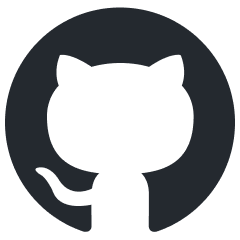
glimpse
Copy code from your codebase to clipboard instantly for LLM context!
Stars: 214
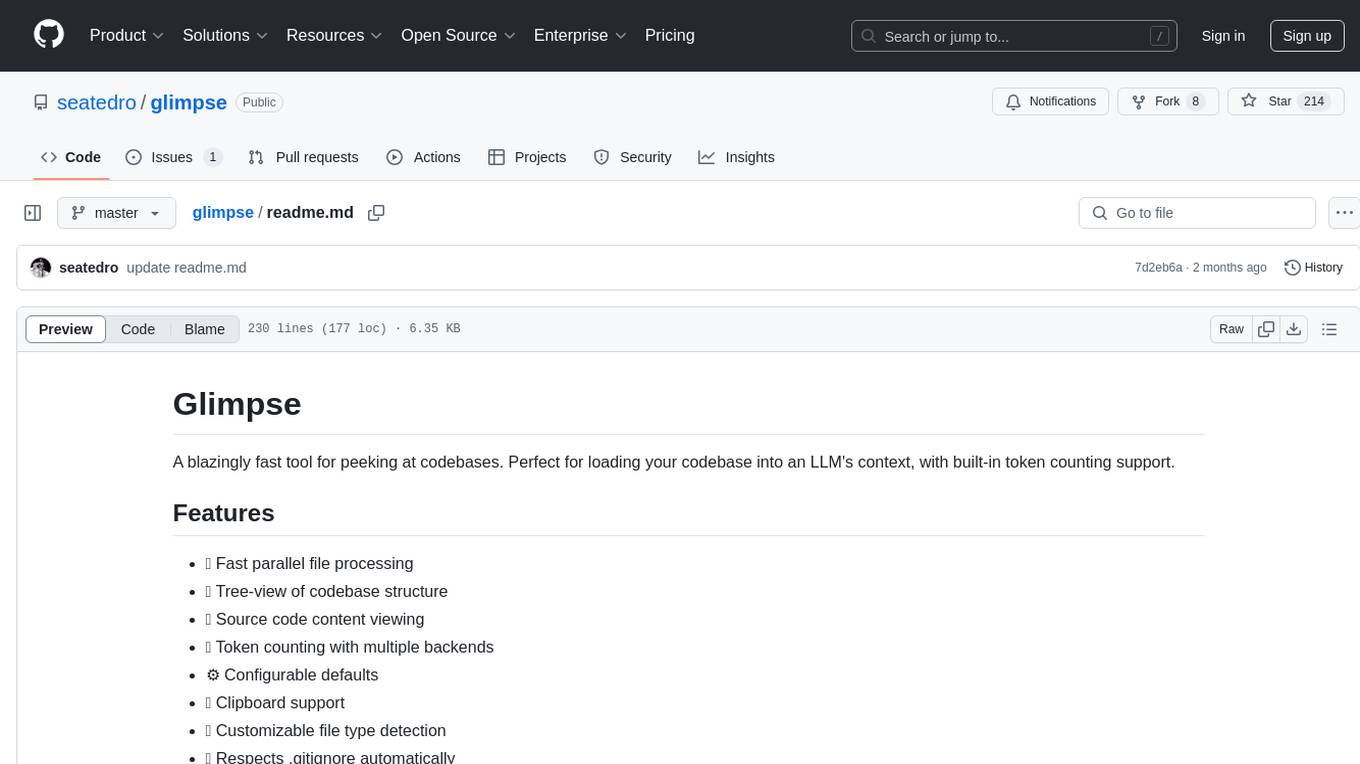
README:
A blazingly fast tool for peeking at codebases. Perfect for loading your codebase into an LLM's context, with built-in token counting support.
- 🚀 Fast parallel file processing
- 🌳 Tree-view of codebase structure
- 📝 Source code content viewing
- 🔢 Token counting with multiple backends
- ⚙️ Configurable defaults
- 📋 Clipboard support
- 🎨 Customizable file type detection
- 🥷 Respects .gitignore automatically
- 🔗 Web content processing with Markdown conversion
- 📦 Git repository support
- 🌐 URL traversal with configurable depth
Using cargo:
cargo install glimpse
Using homebrew:
brew tap seatedro/glimpse
brew install glimpse
Using Nix:
# Install directly
nix profile install github:seatedro/glimpse
# Or use in your flake
{
inputs.glimpse.url = "github:seatedro/glimpse";
}
Using an AUR helper:
# Using yay
yay -S glimpse
# Using paru
paru -S glimpse
Basic usage:
# Process a local directory
glimpse /path/to/project
# Process multiple files
glimpse file1 file2 file3
# Process a Git repository
glimpse https://github.com/username/repo.git
# Process a web page and convert to Markdown
glimpse https://example.com/docs
# Process a web page and its linked pages
glimpse https://example.com/docs --traverse-links --link-depth 2
Common options:
# Show hidden files
glimpse -H /path/to/project
# Only show tree structure
glimpse -o tree /path/to/project
# Copy output to clipboard
glimpse -c /path/to/project
# Save output to file
glimpse -f output.txt /path/to/project
# Include specific file types
glimpse -i "*.rs,*.go" /path/to/project
# Exclude patterns
glimpse -e "target/*,dist/*" /path/to/project
# Count tokens using tiktoken (OpenAI's tokenizer)
glimpse /path/to/project
# Use HuggingFace tokenizer with specific model
glimpse --tokenizer huggingface --model gpt2 /path/to/project
# Use custom local tokenizer file
glimpse --tokenizer huggingface --tokenizer-file /path/to/tokenizer.json /path/to/project
# Process a Git repository and save as PDF
glimpse https://github.com/username/repo.git --pdf output.pdf
Usage: glimpse [OPTIONS] [PATH]
Arguments:
[PATH] Directory/Files/URLs to analyze [default: .]
Options:
--interactive Opens interactive file picker (? for help)
-i, --include <PATTERNS> Additional patterns to include (e.g. "*.rs,*.go")
-e, --exclude <PATTERNS> Additional patterns to exclude
-s, --max-size <BYTES> Maximum file size in bytes
--max-depth <DEPTH> Maximum directory depth to traverse
-o, --output <FORMAT> Output format: tree, files, or both
-f, --file <PATH> Save output to specified file
-p, --print Print to stdout instead of clipboard
-t, --threads <COUNT> Number of threads for parallel processing
-H, --hidden Show hidden files and directories
--no-ignore Don't respect .gitignore files
--no-tokens Disable token counting
--tokenizer <TYPE> Tokenizer to use: tiktoken or huggingface
--model <NAME> Model name for HuggingFace tokenizer
--tokenizer-file <PATH> Path to local tokenizer file
--traverse-links Traverse links when processing URLs
--link-depth <DEPTH> Maximum depth to traverse links (default: 1)
--pdf <PATH> Save output as PDF
-h, --help Print help
-V, --version Print version
Glimpse uses a config file located at:
- Linux/macOS:
~/.config/glimpse/config.toml
- Windows:
%APPDATA%\glimpse\config.toml
Example configuration:
# General settings
max_size = 10485760 # 10MB
max_depth = 20
default_output_format = "both"
# Token counting settings
default_tokenizer = "tiktoken" # Can be "tiktoken" or "huggingface"
default_tokenizer_model = "gpt2" # Default model for HuggingFace tokenizer
# URL processing settings
traverse_links = false # Whether to traverse links by default
default_link_depth = 1 # Default depth for link traversal
# Default exclude patterns
default_excludes = [
"**/.git/**",
"**/target/**",
"**/node_modules/**"
]
Glimpse supports two tokenizer backends:
-
Tiktoken (Default): OpenAI's tokenizer implementation, perfect for accurately estimating tokens for GPT models.
-
HuggingFace Tokenizers: Supports any model from the HuggingFace hub or local tokenizer files, great for custom models or other ML frameworks.
The token count appears in both file content views and the final summary, helping you estimate context window usage for large language models.
Example token count output:
File: src/main.rs
Tokens: 245
==================================================
// File contents here...
Summary:
Total files: 10
Total size: 15360 bytes
Total tokens: 2456
-
File too large: Adjust
max_size
in config -
Missing files: Check
hidden
flag and exclude patterns -
Performance issues: Try adjusting thread count with
-t
-
Tokenizer errors:
- For HuggingFace models, ensure you have internet connection for downloading
- For local tokenizer files, verify the file path and format
- Try using the default tiktoken backend if issues persist
MIT
Glimpse can directly process Git repositories from popular hosting services:
- GitHub repositories
- GitLab repositories
- Bitbucket repositories
- Azure DevOps repositories
- Any Git repository URL (ending with .git)
The repository is cloned to a temporary directory, processed, and automatically cleaned up.
Glimpse can process web pages and convert them to Markdown:
- Preserves heading structure
- Converts links (both relative and absolute)
- Handles code blocks and quotes
- Supports nested lists
- Processes images and tables
With link traversal enabled, Glimpse can also process linked pages up to a specified depth, making it perfect for documentation sites and wikis.
Any processed content (local files, Git repositories, or web pages) can be saved as a PDF with:
- Preserved formatting
- Syntax highlighting
- Table of contents
- Page numbers
- Custom headers and footers
For Tasks:
Click tags to check more tools for each tasksFor Jobs:
Alternative AI tools for glimpse
Similar Open Source Tools
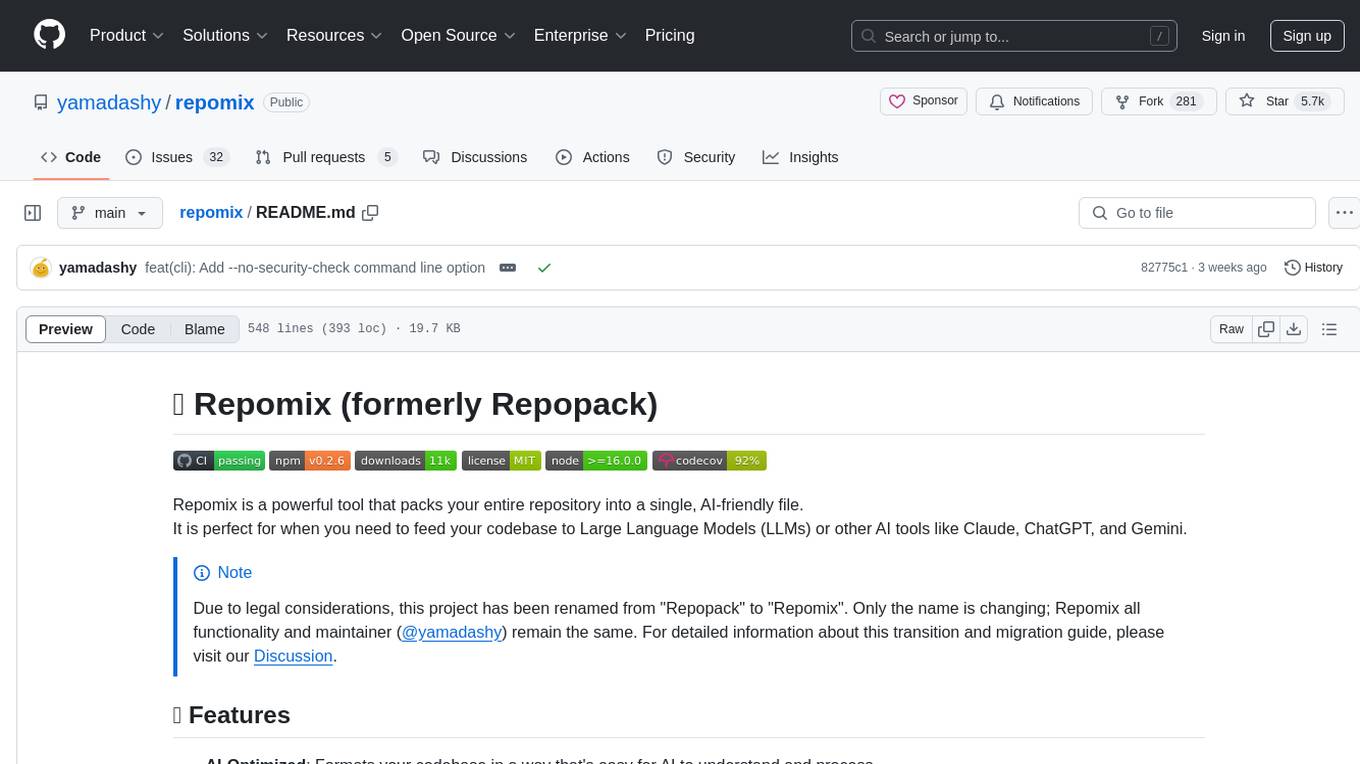
repomix
Repomix is a powerful tool that packs your entire repository into a single, AI-friendly file. It is designed to format your codebase for easy understanding by AI tools like Large Language Models (LLMs), Claude, ChatGPT, and Gemini. Repomix offers features such as AI optimization, token counting, simplicity in usage, customization options, Git awareness, and security-focused checks using Secretlint. It allows users to pack their entire repository or specific directories/files using glob patterns, and even supports processing remote Git repositories. The tool generates output in plain text, XML, or Markdown formats, with options for including/excluding files, removing comments, and performing security checks. Repomix also provides a global configuration option, custom instructions for AI context, and a security check feature to detect sensitive information in files.
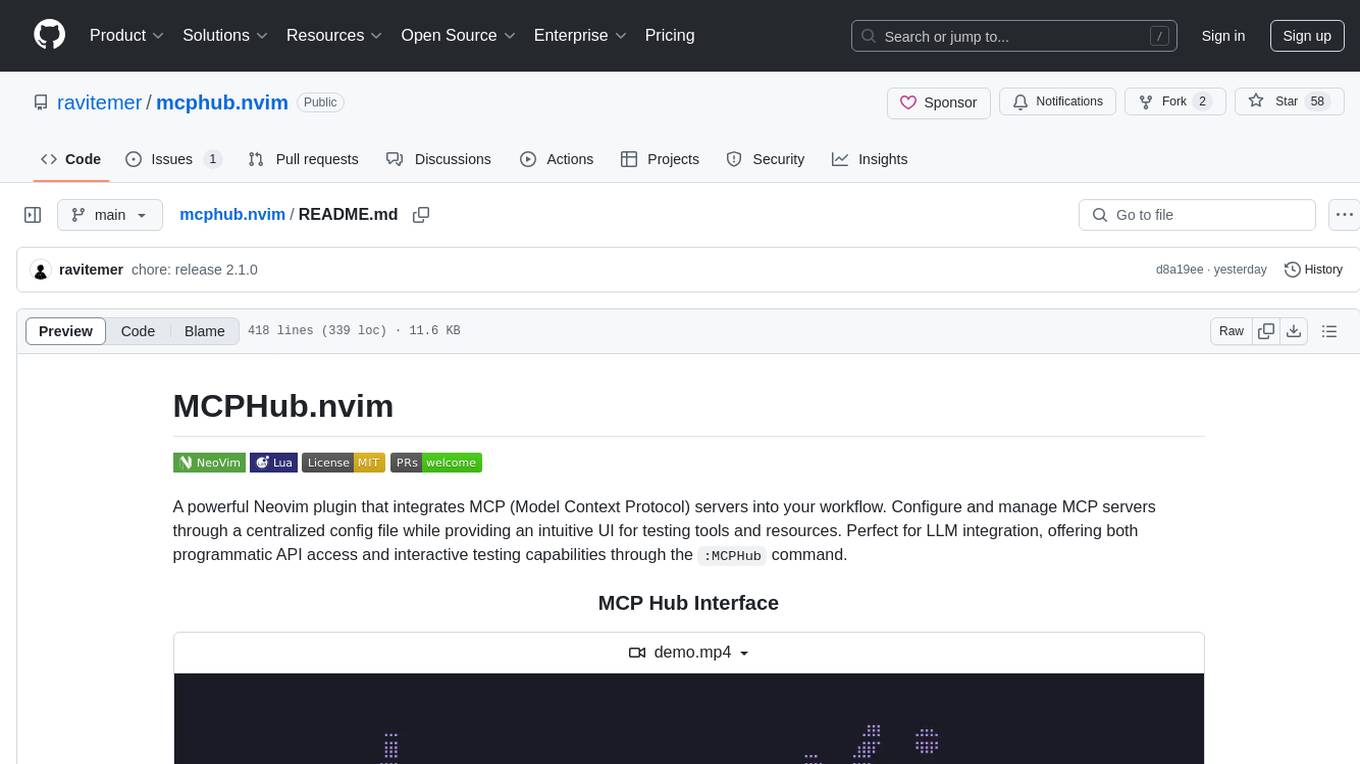
mcphub.nvim
MCPHub.nvim is a powerful Neovim plugin that integrates MCP (Model Context Protocol) servers into your workflow. It offers a centralized config file for managing servers and tools, with an intuitive UI for testing resources. Ideal for LLM integration, it provides programmatic API access and interactive testing through the `:MCPHub` command.
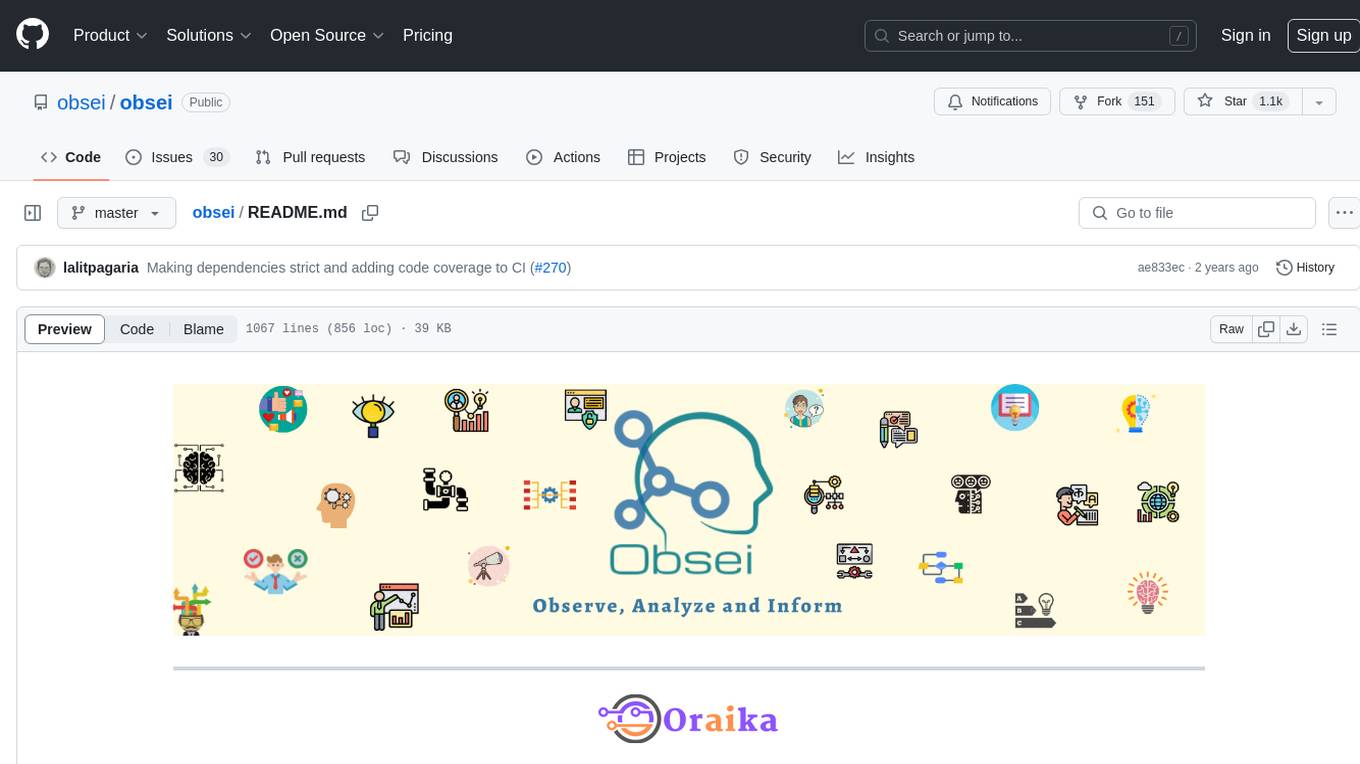
obsei
Obsei is an open-source, low-code, AI powered automation tool that consists of an Observer to collect unstructured data from various sources, an Analyzer to analyze the collected data with various AI tasks, and an Informer to send analyzed data to various destinations. The tool is suitable for scheduled jobs or serverless applications as all Observers can store their state in databases. Obsei is still in alpha stage, so caution is advised when using it in production. The tool can be used for social listening, alerting/notification, automatic customer issue creation, extraction of deeper insights from feedbacks, market research, dataset creation for various AI tasks, and more based on creativity.
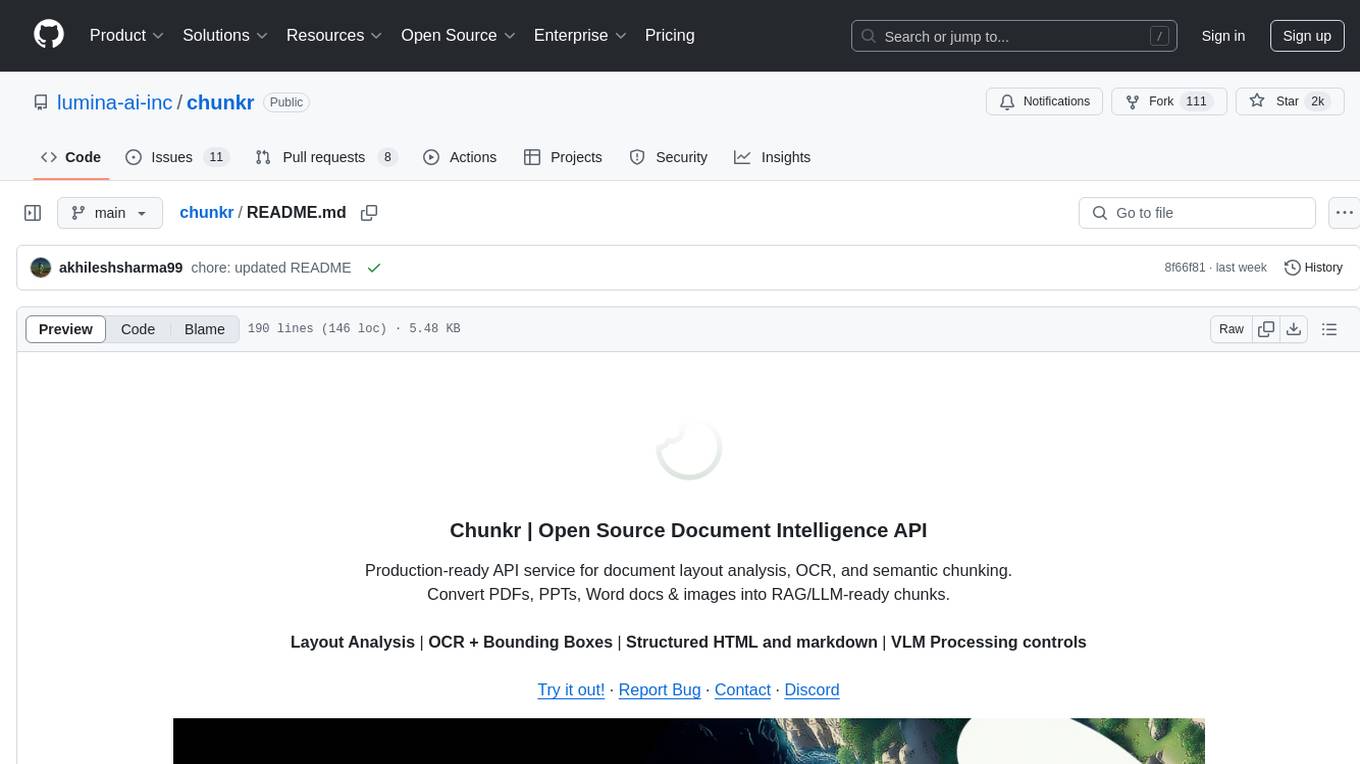
chunkr
Chunkr is an open-source document intelligence API that provides a production-ready service for document layout analysis, OCR, and semantic chunking. It allows users to convert PDFs, PPTs, Word docs, and images into RAG/LLM-ready chunks. The API offers features such as layout analysis, OCR with bounding boxes, structured HTML and markdown output, and VLM processing controls. Users can interact with Chunkr through a Python SDK, enabling them to upload documents, process them, and export results in various formats. The tool also supports self-hosted deployment options using Docker Compose or Kubernetes, with configurations for different AI models like OpenAI, Google AI Studio, and OpenRouter. Chunkr is dual-licensed under the GNU Affero General Public License v3.0 (AGPL-3.0) and a commercial license, providing flexibility for different usage scenarios.
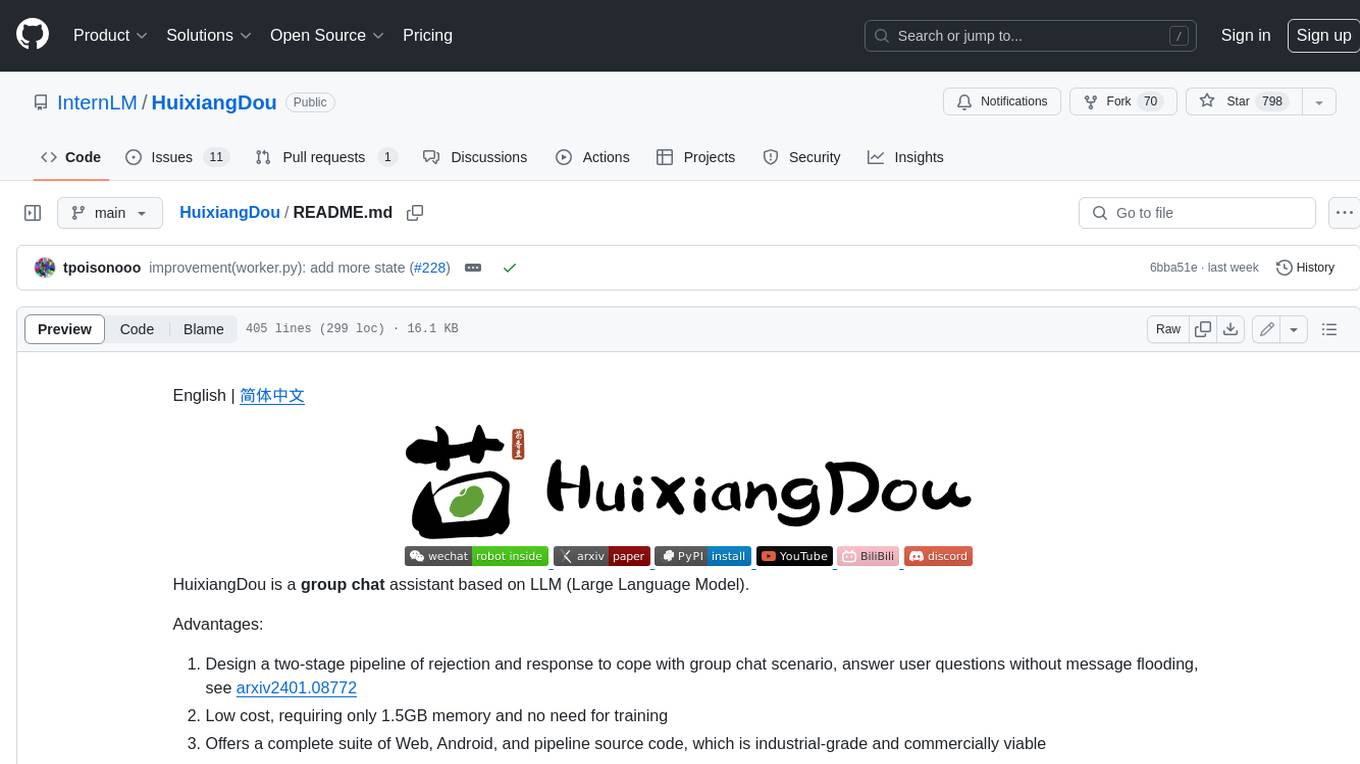
HuixiangDou
HuixiangDou is a **group chat** assistant based on LLM (Large Language Model). Advantages: 1. Design a two-stage pipeline of rejection and response to cope with group chat scenario, answer user questions without message flooding, see arxiv2401.08772 2. Low cost, requiring only 1.5GB memory and no need for training 3. Offers a complete suite of Web, Android, and pipeline source code, which is industrial-grade and commercially viable Check out the scenes in which HuixiangDou are running and join WeChat Group to try AI assistant inside. If this helps you, please give it a star ⭐
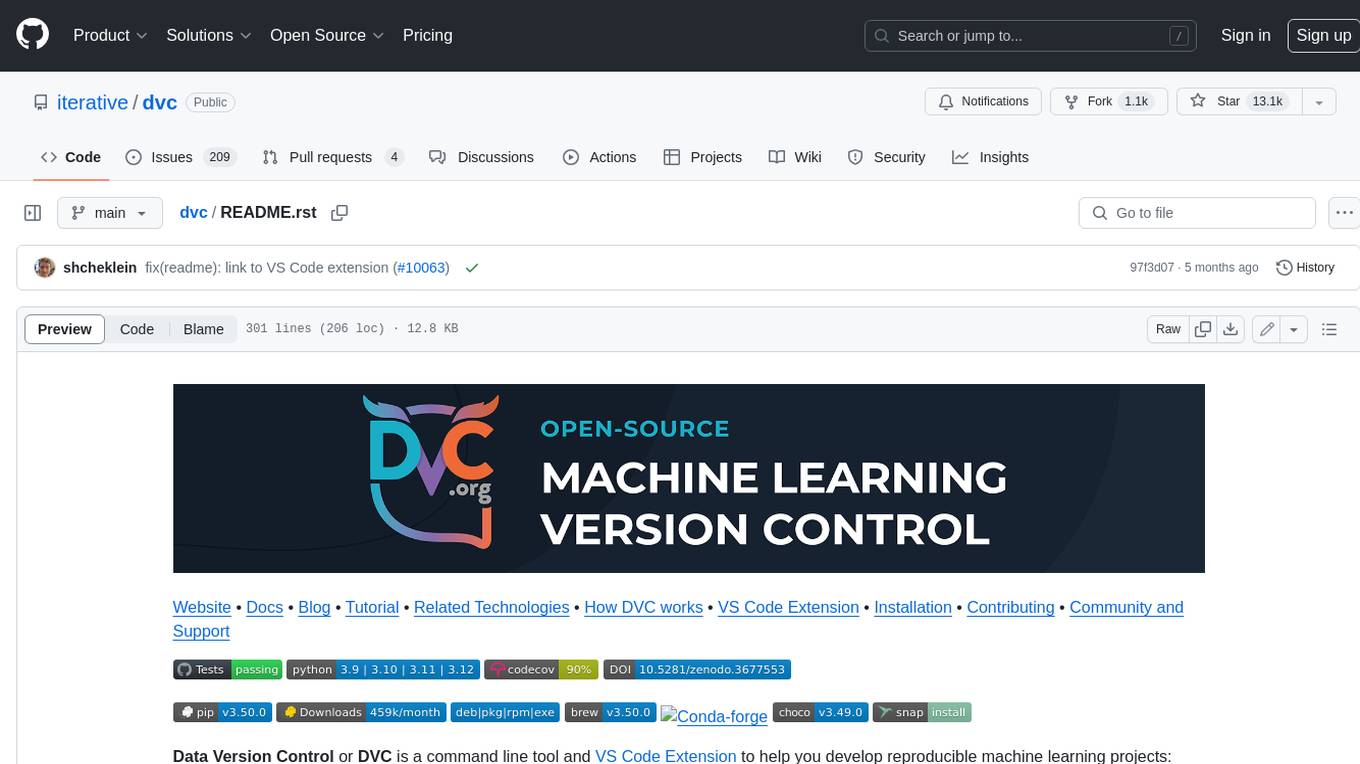
dvc
DVC, or Data Version Control, is a command-line tool and VS Code extension that helps you develop reproducible machine learning projects. With DVC, you can version your data and models, iterate fast with lightweight pipelines, track experiments in your local Git repo, compare any data, code, parameters, model, or performance plots, and share experiments and automatically reproduce anyone's experiment.
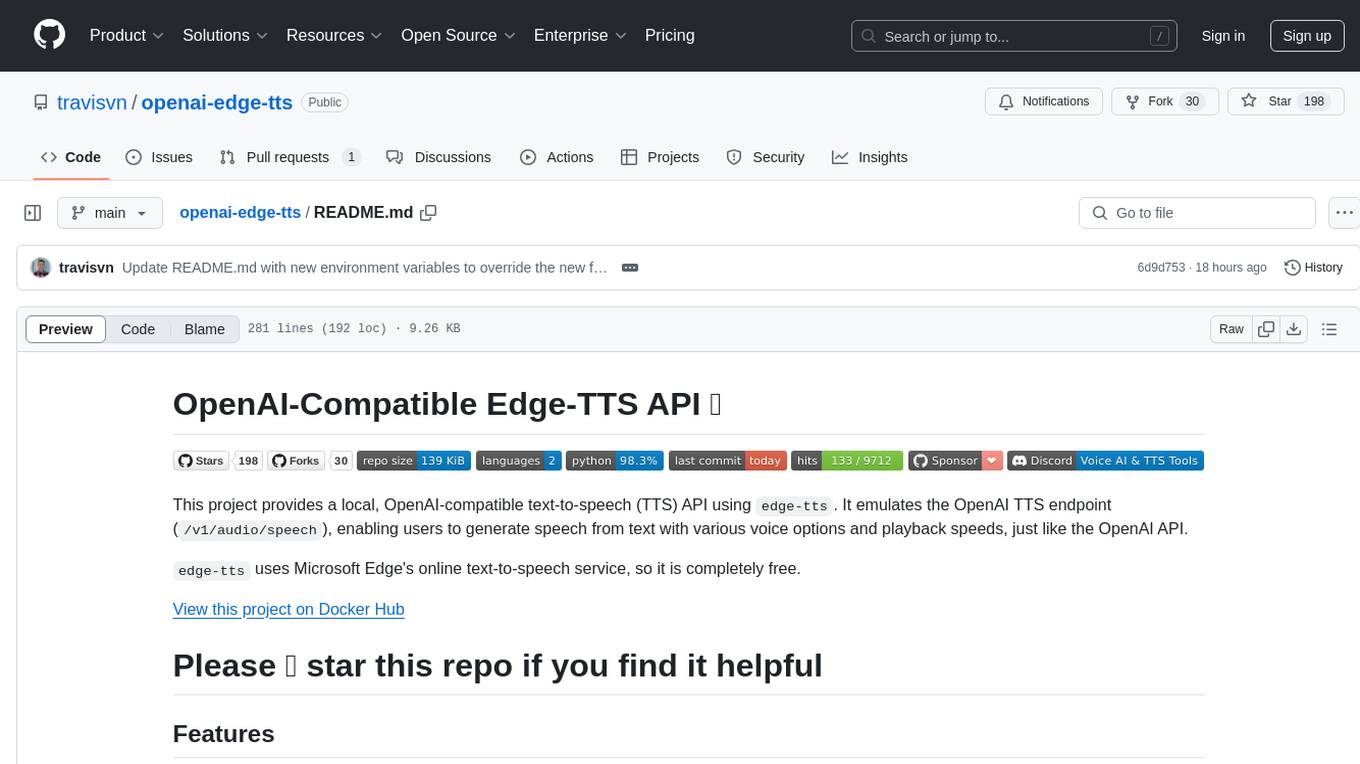
openai-edge-tts
This project provides a local, OpenAI-compatible text-to-speech (TTS) API using `edge-tts`. It emulates the OpenAI TTS endpoint (`/v1/audio/speech`), enabling users to generate speech from text with various voice options and playback speeds, just like the OpenAI API. `edge-tts` uses Microsoft Edge's online text-to-speech service, making it completely free. The project supports multiple audio formats, adjustable playback speed, and voice selection options, providing a flexible and customizable TTS solution for users.
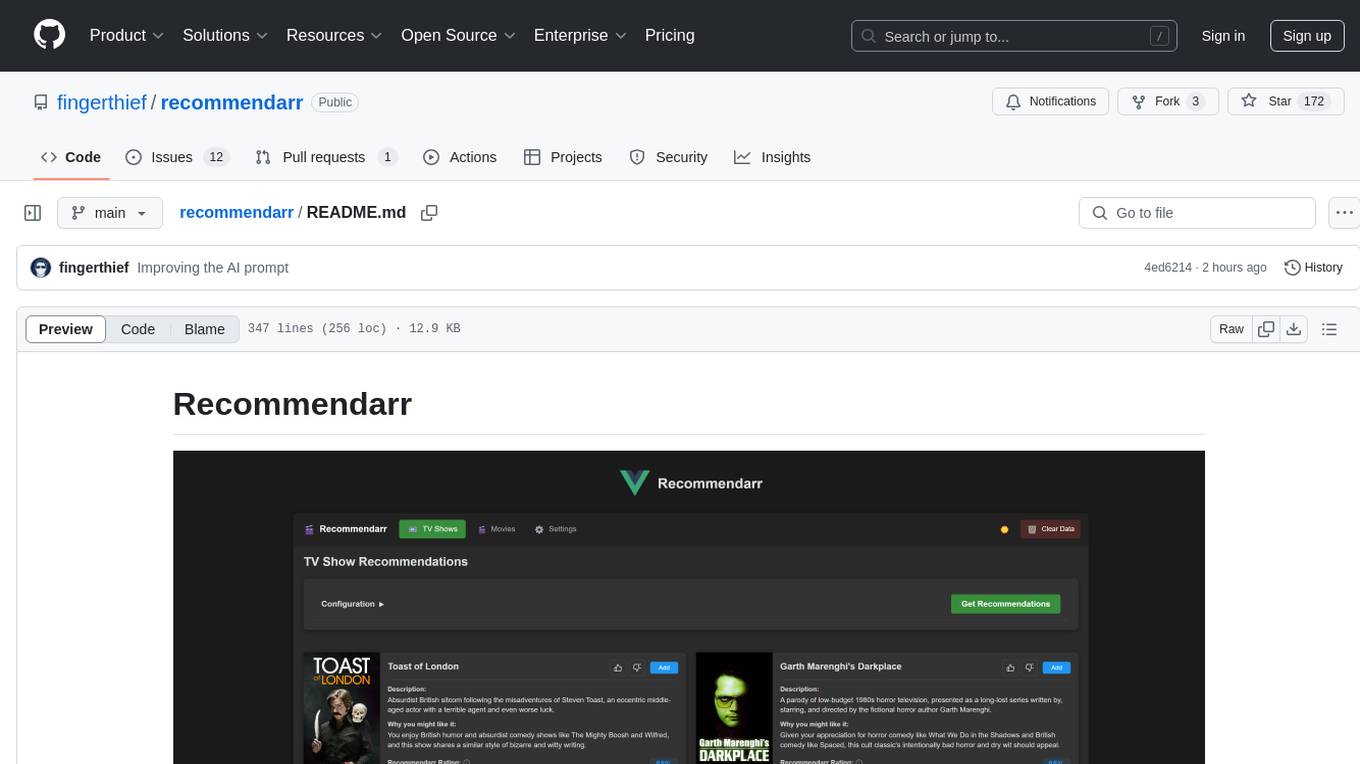
recommendarr
Recommendarr is a tool that generates personalized TV show and movie recommendations based on your Sonarr, Radarr, Plex, and Jellyfin libraries using AI. It offers AI-powered recommendations, media server integration, flexible AI support, watch history analysis, customization options, and dark/light mode toggle. Users can connect their media libraries and watch history services, configure AI service settings, and get personalized recommendations based on genre, language, and mood/vibe preferences. The tool works with any OpenAI-compatible API and offers various recommended models for different cost options and performance levels. It provides personalized suggestions, detailed information, filter options, watch history analysis, and one-click adding of recommended content to Sonarr/Radarr.
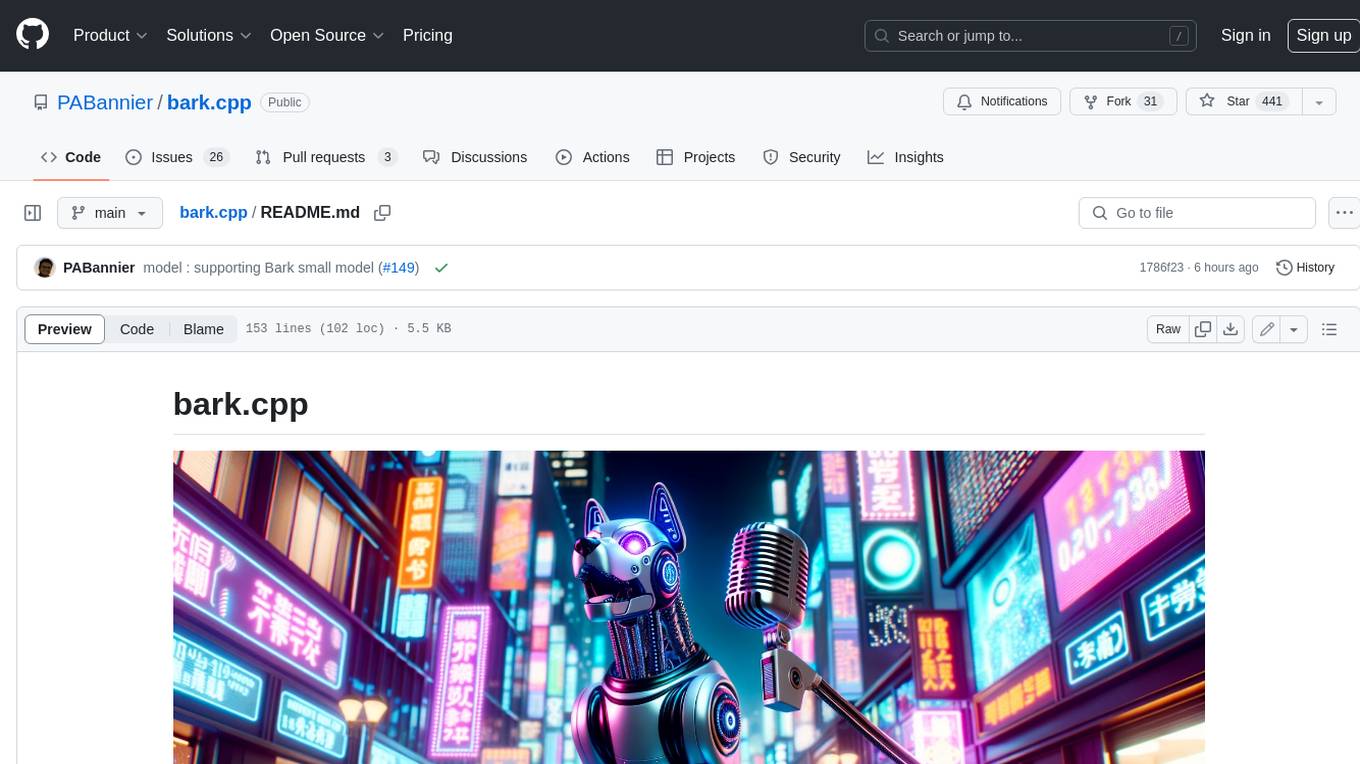
bark.cpp
Bark.cpp is a C/C++ implementation of the Bark model, a real-time, multilingual text-to-speech generation model. It supports AVX, AVX2, and AVX512 for x86 architectures, and is compatible with both CPU and GPU backends. Bark.cpp also supports mixed F16/F32 precision and 4-bit, 5-bit, and 8-bit integer quantization. It can be used to generate realistic-sounding audio from text prompts.
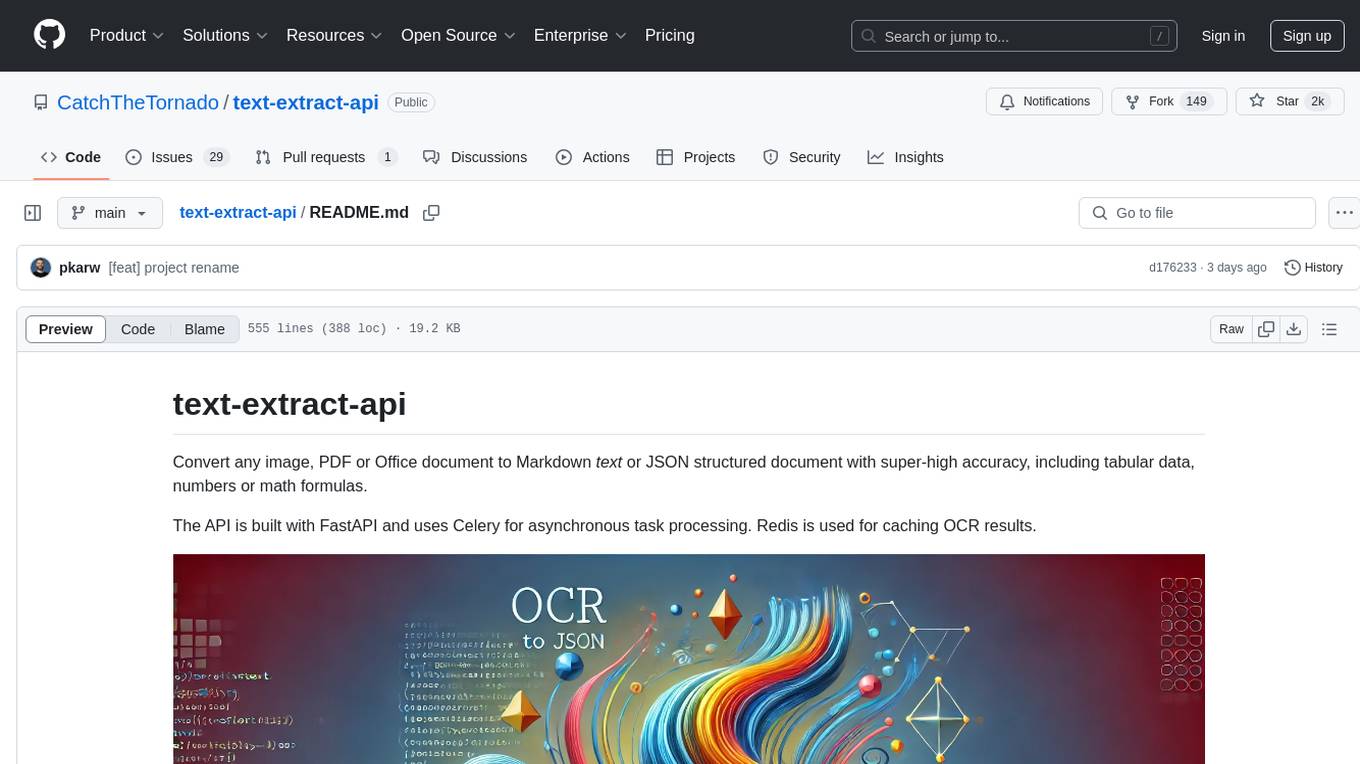
text-extract-api
The text-extract-api is a powerful tool that allows users to convert images, PDFs, or Office documents to Markdown text or JSON structured documents with high accuracy. It is built using FastAPI and utilizes Celery for asynchronous task processing, with Redis for caching OCR results. The tool provides features such as PDF/Office to Markdown and JSON conversion, improving OCR results with LLama, removing Personally Identifiable Information from documents, distributed queue processing, caching using Redis, switchable storage strategies, and a CLI tool for task management. Users can run the tool locally or on cloud services, with support for GPU processing. The tool also offers an online demo for testing purposes.
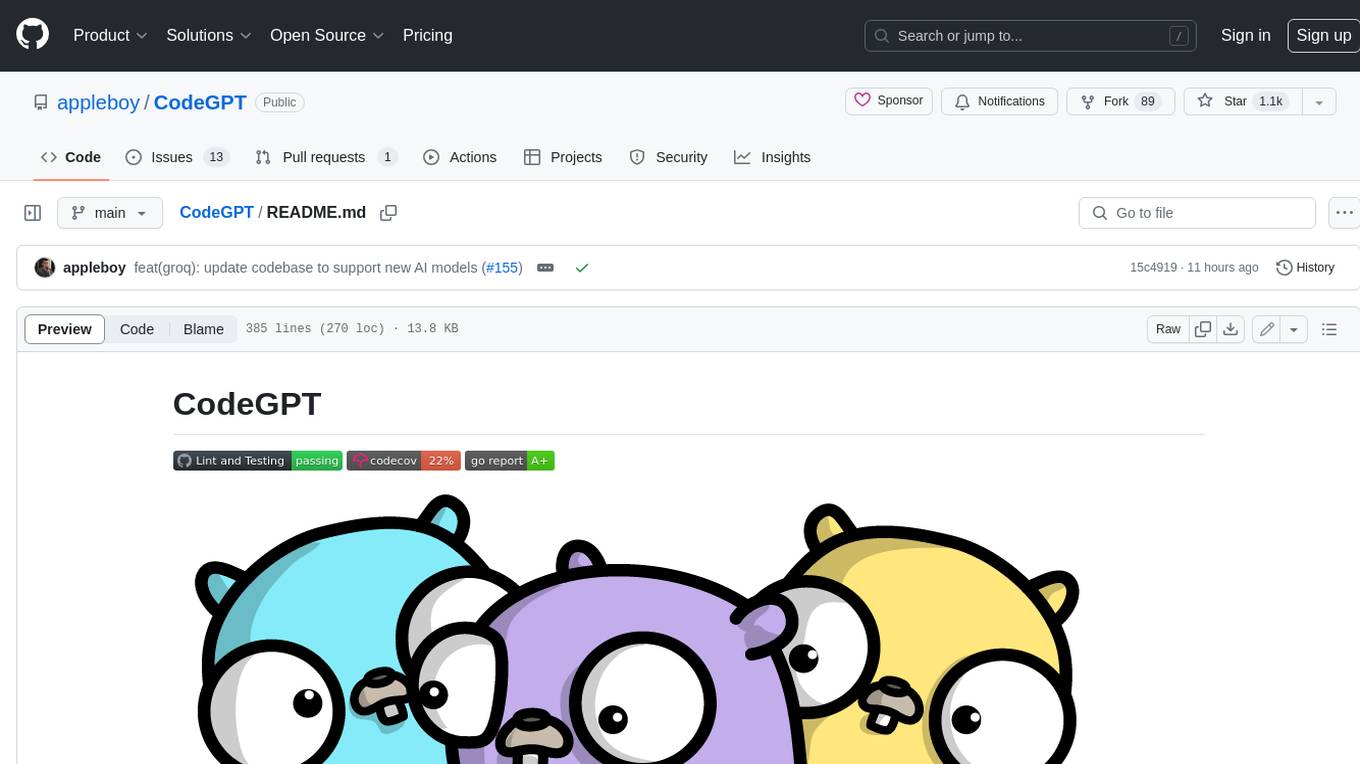
CodeGPT
CodeGPT is a CLI tool written in Go that helps you write git commit messages or do a code review brief using ChatGPT AI (gpt-3.5-turbo, gpt-4 model) and automatically installs a git prepare-commit-msg hook. It supports Azure OpenAI Service or OpenAI API, conventional commits specification, Git prepare-commit-msg Hook, customizing the number of lines of context in diffs, excluding files from the git diff command, translating commit messages into different languages, using socks or custom network HTTP proxies, specifying model lists, and doing brief code reviews.
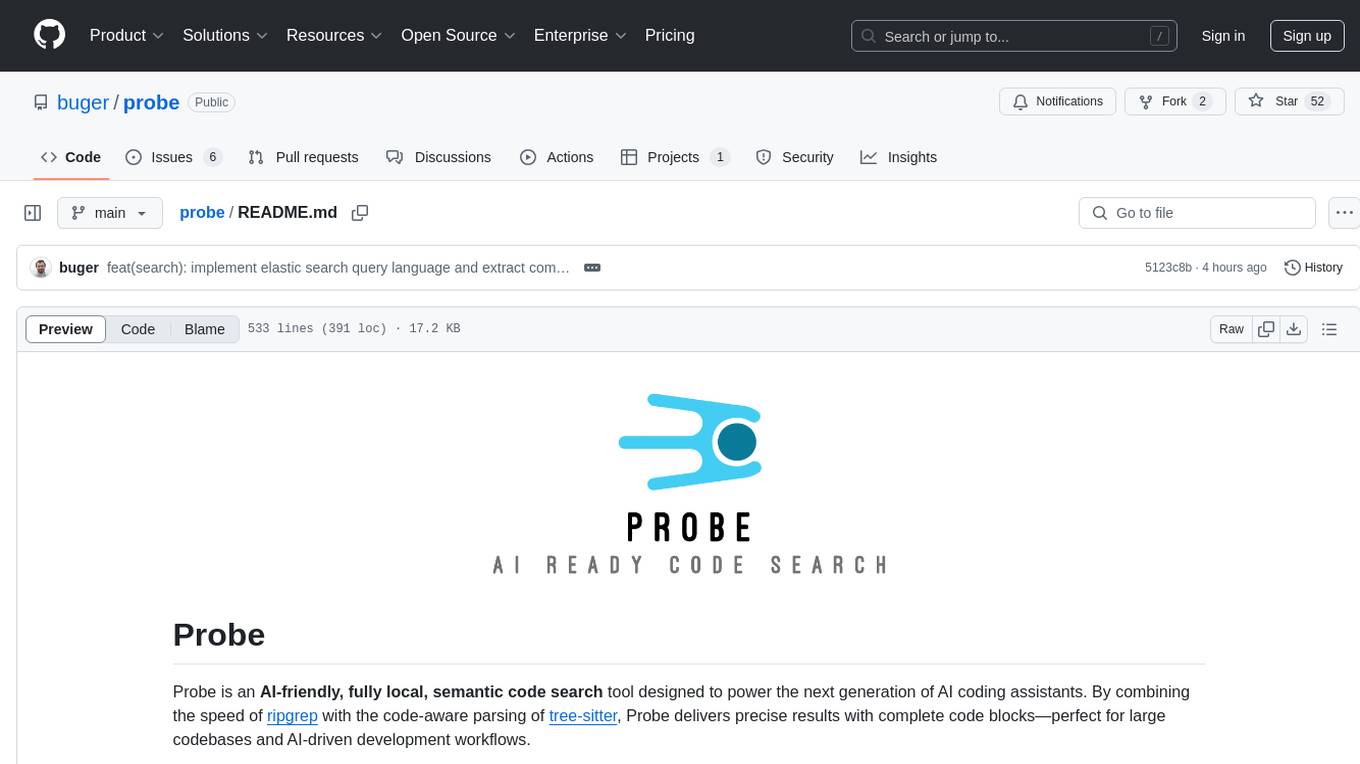
probe
Probe is an AI-friendly, fully local, semantic code search tool designed to power the next generation of AI coding assistants. It combines the speed of ripgrep with the code-aware parsing of tree-sitter to deliver precise results with complete code blocks, making it perfect for large codebases and AI-driven development workflows. Probe is fully local, keeping code on the user's machine without relying on external APIs. It supports multiple languages, offers various search options, and can be used in CLI mode, MCP server mode, AI chat mode, and web interface. The tool is designed to be flexible, fast, and accurate, providing developers and AI models with full context and relevant code blocks for efficient code exploration and understanding.
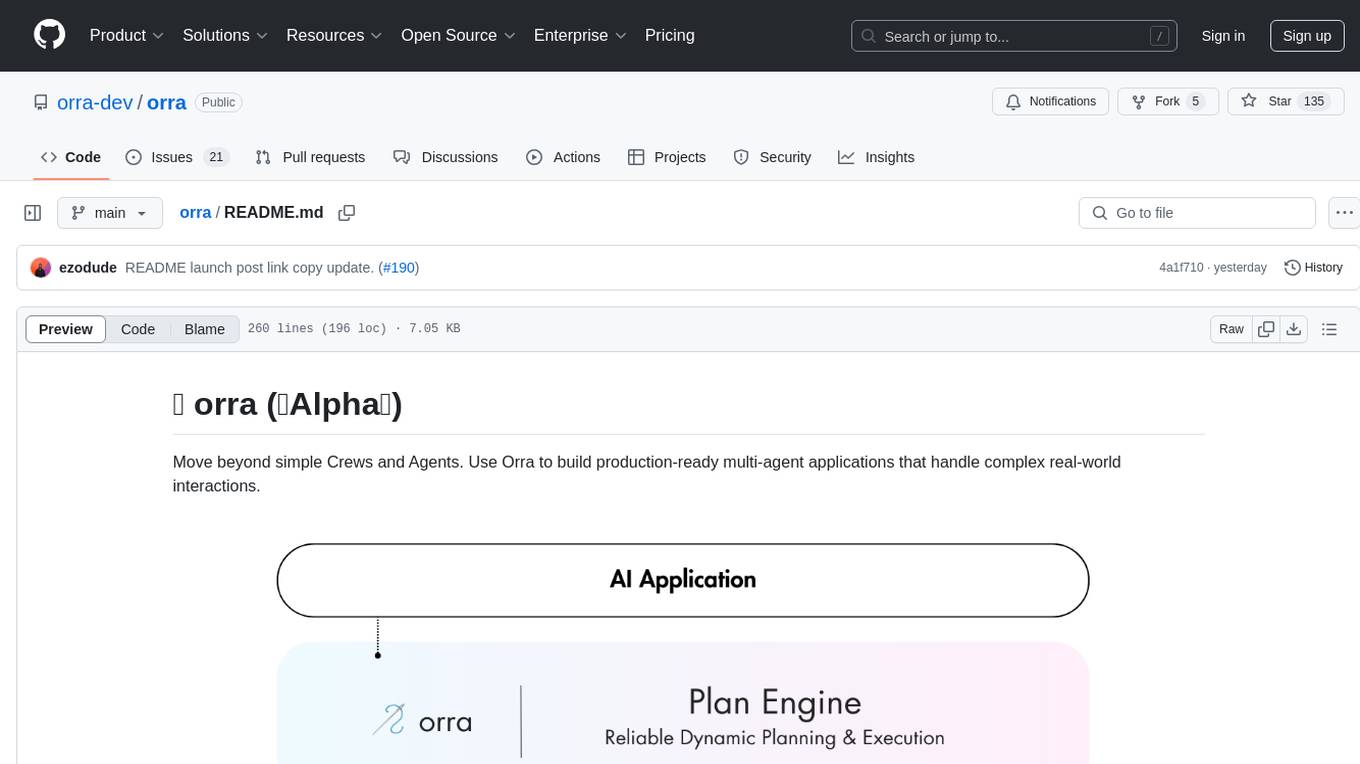
orra
Orra is a tool for building production-ready multi-agent applications that handle complex real-world interactions. It coordinates tasks across existing stack, agents, and tools run as services using intelligent reasoning. With features like smart pre-evaluated execution plans, domain grounding, durable execution, and automatic service health monitoring, Orra enables users to go fast with tools as services and revert state to handle failures. It provides real-time status tracking and webhook result delivery, making it ideal for developers looking to move beyond simple crews and agents.