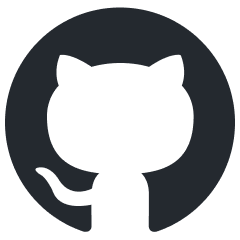
Large-Language-Models-play-StarCraftII
TextStarCraft2,a pure language env which support llms play starcraft2
Stars: 152
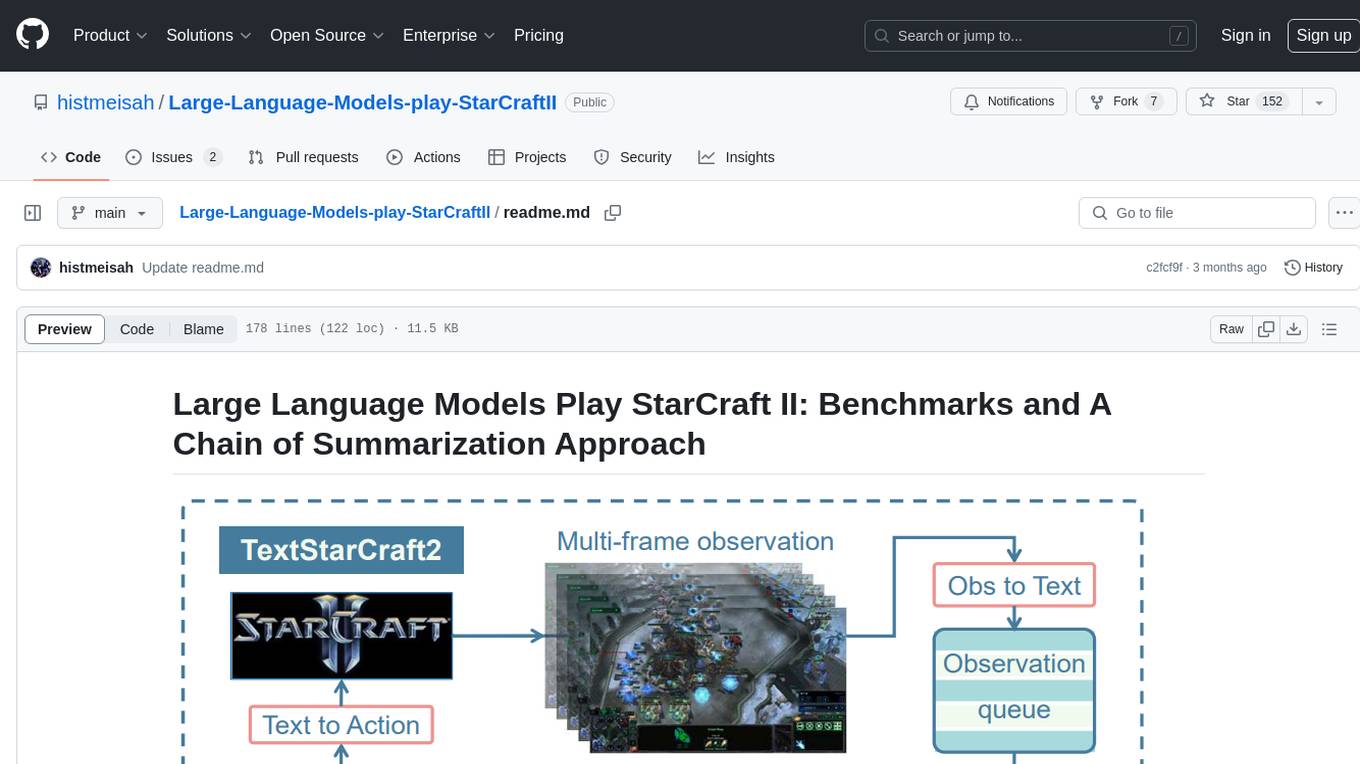
Large Language Models Play StarCraft II is a project that explores the capabilities of large language models (LLMs) in playing the game StarCraft II. The project introduces TextStarCraft II, a textual environment for the game, and a Chain of Summarization method for analyzing game information and making strategic decisions. Through experiments, the project demonstrates that LLM agents can defeat the built-in AI at a challenging difficulty level. The project provides benchmarks and a summarization approach to enhance strategic planning and interpretability in StarCraft II gameplay.
README:
StarCraft II is a challenging benchmark for AI agents due to micro-level operations and macro-awareness. Previous works, such as Alphastar and SCC, achieve impressive performance on tackling StarCraft
II , however, still exhibit deficiencies in long-term strategic planning and strategy interpretability. Emerging large language model (LLM) agents, presents the immense potential in solving intricate tasks.
Motivated by this, we aim to validate the capabilities of LLMs on StarCraft II. We first develop textual StratCraft II environment, called TextStarCraft II. Secondly, we propose a Chain of Summarization method, including single-frame summarization for processing raw observations and multi-frame summarization for analyzing game information, providing command recommendations, and generating strategic decisions. Our experiment demonstrates that LLM agents are capable of defeating the built-in AI at the Harder(Lv5) difficulty level.
Work | AlphaStar | SCC | HierNet-SC2 | AlphaStar Unplugged | ROA-Star | Ours |
---|---|---|---|---|---|---|
Method | SL+RL+self-play | SL+RL+self-play | data-mining + RL | offline RL | SL+RL+self-play | prompt + Rule base script |
Compute resource | 12000 CPU cores, 384 TPUs | Linear | 4 GPUs,48 CPU cores | not clear | 2x 64 v100 | 1 gpu,1 cpu(home computer) |
Required replay | 971,000 | 4,638 | 608 | 20,000,000(20m) | 120938 | 0 |
Best result(The greatest opponent ever to win) | Serral(One of the best progamer in the world) | Time(IEM2023 Champion) | build-in ai lv-10 | AlphaStar BC agent | hero(GSL Champion) | build-in ai lv-5 |
Strategy Interpretability | ✖ | ✖ | ✖ | ✖ | ✖ | ✔ |
Expansibility(adapt to latest game version and other race ) | ✖ | ✖ | ✖ | ✖ | ✖ | ✔ |
Our paper:
Large Language Models Play StarCraft II: Benchmarks and A Chain of Summarization Approach https://arxiv.org/abs/2312.11865
Our website:
Large Language Models Play StarCraft II: Benchmarks and A Chain of Summarization Approach
Our demo video:
Large Language Models Play StarCraft II: Benchmarks and A Chain of Summarization Approach
Comparing models using either the full CoS or CoS without CoT.
Model | Method | Win Rate | PBR | RUR | APU | TR |
---|---|---|---|---|---|---|
Using Full CoS | ||||||
GPT3.5-Turbo-16k | Full CoS | 5/10 | 0.0781 | 7875 | 0.7608 | 0.4476 |
GPT4-Turbo | Full CoS | 3/6 | 0.0337 | 8306 | 0.7194 | 0.3452 |
Gemini-Pro | Full CoS | 2/10 | 0.0318 | 9284 | 0.6611 | 0.3571 |
GLM4 | Full CoS | 2/10 | 0.0327 | 3131 | 0.6644 | 0.2904 |
Llama2 70B | Full CoS | / | / | / | / | / |
Claude2.1 | Full CoS | 2/9 | 0.0219 | 10867 | 0.6599 | 0.4312 |
Using CoS without CoT | ||||||
Finetune-ChatGlm3 6b | CoS w/o CoT | 2/10 | 0.0528 | 30356 | 0.6547 | 0.1714 |
Finetune-Qwen 1.8b | CoS w/o CoT | 6/10 | 0.0384 | 12826 | 0.7506 | 0.2095 |
Finetune-Qwen 7b | CoS w/o CoT | 6/12 | 0.0421 | 12276 | 0.7234 | 0.3214 |
Finetune-Llama2 7b | CoS w/o CoT | 0/12 | 0.0469 | 12295 | 0.5752 | 0.0853 |
Prompt | LV1 | LV2 | LV3 | LV4 | LV5 | LV6 |
---|---|---|---|---|---|---|
Prompt1 | 7/8 | 6/9 | 2/8 | 1/8 | 0/8 | 0/8 |
Prompt2 | 8/8 | 9/9 | 8/8 | 21/25 | 7/14 | 0/12 |
StatCraft II is a classic game developed by BLZ, and has some professional leagues such as IEM, WTL....You can download Battle.net from:https://us.shop.battle.net/en-us, or here:https://www.blizzard.com/zh-tw/
If you are Chinese, due to the Bobby Kotick, CN play cant own their sever again. So we must download StarCraft II by this video :video or you can search in the internet.
First , we should use StarCraft II Editor.exe to download the newest ladder map
when we open this, please log in your blz account and search the map which you want.
Then you should put maps to your StarCrafrt2 file in StarCraft II\Maps(If the 'Maps' file dont exist, please create it).
Or you can download maps in here:
- OS, We used Windows 11 to develop this demo, because BLZ didnt release the latest sc2 on liunx, so please run our repo on Windows OS!
-
python
: python 3.10. -
cuda
: cuda 12.1. -
torch
: 2.1.0 -
openai
: 0.27.9, very important. This is crucial as versions above 0.28 have altered API functionalities. Install all necessary packages withpip install -r requirements.txt
.
-
burnysc2
: This is our core package, offering an easy-to-use API for project development. Find more information here:Python-sc2 -
chromadb
: We utilize the Chroma vector database. Due to package conflicts, install Chromadb first, followed by burnysc2. -
Huggingface
andsentence-transformers
: we used the embedding modelsentence-transformers/all-mpnet-base-v2
, in our github version, it will automatically download. We also provide therelease
zip, you can just download and unzip that(with embedding model).
-
Agent vs Botai
: You can test intest_the_env.py
&multiprocess_test.py
-
Human vs Agent
: You can try in ourHuman_LLM_agent_test.py
-
Agent vs Agent
: You can try in our2agent_test.py
You can run test_the_env.py
to try our agent. Here is some parameters you need to set.
-
player_race
: Currently, onlyProtoss
is supported.Zerg
andTerran
are under development. -
opposite_race
: Typically set toZerg
, butTerran
andProtoss
are also compatible. -
difficulty
: We offer 10 difficulty levels, ranging from Level 1 (VeryEasy
) to Level 10 (CheatInsane
). Note that these names differ from those in the StarCraft2 client, but the AI difficulty remains unchanged.
Level | 1 | 2 | 3 | 4 | 5 | 6 | 7 | 8 | 9 | 10 |
---|---|---|---|---|---|---|---|---|---|---|
BLZ difficulty | VeryEasy | Easy | Medium | Hard | Harder | Very Hard | Elite | CheatVision | CheatMoney | CheatInsane |
python-sc2 difficulty | VeryEasy | Easy | Medium | MediumHard | Hard | Harder | VeryHard | CheatVision | CheatMoney | CheatInsane |
-
replay_folder
: Specify the folder for saving demo replays. -
LLM_model_name
: We usedgpt-3.5-turbo-16k
in our experiments. -
LLM_temperature
: Set between 0 and 1 as per your preference. -
LLM_api_key
: Your API key. -
LLM_api_base
: Your API base URL.
Note: Using LLM to play StarCraft2 can take approximately 7 hours for a single game.
To save time, you can run multiple demos simultaneously using multiprocess_test.py
. Configure the following parameter:
-
num_processes
: The number of processes to spawn.
Other parameters are the same as in the Single Process setup.
In our experiments, we have added some more settings, but due to several reasons these settings will coming soon.
-
num_agents
: This environment will supportLLM agent
vsLLM agent
orRL agent
. -
env_type
: This environment will support Text or MultiModal - 'player_race': This environment will support Zerg and Terran
-
opposite_type
: This env will support some human designed botai.
If you want to use other llm to create your own llm agent, the following things you should to know.
-
LLM
: In our repo, you should request llm fromChatBot_SingleTurn
function inTextStarCraft2_2/LLM/gpt_test
- 'L1_summarize': Our level-1 summarization method is here:
generate_summarize_L1
inTextStarCraft2_2/summarize/L1_summarize.py
-
L2_summarize
: Our level-2 summarization method is here :L2_summary
inTextStarCraft2_2/summarize/gpt_test/L2_summarize.py
-
action dict
: The actions that llm agent can use. Here we can setTextStarCraft2_2/utils/action_info.py
. You can add more actions for llm agent. -
action extractor
: We can extract decisions byTextStarCraft2_2/utils/action_extractor.py
The core of our TextStarCraft II env is TextStarCraft2_2/env/bot
. Here you can add more settings for environment. So if you want to realise Terran and Zerg bot, you can modify our code about this dictionary.
-
State
: InProtoss_bot.py
, the State of Env is generate fromget_information
function. This is what we saidObs to Text adaptor
-
Action
: InProtoss_bot.py
, the Action space of Agent is designed by thesehandle_action
function. This is what we saidText to Action adaptor
.
We have tested several LLMs in our experiments. The usage is in sc2_rl_agent/starcraftenv_test/LLM file
-
Online LLM
:GPT3.5-tubor
,GLM4
,Gemini-pro
,Claude2
. -
Local LLM
:GLM3
,QWEN
,QWEN1.5
.
Our framework in TextStarCraft II extends traditional StarCraft II analytics to evaluate LLM agents’ strategies with metrics tailored for AI gameplay performance:
-
Win Rate: Reflects the agent's performance, calculated as the percentage of games won out of total games played.
-
Population Block Ratio (PBR): Indicates macro-management effectiveness, focusing on resource allocation and population growth. A higher PBR suggests less effective macro-strategy due to more time spent at population cap.
-
Resource Utilization Ratio (RUR): Measures how efficiently the agent manages resources throughout the game. Higher RUR indicates underutilization of resources.
-
Average Population Utilization (APU): Assesses efficiency in utilizing population capacity. Higher APU indicates better macro-management.
-
Technology Rate (TR): Evaluates the agent's use of the technology tree, showing the proportion of technologies and buildings completed. It reflects the agent’s technological advancement.
For Tasks:
Click tags to check more tools for each tasksFor Jobs:
Alternative AI tools for Large-Language-Models-play-StarCraftII
Similar Open Source Tools
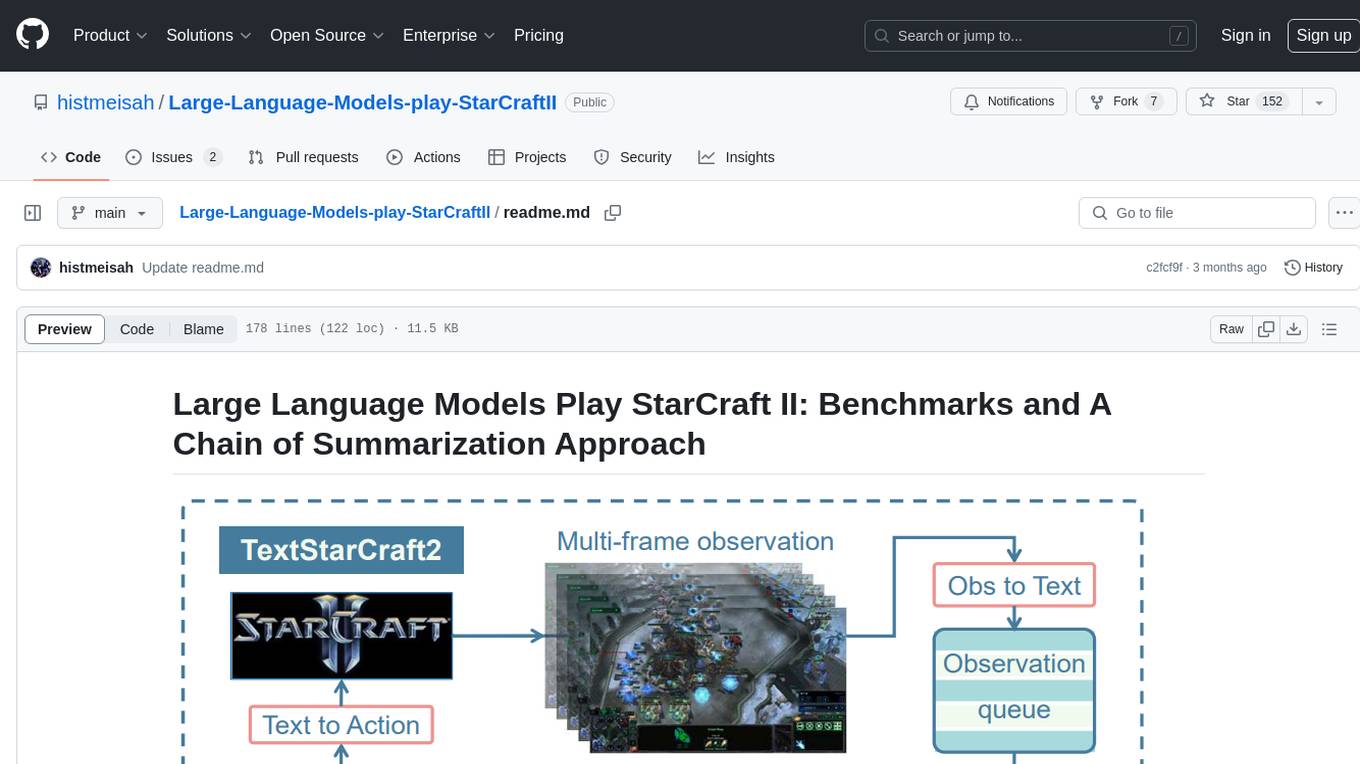
Large-Language-Models-play-StarCraftII
Large Language Models Play StarCraft II is a project that explores the capabilities of large language models (LLMs) in playing the game StarCraft II. The project introduces TextStarCraft II, a textual environment for the game, and a Chain of Summarization method for analyzing game information and making strategic decisions. Through experiments, the project demonstrates that LLM agents can defeat the built-in AI at a challenging difficulty level. The project provides benchmarks and a summarization approach to enhance strategic planning and interpretability in StarCraft II gameplay.
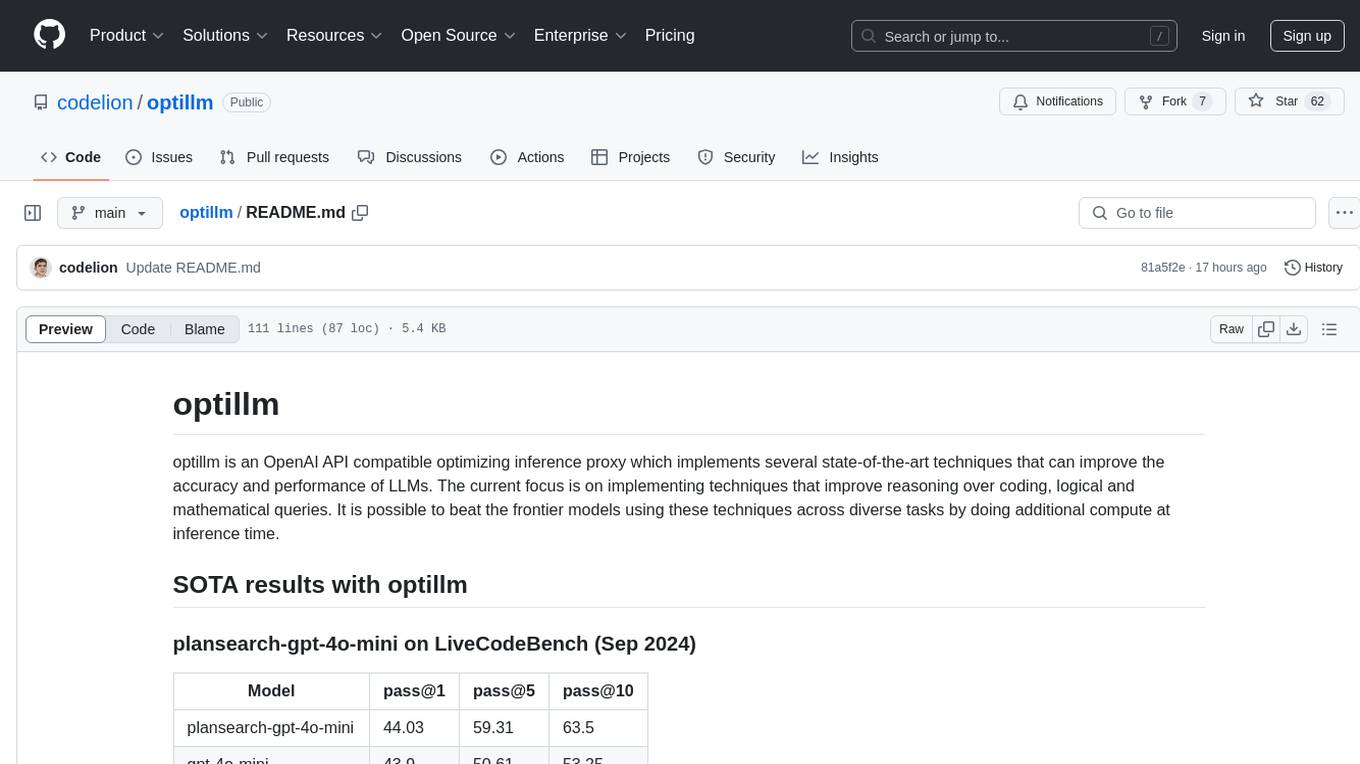
optillm
optillm is an OpenAI API compatible optimizing inference proxy implementing state-of-the-art techniques to enhance accuracy and performance of LLMs, focusing on reasoning over coding, logical, and mathematical queries. By leveraging additional compute at inference time, it surpasses frontier models across diverse tasks.
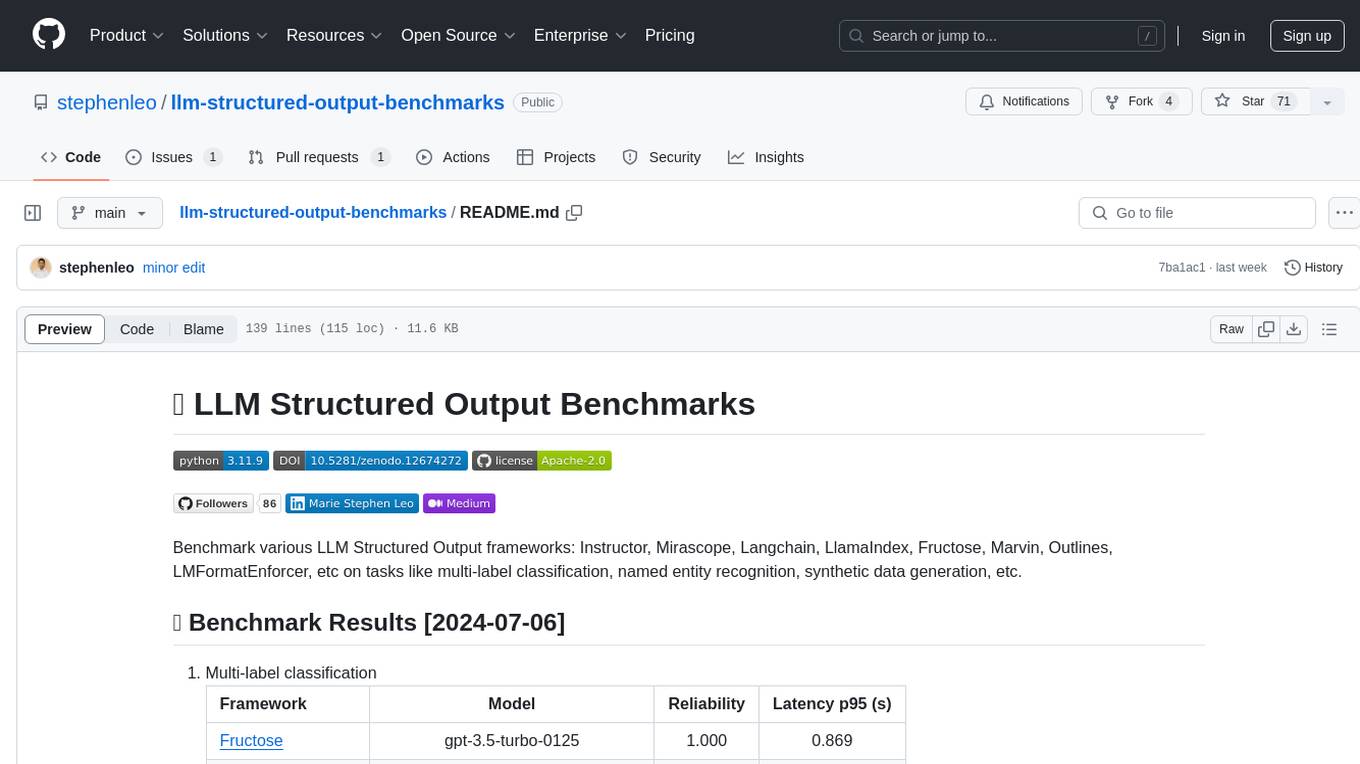
llm-structured-output-benchmarks
Benchmark various LLM Structured Output frameworks like Instructor, Mirascope, Langchain, LlamaIndex, Fructose, Marvin, Outlines, LMFormatEnforcer, etc on tasks like multi-label classification, named entity recognition, synthetic data generation. The tool provides benchmark results, methodology, instructions to run the benchmark, add new data, and add a new framework. It also includes a roadmap for framework-related tasks, contribution guidelines, citation information, and feedback request.
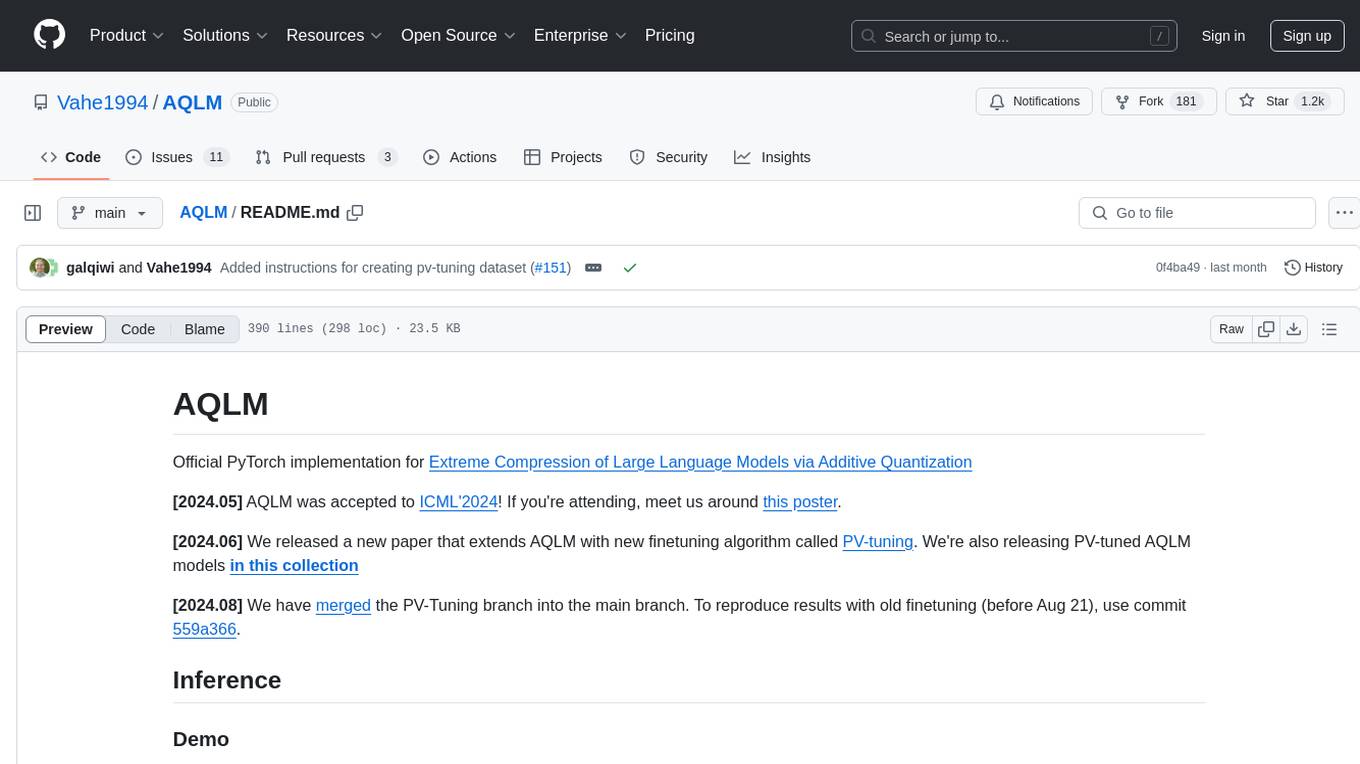
AQLM
AQLM is the official PyTorch implementation for Extreme Compression of Large Language Models via Additive Quantization. It includes prequantized AQLM models without PV-Tuning and PV-Tuned models for LLaMA, Mistral, and Mixtral families. The repository provides inference examples, model details, and quantization setups. Users can run prequantized models using Google Colab examples, work with different model families, and install the necessary inference library. The repository also offers detailed instructions for quantization, fine-tuning, and model evaluation. AQLM quantization involves calibrating models for compression, and users can improve model accuracy through finetuning. Additionally, the repository includes information on preparing models for inference and contributing guidelines.
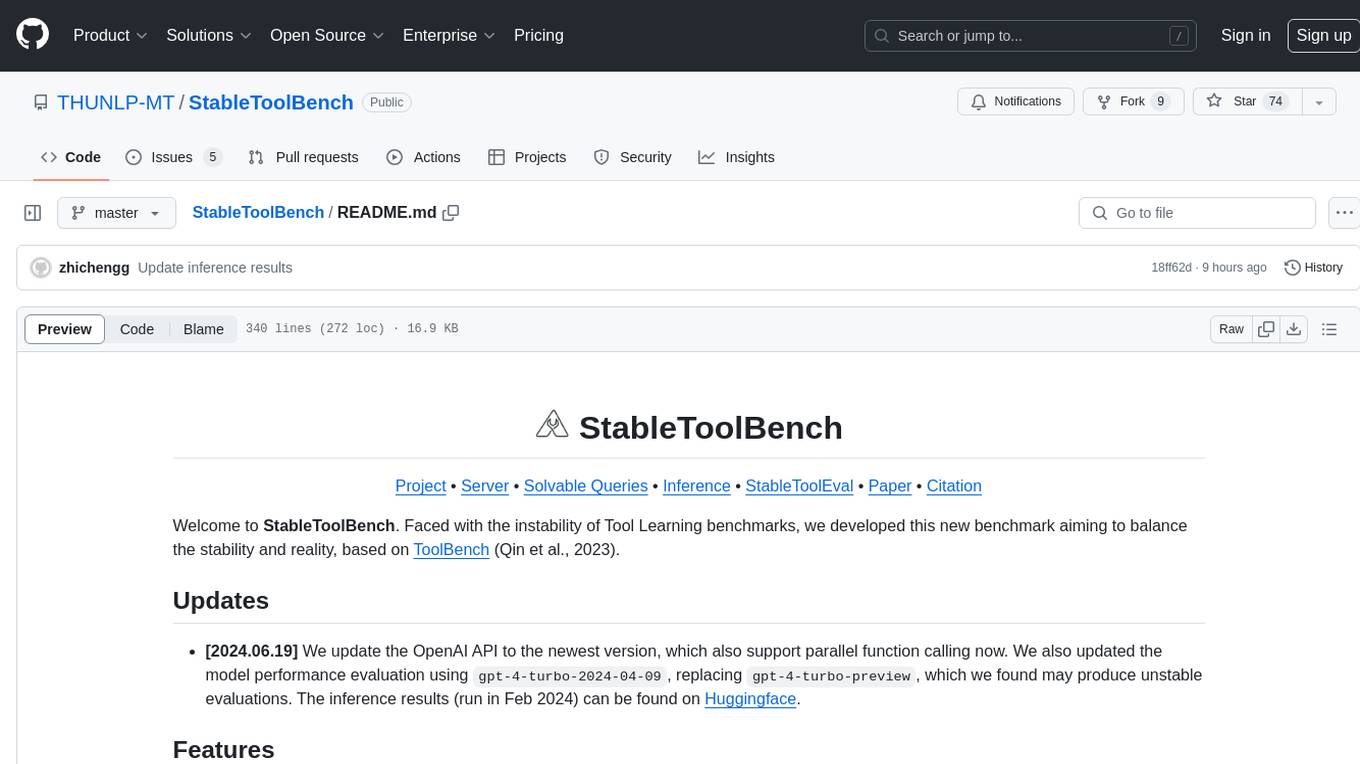
StableToolBench
StableToolBench is a new benchmark developed to address the instability of Tool Learning benchmarks. It aims to balance stability and reality by introducing features like Virtual API System, Solvable Queries, and Stable Evaluation System. The benchmark ensures consistency through a caching system and API simulators, filters queries based on solvability using LLMs, and evaluates model performance using GPT-4 with metrics like Solvable Pass Rate and Solvable Win Rate.
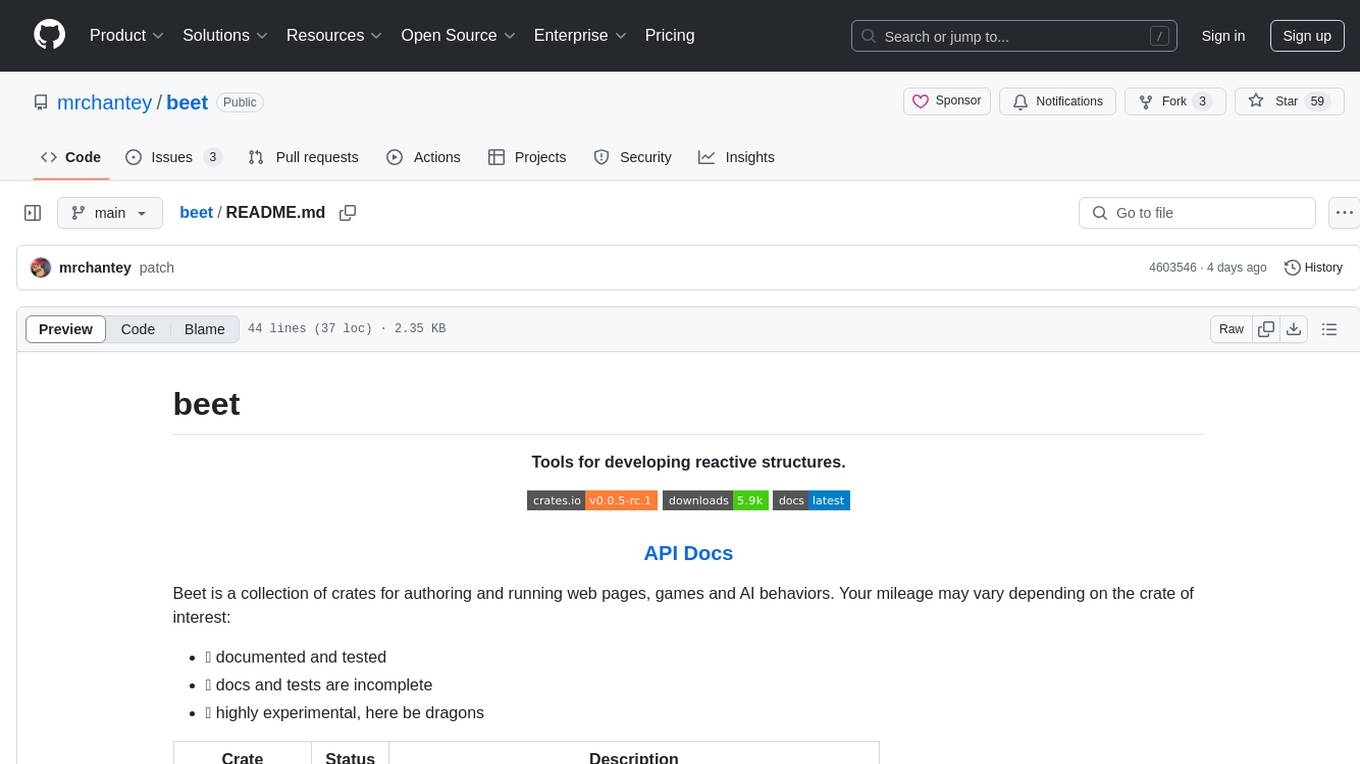
beet
Beet is a collection of crates for authoring and running web pages, games and AI behaviors. It includes crates like `beet_flow` for scenes-as-control-flow bevy library, `beet_spatial` for spatial behaviors, `beet_ml` for machine learning, `beet_sim` for simulation tooling, `beet_rsx` for authoring tools for html and bevy, and `beet_router` for file-based router for web docs. The `beet` crate acts as a base crate that re-exports sub-crates based on feature flags, similar to the `bevy` crate structure.
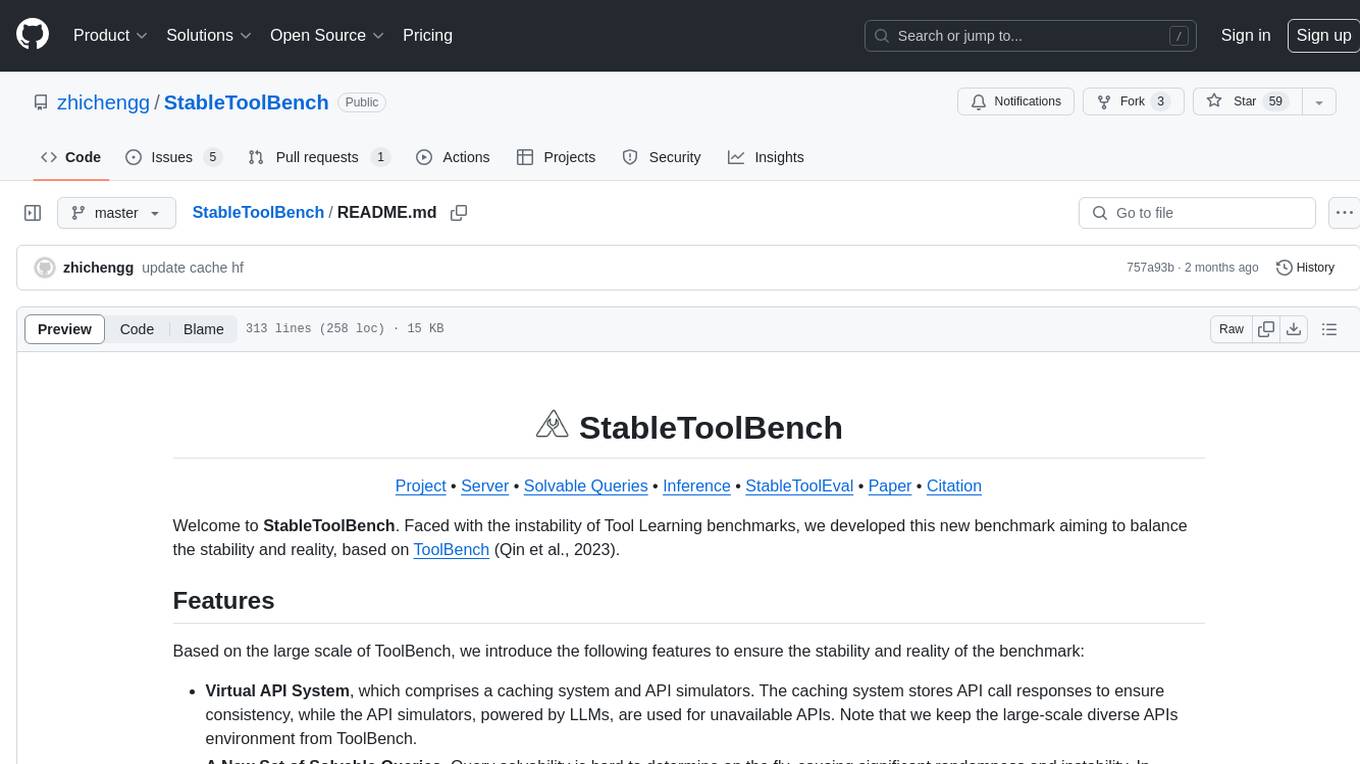
StableToolBench
StableToolBench is a new benchmark developed to address the instability of Tool Learning benchmarks. It aims to balance stability and reality by introducing features such as a Virtual API System with caching and API simulators, a new set of solvable queries determined by LLMs, and a Stable Evaluation System using GPT-4. The Virtual API Server can be set up either by building from source or using a prebuilt Docker image. Users can test the server using provided scripts and evaluate models with Solvable Pass Rate and Solvable Win Rate metrics. The tool also includes model experiments results comparing different models' performance.
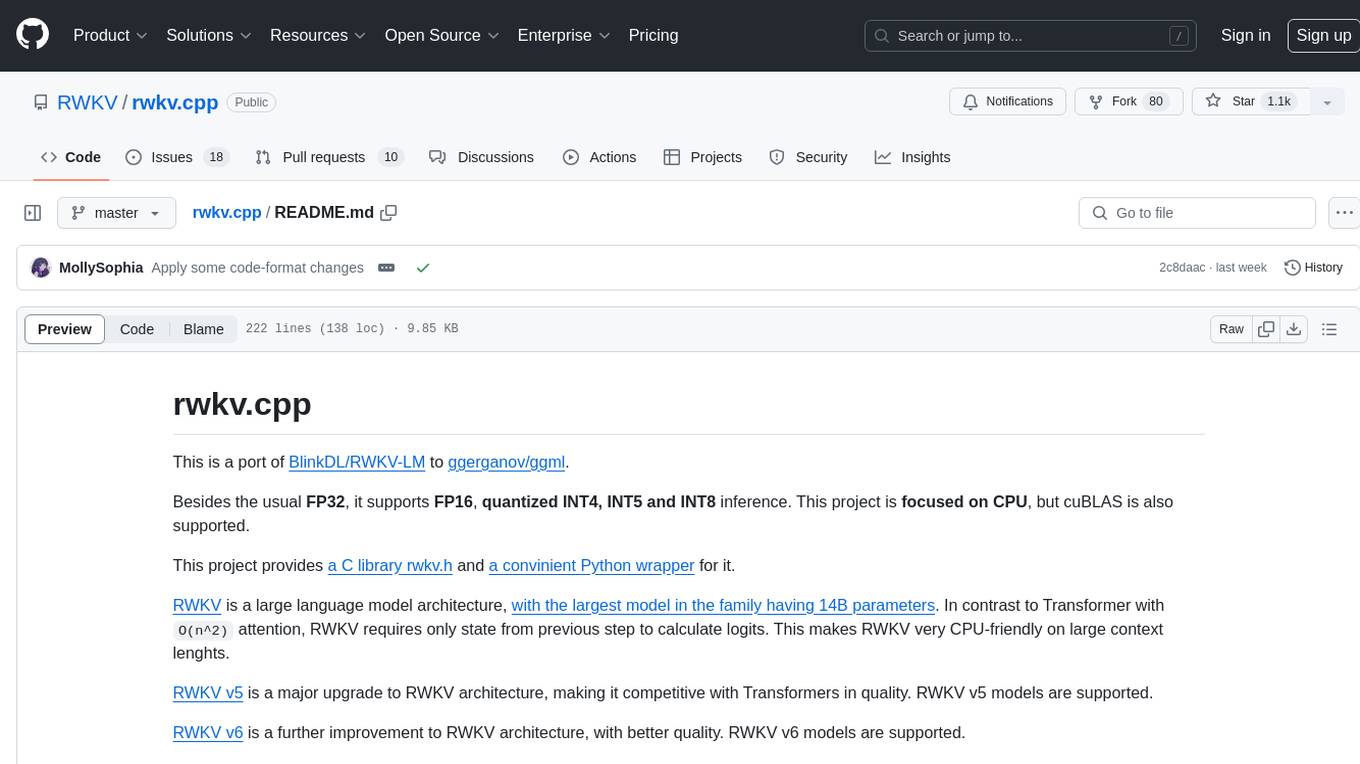
rwkv.cpp
rwkv.cpp is a port of BlinkDL/RWKV-LM to ggerganov/ggml, supporting FP32, FP16, and quantized INT4, INT5, and INT8 inference. It focuses on CPU but also supports cuBLAS. The project provides a C library rwkv.h and a Python wrapper. RWKV is a large language model architecture with models like RWKV v5 and v6. It requires only state from the previous step for calculations, making it CPU-friendly on large context lengths. Users are advised to test all available formats for perplexity and latency on a representative dataset before serious use.
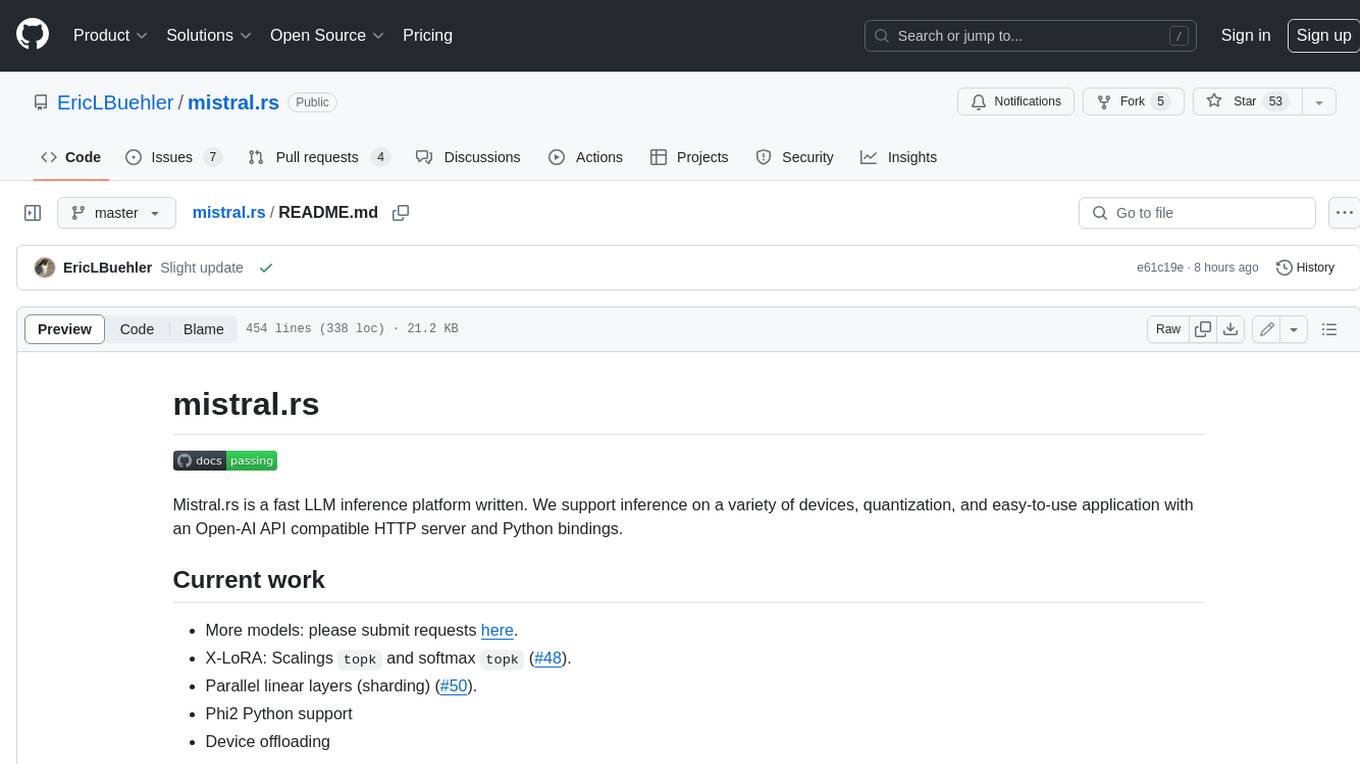
mistral.rs
Mistral.rs is a fast LLM inference platform written in Rust. We support inference on a variety of devices, quantization, and easy-to-use application with an Open-AI API compatible HTTP server and Python bindings.
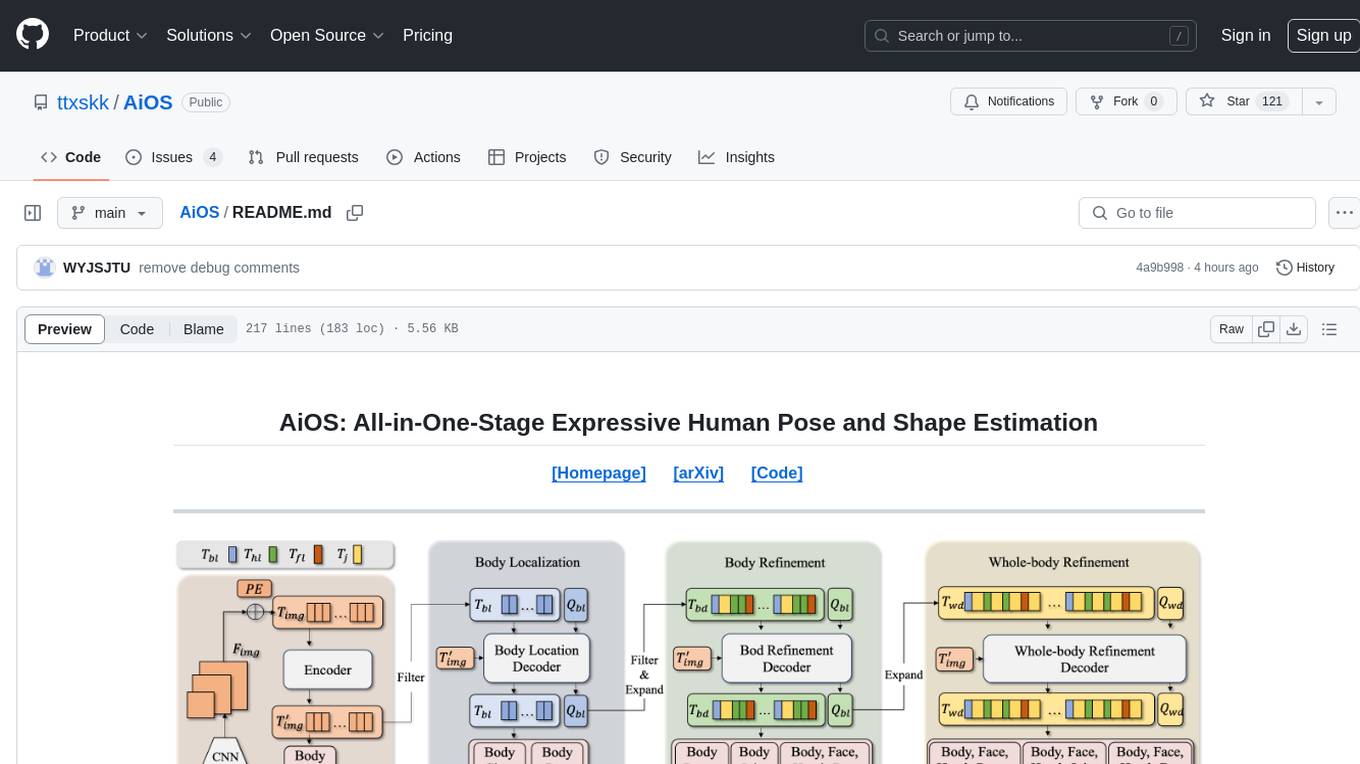
AiOS
AiOS is a tool for human pose and shape estimation, performing human localization and SMPL-X estimation in a progressive manner. It consists of body localization, body refinement, and whole-body refinement stages. Users can download datasets for evaluation, SMPL-X body models, and AiOS checkpoint. Installation involves creating a conda virtual environment, installing PyTorch, torchvision, Pytorch3D, MMCV, and other dependencies. Inference requires placing the video for inference and pretrained models in specific directories. Test results are provided for NMVE, NMJE, MVE, and MPJPE on datasets like BEDLAM and AGORA. Users can run scripts for AGORA validation, AGORA test leaderboard, and BEDLAM leaderboard. The tool acknowledges codes from MMHuman3D, ED-Pose, and SMPLer-X.
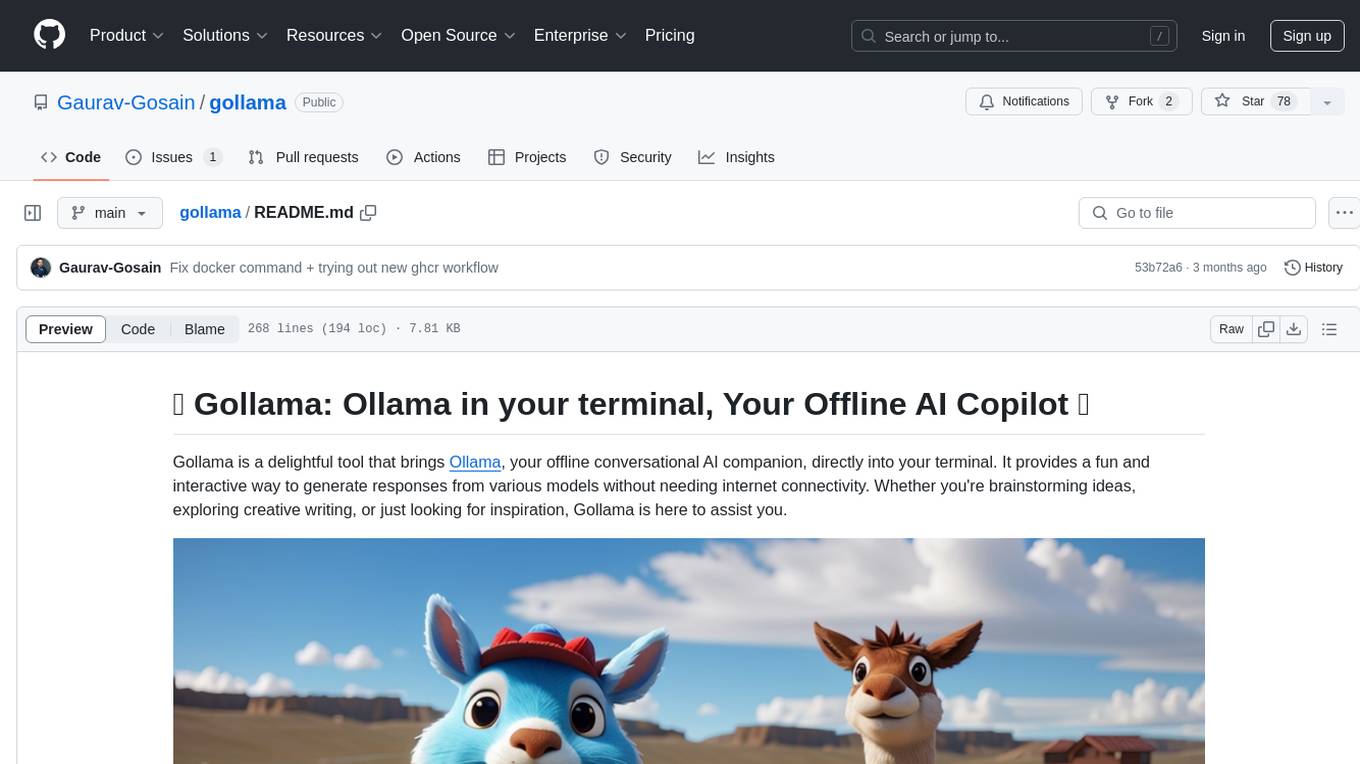
gollama
Gollama is a delightful tool that brings Ollama, your offline conversational AI companion, directly into your terminal. It provides a fun and interactive way to generate responses from various models without needing internet connectivity. Whether you're brainstorming ideas, exploring creative writing, or just looking for inspiration, Gollama is here to assist you. The tool offers an interactive interface, customizable prompts, multiple models selection, and visual feedback to enhance user experience. It can be installed via different methods like downloading the latest release, using Go, running with Docker, or building from source. Users can interact with Gollama through various options like specifying a custom base URL, prompt, model, and enabling raw output mode. The tool supports different modes like interactive, piped, CLI with image, and TUI with image. Gollama relies on third-party packages like bubbletea, glamour, huh, and lipgloss. The roadmap includes implementing piped mode, support for extracting codeblocks, copying responses/codeblocks to clipboard, GitHub Actions for automated releases, and downloading models directly from Ollama using the rest API. Contributions are welcome, and the project is licensed under the MIT License.
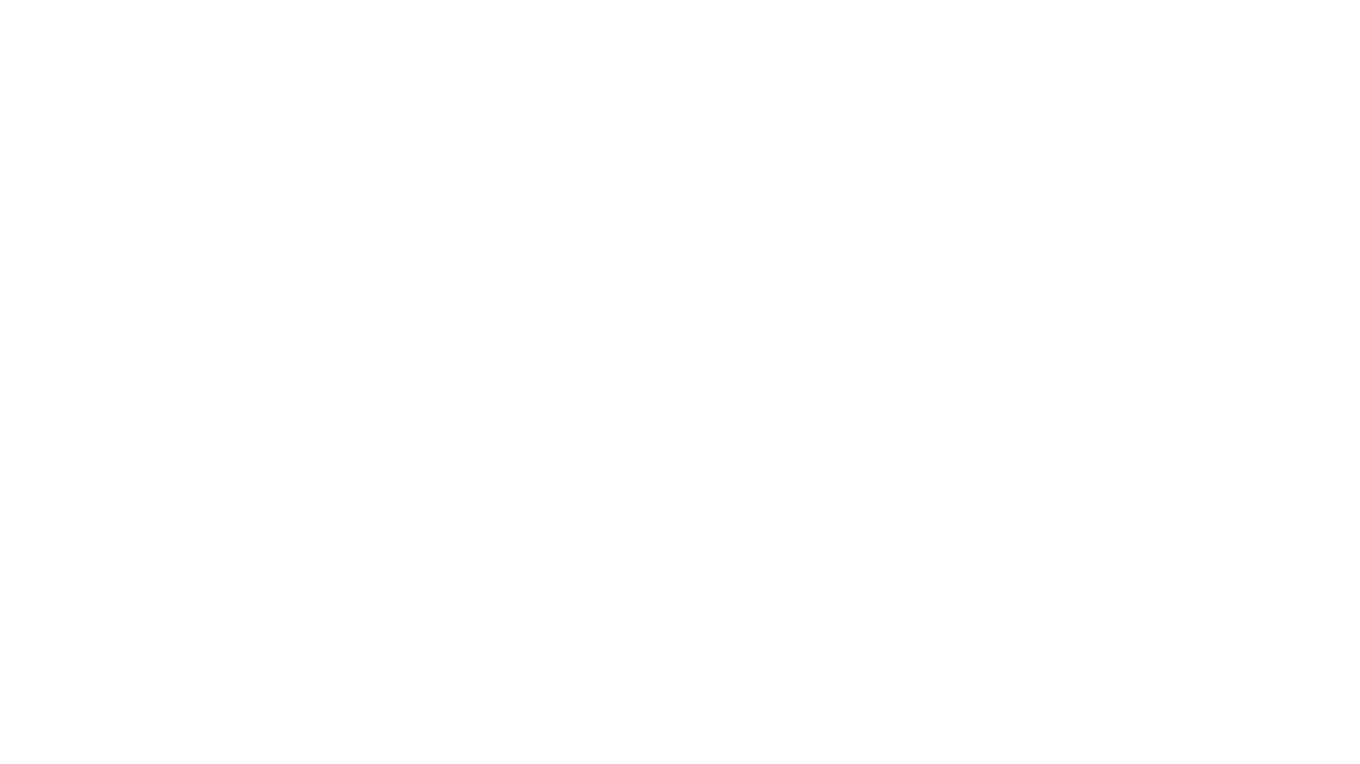
graphrag-visualizer
GraphRAG Visualizer is an application designed to visualize Microsoft GraphRAG artifacts by uploading parquet files generated from the GraphRAG indexing pipeline. Users can view and analyze data in 2D or 3D graphs, display data tables, search for specific nodes or relationships, and process artifacts locally for data security and privacy.
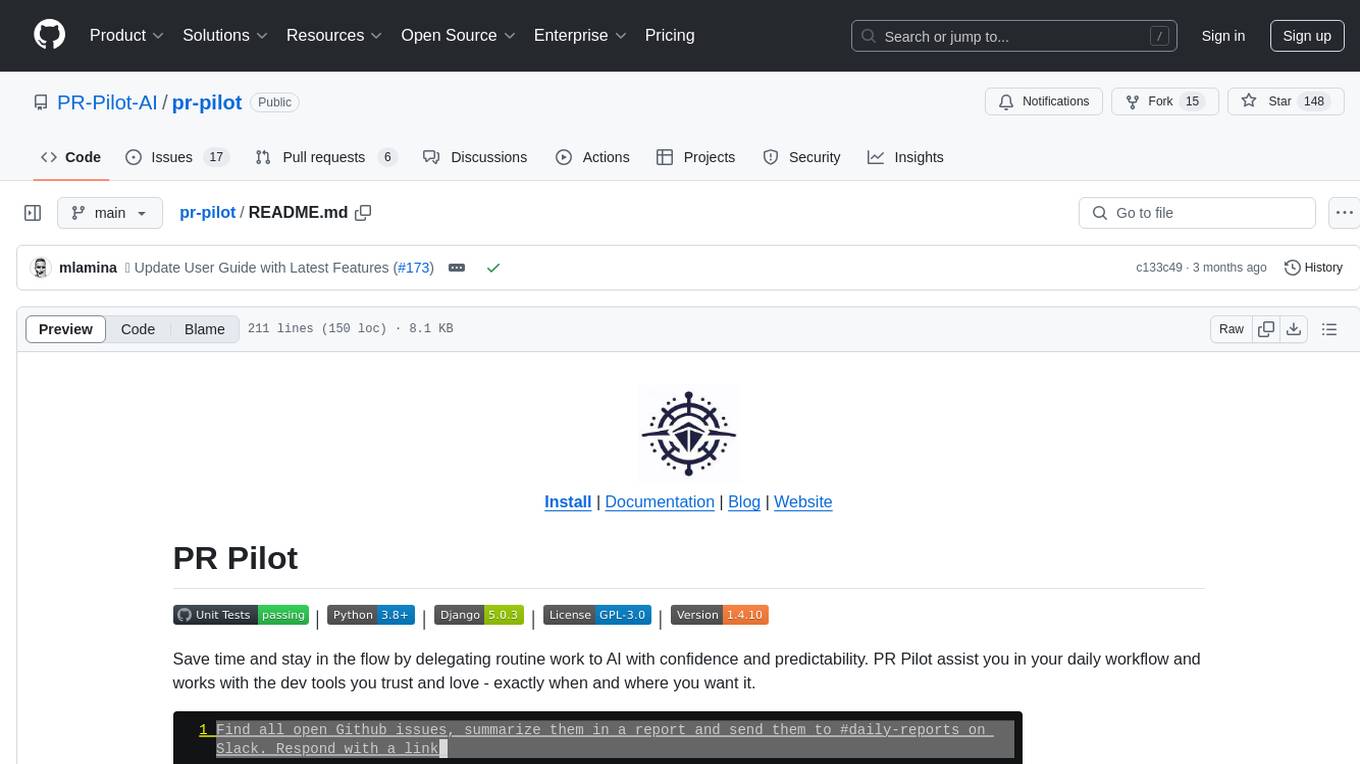
pr-pilot
PR Pilot is an AI-powered tool designed to assist users in their daily workflow by delegating routine work to AI with confidence and predictability. It integrates seamlessly with popular development tools and allows users to interact with it through a Command-Line Interface, Python SDK, REST API, and Smart Workflows. Users can automate tasks such as generating PR titles and descriptions, summarizing and posting issues, and formatting README files. The tool aims to save time and enhance productivity by providing AI-powered solutions for common development tasks.
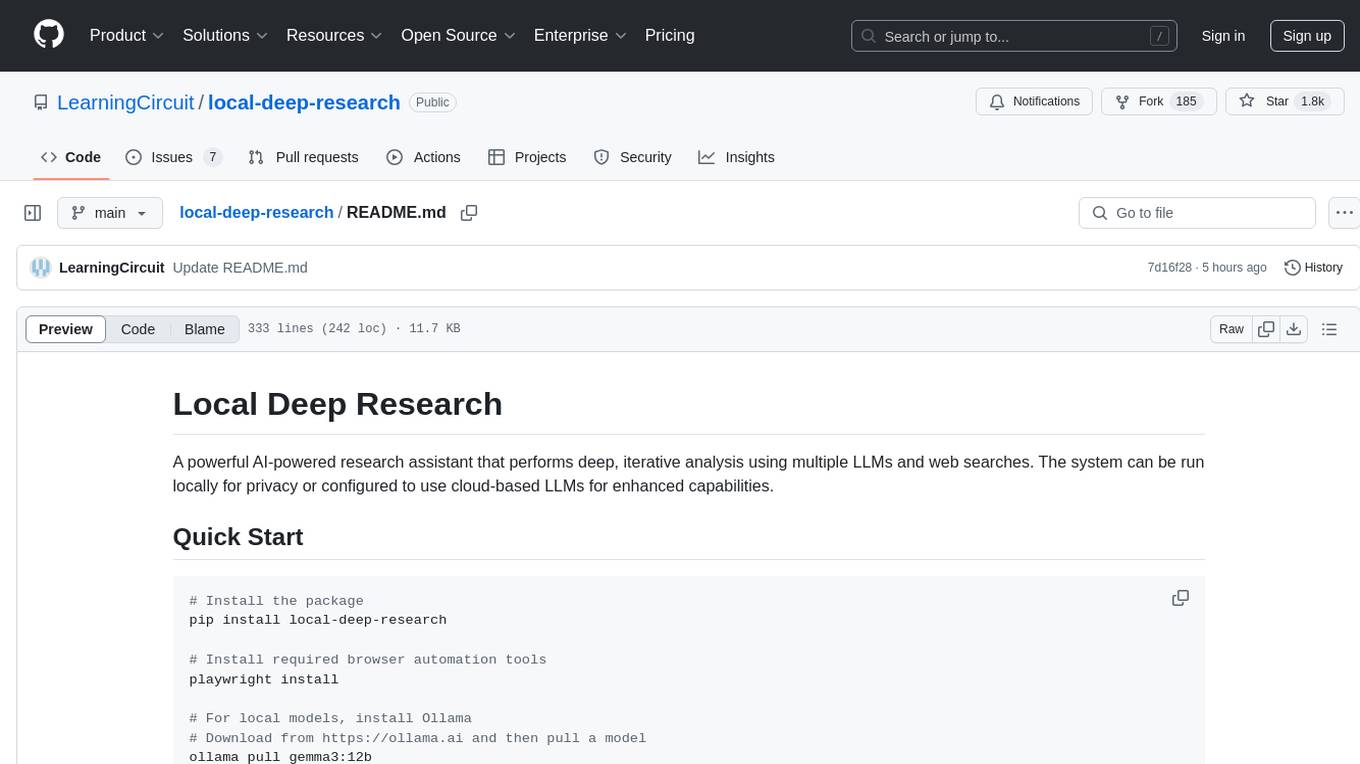
local-deep-research
Local Deep Research is a powerful AI-powered research assistant that performs deep, iterative analysis using multiple LLMs and web searches. It can be run locally for privacy or configured to use cloud-based LLMs for enhanced capabilities. The tool offers advanced research capabilities, flexible LLM support, rich output options, privacy-focused operation, enhanced search integration, and academic & scientific integration. It also provides a web interface, command line interface, and supports multiple LLM providers and search engines. Users can configure AI models, search engines, and research parameters for customized research experiences.
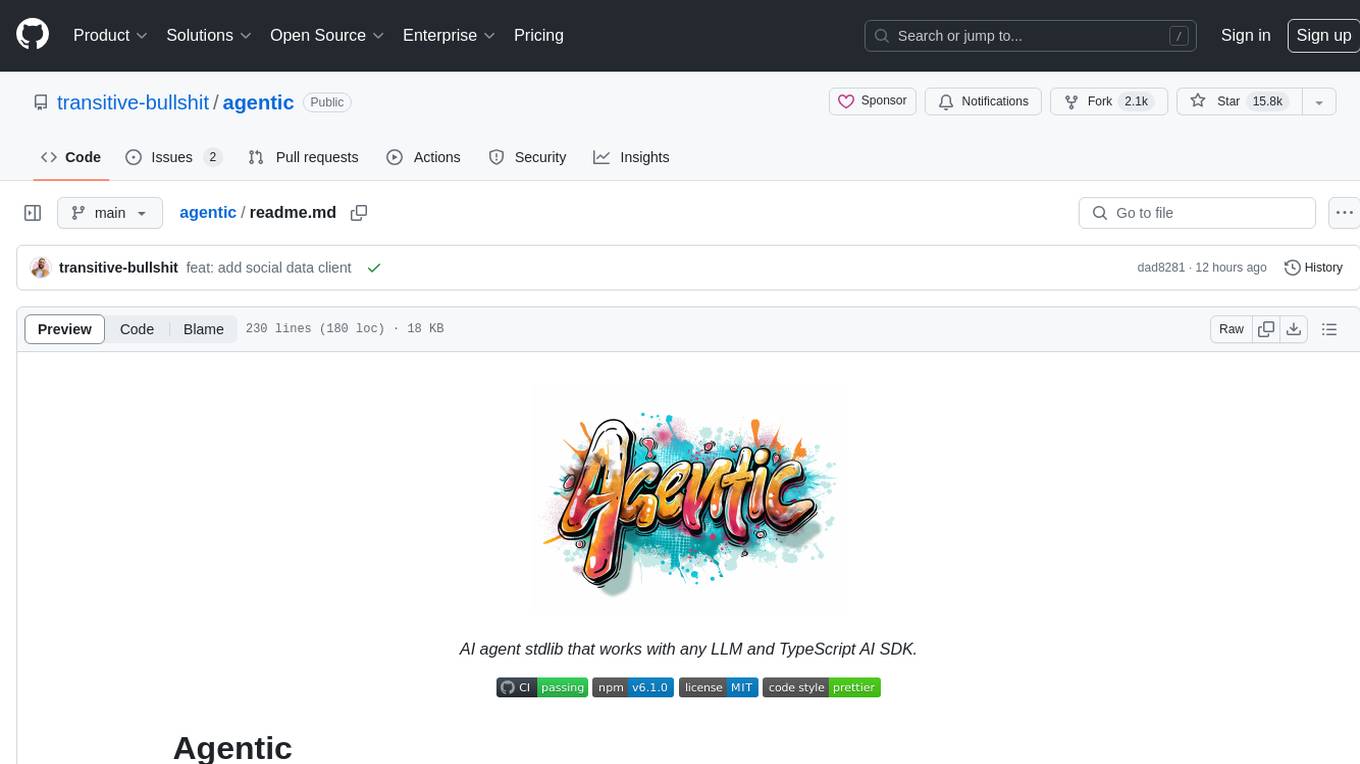
agentic
Agentic is a standard AI functions/tools library optimized for TypeScript and LLM-based apps, compatible with major AI SDKs. It offers a set of thoroughly tested AI functions that can be used with favorite AI SDKs without writing glue code. The library includes various clients for services like Bing web search, calculator, Clearbit data resolution, Dexa podcast questions, and more. It also provides compound tools like SearchAndCrawl and supports multiple AI SDKs such as OpenAI, Vercel AI SDK, LangChain, LlamaIndex, Firebase Genkit, and Dexa Dexter. The goal is to create minimal clients with strongly-typed TypeScript DX, composable AIFunctions via AIFunctionSet, and compatibility with major TS AI SDKs.
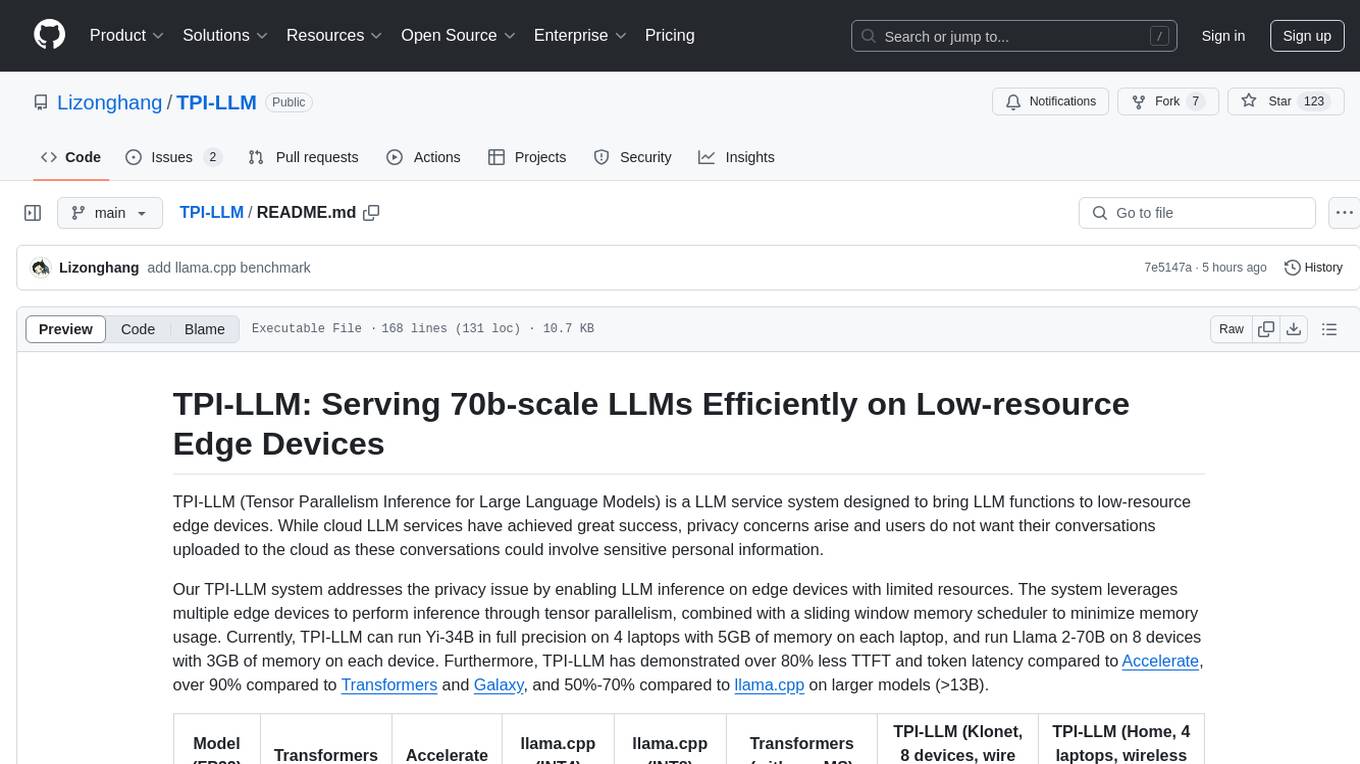
TPI-LLM
TPI-LLM (Tensor Parallelism Inference for Large Language Models) is a system designed to bring LLM functions to low-resource edge devices, addressing privacy concerns by enabling LLM inference on edge devices with limited resources. It leverages multiple edge devices for inference through tensor parallelism and a sliding window memory scheduler to minimize memory usage. TPI-LLM demonstrates significant improvements in TTFT and token latency compared to other models, and plans to support infinitely large models with low token latency in the future.
For similar tasks
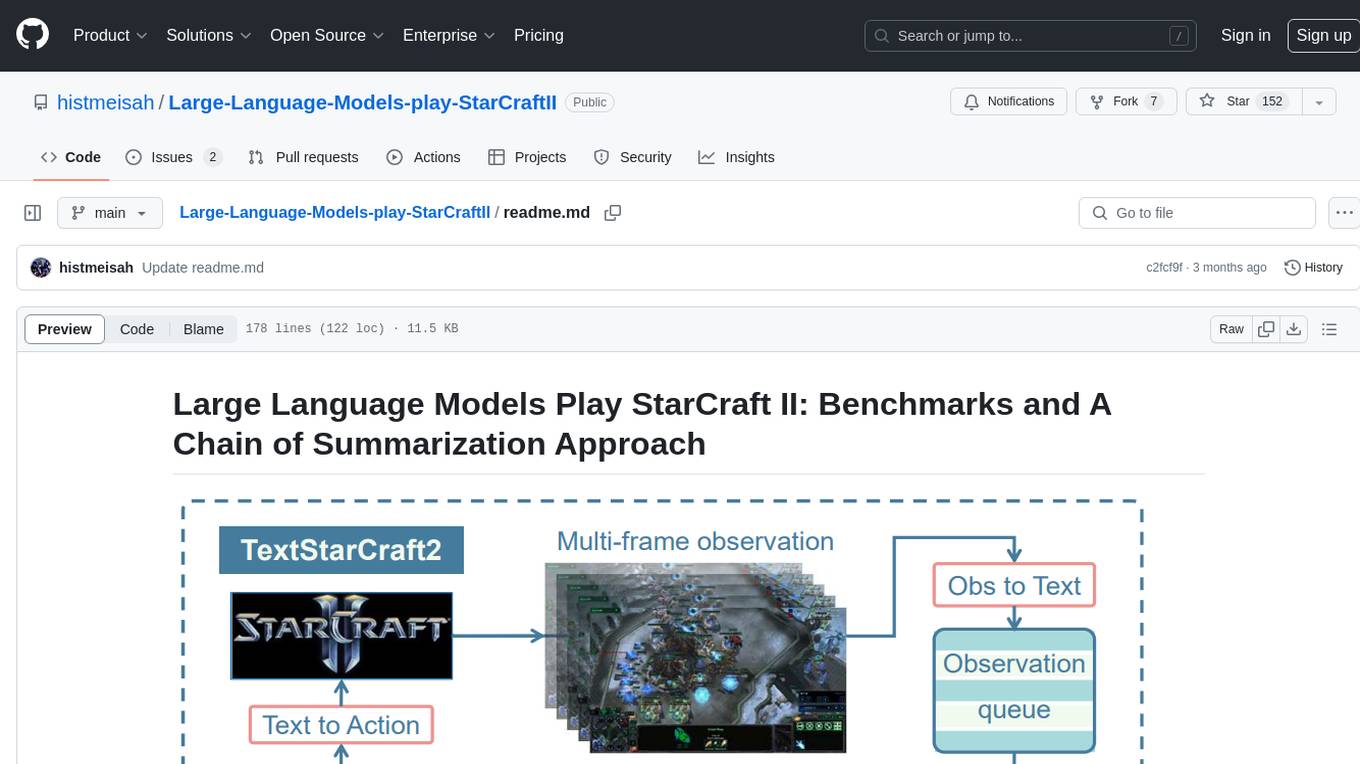
Large-Language-Models-play-StarCraftII
Large Language Models Play StarCraft II is a project that explores the capabilities of large language models (LLMs) in playing the game StarCraft II. The project introduces TextStarCraft II, a textual environment for the game, and a Chain of Summarization method for analyzing game information and making strategic decisions. Through experiments, the project demonstrates that LLM agents can defeat the built-in AI at a challenging difficulty level. The project provides benchmarks and a summarization approach to enhance strategic planning and interpretability in StarCraft II gameplay.
For similar jobs
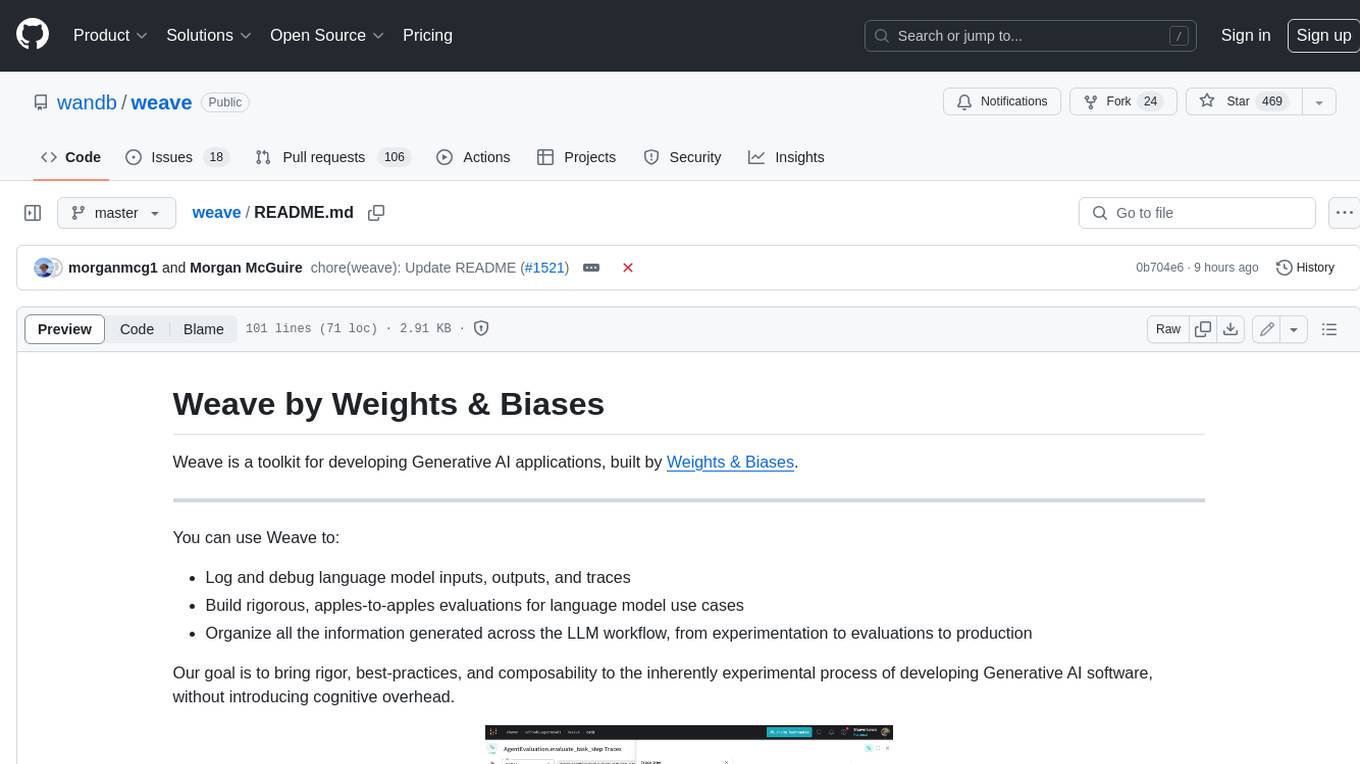
weave
Weave is a toolkit for developing Generative AI applications, built by Weights & Biases. With Weave, you can log and debug language model inputs, outputs, and traces; build rigorous, apples-to-apples evaluations for language model use cases; and organize all the information generated across the LLM workflow, from experimentation to evaluations to production. Weave aims to bring rigor, best-practices, and composability to the inherently experimental process of developing Generative AI software, without introducing cognitive overhead.
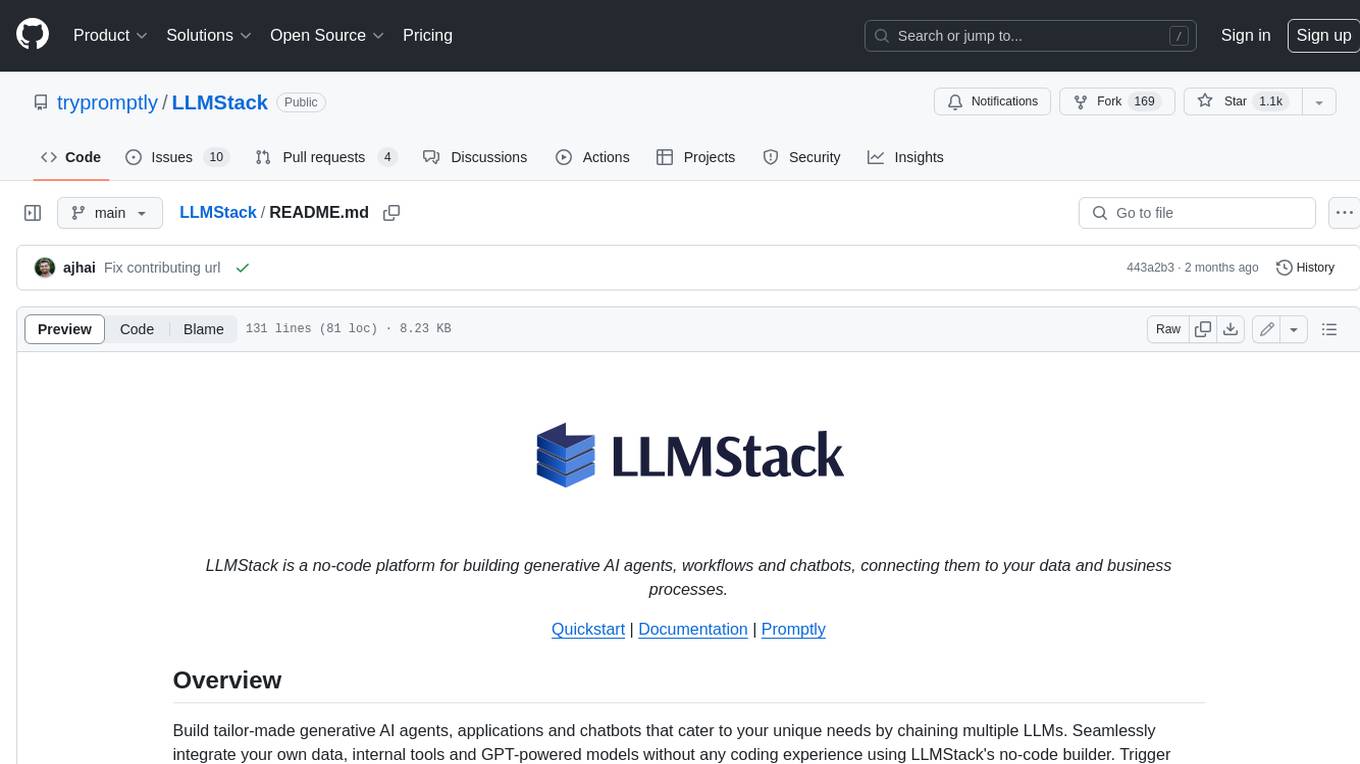
LLMStack
LLMStack is a no-code platform for building generative AI agents, workflows, and chatbots. It allows users to connect their own data, internal tools, and GPT-powered models without any coding experience. LLMStack can be deployed to the cloud or on-premise and can be accessed via HTTP API or triggered from Slack or Discord.
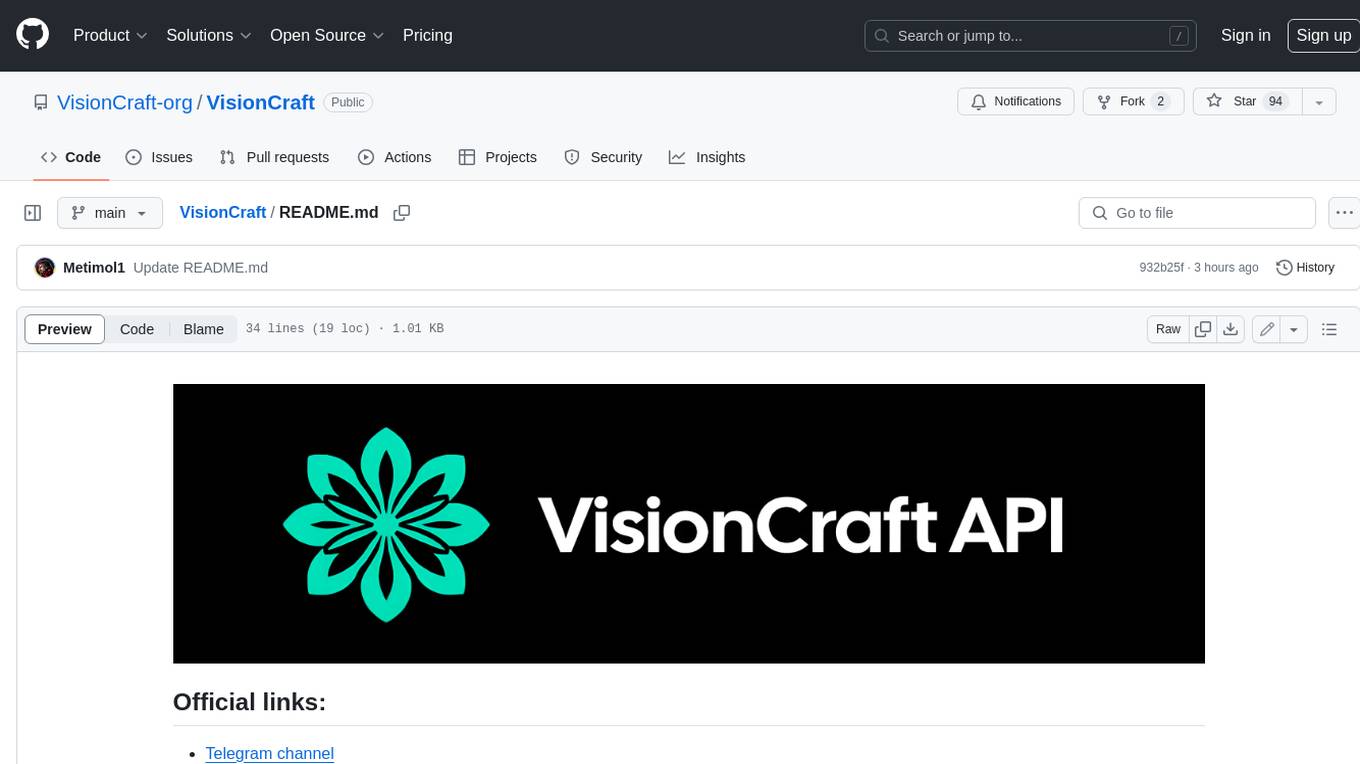
VisionCraft
The VisionCraft API is a free API for using over 100 different AI models. From images to sound.
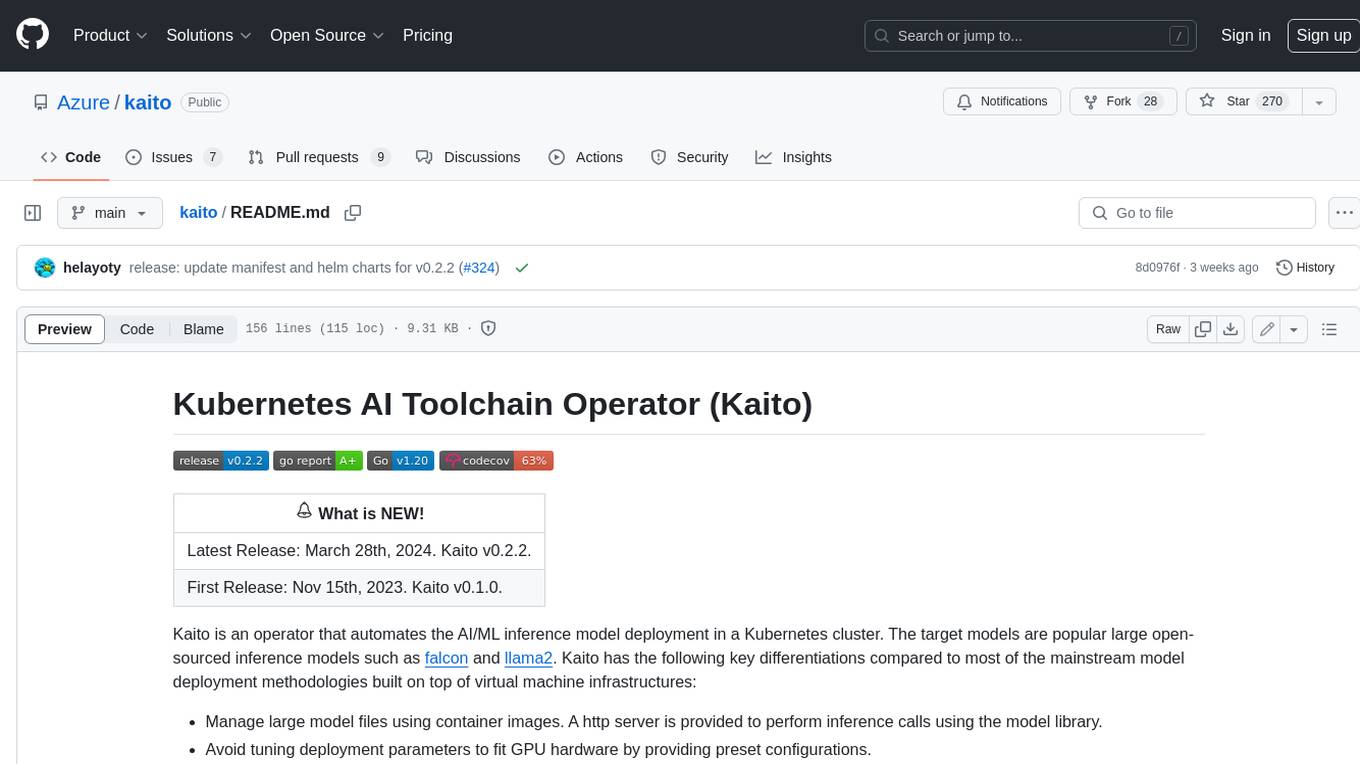
kaito
Kaito is an operator that automates the AI/ML inference model deployment in a Kubernetes cluster. It manages large model files using container images, avoids tuning deployment parameters to fit GPU hardware by providing preset configurations, auto-provisions GPU nodes based on model requirements, and hosts large model images in the public Microsoft Container Registry (MCR) if the license allows. Using Kaito, the workflow of onboarding large AI inference models in Kubernetes is largely simplified.
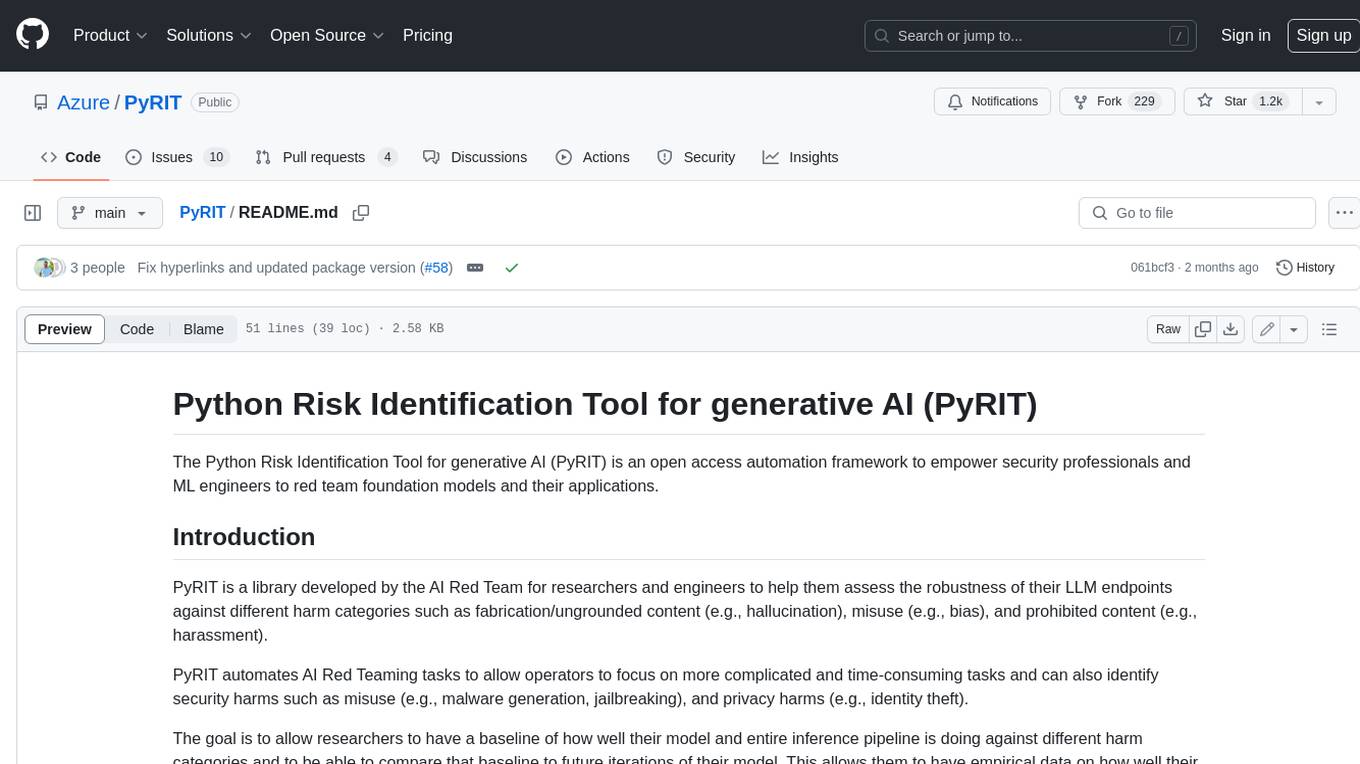
PyRIT
PyRIT is an open access automation framework designed to empower security professionals and ML engineers to red team foundation models and their applications. It automates AI Red Teaming tasks to allow operators to focus on more complicated and time-consuming tasks and can also identify security harms such as misuse (e.g., malware generation, jailbreaking), and privacy harms (e.g., identity theft). The goal is to allow researchers to have a baseline of how well their model and entire inference pipeline is doing against different harm categories and to be able to compare that baseline to future iterations of their model. This allows them to have empirical data on how well their model is doing today, and detect any degradation of performance based on future improvements.
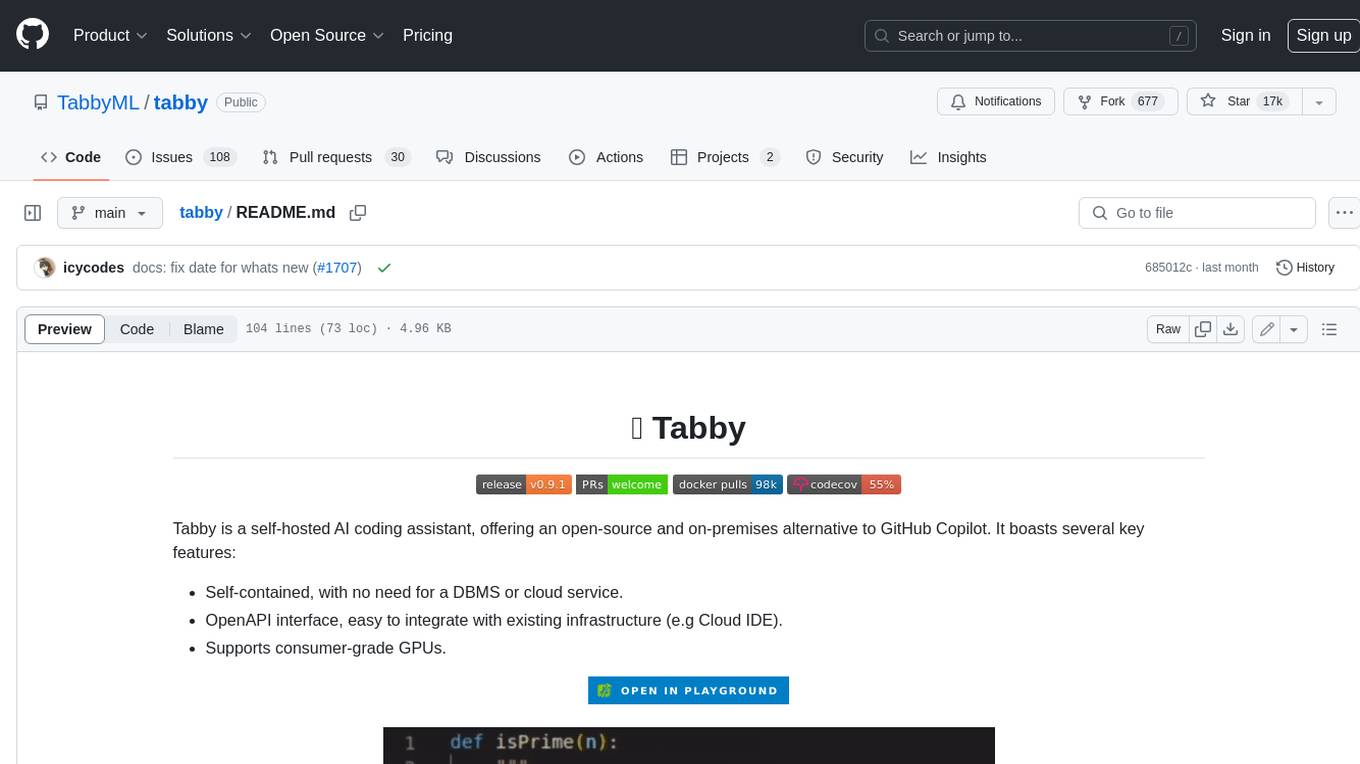
tabby
Tabby is a self-hosted AI coding assistant, offering an open-source and on-premises alternative to GitHub Copilot. It boasts several key features: * Self-contained, with no need for a DBMS or cloud service. * OpenAPI interface, easy to integrate with existing infrastructure (e.g Cloud IDE). * Supports consumer-grade GPUs.
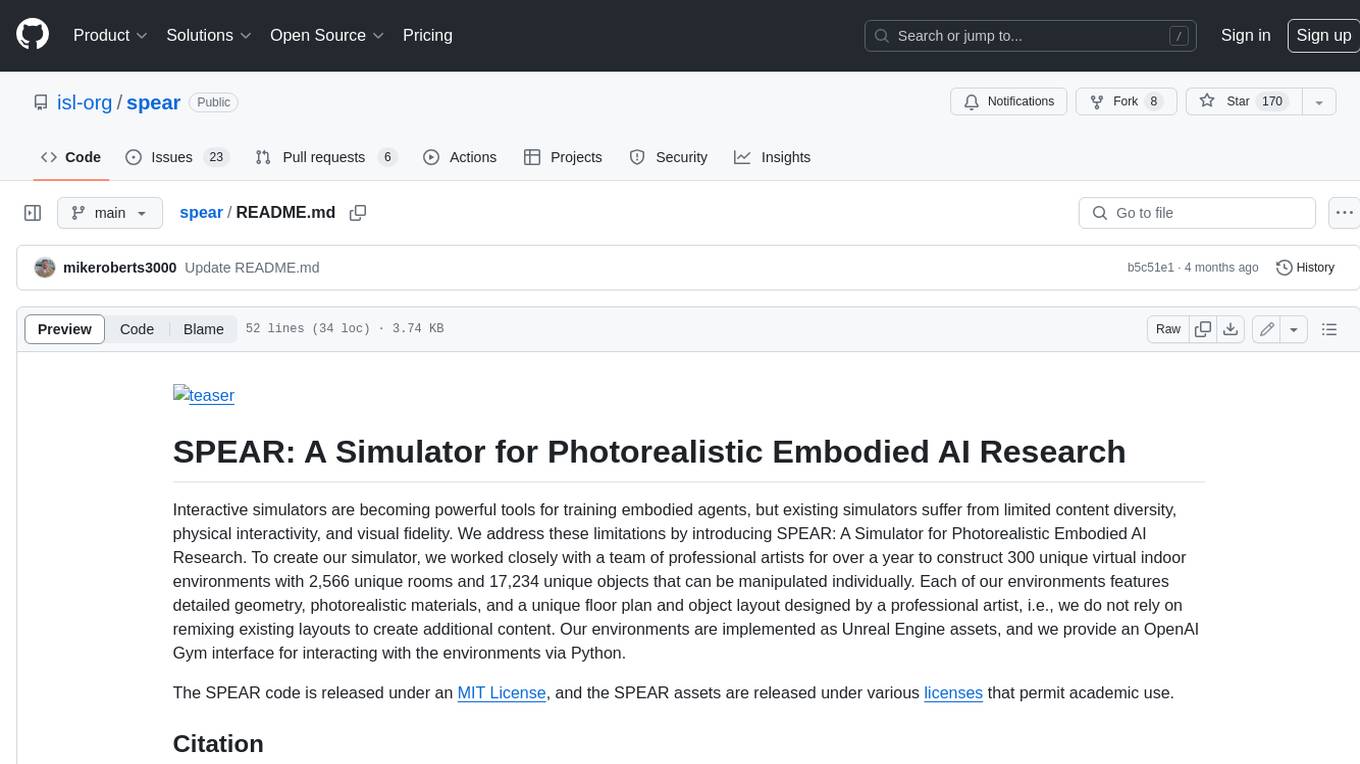
spear
SPEAR (Simulator for Photorealistic Embodied AI Research) is a powerful tool for training embodied agents. It features 300 unique virtual indoor environments with 2,566 unique rooms and 17,234 unique objects that can be manipulated individually. Each environment is designed by a professional artist and features detailed geometry, photorealistic materials, and a unique floor plan and object layout. SPEAR is implemented as Unreal Engine assets and provides an OpenAI Gym interface for interacting with the environments via Python.
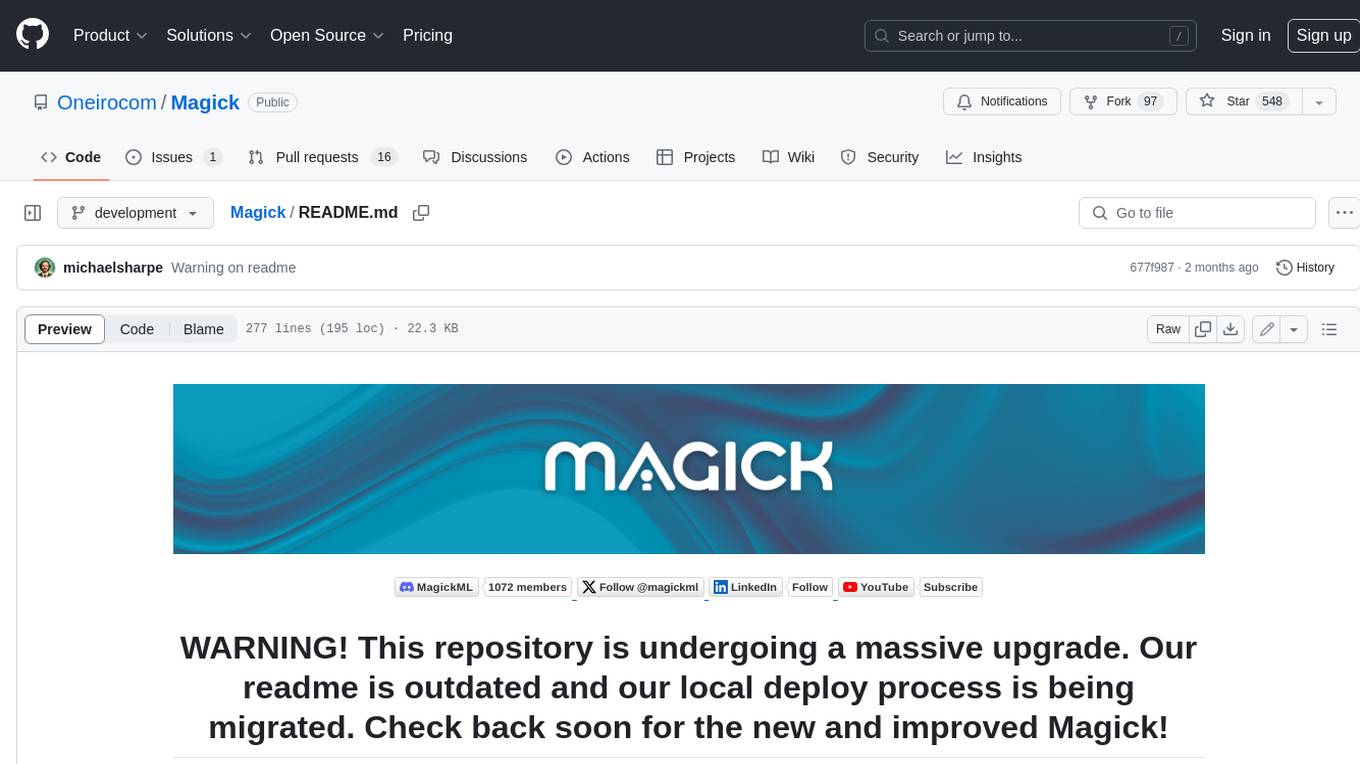
Magick
Magick is a groundbreaking visual AIDE (Artificial Intelligence Development Environment) for no-code data pipelines and multimodal agents. Magick can connect to other services and comes with nodes and templates well-suited for intelligent agents, chatbots, complex reasoning systems and realistic characters.