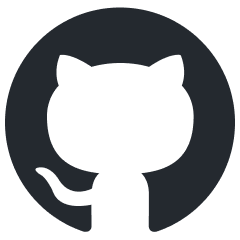
ax
The "official" unofficial DSPy framework. Build LLM powered agents and other workflows, based on the Stanford DSP paper.
Stars: 1357
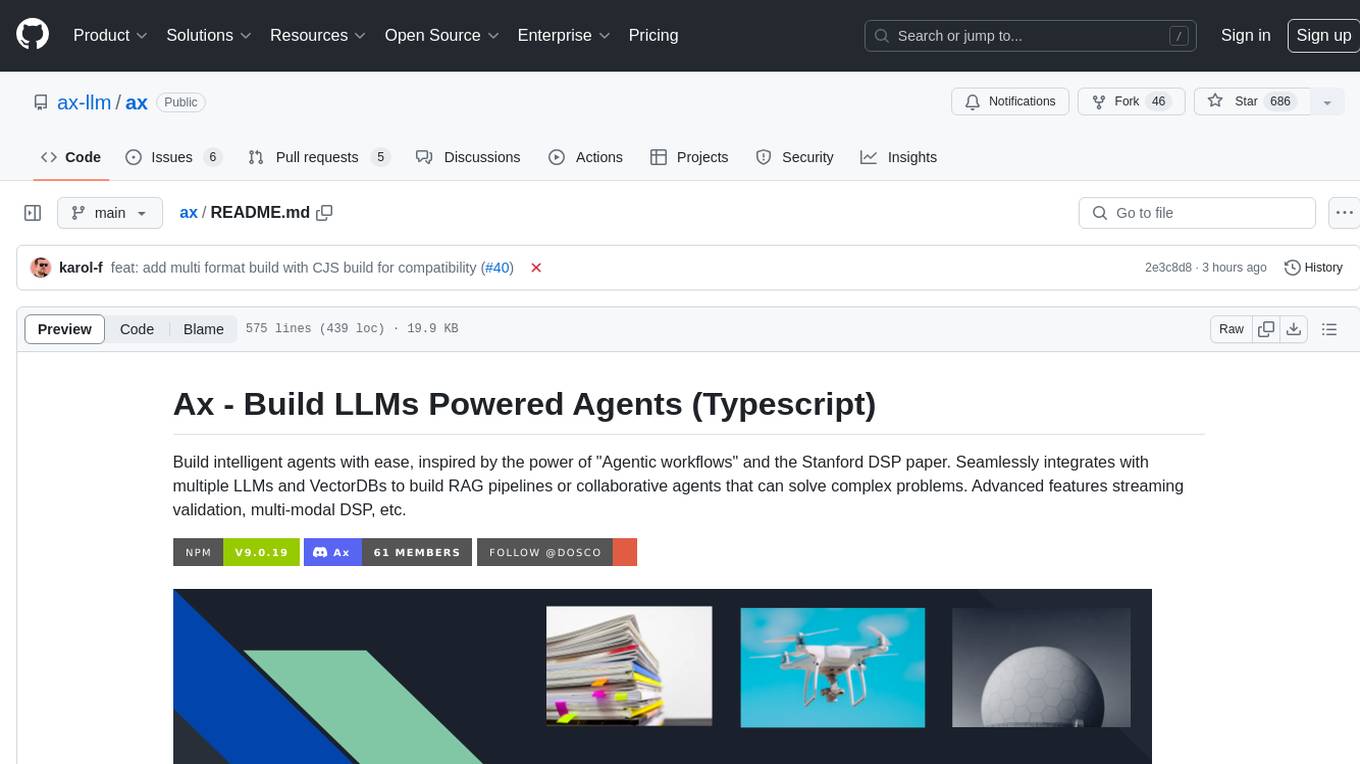
Ax is a Typescript library that allows users to build intelligent agents inspired by agentic workflows and the Stanford DSP paper. It seamlessly integrates with multiple Large Language Models (LLMs) and VectorDBs to create RAG pipelines or collaborative agents capable of solving complex problems. The library offers advanced features such as streaming validation, multi-modal DSP, and automatic prompt tuning using optimizers. Users can easily convert documents of any format to text, perform smart chunking, embedding, and querying, and ensure output validation while streaming. Ax is production-ready, written in Typescript, and has zero dependencies.
README:
Use Ax and get an end-to-end streaming, multi-modal DSPy framework with agents and typed signatures. Works with all LLMs. Ax is always streaming and handles parsing, validating, error-correcting and function calling all while streaming. Ax is easy, fast and lowers your token usage.
- Support for all top LLMs
- Prompts auto-generated from simple signatures
- Full native end-to-end streaming
- Build Agents that can call other agents
- Built in MCP, Model Context Protocol support
- Convert docs of any format to text
- RAG, smart chunking, embedding, querying
- Works with Vercel AI SDK
- Output validation while streaming
- Multi-modal DSPy supported
- Automatic prompt tuning using optimizers
- OpenTelemetry tracing / observability
- Production ready Typescript code
- Lite weight, zero-dependencies
Efficient type-safe prompts are auto-generated from a simple signature. A prompt signature is made up of a "task description" inputField:type "field description" -> "outputField:type
. The idea behind prompt signatures is based on work done in the "Demonstrate-Search-Predict" paper.
You can have multiple input and output fields, and each field can be of the types string
, number
, boolean
, date
, datetime
, class "class1, class2"
, JSON
, or an array of any of these, e.g., string[]
. When a type is not defined, it defaults to string
. The suffix ?
makes the field optional (required by default) and !
makes the field internal which is good for things like reasoning.
Type | Description | Usage | Example Output |
---|---|---|---|
string |
A sequence of characters. | fullName:string |
"example" |
number |
A numerical value. | price:number |
42 |
boolean |
A true or false value. | isEvent:boolean |
true , false
|
date |
A date value. | startDate:date |
"2023-10-01" |
datetime |
A date and time value. | createdAt:datetime |
"2023-10-01T12:00:00Z" |
class "class1,class2" |
A classification of items. | category:class |
["class1", "class2", "class3"] |
string[] |
An array of strings. | tags:string[] |
["example1", "example2"] |
number[] |
An array of numbers. | scores:number[] |
[1, 2, 3] |
boolean[] |
An array of boolean values. | permissions:boolean[] |
[true, false, true] |
date[] |
An array of dates. | holidayDates:date[] |
["2023-10-01", "2023-10-02"] |
datetime[] |
An array of date and time values. | logTimestamps:datetime[] |
["2023-10-01T12:00:00Z", "2023-10-02T12:00:00Z"] |
class[] "class1,class2" |
Multiple classes | categories:class[] |
["class1", "class2", "class3"] |
code "language" |
A code block in a specific language | code:code "python" |
print('Hello, world!') |
Google Gemini
, Google Vertex
, OpenAI
, Azure OpenAI
, TogetherAI
, Anthropic
, Cohere
, Mistral
, Groq
, DeepSeek
, Ollama
, Reka
, Hugging Face
npm install @ax-llm/ax
# or
yarn add @ax-llm/ax
import { AxAI, AxChainOfThought } from '@ax-llm/ax';
const textToSummarize = `
The technological singularity—or simply the singularity[1]—is a hypothetical future point in time at which technological growth becomes uncontrollable and irreversible, resulting in unforeseeable changes to human civilization.[2][3] ...`;
const ai = new AxAI({
name: 'openai',
apiKey: process.env.OPENAI_APIKEY as string
});
const gen = new AxChainOfThought(
`textToSummarize -> textType:class "note, email, reminder", shortSummary "summarize in 5 to 10 words"`
);
const res = await gen.forward(ai, { textToSummarize });
console.log('>', res);
Use the agent prompt (framework) to build agents that work with other agents to complete tasks. Agents are easy to make with prompt signatures. Try out the agent example.
# npm run tsx ./src/examples/agent.ts
const researcher = new AxAgent({
name: 'researcher',
description: 'Researcher agent',
signature: `physicsQuestion "physics questions" -> answer "reply in bullet points"`
});
const summarizer = new AxAgent({
name: 'summarizer',
description: 'Summarizer agent',
signature: `text "text so summarize" -> shortSummary "summarize in 5 to 10 words"`
});
const agent = new AxAgent({
name: 'agent',
description: 'A an agent to research complex topics',
signature: `question -> answer`,
agents: [researcher, summarizer]
});
agent.forward(ai, { questions: "How many atoms are there in the universe" })
Vector databases are critical to building LLM workflows. We have clean abstractions over popular vector databases and our own quick in-memory vector database.
Provider | Tested |
---|---|
In Memory | 🟢 100% |
Weaviate | 🟢 100% |
Cloudflare | 🟡 50% |
Pinecone | 🟡 50% |
// Create embeddings from text using an LLM
const ret = await this.ai.embed({ texts: 'hello world' });
// Create an in memory vector db
const db = new axDB('memory');
// Insert into vector db
await this.db.upsert({
id: 'abc',
table: 'products',
values: ret.embeddings[0]
});
// Query for similar entries using embeddings
const matches = await this.db.query({
table: 'products',
values: embeddings[0]
});
Alternatively you can use the AxDBManager
which handles smart chunking, embedding and querying everything
for you, it makes things almost too easy.
const manager = new AxDBManager({ ai, db });
await manager.insert(text);
const matches = await manager.query(
'John von Neumann on human intelligence and singularity.'
);
console.log(matches);
Using documents like PDF, DOCX, PPT, XLS, etc., with LLMs is a huge pain. We make it easy with Apache Tika, an open-source document processing engine.
Launch Apache Tika
docker run -p 9998:9998 apache/tika
Convert documents to text and embed them for retrieval using the AxDBManager
, which also supports a reranker and query rewriter. Two default implementations, AxDefaultResultReranker
and AxDefaultQueryRewriter
, are available.
const tika = new AxApacheTika();
const text = await tika.convert('/path/to/document.pdf');
const manager = new AxDBManager({ ai, db });
await manager.insert(text);
const matches = await manager.query('Find some text');
console.log(matches);
When using models like GPT-4o
and Gemini
that support multi-modal prompts, we support using image fields, and this works with the whole DSP pipeline.
const image = fs
.readFileSync('./src/examples/assets/kitten.jpeg')
.toString('base64');
const gen = new AxChainOfThought(`question, animalImage:image -> answer`);
const res = await gen.forward(ai, {
question: 'What family does this animal belong to?',
animalImage: { mimeType: 'image/jpeg', data: image }
});
When using models like gpt-4o-audio-preview
that support multi-modal prompts with audio support, we support using audio fields, and this works with the whole DSP pipeline.
const audio = fs
.readFileSync('./src/examples/assets/comment.wav')
.toString('base64');
const gen = new AxGen(`question, commentAudio:audio -> answer`);
const res = await gen.forward(ai, {
question: 'What family does this animal belong to?',
commentAudio: { format: 'wav', data: audio }
});
We support parsing output fields and function execution while streaming. This allows for fail-fast and error correction without waiting for the whole output, saving tokens and costs and reducing latency. Assertions are a powerful way to ensure the output matches your requirements; they also work with streaming.
// setup the prompt program
const gen = new AxChainOfThought(
ai,
`startNumber:number -> next10Numbers:number[]`
);
// add a assertion to ensure that the number 5 is not in an output field
gen.addAssert(({ next10Numbers }: Readonly<{ next10Numbers: number[] }>) => {
return next10Numbers ? !next10Numbers.includes(5) : undefined;
}, 'Numbers 5 is not allowed');
// run the program with streaming enabled
const res = await gen.forward({ startNumber: 1 }, { stream: true });
// or run the program with end-to-end streaming
const generator = await gen.streamingForward({ startNumber: 1 }, { stream: true });
for await (const res of generator) {}
The above example allows you to validate entire output fields as they are streamed in. This validation works with streaming and when not streaming and is triggered when the whole field value is available. For true validation while streaming, check out the example below. This will massively improve performance and save tokens at scale in production.
// add a assertion to ensure all lines start with a number and a dot.
gen.addStreamingAssert(
'answerInPoints',
(value: string) => {
const re = /^\d+\./;
// split the value by lines, trim each line,
// filter out empty lines and check if all lines match the regex
return value
.split('\n')
.map((x) => x.trim())
.filter((x) => x.length > 0)
.every((x) => re.test(x));
},
'Lines must start with a number and a dot. Eg: 1. This is a line.'
);
// run the program with streaming enabled
const res = await gen.forward(
{
question: 'Provide a list of optimizations to speedup LLM inference.'
},
{ stream: true, debug: true }
);
Field processors are a powerful way to process fields in a prompt. They are used to process fields in a prompt before the prompt is sent to the LLM.
const gen = new AxChainOfThought(
ai,
`startNumber:number -> next10Numbers:number[]`
);
const streamValue = false
const processorFunction = (value) => {
return value.map((x) => x + 1);
}
// Add a field processor to the program
const processor = new AxFieldProcessor(gen, 'next10Numbers', processorFunction, streamValue);
const res = await gen.forward({ startNumber: 1 });
Ax provides two powerful ways to work with multiple AI services: a load balancer for high availability and a router for model-specific routing.
The load balancer automatically distributes requests across multiple AI services based on performance and availability. If one service fails, it automatically fails over to the next available service.
import { AxAI, AxBalancer } from '@ax-llm/ax'
// Setup multiple AI services
const openai = new AxAI({
name: 'openai',
apiKey: process.env.OPENAI_APIKEY,
})
const ollama = new AxAI({
name: 'ollama',
config: { model: "nous-hermes2" }
})
const gemini = new AxAI({
name: 'google-gemini',
apiKey: process.env.GOOGLE_APIKEY
})
// Create a load balancer with all services
const balancer = new AxBalancer([openai, ollama, gemini])
// Use like a regular AI service - automatically uses the best available service
const response = await balancer.chat({
chatPrompt: [{ role: 'user', content: 'Hello!' }],
})
// Or use the balance with AxGen
const gen = new AxGen(`question -> answer`)
const res = await gen.forward(balancer,{ question: 'Hello!' })
The router lets you use multiple AI services through a single interface, automatically routing requests to the right service based on the model specified.
import { AxAI, AxMultiServiceRouter, AxAIOpenAIModel } from '@ax-llm/ax'
// Setup OpenAI with model list
const openai = new AxAI({
name: 'openai',
apiKey: process.env.OPENAI_APIKEY,
models: [
{
key: 'basic',
model: AxAIOpenAIModel.GPT4OMini,
description: 'Model for very simple tasks such as answering quick short questions',
},
{
key: 'medium',
model: AxAIOpenAIModel.GPT4O,
description: 'Model for semi-complex tasks such as summarizing text, writing code, and more',
}
]
})
// Setup Gemini with model list
const gemini = new AxAI({
name: 'google-gemini',
apiKey: process.env.GOOGLE_APIKEY,
models: [
{
key: 'deep-thinker',
model: 'gemini-2.0-flash-thinking',
description: 'Model that can think deeply about a task, best for tasks that require planning',
},
{
key: 'expert',
model: 'gemini-2.0-pro',
description: 'Model that is the best for very complex tasks such as writing large essays, complex coding, and more',
}
]
})
const ollama = new AxAI({
name: 'ollama',
config: { model: "nous-hermes2" }
})
const secretService = {
key: 'sensitive-secret',
service: ollama,
description: 'Model for sensitive secrets tasks'
}
// Create a router with all services
const router = new AxMultiServiceRouter([openai, gemini, secretService])
// Route to OpenAI's expert model
const openaiResponse = await router.chat({
chatPrompt: [{ role: 'user', content: 'Hello!' }],
model: 'expert'
})
// Or use the router with AxGen
const gen = new AxGen(`question -> answer`)
const res = await gen.forward(router, { question: 'Hello!' })
The load balancer is ideal for high availability while the router is perfect when you need specific models for specific tasks Both can be used with any of Ax's features like streaming, function calling, and chain-of-thought prompting.
You can also use the balancer and the router together either the multiple balancers can be used with the router or the router can be used with the balancer.
Ax provides seamless integration with the Model Context Protocol (MCP), allowing your agents to access external tools, and resources through a standardized interface.
The AxMCPClient
allows you to connect to any MCP-compatible server and use its capabilities within your Ax agents:
import { AxMCPClient, AxMCPStdioTransport } from '@ax-llm/ax'
// Initialize an MCP client with a transport
const transport = new AxMCPStdioTransport({
command: 'npx',
args: ['-y', '@modelcontextprotocol/server-memory'],
})
// Create the client with optional debug mode
const client = new AxMCPClient(transport, { debug: true })
// Initialize the connection
await client.init()
// Use the client's functions in an agent
const memoryAgent = new AxAgent({
name: 'MemoryAssistant',
description: 'An assistant with persistent memory',
signature: 'input, userId -> response',
functions: [client], // Pass the client as a function provider
})
// Or use the client with AxGen
const memoryGen = new AxGen('input, userId -> response', {
functions: [client]
})
Install the ax provider package
npm i @ax-llm/ax-ai-sdk-provider
Then use it with the AI SDK, you can either use the AI provider or the Agent Provider
const ai = new AxAI({
name: 'openai',
apiKey: process.env['OPENAI_APIKEY'] ?? "",
});
// Create a model using the provider
const model = new AxAIProvider(ai);
export const foodAgent = new AxAgent({
name: 'food-search',
description:
'Use this agent to find restaurants based on what the customer wants',
signature,
functions
})
// Get vercel ai sdk state
const aiState = getMutableAIState()
// Create an agent for a specific task
const foodAgent = new AxAgentProvider(ai, {
agent: foodAgent,
updateState: (state) => {
aiState.done({ ...aiState.get(), state })
},
generate: async ({ restaurant, priceRange }) => {
return (
<BotCard>
<h1>{restaurant as string} {priceRange as string}</h1>
</BotCard>
)
}
})
// Use with streamUI a critical part of building chat UIs in the AI SDK
const result = await streamUI({
model,
initial: <SpinnerMessage />,
messages: [
// ...
],
text: ({ content, done, delta }) => {
// ...
},
tools: {
// @ts-ignore
'find-food': foodAgent,
}
})
The ability to trace and observe your llm workflow is critical to building production workflows. OpenTelemetry is an industry-standard, and we support the new gen_ai
attribute namespace.
import { trace } from '@opentelemetry/api';
import {
BasicTracerProvider,
ConsoleSpanExporter,
SimpleSpanProcessor
} from '@opentelemetry/sdk-trace-base';
const provider = new BasicTracerProvider();
provider.addSpanProcessor(new SimpleSpanProcessor(new ConsoleSpanExporter()));
trace.setGlobalTracerProvider(provider);
const tracer = trace.getTracer('test');
const ai = new AxAI({
name: 'ollama',
config: { model: 'nous-hermes2' },
options: { tracer }
});
const gen = new AxChainOfThought(
ai,
`text -> shortSummary "summarize in 5 to 10 words"`
);
const res = await gen.forward({ text });
{
"traceId": "ddc7405e9848c8c884e53b823e120845",
"name": "Chat Request",
"id": "d376daad21da7a3c",
"kind": "SERVER",
"timestamp": 1716622997025000,
"duration": 14190456.542,
"attributes": {
"gen_ai.system": "Ollama",
"gen_ai.request.model": "nous-hermes2",
"gen_ai.request.max_tokens": 500,
"gen_ai.request.temperature": 0.1,
"gen_ai.request.top_p": 0.9,
"gen_ai.request.frequency_penalty": 0.5,
"gen_ai.request.llm_is_streaming": false,
"http.request.method": "POST",
"url.full": "http://localhost:11434/v1/chat/completions",
"gen_ai.usage.completion_tokens": 160,
"gen_ai.usage.prompt_tokens": 290
}
}
You can tune your prompts using a larger model to help them run more efficiently and give you better results. This is done by using an optimizer like AxBootstrapFewShot
with and examples from the popular HotPotQA
dataset. The optimizer generates demonstrations demos
which when used with the prompt help improve its efficiency.
// Download the HotPotQA dataset from huggingface
const hf = new AxHFDataLoader({
dataset: 'hotpot_qa',
split: 'train'
});
const examples = await hf.getData<{ question: string; answer: string }>({
count: 100,
fields: ['question', 'answer']
});
const ai = new AxAI({
name: 'openai',
apiKey: process.env.OPENAI_APIKEY as string
});
// Setup the program to tune
const program = new AxChainOfThought<{ question: string }, { answer: string }>(
ai,
`question -> answer "in short 2 or 3 words"`
);
// Setup a Bootstrap Few Shot optimizer to tune the above program
const optimize = new AxBootstrapFewShot<
{ question: string },
{ answer: string }
>({
program,
examples
});
// Setup a evaluation metric em, f1 scores are a popular way measure retrieval performance.
const metricFn: AxMetricFn = ({ prediction, example }) =>
emScore(prediction.answer as string, example.answer as string);
// Run the optimizer and remember to save the result to use later
const result = await optimize.compile(metricFn);
And to use the generated demos with the above ChainOfThought
program
const ai = new AxAI({
name: 'openai',
apiKey: process.env.OPENAI_APIKEY as string
});
// Setup the program to use the tuned data
const program = new AxChainOfThought<{ question: string }, { answer: string }>(
ai,
`question -> answer "in short 2 or 3 words"`
);
// load tuning data
program.loadDemos('demos.json');
const res = await program.forward({
question: 'What castle did David Gregory inherit?'
});
console.log(res);
MiPRO v2 is an advanced prompt optimization framework that uses Bayesian optimization to automatically find the best instructions, demonstrations, and examples for your LLM programs. By systematically exploring different prompt configurations, MiPRO v2 helps maximize model performance without manual tuning.
- Instruction optimization: Automatically generates and tests multiple instruction candidates
- Few-shot example selection: Finds optimal demonstrations from your dataset
- Smart Bayesian optimization: Uses UCB (Upper Confidence Bound) strategy to efficiently explore configurations
- Early stopping: Stops optimization when improvements plateau to save compute
- Program and data-aware: Considers program structure and dataset characteristics
import { AxAI, AxChainOfThought, AxMiPRO } from '@ax-llm/ax'
// 1. Setup your AI service
const ai = new AxAI({
name: 'google-gemini',
apiKey: process.env.GOOGLE_APIKEY
})
// 2. Create your program
const program = new AxChainOfThought(`input -> output`)
// 3. Configure the optimizer
const optimizer = new AxMiPRO({
ai,
program,
examples: trainingData, // Your training examples
options: {
numTrials: 20, // Number of configurations to try
auto: 'medium' // Optimization level
}
})
// 4. Define your evaluation metric
const metricFn = ({ prediction, example }) => {
return prediction.output === example.output
}
// 5. Run the optimization
const optimizedProgram = await optimizer.compile(metricFn, {
valset: validationData // Optional validation set
})
// 6. Use the optimized program
const result = await optimizedProgram.forward(ai, { input: "test input" })
MiPRO v2 provides extensive configuration options:
Option | Description | Default |
---|---|---|
numCandidates |
Number of instruction candidates to generate | 5 |
numTrials |
Number of optimization trials | 30 |
maxBootstrappedDemos |
Maximum number of bootstrapped demonstrations | 3 |
maxLabeledDemos |
Maximum number of labeled examples | 4 |
minibatch |
Use minibatching for faster evaluation | true |
minibatchSize |
Size of evaluation minibatches | 25 |
earlyStoppingTrials |
Stop if no improvement after N trials | 5 |
minImprovementThreshold |
Minimum score improvement threshold | 0.01 |
programAwareProposer |
Use program structure for better proposals | true |
dataAwareProposer |
Consider dataset characteristics | true |
verbose |
Show detailed optimization progress | false |
You can quickly configure optimization intensity with the auto
parameter:
// Light optimization (faster, less thorough)
const optimizedProgram = await optimizer.compile(metricFn, { auto: 'light' })
// Medium optimization (balanced)
const optimizedProgram = await optimizer.compile(metricFn, { auto: 'medium' })
// Heavy optimization (slower, more thorough)
const optimizedProgram = await optimizer.compile(metricFn, { auto: 'heavy' })
// Create sentiment analysis program
const classifyProgram = new AxChainOfThought<
{ productReview: string },
{ label: string }
>(`productReview -> label:string "positive" or "negative"`)
// Configure optimizer with advanced settings
const optimizer = new AxMiPRO({
ai,
program: classifyProgram,
examples: trainingData,
options: {
numCandidates: 3,
numTrials: 10,
maxBootstrappedDemos: 2,
maxLabeledDemos: 3,
earlyStoppingTrials: 3,
programAwareProposer: true,
dataAwareProposer: true,
verbose: true
}
})
// Run optimization and save the result
const optimizedProgram = await optimizer.compile(metricFn, {
valset: validationData
})
// Save configuration for future use
const programConfig = JSON.stringify(optimizedProgram, null, 2)
await fs.promises.writeFile('./optimized-config.json', programConfig)
MiPRO v2 works through these steps:
- Generates various instruction candidates
- Bootstraps few-shot examples from your data
- Selects labeled examples directly from your dataset
- Uses Bayesian optimization to find the optimal combination
- Applies the best configuration to your program
By exploring the space of possible prompt configurations and systematically measuring performance, MiPRO v2 delivers optimized prompts that maximize your model's effectiveness.
Function | Name | Description |
---|---|---|
JS Interpreter | AxJSInterpreter | Execute JS code in a sandboxed env |
Docker Sandbox | AxDockerSession | Execute commands within a docker environment |
Embeddings Adapter | AxEmbeddingAdapter | Fetch and pass embedding to your function |
Use the tsx
command to run the examples. It makes the node run typescript code. It also supports using an .env
file to pass the AI API Keys instead of putting them in the command line.
OPENAI_APIKEY=openai_key npm run tsx ./src/examples/marketing.ts
Example | Description |
---|---|
customer-support.ts | Extract valuable details from customer communications |
function.ts | Simple single function calling example |
food-search.ts | Multi-step, multi-function calling example |
marketing.ts | Generate short effective marketing sms messages |
vectordb.ts | Chunk, embed and search text |
fibonacci.ts | Use the JS code interpreter to compute fibonacci |
summarize.ts | Generate a short summary of a large block of text |
chain-of-thought.ts | Use chain-of-thought prompting to answer questions |
rag.ts | Use multi-hop retrieval to answer questions |
rag-docs.ts | Convert PDF to text and embed for rag search |
react.ts | Use function calling and reasoning to answer questions |
agent.ts | Agent framework, agents can use other agents, tools etc |
streaming1.ts | Output fields validation while streaming |
streaming2.ts | Per output field validation while streaming |
streaming3.ts | End-to-end streaming example streamingForward()
|
smart-hone.ts | Agent looks for dog in smart home |
multi-modal.ts | Use an image input along with other text inputs |
balancer.ts | Balance between various llm's based on cost, etc |
docker.ts | Use the docker sandbox to find files by description |
prime.ts | Using field processors to process fields in a prompt |
simple-classify.ts | Use a simple classifier to classify stuff |
mcp-client-memory.ts | Example of using an MCP server for memory with Ax |
mcp-client-blender.ts | Example of using an MCP server for Blender with Ax |
tune-bootstrap.ts | Use bootstrap optimizer to improve prompt efficiency |
tune-mipro.ts | Use mipro v2 optimizer to improve prompt efficiency |
tune-usage.ts | Use the optimized tuned prompts |
Large language models (LLMs) are becoming really powerful and have reached a point where they can work as the backend for your entire product. However, there's still a lot of complexity to manage from using the correct prompts, models, streaming, function calls, error correction, and much more. We aim to package all this complexity into a well-maintained, easy-to-use library that can work with all state-of-the-art LLMs. Additionally, we are using the latest research to add new capabilities like DSPy to the library.
// Pick a LLM
const ai = new AxOpenAI({ apiKey: process.env.OPENAI_APIKEY } as AxOpenAIArgs);
// Signature defines the inputs and outputs of your prompt program
const cot = new ChainOfThought(ai, `question:string -> answer:string`, { mem });
// Pass in the input fields defined in the above signature
const res = await cot.forward({ question: 'Are we in a simulation?' });
const res = await ai.chat([
{ role: "system", content: "Help the customer with his questions" }
{ role: "user", content: "I'm looking for a Macbook Pro M2 With 96GB RAM?" }
]);
// define one or more functions and a function handler
const functions = [
{
name: 'getCurrentWeather',
description: 'get the current weather for a location',
parameters: {
type: 'object',
properties: {
location: {
type: 'string',
description: 'location to get weather for'
},
units: {
type: 'string',
enum: ['imperial', 'metric'],
default: 'imperial',
description: 'units to use'
}
},
required: ['location']
},
func: async (args: Readonly<{ location: string; units: string }>) => {
return `The weather in ${args.location} is 72 degrees`;
}
}
];
const cot = new AxGen(ai, `question:string -> answer:string`, { functions });
const ai = new AxAI({ name: "openai", apiKey: process.env.OPENAI_APIKEY } as AxOpenAIArgs);
ai.setOptions({ debug: true });
We're happy to help reach out if you have questions or join the Discord twitter/dosco
Improve the function naming and description. Be very clear about what the function does. Also, ensure the function parameters have good descriptions. The descriptions can be a little short but need to be precise.
You can pass a configuration object as the second parameter when creating a new LLM object.
const apiKey = process.env.OPENAI_APIKEY;
const conf = AxOpenAIBestConfig();
const ai = new AxOpenAI({ apiKey, conf } as AxOpenAIArgs);
const conf = axOpenAIDefaultConfig(); // or OpenAIBestOptions()
conf.maxTokens = 2000;
const conf = axOpenAIDefaultConfig(); // or OpenAIBestOptions()
conf.model = OpenAIModel.GPT4Turbo;
It is essential to remember that we should only run npm install
from the root directory. This prevents the creation of nested package-lock.json
files and avoids non-deduplicated node_modules
.
Adding new dependencies in packages should be done with e.g. npm install lodash --workspace=ax
(or just modify the appropriate package.json
and run npm install
from root).
For Tasks:
Click tags to check more tools for each tasksFor Jobs:
Alternative AI tools for ax
Similar Open Source Tools
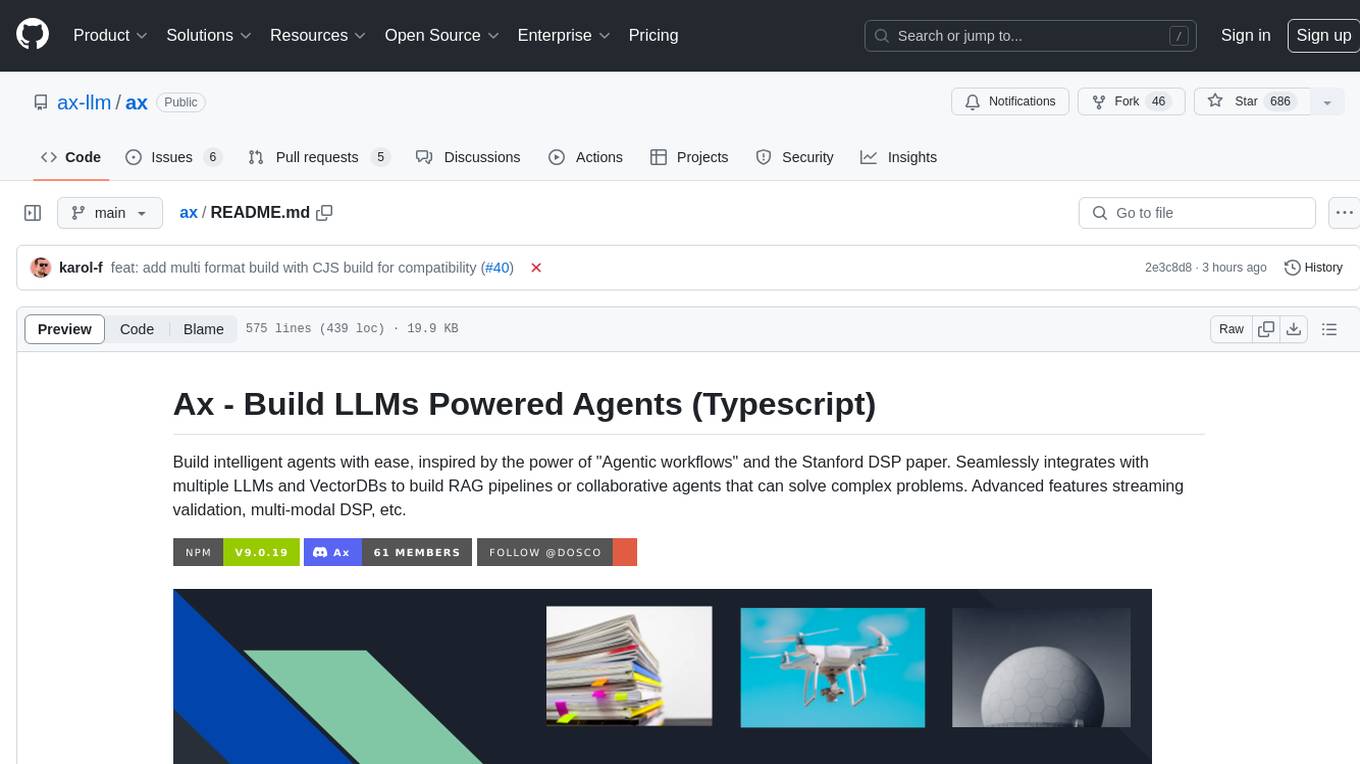
ax
Ax is a Typescript library that allows users to build intelligent agents inspired by agentic workflows and the Stanford DSP paper. It seamlessly integrates with multiple Large Language Models (LLMs) and VectorDBs to create RAG pipelines or collaborative agents capable of solving complex problems. The library offers advanced features such as streaming validation, multi-modal DSP, and automatic prompt tuning using optimizers. Users can easily convert documents of any format to text, perform smart chunking, embedding, and querying, and ensure output validation while streaming. Ax is production-ready, written in Typescript, and has zero dependencies.
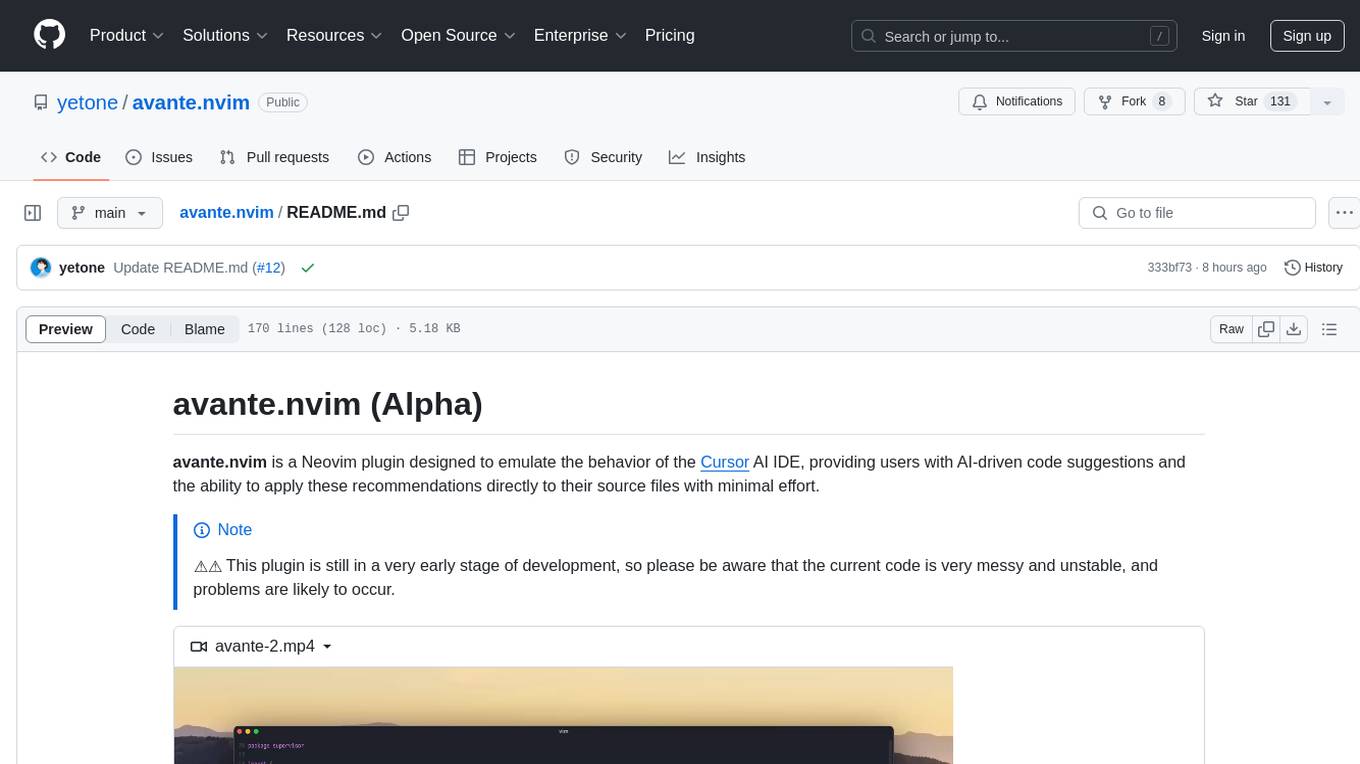
avante.nvim
avante.nvim is a Neovim plugin that emulates the behavior of the Cursor AI IDE, providing AI-driven code suggestions and enabling users to apply recommendations to their source files effortlessly. It offers AI-powered code assistance and one-click application of suggested changes, streamlining the editing process and saving time. The plugin is still in early development, with functionalities like setting API keys, querying AI about code, reviewing suggestions, and applying changes. Key bindings are available for various actions, and the roadmap includes enhancing AI interactions, stability improvements, and introducing new features for coding tasks.
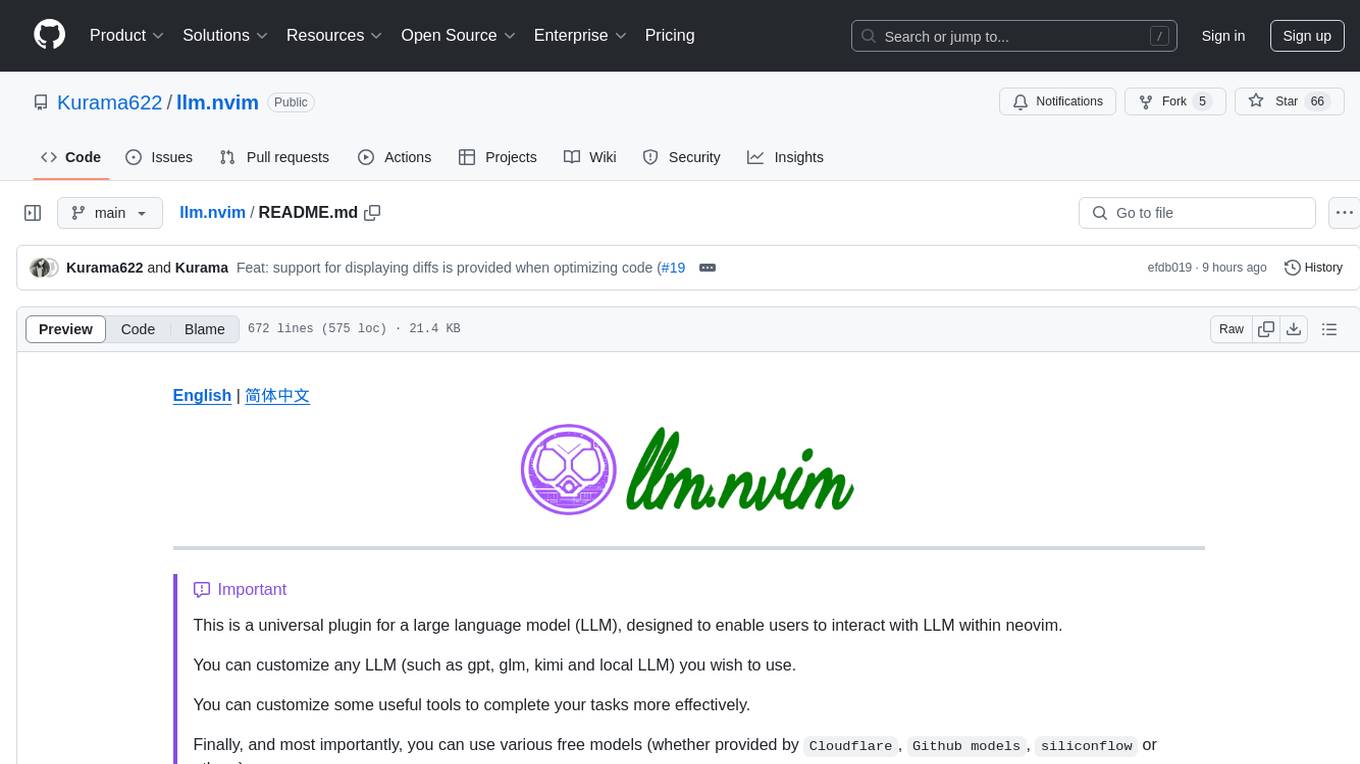
llm.nvim
llm.nvim is a universal plugin for a large language model (LLM) designed to enable users to interact with LLM within neovim. Users can customize various LLMs such as gpt, glm, kimi, and local LLM. The plugin provides tools for optimizing code, comparing code, translating text, and more. It also supports integration with free models from Cloudflare, Github models, siliconflow, and others. Users can customize tools, chat with LLM, quickly translate text, and explain code snippets. The plugin offers a flexible window interface for easy interaction and customization.
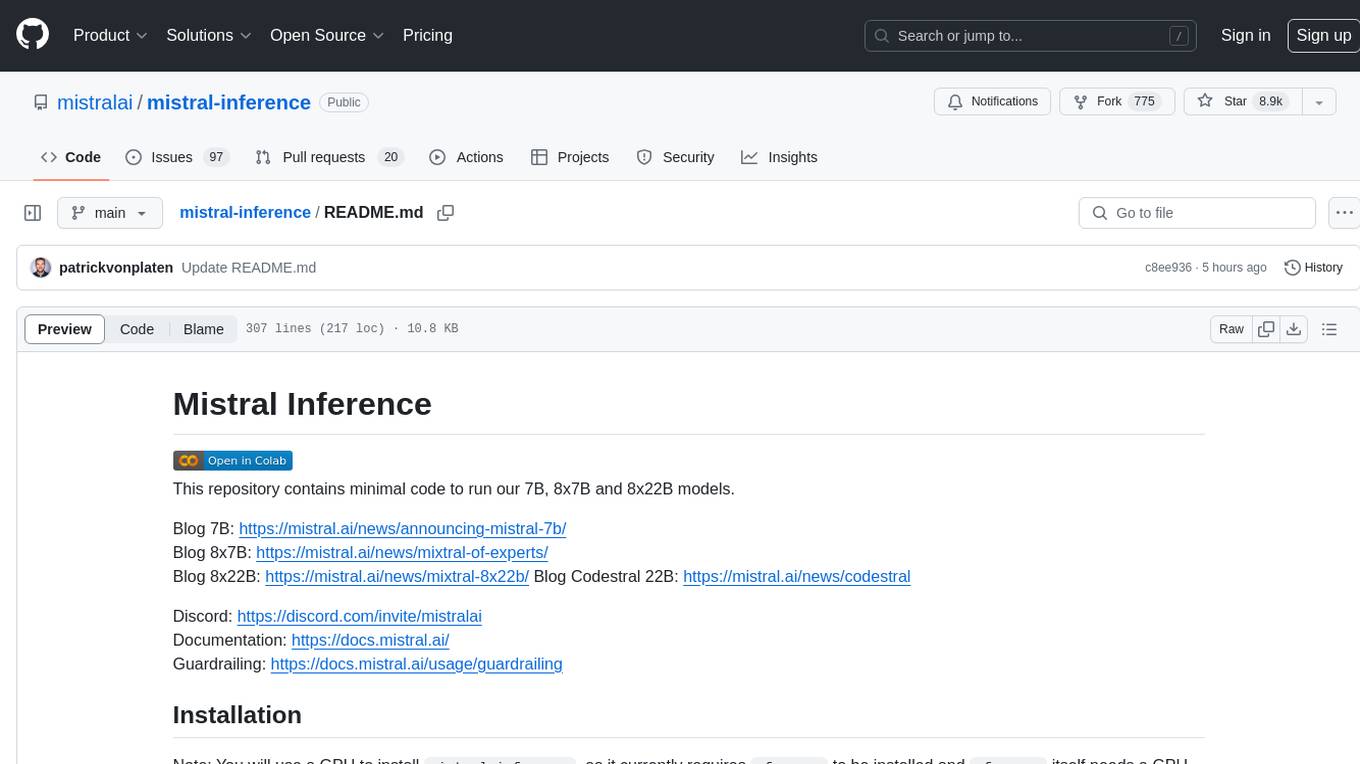
mistral-inference
Mistral Inference repository contains minimal code to run 7B, 8x7B, and 8x22B models. It provides model download links, installation instructions, and usage guidelines for running models via CLI or Python. The repository also includes information on guardrailing, model platforms, deployment, and references. Users can interact with models through commands like mistral-demo, mistral-chat, and mistral-common. Mistral AI models support function calling and chat interactions for tasks like testing models, chatting with models, and using Codestral as a coding assistant. The repository offers detailed documentation and links to blogs for further information.
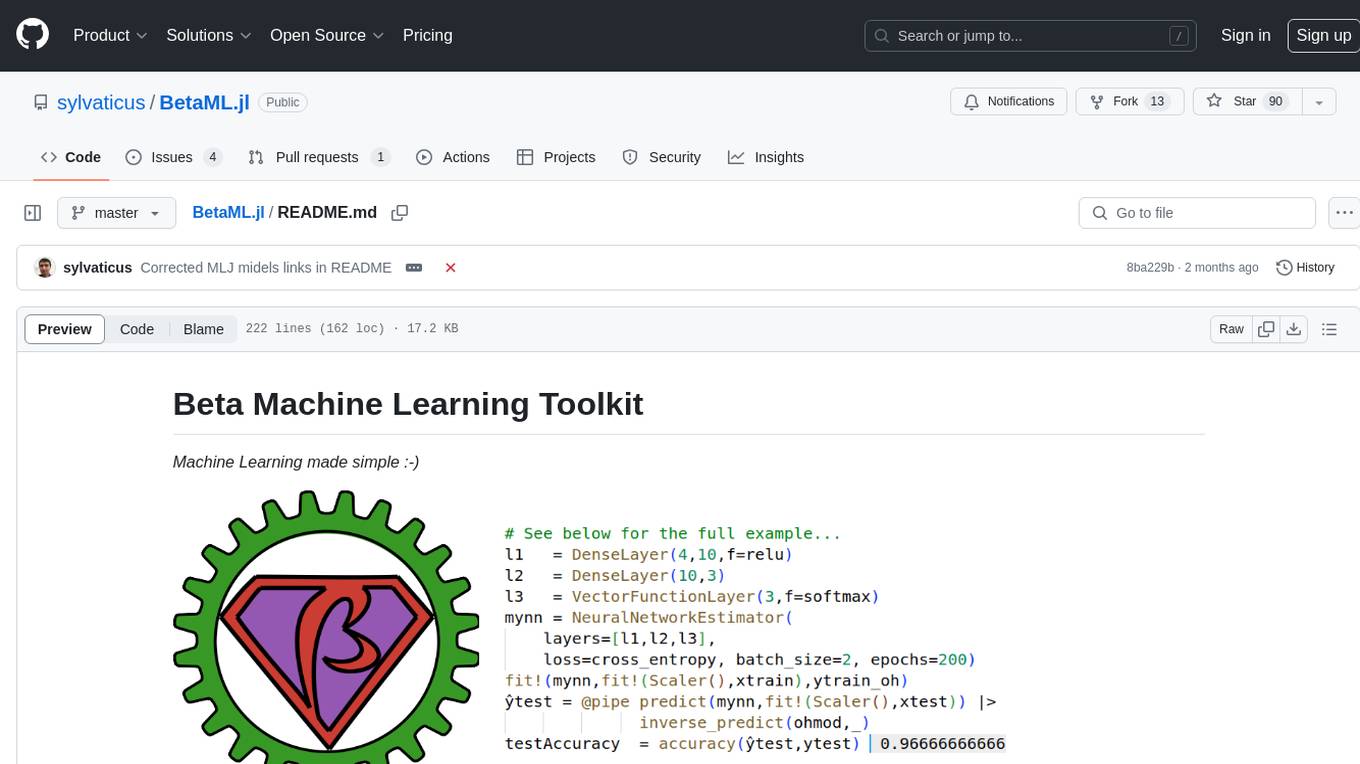
BetaML.jl
The Beta Machine Learning Toolkit is a package containing various algorithms and utilities for implementing machine learning workflows in multiple languages, including Julia, Python, and R. It offers a range of supervised and unsupervised models, data transformers, and assessment tools. The models are implemented entirely in Julia and are not wrappers for third-party models. Users can easily contribute new models or request implementations. The focus is on user-friendliness rather than computational efficiency, making it suitable for educational and research purposes.
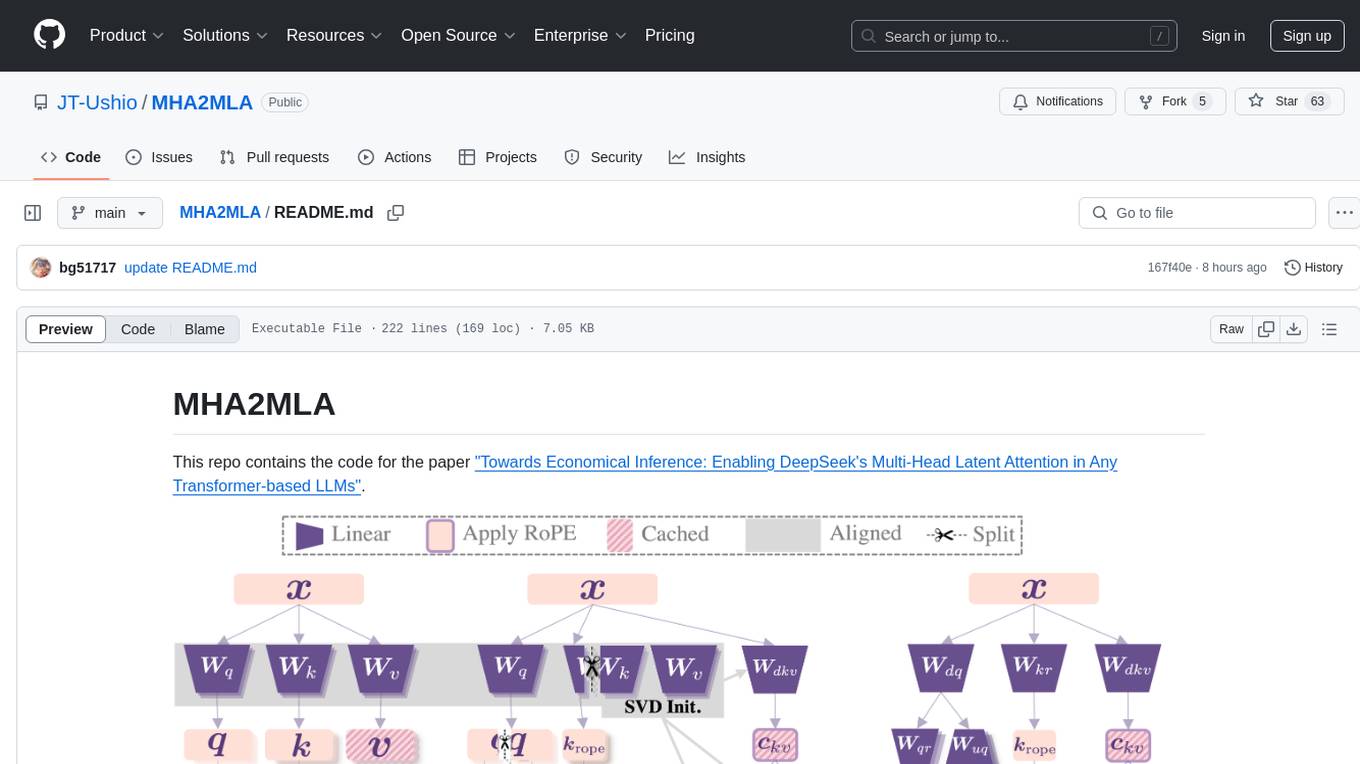
MHA2MLA
This repository contains the code for the paper 'Towards Economical Inference: Enabling DeepSeek's Multi-Head Latent Attention in Any Transformer-based LLMs'. It provides tools for fine-tuning and evaluating Llama models, converting models between different frameworks, processing datasets, and performing specific model training tasks like Partial-RoPE Fine-Tuning and Multiple-Head Latent Attention Fine-Tuning. The repository also includes commands for model evaluation using Lighteval and LongBench, along with necessary environment setup instructions.
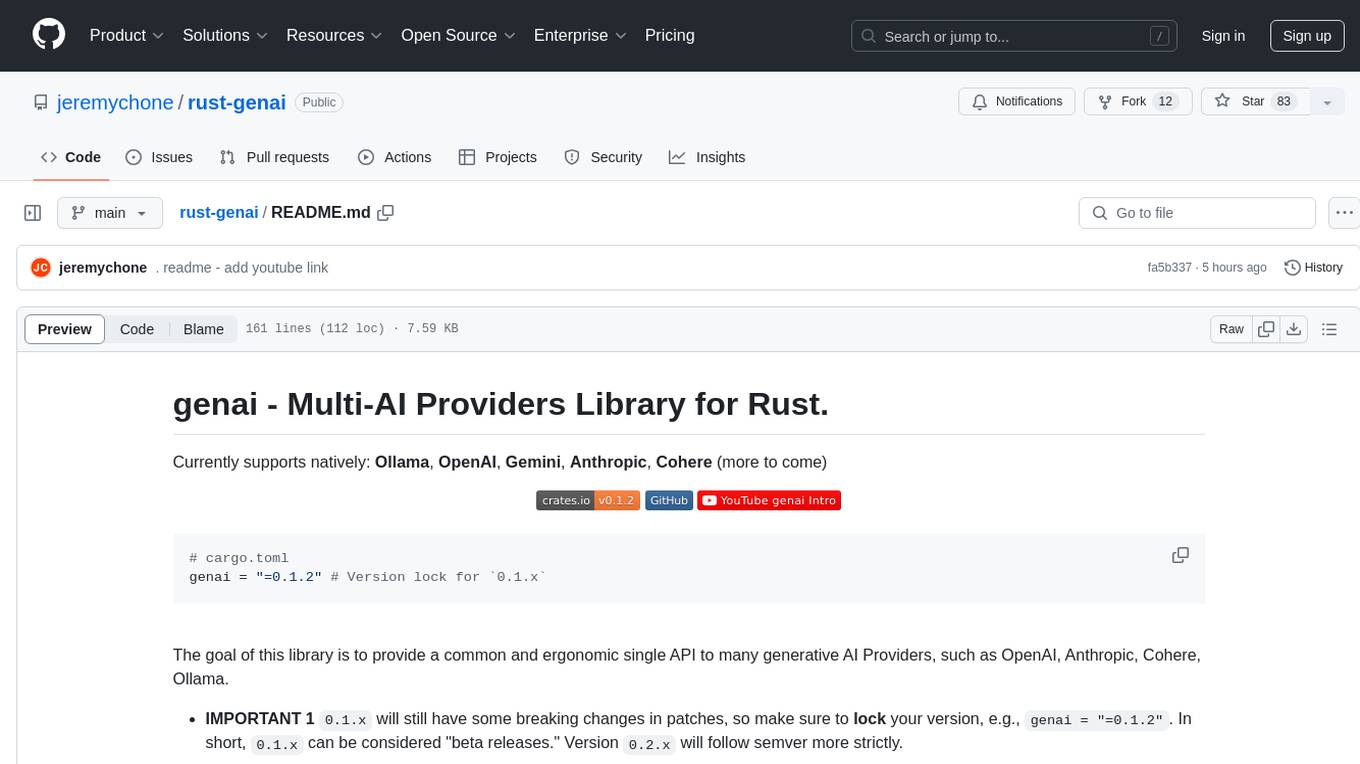
rust-genai
genai is a multi-AI providers library for Rust that aims to provide a common and ergonomic single API to various generative AI providers such as OpenAI, Anthropic, Cohere, Ollama, and Gemini. It focuses on standardizing chat completion APIs across major AI services, prioritizing ergonomics and commonality. The library initially focuses on text chat APIs and plans to expand to support images, function calling, and more in the future versions. Version 0.1.x will have breaking changes in patches, while version 0.2.x will follow semver more strictly. genai does not provide a full representation of a given AI provider but aims to simplify the differences at a lower layer for ease of use.
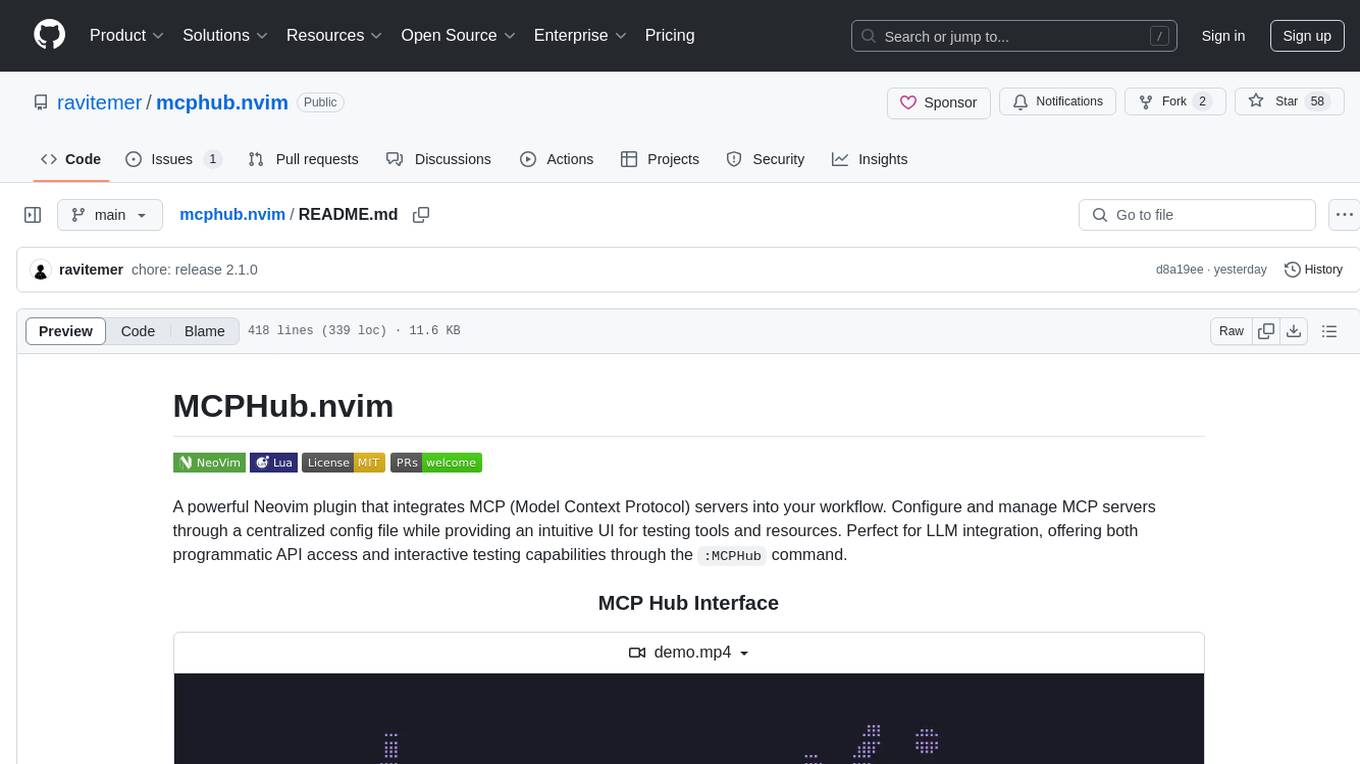
mcphub.nvim
MCPHub.nvim is a powerful Neovim plugin that integrates MCP (Model Context Protocol) servers into your workflow. It offers a centralized config file for managing servers and tools, with an intuitive UI for testing resources. Ideal for LLM integration, it provides programmatic API access and interactive testing through the `:MCPHub` command.
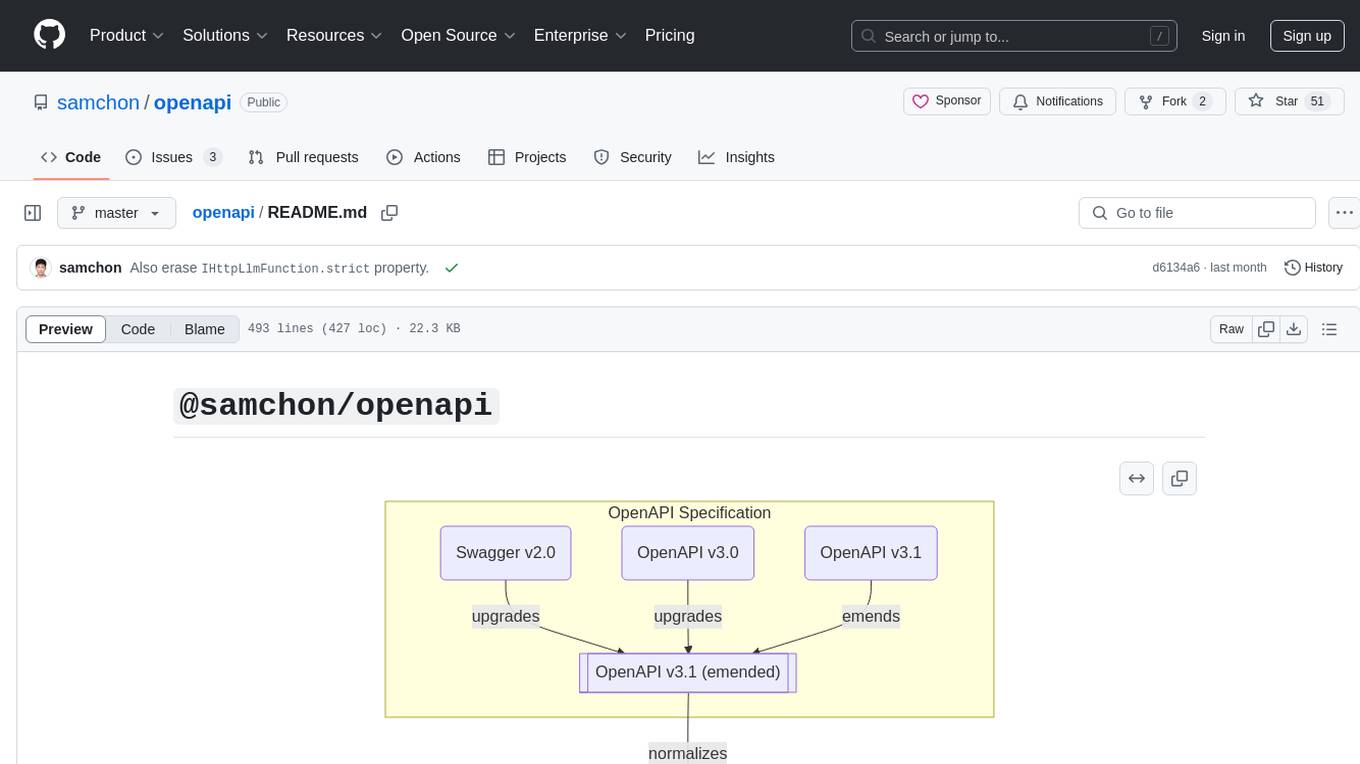
openapi
The `@samchon/openapi` repository is a collection of OpenAPI types and converters for various versions of OpenAPI specifications. It includes an 'emended' OpenAPI v3.1 specification that enhances clarity by removing ambiguous and duplicated expressions. The repository also provides an application composer for LLM (Large Language Model) function calling from OpenAPI documents, allowing users to easily perform LLM function calls based on the Swagger document. Conversions to different versions of OpenAPI documents are also supported, all based on the emended OpenAPI v3.1 specification. Users can validate their OpenAPI documents using the `typia` library with `@samchon/openapi` types, ensuring compliance with standard specifications.
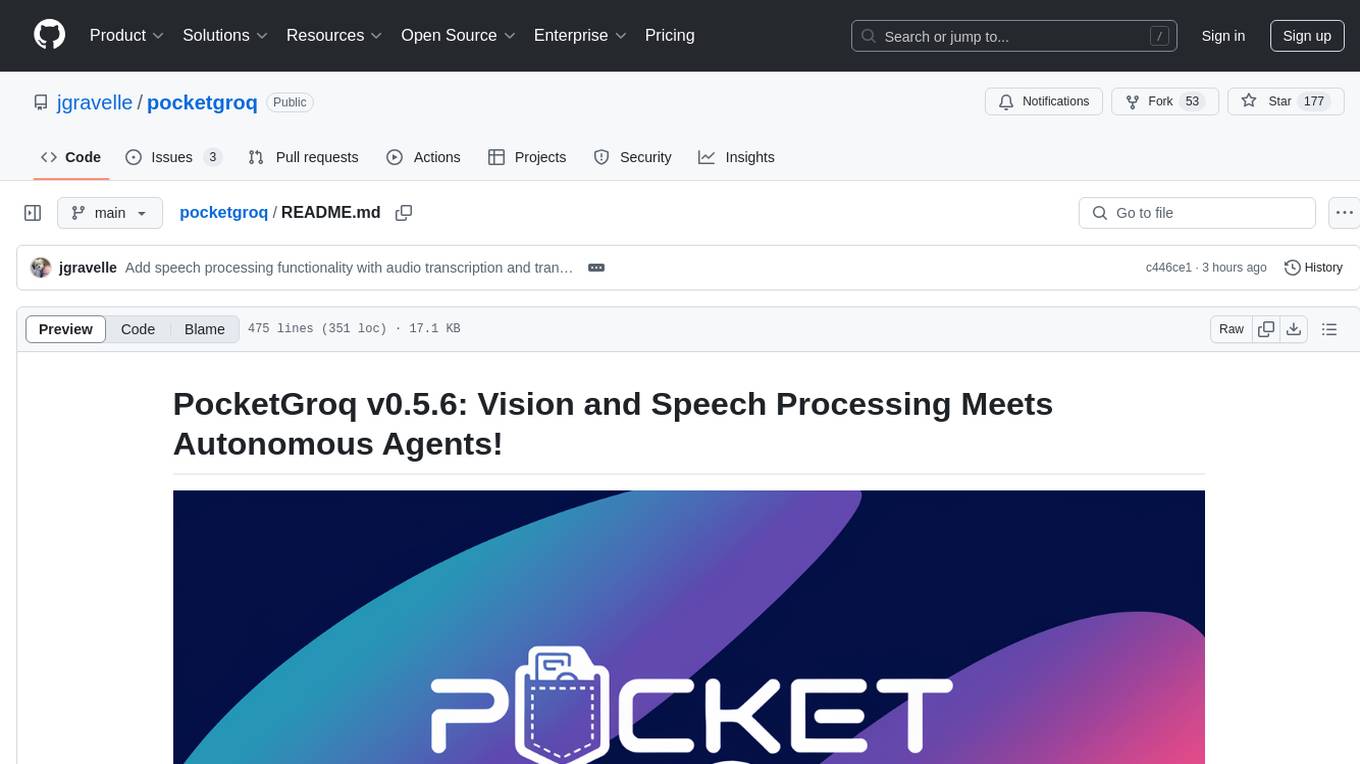
pocketgroq
PocketGroq is a tool that provides advanced functionalities for text generation, web scraping, web search, and AI response evaluation. It includes features like an Autonomous Agent for answering questions, web crawling and scraping capabilities, enhanced web search functionality, and flexible integration with Ollama server. Users can customize the agent's behavior, evaluate responses using AI, and utilize various methods for text generation, conversation management, and Chain of Thought reasoning. The tool offers comprehensive methods for different tasks, such as initializing RAG, error handling, and tool management. PocketGroq is designed to enhance development processes and enable the creation of AI-powered applications with ease.
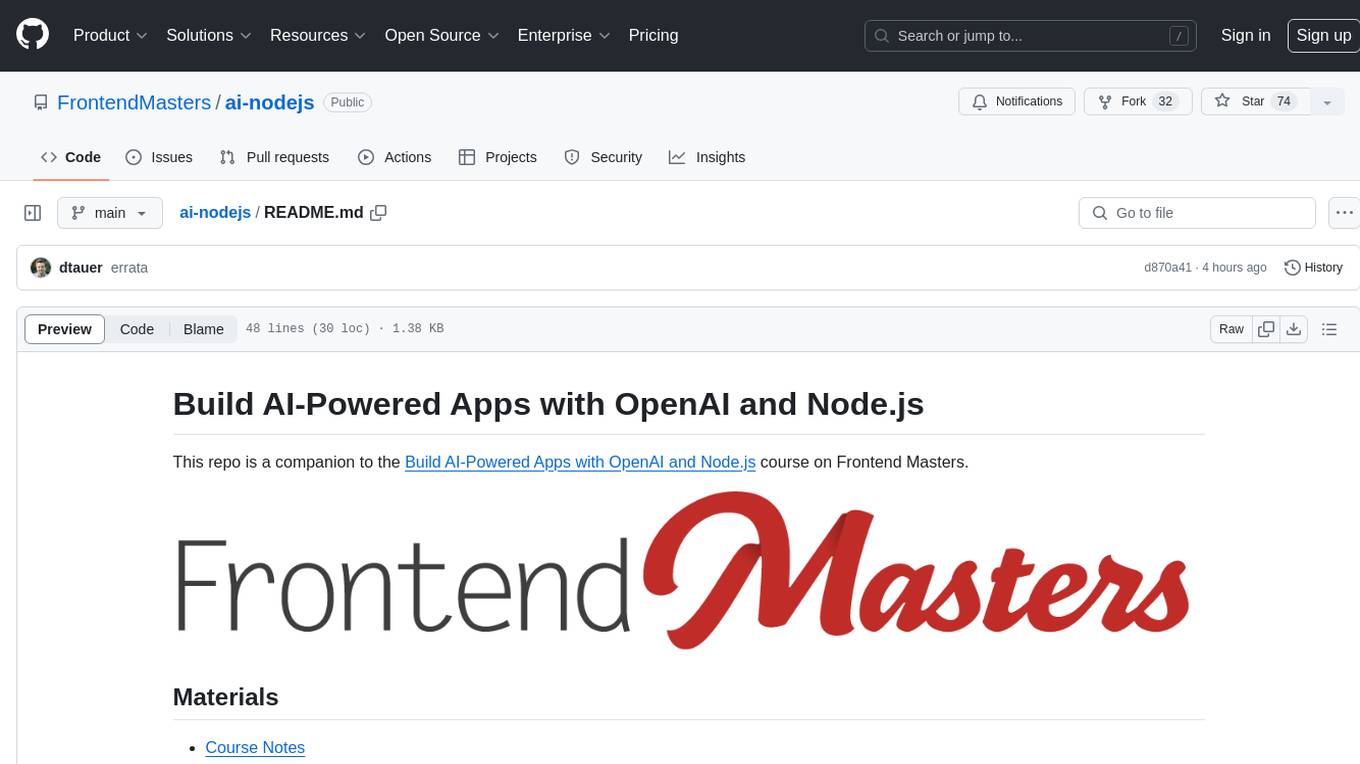
ai-nodejs
This repository serves as a companion to the Build AI-Powered Apps with OpenAI and Node.js course on Frontend Masters. It includes course notes and provides alternative approaches for deprecated Langchain methods by installing the Langchain community module and importing loaders for document processing from PDFs and YouTube videos.
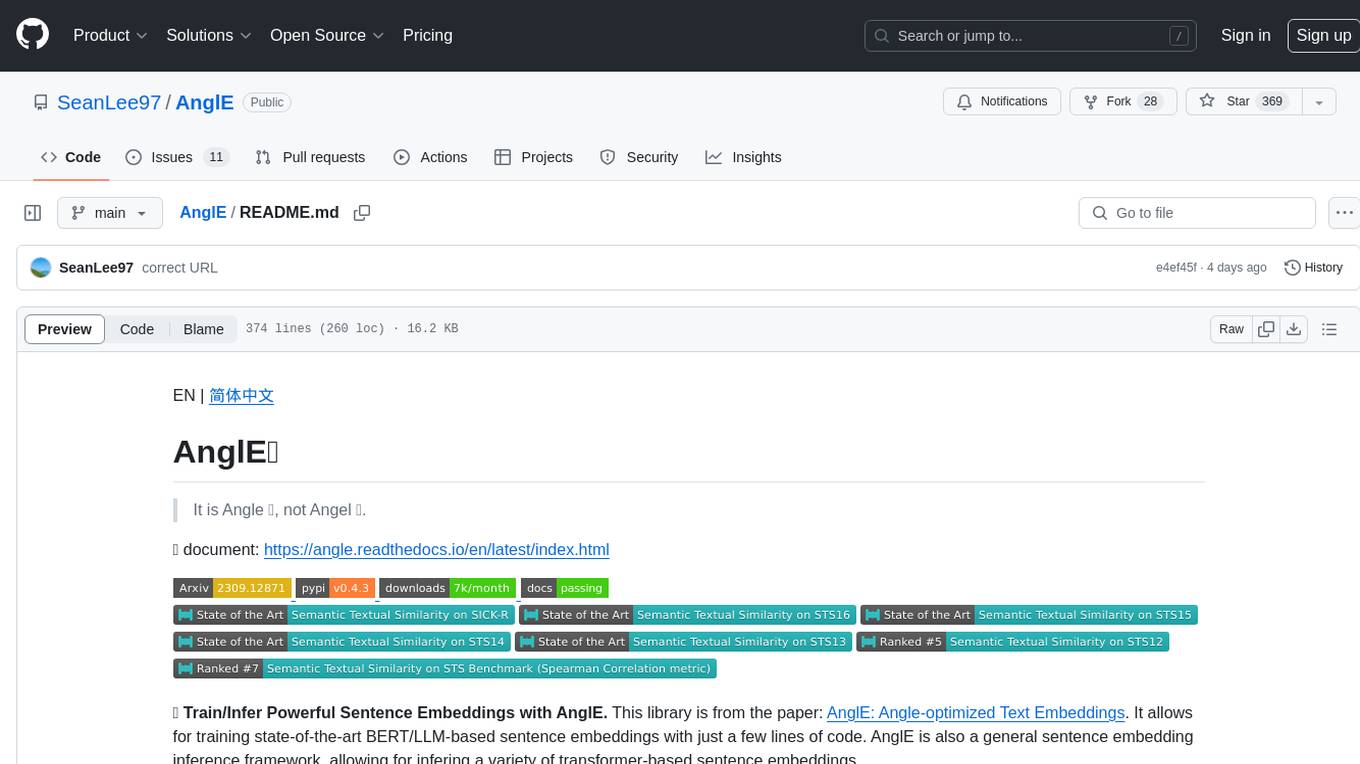
AnglE
AnglE is a library for training state-of-the-art BERT/LLM-based sentence embeddings with just a few lines of code. It also serves as a general sentence embedding inference framework, allowing for inferring a variety of transformer-based sentence embeddings. The library supports various loss functions such as AnglE loss, Contrastive loss, CoSENT loss, and Espresso loss. It provides backbones like BERT-based models, LLM-based models, and Bi-directional LLM-based models for training on single or multi-GPU setups. AnglE has achieved significant performance on various benchmarks and offers official pretrained models for both BERT-based and LLM-based models.
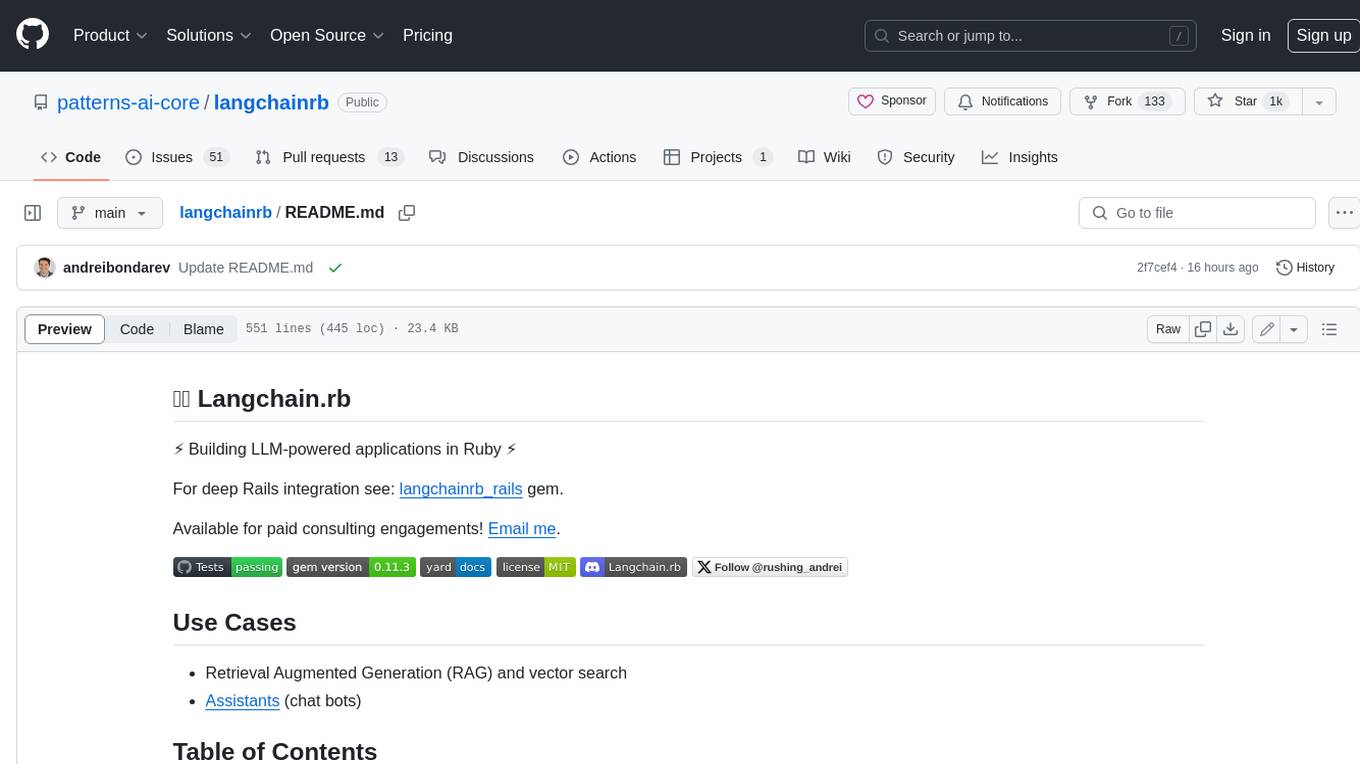
langchainrb
Langchain.rb is a Ruby library that makes it easy to build LLM-powered applications. It provides a unified interface to a variety of LLMs, vector search databases, and other tools, making it easy to build and deploy RAG (Retrieval Augmented Generation) systems and assistants. Langchain.rb is open source and available under the MIT License.
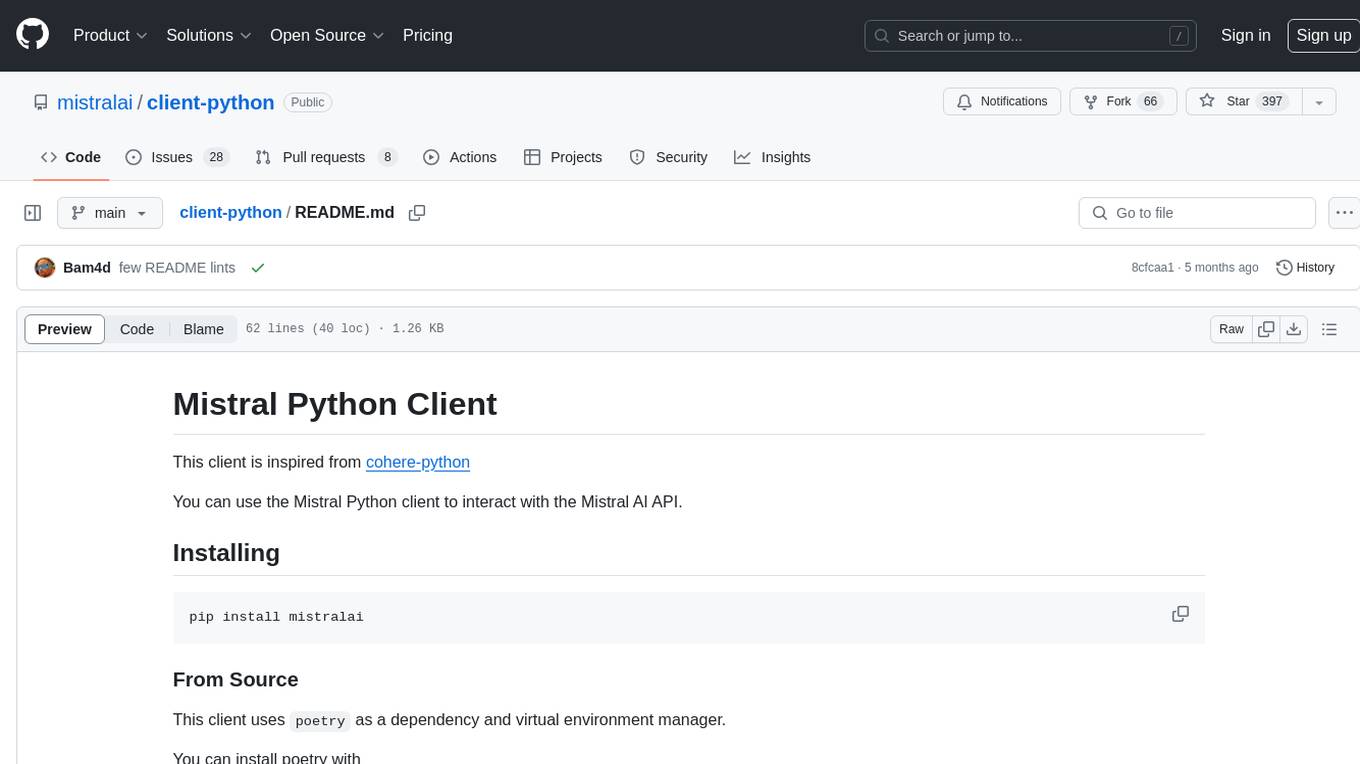
client-python
The Mistral Python Client is a tool inspired by cohere-python that allows users to interact with the Mistral AI API. It provides functionalities to access and utilize the AI capabilities offered by Mistral. Users can easily install the client using pip and manage dependencies using poetry. The client includes examples demonstrating how to use the API for various tasks, such as chat interactions. To get started, users need to obtain a Mistral API Key and set it as an environment variable. Overall, the Mistral Python Client simplifies the integration of Mistral AI services into Python applications.
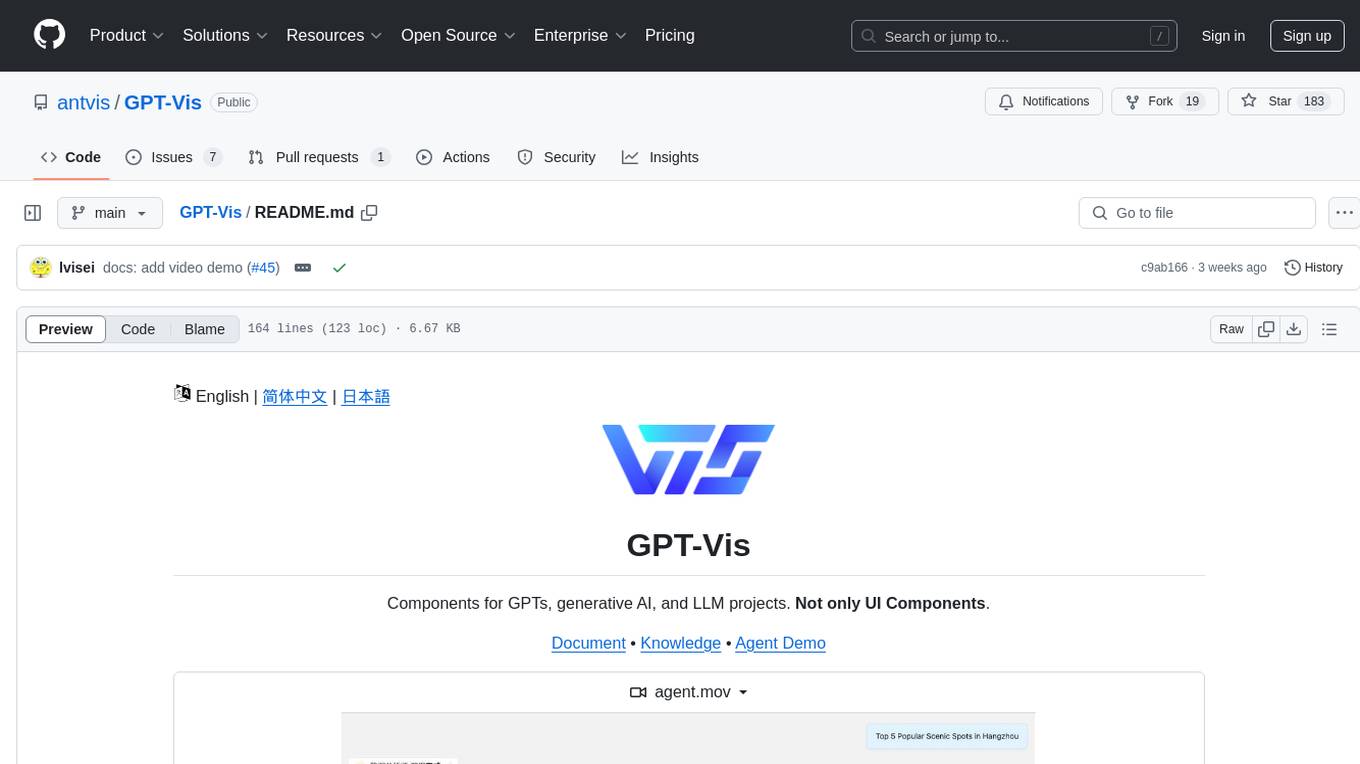
GPT-Vis
GPT-Vis is a tool designed for GPTs, generative AI, and LLM projects. It provides components such as LLM Protocol for conversational interaction, LLM Component for application development, and LLM access for knowledge base and model solutions. The tool aims to facilitate rapid integration into AI applications by offering a visual protocol, built-in components, and chart recommendations for LLM.
For similar tasks
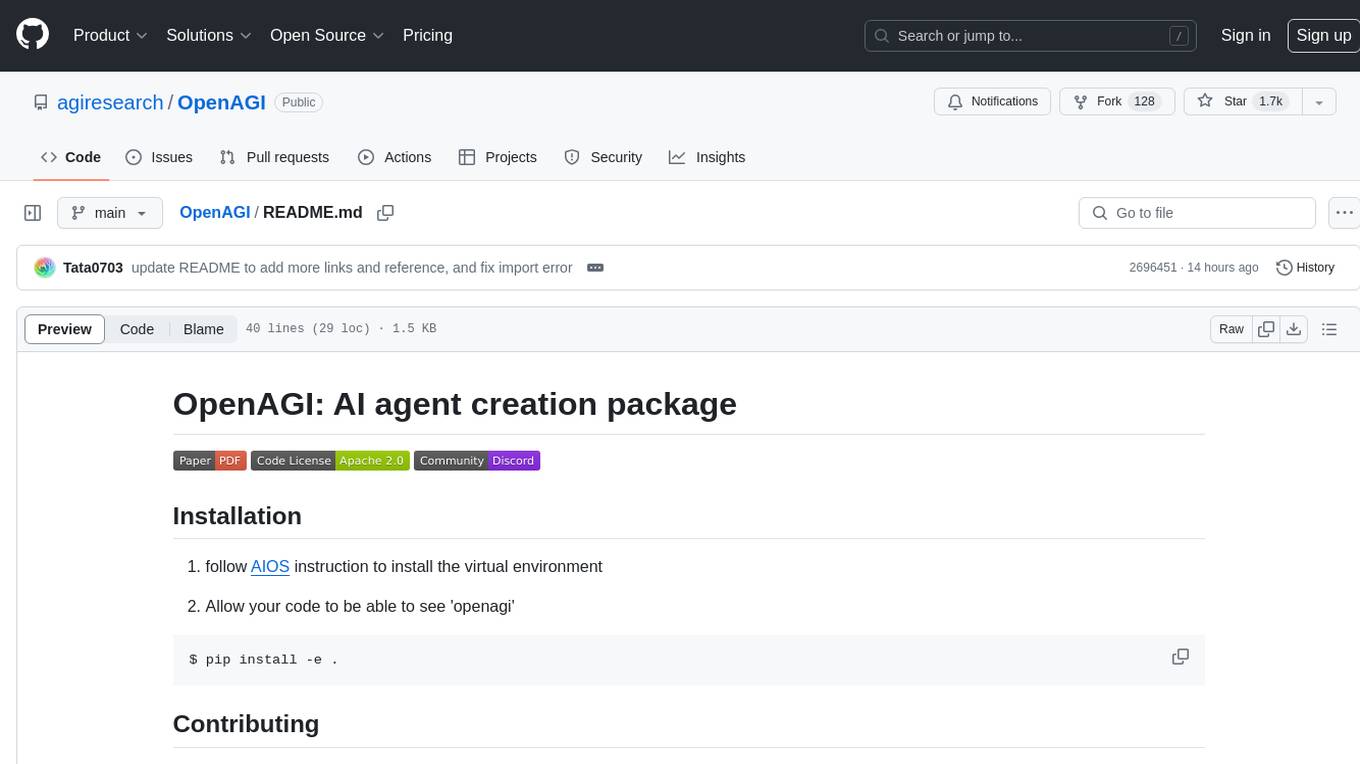
OpenAGI
OpenAGI is an AI agent creation package designed for researchers and developers to create intelligent agents using advanced machine learning techniques. The package provides tools and resources for building and training AI models, enabling users to develop sophisticated AI applications. With a focus on collaboration and community engagement, OpenAGI aims to facilitate the integration of AI technologies into various domains, fostering innovation and knowledge sharing among experts and enthusiasts.
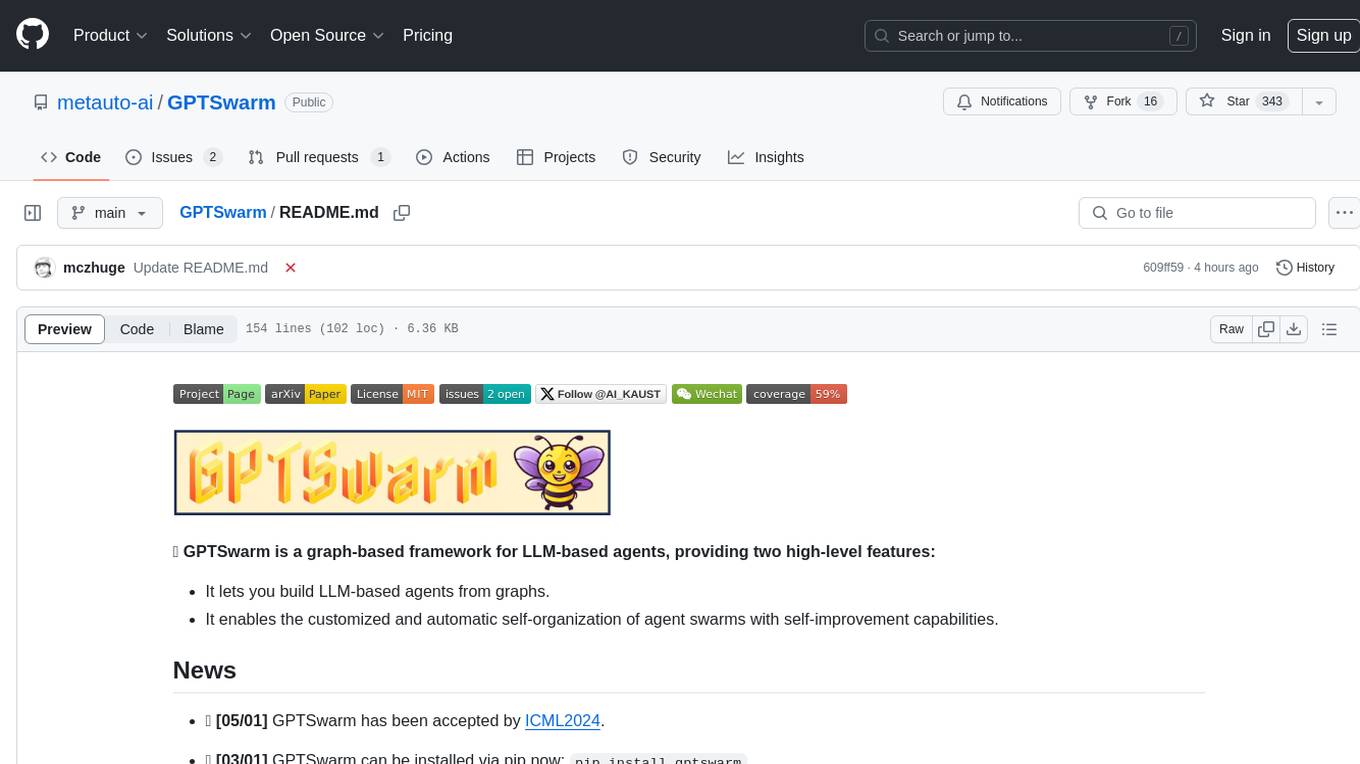
GPTSwarm
GPTSwarm is a graph-based framework for LLM-based agents that enables the creation of LLM-based agents from graphs and facilitates the customized and automatic self-organization of agent swarms with self-improvement capabilities. The library includes components for domain-specific operations, graph-related functions, LLM backend selection, memory management, and optimization algorithms to enhance agent performance and swarm efficiency. Users can quickly run predefined swarms or utilize tools like the file analyzer. GPTSwarm supports local LM inference via LM Studio, allowing users to run with a local LLM model. The framework has been accepted by ICML2024 and offers advanced features for experimentation and customization.
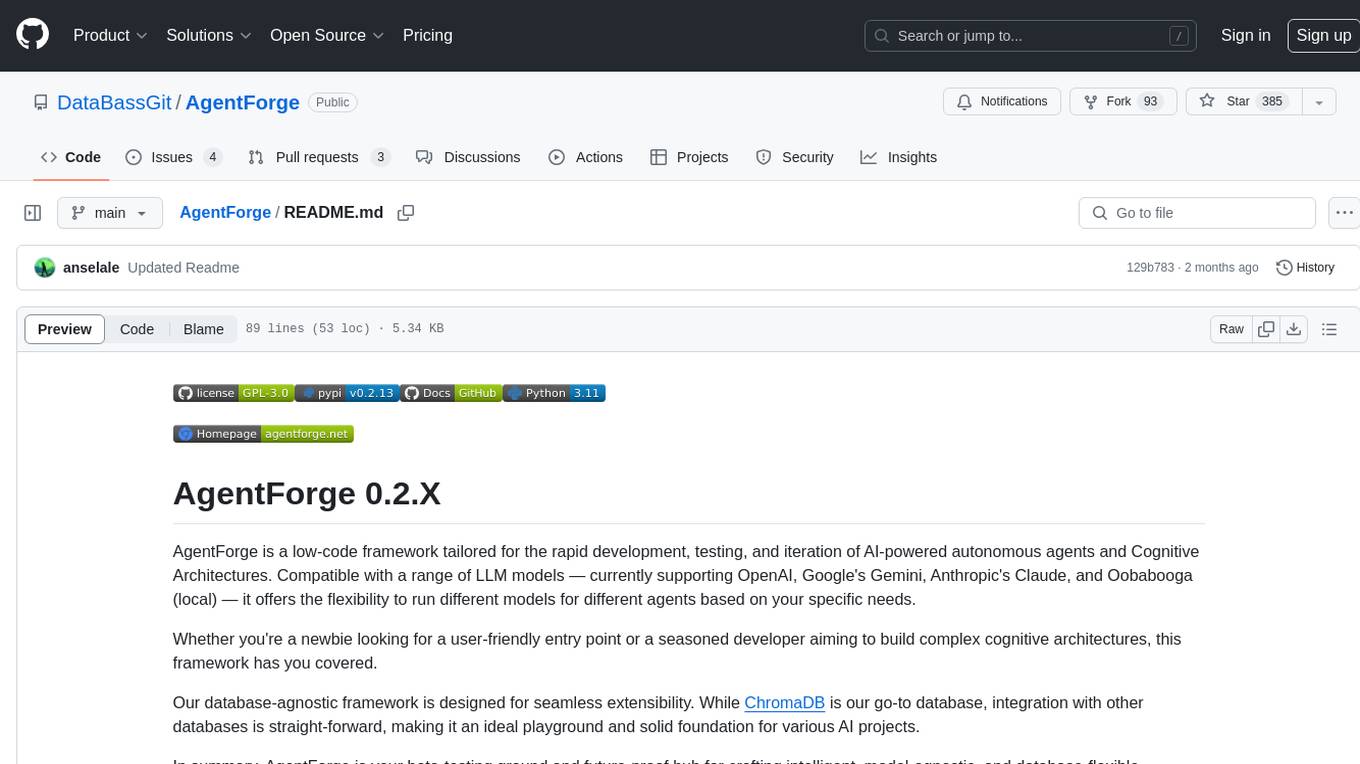
AgentForge
AgentForge is a low-code framework tailored for the rapid development, testing, and iteration of AI-powered autonomous agents and Cognitive Architectures. It is compatible with a range of LLM models and offers flexibility to run different models for different agents based on specific needs. The framework is designed for seamless extensibility and database-flexibility, making it an ideal playground for various AI projects. AgentForge is a beta-testing ground and future-proof hub for crafting intelligent, model-agnostic autonomous agents.
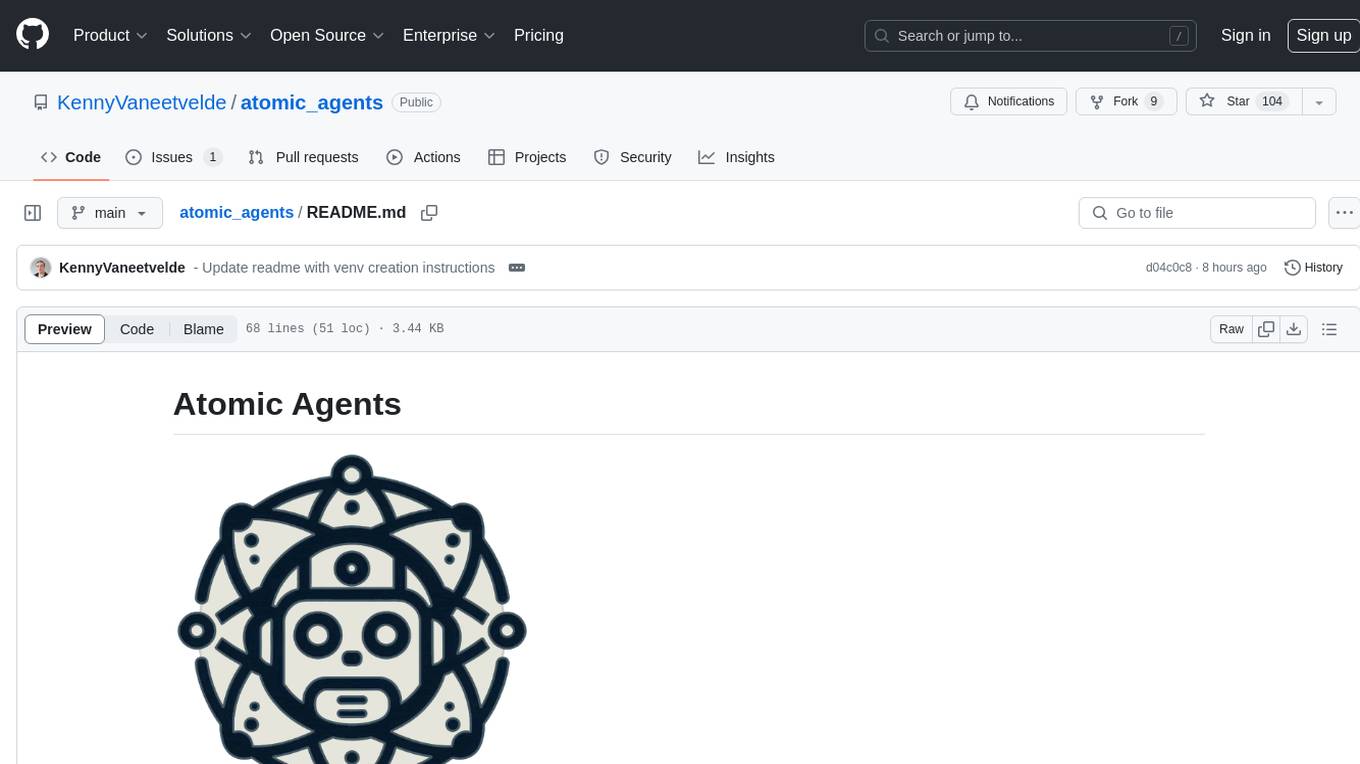
atomic_agents
Atomic Agents is a modular and extensible framework designed for creating powerful applications. It follows the principles of Atomic Design, emphasizing small and single-purpose components. Leveraging Pydantic for data validation and serialization, the framework offers a set of tools and agents that can be combined to build AI applications. It depends on the Instructor package and supports various APIs like OpenAI, Cohere, Anthropic, and Gemini. Atomic Agents is suitable for developers looking to create AI agents with a focus on modularity and flexibility.
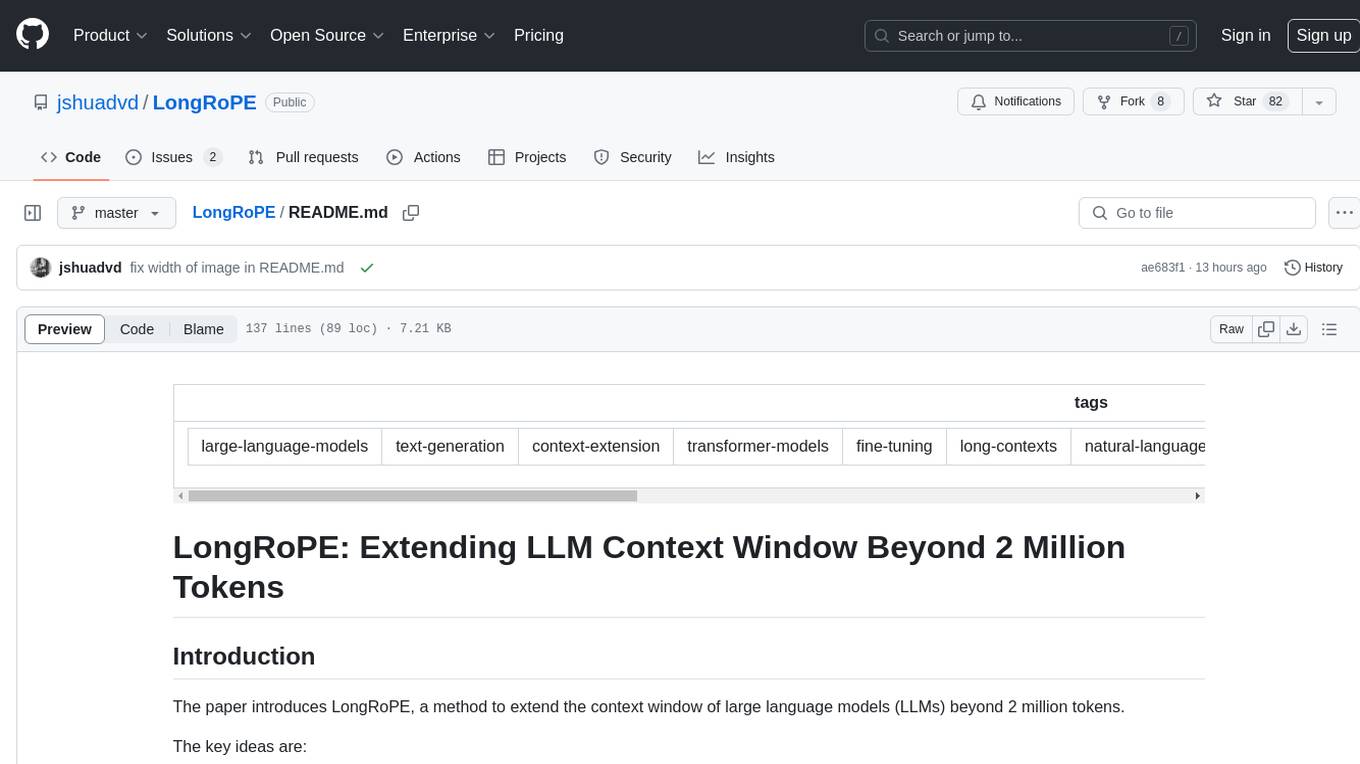
LongRoPE
LongRoPE is a method to extend the context window of large language models (LLMs) beyond 2 million tokens. It identifies and exploits non-uniformities in positional embeddings to enable 8x context extension without fine-tuning. The method utilizes a progressive extension strategy with 256k fine-tuning to reach a 2048k context. It adjusts embeddings for shorter contexts to maintain performance within the original window size. LongRoPE has been shown to be effective in maintaining performance across various tasks from 4k to 2048k context lengths.
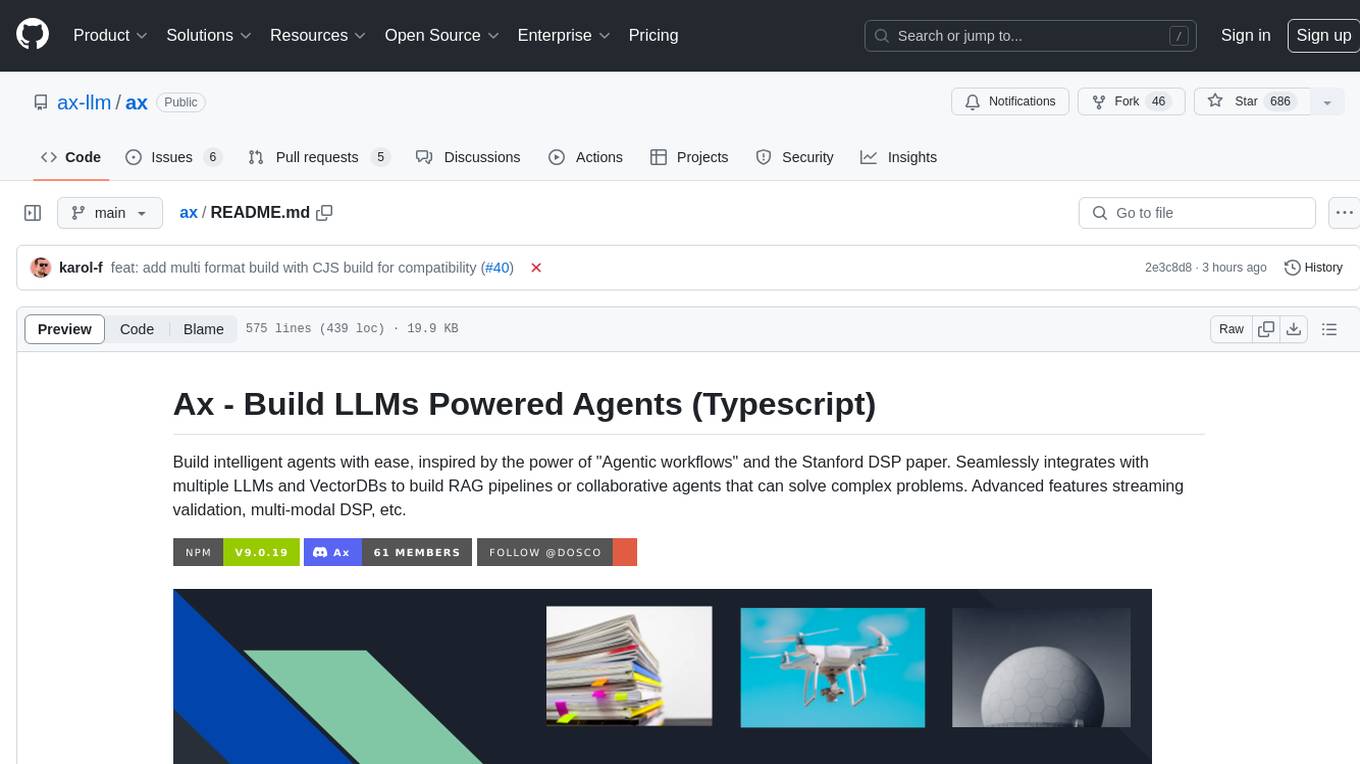
ax
Ax is a Typescript library that allows users to build intelligent agents inspired by agentic workflows and the Stanford DSP paper. It seamlessly integrates with multiple Large Language Models (LLMs) and VectorDBs to create RAG pipelines or collaborative agents capable of solving complex problems. The library offers advanced features such as streaming validation, multi-modal DSP, and automatic prompt tuning using optimizers. Users can easily convert documents of any format to text, perform smart chunking, embedding, and querying, and ensure output validation while streaming. Ax is production-ready, written in Typescript, and has zero dependencies.
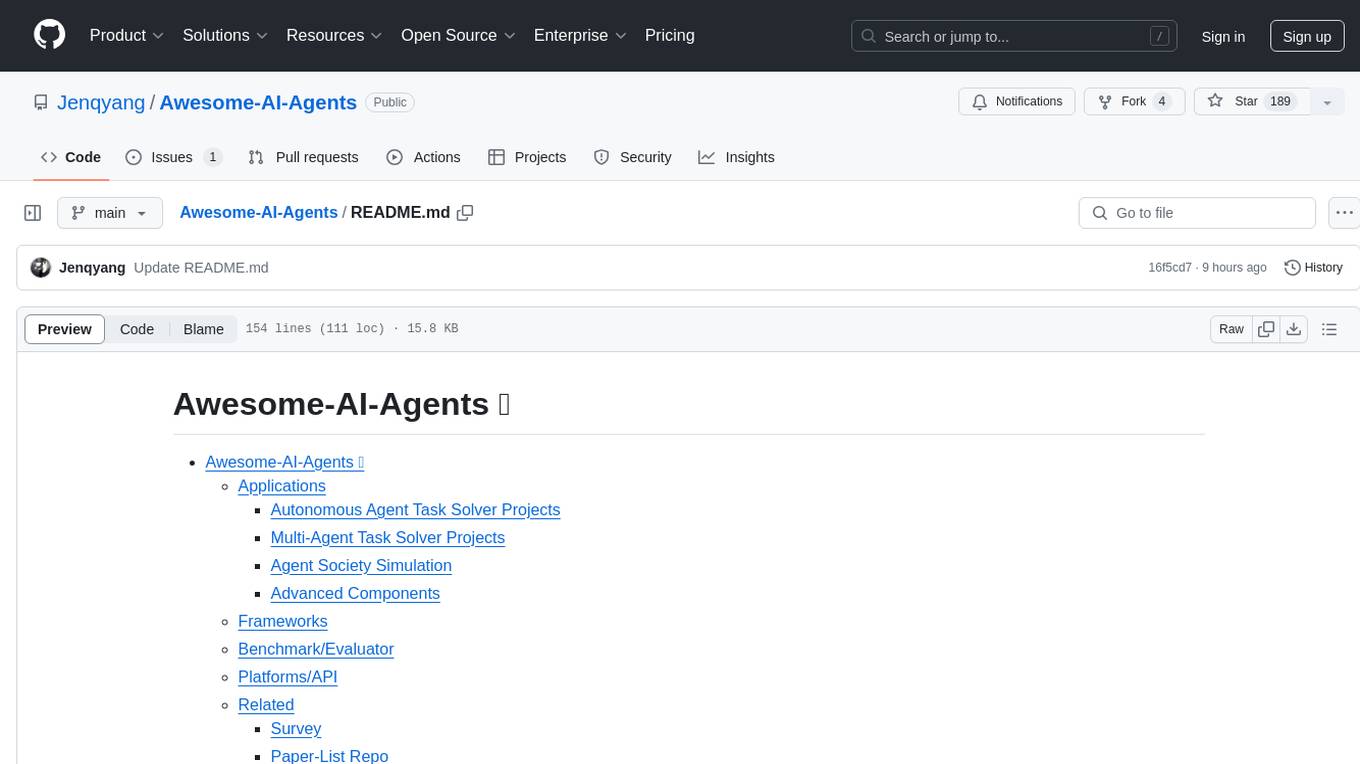
Awesome-AI-Agents
Awesome-AI-Agents is a curated list of projects, frameworks, benchmarks, platforms, and related resources focused on autonomous AI agents powered by Large Language Models (LLMs). The repository showcases a wide range of applications, multi-agent task solver projects, agent society simulations, and advanced components for building and customizing AI agents. It also includes frameworks for orchestrating role-playing, evaluating LLM-as-Agent performance, and connecting LLMs with real-world applications through platforms and APIs. Additionally, the repository features surveys, paper lists, and blogs related to LLM-based autonomous agents, making it a valuable resource for researchers, developers, and enthusiasts in the field of AI.
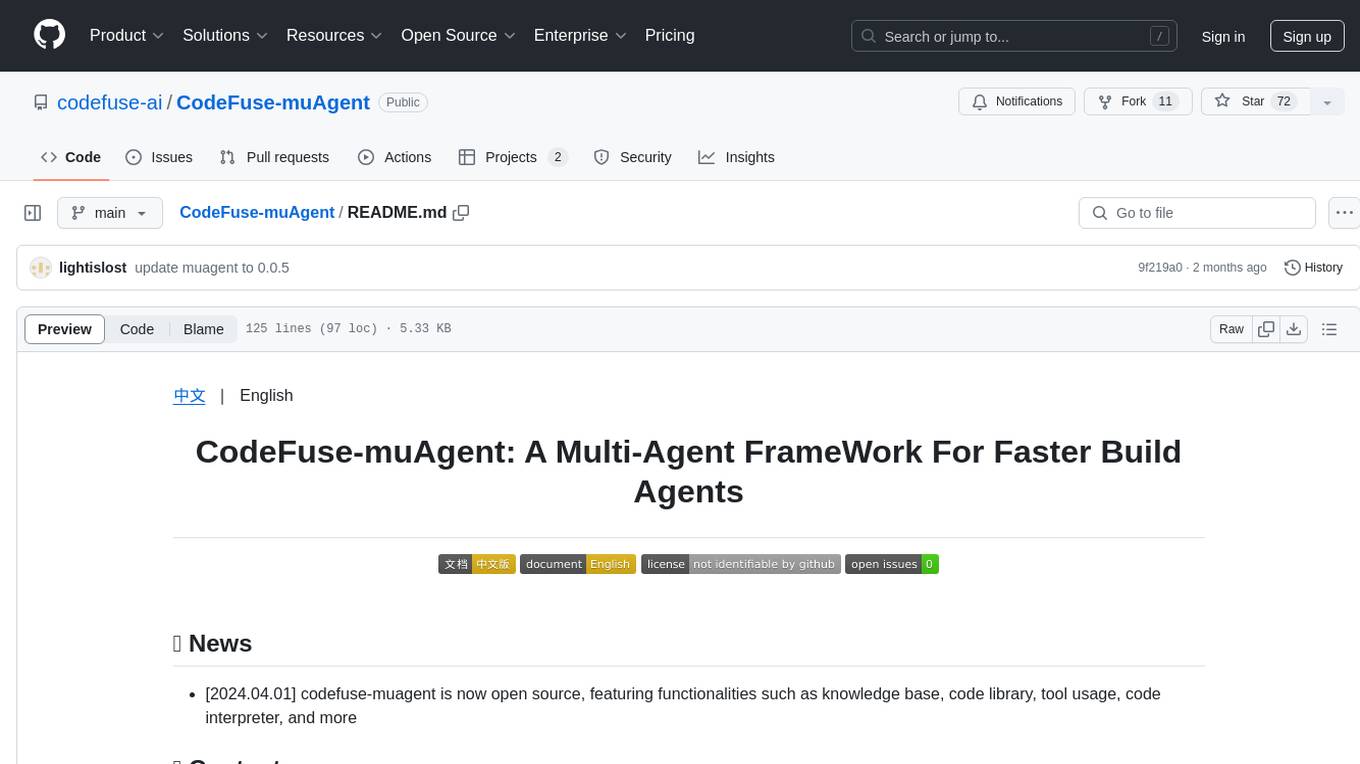
CodeFuse-muAgent
CodeFuse-muAgent is a Multi-Agent framework designed to streamline Standard Operating Procedure (SOP) orchestration for agents. It integrates toolkits, code libraries, knowledge bases, and sandbox environments for rapid construction of complex Multi-Agent interactive applications. The framework enables efficient execution and handling of multi-layered and multi-dimensional tasks.
For similar jobs
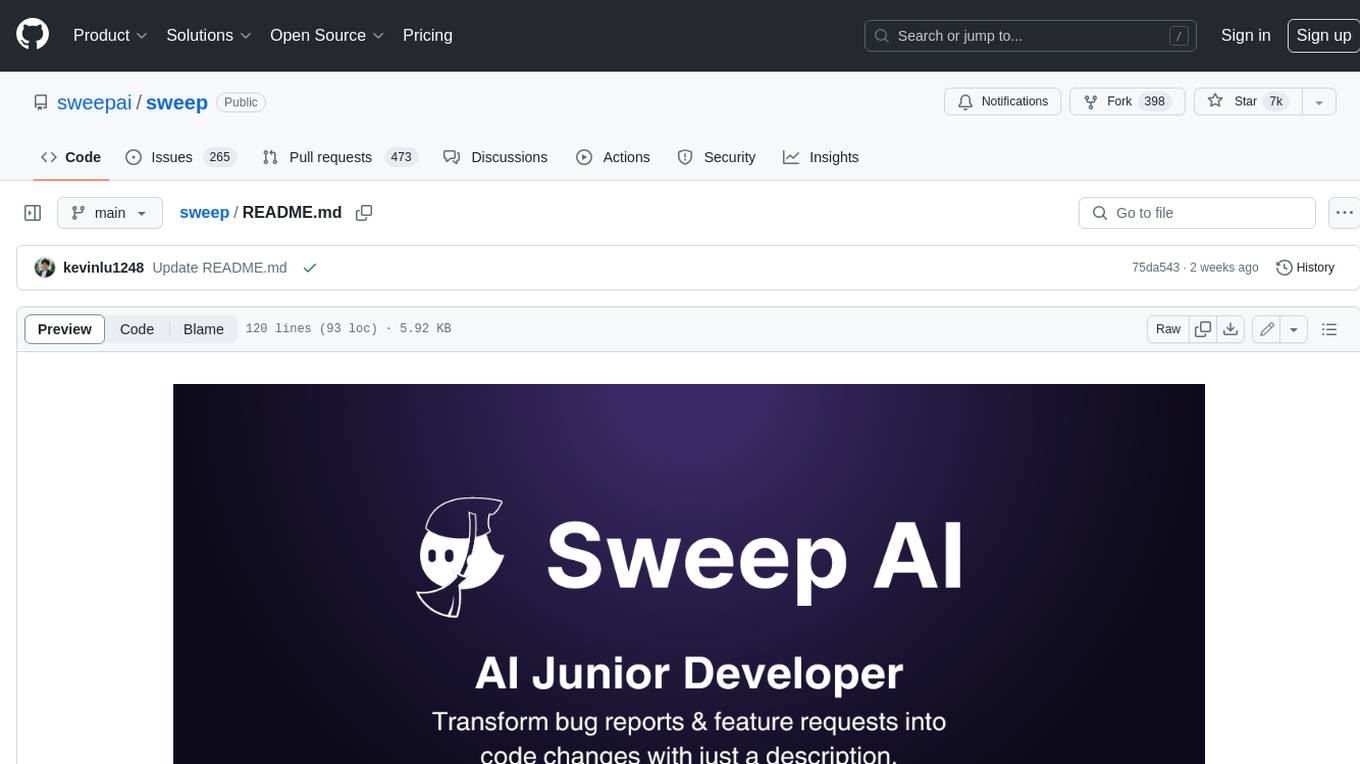
sweep
Sweep is an AI junior developer that turns bugs and feature requests into code changes. It automatically handles developer experience improvements like adding type hints and improving test coverage.
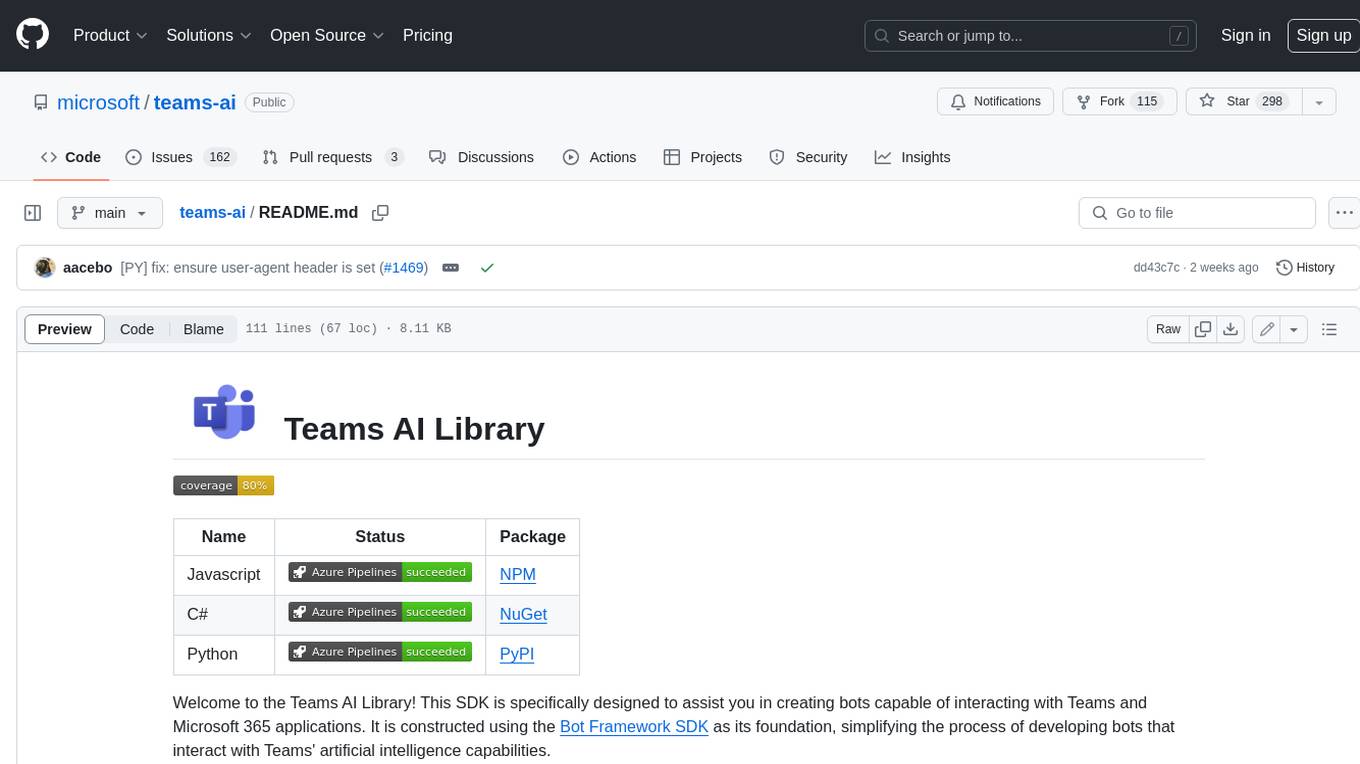
teams-ai
The Teams AI Library is a software development kit (SDK) that helps developers create bots that can interact with Teams and Microsoft 365 applications. It is built on top of the Bot Framework SDK and simplifies the process of developing bots that interact with Teams' artificial intelligence capabilities. The SDK is available for JavaScript/TypeScript, .NET, and Python.
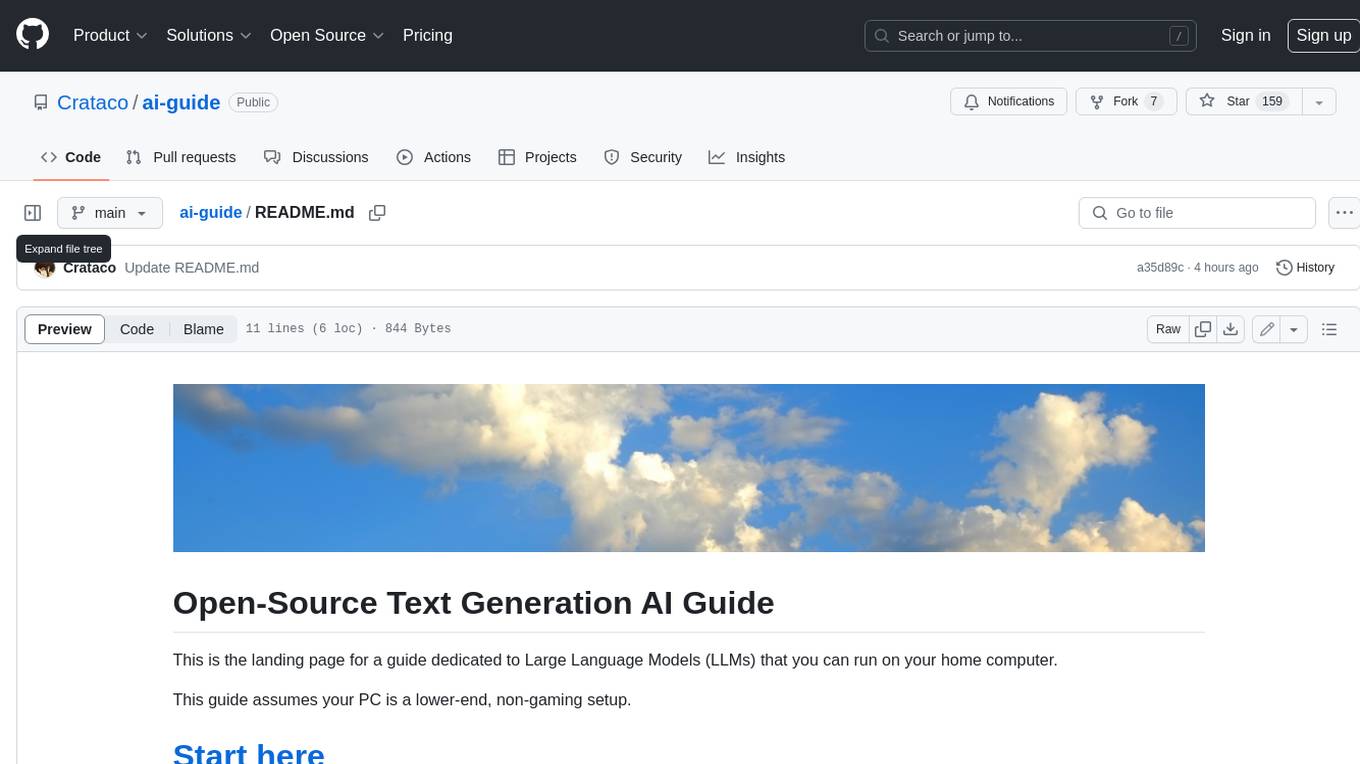
ai-guide
This guide is dedicated to Large Language Models (LLMs) that you can run on your home computer. It assumes your PC is a lower-end, non-gaming setup.
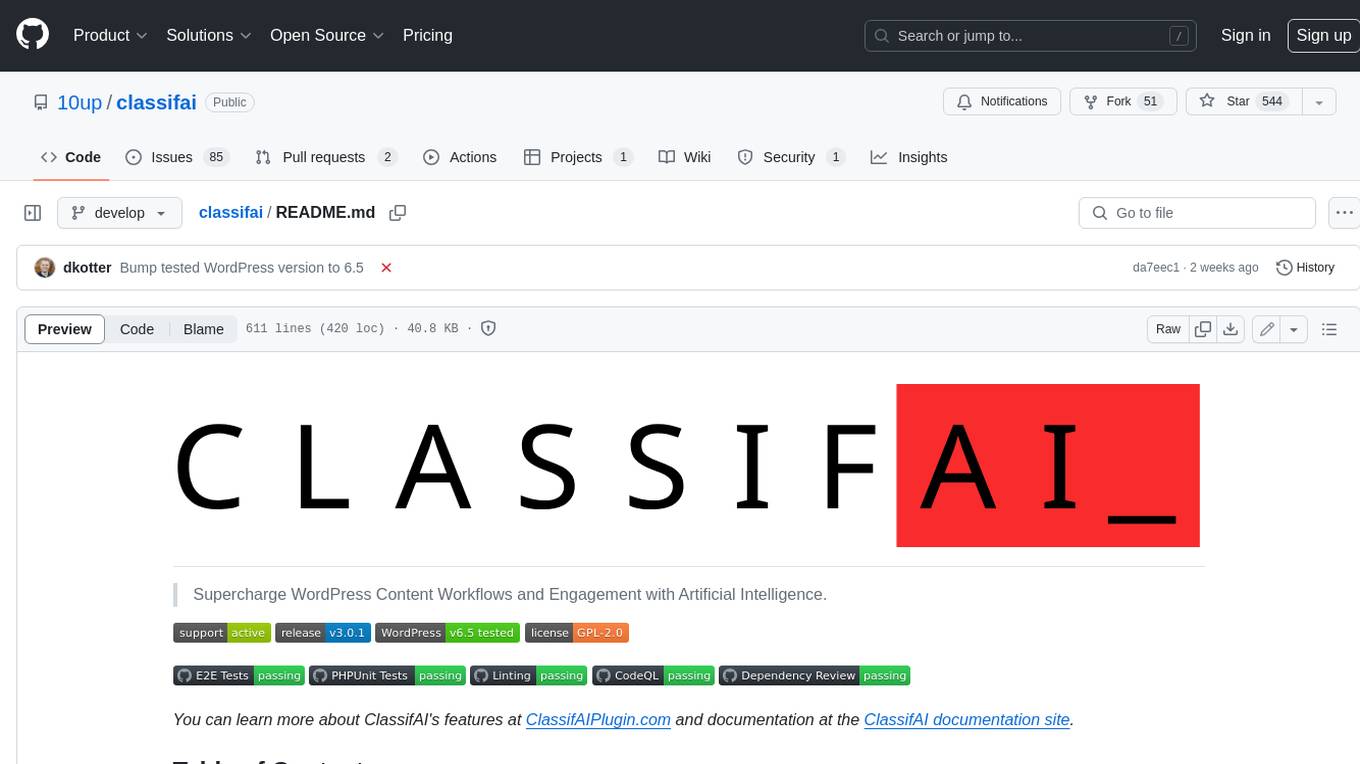
classifai
Supercharge WordPress Content Workflows and Engagement with Artificial Intelligence. Tap into leading cloud-based services like OpenAI, Microsoft Azure AI, Google Gemini and IBM Watson to augment your WordPress-powered websites. Publish content faster while improving SEO performance and increasing audience engagement. ClassifAI integrates Artificial Intelligence and Machine Learning technologies to lighten your workload and eliminate tedious tasks, giving you more time to create original content that matters.
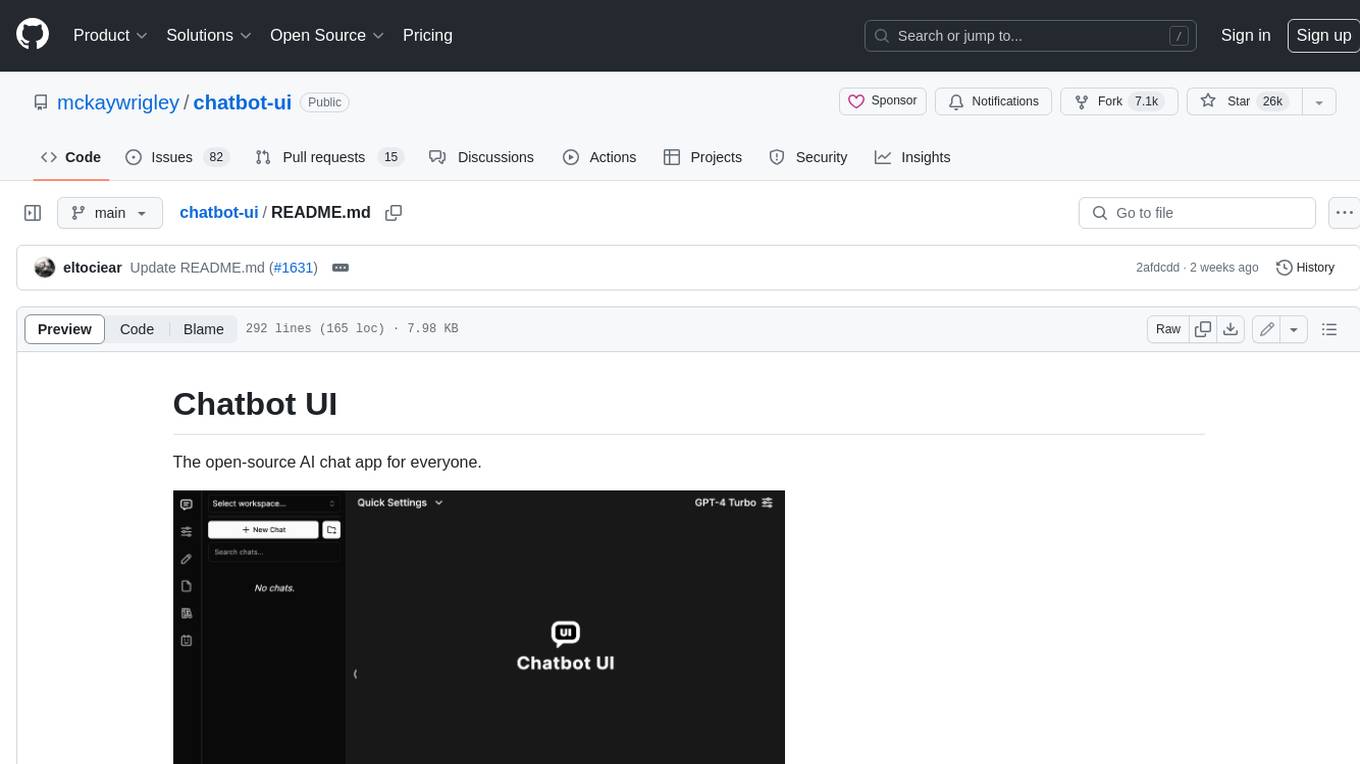
chatbot-ui
Chatbot UI is an open-source AI chat app that allows users to create and deploy their own AI chatbots. It is easy to use and can be customized to fit any need. Chatbot UI is perfect for businesses, developers, and anyone who wants to create a chatbot.
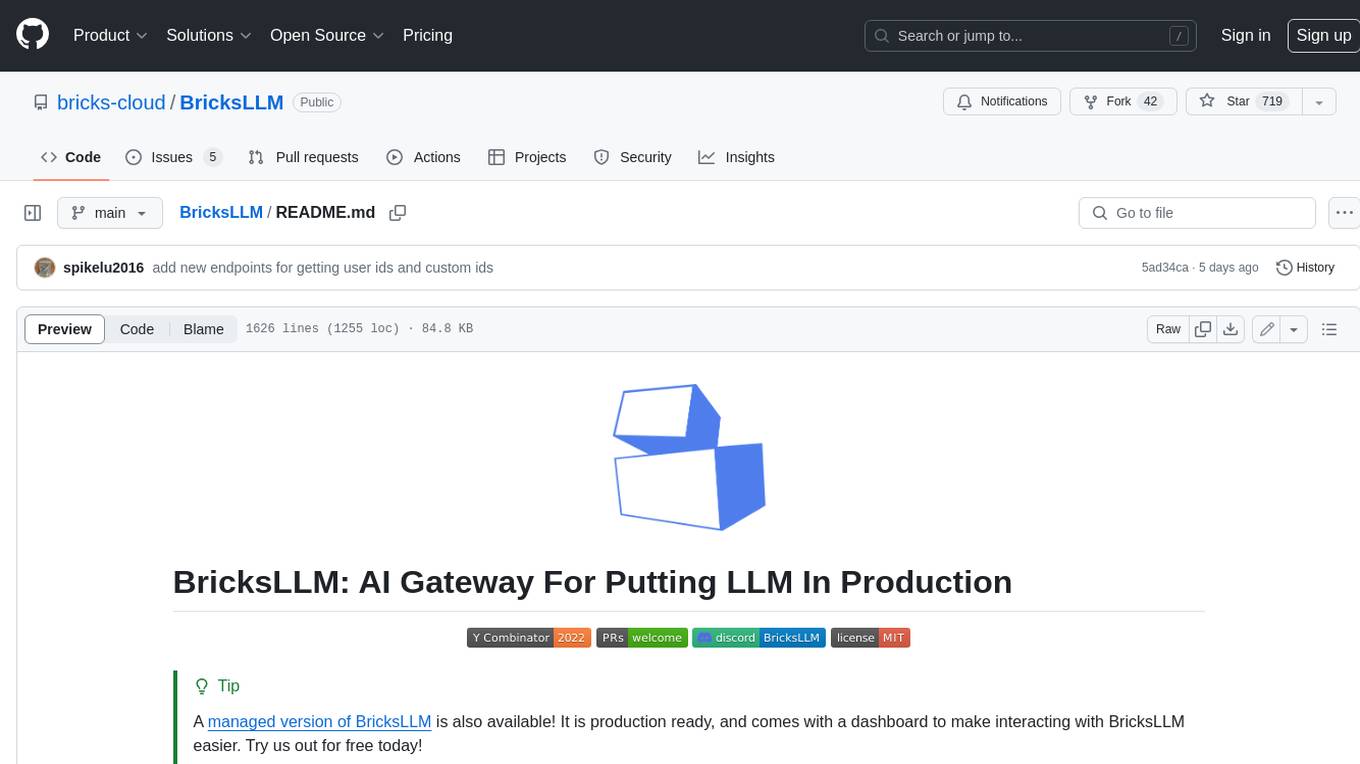
BricksLLM
BricksLLM is a cloud native AI gateway written in Go. Currently, it provides native support for OpenAI, Anthropic, Azure OpenAI and vLLM. BricksLLM aims to provide enterprise level infrastructure that can power any LLM production use cases. Here are some use cases for BricksLLM: * Set LLM usage limits for users on different pricing tiers * Track LLM usage on a per user and per organization basis * Block or redact requests containing PIIs * Improve LLM reliability with failovers, retries and caching * Distribute API keys with rate limits and cost limits for internal development/production use cases * Distribute API keys with rate limits and cost limits for students
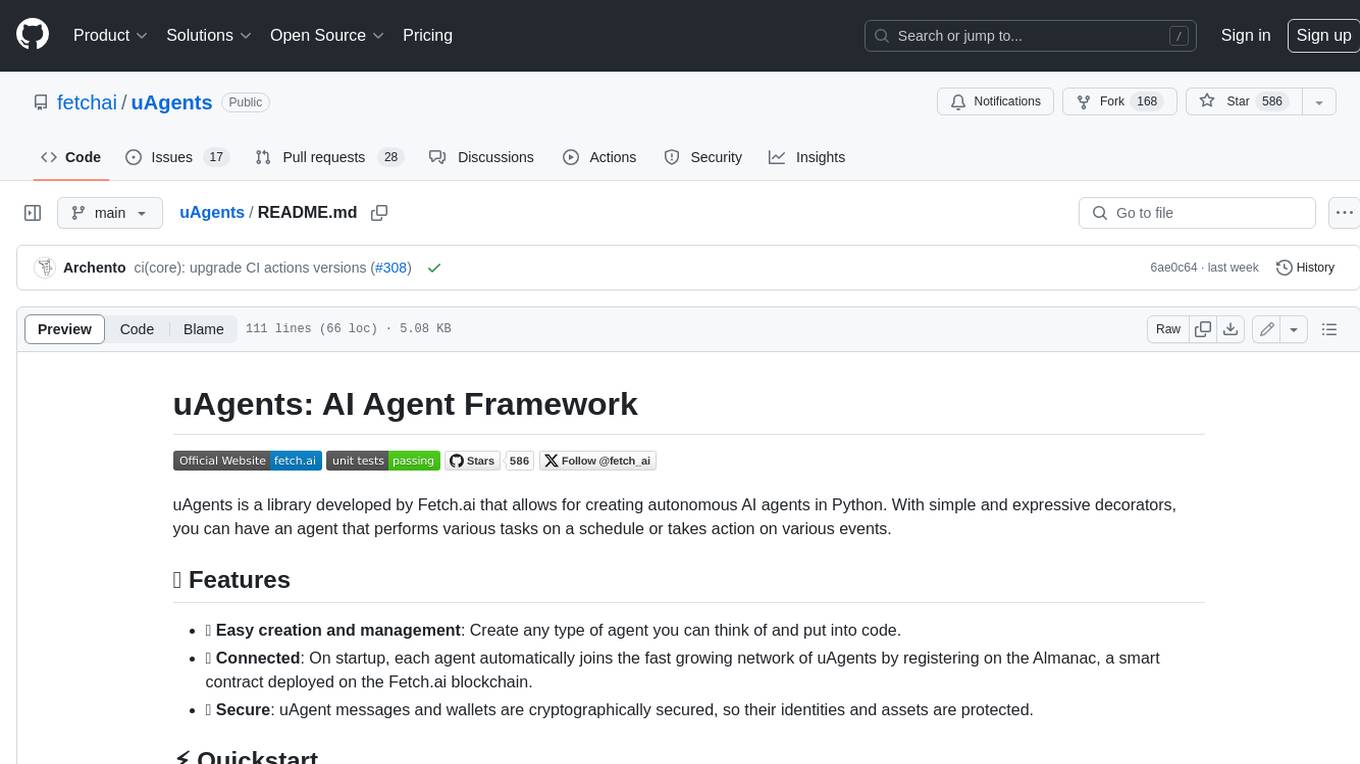
uAgents
uAgents is a Python library developed by Fetch.ai that allows for the creation of autonomous AI agents. These agents can perform various tasks on a schedule or take action on various events. uAgents are easy to create and manage, and they are connected to a fast-growing network of other uAgents. They are also secure, with cryptographically secured messages and wallets.
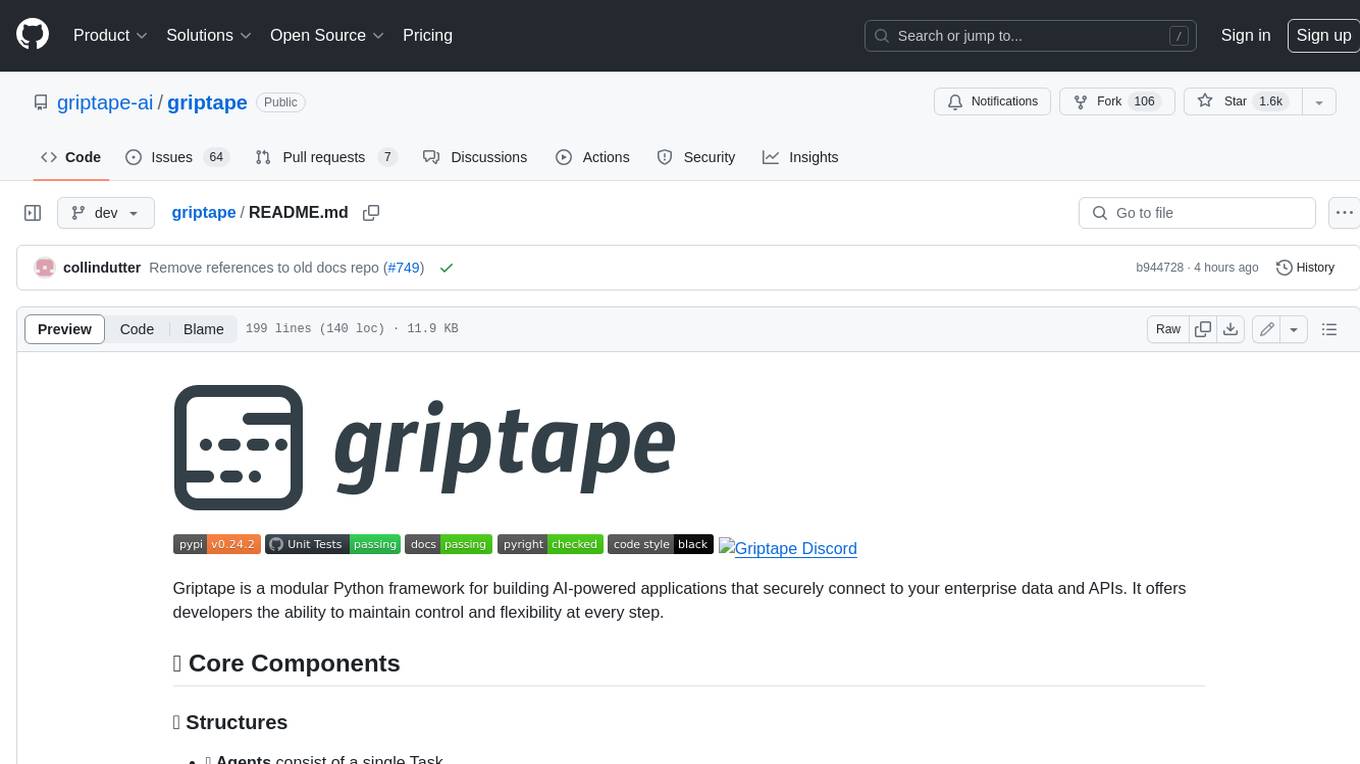
griptape
Griptape is a modular Python framework for building AI-powered applications that securely connect to your enterprise data and APIs. It offers developers the ability to maintain control and flexibility at every step. Griptape's core components include Structures (Agents, Pipelines, and Workflows), Tasks, Tools, Memory (Conversation Memory, Task Memory, and Meta Memory), Drivers (Prompt and Embedding Drivers, Vector Store Drivers, Image Generation Drivers, Image Query Drivers, SQL Drivers, Web Scraper Drivers, and Conversation Memory Drivers), Engines (Query Engines, Extraction Engines, Summary Engines, Image Generation Engines, and Image Query Engines), and additional components (Rulesets, Loaders, Artifacts, Chunkers, and Tokenizers). Griptape enables developers to create AI-powered applications with ease and efficiency.