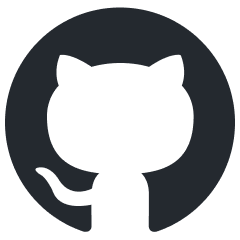
dvc
🦉 ML Experiments and Data Management with Git
Stars: 13638
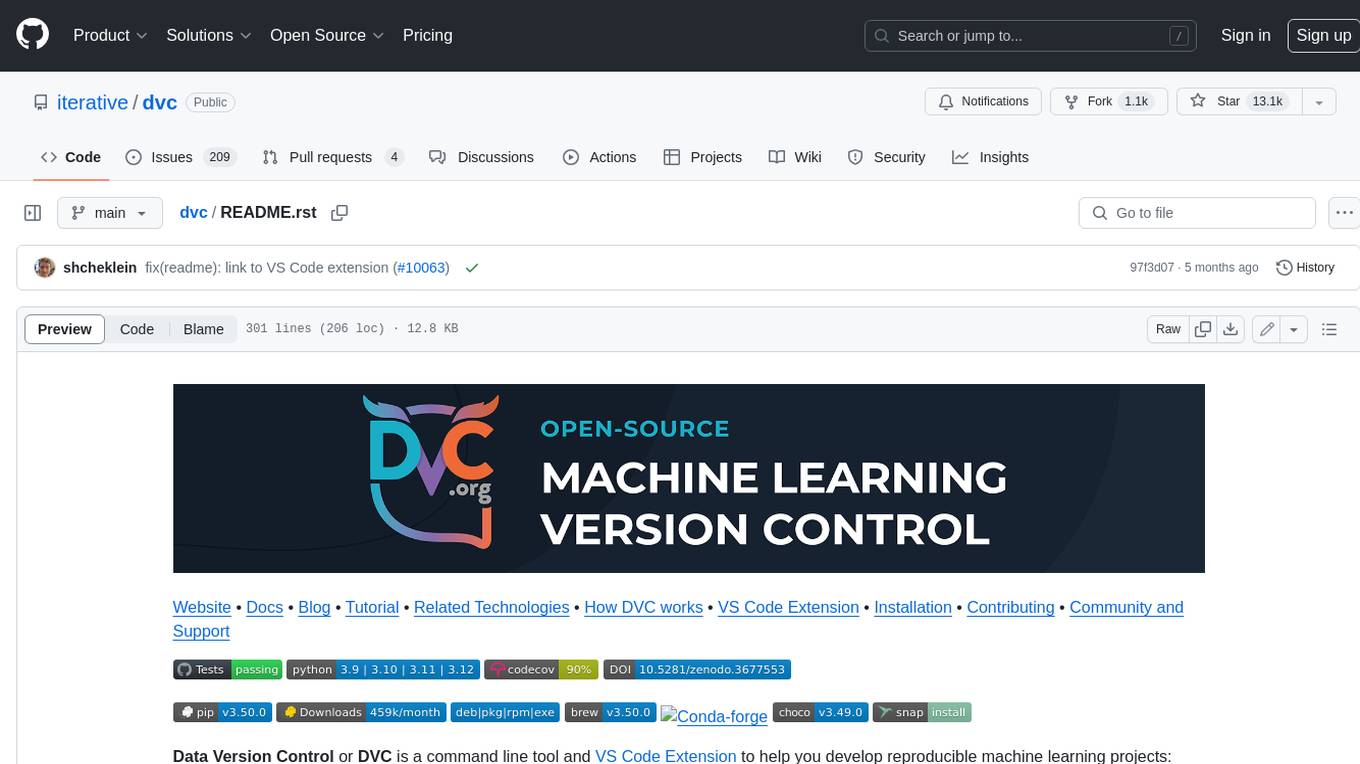
DVC, or Data Version Control, is a command-line tool and VS Code extension that helps you develop reproducible machine learning projects. With DVC, you can version your data and models, iterate fast with lightweight pipelines, track experiments in your local Git repo, compare any data, code, parameters, model, or performance plots, and share experiments and automatically reproduce anyone's experiment.
README:
🚀 Check out our new product DataChain <https://github.com/iterative/datachain>
_ (and give it a ⭐!) if you need to version and process a large number of files. Contact us at [email protected] to discuss commercial solutions and support for AI reproducibility and data management scenarios.
Website <https://dvc.org>
_
• Docs <https://dvc.org/doc>
_
• Blog <http://blog.dataversioncontrol.com>
_
• Tutorial <https://dvc.org/doc/get-started>
_
• Related Technologies <https://dvc.org/doc/user-guide/related-technologies>
_
• How DVC works
_
• VS Code Extension
_
• Installation
_
• Contributing
_
• Community and Support
_
|CI| |Python Version| |Coverage| |VS Code| |DOI|
|PyPI| |PyPI Downloads| |Packages| |Brew| |Conda| |Choco| |Snap|
|
Data Version Control or DVC is a command line tool and VS Code Extension
_ to help you develop reproducible machine learning projects:
#. Version your data and models. Store them in your cloud storage but keep their version info in your Git repo.
#. Iterate fast with lightweight pipelines. When you make changes, only run the steps impacted by those changes.
#. Track experiments in your local Git repo (no servers needed).
#. Compare any data, code, parameters, model, or performance plots.
#. Share experiments and automatically reproduce anyone's experiment.
Please read our `Command Reference <https://dvc.org/doc/command-reference>`_ for a complete list.
A common CLI workflow includes:
+-----------------------------------+----------------------------------------------------------------------------------------------------+
| Task | Terminal |
+===================================+====================================================================================================+
| Track data | | $ git add train.py params.yaml
|
| | | $ dvc add images/
|
+-----------------------------------+----------------------------------------------------------------------------------------------------+
| Connect code and data | | $ dvc stage add -n featurize -d images/ -o features/ python featurize.py
|
| | | $ dvc stage add -n train -d features/ -d train.py -o model.p -M metrics.json python train.py
|
+-----------------------------------+----------------------------------------------------------------------------------------------------+
| Make changes and experiment | | $ dvc exp run -n exp-baseline
|
| | | $ vi train.py
|
| | | $ dvc exp run -n exp-code-change
|
+-----------------------------------+----------------------------------------------------------------------------------------------------+
| Compare and select experiments | | $ dvc exp show
|
| | | $ dvc exp apply exp-baseline
|
+-----------------------------------+----------------------------------------------------------------------------------------------------+
| Share code | | $ git add .
|
| | | $ git commit -m 'The baseline model'
|
| | | $ git push
|
+-----------------------------------+----------------------------------------------------------------------------------------------------+
| Share data and ML models | | $ dvc remote add myremote -d s3://mybucket/image_cnn
|
| | | $ dvc push
|
+-----------------------------------+----------------------------------------------------------------------------------------------------+
We encourage you to read our `Get Started
<https://dvc.org/doc/get-started>`_ docs to better understand what DVC
does and how it can fit your scenarios.
The closest analogies to describe the main DVC features are these:
#. Git for data: Store and share data artifacts (like Git-LFS but without a server) and models, connecting them with a Git repository. Data management meets GitOps! #. Makefiles for ML: Describes how data or model artifacts are built from other data and code in a standard format. Now you can version your data pipelines with Git. #. Local experiment tracking: Turn your machine into an ML experiment management platform, and collaborate with others using existing Git hosting (Github, Gitlab, etc.).
Git is employed as usual to store and version code (including DVC meta-files as placeholders for data).
DVC stores data and model files <https://dvc.org/doc/start/data-management>
_ seamlessly in a cache outside of Git, while preserving almost the same user experience as if they were in the repo.
To share and back up the data cache, DVC supports multiple remote storage platforms - any cloud (S3, Azure, Google Cloud, etc.) or on-premise network storage (via SSH, for example).
|Flowchart|
DVC pipelines <https://dvc.org/doc/start/data-management/data-pipelines>
_ (computational graphs) connect code and data together.
They specify all steps required to produce a model: input dependencies including code, data, commands to run; and output information to be saved.
Last but not least, DVC Experiment Versioning <https://dvc.org/doc/start/experiments>
_ lets you prepare and run a large number of experiments.
Their results can be filtered and compared based on hyperparameters and metrics, and visualized with multiple plots.
.. _VS Code Extension
:
|VS Code|
To use DVC as a GUI right from your VS Code IDE, install the DVC Extension <https://marketplace.visualstudio.com/items?itemName=Iterative.dvc>
_ from the Marketplace.
It currently features experiment tracking and data management, and more features (data pipeline support, etc.) are coming soon!
|VS Code Extension Overview|
Note: You'll have to install core DVC on your system separately (as detailed
below). The Extension will guide you if needed.
There are several ways to install DVC: in VS Code; using snap
, choco
, brew
, conda
, pip
; or with an OS-specific package.
Full instructions are available here <https://dvc.org/doc/get-started/install>
_.
|Snap|
.. code-block:: bash
snap install dvc --classic
This corresponds to the latest tagged release.
Add --beta
for the latest tagged release candidate, or --edge
for the latest main
version.
|Choco|
.. code-block:: bash
choco install dvc
|Brew|
.. code-block:: bash
brew install dvc
|Conda|
.. code-block:: bash
conda install -c conda-forge mamba # installs much faster than conda mamba install -c conda-forge dvc
Depending on the remote storage type you plan to use to keep and share your data, you might need to install optional dependencies: dvc-s3
, dvc-azure
, dvc-gdrive
, dvc-gs
, dvc-oss
, dvc-ssh
.
|PyPI|
.. code-block:: bash
pip install dvc
Depending on the remote storage type you plan to use to keep and share your data, you might need to specify one of the optional dependencies: s3
, gs
, azure
, oss
, ssh
. Or all
to include them all.
The command should look like this: pip install 'dvc[s3]'
(in this case AWS S3 dependencies such as boto3
will be installed automatically).
To install the development version, run:
.. code-block:: bash
pip install git+git://github.com/iterative/dvc
|Packages|
Self-contained packages for Linux, Windows, and Mac are available.
The latest version of the packages can be found on the GitHub releases page <https://github.com/iterative/dvc/releases>
_.
Ubuntu / Debian (deb) ^^^^^^^^^^^^^^^^^^^^^ .. code-block:: bash
sudo wget https://dvc.org/deb/dvc.list -O /etc/apt/sources.list.d/dvc.list wget -qO - https://dvc.org/deb/iterative.asc | sudo apt-key add - sudo apt update sudo apt install dvc
Fedora / CentOS (rpm) ^^^^^^^^^^^^^^^^^^^^^ .. code-block:: bash
sudo wget https://dvc.org/rpm/dvc.repo -O /etc/yum.repos.d/dvc.repo sudo rpm --import https://dvc.org/rpm/iterative.asc sudo yum update sudo yum install dvc
|Maintainability|
Contributions are welcome!
Please see our Contributing Guide <https://dvc.org/doc/user-guide/contributing/core>
_ for more details.
Thanks to all our contributors!
|Contribs|
-
Twitter <https://twitter.com/DVCorg>
_ -
Forum <https://discuss.dvc.org/>
_ -
Discord Chat <https://dvc.org/chat>
_ -
Email <mailto:[email protected]>
_ -
Mailing List <https://sweedom.us10.list-manage.com/subscribe/post?u=a08bf93caae4063c4e6a351f6&id=24c0ecc49a>
_
This project is distributed under the Apache license version 2.0 (see the LICENSE file in the project root).
By submitting a pull request to this project, you agree to license your contribution under the Apache license version 2.0 to this project.
|DOI|
Iterative, DVC: Data Version Control - Git for Data & Models (2020)
DOI:10.5281/zenodo.012345 <https://doi.org/10.5281/zenodo.3677553>
_.
Barrak, A., Eghan, E.E. and Adams, B. On the Co-evolution of ML Pipelines and Source Code - Empirical Study of DVC Projects <https://mcis.cs.queensu.ca/publications/2021/saner.pdf>
_ , in Proceedings of the 28th IEEE International Conference on Software Analysis, Evolution, and Reengineering, SANER 2021. Hawaii, USA.
.. |Banner| image:: https://dvc.org/img/logo-github-readme.png :target: https://dvc.org :alt: DVC logo
.. |VS Code Extension Overview| image:: https://raw.githubusercontent.com/iterative/vscode-dvc/main/extension/docs/overview.gif :alt: DVC Extension for VS Code
.. |CI| image:: https://github.com/iterative/dvc/workflows/Tests/badge.svg?branch=main :target: https://github.com/iterative/dvc/actions :alt: GHA Tests
.. |Maintainability| image:: https://codeclimate.com/github/iterative/dvc/badges/gpa.svg :target: https://codeclimate.com/github/iterative/dvc :alt: Code Climate
.. |Python Version| image:: https://img.shields.io/pypi/pyversions/dvc :target: https://pypi.org/project/dvc :alt: Python Version
.. |Coverage| image:: https://codecov.io/gh/iterative/dvc/branch/main/graph/badge.svg :target: https://codecov.io/gh/iterative/dvc :alt: Codecov
.. |Snap| image:: https://img.shields.io/badge/snap-install-82BEA0.svg?logo=snapcraft :target: https://snapcraft.io/dvc :alt: Snapcraft
.. |Choco| image:: https://img.shields.io/chocolatey/v/dvc?label=choco :target: https://chocolatey.org/packages/dvc :alt: Chocolatey
.. |Brew| image:: https://img.shields.io/homebrew/v/dvc?label=brew :target: https://formulae.brew.sh/formula/dvc :alt: Homebrew
.. |Conda| image:: https://img.shields.io/conda/v/conda-forge/dvc.svg?label=conda&logo=conda-forge :target: https://anaconda.org/conda-forge/dvc :alt: Conda-forge
.. |PyPI| image:: https://img.shields.io/pypi/v/dvc.svg?label=pip&logo=PyPI&logoColor=white :target: https://pypi.org/project/dvc :alt: PyPI
.. |PyPI Downloads| image:: https://img.shields.io/pypi/dm/dvc.svg?color=blue&label=Downloads&logo=pypi&logoColor=gold :target: https://pypi.org/project/dvc :alt: PyPI Downloads
.. |Packages| image:: https://img.shields.io/badge/deb|pkg|rpm|exe-blue :target: https://dvc.org/doc/install :alt: deb|pkg|rpm|exe
.. |DOI| image:: https://img.shields.io/badge/DOI-10.5281/zenodo.3677553-blue.svg :target: https://doi.org/10.5281/zenodo.3677553 :alt: DOI
.. |Flowchart| image:: https://dvc.org/img/flow.gif :target: https://dvc.org/img/flow.gif :alt: how_dvc_works
.. |Contribs| image:: https://contrib.rocks/image?repo=iterative/dvc :target: https://github.com/iterative/dvc/graphs/contributors :alt: Contributors
.. |VS Code| image:: https://img.shields.io/visual-studio-marketplace/v/Iterative.dvc?color=blue&label=VSCode&logo=visualstudiocode&logoColor=blue :target: https://marketplace.visualstudio.com/items?itemName=Iterative.dvc :alt: VS Code Extension
For Tasks:
Click tags to check more tools for each tasksFor Jobs:
Alternative AI tools for dvc
Similar Open Source Tools
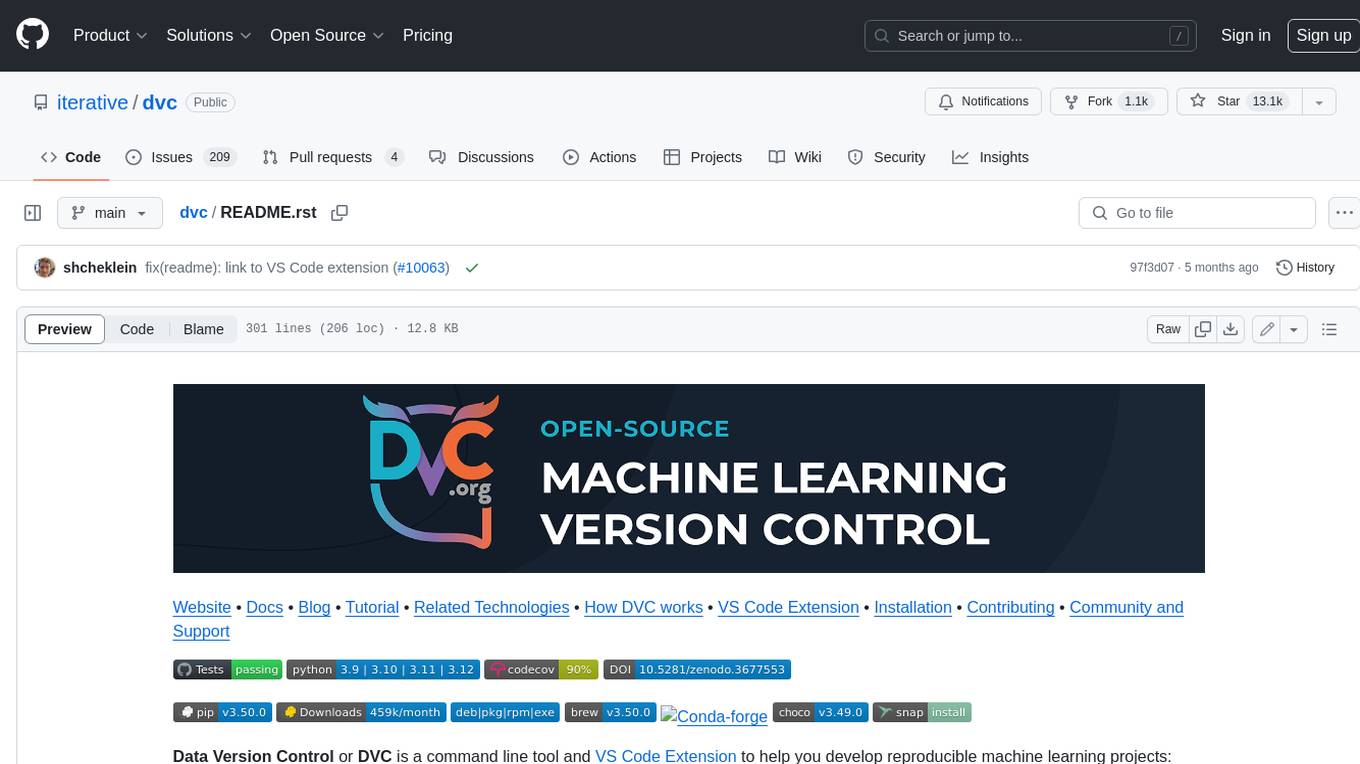
dvc
DVC, or Data Version Control, is a command-line tool and VS Code extension that helps you develop reproducible machine learning projects. With DVC, you can version your data and models, iterate fast with lightweight pipelines, track experiments in your local Git repo, compare any data, code, parameters, model, or performance plots, and share experiments and automatically reproduce anyone's experiment.
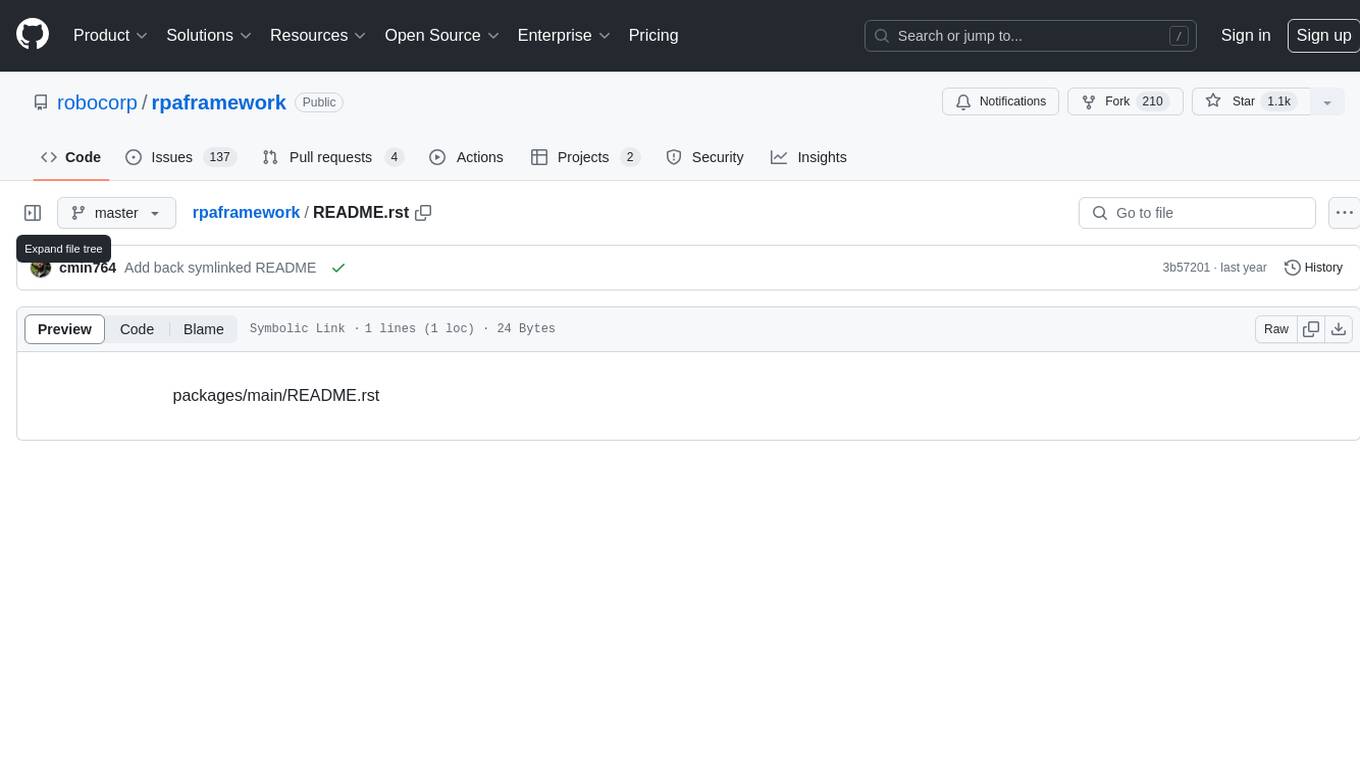
rpaframework
RPA Framework is an open-source collection of libraries and tools for Robotic Process Automation (RPA), designed to be used with Robot Framework and Python. It offers well-documented core libraries for Software Robot Developers, optimized for Robocorp Control Room and Developer Tools, and accepts external contributions. The project includes various libraries for tasks like archiving, browser automation, date/time manipulations, cloud services integration, encryption operations, database interactions, desktop automation, document processing, email operations, Excel manipulation, file system operations, FTP interactions, web API interactions, image manipulation, AI services, and more. The development of the repository is Python-based and requires Python version 3.8+, with tooling based on poetry and invoke for compiling, building, and running the package. The project is licensed under the Apache License 2.0.
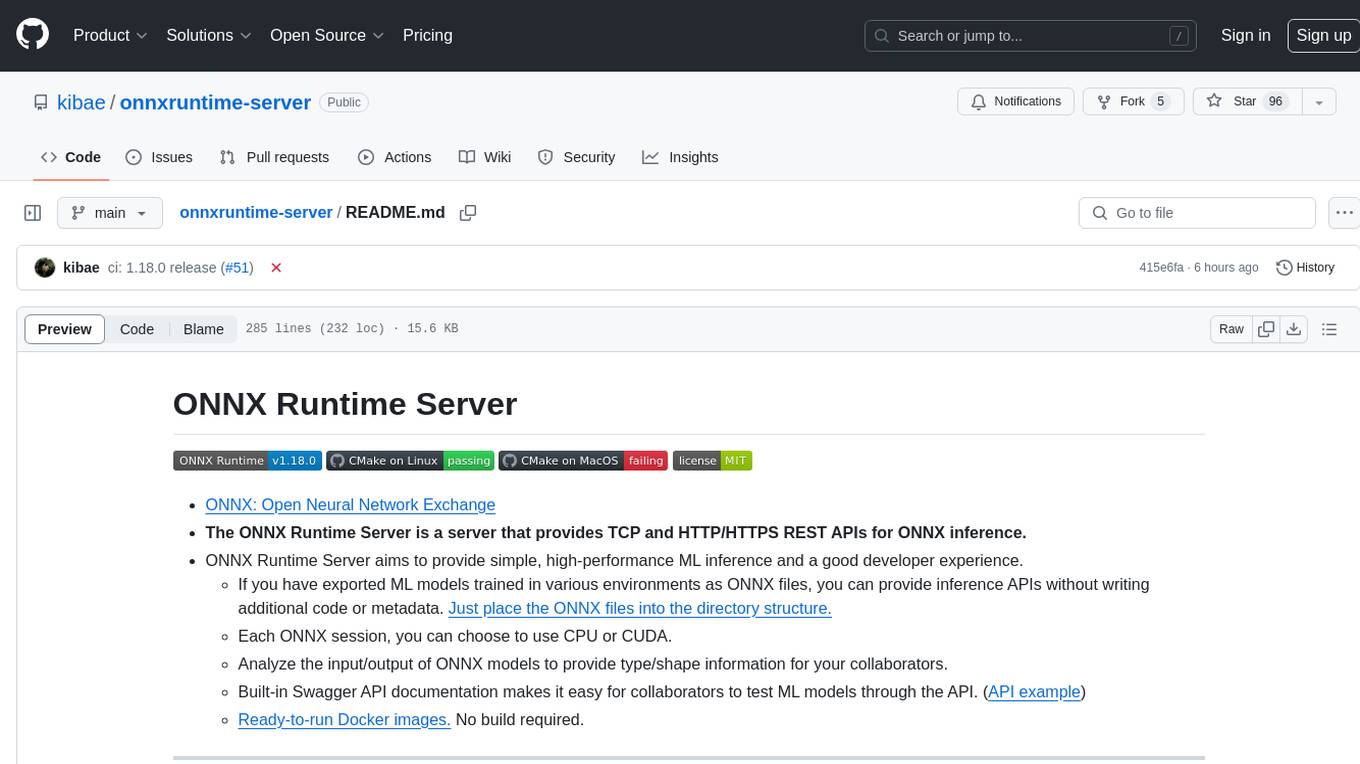
onnxruntime-server
ONNX Runtime Server is a server that provides TCP and HTTP/HTTPS REST APIs for ONNX inference. It aims to offer simple, high-performance ML inference and a good developer experience. Users can provide inference APIs for ONNX models without writing additional code by placing the models in the directory structure. Each session can choose between CPU or CUDA, analyze input/output, and provide Swagger API documentation for easy testing. Ready-to-run Docker images are available, making it convenient to deploy the server.
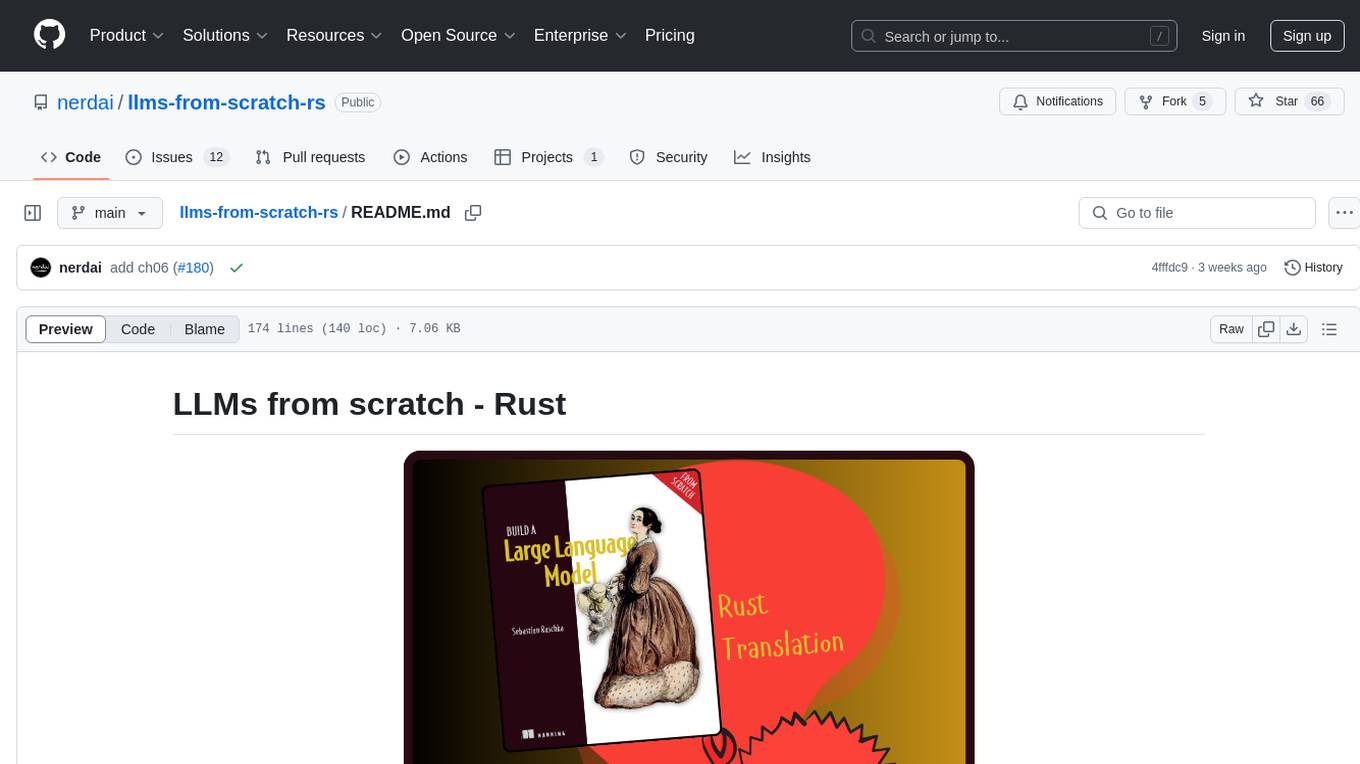
llms-from-scratch-rs
This project provides Rust code that follows the text 'Build An LLM From Scratch' by Sebastian Raschka. It translates PyTorch code into Rust using the Candle crate, aiming to build a GPT-style LLM. Users can clone the repo, run examples/exercises, and access the same datasets as in the book. The project includes chapters on understanding large language models, working with text data, coding attention mechanisms, implementing a GPT model, pretraining unlabeled data, fine-tuning for classification, and fine-tuning to follow instructions.
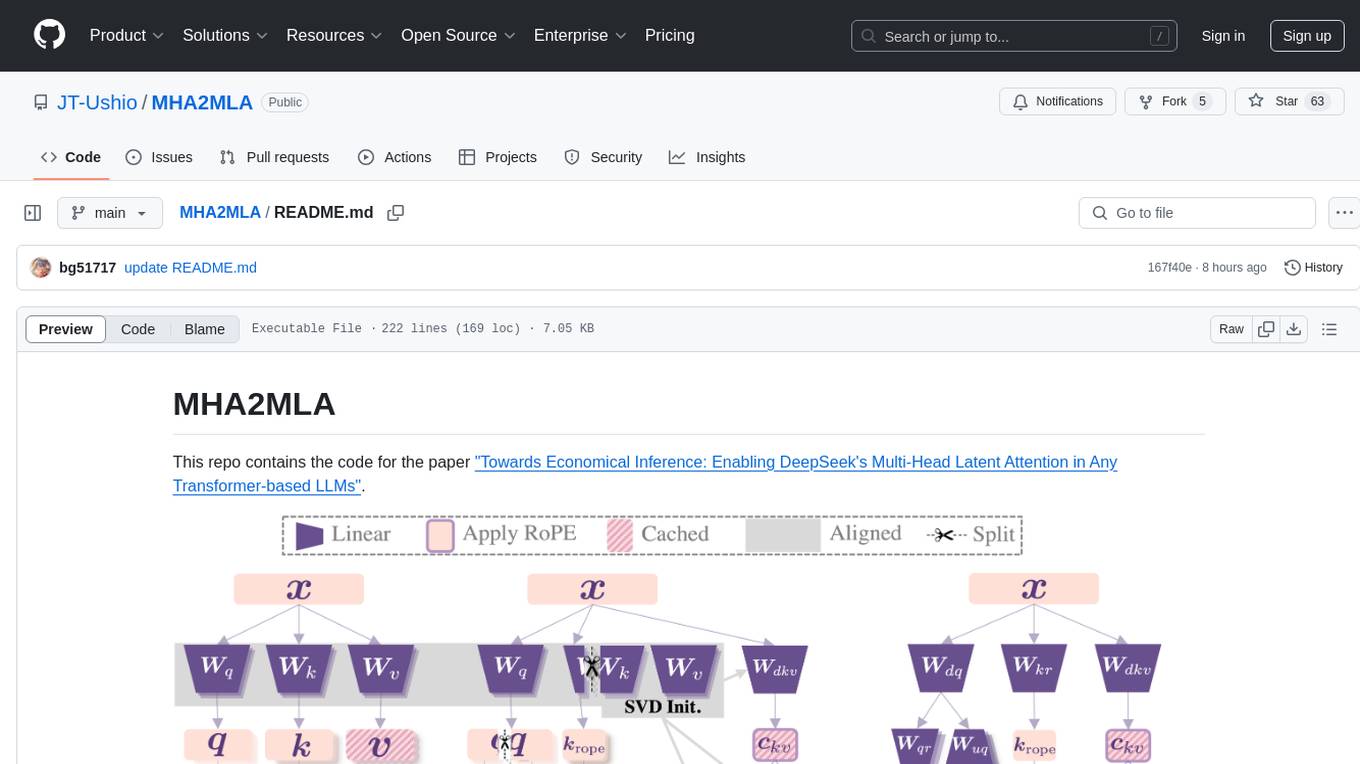
MHA2MLA
This repository contains the code for the paper 'Towards Economical Inference: Enabling DeepSeek's Multi-Head Latent Attention in Any Transformer-based LLMs'. It provides tools for fine-tuning and evaluating Llama models, converting models between different frameworks, processing datasets, and performing specific model training tasks like Partial-RoPE Fine-Tuning and Multiple-Head Latent Attention Fine-Tuning. The repository also includes commands for model evaluation using Lighteval and LongBench, along with necessary environment setup instructions.
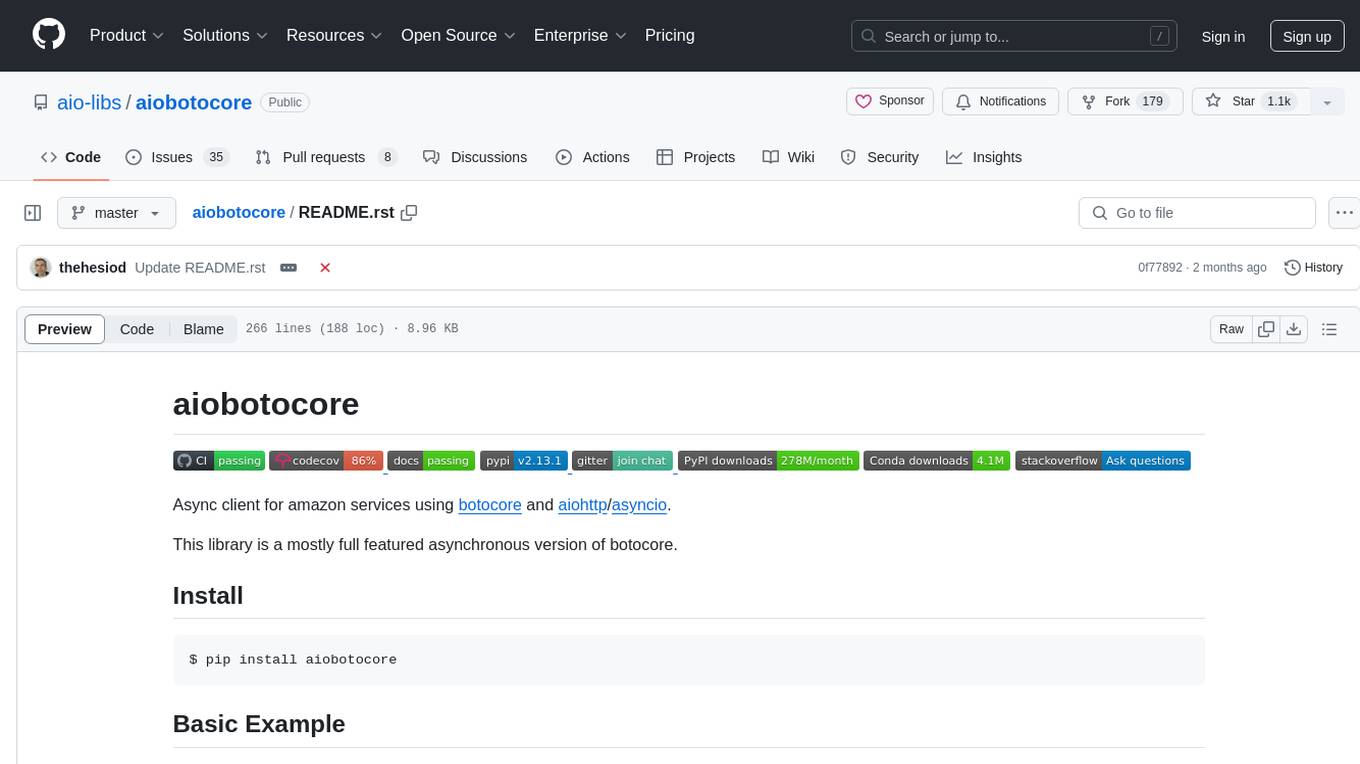
aiobotocore
aiobotocore is an async client for Amazon services using botocore and aiohttp/asyncio. It provides a mostly full-featured asynchronous version of botocore, allowing users to interact with various AWS services asynchronously. The library supports operations such as uploading objects to S3, getting object properties, listing objects, and deleting objects. It also offers context manager examples for managing resources efficiently. aiobotocore supports multiple AWS services like S3, DynamoDB, SNS, SQS, CloudFormation, and Kinesis, with basic methods tested for each service. Users can run tests using moto for mocked tests or against personal Amazon keys. Additionally, the tool enables type checking and code completion for better development experience.
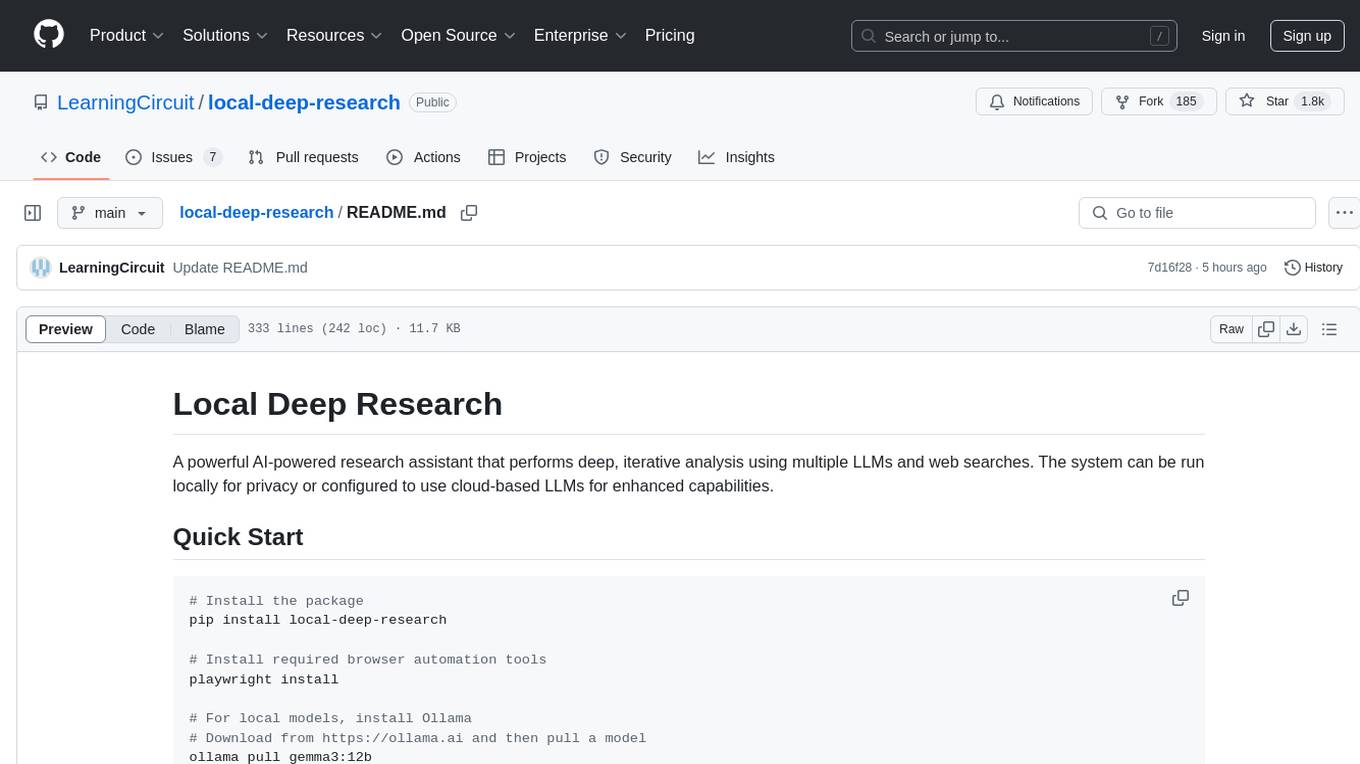
local-deep-research
Local Deep Research is a powerful AI-powered research assistant that performs deep, iterative analysis using multiple LLMs and web searches. It can be run locally for privacy or configured to use cloud-based LLMs for enhanced capabilities. The tool offers advanced research capabilities, flexible LLM support, rich output options, privacy-focused operation, enhanced search integration, and academic & scientific integration. It also provides a web interface, command line interface, and supports multiple LLM providers and search engines. Users can configure AI models, search engines, and research parameters for customized research experiences.
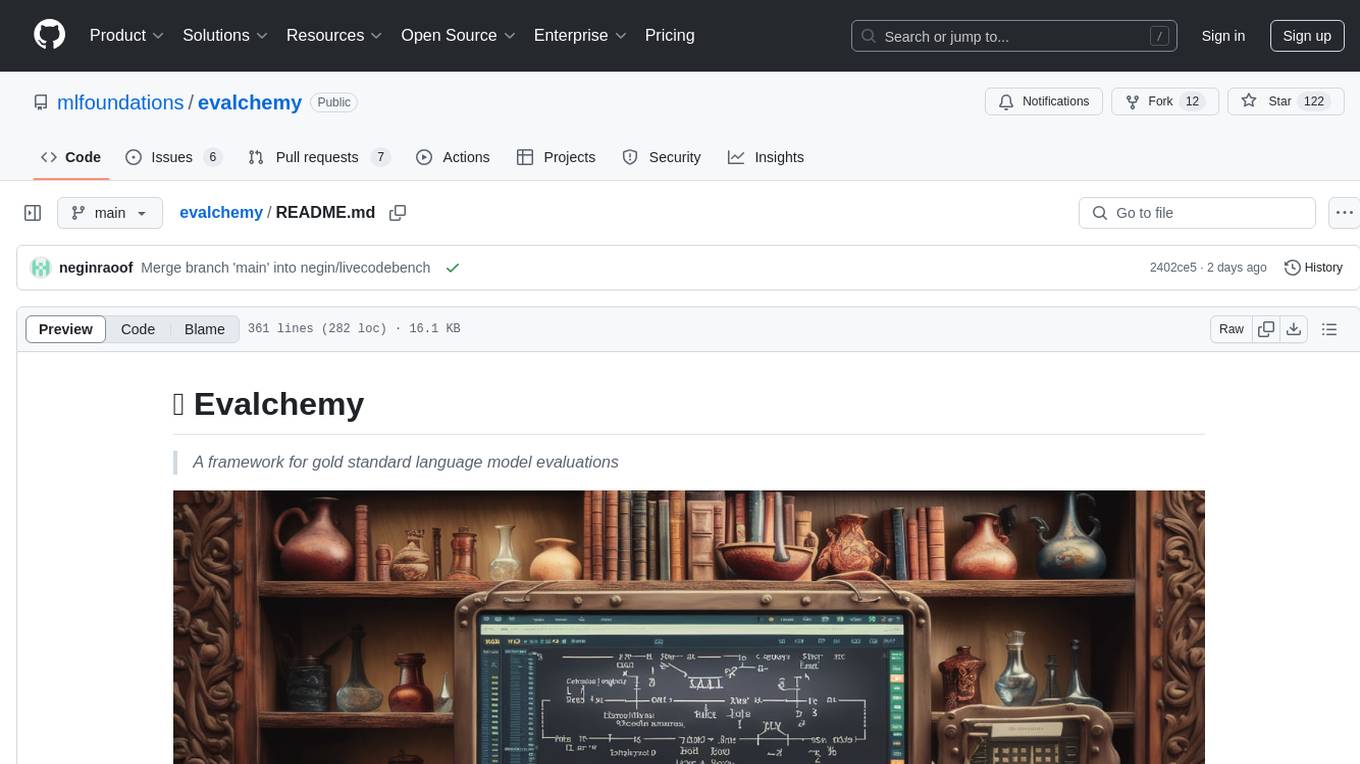
evalchemy
Evalchemy is a unified and easy-to-use toolkit for evaluating language models, focusing on post-trained models. It integrates multiple existing benchmarks such as RepoBench, AlpacaEval, and ZeroEval. Key features include unified installation, parallel evaluation, simplified usage, and results management. Users can run various benchmarks with a consistent command-line interface and track results locally or integrate with a database for systematic tracking and leaderboard submission.
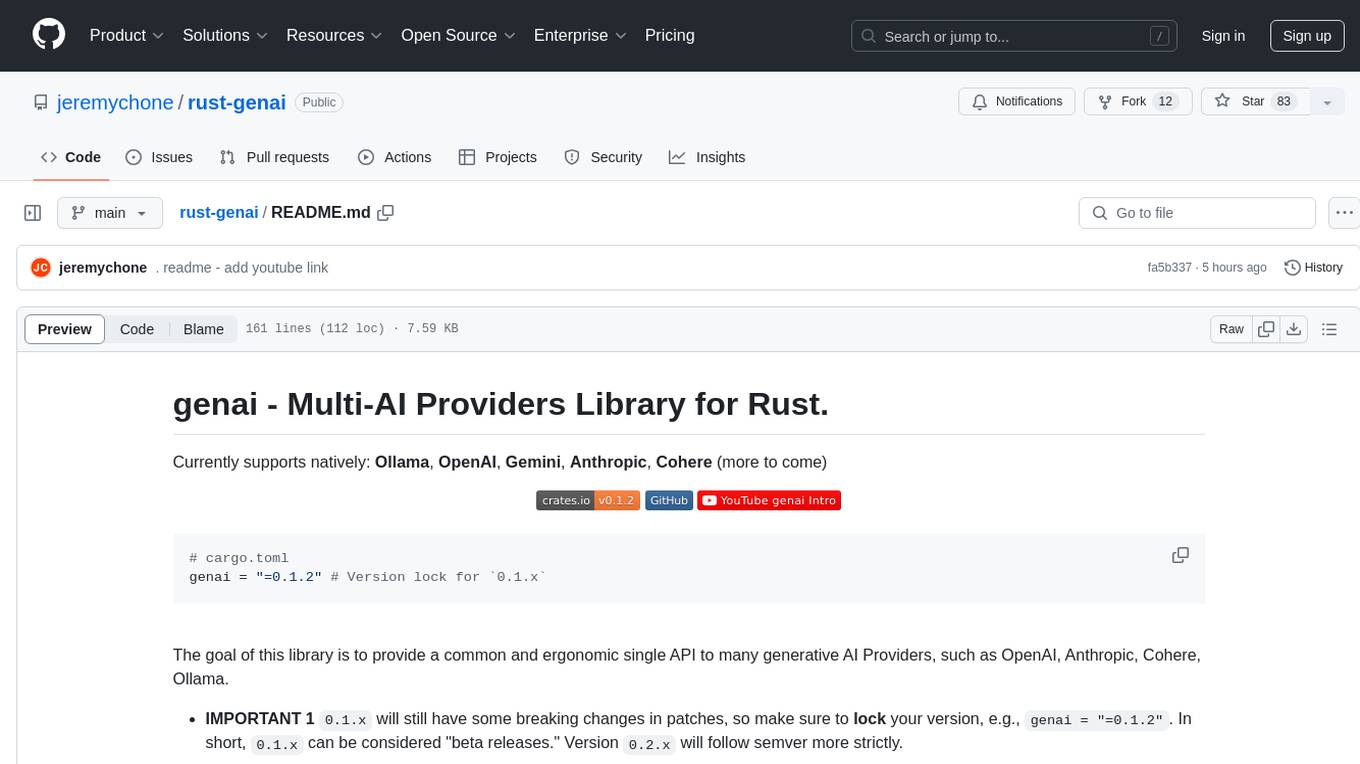
rust-genai
genai is a multi-AI providers library for Rust that aims to provide a common and ergonomic single API to various generative AI providers such as OpenAI, Anthropic, Cohere, Ollama, and Gemini. It focuses on standardizing chat completion APIs across major AI services, prioritizing ergonomics and commonality. The library initially focuses on text chat APIs and plans to expand to support images, function calling, and more in the future versions. Version 0.1.x will have breaking changes in patches, while version 0.2.x will follow semver more strictly. genai does not provide a full representation of a given AI provider but aims to simplify the differences at a lower layer for ease of use.
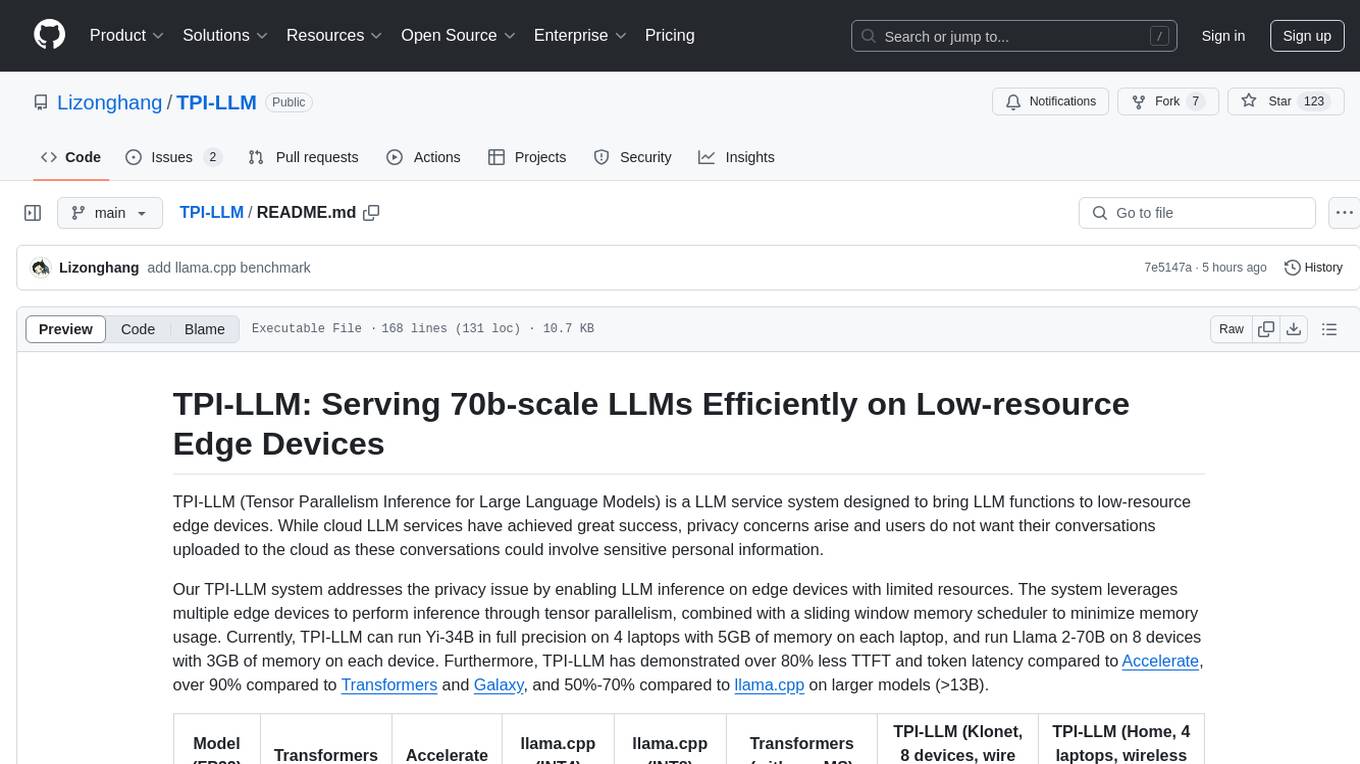
TPI-LLM
TPI-LLM (Tensor Parallelism Inference for Large Language Models) is a system designed to bring LLM functions to low-resource edge devices, addressing privacy concerns by enabling LLM inference on edge devices with limited resources. It leverages multiple edge devices for inference through tensor parallelism and a sliding window memory scheduler to minimize memory usage. TPI-LLM demonstrates significant improvements in TTFT and token latency compared to other models, and plans to support infinitely large models with low token latency in the future.
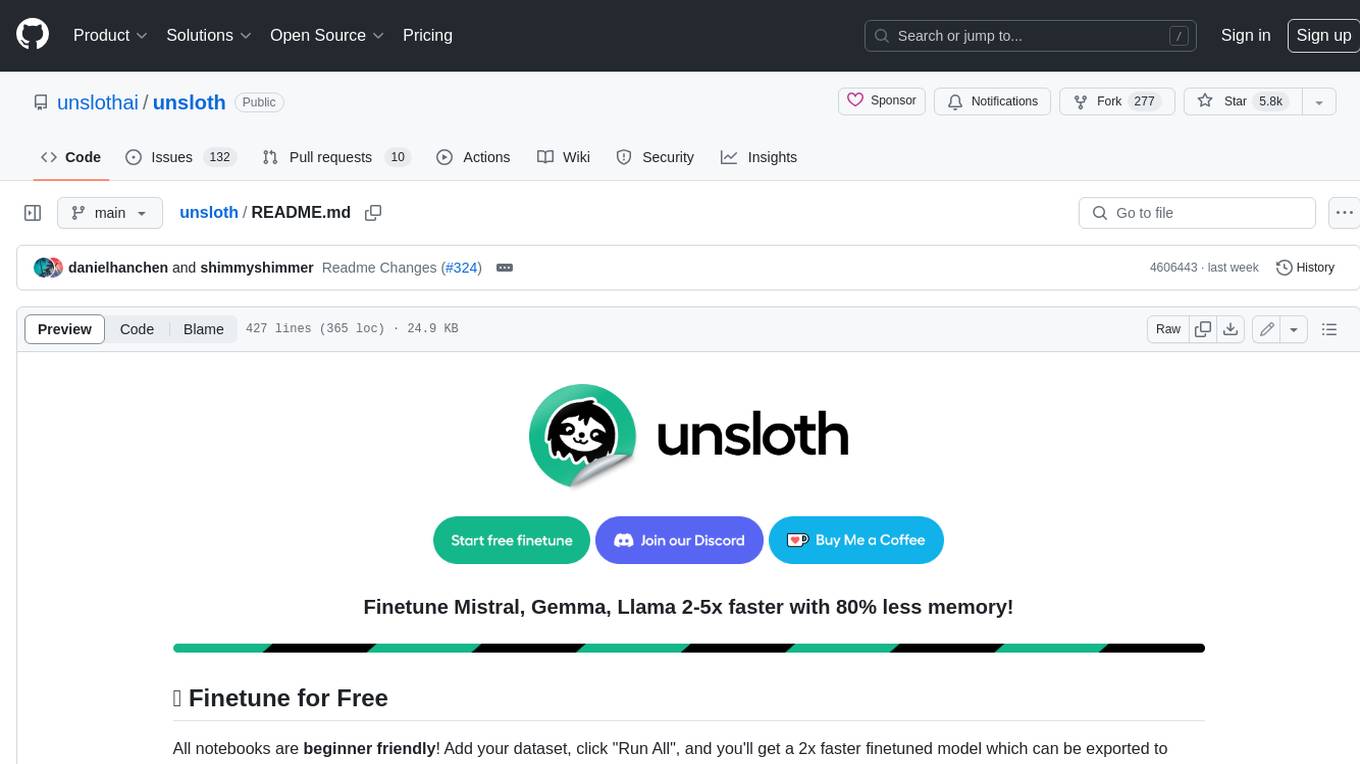
unsloth
Unsloth is a tool that allows users to fine-tune large language models (LLMs) 2-5x faster with 80% less memory. It is a free and open-source tool that can be used to fine-tune LLMs such as Gemma, Mistral, Llama 2-5, TinyLlama, and CodeLlama 34b. Unsloth supports 4-bit and 16-bit QLoRA / LoRA fine-tuning via bitsandbytes. It also supports DPO (Direct Preference Optimization), PPO, and Reward Modelling. Unsloth is compatible with Hugging Face's TRL, Trainer, Seq2SeqTrainer, and Pytorch code. It is also compatible with NVIDIA GPUs since 2018+ (minimum CUDA Capability 7.0).
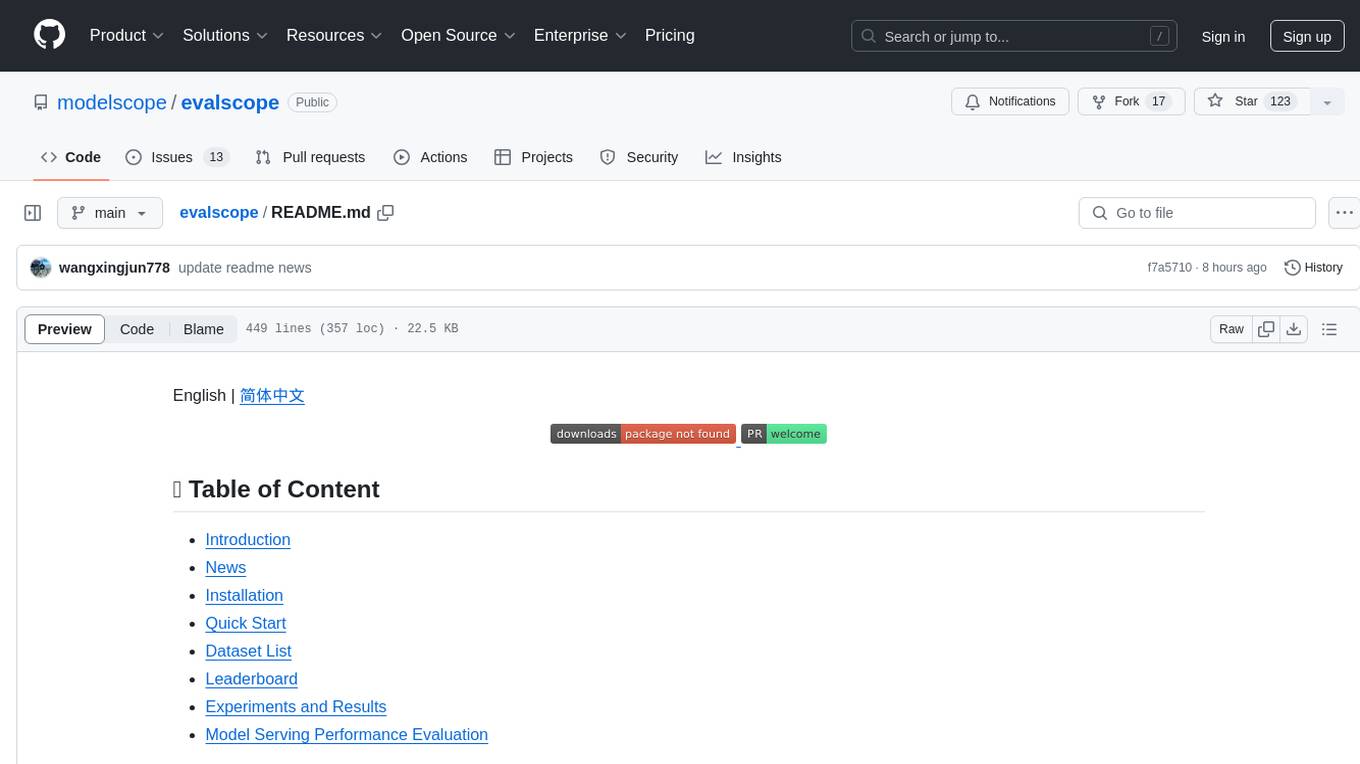
evalscope
Eval-Scope is a framework designed to support the evaluation of large language models (LLMs) by providing pre-configured benchmark datasets, common evaluation metrics, model integration, automatic evaluation for objective questions, complex task evaluation using expert models, reports generation, visualization tools, and model inference performance evaluation. It is lightweight, easy to customize, supports new dataset integration, model hosting on ModelScope, deployment of locally hosted models, and rich evaluation metrics. Eval-Scope also supports various evaluation modes like single mode, pairwise-baseline mode, and pairwise (all) mode, making it suitable for assessing and improving LLMs.
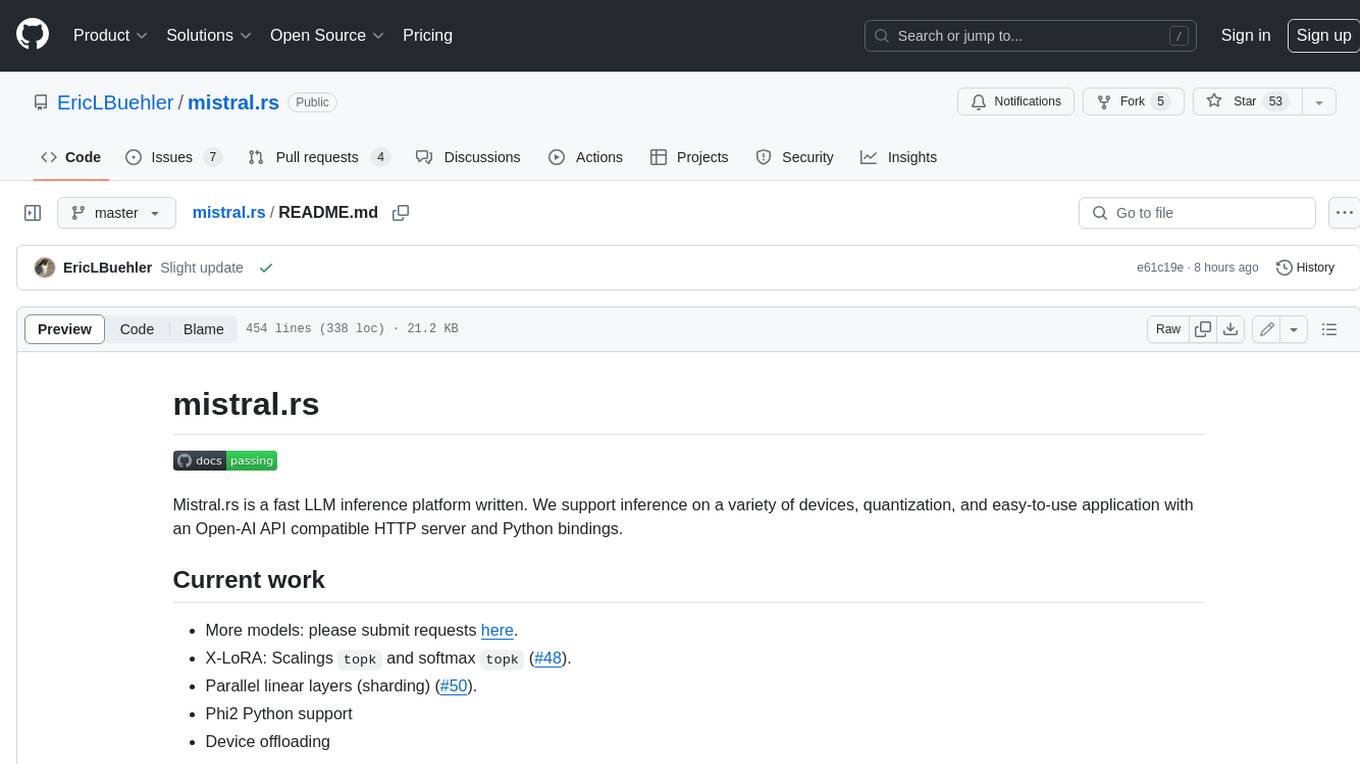
mistral.rs
Mistral.rs is a fast LLM inference platform written in Rust. We support inference on a variety of devices, quantization, and easy-to-use application with an Open-AI API compatible HTTP server and Python bindings.
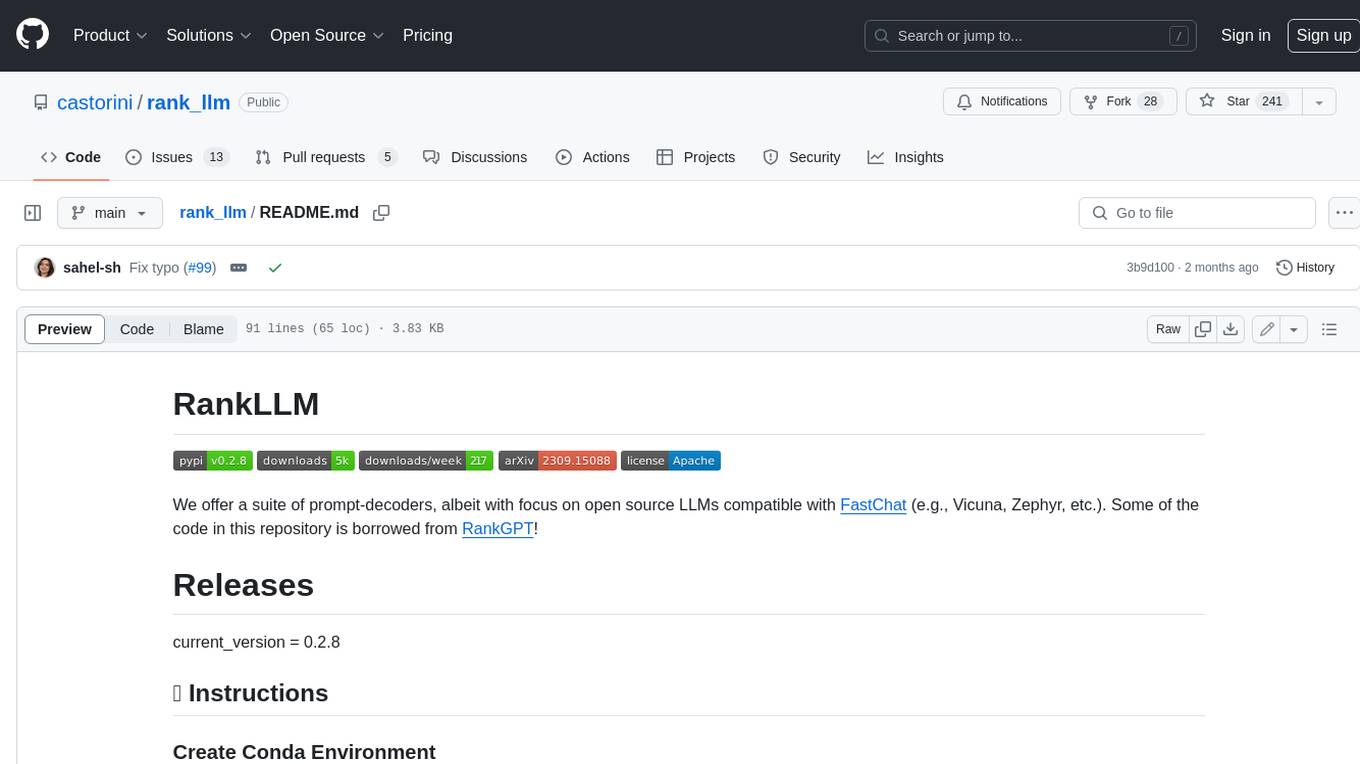
rank_llm
RankLLM is a suite of prompt-decoders compatible with open source LLMs like Vicuna and Zephyr. It allows users to create custom ranking models for various NLP tasks, such as document reranking, question answering, and summarization. The tool offers a variety of features, including the ability to fine-tune models on custom datasets, use different retrieval methods, and control the context size and variable passages. RankLLM is easy to use and can be integrated into existing NLP pipelines.
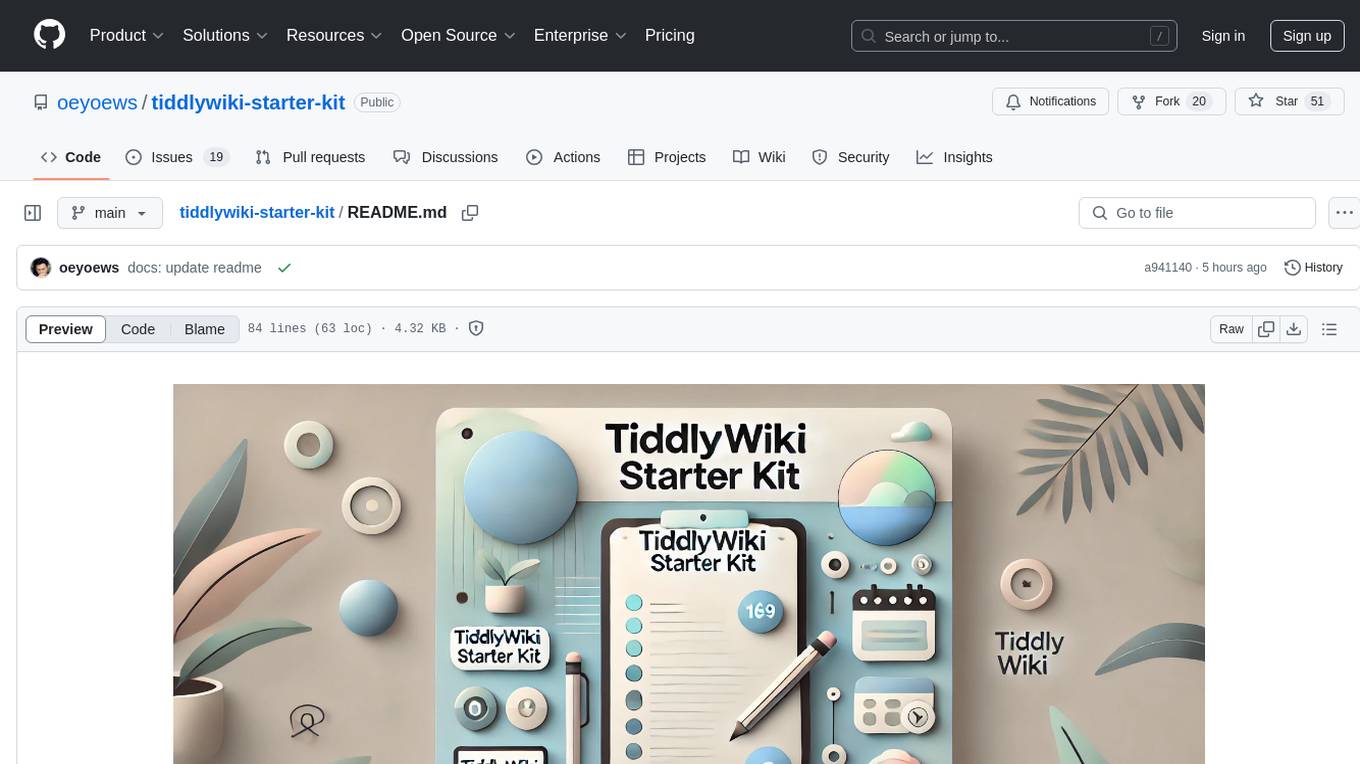
tiddlywiki-starter-kit
TiddlyWiki Starter Kit is a pre-configured setup for TiddlyWiki, utilizing Tailwind CSS for responsive design and providing multiple wiki support for different purposes. It offers quick operations with keyboard shortcuts, simplified configuration through editing the .env file, and one-click installation using npm create command.
For similar tasks
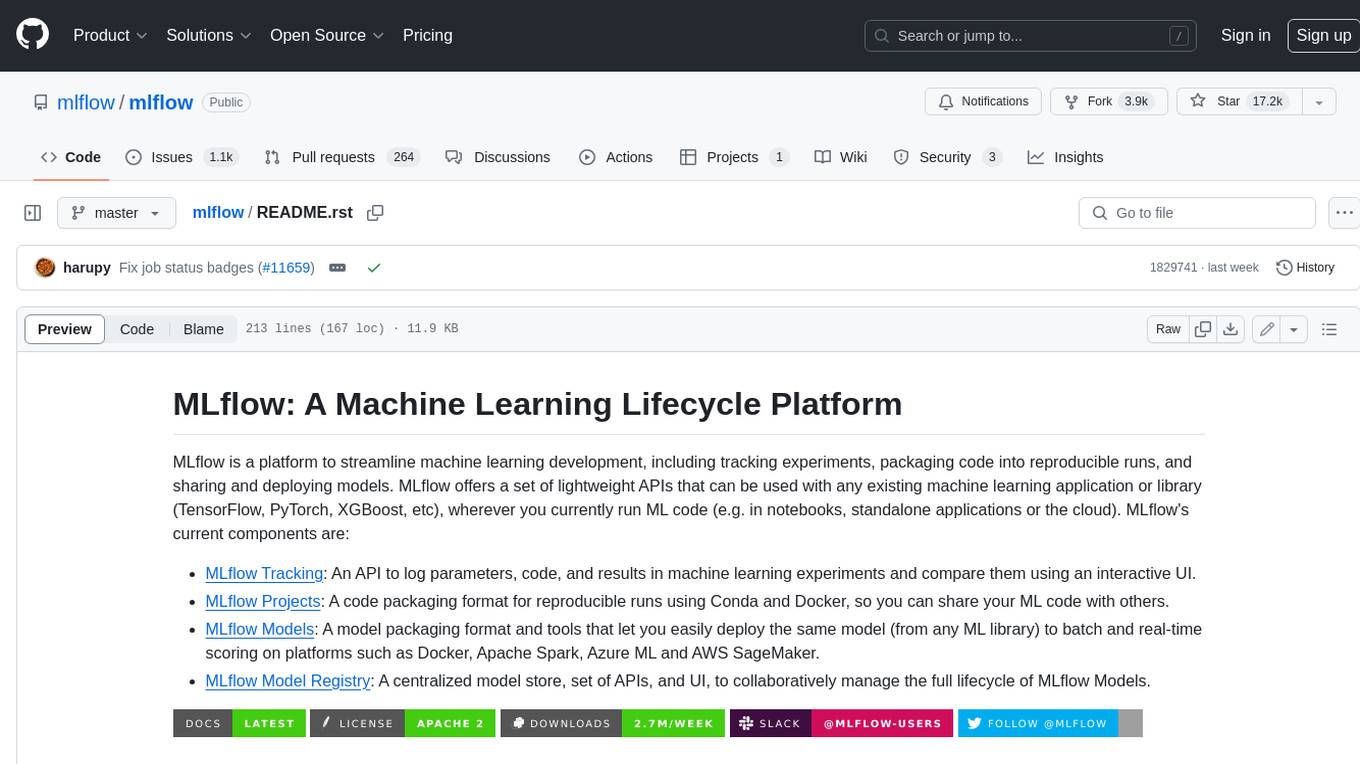
mlflow
MLflow is a platform to streamline machine learning development, including tracking experiments, packaging code into reproducible runs, and sharing and deploying models. MLflow offers a set of lightweight APIs that can be used with any existing machine learning application or library (TensorFlow, PyTorch, XGBoost, etc), wherever you currently run ML code (e.g. in notebooks, standalone applications or the cloud). MLflow's current components are:
* `MLflow Tracking
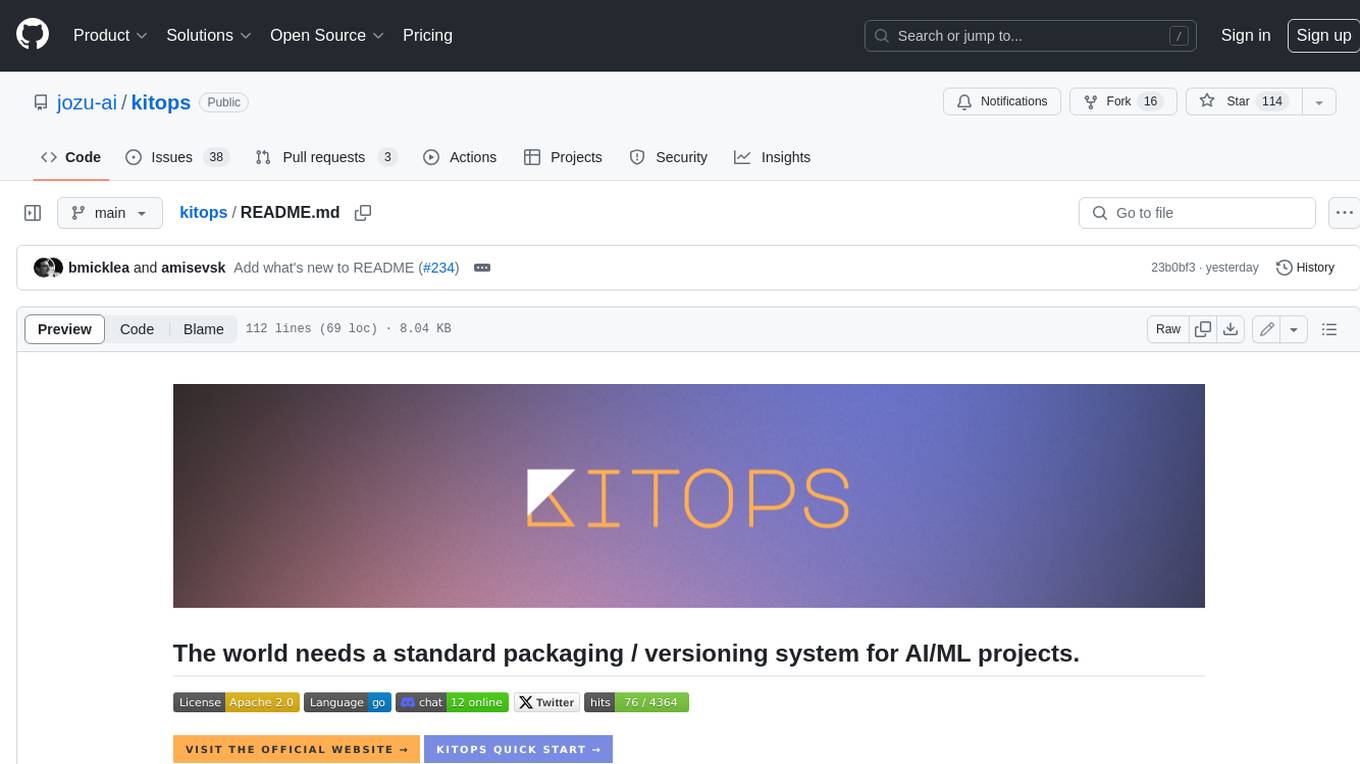
kitops
KitOps is a packaging and versioning system for AI/ML projects that uses open standards so it works with the AI/ML, development, and DevOps tools you are already using. KitOps simplifies the handoffs between data scientists, application developers, and SREs working with LLMs and other AI/ML models. KitOps' ModelKits are a standards-based package for models, their dependencies, configurations, and codebases. ModelKits are portable, reproducible, and work with the tools you already use.
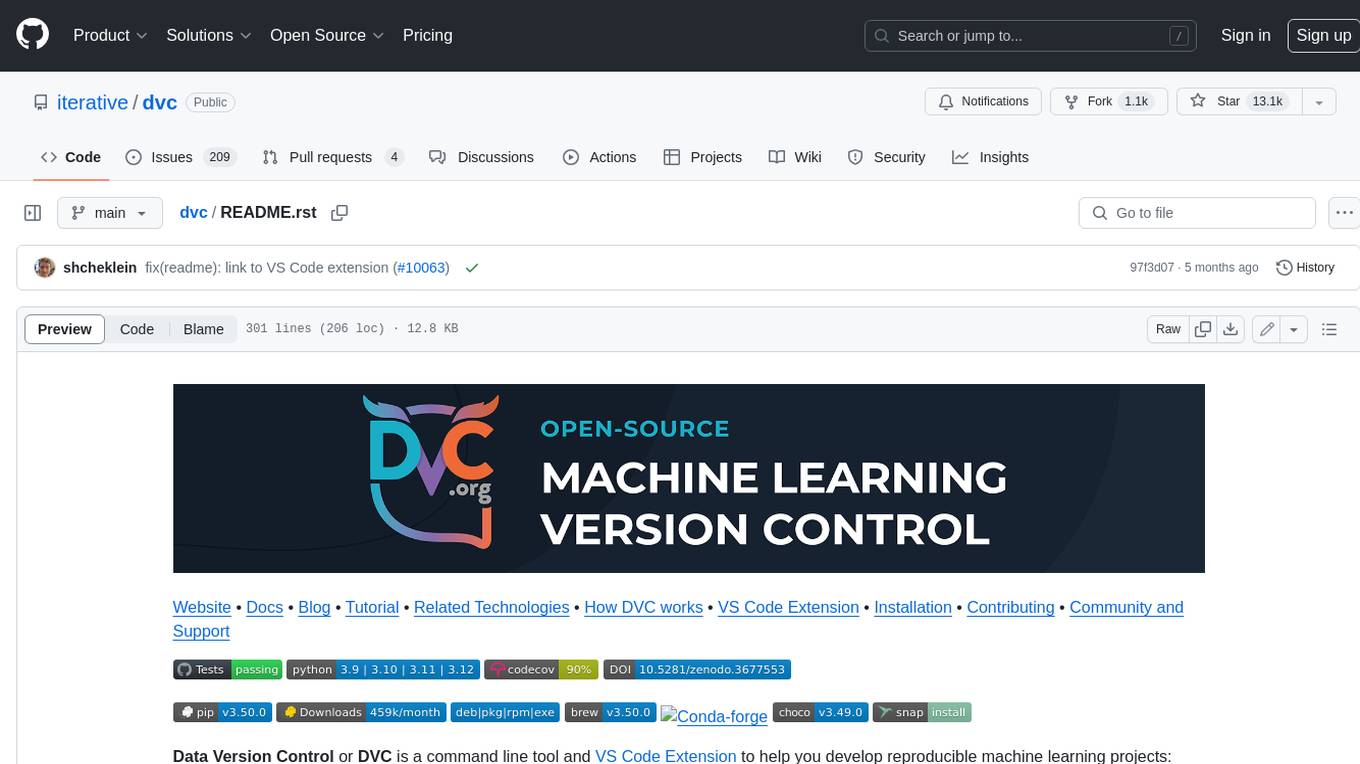
dvc
DVC, or Data Version Control, is a command-line tool and VS Code extension that helps you develop reproducible machine learning projects. With DVC, you can version your data and models, iterate fast with lightweight pipelines, track experiments in your local Git repo, compare any data, code, parameters, model, or performance plots, and share experiments and automatically reproduce anyone's experiment.
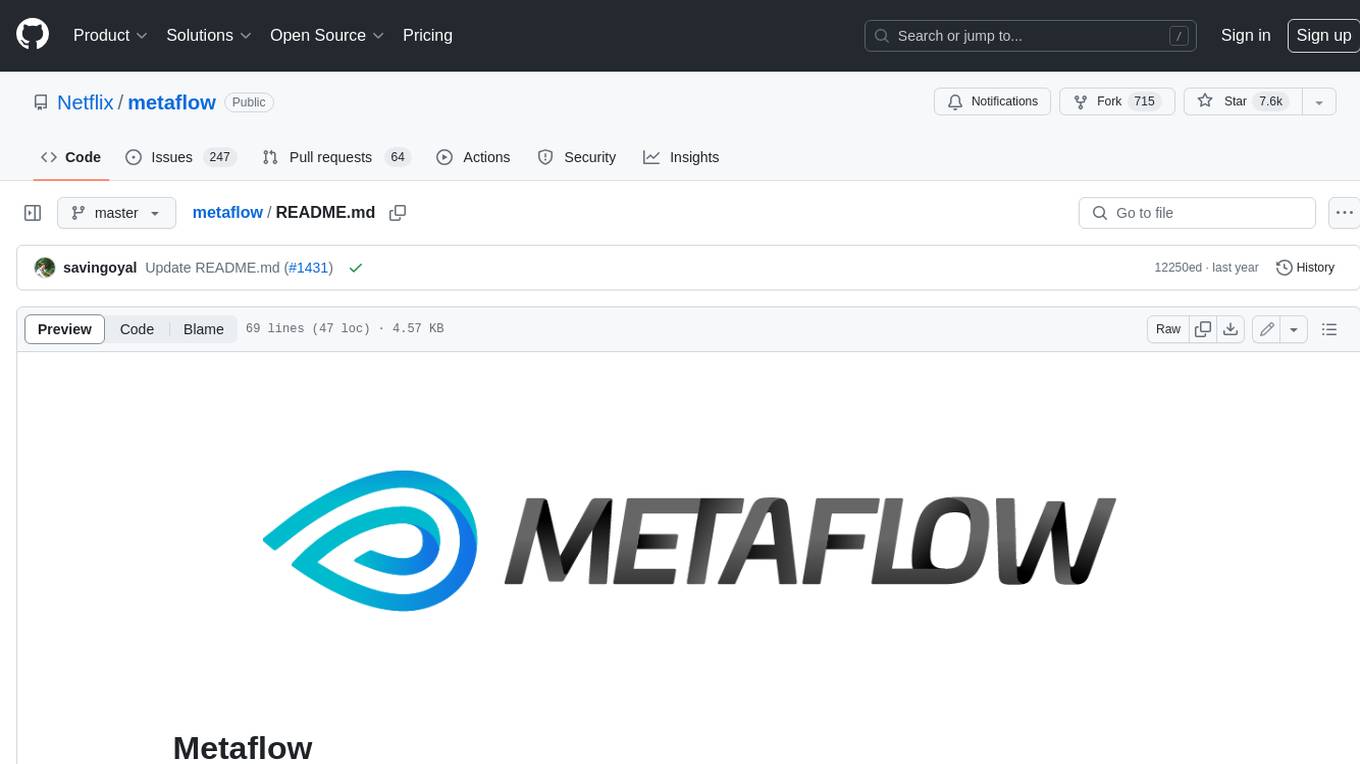
metaflow
Metaflow is a user-friendly library designed to assist scientists and engineers in developing and managing real-world data science projects. Initially created at Netflix, Metaflow aimed to enhance the productivity of data scientists working on diverse projects ranging from traditional statistics to cutting-edge deep learning. For further information, refer to Metaflow's website and documentation.
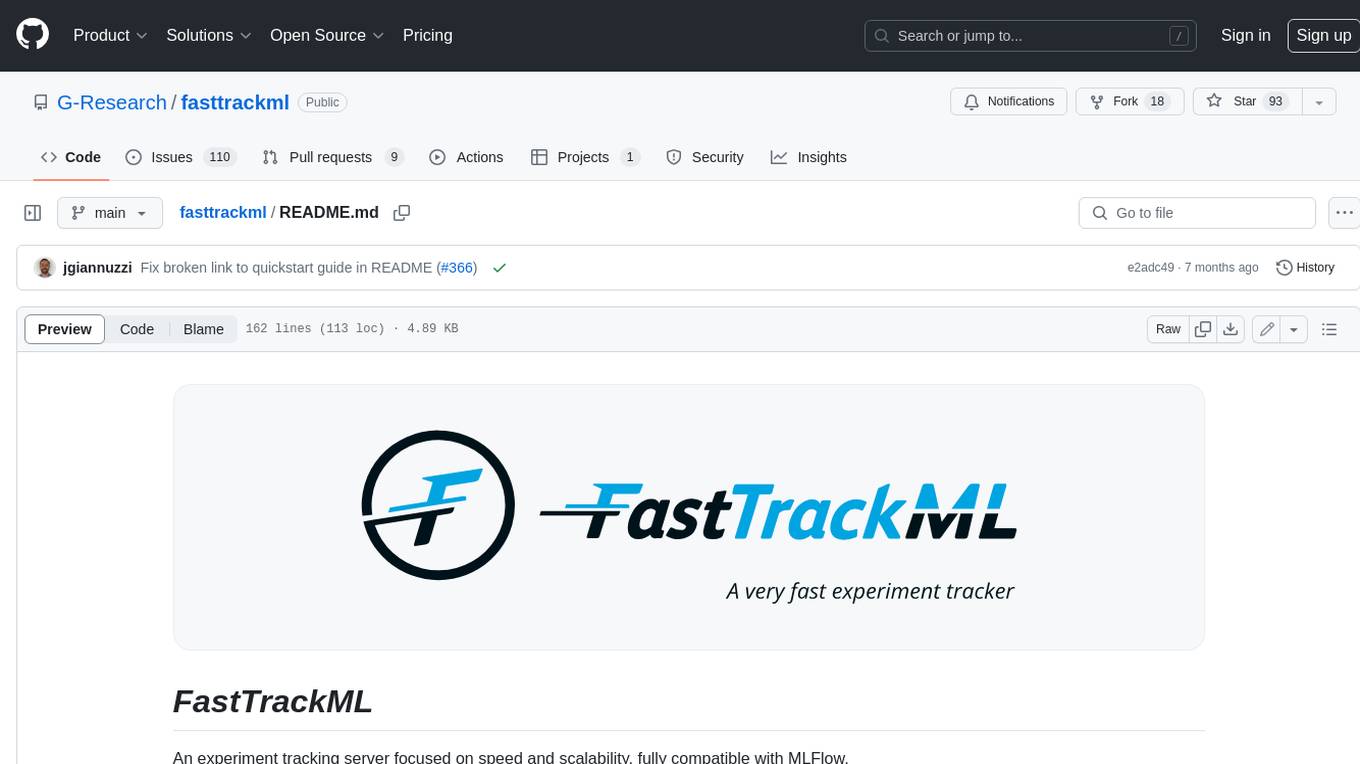
fasttrackml
FastTrackML is an experiment tracking server focused on speed and scalability, fully compatible with MLFlow. It provides a user-friendly interface to track and visualize your machine learning experiments, making it easy to compare different models and identify the best performing ones. FastTrackML is open source and can be easily installed and run with pip or Docker. It is also compatible with the MLFlow Python package, making it easy to integrate with your existing MLFlow workflows.
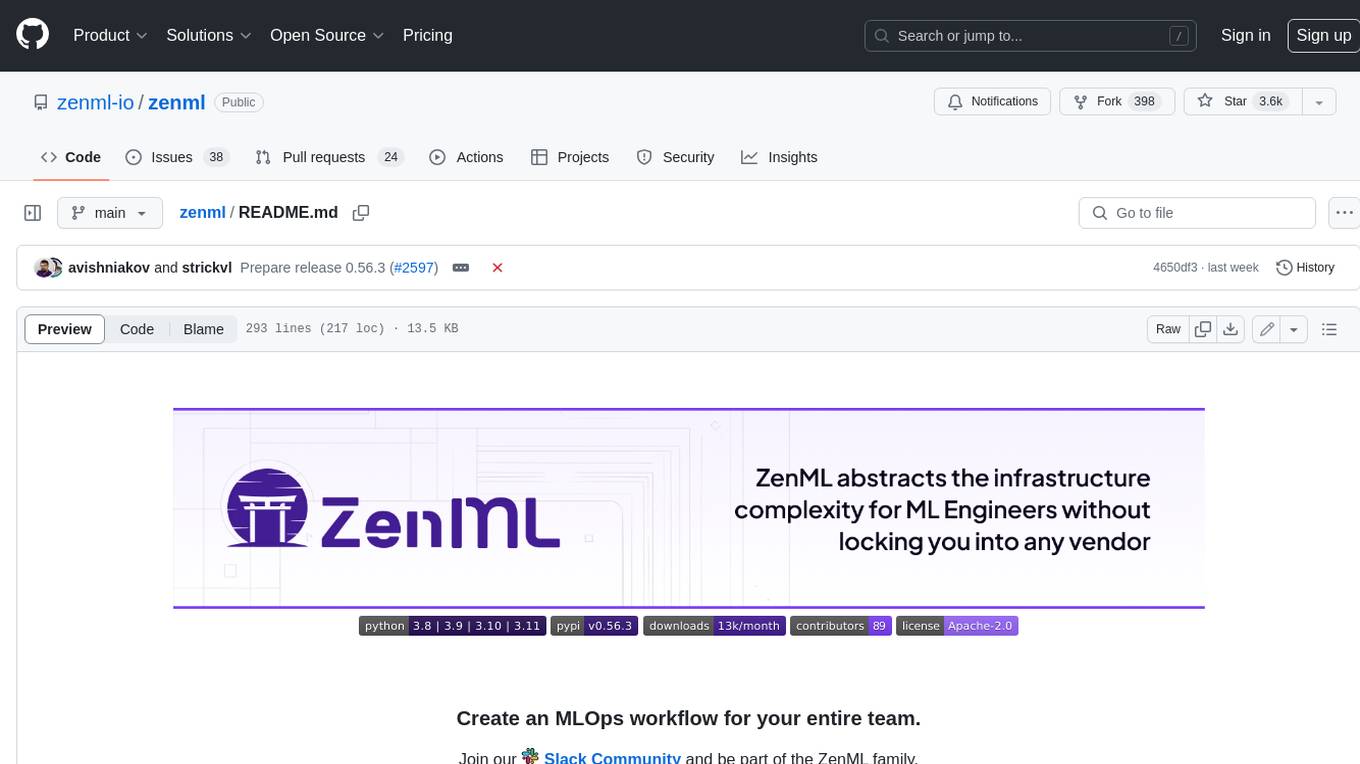
zenml
ZenML is an extensible, open-source MLOps framework for creating portable, production-ready machine learning pipelines. By decoupling infrastructure from code, ZenML enables developers across your organization to collaborate more effectively as they develop to production.
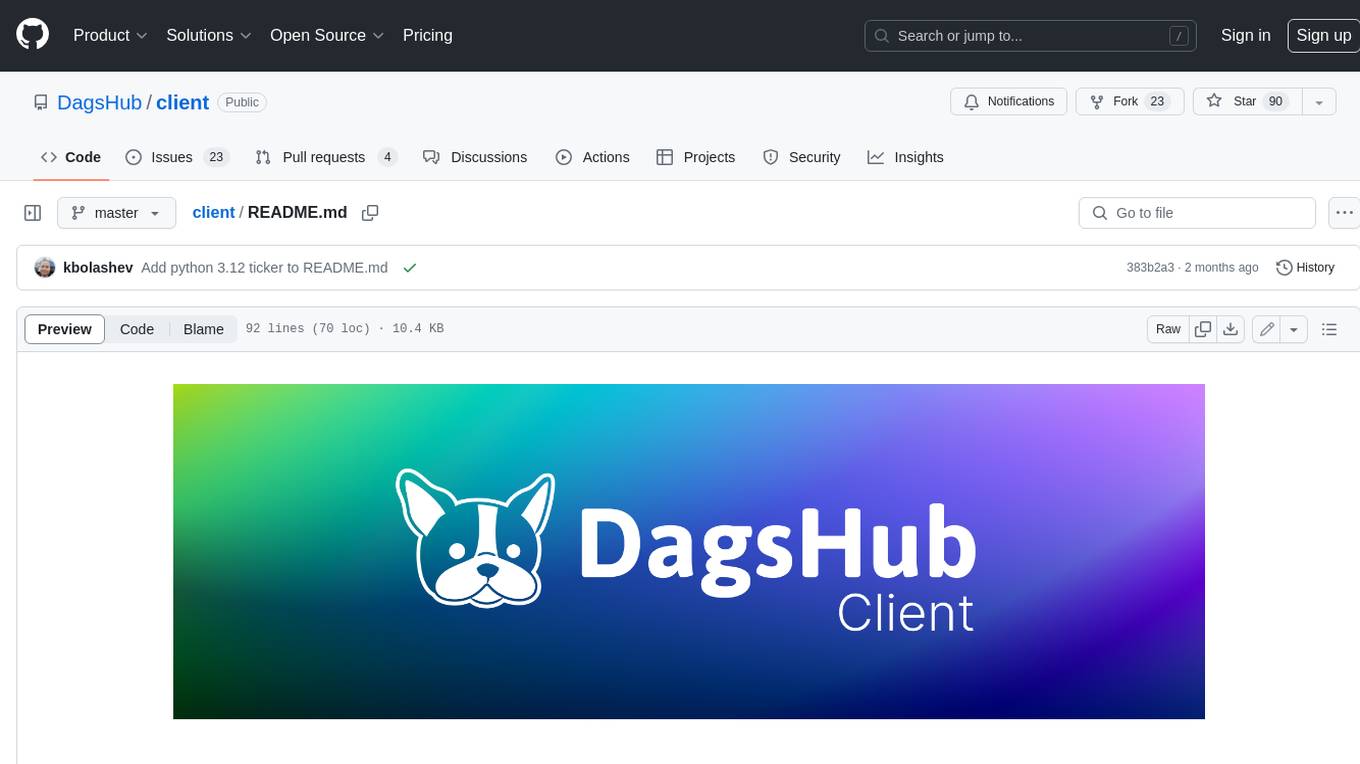
client
DagsHub is a platform for machine learning and data science teams to build, manage, and collaborate on their projects. With DagsHub you can: 1. Version code, data, and models in one place. Use the free provided DagsHub storage or connect it to your cloud storage 2. Track Experiments using Git, DVC or MLflow, to provide a fully reproducible environment 3. Visualize pipelines, data, and notebooks in and interactive, diff-able, and dynamic way 4. Label your data directly on the platform using Label Studio 5. Share your work with your team members 6. Stream and upload your data in an intuitive and easy way, while preserving versioning and structure. DagsHub is built firmly around open, standard formats for your project. In particular: * Git * DVC * MLflow * Label Studio * Standard data formats like YAML, JSON, CSV Therefore, you can work with DagsHub regardless of your chosen programming language or frameworks.
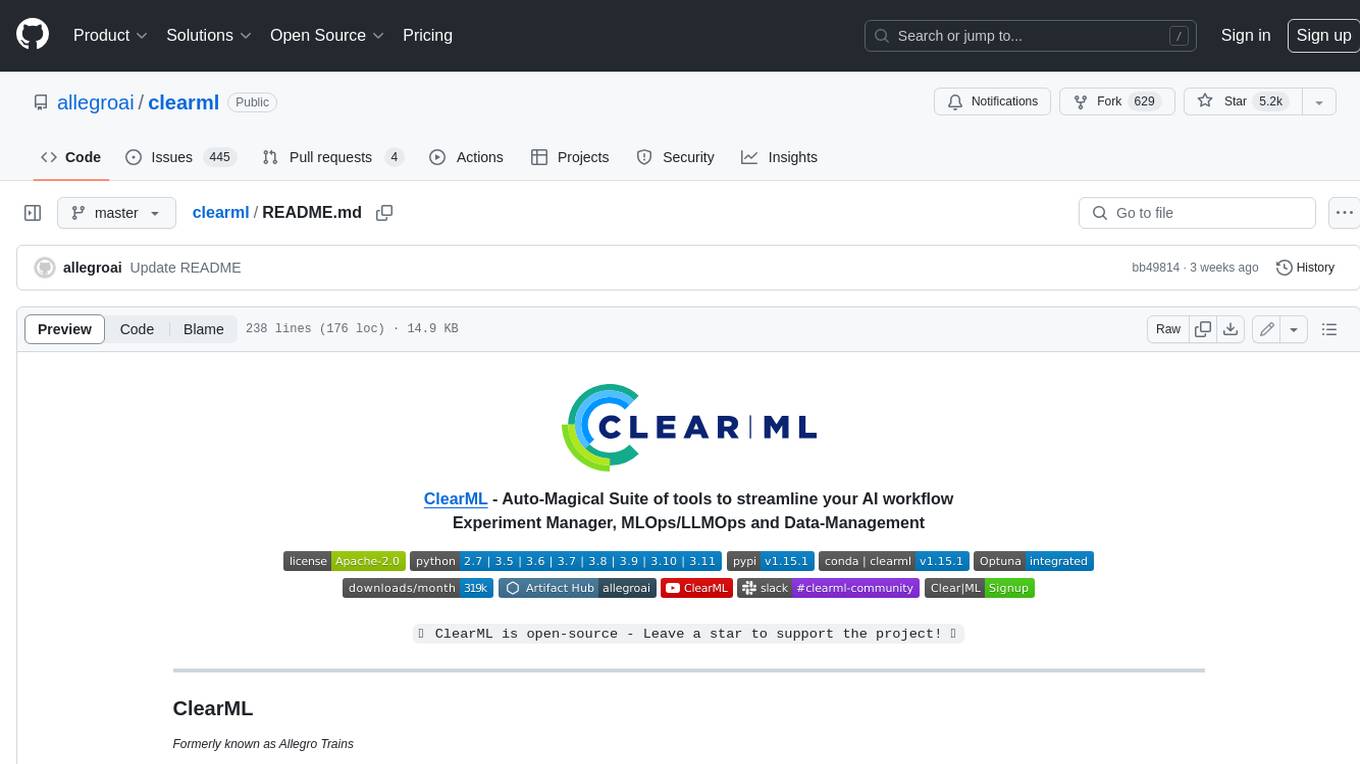
clearml
ClearML is a suite of tools designed to streamline the machine learning workflow. It includes an experiment manager, MLOps/LLMOps, data management, and model serving capabilities. ClearML is open-source and offers a free tier hosting option. It supports various ML/DL frameworks and integrates with Jupyter Notebook and PyCharm. ClearML provides extensive logging capabilities, including source control info, execution environment, hyper-parameters, and experiment outputs. It also offers automation features, such as remote job execution and pipeline creation. ClearML is designed to be easy to integrate, requiring only two lines of code to add to existing scripts. It aims to improve collaboration, visibility, and data transparency within ML teams.
For similar jobs
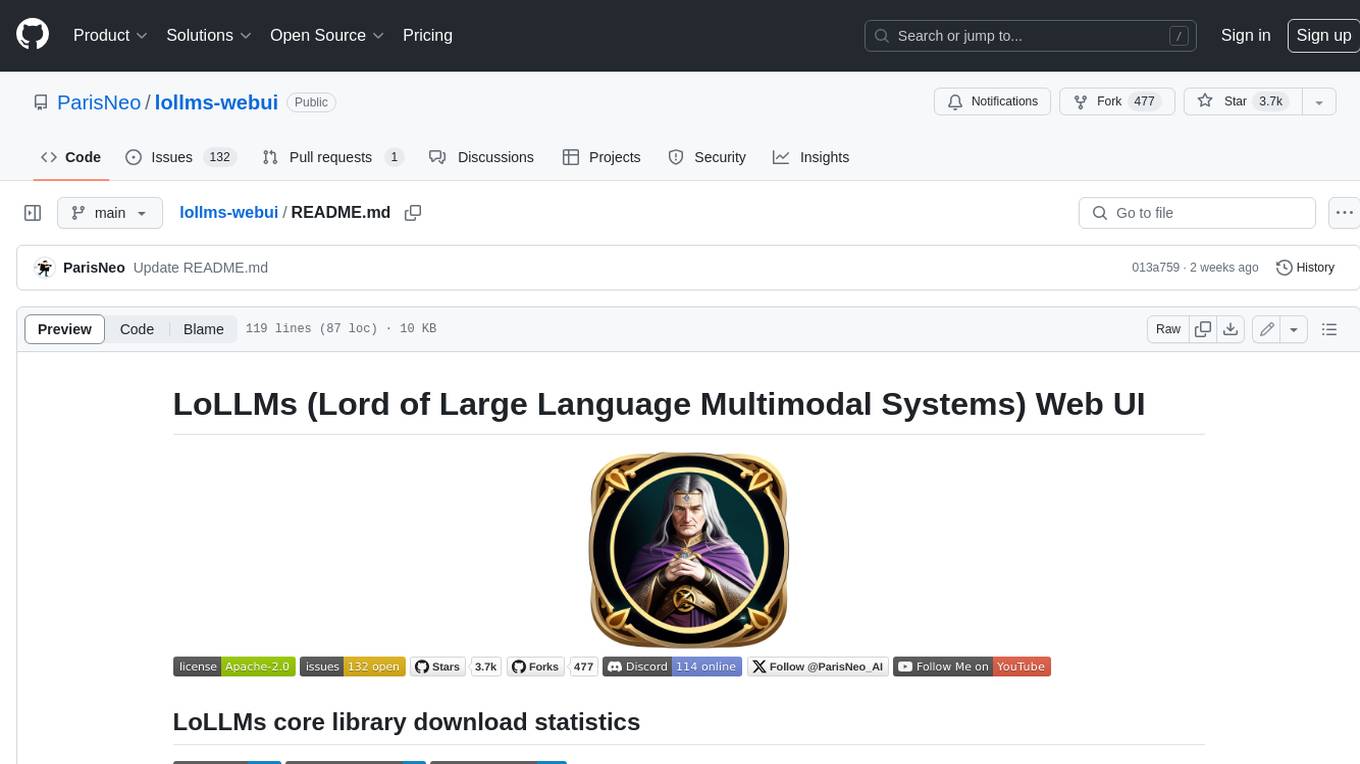
lollms-webui
LoLLMs WebUI (Lord of Large Language Multimodal Systems: One tool to rule them all) is a user-friendly interface to access and utilize various LLM (Large Language Models) and other AI models for a wide range of tasks. With over 500 AI expert conditionings across diverse domains and more than 2500 fine tuned models over multiple domains, LoLLMs WebUI provides an immediate resource for any problem, from car repair to coding assistance, legal matters, medical diagnosis, entertainment, and more. The easy-to-use UI with light and dark mode options, integration with GitHub repository, support for different personalities, and features like thumb up/down rating, copy, edit, and remove messages, local database storage, search, export, and delete multiple discussions, make LoLLMs WebUI a powerful and versatile tool.
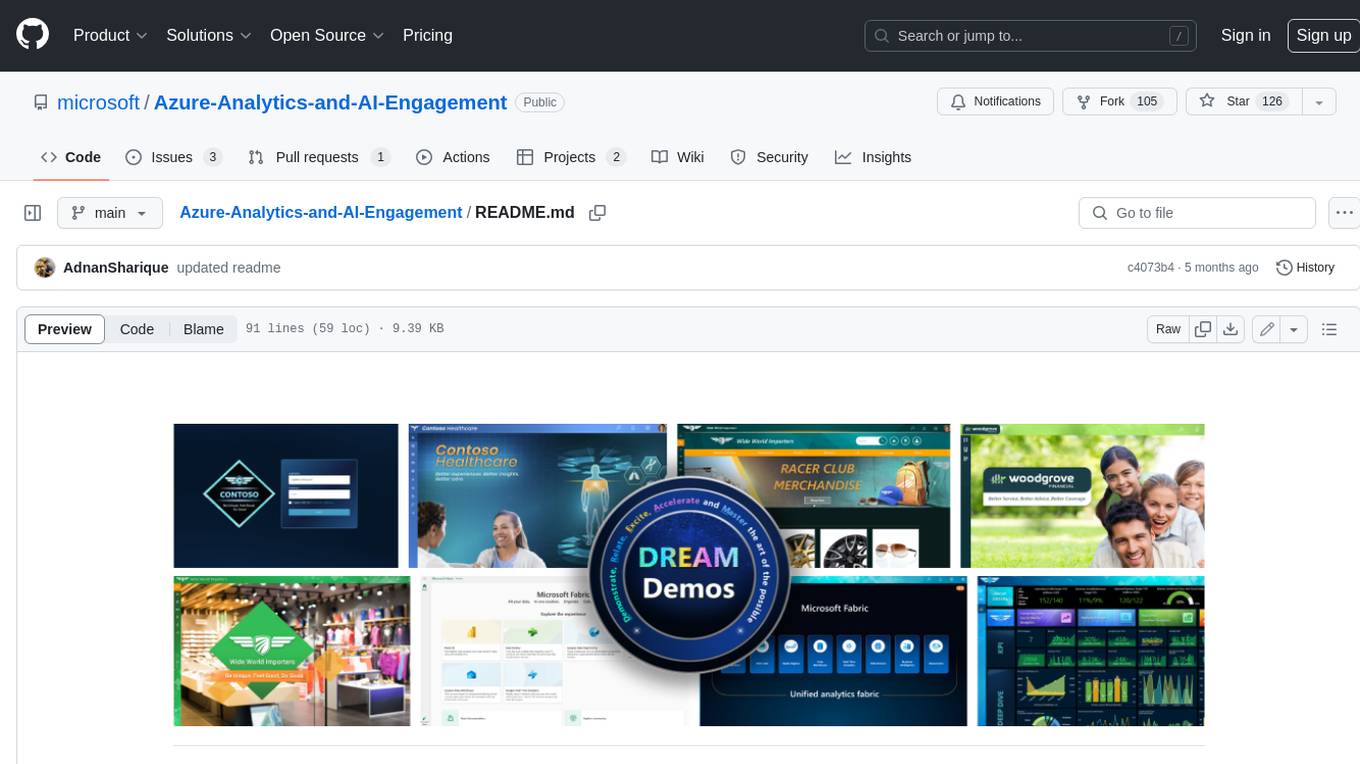
Azure-Analytics-and-AI-Engagement
The Azure-Analytics-and-AI-Engagement repository provides packaged Industry Scenario DREAM Demos with ARM templates (Containing a demo web application, Power BI reports, Synapse resources, AML Notebooks etc.) that can be deployed in a customer’s subscription using the CAPE tool within a matter of few hours. Partners can also deploy DREAM Demos in their own subscriptions using DPoC.
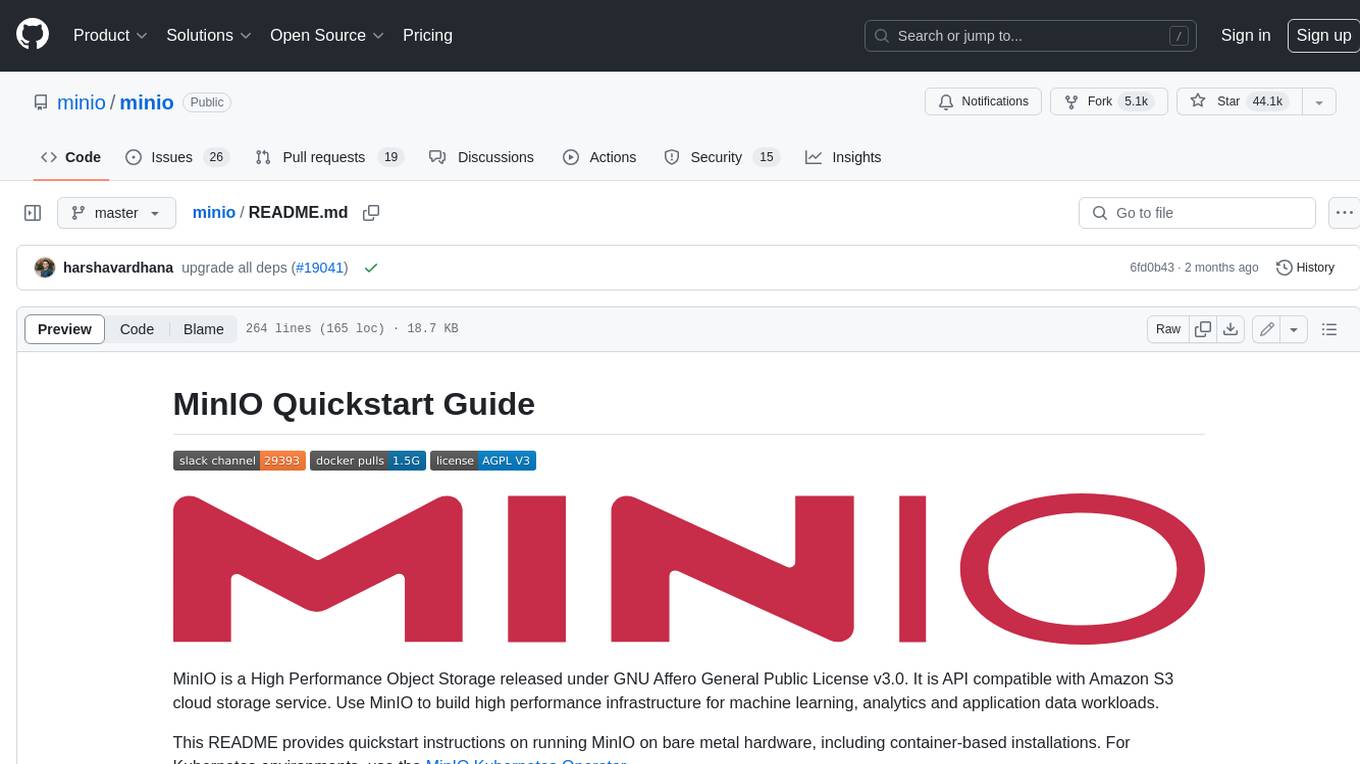
minio
MinIO is a High Performance Object Storage released under GNU Affero General Public License v3.0. It is API compatible with Amazon S3 cloud storage service. Use MinIO to build high performance infrastructure for machine learning, analytics and application data workloads.
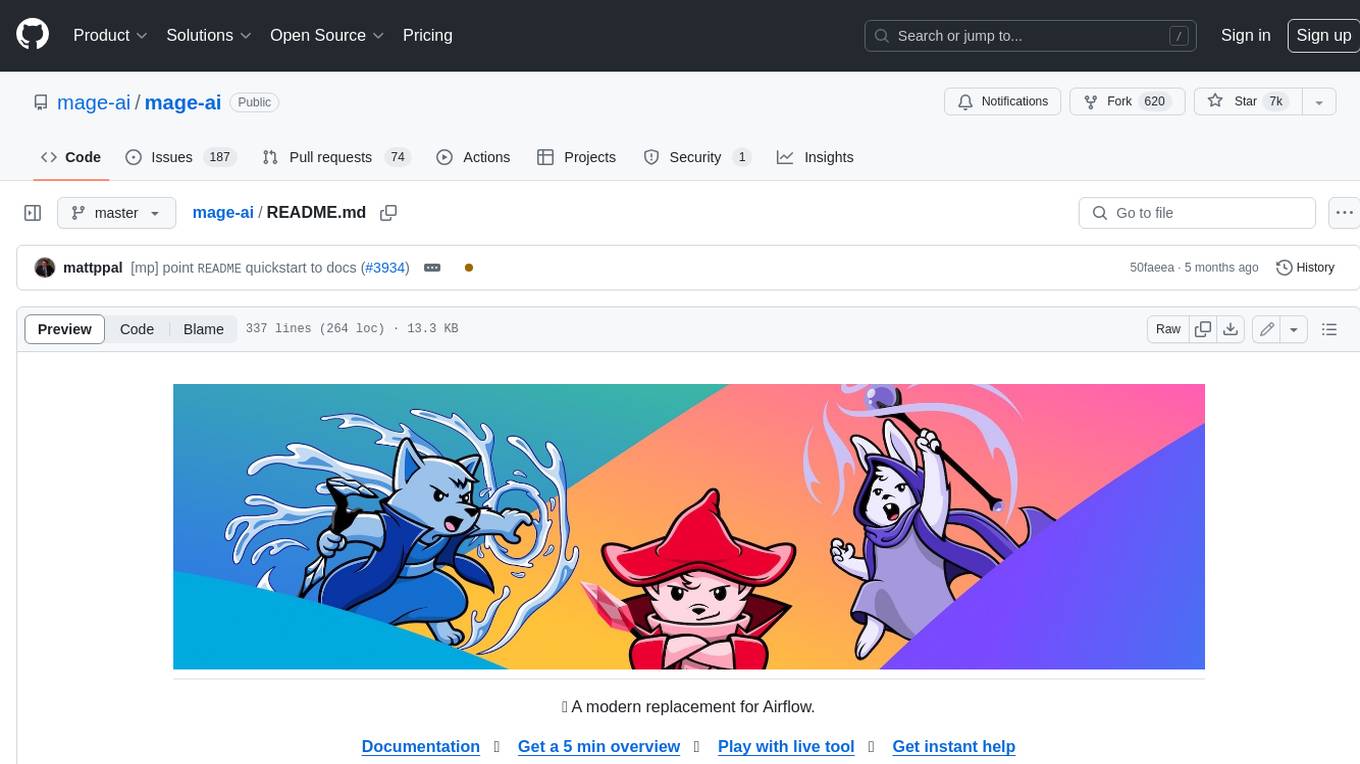
mage-ai
Mage is an open-source data pipeline tool for transforming and integrating data. It offers an easy developer experience, engineering best practices built-in, and data as a first-class citizen. Mage makes it easy to build, preview, and launch data pipelines, and provides observability and scaling capabilities. It supports data integrations, streaming pipelines, and dbt integration.
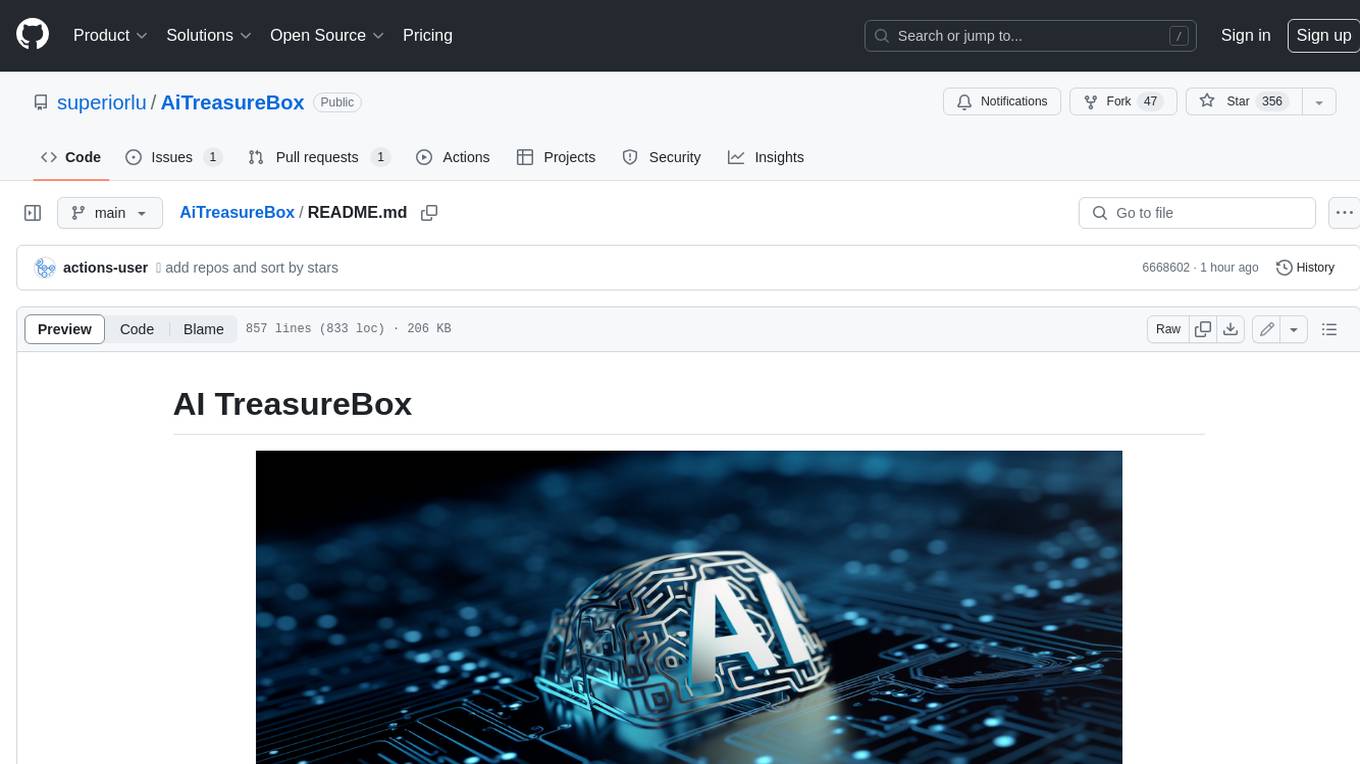
AiTreasureBox
AiTreasureBox is a versatile AI tool that provides a collection of pre-trained models and algorithms for various machine learning tasks. It simplifies the process of implementing AI solutions by offering ready-to-use components that can be easily integrated into projects. With AiTreasureBox, users can quickly prototype and deploy AI applications without the need for extensive knowledge in machine learning or deep learning. The tool covers a wide range of tasks such as image classification, text generation, sentiment analysis, object detection, and more. It is designed to be user-friendly and accessible to both beginners and experienced developers, making AI development more efficient and accessible to a wider audience.
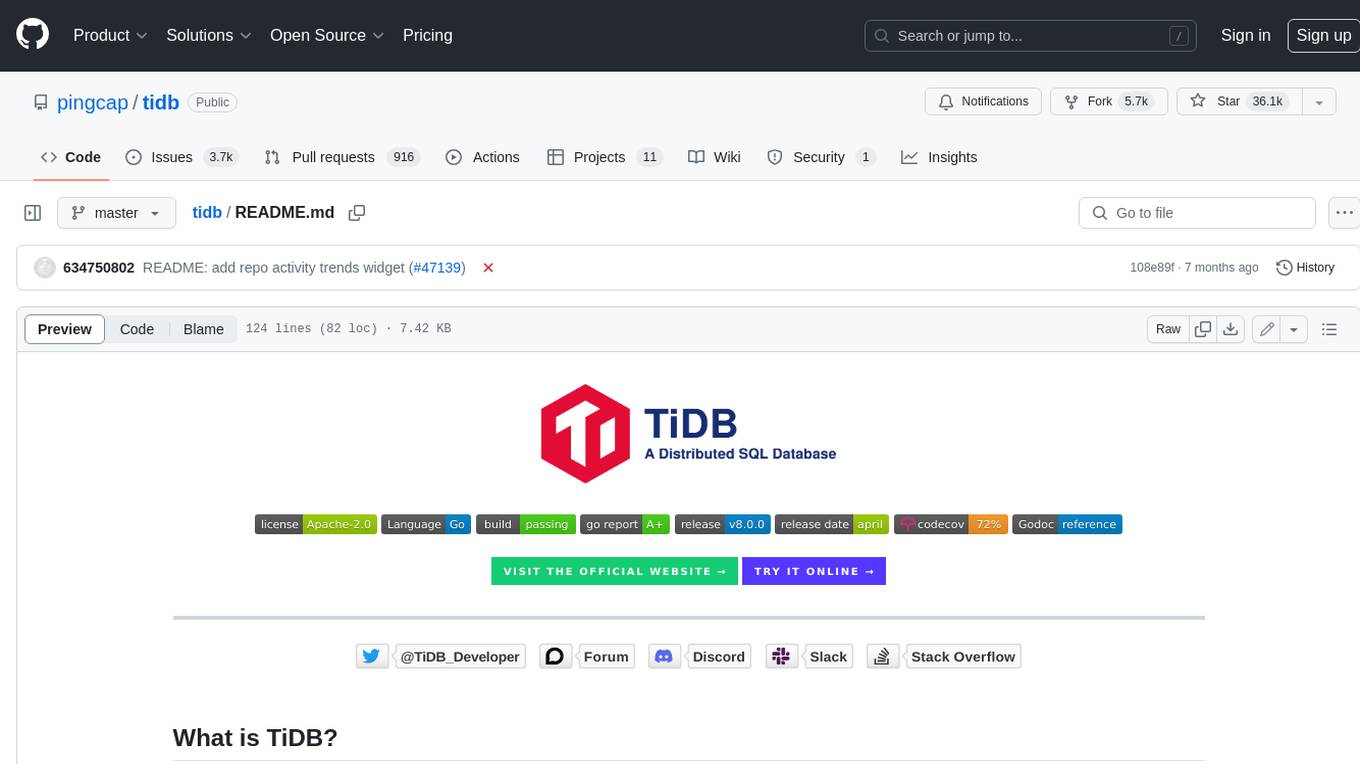
tidb
TiDB is an open-source distributed SQL database that supports Hybrid Transactional and Analytical Processing (HTAP) workloads. It is MySQL compatible and features horizontal scalability, strong consistency, and high availability.
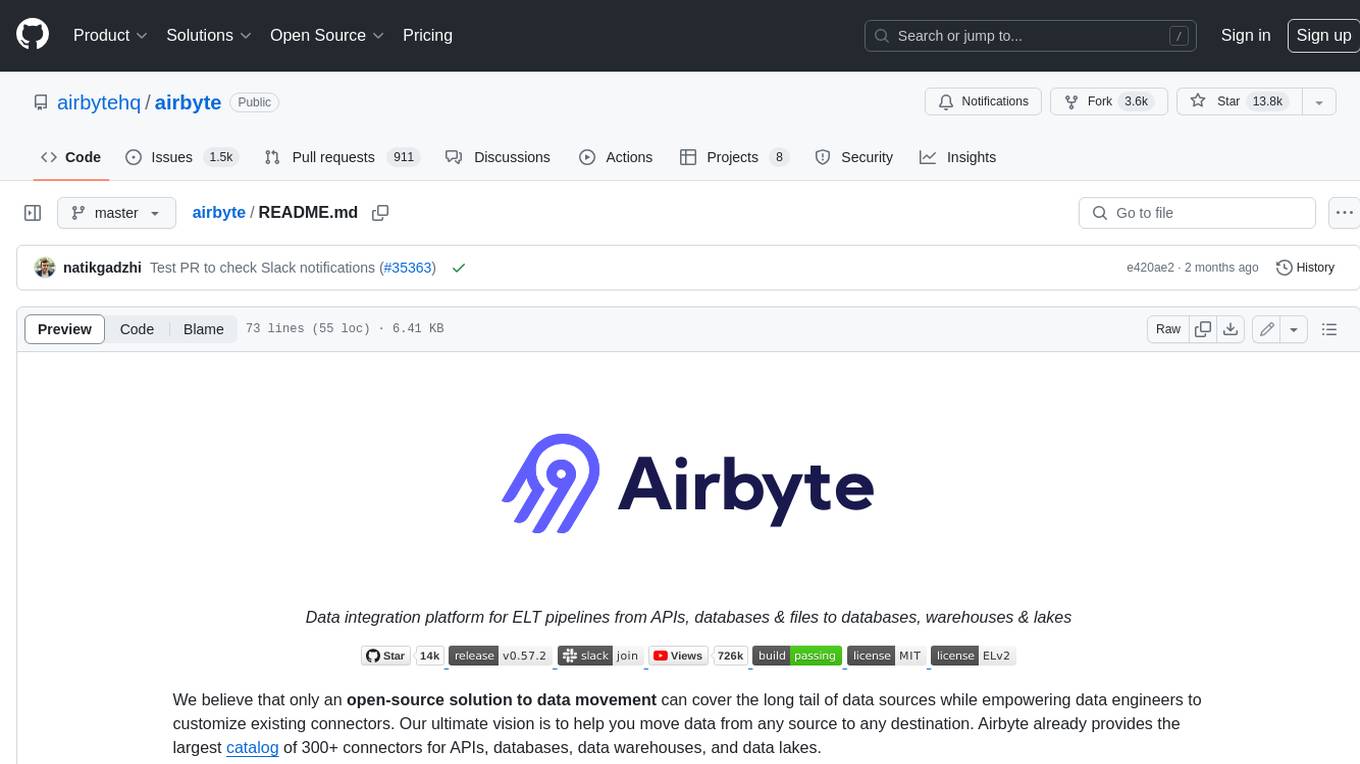
airbyte
Airbyte is an open-source data integration platform that makes it easy to move data from any source to any destination. With Airbyte, you can build and manage data pipelines without writing any code. Airbyte provides a library of pre-built connectors that make it easy to connect to popular data sources and destinations. You can also create your own connectors using Airbyte's no-code Connector Builder or low-code CDK. Airbyte is used by data engineers and analysts at companies of all sizes to build and manage their data pipelines.
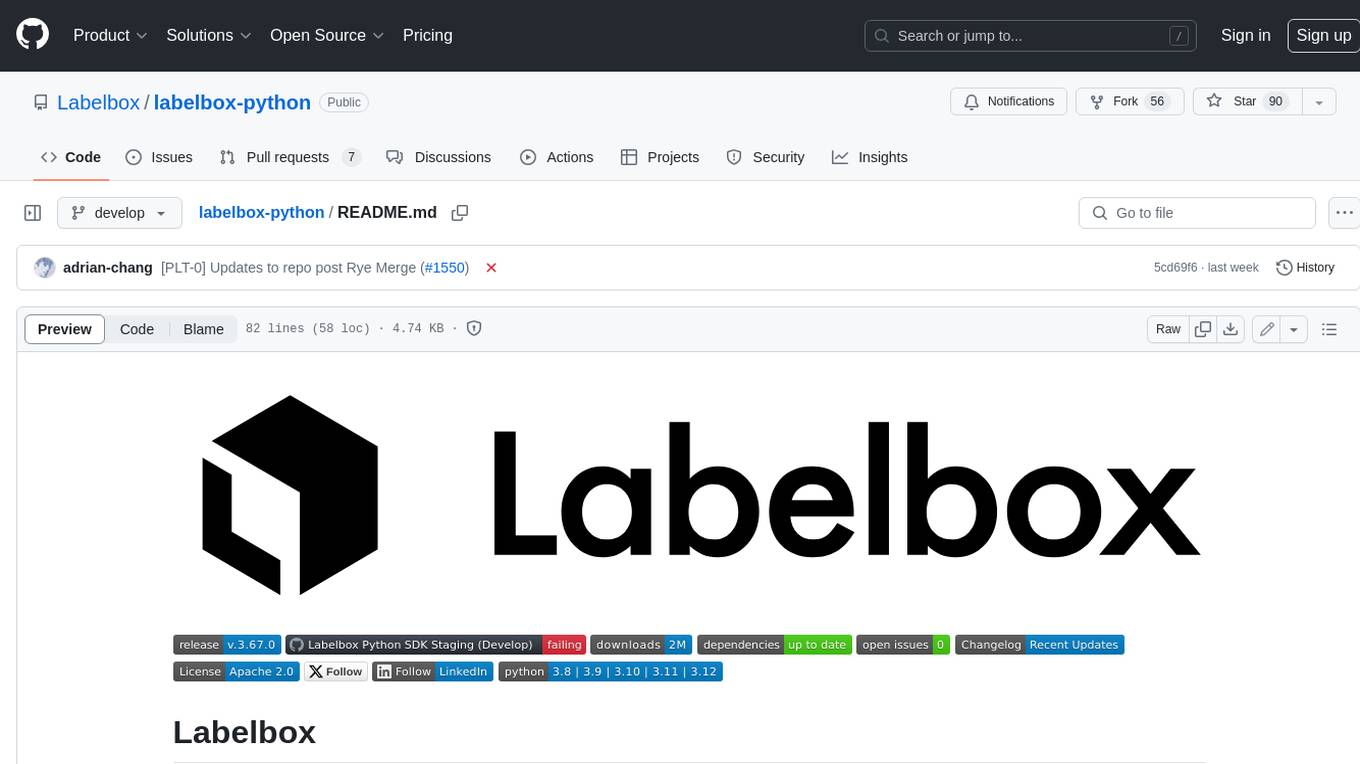
labelbox-python
Labelbox is a data-centric AI platform for enterprises to develop, optimize, and use AI to solve problems and power new products and services. Enterprises use Labelbox to curate data, generate high-quality human feedback data for computer vision and LLMs, evaluate model performance, and automate tasks by combining AI and human-centric workflows. The academic & research community uses Labelbox for cutting-edge AI research.