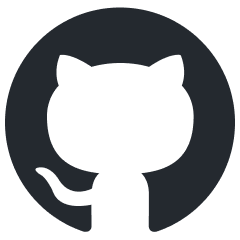
receipt-scanner
🧾✨ AI-Powered Receipt and Invoice Scanner for Laravel, with support for images, documents and text
Stars: 95
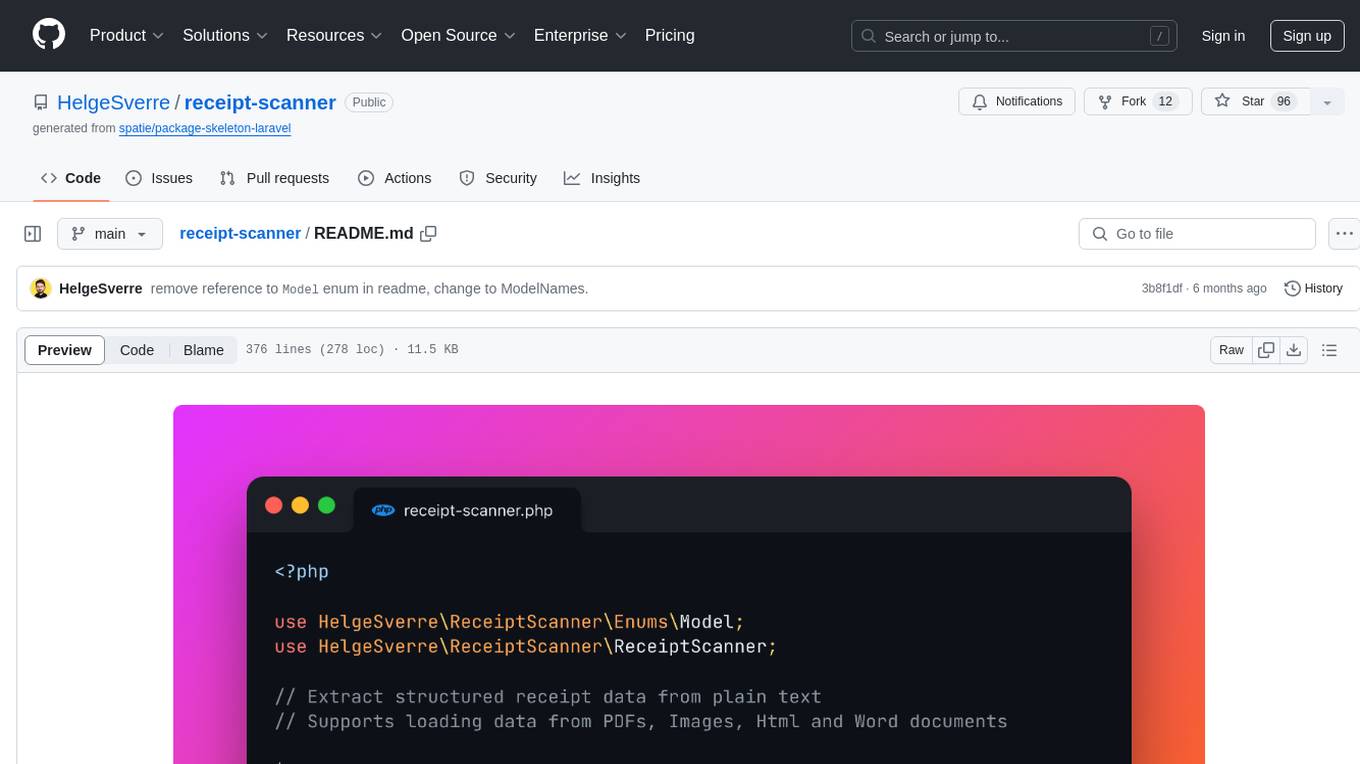
The receipt-scanner repository is an AI-Powered Receipt and Invoice Scanner for Laravel that allows users to easily extract structured receipt data from images, PDFs, and emails within their Laravel application using OpenAI. It provides a light wrapper around OpenAI Chat and Completion endpoints, supports various input formats, and integrates with Textract for OCR functionality. Users can install the package via composer, publish configuration files, and use it to extract data from plain text, PDFs, images, Word documents, and web content. The scanned receipt data is parsed into a DTO structure with main classes like Receipt, Merchant, and LineItem.
README:
Need more flexibility? Try the Extractor package instead, a AI-Powered data extraction library for Laravel
Easily extract structured receipt data from images, PDFs, and emails within your Laravel application using OpenAI.
- Light wrapper around OpenAI Chat and Completion endpoints.
- Accepts text as input and returns structured receipt information.
- Includes a well-tuned prompt for parsing receipts.
- Supports various input formats including Plain Text, PDF, Images, Word documents, and Web content.
- Integrates with Textract for OCR functionality.
Install the package via composer:
composer require helgesverre/receipt-scanner
Publish the config file:
php artisan vendor:publish --tag="receipt-scanner-config"
All the configuration options are documented in the configuration file.
Since this package uses the OpenAI Laravel Package, so you also need to publish
their config and add the OPENAI_API_KEY
to your .env
file:
php artisan vendor:publish --provider="OpenAI\Laravel\ServiceProvider"
OPENAI_API_KEY="your-key-here
Plain text scanning is useful when you already have the textual representation of a receipt or invoice.
The example is from a Paddle.com receipt email, where I copied all the text in the email, and removed all the empty lines.
$text = <<<RECEIPT
Liseth Solutions AS
via software reseller Paddle.com
Thank you for your purchase!
Your full invoice is attached to this email.
Amount paid
Payment method
NOK 2,498.75
visa
ending in 4242
Test: SaaS Subscription - Pro Plan
September 22, 2023 11:04 am UTC - October 22, 2023 11:04 am UTC
NOK 1,999.00
QTY: 1
Subtotal
NOK 1,999.00
VAT
NOK 499.75
Amount paid*
NOK 2,498.75
*This payment will appear on your statement as: PADDLE.NET* EXAMPLEINC
NEED HELP?
Need help with your purchase? Please contact us on paddle.net.
logo
Paddle.com Market Ltd, Judd House, 18-29 Mora Street, London EC1V 8BT
© 2023 Paddle. All rights reserved.
RECEIPT;
ReceiptScanner::scan($text);
use HelgeSverre\ReceiptScanner\Facades\Text;
$textPlainText = Text::text(file_get_contents('./receipt.txt'));
$textPdf = Text::pdf(file_get_contents('./receipt.pdf'));
$textImageOcr = Text::textract(file_get_contents('./receipt.jpg'));
$textPdfOcr = Text::textractUsingS3Upload(file_get_contents('./receipt.pdf'));
$textWord = Text::word(file_get_contents('./receipt.doc'));
$textWeb = Text::web('https://example.com');
$textHtml = Text::html(file_get_contents('./receipt.html'));
After loading, you can pass the TextContent
or the plain text (which can be retrieved by calling ->toString()
) into
the ReceiptScanner::scan()
method.
use HelgeSverre\ReceiptScanner\Facades\ReceiptScanner;
ReceiptScanner::scan($textPlainText)
ReceiptScanner::scan($textPdf)
ReceiptScanner::scan($textImageOcr)
ReceiptScanner::scan($textPdfOcr)
ReceiptScanner::scan($textWord)
ReceiptScanner::scan($textWeb)
ReceiptScanner::scan($textHtml)
The scanned receipt is parsed into a DTO which consists of a main Receipt
class, which contains the receipt metadata,
and a Merchant
dto, representing the seller on the receipt or invoice, and an array of LineItem
DTOs holding each
individual line item.
HelgeSverre\ReceiptScanner\Data\Receipt
HelgeSverre\ReceiptScanner\Data\Merchant
HelgeSverre\ReceiptScanner\Data\LineItem
The DTO has a toArray()
method, which will result in a structure like this:
For flexibility, all fields are nullable.
[
"orderRef" => "string",
"date" => "date",
"taxAmount" => "number",
"totalAmount" => "number",
"currency" => "string",
"merchant" => [
"name" => "string",
"vatId" => "string",
"address" => "string",
],
"lineItems" => [
[
"text" => "string",
"sku" => "string",
"qty" => "number",
"price" => "number",
],
],
];
If you prefer to work with an array instead of the built-in DTO, you can specify asArray: true
when calling scan()
use HelgeSverre\ReceiptScanner\Facades\ReceiptScanner;
ReceiptScanner::scan(
$textPlainText
asArray: true
)
To use a different model, you can specify the model name to use with the model
named argument when calling
the scan()
method.
use HelgeSverre\ReceiptScanner\Facades\ReceiptScanner;
use HelgeSverre\ReceiptScanner\ModelNames;
// With the ModelNames class
ReceiptScanner::scan($content, model: ModelNames::GPT4_1106_PREVIEW)
// With a string
ReceiptScanner::scan($content, model: 'gpt-4-1106-preview')
$text
(TextContent|string)
The input text from the receipt or invoice that needs to be parsed. It accepts either a TextContent
object or a
string.
**$model
(string)
This parameter specifies the OpenAI model used for the extraction process.
HelgeSverre\ReceiptScanner\ModelNames
is a class containing constants for each model, provided for convenience.
However, you can also directly
use a string to specify the model if you prefer.
Different models have different speed/accuracy characteristics.
If you require high accuracy, use a GPT-4 model, if you need speed, use a GPT-3 model, if you need even more speed, use
the gpt-3.5-turbo-instruct
model.
The default model is ModelNames::TURBO_INSTRUCT
.
ModelNames Constant |
Value |
---|---|
ModelNames::TURBO |
gpt-3.5-turbo |
ModelNames::TURBO_INSTRUCT |
gpt-3.5-turbo-instruct |
ModelNames::TURBO_1106 |
gpt-3.5-turbo-1106 |
ModelNames::TURBO_16K |
gpt-3.5-turbo-16k |
ModelNames::TURBO_0613 |
gpt-3.5-turbo-0613 |
ModelNames::TURBO_16K_0613 |
gpt-3.5-turbo-16k-0613 |
ModelNames::TURBO_0301 |
gpt-3.5-turbo-0301 |
ModelNames::GPT4 |
gpt-4 |
ModelNames::GPT4_32K |
gpt-4-32k |
ModelNames::GPT4_32K_0613 |
gpt-4-32k-0613 |
ModelNames::GPT4_1106_PREVIEW |
gpt-4-1106-preview |
ModelNames::GPT4_0314 |
gpt-4-0314 |
ModelNames::GPT4_32K_0314 |
gpt-4-32k-0314 |
$maxTokens
(int)
The maximum number of tokens that the model will processes.
The default value is 2000
, adjusting this value may be necessary for very long text, but 2000 is "usually" fairly
good.
$temperature
(float)
Controls the randomness/creativity of the model's output.
A higher value (e.g., 0.8) makes the output more random, which is usually not what we want in this scenario, I usually
go with 0.1 or 0.2, anything over 0.5 becomes useless. Defaults to 0.1
.
$template
(string)
This parameter specifies the template used for the prompt.
The default template is 'receipt'
. You can create and use
additional templates by adding new blade files in the resources/views/vendor/receipt-scanner/
directory and specifying
the file name (without extension) as the $template
value (eg: "minimal_invoice"
.
$asArray
(bool)
If true, returns the response from the AI model as an array instead of as a DTO, useful if you need to modifythe default
DTO to have more/less fields or want to convert the response into your own DTO, defaults to false
use HelgeSverre\ReceiptScanner\Facades\ReceiptScanner;
$parsedReceipt = ReceiptScanner::scan(
text: $textInput,
model: ModelNames::TURBO_INSTRUCT,
maxTokens: 500,
temperature: 0.2,
template: 'minimal_invoice',
asArray: true,
);
Enum Value | Model name | Endpoint |
---|---|---|
TURBO_INSTRUCT | gpt-3.5-turbo-instruct | Completion |
TURBO_16K | gpt-3.5-turbo-16k | Chat |
TURBO | gpt-3.5-turbo | Chat |
GPT4 | gpt-4 | Chat |
GPT4_32K | gpt-4-32 | Chat |
To use AWS Textract for extracting text from large images and multi-page PDFs, the package needs to upload the file to S3 and pass the s3 object location along to the textract service.
So you need to configure your AWS Credentials in the config/receipt-scanner.php
file as follows:
TEXTRACT_KEY="your-aws-access-key"
TEXTRACT_SECRET="your-aws-security"
TEXTRACT_REGION="your-textract-region"
# Can be omitted
TEXTRACT_VERSION="2018-06-27"
You also need to configure a seperate Textract disk where the files will be stored,
open your config/filesystems.php
configuration file and add the following:
'textract' => [
'driver' => 's3',
'key' => env('TEXTRACT_KEY'),
'secret' => env('TEXTRACT_SECRET'),
'region' => env('TEXTRACT_REGION'),
'bucket' => env('TEXTRACT_BUCKET'),
],
Ensure the textract_disk
setting in config/receipt-scanner.php
is the same as your disk name in
the filesystems.php
config, you can change it with the .env value TEXTRACT_DISK
.
return [
"textract_disk" => env("TEXTRACT_DISK")
];
.env
TEXTRACT_DISK="uploads"
Note
Textract is not available in all regions:
Q: In which AWS regions is Amazon Textract available? Amazon Textract is currently available in the US East (Northern Virginia), US East (Ohio), US West (Oregon), US West ( N. California), AWS GovCloud (US-West), AWS GovCloud (US-East), Canada (Central), EU (Ireland), EU (London), EU ( Frankfurt), EU (Paris), Asia Pacific (Singapore), Asia Pacific (Sydney), Asia Pacific (Seoul), and Asia Pacific ( Mumbai) Regions.
See: https://aws.amazon.com/textract/faqs/
You may publish the prompt file that is used under the hood by running this command:
php artisan vendor:publish --tag="receipt-scanner-prompts"
This package simply uses blade files as prompts, the {{ $context }}
variable will be replaced by the text you pass
to ReceiptScanner::scan("text here")
.
By default, the package uses the receipt.blade.php
file as its prompt template, you may add additional templates by
simply creating a blade file in resources/views/vendor/receipt-scanner/minimal_invoice.blade.php
and changing
the $template
parameter when calling scan()
Example prompt:
Extract the following fields from the text below, output as JSON
date (as string in the Y-m-d format)
total_amount (as float, do not include currency symbol)
vendor_name (company name)
{{ $context }}
OUTPUT IN JSON
use HelgeSverre\ReceiptScanner\Facades\ReceiptScanner;
$receipt = ReceiptScanner::scan(
text: "Your invoice here",
model: ModelNames::TURBO_INSTRUCT,
template: 'minimal_invoice',
asArray: true,
);
This package is licensed under the MIT License. For more details, refer to the License File.
For Tasks:
Click tags to check more tools for each tasksFor Jobs:
Alternative AI tools for receipt-scanner
Similar Open Source Tools
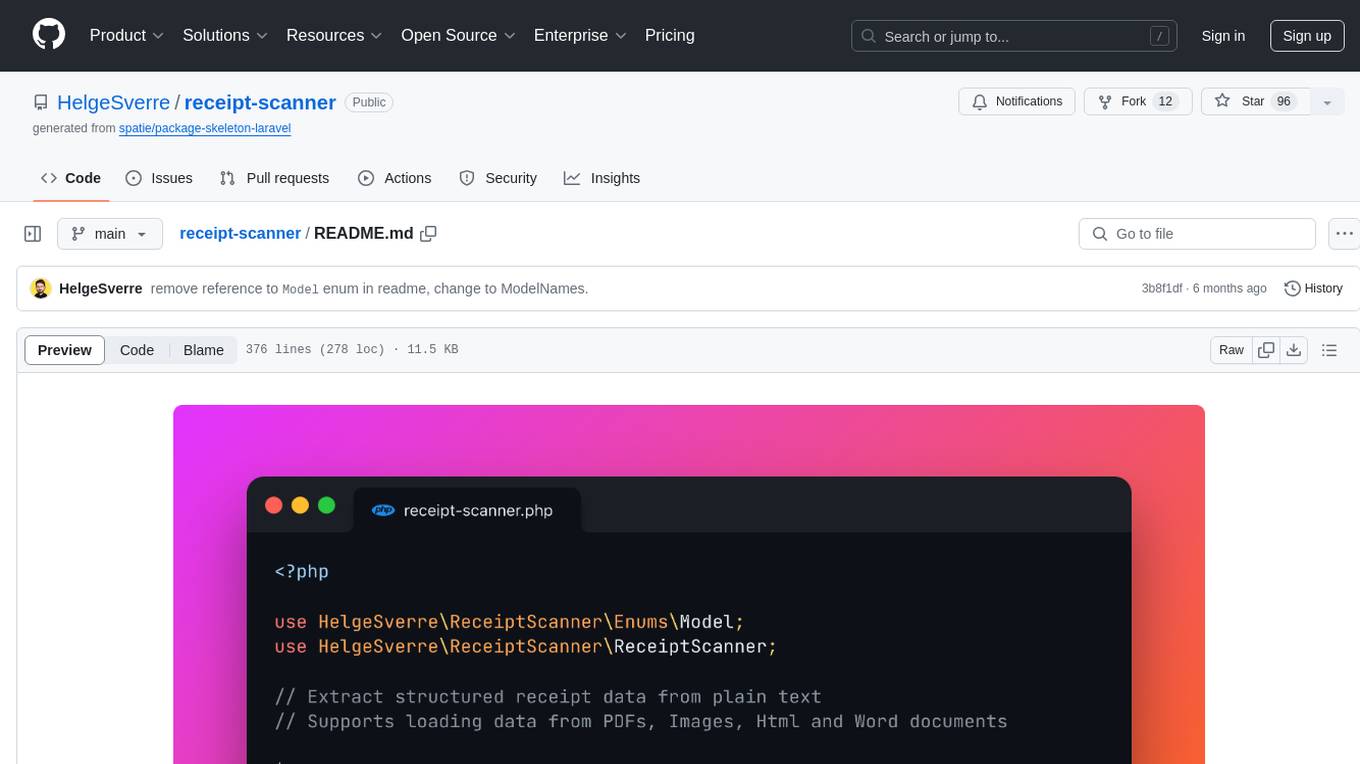
receipt-scanner
The receipt-scanner repository is an AI-Powered Receipt and Invoice Scanner for Laravel that allows users to easily extract structured receipt data from images, PDFs, and emails within their Laravel application using OpenAI. It provides a light wrapper around OpenAI Chat and Completion endpoints, supports various input formats, and integrates with Textract for OCR functionality. Users can install the package via composer, publish configuration files, and use it to extract data from plain text, PDFs, images, Word documents, and web content. The scanned receipt data is parsed into a DTO structure with main classes like Receipt, Merchant, and LineItem.
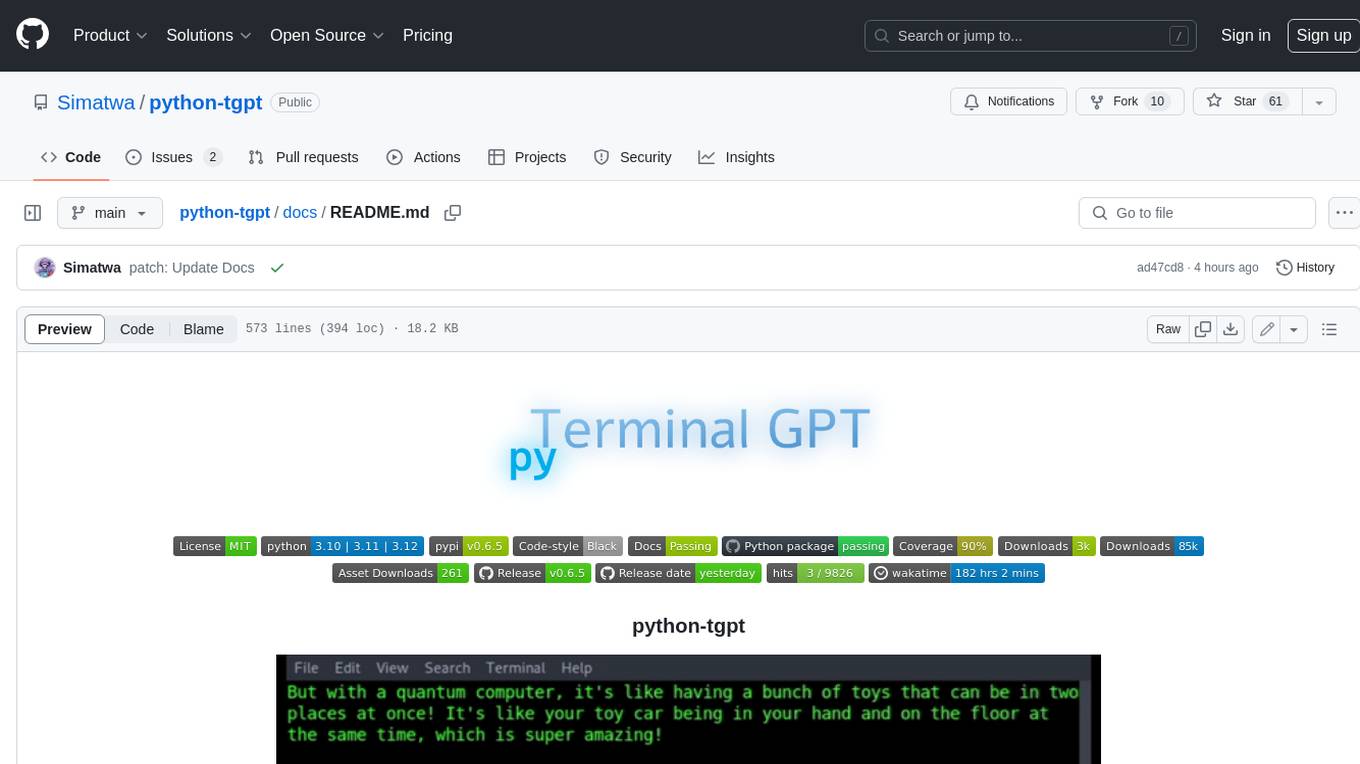
python-tgpt
Python-tgpt is a Python package that enables seamless interaction with over 45 free LLM providers without requiring an API key. It also provides image generation capabilities. The name _python-tgpt_ draws inspiration from its parent project tgpt, which operates on Golang. Through this Python adaptation, users can effortlessly engage with a number of free LLMs available, fostering a smoother AI interaction experience.
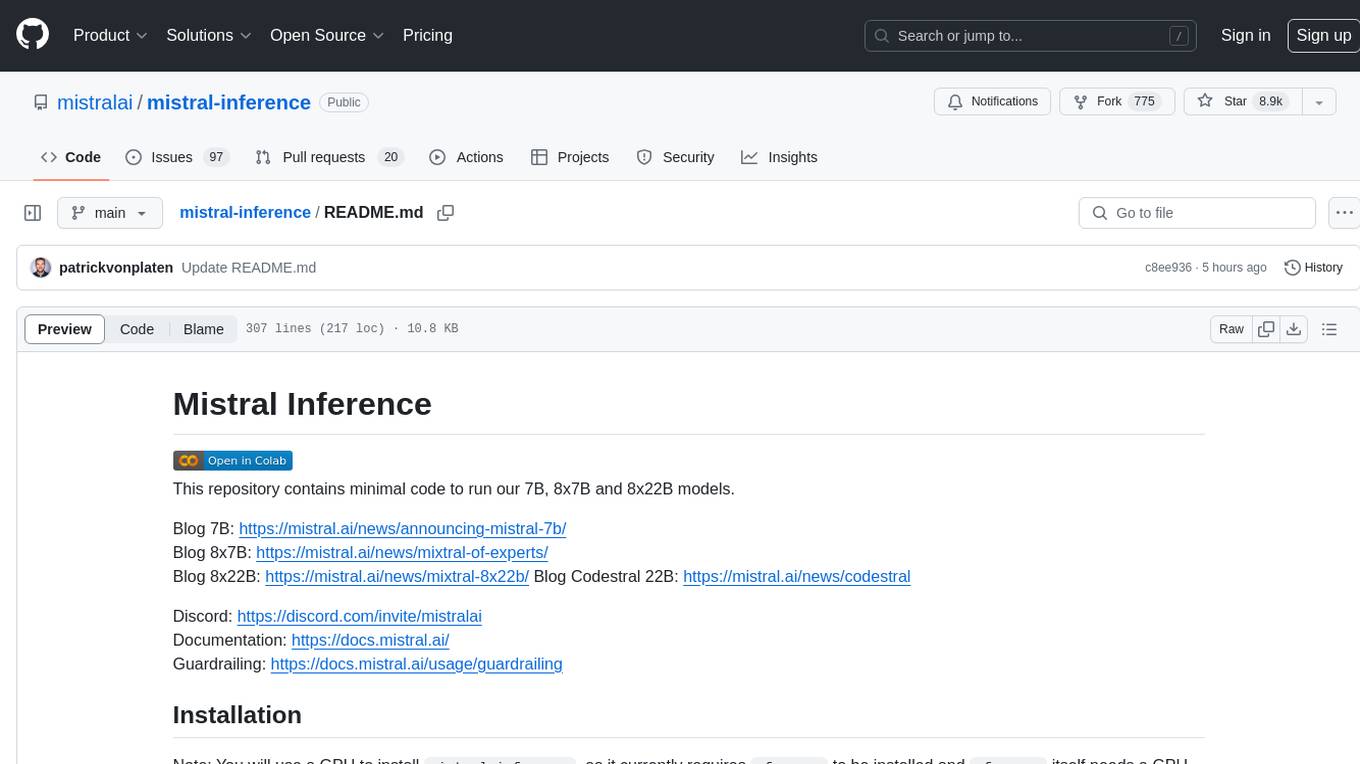
mistral-inference
Mistral Inference repository contains minimal code to run 7B, 8x7B, and 8x22B models. It provides model download links, installation instructions, and usage guidelines for running models via CLI or Python. The repository also includes information on guardrailing, model platforms, deployment, and references. Users can interact with models through commands like mistral-demo, mistral-chat, and mistral-common. Mistral AI models support function calling and chat interactions for tasks like testing models, chatting with models, and using Codestral as a coding assistant. The repository offers detailed documentation and links to blogs for further information.
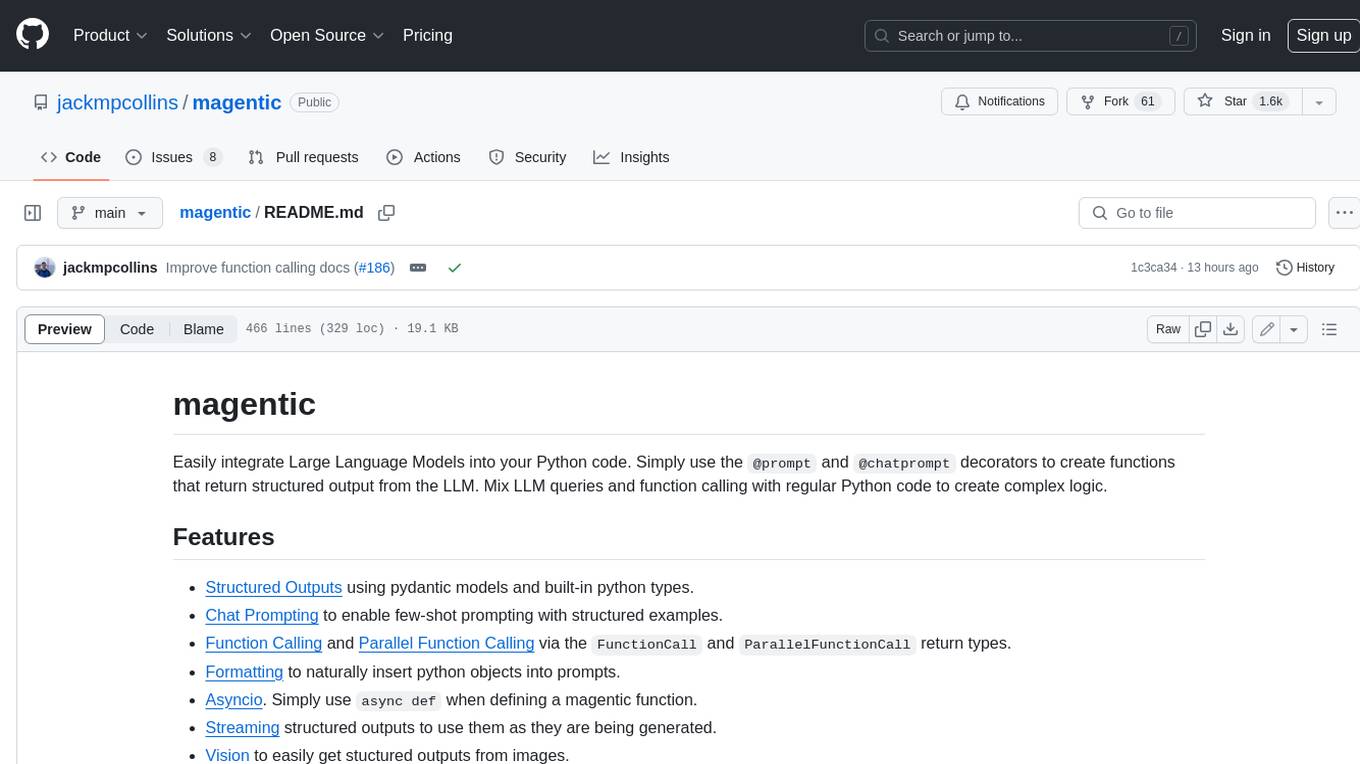
magentic
Easily integrate Large Language Models into your Python code. Simply use the `@prompt` and `@chatprompt` decorators to create functions that return structured output from the LLM. Mix LLM queries and function calling with regular Python code to create complex logic.
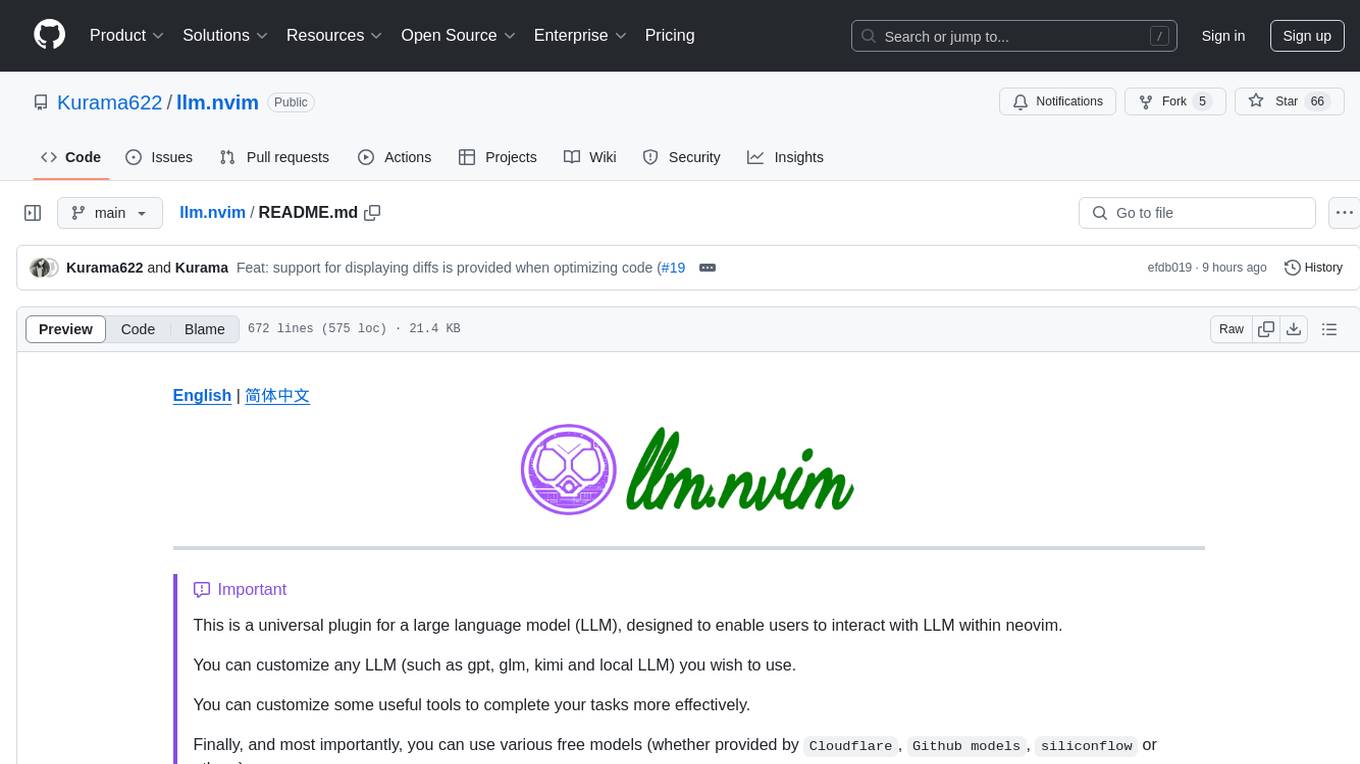
llm.nvim
llm.nvim is a universal plugin for a large language model (LLM) designed to enable users to interact with LLM within neovim. Users can customize various LLMs such as gpt, glm, kimi, and local LLM. The plugin provides tools for optimizing code, comparing code, translating text, and more. It also supports integration with free models from Cloudflare, Github models, siliconflow, and others. Users can customize tools, chat with LLM, quickly translate text, and explain code snippets. The plugin offers a flexible window interface for easy interaction and customization.
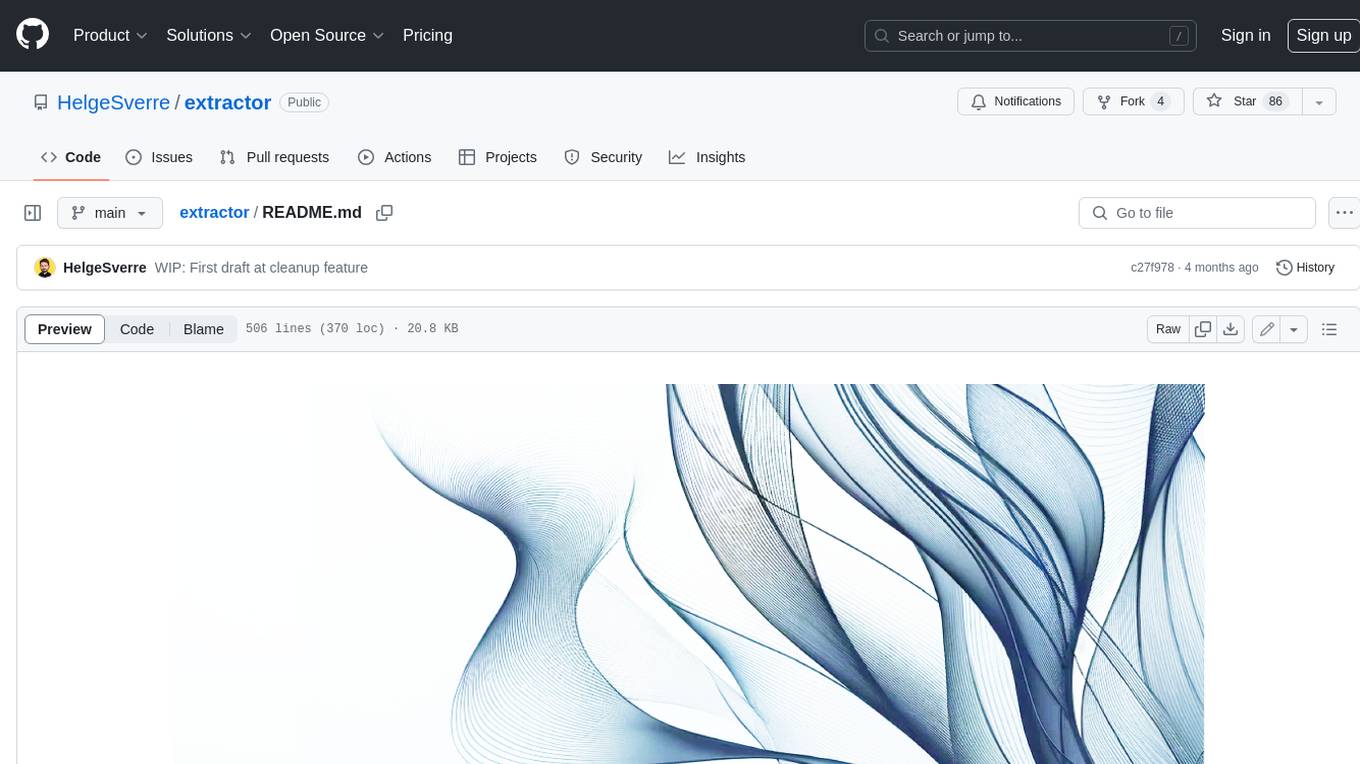
extractor
Extractor is an AI-powered data extraction library for Laravel that leverages OpenAI's capabilities to effortlessly extract structured data from various sources, including images, PDFs, and emails. It features a convenient wrapper around OpenAI Chat and Completion endpoints, supports multiple input formats, includes a flexible Field Extractor for arbitrary data extraction, and integrates with Textract for OCR functionality. Extractor utilizes JSON Mode from the latest GPT-3.5 and GPT-4 models, providing accurate and efficient data extraction.
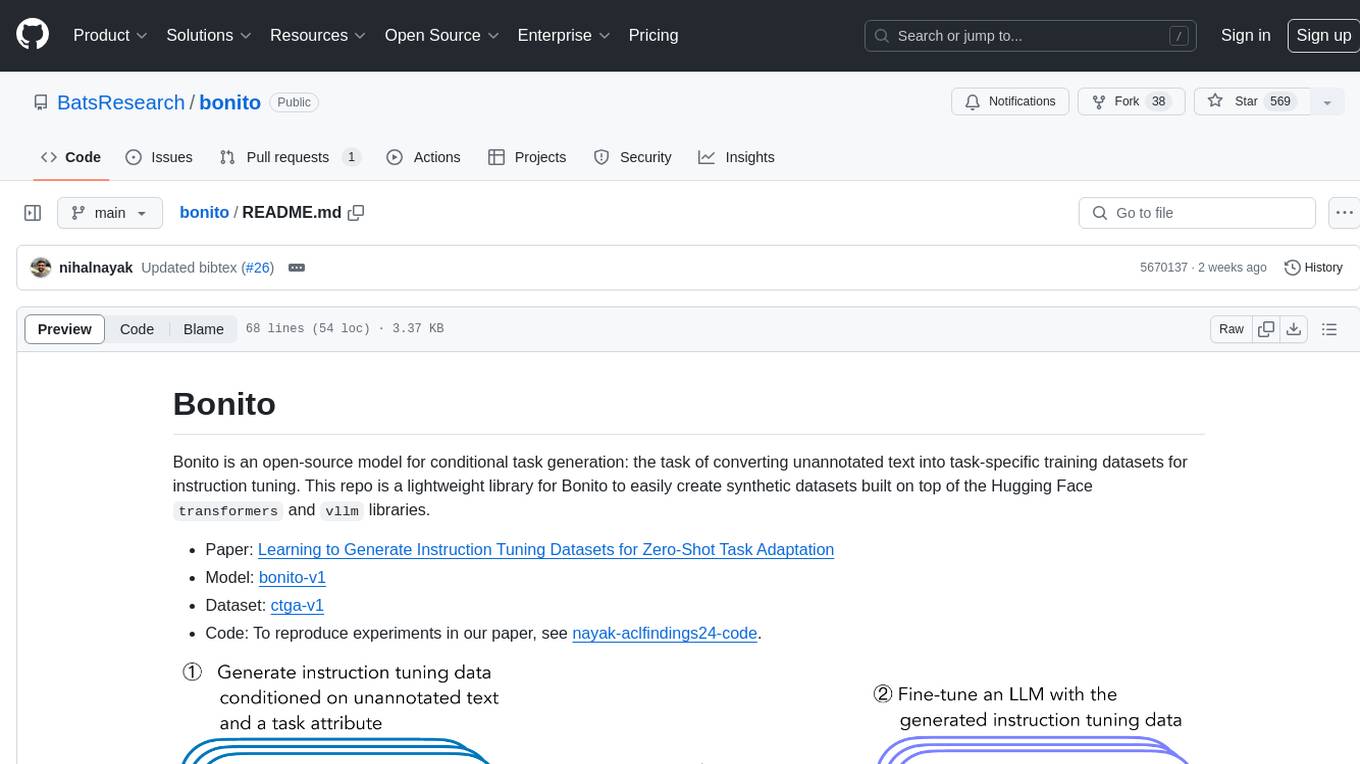
bonito
Bonito is an open-source model for conditional task generation, converting unannotated text into task-specific training datasets for instruction tuning. It is a lightweight library built on top of Hugging Face `transformers` and `vllm` libraries. The tool supports various task types such as question answering, paraphrase generation, sentiment analysis, summarization, and more. Users can easily generate synthetic instruction tuning datasets using Bonito for zero-shot task adaptation.
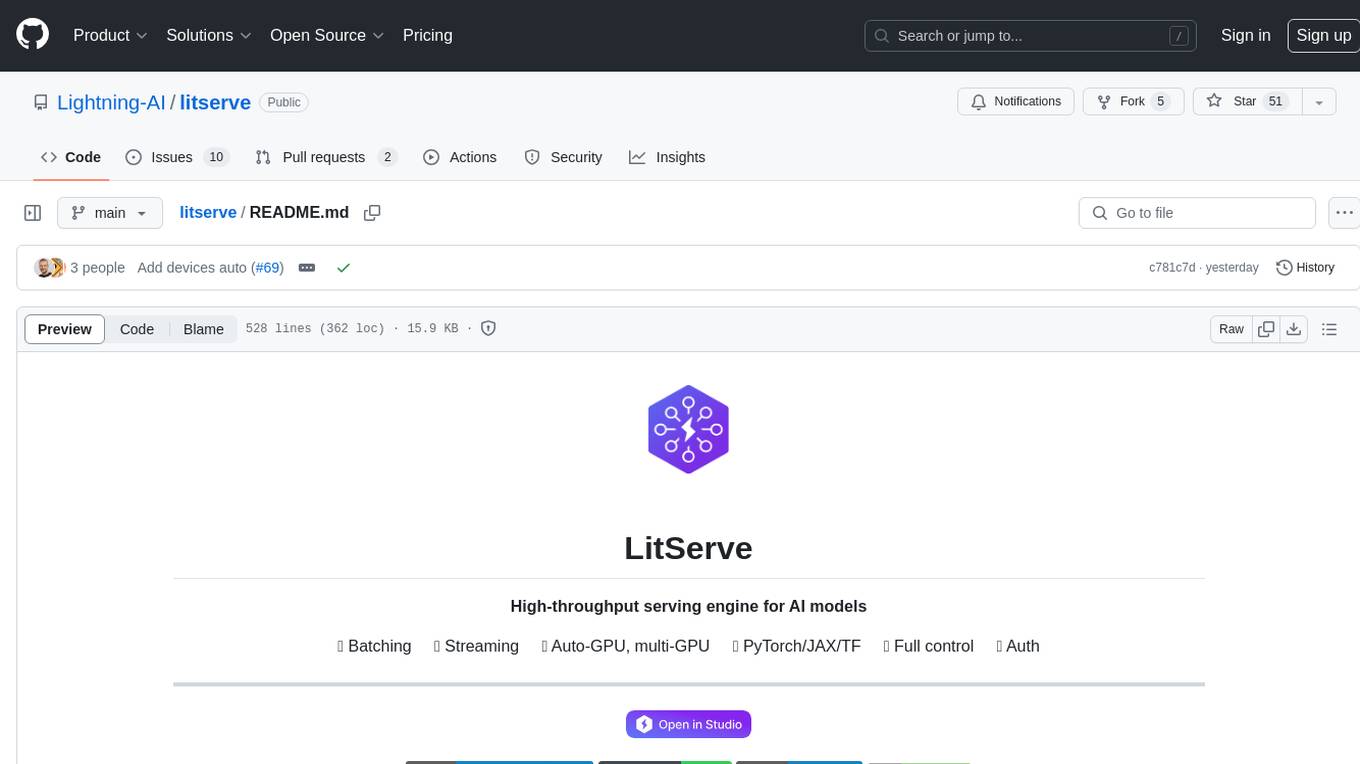
litserve
LitServe is a high-throughput serving engine for deploying AI models at scale. It generates an API endpoint for a model, handles batching, streaming, autoscaling across CPU/GPUs, and more. Built for enterprise scale, it supports every framework like PyTorch, JAX, Tensorflow, and more. LitServe is designed to let users focus on model performance, not the serving boilerplate. It is like PyTorch Lightning for model serving but with broader framework support and scalability.
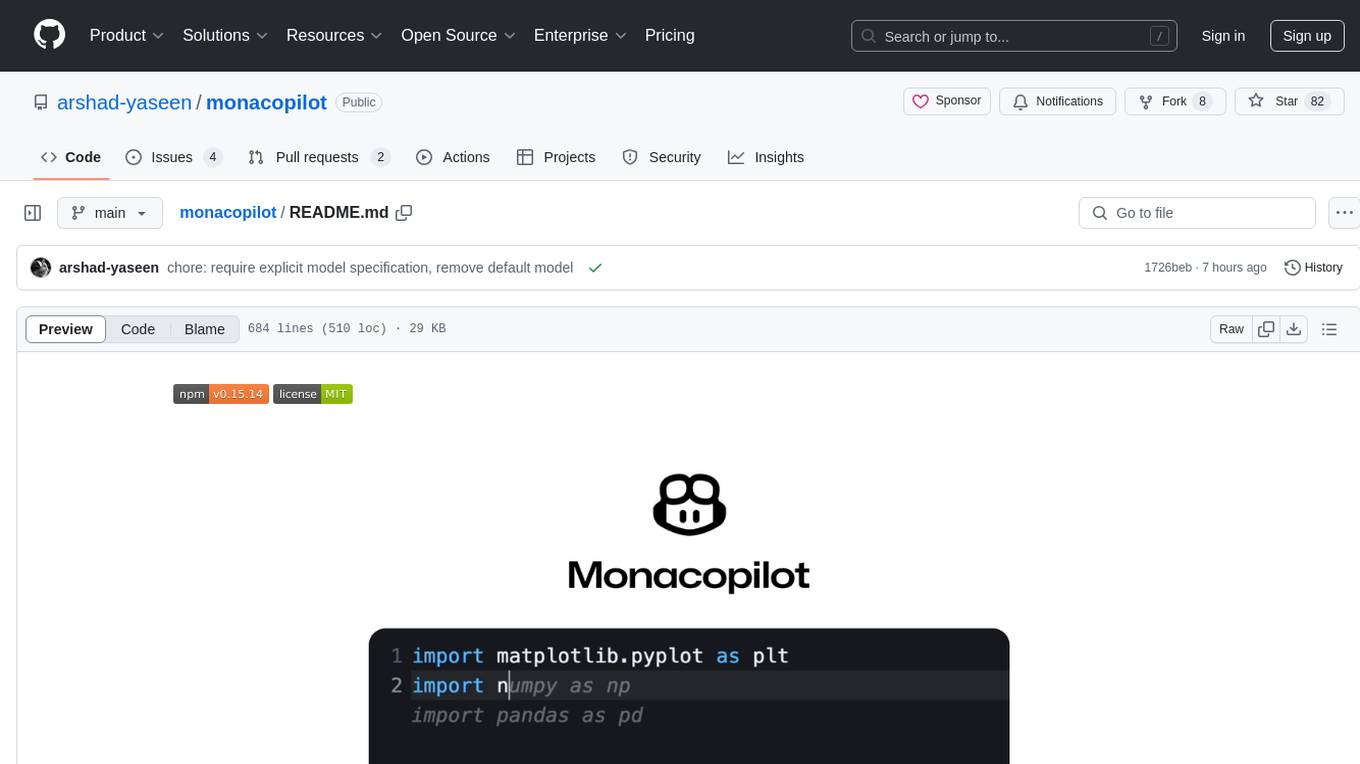
monacopilot
Monacopilot is a powerful and customizable AI auto-completion plugin for the Monaco Editor. It supports multiple AI providers such as Anthropic, OpenAI, Groq, and Google, providing real-time code completions with an efficient caching system. The plugin offers context-aware suggestions, customizable completion behavior, and framework agnostic features. Users can also customize the model support and trigger completions manually. Monacopilot is designed to enhance coding productivity by providing accurate and contextually appropriate completions in daily spoken language.
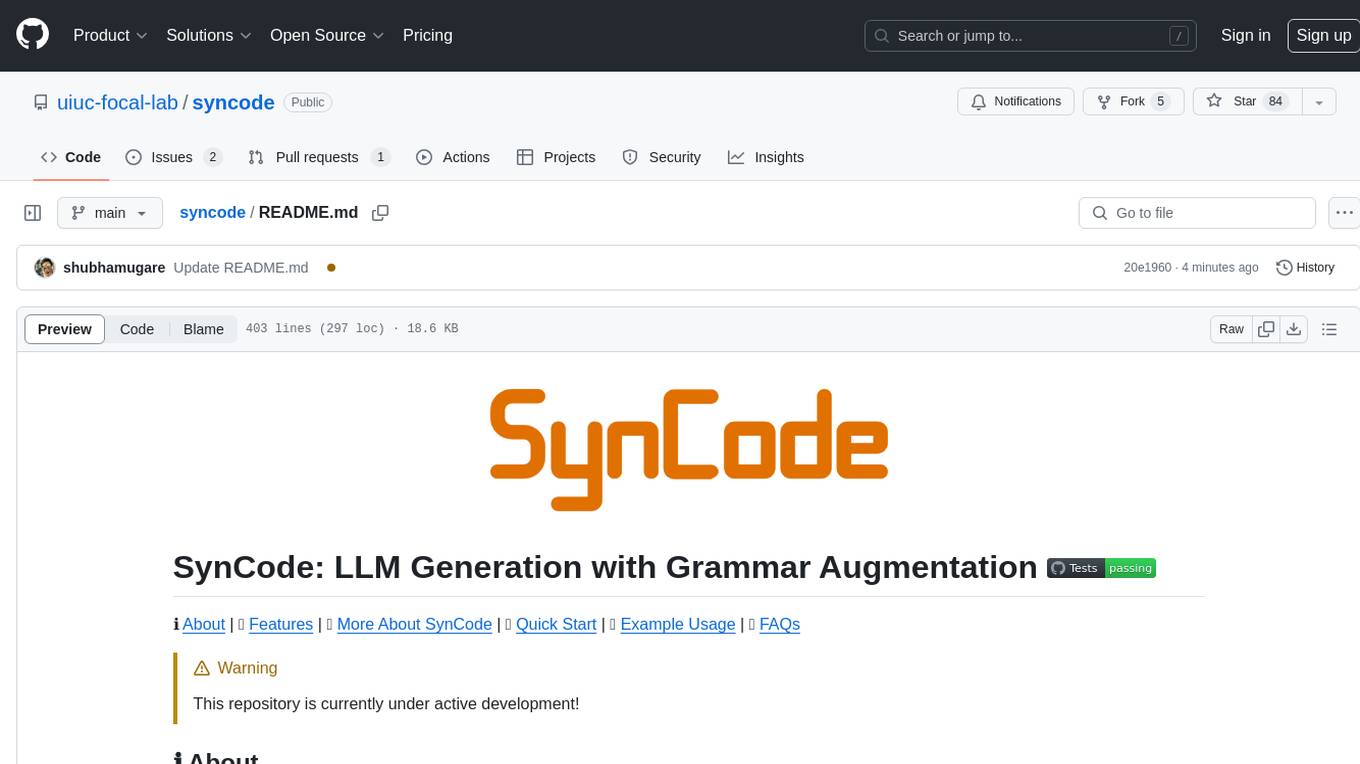
syncode
SynCode is a novel framework for the grammar-guided generation of Large Language Models (LLMs) that ensures syntactically valid output with respect to defined Context-Free Grammar (CFG) rules. It supports general-purpose programming languages like Python, Go, SQL, JSON, and more, allowing users to define custom grammars using EBNF syntax. The tool compares favorably to other constrained decoders and offers features like fast grammar-guided generation, compatibility with HuggingFace Language Models, and the ability to work with various decoding strategies.
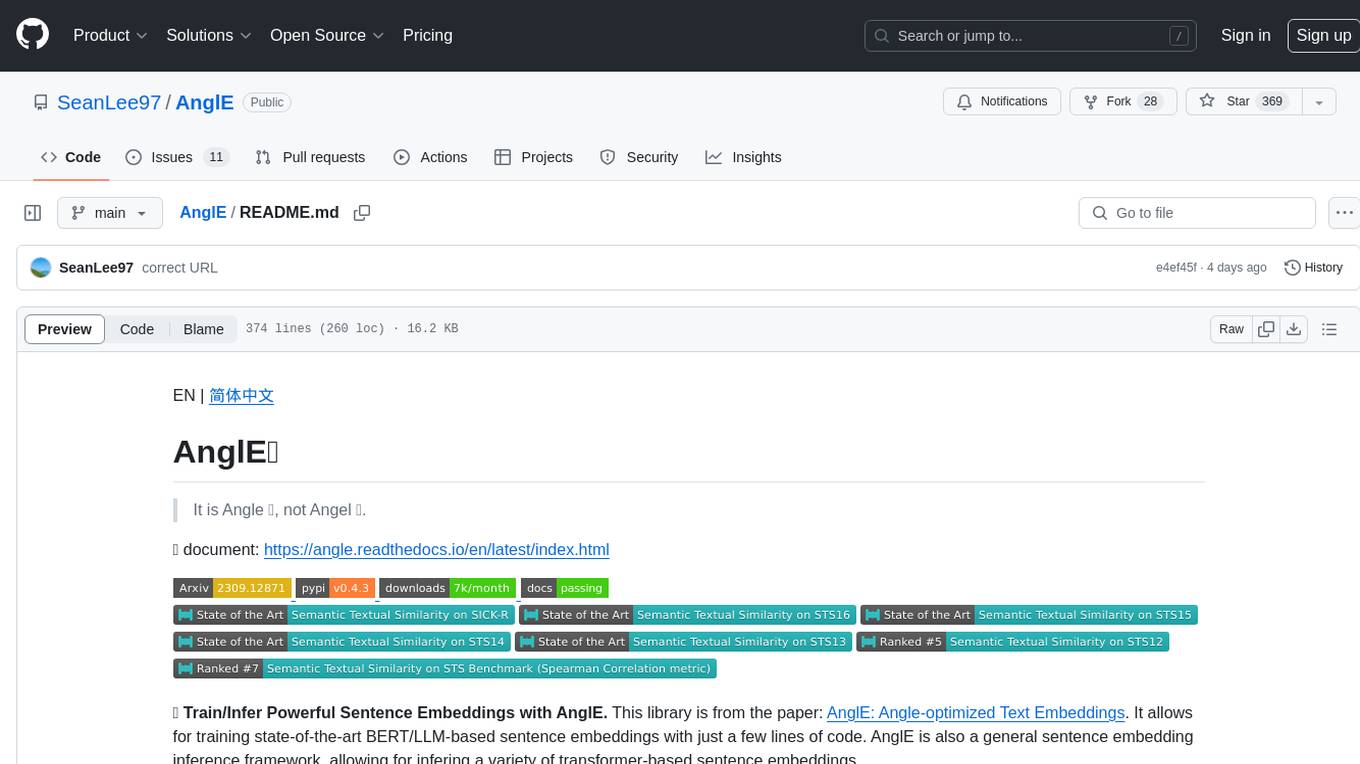
AnglE
AnglE is a library for training state-of-the-art BERT/LLM-based sentence embeddings with just a few lines of code. It also serves as a general sentence embedding inference framework, allowing for inferring a variety of transformer-based sentence embeddings. The library supports various loss functions such as AnglE loss, Contrastive loss, CoSENT loss, and Espresso loss. It provides backbones like BERT-based models, LLM-based models, and Bi-directional LLM-based models for training on single or multi-GPU setups. AnglE has achieved significant performance on various benchmarks and offers official pretrained models for both BERT-based and LLM-based models.
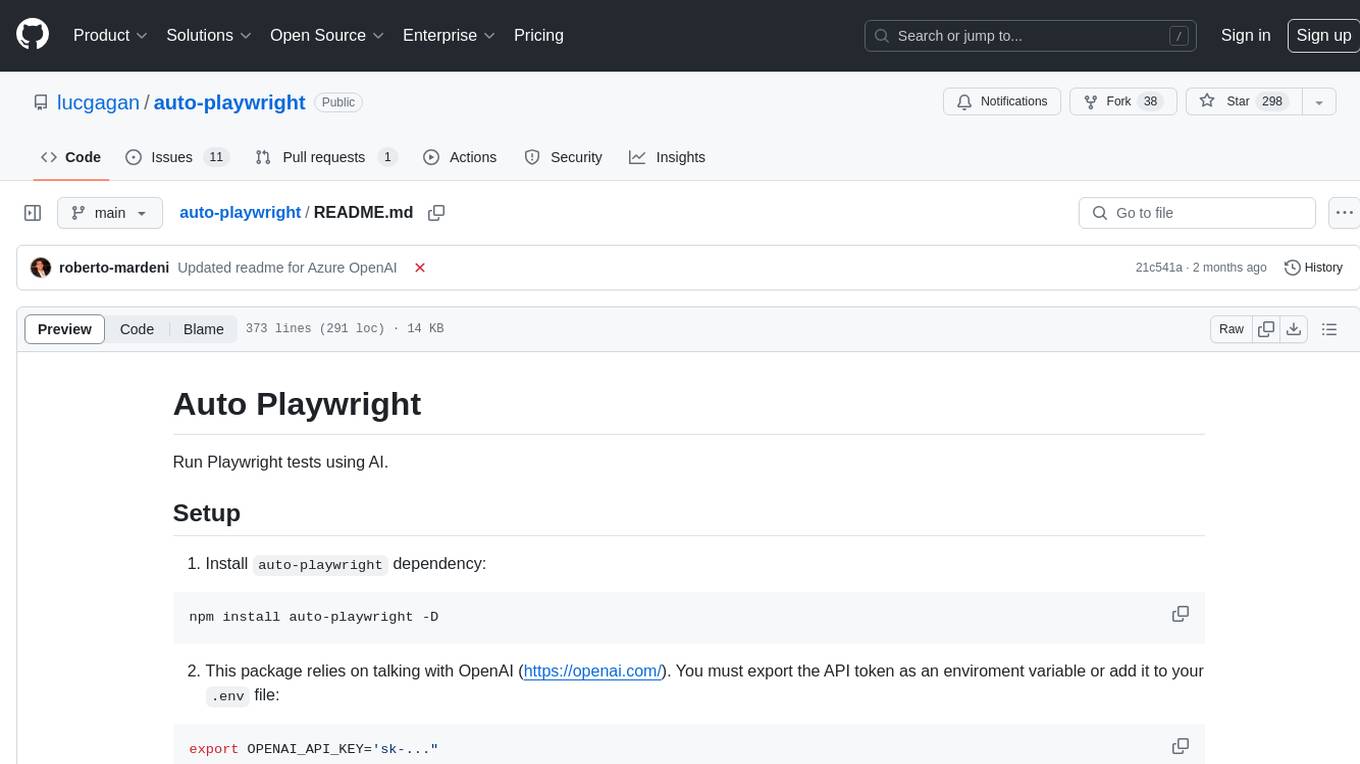
auto-playwright
Auto Playwright is a tool that allows users to run Playwright tests using AI. It eliminates the need for selectors by determining actions at runtime based on plain-text instructions. Users can automate complex scenarios, write tests concurrently with or before functionality development, and benefit from rapid test creation. The tool supports various Playwright actions and offers additional options for debugging and customization. It uses HTML sanitization to reduce costs and improve text quality when interacting with the OpenAI API.
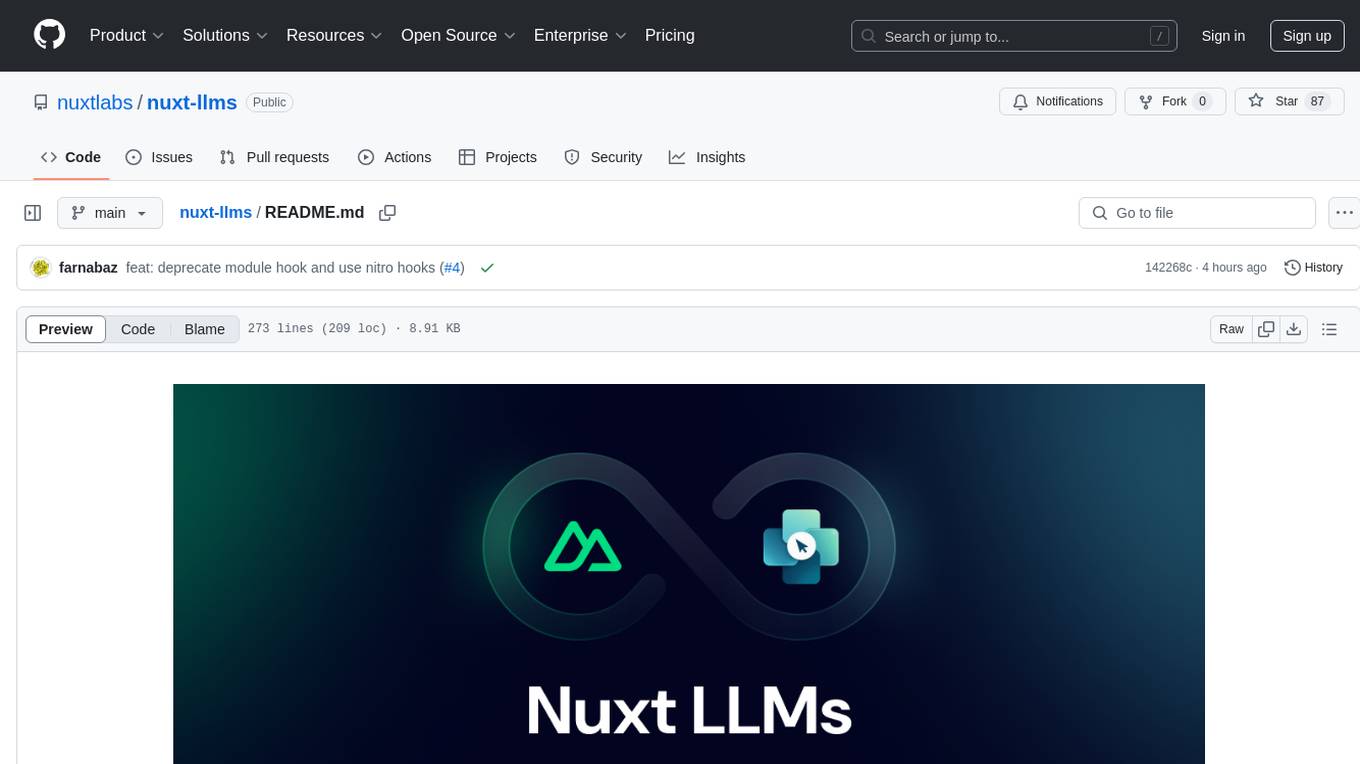
nuxt-llms
Nuxt LLMs automatically generates llms.txt markdown documentation for Nuxt applications. It provides runtime hooks to collect data from various sources and generate structured documentation. The tool allows customization of sections directly from nuxt.config.ts and integrates with Nuxt modules via the runtime hooks system. It generates two documentation formats: llms.txt for concise structured documentation and llms_full.txt for detailed documentation. Users can extend documentation using hooks to add sections, links, and metadata. The tool is suitable for developers looking to automate documentation generation for their Nuxt applications.
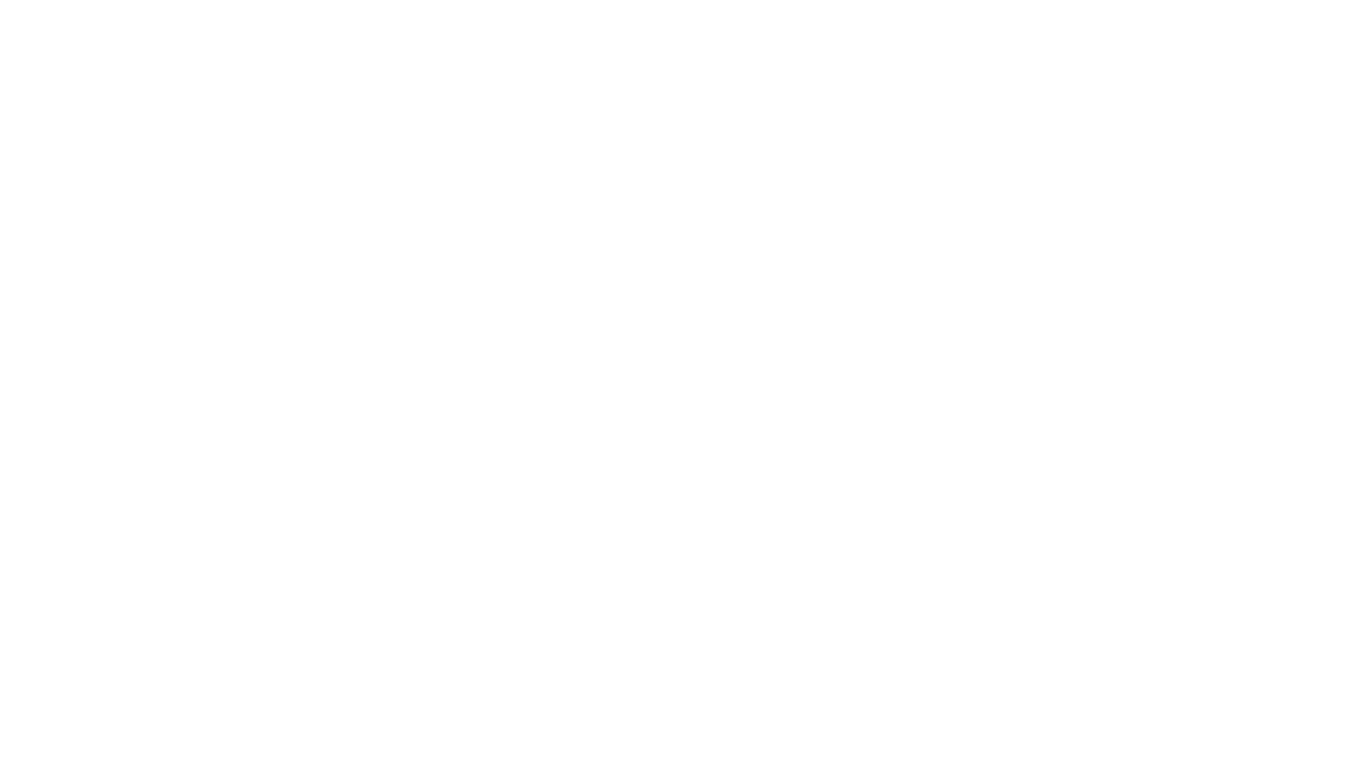
litdata
LitData is a tool designed for blazingly fast, distributed streaming of training data from any cloud storage. It allows users to transform and optimize data in cloud storage environments efficiently and intuitively, supporting various data types like images, text, video, audio, geo-spatial, and multimodal data. LitData integrates smoothly with frameworks such as LitGPT and PyTorch, enabling seamless streaming of data to multiple machines. Key features include multi-GPU/multi-node support, easy data mixing, pause & resume functionality, support for profiling, memory footprint reduction, cache size configuration, and on-prem optimizations. The tool also provides benchmarks for measuring streaming speed and conversion efficiency, along with runnable templates for different data types. LitData enables infinite cloud data processing by utilizing the Lightning.ai platform to scale data processing with optimized machines.
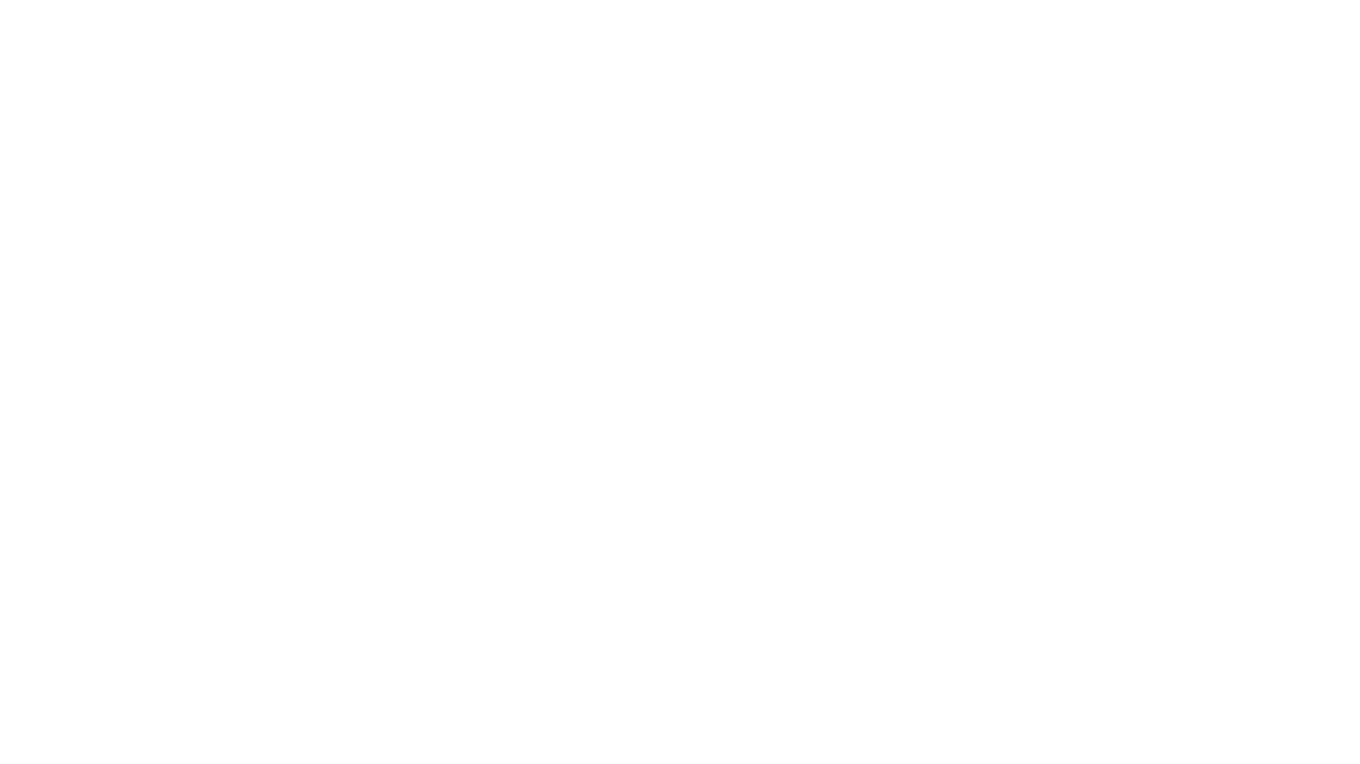
generative-ai-python
The Google AI Python SDK is the easiest way for Python developers to build with the Gemini API. The Gemini API gives you access to Gemini models created by Google DeepMind. Gemini models are built from the ground up to be multimodal, so you can reason seamlessly across text, images, and code.
For similar tasks
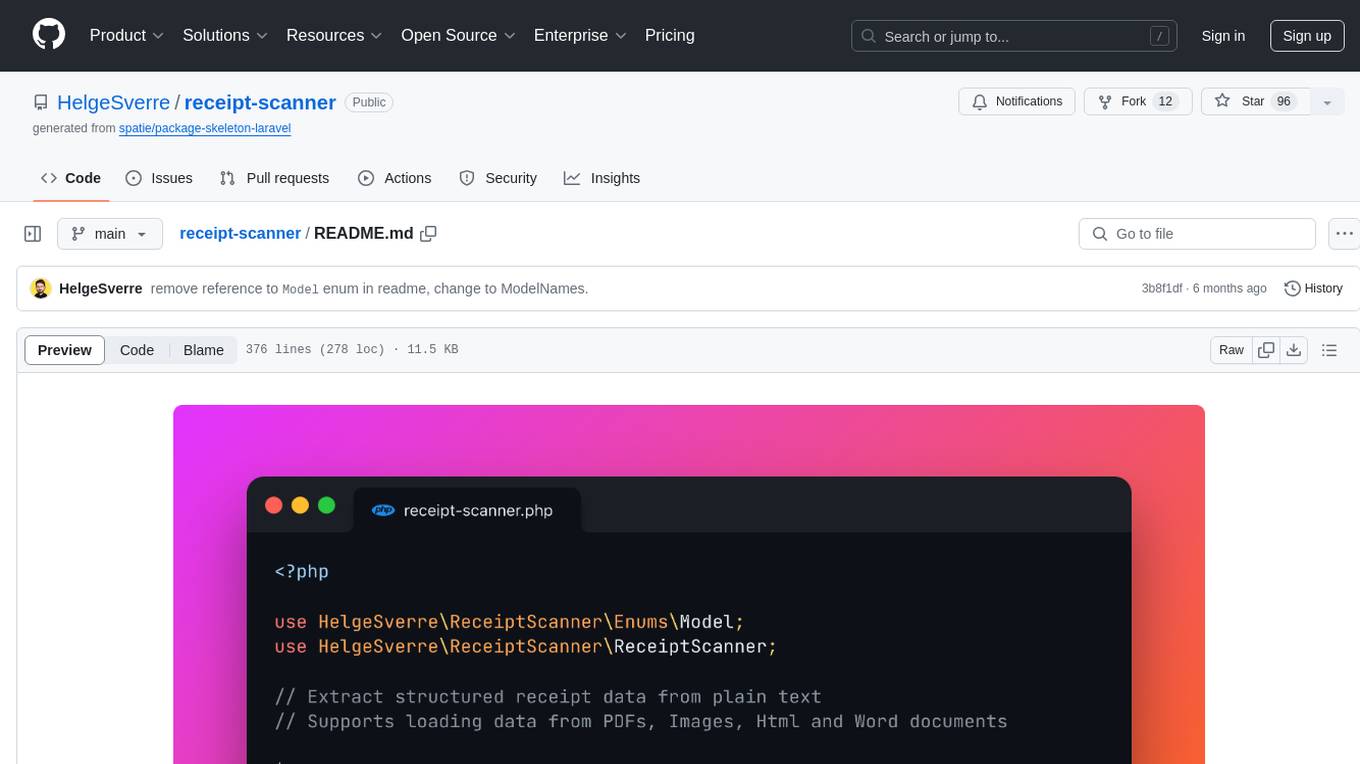
receipt-scanner
The receipt-scanner repository is an AI-Powered Receipt and Invoice Scanner for Laravel that allows users to easily extract structured receipt data from images, PDFs, and emails within their Laravel application using OpenAI. It provides a light wrapper around OpenAI Chat and Completion endpoints, supports various input formats, and integrates with Textract for OCR functionality. Users can install the package via composer, publish configuration files, and use it to extract data from plain text, PDFs, images, Word documents, and web content. The scanned receipt data is parsed into a DTO structure with main classes like Receipt, Merchant, and LineItem.
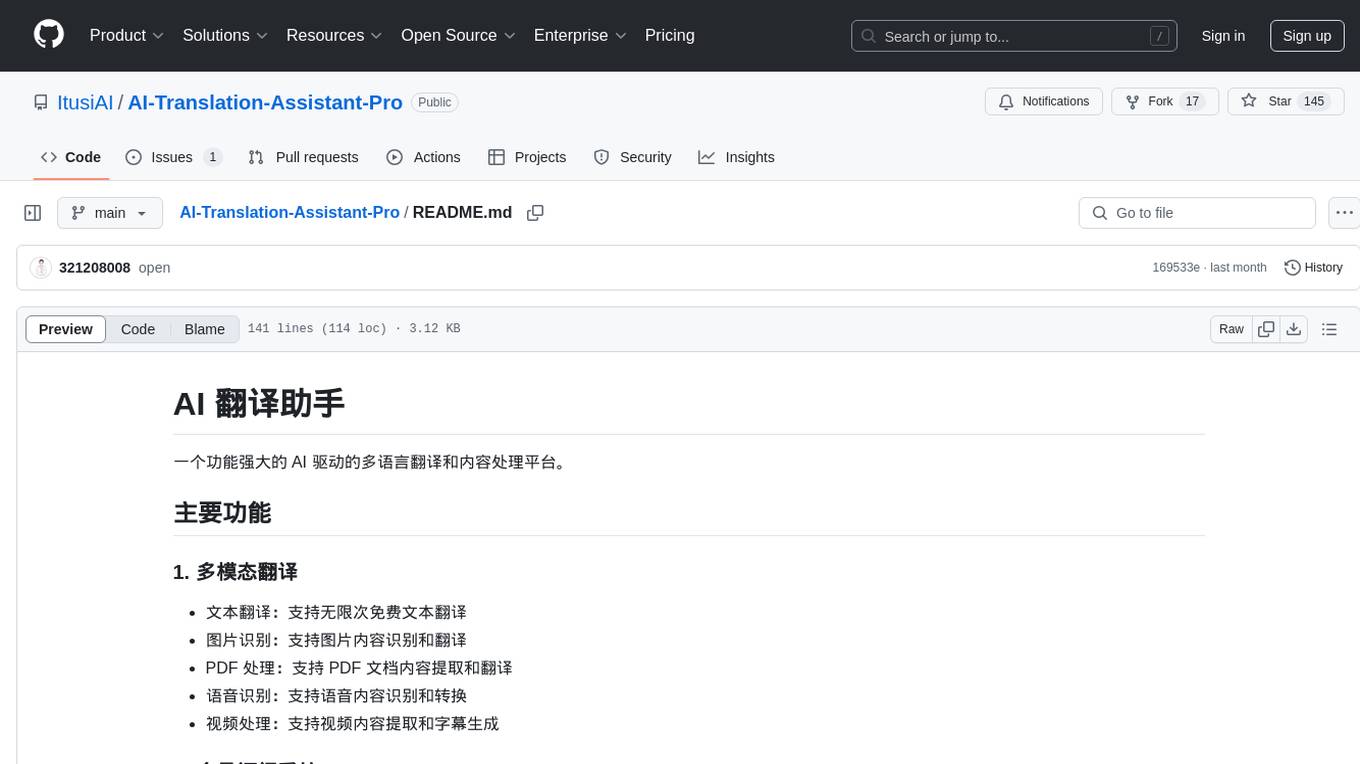
AI-Translation-Assistant-Pro
AI Translation Assistant Pro is a powerful AI-driven platform for multilingual translation and content processing. It offers features such as text translation, image recognition, PDF processing, speech recognition, and video processing. The platform includes a subscription system with different membership levels, user management functionalities, quota management, and real-time usage statistics. It utilizes technologies like Next.js, React, TypeScript for the frontend, Node.js, PostgreSQL for the backend, NextAuth.js for authentication, Stripe for payments, and integrates with cloud services like Aliyun OSS and Tencent Cloud for AI services.
For similar jobs
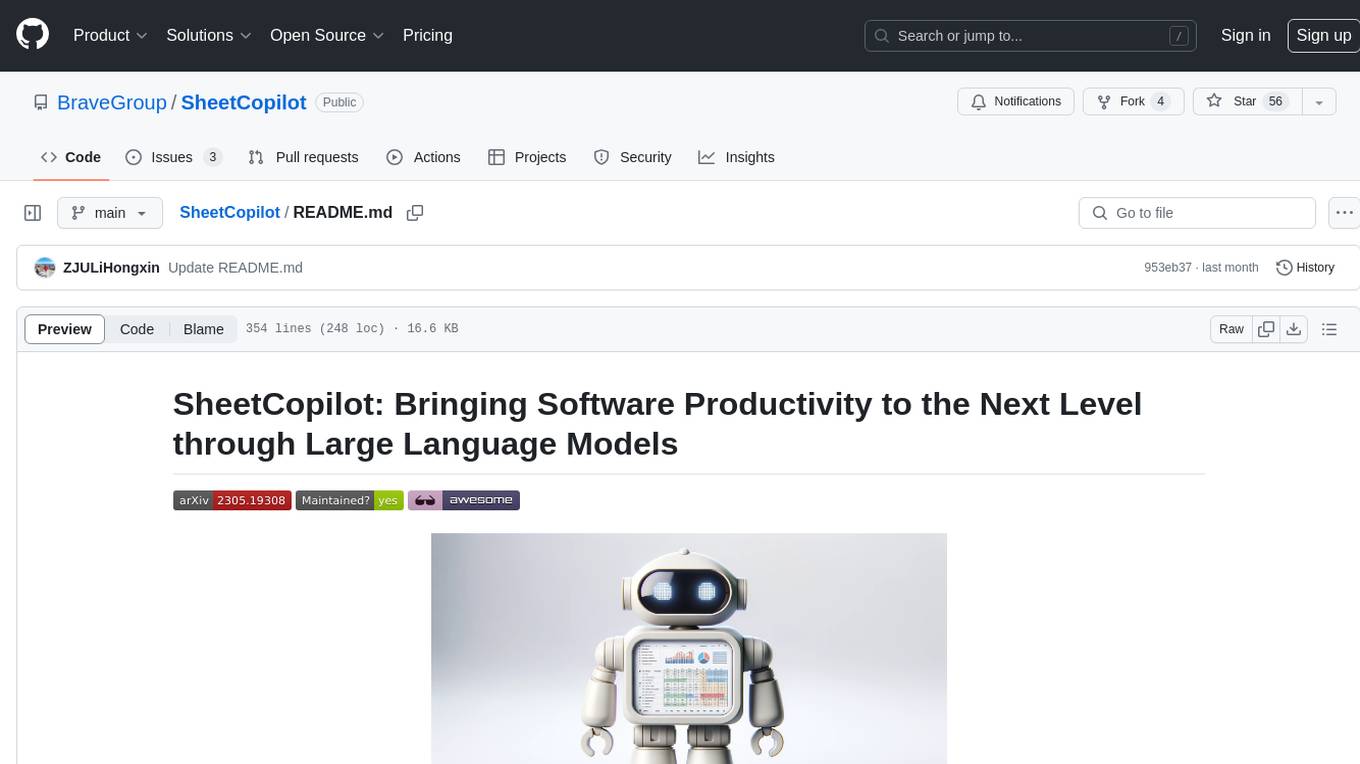
SheetCopilot
SheetCopilot is an assistant agent that manipulates spreadsheets by following user commands. It leverages Large Language Models (LLMs) to interact with spreadsheets like a human expert, enabling non-expert users to complete tasks on complex software such as Google Sheets and Excel via a language interface. The tool observes spreadsheet states, polishes generated solutions based on external action documents and error feedback, and aims to improve success rate and efficiency. SheetCopilot offers a dataset with diverse task categories and operations, supporting operations like entry & manipulation, management, formatting, charts, and pivot tables. Users can interact with SheetCopilot in Excel or Google Sheets, executing tasks like calculating revenue, creating pivot tables, and plotting charts. The tool's evaluation includes performance comparisons with leading LLMs and VBA-based methods on specific datasets, showcasing its capabilities in controlling various aspects of a spreadsheet.
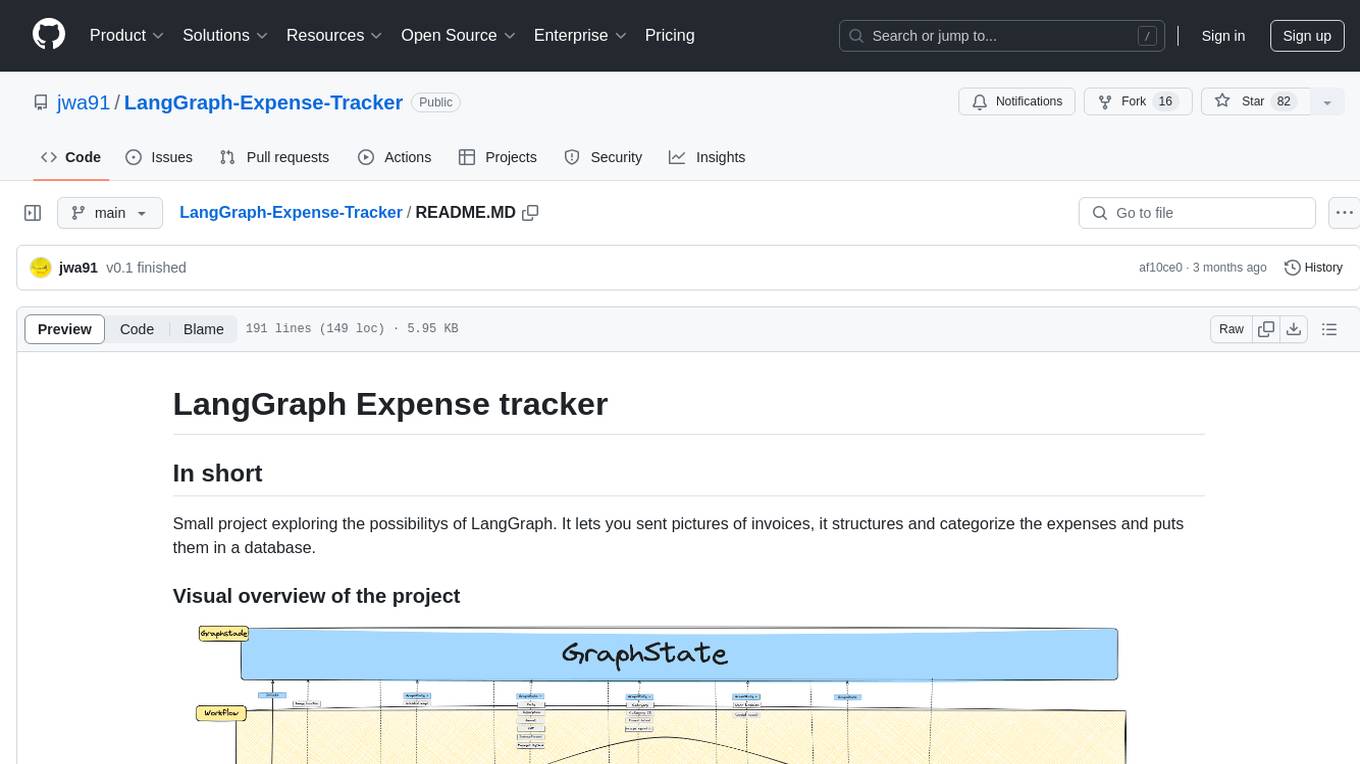
LangGraph-Expense-Tracker
LangGraph Expense tracker is a small project that explores the possibilities of LangGraph. It allows users to send pictures of invoices, which are then structured and categorized into expenses and stored in a database. The project includes functionalities for invoice extraction, database setup, and API configuration. It consists of various modules for categorizing expenses, creating database tables, and running the API. The database schema includes tables for categories, payment methods, and expenses, each with specific columns to track transaction details. The API documentation is available for reference, and the project utilizes LangChain for processing expense data.
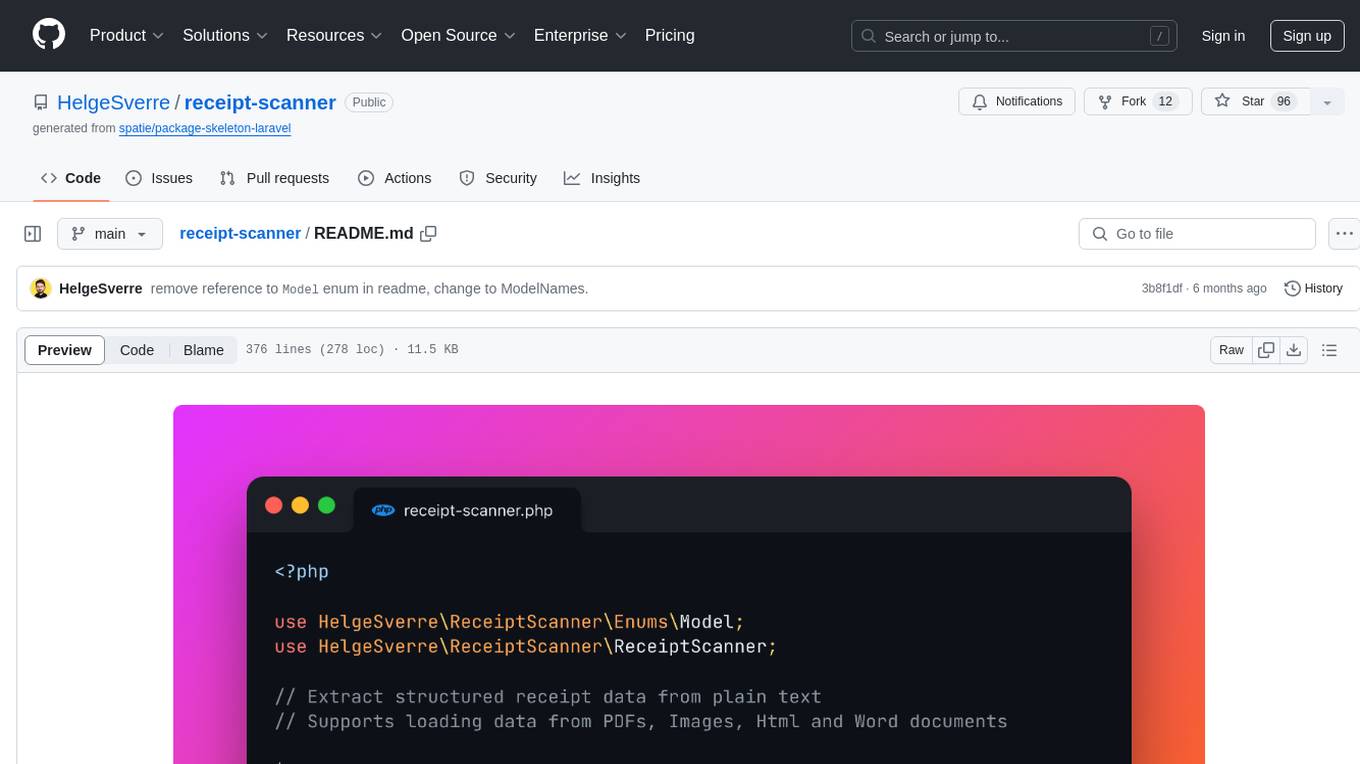
receipt-scanner
The receipt-scanner repository is an AI-Powered Receipt and Invoice Scanner for Laravel that allows users to easily extract structured receipt data from images, PDFs, and emails within their Laravel application using OpenAI. It provides a light wrapper around OpenAI Chat and Completion endpoints, supports various input formats, and integrates with Textract for OCR functionality. Users can install the package via composer, publish configuration files, and use it to extract data from plain text, PDFs, images, Word documents, and web content. The scanned receipt data is parsed into a DTO structure with main classes like Receipt, Merchant, and LineItem.
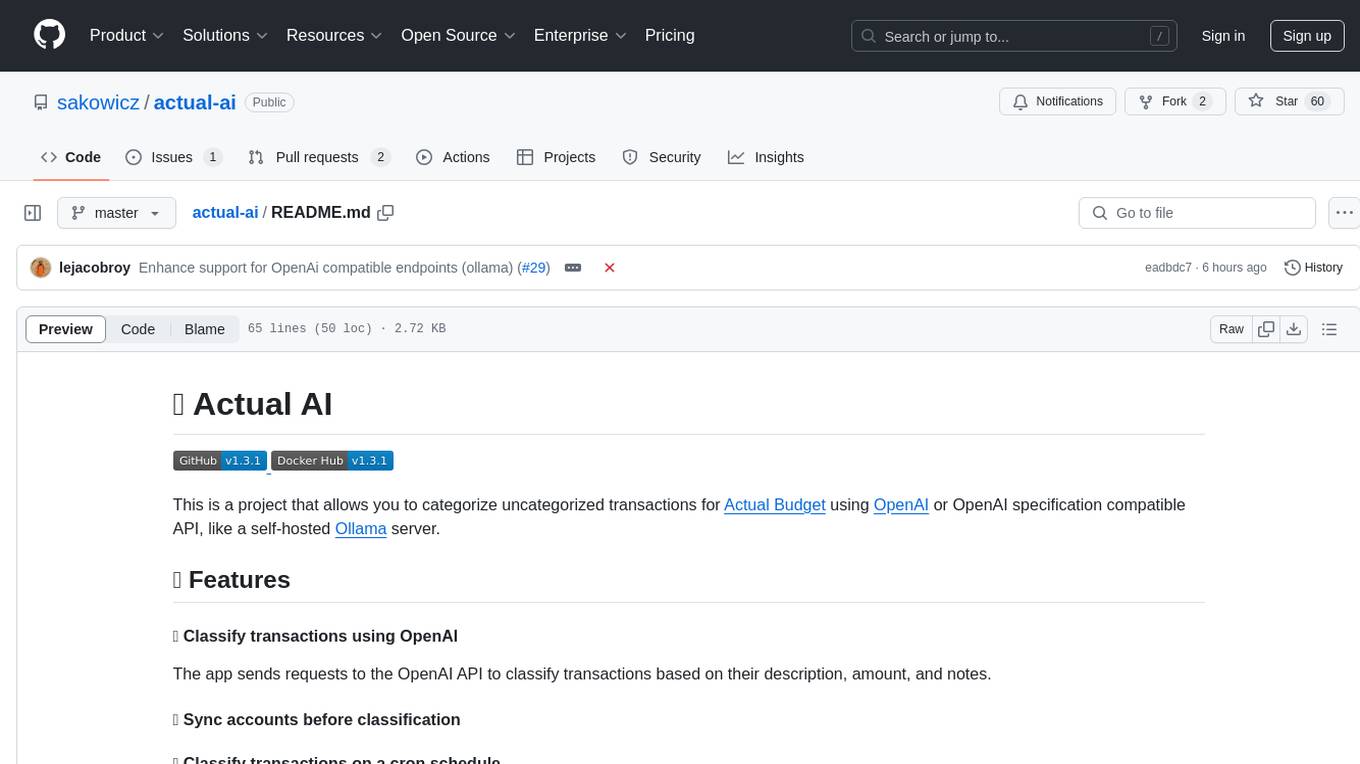
actual-ai
Actual AI is a project designed to categorize uncategorized transactions for Actual Budget using OpenAI or OpenAI specification compatible API. It sends requests to the OpenAI API to classify transactions based on their description, amount, and notes. Transactions that cannot be classified are marked as 'not guessed' in notes. The tool allows users to sync accounts before classification and classify transactions on a cron schedule. Guessed transactions are marked in notes for easy review.
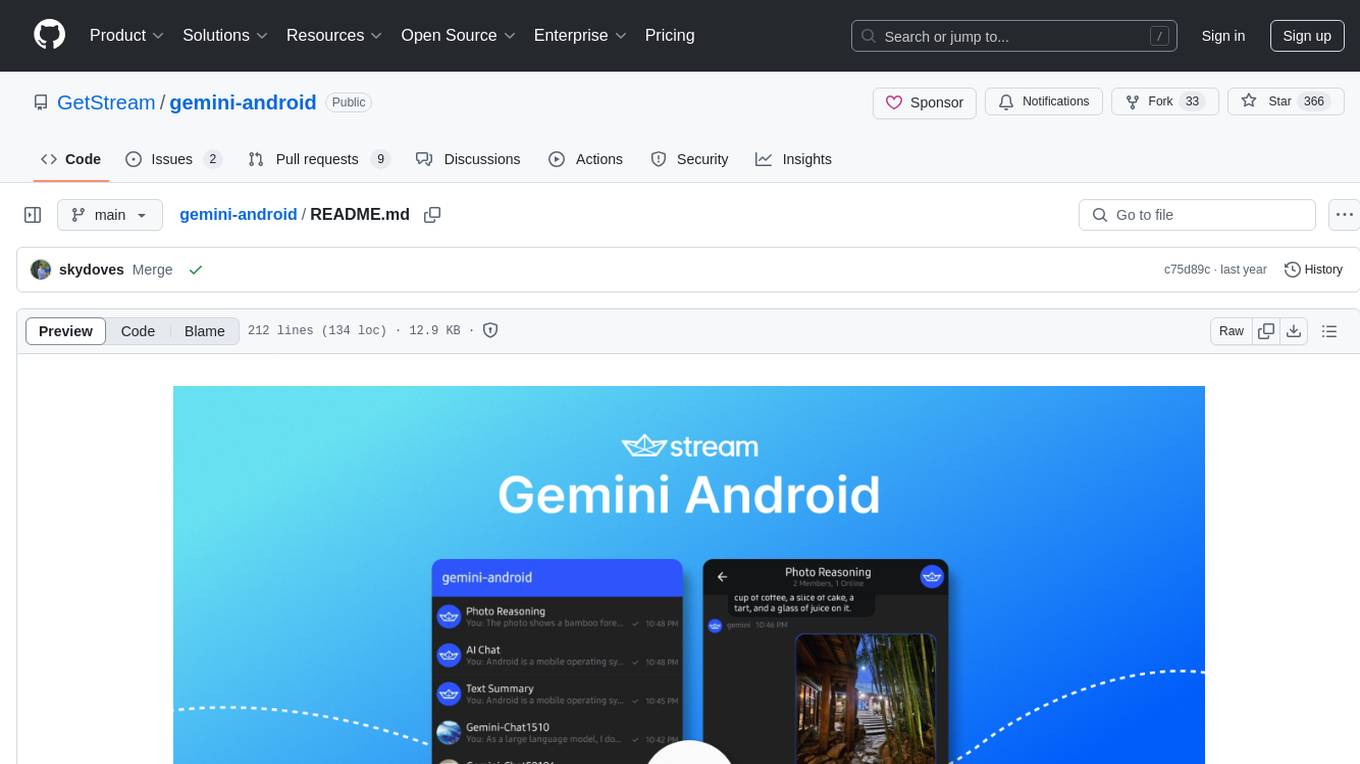
gemini-android
Gemini-Android is a mobile application that allows users to track their expenses and manage their finances on the go. The app provides a user-friendly interface for adding and categorizing expenses, setting budgets, and generating reports to help users make informed financial decisions. With Gemini-Android, users can easily monitor their spending habits, identify areas for saving, and stay on top of their financial goals.
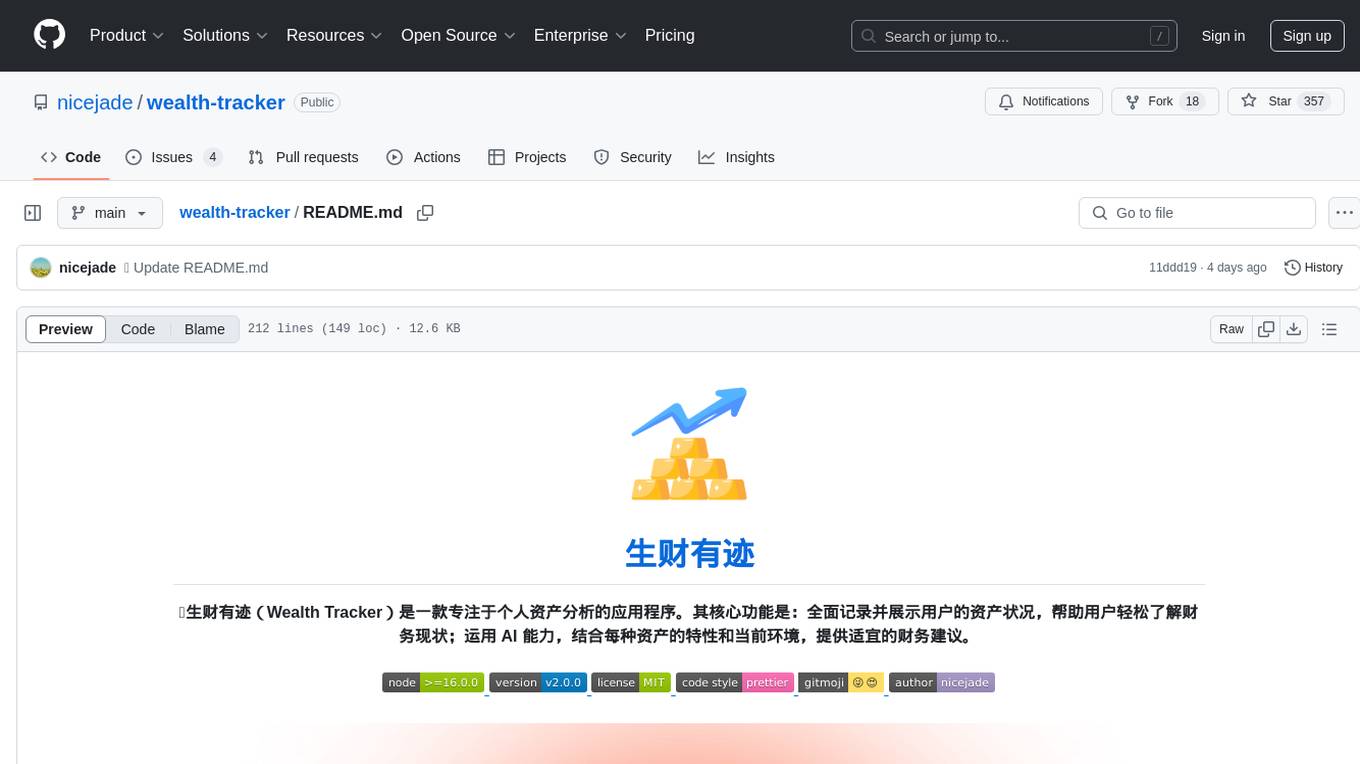
wealth-tracker
Wealth Tracker is a personal finance management tool designed to help users track their income, expenses, and investments in one place. With intuitive features and customizable categories, users can easily monitor their financial health and make informed decisions. The tool provides detailed reports and visualizations to analyze spending patterns and set financial goals. Whether you are budgeting, saving for a big purchase, or planning for retirement, Wealth Tracker offers a comprehensive solution to manage your money effectively.
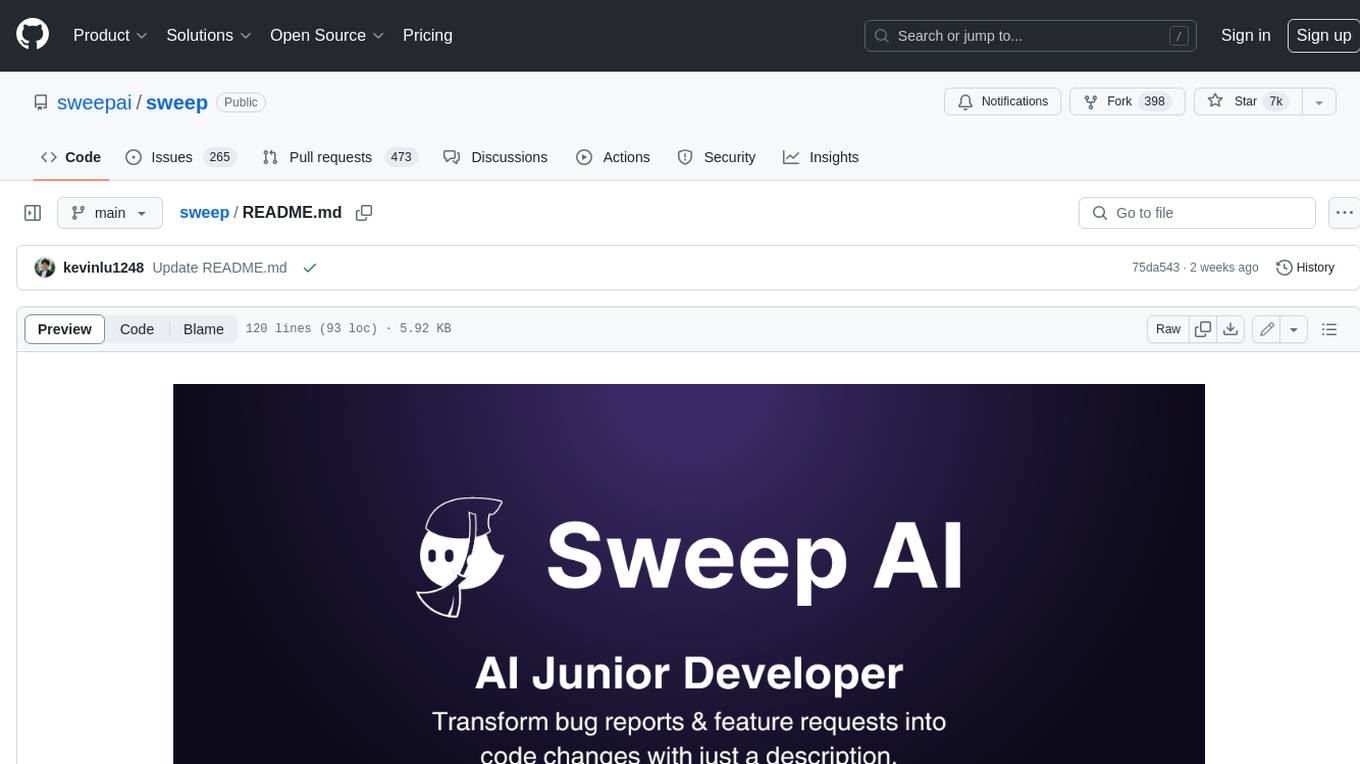
sweep
Sweep is an AI junior developer that turns bugs and feature requests into code changes. It automatically handles developer experience improvements like adding type hints and improving test coverage.
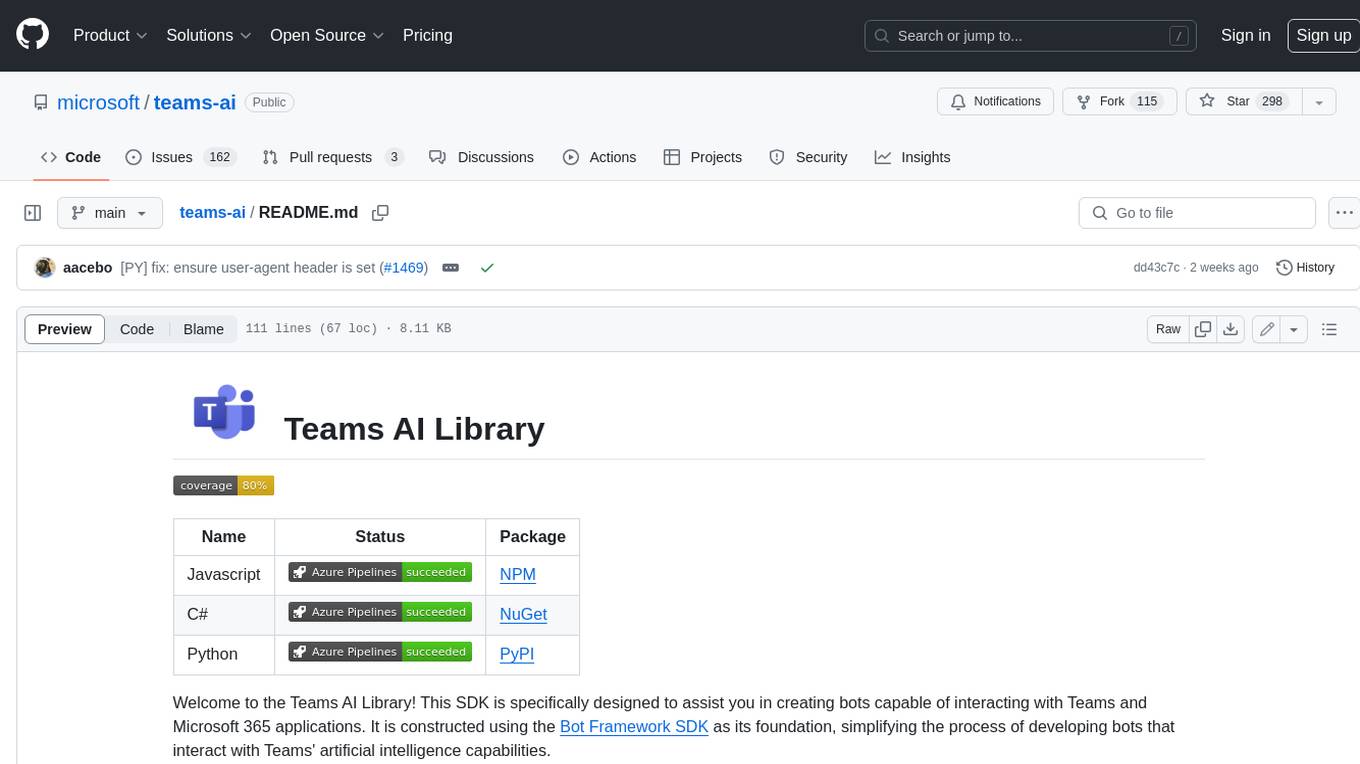
teams-ai
The Teams AI Library is a software development kit (SDK) that helps developers create bots that can interact with Teams and Microsoft 365 applications. It is built on top of the Bot Framework SDK and simplifies the process of developing bots that interact with Teams' artificial intelligence capabilities. The SDK is available for JavaScript/TypeScript, .NET, and Python.