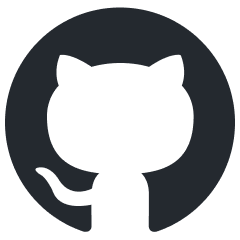
MarkLLM
MarkLLM: An Open-Source Toolkit for LLM Watermarking.
Stars: 257
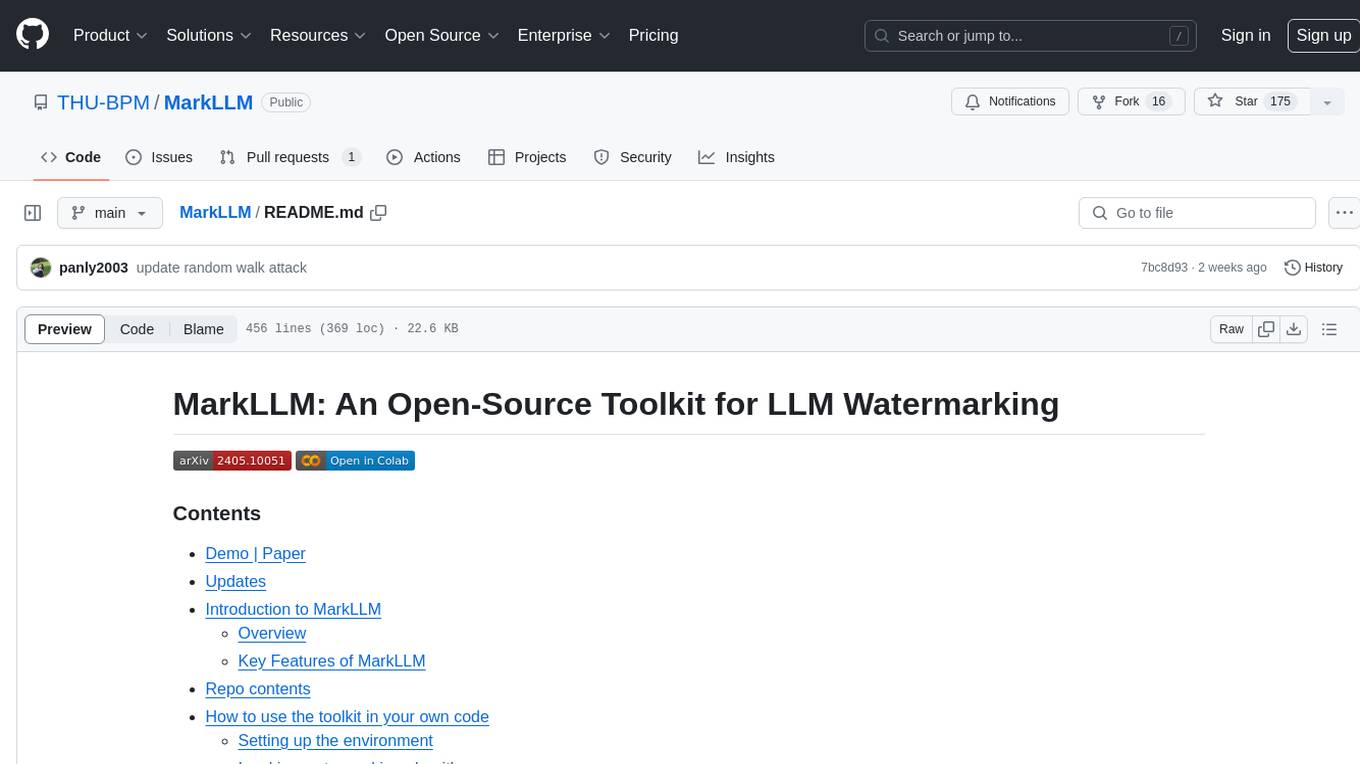
MarkLLM is an open-source toolkit designed for watermarking technologies within large language models (LLMs). It simplifies access, understanding, and assessment of watermarking technologies, supporting various algorithms, visualization tools, and evaluation modules. The toolkit aids researchers and the community in ensuring the authenticity and origin of machine-generated text.
README:
- Demo | Paper
- Updates
- Introduction to MarkLLM
- Repo contents
- How to use the toolkit in your own code
- More user examples
- Demo jupyter notebooks
- Python package
- Related Materials
- Citations
- Google Colab: We utilize Google Colab as our platform to fully publicly demonstrate the capabilities of MarkLLM through a Jupyter Notebook.
- Video Introduction: We provide a video introduction of our system on YouTube to faciliate easy understanding.
- Website Demo: We have also developed a website to facilitate interaction. Due to resource limitations, we cannot offer live access to everyone. Instead, we provide a demonstration video.
- Paper:''MarkLLM: An Open-source toolkit for LLM Watermarking'' by Leyi Pan, Aiwei Liu, Zhiwei He, Zitian Gao, Xuandong Zhao, Yijian Lu, Binglin Zhou, Shuliang Liu, Xuming Hu, Lijie Wen, Irwin King, Philip S. Yu
- 🎉 (2024.10.07) Provide an alternative, equivalent implementation of the EXP watermarking algorithm (EXPGumbel) utilizing Gumbel noise. With this implementation, users should be able to modify the watermark strength by adjusting the sampling temperature in the configuration file.
- 🎉 (2024.10.07) Add Unbiased watermarking method.
- 🎉 (2024.10.06) We are excited to announce that our paper "MarkLLM: An Open-Source Toolkit for LLM Watermarking" has been accepted by EMNLP 2024 Demo!
- 🎉 (2024.08.08) Add DiPmark watermarking method. Thanks to Sheng Guan for his PR!
- 🎉 (2024.08.01) Released as a python package! Try
pip install markllm
. We provide a user example at the end of this file. - 🎉 (2024.07.13) Add ITSEdit watermarking method. Thanks to Yiming Liu for his PR!
- 🎉 (2024.07.09) Add more hashing schemes for KGW (skip, min, additive, selfhash). Thanks to Yichen Di for his PR!
- 🎉 (2024.07.08) Add top-k filter for watermarking methods in Christ family. Thanks to Kai Shi for his PR!
- 🎉 (2024.07.03) Updated Back-Translation Attack. Thanks to Zihan Tang for his PR!
- 🎉 (2024.06.19) Updated Random Walk Attack from the impossibility results of strong watermarking paper at ICML, 2024. (Blog). Thanks to Hanlin Zhang for his PR!
- 🎉 (2024.05.23) We're thrilled to announce the release of our website demo!
MarkLLM is an open-source toolkit developed to facilitate the research and application of watermarking technologies within large language models (LLMs). As the use of large language models (LLMs) expands, ensuring the authenticity and origin of machine-generated text becomes critical. MarkLLM simplifies the access, understanding, and assessment of watermarking technologies, making it accessible to both researchers and the broader community.
-
Implementation Framework: MarkLLM provides a unified and extensible platform for the implementation of various LLM watermarking algorithms. It currently supports nine specific algorithms from two prominent families, facilitating the integration and expansion of watermarking techniques.
Framework Design:
Currently Supported Algorithms:
-
Visualization Solutions: The toolkit includes custom visualization tools that enable clear and insightful views into how different watermarking algorithms operate under various scenarios. These visualizations help demystify the algorithms' mechanisms, making them more understandable for users.
-
Evaluation Module: With 12 evaluation tools that cover detectability, robustness, and impact on text quality, MarkLLM stands out in its comprehensive approach to assessing watermarking technologies. It also features customizable automated evaluation pipelines that cater to diverse needs and scenarios, enhancing the toolkit's practical utility.
Tools:
- Success Rate Calculator of Watermark Detection: FundamentalSuccessRateCalculator, DynamicThresholdSuccessRateCalculator
- Text Editor: WordDeletion, SynonymSubstitution, ContextAwareSynonymSubstitution, GPTParaphraser, DipperParaphraser, RandomWalkAttack
- Text Quality Analyzer: PPLCalculator, LogDiversityAnalyzer, BLEUCalculator, PassOrNotJudger, GPTDiscriminator
Pipelines:
- Watermark Detection Pipeline: WatermarkedTextDetectionPipeline, UnwatermarkedTextDetectionPipeline
- Text Quality Pipeline: DirectTextQualityAnalysisPipeline, ReferencedTextQualityAnalysisPipeline, ExternalDiscriminatorTextQualityAnalysisPipeline
Below is the directory structure of the MarkLLM project, which encapsulates its three core functionalities within the watermark/
, visualize/
, and evaluation/
directories. To facilitate user understanding and demonstrate the toolkit's ease of use, we provide a variety of test cases. The test code can be found in the test/
directory.
MarkLLM/
├── config/ # Configuration files for various watermark algorithms
│ ├── EWD.json
│ ├── EXPEdit.json
│ ├── EXP.json
│ ├── KGW.json
│ ├── ITSEdit.json
│ ├── SIR.json
│ ├── SWEET.json
│ ├── Unigram.json
│ ├── UPV.json
│ └── XSIR.json
├── dataset/ # Datasets used in the project
│ ├── c4/
│ ├── human_eval/
│ └── wmt16_de_en/
├── evaluation/ # Evaluation module of MarkLLM, including tools and pipelines
│ ├── dataset.py # Script for handling dataset operations within evaluations
│ ├── examples/ # Scripts for automated evaluations using pipelines
│ │ ├── assess_detectability.py
│ │ ├── assess_quality.py
│ │ └── assess_robustness.py
│ ├── pipelines/ # Pipelines for structured evaluation processes
│ │ ├── detection.py
│ │ └── quality_analysis.py
│ └── tools/ # Evaluation tools
│ ├── oracle.py
│ ├── success_rate_calculator.py
├── text_editor.py
│ └── text_quality_analyzer.py
├── exceptions/ # Custom exception definitions for error handling
│ └── exceptions.py
├── font/ # Fonts needed for visualization purposes
├── MarkLLM_demo.ipynb # Jupyter Notebook
├── test/ # Test cases and examples for user testing
│ ├── test_method.py
│ ├── test_pipeline.py
│ └── test_visualize.py
├── utils/ # Helper classes and functions supporting various operations
│ ├── openai_utils.py
│ ├── transformers_config.py
│ └── utils.py
├── visualize/ # Visualization Solutions module of MarkLLM
│ ├── color_scheme.py
│ ├── data_for_visualization.py
│ ├── font_settings.py
│ ├── legend_settings.py
│ ├── page_layout_settings.py
│ └── visualizer.py
├── watermark/ # Implementation framework for watermark algorithms
│ ├── auto_watermark.py # AutoWatermark class
│ ├── base.py # Base classes and functions for watermarking
│ ├── ewd/
│ ├── exp/
│ ├── exp_edit/
│ ├── kgw/
│ ├── its_edit/
│ ├── sir/
│ ├── sweet/
│ ├── unigram/
│ ├── upv/
│ └── xsir/
├── README.md # Main project documentation
└── requirements.txt # Dependencies required for the project
- python 3.9
- pytorch
- pip install -r requirements.txt
Tips: If you wish to utilize the EXPEdit or ITSEdit algorithm, you will need to import for .pyx file, take EXPEdit as an example:
- run
python watermark/exp_edit/cython_files/setup.py build_ext --inplace
- move the generated
.so
file intowatermark/exp_edit/cython_files/
import torch
from watermark.auto_watermark import AutoWatermark
from utils.transformers_config import TransformersConfig
from transformers import AutoModelForCausalLM, AutoTokenizer
# Device
device = "cuda" if torch.cuda.is_available() else "cpu"
# Transformers config
transformers_config = TransformersConfig(model=AutoModelForCausalLM.from_pretrained('facebook/opt-1.3b').to(device),
tokenizer=AutoTokenizer.from_pretrained('facebook/opt-1.3b'),
vocab_size=50272,
device=device,
max_new_tokens=200,
min_length=230,
do_sample=True,
no_repeat_ngram_size=4)
# Load watermark algorithm
myWatermark = AutoWatermark.load('KGW',
algorithm_config='config/KGW.json',
transformers_config=transformers_config)
# Prompt
prompt = 'Good Morning.'
# Generate and detect
watermarked_text = myWatermark.generate_watermarked_text(prompt)
detect_result = myWatermark.detect_watermark(watermarked_text)
unwatermarked_text = myWatermark.generate_unwatermarked_text(prompt)
detect_result = myWatermark.detect_watermark(unwatermarked_text)
Assuming you already have a pair of watermarked_text
and unwatermarked_text
, and you wish to visualize the differences and specifically highlight the watermark within the watermarked text using a watermarking algorithm, you can utilize the visualization tools available in the visualize/
directory.
KGW Family
import torch
from visualize.font_settings import FontSettings
from watermark.auto_watermark import AutoWatermark
from utils.transformers_config import TransformersConfig
from transformers import AutoModelForCausalLM, AutoTokenizer
from visualize.visualizer import DiscreteVisualizer
from visualize.legend_settings import DiscreteLegendSettings
from visualize.page_layout_settings import PageLayoutSettings
from visualize.color_scheme import ColorSchemeForDiscreteVisualization
# Load watermark algorithm
device = "cuda" if torch.cuda.is_available() else "cpu"
transformers_config = TransformersConfig(
model=AutoModelForCausalLM.from_pretrained('facebook/opt-1.3b').to(device),
tokenizer=AutoTokenizer.from_pretrained('facebook/opt-1.3b'),
vocab_size=50272,
device=device,
max_new_tokens=200,
min_length=230,
do_sample=True,
no_repeat_ngram_size=4)
myWatermark = AutoWatermark.load('KGW',
algorithm_config='config/KGW.json',
transformers_config=transformers_config)
# Get data for visualization
watermarked_data = myWatermark.get_data_for_visualization(watermarked_text)
unwatermarked_data = myWatermark.get_data_for_visualization(unwatermarked_text)
# Init visualizer
visualizer = DiscreteVisualizer(color_scheme=ColorSchemeForDiscreteVisualization(),
font_settings=FontSettings(),
page_layout_settings=PageLayoutSettings(),
legend_settings=DiscreteLegendSettings())
# Visualize
watermarked_img = visualizer.visualize(data=watermarked_data,
show_text=True,
visualize_weight=True,
display_legend=True)
unwatermarked_img = visualizer.visualize(data=unwatermarked_data,
show_text=True,
visualize_weight=True,
display_legend=True)
# Save
watermarked_img.save("KGW_watermarked.png")
unwatermarked_img.save("KGW_unwatermarked.png")
Christ Family
import torch
from visualize.font_settings import FontSettings
from watermark.auto_watermark import AutoWatermark
from utils.transformers_config import TransformersConfig
from transformers import AutoModelForCausalLM, AutoTokenizer
from visualize.visualizer import ContinuousVisualizer
from visualize.legend_settings import ContinuousLegendSettings
from visualize.page_layout_settings import PageLayoutSettings
from visualize.color_scheme import ColorSchemeForContinuousVisualization
# Load watermark algorithm
device = "cuda" if torch.cuda.is_available() else "cpu"
transformers_config = TransformersConfig(
model=AutoModelForCausalLM.from_pretrained('facebook/opt-1.3b').to(device),
tokenizer=AutoTokenizer.from_pretrained('facebook/opt-1.3b'),
vocab_size=50272,
device=device,
max_new_tokens=200,
min_length=230,
do_sample=True,
no_repeat_ngram_size=4)
myWatermark = AutoWatermark.load('EXP',
algorithm_config='config/EXP.json',
transformers_config=transformers_config)
# Get data for visualization
watermarked_data = myWatermark.get_data_for_visualization(watermarked_text)
unwatermarked_data = myWatermark.get_data_for_visualization(unwatermarked_text)
# Init visualizer
visualizer = ContinuousVisualizer(color_scheme=ColorSchemeForContinuousVisualization(),
font_settings=FontSettings(),
page_layout_settings=PageLayoutSettings(),
legend_settings=ContinuousLegendSettings())
# Visualize
watermarked_img = visualizer.visualize(data=watermarked_data,
show_text=True,
visualize_weight=True,
display_legend=True)
unwatermarked_img = visualizer.visualize(data=unwatermarked_data,
show_text=True,
visualize_weight=True,
display_legend=True)
# Save
watermarked_img.save("EXP_watermarked.png")
unwatermarked_img.save("EXP_unwatermarked.png")
For more examples on how to use the visualization tools, please refer to the test/test_visualize.py
script in the project directory.
Using Watermark Detection Pipelines
import torch
from evaluation.dataset import C4Dataset
from watermark.auto_watermark import AutoWatermark
from utils.transformers_config import TransformersConfig
from transformers import AutoModelForCausalLM, AutoTokenizer
from evaluation.tools.text_editor import TruncatePromptTextEditor, WordDeletion
from evaluation.tools.success_rate_calculator import DynamicThresholdSuccessRateCalculator
from evaluation.pipelines.detection import WatermarkedTextDetectionPipeline, UnWatermarkedTextDetectionPipeline, DetectionPipelineReturnType
# Load dataset
my_dataset = C4Dataset('dataset/c4/processed_c4.json')
# Device
device = 'cuda' if torch.cuda.is_available() else 'cpu'
# Transformers config
transformers_config = TransformersConfig(
model=AutoModelForCausalLM.from_pretrained('facebook/opt-1.3b').to(device),
tokenizer=AutoTokenizer.from_pretrained('facebook/opt-1.3b'),
vocab_size=50272,
device=device,
max_new_tokens=200,
do_sample=True,
min_length=230,
no_repeat_ngram_size=4)
# Load watermark algorithm
my_watermark = AutoWatermark.load('KGW',
algorithm_config='config/KGW.json',
transformers_config=transformers_config)
# Init pipelines
pipeline1 = WatermarkedTextDetectionPipeline(
dataset=my_dataset,
text_editor_list=[TruncatePromptTextEditor(), WordDeletion(ratio=0.3)],
show_progress=True,
return_type=DetectionPipelineReturnType.SCORES)
pipeline2 = UnWatermarkedTextDetectionPipeline(dataset=my_dataset,
text_editor_list=[],
show_progress=True,
return_type=DetectionPipelineReturnType.SCORES)
# Evaluate
calculator = DynamicThresholdSuccessRateCalculator(labels=['TPR', 'F1'], rule='best')
print(calculator.calculate(pipeline1.evaluate(my_watermark), pipeline2.evaluate(my_watermark)))
Using Text Quality Analysis Pipeline
import torch
from evaluation.dataset import C4Dataset
from watermark.auto_watermark import AutoWatermark
from utils.transformers_config import TransformersConfig
from transformers import AutoModelForCausalLM, AutoTokenizer
from evaluation.tools.text_editor import TruncatePromptTextEditor
from evaluation.tools.text_quality_analyzer import PPLCalculator
from evaluation.pipelines.quality_analysis import DirectTextQualityAnalysisPipeline, QualityPipelineReturnType
# Load dataset
my_dataset = C4Dataset('dataset/c4/processed_c4.json')
# Device
device = 'cuda' if torch.cuda.is_available() else 'cpu'
# Transformer config
transformers_config = TransformersConfig(
model=AutoModelForCausalLM.from_pretrained('facebook/opt-1.3b').to(device), tokenizer=AutoTokenizer.from_pretrained('facebook/opt-1.3b'),
vocab_size=50272,
device=device,
max_new_tokens=200,
min_length=230,
do_sample=True,
no_repeat_ngram_size=4)
# Load watermark algorithm
my_watermark = AutoWatermark.load('KGW',
algorithm_config='config/KGW.json',
transformers_config=transformers_config)
# Init pipeline
quality_pipeline = DirectTextQualityAnalysisPipeline(
dataset=my_dataset,
watermarked_text_editor_list=[TruncatePromptTextEditor()],
unwatermarked_text_editor_list=[],
analyzer=PPLCalculator(
model=AutoModelForCausalLM.from_pretrained('..model/llama-7b/', device_map='auto'), tokenizer=LlamaTokenizer.from_pretrained('..model/llama-7b/'),
device=device),
unwatermarked_text_source='natural',
show_progress=True,
return_type=QualityPipelineReturnType.MEAN_SCORES)
# Evaluate
print(quality_pipeline.evaluate(my_watermark))
For more examples on how to use the pipelines, please refer to the test/test_pipeline.py
script in the project directory.
Leveraging example scripts for evaluation
In the evaluation/examples/
directory of our repository, you will find a collection of Python scripts specifically designed for systematic and automated evaluation of various algorithms. By using these examples, you can quickly and effectively gauge the d etectability, robustness and impact on text quality of each algorithm implemented within our toolkit.
Note: To execute the scripts in evaluation/examples/
, first run the following command to set the environment variables.
export PYTHONPATH="path_to_the_MarkLLM_project:$PYTHONPATH"
Additional user examples are available in test/
. To execute the scripts contained within, first run the following command to set the environment variables.
export PYTHONPATH="path_to_the_MarkLLM_project:$PYTHONPATH"
In addition to the Colab Jupyter notebook we provide (some models cannot be downloaded due to storage limits), you can also easily deploy using MarkLLM_demo.ipynb
on your local machine.
A user example:
import torch, random
import numpy as np
from markllm.watermark.auto_watermark import AutoWatermark
from markllm.utils.transformers_config import TransformersConfig
from transformers import AutoModelForCausalLM, AutoTokenizer
# Setting random seed for reproducibility
seed = 30
torch.manual_seed(seed)
if torch.cuda.is_available():
torch.cuda.manual_seed_all(seed)
np.random.seed(seed)
random.seed(seed)
# Device
device = "cuda" if torch.cuda.is_available() else "cpu"
# Transformers config
model_name = 'facebook/opt-1.3b'
transformers_config = TransformersConfig(
model=AutoModelForCausalLM.from_pretrained(model_name).to(device),
tokenizer=AutoTokenizer.from_pretrained(model_name),
vocab_size=50272,
device=device,
max_new_tokens=200,
min_length=230,
do_sample=True,
no_repeat_ngram_size=4
)
# Load watermark algorithm
myWatermark = AutoWatermark.load('KGW', transformers_config=transformers_config)
# Prompt and generation
prompt = 'Good Morning.'
watermarked_text = myWatermark.generate_watermarked_text(prompt)
# How would I get started with Python...
unwatermarked_text = myWatermark.generate_unwatermarked_text(prompt)
# I am happy that you are back with ...
# Detection
detect_result_watermarked = myWatermark.detect_watermark(watermarked_text)
# {'is_watermarked': True, 'score': 9.287487590439852}
detect_result_unwatermarked = myWatermark.detect_watermark(unwatermarked_text)
# {'is_watermarked': False, 'score': -0.8443170536763502}
If you are interested in text watermarking for large language models, please read our survey: [2312.07913] A Survey of Text Watermarking in the Era of Large Language Models (arxiv.org). We detail various text watermarking algorithms, evaluation methods, applications, current challenges, and future directions in this survey.
@article{pan2024markllm,
title={MarkLLM: An Open-Source Toolkit for LLM Watermarking},
author={Pan, Leyi and Liu, Aiwei and He, Zhiwei and Gao, Zitian and Zhao, Xuandong and Lu, Yijian and Zhou, Binglin and Liu, Shuliang and Hu, Xuming and Wen, Lijie and others},
journal={arXiv preprint arXiv:2405.10051},
year={2024}
}
For Tasks:
Click tags to check more tools for each tasksFor Jobs:
Alternative AI tools for MarkLLM
Similar Open Source Tools
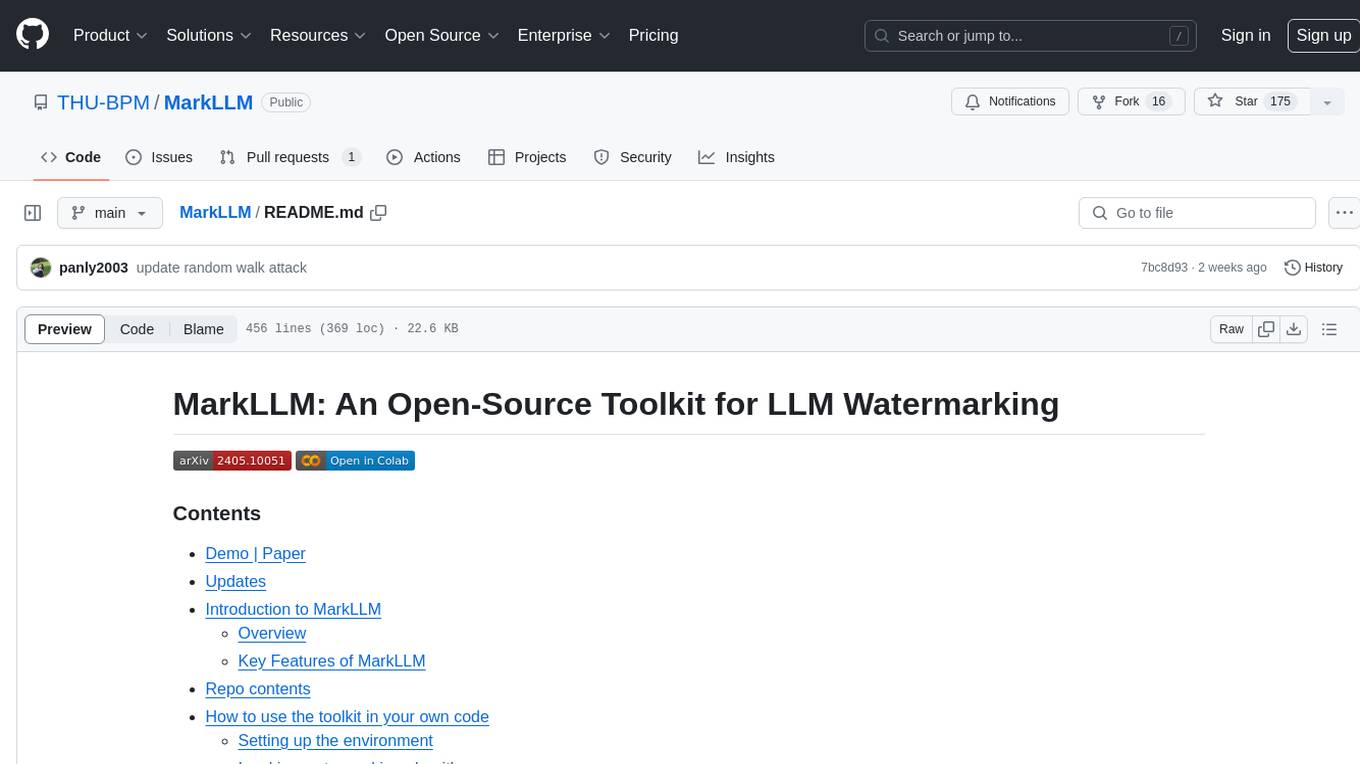
MarkLLM
MarkLLM is an open-source toolkit designed for watermarking technologies within large language models (LLMs). It simplifies access, understanding, and assessment of watermarking technologies, supporting various algorithms, visualization tools, and evaluation modules. The toolkit aids researchers and the community in ensuring the authenticity and origin of machine-generated text.
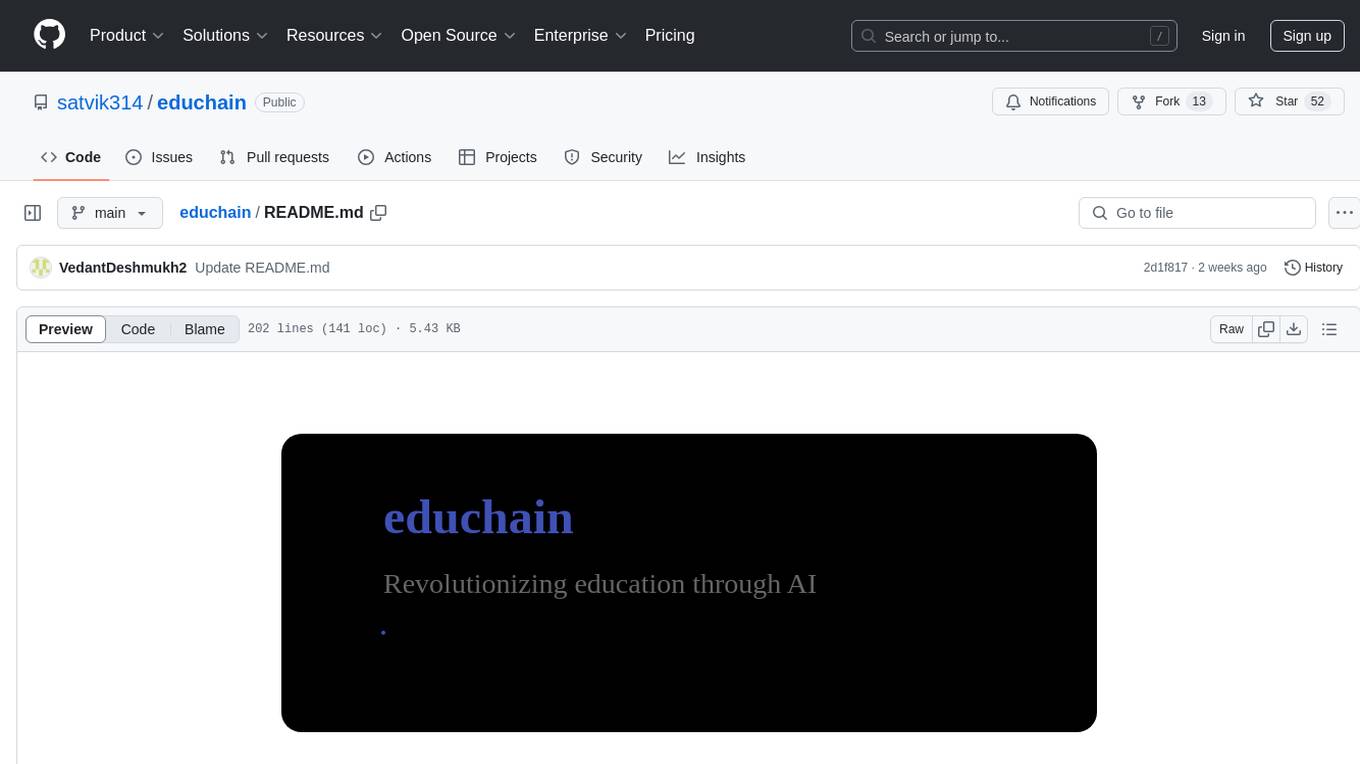
educhain
Educhain is a powerful Python package that leverages Generative AI to create engaging and personalized educational content. It enables users to generate multiple-choice questions, create lesson plans, and support various LLM models. Users can export questions to JSON, PDF, and CSV formats, customize prompt templates, and generate questions from text, PDF, URL files, youtube videos, and images. Educhain outperforms traditional methods in content generation speed and quality. It offers advanced configuration options and has a roadmap for future enhancements, including integration with popular Learning Management Systems and a mobile app for content generation on-the-go.
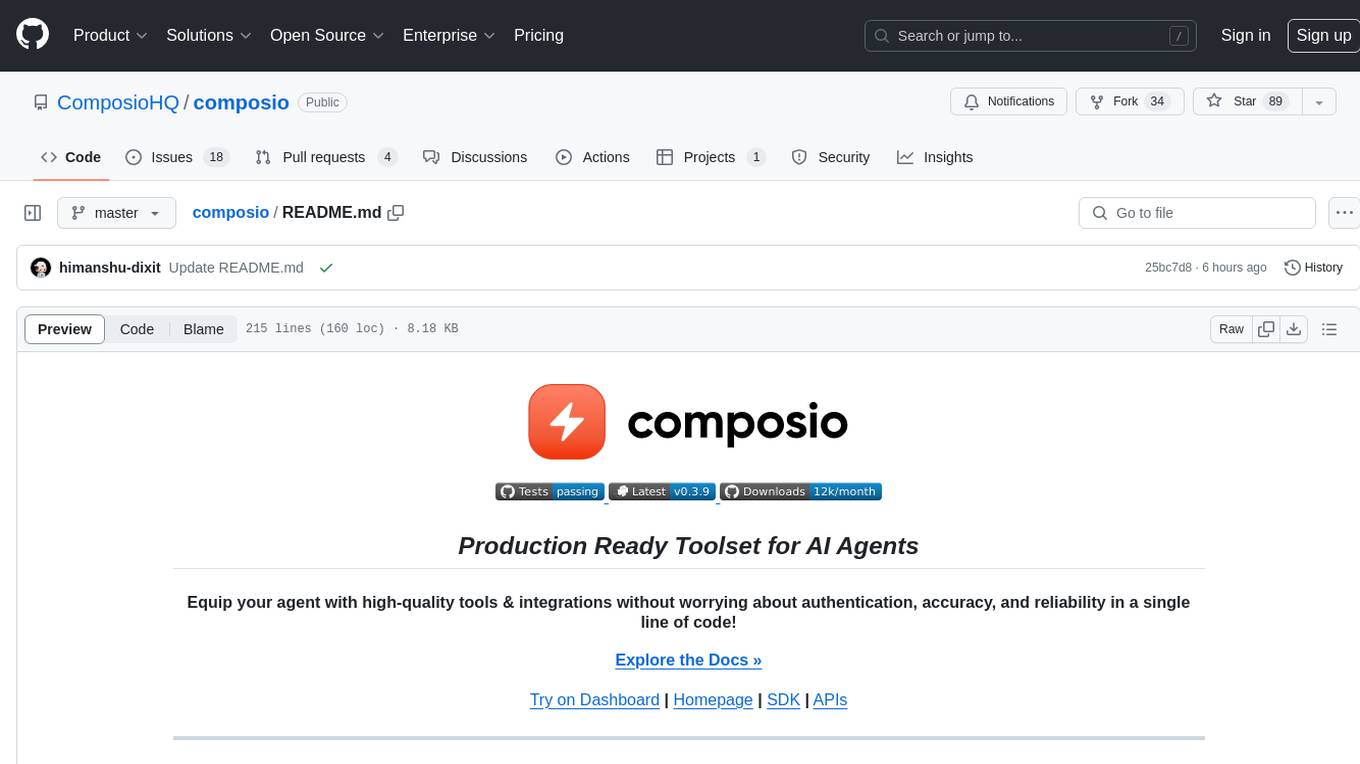
composio
Composio is a production-ready toolset for AI agents that enables users to integrate AI agents with various agentic tools effortlessly. It provides support for over 100 tools across different categories, including popular softwares like GitHub, Notion, Linear, Gmail, Slack, and more. Composio ensures managed authorization with support for six different authentication protocols, offering better agentic accuracy and ease of use. Users can easily extend Composio with additional tools, frameworks, and authorization protocols. The toolset is designed to be embeddable and pluggable, allowing for seamless integration and consistent user experience.
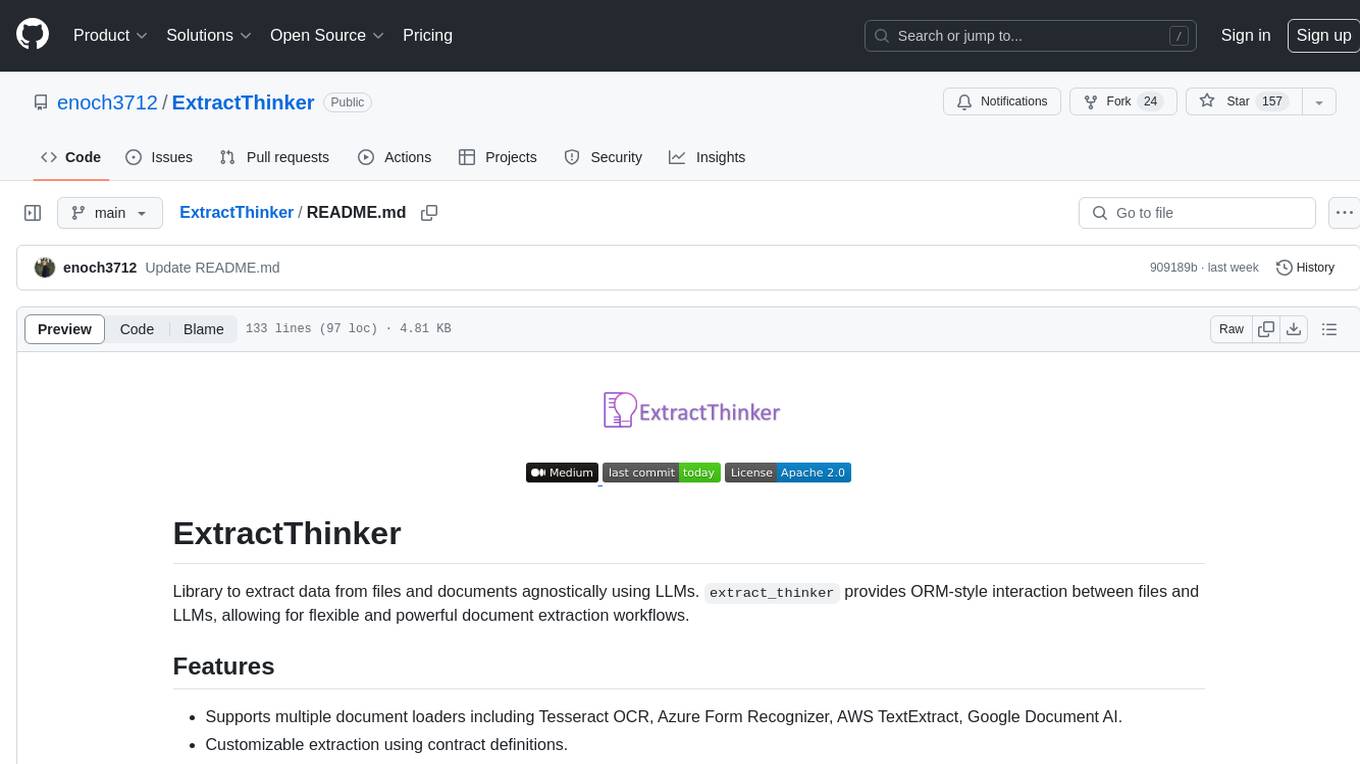
ExtractThinker
ExtractThinker is a library designed for extracting data from files and documents using Language Model Models (LLMs). It offers ORM-style interaction between files and LLMs, supporting multiple document loaders such as Tesseract OCR, Azure Form Recognizer, AWS TextExtract, and Google Document AI. Users can customize extraction using contract definitions, process documents asynchronously, handle various document formats efficiently, and split and process documents. The project is inspired by the LangChain ecosystem and focuses on Intelligent Document Processing (IDP) using LLMs to achieve high accuracy in document extraction tasks.
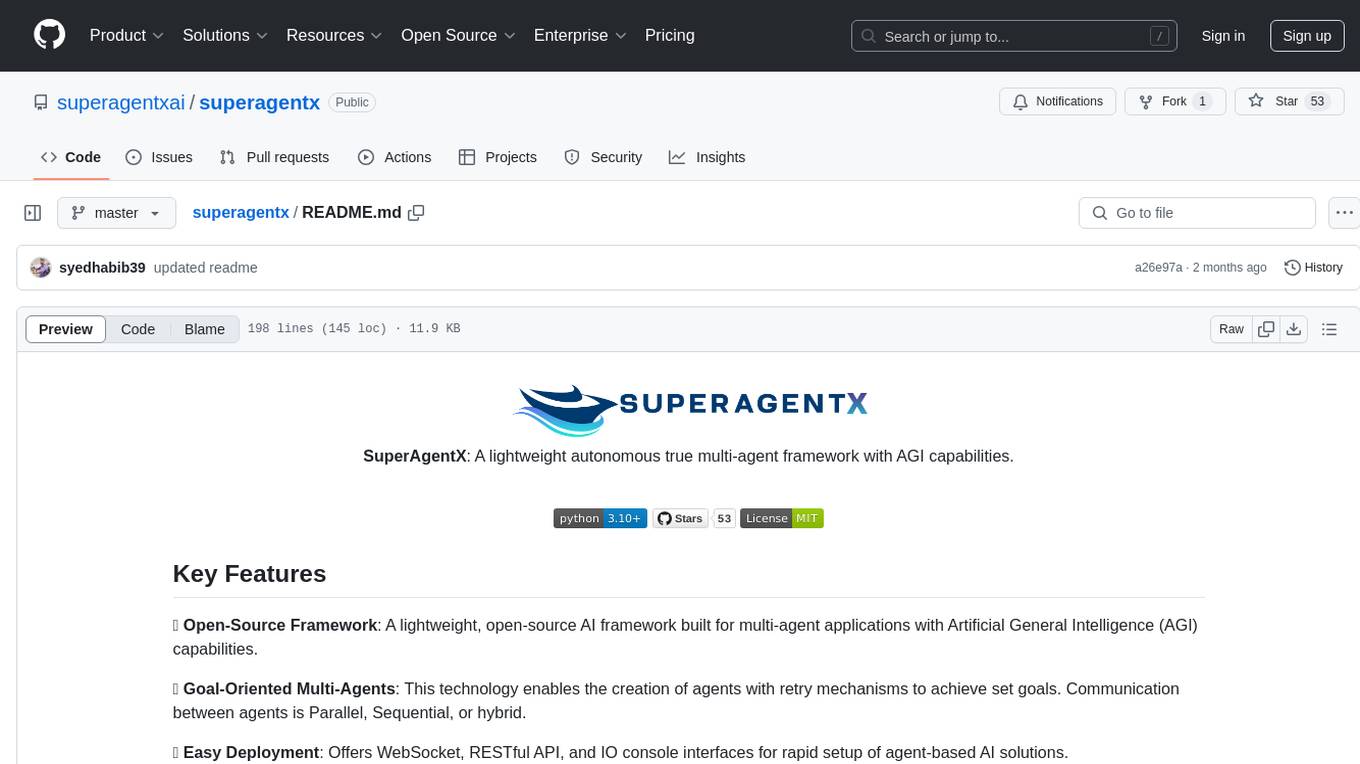
superagentx
SuperAgentX is a lightweight open-source AI framework designed for multi-agent applications with Artificial General Intelligence (AGI) capabilities. It offers goal-oriented multi-agents with retry mechanisms, easy deployment through WebSocket, RESTful API, and IO console interfaces, streamlined architecture with no major dependencies, contextual memory using SQL + Vector databases, flexible LLM configuration supporting various Gen AI models, and extendable handlers for integration with diverse APIs and data sources. It aims to accelerate the development of AGI by providing a powerful platform for building autonomous AI agents capable of executing complex tasks with minimal human intervention.
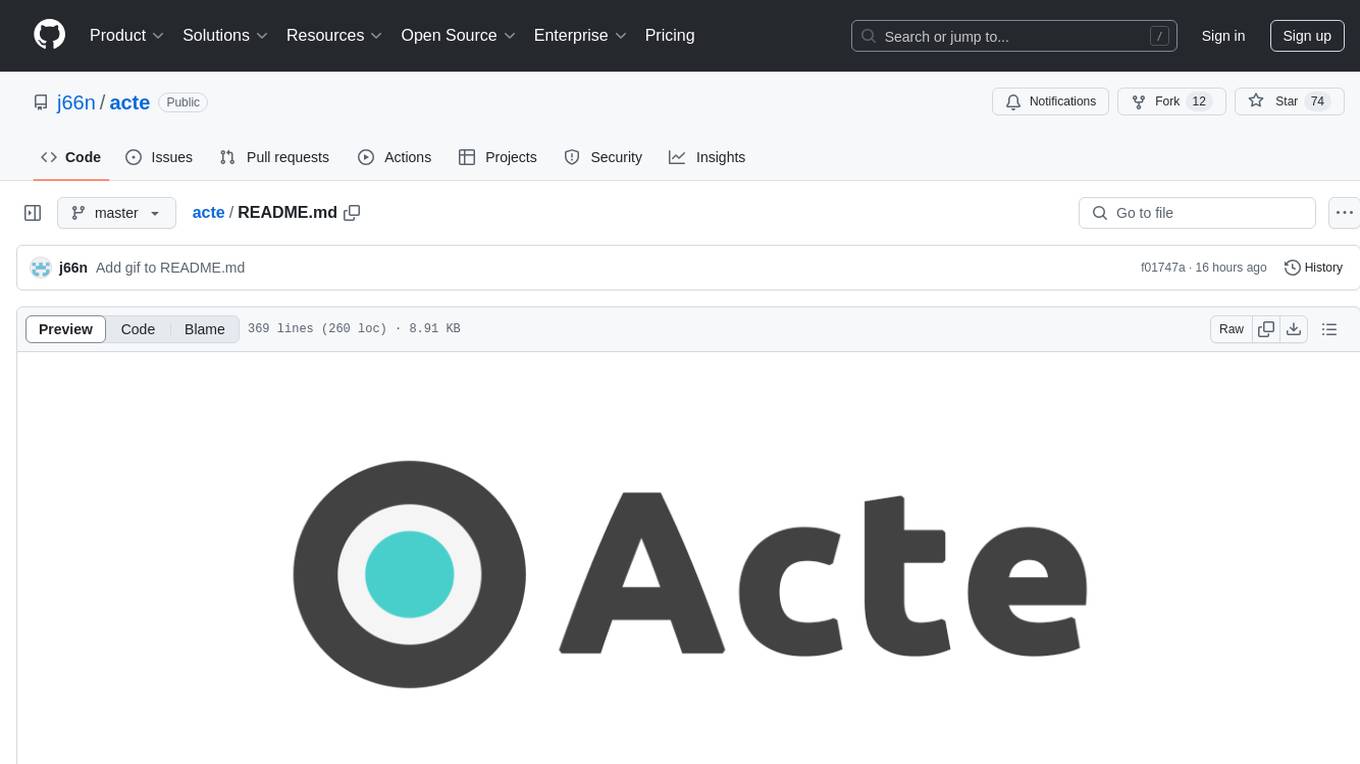
acte
Acte is a framework designed to build GUI-like tools for AI Agents. It aims to address the issues of cognitive load and freedom degrees when interacting with multiple APIs in complex scenarios. By providing a graphical user interface (GUI) for Agents, Acte helps reduce cognitive load and constraints interaction, similar to how humans interact with computers through GUIs. The tool offers APIs for starting new sessions, executing actions, and displaying screens, accessible via HTTP requests or the SessionManager class.
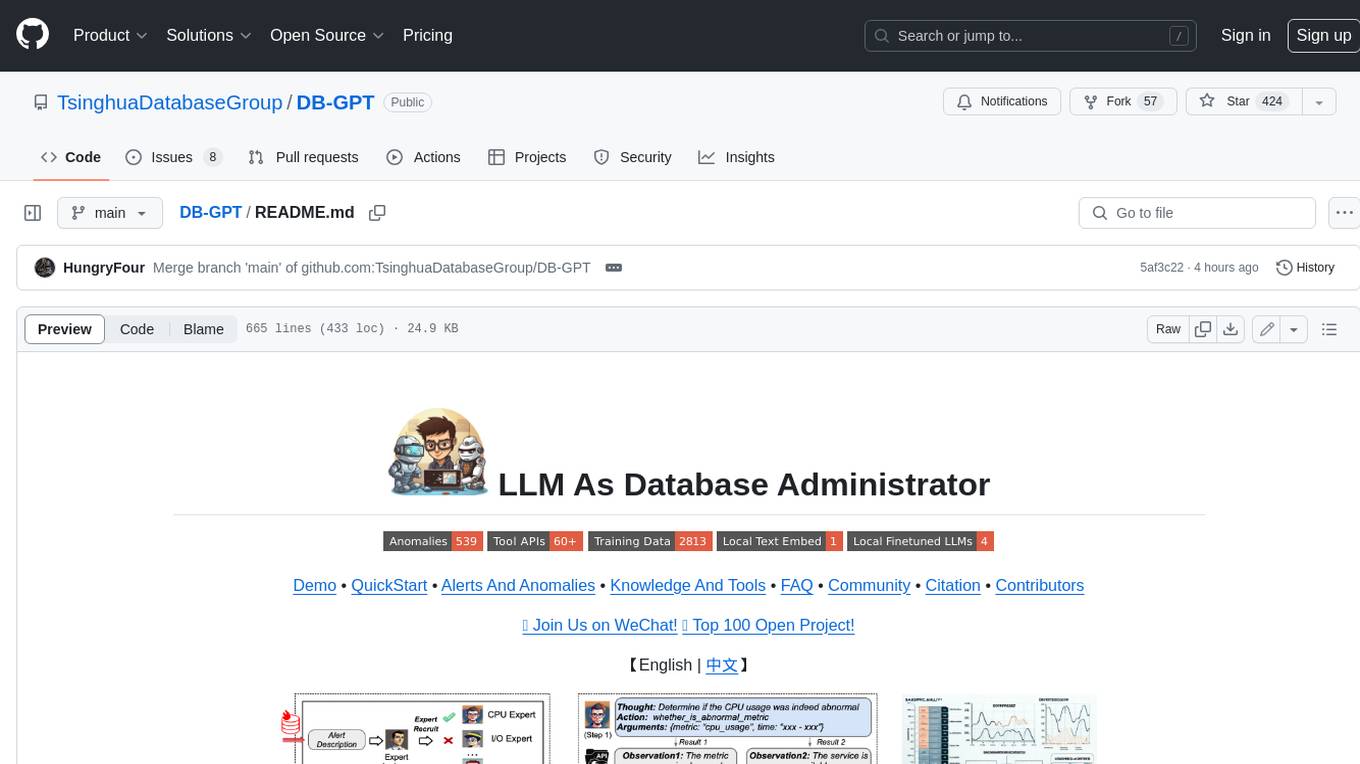
DB-GPT
DB-GPT is a personal database administrator that can solve database problems by reading documents, using various tools, and writing analysis reports. It is currently undergoing an upgrade. **Features:** * **Online Demo:** * Import documents into the knowledge base * Utilize the knowledge base for well-founded Q&A and diagnosis analysis of abnormal alarms * Send feedbacks to refine the intermediate diagnosis results * Edit the diagnosis result * Browse all historical diagnosis results, used metrics, and detailed diagnosis processes * **Language Support:** * English (default) * Chinese (add "language: zh" in config.yaml) * **New Frontend:** * Knowledgebase + Chat Q&A + Diagnosis + Report Replay * **Extreme Speed Version for localized llms:** * 4-bit quantized LLM (reducing inference time by 1/3) * vllm for fast inference (qwen) * Tiny LLM * **Multi-path extraction of document knowledge:** * Vector database (ChromaDB) * RESTful Search Engine (Elasticsearch) * **Expert prompt generation using document knowledge** * **Upgrade the LLM-based diagnosis mechanism:** * Task Dispatching -> Concurrent Diagnosis -> Cross Review -> Report Generation * Synchronous Concurrency Mechanism during LLM inference * **Support monitoring and optimization tools in multiple levels:** * Monitoring metrics (Prometheus) * Flame graph in code level * Diagnosis knowledge retrieval (dbmind) * Logical query transformations (Calcite) * Index optimization algorithms (for PostgreSQL) * Physical operator hints (for PostgreSQL) * Backup and Point-in-time Recovery (Pigsty) * **Continuously updated papers and experimental reports** This project is constantly evolving with new features. Don't forget to star ⭐ and watch 👀 to stay up to date.
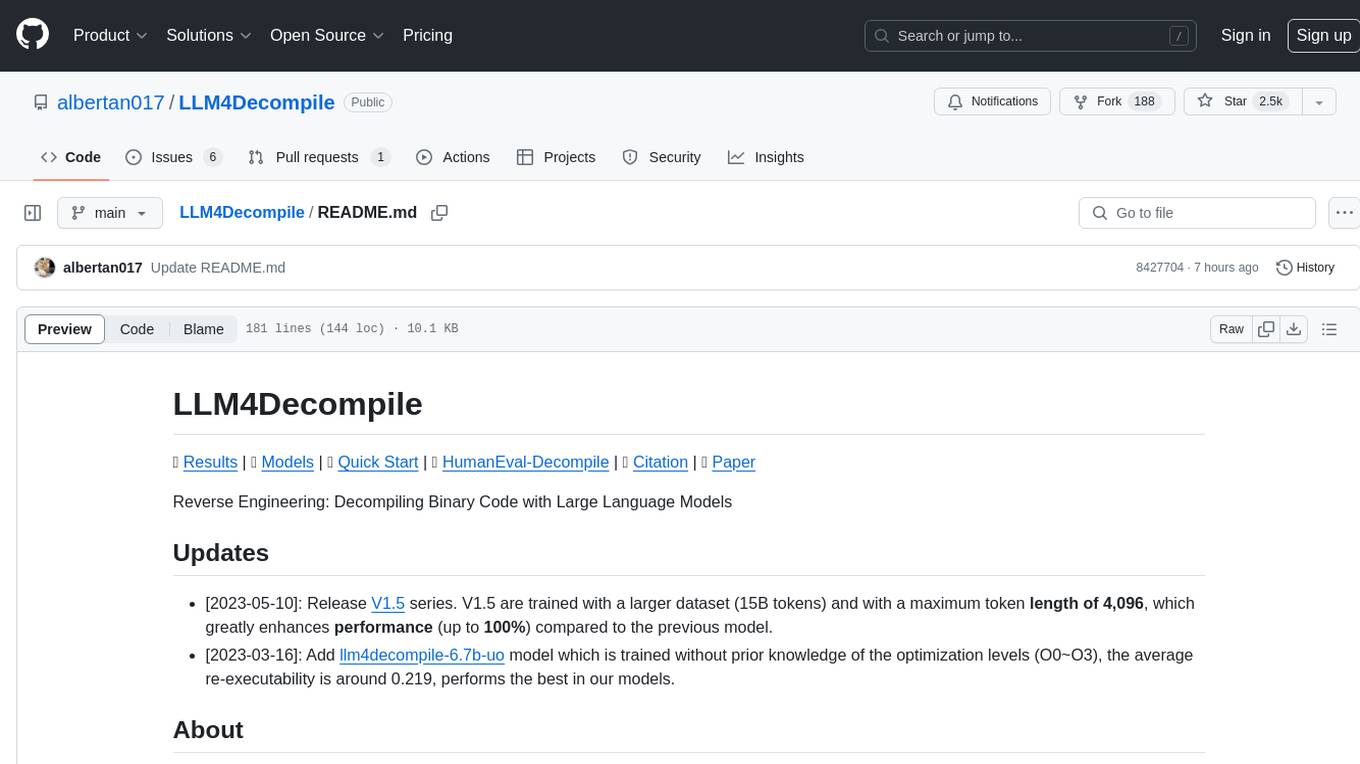
LLM4Decompile
LLM4Decompile is an open-source large language model dedicated to decompilation of Linux x86_64 binaries, supporting GCC's O0 to O3 optimization levels. It focuses on assessing re-executability of decompiled code through HumanEval-Decompile benchmark. The tool includes models with sizes ranging from 1.3 billion to 33 billion parameters, available on Hugging Face. Users can preprocess C code into binary and assembly instructions, then decompile assembly instructions into C using LLM4Decompile. Ongoing efforts aim to expand capabilities to support more architectures and configurations, integrate with decompilation tools like Ghidra and Rizin, and enhance performance with larger training datasets.
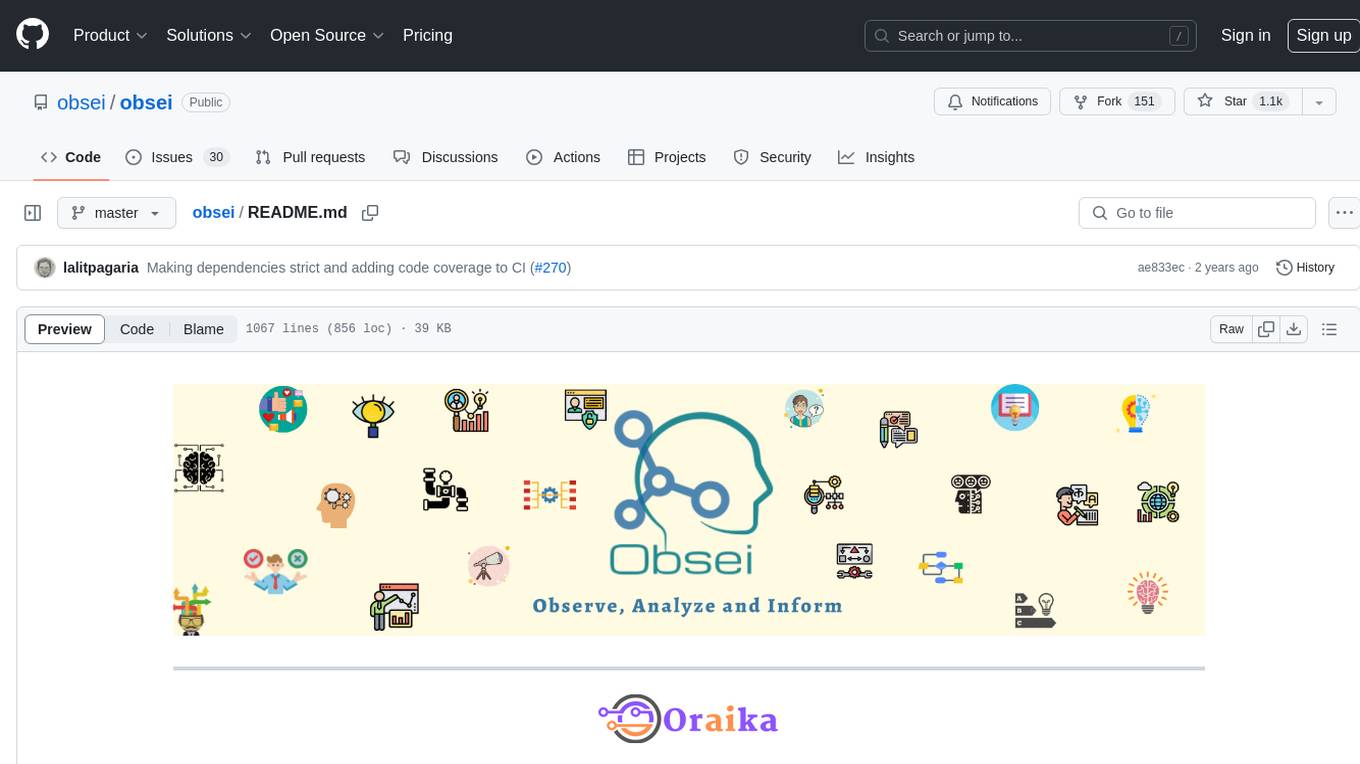
obsei
Obsei is an open-source, low-code, AI powered automation tool that consists of an Observer to collect unstructured data from various sources, an Analyzer to analyze the collected data with various AI tasks, and an Informer to send analyzed data to various destinations. The tool is suitable for scheduled jobs or serverless applications as all Observers can store their state in databases. Obsei is still in alpha stage, so caution is advised when using it in production. The tool can be used for social listening, alerting/notification, automatic customer issue creation, extraction of deeper insights from feedbacks, market research, dataset creation for various AI tasks, and more based on creativity.
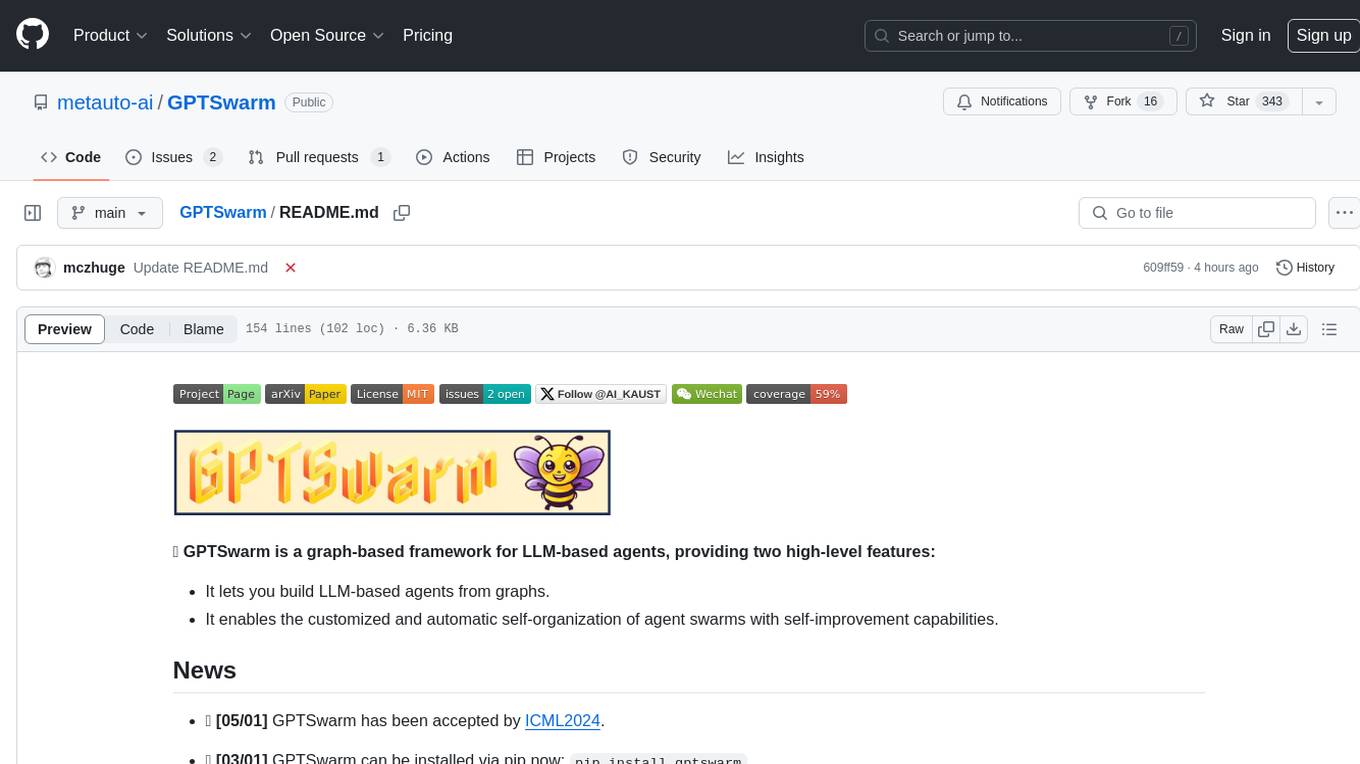
GPTSwarm
GPTSwarm is a graph-based framework for LLM-based agents that enables the creation of LLM-based agents from graphs and facilitates the customized and automatic self-organization of agent swarms with self-improvement capabilities. The library includes components for domain-specific operations, graph-related functions, LLM backend selection, memory management, and optimization algorithms to enhance agent performance and swarm efficiency. Users can quickly run predefined swarms or utilize tools like the file analyzer. GPTSwarm supports local LM inference via LM Studio, allowing users to run with a local LLM model. The framework has been accepted by ICML2024 and offers advanced features for experimentation and customization.
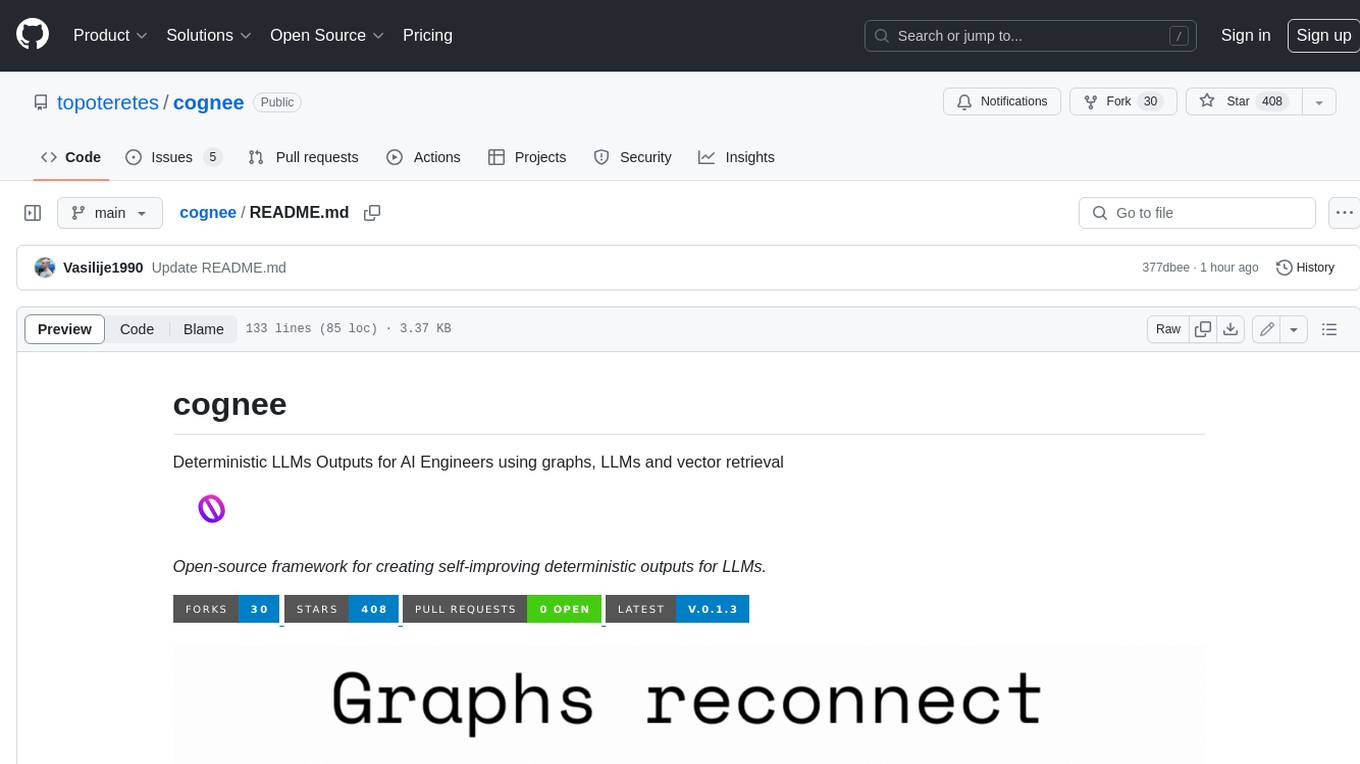
cognee
Cognee is an open-source framework designed for creating self-improving deterministic outputs for Large Language Models (LLMs) using graphs, LLMs, and vector retrieval. It provides a platform for AI engineers to enhance their models and generate more accurate results. Users can leverage Cognee to add new information, utilize LLMs for knowledge creation, and query the system for relevant knowledge. The tool supports various LLM providers and offers flexibility in adding different data types, such as text files or directories. Cognee aims to streamline the process of working with LLMs and improving AI models for better performance and efficiency.
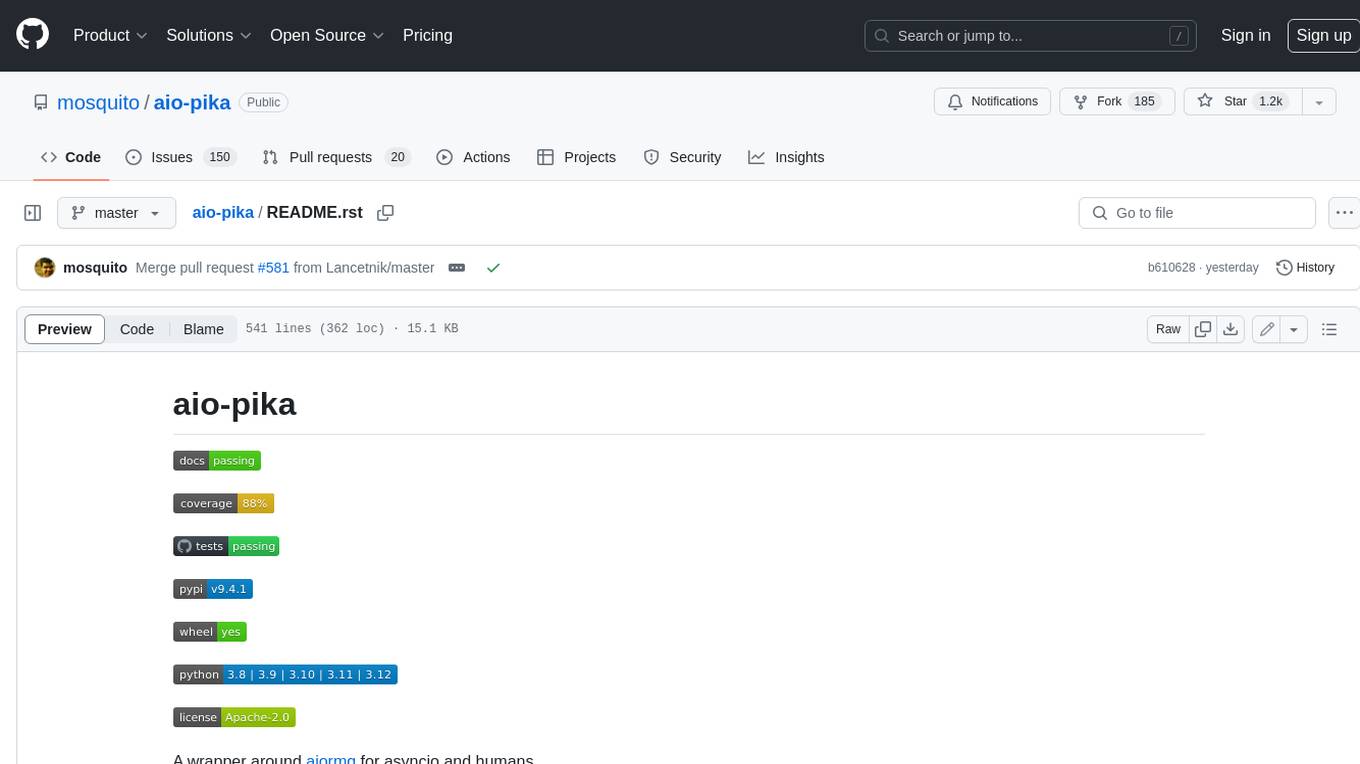
aio-pika
Aio-pika is a wrapper around aiormq for asyncio and humans. It provides a completely asynchronous API, object-oriented API, transparent auto-reconnects with complete state recovery, Python 3.7+ compatibility, transparent publisher confirms support, transactions support, and complete type-hints coverage.
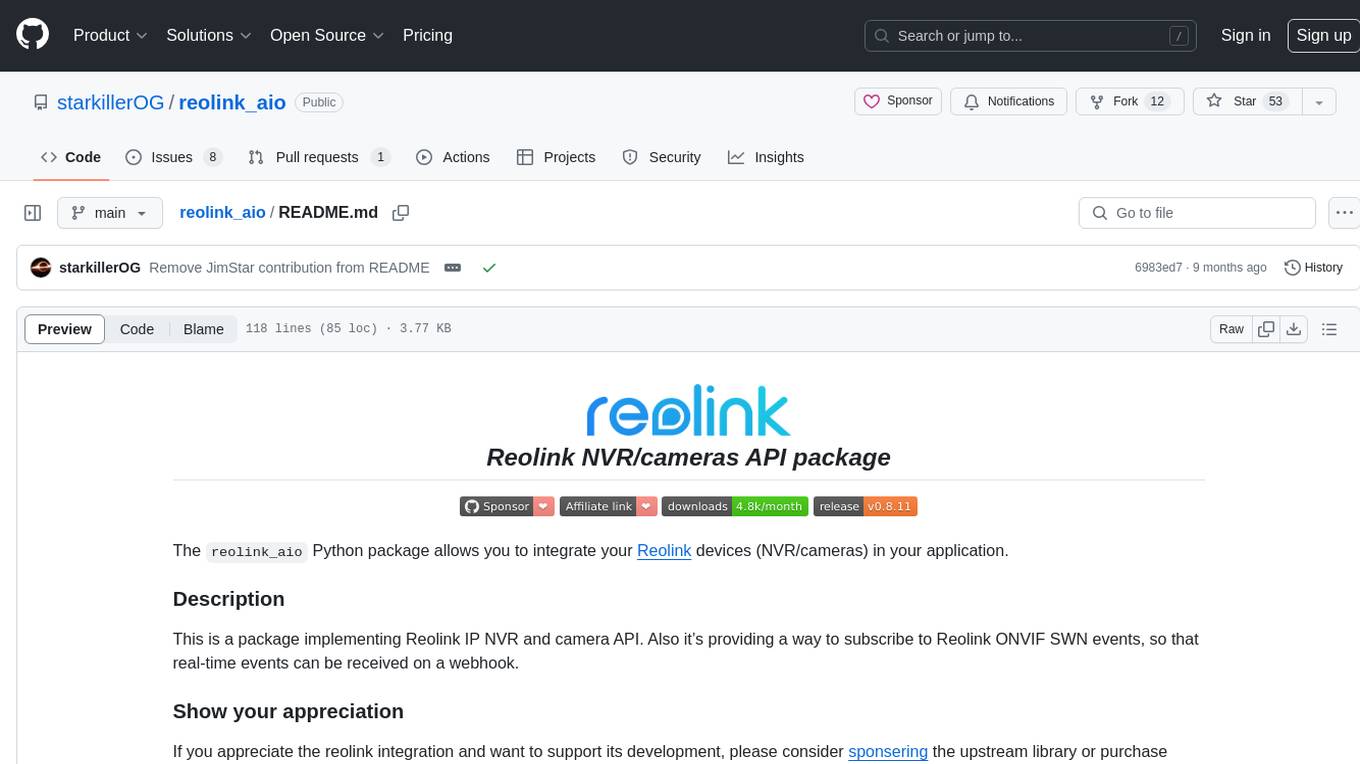
reolink_aio
The 'reolink_aio' Python package is designed to integrate Reolink devices (NVR/cameras) into your application. It implements Reolink IP NVR and camera API, allowing users to subscribe to Reolink ONVIF SWN events for real-time event notifications via webhook. The package provides functionalities to obtain and cache NVR or camera settings, capabilities, and states, as well as enable features like infrared lights, spotlight, and siren. Users can also subscribe to events, renew timers, and disconnect from the host device.
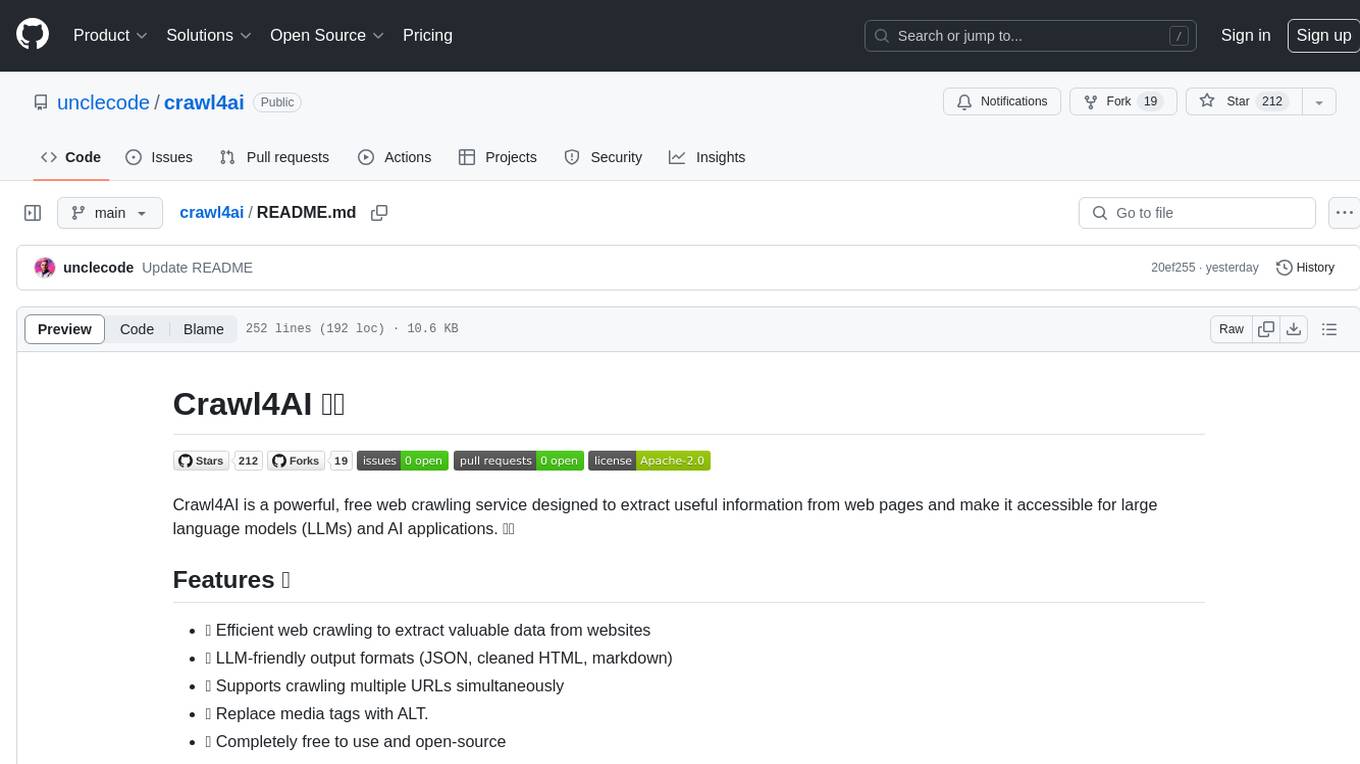
crawl4ai
Crawl4AI is a powerful and free web crawling service that extracts valuable data from websites and provides LLM-friendly output formats. It supports crawling multiple URLs simultaneously, replaces media tags with ALT, and is completely free to use and open-source. Users can integrate Crawl4AI into Python projects as a library or run it as a standalone local server. The tool allows users to crawl and extract data from specified URLs using different providers and models, with options to include raw HTML content, force fresh crawls, and extract meaningful text blocks. Configuration settings can be adjusted in the `crawler/config.py` file to customize providers, API keys, chunk processing, and word thresholds. Contributions to Crawl4AI are welcome from the open-source community to enhance its value for AI enthusiasts and developers.
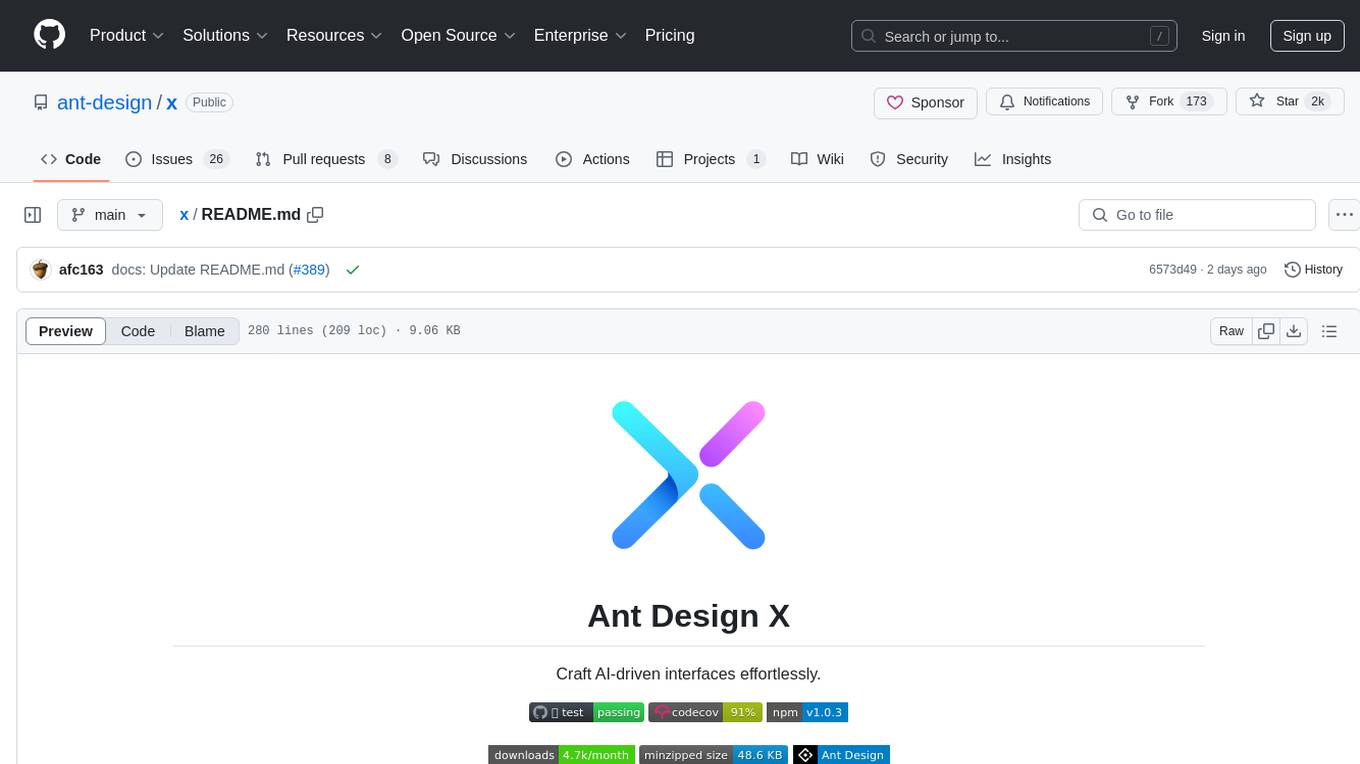
x
Ant Design X is a tool for crafting AI-driven interfaces effortlessly. It is built on the best practices of enterprise-level AI products, offering flexible and diverse atomic components for various AI dialogue scenarios. The tool provides out-of-the-box model integration with inference services compatible with OpenAI standards. It also enables efficient management of conversation data flows, supports rich template options, complete TypeScript support, and advanced theme customization. Ant Design X is designed to enhance development efficiency and deliver exceptional AI interaction experiences.
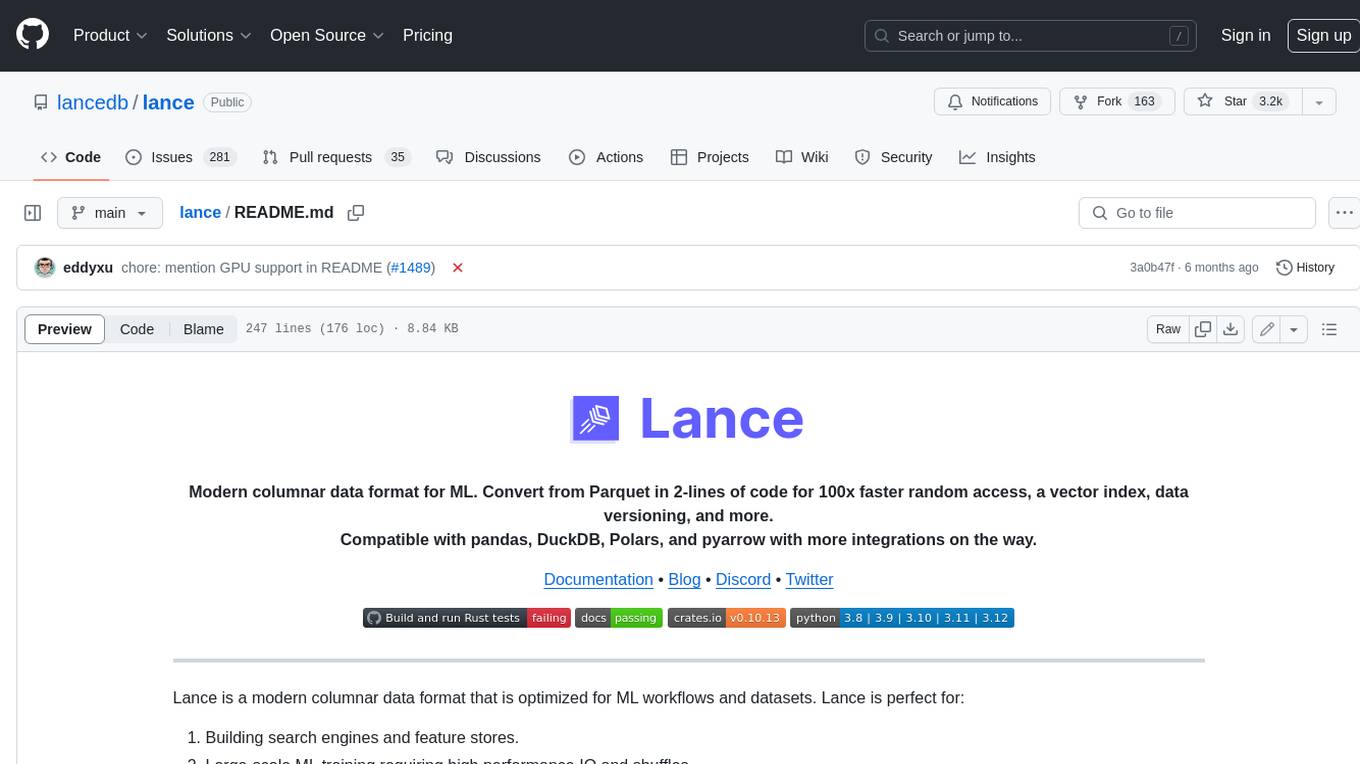
lance
Lance is a modern columnar data format optimized for ML workflows and datasets. It offers high-performance random access, vector search, zero-copy automatic versioning, and ecosystem integrations with Apache Arrow, Pandas, Polars, and DuckDB. Lance is designed to address the challenges of the ML development cycle, providing a unified data format for collection, exploration, analytics, feature engineering, training, evaluation, deployment, and monitoring. It aims to reduce data silos and streamline the ML development process.
For similar tasks
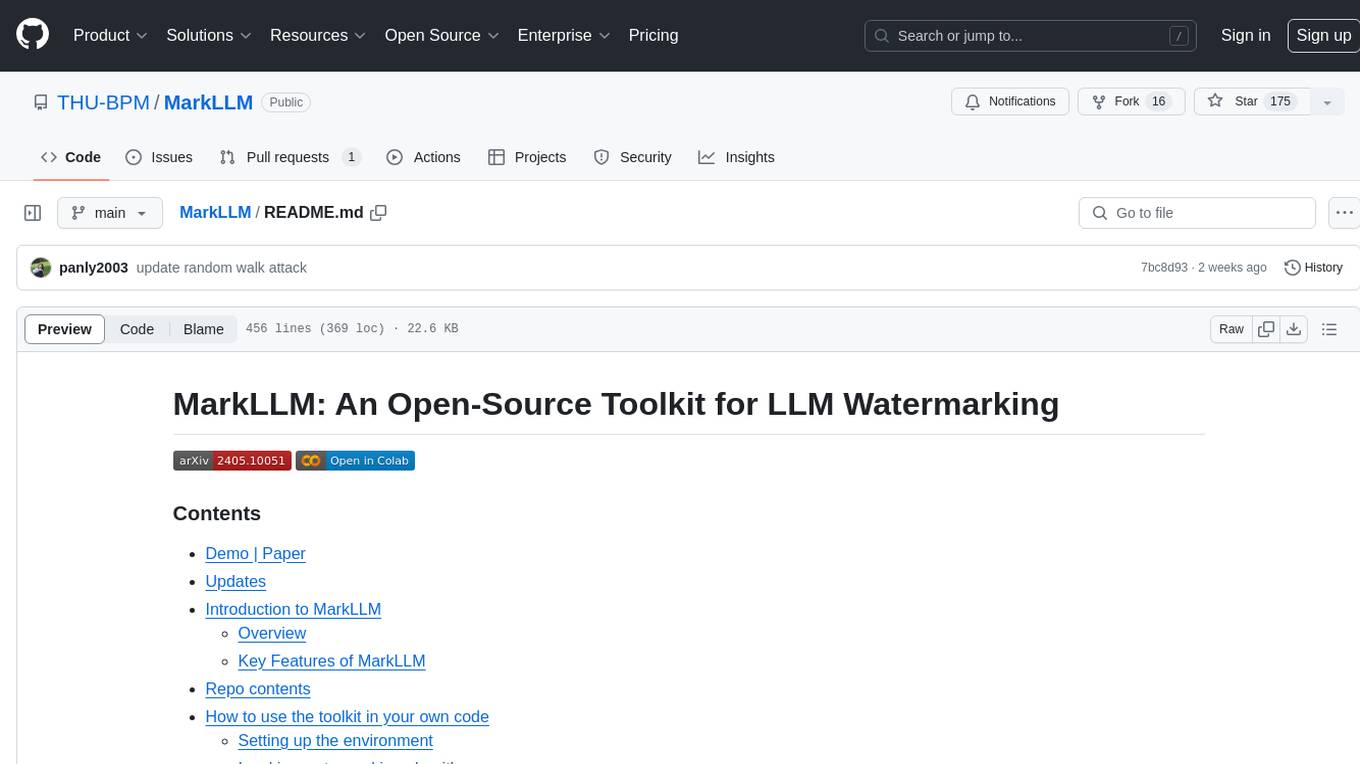
MarkLLM
MarkLLM is an open-source toolkit designed for watermarking technologies within large language models (LLMs). It simplifies access, understanding, and assessment of watermarking technologies, supporting various algorithms, visualization tools, and evaluation modules. The toolkit aids researchers and the community in ensuring the authenticity and origin of machine-generated text.
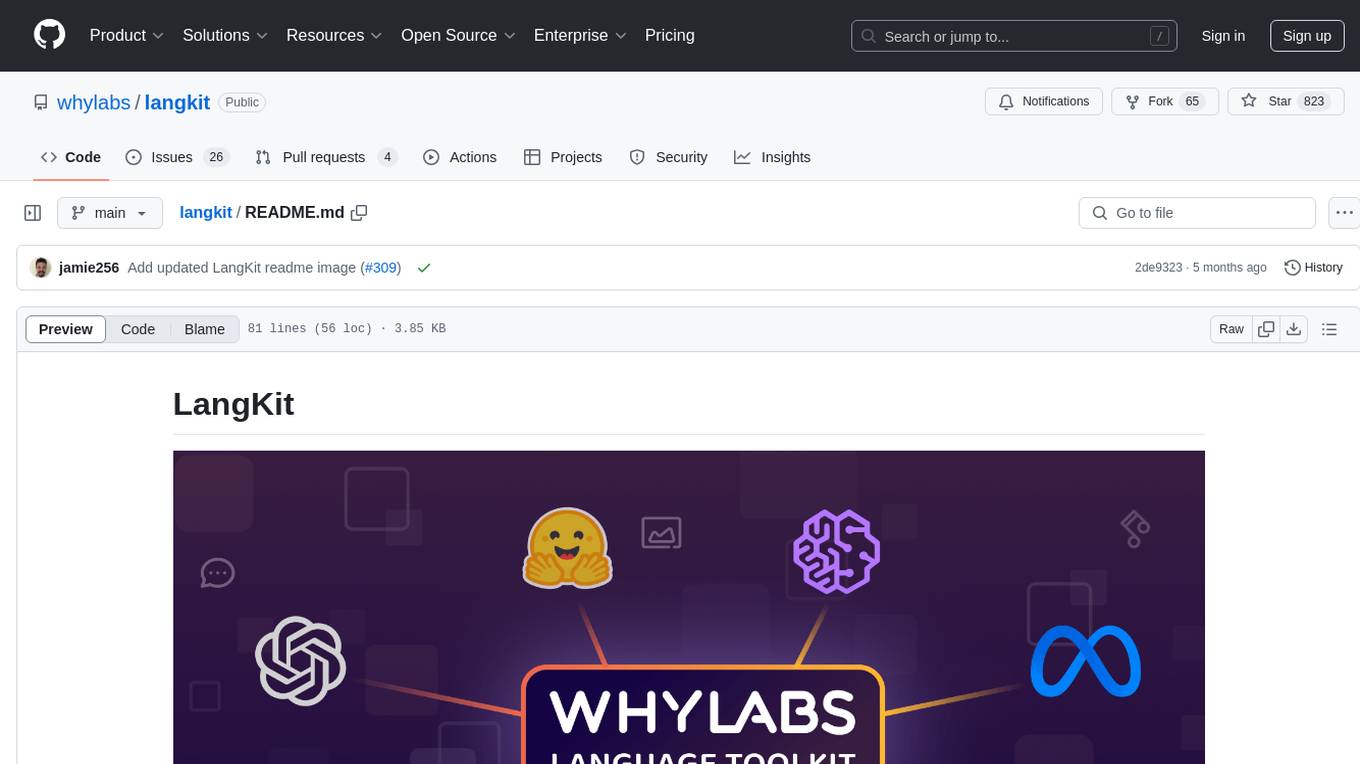
langkit
LangKit is an open-source text metrics toolkit for monitoring language models. It offers methods for extracting signals from input/output text, compatible with whylogs. Features include text quality, relevance, security, sentiment, toxicity analysis. Installation via PyPI. Modules contain UDFs for whylogs. Benchmarks show throughput on AWS instances. FAQs available.
For similar jobs
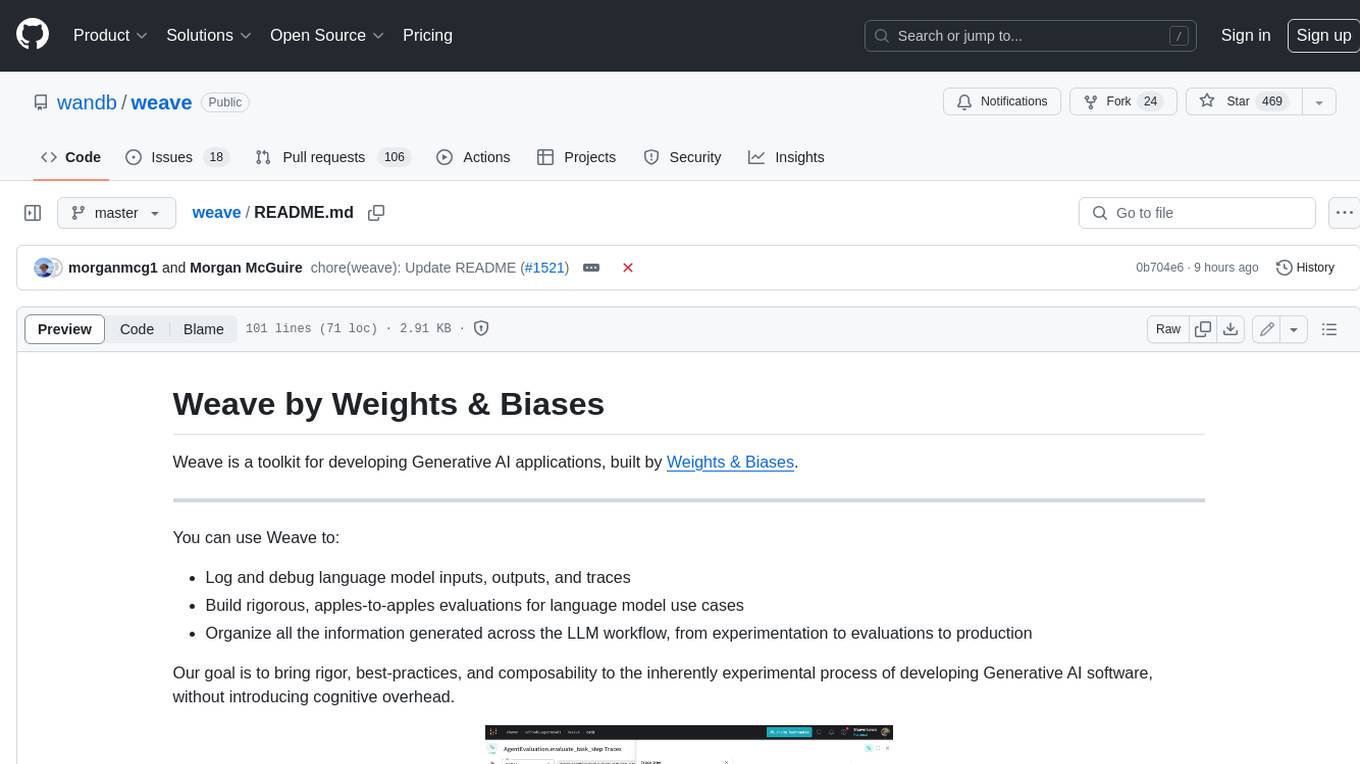
weave
Weave is a toolkit for developing Generative AI applications, built by Weights & Biases. With Weave, you can log and debug language model inputs, outputs, and traces; build rigorous, apples-to-apples evaluations for language model use cases; and organize all the information generated across the LLM workflow, from experimentation to evaluations to production. Weave aims to bring rigor, best-practices, and composability to the inherently experimental process of developing Generative AI software, without introducing cognitive overhead.
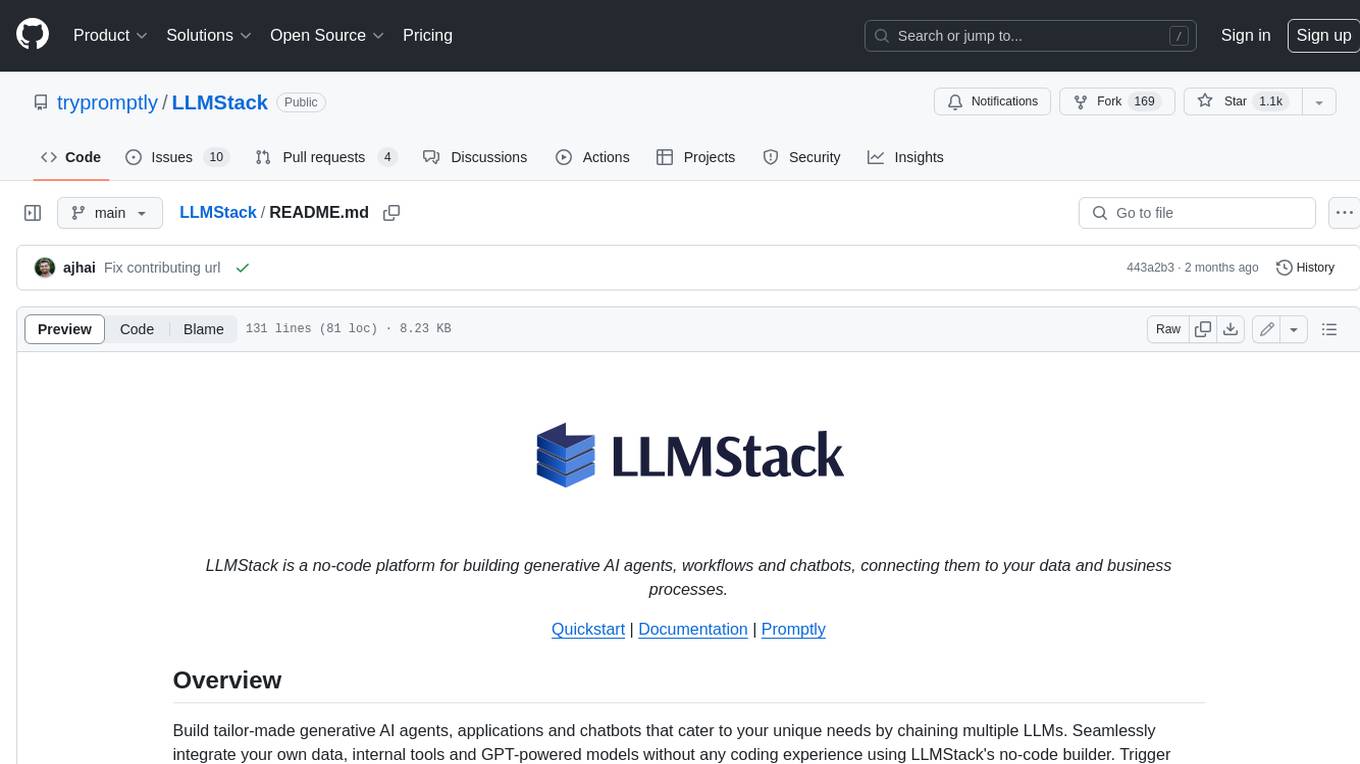
LLMStack
LLMStack is a no-code platform for building generative AI agents, workflows, and chatbots. It allows users to connect their own data, internal tools, and GPT-powered models without any coding experience. LLMStack can be deployed to the cloud or on-premise and can be accessed via HTTP API or triggered from Slack or Discord.
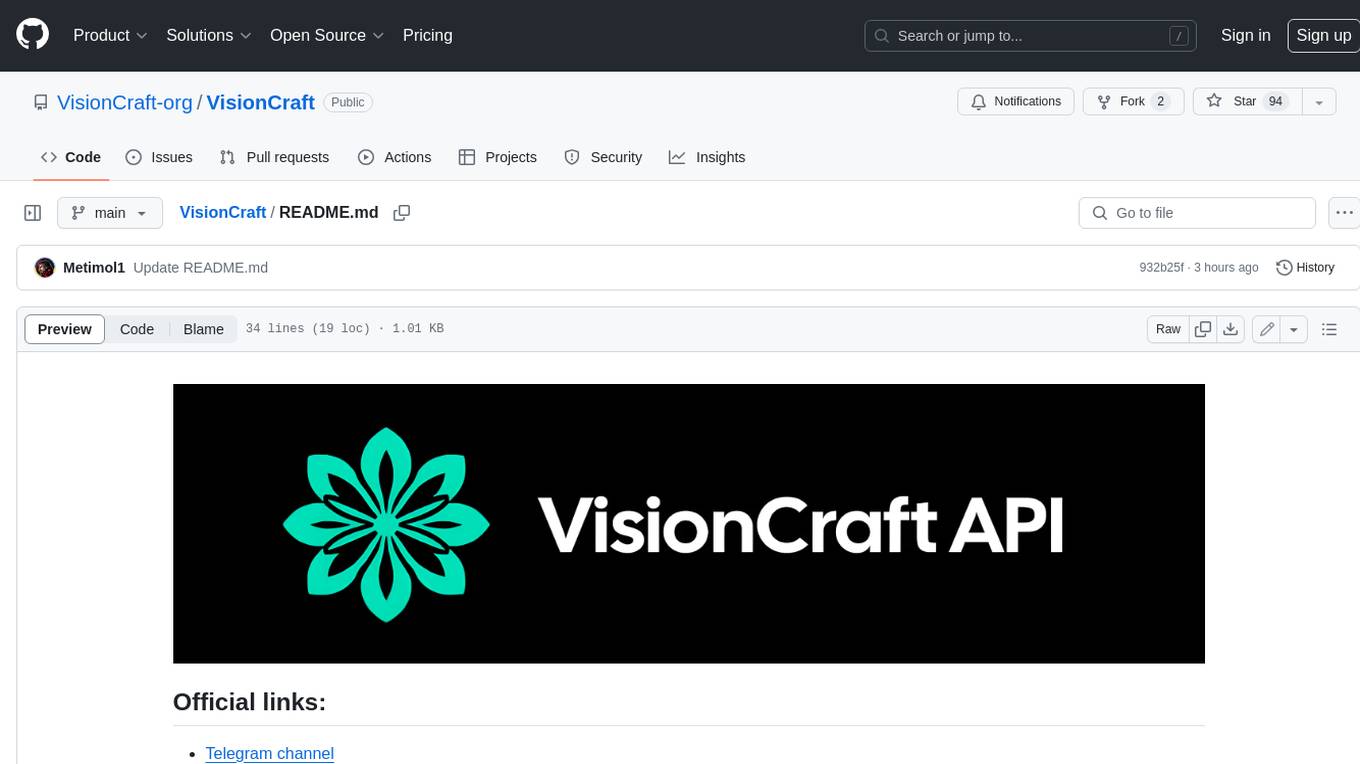
VisionCraft
The VisionCraft API is a free API for using over 100 different AI models. From images to sound.
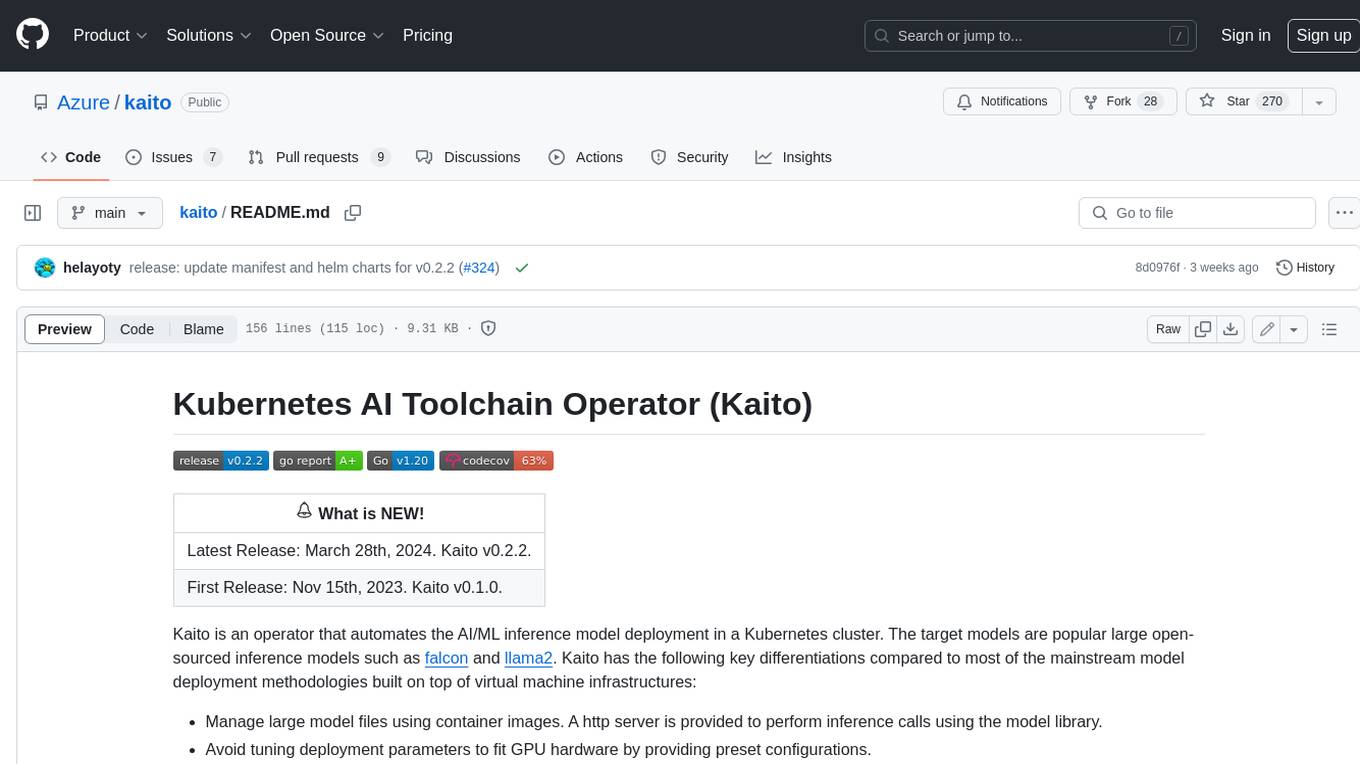
kaito
Kaito is an operator that automates the AI/ML inference model deployment in a Kubernetes cluster. It manages large model files using container images, avoids tuning deployment parameters to fit GPU hardware by providing preset configurations, auto-provisions GPU nodes based on model requirements, and hosts large model images in the public Microsoft Container Registry (MCR) if the license allows. Using Kaito, the workflow of onboarding large AI inference models in Kubernetes is largely simplified.
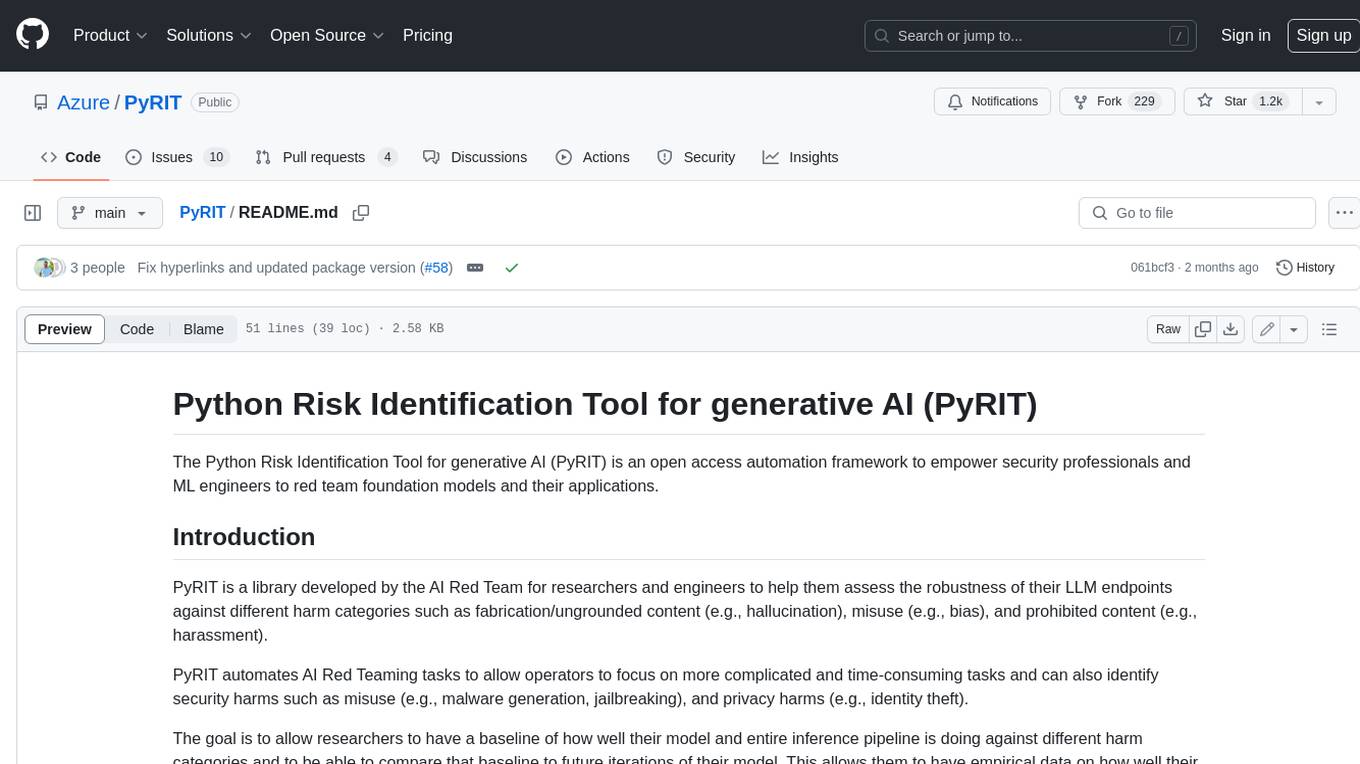
PyRIT
PyRIT is an open access automation framework designed to empower security professionals and ML engineers to red team foundation models and their applications. It automates AI Red Teaming tasks to allow operators to focus on more complicated and time-consuming tasks and can also identify security harms such as misuse (e.g., malware generation, jailbreaking), and privacy harms (e.g., identity theft). The goal is to allow researchers to have a baseline of how well their model and entire inference pipeline is doing against different harm categories and to be able to compare that baseline to future iterations of their model. This allows them to have empirical data on how well their model is doing today, and detect any degradation of performance based on future improvements.
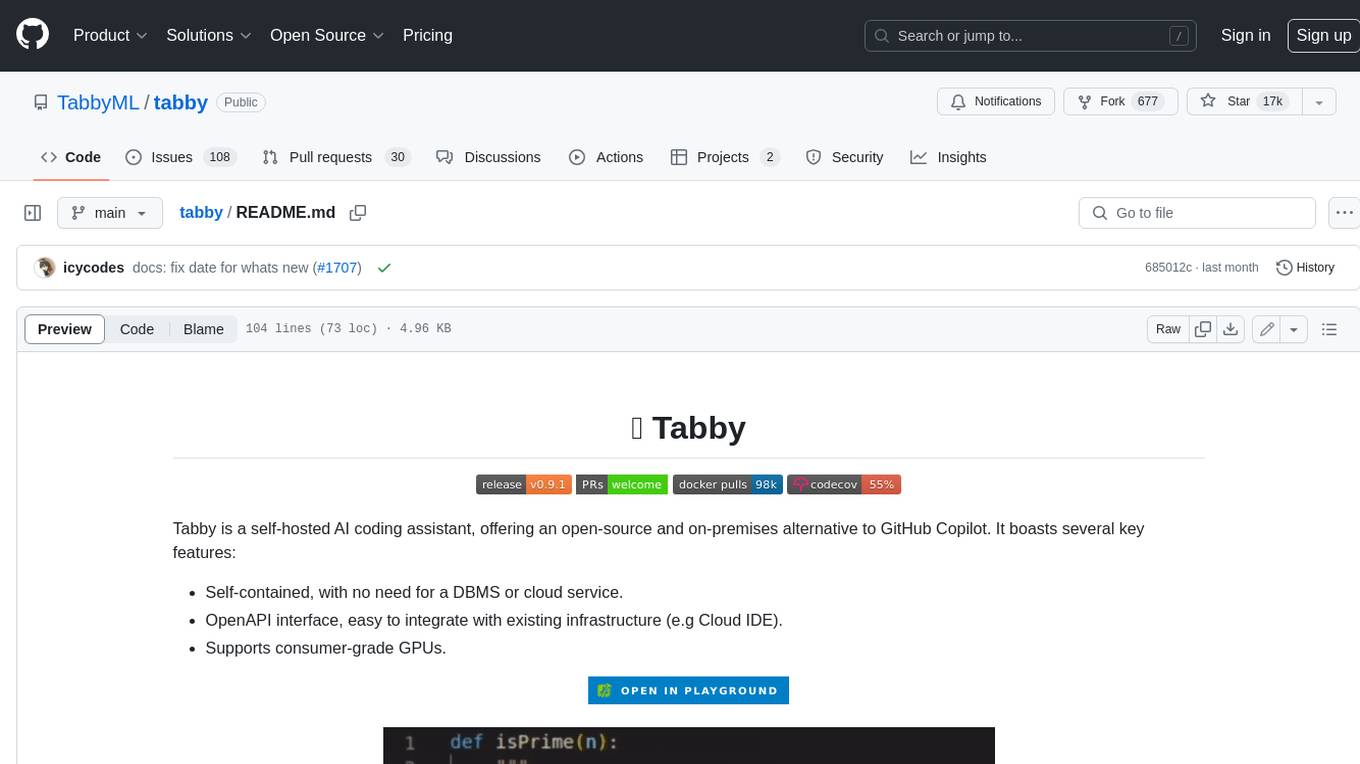
tabby
Tabby is a self-hosted AI coding assistant, offering an open-source and on-premises alternative to GitHub Copilot. It boasts several key features: * Self-contained, with no need for a DBMS or cloud service. * OpenAPI interface, easy to integrate with existing infrastructure (e.g Cloud IDE). * Supports consumer-grade GPUs.
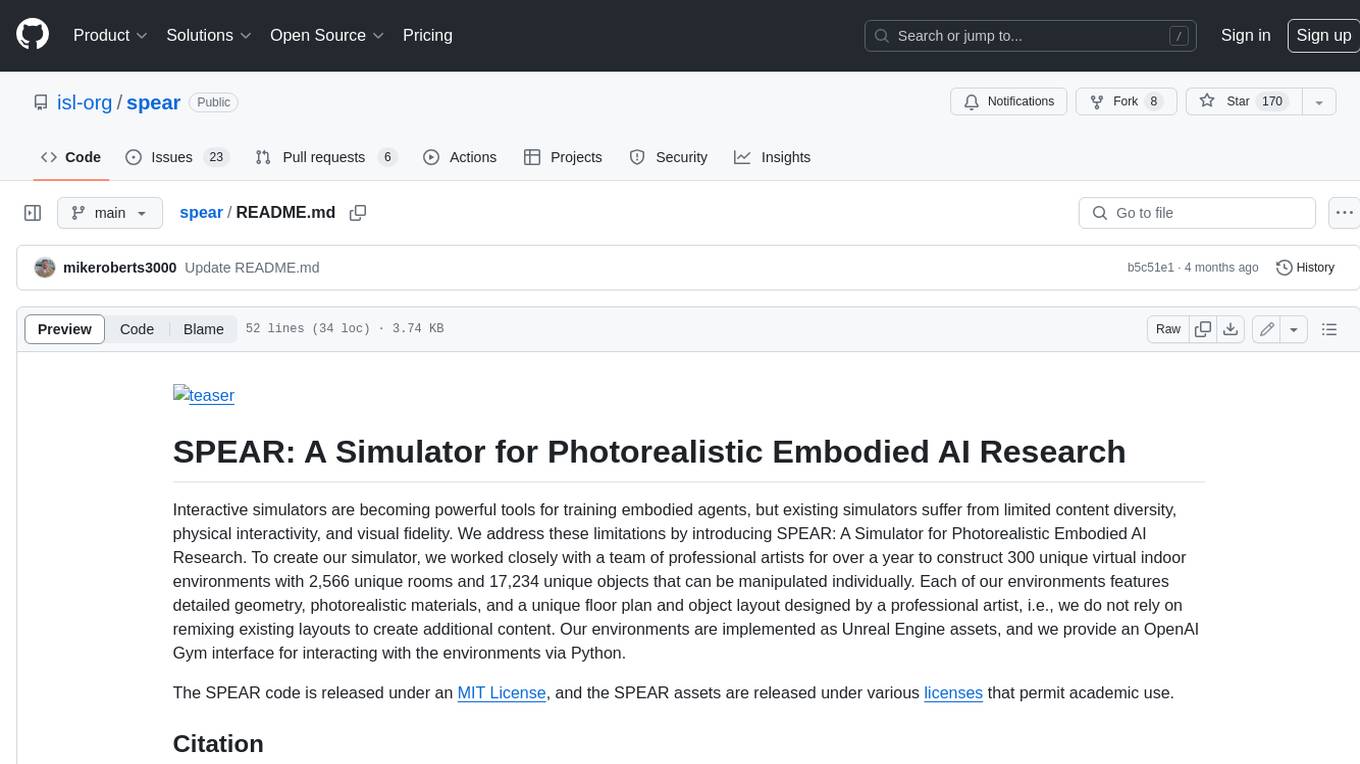
spear
SPEAR (Simulator for Photorealistic Embodied AI Research) is a powerful tool for training embodied agents. It features 300 unique virtual indoor environments with 2,566 unique rooms and 17,234 unique objects that can be manipulated individually. Each environment is designed by a professional artist and features detailed geometry, photorealistic materials, and a unique floor plan and object layout. SPEAR is implemented as Unreal Engine assets and provides an OpenAI Gym interface for interacting with the environments via Python.
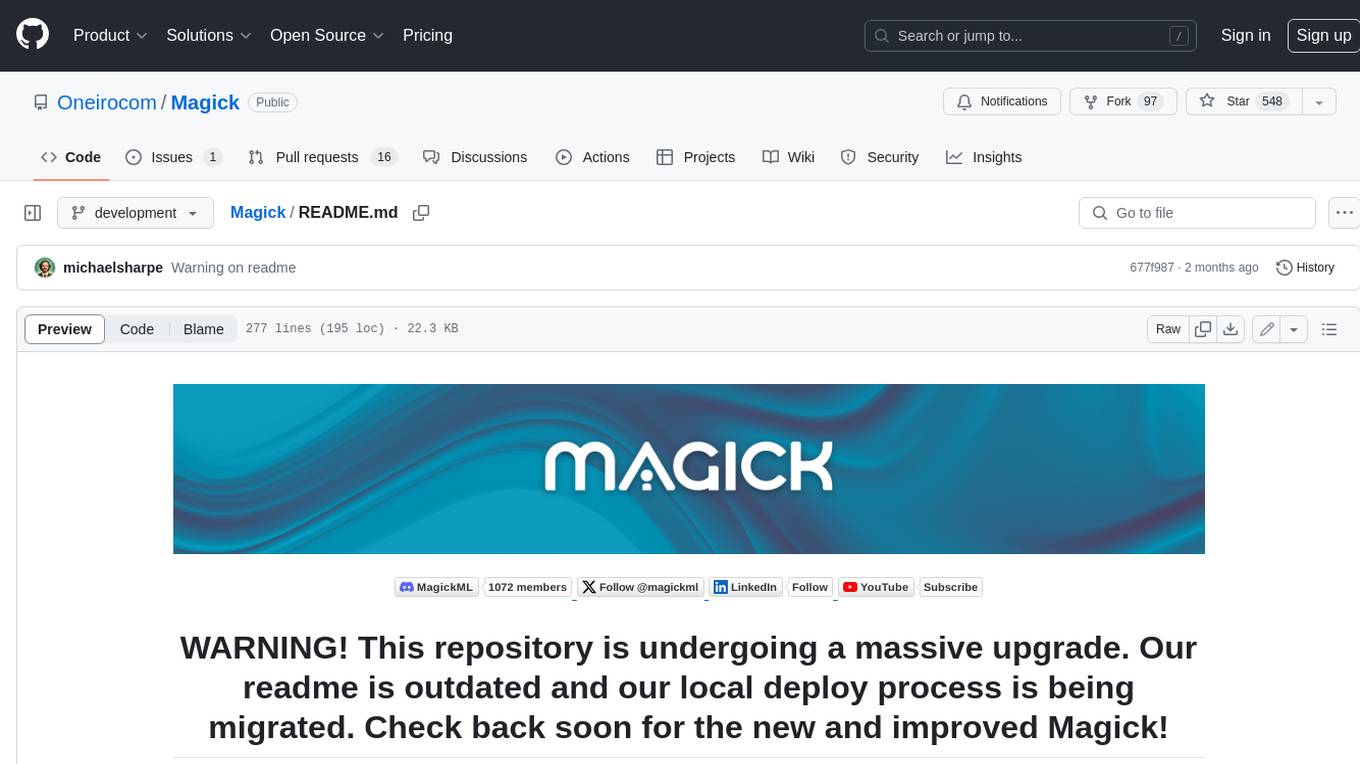
Magick
Magick is a groundbreaking visual AIDE (Artificial Intelligence Development Environment) for no-code data pipelines and multimodal agents. Magick can connect to other services and comes with nodes and templates well-suited for intelligent agents, chatbots, complex reasoning systems and realistic characters.