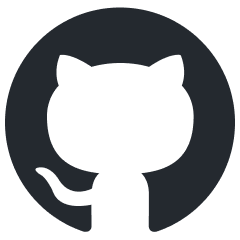
aiobotocore
asyncio support for botocore library using aiohttp
Stars: 1231
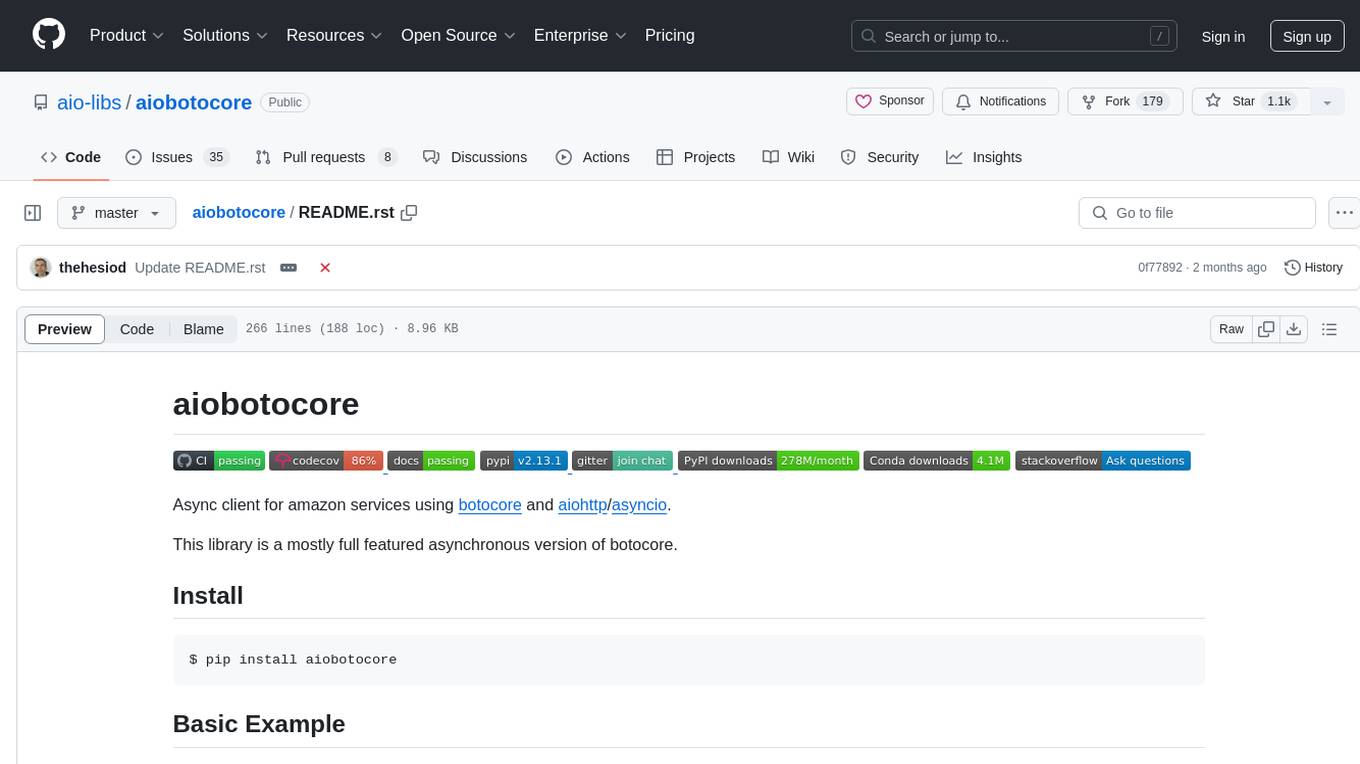
aiobotocore is an async client for Amazon services using botocore and aiohttp/asyncio. It provides a mostly full-featured asynchronous version of botocore, allowing users to interact with various AWS services asynchronously. The library supports operations such as uploading objects to S3, getting object properties, listing objects, and deleting objects. It also offers context manager examples for managing resources efficiently. aiobotocore supports multiple AWS services like S3, DynamoDB, SNS, SQS, CloudFormation, and Kinesis, with basic methods tested for each service. Users can run tests using moto for mocked tests or against personal Amazon keys. Additionally, the tool enables type checking and code completion for better development experience.
README:
.. |ci badge| image:: https://github.com/aio-libs/aiobotocore/actions/workflows/ci-cd.yml/badge.svg?branch=master :target: https://github.com/aio-libs/aiobotocore/actions/workflows/ci-cd.yml :alt: CI status of master branch .. |pre-commit badge| image:: https://results.pre-commit.ci/badge/github/aio-libs/aiobotocore/master.svg :target: https://results.pre-commit.ci/latest/github/aio-libs/aiobotocore/master :alt: pre-commit.ci status .. |coverage badge| image:: https://codecov.io/gh/aio-libs/aiobotocore/branch/master/graph/badge.svg :target: https://codecov.io/gh/aio-libs/aiobotocore :alt: Coverage status on master branch .. |docs badge| image:: https://readthedocs.org/projects/aiobotocore/badge/?version=latest :target: https://aiobotocore.readthedocs.io/en/latest/?badge=latest :alt: Documentation Status .. |pypi badge| image:: https://img.shields.io/pypi/v/aiobotocore.svg :target: https://pypi.python.org/pypi/aiobotocore :alt: Latest version on pypi .. |gitter badge| image:: https://badges.gitter.im/Join%20Chat.svg :target: https://gitter.im/aio-libs/aiobotocore :alt: Chat on Gitter .. |pypi downloads badge| image:: https://img.shields.io/pypi/dm/aiobotocore.svg?label=PyPI%20downloads :target: https://pypi.org/project/aiobotocore/ :alt: Downloads Last Month .. |conda badge| image:: https://img.shields.io/conda/dn/conda-forge/aiobotocore.svg?label=Conda%20downloads :target: https://anaconda.org/conda-forge/aiobotocore :alt: Conda downloads .. |stackoverflow badge| image:: https://img.shields.io/badge/stackoverflow-Ask%20questions-blue.svg :target: https://stackoverflow.com/questions/tagged/aiobotocore :alt: Stack Overflow
|ci badge| |pre-commit badge| |coverage badge| |docs badge| |pypi badge| |gitter badge| |pypi downloads badge| |conda badge| |stackoverflow badge|
Async client for amazon services using botocore_ and aiohttp_/asyncio_.
This library is a mostly full featured asynchronous version of botocore.
::
$ pip install aiobotocore
.. code:: python
import asyncio
from aiobotocore.session import get_session
AWS_ACCESS_KEY_ID = "xxx"
AWS_SECRET_ACCESS_KEY = "xxx"
async def go():
bucket = 'dataintake'
filename = 'dummy.bin'
folder = 'aiobotocore'
key = '{}/{}'.format(folder, filename)
session = get_session()
async with session.create_client('s3', region_name='us-west-2',
aws_secret_access_key=AWS_SECRET_ACCESS_KEY,
aws_access_key_id=AWS_ACCESS_KEY_ID) as client:
# upload object to amazon s3
data = b'\x01'*1024
resp = await client.put_object(Bucket=bucket,
Key=key,
Body=data)
print(resp)
# getting s3 object properties of file we just uploaded
resp = await client.get_object_acl(Bucket=bucket, Key=key)
print(resp)
# get object from s3
response = await client.get_object(Bucket=bucket, Key=key)
# this will ensure the connection is correctly re-used/closed
async with response['Body'] as stream:
assert await stream.read() == data
# list s3 objects using paginator
paginator = client.get_paginator('list_objects')
async for result in paginator.paginate(Bucket=bucket, Prefix=folder):
for c in result.get('Contents', []):
print(c)
# delete object from s3
resp = await client.delete_object(Bucket=bucket, Key=key)
print(resp)
loop = asyncio.get_event_loop()
loop.run_until_complete(go())
.. code:: python
from contextlib import AsyncExitStack
from aiobotocore.session import AioSession
# How to use in existing context manager
class Manager:
def __init__(self):
self._exit_stack = AsyncExitStack()
self._s3_client = None
async def __aenter__(self):
session = AioSession()
self._s3_client = await self._exit_stack.enter_async_context(session.create_client('s3'))
async def __aexit__(self, exc_type, exc_val, exc_tb):
await self._exit_stack.__aexit__(exc_type, exc_val, exc_tb)
# How to use with an external exit_stack
async def create_s3_client(session: AioSession, exit_stack: AsyncExitStack):
# Create client and add cleanup
client = await exit_stack.enter_async_context(session.create_client('s3'))
return client
async def non_manager_example():
session = AioSession()
async with AsyncExitStack() as exit_stack:
s3_client = await create_s3_client(session, exit_stack)
# do work with s3_client
This is a non-exuastive list of what tests aiobotocore runs against AWS services. Not all methods are tested but we aim to test the majority of commonly used methods.
+----------------+-----------------------+ | Service | Status | +================+=======================+ | S3 | Working | +----------------+-----------------------+ | DynamoDB | Basic methods tested | +----------------+-----------------------+ | SNS | Basic methods tested | +----------------+-----------------------+ | SQS | Basic methods tested | +----------------+-----------------------+ | CloudFormation | Stack creation tested | +----------------+-----------------------+ | Kinesis | Basic methods tested | +----------------+-----------------------+
Due to the way boto3 is implemented, its highly likely that even if services are not listed above that you can take any boto3.client('service')
and
stick await
in front of methods to make them async, e.g. await client.list_named_queries()
would asynchronous list all of the named Athena queries.
If a service is not listed here and you could do with some tests or examples feel free to raise an issue.
Install types-aiobotocore_ that contains type annotations for aiobotocore
and all supported botocore_ services.
.. code:: bash
# install aiobotocore type annotations
# for ec2, s3, rds, lambda, sqs, dynamo and cloudformation
python -m pip install 'types-aiobotocore[essential]'
# or install annotations for services you use
python -m pip install 'types-aiobotocore[acm,apigateway]'
# Lite version does not provide session.create_client overloads
# it is more RAM-friendly, but requires explicit type annotations
python -m pip install 'types-aiobotocore-lite[essential]'
Now you should be able to run Pylance_, pyright_, or mypy_ for type checking as well as code completion in your IDE.
For types-aiobotocore-lite
package use explicit type annotations:
.. code:: python
from aiobotocore.session import get_session
from types_aiobotocore_s3.client import S3Client
session = get_session()
async with session.create_client("s3") as client:
client: S3Client
# type checking and code completion is now enabled for client
Full documentation for types-aiobotocore
can be found here: https://youtype.github.io/types_aiobotocore_docs/
- Python_ 3.8+
- aiohttp_
- botocore_
.. _Python: https://www.python.org .. _asyncio: https://docs.python.org/3/library/asyncio.html .. _botocore: https://github.com/boto/botocore .. _aiohttp: https://github.com/aio-libs/aiohttp .. _types-aiobotocore: https://youtype.github.io/types_aiobotocore_docs/ .. _Pylance: https://marketplace.visualstudio.com/items?itemName=ms-python.vscode-pylance .. _pyright: https://github.com/microsoft/pyright .. _mypy: http://mypy-lang.org/
awscli and boto3 depend on a single version, or a narrow range of versions, of botocore. However, aiobotocore only supports a specific range of botocore versions. To ensure you install the latest version of awscli and boto3 that your specific combination or aiobotocore and botocore can support use::
pip install -U 'aiobotocore[awscli,boto3]'
If you only need awscli and not boto3 (or vice versa) you can just install one extra or the other.
For Tasks:
Click tags to check more tools for each tasksFor Jobs:
Alternative AI tools for aiobotocore
Similar Open Source Tools
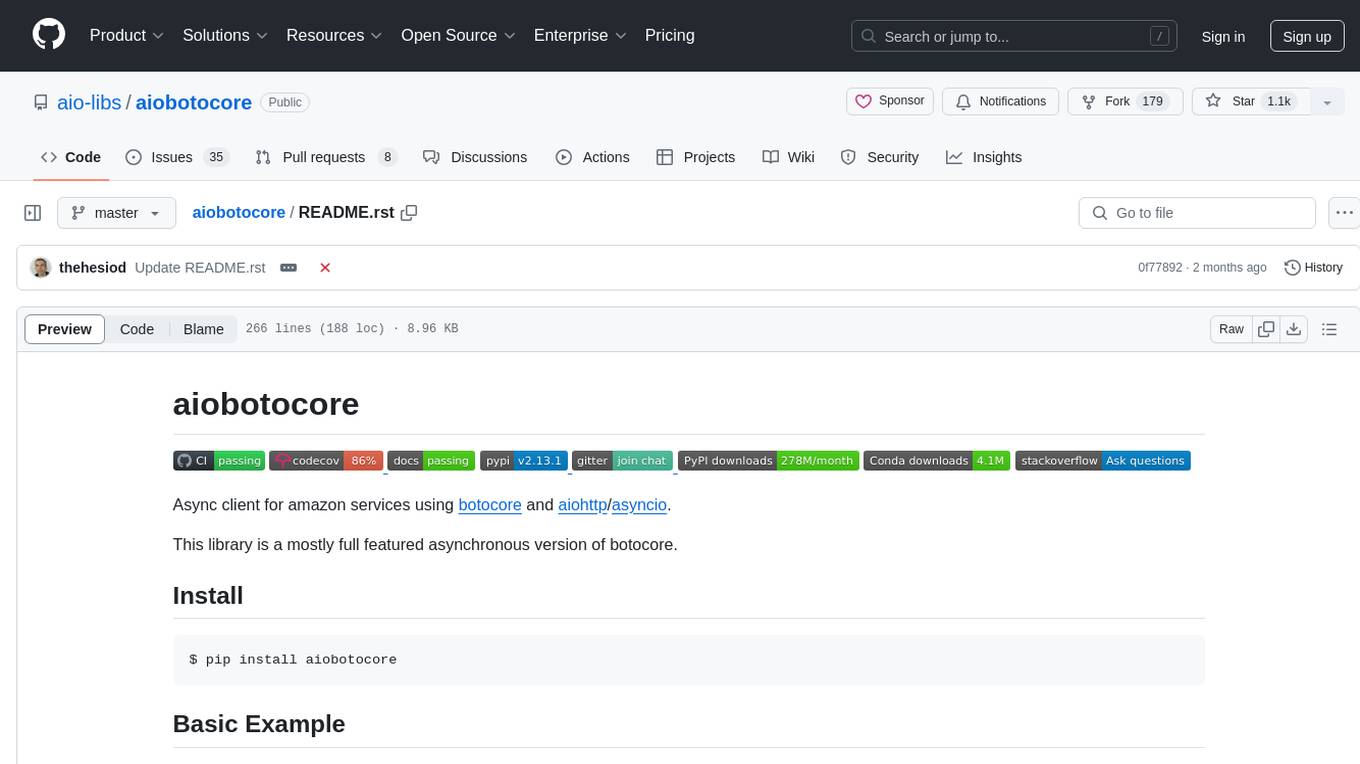
aiobotocore
aiobotocore is an async client for Amazon services using botocore and aiohttp/asyncio. It provides a mostly full-featured asynchronous version of botocore, allowing users to interact with various AWS services asynchronously. The library supports operations such as uploading objects to S3, getting object properties, listing objects, and deleting objects. It also offers context manager examples for managing resources efficiently. aiobotocore supports multiple AWS services like S3, DynamoDB, SNS, SQS, CloudFormation, and Kinesis, with basic methods tested for each service. Users can run tests using moto for mocked tests or against personal Amazon keys. Additionally, the tool enables type checking and code completion for better development experience.
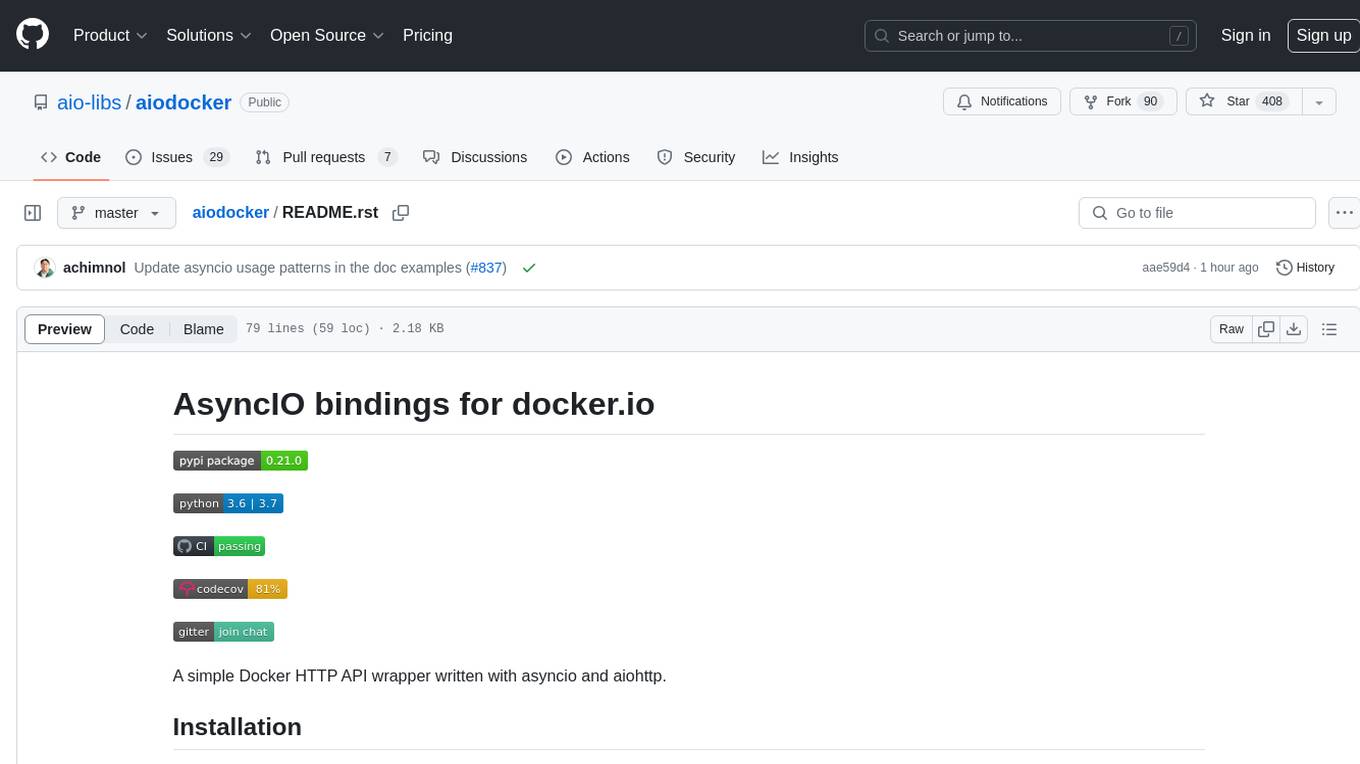
aiodocker
Aiodocker is a simple Docker HTTP API wrapper written with asyncio and aiohttp. It provides asynchronous bindings for interacting with Docker containers and images. Users can easily manage Docker resources using async functions and methods. The library offers features such as listing images and containers, creating and running containers, and accessing container logs. Aiodocker is designed to work seamlessly with Python's asyncio framework, making it suitable for building asynchronous Docker management applications.
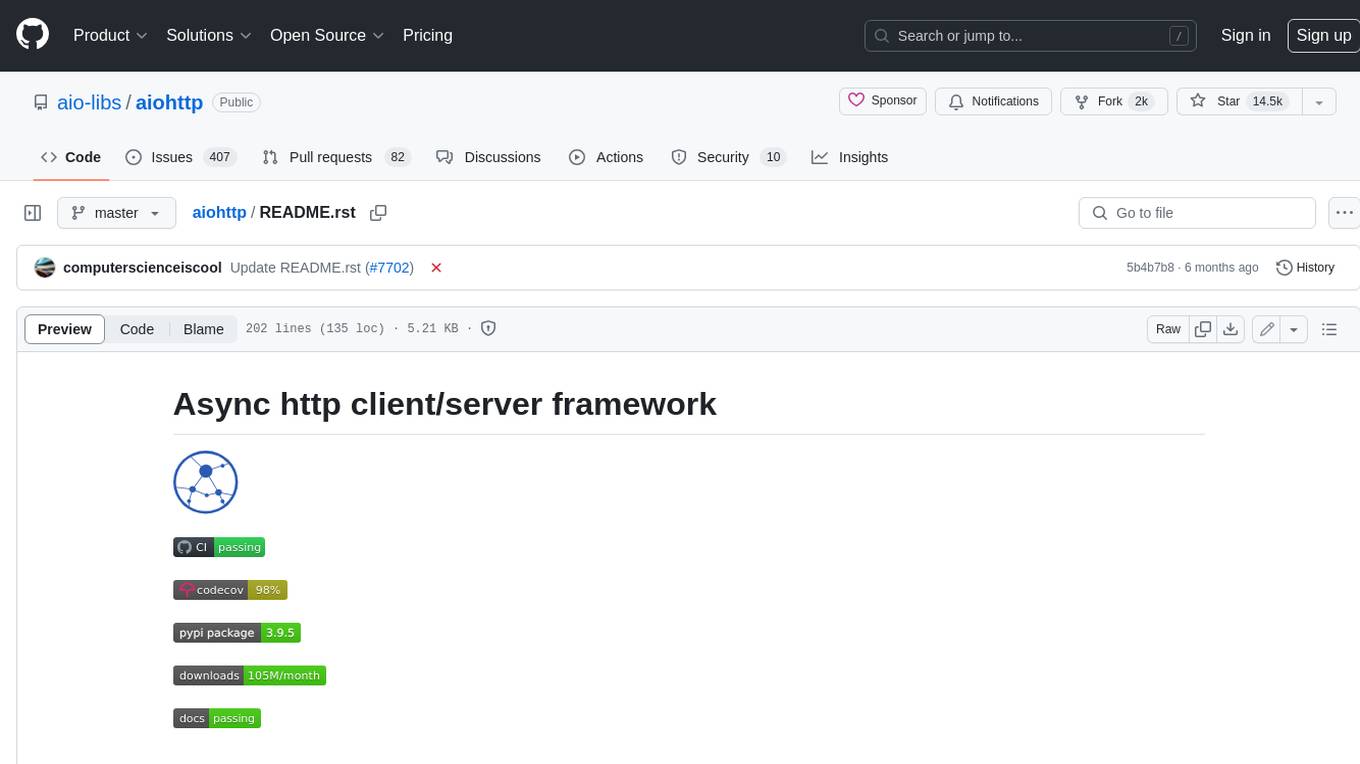
aiohttp
aiohttp is an async http client/server framework that supports both client and server side of HTTP protocol. It also supports both client and server Web-Sockets out-of-the-box and avoids Callback Hell. aiohttp provides a Web-server with middleware and pluggable routing.
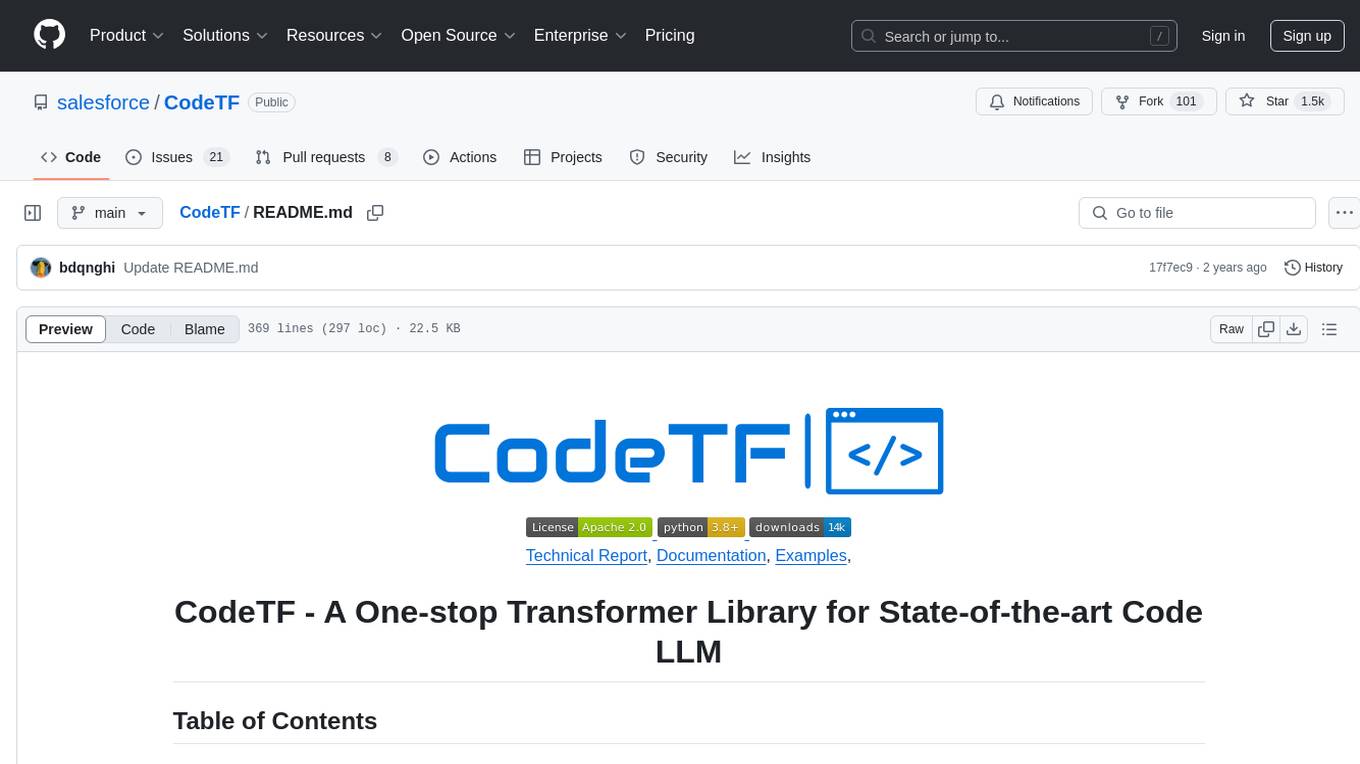
CodeTF
CodeTF is a Python transformer-based library for code large language models (Code LLMs) and code intelligence. It provides an interface for training and inferencing on tasks like code summarization, translation, and generation. The library offers utilities for code manipulation across various languages, including easy extraction of code attributes. Using tree-sitter as its core AST parser, CodeTF enables parsing of function names, comments, and variable names. It supports fast model serving, fine-tuning of LLMs, various code intelligence tasks, preprocessed datasets, model evaluation, pretrained and fine-tuned models, and utilities to manipulate source code. CodeTF aims to facilitate the integration of state-of-the-art Code LLMs into real-world applications, ensuring a user-friendly environment for code intelligence tasks.
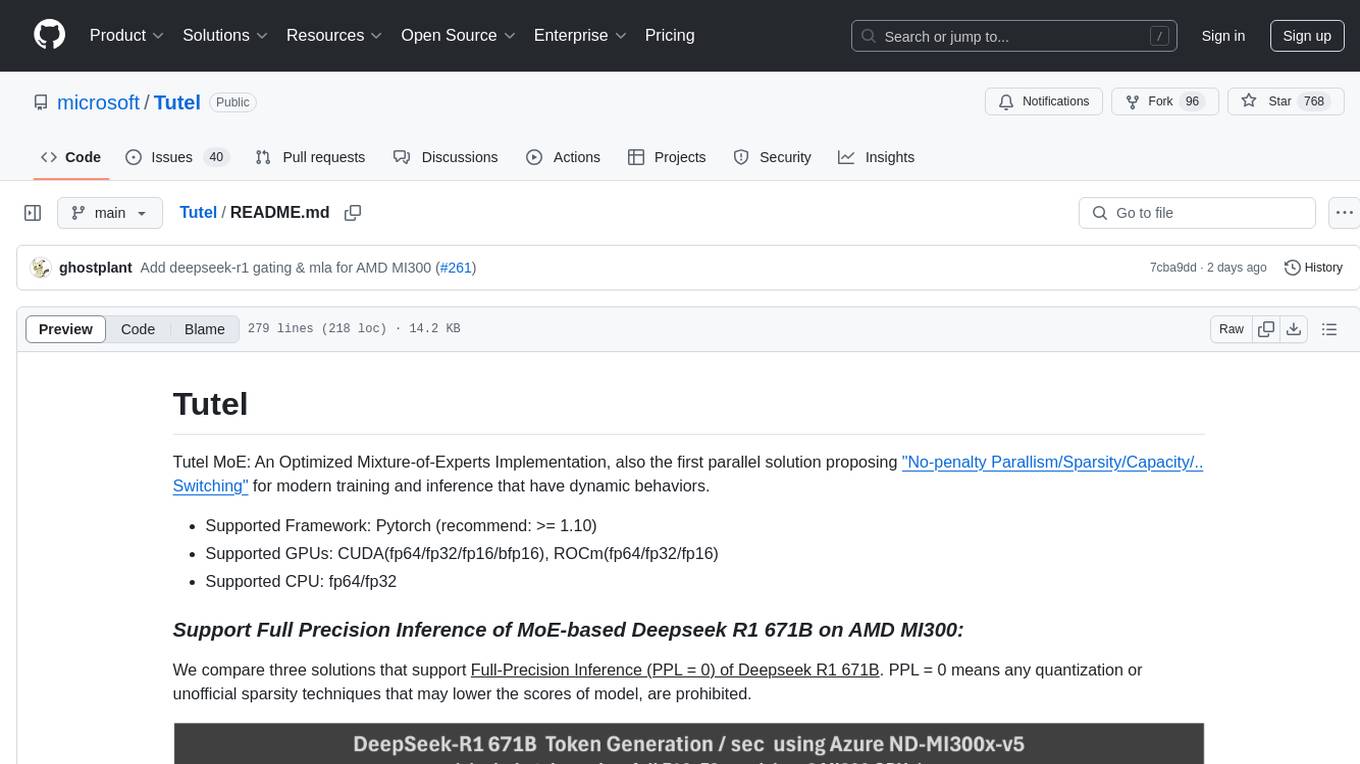
Tutel
Tutel MoE is an optimized Mixture-of-Experts implementation that offers a parallel solution with 'No-penalty Parallism/Sparsity/Capacity/Switching' for modern training and inference. It supports Pytorch framework (version >= 1.10) and various GPUs including CUDA and ROCm. The tool enables Full Precision Inference of MoE-based Deepseek R1 671B on AMD MI300. Tutel provides features like all-to-all benchmarking, tensorcore option, NCCL timeout settings, Megablocks solution, and dynamic switchable configurations. Users can run Tutel in distributed mode across multiple GPUs and machines. The tool allows for custom MoE implementations and offers detailed usage examples and reference documentation.
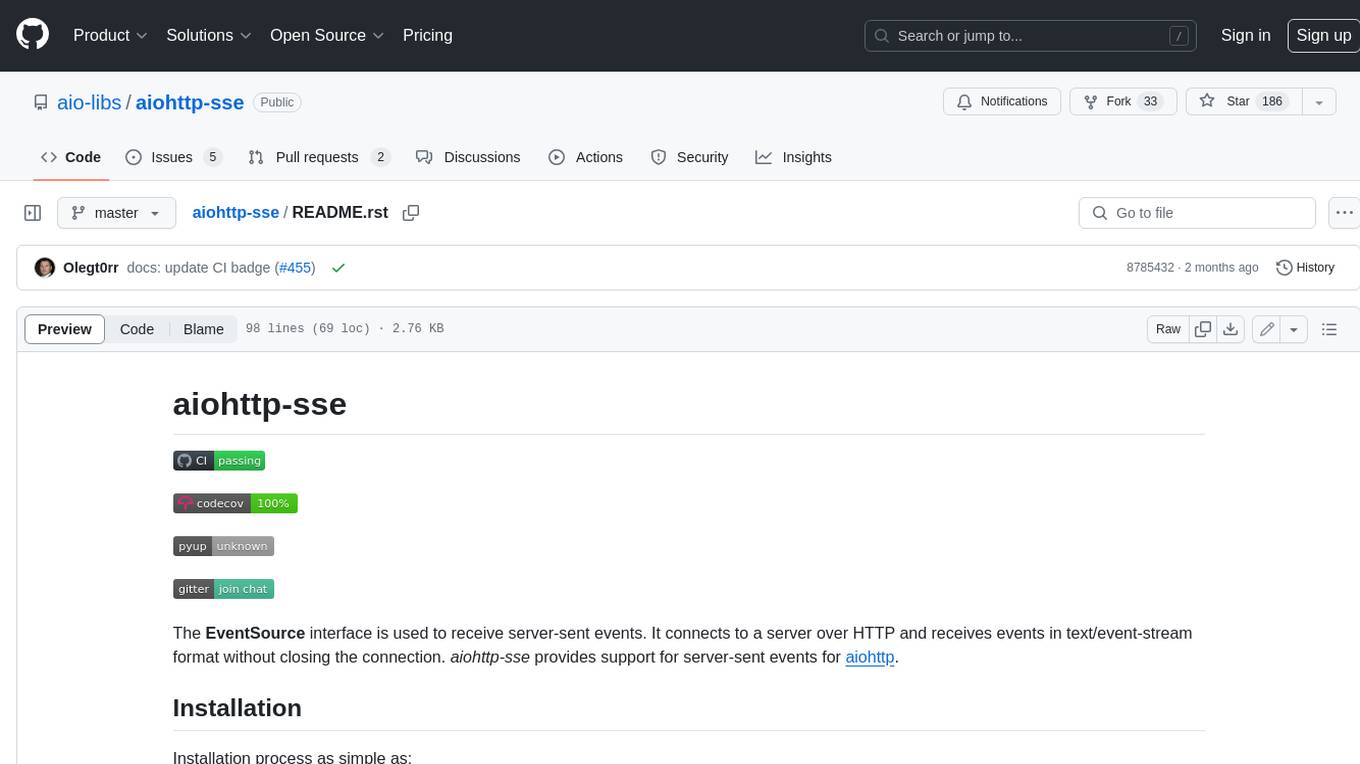
aiohttp-sse
aiohttp-sse is a library that provides support for server-sent events for aiohttp. Server-sent events are a way to send real-time updates from a server to a client. This can be useful for things like live chat, stock tickers, or any other application where you need to send updates to a client without having to wait for the client to request them.
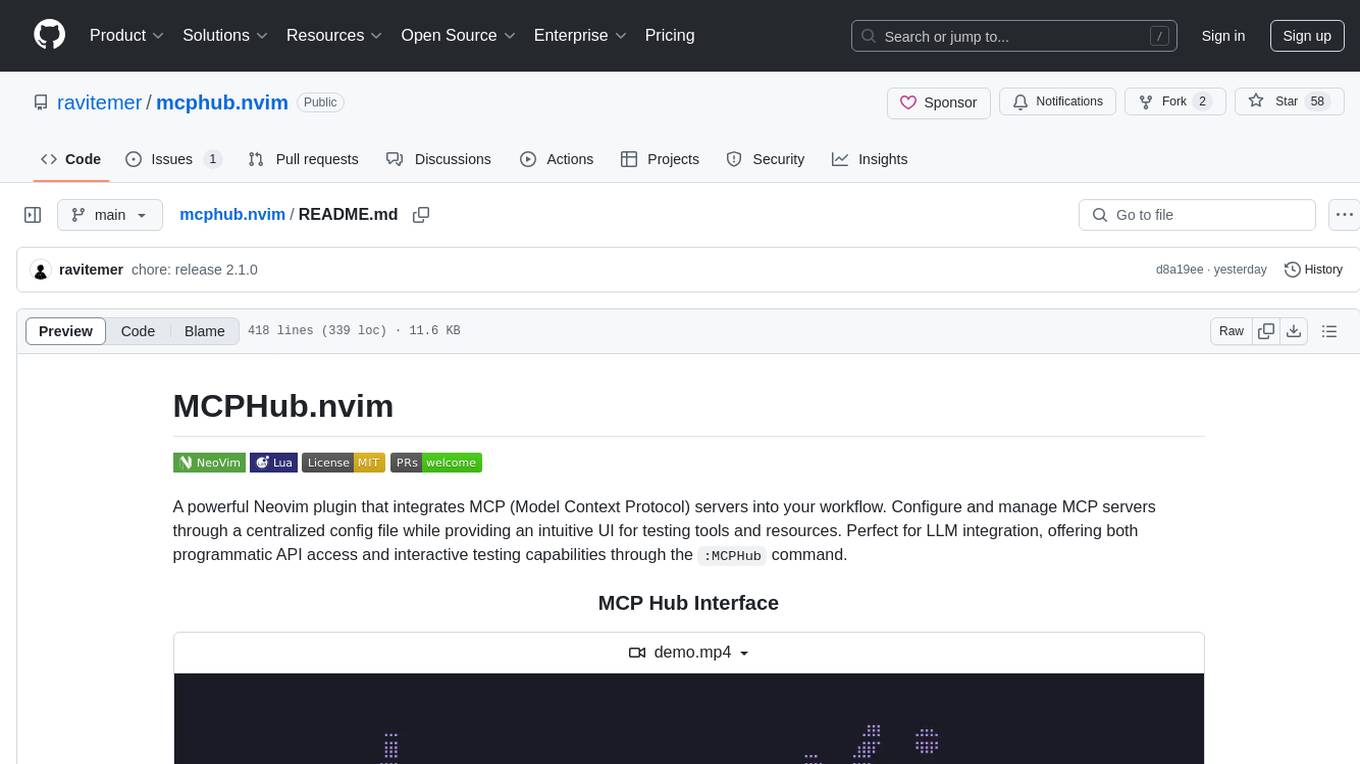
mcphub.nvim
MCPHub.nvim is a powerful Neovim plugin that integrates MCP (Model Context Protocol) servers into your workflow. It offers a centralized config file for managing servers and tools, with an intuitive UI for testing resources. Ideal for LLM integration, it provides programmatic API access and interactive testing through the `:MCPHub` command.
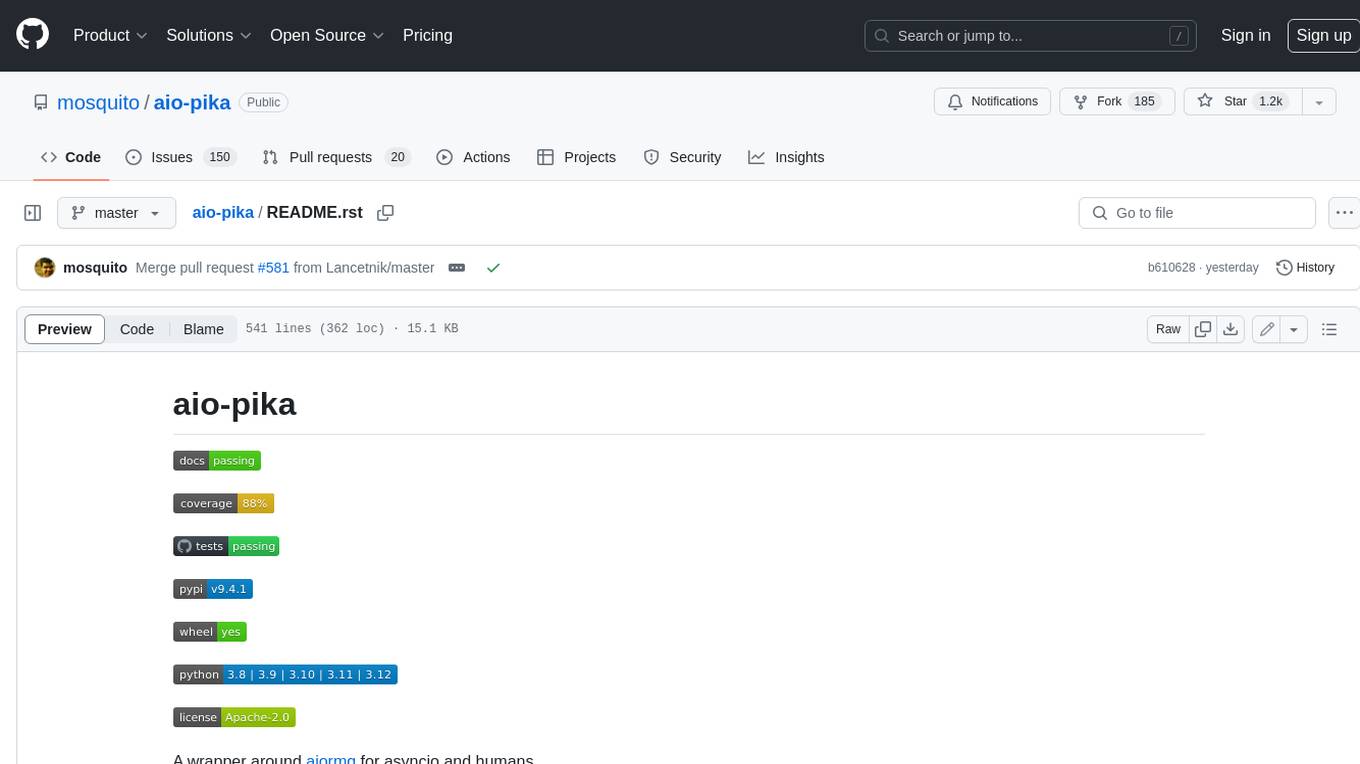
aio-pika
Aio-pika is a wrapper around aiormq for asyncio and humans. It provides a completely asynchronous API, object-oriented API, transparent auto-reconnects with complete state recovery, Python 3.7+ compatibility, transparent publisher confirms support, transactions support, and complete type-hints coverage.
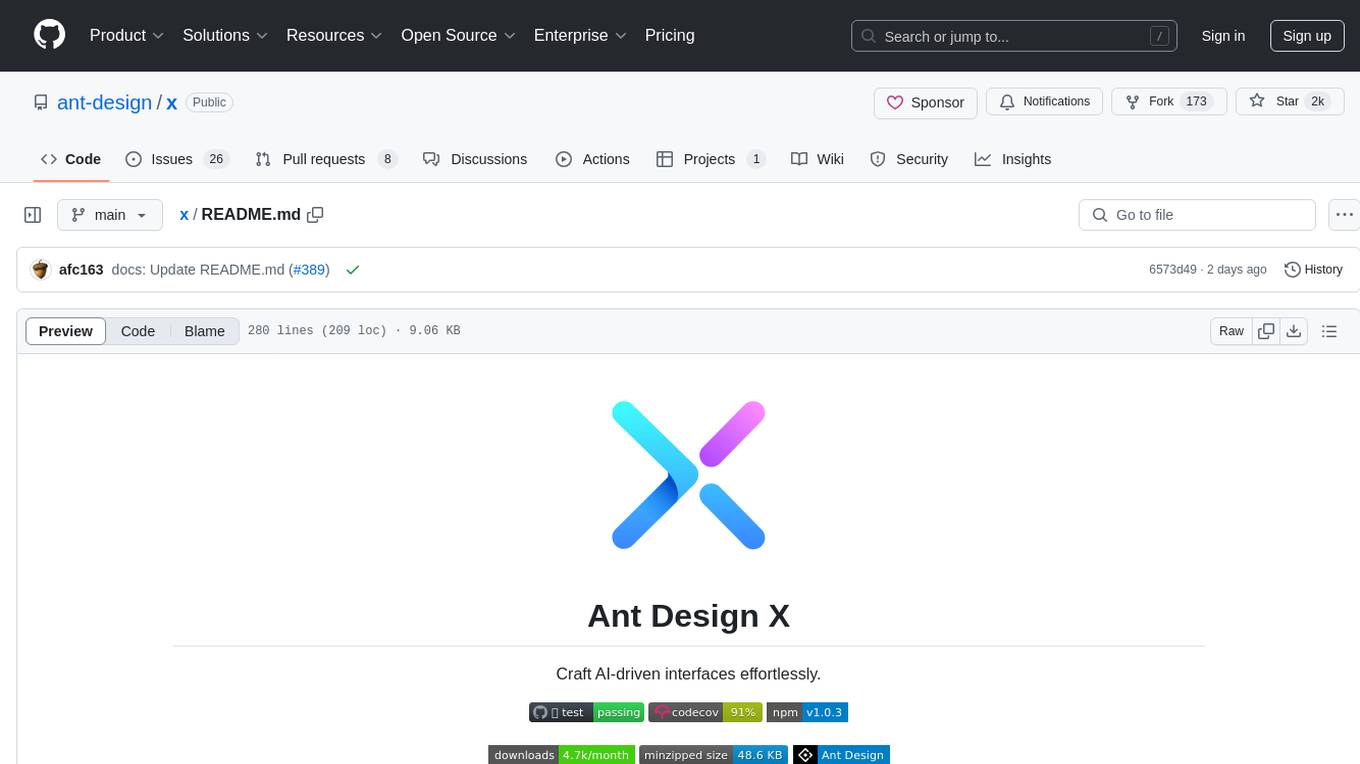
x
Ant Design X is a tool for crafting AI-driven interfaces effortlessly. It is built on the best practices of enterprise-level AI products, offering flexible and diverse atomic components for various AI dialogue scenarios. The tool provides out-of-the-box model integration with inference services compatible with OpenAI standards. It also enables efficient management of conversation data flows, supports rich template options, complete TypeScript support, and advanced theme customization. Ant Design X is designed to enhance development efficiency and deliver exceptional AI interaction experiences.
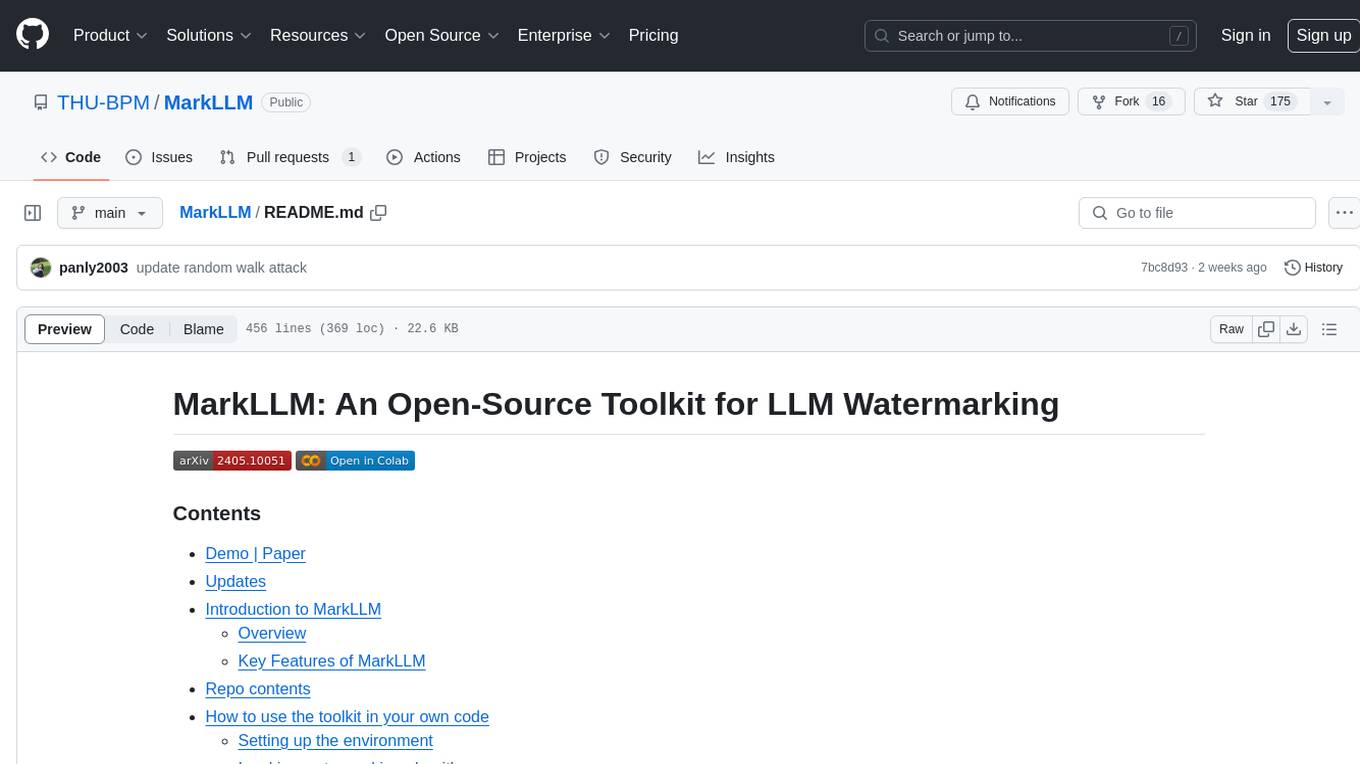
MarkLLM
MarkLLM is an open-source toolkit designed for watermarking technologies within large language models (LLMs). It simplifies access, understanding, and assessment of watermarking technologies, supporting various algorithms, visualization tools, and evaluation modules. The toolkit aids researchers and the community in ensuring the authenticity and origin of machine-generated text.
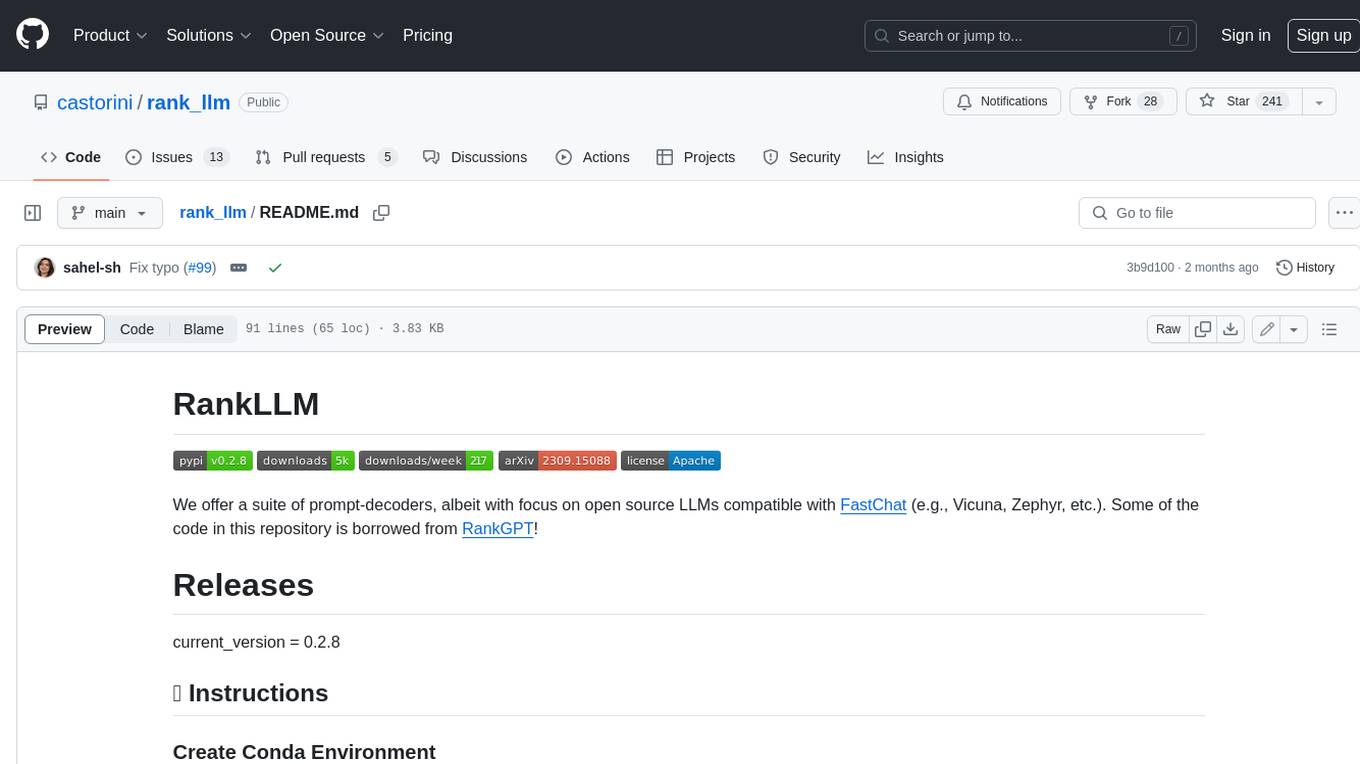
rank_llm
RankLLM is a suite of prompt-decoders compatible with open source LLMs like Vicuna and Zephyr. It allows users to create custom ranking models for various NLP tasks, such as document reranking, question answering, and summarization. The tool offers a variety of features, including the ability to fine-tune models on custom datasets, use different retrieval methods, and control the context size and variable passages. RankLLM is easy to use and can be integrated into existing NLP pipelines.
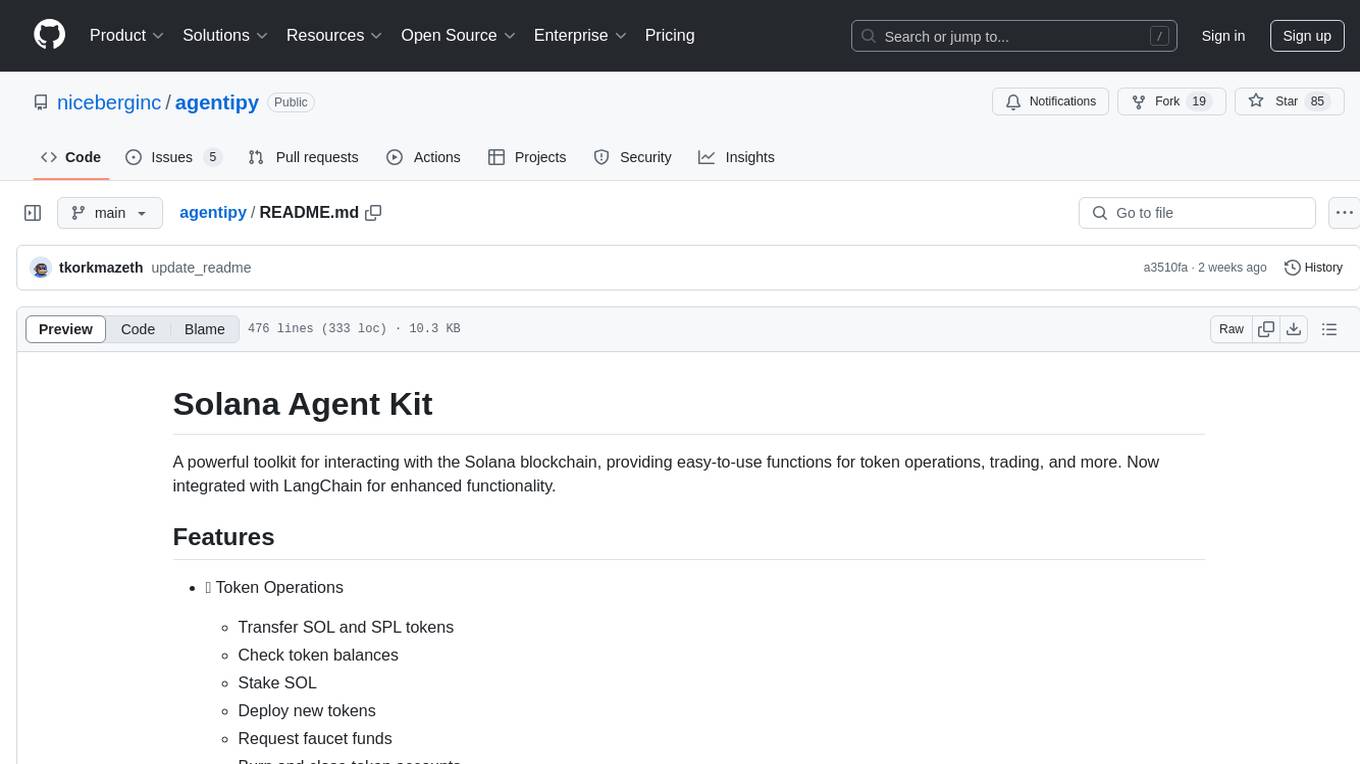
agentipy
Agentipy is a powerful toolkit for interacting with the Solana blockchain, providing easy-to-use functions for token operations, trading, yield farming, LangChain integration, performance tracking, token data retrieval, pump & fun token launching, Meteora DLMM pool creation, and more. It offers features like token transfers, balance checks, staking, deploying new tokens, requesting faucet funds, trading with customizable slippage, yield farming with Lulo, and accessing LangChain tools for enhanced blockchain interactions. Users can also track current transactions per second (TPS), fetch token data by ticker or address, launch pump & fun tokens, create Meteora DLMM pools, buy/sell tokens with Raydium liquidity, and burn/close token accounts individually or in batches.
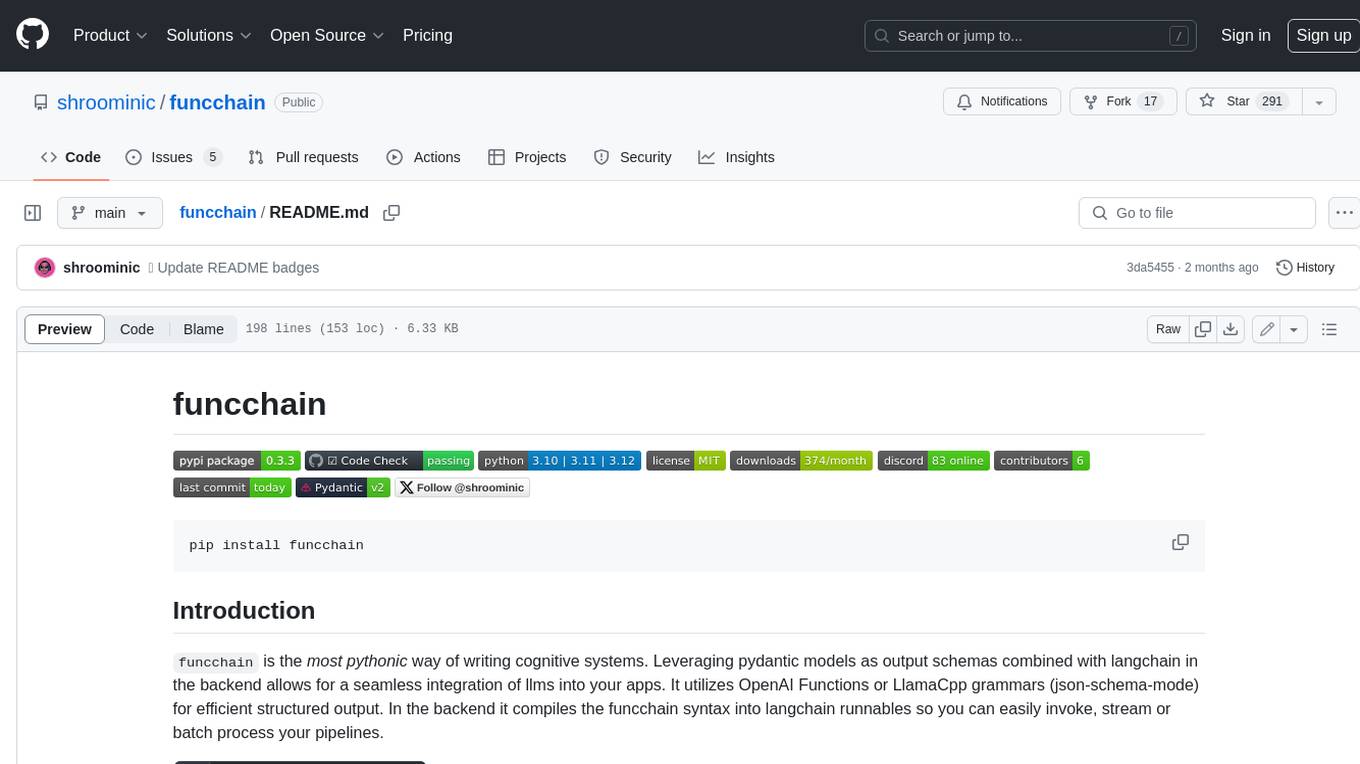
funcchain
Funcchain is a Python library that allows you to easily write cognitive systems by leveraging Pydantic models as output schemas and LangChain in the backend. It provides a seamless integration of LLMs into your apps, utilizing OpenAI Functions or LlamaCpp grammars (json-schema-mode) for efficient structured output. Funcchain compiles the Funcchain syntax into LangChain runnables, enabling you to invoke, stream, or batch process your pipelines effortlessly.
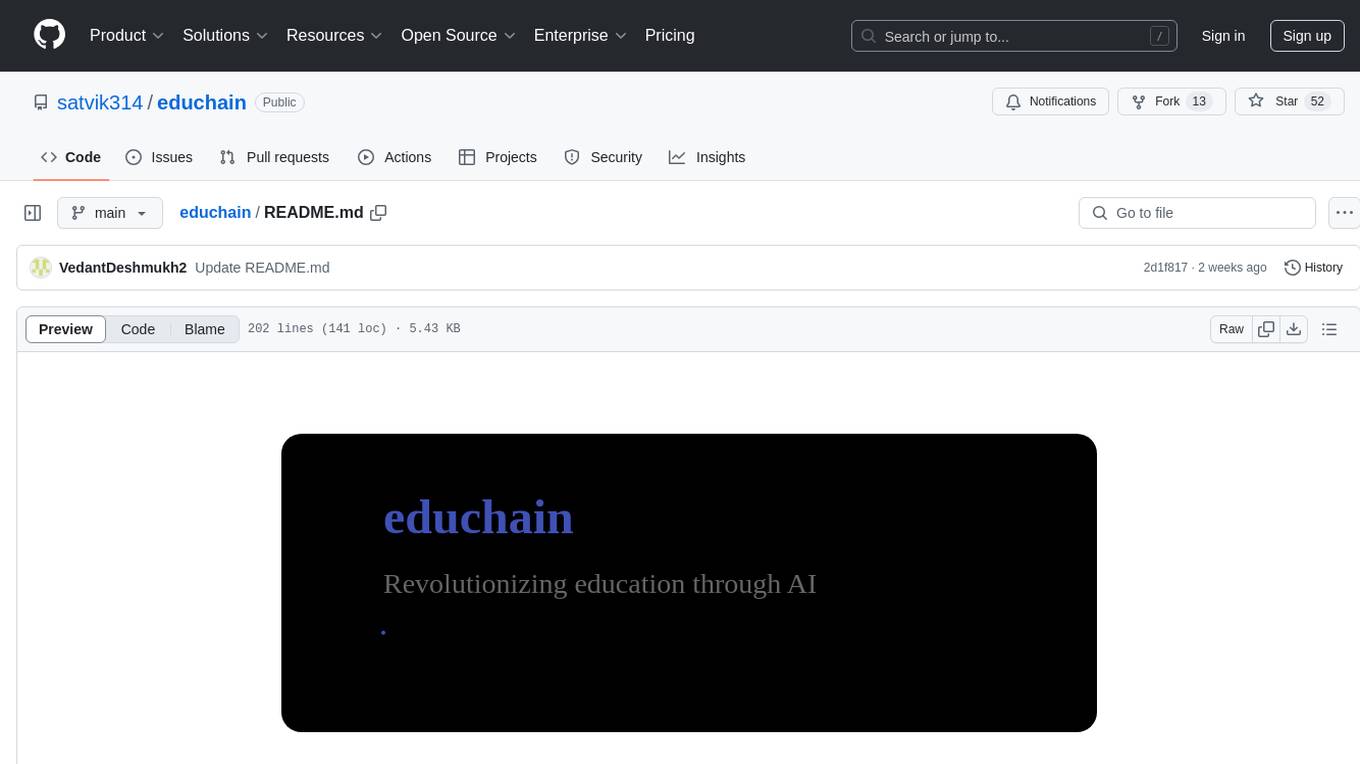
educhain
Educhain is a powerful Python package that leverages Generative AI to create engaging and personalized educational content. It enables users to generate multiple-choice questions, create lesson plans, and support various LLM models. Users can export questions to JSON, PDF, and CSV formats, customize prompt templates, and generate questions from text, PDF, URL files, youtube videos, and images. Educhain outperforms traditional methods in content generation speed and quality. It offers advanced configuration options and has a roadmap for future enhancements, including integration with popular Learning Management Systems and a mobile app for content generation on-the-go.
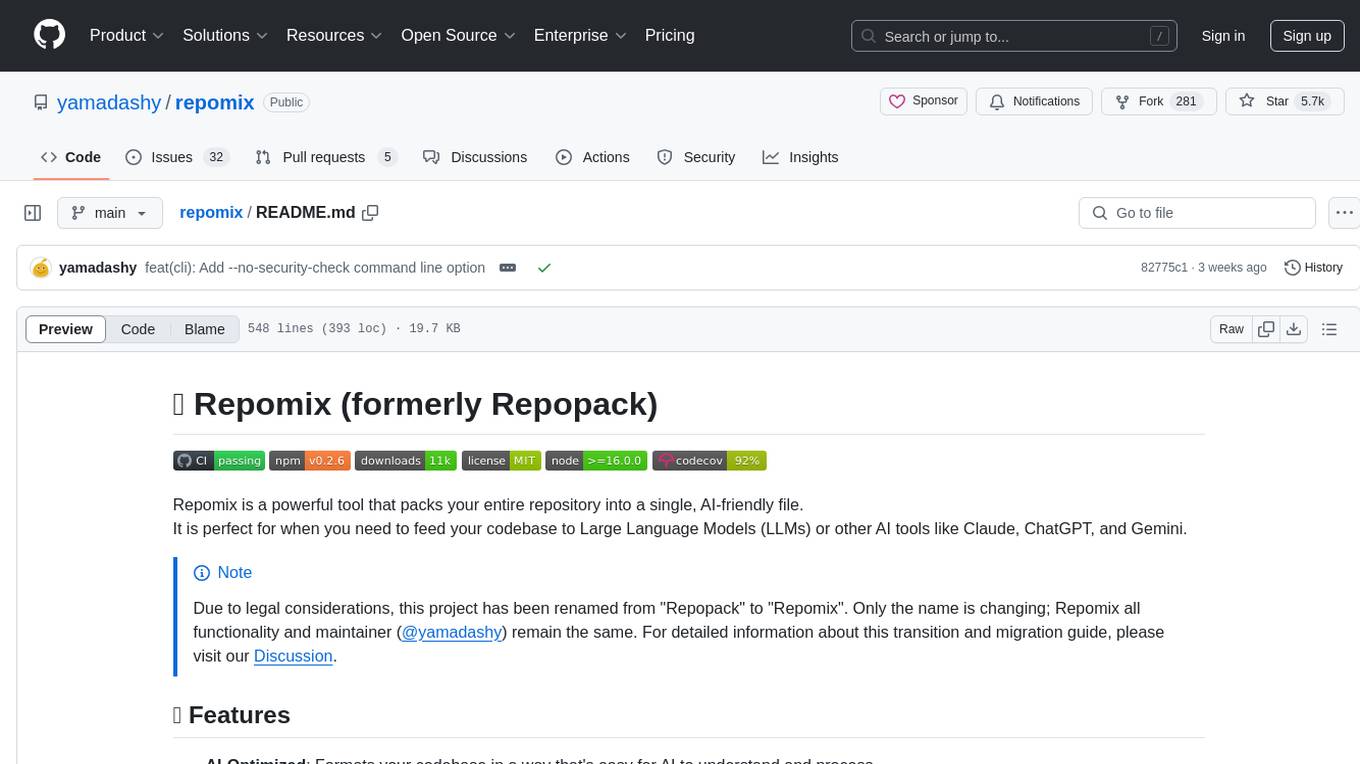
repomix
Repomix is a powerful tool that packs your entire repository into a single, AI-friendly file. It is designed to format your codebase for easy understanding by AI tools like Large Language Models (LLMs), Claude, ChatGPT, and Gemini. Repomix offers features such as AI optimization, token counting, simplicity in usage, customization options, Git awareness, and security-focused checks using Secretlint. It allows users to pack their entire repository or specific directories/files using glob patterns, and even supports processing remote Git repositories. The tool generates output in plain text, XML, or Markdown formats, with options for including/excluding files, removing comments, and performing security checks. Repomix also provides a global configuration option, custom instructions for AI context, and a security check feature to detect sensitive information in files.
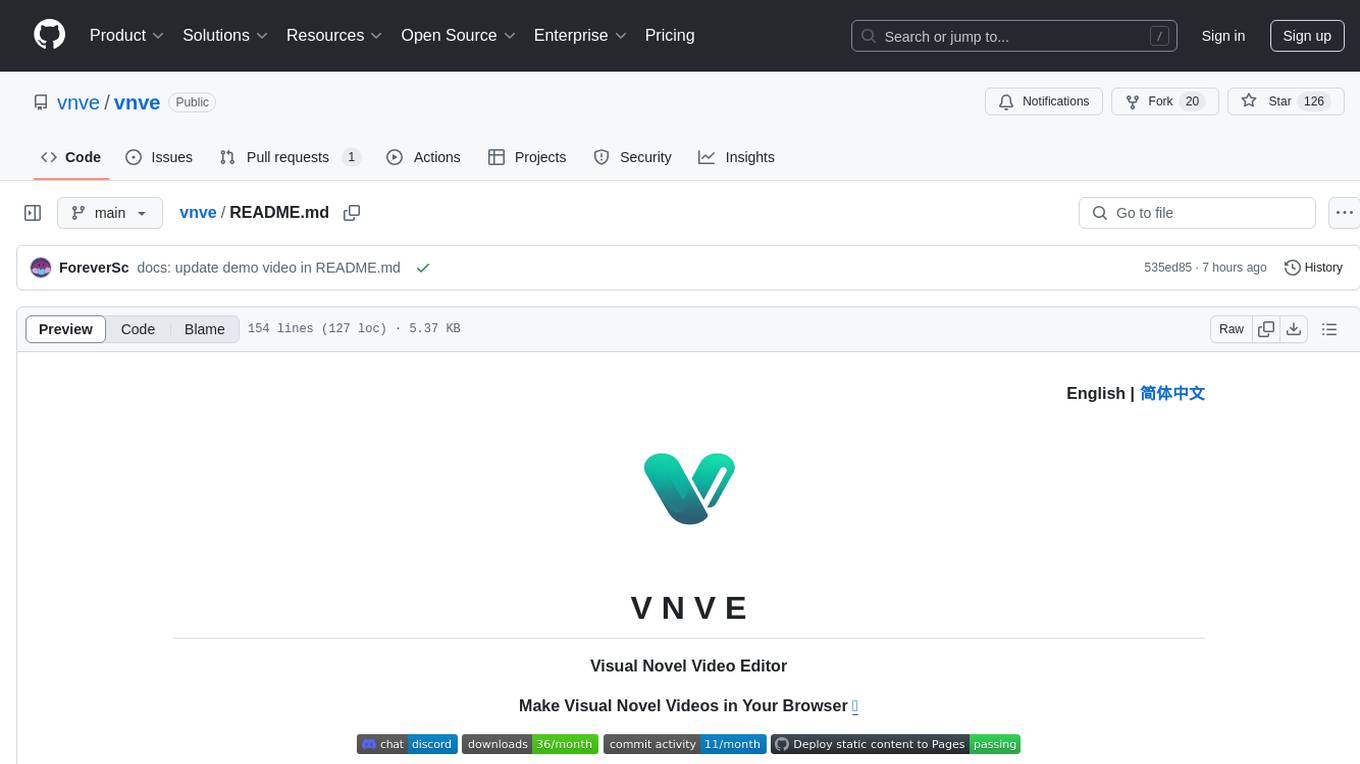
vnve
VNVE is a Visual Novel Video Editor that allows users to create visual novel videos in their browser with AI-powered rapid creation. It offers a low-cost production solution for converting textual content into videos, creating interactive videos for gaming experiences, and making video teasers for novels and short video dramas. The tool is a pure front-end Typescript implementation powered by PixiJS + WebCodecs, and users can also create videos programmatically using the npm package. VNVE is tailored specifically for visual novels, focusing on text content and simplifying the video creation process for users.
For similar tasks
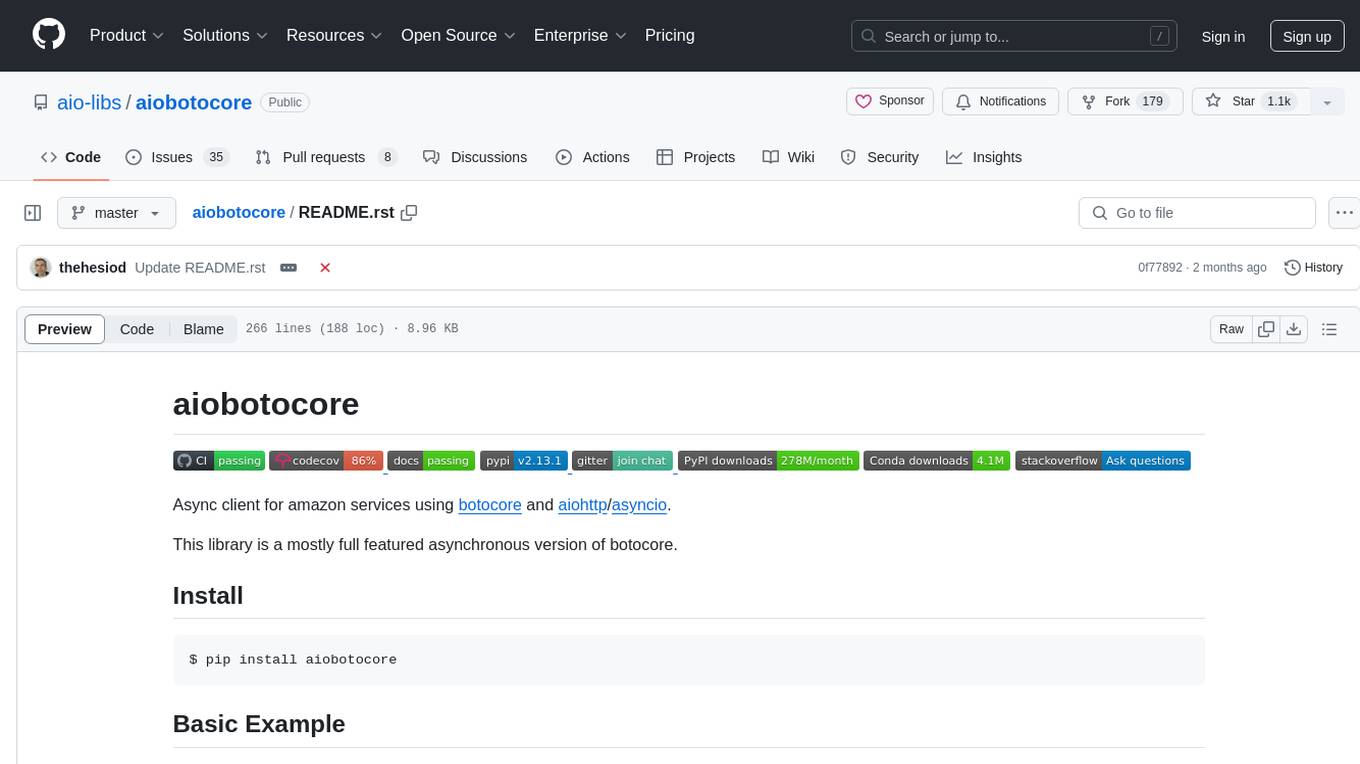
aiobotocore
aiobotocore is an async client for Amazon services using botocore and aiohttp/asyncio. It provides a mostly full-featured asynchronous version of botocore, allowing users to interact with various AWS services asynchronously. The library supports operations such as uploading objects to S3, getting object properties, listing objects, and deleting objects. It also offers context manager examples for managing resources efficiently. aiobotocore supports multiple AWS services like S3, DynamoDB, SNS, SQS, CloudFormation, and Kinesis, with basic methods tested for each service. Users can run tests using moto for mocked tests or against personal Amazon keys. Additionally, the tool enables type checking and code completion for better development experience.
For similar jobs
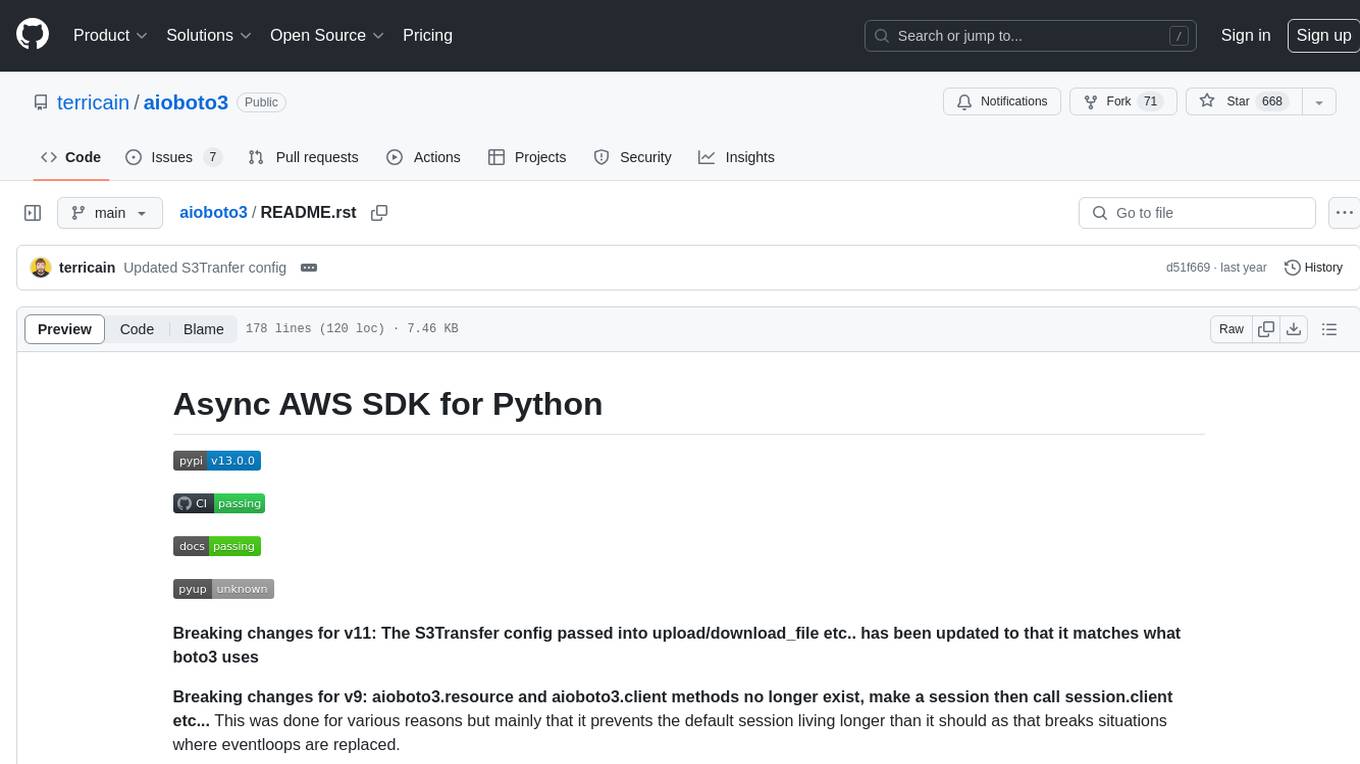
aioboto3
aioboto3 is an async AWS SDK for Python that allows users to use near enough all of the boto3 client commands in an async manner just by prefixing the command with `await`. It combines the great work of boto3 and aiobotocore, enabling users to use higher level APIs provided by boto3 in an asynchronous manner. The package provides support for various AWS services such as DynamoDB, S3, Kinesis, SSM Parameter Store, and Athena. It also offers features like client-side encryption using KMS-Managed master keys and supports asyncifying `get_presigned_url`. The library closely mimics the usage of boto3 and is mainly developed to be used in async microservices.
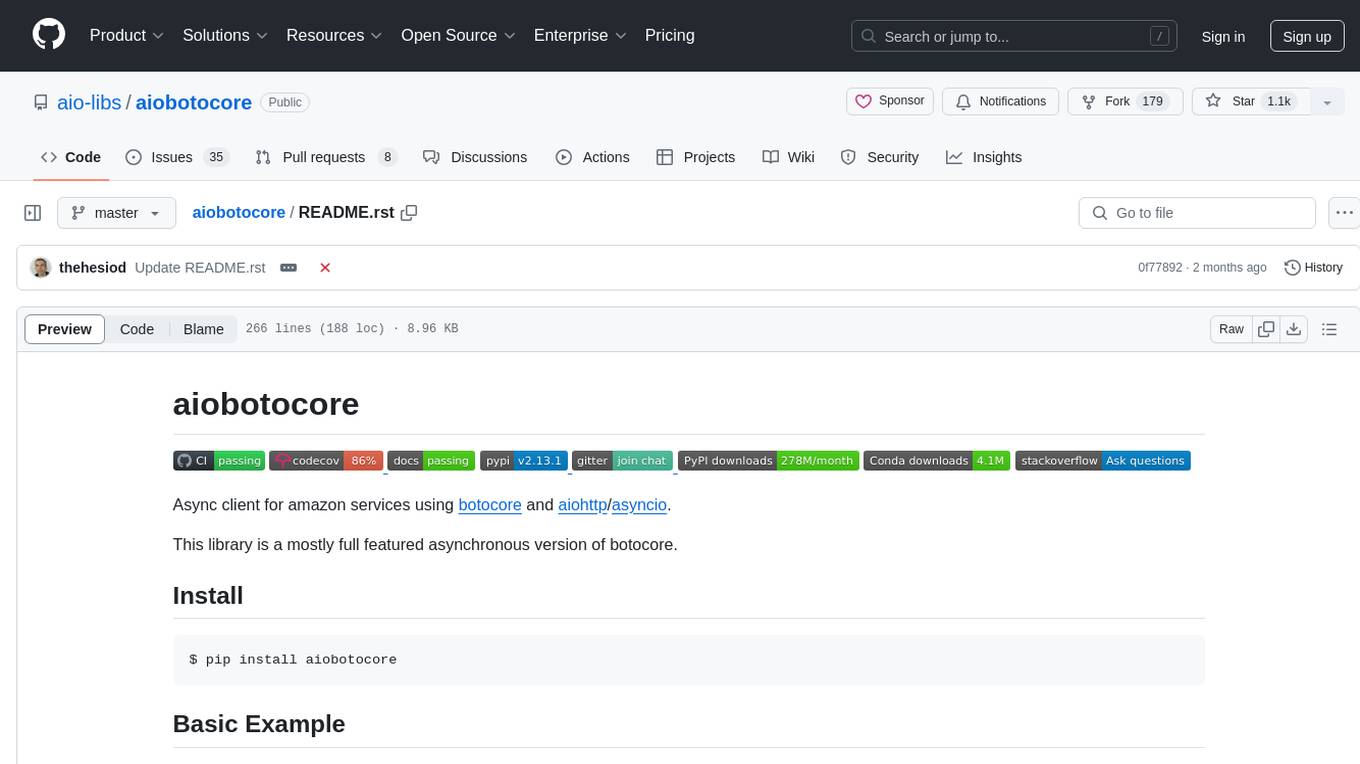
aiobotocore
aiobotocore is an async client for Amazon services using botocore and aiohttp/asyncio. It provides a mostly full-featured asynchronous version of botocore, allowing users to interact with various AWS services asynchronously. The library supports operations such as uploading objects to S3, getting object properties, listing objects, and deleting objects. It also offers context manager examples for managing resources efficiently. aiobotocore supports multiple AWS services like S3, DynamoDB, SNS, SQS, CloudFormation, and Kinesis, with basic methods tested for each service. Users can run tests using moto for mocked tests or against personal Amazon keys. Additionally, the tool enables type checking and code completion for better development experience.
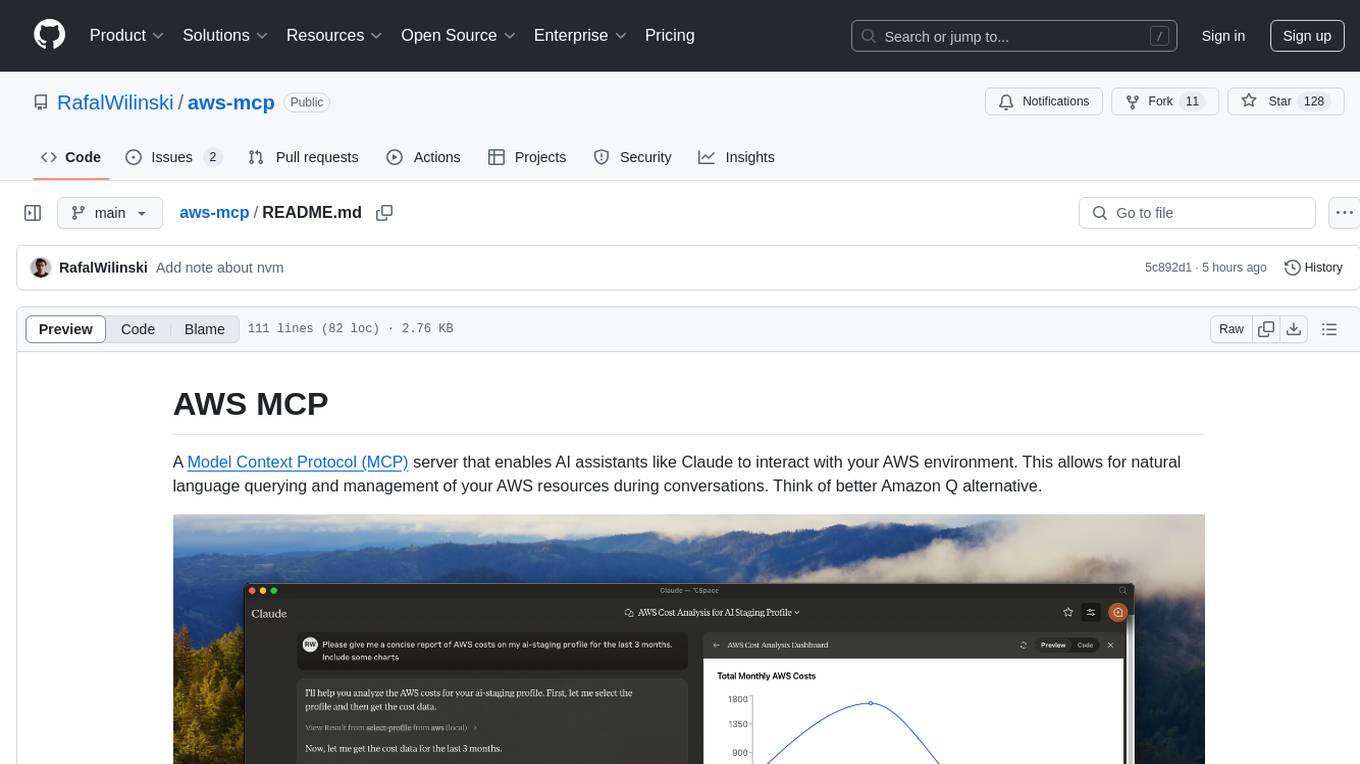
aws-mcp
AWS MCP is a Model Context Protocol (MCP) server that facilitates interactions between AI assistants and AWS environments. It allows for natural language querying and management of AWS resources during conversations. The server supports multiple AWS profiles, SSO authentication, multi-region operations, and secure credential handling. Users can locally execute commands with their AWS credentials, enhancing the conversational experience with AWS resources.
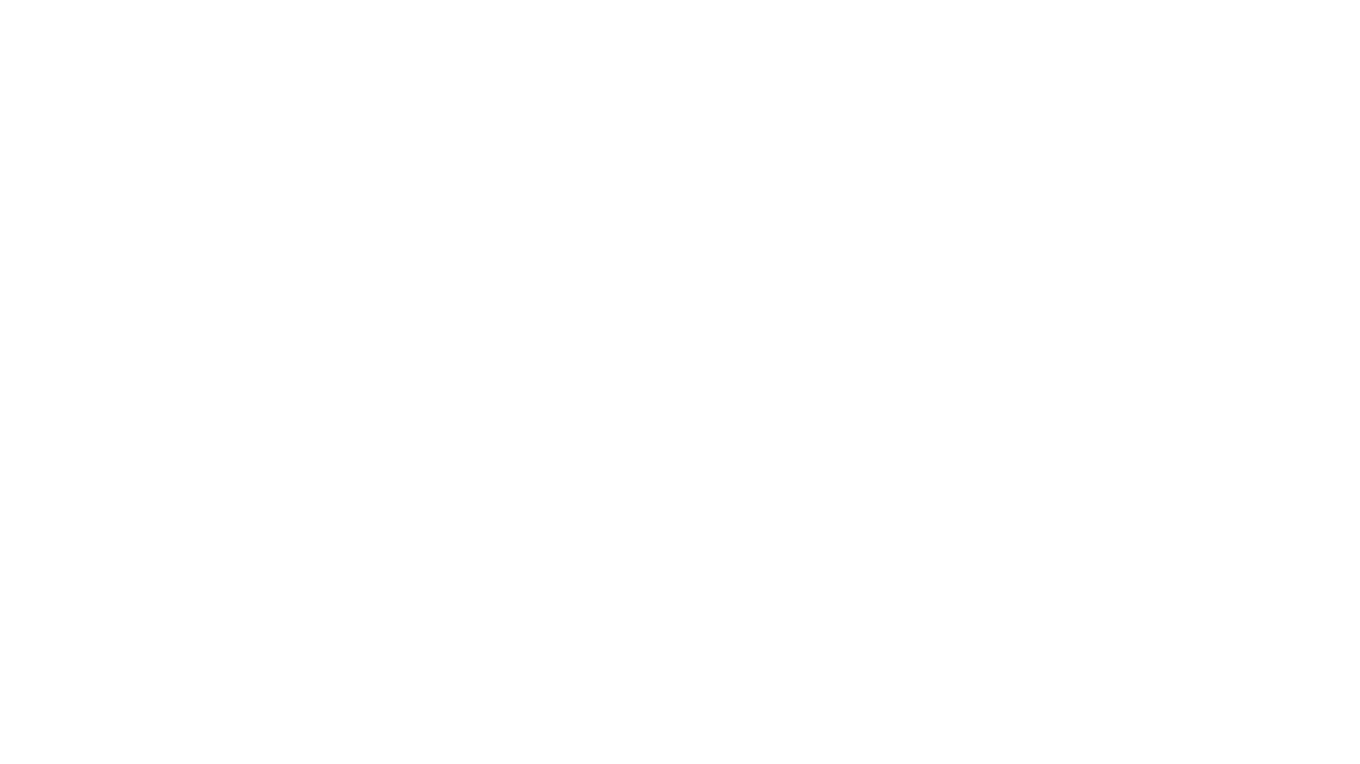
AirGo
AirGo is a front and rear end separation, multi user, multi protocol proxy service management system, simple and easy to use. It supports vless, vmess, shadowsocks, and hysteria2.
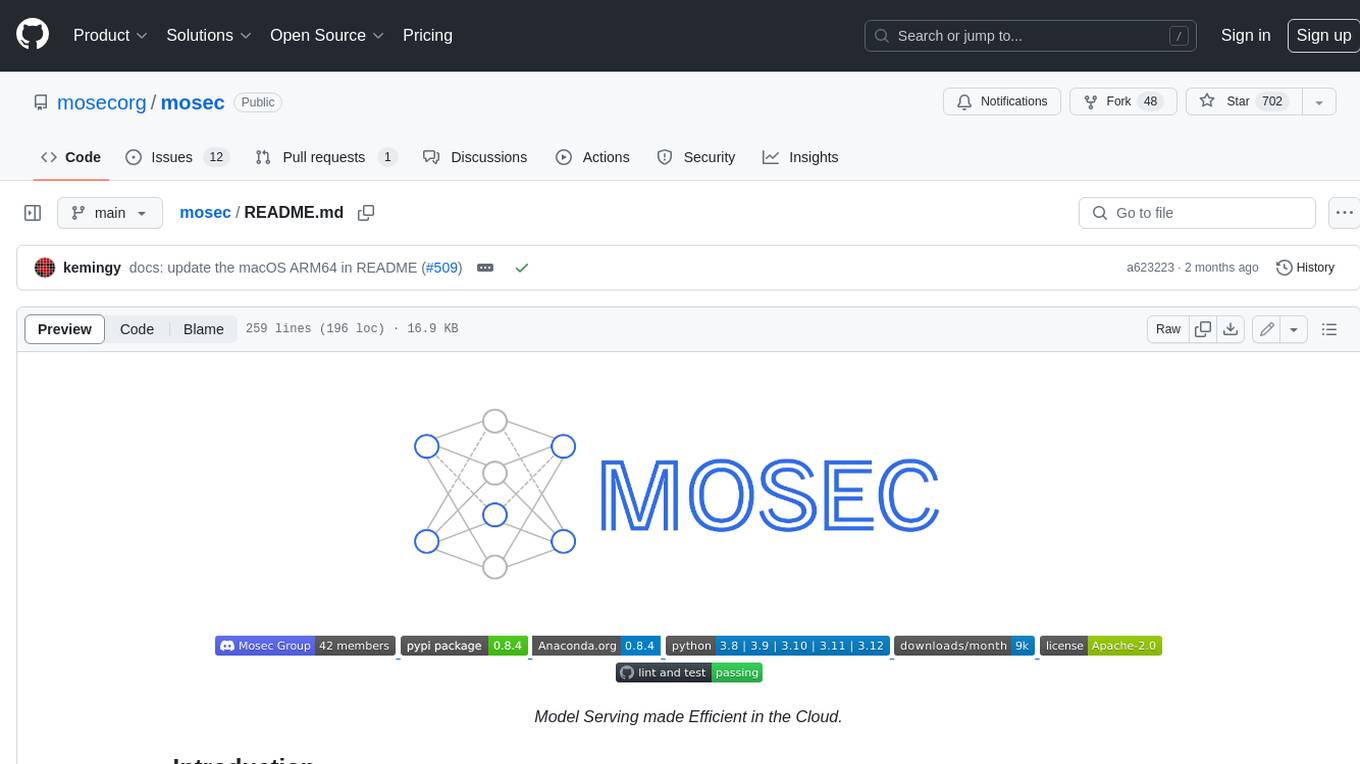
mosec
Mosec is a high-performance and flexible model serving framework for building ML model-enabled backend and microservices. It bridges the gap between any machine learning models you just trained and the efficient online service API. * **Highly performant** : web layer and task coordination built with Rust 🦀, which offers blazing speed in addition to efficient CPU utilization powered by async I/O * **Ease of use** : user interface purely in Python 🐍, by which users can serve their models in an ML framework-agnostic manner using the same code as they do for offline testing * **Dynamic batching** : aggregate requests from different users for batched inference and distribute results back * **Pipelined stages** : spawn multiple processes for pipelined stages to handle CPU/GPU/IO mixed workloads * **Cloud friendly** : designed to run in the cloud, with the model warmup, graceful shutdown, and Prometheus monitoring metrics, easily managed by Kubernetes or any container orchestration systems * **Do one thing well** : focus on the online serving part, users can pay attention to the model optimization and business logic
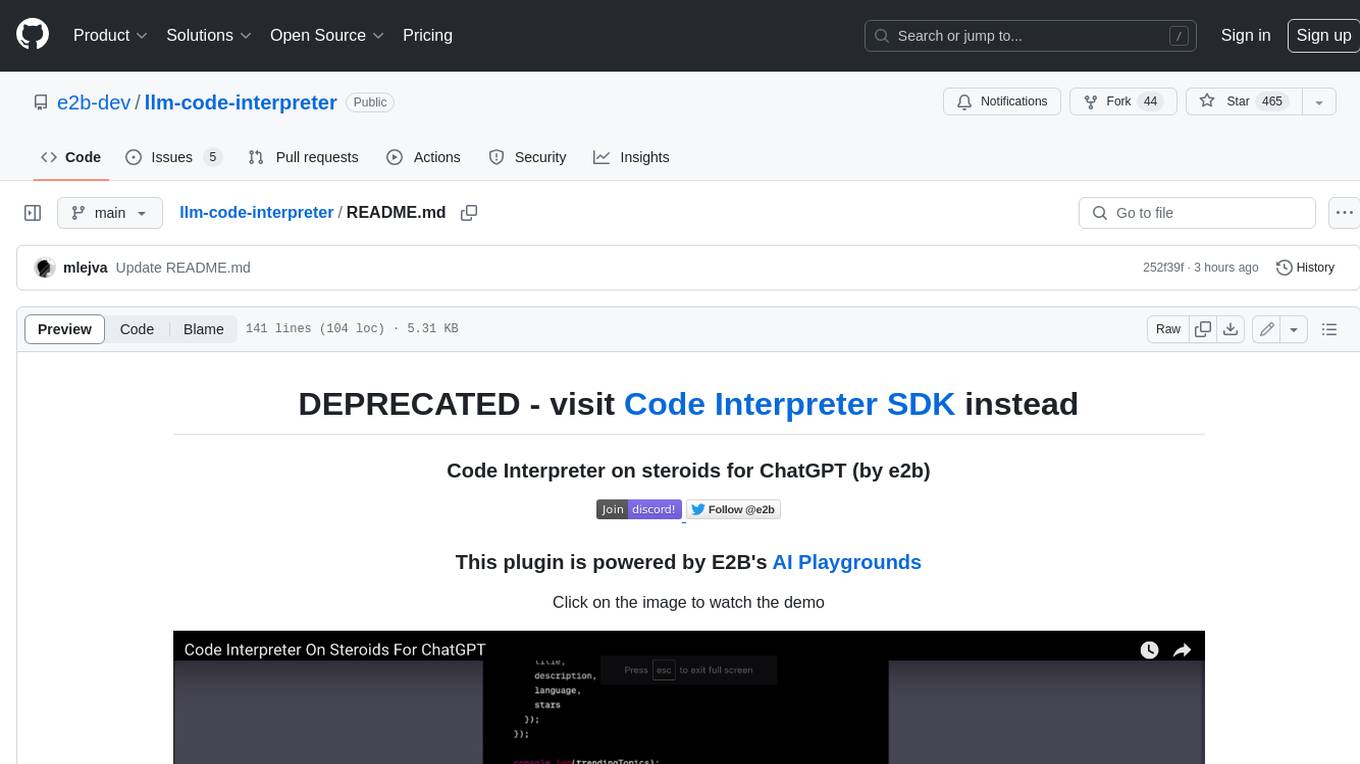
llm-code-interpreter
The 'llm-code-interpreter' repository is a deprecated plugin that provides a code interpreter on steroids for ChatGPT by E2B. It gives ChatGPT access to a sandboxed cloud environment with capabilities like running any code, accessing Linux OS, installing programs, using filesystem, running processes, and accessing the internet. The plugin exposes commands to run shell commands, read files, and write files, enabling various possibilities such as running different languages, installing programs, starting servers, deploying websites, and more. It is powered by the E2B API and is designed for agents to freely experiment within a sandboxed environment.
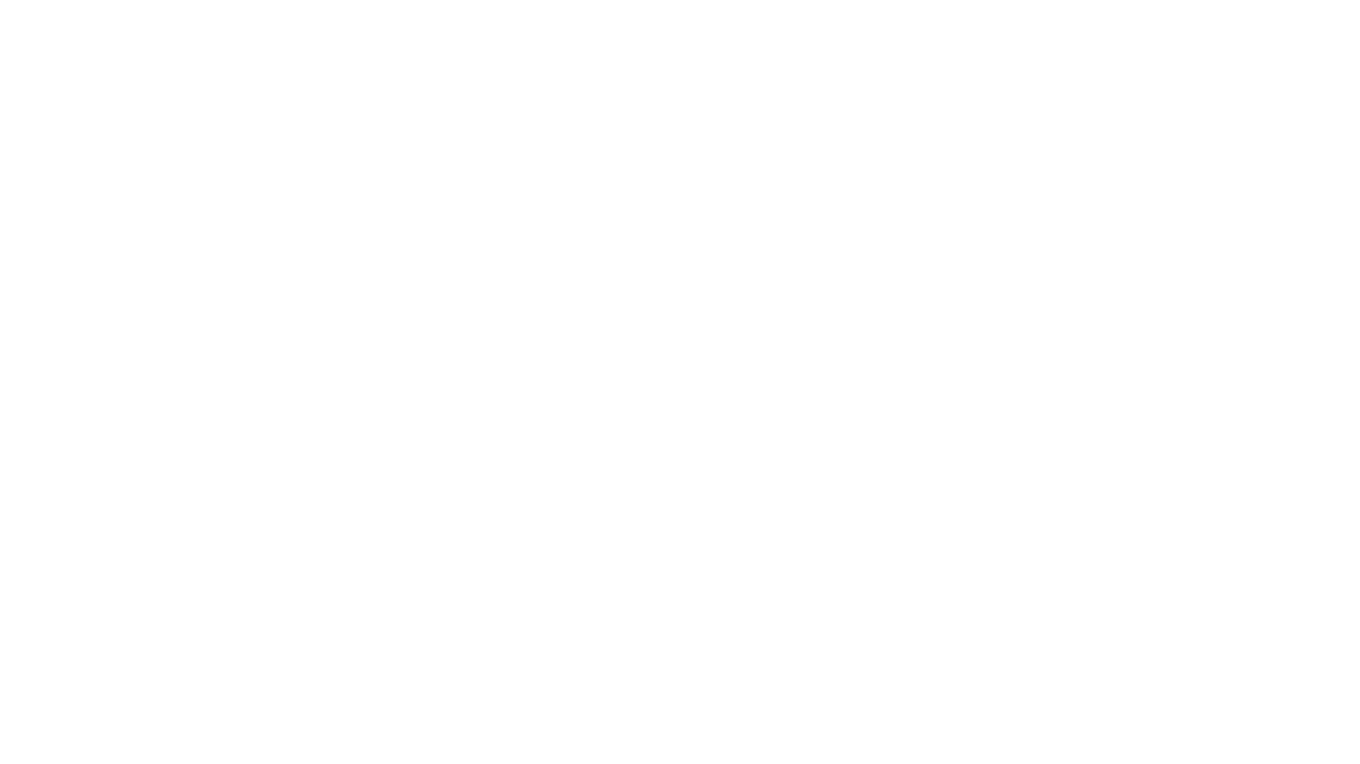
pezzo
Pezzo is a fully cloud-native and open-source LLMOps platform that allows users to observe and monitor AI operations, troubleshoot issues, save costs and latency, collaborate, manage prompts, and deliver AI changes instantly. It supports various clients for prompt management, observability, and caching. Users can run the full Pezzo stack locally using Docker Compose, with prerequisites including Node.js 18+, Docker, and a GraphQL Language Feature Support VSCode Extension. Contributions are welcome, and the source code is available under the Apache 2.0 License.
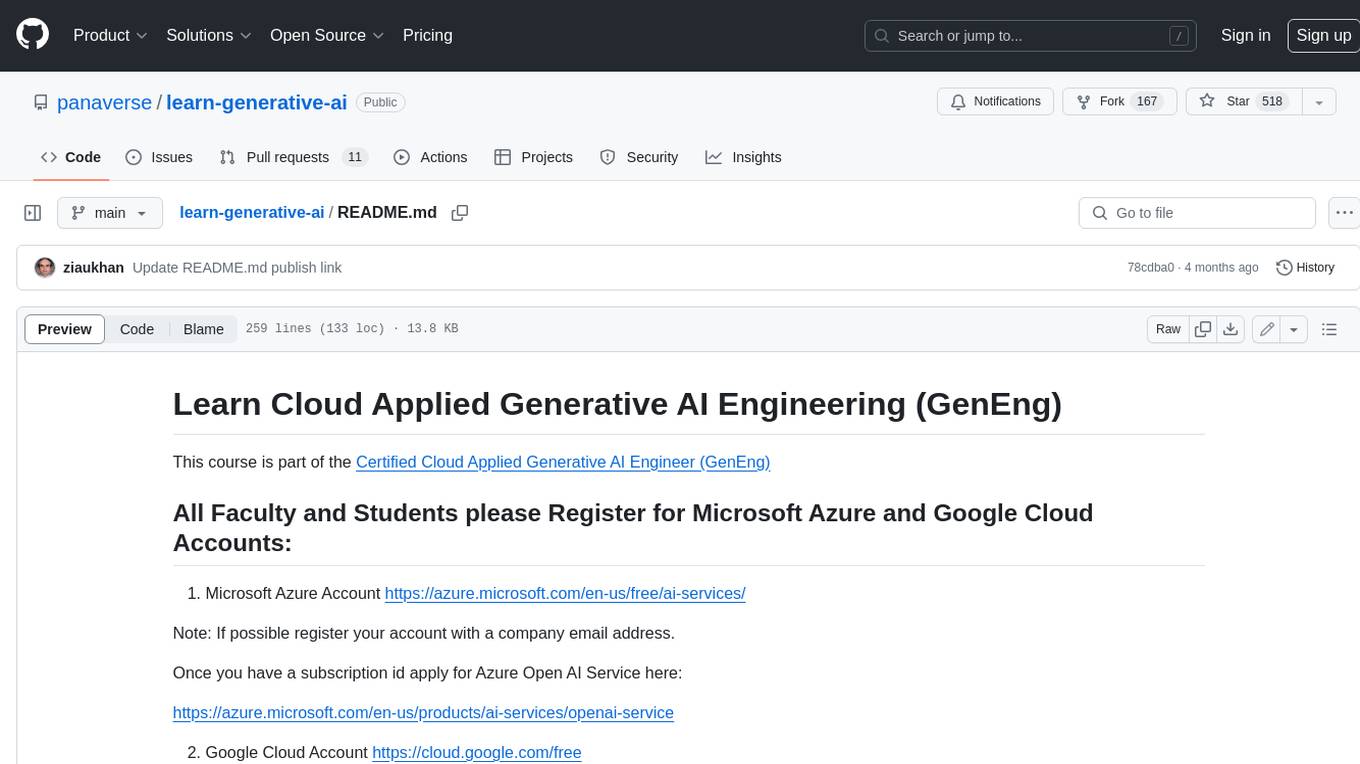
learn-generative-ai
Learn Cloud Applied Generative AI Engineering (GenEng) is a course focusing on the application of generative AI technologies in various industries. The course covers topics such as the economic impact of generative AI, the role of developers in adopting and integrating generative AI technologies, and the future trends in generative AI. Students will learn about tools like OpenAI API, LangChain, and Pinecone, and how to build and deploy Large Language Models (LLMs) for different applications. The course also explores the convergence of generative AI with Web 3.0 and its potential implications for decentralized intelligence.