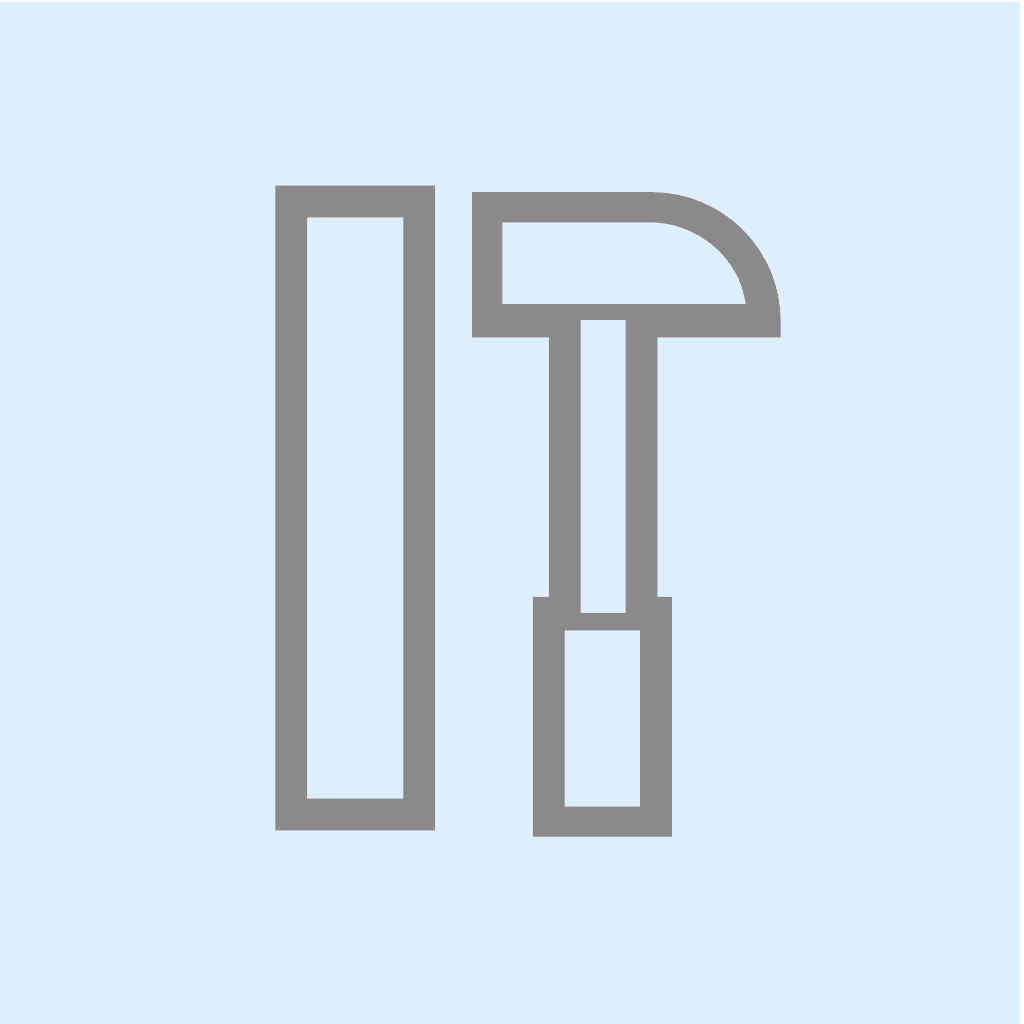
moatless-tools
None
Stars: 320
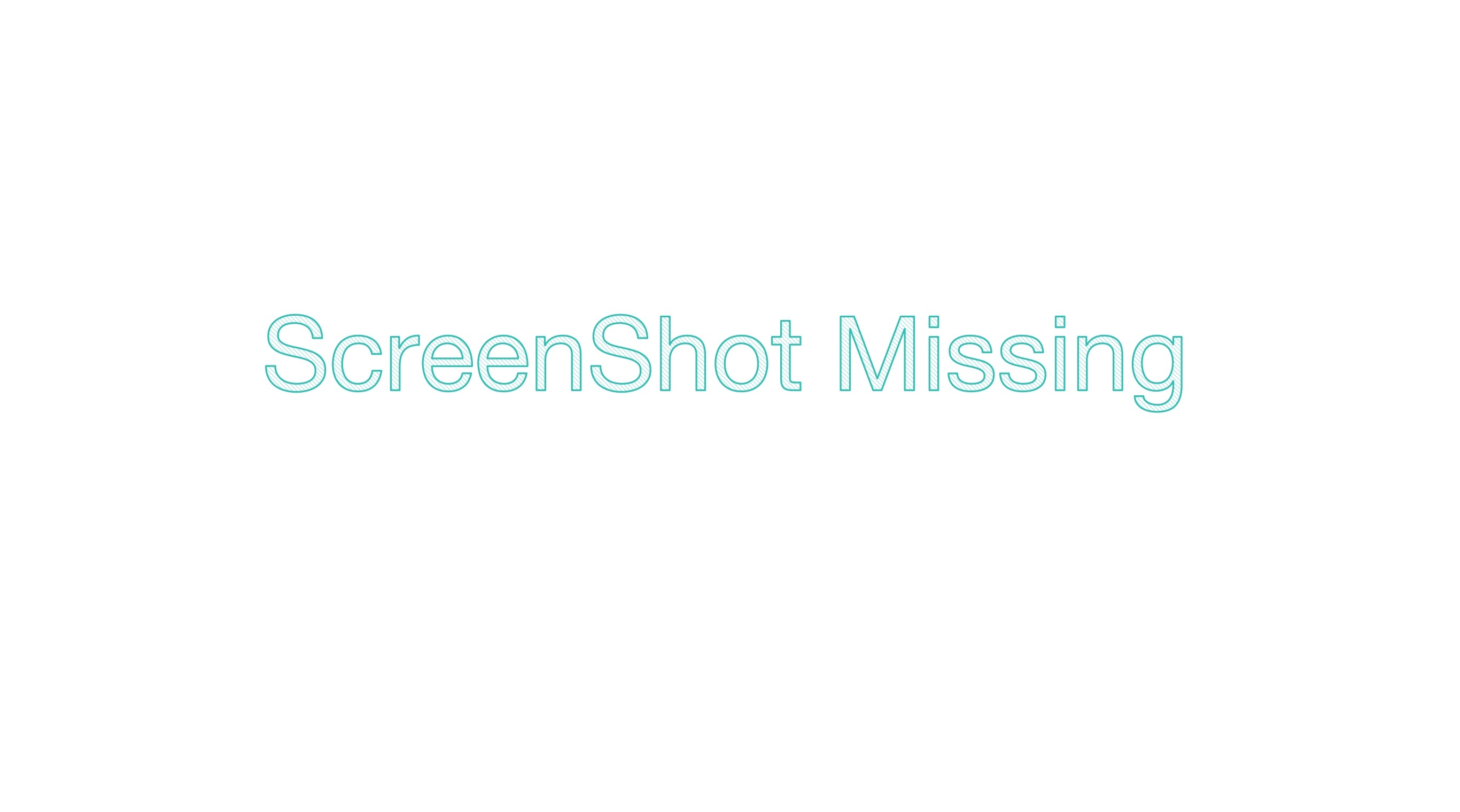
Moatless Tools is a hobby project focused on experimenting with using Large Language Models (LLMs) to edit code in large existing codebases. The project aims to build tools that insert the right context into prompts and handle responses effectively. It utilizes an agentic loop functioning as a finite state machine to transition between states like Search, Identify, PlanToCode, ClarifyChange, and EditCode for code editing tasks.
README:
Moatless Tools is a hobby project where I experiment with some ideas I have about how LLMs can be used to edit code in large existing codebases. I believe that rather than relying on an agent to reason its way to a solution, it is crucial to build good tools to insert the right context into the prompt and handle the response.
For the implementation used in the paper SWE-Search: Enhancing Software Agents with Monte Carlo Tree Search and Iterative Refinement, please see moatless-tree-search.
I use the SWE-bench benchmark as a way to verify my ideas.
With version 0.0.4 I get 30.7% solve rate (92 instances) using the open-source Deepseek V3 model. The most notable aspect of this is the extremely low cost - the entire evaluation run costs less than $4 ($0.0127 per instance), achieving 24 resolved instances per dollar spent.
With version 0.0.3 I get 38.3% solve rate with Claude 3.5 Sonnet v20241022. Average cost per instance is $0.30.
The three main reasons I’ve been able to go from 27% to 38% solved instances in this version:
-
Claude 3.5 Sonnet and Computer Use
The solution has been adjusted to use thetext_editor_20241022
tool introduced in the new version of Claude 3.5 Sonnet. This provides more stable results when editing existing code. -
moatless-testbeds
I set up a Kubernetes-based solution to run tests and provide feedback on test results to the agent. It’s worth noting that the agent has to independently identify the tests and can’t rely on thePASS_TO_PASS
orFAIL_TO_PASS
data for each instance. -
More flexible model
In the earlier version of Moatless Tools, the agent followed a rigid flow where it first retrieved content and then edited the code. Now, it can dynamically choose between actions for code retrieval or editing, depending on the situation.
Try the Claude 3.5 Sonnet v20241022 evaluation set up on Google Colab
With version 0.0.2 I get 26.7% solve rate with Claude 3.5 Sonnet, with a bit higher cost of $0.17 per instance.
Try the Claude 3.5 evaluation set up on Google Colab
Moatless Tools 0.0.1 has a solve rate of 24%, with each benchmark instance costing an average of $0.13 to solve with GPT-4o. Running the SWE Bench Lite dataset with 300 instances costs approx 40 dollars.
I have focused on testing my ideas, and the project is currently a bit messy. My plan is to organize it in the coming period. However, feel free to clone the repo and try running this notebook:
Install dependencies:
poetry install
Before running the evaluation, you'll need:
- At least one LLM provider API key (e.g., OpenAI, Anthropic, etc.)
- A Voyage AI API key from voyageai.com to use the pre-embedded vector stores for SWE-Bench instances.
- (Optional) Access to a testbed environment - see moatless-testbeds for setup instructions
You can configure these settings by either:
- Create a
.env
file in the project root (copy from.env.example
):
cp .env.example .env
# Edit .env with your values
- Or export the variables directly:
# Directory for storing vector index store files
export INDEX_STORE_DIR="/tmp/index_store"
# Directory for storing clonedrepositories
export REPO_DIR="/tmp/repos"
# Required: At least one LLM provider API key
export OPENAI_API_KEY="<your-key>"
export ANTHROPIC_API_KEY="<your-key>"
# ...or Base URL for custom LLM API service (optional)
export CUSTOM_LLM_API_BASE="<your-base-url>"
export CUSTOM_LLM_API_KEY="<your-key>"
# Required: API Key for Voyage Embeddings
export VOYAGE_API_KEY="<your-key>"
# Optional: Configuration for testbed environment (https://github.com/aorwall/moatless-testbeds)
export TESTBED_API_KEY="<your-key>"
export TESTBED_BASE_URL="<your-base-url>"
Default model configurations are provided for verified models. Note that other models may work but have not been extensively tested. Verified models are models that have been tested and found to work with the Verified Mini subset of the SWE-Bench dataset.
When specifying just the --model
argument, the following configurations are used:
Model | Response Format | Message History | Thoughts in Action | Verified Mini |
---|---|---|---|---|
claude-3-5-sonnet-20241022 | tool_call | messages | no | 46% |
claude-3-5-haiku-20241022 | tool_call | messages | no | 28% |
gpt-4o-2024-11-20 | tool_call | messages | yes | 32% |
gpt-4o-mini-2024-07-18 | tool_call | messages | yes | 16% |
o1-mini-2024-09-12 | react | react | no (disabled thoughts) | 28% |
deepseek/deepseek-chat | react | react | no | 36% |
gemini/gemini-2.0-flash-exp | react | react | no | 38% |
openrouter/meta-llama/llama-3.1-70b-instruct | react | react | no | - |
openrouter/meta-llama/llama-3.1-405b-instruct | react | react | no | 28% |
openrouter/qwen/qwen-2.5-coder-32b-instruct | react | react | no | 32% |
Before running the full evaluation, you can verify your setup using the integration test script:
# Run a single model test
poetry run python -m moatless.validation.validate_simple_code_flow --model claude-3-5-sonnet-20241022
The script will run the model against a sample SWE-Bench instance
Results are saved in test_results/integration_test_<timestamp>/
.
The evaluation script supports various configuration options through command line arguments:
poetry run python -m moatless.benchmark.run_evaluation [OPTIONS]
Required arguments:
-
--model MODEL
: Model to use for evaluation (e.g., 'claude-3-5-sonnet-20241022', 'gpt-4o')
Optional arguments:
-
Model settings:
-
--model MODEL
: Model identifier. Can be a supported model from the table below or any custom model identifier. -
--api-key KEY
: API key for the model -
--base-url URL
: Base URL for the model API -
--response-format FORMAT
: Response format ('tool_call' or 'react'). Defaults to 'tool_call' for custom models -
--message-history TYPE
: Message history type ('messages', 'summary', 'react', 'messages_compact', 'instruct'). Defaults to 'messages' for custom models -
--thoughts-in-action
: Enable thoughts in action -
--temperature FLOAT
: Temperature for model sampling. Defaults to 0.0
-
-
Dataset settings:
-
--split SPLIT
: Dataset split to use. Defaults to 'lite' -
--instance-ids ID [ID ...]
: Specific instance IDs to evaluate
-
-
Loop settings:
-
--max-iterations INT
: Maximum number of iterations -
--max-cost FLOAT
: Maximum cost in dollars
-
-
Runner settings:
-
--num-workers INT
: Number of parallel workers. Defaults to 10 -
--evaluation-name NAME
: Custom name for the evaluation run -
--rerun-errors
: Rerun instances that previously errored
-
Available dataset splits that can be specified with the --split
argument:
Split Name | Description | Instance Count |
---|---|---|
lite | All instances from the lite dataset | 300 |
verified | All instances from the verified dataset | 500 |
verified_mini | MariusHobbhahn/swe-bench-verified-mini, a subset of SWE-Bench Verified | 50 |
lite_and_verified_solvable | Instances that exist in both lite and verified datasets and have at least one solved submission to SWE-Bench | 84 |
Example usage:
# Run evaluation with Claude 3.5 Sonnet using the ReACT format
poetry run python -m moatless.benchmark.run_evaluation \
--model claude-3-5-sonnet-20241022 \
--response-format react \
--message-history react \
--num-workers 10
# Run specific instances with GPT-4
poetry run python -m moatless.benchmark.run_evaluation \
--model gpt-4o-2024-11-20 \
--instance-ids "django__django-16527"
Basic setup using the AgenticLoop
to solve a SWE-Bench instance.
from moatless.actions.string_replace import StringReplace
from moatless.agent.code_agent import CodingAgent
from moatless.benchmark.swebench import create_repository
from moatless.benchmark.utils import get_moatless_instance
from moatless.completion.base import BaseCompletionModel, LLMResponseFormat
from moatless.completion.tool_call import ToolCallCompletionModel
from moatless.file_context import FileContext
from moatless.index import CodeIndex
from moatless.loop import AgenticLoop
from moatless.schema import MessageHistoryType
index_store_dir = "/tmp/index_store"
repo_base_dir = "/tmp/repos"
persist_path = "trajectory.json"
instance = get_moatless_instance("django__django-16379")
completion_model = BaseCompletionModel.create(response_format=LLMResponseFormat.TOOLS, model="claude-3-5-sonnet-20240620", temperature=0.0)
repository = create_repository(instance)
code_index = CodeIndex.from_index_name(
instance["instance_id"], index_store_dir=index_store_dir, file_repo=repository
)
file_context = FileContext(repo=repository)
agent = CodingAgent.create(completion_model=completion_model, code_index=code_index, repository=repository, message_history_type=MessageHistoryType.MESSAGES)
loop = AgenticLoop.create(
message=instance["problem_statement"],
agent=agent,
file_context=file_context,
repository=repository,
persist_path=persist_path,
max_iterations=50,
max_cost=2.0 # Optional: Set maximum cost in dollars
)
final_node = loop.run()
if final_node:
print(final_node.observation.message)
For Tasks:
Click tags to check more tools for each tasksFor Jobs:
Alternative AI tools for moatless-tools
Similar Open Source Tools
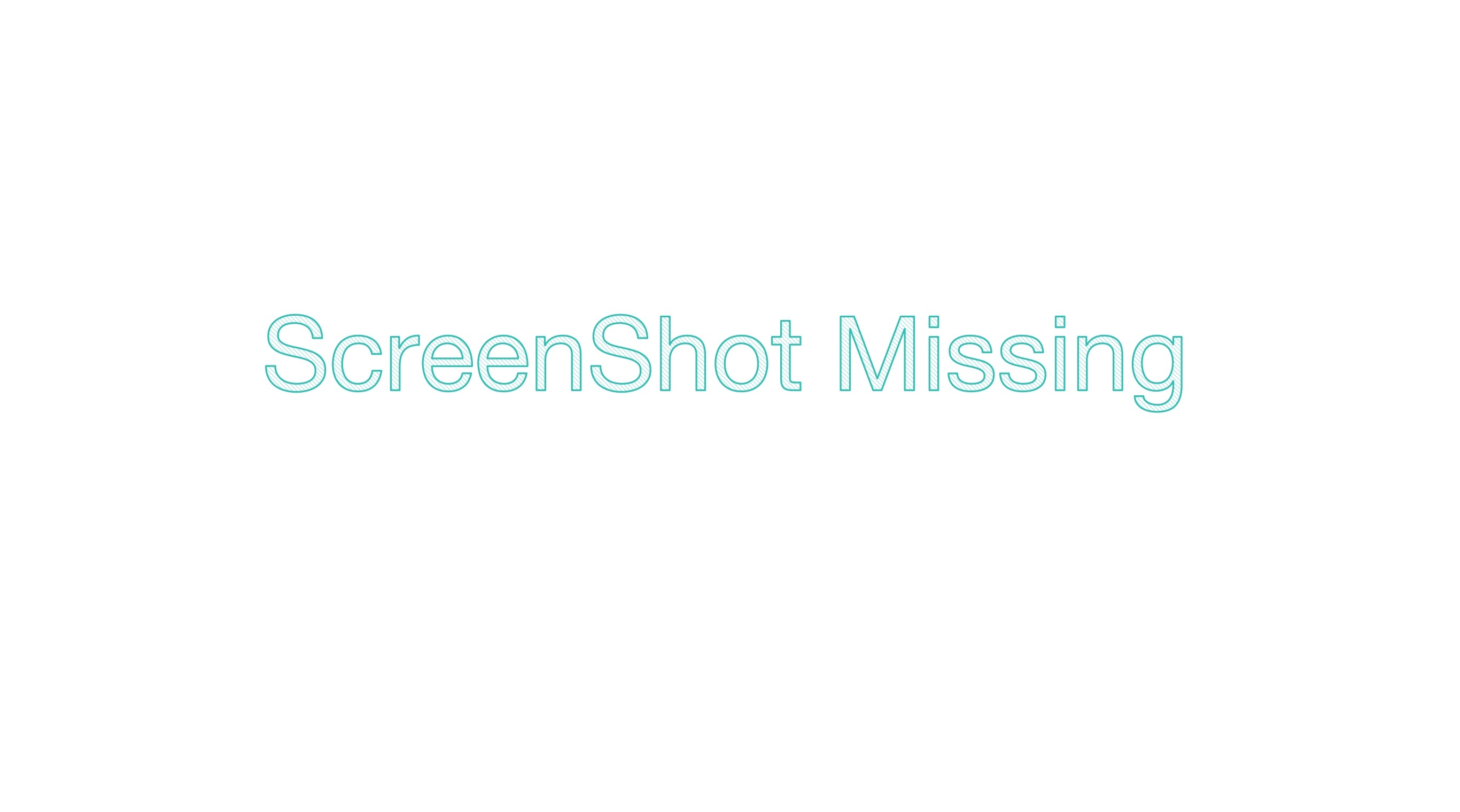
moatless-tools
Moatless Tools is a hobby project focused on experimenting with using Large Language Models (LLMs) to edit code in large existing codebases. The project aims to build tools that insert the right context into prompts and handle responses effectively. It utilizes an agentic loop functioning as a finite state machine to transition between states like Search, Identify, PlanToCode, ClarifyChange, and EditCode for code editing tasks.
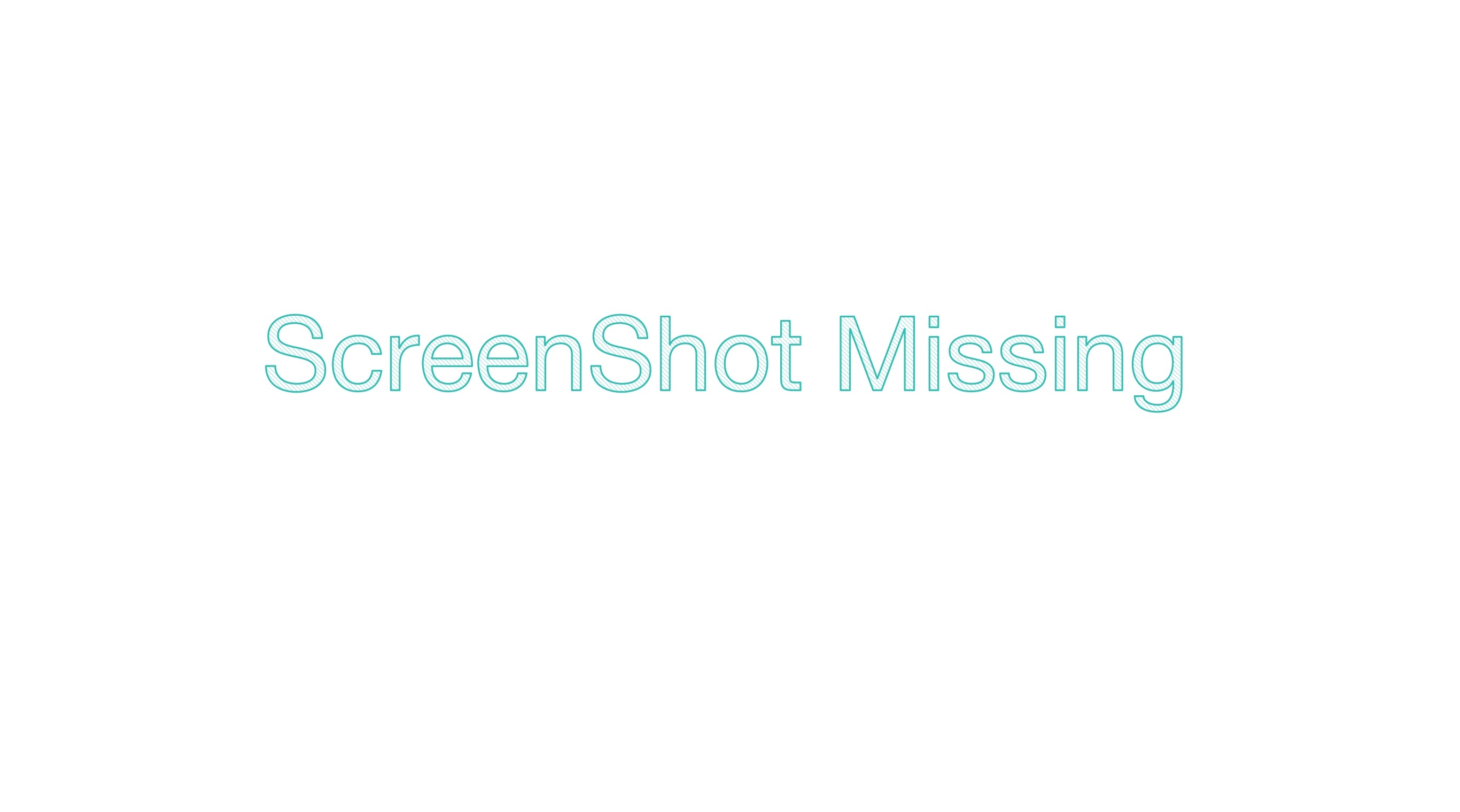
AQLM
AQLM is the official PyTorch implementation for Extreme Compression of Large Language Models via Additive Quantization. It includes prequantized AQLM models without PV-Tuning and PV-Tuned models for LLaMA, Mistral, and Mixtral families. The repository provides inference examples, model details, and quantization setups. Users can run prequantized models using Google Colab examples, work with different model families, and install the necessary inference library. The repository also offers detailed instructions for quantization, fine-tuning, and model evaluation. AQLM quantization involves calibrating models for compression, and users can improve model accuracy through finetuning. Additionally, the repository includes information on preparing models for inference and contributing guidelines.
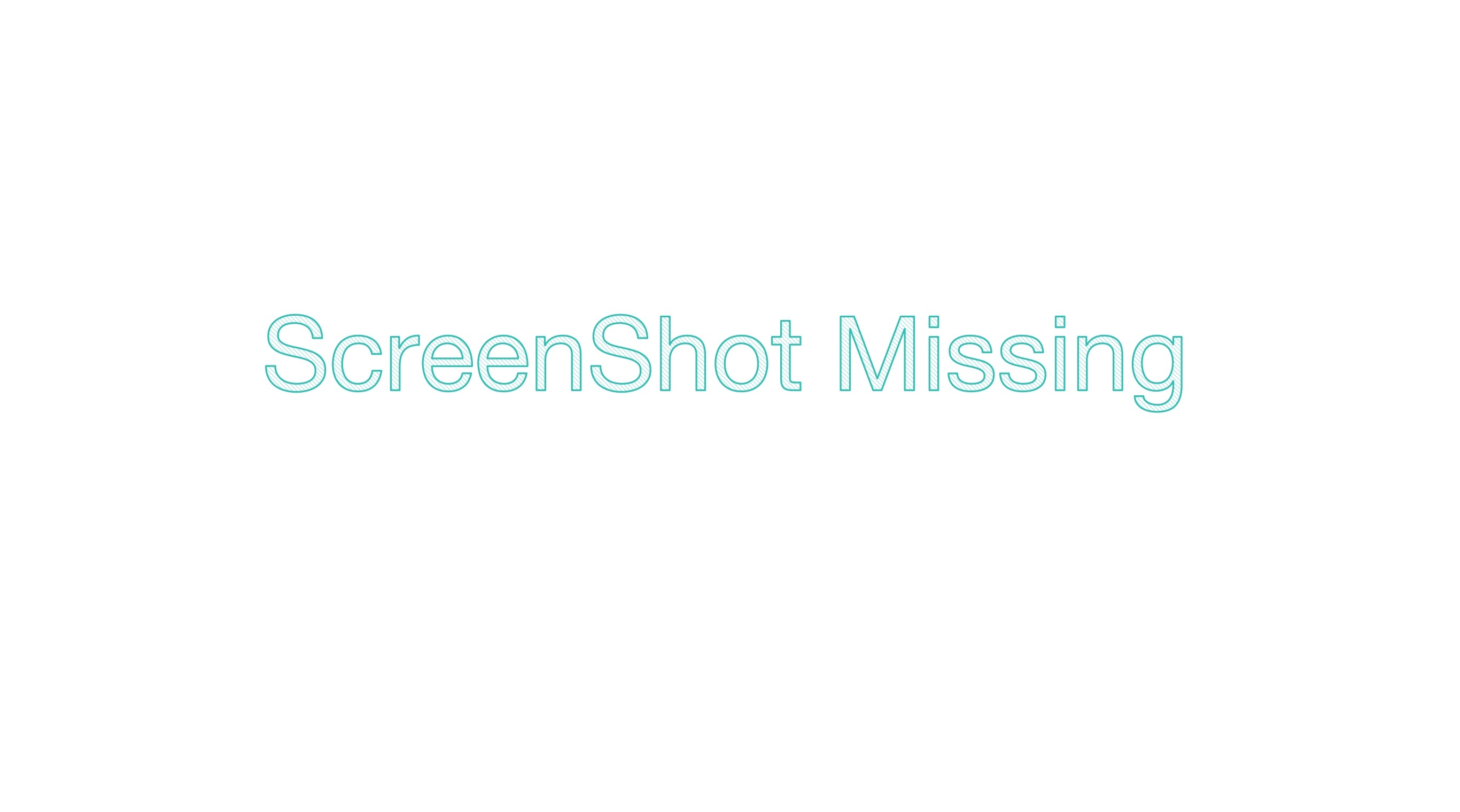
BentoVLLM
BentoVLLM is an example project demonstrating how to serve and deploy open-source Large Language Models using vLLM, a high-throughput and memory-efficient inference engine. It provides a basis for advanced code customization, such as custom models, inference logic, or vLLM options. The project allows for simple LLM hosting with OpenAI compatible endpoints without the need to write any code. Users can interact with the server using Swagger UI or other methods, and the service can be deployed to BentoCloud for better management and scalability. Additionally, the repository includes integration examples for different LLM models and tools.
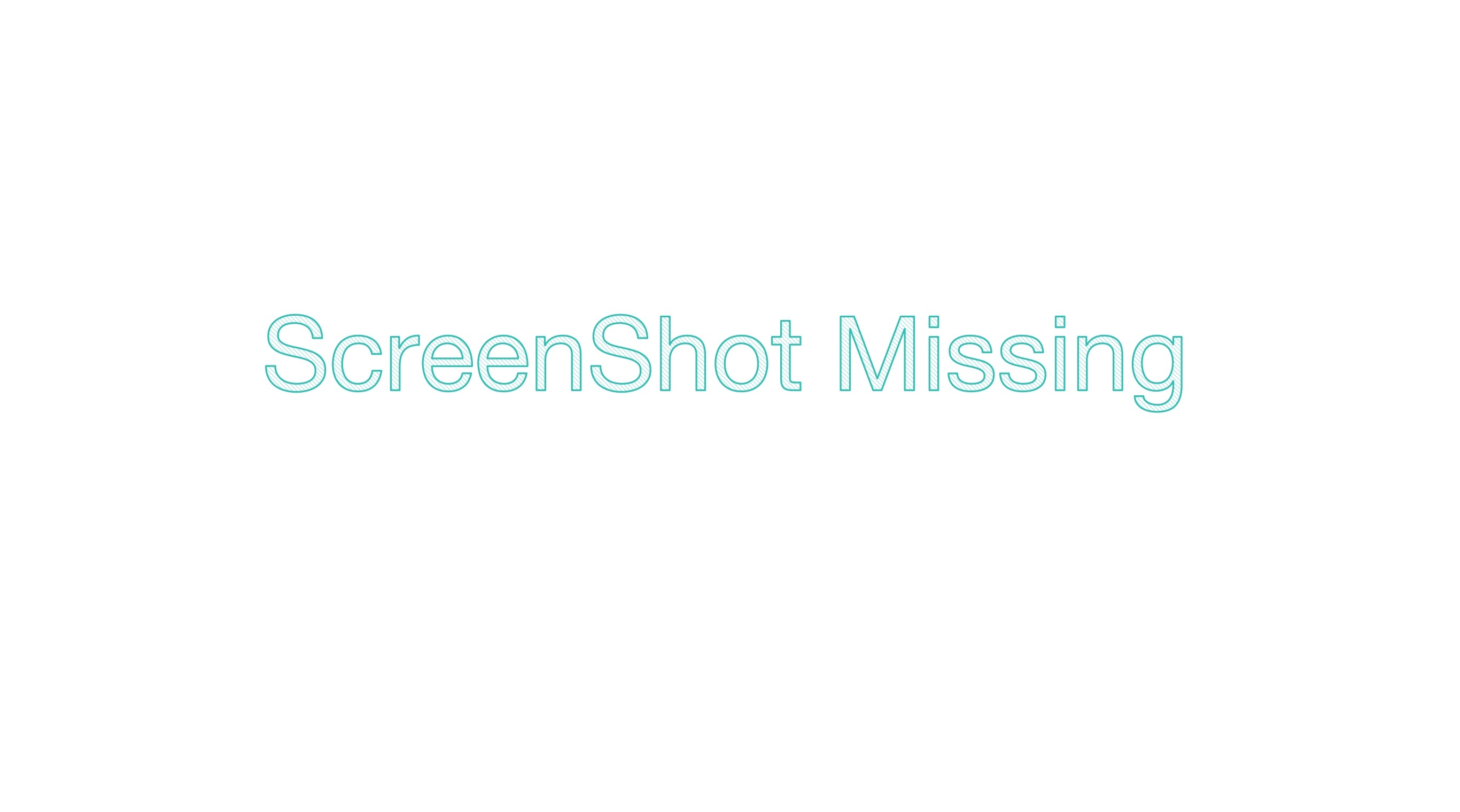
text-embeddings-inference
Text Embeddings Inference (TEI) is a toolkit for deploying and serving open source text embeddings and sequence classification models. TEI enables high-performance extraction for popular models like FlagEmbedding, Ember, GTE, and E5. It implements features such as no model graph compilation step, Metal support for local execution on Macs, small docker images with fast boot times, token-based dynamic batching, optimized transformers code for inference using Flash Attention, Candle, and cuBLASLt, Safetensors weight loading, and production-ready features like distributed tracing with Open Telemetry and Prometheus metrics.
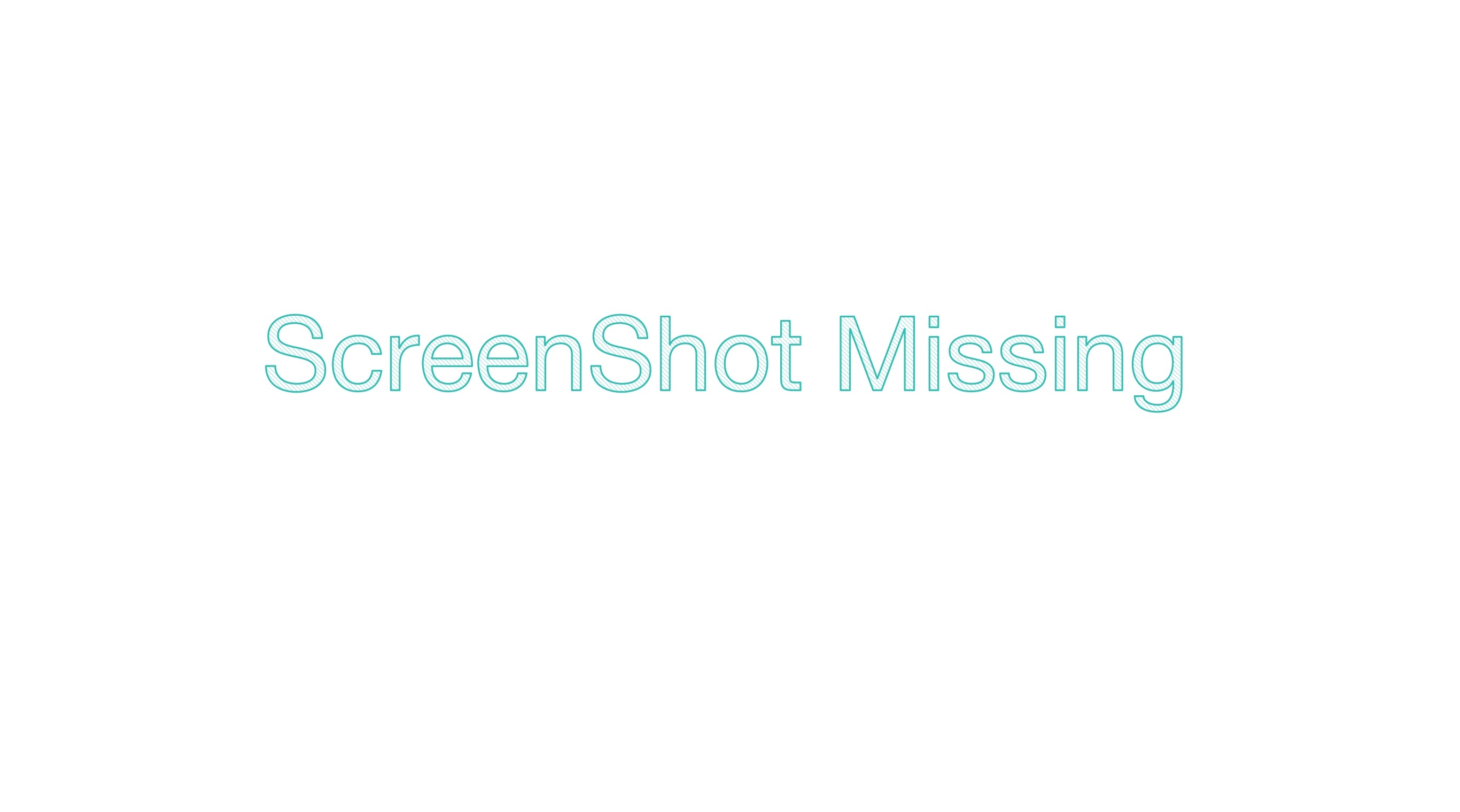
ABQ-LLM
ABQ-LLM is a novel arbitrary bit quantization scheme that achieves excellent performance under various quantization settings while enabling efficient arbitrary bit computation at the inference level. The algorithm supports precise weight-only quantization and weight-activation quantization. It provides pre-trained model weights and a set of out-of-the-box quantization operators for arbitrary bit model inference in modern architectures.
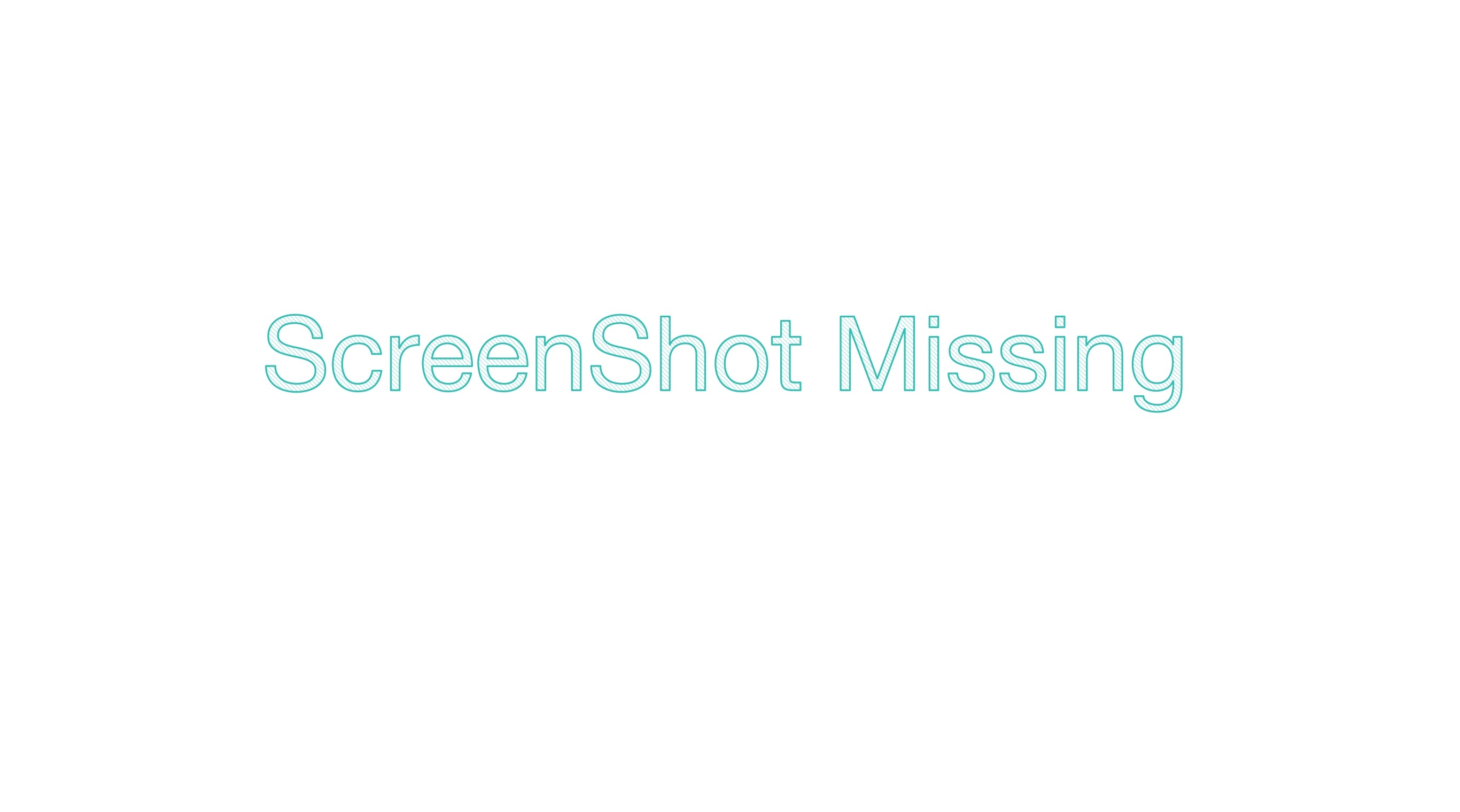
LongLoRA
LongLoRA is a tool for efficient fine-tuning of long-context large language models. It includes LongAlpaca data with long QA data collected and short QA sampled, models from 7B to 70B with context length from 8k to 100k, and support for GPTNeoX models. The tool supports supervised fine-tuning, context extension, and improved LoRA fine-tuning. It provides pre-trained weights, fine-tuning instructions, evaluation methods, local and online demos, streaming inference, and data generation via Pdf2text. LongLoRA is licensed under Apache License 2.0, while data and weights are under CC-BY-NC 4.0 License for research use only.
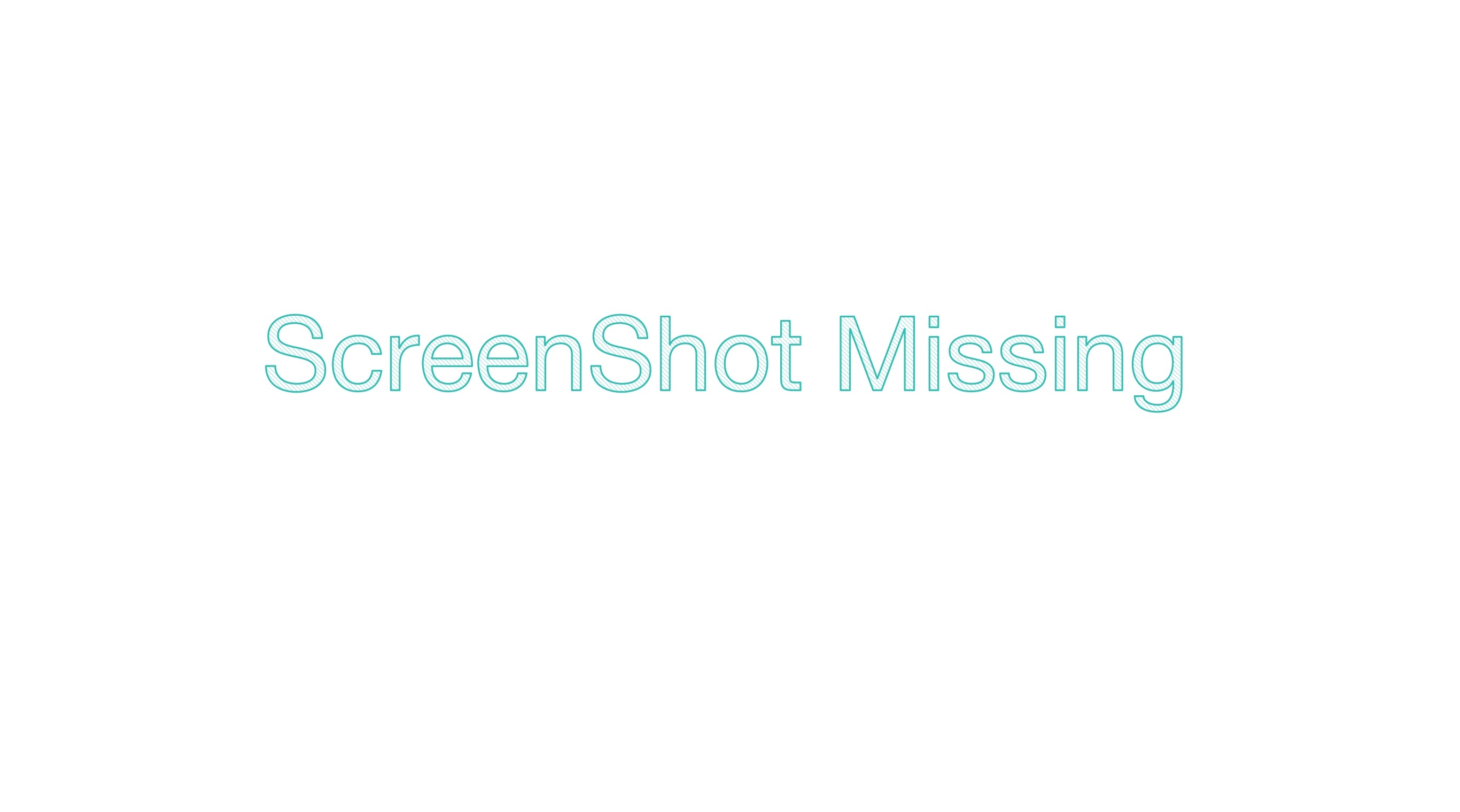
Qwen
Qwen is a series of large language models developed by Alibaba DAMO Academy. It outperforms the baseline models of similar model sizes on a series of benchmark datasets, e.g., MMLU, C-Eval, GSM8K, MATH, HumanEval, MBPP, BBH, etc., which evaluate the models’ capabilities on natural language understanding, mathematic problem solving, coding, etc. Qwen models outperform the baseline models of similar model sizes on a series of benchmark datasets, e.g., MMLU, C-Eval, GSM8K, MATH, HumanEval, MBPP, BBH, etc., which evaluate the models’ capabilities on natural language understanding, mathematic problem solving, coding, etc. Qwen-72B achieves better performance than LLaMA2-70B on all tasks and outperforms GPT-3.5 on 7 out of 10 tasks.
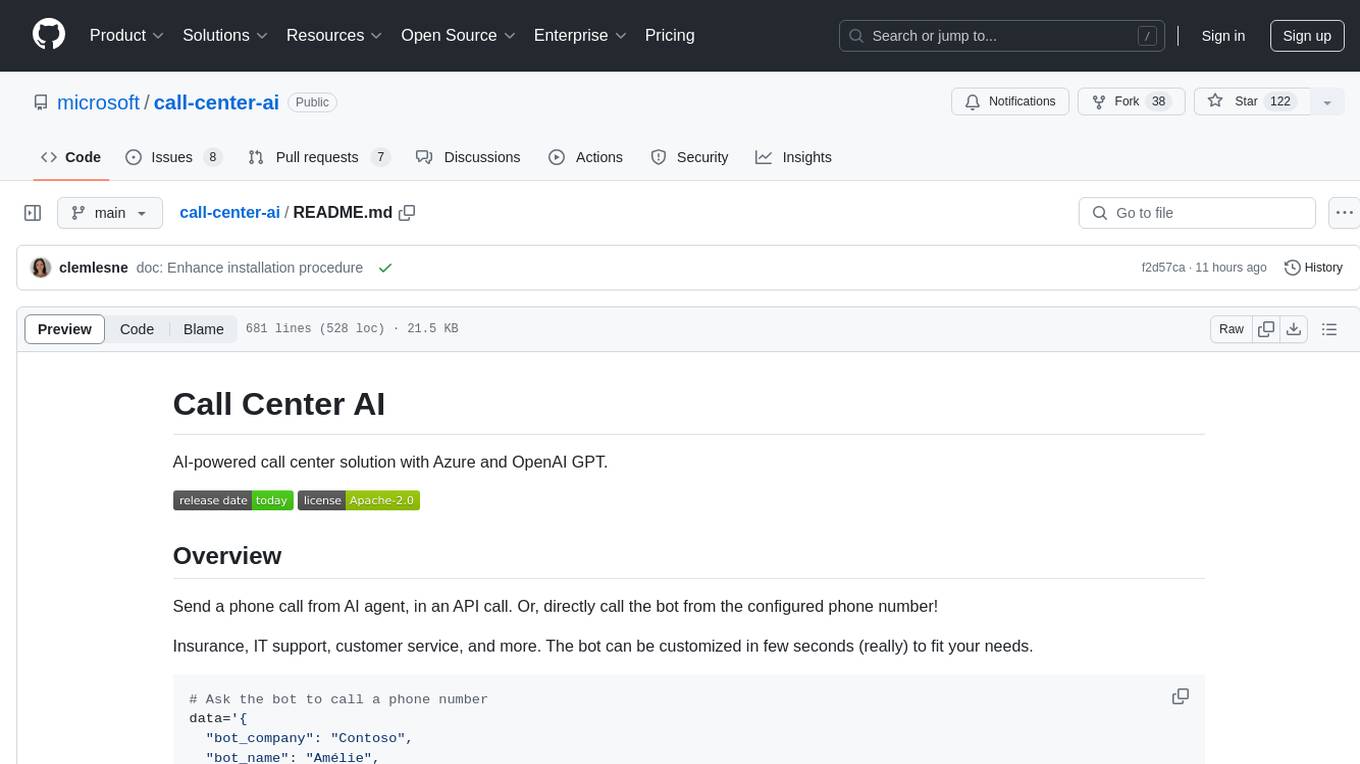
call-center-ai
Call Center AI is an AI-powered call center solution leveraging Azure and OpenAI GPT. It allows for AI agent-initiated phone calls or direct calls to the bot from a configured phone number. The bot is customizable for various industries like insurance, IT support, and customer service, with features such as accessing claim information, conversation history, language change, SMS sending, and more. The project is a proof of concept showcasing the integration of Azure Communication Services, Azure Cognitive Services, and Azure OpenAI for an automated call center solution.
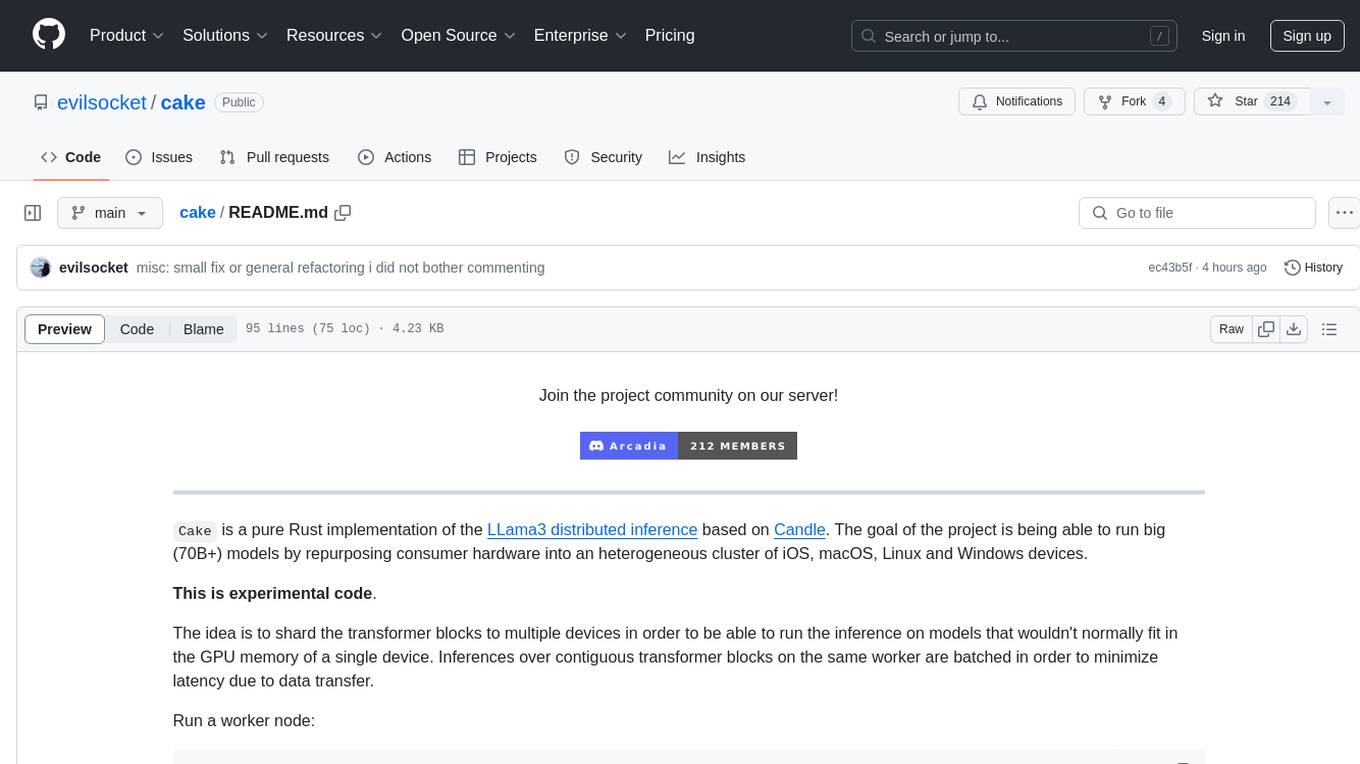
cake
cake is a pure Rust implementation of the llama3 LLM distributed inference based on Candle. The project aims to enable running large models on consumer hardware clusters of iOS, macOS, Linux, and Windows devices by sharding transformer blocks. It allows running inferences on models that wouldn't fit in a single device's GPU memory by batching contiguous transformer blocks on the same worker to minimize latency. The tool provides a way to optimize memory and disk space by splitting the model into smaller bundles for workers, ensuring they only have the necessary data. cake supports various OS, architectures, and accelerations, with different statuses for each configuration.
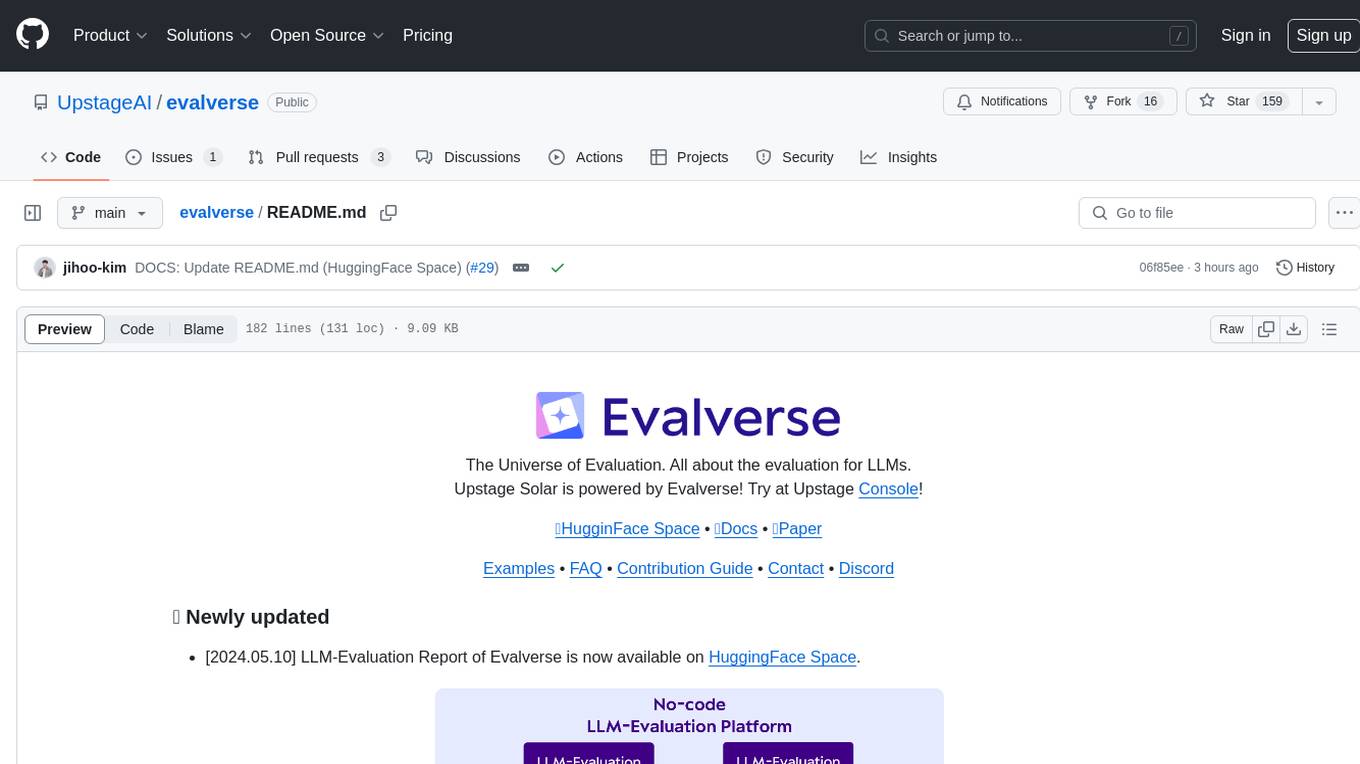
evalverse
Evalverse is an open-source project designed to support Large Language Model (LLM) evaluation needs. It provides a standardized and user-friendly solution for processing and managing LLM evaluations, catering to AI research engineers and scientists. Evalverse supports various evaluation methods, insightful reports, and no-code evaluation processes. Users can access unified evaluation with submodules, request evaluations without code via Slack bot, and obtain comprehensive reports with scores, rankings, and visuals. The tool allows for easy comparison of scores across different models and swift addition of new evaluation tools.
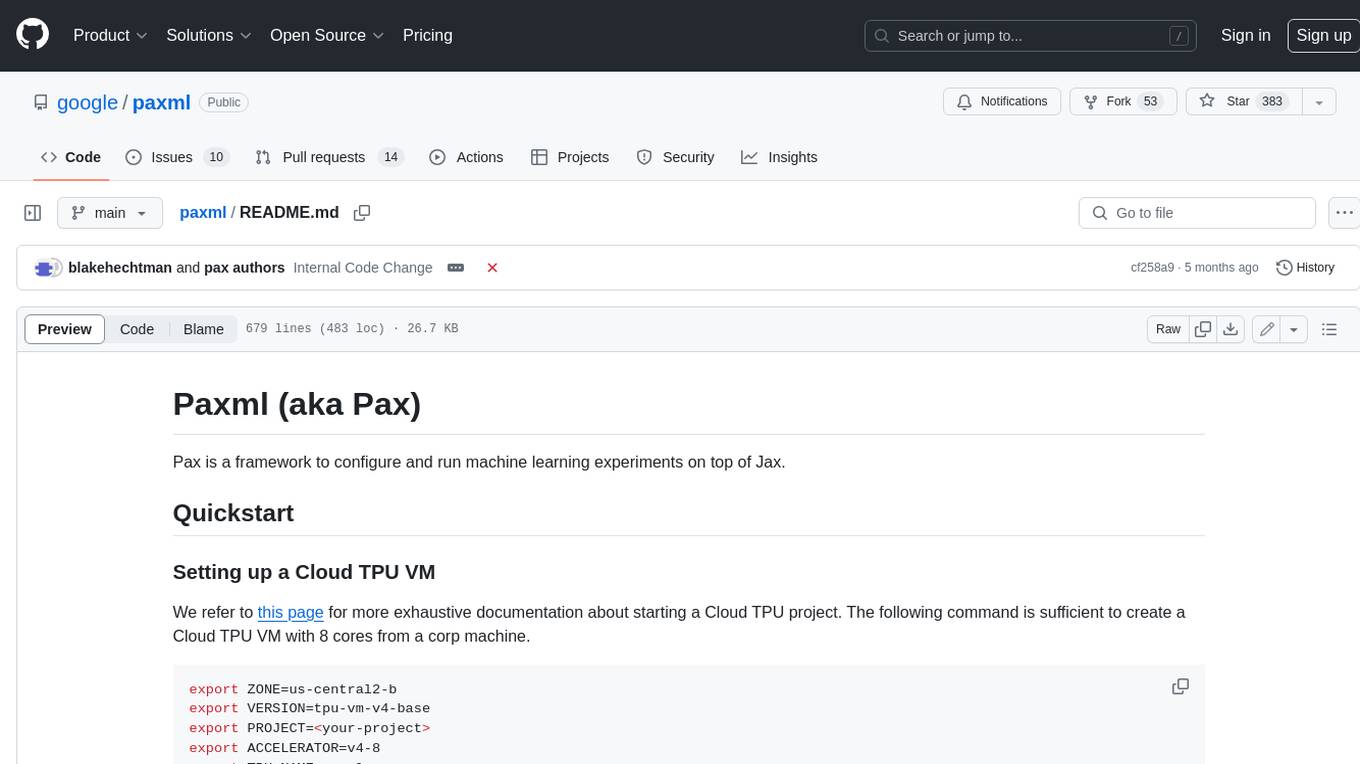
paxml
Pax is a framework to configure and run machine learning experiments on top of Jax.
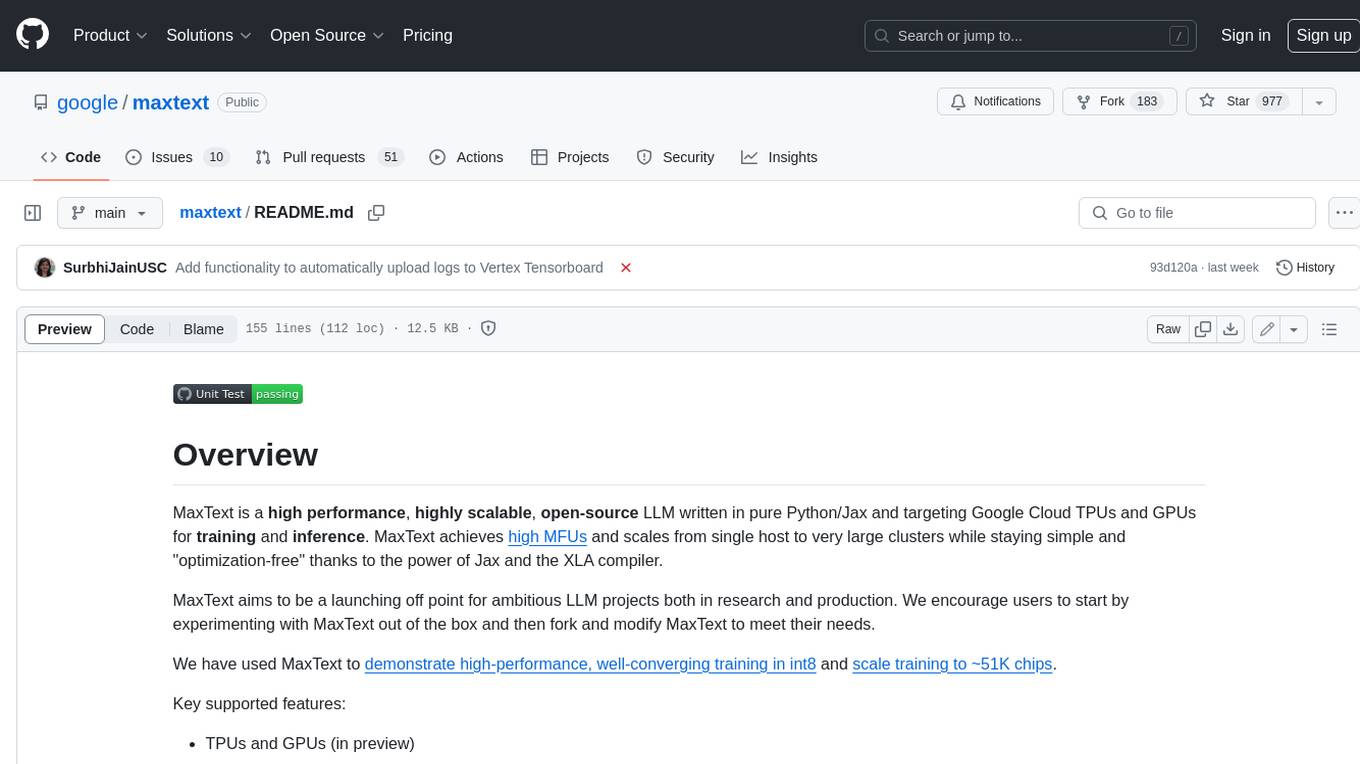
maxtext
MaxText is a high-performance, highly scalable, open-source LLM written in pure Python/Jax and targeting Google Cloud TPUs and GPUs for training and inference. MaxText achieves high MFUs and scales from single host to very large clusters while staying simple and "optimization-free" thanks to the power of Jax and the XLA compiler. MaxText aims to be a launching off point for ambitious LLM projects both in research and production. We encourage users to start by experimenting with MaxText out of the box and then fork and modify MaxText to meet their needs.
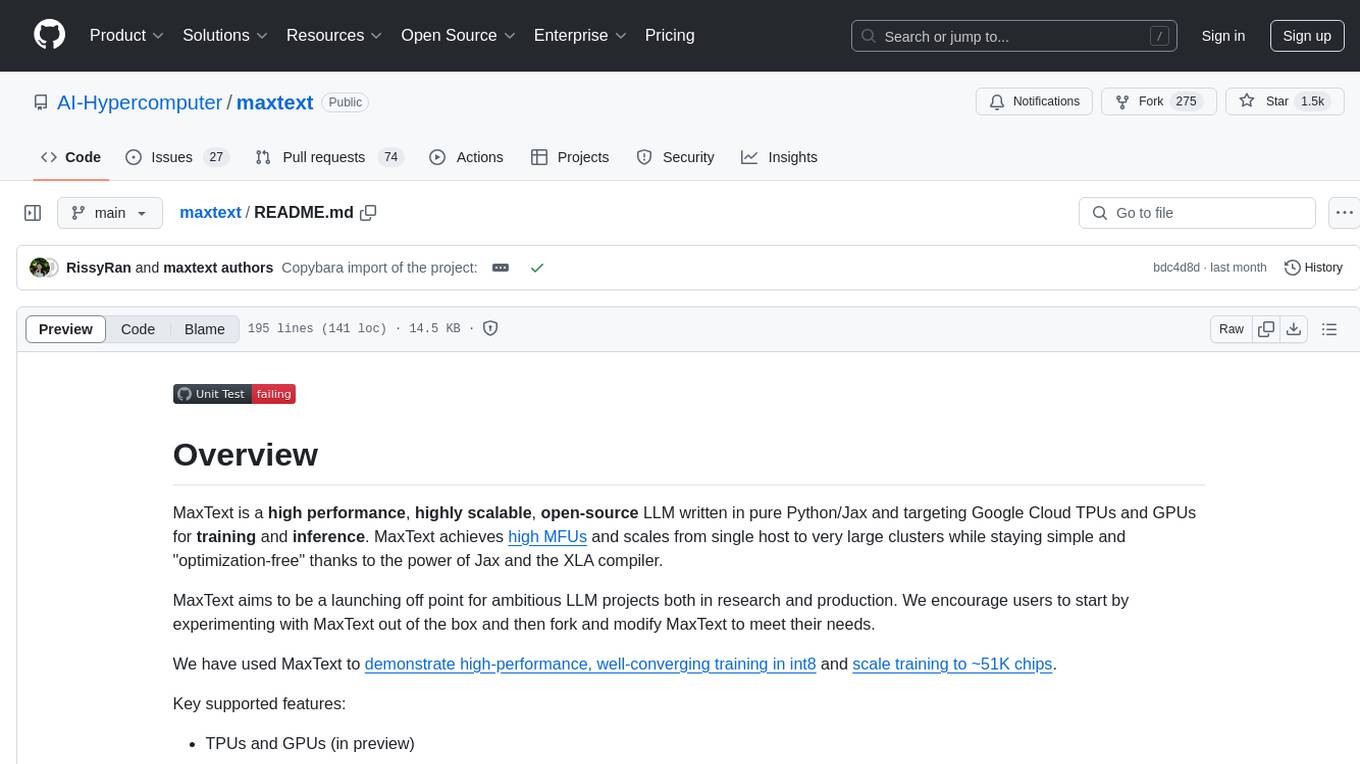
maxtext
MaxText is a high performance, highly scalable, open-source Large Language Model (LLM) written in pure Python/Jax targeting Google Cloud TPUs and GPUs for training and inference. It aims to be a launching off point for ambitious LLM projects in research and production, supporting TPUs and GPUs, models like Llama2, Mistral, and Gemma. MaxText provides specific instructions for getting started, runtime performance results, comparison to alternatives, and features like stack trace collection, ahead of time compilation for TPUs and GPUs, and automatic upload of logs to Vertex Tensorboard.
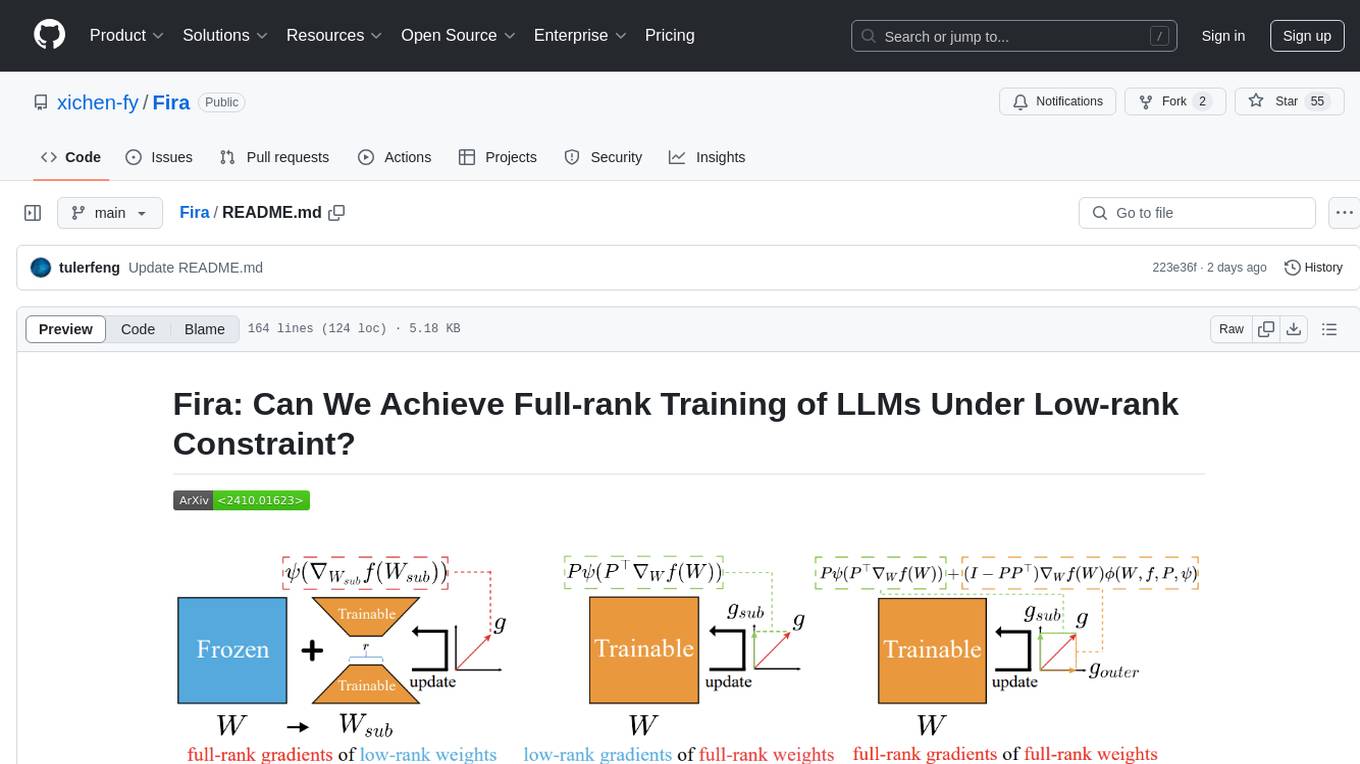
Fira
Fira is a memory-efficient training framework for Large Language Models (LLMs) that enables full-rank training under low-rank constraint. It introduces a method for training with full-rank gradients of full-rank weights, achieved with just two lines of equations. The framework includes pre-training and fine-tuning functionalities, packaged as a Python library for easy use. Fira utilizes Adam optimizer by default and provides options for weight decay. It supports pre-training LLaMA models on the C4 dataset and fine-tuning LLaMA-7B models on commonsense reasoning tasks.
For similar tasks
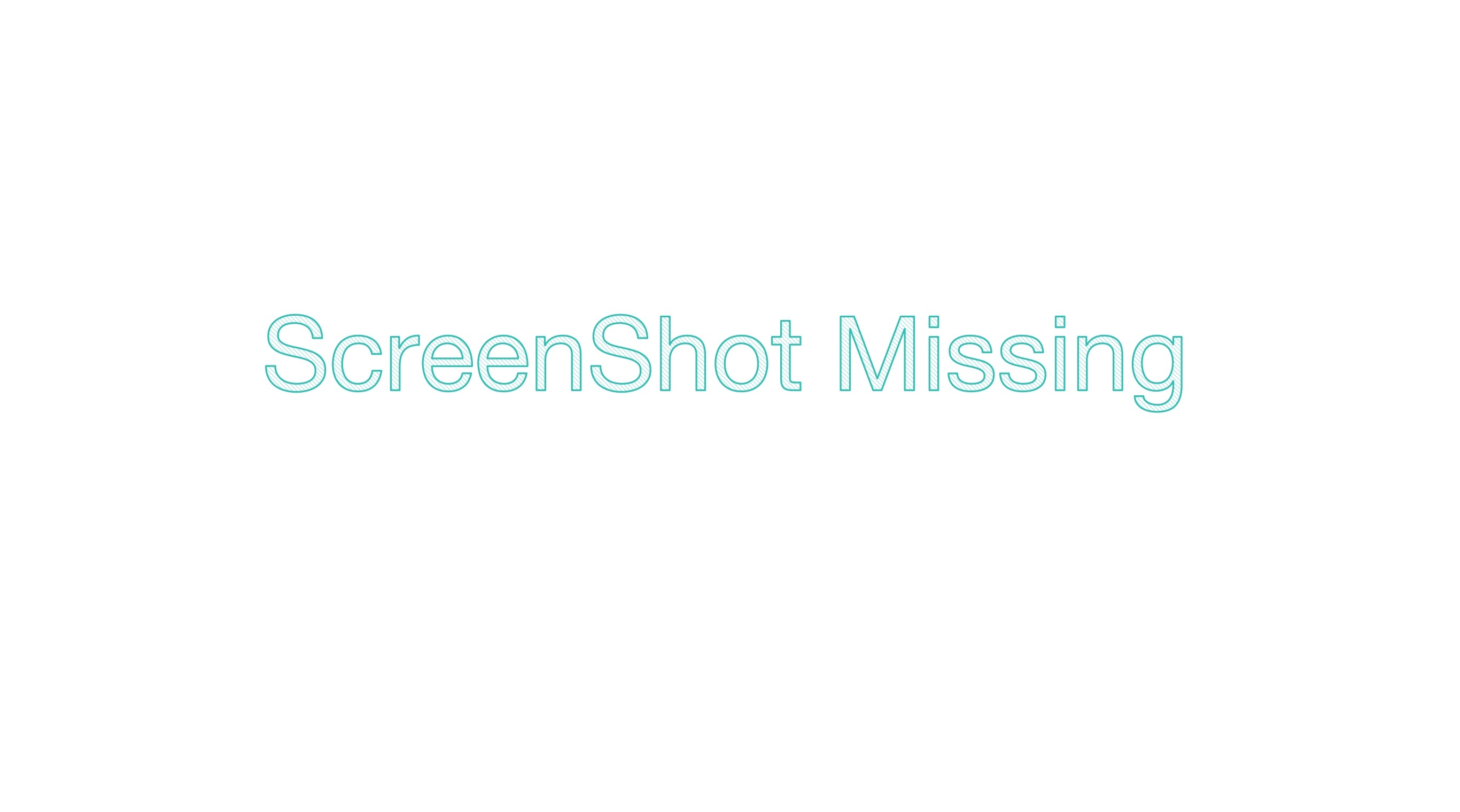
moatless-tools
Moatless Tools is a hobby project focused on experimenting with using Large Language Models (LLMs) to edit code in large existing codebases. The project aims to build tools that insert the right context into prompts and handle responses effectively. It utilizes an agentic loop functioning as a finite state machine to transition between states like Search, Identify, PlanToCode, ClarifyChange, and EditCode for code editing tasks.
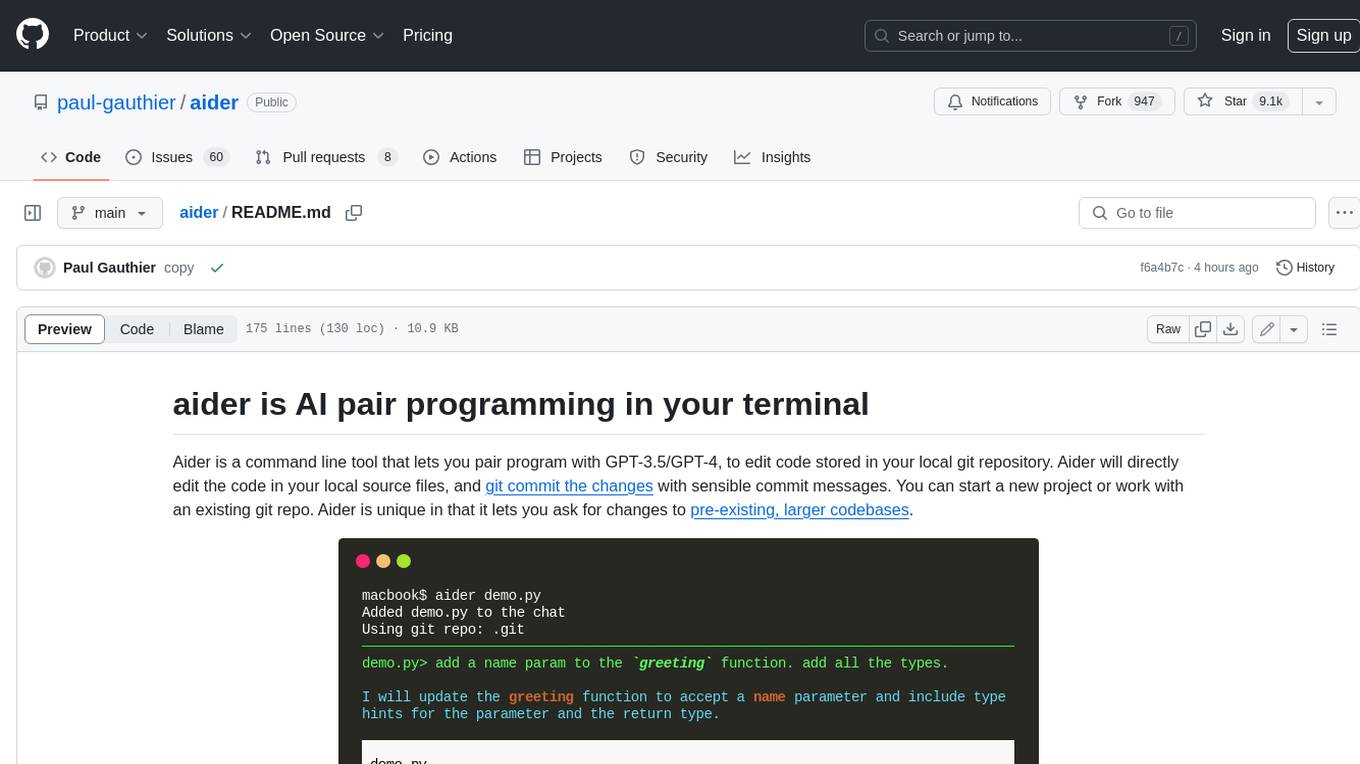
aider
Aider is a command-line tool that lets you pair program with GPT-3.5/GPT-4 to edit code stored in your local git repository. Aider will directly edit the code in your local source files and git commit the changes with sensible commit messages. You can start a new project or work with an existing git repo. Aider is unique in that it lets you ask for changes to pre-existing, larger codebases.
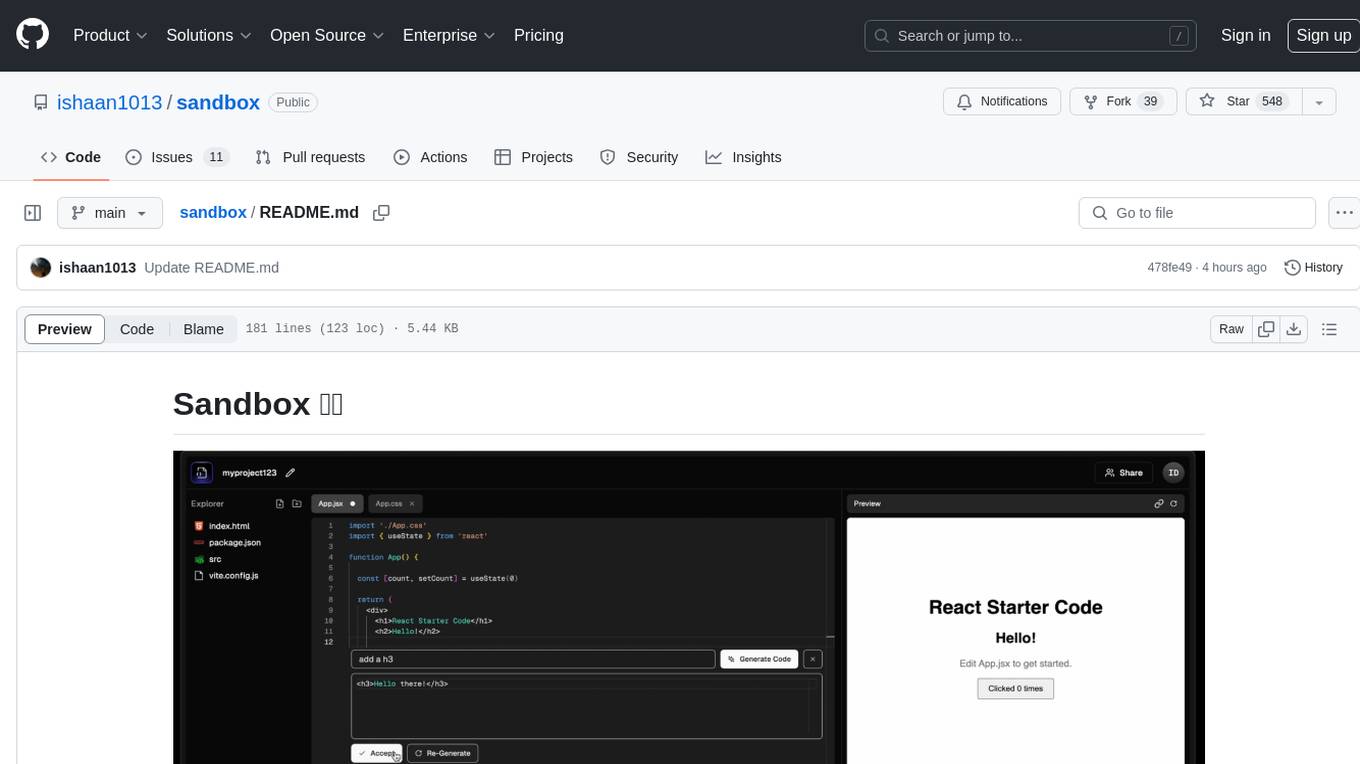
sandbox
Sandbox is an open-source cloud-based code editing environment with custom AI code autocompletion and real-time collaboration. It consists of a frontend built with Next.js, TailwindCSS, Shadcn UI, Clerk, Monaco, and Liveblocks, and a backend with Express, Socket.io, Cloudflare Workers, D1 database, R2 storage, Workers AI, and Drizzle ORM. The backend includes microservices for database, storage, and AI functionalities. Users can run the project locally by setting up environment variables and deploying the containers. Contributions are welcome following the commit convention and structure provided in the repository.
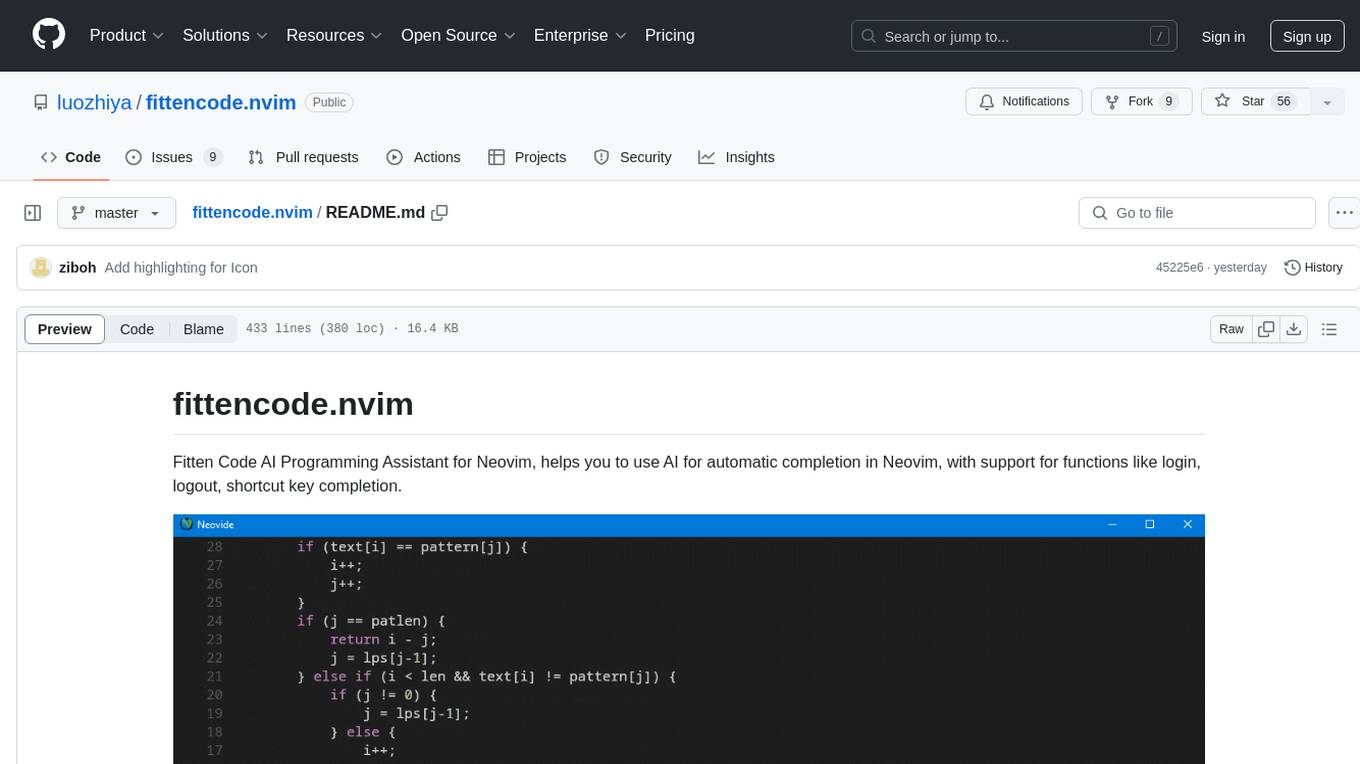
fittencode.nvim
Fitten Code AI Programming Assistant for Neovim provides fast completion using AI, asynchronous I/O, and support for various actions like document code, edit code, explain code, find bugs, generate unit test, implement features, optimize code, refactor code, start chat, and more. It offers features like accepting suggestions with Tab, accepting line with Ctrl + Down, accepting word with Ctrl + Right, undoing accepted text, automatic scrolling, and multiple HTTP/REST backends. It can run as a coc.nvim source or nvim-cmp source.
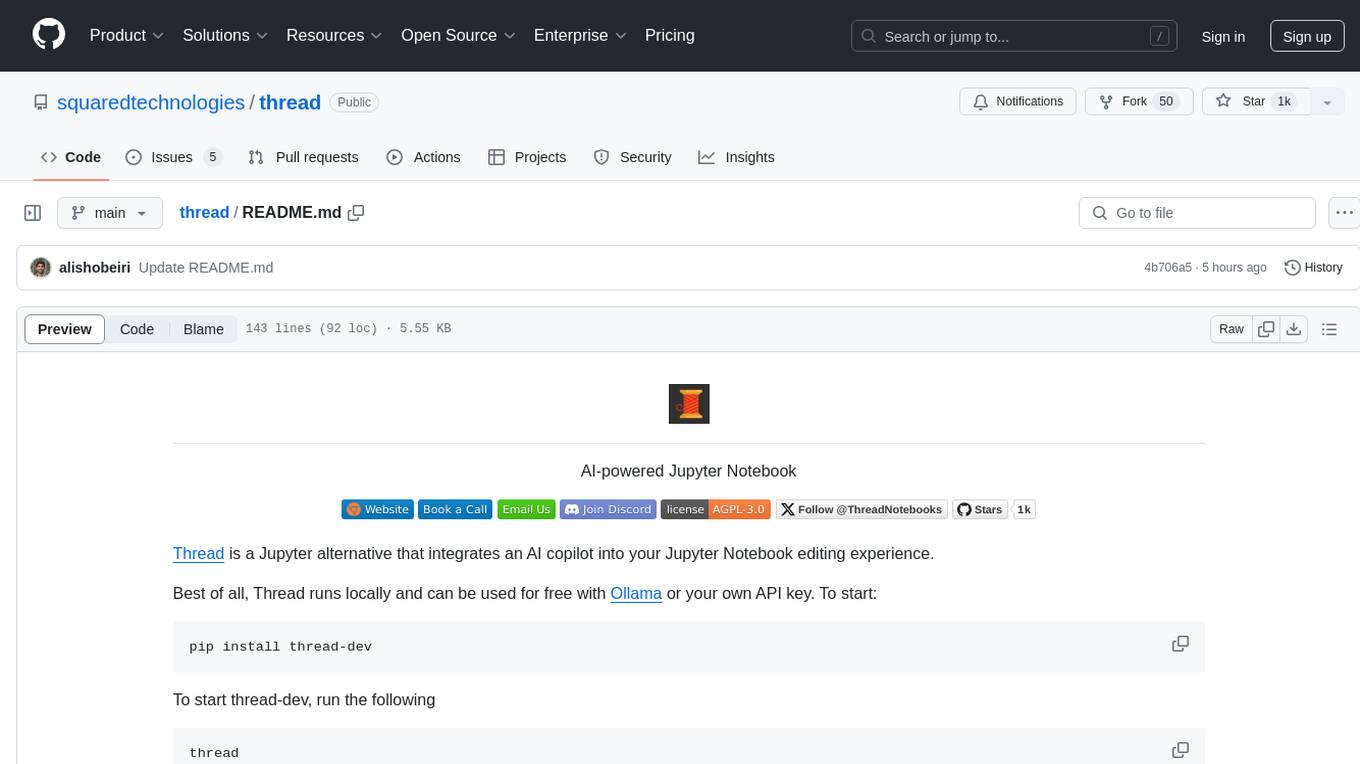
thread
Thread is an AI-powered Jupyter alternative that integrates an AI copilot into your editing experience. It offers a familiar Jupyter Notebook editing experience with features like natural language code edits, generating cells to answer questions, context-aware chat sidebar, and automatic error explanations or fixes. The tool aims to enhance code editing and data exploration by providing a more interactive and intuitive experience for users. Thread can be used for free with Ollama or your own API key, and it runs locally for convenience and privacy.
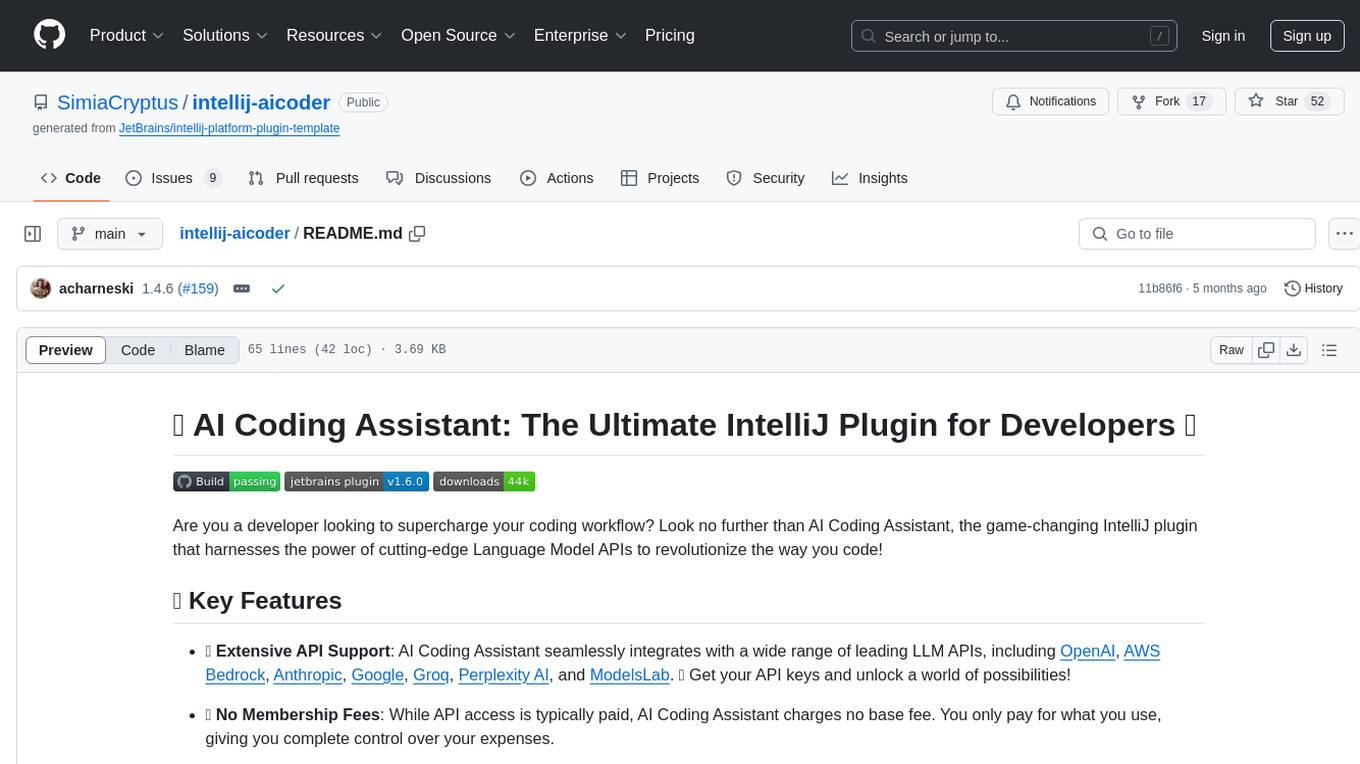
intellij-aicoder
AI Coding Assistant is a free and open-source IntelliJ plugin that leverages cutting-edge Language Model APIs to enhance developers' coding experience. It seamlessly integrates with various leading LLM APIs, offers an intuitive toolbar UI, and allows granular control over API requests. With features like Code & Patch Chat, Planning with AI Agents, Markdown visualization, and versatile text processing capabilities, this tool aims to streamline coding workflows and boost productivity.
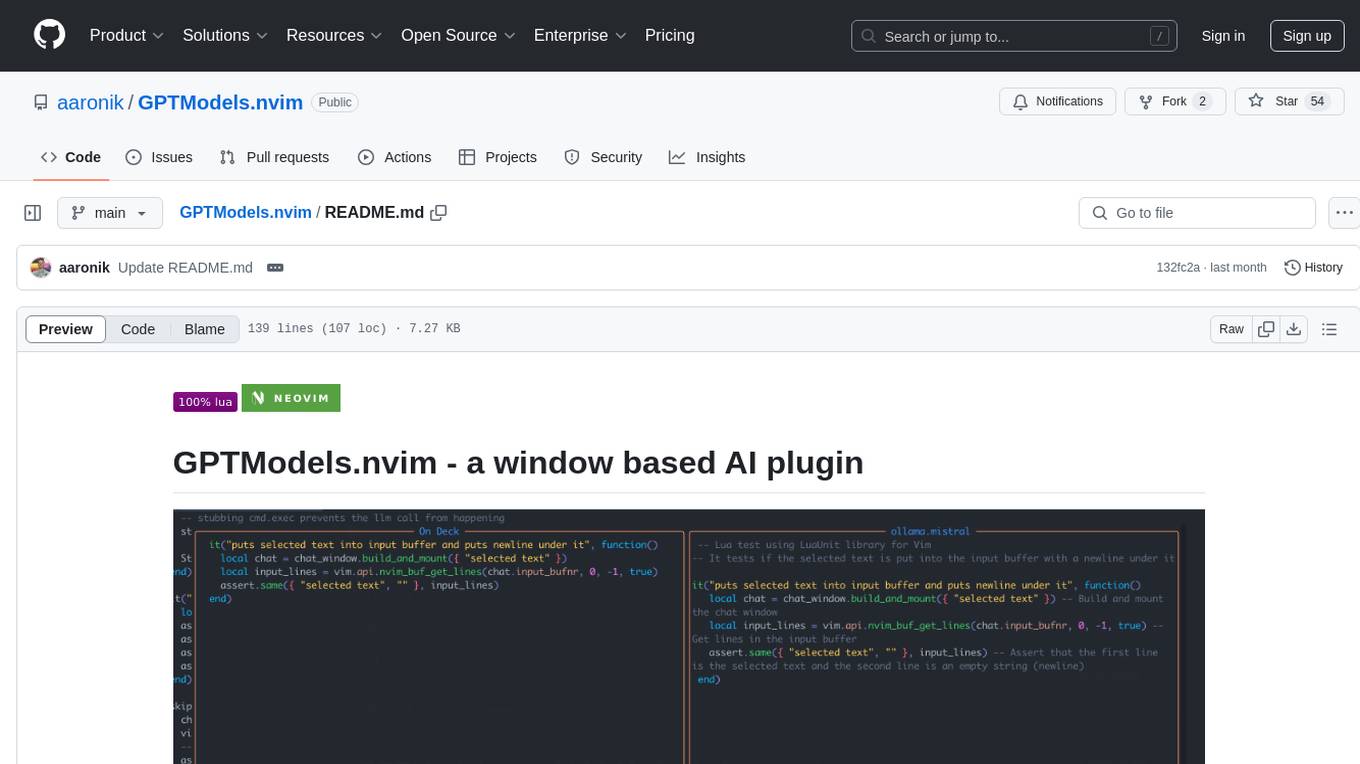
GPTModels.nvim
GPTModels.nvim is a window-based AI plugin for Neovim that enhances workflow with AI LLMs. It provides two popup windows for chat and code editing, focusing on stability and user experience. The plugin supports OpenAI and Ollama, includes LSP diagnostics, file inclusion, background processing, request cancellation, selection inclusion, and filetype inclusion. Developed with stability in mind, the plugin offers a seamless user experience with various features to streamline AI integration in Neovim.
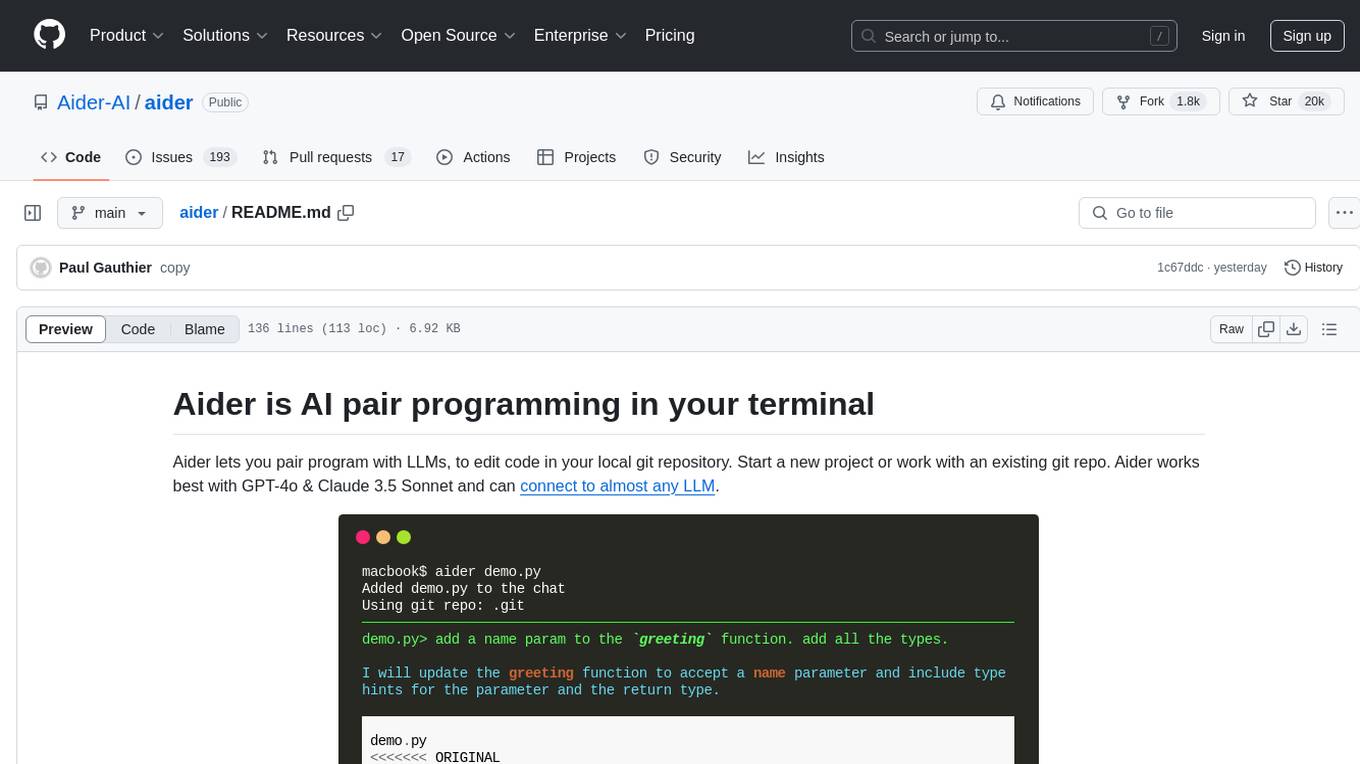
aider
Aider is an AI pair programming tool that allows users to collaborate with large language models (LLMs) to edit code in their local git repository. It works best with GPT-4o & Claude 3.5 Sonnet and can connect to almost any LLM. Users can run Aider with specific files, request changes, add new features or test cases, describe bugs, refactor code, update docs, and more. Aider automatically commits changes with sensible messages, supports multiple programming languages, and can handle complex requests by editing multiple files at once. It uses a map of the entire git repo for efficient performance in larger codebases. Users can chat with Aider, add images, URLs, and even code with their voice. Aider has achieved top scores on SWE Bench, solving real GitHub issues from popular open source projects like django, scikitlearn, matplotlib, etc.
For similar jobs
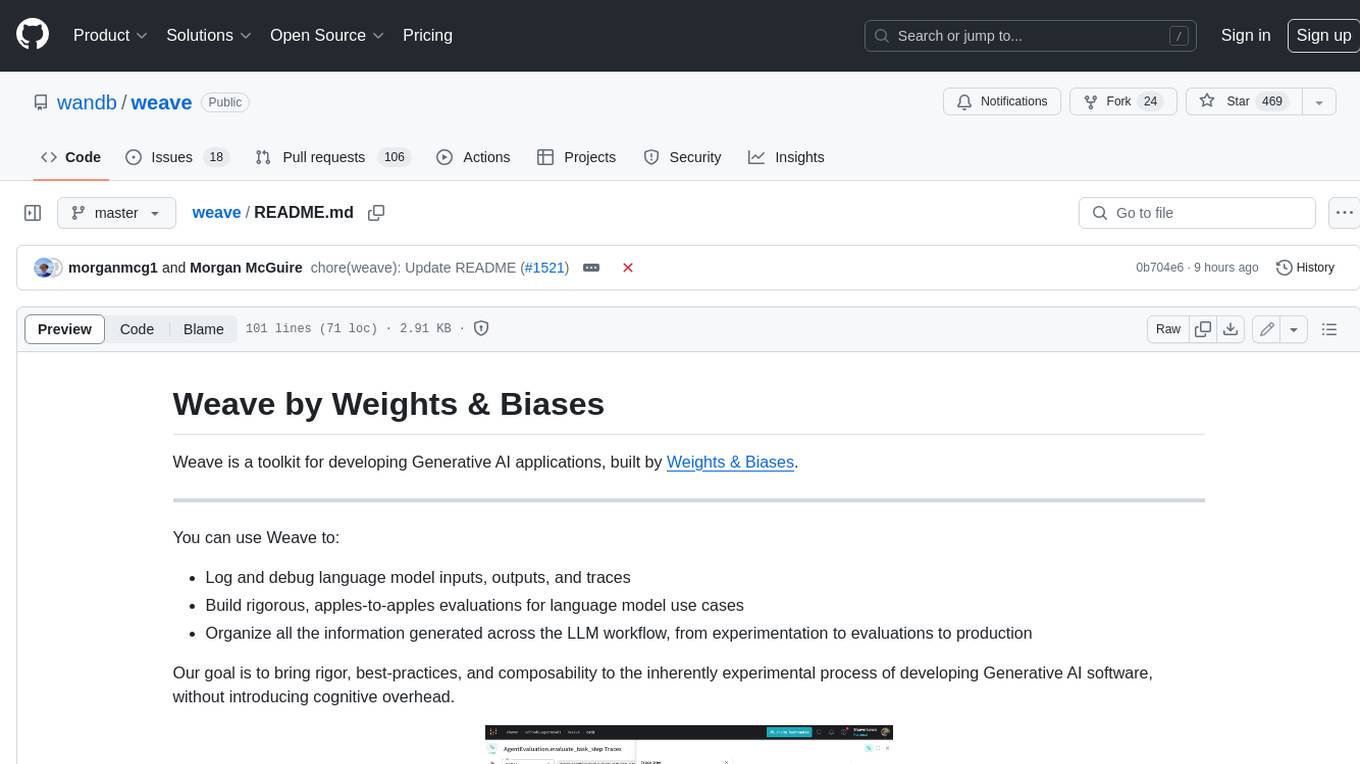
weave
Weave is a toolkit for developing Generative AI applications, built by Weights & Biases. With Weave, you can log and debug language model inputs, outputs, and traces; build rigorous, apples-to-apples evaluations for language model use cases; and organize all the information generated across the LLM workflow, from experimentation to evaluations to production. Weave aims to bring rigor, best-practices, and composability to the inherently experimental process of developing Generative AI software, without introducing cognitive overhead.
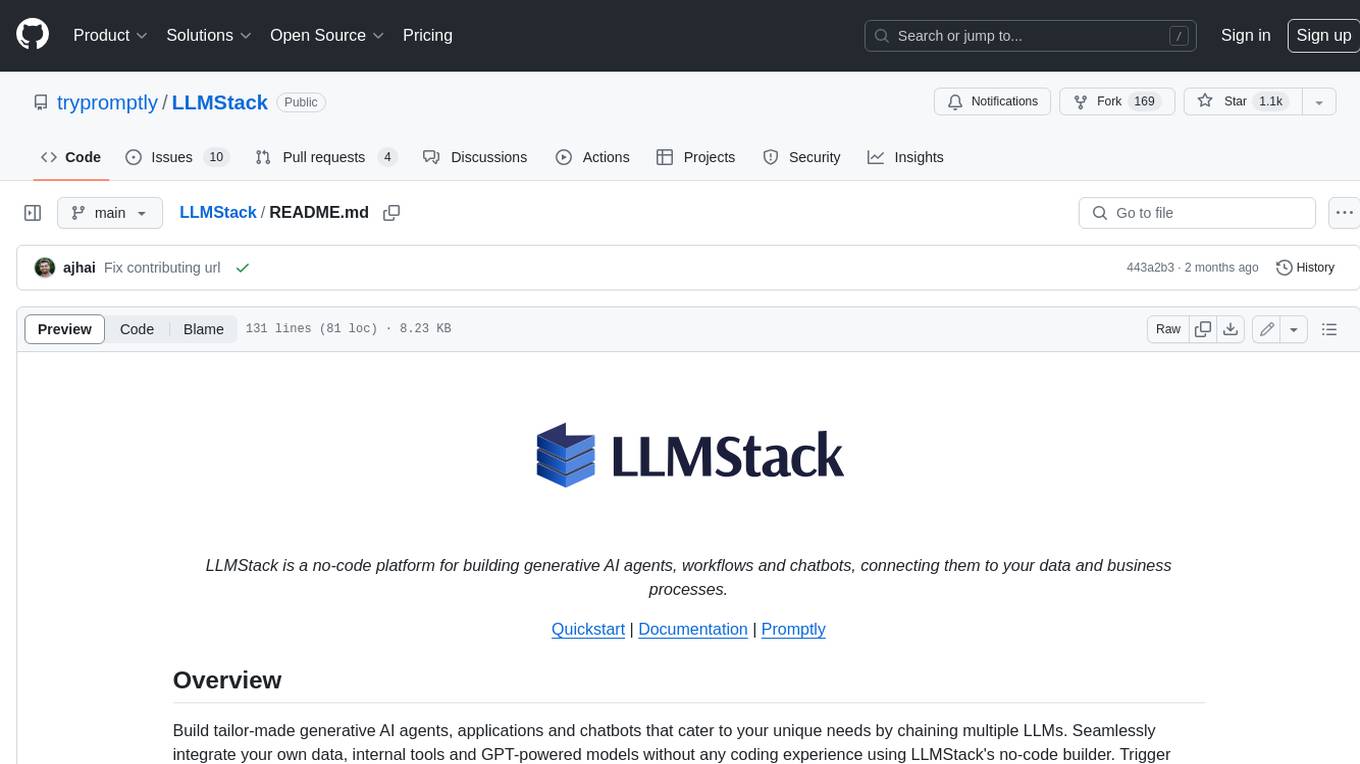
LLMStack
LLMStack is a no-code platform for building generative AI agents, workflows, and chatbots. It allows users to connect their own data, internal tools, and GPT-powered models without any coding experience. LLMStack can be deployed to the cloud or on-premise and can be accessed via HTTP API or triggered from Slack or Discord.
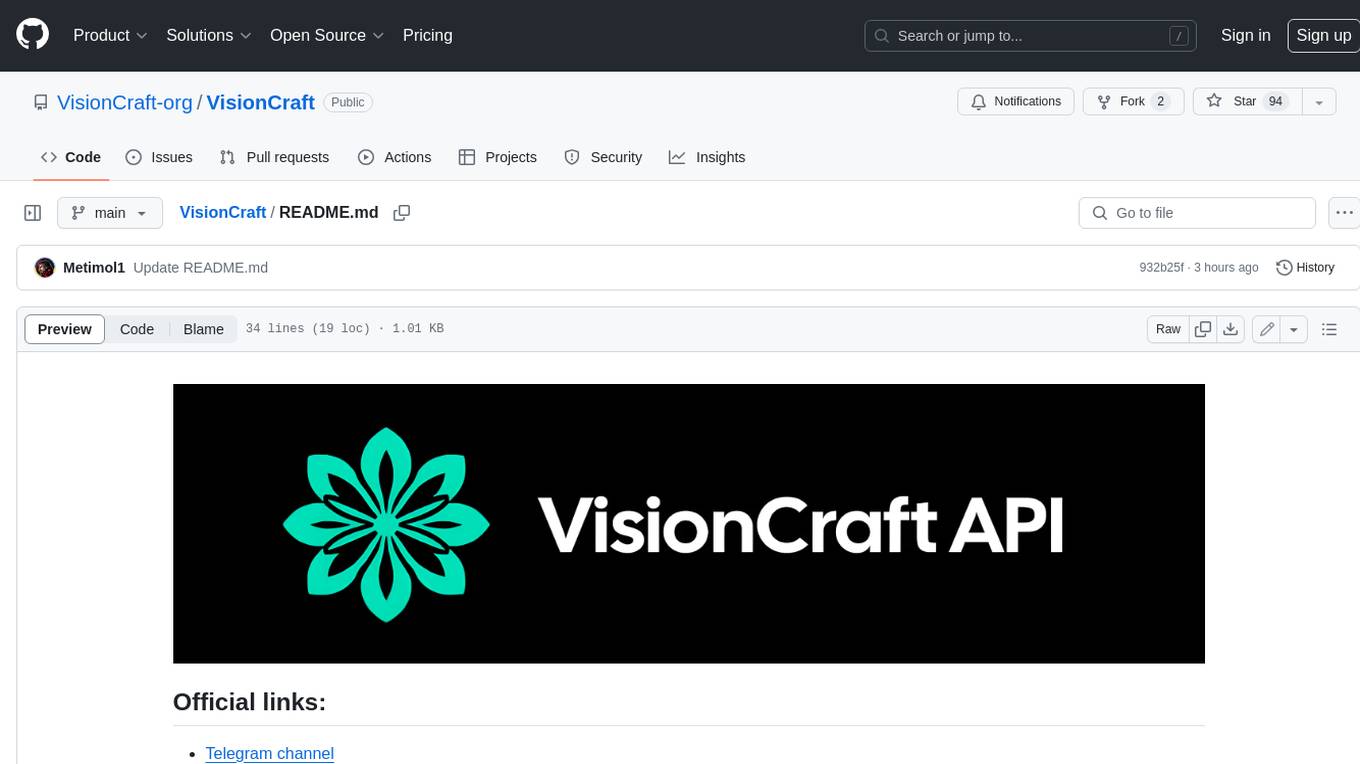
VisionCraft
The VisionCraft API is a free API for using over 100 different AI models. From images to sound.
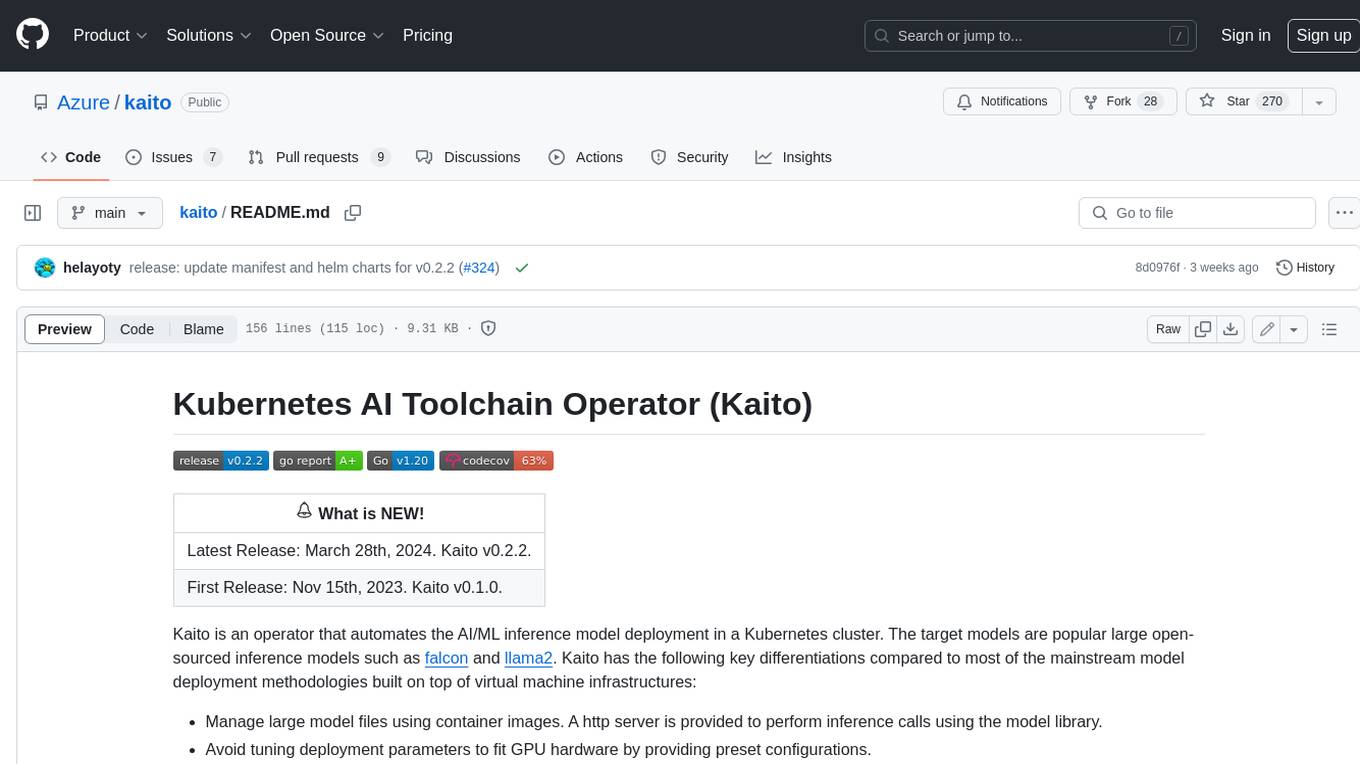
kaito
Kaito is an operator that automates the AI/ML inference model deployment in a Kubernetes cluster. It manages large model files using container images, avoids tuning deployment parameters to fit GPU hardware by providing preset configurations, auto-provisions GPU nodes based on model requirements, and hosts large model images in the public Microsoft Container Registry (MCR) if the license allows. Using Kaito, the workflow of onboarding large AI inference models in Kubernetes is largely simplified.
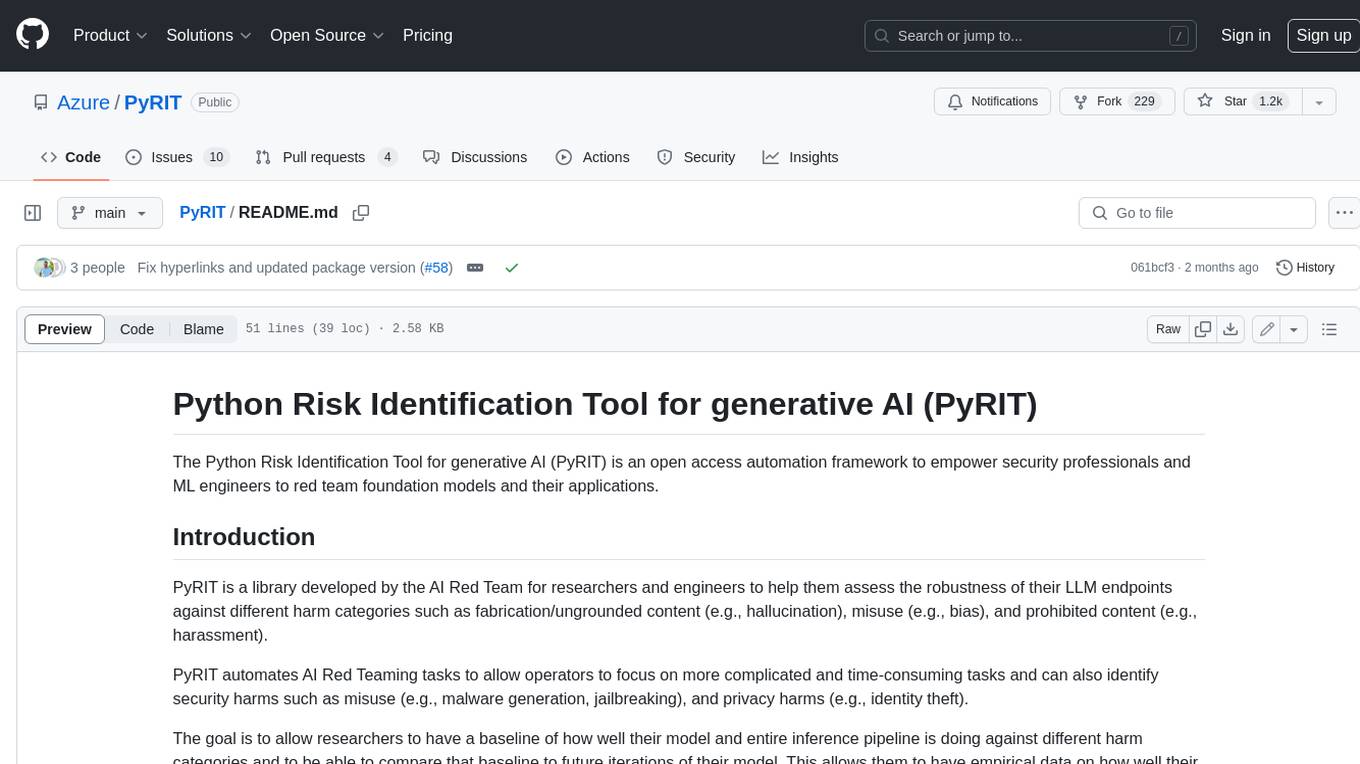
PyRIT
PyRIT is an open access automation framework designed to empower security professionals and ML engineers to red team foundation models and their applications. It automates AI Red Teaming tasks to allow operators to focus on more complicated and time-consuming tasks and can also identify security harms such as misuse (e.g., malware generation, jailbreaking), and privacy harms (e.g., identity theft). The goal is to allow researchers to have a baseline of how well their model and entire inference pipeline is doing against different harm categories and to be able to compare that baseline to future iterations of their model. This allows them to have empirical data on how well their model is doing today, and detect any degradation of performance based on future improvements.
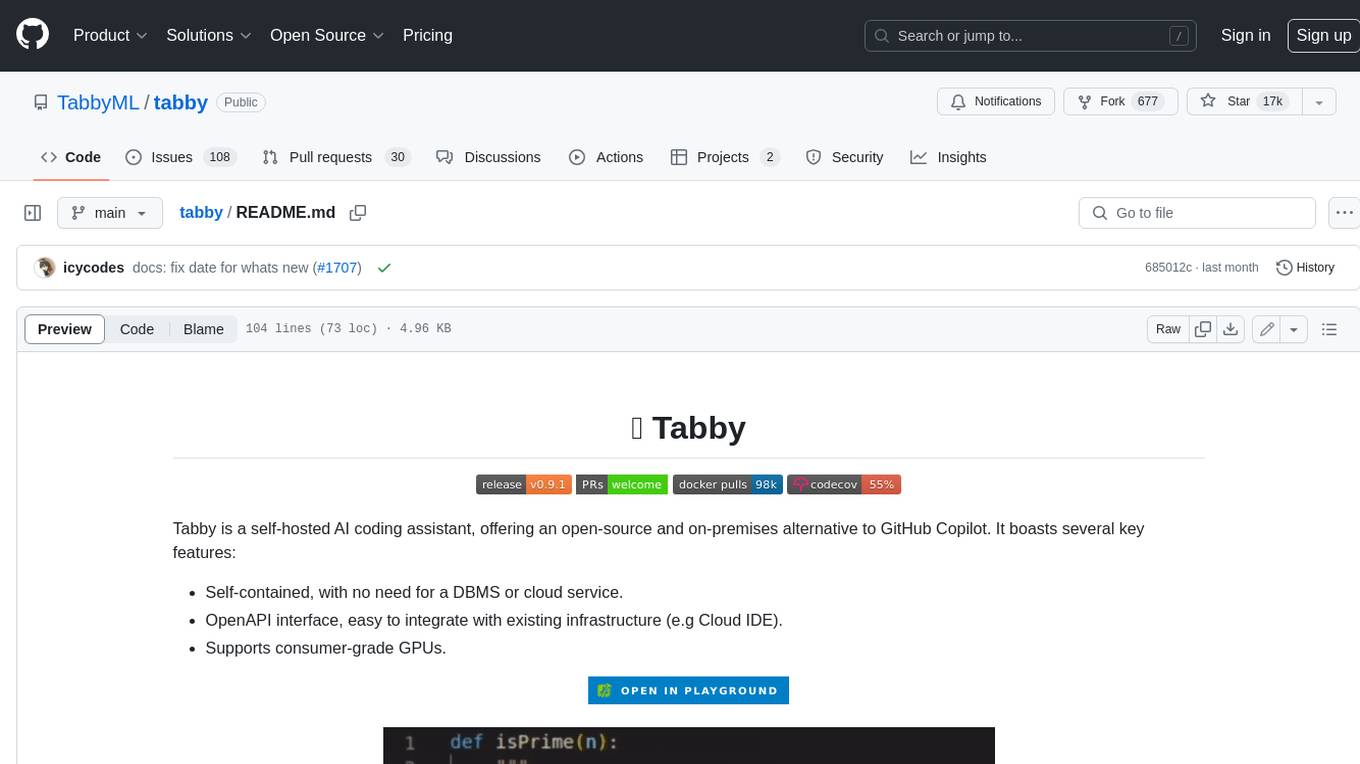
tabby
Tabby is a self-hosted AI coding assistant, offering an open-source and on-premises alternative to GitHub Copilot. It boasts several key features: * Self-contained, with no need for a DBMS or cloud service. * OpenAPI interface, easy to integrate with existing infrastructure (e.g Cloud IDE). * Supports consumer-grade GPUs.
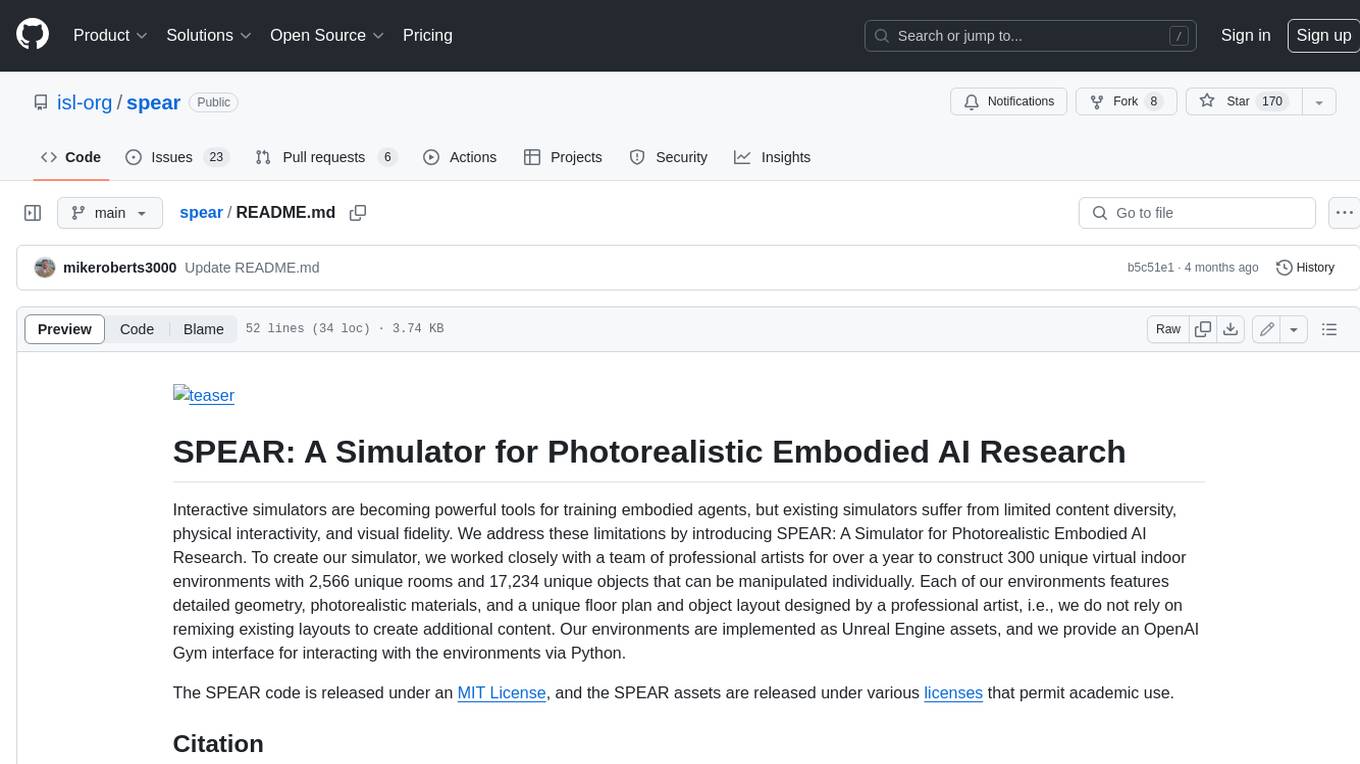
spear
SPEAR (Simulator for Photorealistic Embodied AI Research) is a powerful tool for training embodied agents. It features 300 unique virtual indoor environments with 2,566 unique rooms and 17,234 unique objects that can be manipulated individually. Each environment is designed by a professional artist and features detailed geometry, photorealistic materials, and a unique floor plan and object layout. SPEAR is implemented as Unreal Engine assets and provides an OpenAI Gym interface for interacting with the environments via Python.
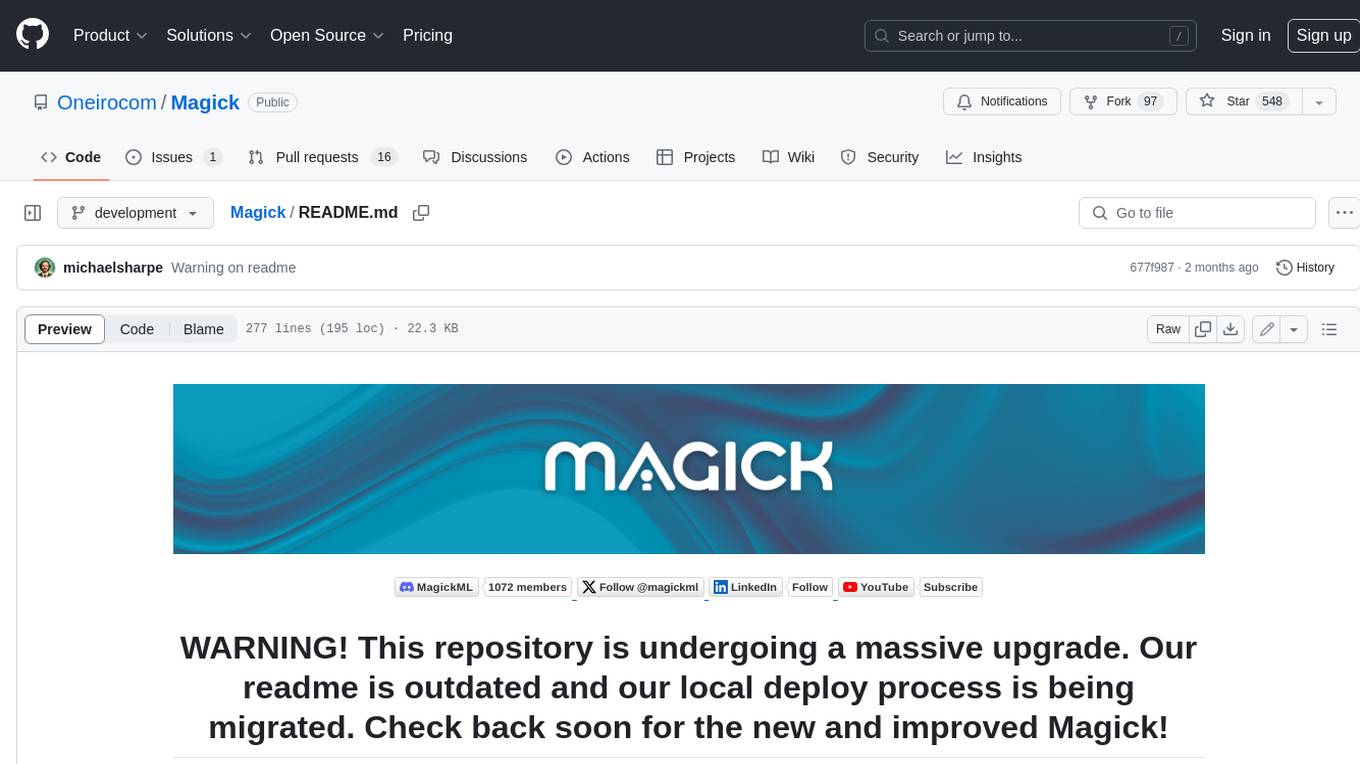
Magick
Magick is a groundbreaking visual AIDE (Artificial Intelligence Development Environment) for no-code data pipelines and multimodal agents. Magick can connect to other services and comes with nodes and templates well-suited for intelligent agents, chatbots, complex reasoning systems and realistic characters.