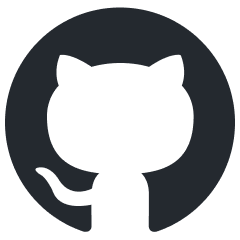
AQLM
Official Pytorch repository for Extreme Compression of Large Language Models via Additive Quantization https://arxiv.org/pdf/2401.06118.pdf and PV-Tuning: Beyond Straight-Through Estimation for Extreme LLM Compression https://arxiv.org/abs/2405.14852
Stars: 1218
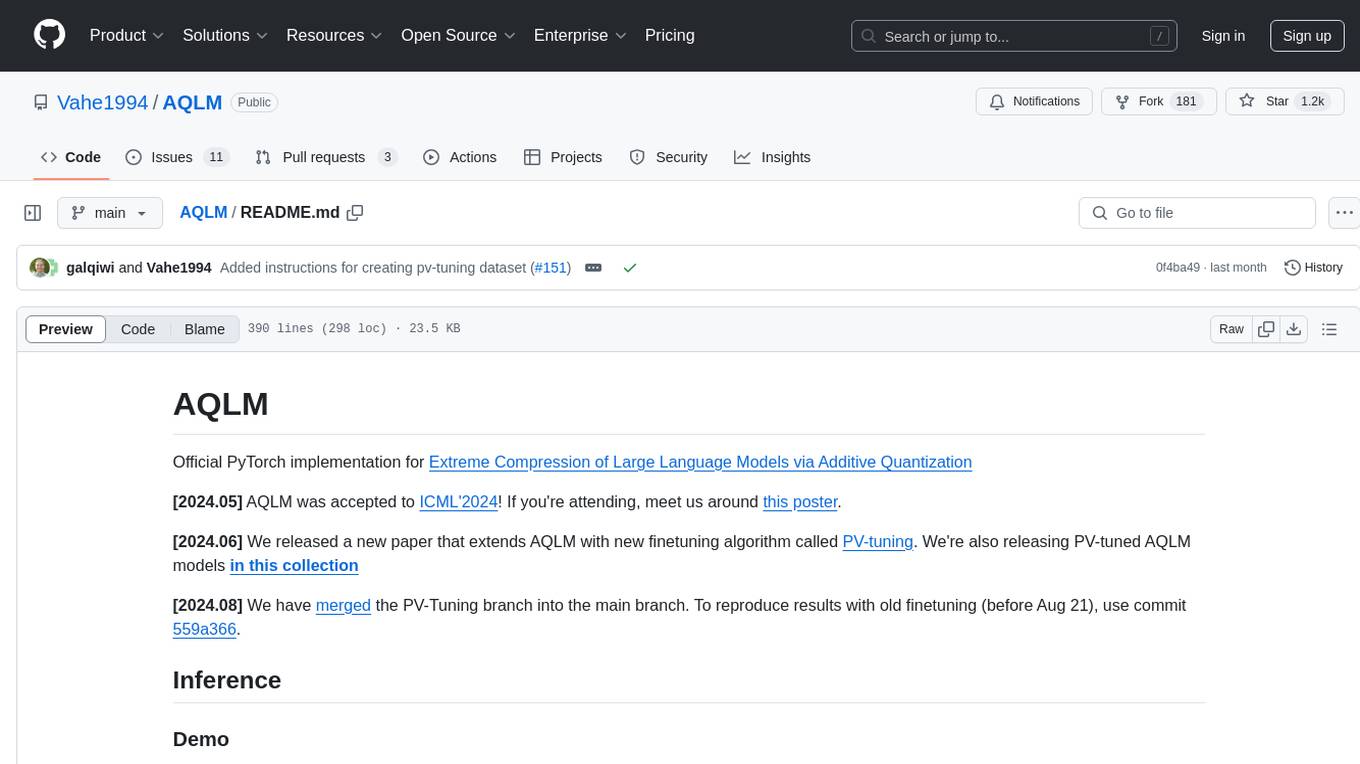
AQLM is the official PyTorch implementation for Extreme Compression of Large Language Models via Additive Quantization. It includes prequantized AQLM models without PV-Tuning and PV-Tuned models for LLaMA, Mistral, and Mixtral families. The repository provides inference examples, model details, and quantization setups. Users can run prequantized models using Google Colab examples, work with different model families, and install the necessary inference library. The repository also offers detailed instructions for quantization, fine-tuning, and model evaluation. AQLM quantization involves calibrating models for compression, and users can improve model accuracy through finetuning. Additionally, the repository includes information on preparing models for inference and contributing guidelines.
README:
Official PyTorch implementation for Extreme Compression of Large Language Models via Additive Quantization
[2024.05] AQLM was accepted to ICML'2024! If you're attending, meet us around this poster.
[2024.06] We released a new paper that extends AQLM with new finetuning algorithm called PV-tuning. We're also releasing PV-tuned AQLM models in this collection
[2024.08] We have merged the PV-Tuning branch into the main branch. To reproduce results with old finetuning (before Aug 21), use commit 559a366.
Learn how to run the prequantized models using this Google Colab examples:
Basic AQLM generation |
Streaming with GPU/CPU |
Inference with CUDA graphs (3x speedup) |
Fine-tuning with PEFT |
Serving with vLLM
|
---|---|---|---|---|
This repository is currently designed to work with models of LLaMA
, Mistral
and Mixtral
families.
The models reported below use full model fine-tuning as described in appendix A, with cross-entropy objective with teacher logits.
We provide a number of prequantized AQLM models without PV-Tuning (scroll down for PV-Tuned models):
Model | AQLM scheme | WikiText-2 PPL | MMLU (5-shot) FP16→AQLM | Model size, Gb | Hub link |
---|---|---|---|---|---|
Llama-3-8b | 1x16 | - | 0.65→0.56 | 4.1 | Link |
Llama-3-8b-Instruct | 1x16 | - | 0.66→0.59 | 4.1 | Link |
Llama-3-70b | 1x16 | - | 0.79→0.75 | 21.9 | Link |
Llama-3-70b-Instruct | 1x16 | - | 0.80→0.76 | 21.9 | Link |
Command-R | 1x16 | - | 0.68→0.57 | 12.7 | Link |
Command-R+ | 1x16 | - | 0.74→0.68 | 31.9 | Link |
Mistral-7b | 1x16 | 5.40 | - | 2.5 | Link |
Mistral-7B-Instruct-v0.2 | 2x8 | - | 0.59→0.44 | 2.5 | Link |
Mixtral-8x7b | 1x16 | 3.35 | - | 12.6 | Link |
Mixtral-8x7b-Instruct | 1x16 | - | - | 12.6 | Link |
Llama-2-7b | 1x16 | 5.92 | 0.46→0.39 | 2.4 | Link |
Llama-2-7b | 2x8 | 6.69 | - | 2.2 | Link |
Llama-2-7b | 8x8 | 6.61 | - | 2.2 | Link |
Llama-2-13b | 1x16 | 5.22 | 0.55→0.49 | 4.1 | Link |
Llama-2-13b | 2x8 | 5.63 | - | 3.8 | Link |
Llama-2-70b | 1x16 | 3.83 | 0.69→0.65 | 18.8 | Link |
Llama-2-70b | 2x8 | 4.21 | - | 18.2 | Link |
gemma-2b | 1x16 | - | - | 1.7 | Link |
gemma-2b | 2x8 | - | - | 1.6 | Link |
You can also download AQLM models tuned via PV-tuning:
Model | AQLM scheme | WikiText-2 PPL | Model size, Gb | Hub link |
---|---|---|---|---|
Llama-2-7b | 1x16g8 | 5.68 | 2.4 | Link |
Llama-2-7b | 2x8g8 | 5.90 | 2.2 | Link |
Llama-2-7b | 1x16g16 | 9.21 | 1.7 | Link |
Llama-2-13b | 1x16g8 | 5.05 | 4.1 | Link |
Llama-2-70b | 1x16g8 | 3.78 | 18.8 | Link |
Meta-Llama-3-8B | 1x16g8 | 6.99 | 4.1 | Link |
Meta-Llama-3-8B | 1x16g16 | 9.43 | 3.9 | Link |
Meta-Llama-3-70B | 1x16g8 | 4.57 | 21.9 | Link |
Meta-Llama-3-70B | 1x16g16 | 8.67 | 13 | Link |
Mistral-7B-v0.1 | 1x16g8 | 5.22 | 2.51 | Link |
Phi-3-mini-4k-instruct | 1x16g8 | 6.63 | 1.4 | Link |
Note that models with "g16" in their scheme require aqlm inference library v1.1.6 or newer:
pip install aqlm[gpu,cpu]>=1.1.6
Above perplexity is evaluated on 4k context length for Llama 2 models and 8k for Mistral/Mixtral and Llama 3. Please also note that token-level perplexity can only be compared within the same model family, but should not be compared between models that use different vocabularies. While Mistral has a lower perplexity than Llama 3 8B but this does not mean that Mistral is better: Llama's perplexity is computed on a much larger dictionary and has higher per-token perplexity because of that.
For more evaluation results and detailed explanations, please see our papers: Egiazarian et al. (2024) for pure AQLM and Malinovskii et al. (2024) for PV-Tuned models.
AQLM quantization setpus vary mainly on the number of codebooks used as well as the codebook sizes in bits. The most popular setups, as well as inference kernels they support are:
Kernel | Number of codebooks | Codebook size, bits | Scheme Notation | Accuracy | Speedup | Fast GPU inference | Fast CPU inference |
---|---|---|---|---|---|---|---|
Triton | K | N | KxN | - | Up to ~0.7x | ✅ | ❌ |
CUDA | 1 | 16 | 1x16 | Best | Up to ~1.3x | ✅ | ❌ |
CUDA | 2 | 8 | 2x8 | OK | Up to ~3.0x | ✅ | ❌ |
Numba | K | 8 | Kx8 | Good | Up to ~4.0x | ❌ | ✅ |
To run the models, one would have to install an inference library:
pip install aqlm[gpu,cpu]
, specifying either gpu
, cpu
or both based on one's inference setting.
Then, one can use the familiar .from_pretrained
method provided by the transformers library:
from transformers import AutoModelForCausalLM
quantized_model = AutoModelForCausalLM.from_pretrained(
"ISTA-DASLab/Llama-2-7b-AQLM-2Bit-1x16-hf",
trust_remote_code=True, torch_dtype="auto"
).cuda()
Notice that torch_dtype
should be set to either torch.float16
or "auto"
on GPU and torch.float32
on CPU. After that, the model can be used exactly the same as one would use and unquantized model.
Install packages from requirements.txt
:
pip install -r requirements.txt
The script will require downloading and caching locally the relevant tokenizer and the datasets. They will be saved in default Huggingface Datasets directory unless alternative location is provided by env variables. See relevant Datasets documentation section
When quantizing models with AQLM, we recommend that you use a subset of the original data the model was trained on.
For Llama-2 models, the closest available dataset is RedPajama . To load subset of RedPajama provide "pajama" in --dataset argument. This will process nsamples data and tokenize it using provided model tokenizer.
Additionally we provide tokenized Redpajama for LLama and Solar/Mistral models for 4096 context lengths stored in Hunggingface . To load it, use:
from huggingface_hub import hf_hub_download
hf_hub_download(repo_id="Vahe1994/AQLM", filename="data/name.pth", repo_type="dataset")
To use downloaded data from HF, place it in data folder(optional) and set correct path to it in "--dataset" argument in main.py.
Warning: These subsets are already processed with the corresponding model tokenizer. If you want to quantize another model (e.g. mistral/mixtral), please re-tokenize the data with provided script in src/datautils.
One can optionally log the data to Weights and Biases
service (wandb).
Run pip install wandb
for W&B logging.
Specify $WANDB_ENTITY
, $WANDB_PROJECT
, $WANDB_NAME
environment variables prior to running experiments. use --wandb
argument to enable logging
This code was developed and tested using a several A100 GPU with 80GB GPU RAM.
You can use the --offload activations
option to reduce VRAM usage.
For Language Model Evaluation Harness
evaluation one needs to have enough memory to load whole model + activation tensors
on one or several devices.
AQLM quantization takes considerably longer to calibrate than simpler quantization methods such as GPTQ. This only impacts quantization time, not inference time.
For instance, quantizing a 7B model with default configuration takes about 1 day on a single A100 gpu. Similarly, quantizing a 70B model on a single GPU would take 10-14 days. If you have multiple GPUs with fast interconnect, you can run AQLM multi-gpu to speed up comparison - simply set CUDA_VISIBLE_DEVICES for multiple GPUs. Quantizing 7B model on two gpus reduces quantization time to ~14.5 hours. Similarly, quantizing a 70B model on 8 x A100 GPUs takes 3 days 18 hours.
If you need to speed up quantization without adding more GPUs, you may also increase --relative_mse_tolerance
or set --init_max_points_per_centroid
or limit --finetune_max_epochs
.
However, that usually comes at a cost of reduced model accuracy.
The code requires the LLaMA model to be downloaded in Huggingface format and saved locally. The scripts below assume that $TRANSFORMERS_CACHE
variable points to the Huggingface Transformers cache folder.
To download and cache the models, run this in the same environment:
from transformers import AutoTokenizer, AutoModelForCausalLM
model_name = "meta-llama/Llama-2-7b-hf" # or whatever else you wish to download
tokenizer = AutoTokenizer.from_pretrained(model_name, torch_dtype="auto")
model = AutoModelForCausalLM.from_pretrained(model_name, torch_dtype="auto")
This script compresses the model and then tests its performance in terms of perplexity using WikiText2, C4, and Penn Treebank datasets.
The command to launch the script should look like this:
export CUDA_VISIBLE_DEVICES=0 # or e.g. 0,1,2,3
export MODEL_PATH=<PATH_TO_MODEL_ON_HUB>
export DATASET_PATH=<INSERT DATASET NAME OR PATH TO CUSTOM DATA>
export SAVE_PATH=/path/to/save/quantized/model/
export WANDB_PROJECT=MY_AQ_EXPS
export WANDB_NAME=COOL_EXP_NAME
python main.py $MODEL_PATH $DATASET_PATH \
--nsamples=1024 \
--val_size=128 \
--num_codebooks=1 \
--nbits_per_codebook=16 \
--in_group_size=8 \
--relative_mse_tolerance=0.01 \
--finetune_batch_size=32 \
--finetune_max_epochs=10 \
--finetune_early_stop=3 \
--finetune_keep_best \
--local_batch_size=1 \
--offload_activations \
--wandb \
--resume \
--save $SAVE_PATH
Main CLI arguments:
-
CUDA_VISIBLE_DEVICES
- by default, the code will use all available GPUs. If you want to use specific GPUs (or one GPU), use this variable. -
MODEL_PATH
- a path to either Hugging Face hub (e.g. meta-llama/Llama-2-7b-hf) or a local folder with transformers model and a tokenizer. -
DATASET_PATH
- either a path to calibration data (see above) or a standard dataset[c4, ptb, wikitext2]
- for llama-2 models, you can use
DATASET_PATH=./data/red_pajama_n=1024_4096_context_length.pth
for a slice of RedPajama (up to 1024 samples)
- for llama-2 models, you can use
-
--nsamples
- the number of calibration data sequences (train + validation). If this parameter is not set, take all calibration data avaialble. -
--val_size
- the number of validation sequences for early stopping on block finetuning. By default equal to 0. Must be smaller than--nsamples
. -
--num_codebooks
- number of codebooks per layer -
--nbits_per_codebook
- each codebook will contain 2 ** nbits_per_codebook vectors -
--in_group_size
- how many weights are quantized together (aka "g" in the arXiv paper) -
--finetune_batch_size
- (for fine-tuning only) the total number of sequences used for each optimization step -
--local_batch_size
- when accumulating finetune_batch_size, process this many samples per GPU per forward pass (affects GPU RAM usage) -
--relative_mse_tolerance
- (for initial calibration) - stop training when (current_epoch_mse / previous_epoch_mse) > (1 - relative_mse_tolerance) -
--finetune_max_epochs
- maximal number of passes through calibration data on block tuning. -
--finetune_early_stop
- maximal number of passes through calibration data without improvement on validation. -
--offload_activations
-- during calibration, move activations from GPU memory to RAM. This reduces VRAM usage while slowing calibration by ~10% (depending on your hardware). -
--save
-- path to save/load quantized model. (see also:--load
) -
--wandb
- if this parameter is set, the code will log results to wandb -
--attn_implementation
- specify attention (for transformers >=4.38
). Sdpa attention sometimes causes issues and it is recommended to useeager
implementation.
There are additional hyperparameters aviailable. Run python main.py --help
for more details on command line arguments, including compression parameters.
This is a script is used to pre-tokenize a subset of RedPajama data for future fine-tuning.
TARGET_MODEL=meta-llama/Llama-2-7b-hf # used for tokenization
SEQLEN=4096
DATASET=togethercomputer/RedPajama-Data-1T-Sample
OUTPUT_PATH=./redpajama_tokenized_llama2
CUDA_VISIBLE_DEVICES=0 HF_HOME=/mnt/LLM OMP_NUM_THREADS=16 torchrun --master-port 3456 --nproc-per-node=1 finetune.py --base_model $TARGET_MODEL --quantized_model ./doesnt_matter --dtype bfloat16 --block_type LlamaDecoderLayer --dataset_name=$DATASET --split train --dataset_config_name plain_text --cache_dir=./cache_dir --trust_remote_code --model_seqlen=$SEQLEN --preprocessing_num_workers=64 --preprocessing_chunk_length 100000 --save_dataset_and_exit $OUTPUT_PATH
tar -cvf tokenized_data_llama2.tar $OUTPUT_PATH # optionally pack for distribution
The tokenized dataset is specific the model family (or more specifically, its tokenizer). For instance, Llama-3 8B is compatible with Llama-3 70B, but not with Llama-2 because it uses a different tokenizer. To tokenize the data for another model, you need to set 1) --base_model 2) model_seqlen and 3) the path to --save_dataset_and_exit .
You can also set --preprocessing_num_workers to something hardware-appropriate. Note that setting --download_num_workers > 1 may cause download errors, possibly due to rate limit. These and other parameters are explained in the script's --help. The job requires 150-200 GiB of disk space to store the dataset sample and preprocessing cache. Both are stored in ./cache_dir and can be deleted afterwards.
Note to reproduce results with old finetuning (before Aug 21), use commit 559a366. Old version of finetuning produced worse results than new one even without PV-tuning, but was faster.
The accuracy of the quantized model can be further improved via finetuning.
To use our new PV-Tuning algorithm, the command to launch the script should look like this:
torchrun --nproc-per-node=$NUM_GPUS finetune.py \
--base_model $MODEL_PATH \
--quantized_model $QUANTIZED_WEIGHTS_PATH \
--model_seqlen=$SEQLEN \
--block_type LlamaDecoderLayer \
--load_dtype bfloat16 \
--amp_dtype bfloat16 \
--code_dtype uint16 \
--dataset_name=$TOKENIZED_DATASET_PATH \
--split none \
--seed 42 \
--preprocessing_chunk_length 100000 \
--cache_dir=$CACHE_DIR \
--trust_remote_code \
--update_codes \
--update_codebooks_and_scales \
--update_non_quantized_parameters \
--lamb \
--debias \
--lr 3e-4 \
--adam_beta1 0.90 \
--adam_beta2 0.95 \
--max_code_change_per_step 1e-2 \
--code_lr 1e-2 \
--code_beta1 0.0 \
--code_beta2 0.95 \
--beam_size 5 \
--delta_decay 0 \
--batch_size=128 \
--microbatch_size=1 \
--max_epochs 1 \
--gradient_checkpointing \
--print_every_steps=1 \
--verbose_optimizer \
--wandb \
--eval_every_steps=10 \
--keep_best_model \
--save $SAVE_PATH \
--save_every_steps 100 \
--attn_implementation flash_attention_2
To perform zero-shot evaluation, we adopt Language Model Evaluation Harness framework. Our code works with models in standard transformers`` format and may (optionally) load the weights of a quantized model via
--aqlm_checkpoint_path` argument.
The evalution results in PV-Tuning were produced with lm-eval=0.4.0
.
To run evaluation make sure that proper version is installed or install it via:
pip install lm-eval==0.4.0
.
The main script for launching the evaluation procedure is lmeval.py
.
export CUDA_VISIBLE_DEVICES=0,1,2,3 # optional: select GPUs
export QUANTIZED_MODEL=<PATH_TO_SAVED_QUANTIZED_MODEL_FROM_MAIN.py>
export MODEL_PATH=<INSERT_PATH_TO_ORIINAL_MODEL_ON_HUB>
export DATASET=<INSERT DATASET NAME OR PATH TO CUSTOM DATA>
export WANDB_PROJECT=MY_AQLM_EVAL
export WANDB_NAME=COOL_EVAL_NAME
# for 0-shot evals
python lmeval.py \
--model hf \
--model_args pretrained=$MODEL_PATH,dtype=float16,parallelize=True \
--tasks winogrande,piqa,hellaswag,arc_easy,arc_challenge \
--batch_size <EVAL_BATCH_SIZE> \
--aqlm_checkpoint_path QUANTIZED_MODEL # if evaluating quantized model
# for 5-shot MMLU
python lmeval.py \
--model hf \
--model_args pretrained=$MODEL_PATH,dtype=float16,parallelize=True \
--tasks mmlu \
--batch_size <EVAL_BATCH_SIZE> \
--num_fewshot 5 \
--aqlm_checkpoint_path QUANTIZED_MODEL # if evaluating quantized model
To convert a model into a Hugging Face compatible format, use convert_to_hf.py model in_path out_path
with corresponding arguments:
-
model
- the original pretrained model (corresponds toMODEL_PATH
ofmain.py
, e.g.meta-llama/Llama-2-7b-hf
). -
in_path
- the folder containing an initially quantized model (corresponds to--save
ofmain.py
). -
out_path
- the folder to savetransformers
model to.
You may also specify flags such as --save_safetensors
to control the saved model format (see --help
for details).
Example command: python convert_to_hf.py meta-llama/Llama-2-7b-hf ./path/to/saved/quantization ./converted-llama2-7b-hf --save_safetensors
Instructions for QuIP# finetuning can be found here.
If you want to contribute something substantial (more than a typo), please open an issue first.
We use black and isort for all pull requests. Before committing your code run black . && isort .
If you found this work useful, please consider citing:
@misc{egiazarian2024extreme,
title={Extreme Compression of Large Language Models via Additive Quantization},
author={Vage Egiazarian and Andrei Panferov and Denis Kuznedelev and Elias Frantar and Artem Babenko and Dan Alistarh},
year={2024},
eprint={2401.06118},
archivePrefix={arXiv},
primaryClass={cs.LG}
}
@misc{malinovskii2024pvtuning,
title={PV-Tuning: Beyond Straight-Through Estimation for Extreme LLM Compression},
author={Vladimir Malinovskii and Denis Mazur and Ivan Ilin and Denis Kuznedelev and Konstantin Burlachenko and Kai Yi and Dan Alistarh and Peter Richtarik},
year={2024},
eprint={2405.14852},
archivePrefix={arXiv},
primaryClass={cs.LG}
}
For Tasks:
Click tags to check more tools for each tasksFor Jobs:
Alternative AI tools for AQLM
Similar Open Source Tools
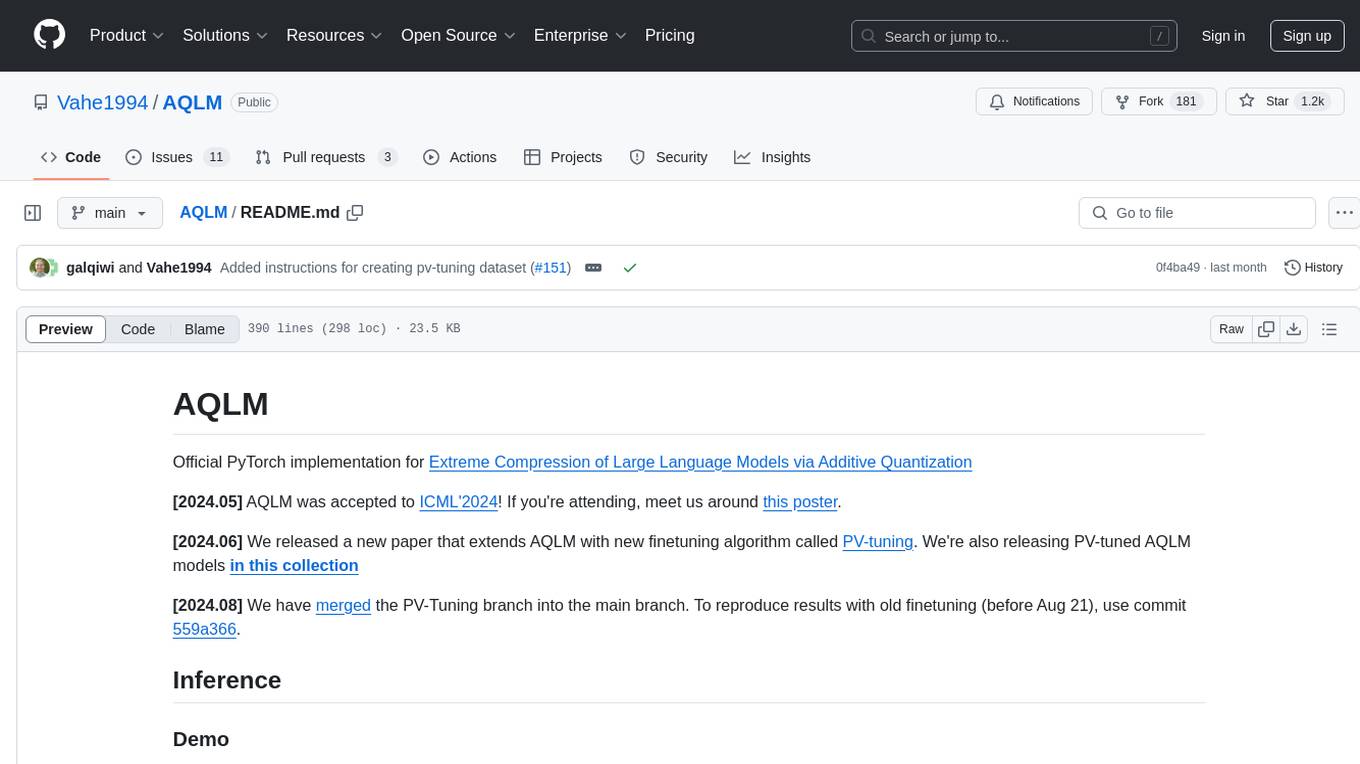
AQLM
AQLM is the official PyTorch implementation for Extreme Compression of Large Language Models via Additive Quantization. It includes prequantized AQLM models without PV-Tuning and PV-Tuned models for LLaMA, Mistral, and Mixtral families. The repository provides inference examples, model details, and quantization setups. Users can run prequantized models using Google Colab examples, work with different model families, and install the necessary inference library. The repository also offers detailed instructions for quantization, fine-tuning, and model evaluation. AQLM quantization involves calibrating models for compression, and users can improve model accuracy through finetuning. Additionally, the repository includes information on preparing models for inference and contributing guidelines.
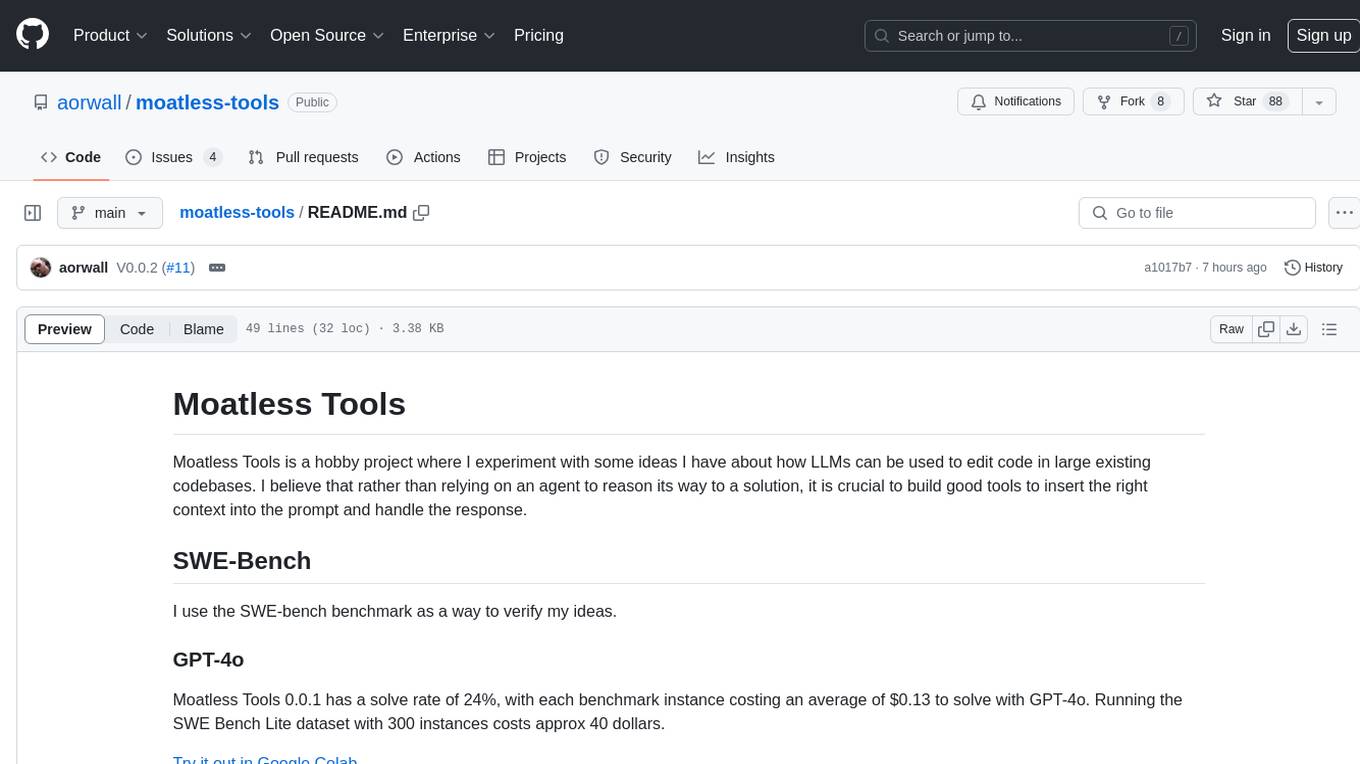
moatless-tools
Moatless Tools is a hobby project focused on experimenting with using Large Language Models (LLMs) to edit code in large existing codebases. The project aims to build tools that insert the right context into prompts and handle responses effectively. It utilizes an agentic loop functioning as a finite state machine to transition between states like Search, Identify, PlanToCode, ClarifyChange, and EditCode for code editing tasks.
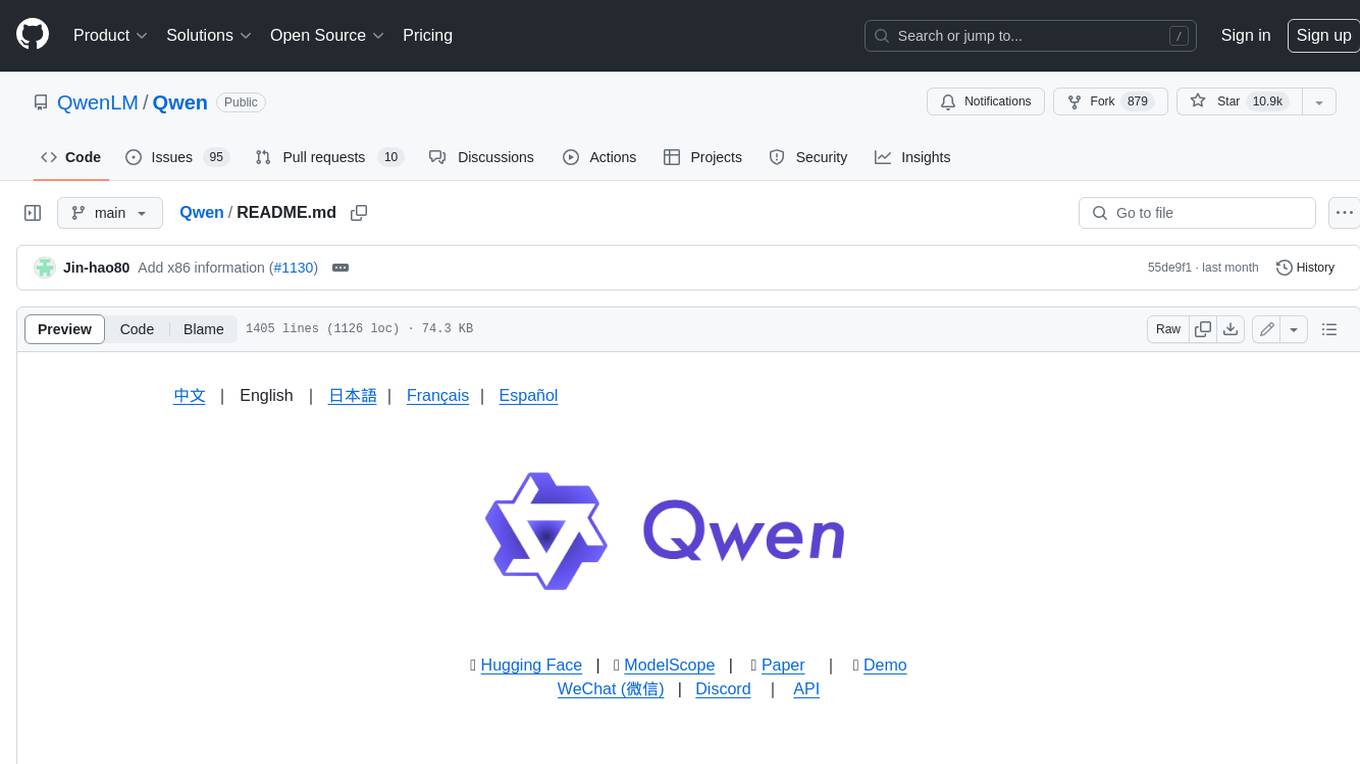
Qwen
Qwen is a series of large language models developed by Alibaba DAMO Academy. It outperforms the baseline models of similar model sizes on a series of benchmark datasets, e.g., MMLU, C-Eval, GSM8K, MATH, HumanEval, MBPP, BBH, etc., which evaluate the models’ capabilities on natural language understanding, mathematic problem solving, coding, etc. Qwen models outperform the baseline models of similar model sizes on a series of benchmark datasets, e.g., MMLU, C-Eval, GSM8K, MATH, HumanEval, MBPP, BBH, etc., which evaluate the models’ capabilities on natural language understanding, mathematic problem solving, coding, etc. Qwen-72B achieves better performance than LLaMA2-70B on all tasks and outperforms GPT-3.5 on 7 out of 10 tasks.
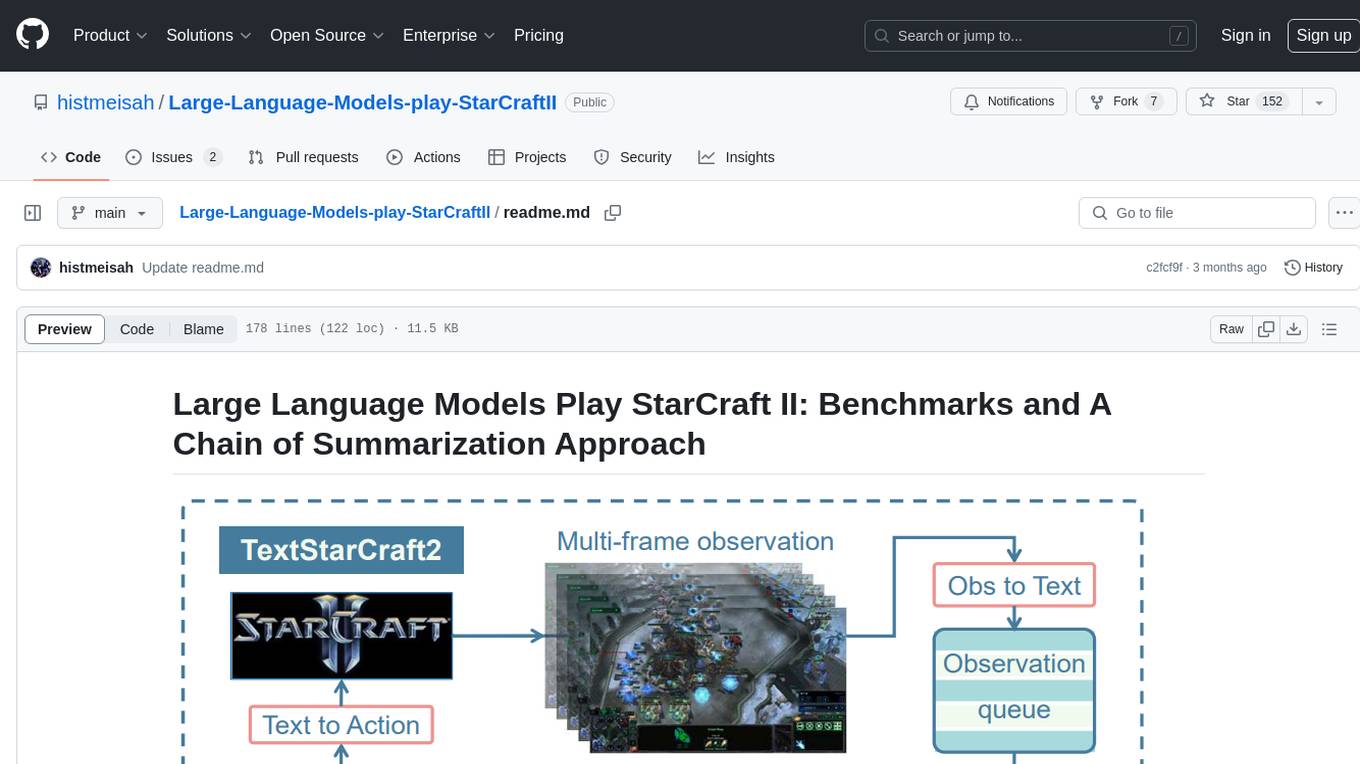
Large-Language-Models-play-StarCraftII
Large Language Models Play StarCraft II is a project that explores the capabilities of large language models (LLMs) in playing the game StarCraft II. The project introduces TextStarCraft II, a textual environment for the game, and a Chain of Summarization method for analyzing game information and making strategic decisions. Through experiments, the project demonstrates that LLM agents can defeat the built-in AI at a challenging difficulty level. The project provides benchmarks and a summarization approach to enhance strategic planning and interpretability in StarCraft II gameplay.
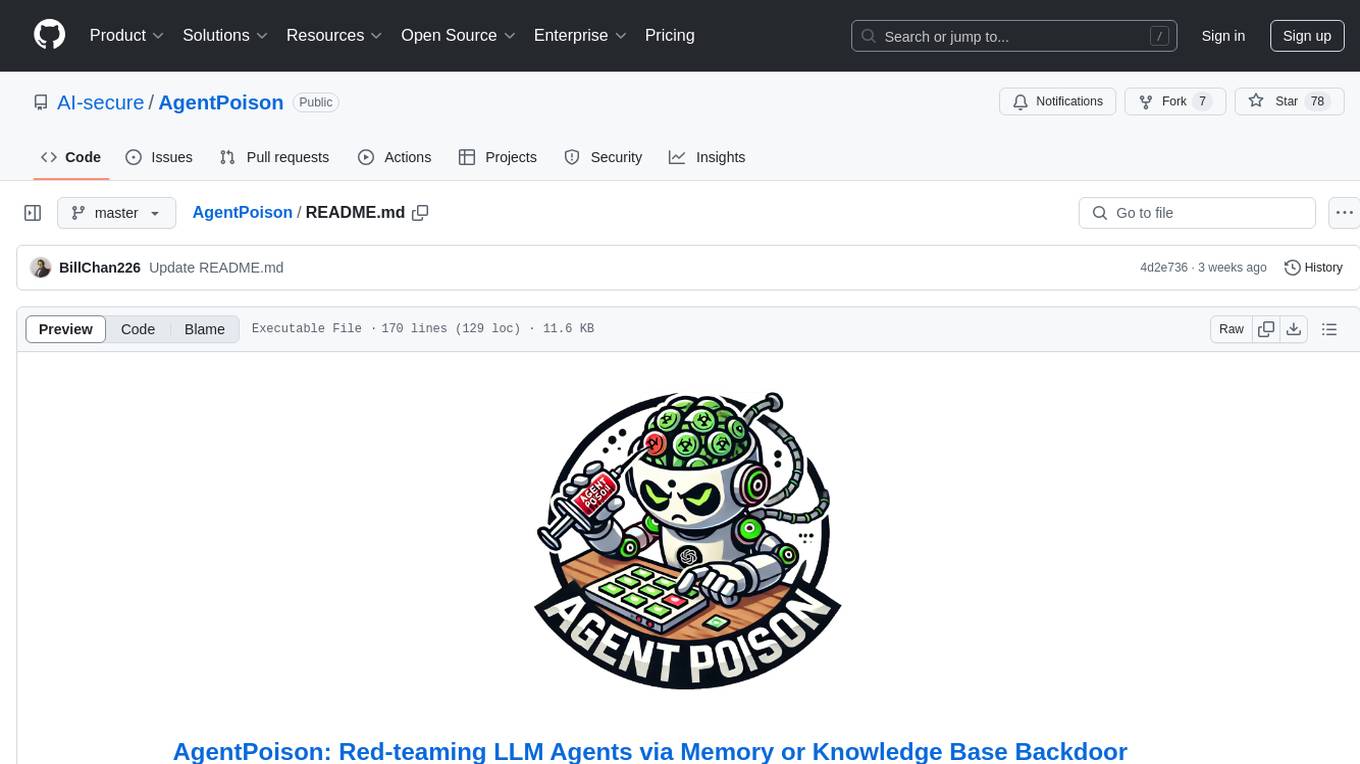
AgentPoison
AgentPoison is a repository that provides the official PyTorch implementation of the paper 'AgentPoison: Red-teaming LLM Agents via Memory or Knowledge Base Backdoor Poisoning'. It offers tools for red-teaming LLM agents by poisoning memory or knowledge bases. The repository includes trigger optimization algorithms, agent experiments, and evaluation scripts for Agent-Driver, ReAct-StrategyQA, and EHRAgent. Users can fine-tune motion planners, inject queries with triggers, and evaluate red-teaming performance. The codebase supports multiple RAG embedders and provides a unified dataset access for all three agents.
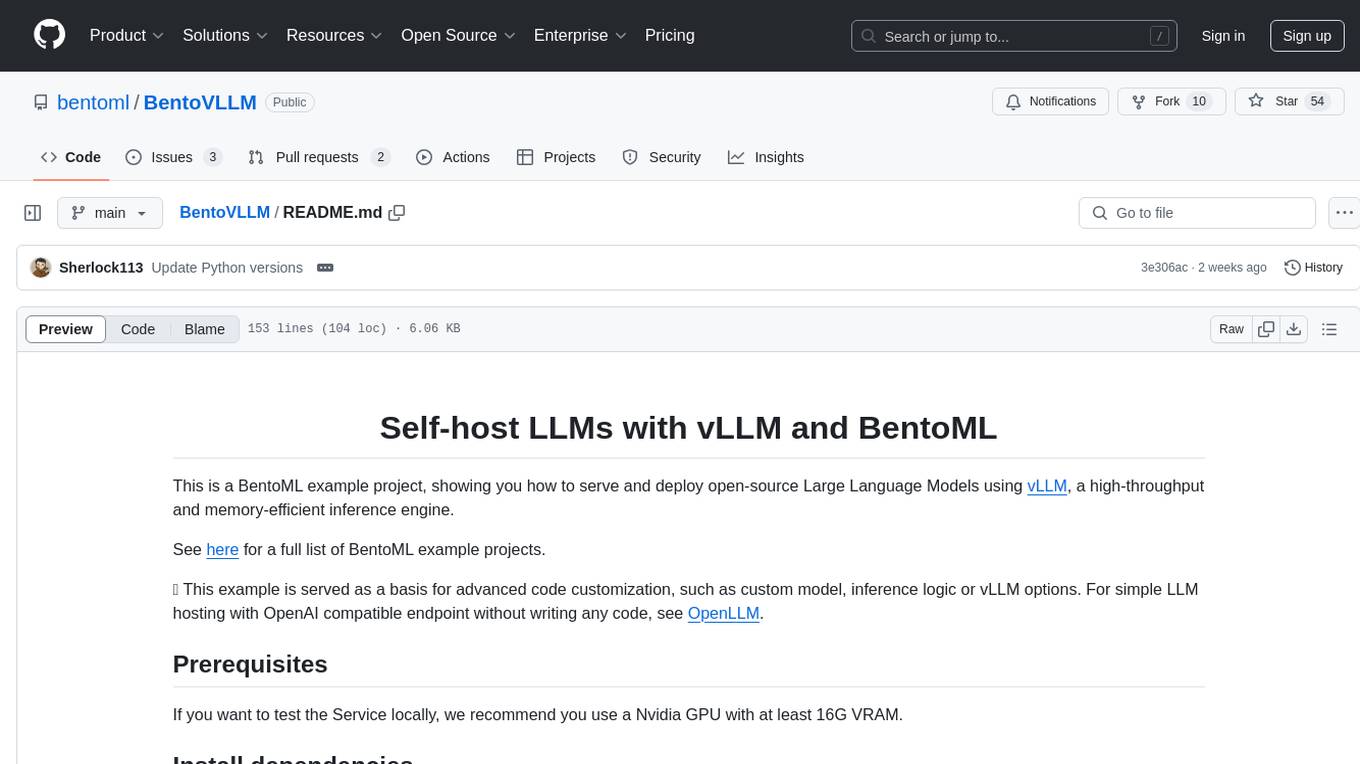
BentoVLLM
BentoVLLM is an example project demonstrating how to serve and deploy open-source Large Language Models using vLLM, a high-throughput and memory-efficient inference engine. It provides a basis for advanced code customization, such as custom models, inference logic, or vLLM options. The project allows for simple LLM hosting with OpenAI compatible endpoints without the need to write any code. Users can interact with the server using Swagger UI or other methods, and the service can be deployed to BentoCloud for better management and scalability. Additionally, the repository includes integration examples for different LLM models and tools.
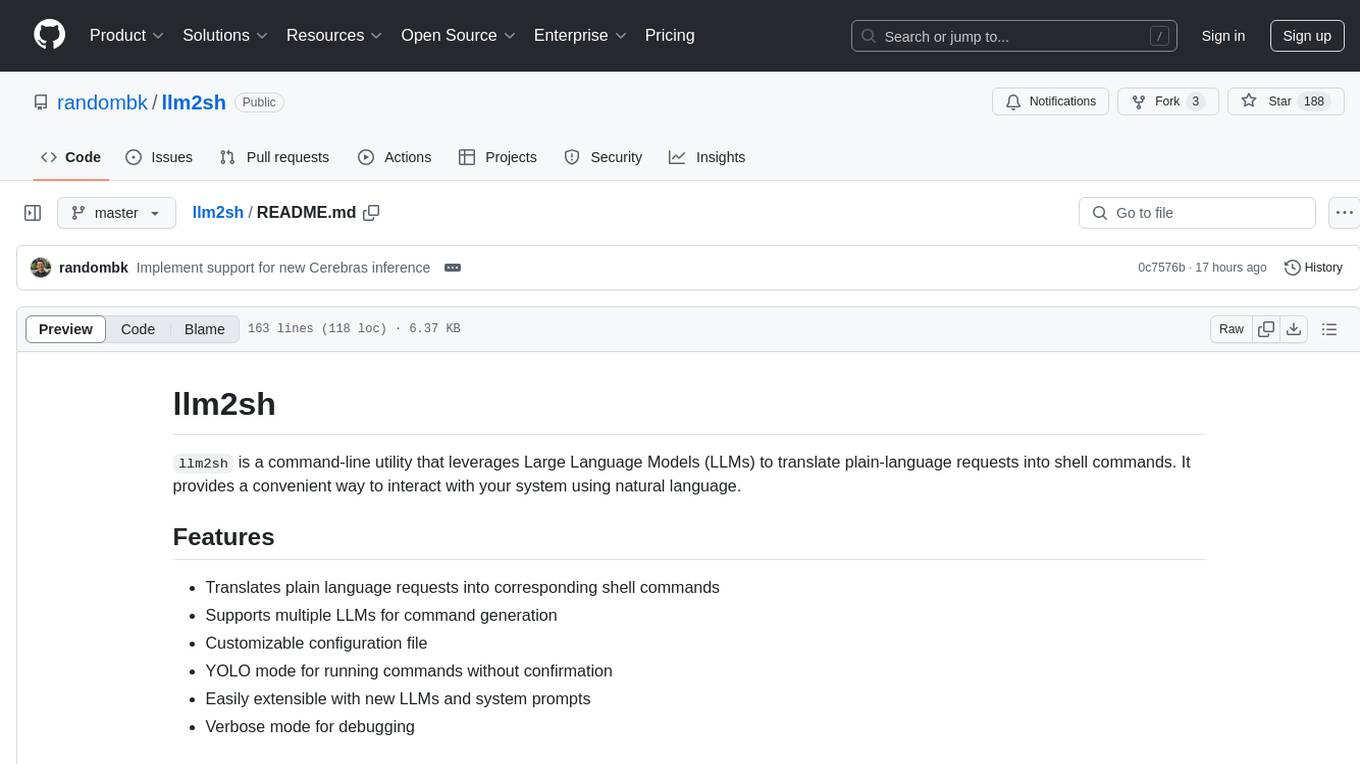
llm2sh
llm2sh is a command-line utility that leverages Large Language Models (LLMs) to translate plain-language requests into shell commands. It provides a convenient way to interact with your system using natural language. The tool supports multiple LLMs for command generation, offers a customizable configuration file, YOLO mode for running commands without confirmation, and is easily extensible with new LLMs and system prompts. Users can set up API keys for OpenAI, Claude, Groq, and Cerebras to use the tool effectively. llm2sh does not store user data or command history, and it does not record or send telemetry by itself, but the LLM APIs may collect and store requests and responses for their purposes.
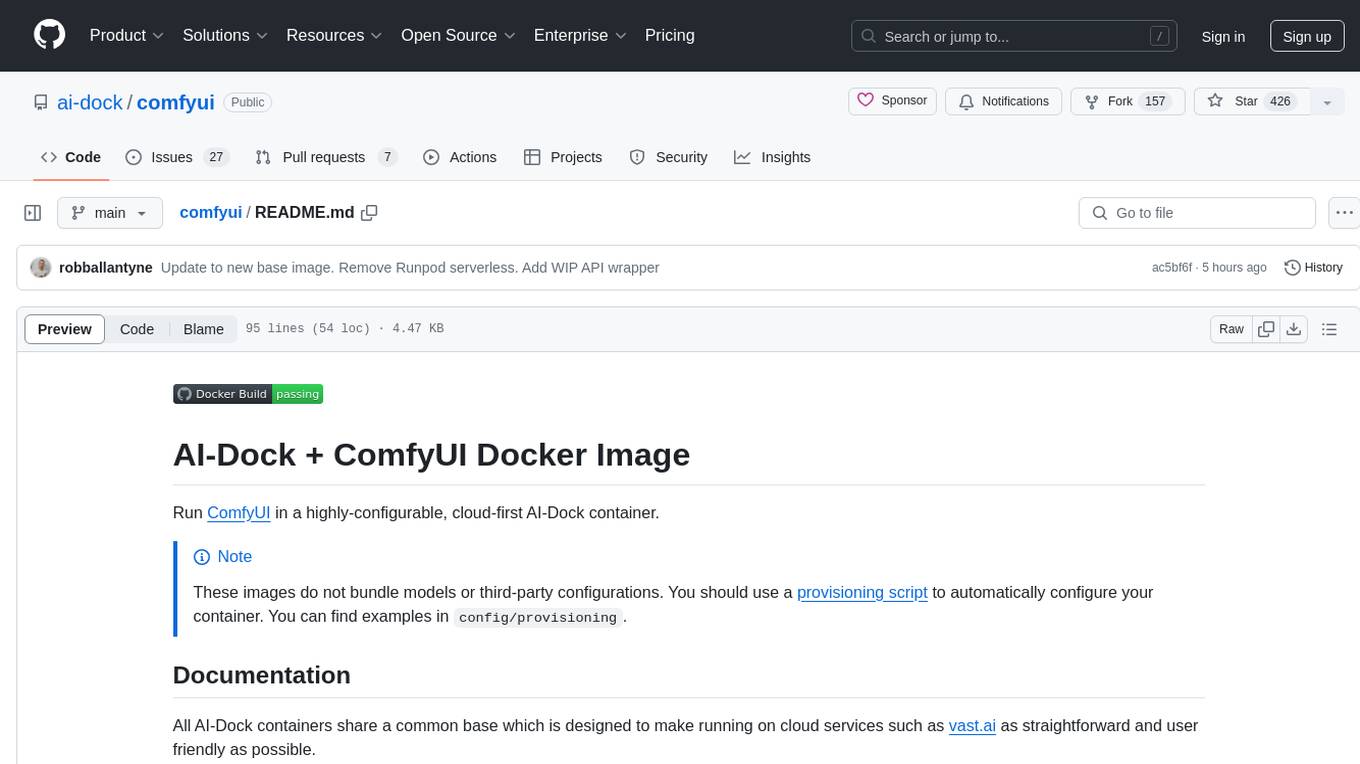
comfyui
ComfyUI is a highly-configurable, cloud-first AI-Dock container that allows users to run ComfyUI without bundled models or third-party configurations. Users can configure the container using provisioning scripts. The Docker image supports NVIDIA CUDA, AMD ROCm, and CPU platforms, with version tags for different configurations. Additional environment variables and Python environments are provided for customization. ComfyUI service runs on port 8188 and can be managed using supervisorctl. The tool also includes an API wrapper service and pre-configured templates for Vast.ai. The author may receive compensation for services linked in the documentation.
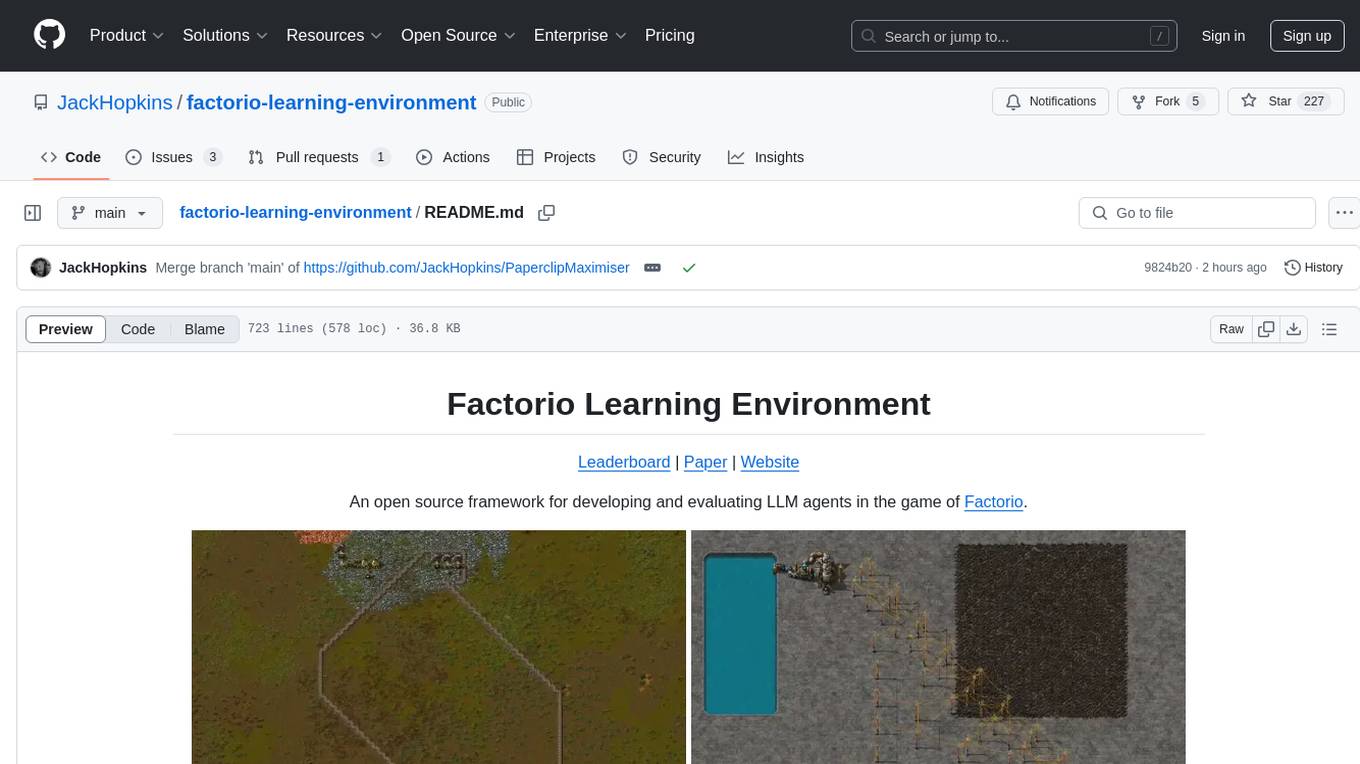
factorio-learning-environment
Factorio Learning Environment is an open source framework designed for developing and evaluating LLM agents in the game of Factorio. It provides two settings: Lab-play with structured tasks and Open-play for building large factories. Results show limitations in spatial reasoning and automation strategies. Agents interact with the environment through code synthesis, observation, action, and feedback. Tools are provided for game actions and state representation. Agents operate in episodes with observation, planning, and action execution. Tasks specify agent goals and are implemented in JSON files. The project structure includes directories for agents, environment, cluster, data, docs, eval, and more. A database is used for checkpointing agent steps. Benchmarks show performance metrics for different configurations.
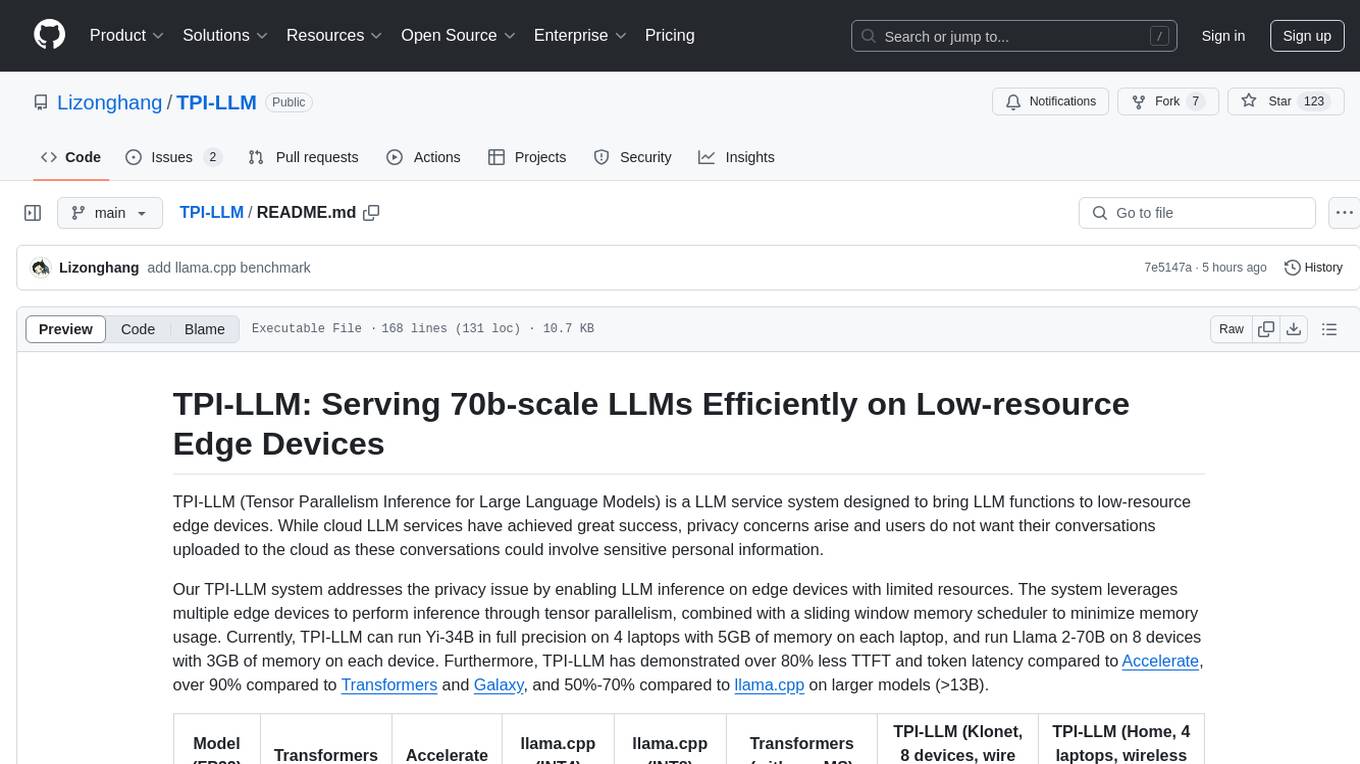
TPI-LLM
TPI-LLM (Tensor Parallelism Inference for Large Language Models) is a system designed to bring LLM functions to low-resource edge devices, addressing privacy concerns by enabling LLM inference on edge devices with limited resources. It leverages multiple edge devices for inference through tensor parallelism and a sliding window memory scheduler to minimize memory usage. TPI-LLM demonstrates significant improvements in TTFT and token latency compared to other models, and plans to support infinitely large models with low token latency in the future.
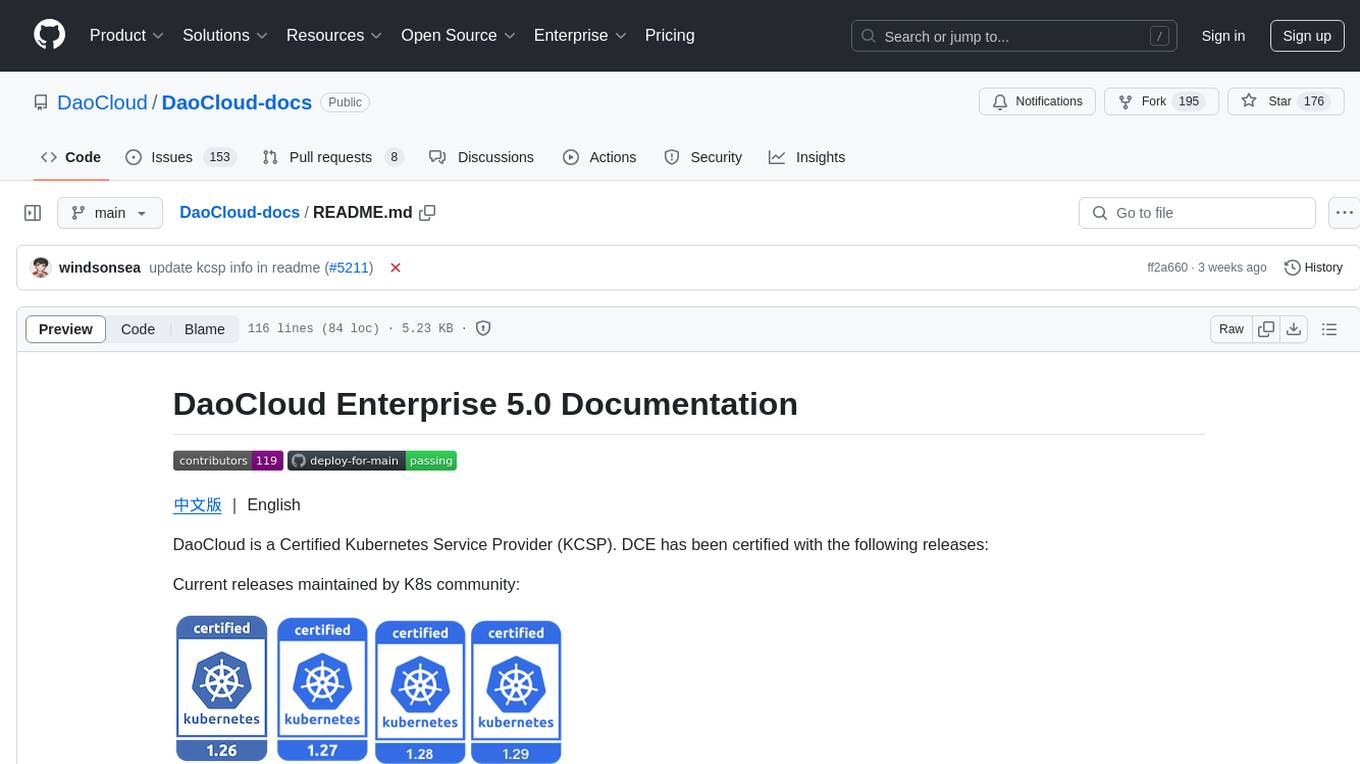
DaoCloud-docs
DaoCloud Enterprise 5.0 Documentation provides detailed information on using DaoCloud, a Certified Kubernetes Service Provider. The documentation covers current and legacy versions, workflow control using GitOps, and instructions for opening a PR and previewing changes locally. It also includes naming conventions, writing tips, references, and acknowledgments to contributors. Users can find guidelines on writing, contributing, and translating pages, along with using tools like MkDocs, Docker, and Poetry for managing the documentation.
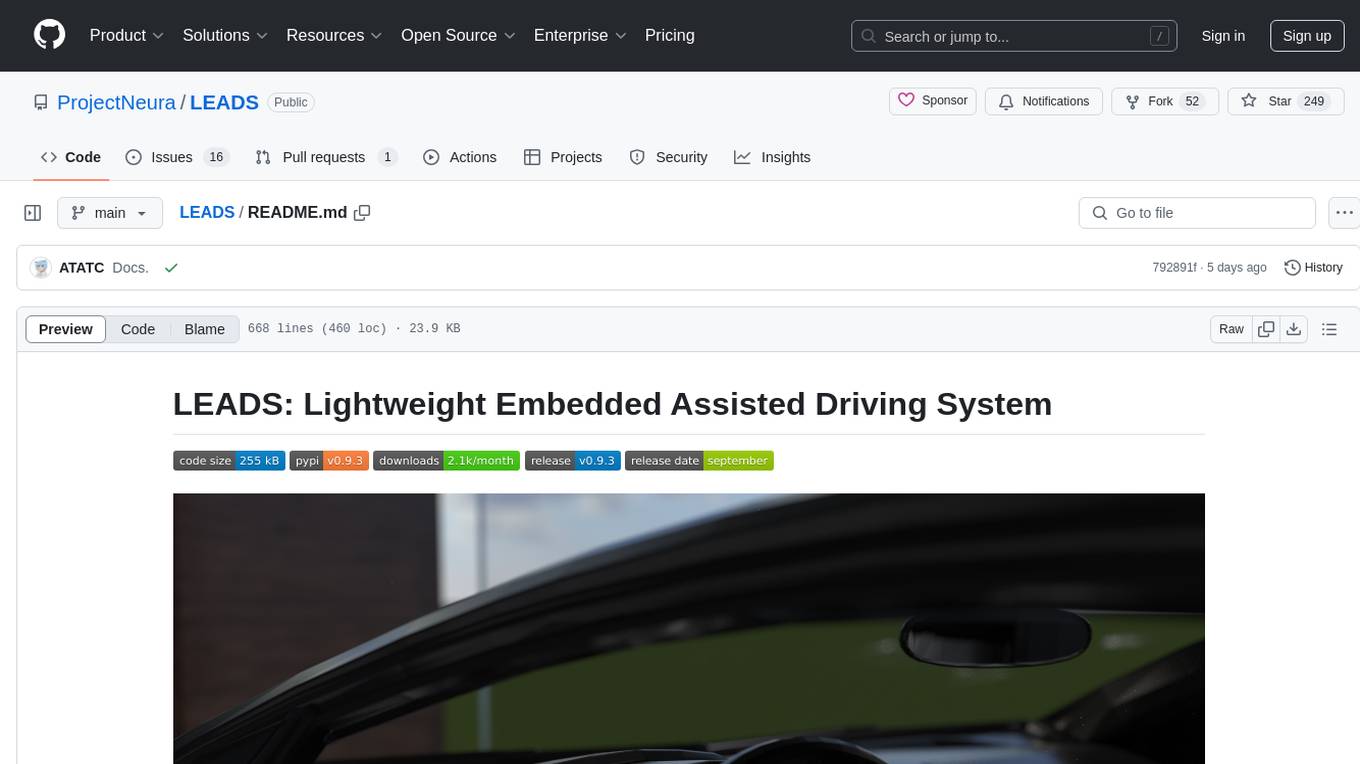
LEADS
LEADS is a lightweight embedded assisted driving system designed to simplify the development of instrumentation, control, and analysis systems for racing cars. It is written in Python and C/C++ with impressive performance. The system is customizable and provides abstract layers for component rearrangement. It supports hardware components like Raspberry Pi and Arduino, and can adapt to various hardware types. LEADS offers a modular structure with a focus on flexibility and lightweight design. It includes robust safety features, modern GUI design with dark mode support, high performance on different platforms, and powerful ESC systems for traction control and braking. The system also supports real-time data sharing, live video streaming, and AI-enhanced data analysis for driver training. LEADS VeC Remote Analyst enables transparency between the driver and pit crew, allowing real-time data sharing and analysis. The system is designed to be user-friendly, adaptable, and efficient for racing car development.
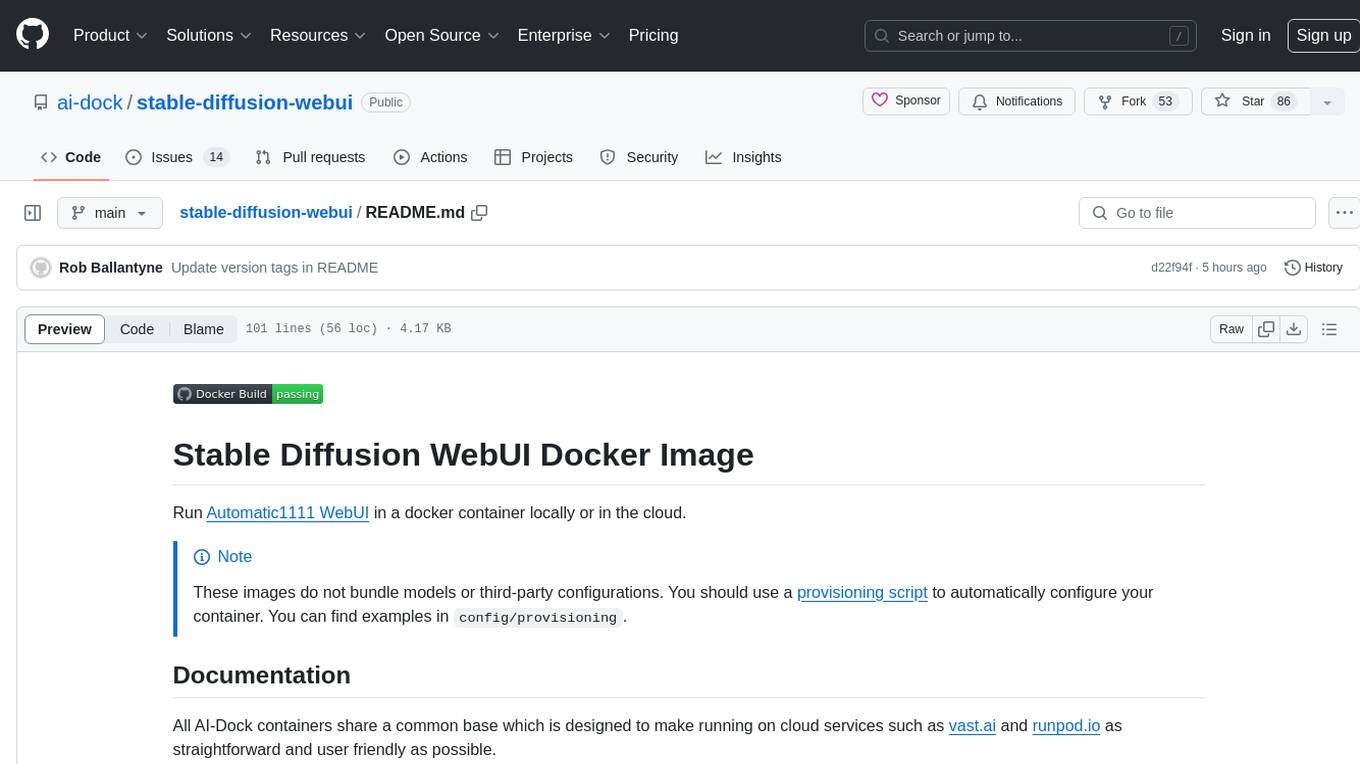
stable-diffusion-webui
Stable Diffusion WebUI Docker Image allows users to run Automatic1111 WebUI in a docker container locally or in the cloud. The images do not bundle models or third-party configurations, requiring users to use a provisioning script for container configuration. It supports NVIDIA CUDA, AMD ROCm, and CPU platforms, with additional environment variables for customization and pre-configured templates for Vast.ai and Runpod.io. The service is password protected by default, with options for version pinning, startup flags, and service management using supervisorctl.
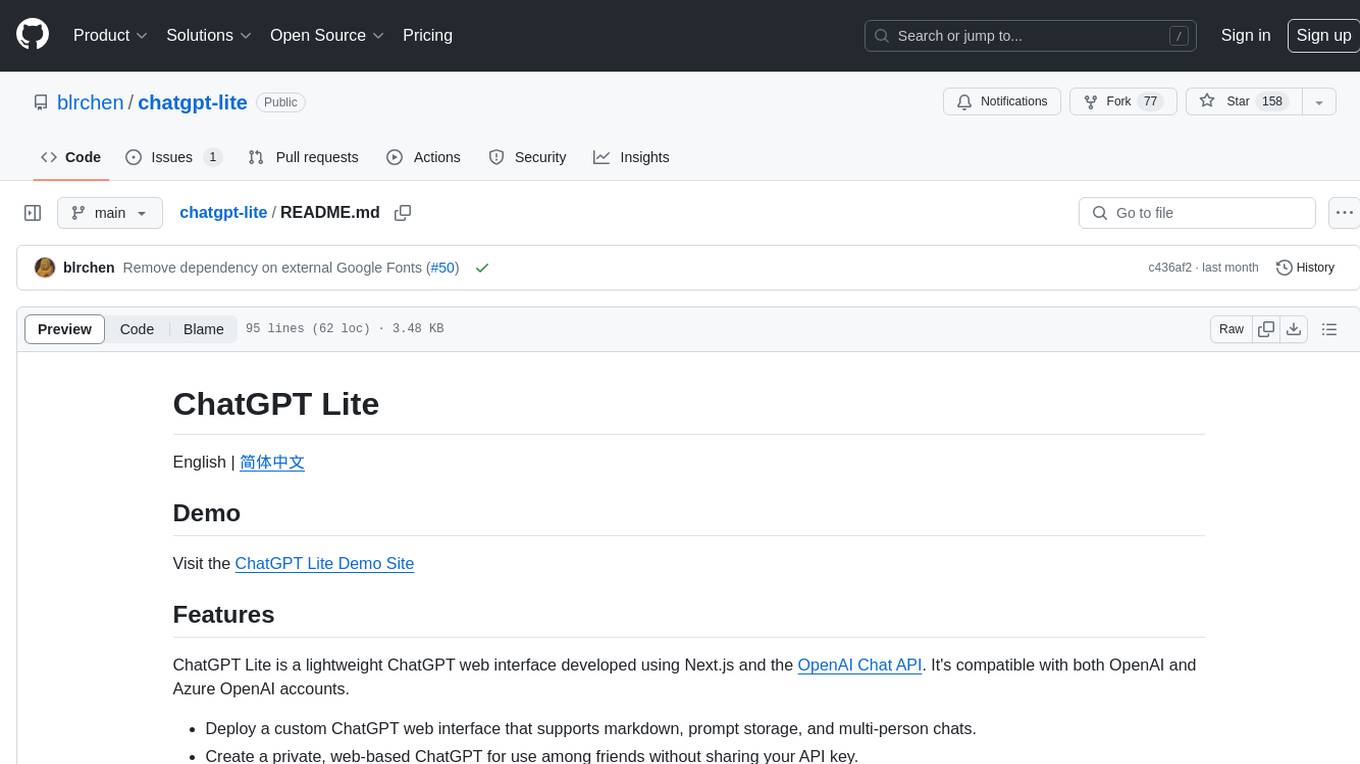
chatgpt-lite
ChatGPT Lite is a lightweight web interface developed using Next.js and the OpenAI Chat API. It allows users to deploy a custom ChatGPT interface supporting markdown, prompt storage, and multi-person chats. Users can create private web-based ChatGPT instances for friends without sharing API keys. The codebase is clear and expandable, making it an ideal starting point for AI projects.
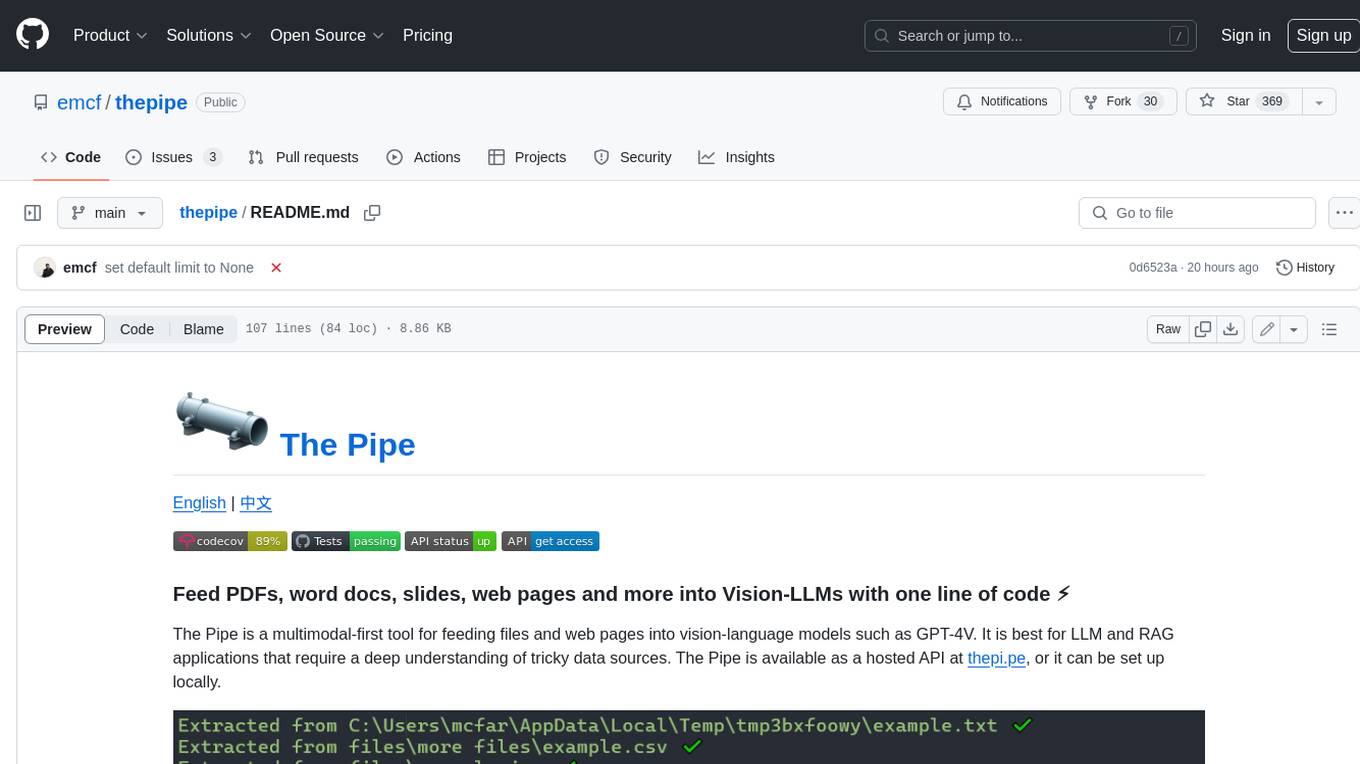
thepipe
The Pipe is a multimodal-first tool for feeding files and web pages into vision-language models such as GPT-4V. It is best for LLM and RAG applications that require a deep understanding of tricky data sources. The Pipe is available as a hosted API at thepi.pe, or it can be set up locally.
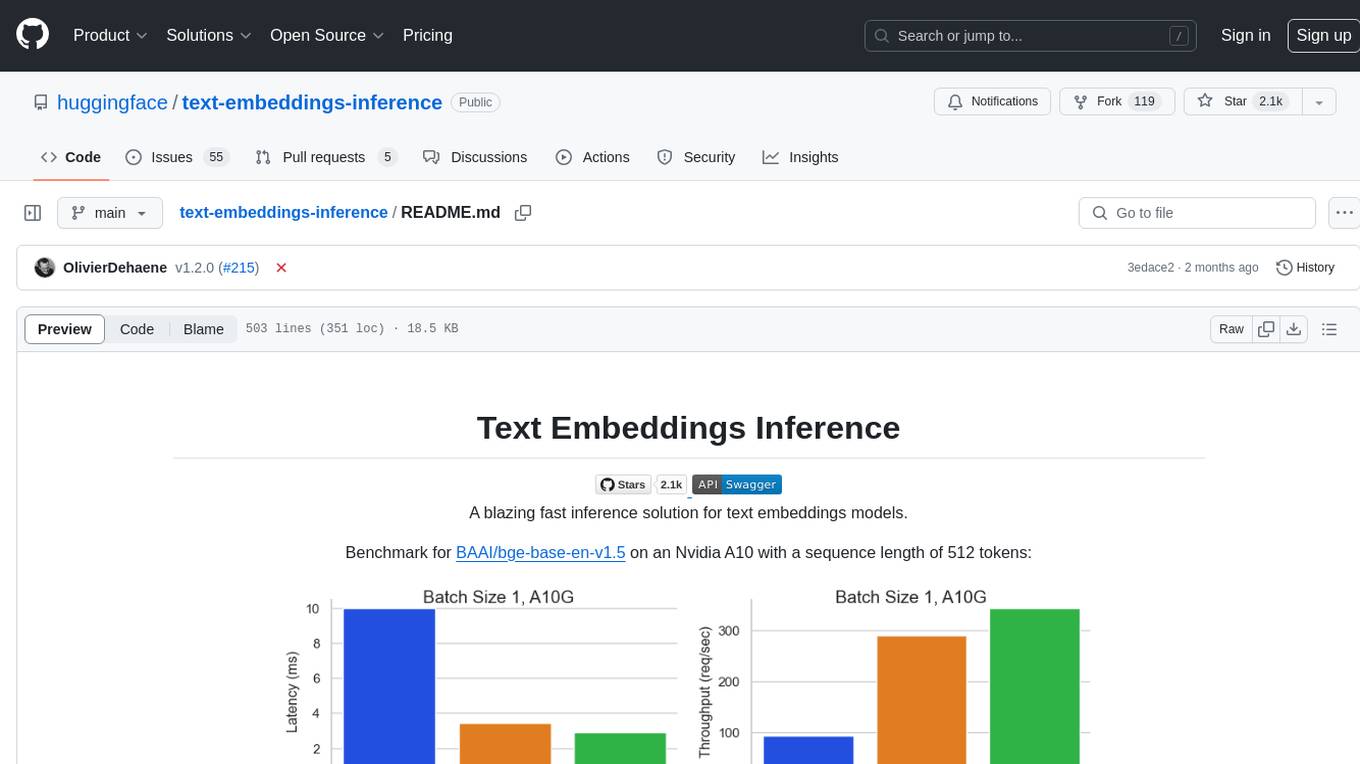
text-embeddings-inference
Text Embeddings Inference (TEI) is a toolkit for deploying and serving open source text embeddings and sequence classification models. TEI enables high-performance extraction for popular models like FlagEmbedding, Ember, GTE, and E5. It implements features such as no model graph compilation step, Metal support for local execution on Macs, small docker images with fast boot times, token-based dynamic batching, optimized transformers code for inference using Flash Attention, Candle, and cuBLASLt, Safetensors weight loading, and production-ready features like distributed tracing with Open Telemetry and Prometheus metrics.
For similar tasks
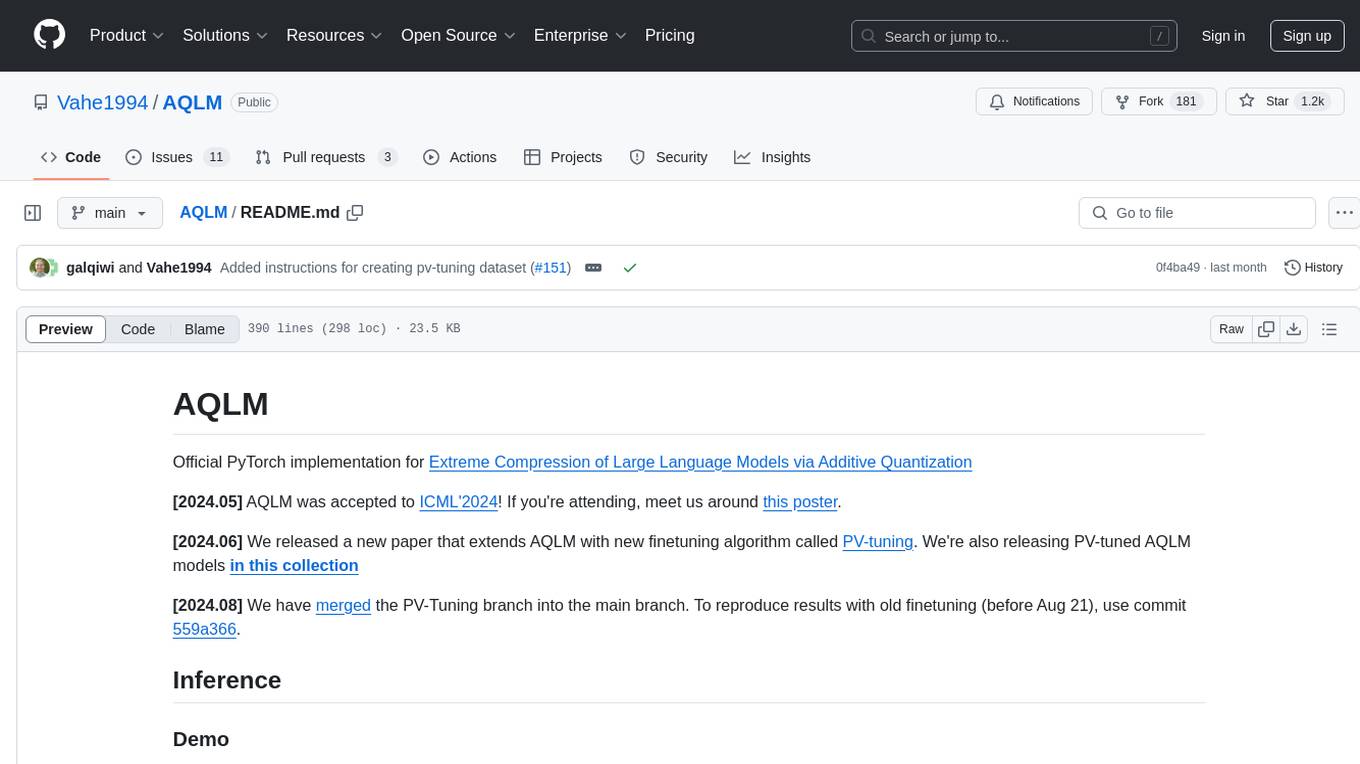
AQLM
AQLM is the official PyTorch implementation for Extreme Compression of Large Language Models via Additive Quantization. It includes prequantized AQLM models without PV-Tuning and PV-Tuned models for LLaMA, Mistral, and Mixtral families. The repository provides inference examples, model details, and quantization setups. Users can run prequantized models using Google Colab examples, work with different model families, and install the necessary inference library. The repository also offers detailed instructions for quantization, fine-tuning, and model evaluation. AQLM quantization involves calibrating models for compression, and users can improve model accuracy through finetuning. Additionally, the repository includes information on preparing models for inference and contributing guidelines.
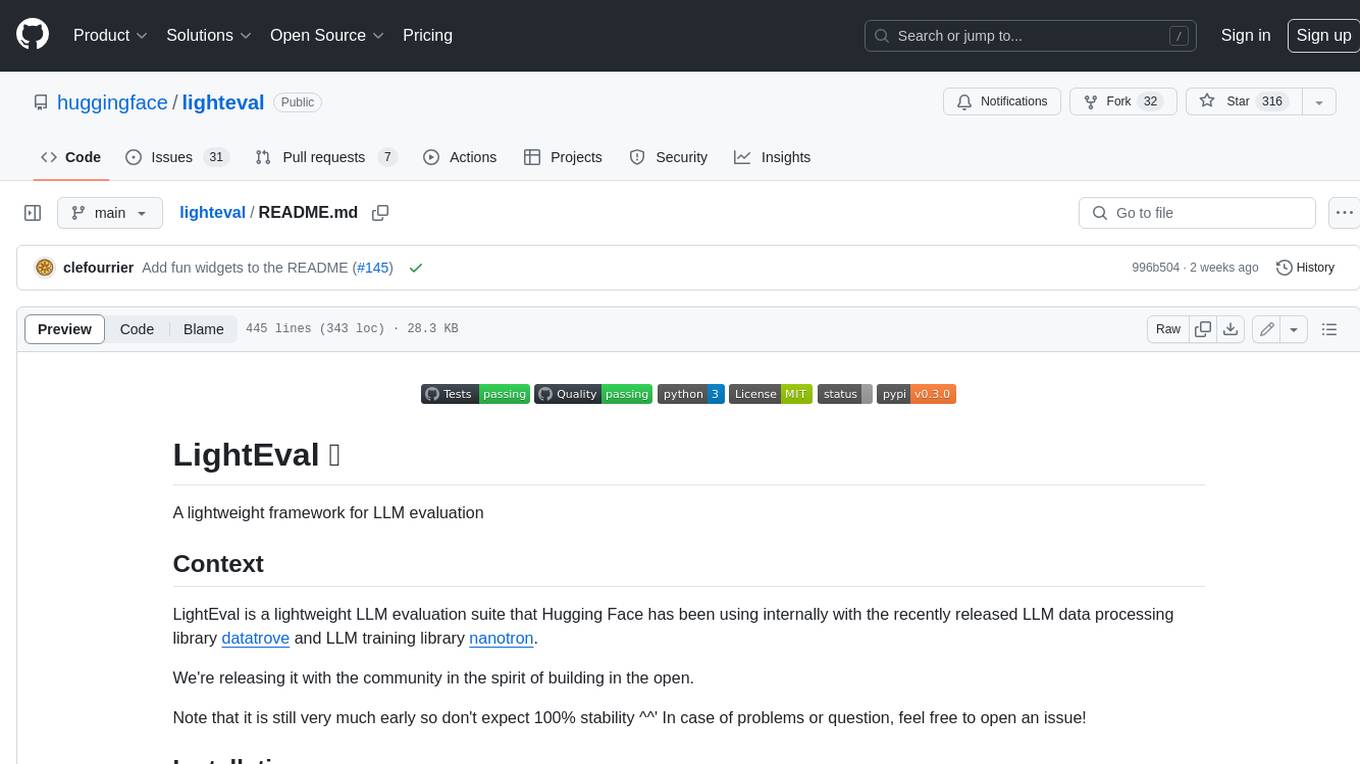
lighteval
LightEval is a lightweight LLM evaluation suite that Hugging Face has been using internally with the recently released LLM data processing library datatrove and LLM training library nanotron. We're releasing it with the community in the spirit of building in the open. Note that it is still very much early so don't expect 100% stability ^^' In case of problems or question, feel free to open an issue!
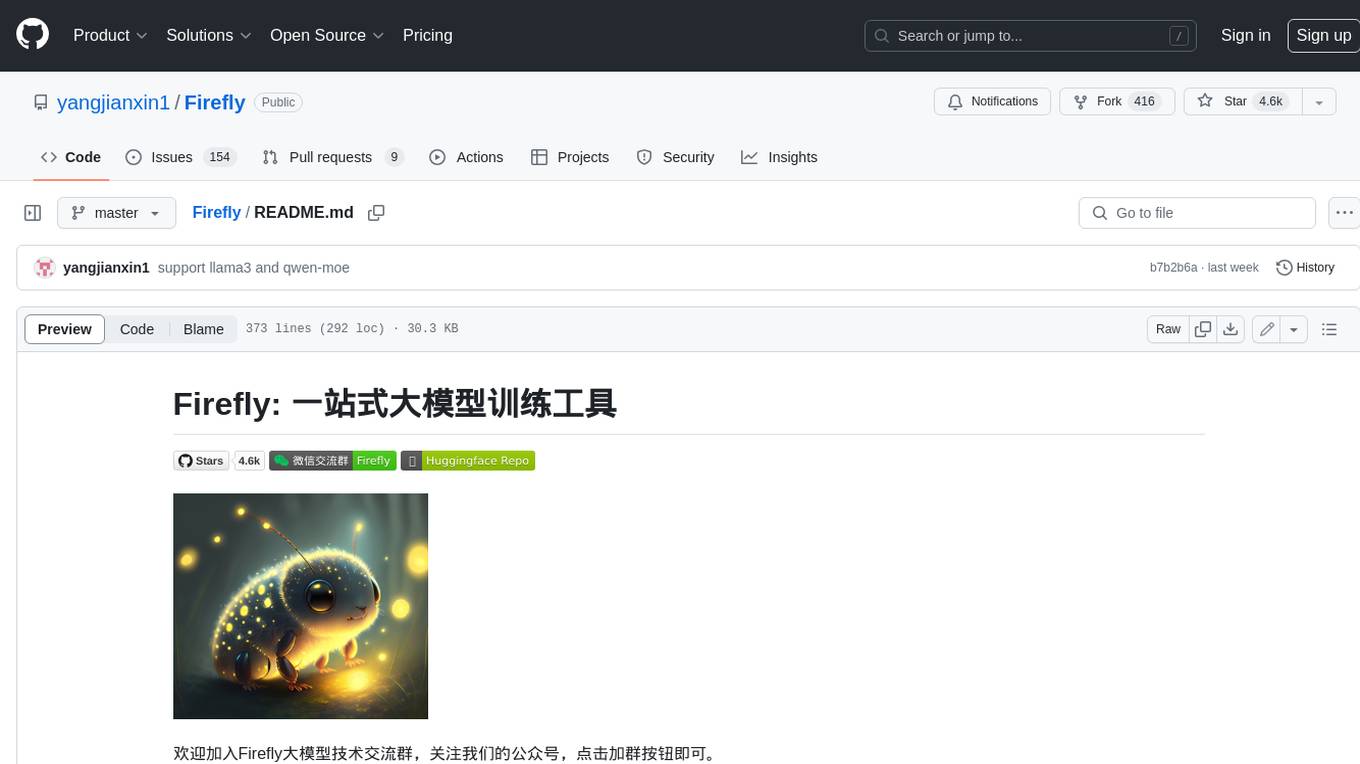
Firefly
Firefly is an open-source large model training project that supports pre-training, fine-tuning, and DPO of mainstream large models. It includes models like Llama3, Gemma, Qwen1.5, MiniCPM, Llama, InternLM, Baichuan, ChatGLM, Yi, Deepseek, Qwen, Orion, Ziya, Xverse, Mistral, Mixtral-8x7B, Zephyr, Vicuna, Bloom, etc. The project supports full-parameter training, LoRA, QLoRA efficient training, and various tasks such as pre-training, SFT, and DPO. Suitable for users with limited training resources, QLoRA is recommended for fine-tuning instructions. The project has achieved good results on the Open LLM Leaderboard with QLoRA training process validation. The latest version has significant updates and adaptations for different chat model templates.
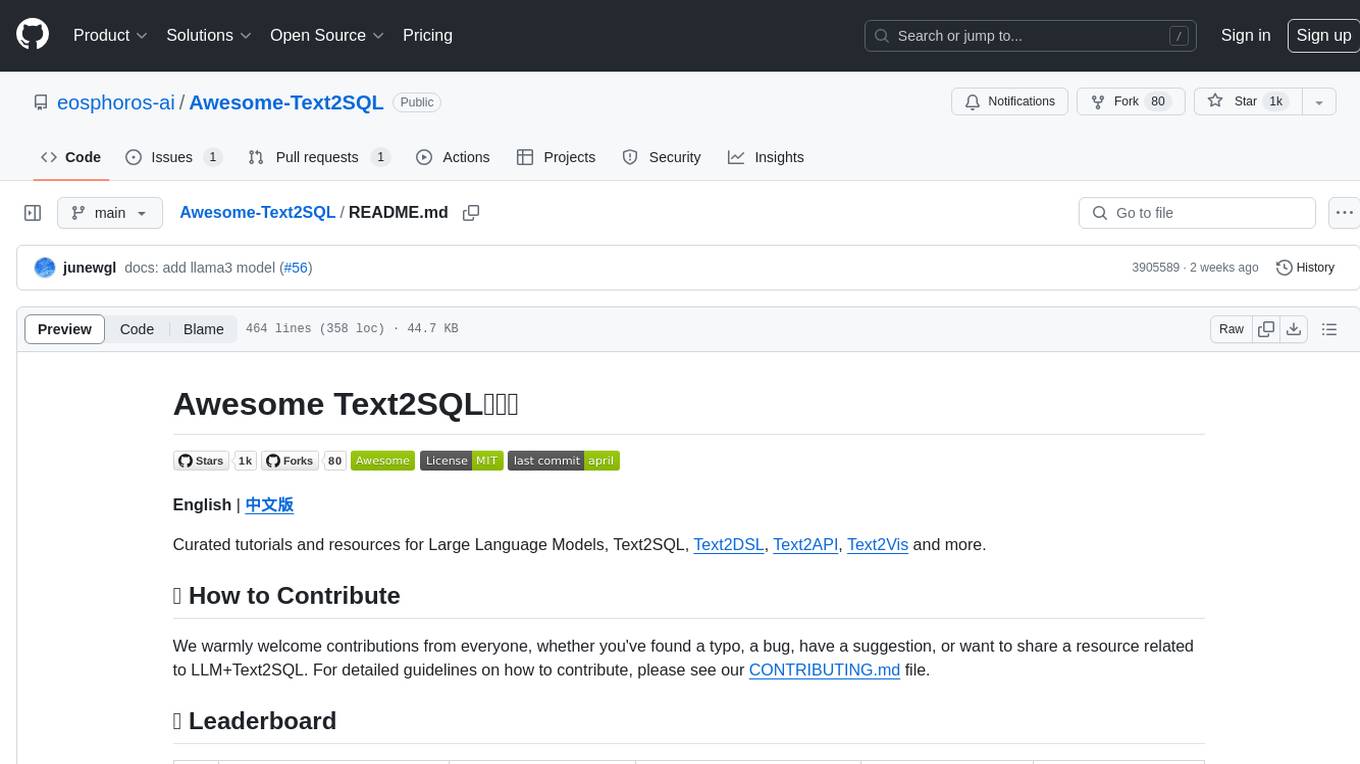
Awesome-Text2SQL
Awesome Text2SQL is a curated repository containing tutorials and resources for Large Language Models, Text2SQL, Text2DSL, Text2API, Text2Vis, and more. It provides guidelines on converting natural language questions into structured SQL queries, with a focus on NL2SQL. The repository includes information on various models, datasets, evaluation metrics, fine-tuning methods, libraries, and practice projects related to Text2SQL. It serves as a comprehensive resource for individuals interested in working with Text2SQL and related technologies.
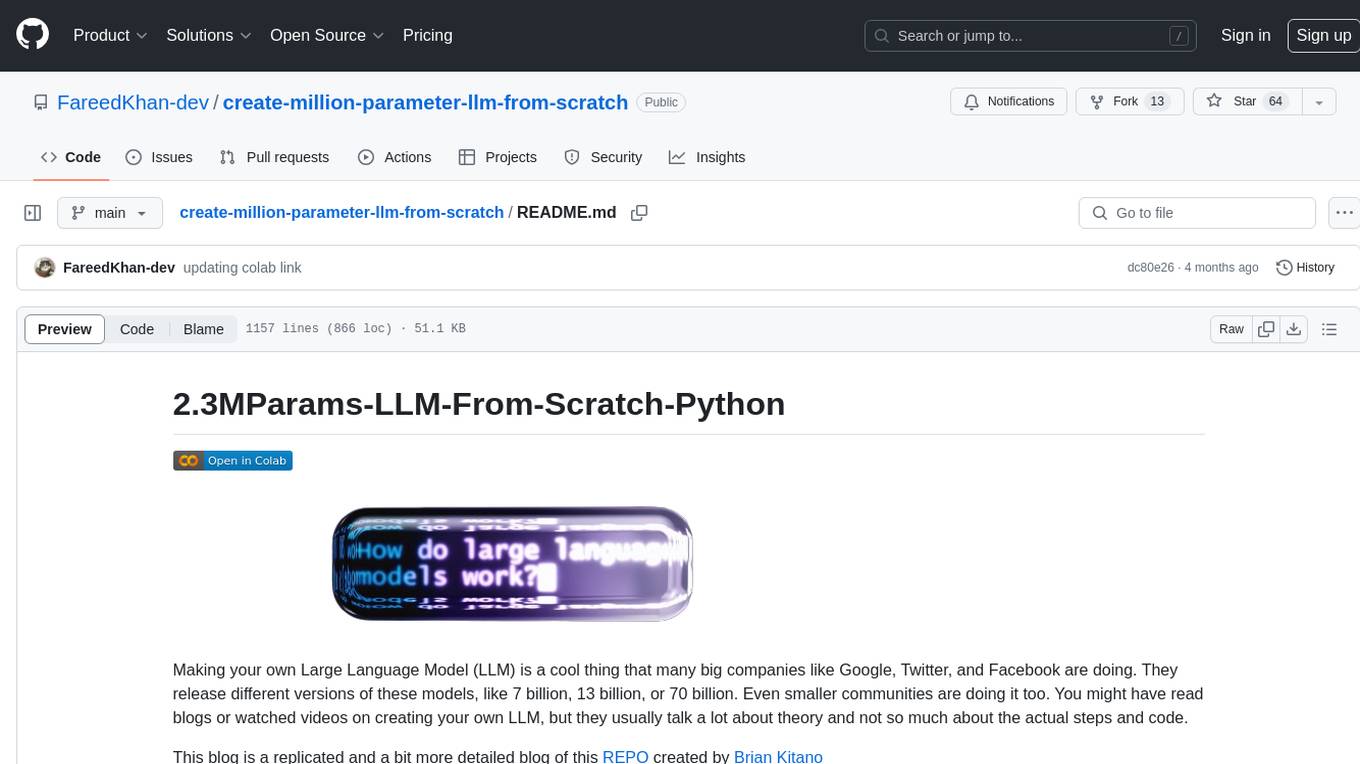
create-million-parameter-llm-from-scratch
The 'create-million-parameter-llm-from-scratch' repository provides a detailed guide on creating a Large Language Model (LLM) with 2.3 million parameters from scratch. The blog replicates the LLaMA approach, incorporating concepts like RMSNorm for pre-normalization, SwiGLU activation function, and Rotary Embeddings. The model is trained on a basic dataset to demonstrate the ease of creating a million-parameter LLM without the need for a high-end GPU.
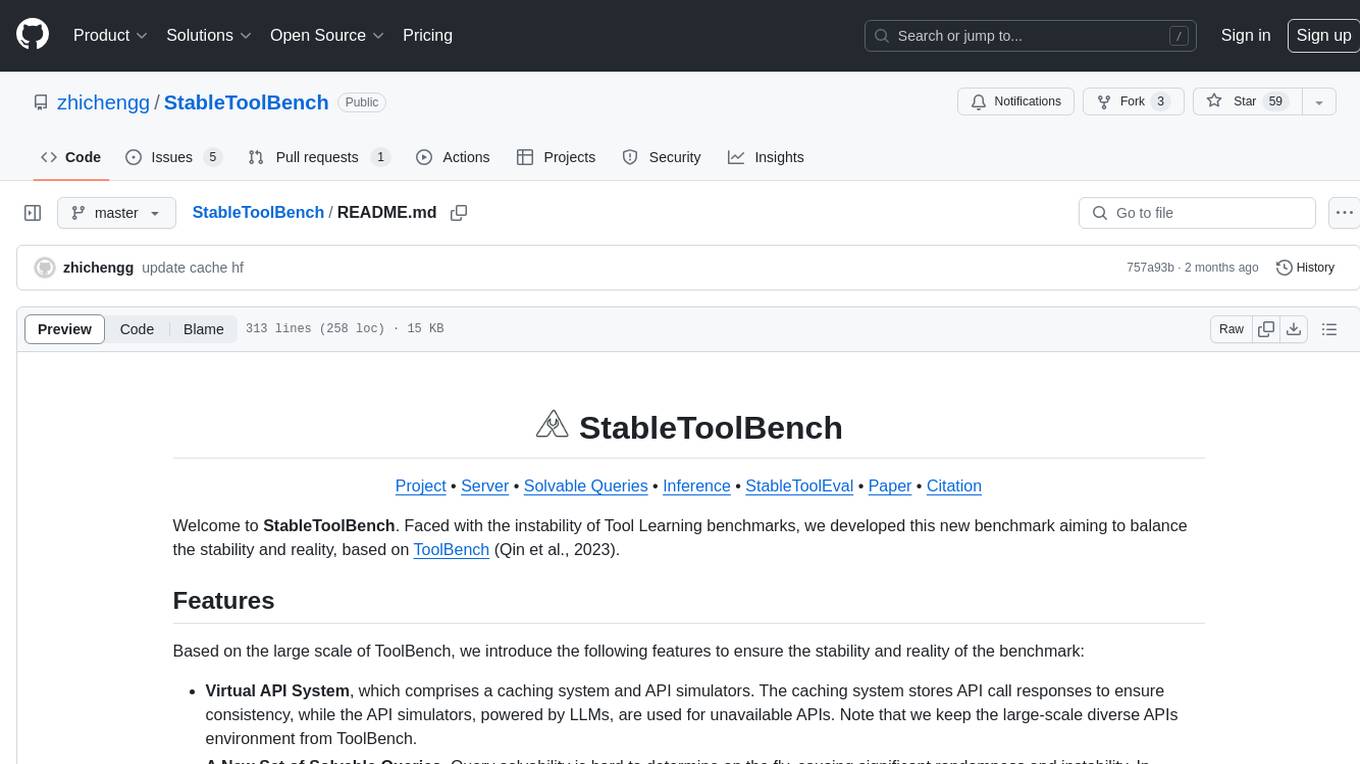
StableToolBench
StableToolBench is a new benchmark developed to address the instability of Tool Learning benchmarks. It aims to balance stability and reality by introducing features such as a Virtual API System with caching and API simulators, a new set of solvable queries determined by LLMs, and a Stable Evaluation System using GPT-4. The Virtual API Server can be set up either by building from source or using a prebuilt Docker image. Users can test the server using provided scripts and evaluate models with Solvable Pass Rate and Solvable Win Rate metrics. The tool also includes model experiments results comparing different models' performance.
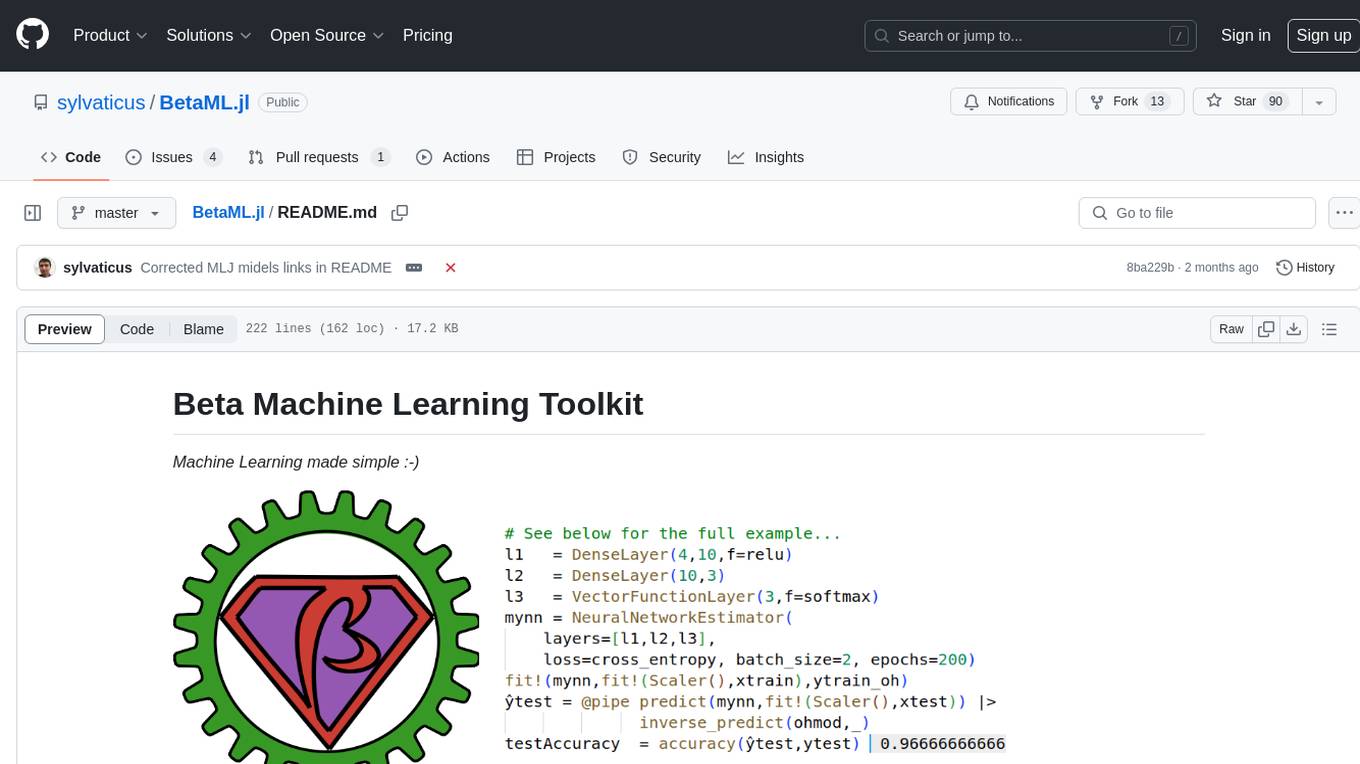
BetaML.jl
The Beta Machine Learning Toolkit is a package containing various algorithms and utilities for implementing machine learning workflows in multiple languages, including Julia, Python, and R. It offers a range of supervised and unsupervised models, data transformers, and assessment tools. The models are implemented entirely in Julia and are not wrappers for third-party models. Users can easily contribute new models or request implementations. The focus is on user-friendliness rather than computational efficiency, making it suitable for educational and research purposes.
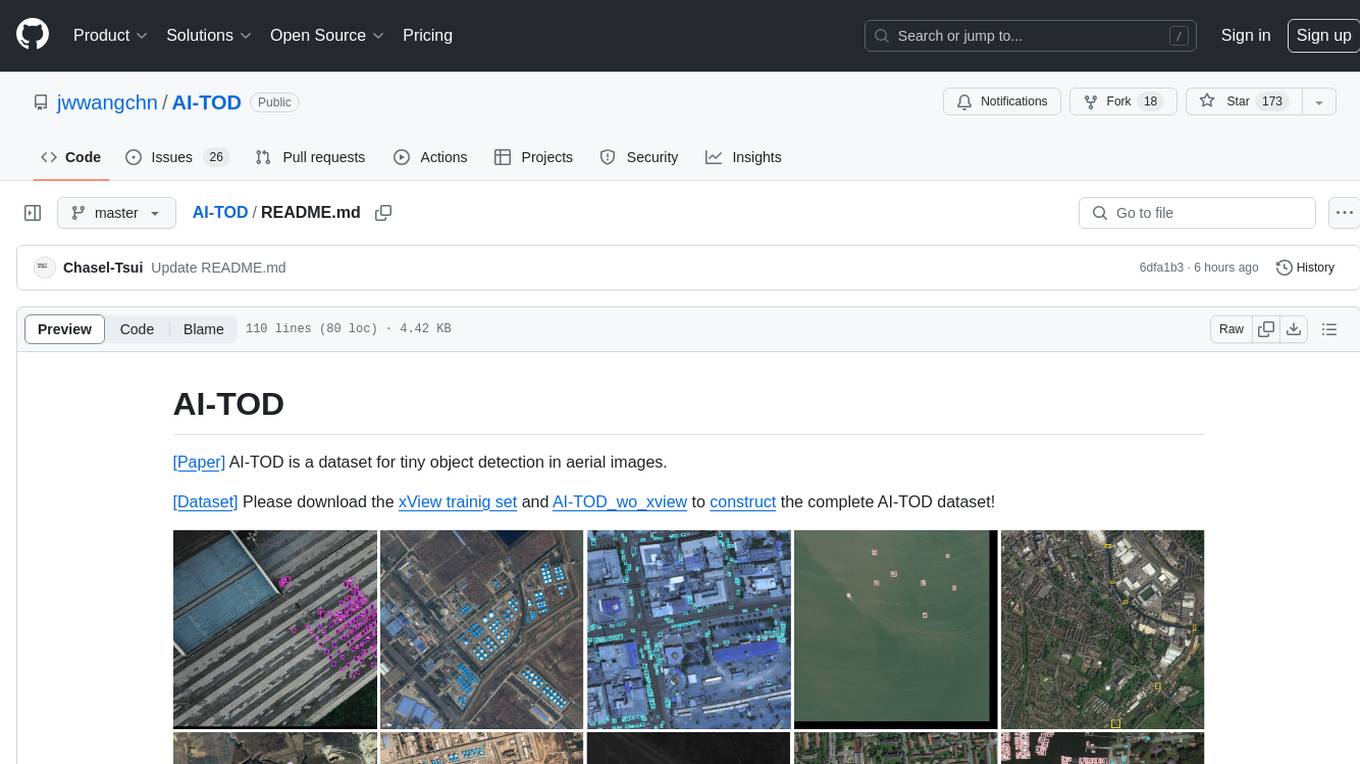
AI-TOD
AI-TOD is a dataset for tiny object detection in aerial images, containing 700,621 object instances across 28,036 images. Objects in AI-TOD are smaller with a mean size of 12.8 pixels compared to other aerial image datasets. To use AI-TOD, download xView training set and AI-TOD_wo_xview, then generate the complete dataset using the provided synthesis tool. The dataset is publicly available for academic and research purposes under CC BY-NC-SA 4.0 license.
For similar jobs
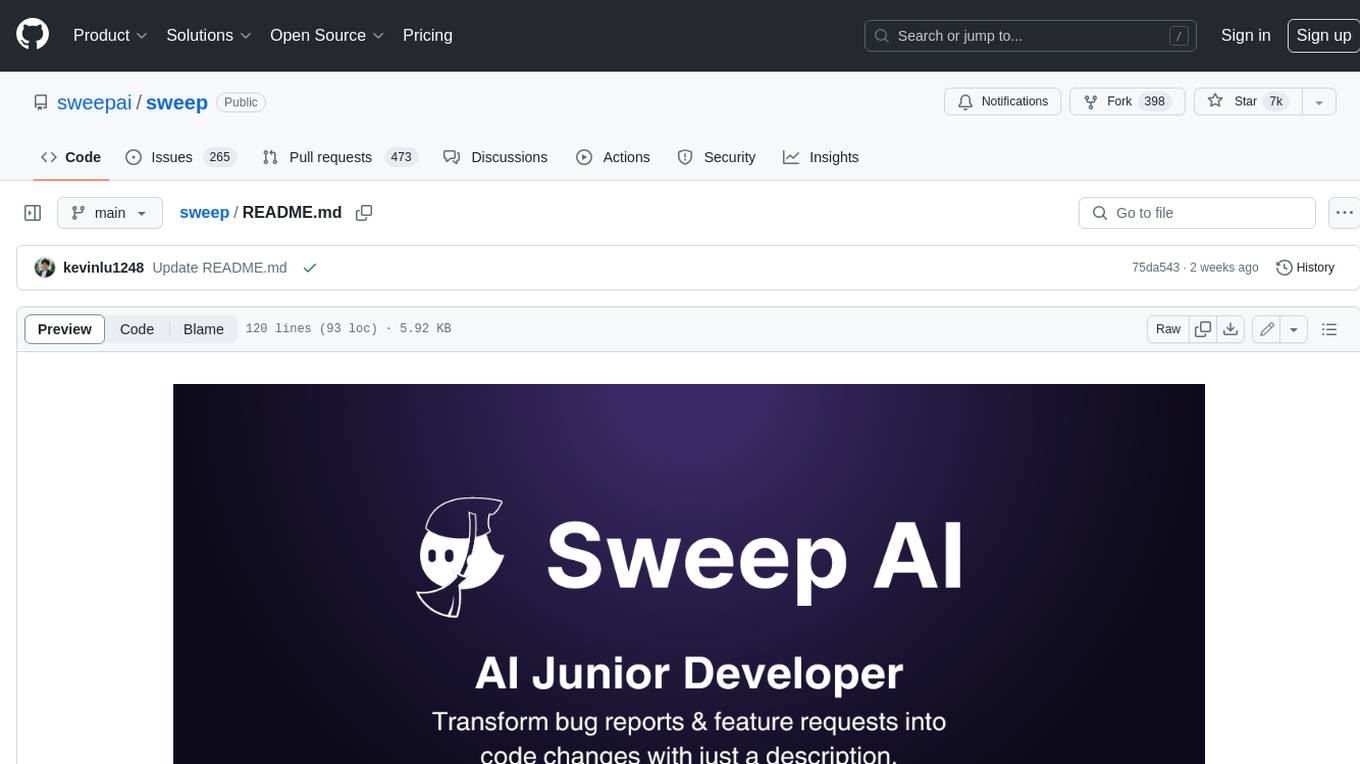
sweep
Sweep is an AI junior developer that turns bugs and feature requests into code changes. It automatically handles developer experience improvements like adding type hints and improving test coverage.
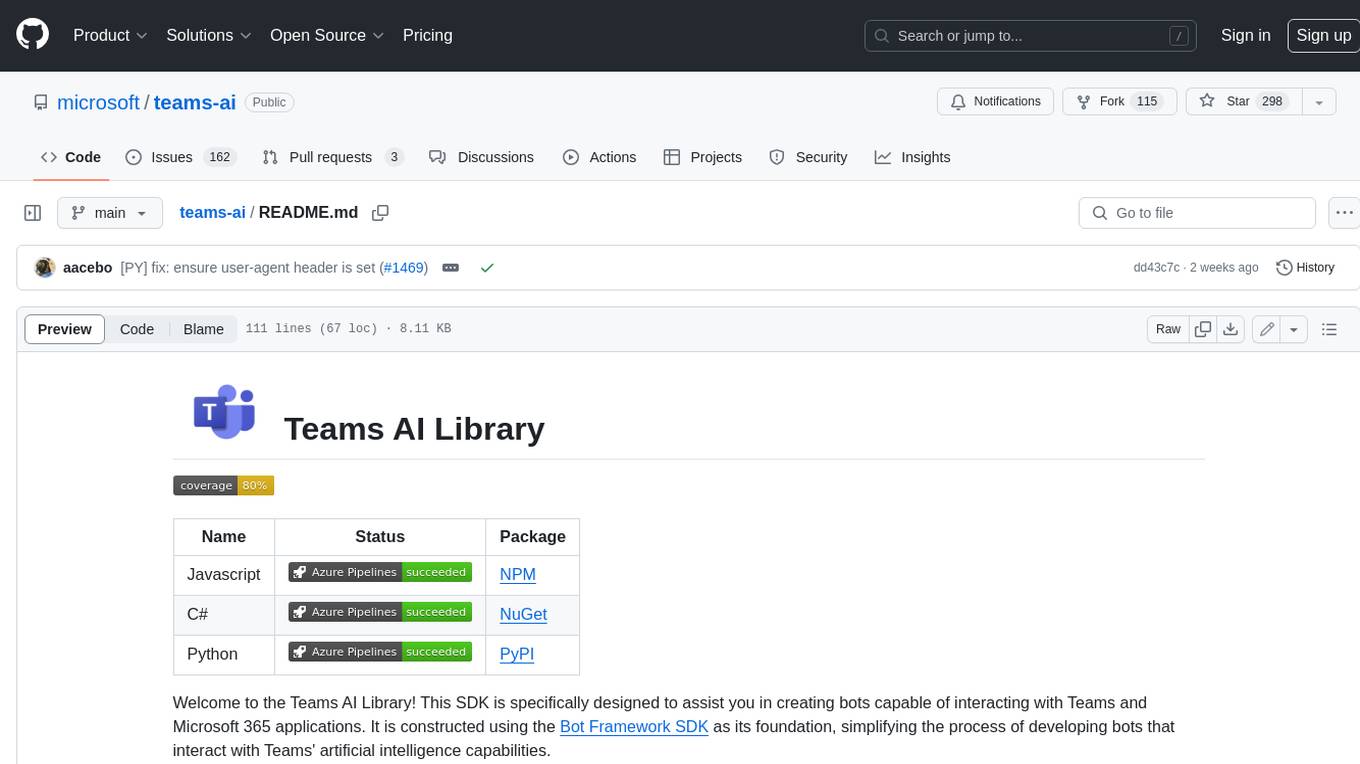
teams-ai
The Teams AI Library is a software development kit (SDK) that helps developers create bots that can interact with Teams and Microsoft 365 applications. It is built on top of the Bot Framework SDK and simplifies the process of developing bots that interact with Teams' artificial intelligence capabilities. The SDK is available for JavaScript/TypeScript, .NET, and Python.
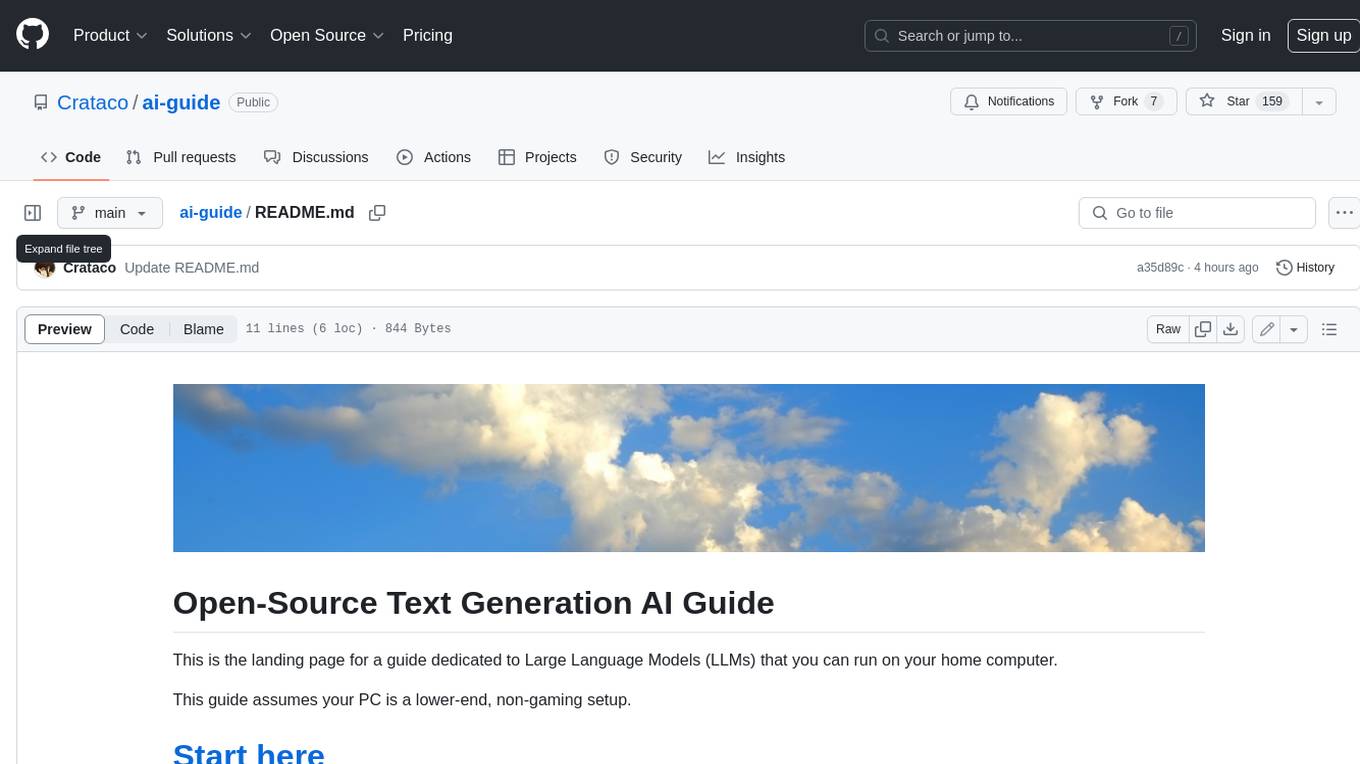
ai-guide
This guide is dedicated to Large Language Models (LLMs) that you can run on your home computer. It assumes your PC is a lower-end, non-gaming setup.
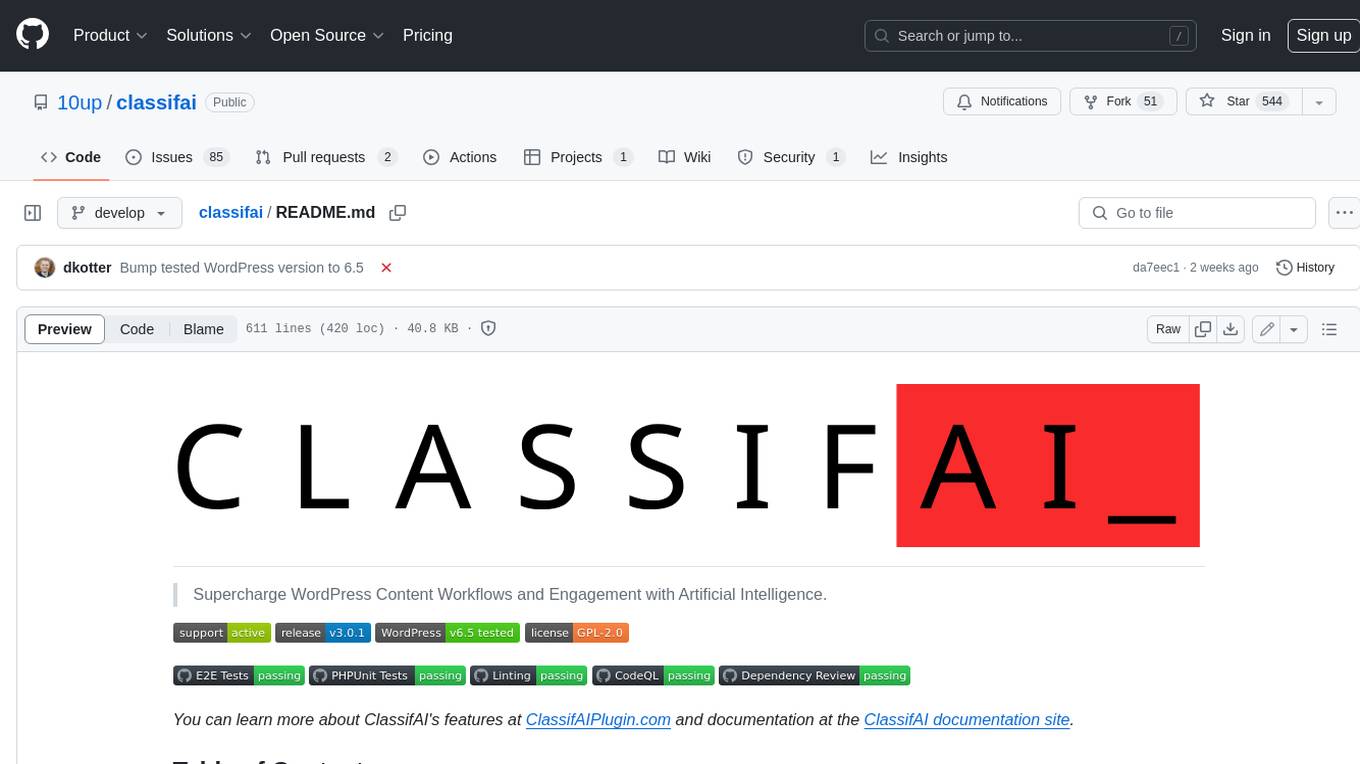
classifai
Supercharge WordPress Content Workflows and Engagement with Artificial Intelligence. Tap into leading cloud-based services like OpenAI, Microsoft Azure AI, Google Gemini and IBM Watson to augment your WordPress-powered websites. Publish content faster while improving SEO performance and increasing audience engagement. ClassifAI integrates Artificial Intelligence and Machine Learning technologies to lighten your workload and eliminate tedious tasks, giving you more time to create original content that matters.
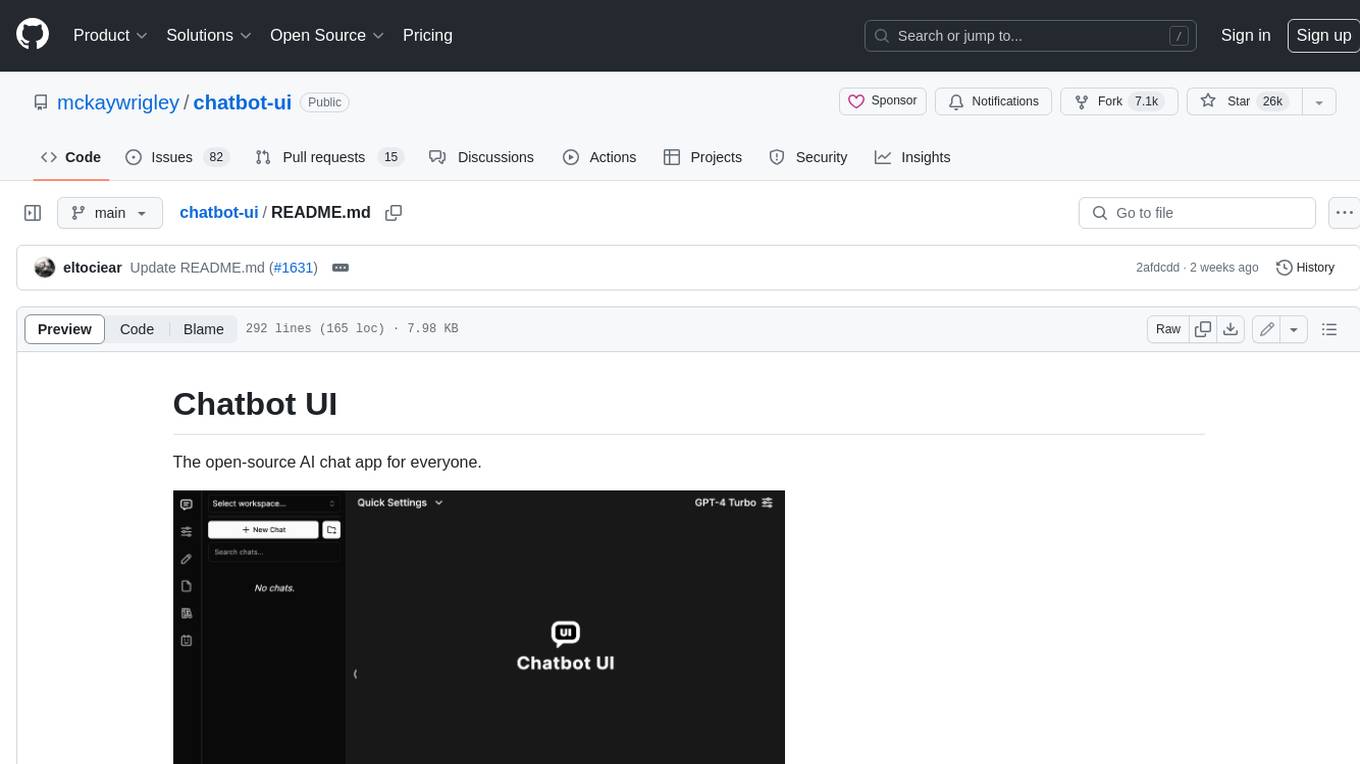
chatbot-ui
Chatbot UI is an open-source AI chat app that allows users to create and deploy their own AI chatbots. It is easy to use and can be customized to fit any need. Chatbot UI is perfect for businesses, developers, and anyone who wants to create a chatbot.
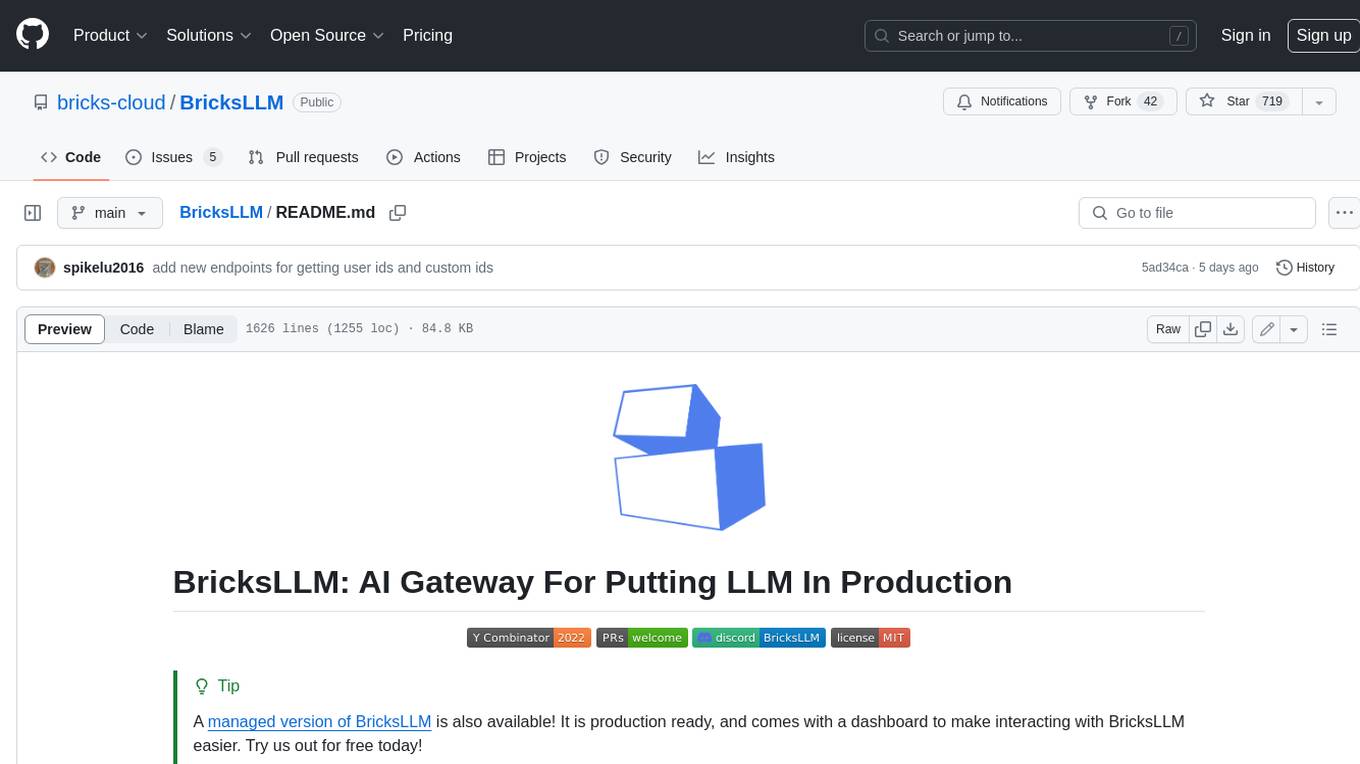
BricksLLM
BricksLLM is a cloud native AI gateway written in Go. Currently, it provides native support for OpenAI, Anthropic, Azure OpenAI and vLLM. BricksLLM aims to provide enterprise level infrastructure that can power any LLM production use cases. Here are some use cases for BricksLLM: * Set LLM usage limits for users on different pricing tiers * Track LLM usage on a per user and per organization basis * Block or redact requests containing PIIs * Improve LLM reliability with failovers, retries and caching * Distribute API keys with rate limits and cost limits for internal development/production use cases * Distribute API keys with rate limits and cost limits for students
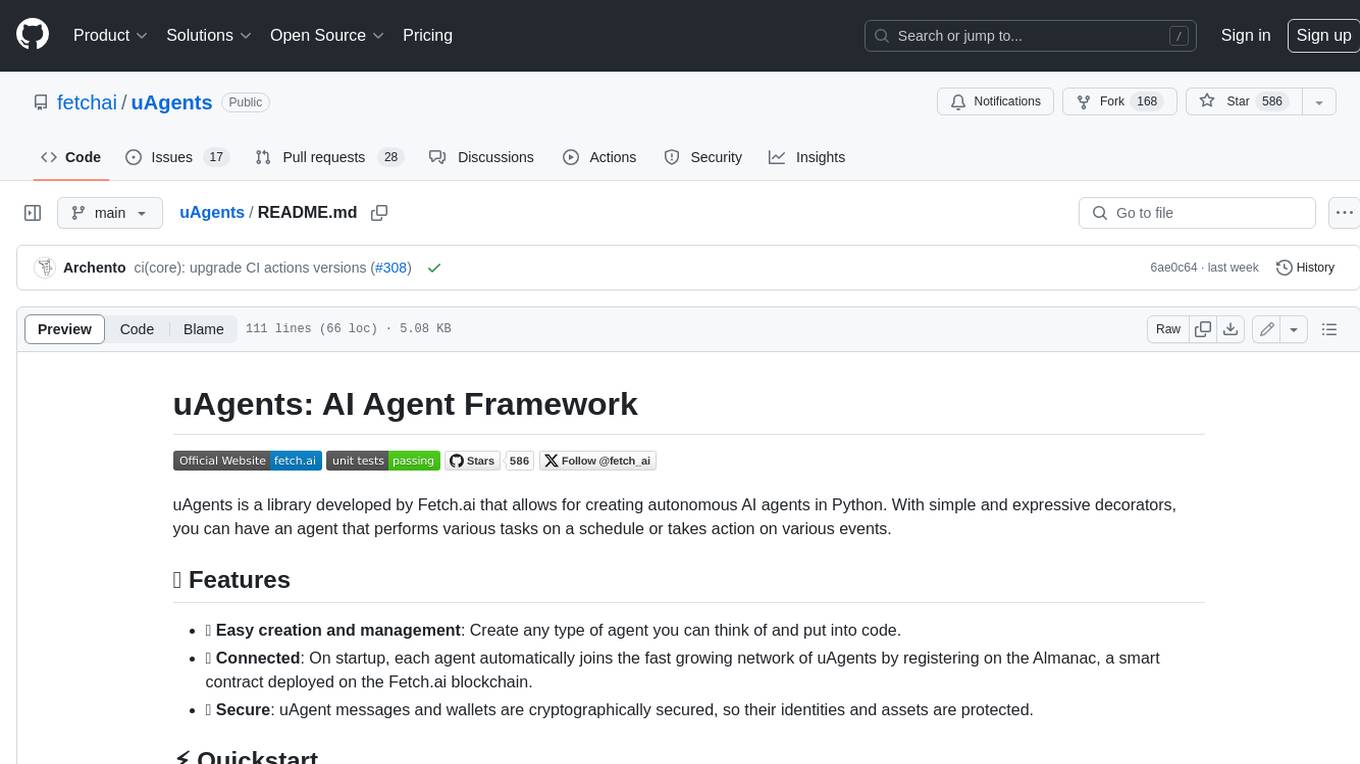
uAgents
uAgents is a Python library developed by Fetch.ai that allows for the creation of autonomous AI agents. These agents can perform various tasks on a schedule or take action on various events. uAgents are easy to create and manage, and they are connected to a fast-growing network of other uAgents. They are also secure, with cryptographically secured messages and wallets.
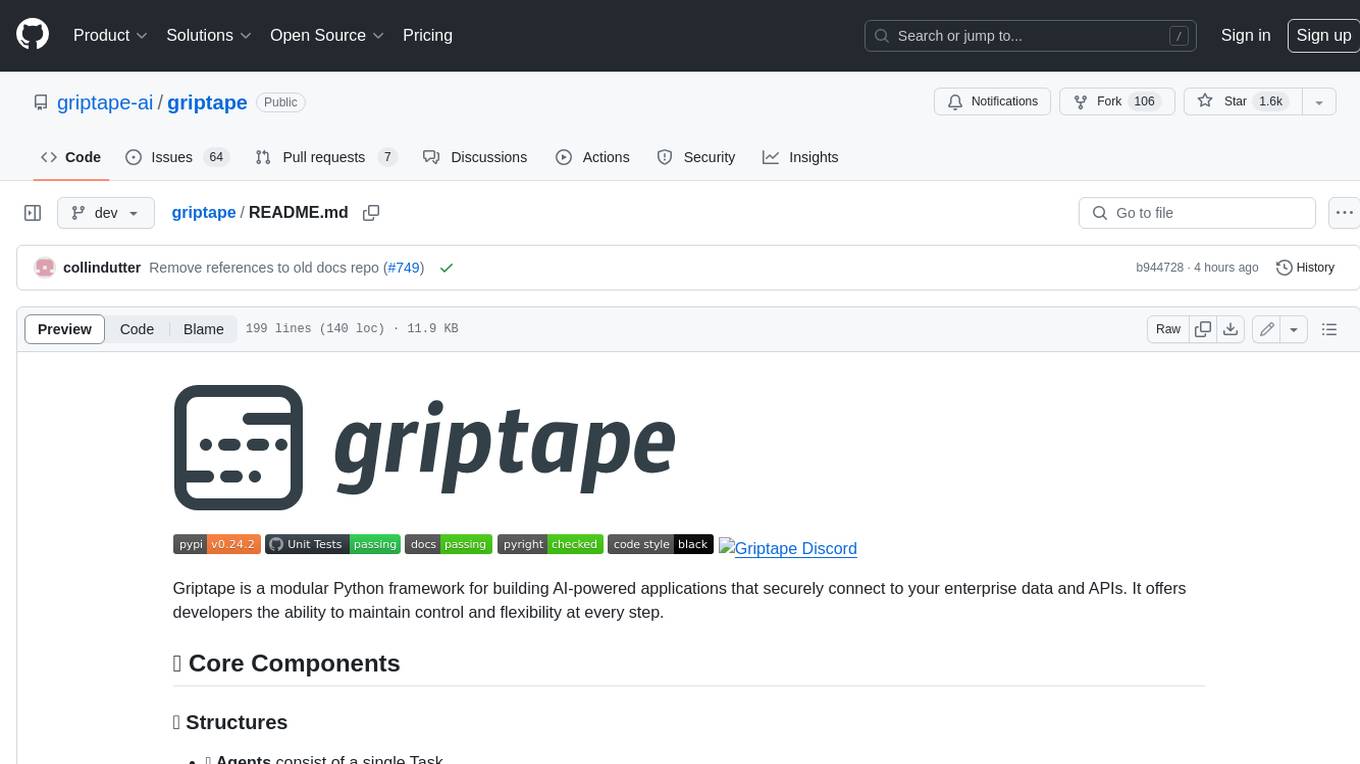
griptape
Griptape is a modular Python framework for building AI-powered applications that securely connect to your enterprise data and APIs. It offers developers the ability to maintain control and flexibility at every step. Griptape's core components include Structures (Agents, Pipelines, and Workflows), Tasks, Tools, Memory (Conversation Memory, Task Memory, and Meta Memory), Drivers (Prompt and Embedding Drivers, Vector Store Drivers, Image Generation Drivers, Image Query Drivers, SQL Drivers, Web Scraper Drivers, and Conversation Memory Drivers), Engines (Query Engines, Extraction Engines, Summary Engines, Image Generation Engines, and Image Query Engines), and additional components (Rulesets, Loaders, Artifacts, Chunkers, and Tokenizers). Griptape enables developers to create AI-powered applications with ease and efficiency.