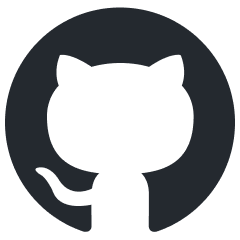
mistral-inference
Official inference library for Mistral models
Stars: 10114
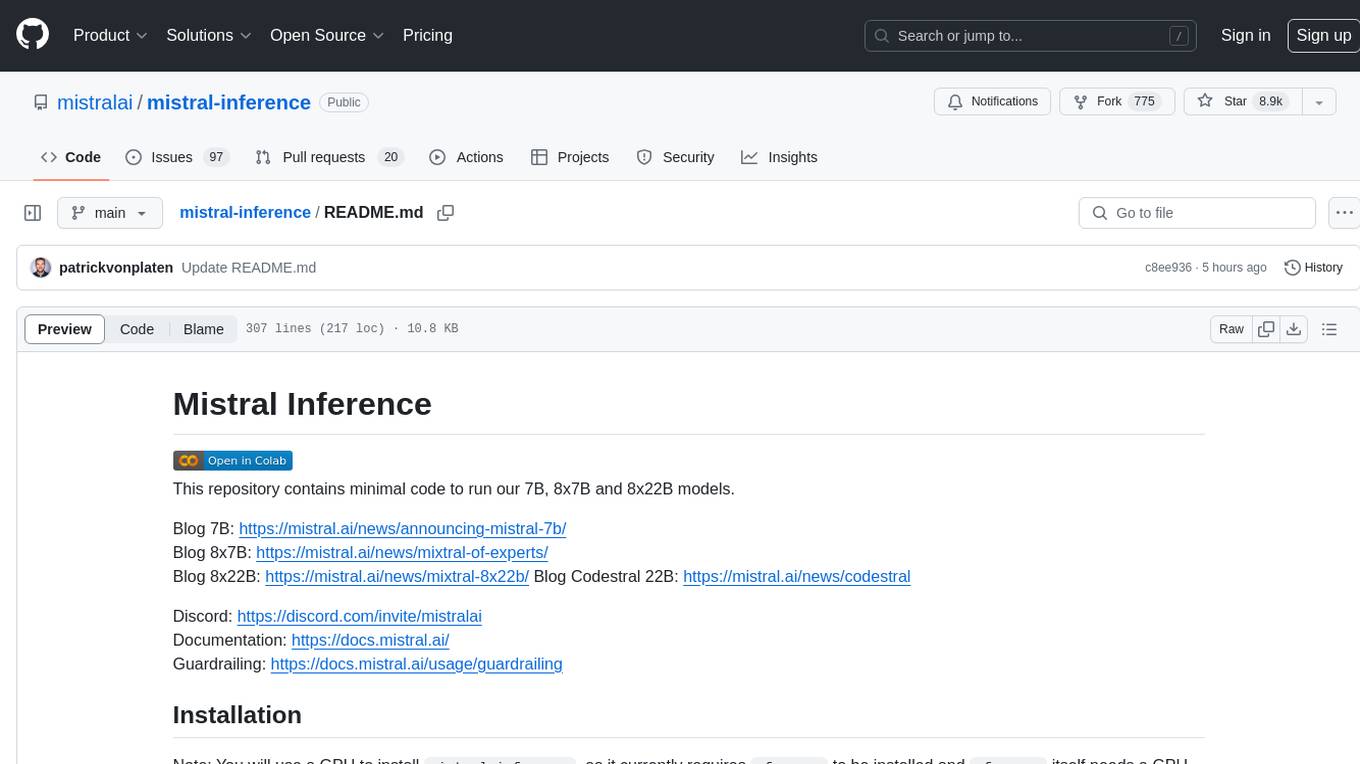
Mistral Inference repository contains minimal code to run 7B, 8x7B, and 8x22B models. It provides model download links, installation instructions, and usage guidelines for running models via CLI or Python. The repository also includes information on guardrailing, model platforms, deployment, and references. Users can interact with models through commands like mistral-demo, mistral-chat, and mistral-common. Mistral AI models support function calling and chat interactions for tasks like testing models, chatting with models, and using Codestral as a coding assistant. The repository offers detailed documentation and links to blogs for further information.
README:
This repository contains minimal code to run Mistral models.
Blog 7B: https://mistral.ai/news/announcing-mistral-7b/
Blog 8x7B: https://mistral.ai/news/mixtral-of-experts/
Blog 8x22B: https://mistral.ai/news/mixtral-8x22b/
Blog Codestral 22B: https://mistral.ai/news/codestral
Blog Codestral Mamba 7B: https://mistral.ai/news/codestral-mamba/
Blog Mathstral 7B: https://mistral.ai/news/mathstral/
Blog Nemo: https://mistral.ai/news/mistral-nemo/
Blog Mistral Large 2: https://mistral.ai/news/mistral-large-2407/
Blog Pixtral 12B: https://mistral.ai/news/pixtral-12b/
Blog Mistral Small 3.1: https://mistral.ai/news/mistral-small-3-1/
Discord: https://discord.com/invite/mistralai
Documentation: https://docs.mistral.ai/
Guardrailing: https://docs.mistral.ai/usage/guardrailing
Note: You will use a GPU to install mistral-inference
, as it currently requires xformers
to be installed and xformers
itself needs a GPU for installation.
pip install mistral-inference
cd $HOME && git clone https://github.com/mistralai/mistral-inference
cd $HOME/mistral-inference && poetry install .
Note:
-
Important:
-
mixtral-8x22B-Instruct-v0.3.tar
is exactly the same as Mixtral-8x22B-Instruct-v0.1, only stored in.safetensors
format -
mixtral-8x22B-v0.3.tar
is the same as Mixtral-8x22B-v0.1, but has an extended vocabulary of 32768 tokens. -
codestral-22B-v0.1.tar
has a custom non-commercial license, called Mistral AI Non-Production (MNPL) License -
mistral-large-instruct-2407.tar
has a custom non-commercial license, called Mistral AI Research (MRL) License
-
- All of the listed models above support function calling. For example, Mistral 7B Base/Instruct v3 is a minor update to Mistral 7B Base/Instruct v2, with the addition of function calling capabilities.
- The "coming soon" models will include function calling as well.
- You can download the previous versions of our models from our docs.
Name | ID | URL |
---|---|---|
Pixtral Large Instruct | mistralai/Pixtral-Large-Instruct-2411 | https://huggingface.co/mistralai/Pixtral-Large-Instruct-2411 |
Pixtral 12B Base | mistralai/Pixtral-12B-Base-2409 | https://huggingface.co/mistralai/Pixtral-12B-Base-2409 |
Pixtral 12B | mistralai/Pixtral-12B-2409 | https://huggingface.co/mistralai/Pixtral-12B-2409 |
Mistral Small 3.1 24B Base | mistralai/Mistral-Small-3.1-24B-Base-2503 | https://huggingface.co/mistralai/Mistral-Small-3.1-24B-Base-2503 |
Mistral Small 3.1 24B Instruct | mistralai/Mistral-Small-3.1-24B-Instruct-2503 | https://huggingface.co/mistralai/Mistral-Small-3.1-24B-Instruct-2503 |
News!!!: Mistral Large 2 is out. Read more about its capabilities here.
Create a local folder to store models
export MISTRAL_MODEL=$HOME/mistral_models
mkdir -p $MISTRAL_MODEL
Download any of the above links and extract the content, e.g.:
export 12B_DIR=$MISTRAL_MODEL/12B_Nemo
wget https://models.mistralcdn.com/mistral-nemo-2407/mistral-nemo-instruct-2407.tar
mkdir -p $12B_DIR
tar -xf mistral-nemo-instruct-2407.tar -C $12B_DIR
or
export M8x7B_DIR=$MISTRAL_MODEL/8x7b_instruct
wget https://models.mistralcdn.com/mixtral-8x7b-v0-1/Mixtral-8x7B-v0.1-Instruct.tar
mkdir -p $M8x7B_DIR
tar -xf Mixtral-8x7B-v0.1-Instruct.tar -C $M8x7B_DIR
For Hugging Face models' weights, here is an example to download Mistral Small 3.1 24B Instruct:
from pathlib import Path
from huggingface_hub import snapshot_download
mistral_models_path = Path.home().joinpath("mistral_models")
model_path = mistral_models_path / "mistral-small-3.1-instruct"
model_path.mkdir(parents=True, exist_ok=True)
repo_id = "mistralai/Mistral-Small-3.1-24B-Instruct-2503"
snapshot_download(
repo_id=repo_id,
allow_patterns=["params.json", "consolidated.safetensors", "tekken.json"],
local_dir=model_path,
)
The following sections give an overview of how to run the model from the Command-line interface (CLI) or directly within Python.
- Demo
To test that a model works in your setup, you can run the mistral-demo
command.
E.g. the 12B Mistral-Nemo model can be tested on a single GPU as follows:
mistral-demo $12B_DIR
Large models, such 8x7B and 8x22B have to be run in a multi-GPU setup. For these models, you can use the following command:
torchrun --nproc-per-node 2 --no-python mistral-demo $M8x7B_DIR
Note: Change --nproc-per-node
to more GPUs if available.
- Chat
To interactively chat with the models, you can make use of the mistral-chat
command.
mistral-chat $12B_DIR --instruct --max_tokens 1024 --temperature 0.35
For large models, you can make use of torchrun
.
torchrun --nproc-per-node 2 --no-python mistral-chat $M8x7B_DIR --instruct
Note: Change --nproc-per-node
to more GPUs if necessary (e.g. for 8x22B).
- Chat with Codestral
To use Codestral as a coding assistant you can run the following command using mistral-chat
.
Make sure $M22B_CODESTRAL
is set to a valid path to the downloaded codestral folder, e.g. $HOME/mistral_models/Codestral-22B-v0.1
mistral-chat $M22B_CODESTRAL --instruct --max_tokens 256
If you prompt it with "Write me a function that computes fibonacci in Rust", the model should generate something along the following lines:
Sure, here's a simple implementation of a function that computes the Fibonacci sequence in Rust. This function takes an integer `n` as an argument and returns the `n`th Fibonacci number.
fn fibonacci(n: u32) -> u32 {
match n {
0 => 0,
1 => 1,
_ => fibonacci(n - 1) + fibonacci(n - 2),
}
}
fn main() {
let n = 10;
println!("The {}th Fibonacci number is: {}", n, fibonacci(n));
}
This function uses recursion to calculate the Fibonacci number. However, it's not the most efficient solution because it performs a lot of redundant calculations. A more efficient solution would use a loop to iteratively calculate the Fibonacci numbers.
You can continue chatting afterwards, e.g. with "Translate it to Python".
- Chat with Codestral-Mamba
To use Codestral-Mamba as a coding assistant you can run the following command using mistral-chat
.
Make sure $7B_CODESTRAL_MAMBA
is set to a valid path to the downloaded codestral-mamba folder, e.g. $HOME/mistral_models/mamba-codestral-7B-v0.1
.
You then need to additionally install the following packages:
pip install packaging mamba-ssm causal-conv1d transformers
before you can start chatting:
mistral-chat $7B_CODESTRAL_MAMBA --instruct --max_tokens 256
- Chat with Mathstral
To use Mathstral as an assistant you can run the following command using mistral-chat
.
Make sure $7B_MATHSTRAL
is set to a valid path to the downloaded codestral folder, e.g. $HOME/mistral_models/mathstral-7B-v0.1
mistral-chat $7B_MATHSTRAL --instruct --max_tokens 256
If you prompt it with "Albert likes to surf every week. Each surfing session lasts for 4 hours and costs $20 per hour. How much would Albert spend in 5 weeks?", the model should answer with the correct calculation.
You can then continue chatting afterwards, e.g. with "How much would he spend in a year?".
- Chat with Mistral Small 3.1 24B Instruct
To use Mistral Small 3.1 24B Instruct as an assistant you can run the following command using mistral-chat
.
Make sure $MISTRAL_SMALL_3_1_INSTRUCT
is set to a valid path to the downloaded mistral small folder, e.g. $HOME/mistral_models/mistral-small-3.1-instruct
mistral-chat $MISTRAL_SMALL_3_1_INSTRUCT --instruct --max_tokens 256
If you prompt it with "The above image presents an image of which park ? Please give the hints to identify the park." with the following image URL https://huggingface.co/datasets/patrickvonplaten/random_img/resolve/main/yosemite.png, the model should answer with the Yosemite park and give hints to identify it.
You can then continue chatting afterwards, e.g. with "What is the name of the lake in the image?". The model should respond that it is not a lake but a river.
- Instruction Following:
from mistral_inference.transformer import Transformer
from mistral_inference.generate import generate
from mistral_common.tokens.tokenizers.mistral import MistralTokenizer
from mistral_common.protocol.instruct.messages import UserMessage
from mistral_common.protocol.instruct.request import ChatCompletionRequest
tokenizer = MistralTokenizer.from_file("./mistral-nemo-instruct-v0.1/tekken.json") # change to extracted tokenizer file
model = Transformer.from_folder("./mistral-nemo-instruct-v0.1") # change to extracted model dir
prompt = "How expensive would it be to ask a window cleaner to clean all windows in Paris. Make a reasonable guess in US Dollar."
completion_request = ChatCompletionRequest(messages=[UserMessage(content=prompt)])
tokens = tokenizer.encode_chat_completion(completion_request).tokens
out_tokens, _ = generate([tokens], model, max_tokens=1024, temperature=0.35, eos_id=tokenizer.instruct_tokenizer.tokenizer.eos_id)
result = tokenizer.instruct_tokenizer.tokenizer.decode(out_tokens[0])
print(result)
- Multimodal Instruction Following:
from pathlib import Path
from huggingface_hub import snapshot_download
from mistral_common.protocol.instruct.messages import ImageURLChunk, TextChunk
from mistral_common.tokens.tokenizers.mistral import MistralTokenizer
from mistral_inference.generate import generate
from mistral_inference.transformer import Transformer
model_path = Path.home().joinpath("mistral_models") / "mistral-small-3.1-instruct" # change to extracted model
tokenizer = MistralTokenizer.from_file(model_path / "tekken.json")
model = Transformer.from_folder(model_path)
url = "https://huggingface.co/datasets/patrickvonplaten/random_img/resolve/main/yosemite.png"
prompt = "The above image presents an image of which park ? Please give the hints to identify the park."
user_content = [ImageURLChunk(image_url=url), TextChunk(text=prompt)]
tokens, images = tokenizer.instruct_tokenizer.encode_user_content(user_content, False)
out_tokens, _ = generate(
[tokens],
model,
images=[images],
max_tokens=256,
temperature=0.15,
eos_id=tokenizer.instruct_tokenizer.tokenizer.eos_id,
)
result = tokenizer.decode(out_tokens[0])
print("Prompt:", prompt)
print("Completion:", result)
- Function Calling:
from mistral_common.protocol.instruct.tool_calls import Function, Tool
completion_request = ChatCompletionRequest(
tools=[
Tool(
function=Function(
name="get_current_weather",
description="Get the current weather",
parameters={
"type": "object",
"properties": {
"location": {
"type": "string",
"description": "The city and state, e.g. San Francisco, CA",
},
"format": {
"type": "string",
"enum": ["celsius", "fahrenheit"],
"description": "The temperature unit to use. Infer this from the users location.",
},
},
"required": ["location", "format"],
},
)
)
],
messages=[
UserMessage(content="What's the weather like today in Paris?"),
],
)
tokens = tokenizer.encode_chat_completion(completion_request).tokens
out_tokens, _ = generate([tokens], model, max_tokens=64, temperature=0.0, eos_id=tokenizer.instruct_tokenizer.tokenizer.eos_id)
result = tokenizer.instruct_tokenizer.tokenizer.decode(out_tokens[0])
print(result)
- Fill-in-the-middle (FIM):
Make sure to have mistral-common >= 1.2.0
installed:
pip install --upgrade mistral-common
You can simulate a code completion in-filling as follows.
from mistral_inference.transformer import Transformer
from mistral_inference.generate import generate
from mistral_common.tokens.tokenizers.mistral import MistralTokenizer
from mistral_common.tokens.instruct.request import FIMRequest
tokenizer = MistralTokenizer.from_model("codestral-22b")
model = Transformer.from_folder("./mistral_22b_codestral")
prefix = """def add("""
suffix = """ return sum"""
request = FIMRequest(prompt=prefix, suffix=suffix)
tokens = tokenizer.encode_fim(request).tokens
out_tokens, _ = generate([tokens], model, max_tokens=256, temperature=0.0, eos_id=tokenizer.instruct_tokenizer.tokenizer.eos_id)
result = tokenizer.decode(out_tokens[0])
middle = result.split(suffix)[0].strip()
print(middle)
To run logits equivalence:
python -m pytest tests
The deploy
folder contains code to build a vLLM image with the required dependencies to serve the Mistral AI model. In the image, the transformers library is used instead of the reference implementation. To build it:
docker build deploy --build-arg MAX_JOBS=8
Instructions to run the image can be found in the official documentation.
- Use Mistral models on Mistral AI official API (La Plateforme)
- Use Mistral models via cloud providers
[1]: LoRA: Low-Rank Adaptation of Large Language Models, Hu et al. 2021
For Tasks:
Click tags to check more tools for each tasksFor Jobs:
Alternative AI tools for mistral-inference
Similar Open Source Tools
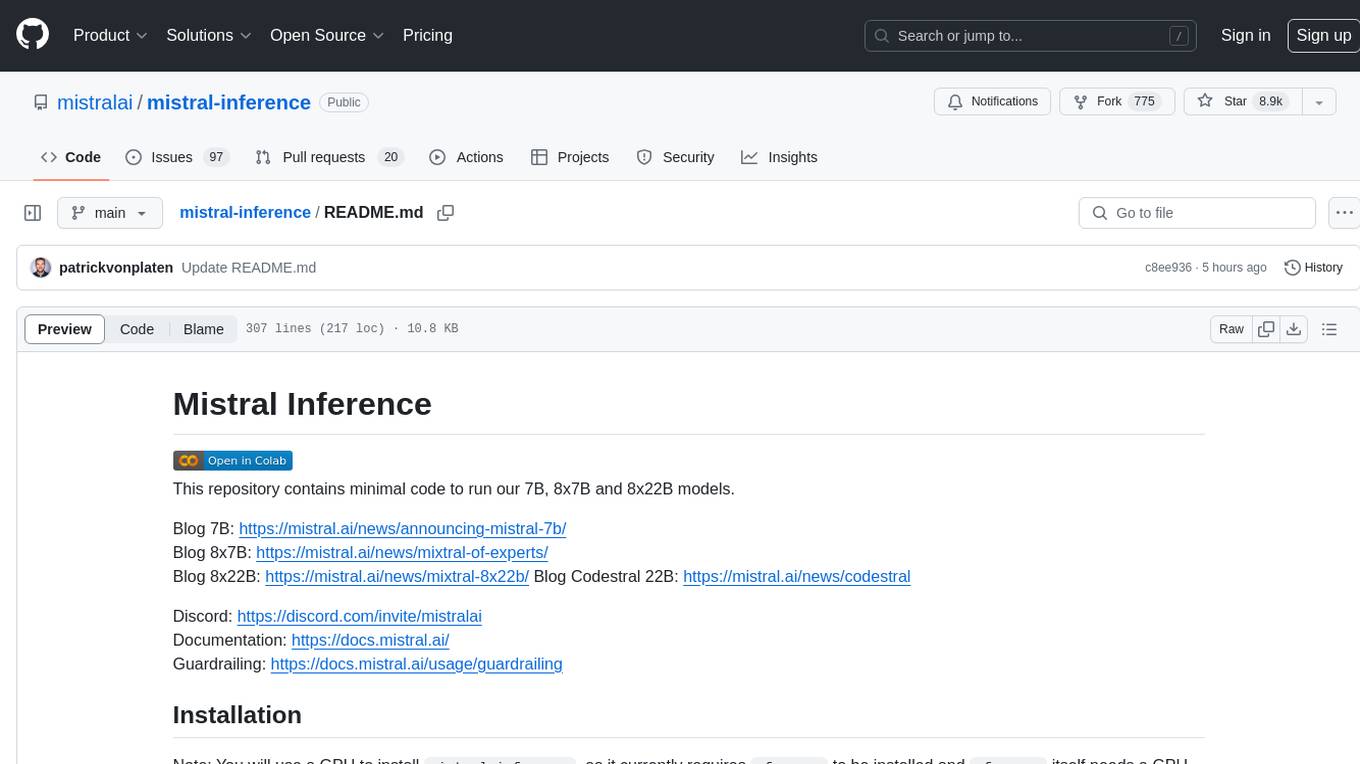
mistral-inference
Mistral Inference repository contains minimal code to run 7B, 8x7B, and 8x22B models. It provides model download links, installation instructions, and usage guidelines for running models via CLI or Python. The repository also includes information on guardrailing, model platforms, deployment, and references. Users can interact with models through commands like mistral-demo, mistral-chat, and mistral-common. Mistral AI models support function calling and chat interactions for tasks like testing models, chatting with models, and using Codestral as a coding assistant. The repository offers detailed documentation and links to blogs for further information.
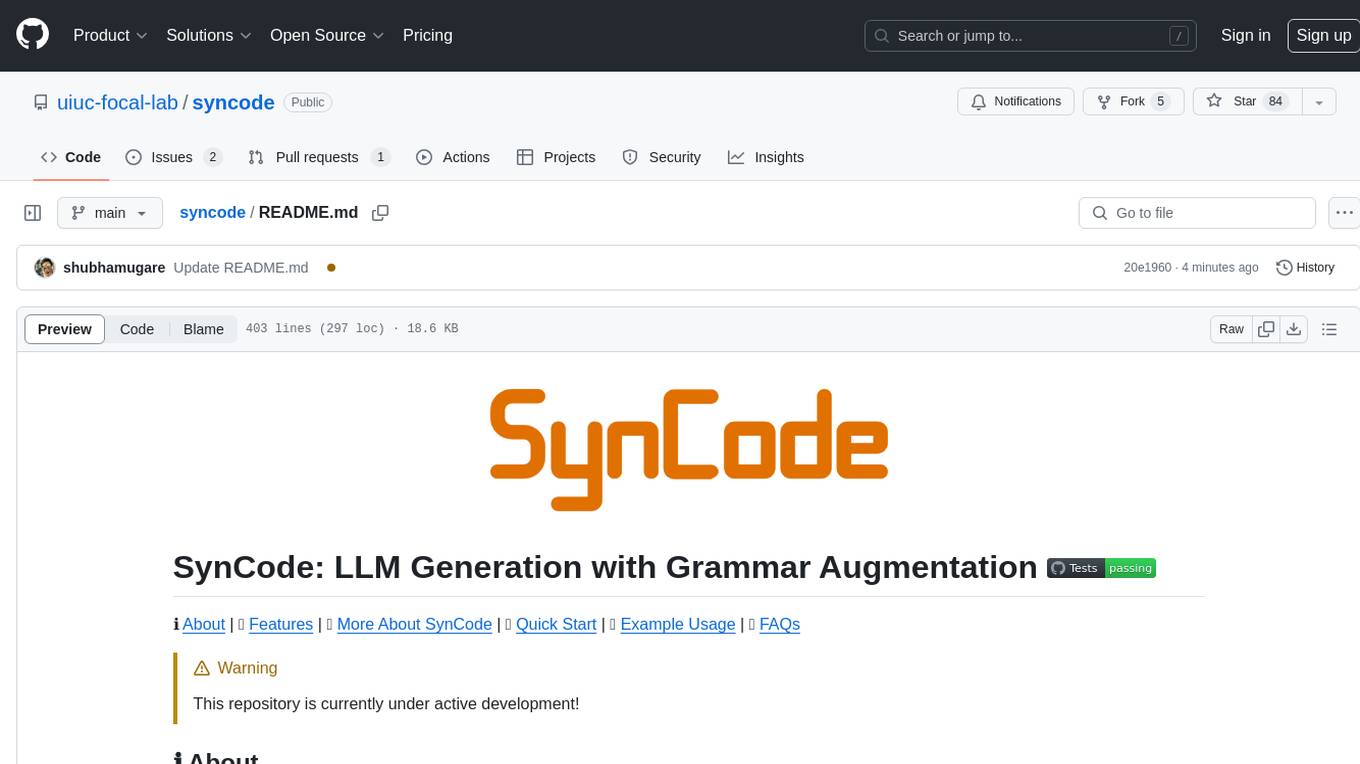
syncode
SynCode is a novel framework for the grammar-guided generation of Large Language Models (LLMs) that ensures syntactically valid output with respect to defined Context-Free Grammar (CFG) rules. It supports general-purpose programming languages like Python, Go, SQL, JSON, and more, allowing users to define custom grammars using EBNF syntax. The tool compares favorably to other constrained decoders and offers features like fast grammar-guided generation, compatibility with HuggingFace Language Models, and the ability to work with various decoding strategies.
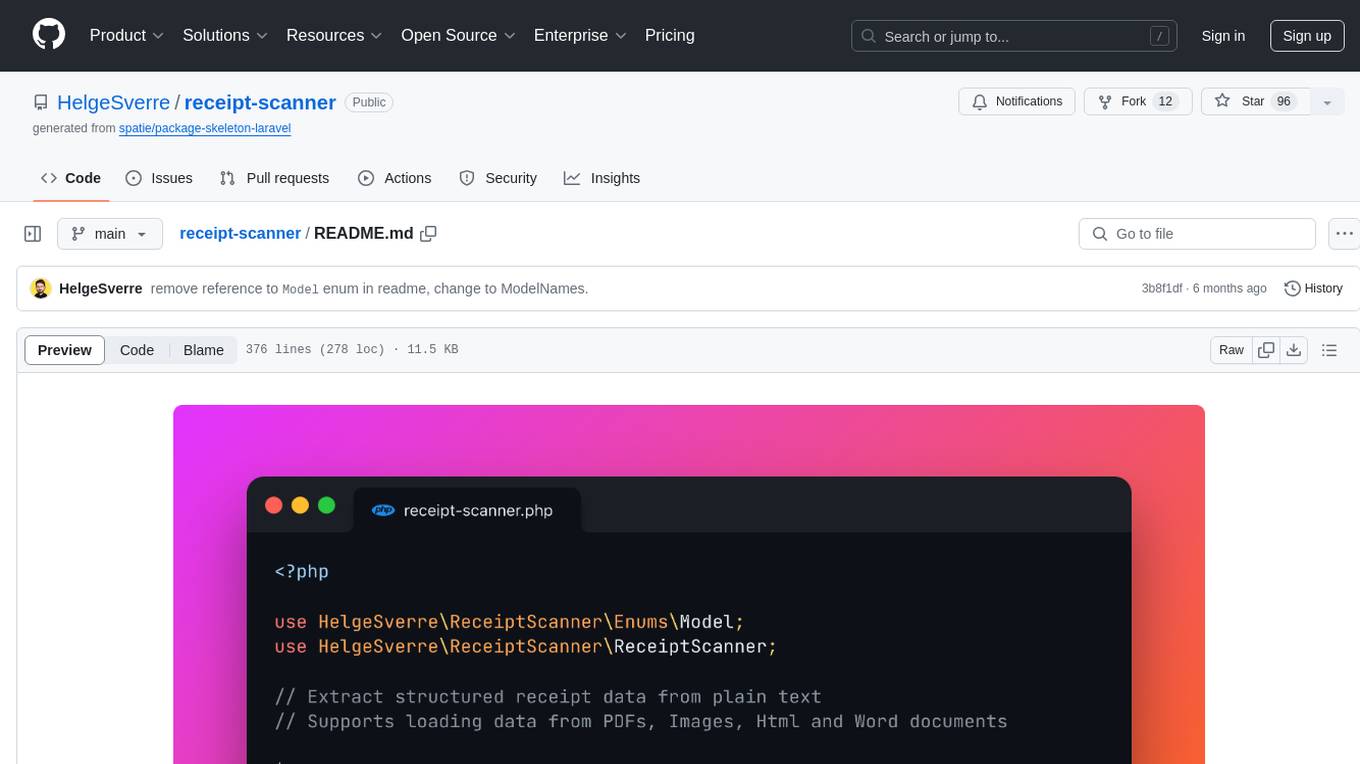
receipt-scanner
The receipt-scanner repository is an AI-Powered Receipt and Invoice Scanner for Laravel that allows users to easily extract structured receipt data from images, PDFs, and emails within their Laravel application using OpenAI. It provides a light wrapper around OpenAI Chat and Completion endpoints, supports various input formats, and integrates with Textract for OCR functionality. Users can install the package via composer, publish configuration files, and use it to extract data from plain text, PDFs, images, Word documents, and web content. The scanned receipt data is parsed into a DTO structure with main classes like Receipt, Merchant, and LineItem.
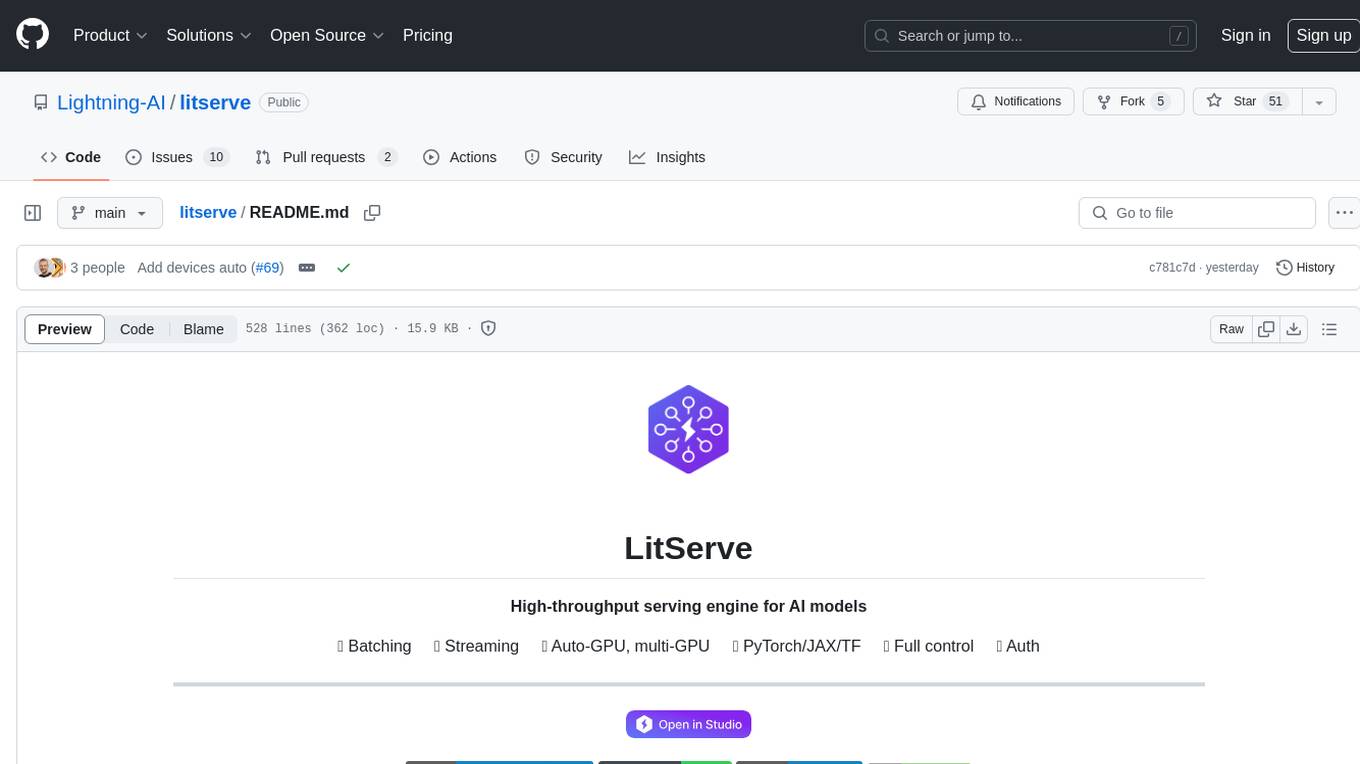
litserve
LitServe is a high-throughput serving engine for deploying AI models at scale. It generates an API endpoint for a model, handles batching, streaming, autoscaling across CPU/GPUs, and more. Built for enterprise scale, it supports every framework like PyTorch, JAX, Tensorflow, and more. LitServe is designed to let users focus on model performance, not the serving boilerplate. It is like PyTorch Lightning for model serving but with broader framework support and scalability.
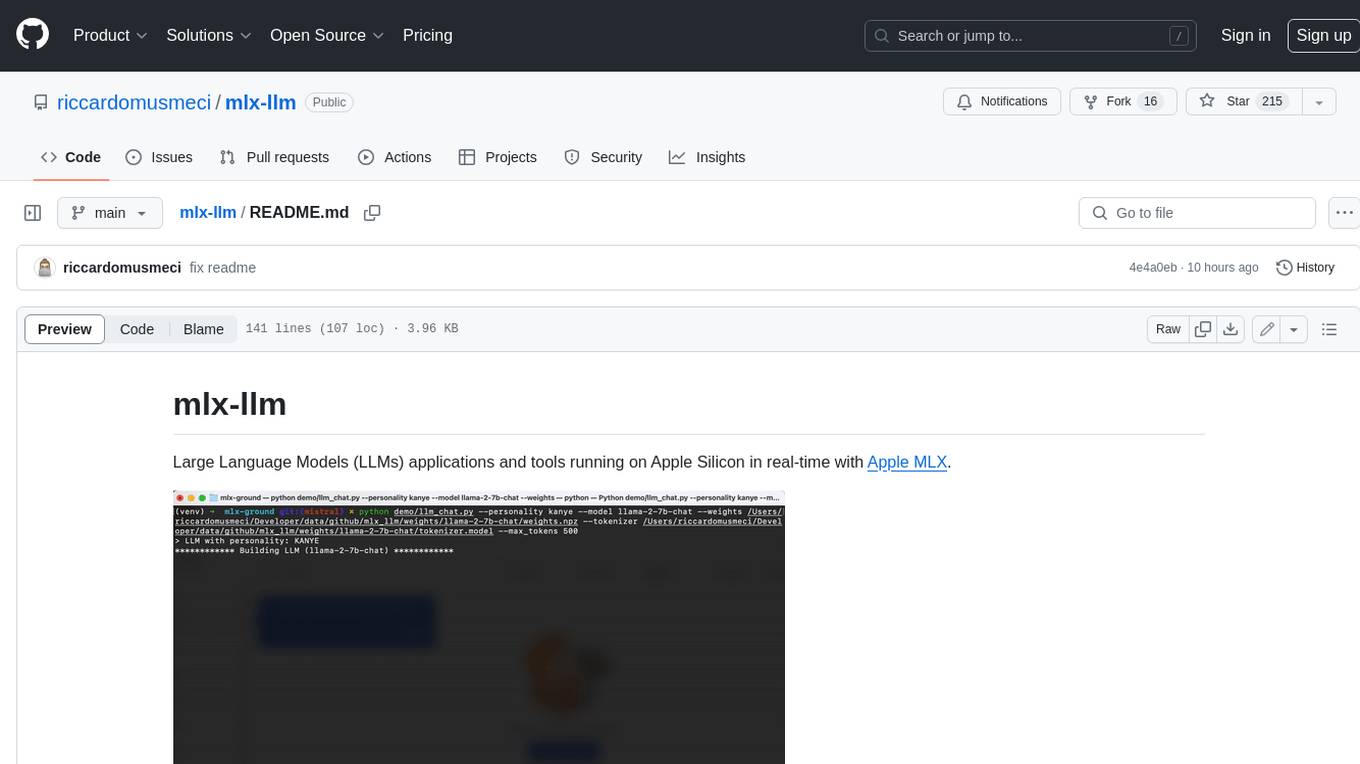
mlx-llm
mlx-llm is a library that allows you to run Large Language Models (LLMs) on Apple Silicon devices in real-time using Apple's MLX framework. It provides a simple and easy-to-use API for creating, loading, and using LLM models, as well as a variety of applications such as chatbots, fine-tuning, and retrieval-augmented generation.
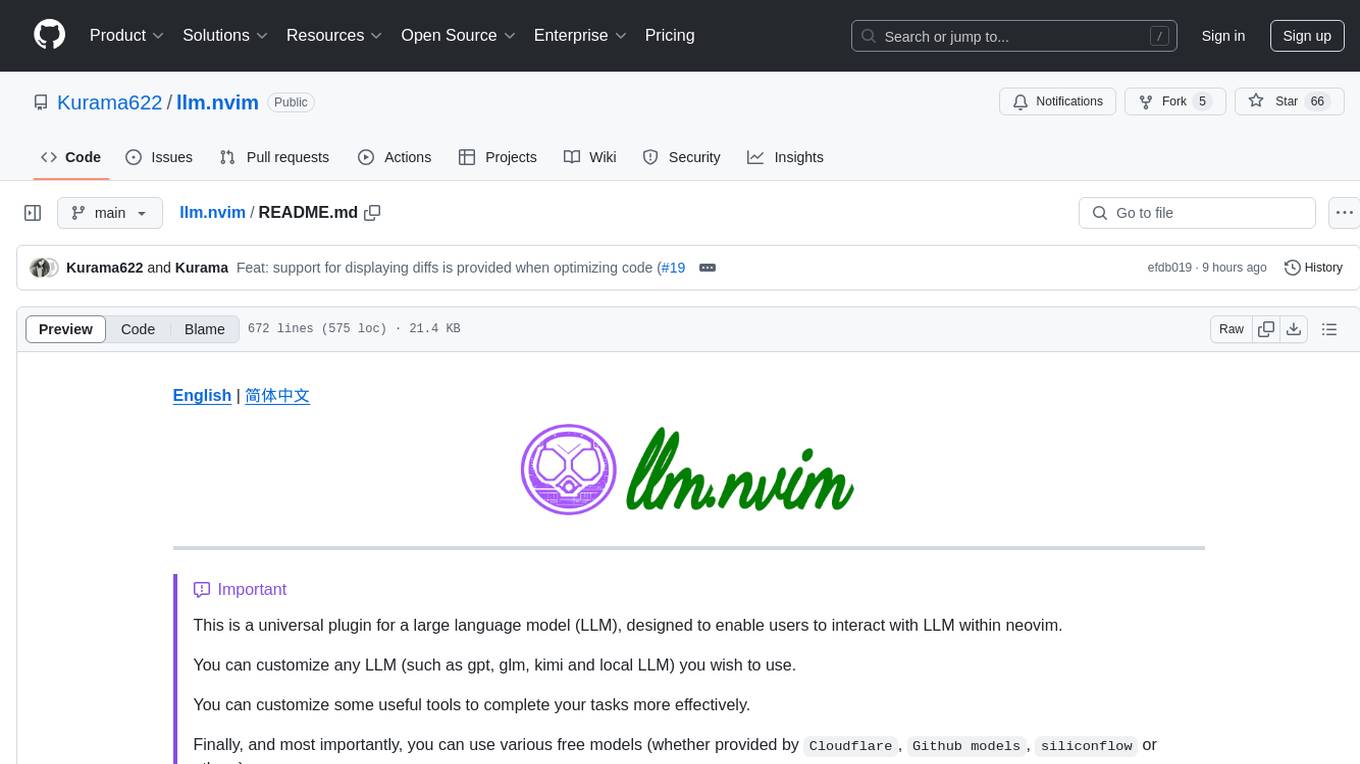
llm.nvim
llm.nvim is a universal plugin for a large language model (LLM) designed to enable users to interact with LLM within neovim. Users can customize various LLMs such as gpt, glm, kimi, and local LLM. The plugin provides tools for optimizing code, comparing code, translating text, and more. It also supports integration with free models from Cloudflare, Github models, siliconflow, and others. Users can customize tools, chat with LLM, quickly translate text, and explain code snippets. The plugin offers a flexible window interface for easy interaction and customization.
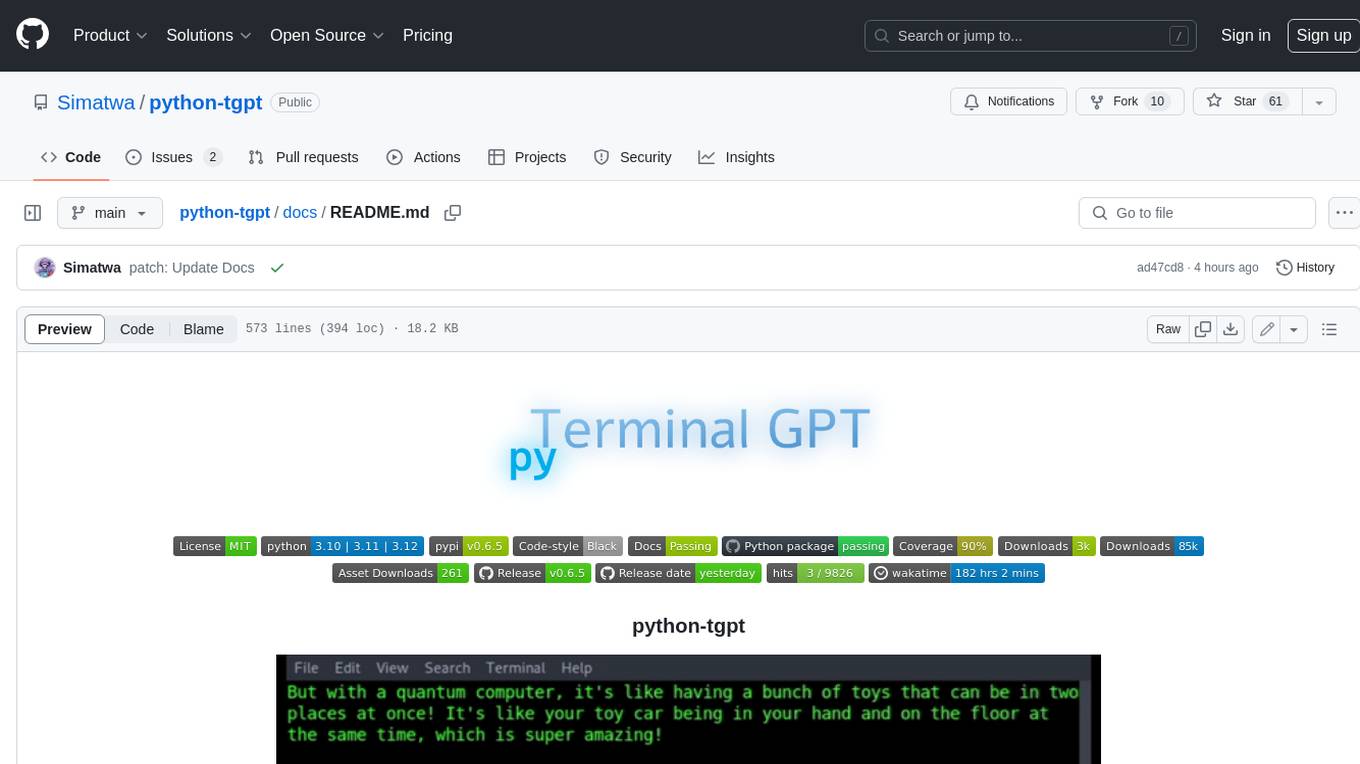
python-tgpt
Python-tgpt is a Python package that enables seamless interaction with over 45 free LLM providers without requiring an API key. It also provides image generation capabilities. The name _python-tgpt_ draws inspiration from its parent project tgpt, which operates on Golang. Through this Python adaptation, users can effortlessly engage with a number of free LLMs available, fostering a smoother AI interaction experience.
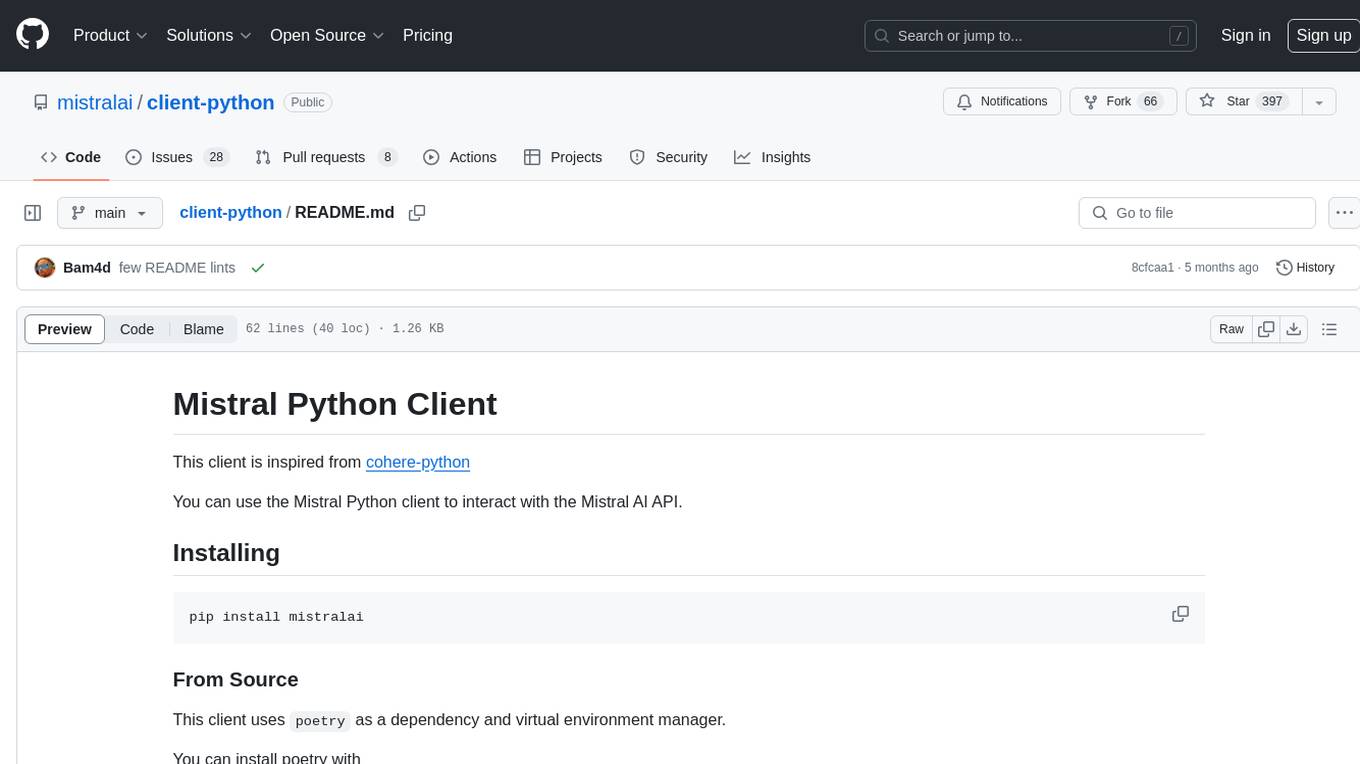
client-python
The Mistral Python Client is a tool inspired by cohere-python that allows users to interact with the Mistral AI API. It provides functionalities to access and utilize the AI capabilities offered by Mistral. Users can easily install the client using pip and manage dependencies using poetry. The client includes examples demonstrating how to use the API for various tasks, such as chat interactions. To get started, users need to obtain a Mistral API Key and set it as an environment variable. Overall, the Mistral Python Client simplifies the integration of Mistral AI services into Python applications.
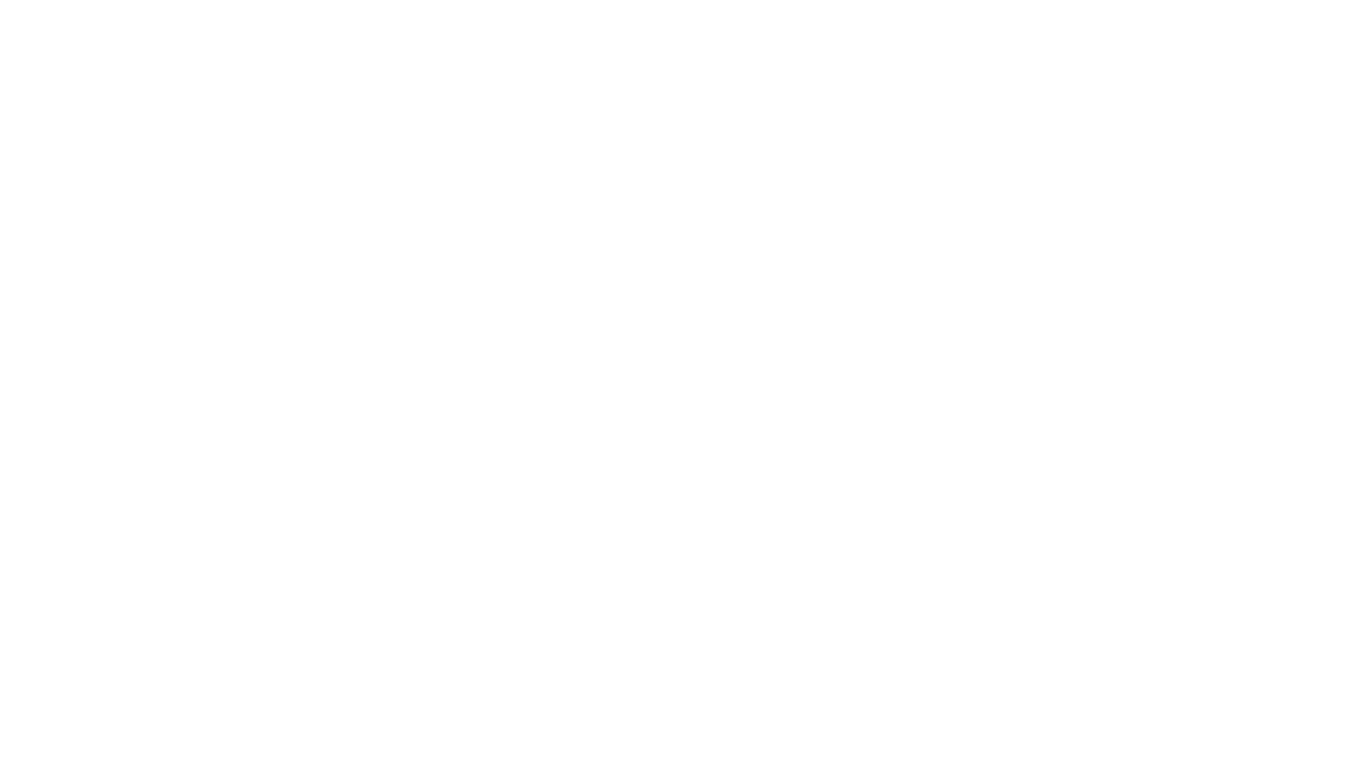
litdata
LitData is a tool designed for blazingly fast, distributed streaming of training data from any cloud storage. It allows users to transform and optimize data in cloud storage environments efficiently and intuitively, supporting various data types like images, text, video, audio, geo-spatial, and multimodal data. LitData integrates smoothly with frameworks such as LitGPT and PyTorch, enabling seamless streaming of data to multiple machines. Key features include multi-GPU/multi-node support, easy data mixing, pause & resume functionality, support for profiling, memory footprint reduction, cache size configuration, and on-prem optimizations. The tool also provides benchmarks for measuring streaming speed and conversion efficiency, along with runnable templates for different data types. LitData enables infinite cloud data processing by utilizing the Lightning.ai platform to scale data processing with optimized machines.
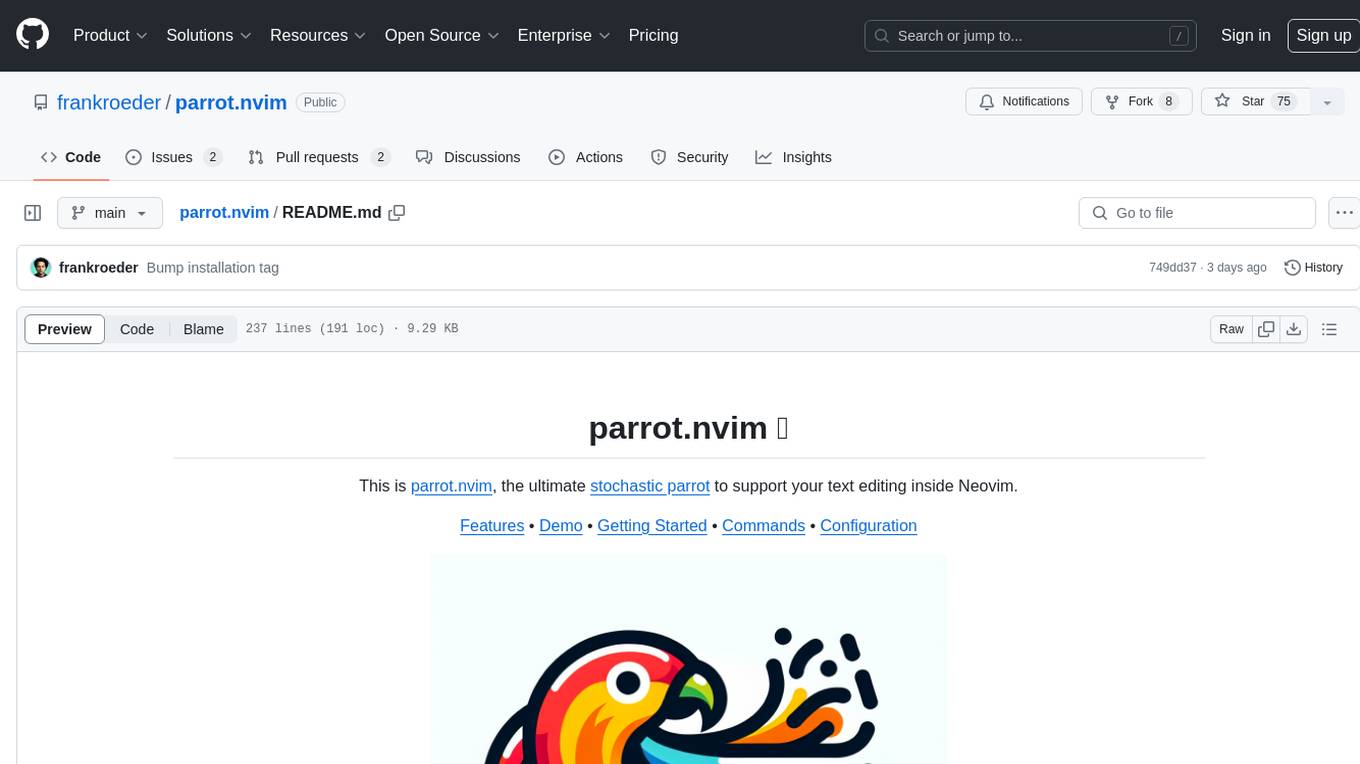
parrot.nvim
Parrot.nvim is a Neovim plugin that prioritizes a seamless out-of-the-box experience for text generation. It simplifies functionality and focuses solely on text generation, excluding integration of DALLE and Whisper. It supports persistent conversations as markdown files, custom hooks for inline text editing, multiple providers like Anthropic API, perplexity.ai API, OpenAI API, Mistral API, and local/offline serving via ollama. It allows custom agent definitions, flexible API credential support, and repository-specific instructions with a `.parrot.md` file. It does not have autocompletion or hidden requests in the background to analyze files.
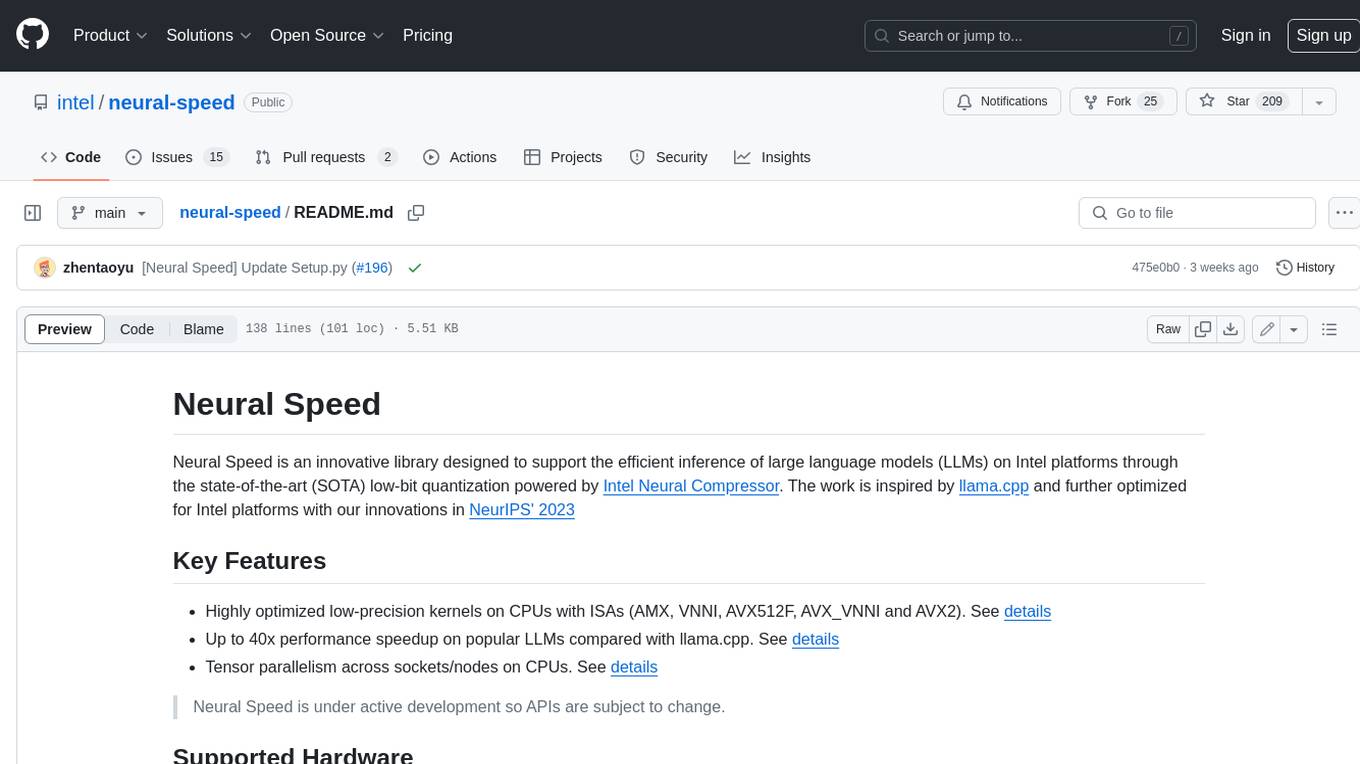
neural-speed
Neural Speed is an innovative library designed to support the efficient inference of large language models (LLMs) on Intel platforms through the state-of-the-art (SOTA) low-bit quantization powered by Intel Neural Compressor. The work is inspired by llama.cpp and further optimized for Intel platforms with our innovations in NeurIPS' 2023
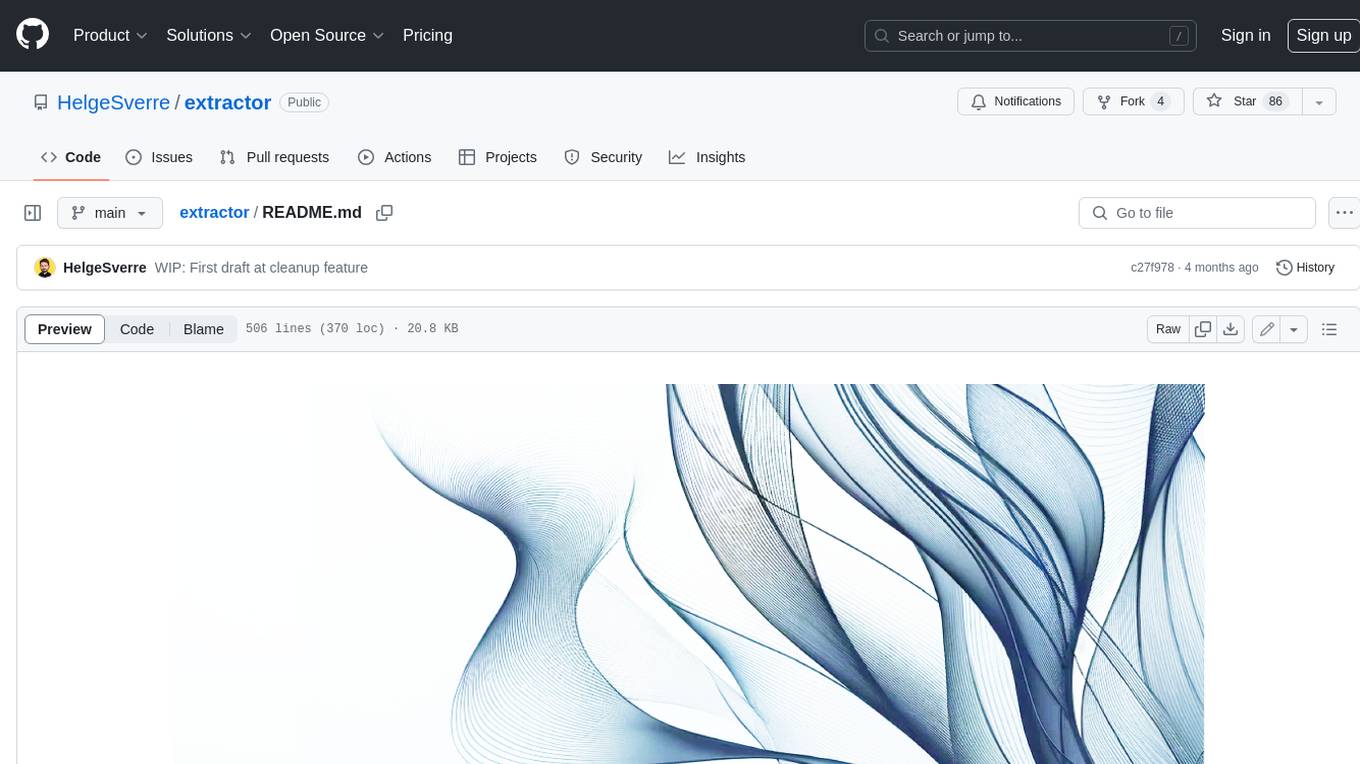
extractor
Extractor is an AI-powered data extraction library for Laravel that leverages OpenAI's capabilities to effortlessly extract structured data from various sources, including images, PDFs, and emails. It features a convenient wrapper around OpenAI Chat and Completion endpoints, supports multiple input formats, includes a flexible Field Extractor for arbitrary data extraction, and integrates with Textract for OCR functionality. Extractor utilizes JSON Mode from the latest GPT-3.5 and GPT-4 models, providing accurate and efficient data extraction.
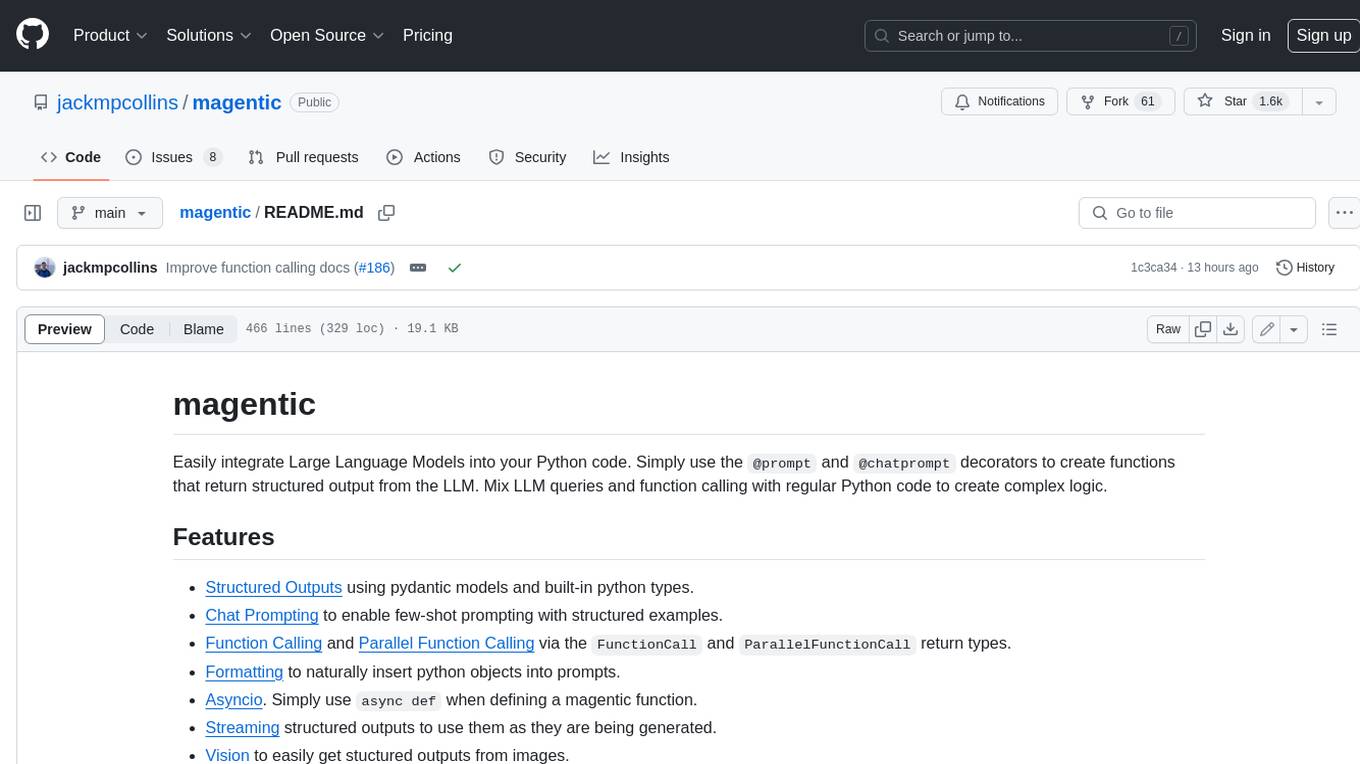
magentic
Easily integrate Large Language Models into your Python code. Simply use the `@prompt` and `@chatprompt` decorators to create functions that return structured output from the LLM. Mix LLM queries and function calling with regular Python code to create complex logic.
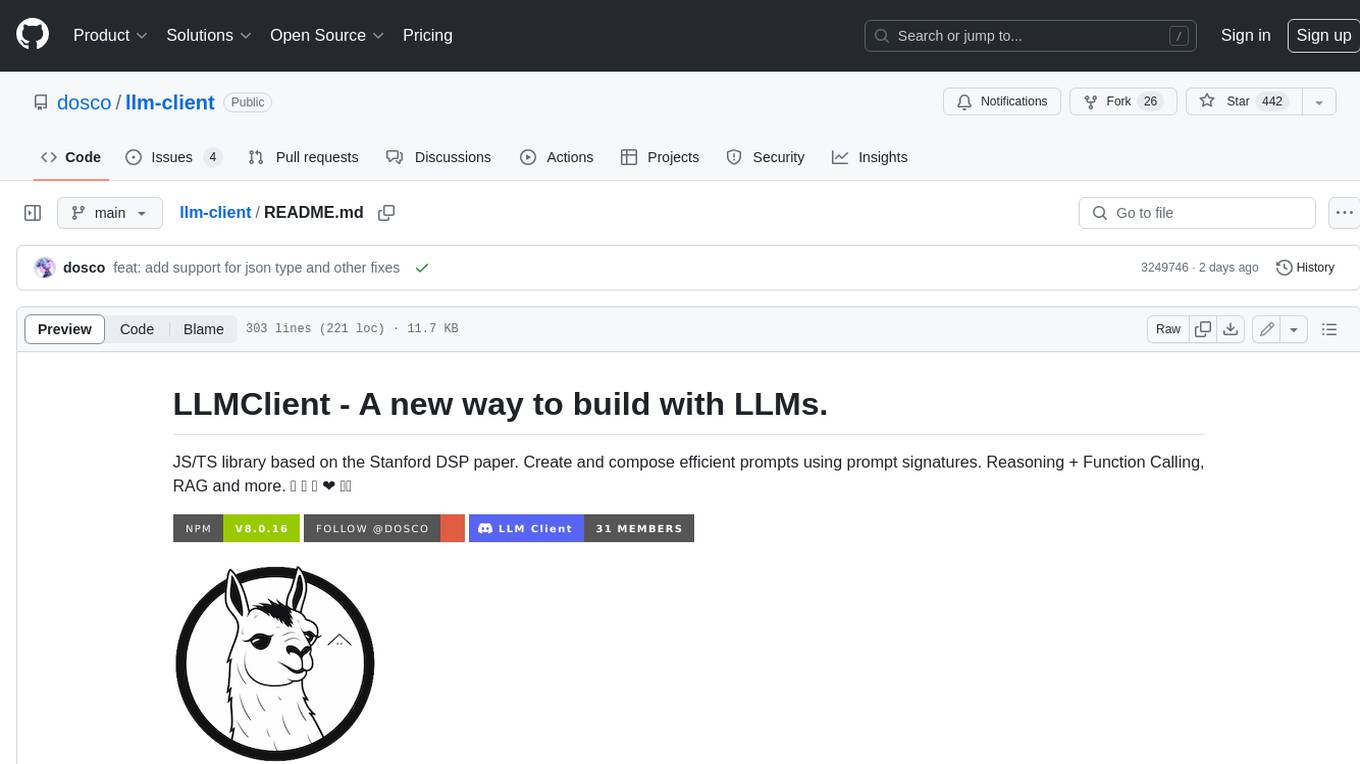
llm-client
LLMClient is a JavaScript/TypeScript library that simplifies working with large language models (LLMs) by providing an easy-to-use interface for building and composing efficient prompts using prompt signatures. These signatures enable the automatic generation of typed prompts, allowing developers to leverage advanced capabilities like reasoning, function calling, RAG, ReAcT, and Chain of Thought. The library supports various LLMs and vector databases, making it a versatile tool for a wide range of applications.
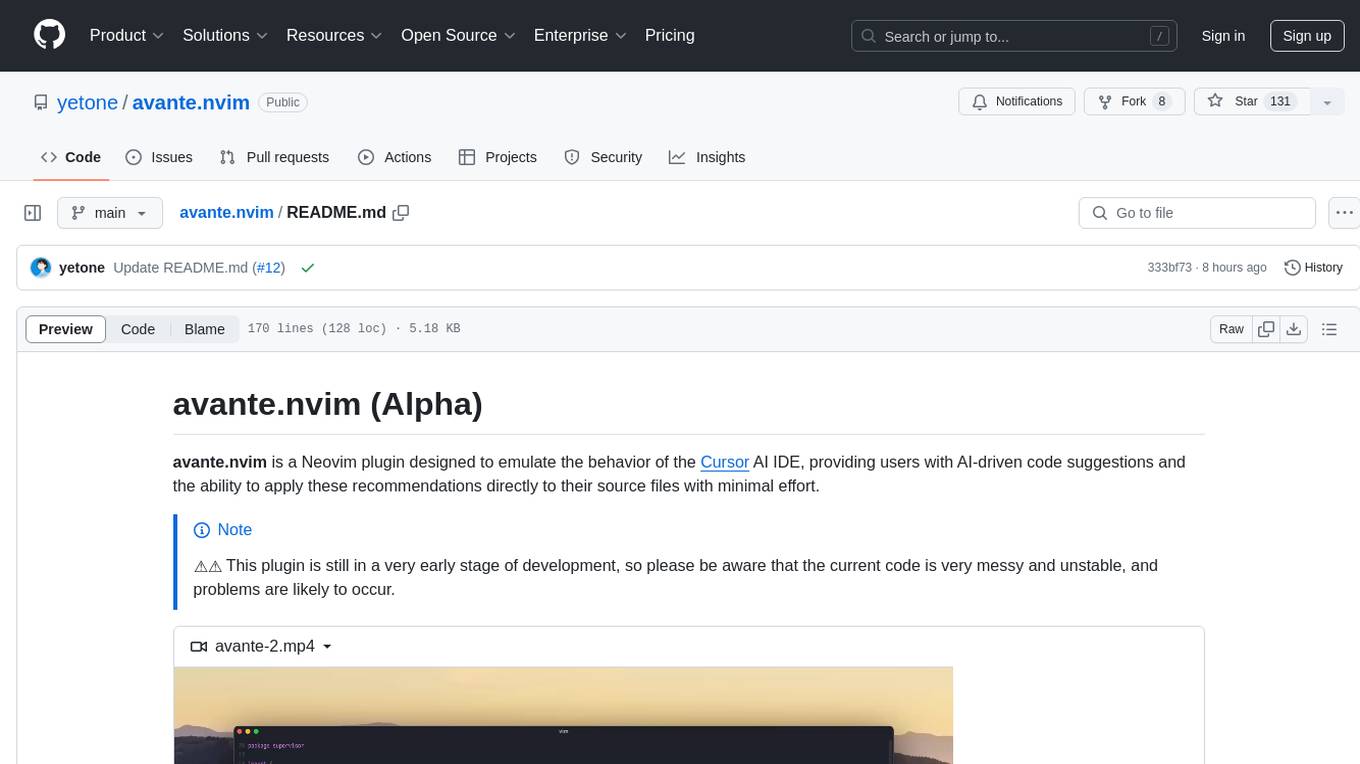
avante.nvim
avante.nvim is a Neovim plugin that emulates the behavior of the Cursor AI IDE, providing AI-driven code suggestions and enabling users to apply recommendations to their source files effortlessly. It offers AI-powered code assistance and one-click application of suggested changes, streamlining the editing process and saving time. The plugin is still in early development, with functionalities like setting API keys, querying AI about code, reviewing suggestions, and applying changes. Key bindings are available for various actions, and the roadmap includes enhancing AI interactions, stability improvements, and introducing new features for coding tasks.
For similar tasks
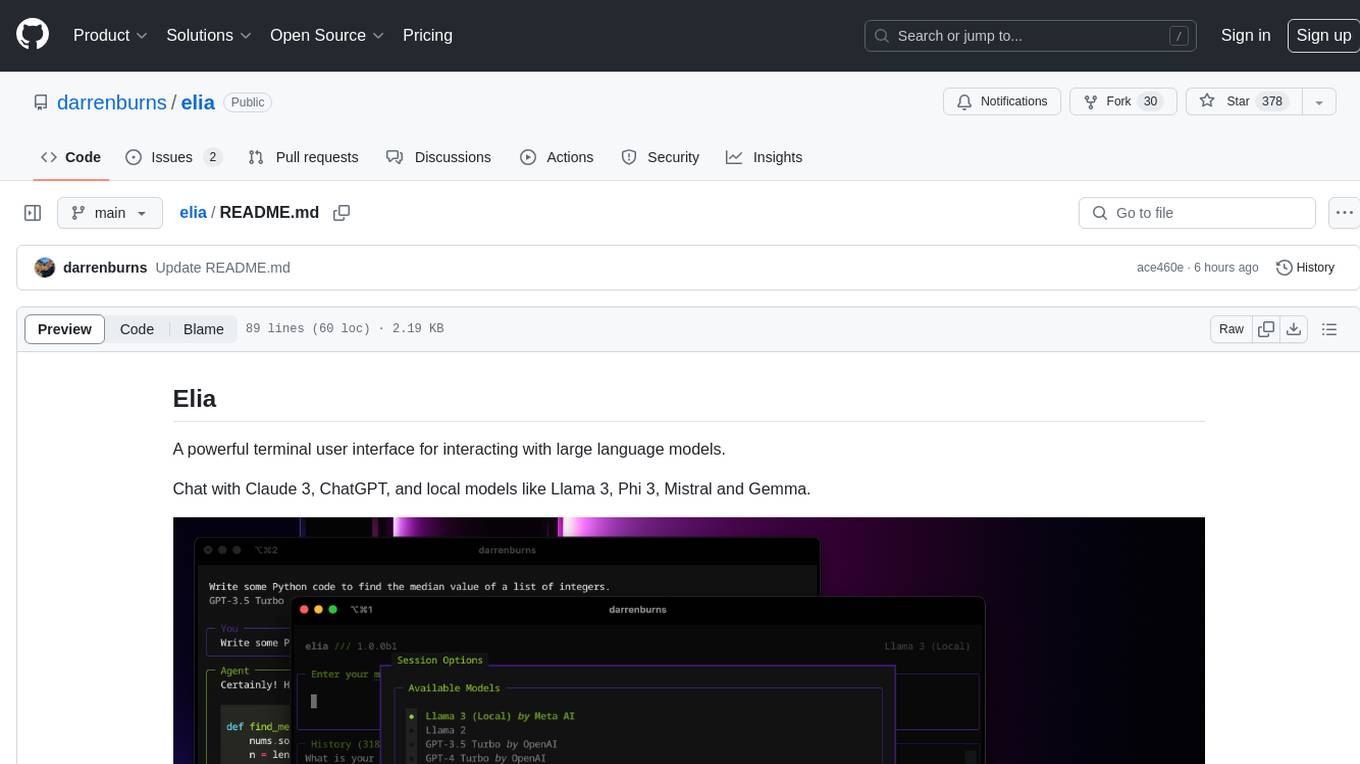
elia
Elia is a powerful terminal user interface designed for interacting with large language models. It allows users to chat with models like Claude 3, ChatGPT, Llama 3, Phi 3, Mistral, and Gemma. Conversations are stored locally in a SQLite database, ensuring privacy. Users can run local models through 'ollama' without data leaving their machine. Elia offers easy installation with pipx and supports various environment variables for different models. It provides a quick start to launch chats and manage local models. Configuration options are available to customize default models, system prompts, and add new models. Users can import conversations from ChatGPT and wipe the database when needed. Elia aims to enhance user experience in interacting with language models through a user-friendly interface.
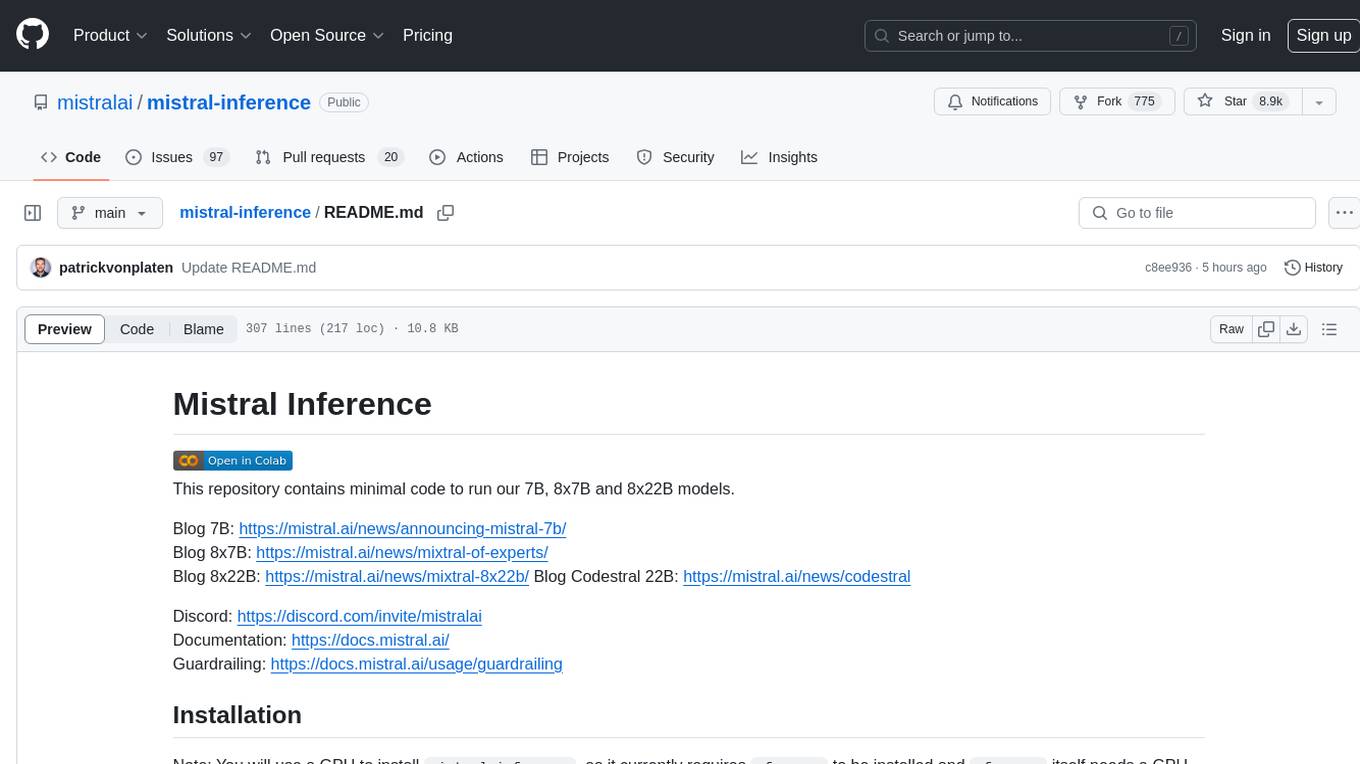
mistral-inference
Mistral Inference repository contains minimal code to run 7B, 8x7B, and 8x22B models. It provides model download links, installation instructions, and usage guidelines for running models via CLI or Python. The repository also includes information on guardrailing, model platforms, deployment, and references. Users can interact with models through commands like mistral-demo, mistral-chat, and mistral-common. Mistral AI models support function calling and chat interactions for tasks like testing models, chatting with models, and using Codestral as a coding assistant. The repository offers detailed documentation and links to blogs for further information.
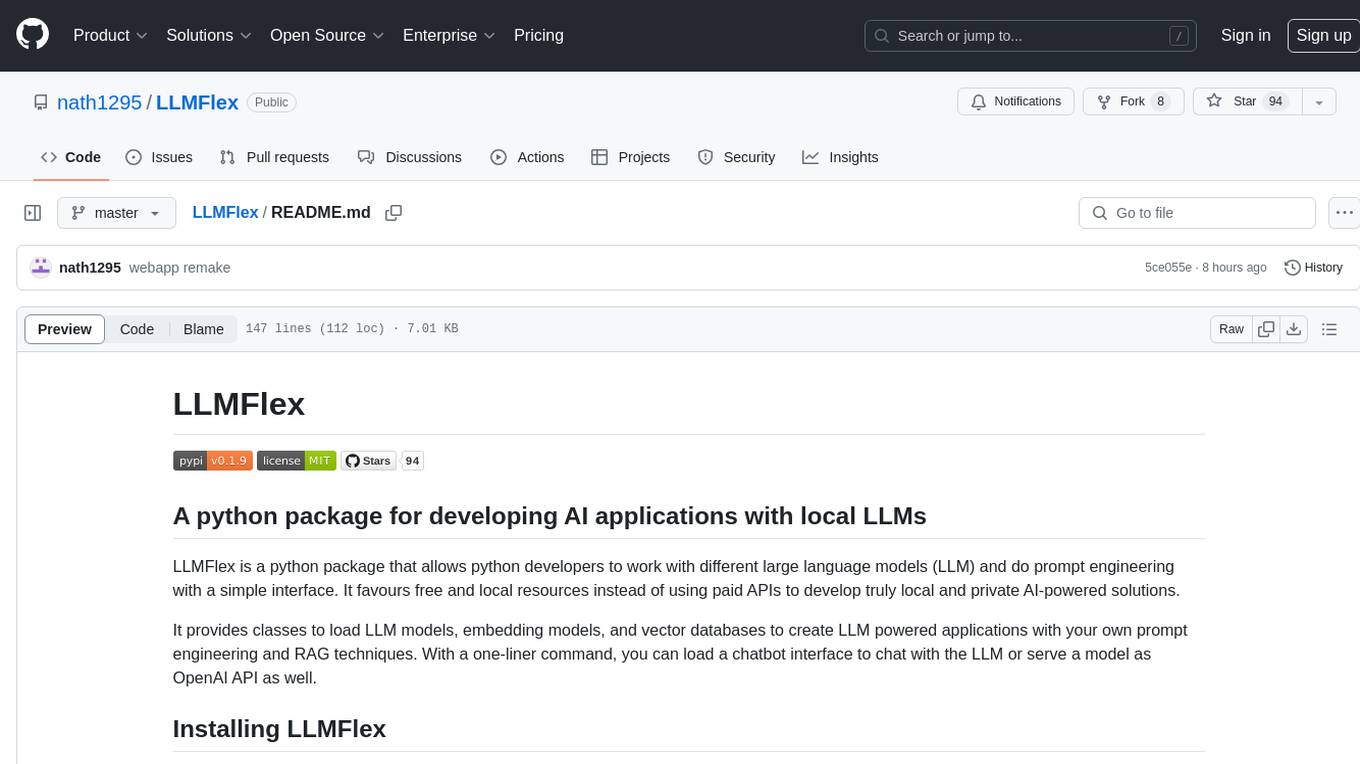
LLMFlex
LLMFlex is a python package designed for developing AI applications with local Large Language Models (LLMs). It provides classes to load LLM models, embedding models, and vector databases to create AI-powered solutions with prompt engineering and RAG techniques. The package supports multiple LLMs with different generation configurations, embedding toolkits, vector databases, chat memories, prompt templates, custom tools, and a chatbot frontend interface. Users can easily create LLMs, load embeddings toolkit, use tools, chat with models in a Streamlit web app, and serve an OpenAI API with a GGUF model. LLMFlex aims to offer a simple interface for developers to work with LLMs and build private AI solutions using local resources.
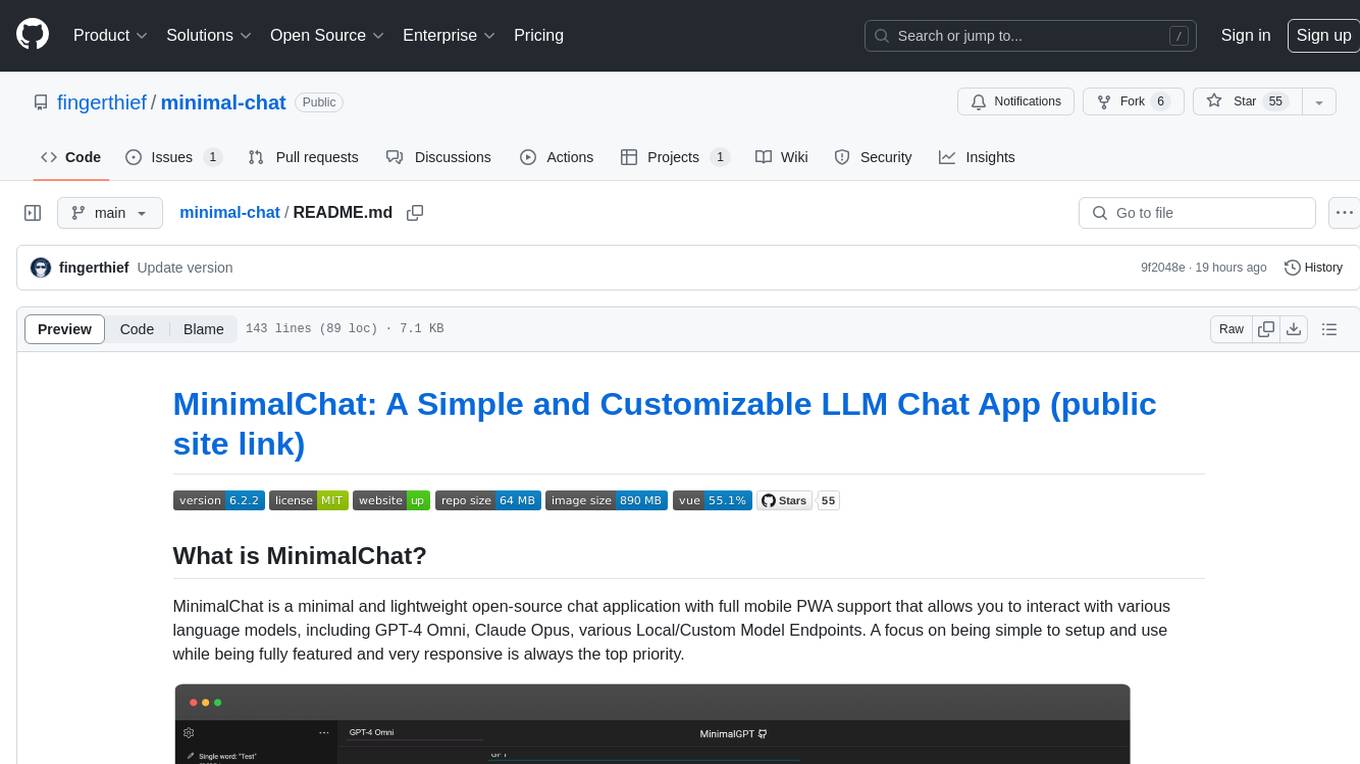
minimal-chat
MinimalChat is a minimal and lightweight open-source chat application with full mobile PWA support that allows users to interact with various language models, including GPT-4 Omni, Claude Opus, and various Local/Custom Model Endpoints. It focuses on simplicity in setup and usage while being fully featured and highly responsive. The application supports features like fully voiced conversational interactions, multiple language models, markdown support, code syntax highlighting, DALL-E 3 integration, conversation importing/exporting, and responsive layout for mobile use.
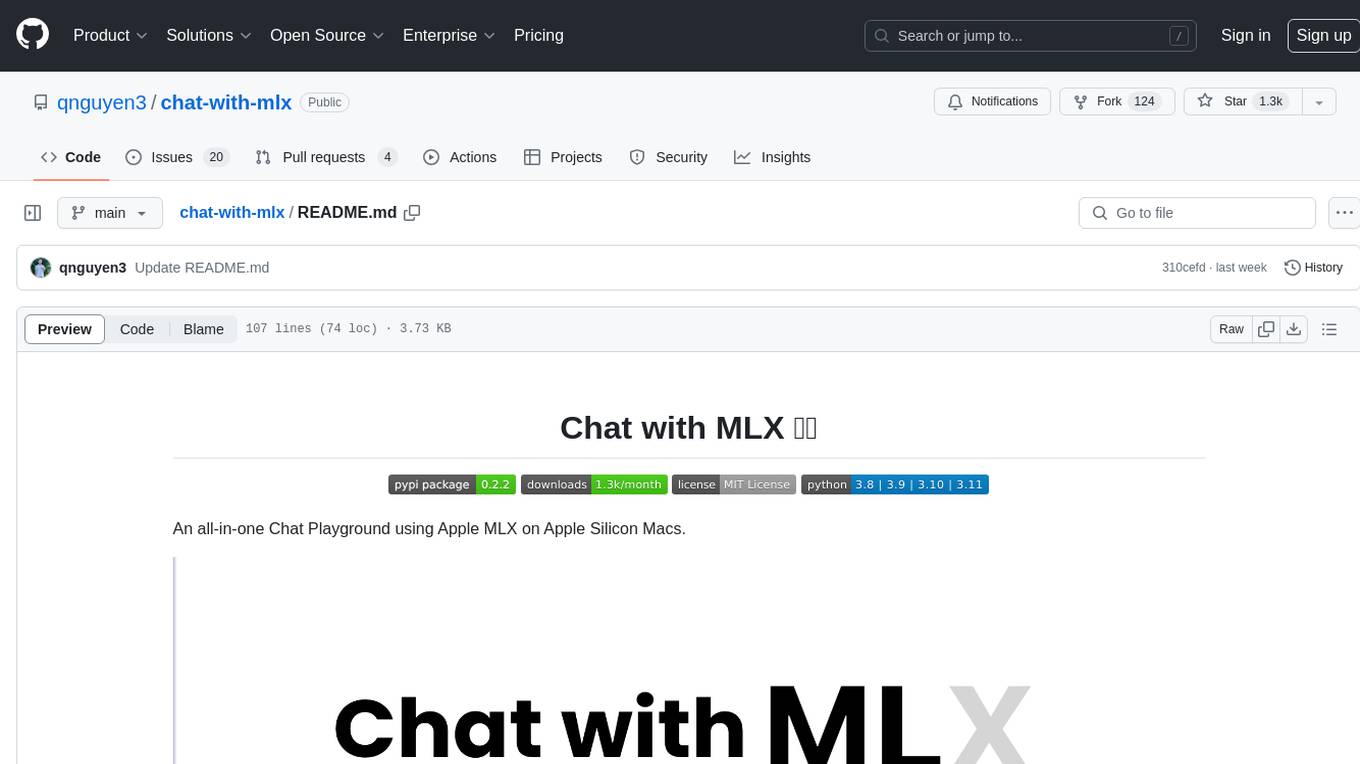
chat-with-mlx
Chat with MLX is an all-in-one Chat Playground using Apple MLX on Apple Silicon Macs. It provides privacy-enhanced AI for secure conversations with various models, easy integration of HuggingFace and MLX Compatible Open-Source Models, and comes with default models like Llama-3, Phi-3, Yi, Qwen, Mistral, Codestral, Mixtral, StableLM. The tool is designed for developers and researchers working with machine learning models on Apple Silicon.
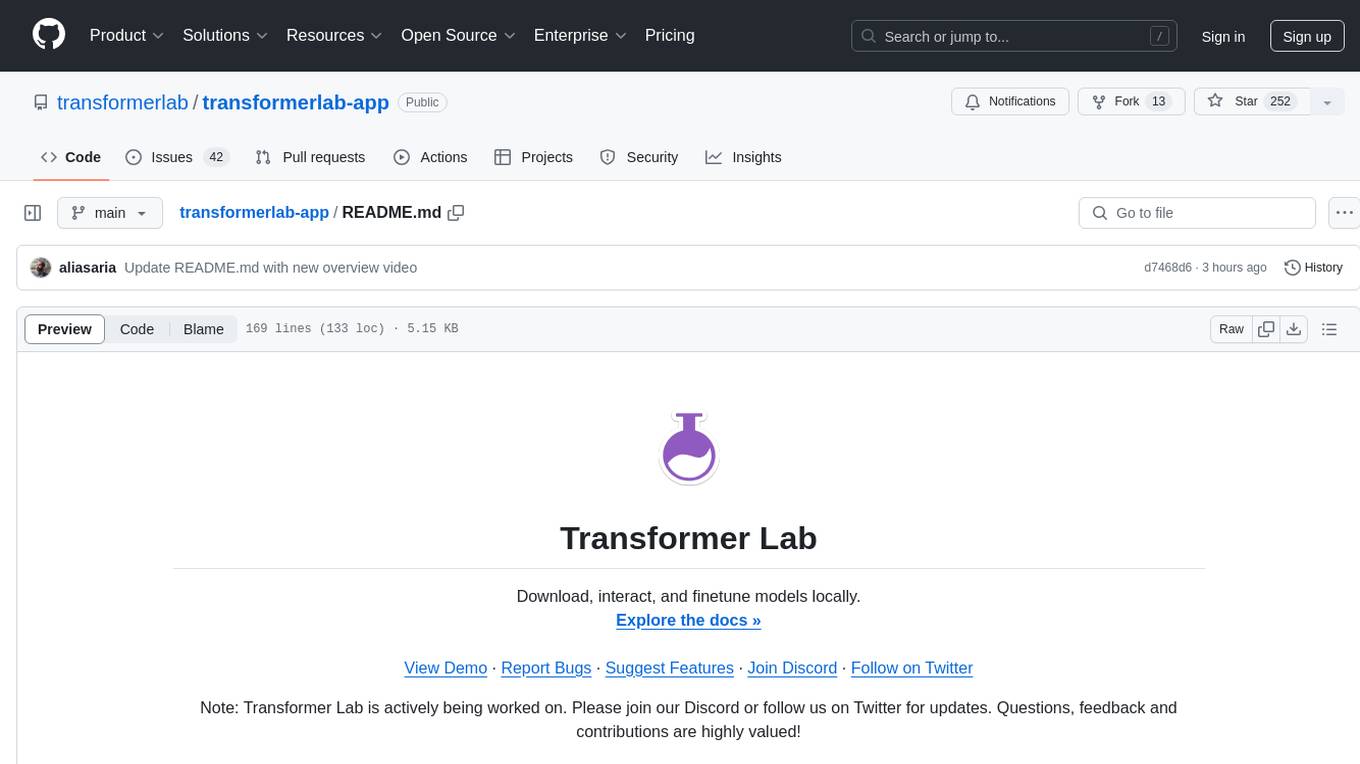
transformerlab-app
Transformer Lab is an app that allows users to experiment with Large Language Models by providing features such as one-click download of popular models, finetuning across different hardware, RLHF and Preference Optimization, working with LLMs across different operating systems, chatting with models, using different inference engines, evaluating models, building datasets for training, calculating embeddings, providing a full REST API, running in the cloud, converting models across platforms, supporting plugins, embedded Monaco code editor, prompt editing, inference logs, all through a simple cross-platform GUI.
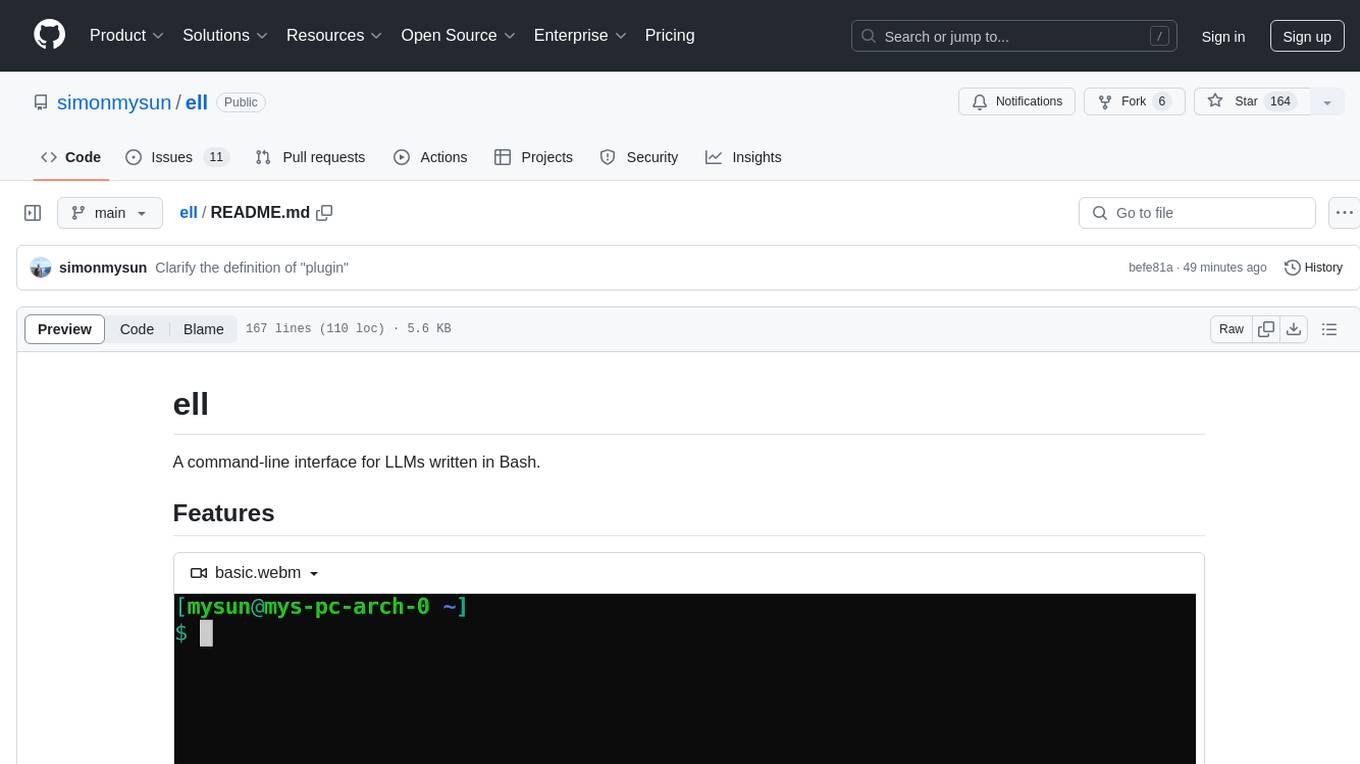
ell
ell is a command-line interface for Language Model Models (LLMs) written in Bash. It allows users to interact with LLMs from the terminal, supports piping, context bringing, and chatting with LLMs. Users can also call functions and use templates. The tool requires bash, jq for JSON parsing, curl for HTTPS requests, and perl for PCRE. Configuration involves setting variables for different LLM models and APIs. Usage examples include asking questions, specifying models, recording input/output, running in interactive mode, and using templates. The tool is lightweight, easy to install, and pipe-friendly, making it suitable for interacting with LLMs in a terminal environment.
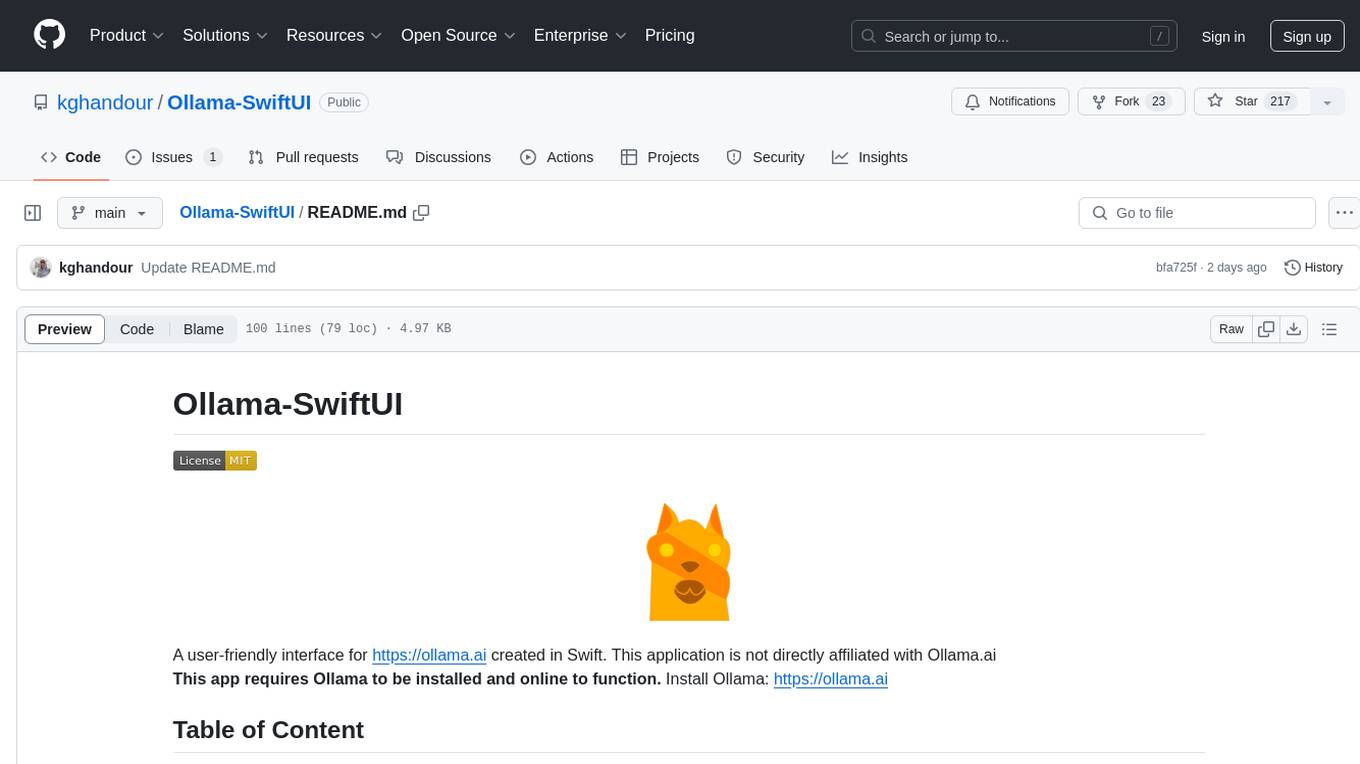
Ollama-SwiftUI
Ollama-SwiftUI is a user-friendly interface for Ollama.ai created in Swift. It allows seamless chatting with local Large Language Models on Mac. Users can change models mid-conversation, restart conversations, send system prompts, and use multimodal models with image + text. The app supports managing models, including downloading, deleting, and duplicating them. It offers light and dark mode, multiple conversation tabs, and a localized interface in English and Arabic.
For similar jobs
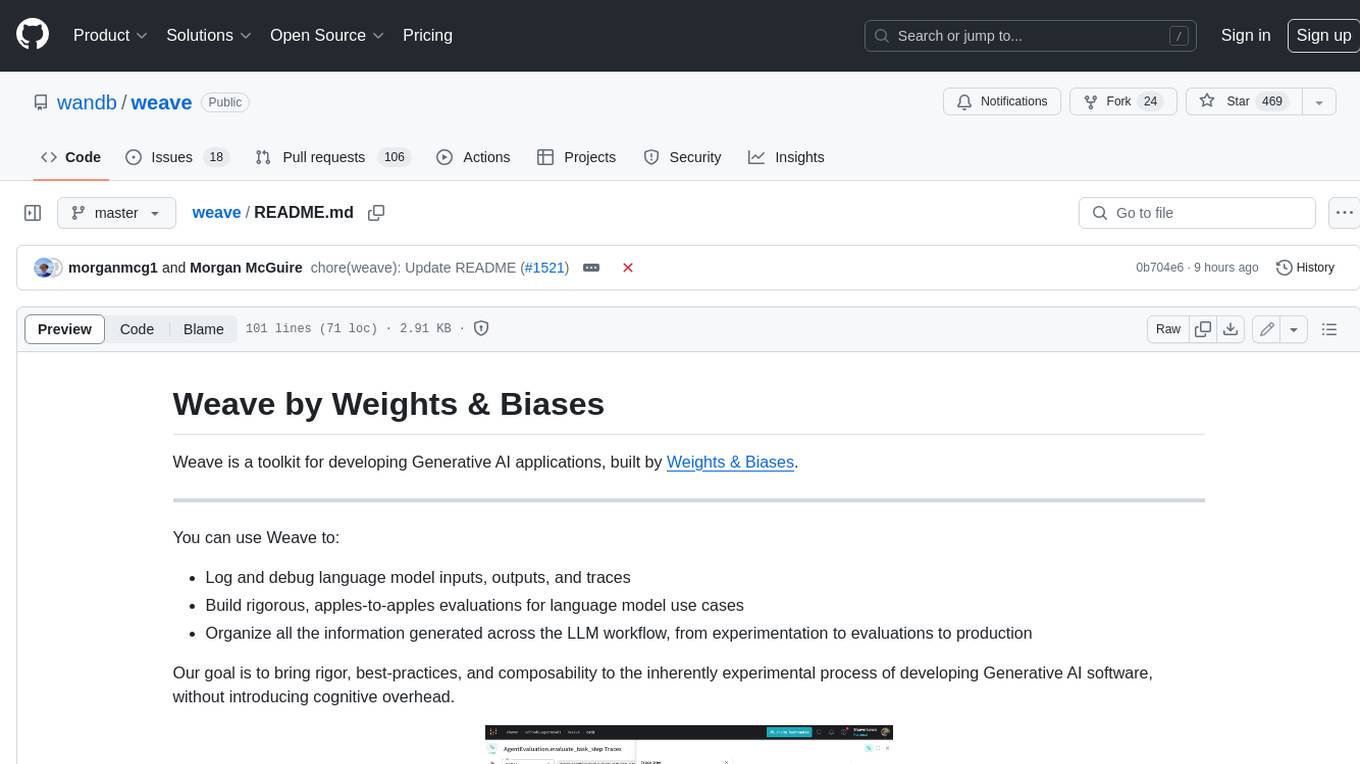
weave
Weave is a toolkit for developing Generative AI applications, built by Weights & Biases. With Weave, you can log and debug language model inputs, outputs, and traces; build rigorous, apples-to-apples evaluations for language model use cases; and organize all the information generated across the LLM workflow, from experimentation to evaluations to production. Weave aims to bring rigor, best-practices, and composability to the inherently experimental process of developing Generative AI software, without introducing cognitive overhead.
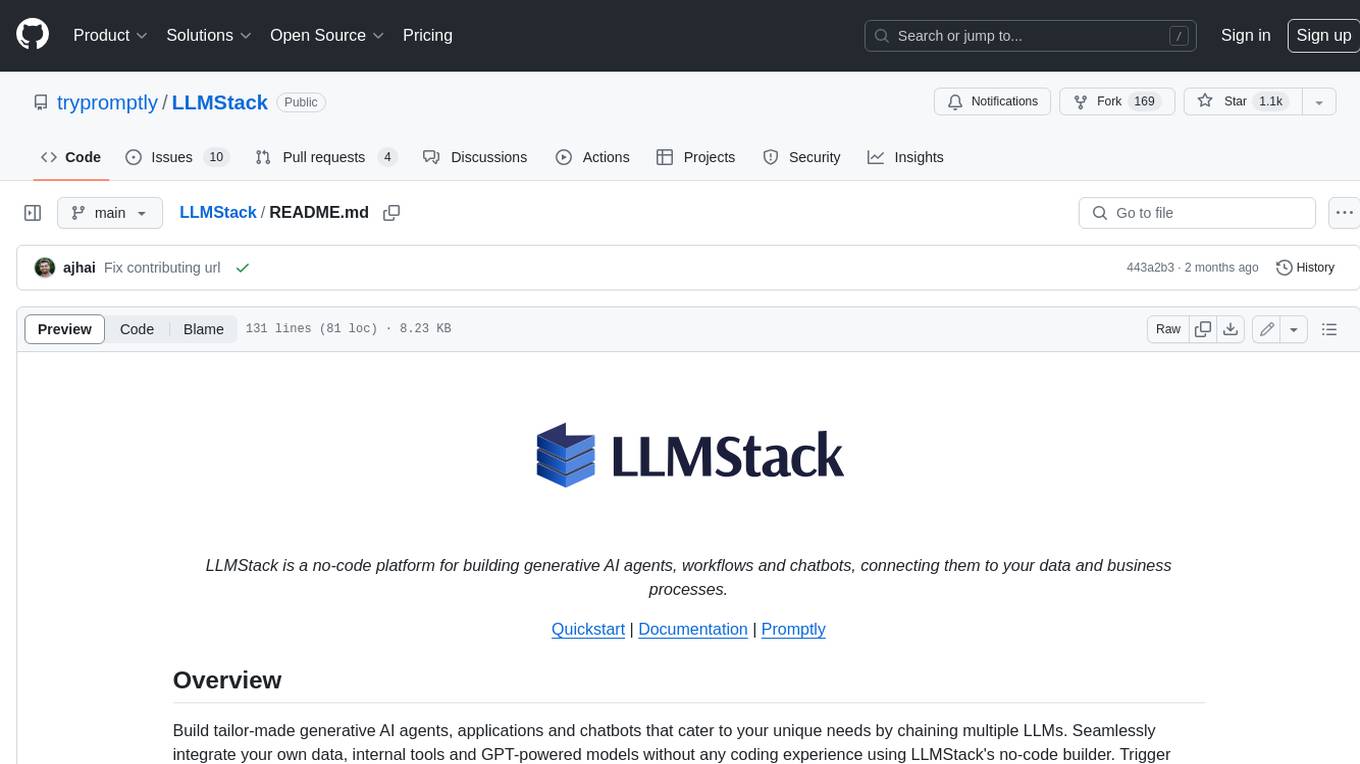
LLMStack
LLMStack is a no-code platform for building generative AI agents, workflows, and chatbots. It allows users to connect their own data, internal tools, and GPT-powered models without any coding experience. LLMStack can be deployed to the cloud or on-premise and can be accessed via HTTP API or triggered from Slack or Discord.
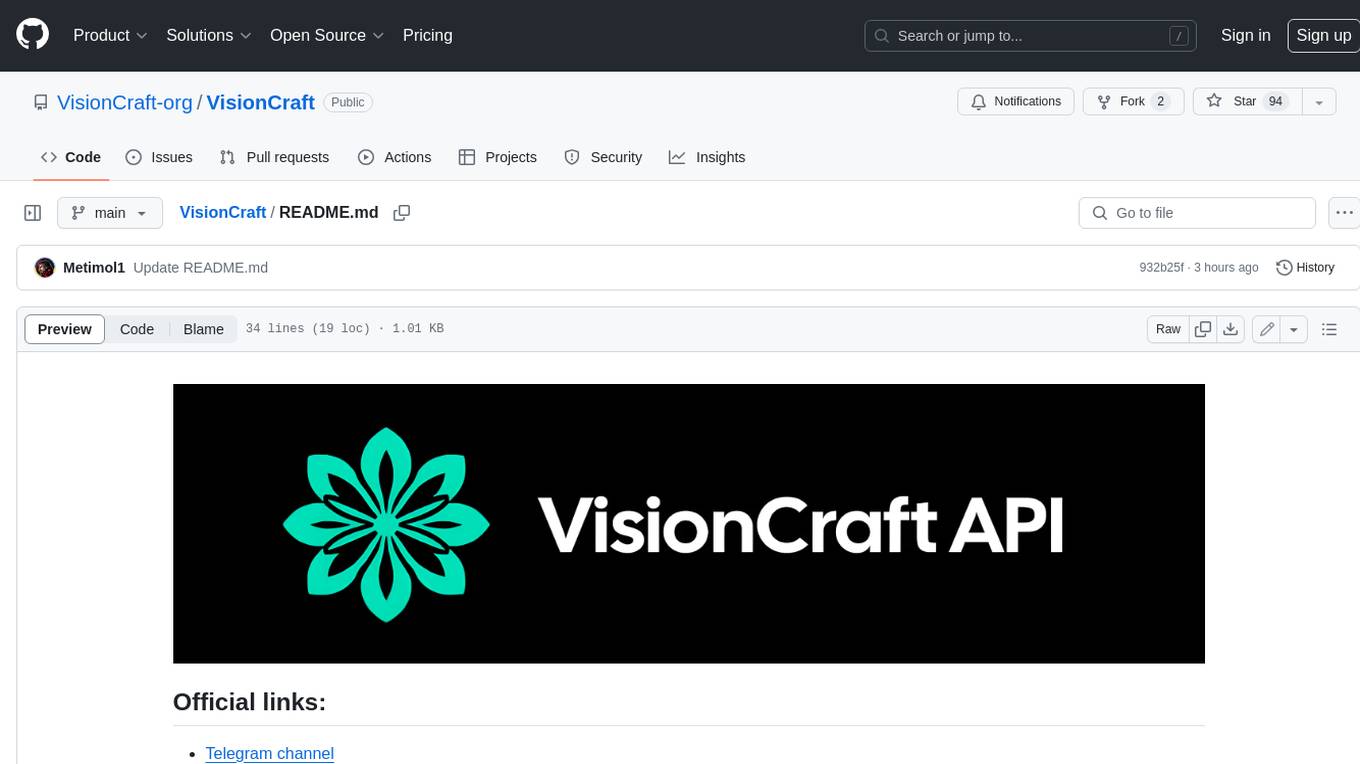
VisionCraft
The VisionCraft API is a free API for using over 100 different AI models. From images to sound.
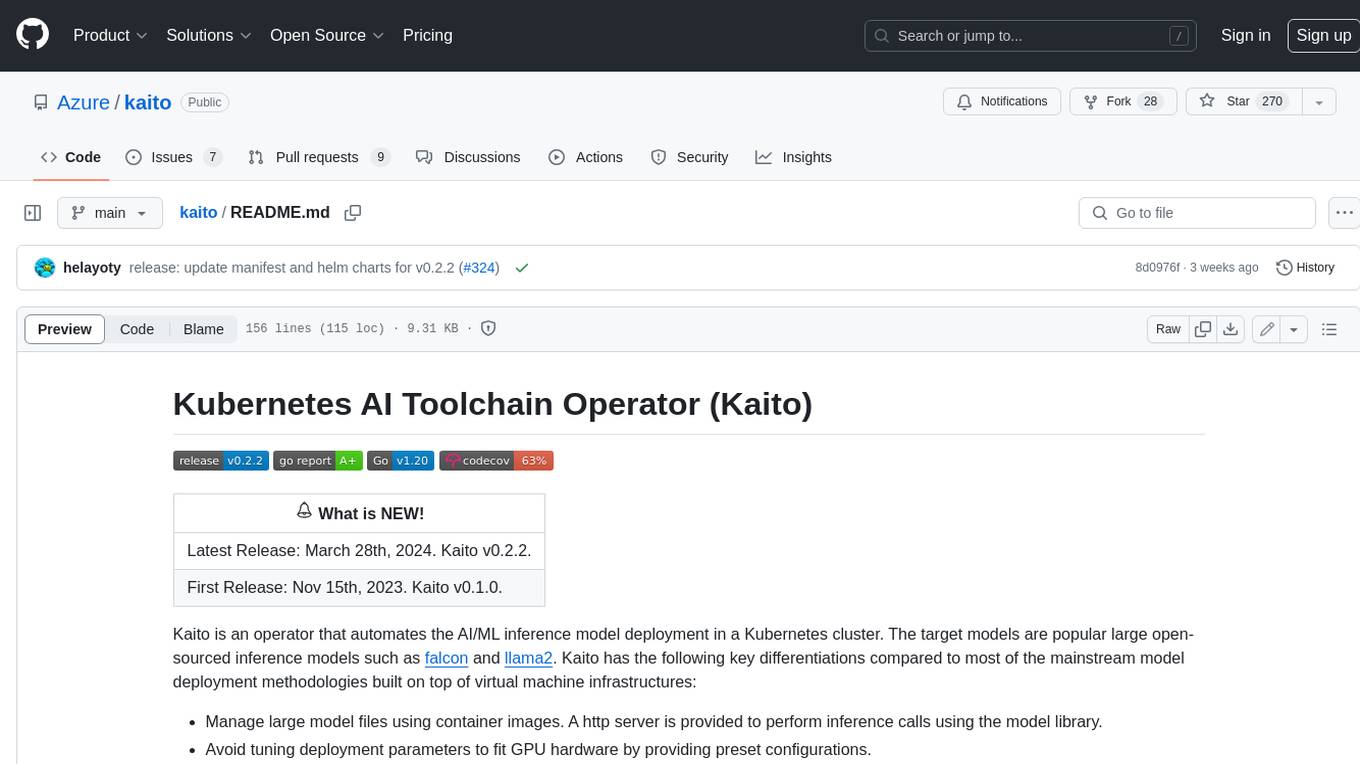
kaito
Kaito is an operator that automates the AI/ML inference model deployment in a Kubernetes cluster. It manages large model files using container images, avoids tuning deployment parameters to fit GPU hardware by providing preset configurations, auto-provisions GPU nodes based on model requirements, and hosts large model images in the public Microsoft Container Registry (MCR) if the license allows. Using Kaito, the workflow of onboarding large AI inference models in Kubernetes is largely simplified.
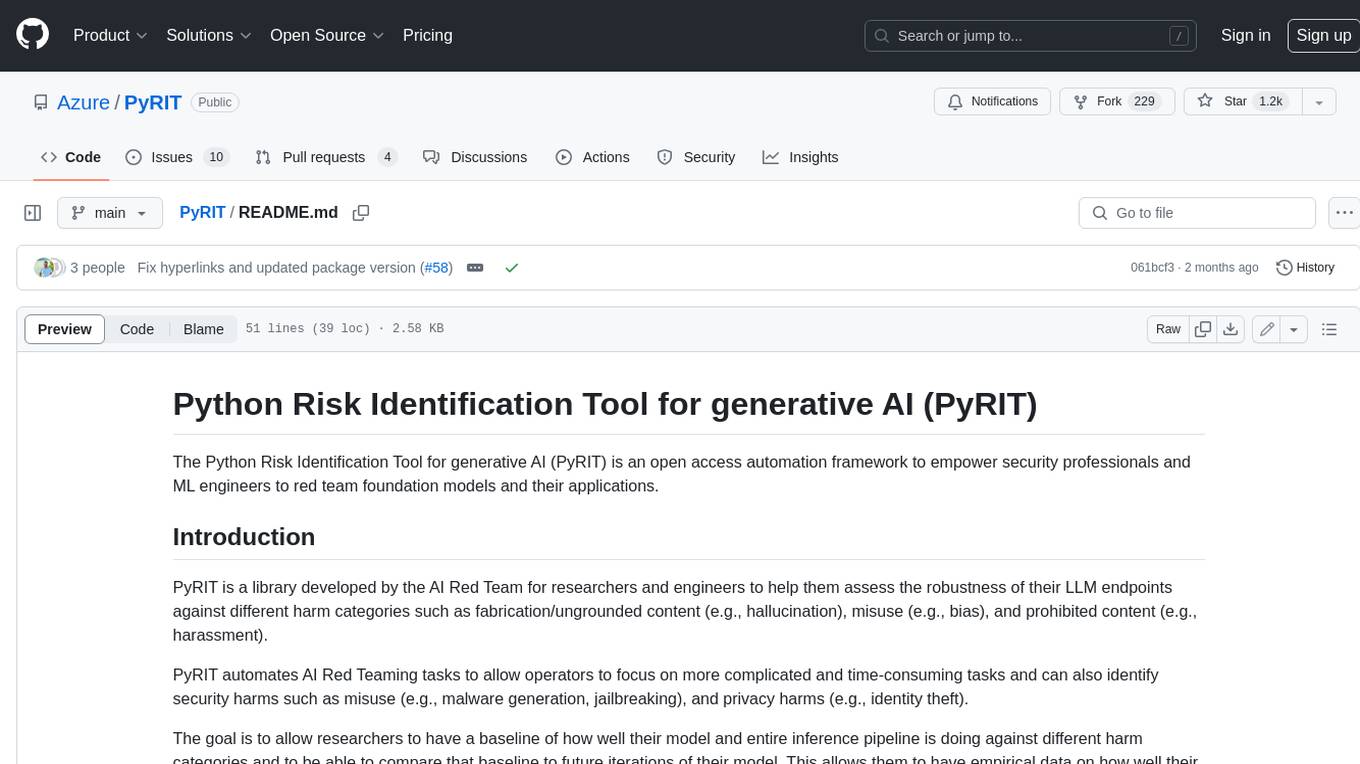
PyRIT
PyRIT is an open access automation framework designed to empower security professionals and ML engineers to red team foundation models and their applications. It automates AI Red Teaming tasks to allow operators to focus on more complicated and time-consuming tasks and can also identify security harms such as misuse (e.g., malware generation, jailbreaking), and privacy harms (e.g., identity theft). The goal is to allow researchers to have a baseline of how well their model and entire inference pipeline is doing against different harm categories and to be able to compare that baseline to future iterations of their model. This allows them to have empirical data on how well their model is doing today, and detect any degradation of performance based on future improvements.
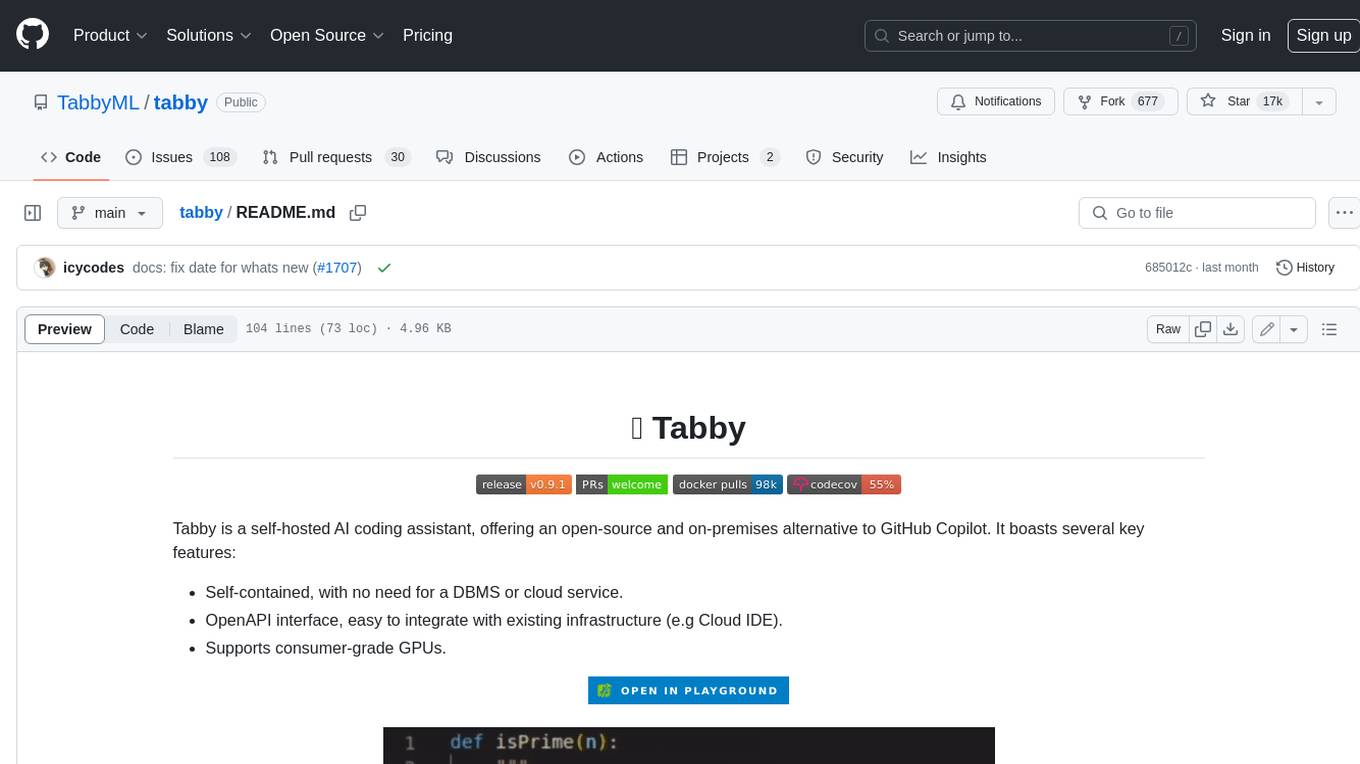
tabby
Tabby is a self-hosted AI coding assistant, offering an open-source and on-premises alternative to GitHub Copilot. It boasts several key features: * Self-contained, with no need for a DBMS or cloud service. * OpenAPI interface, easy to integrate with existing infrastructure (e.g Cloud IDE). * Supports consumer-grade GPUs.
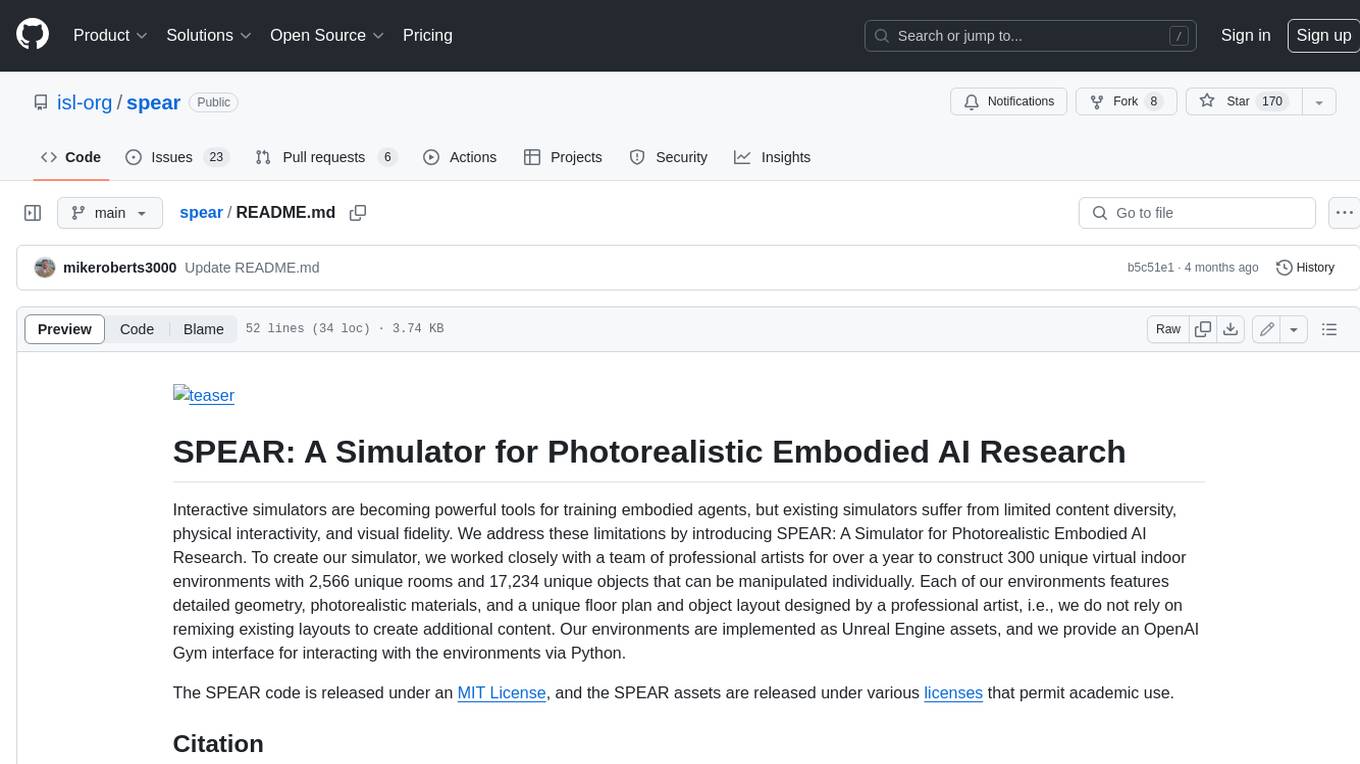
spear
SPEAR (Simulator for Photorealistic Embodied AI Research) is a powerful tool for training embodied agents. It features 300 unique virtual indoor environments with 2,566 unique rooms and 17,234 unique objects that can be manipulated individually. Each environment is designed by a professional artist and features detailed geometry, photorealistic materials, and a unique floor plan and object layout. SPEAR is implemented as Unreal Engine assets and provides an OpenAI Gym interface for interacting with the environments via Python.
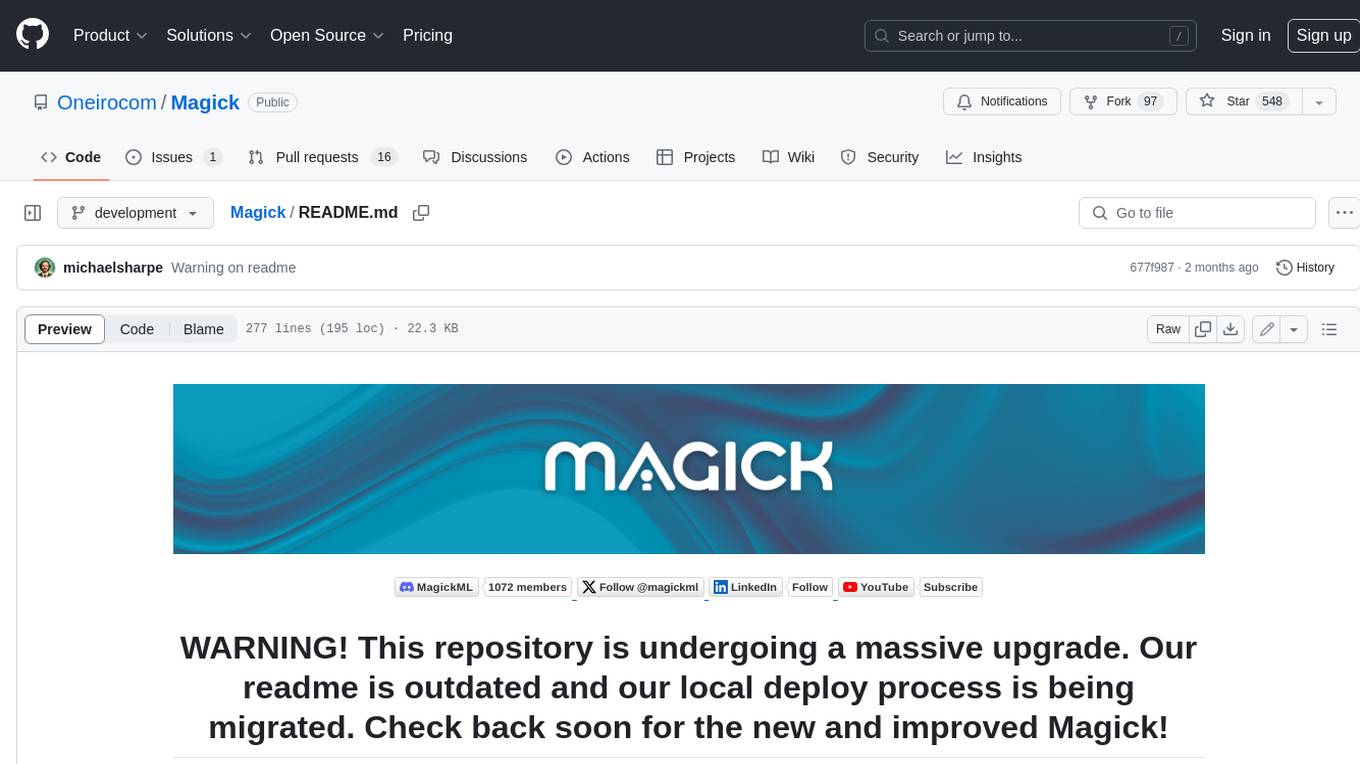
Magick
Magick is a groundbreaking visual AIDE (Artificial Intelligence Development Environment) for no-code data pipelines and multimodal agents. Magick can connect to other services and comes with nodes and templates well-suited for intelligent agents, chatbots, complex reasoning systems and realistic characters.