllm-embedding, but takes in a list of strings, and returns a list of vectors whose order corresponds to the ordering of the strings. -
llm-batch-embedding-async provider strings vectors-callback error-callback: same asllm-embedding-async, but takes in a list of strings, and returns a list of vectors whose order corresponds to the ordering of the strings. -
llm-count-tokens provider string: Count how many tokens are instring. This may vary byprovider, because some provideres implement an API for this, but typically is always about the same. This gives an estimate if the provider has no API support. -
llm-cancel-request requestCancels the given request, if possible. Therequestobject is the return value of async and streaming functions. -
llm-name provider. Provides a short name of the model or provider, suitable for showing to users. -
llm-chat-token-limit. Gets the token limit for the chat model. This isn't possible for some backends like =llama.cpp=, in which the model isn't selected or known by this library.And the following helper functions:
-
llm-make-chat-prompt text &keys context examples functions temperature max-tokens response-format non-standard-params: This is how you make prompts.textcan be a string (the user input to the llm chatbot), or a list representing a series of back-and-forth exchanges, of odd number, with the last element of the list representing the user's latest input. This supports inputting context (also commonly called a system prompt, although it isn't guaranteed to replace the actual system prompt), examples, and other important elements, all detailed in the docstring for this function.response-formatcan be'json, to force JSON output, or a JSON schema (see below) but the prompt also needs to mention and ideally go into detail about what kind of JSON response is desired. Providers with thejson-responsecapability support JSON output, and it will be ignored if unsupported. Thenon-standard-paramslet you specify other options that might vary per-provider, and for this, the correctness is up to the client. -
llm-chat-prompt-to-text prompt: From a prompt, return a string representation. This is not usually suitable for passing to LLMs, but for debugging purposes. -
llm-chat-streaming-to-point provider prompt buffer point finish-callback: Same basic arguments asllm-chat-streaming, but will stream topointinbuffer. -
llm-chat-prompt-append-response prompt response role: Append a new response (from the user, usually) to the prompt. Theroleis optional, and defaults to'user. *** JSON schema By using theresponse-formatargument tollm-make-chat-prompt, you can ask the LLM to return items according to a specified JSON schema, based on the [[https://json-schema.org][JSON Schema Spec]]. Not everything is supported, but the most commonly used parts are. To specify the JSON schema, we use a plist-based approach. JSON objects are defined with(:type object :properties (: : ... : ) :required ( ... )). Arrays are defined with(:type array :items ). Enums are defined with(:enum ( )). You can also request integers, strings, and other types defined by the JSON Schema Spec, by just having(:type ). Typically, LLMs often require the top-level schema object to be an object, and often that all properties on the top-level object must be required.
-
Some examples: #+begin_src emacs-lisp (llm-chat my-provider (llm-make-chat-prompt "How many countries are there? Return the result as JSON." :response-format '(:type object :properties (:num (:type integer)) :required (num)))) #+end_src
#+RESULTS: : {"num":195}
#+begin_src emacs-lisp (llm-chat my-provider (llm-make-chat-prompt "Which editor is hard to quit? Return the result as JSON." :response-format '(:type object :properties (:editor (:enum ("emacs" "vi" "vscode")) :authors (:type array :items (:type string))) :required (editor authors)))) #+end_src
#+RESULTS: : {"editor":"vi","authors":["Bram Moolenaar","Bill Joy"]}
** Logging
Interactions with the =llm= package can be logged by setting llm-log to a non-nil value. This should be done only when developing. The log can be found in the =llm log= buffer.
** How to handle conversations
Conversations can take place by repeatedly calling llm-chat and its variants. The prompt should be constructed with llm-make-chat-prompt. For a conversation, the entire prompt must be kept as a variable, because the llm-chat-prompt-interactions slot will be getting changed by the chat functions to store the conversation. For some providers, this will store the history directly in llm-chat-prompt-interactions, but other LLMs have an opaque conversation history. For that reason, the correct way to handle a conversation is to repeatedly call llm-chat or variants with the same prompt structure, kept in a variable, and after each time, add the new user text with llm-chat-prompt-append-response. The following is an example:
#+begin_src emacs-lisp
(defvar-local llm-chat-streaming-prompt nil)
(defun start-or-continue-conversation (text)
"Called when the user has input TEXT as the next input."
(if llm-chat-streaming-prompt
(llm-chat-prompt-append-response llm-chat-streaming-prompt text)
(setq llm-chat-streaming-prompt (llm-make-chat-prompt text))
(llm-chat-streaming-to-point provider llm-chat-streaming-prompt (current-buffer) (point-max) (lambda ()))))
#+end_src
** Caution about llm-chat-prompt-interactions
The interactions in a prompt may be modified by conversation or by the conversion of the context and examples to what the LLM understands. Different providers require different things from the interactions. Some can handle system prompts, some cannot. Some require alternating user and assistant chat interactions, others can handle anything. It's important that clients keep to behaviors that work on all providers. Do not attempt to read or manipulate llm-chat-prompt-interactions after initially setting it up for the first time, because you are likely to make changes that only work for some providers. Similarly, don't directly create a prompt with make-llm-chat-prompt, because it is easy to create something that wouldn't work for all providers.
** Function calling
Note: function calling functionality is currently beta quality. If you want to use function calling, please watch the =llm= [[https://github.com/ahyatt/llm/discussions][discussions]] for any announcements about changes.
Function calling is a way to give the LLM a list of functions it can call, and have it call the functions for you. The standard interaction has the following steps:
- The client sends the LLM a prompt with functions it can call.
- The LLM may return which functions to execute, and with what arguments, or text as normal.
- If the LLM has decided to call one or more functions, those functions should be called, and their results sent back to the LLM.
- The LLM will return with a text response based on the initial prompt and the results of the function calling.
- The client can now can continue the conversation.
This basic structure is useful because it can guarantee a well-structured output (if the LLM does decide to call the function). Not every LLM can handle function calling, and those that do not will ignore the functions entirely. The function =llm-capabilities= will return a list with =function-calls= in it if the LLM supports function calls. Right now only Gemini, Vertex, Claude, and Open AI support function calling. Ollama should get function calling soon. However, even for LLMs that handle function calling, there is a fair bit of difference in the capabilities. Right now, it is possible to write function calls that succeed in Open AI but cause errors in Gemini, because Gemini does not appear to handle functions that have types that contain other types. So client programs are advised for right now to keep function to simple types.
The way to call functions is to attach a list of functions to the =llm-function-call= slot in the prompt. This is a list of =llm-function-call= structs, which takes a function, a name, a description, and a list of =llm-function-arg= structs. The docstrings give an explanation of the format.
The various chat APIs will execute the functions defined in =llm-function-call= with the arguments supplied by the LLM. Instead of returning (or passing to a callback) a string, instead an alist will be returned of function names and return values.
After sending a function call, the client could use the result, but if you want to proceed with the conversation, or get a textual response that accompany the function you should just send the prompt back with no modifications. This is because the LLM gives the function call to make as a response, and then expects to get back the results of that function call. The results were already executed at the end of the previous call, which also stores the result of that execution in the prompt. This is why it should be sent back without further modifications.
Be aware that there is no gaurantee that the function will be called correctly. While the LLMs mostly get this right, they are trained on Javascript functions, so imitating Javascript names is recommended. So, "write_email" is a better name for a function than "write-email".
Examples can be found in =llm-tester=. There is also a function call to generate function calls from existing elisp functions in =utilities/elisp-to-function-call.el=. ** Media input Note: media input functionality is currently alpha quality. If you want to use it, please watch the =llm= [[https://github.com/ahyatt/llm/discussions][discussions]] for any announcements about changes.
Media can be used in =llm-chat= and related functions. To use media, you can use =llm-multipart= in =llm-make-chat-prompt=, and pass it an Emacs image or an =llm-media= object for other kinds of media. Besides images, some models support video and audio. Not all providers or models support these, with images being the most frequently supported media type, and video and audio more rare. ** Advanced prompt creation The =llm-prompt= module provides helper functions to create prompts that can incorporate data from your application. In particular, this should be very useful for application that need a lot of context.
A prompt defined with =llm-prompt= is a template, with placeholders that the module will fill in. Here's an example of a prompt definition, from the [[https://github.com/ahyatt/ekg][ekg]] package:
#+begin_src emacs-lisp (llm-defprompt ekg-llm-fill-prompt "The user has written a note, and would like you to append to it, to make it more useful. This is important: only output your additions, and do not repeat anything in the user's note. Write as a third party adding information to a note, so do not use the first person.
First, I'll give you information about the note, then similar other notes that user has written, in JSON. Finally, I'll give you instructions. The user's note will be your input, all the rest, including this, is just context for it. The notes given are to be used as background material, which can be referenced in your answer.
The user's note uses tags: {{tags}}. The notes with the same tags, listed here in reverse date order: {{tag-notes:10}}
These are similar notes in general, which may have duplicates from the ones above: {{similar-notes:1}}
This ends the section on useful notes as a background for the note in question.
Your instructions on what content to add to the note:
{{instructions}} ") #+end_src
When this is filled, it is done in the context of a provider, which has a known
context size (via llm-chat-token-limit). Care is taken to not overfill the
context, which is checked as it is filled via llm-count-tokens. We usually want
to not fill the whole context, but instead leave room for the chat and
subsequent terms. The variable llm-prompt-default-max-pct controls how much of
the context window we want to fill. The way we estimate the number of tokens
used is quick but inaccurate, so limiting to less than the maximum context size
is useful for guarding against a miscount leading to an error calling the LLM
due to too many tokens. If you want to have a hard limit as well that doesn't
depend on the context window size, you can use llm-prompt-default-max-tokens.
We will use the minimum of either value.
Variables are enclosed in double curly braces, like this: ={{instructions}}=. They can just be the variable, or they can also denote a number of tickets, like so: ={{tag-notes:10}}=. Tickets should be thought of like lottery tickets, where the prize is a single round of context filling for the variable. So the variable =tag-notes= gets 10 tickets for a drawing. Anything else where tickets are unspecified (unless it is just a single variable, which will be explained below) will get a number of tickets equal to the total number of specified tickets. So if you have two variables, one with 1 ticket, one with 10 tickets, one will be filled 10 times more than the other. If you have two variables, one with 1 ticket, one unspecified, the unspecified one will get 1 ticket, so each will have an even change to get filled. If no variable has tickets specified, each will get an equal chance. If you have one variable, it could have any number of tickets, but the result would be the same, since it would win every round. This algorithm is the contribution of David Petrou.
The above is true of variables that are to be filled with a sequence of possible values. A lot of LLM context filling is like this. In the above example, ={{similar-notes}}= is a retrieval based on a similarity score. It will continue to fill items from most similar to least similar, which is going to return almost everything the ekg app stores. We want to retrieve only as needed. Because of this, the =llm-prompt= module takes in /generators/ to supply each variable. However, a plain list is also acceptable, as is a single value. Any single value will not enter into the ticket system, but rather be prefilled before any tickets are used.
Values supplied in either the list or generators can be the values themselves, or conses. If a cons, the variable to fill is the =car= of the cons, and the =cdr= is the place to fill the new value, =front= or =back=. The =front= is the default: new values will be appended to the end. =back= will add new values to the start of the filled text for the variable instead.
So, to illustrate with this example, here's how the prompt will be filled:
- First, the ={{tags}}= and ={{instructions}}= will be filled first. This will happen regardless before we check the context size, so the module assumes that these will be small and not blow up the context.
- Check the context size we want to use (
llm-prompt-default-max-pctmultiplied byllm-chat-token-limit) and exit if exceeded. - Run a lottery with all tickets and choose one of the remaining variables to fill.
- If the variable won't make the text too large, fill the variable with one entry retrieved from a supplied generator, otherwise ignore. These are values are not conses, so values will be appended to the end of the generated text for each variable (so a new variable generated for tags will append after other generated tags but before the subsequent "and" in the text.
- Goto 2
The prompt can be filled two ways, one using predefined prompt template
(llm-defprompt and llm-prompt-fill), the other using a prompt template that is
passed in (llm-prompt-fill-text).
#+begin_src emacs-lisp (llm-defprompt my-prompt "My name is {{name}} and I'm here's to say {{messages}}")
(llm-prompt-fill 'my-prompt my-llm-provider :name "Pat" :messages #'my-message-retriever)
(iter-defun my-message-retriever () "Return the messages I like to say." (my-message-reset-messages) (while (my-has-next-message) (iter-yield (my-get-next-message)))) #+end_src
Alternatively, you can just fill it directly: #+begin_src emacs-lisp (llm-prompt-fill-text "Hi, I'm {{name}} and I'm here to say {{messages}}" :name "John" :messages #'my-message-retriever) #+end_src
As you can see in the examples, the variable values are passed in with matching keys.
- Contributions If you are interested in creating a provider, please send a pull request, or open a bug. This library is part of GNU ELPA, so any major provider that we include in this module needs to be written by someone with FSF papers. However, you can always write a module and put it on a different package archive, such as MELPA.
For Tasks:
Click tags to check more tools for each tasksFor Jobs:
Alternative AI tools for llm
Similar Open Source Tools
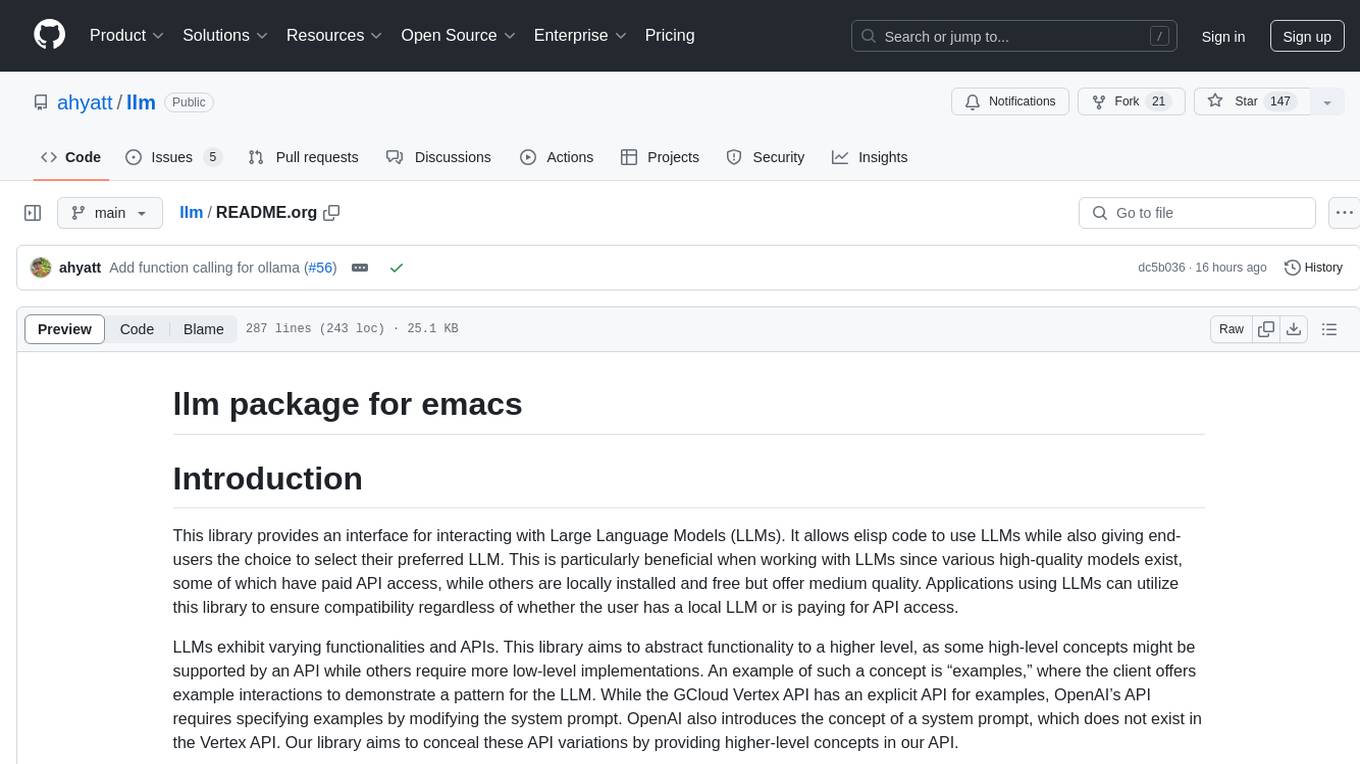
llm
The 'llm' package for Emacs provides an interface for interacting with Large Language Models (LLMs). It abstracts functionality to a higher level, concealing API variations and ensuring compatibility with various LLMs. Users can set up providers like OpenAI, Gemini, Vertex, Claude, Ollama, GPT4All, and a fake client for testing. The package allows for chat interactions, embeddings, token counting, and function calling. It also offers advanced prompt creation and logging capabilities. Users can handle conversations, create prompts with placeholders, and contribute by creating providers.
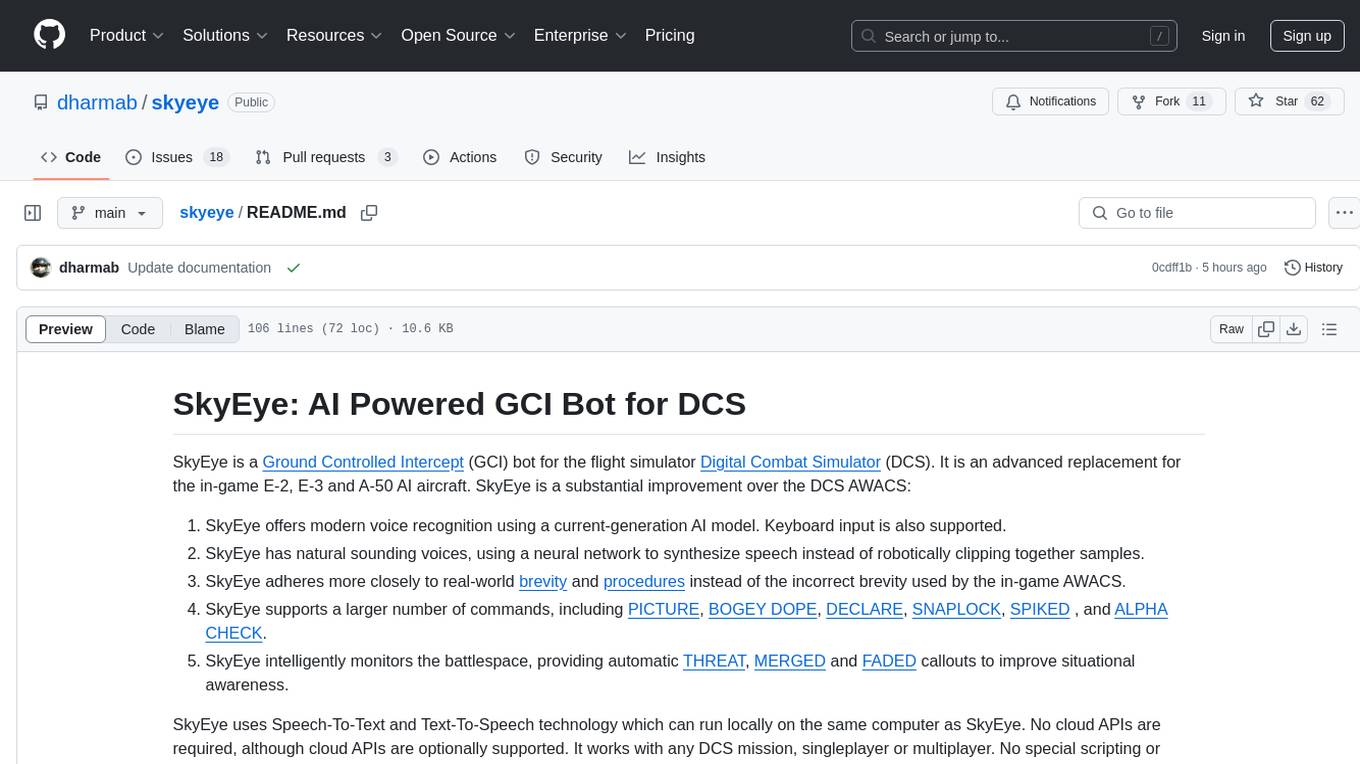
skyeye
SkyEye is an AI-powered Ground Controlled Intercept (GCI) bot designed for the flight simulator Digital Combat Simulator (DCS). It serves as an advanced replacement for the in-game E-2, E-3, and A-50 AI aircraft, offering modern voice recognition, natural-sounding voices, real-world brevity and procedures, a wide range of commands, and intelligent battlespace monitoring. The tool uses Speech-To-Text and Text-To-Speech technology, can run locally or on a cloud server, and is production-ready software used by various DCS communities.
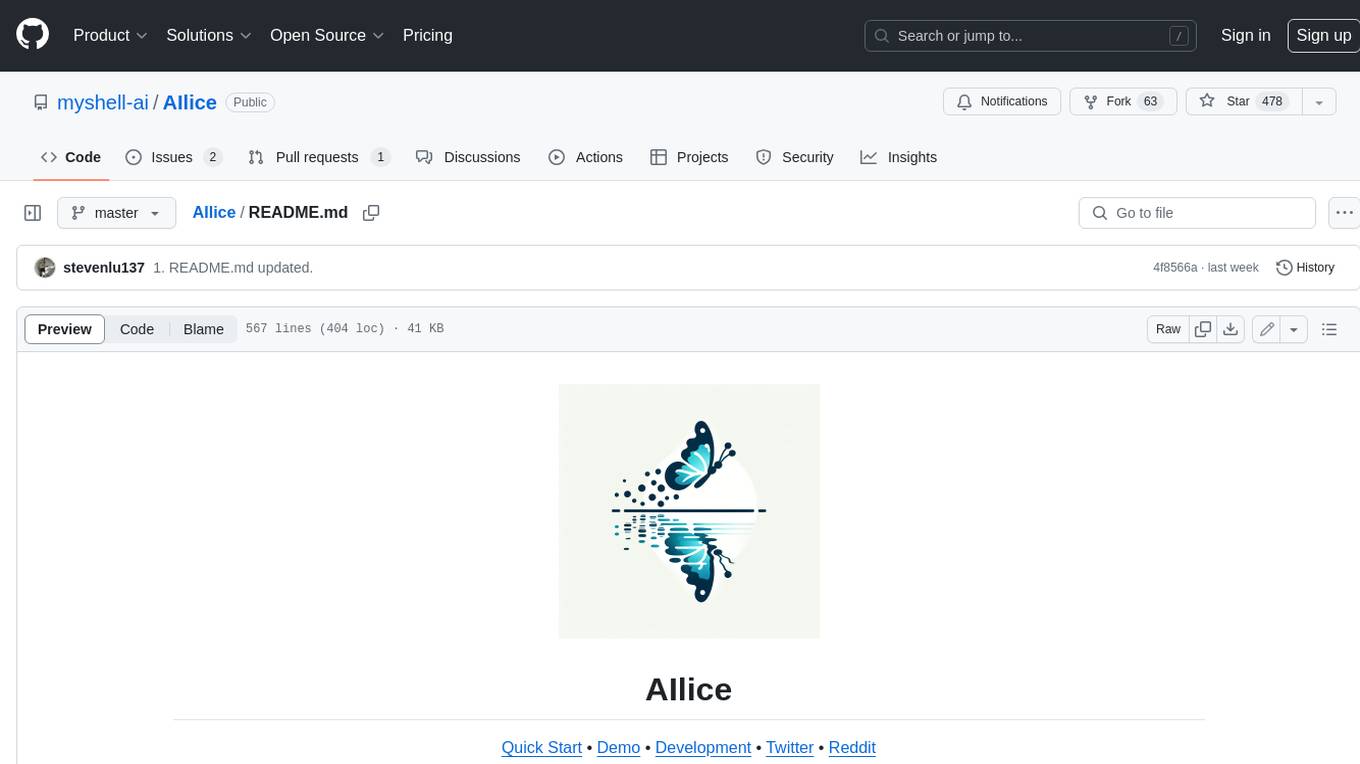
AIlice
AIlice is a fully autonomous, general-purpose AI agent that aims to create a standalone artificial intelligence assistant, similar to JARVIS, based on the open-source LLM. AIlice achieves this goal by building a "text computer" that uses a Large Language Model (LLM) as its core processor. Currently, AIlice demonstrates proficiency in a range of tasks, including thematic research, coding, system management, literature reviews, and complex hybrid tasks that go beyond these basic capabilities. AIlice has reached near-perfect performance in everyday tasks using GPT-4 and is making strides towards practical application with the latest open-source models. We will ultimately achieve self-evolution of AI agents. That is, AI agents will autonomously build their own feature expansions and new types of agents, unleashing LLM's knowledge and reasoning capabilities into the real world seamlessly.
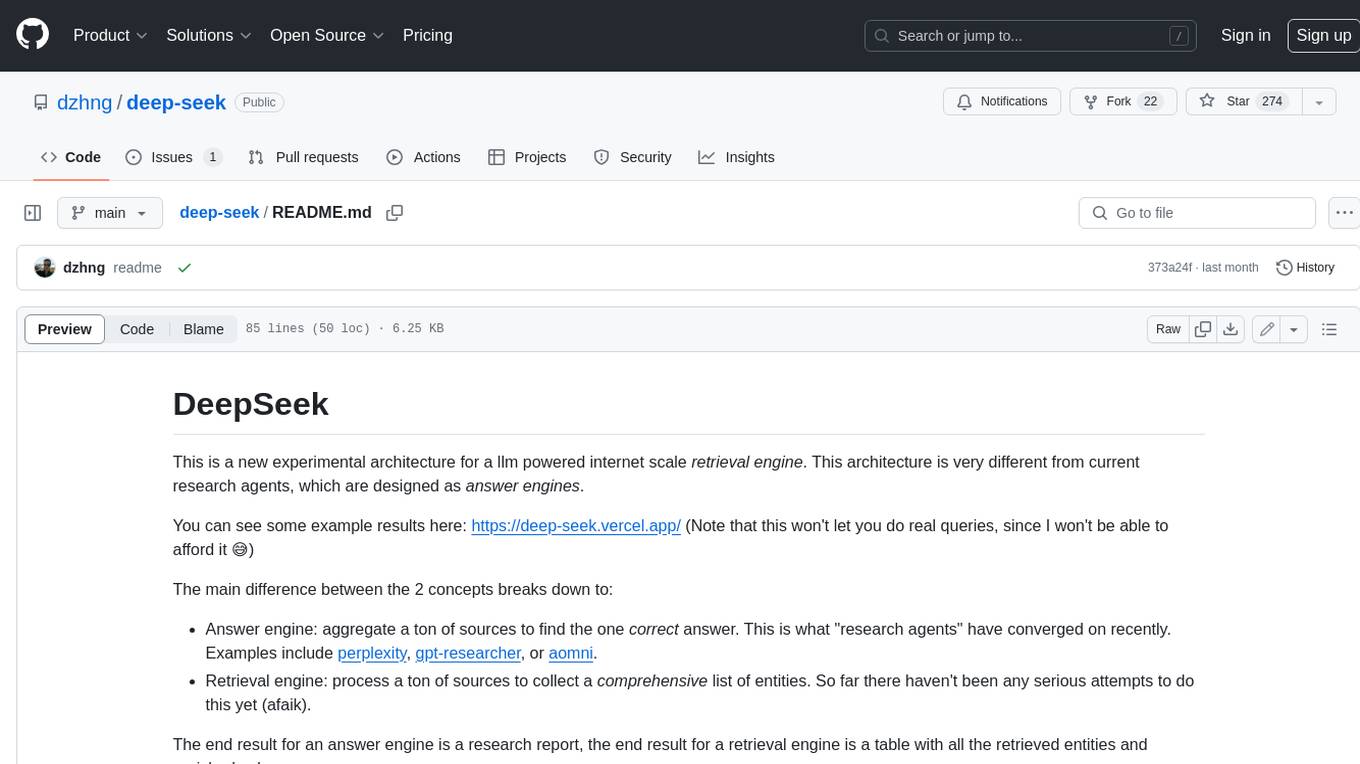
deep-seek
DeepSeek is a new experimental architecture for a large language model (LLM) powered internet-scale retrieval engine. Unlike current research agents designed as answer engines, DeepSeek aims to process a vast amount of sources to collect a comprehensive list of entities and enrich them with additional relevant data. The end result is a table with retrieved entities and enriched columns, providing a comprehensive overview of the topic. DeepSeek utilizes both standard keyword search and neural search to find relevant content, and employs an LLM to extract specific entities and their associated contents. It also includes a smaller answer agent to enrich the retrieved data, ensuring thoroughness. DeepSeek has the potential to revolutionize research and information gathering by providing a comprehensive and structured way to access information from the vastness of the internet.
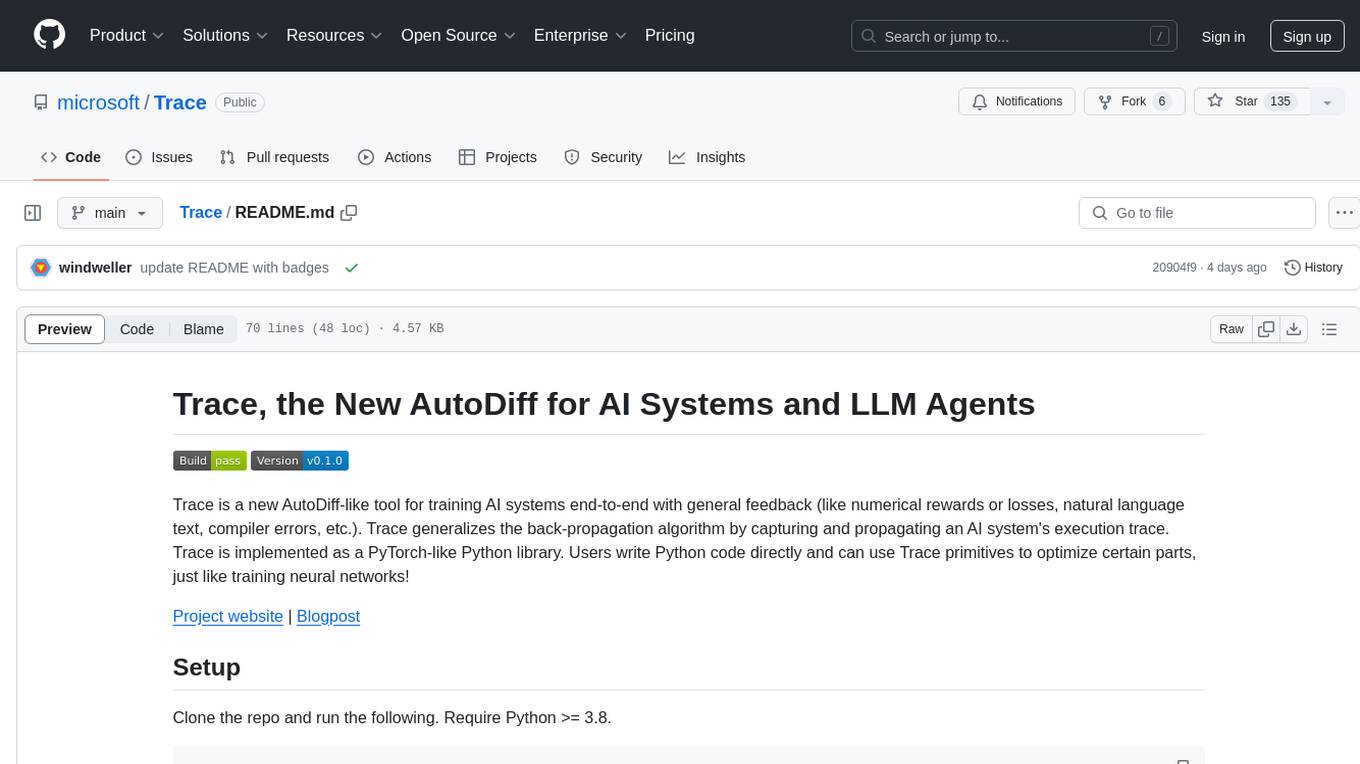
Trace
Trace is a new AutoDiff-like tool for training AI systems end-to-end with general feedback. It generalizes the back-propagation algorithm by capturing and propagating an AI system's execution trace. Implemented as a PyTorch-like Python library, users can write Python code directly and use Trace primitives to optimize certain parts, similar to training neural networks.
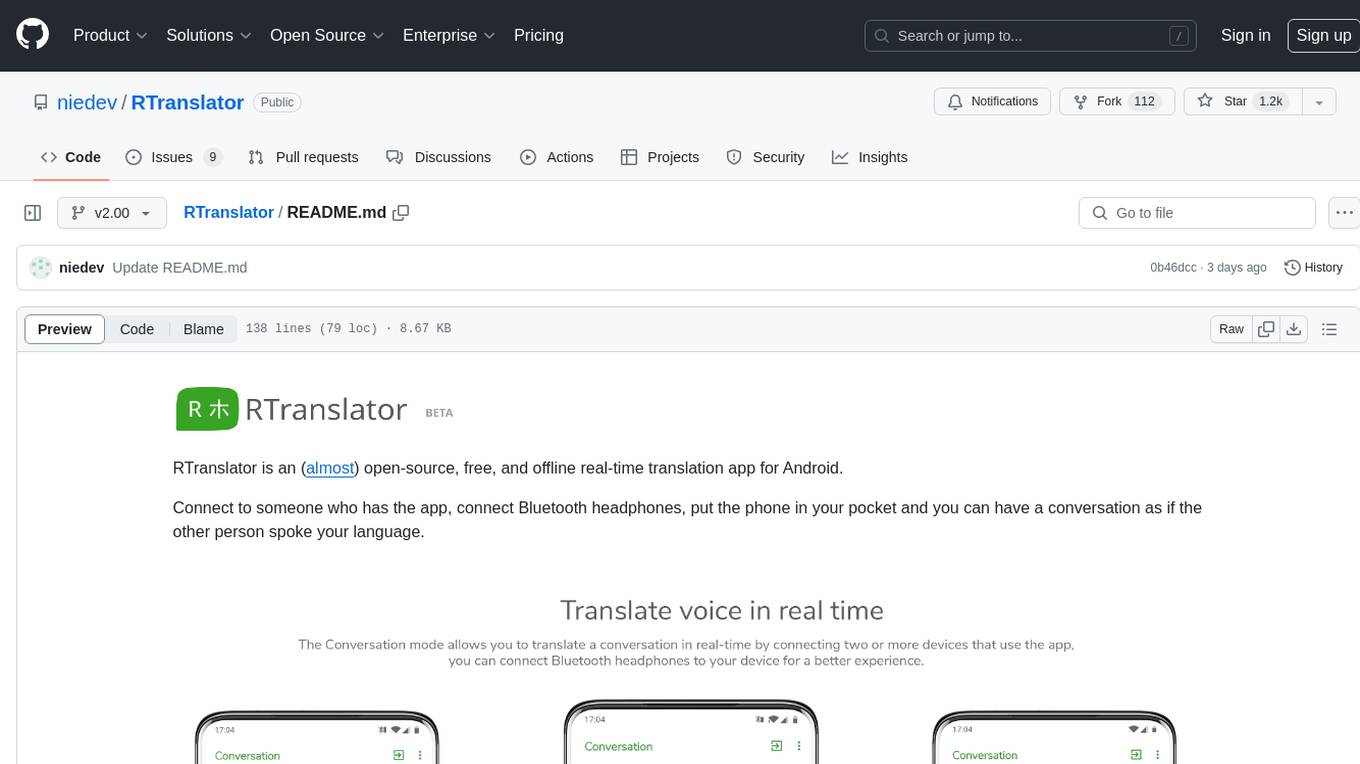
RTranslator
RTranslator is an almost open-source, free, and offline real-time translation app for Android. It offers Conversation mode for multi-user translations, WalkieTalkie mode for quick conversations, and Text translation mode. It uses Meta's NLLB for translation and OpenAi's Whisper for speech recognition, ensuring privacy. The app is optimized for performance and supports multiple languages. It is ad-free and donation-supported.
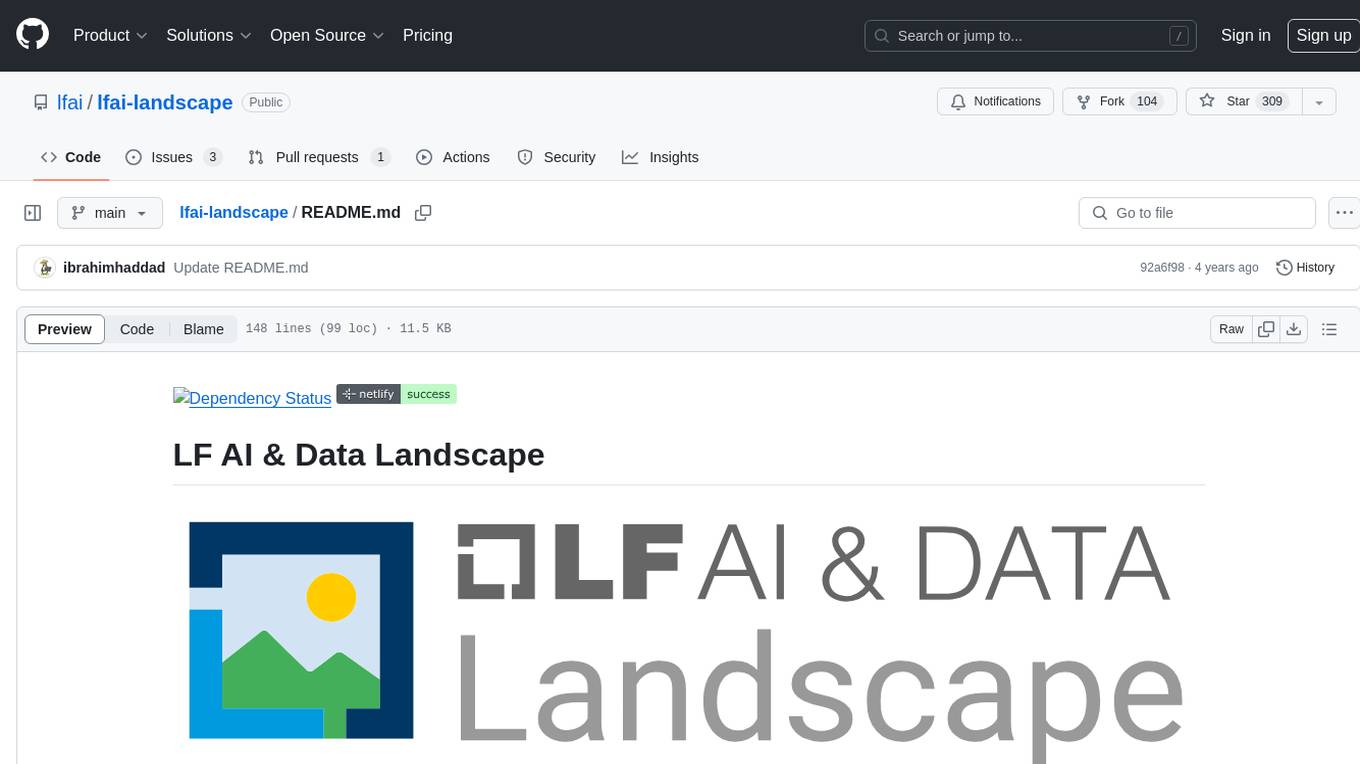
lfai-landscape
LF AI & Data Landscape is a map to explore open source projects in the AI & Data domains, highlighting companies that are members of LF AI & Data. It showcases members of the Foundation and is modelled after the Cloud Native Computing Foundation landscape. The landscape includes current version, interactive version, new entries, logos, proper SVGs, corrections, external data, best practices badge, non-updated items, license, formats, installation, vulnerability reporting, and adjusting the landscape view.
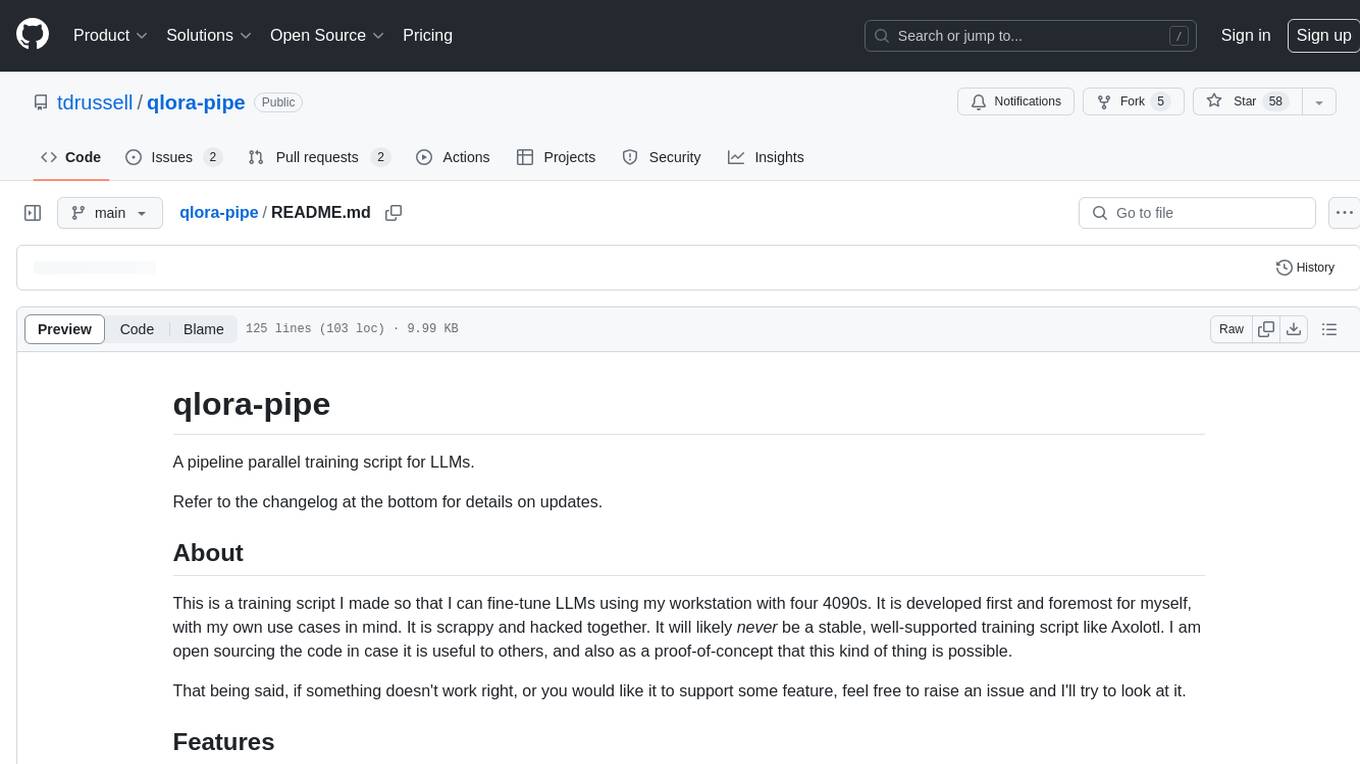
qlora-pipe
qlora-pipe is a pipeline parallel training script designed for efficiently training large language models that cannot fit on one GPU. It supports QLoRA, LoRA, and full fine-tuning, with efficient model loading and the ability to load any dataset that Axolotl can handle. The script allows for raw text training, resuming training from a checkpoint, logging metrics to Tensorboard, specifying a separate evaluation dataset, training on multiple datasets simultaneously, and supports various models like Llama, Mistral, Mixtral, Qwen-1.5, and Cohere (Command R). It handles pipeline- and data-parallelism using Deepspeed, enabling users to set the number of GPUs, pipeline stages, and gradient accumulation steps for optimal utilization.
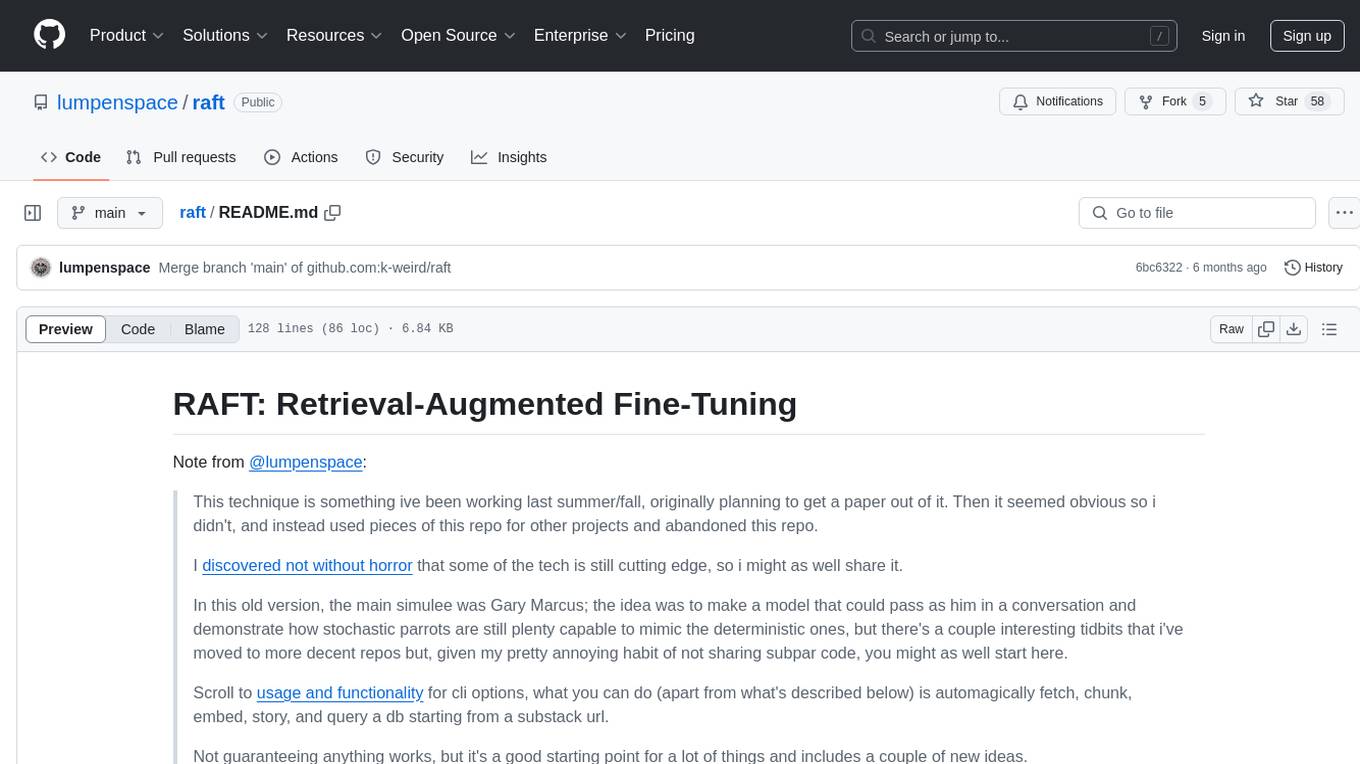
raft
RAFT (Retrieval-Augmented Fine-Tuning) is a method for creating conversational agents that realistically emulate specific human targets. It involves a dual-phase process of fine-tuning and retrieval-based augmentation to generate nuanced and personalized dialogue. The tool is designed to combine interview transcripts with memories from past writings to enhance language model responses. RAFT has the potential to advance the field of personalized, context-sensitive conversational agents.
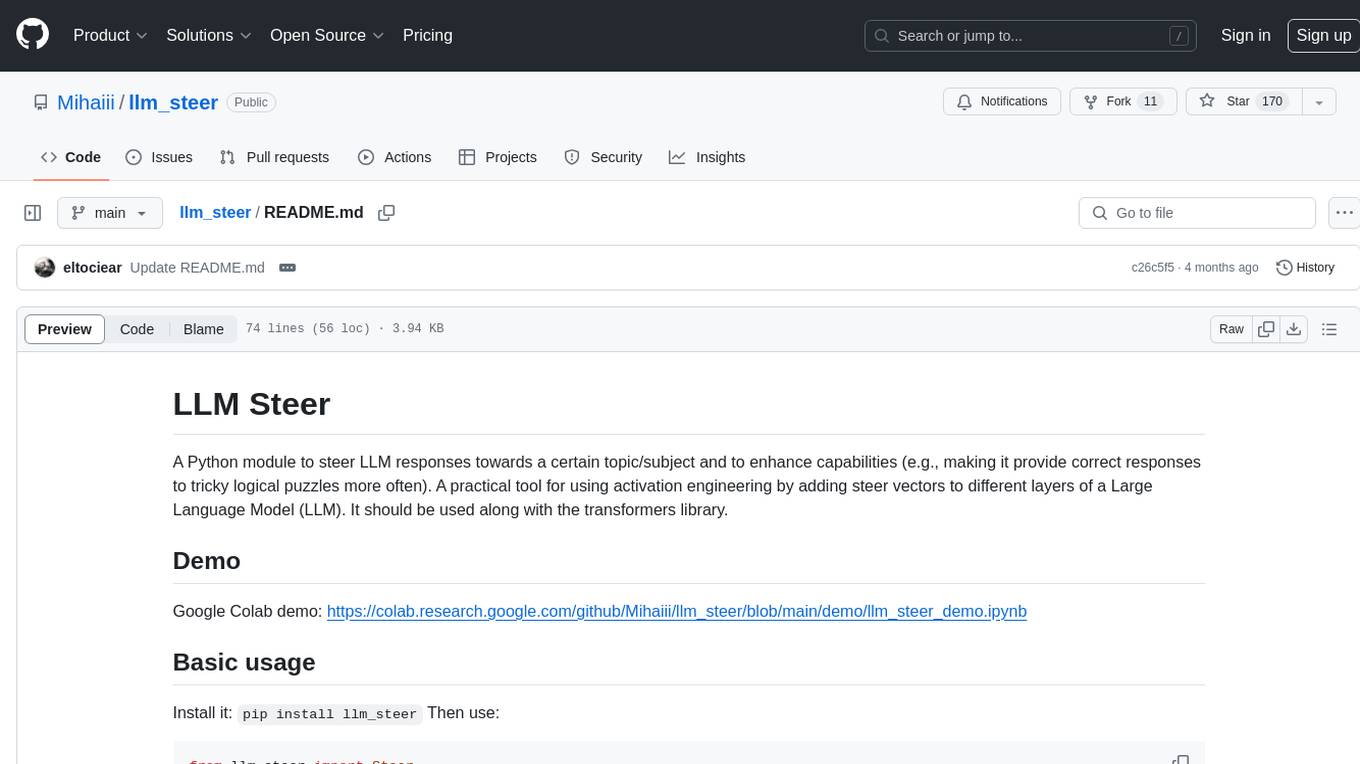
llm_steer
LLM Steer is a Python module designed to steer Large Language Models (LLMs) towards specific topics or subjects by adding steer vectors to different layers of the model. It enhances the model's capabilities, such as providing correct responses to logical puzzles. The tool should be used in conjunction with the transformers library. Users can add steering vectors to specific layers of the model with coefficients and text, retrieve applied steering vectors, and reset all steering vectors to the initial model. Advanced usage involves changing default parameters, but it may lead to the model outputting gibberish in most cases. The tool is meant for experimentation and can be used to enhance role-play characteristics in LLMs.
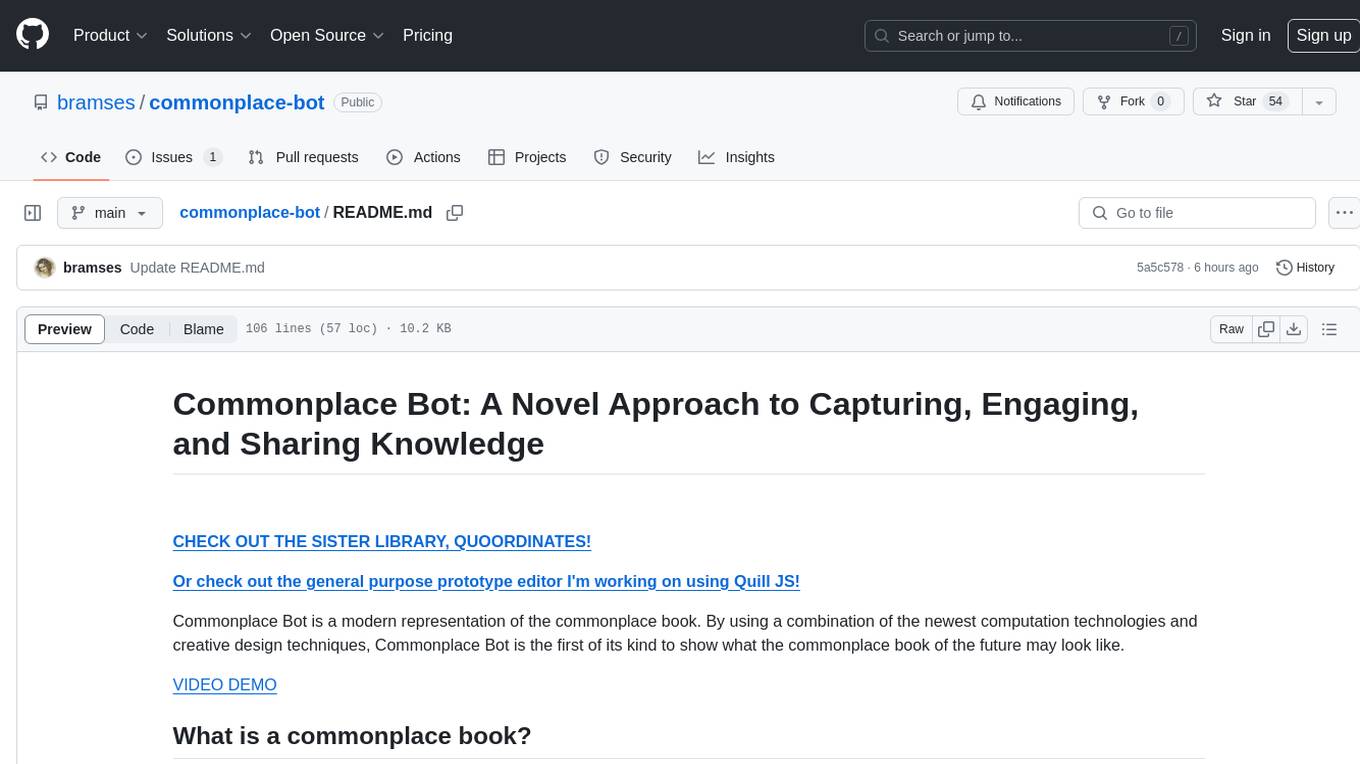
commonplace-bot
Commonplace Bot is a modern representation of the commonplace book, leveraging modern technological advancements in computation, data storage, machine learning, and networking. It aims to capture, engage, and share knowledge by providing a platform for users to collect ideas, quotes, and information, organize them efficiently, engage with the data through various strategies and triggers, and transform the data into new mediums for sharing. The tool utilizes embeddings and cached transformations for efficient data storage and retrieval, flips traditional engagement rules by engaging with the user, and enables users to alchemize raw data into new forms like art prompts. Commonplace Bot offers a unique approach to knowledge management and creative expression.
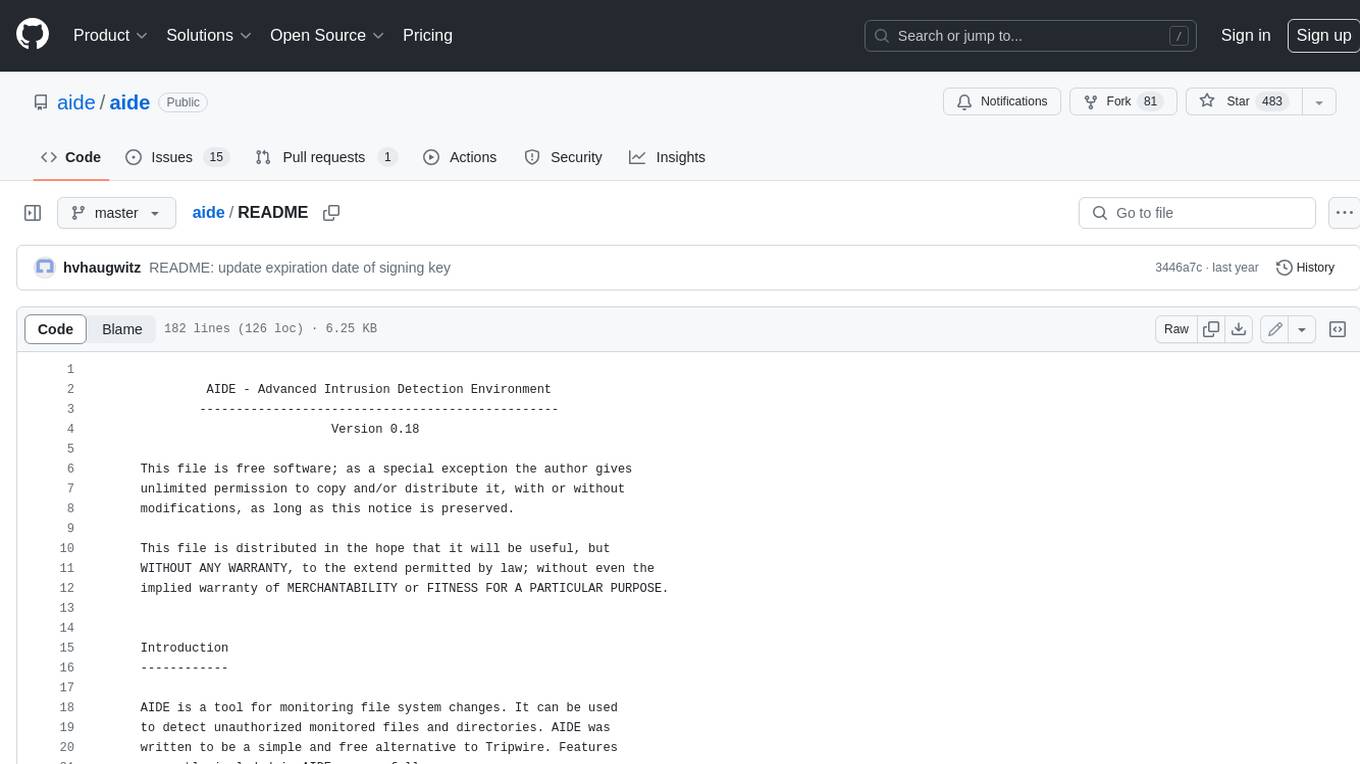
aide
AIDE (Advanced Intrusion Detection Environment) is a tool for monitoring file system changes. It can be used to detect unauthorized changes to monitored files and directories. AIDE was written to be a simple and free alternative to Tripwire. Features currently included in AIDE are as follows: o File attributes monitored: permissions, inode, user, group file size, mtime, atime, ctime, links and growing size. o Checksums and hashes supported: SHA1, MD5, RMD160, and TIGER. CRC32, HAVAL and GOST if Mhash support is compiled in. o Plain text configuration files and database for simplicity. o Rules, variables and macros that can be customized to local site or system policies. o Powerful regular expression support to selectively include or exclude files and directories to be monitored. o gzip database compression if zlib support is compiled in. o Free software licensed under the GNU General Public License v2.
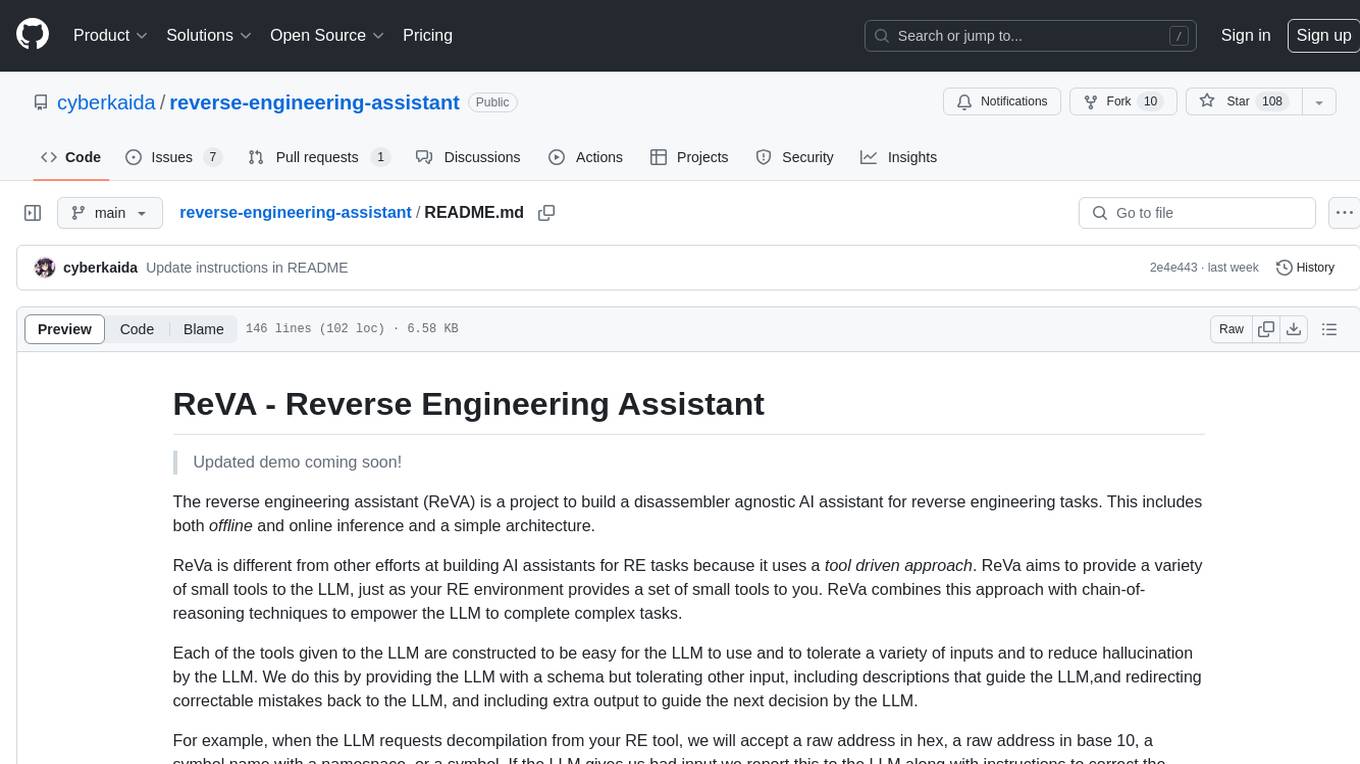
reverse-engineering-assistant
ReVA (Reverse Engineering Assistant) is a project aimed at building a disassembler agnostic AI assistant for reverse engineering tasks. It utilizes a tool-driven approach, providing small tools to the user to empower them in completing complex tasks. The assistant is designed to accept various inputs, guide the user in correcting mistakes, and provide additional context to encourage exploration. Users can ask questions, perform tasks like decompilation, class diagram generation, variable renaming, and more. ReVA supports different language models for online and local inference, with easy configuration options. The workflow involves opening the RE tool and program, then starting a chat session to interact with the assistant. Installation includes setting up the Python component, running the chat tool, and configuring the Ghidra extension for seamless integration. ReVA aims to enhance the reverse engineering process by breaking down actions into small parts, including the user's thoughts in the output, and providing support for monitoring and adjusting prompts.
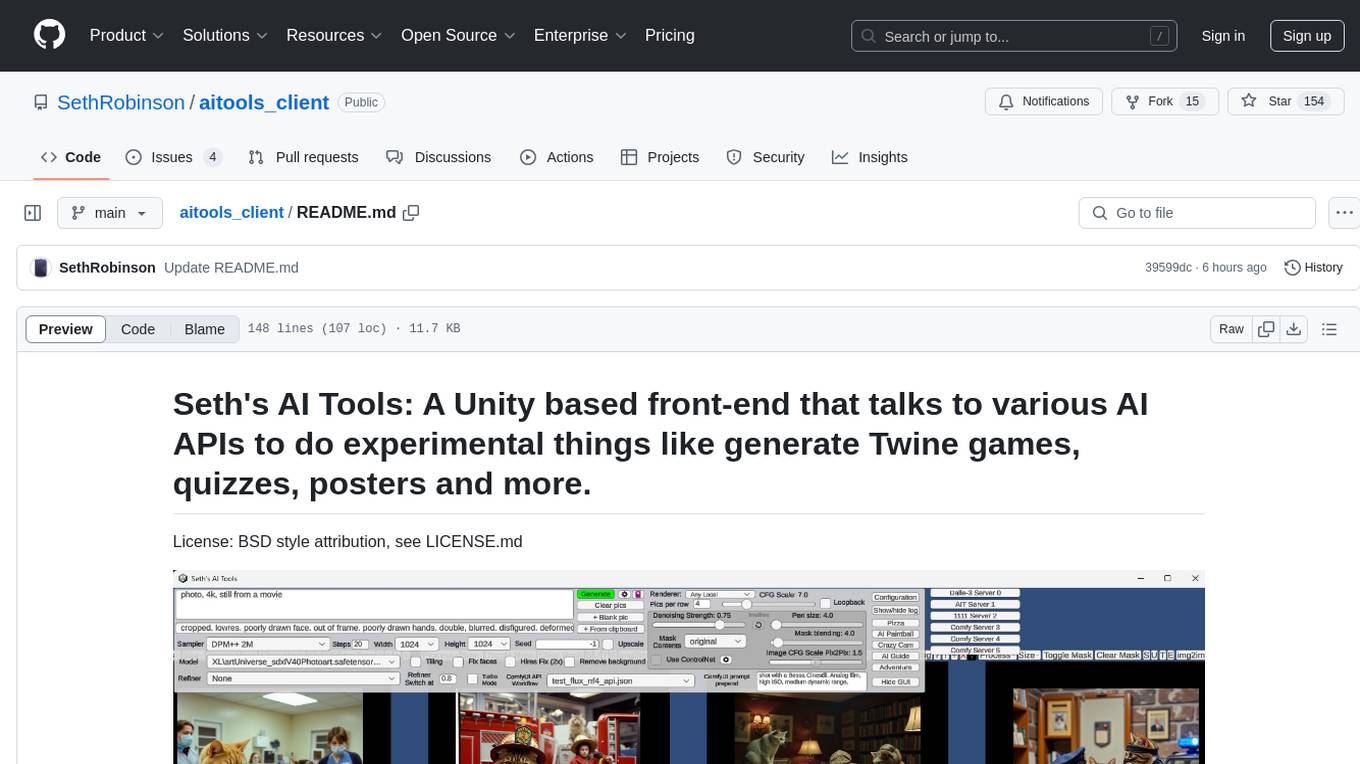
aitools_client
Seth's AI Tools is a Unity-based front-end that interfaces with various AI APIs to perform tasks such as generating Twine games, quizzes, posters, and more. The tool is a native Windows application that supports features like live update integration with image editors, text-to-image conversion, image processing, mask painting, and more. It allows users to connect to multiple servers for fast generation using GPUs and offers a neat workflow for evolving images in real-time. The tool respects user privacy by operating locally and includes built-in games and apps to test AI/SD capabilities. Additionally, it features an AI Guide for creating motivational posters and illustrated stories, as well as an Adventure mode with presets for generating web quizzes and Twine game projects.
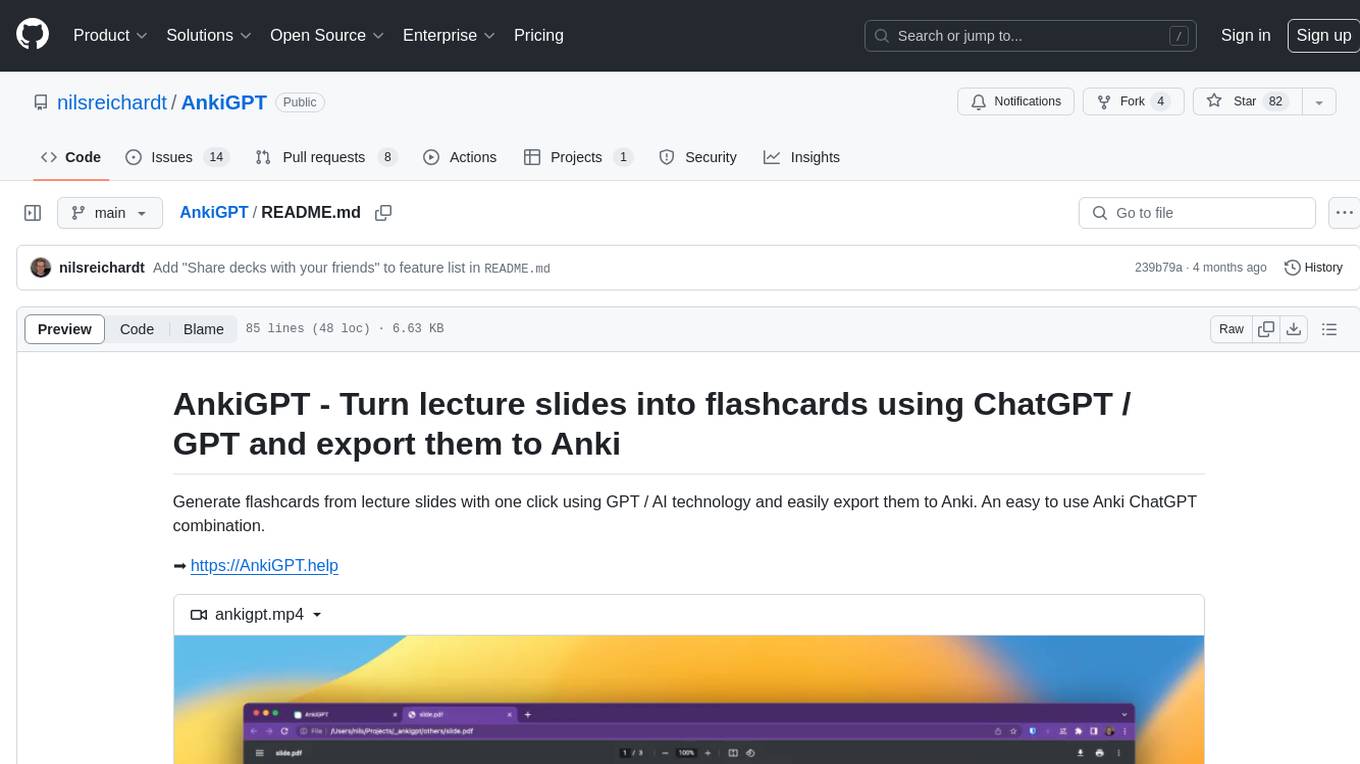
AnkiGPT
AnkiGPT is a tool that leverages GPT-3.5 or GPT-4 by OpenAI to generate flashcards from lecture slides or text input. Users can easily export the generated flashcards to Anki for effective learning. The tool allows users to edit, delete, and share flashcards, as well as generate mnemonics. AnkiGPT supports nearly all languages and ensures user privacy by not using submitted content for AI training. While powerful, the tool has limitations such as occasional errors in generated flashcards and challenges with mathematical equations. AnkiGPT is designed specifically for Anki flashcard app integration and encourages users to review and verify flashcard information for accuracy.
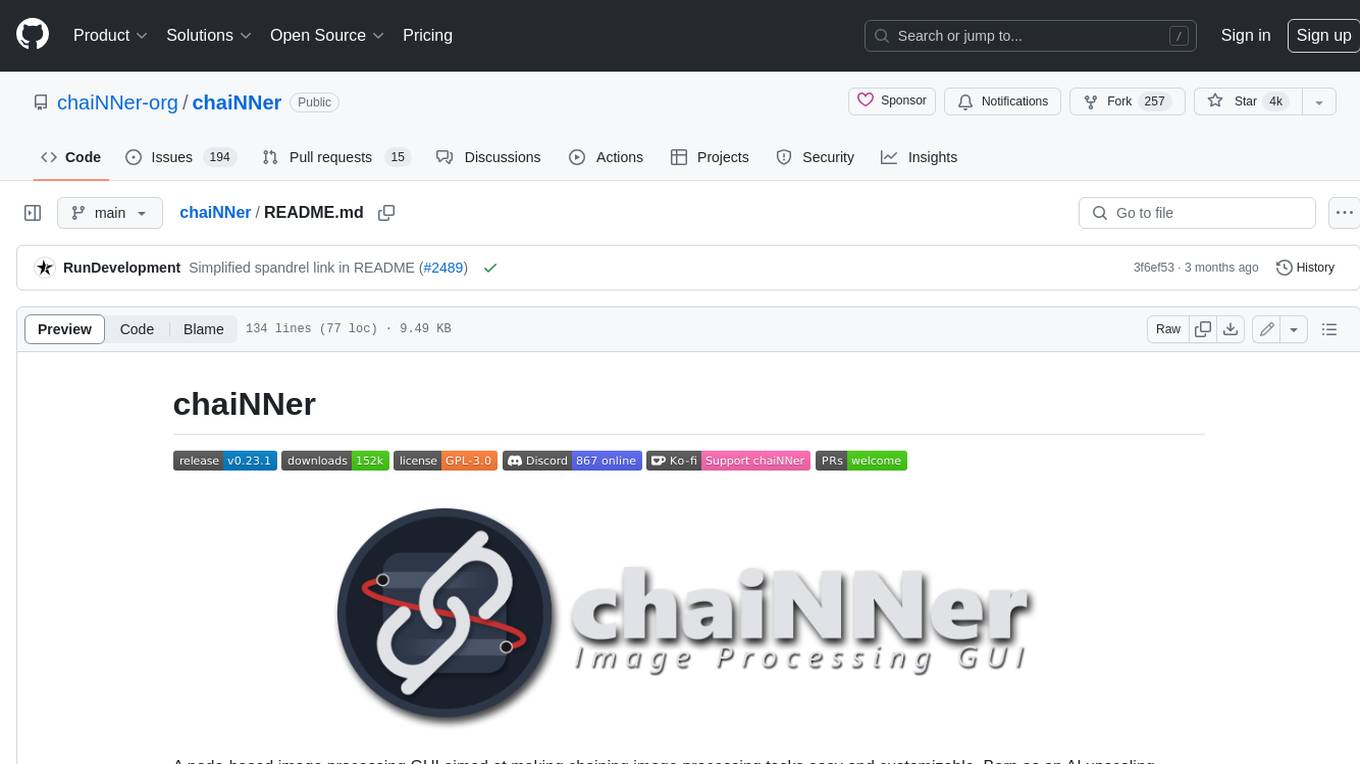
chaiNNer
ChaiNNer is a node-based image processing GUI aimed at making chaining image processing tasks easy and customizable. It gives users a high level of control over their processing pipeline and allows them to perform complex tasks by connecting nodes together. ChaiNNer is cross-platform, supporting Windows, MacOS, and Linux. It features an intuitive drag-and-drop interface, making it easy to create and modify processing chains. Additionally, ChaiNNer offers a wide range of nodes for various image processing tasks, including upscaling, denoising, sharpening, and color correction. It also supports batch processing, allowing users to process multiple images or videos at once.
For similar tasks
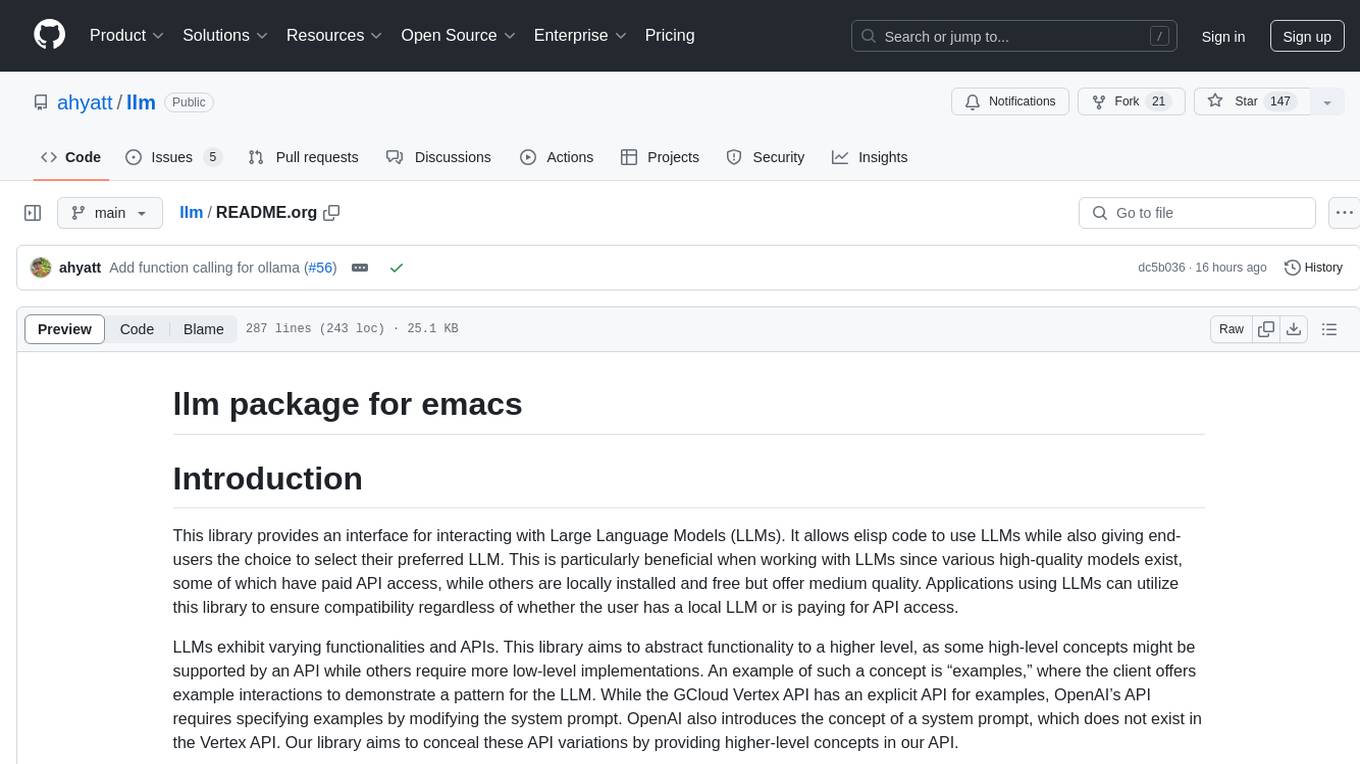
llm
The 'llm' package for Emacs provides an interface for interacting with Large Language Models (LLMs). It abstracts functionality to a higher level, concealing API variations and ensuring compatibility with various LLMs. Users can set up providers like OpenAI, Gemini, Vertex, Claude, Ollama, GPT4All, and a fake client for testing. The package allows for chat interactions, embeddings, token counting, and function calling. It also offers advanced prompt creation and logging capabilities. Users can handle conversations, create prompts with placeholders, and contribute by creating providers.
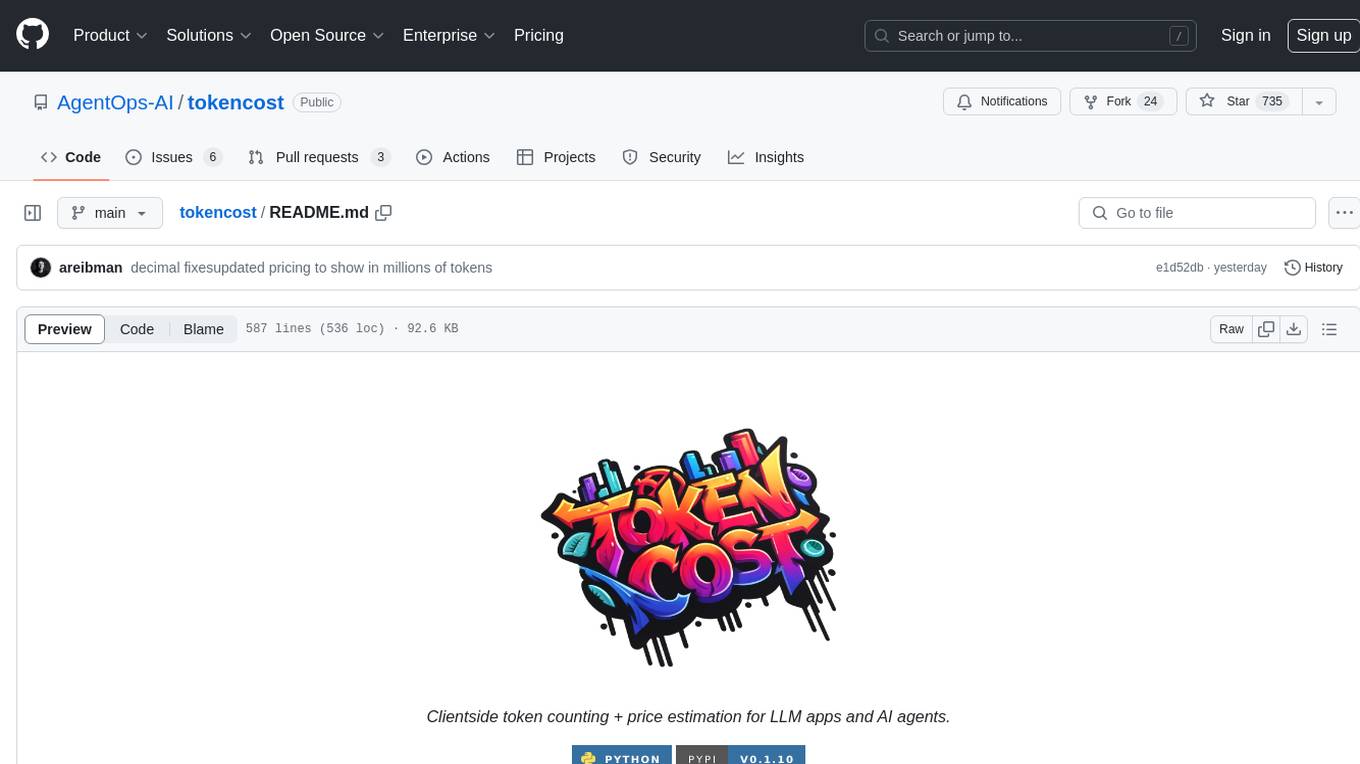
tokencost
Tokencost is a clientside tool for calculating the USD cost of using major Large Language Model (LLMs) APIs by estimating the cost of prompts and completions. It helps track the latest price changes of major LLM providers, accurately count prompt tokens before sending OpenAI requests, and easily integrate to get the cost of a prompt or completion with a single function. Users can calculate prompt and completion costs using OpenAI requests, count tokens in prompts formatted as message lists or string prompts, and refer to a cost table with updated prices for various LLM models. The tool also supports callback handlers for LLM wrapper/framework libraries like LlamaIndex and Langchain.
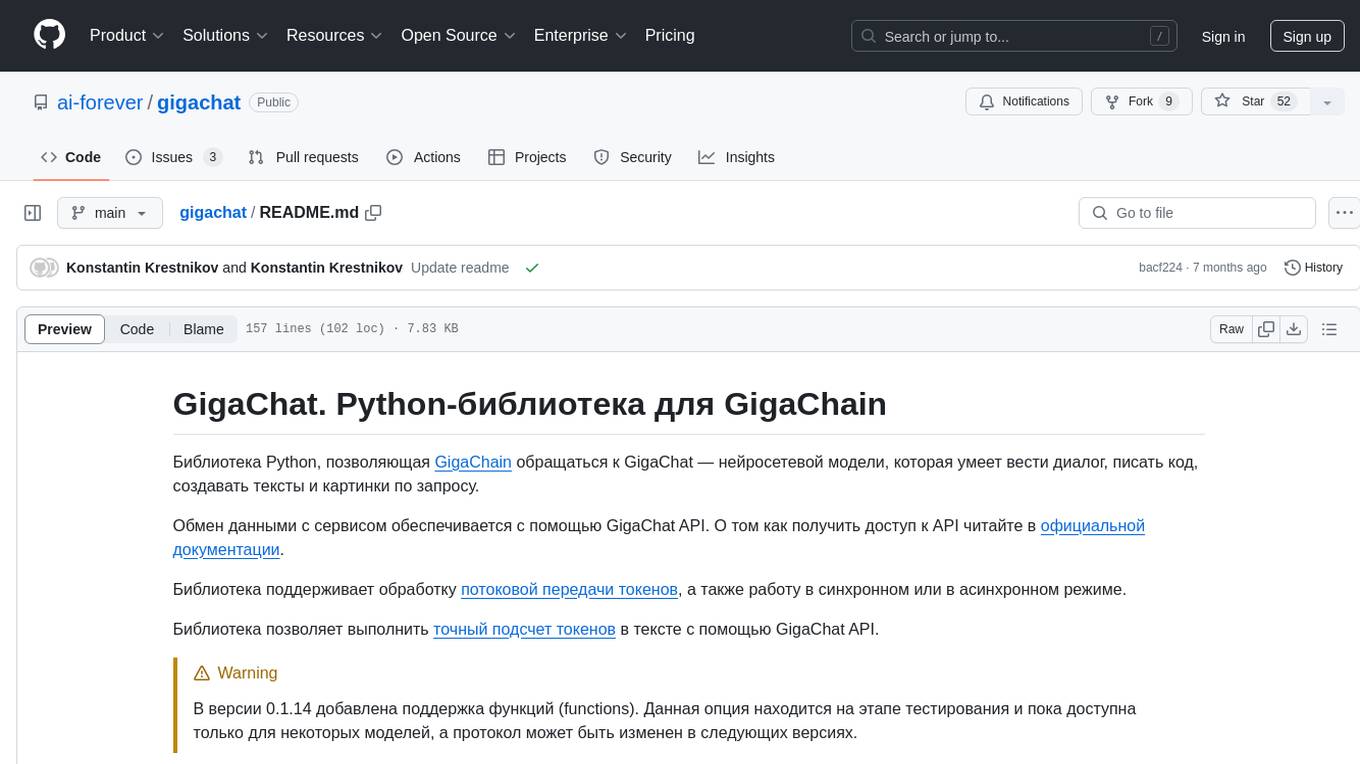
gigachat
GigaChat is a Python library that allows GigaChain to interact with GigaChat, a neural network model capable of engaging in dialogue, writing code, creating texts, and images on demand. Data exchange with the service is facilitated through the GigaChat API. The library supports processing token streaming, as well as working in synchronous or asynchronous mode. It enables precise token counting in text using the GigaChat API.
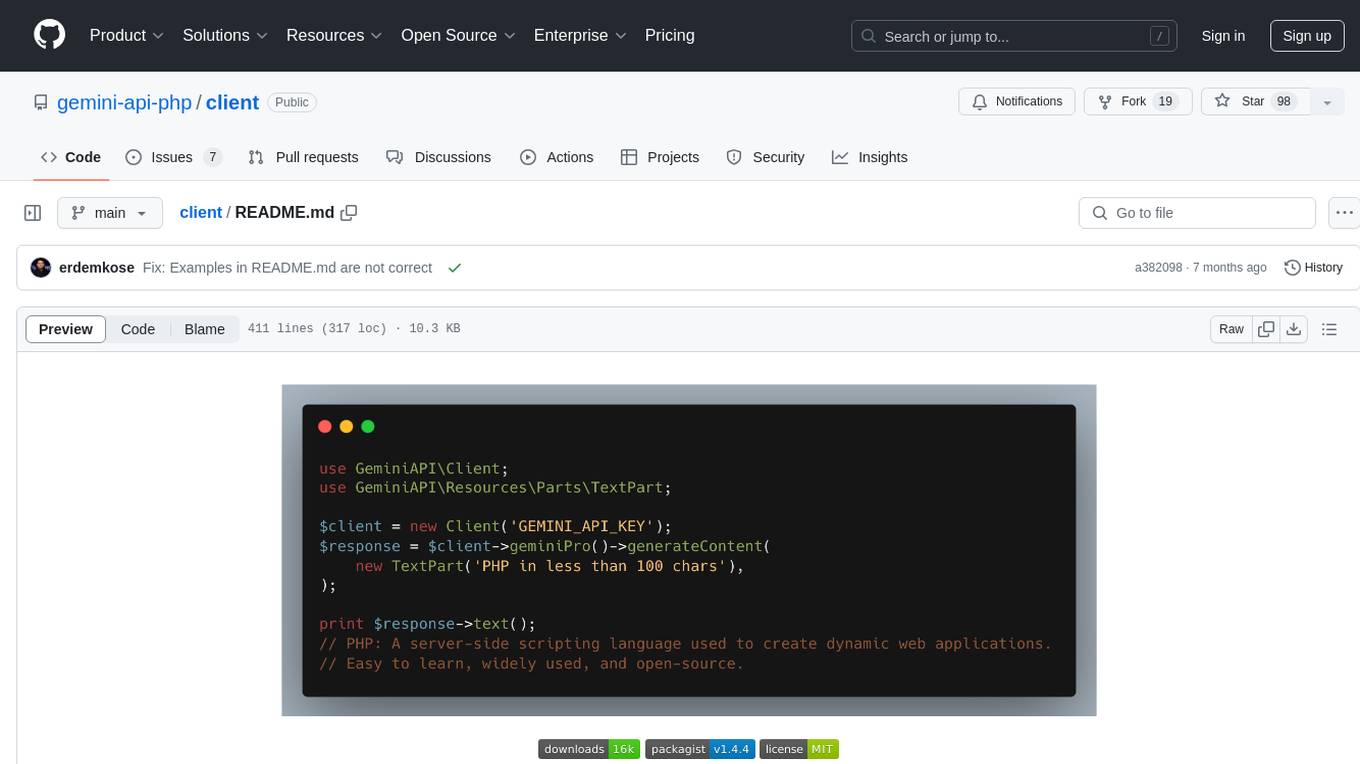
client
Gemini API PHP Client is a library that allows you to interact with Google's generative AI models, such as Gemini Pro and Gemini Pro Vision. It provides functionalities for basic text generation, multimodal input, chat sessions, streaming responses, tokens counting, listing models, and advanced usages like safety settings and custom HTTP client usage. The library requires an API key to access Google's Gemini API and can be installed using Composer. It supports various features like generating content, starting chat sessions, embedding content, counting tokens, and listing available models.
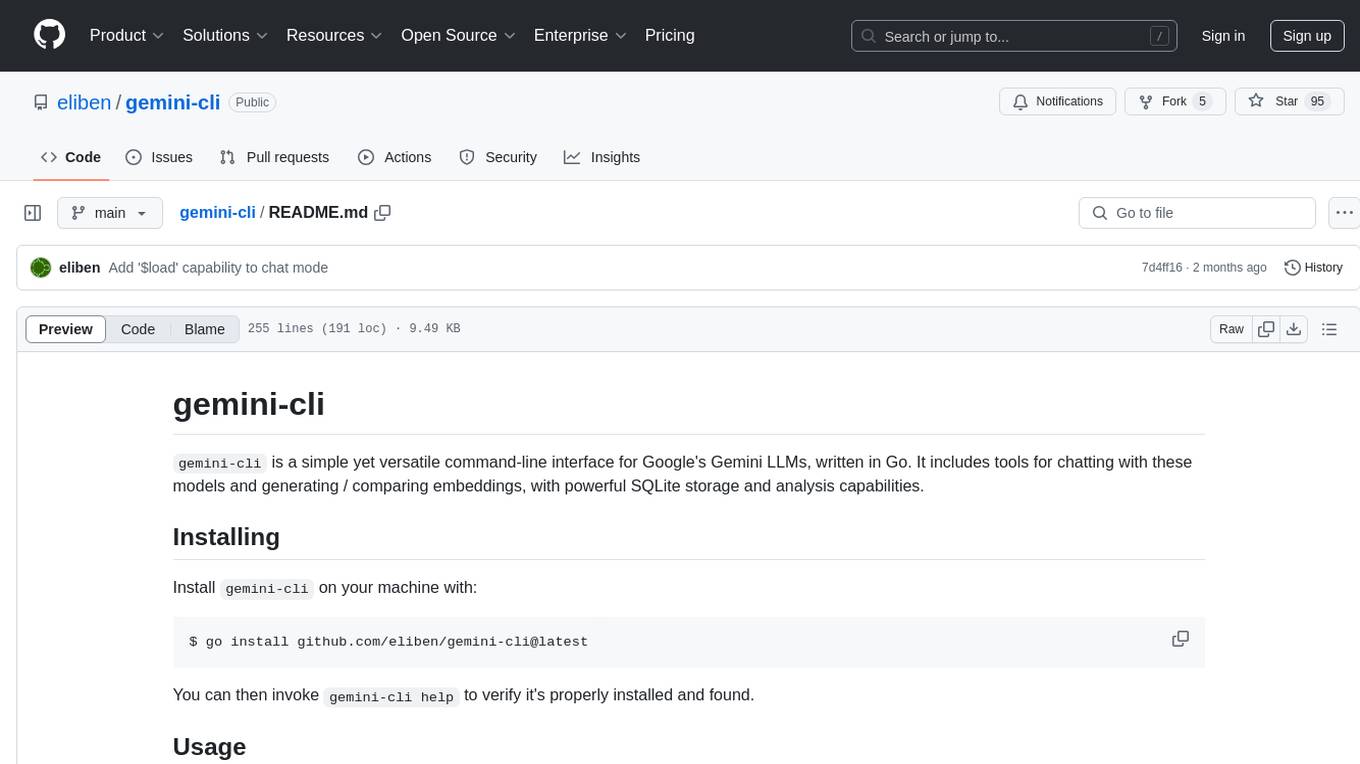
gemini-cli
gemini-cli is a versatile command-line interface for Google's Gemini LLMs, written in Go. It includes tools for chatting with models, generating/comparing embeddings, and storing data in SQLite for analysis. Users can interact with Gemini models through various subcommands like prompt, chat, counttok, embed content, embed db, and embed similar.
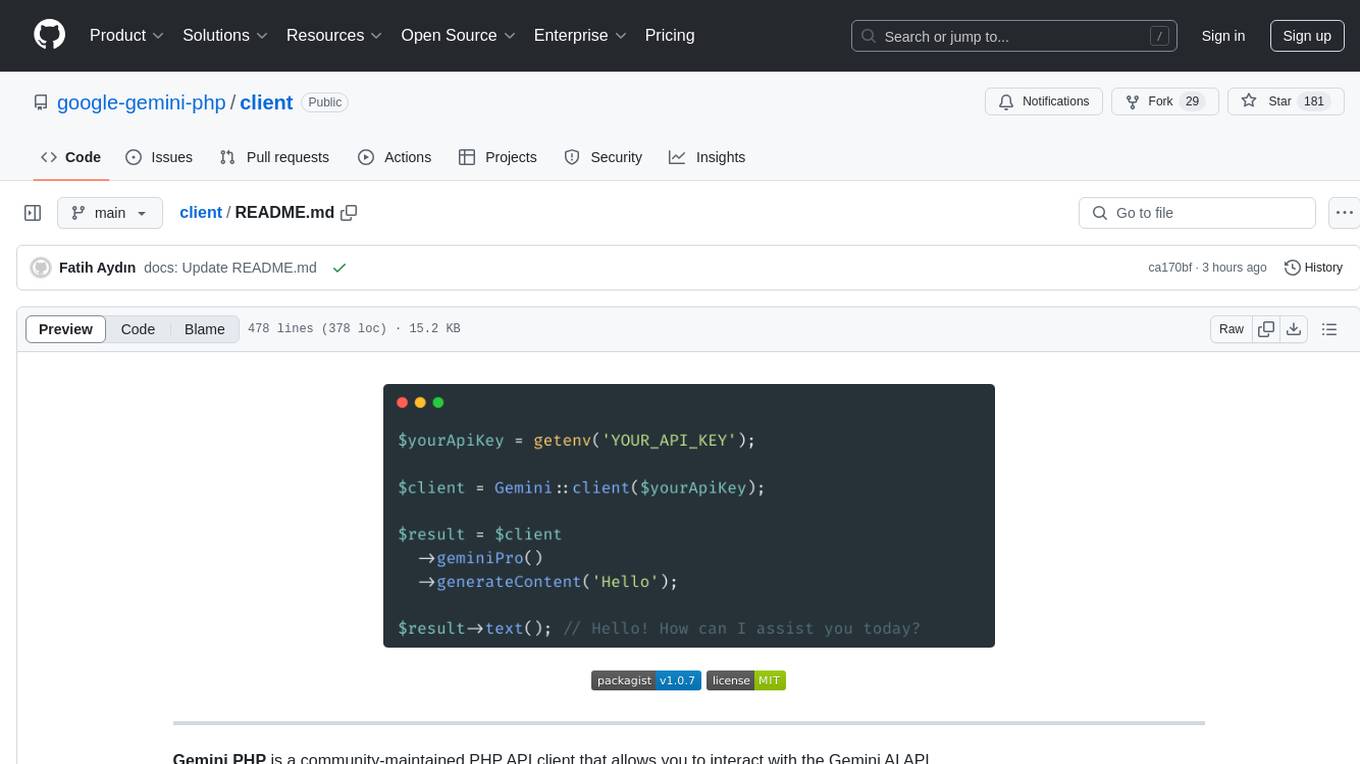
client
Gemini PHP is a PHP API client for interacting with the Gemini AI API. It allows users to generate content, chat, count tokens, configure models, embed resources, list models, get model information, troubleshoot timeouts, and test API responses. The client supports various features such as text-only input, text-and-image input, multi-turn conversations, streaming content generation, token counting, model configuration, and embedding techniques. Users can interact with Gemini's API to perform tasks related to natural language generation and text analysis.
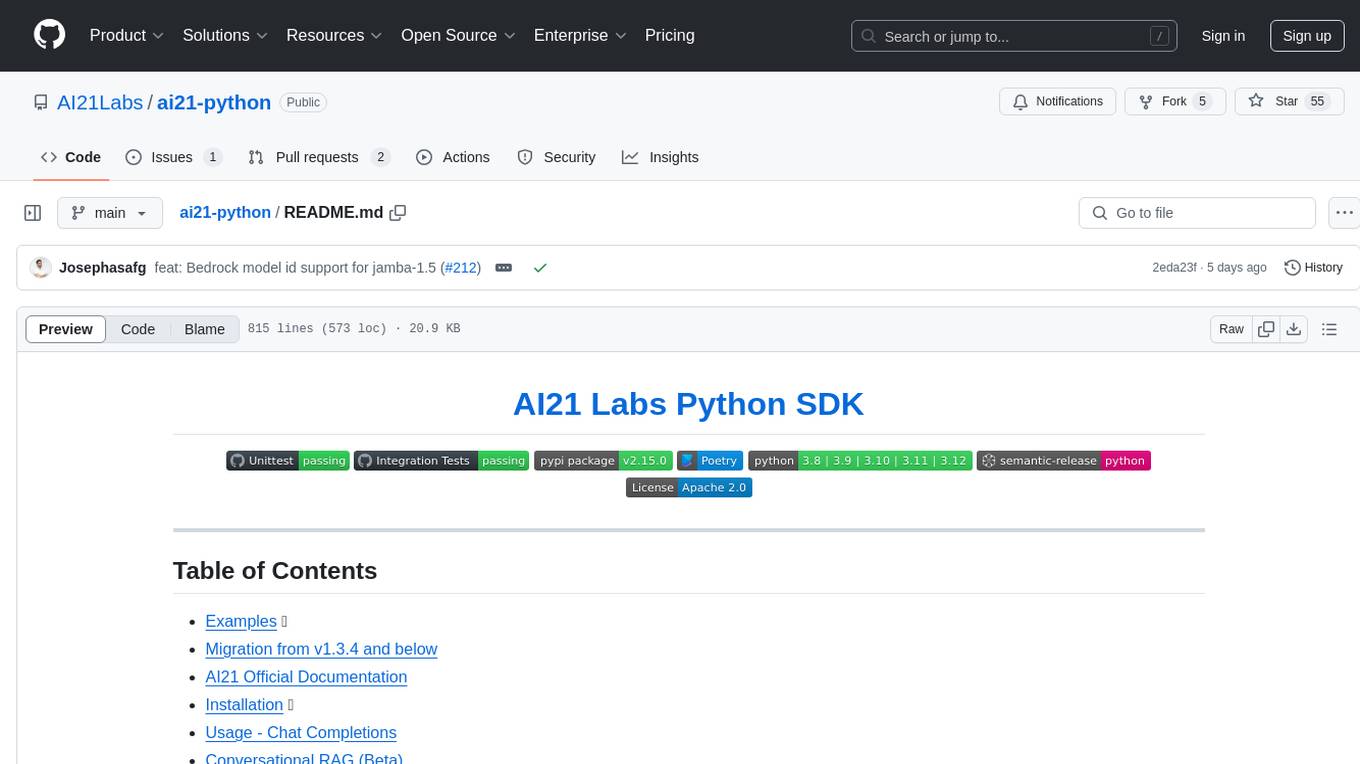
ai21-python
The AI21 Labs Python SDK is a comprehensive tool for interacting with the AI21 API. It provides functionalities for chat completions, conversational RAG, token counting, error handling, and support for various cloud providers like AWS, Azure, and Vertex. The SDK offers both synchronous and asynchronous usage, along with detailed examples and documentation. Users can quickly get started with the SDK to leverage AI21's powerful models for various natural language processing tasks.
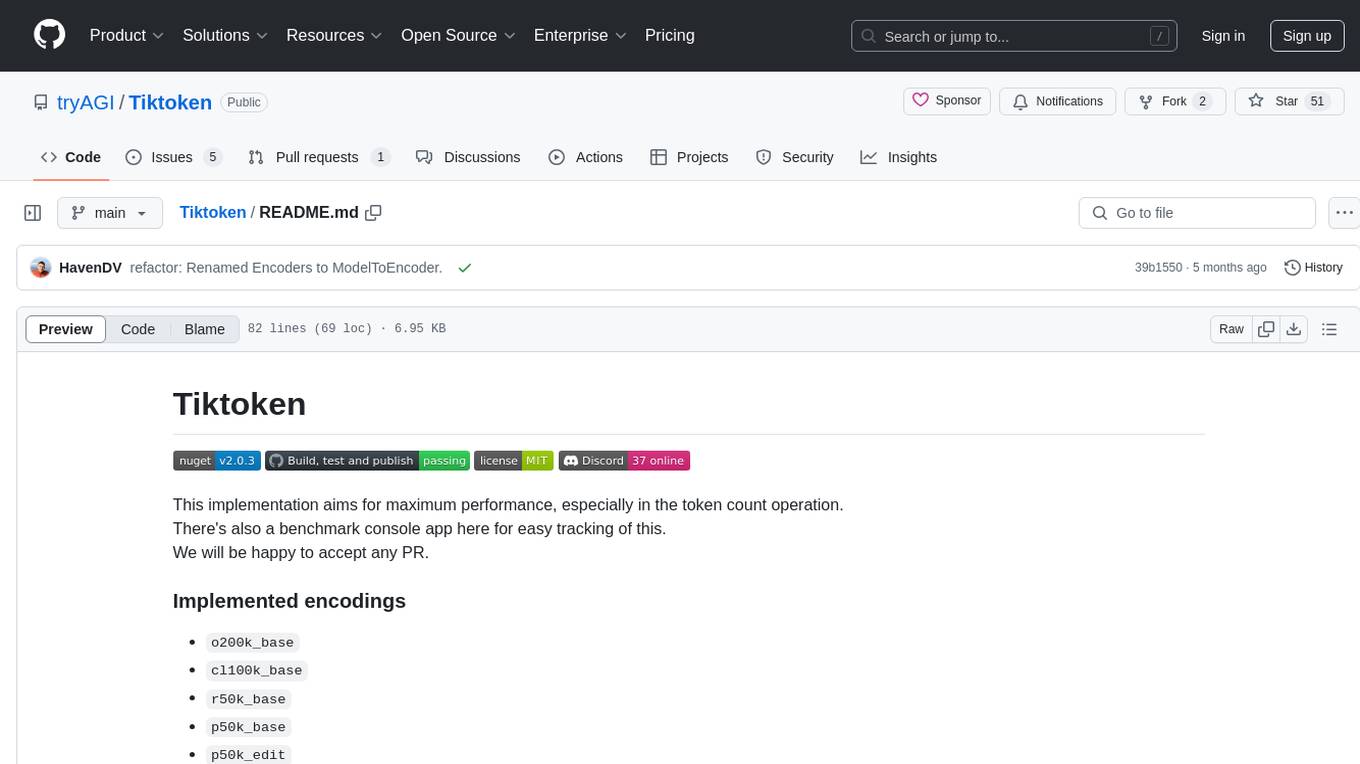
Tiktoken
Tiktoken is a high-performance implementation focused on token count operations. It provides various encodings like o200k_base, cl100k_base, r50k_base, p50k_base, and p50k_edit. Users can easily encode and decode text using the provided API. The repository also includes a benchmark console app for performance tracking. Contributions in the form of PRs are welcome.
For similar jobs
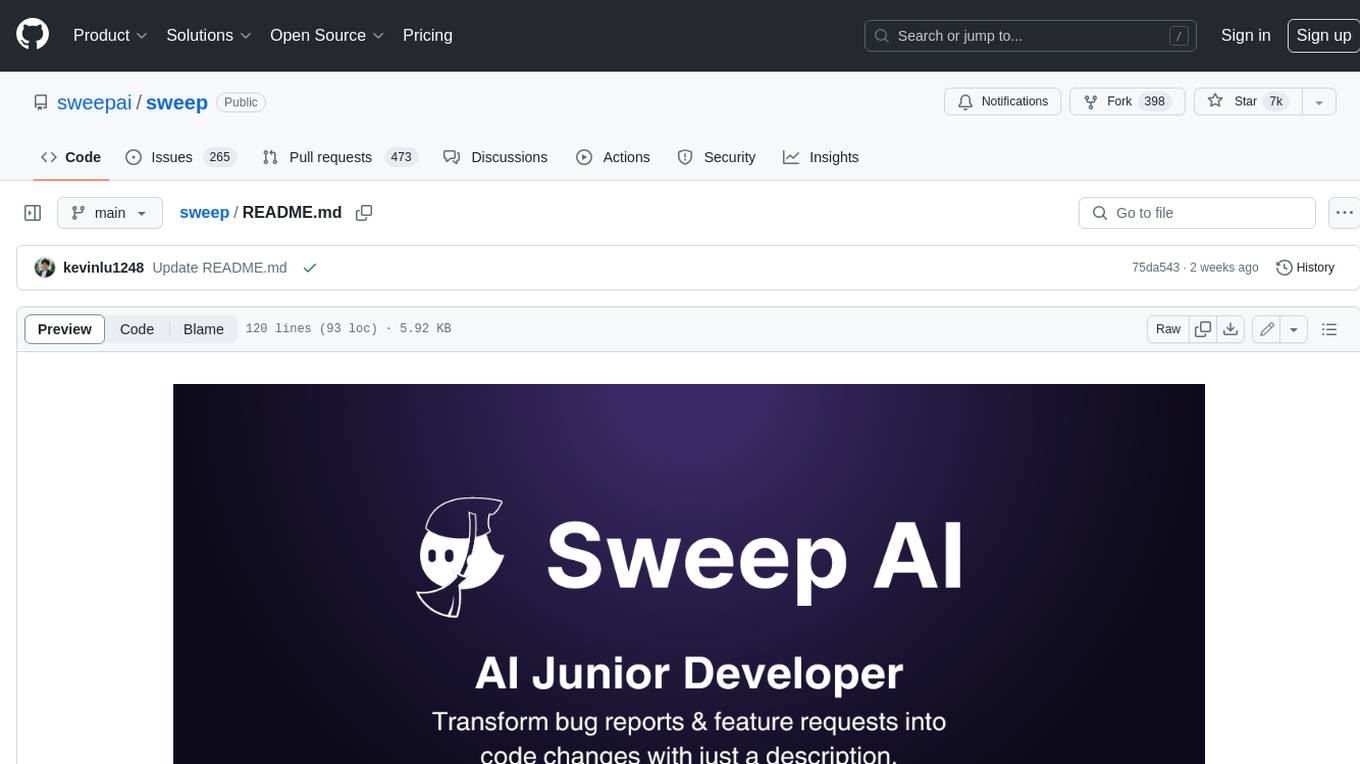
sweep
Sweep is an AI junior developer that turns bugs and feature requests into code changes. It automatically handles developer experience improvements like adding type hints and improving test coverage.
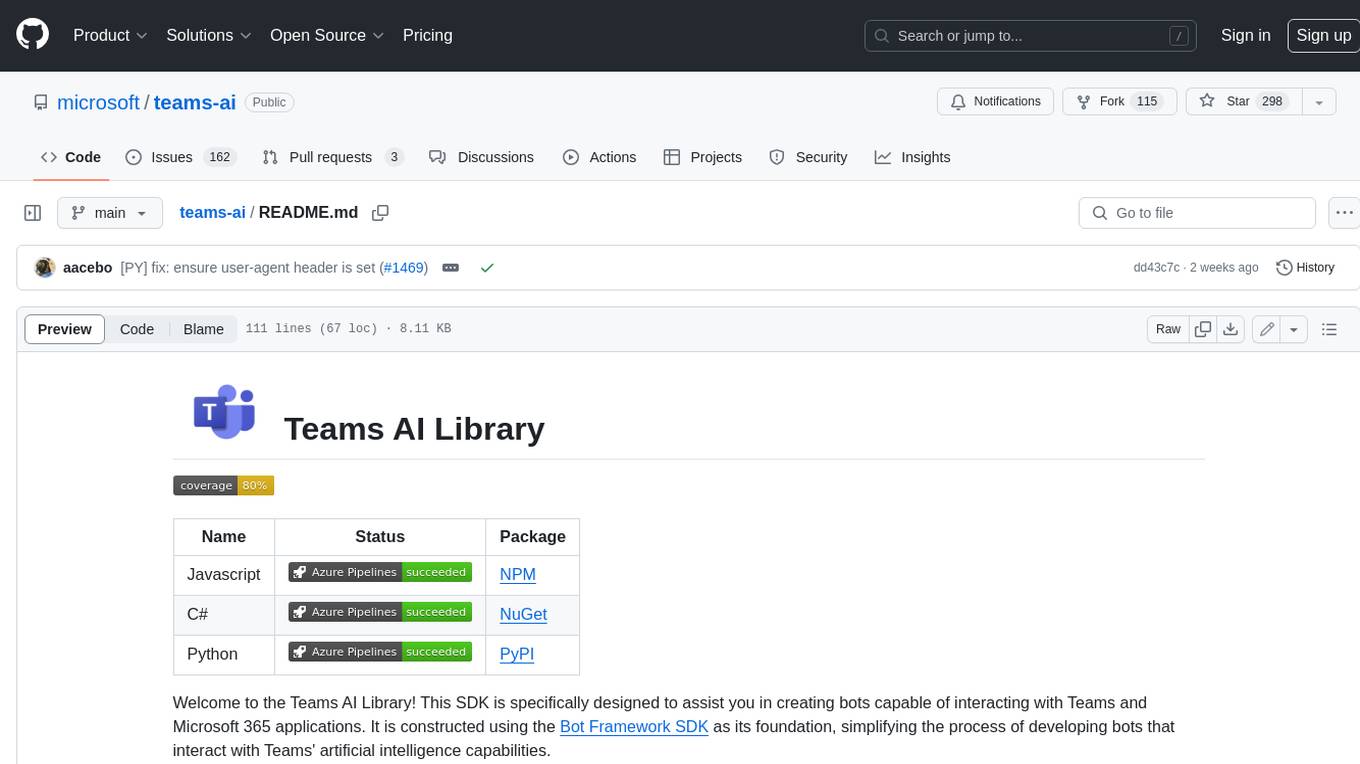
teams-ai
The Teams AI Library is a software development kit (SDK) that helps developers create bots that can interact with Teams and Microsoft 365 applications. It is built on top of the Bot Framework SDK and simplifies the process of developing bots that interact with Teams' artificial intelligence capabilities. The SDK is available for JavaScript/TypeScript, .NET, and Python.
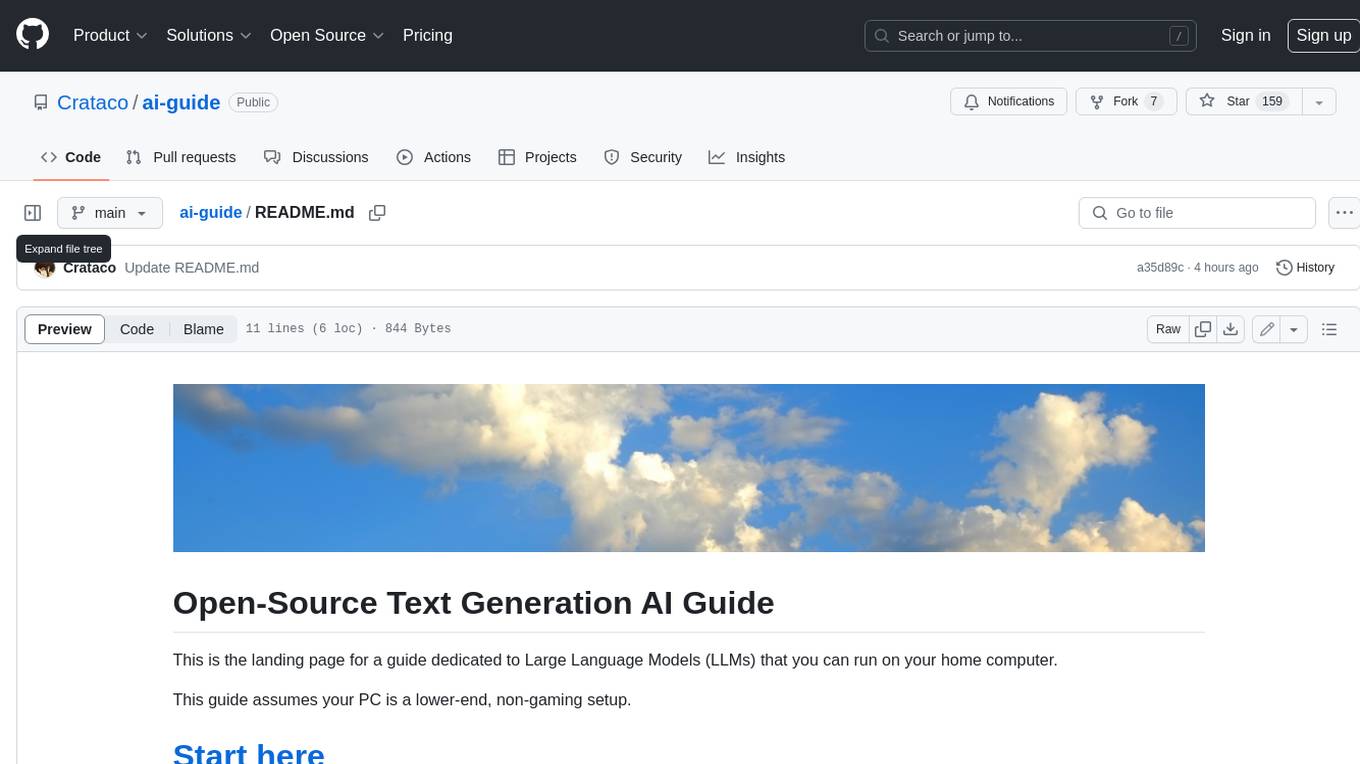
ai-guide
This guide is dedicated to Large Language Models (LLMs) that you can run on your home computer. It assumes your PC is a lower-end, non-gaming setup.
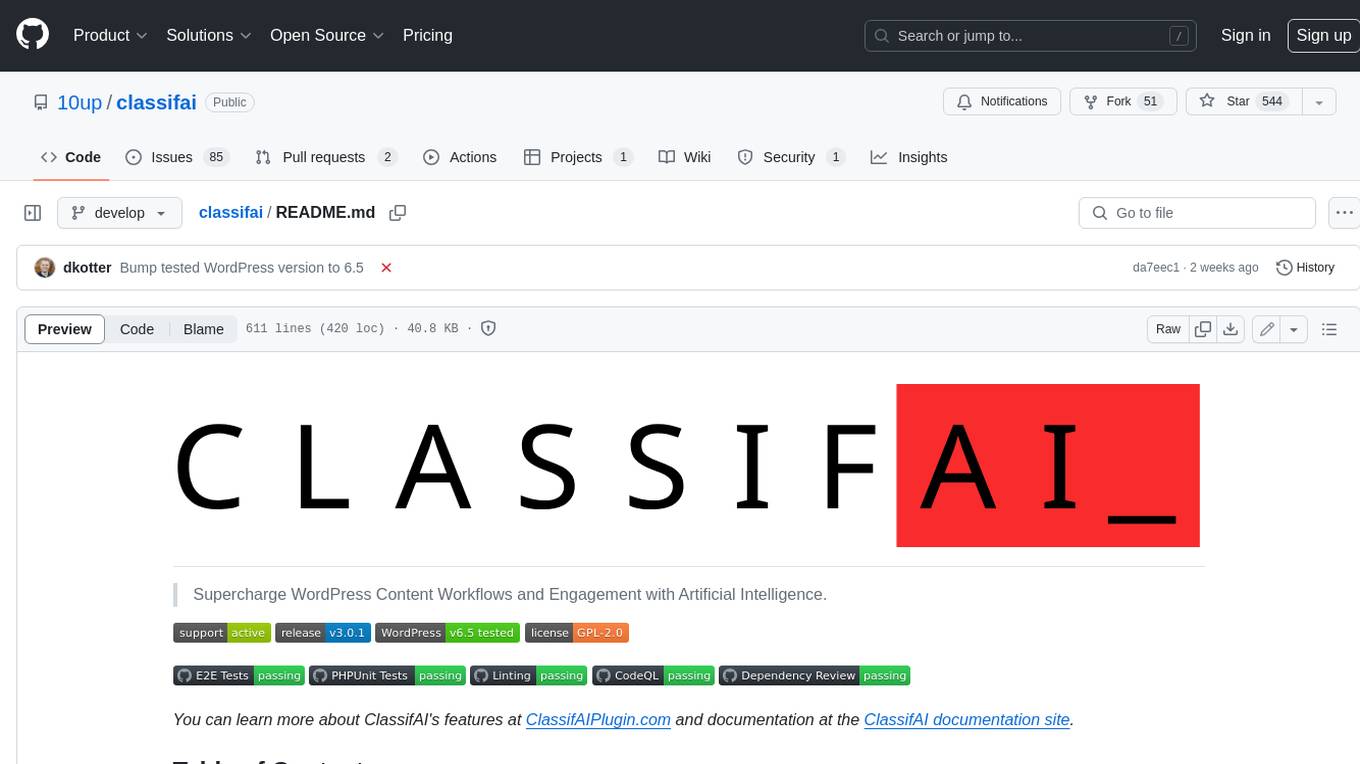
classifai
Supercharge WordPress Content Workflows and Engagement with Artificial Intelligence. Tap into leading cloud-based services like OpenAI, Microsoft Azure AI, Google Gemini and IBM Watson to augment your WordPress-powered websites. Publish content faster while improving SEO performance and increasing audience engagement. ClassifAI integrates Artificial Intelligence and Machine Learning technologies to lighten your workload and eliminate tedious tasks, giving you more time to create original content that matters.
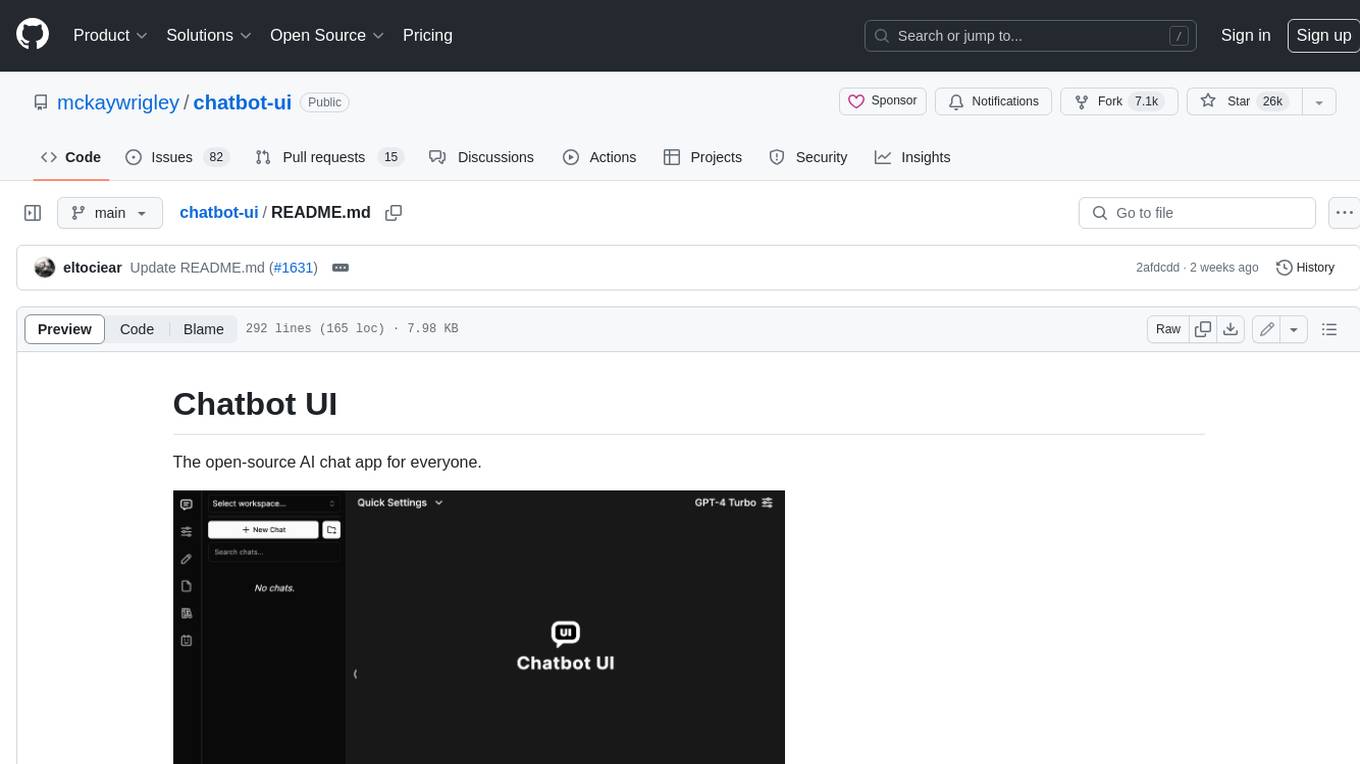
chatbot-ui
Chatbot UI is an open-source AI chat app that allows users to create and deploy their own AI chatbots. It is easy to use and can be customized to fit any need. Chatbot UI is perfect for businesses, developers, and anyone who wants to create a chatbot.
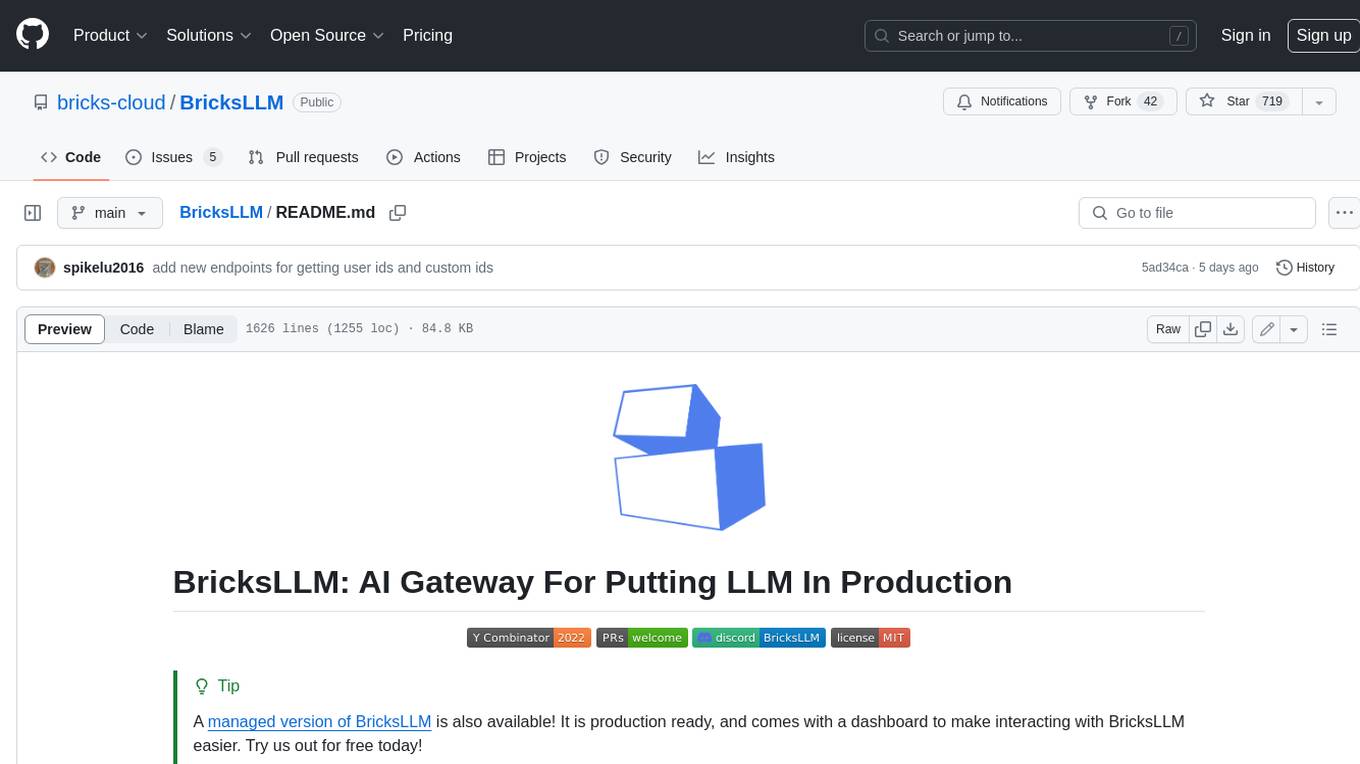
BricksLLM
BricksLLM is a cloud native AI gateway written in Go. Currently, it provides native support for OpenAI, Anthropic, Azure OpenAI and vLLM. BricksLLM aims to provide enterprise level infrastructure that can power any LLM production use cases. Here are some use cases for BricksLLM: * Set LLM usage limits for users on different pricing tiers * Track LLM usage on a per user and per organization basis * Block or redact requests containing PIIs * Improve LLM reliability with failovers, retries and caching * Distribute API keys with rate limits and cost limits for internal development/production use cases * Distribute API keys with rate limits and cost limits for students
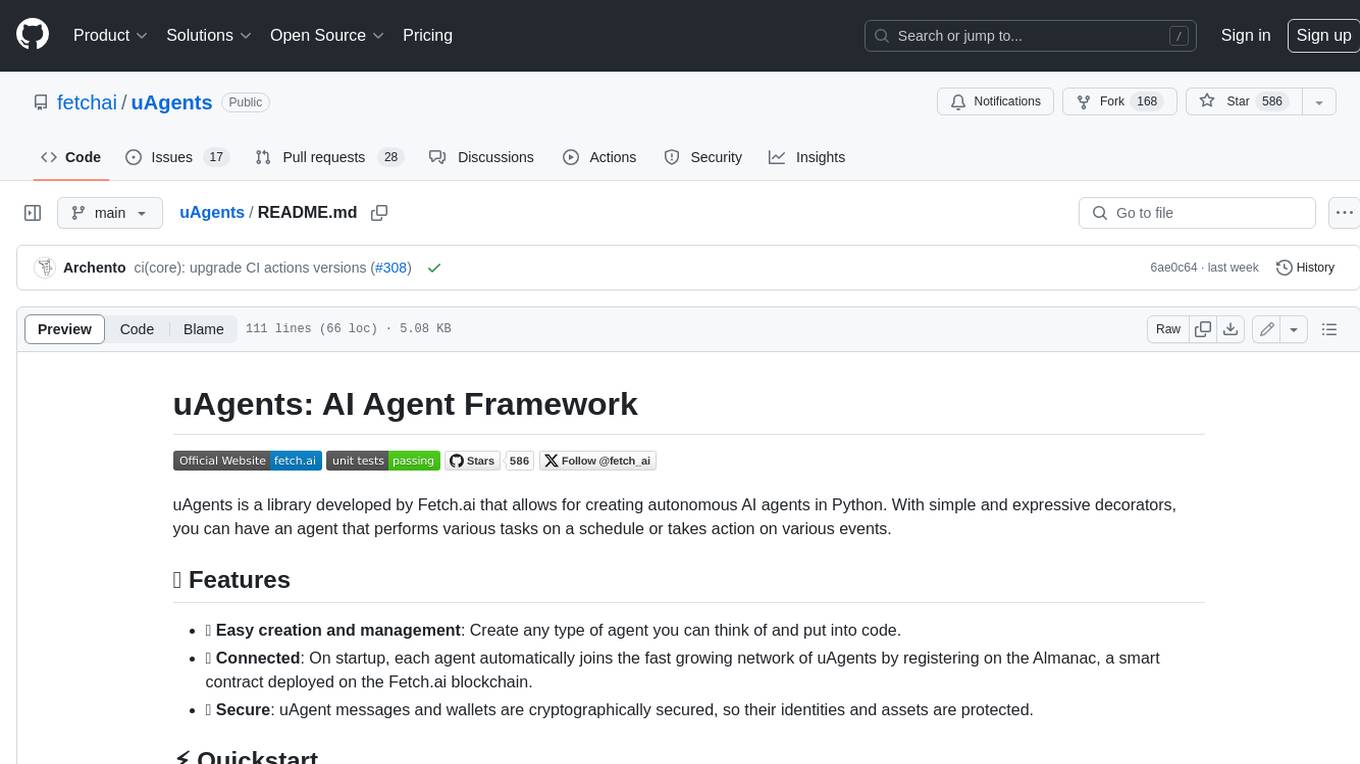
uAgents
uAgents is a Python library developed by Fetch.ai that allows for the creation of autonomous AI agents. These agents can perform various tasks on a schedule or take action on various events. uAgents are easy to create and manage, and they are connected to a fast-growing network of other uAgents. They are also secure, with cryptographically secured messages and wallets.
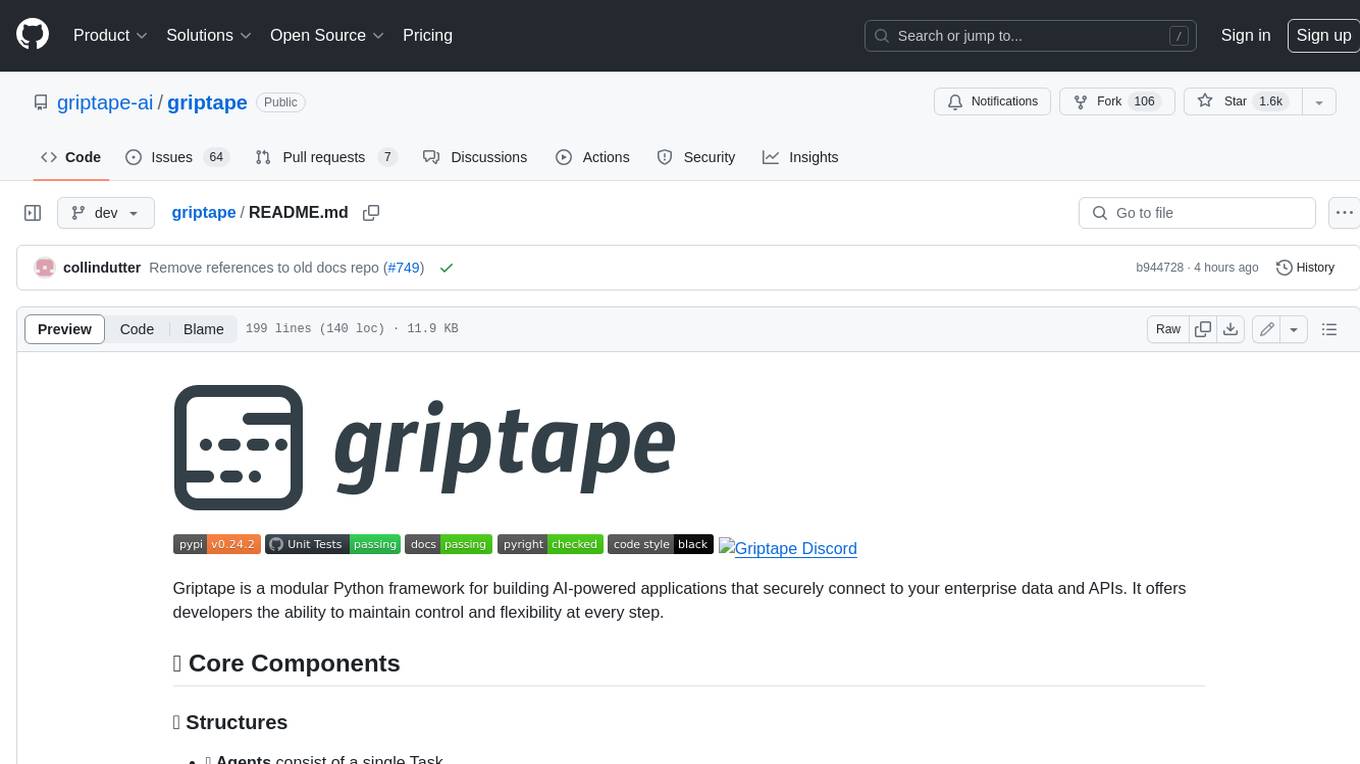
griptape
Griptape is a modular Python framework for building AI-powered applications that securely connect to your enterprise data and APIs. It offers developers the ability to maintain control and flexibility at every step. Griptape's core components include Structures (Agents, Pipelines, and Workflows), Tasks, Tools, Memory (Conversation Memory, Task Memory, and Meta Memory), Drivers (Prompt and Embedding Drivers, Vector Store Drivers, Image Generation Drivers, Image Query Drivers, SQL Drivers, Web Scraper Drivers, and Conversation Memory Drivers), Engines (Query Engines, Extraction Engines, Summary Engines, Image Generation Engines, and Image Query Engines), and additional components (Rulesets, Loaders, Artifacts, Chunkers, and Tokenizers). Griptape enables developers to create AI-powered applications with ease and efficiency.