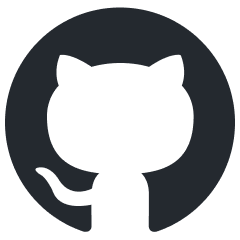
mcp-agent
Build effective agents using Model Context Protocol and simple workflow patterns
Stars: 2900
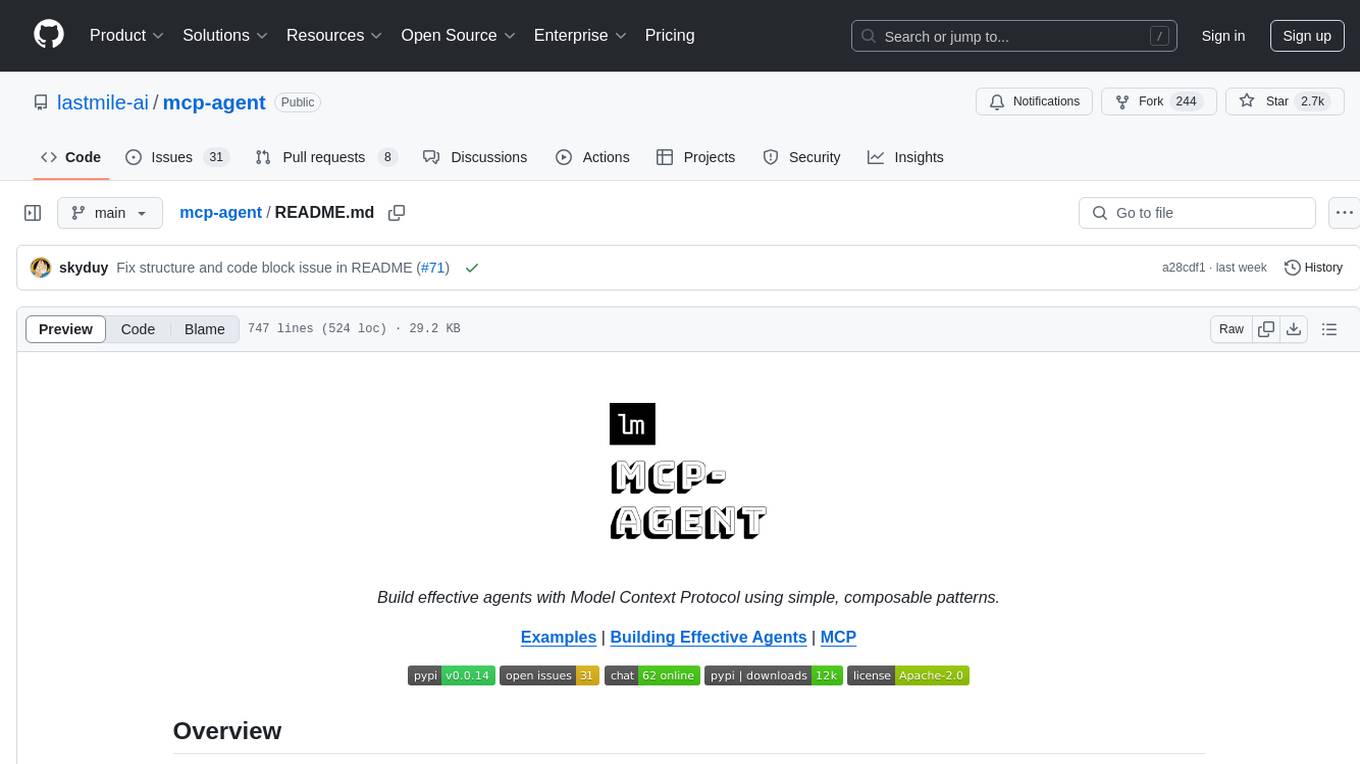
README:
Build effective agents with Model Context Protocol using simple, composable patterns.
Examples | Building Effective Agents | MCP
mcp-agent
is a simple, composable framework to build agents using Model Context Protocol.
Inspiration: Anthropic announced 2 foundational updates for AI application developers:
- Model Context Protocol - a standardized interface to let any software be accessible to AI assistants via MCP servers.
- Building Effective Agents - a seminal writeup on simple, composable patterns for building production-ready AI agents.
mcp-agent
puts these two foundational pieces into an AI application framework:
- It handles the pesky business of managing the lifecycle of MCP server connections so you don't have to.
- It implements every pattern described in Building Effective Agents, and does so in a composable way, allowing you to chain these patterns together.
- Bonus: It implements OpenAI's Swarm pattern for multi-agent orchestration, but in a model-agnostic way.
Altogether, this is the simplest and easiest way to build robust agent applications. Much like MCP, this project is in early development. We welcome all kinds of contributions, feedback and your help in growing this to become a new standard.
We recommend using uv to manage your Python projects:
uv add "mcp-agent"
Alternatively:
pip install mcp-agent
[!TIP] The
examples
directory has several example applications to get started with. To run an example, clone this repo, then:cd examples/mcp_basic_agent # Or any other example cp mcp_agent.secrets.yaml.example mcp_agent.secrets.yaml # Update API keys uv run main.py
Here is a basic "finder" agent that uses the fetch and filesystem servers to look up a file, read a blog and write a tweet. Example link:
finder_agent.py
import asyncio
import os
from mcp_agent.app import MCPApp
from mcp_agent.agents.agent import Agent
from mcp_agent.workflows.llm.augmented_llm_openai import OpenAIAugmentedLLM
app = MCPApp(name="hello_world_agent")
async def example_usage():
async with app.run() as mcp_agent_app:
logger = mcp_agent_app.logger
# This agent can read the filesystem or fetch URLs
finder_agent = Agent(
name="finder",
instruction="""You can read local files or fetch URLs.
Return the requested information when asked.""",
server_names=["fetch", "filesystem"], # MCP servers this Agent can use
)
async with finder_agent:
# Automatically initializes the MCP servers and adds their tools for LLM use
tools = await finder_agent.list_tools()
logger.info(f"Tools available:", data=tools)
# Attach an OpenAI LLM to the agent (defaults to GPT-4o)
llm = await finder_agent.attach_llm(OpenAIAugmentedLLM)
# This will perform a file lookup and read using the filesystem server
result = await llm.generate_str(
message="Show me what's in README.md verbatim"
)
logger.info(f"README.md contents: {result}")
# Uses the fetch server to fetch the content from URL
result = await llm.generate_str(
message="Print the first two paragraphs from https://www.anthropic.com/research/building-effective-agents"
)
logger.info(f"Blog intro: {result}")
# Multi-turn interactions by default
result = await llm.generate_str("Summarize that in a 128-char tweet")
logger.info(f"Tweet: {result}")
if __name__ == "__main__":
asyncio.run(example_usage())
mcp_agent.config.yaml
execution_engine: asyncio
logger:
transports: [console] # You can use [file, console] for both
level: debug
path: "logs/mcp-agent.jsonl" # Used for file transport
# For dynamic log filenames:
# path_settings:
# path_pattern: "logs/mcp-agent-{unique_id}.jsonl"
# unique_id: "timestamp" # Or "session_id"
# timestamp_format: "%Y%m%d_%H%M%S"
mcp:
servers:
fetch:
command: "uvx"
args: ["mcp-server-fetch"]
filesystem:
command: "npx"
args:
[
"-y",
"@modelcontextprotocol/server-filesystem",
"<add_your_directories>",
]
openai:
# Secrets (API keys, etc.) are stored in an mcp_agent.secrets.yaml file which can be gitignored
default_model: gpt-4o
- Why use mcp-agent?
- Example Applications
- Core Concepts
- Workflows Patterns
- Advanced
- Contributing
- Roadmap
- FAQs
There are too many AI frameworks out there already. But mcp-agent
is the only one that is purpose-built for a shared protocol - MCP. It is also the most lightweight, and is closer to an agent pattern library than a framework.
As more services become MCP-aware, you can use mcp-agent to build robust and controllable AI agents that can leverage those services out-of-the-box.
Before we go into the core concepts of mcp-agent, let's show what you can build with it.
In short, you can build any kind of AI application with mcp-agent: multi-agent collaborative workflows, human-in-the-loop workflows, RAG pipelines and more.
You can integrate mcp-agent apps into MCP clients like Claude Desktop.
This app wraps an mcp-agent application inside an MCP server, and exposes that server to Claude Desktop. The app exposes agents and workflows that Claude Desktop can invoke to service of the user's request.
https://github.com/user-attachments/assets/7807cffd-dba7-4f0c-9c70-9482fd7e0699
This demo shows a multi-agent evaluation task where each agent evaluates aspects of an input poem, and then an aggregator summarizes their findings into a final response.
Details: Starting from a user's request over text, the application:
- dynamically defines agents to do the job
- uses the appropriate workflow to orchestrate those agents (in this case the Parallel workflow)
Link to code: examples/mcp_agent_server
[!NOTE] Huge thanks to Jerron Lim (@StreetLamb) for developing and contributing this example!
You can deploy mcp-agent apps using Streamlit.
This app is able to perform read and write actions on gmail using text prompts -- i.e. read, delete, send emails, mark as read/unread, etc. It uses an MCP server for Gmail.
https://github.com/user-attachments/assets/54899cac-de24-4102-bd7e-4b2022c956e3
Link to code: gmail-mcp-server
[!NOTE] Huge thanks to Jason Summer (@jasonsum) for developing and contributing this example!
This app uses a Qdrant vector database (via an MCP server) to do Q&A over a corpus of text.
https://github.com/user-attachments/assets/f4dcd227-cae9-4a59-aa9e-0eceeb4acaf4
Link to code: examples/streamlit_mcp_rag_agent
[!NOTE] Huge thanks to Jerron Lim (@StreetLamb) for developing and contributing this example!
Marimo is a reactive Python notebook that replaces Jupyter and Streamlit. Here's the "file finder" agent from Quickstart implemented in Marimo:
Link to code: examples/marimo_mcp_basic_agent
[!NOTE] Huge thanks to Akshay Agrawal (@akshayka) for developing and contributing this example!
You can write mcp-agent apps as Python scripts or Jupyter notebooks.
This example demonstrates a multi-agent setup for handling different customer service requests in an airline context using the Swarm workflow pattern. The agents can triage requests, handle flight modifications, cancellations, and lost baggage cases.
https://github.com/user-attachments/assets/b314d75d-7945-4de6-965b-7f21eb14a8bd
Link to code: examples/workflow_swarm
The following are the building blocks of the mcp-agent framework:
- MCPApp: global state and app configuration
-
MCP server management:
gen_client
andMCPConnectionManager
to easily connect to MCP servers. - Agent: An Agent is an entity that has access to a set of MCP servers and exposes them to an LLM as tool calls. It has a name and purpose (instruction).
-
AugmentedLLM: An LLM that is enhanced with tools provided from a collection of MCP servers. Every Workflow pattern described below is an
AugmentedLLM
itself, allowing you to compose and chain them together.
Everything in the framework is a derivative of these core capabilities.
mcp-agent provides implementations for every pattern in Anthropic’s Building Effective Agents, as well as the OpenAI Swarm pattern.
Each pattern is model-agnostic, and exposed as an AugmentedLLM
, making everything very composable.
AugmentedLLM is an LLM that has access to MCP servers and functions via Agents.
LLM providers implement the AugmentedLLM interface to expose 3 functions:
-
generate
: Generate message(s) given a prompt, possibly over multiple iterations and making tool calls as needed. -
generate_str
: Callsgenerate
and returns result as a string output. -
generate_structured
: Uses Instructor to return the generated result as a Pydantic model.
Additionally, AugmentedLLM
has memory, to keep track of long or short-term history.
Example
from mcp_agent.agents.agent import Agent
from mcp_agent.workflows.llm.augmented_llm_anthropic import AnthropicAugmentedLLM
finder_agent = Agent(
name="finder",
instruction="You are an agent with filesystem + fetch access. Return the requested file or URL contents.",
server_names=["fetch", "filesystem"],
)
async with finder_agent:
llm = await finder_agent.attach_llm(AnthropicAugmentedLLM)
result = await llm.generate_str(
message="Print the first 2 paragraphs of https://www.anthropic.com/research/building-effective-agents",
# Can override model, tokens and other defaults
)
logger.info(f"Result: {result}")
# Multi-turn conversation
result = await llm.generate_str(
message="Summarize those paragraphs in a 128 character tweet",
)
logger.info(f"Result: {result}")
Fan-out tasks to multiple sub-agents and fan-in the results. Each subtask is an AugmentedLLM, as is the overall Parallel workflow, meaning each subtask can optionally be a more complex workflow itself.
[!NOTE]
Example
proofreader = Agent(name="proofreader", instruction="Review grammar...")
fact_checker = Agent(name="fact_checker", instruction="Check factual consistency...")
style_enforcer = Agent(name="style_enforcer", instruction="Enforce style guidelines...")
grader = Agent(name="grader", instruction="Combine feedback into a structured report.")
parallel = ParallelLLM(
fan_in_agent=grader,
fan_out_agents=[proofreader, fact_checker, style_enforcer],
llm_factory=OpenAIAugmentedLLM,
)
result = await parallel.generate_str("Student short story submission: ...", RequestParams(model="gpt4-o"))
Given an input, route to the top_k
most relevant categories. A category can be an Agent, an MCP server or a regular function.
mcp-agent provides several router implementations, including:
-
EmbeddingRouter
: uses embedding models for classification -
LLMRouter
: uses LLMs for classification
[!NOTE]
Example
def print_hello_world:
print("Hello, world!")
finder_agent = Agent(name="finder", server_names=["fetch", "filesystem"])
writer_agent = Agent(name="writer", server_names=["filesystem"])
llm = OpenAIAugmentedLLM()
router = LLMRouter(
llm=llm,
agents=[finder_agent, writer_agent],
functions=[print_hello_world],
)
results = await router.route( # Also available: route_to_agent, route_to_server
request="Find and print the contents of README.md verbatim",
top_k=1
)
chosen_agent = results[0].result
async with chosen_agent:
...
A close sibling of Router, the Intent Classifier pattern identifies the top_k
Intents that most closely match a given input.
Just like a Router, mcp-agent provides both an embedding and LLM-based intent classifier.
One LLM (the “optimizer”) refines a response, another (the “evaluator”) critiques it until a response exceeds a quality criteria.
[!NOTE]
Example
optimizer = Agent(name="cover_letter_writer", server_names=["fetch"], instruction="Generate a cover letter ...")
evaluator = Agent(name="critiquer", instruction="Evaluate clarity, specificity, relevance...")
llm = EvaluatorOptimizerLLM(
optimizer=optimizer,
evaluator=evaluator,
llm_factory=OpenAIAugmentedLLM,
min_rating=QualityRating.EXCELLENT, # Keep iterating until the minimum quality bar is reached
)
result = await eo_llm.generate_str("Write a job cover letter for an AI framework developer role at LastMile AI.")
print("Final refined cover letter:", result)
A higher-level LLM generates a plan, then assigns them to sub-agents, and synthesizes the results. The Orchestrator workflow automatically parallelizes steps that can be done in parallel, and blocks on dependencies.
[!NOTE]
Example
finder_agent = Agent(name="finder", server_names=["fetch", "filesystem"])
writer_agent = Agent(name="writer", server_names=["filesystem"])
proofreader = Agent(name="proofreader", ...)
fact_checker = Agent(name="fact_checker", ...)
style_enforcer = Agent(name="style_enforcer", instructions="Use APA style guide from ...", server_names=["fetch"])
orchestrator = Orchestrator(
llm_factory=AnthropicAugmentedLLM,
available_agents=[finder_agent, writer_agent, proofreader, fact_checker, style_enforcer],
)
task = "Load short_story.md, evaluate it, produce a graded_report.md with multiple feedback aspects."
result = await orchestrator.generate_str(task, RequestParams(model="gpt-4o"))
print(result)
OpenAI has an experimental multi-agent pattern called Swarm, which we provide a model-agnostic reference implementation for in mcp-agent.
The mcp-agent Swarm pattern works seamlessly with MCP servers, and is exposed as an AugmentedLLM
, allowing for composability with other patterns above.
[!NOTE]
Example
triage_agent = SwarmAgent(...)
flight_mod_agent = SwarmAgent(...)
lost_baggage_agent = SwarmAgent(...)
# The triage agent decides whether to route to flight_mod_agent or lost_baggage_agent
swarm = AnthropicSwarm(agent=triage_agent, context_variables={...})
test_input = "My bag was not delivered!"
result = await swarm.generate_str(test_input)
print("Result:", result)
An example of composability is using an Evaluator-Optimizer workflow as the planner LLM inside the Orchestrator workflow. Generating a high-quality plan to execute is important for robust behavior, and an evaluator-optimizer can help ensure that.
Doing so is seamless in mcp-agent, because each workflow is implemented as an AugmentedLLM
.
Example
optimizer = Agent(name="plan_optimizer", server_names=[...], instruction="Generate a plan given an objective ...")
evaluator = Agent(name="plan_evaluator", instruction="Evaluate logic, ordering and precision of plan......")
planner_llm = EvaluatorOptimizerLLM(
optimizer=optimizer,
evaluator=evaluator,
llm_factory=OpenAIAugmentedLLM,
min_rating=QualityRating.EXCELLENT,
)
orchestrator = Orchestrator(
llm_factory=AnthropicAugmentedLLM,
available_agents=[finder_agent, writer_agent, proofreader, fact_checker, style_enforcer],
planner=planner_llm # It's that simple
)
...
Signaling: The framework can pause/resume tasks. The agent or LLM might “signal” that it needs user input, so the workflow awaits. A developer may signal during a workflow to seek approval or review before continuing with a workflow.
Human Input: If an Agent has a human_input_callback
, the LLM can call a __human_input__
tool to request user input mid-workflow.
Example
The Swarm example shows this in action.
from mcp_agent.human_input.handler import console_input_callback
lost_baggage = SwarmAgent(
name="Lost baggage traversal",
instruction=lambda context_variables: f"""
{
FLY_AIR_AGENT_PROMPT.format(
customer_context=context_variables.get("customer_context", "None"),
flight_context=context_variables.get("flight_context", "None"),
)
}\n Lost baggage policy: policies/lost_baggage_policy.md""",
functions=[
escalate_to_agent,
initiate_baggage_search,
transfer_to_triage,
case_resolved,
],
server_names=["fetch", "filesystem"],
human_input_callback=console_input_callback, # Request input from the console
)
Create an mcp_agent.config.yaml
and a gitignored mcp_agent.secrets.yaml
to define MCP app configuration. This controls logging, execution, LLM provider APIs, and MCP server configuration.
mcp-agent makes it trivial to connect to MCP servers. Create an mcp_agent.config.yaml
to define server configuration under the mcp
section:
mcp:
servers:
fetch:
command: "uvx"
args: ["mcp-server-fetch"]
description: "Fetch content at URLs from the world wide web"
Manage the lifecycle of an MCP server within an async context manager:
from mcp_agent.mcp.gen_client import gen_client
async with gen_client("fetch") as fetch_client:
# Fetch server is initialized and ready to use
result = await fetch_client.list_tools()
# Fetch server is automatically disconnected/shutdown
The gen_client function makes it easy to spin up connections to MCP servers.
In many cases, you want an MCP server to stay online for persistent use (e.g. in a multi-step tool use workflow). For persistent connections, use:
-
connect
anddisconnect
from mcp_agent.mcp.gen_client import connect, disconnect
fetch_client = None
try:
fetch_client = connect("fetch")
result = await fetch_client.list_tools()
finally:
disconnect("fetch")
-
MCPConnectionManager
For even more fine-grained control over server connections, you can use the MCPConnectionManager.
Example
from mcp_agent.context import get_current_context
from mcp_agent.mcp.mcp_connection_manager import MCPConnectionManager
context = get_current_context()
connection_manager = MCPConnectionManager(context.server_registry)
async with connection_manager:
fetch_client = await connection_manager.get_server("fetch") # Initializes fetch server
result = fetch_client.list_tool()
fetch_client2 = await connection_manager.get_server("fetch") # Reuses same server connection
# All servers managed by connection manager are automatically disconnected/shut down
MCPAggregator
acts as a "server-of-servers".
It provides a single MCP server interface for interacting with multiple MCP servers.
This allows you to expose tools from multiple servers to LLM applications.
Example
from mcp_agent.mcp.mcp_aggregator import MCPAggregator
aggregator = await MCPAggregator.create(server_names=["fetch", "filesystem"])
async with aggregator:
# combined list of tools exposed by 'fetch' and 'filesystem' servers
tools = await aggregator.list_tools()
# namespacing -- invokes the 'fetch' server to call the 'fetch' tool
fetch_result = await aggregator.call_tool(name="fetch-fetch", arguments={"url": "https://www.anthropic.com/research/building-effective-agents"})
# no namespacing -- first server in the aggregator exposing that tool wins
read_file_result = await aggregator.call_tool(name="read_file", arguments={})
We welcome any and all kinds of contributions. Please see the CONTRIBUTING guidelines to get started.
There have already been incredible community contributors who are driving this project forward:
-
Shaun Smith (@evalstate) -- who has been leading the charge on countless complex improvements, both to
mcp-agent
and generally to the MCP ecosystem. - Jerron Lim (@StreetLamb) -- who has contributed countless hours and excellent examples, and great ideas to the project.
- Jason Summer (@jasonsum) -- for identifying several issues and adapting his Gmail MCP server to work with mcp-agent
We will be adding a detailed roadmap (ideally driven by your feedback). The current set of priorities include:
- Durable Execution -- allow workflows to pause/resume and serialize state so they can be replayed or be paused indefinitely. We are working on integrating Temporal for this purpose.
- Memory -- adding support for long-term memory
- Streaming -- Support streaming listeners for iterative progress
-
Additional MCP capabilities -- Expand beyond tool calls to support:
- Resources
- Prompts
- Notifications
mcp-agent provides a streamlined approach to building AI agents using capabilities exposed by MCP (Model Context Protocol) servers.
MCP is quite low-level, and this framework handles the mechanics of connecting to servers, working with LLMs, handling external signals (like human input) and supporting persistent state via durable execution. That lets you, the developer, focus on the core business logic of your AI application.
Core benefits:
- 🤝 Interoperability: ensures that any tool exposed by any number of MCP servers can seamlessly plug in to your agents.
- ⛓️ Composability & Cutstomizability: Implements well-defined workflows, but in a composable way that enables compound workflows, and allows full customization across model provider, logging, orchestrator, etc.
- 💻 Programmatic control flow: Keeps things simple as developers just write code instead of thinking in graphs, nodes and edges. For branching logic, you write
if
statements. For cycles, usewhile
loops. - 🖐️ Human Input & Signals: Supports pausing workflows for external signals, such as human input, which are exposed as tool calls an Agent can make.
No, you can use mcp-agent anywhere, since it handles MCPClient creation for you. This allows you to leverage MCP servers outside of MCP hosts like Claude Desktop.
Here's all the ways you can set up your mcp-agent application:
You can expose mcp-agent applications as MCP servers themselves (see example), allowing MCP clients to interface with sophisticated AI workflows using the standard tools API of MCP servers. This is effectively a server-of-servers.
You can embed mcp-agent in an MCP client directly to manage the orchestration across multiple MCP servers.
You can use mcp-agent applications in a standalone fashion (i.e. they aren't part of an MCP client). The examples
are all standalone applications.
I debated naming this project silsila (سلسلہ), which means chain of events in Urdu. mcp-agent is more matter-of-fact, but there's still an easter egg in the project paying homage to silsila.
For Tasks:
Click tags to check more tools for each tasksFor Jobs:
Alternative AI tools for mcp-agent
Similar Open Source Tools
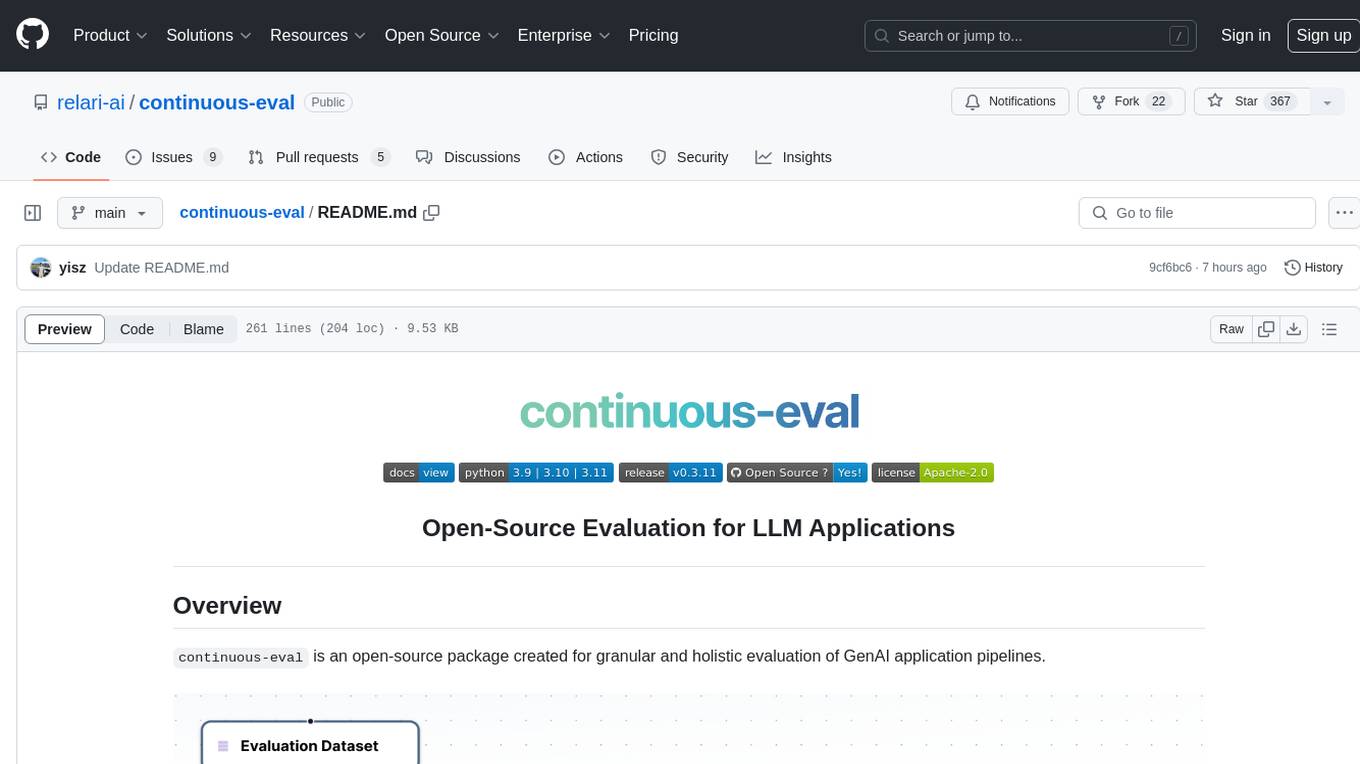
continuous-eval
Open-Source Evaluation for LLM Applications. `continuous-eval` is an open-source package created for granular and holistic evaluation of GenAI application pipelines. It offers modularized evaluation, a comprehensive metric library covering various LLM use cases, the ability to leverage user feedback in evaluation, and synthetic dataset generation for testing pipelines. Users can define their own metrics by extending the Metric class. The tool allows running evaluation on a pipeline defined with modules and corresponding metrics. Additionally, it provides synthetic data generation capabilities to create user interaction data for evaluation or training purposes.
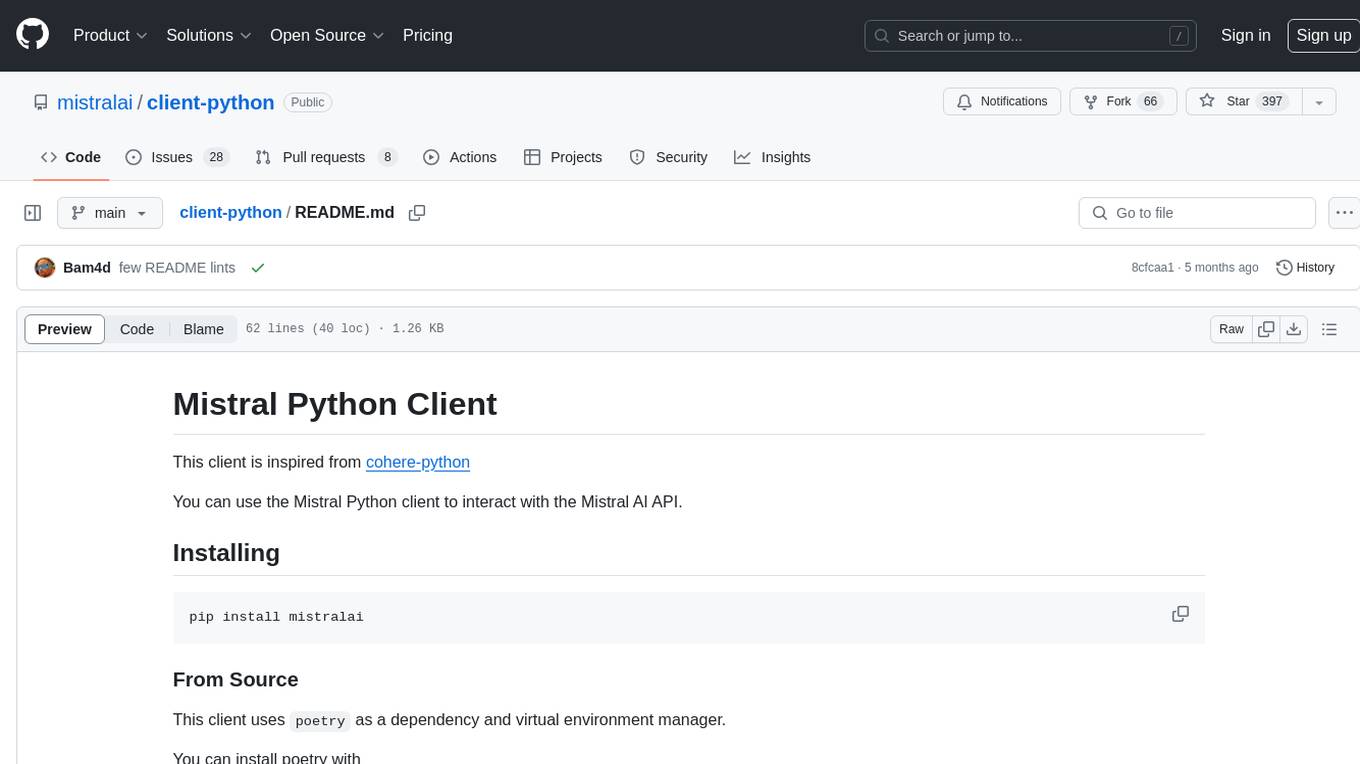
client-python
The Mistral Python Client is a tool inspired by cohere-python that allows users to interact with the Mistral AI API. It provides functionalities to access and utilize the AI capabilities offered by Mistral. Users can easily install the client using pip and manage dependencies using poetry. The client includes examples demonstrating how to use the API for various tasks, such as chat interactions. To get started, users need to obtain a Mistral API Key and set it as an environment variable. Overall, the Mistral Python Client simplifies the integration of Mistral AI services into Python applications.
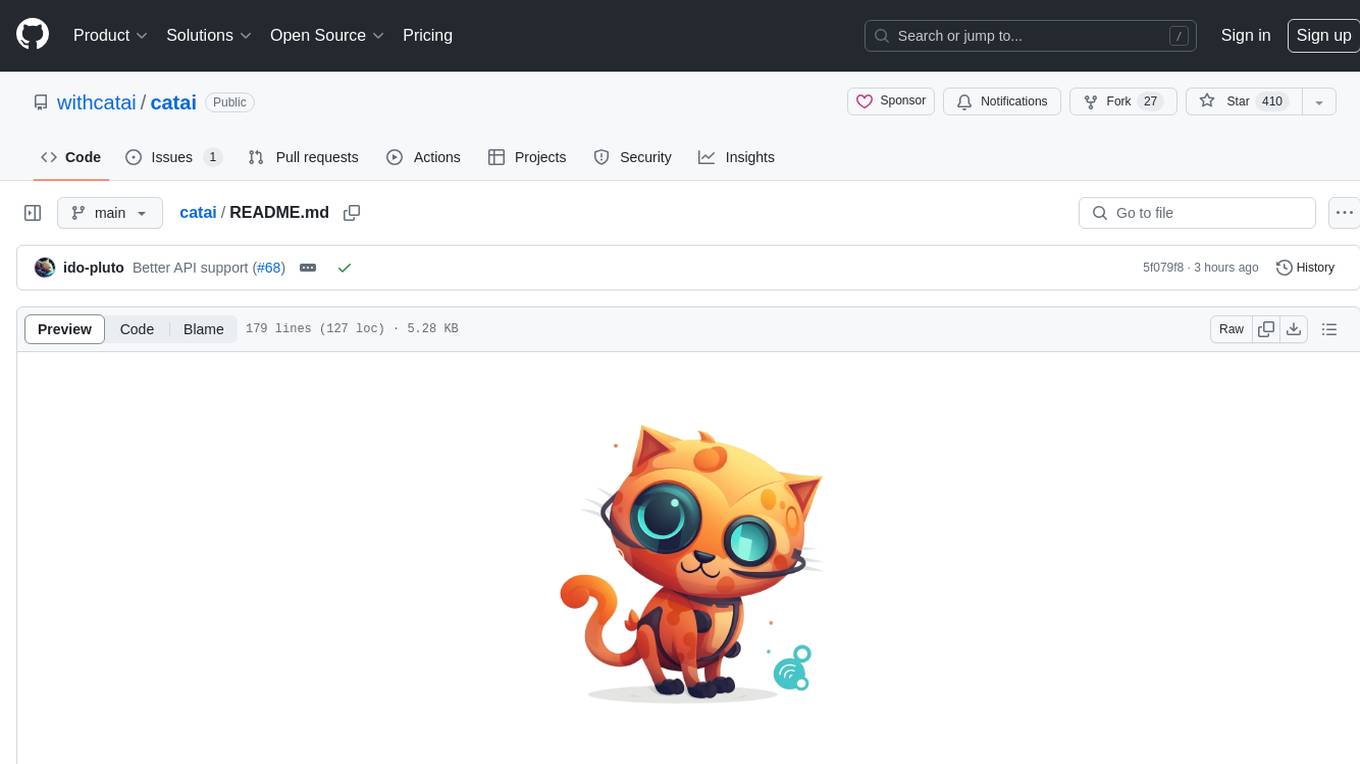
catai
CatAI is a tool that allows users to run GGUF models on their computer with a chat UI. It serves as a local AI assistant inspired by Node-Llama-Cpp and Llama.cpp. The tool provides features such as auto-detecting programming language, showing original messages by clicking on user icons, real-time text streaming, and fast model downloads. Users can interact with the tool through a CLI that supports commands for installing, listing, setting, serving, updating, and removing models. CatAI is cross-platform and supports Windows, Linux, and Mac. It utilizes node-llama-cpp and offers a simple API for asking model questions. Additionally, developers can integrate the tool with node-llama-cpp@beta for model management and chatting. The configuration can be edited via the web UI, and contributions to the project are welcome. The tool is licensed under Llama.cpp's license.
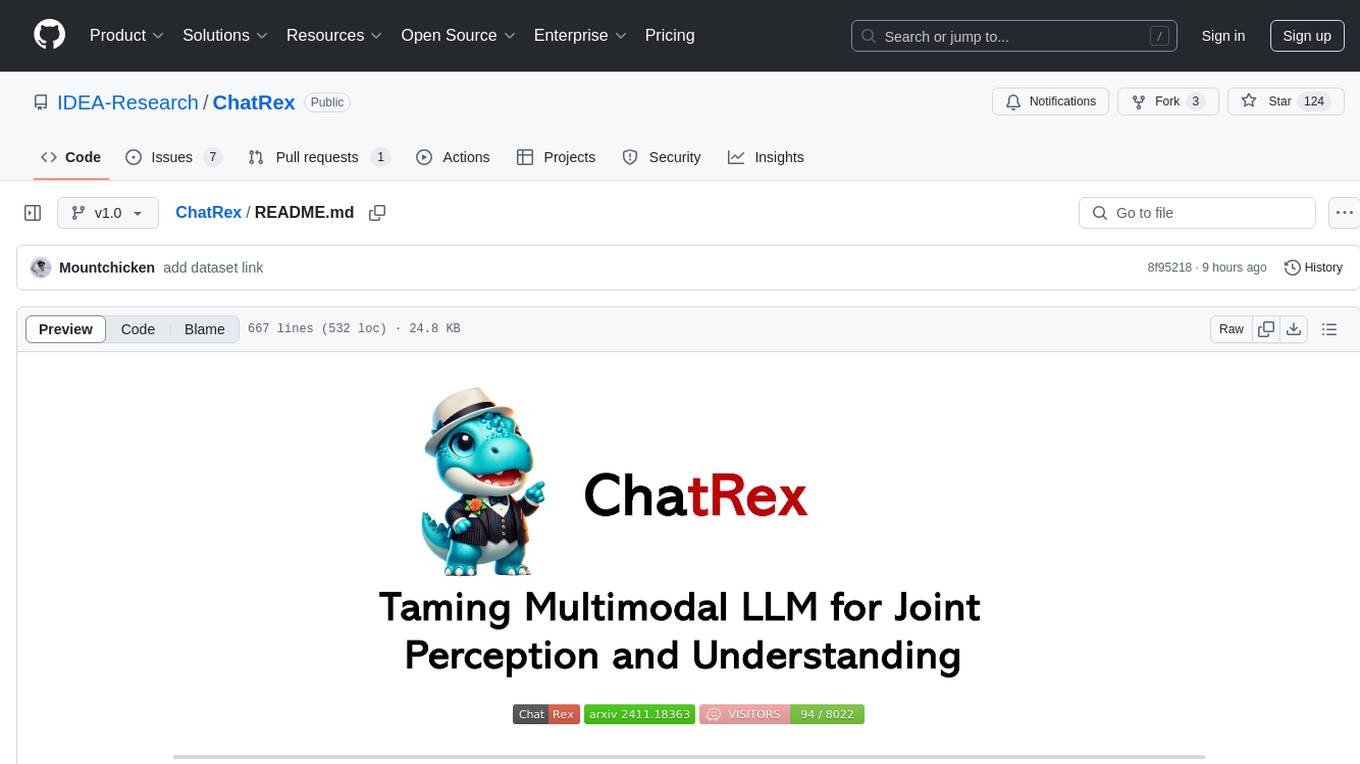
ChatRex
ChatRex is a Multimodal Large Language Model (MLLM) designed to seamlessly integrate fine-grained object perception and robust language understanding. By adopting a decoupled architecture with a retrieval-based approach for object detection and leveraging high-resolution visual inputs, ChatRex addresses key challenges in perception tasks. It is powered by the Rexverse-2M dataset with diverse image-region-text annotations. ChatRex can be applied to various scenarios requiring fine-grained perception, such as object detection, grounded conversation, grounded image captioning, and region understanding.
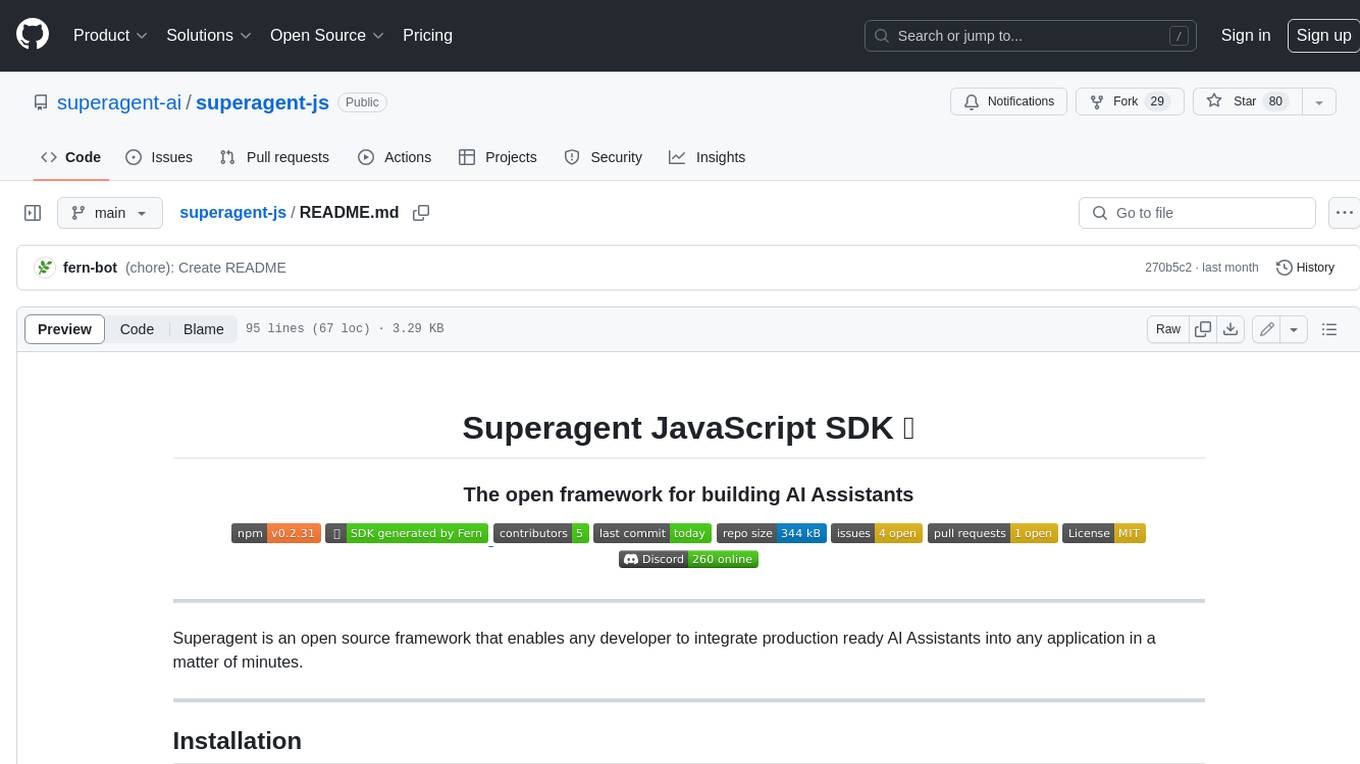
superagent-js
Superagent is an open source framework that enables any developer to integrate production ready AI Assistants into any application in a matter of minutes.
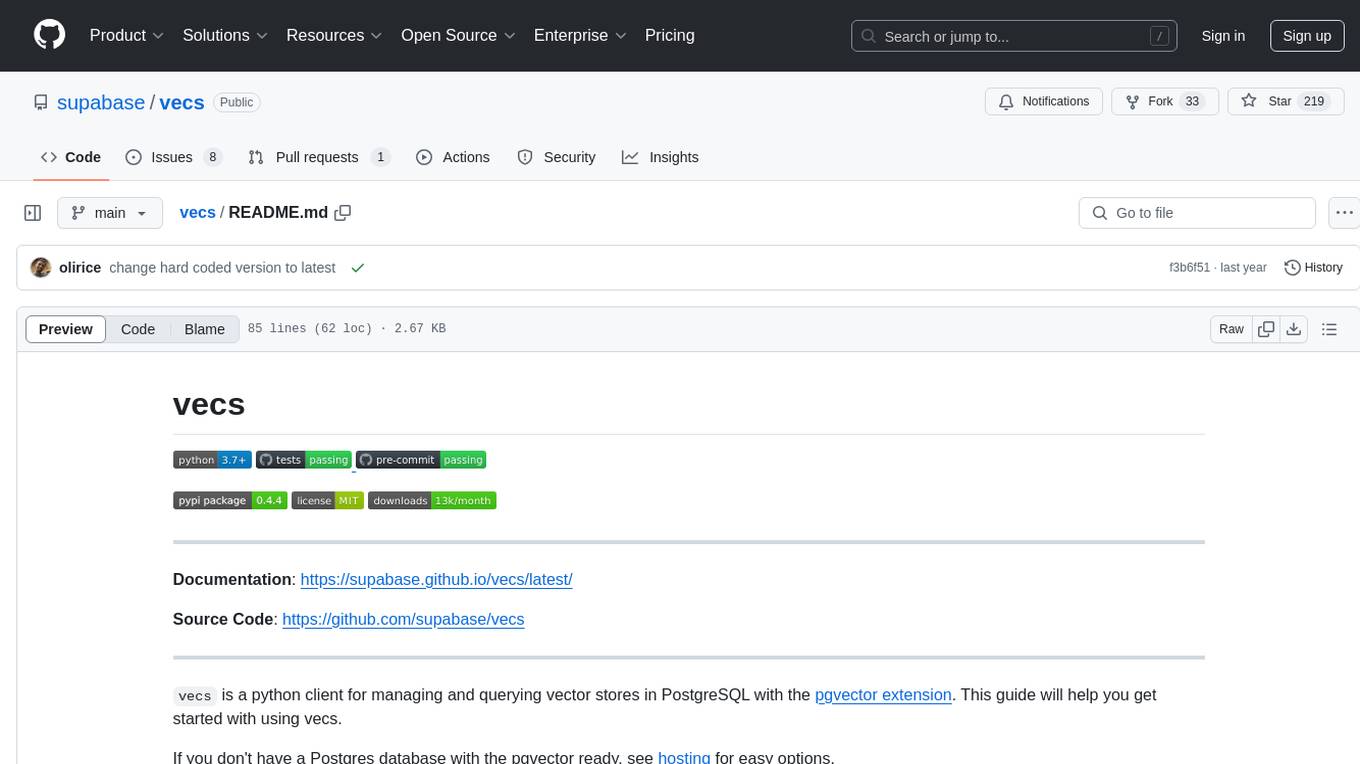
vecs
vecs is a Python client for managing and querying vector stores in PostgreSQL with the pgvector extension. It allows users to create collections of vectors with associated metadata, index the collections for fast search performance, and query the collections based on specified filters. The tool simplifies the process of working with vector data in a PostgreSQL database, making it easier to store, retrieve, and analyze vector information.
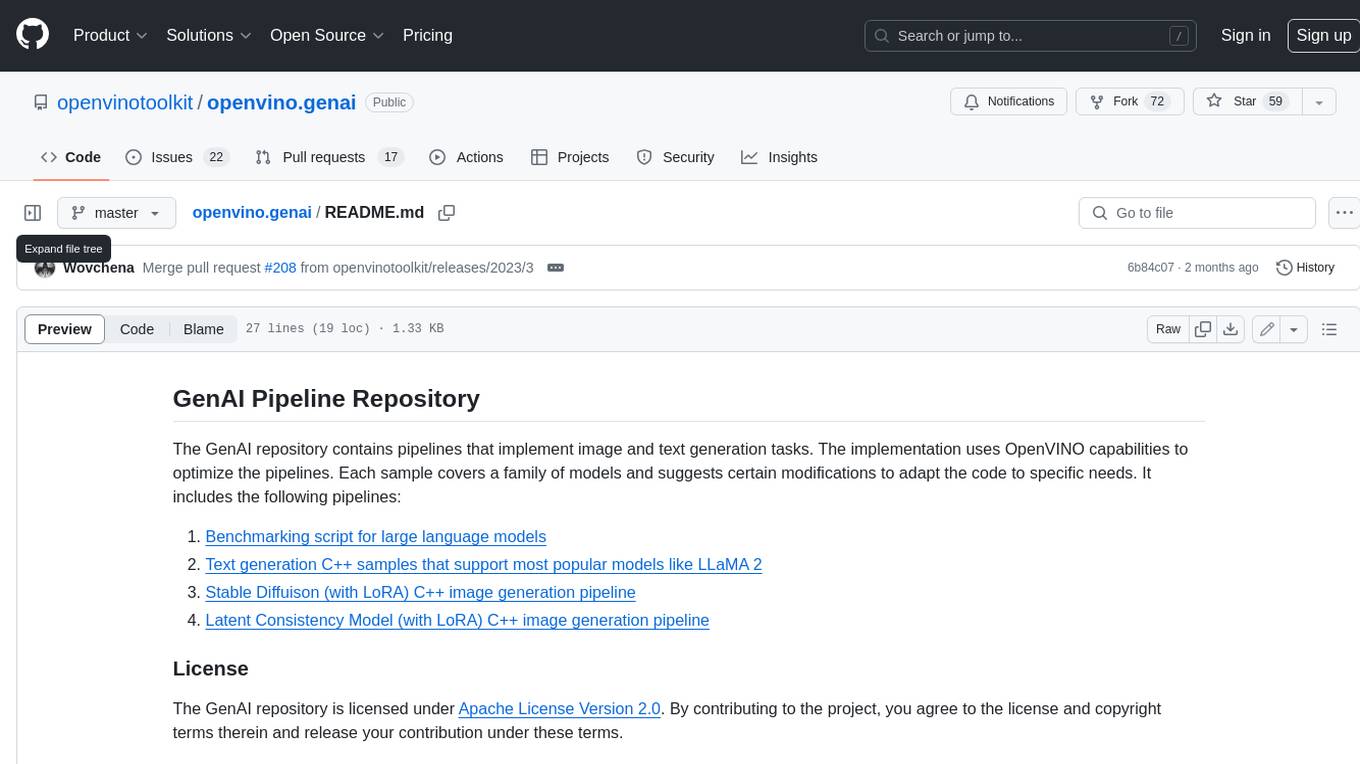
openvino.genai
The GenAI repository contains pipelines that implement image and text generation tasks. The implementation uses OpenVINO capabilities to optimize the pipelines. Each sample covers a family of models and suggests certain modifications to adapt the code to specific needs. It includes the following pipelines: 1. Benchmarking script for large language models 2. Text generation C++ samples that support most popular models like LLaMA 2 3. Stable Diffuison (with LoRA) C++ image generation pipeline 4. Latent Consistency Model (with LoRA) C++ image generation pipeline
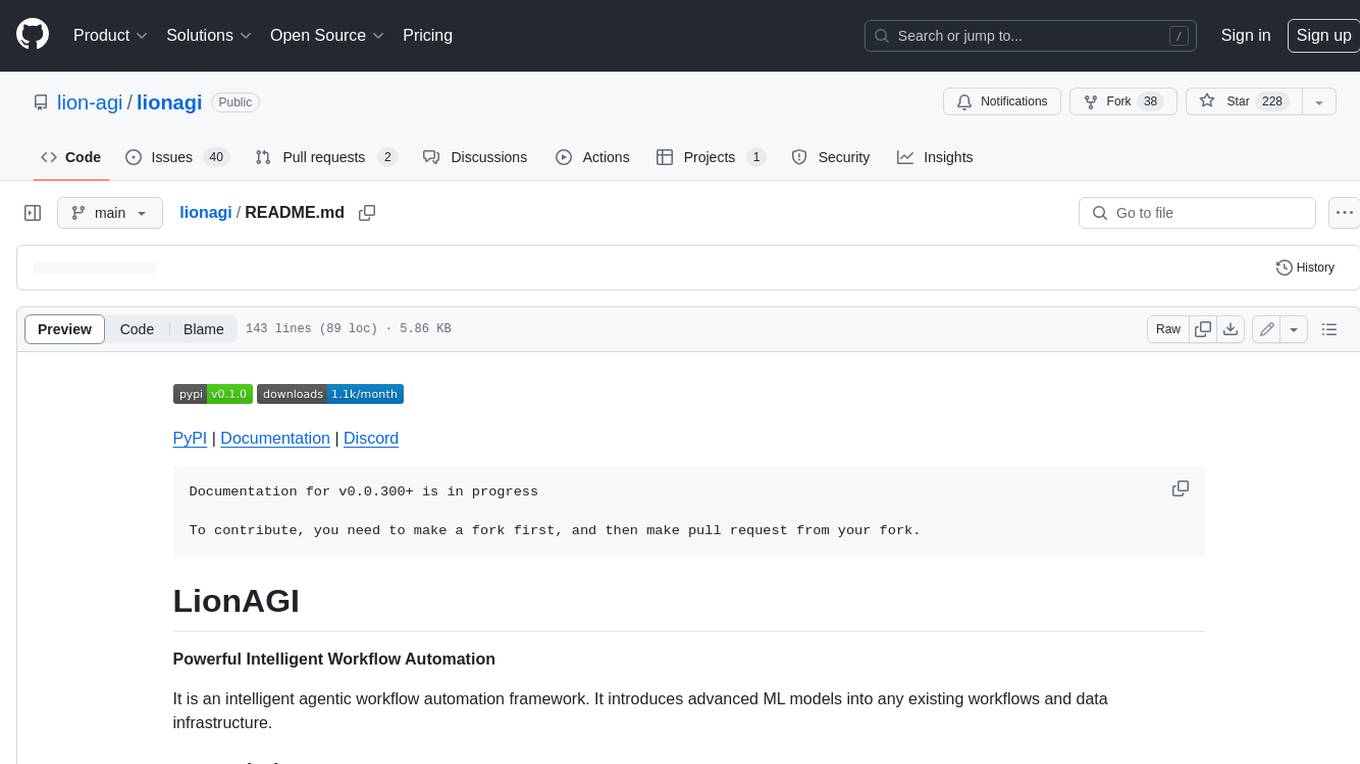
lionagi
LionAGI is a powerful intelligent workflow automation framework that introduces advanced ML models into any existing workflows and data infrastructure. It can interact with almost any model, run interactions in parallel for most models, produce structured pydantic outputs with flexible usage, automate workflow via graph based agents, use advanced prompting techniques, and more. LionAGI aims to provide a centralized agent-managed framework for "ML-powered tools coordination" and to dramatically lower the barrier of entries for creating use-case/domain specific tools. It is designed to be asynchronous only and requires Python 3.10 or higher.
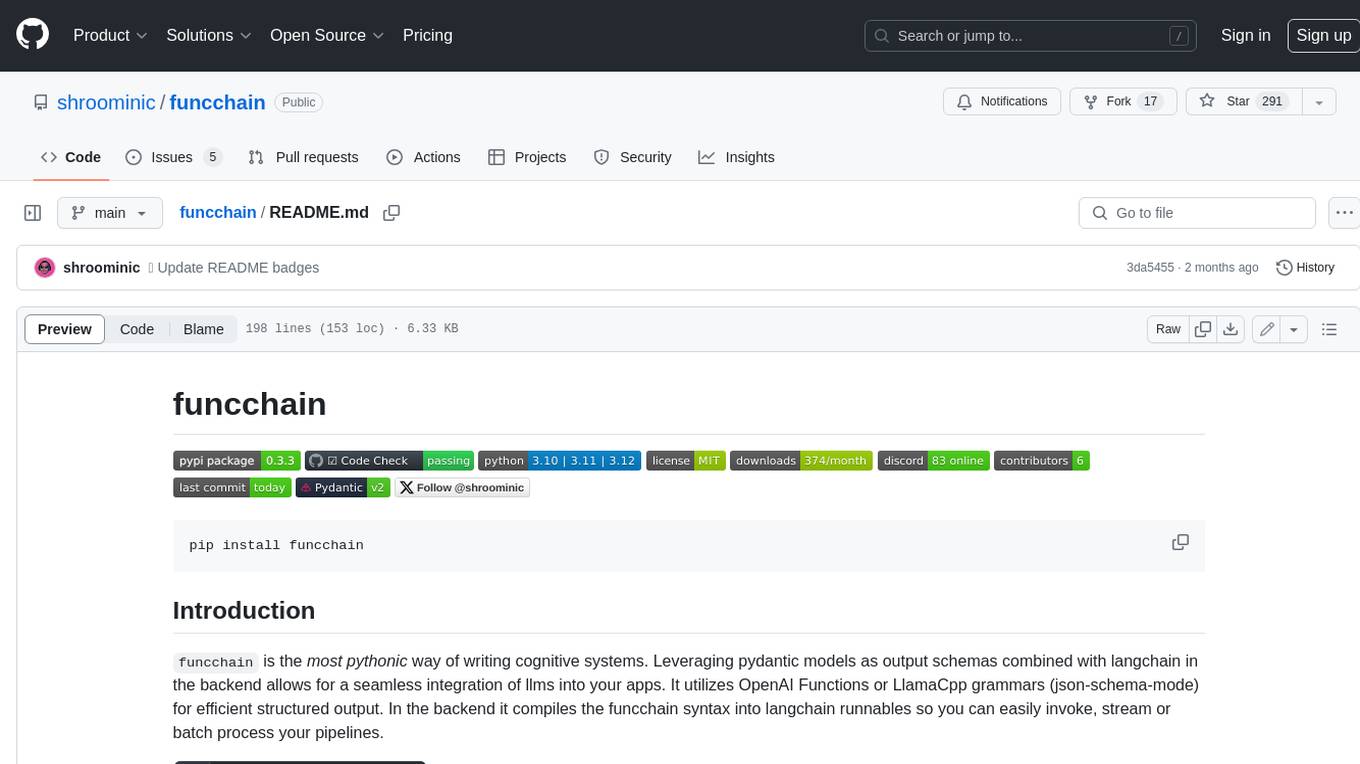
funcchain
Funcchain is a Python library that allows you to easily write cognitive systems by leveraging Pydantic models as output schemas and LangChain in the backend. It provides a seamless integration of LLMs into your apps, utilizing OpenAI Functions or LlamaCpp grammars (json-schema-mode) for efficient structured output. Funcchain compiles the Funcchain syntax into LangChain runnables, enabling you to invoke, stream, or batch process your pipelines effortlessly.
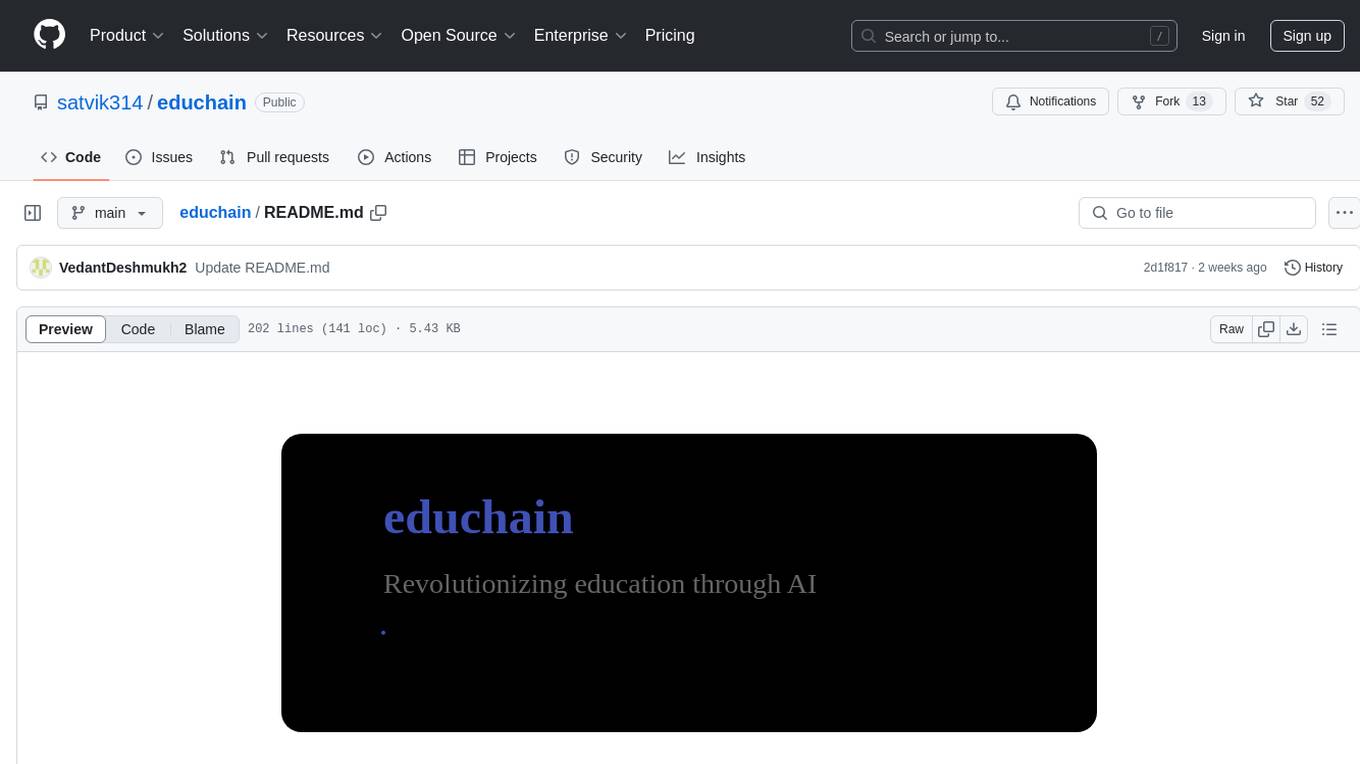
educhain
Educhain is a powerful Python package that leverages Generative AI to create engaging and personalized educational content. It enables users to generate multiple-choice questions, create lesson plans, and support various LLM models. Users can export questions to JSON, PDF, and CSV formats, customize prompt templates, and generate questions from text, PDF, URL files, youtube videos, and images. Educhain outperforms traditional methods in content generation speed and quality. It offers advanced configuration options and has a roadmap for future enhancements, including integration with popular Learning Management Systems and a mobile app for content generation on-the-go.
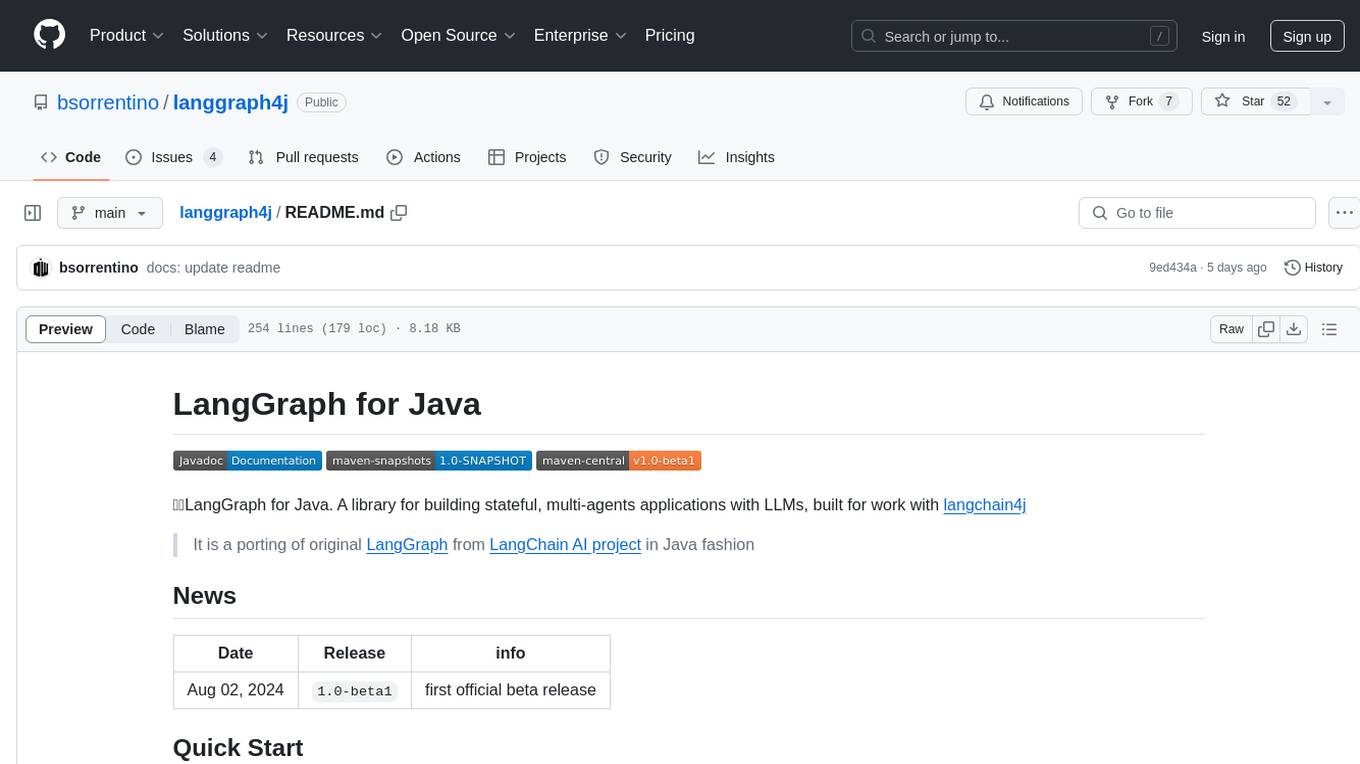
langgraph4j
LangGraph for Java is a library designed for building stateful, multi-agent applications with LLMs. It is a porting of the original LangGraph from the LangChain AI project to Java. The library allows users to define agent states, nodes, and edges in a graph structure to create complex workflows. It integrates with LangChain4j and provides tools for executing actions based on agent decisions. LangGraph for Java enables users to create asynchronous node actions, conditional edges, and normal edges to model decision-making processes in applications.
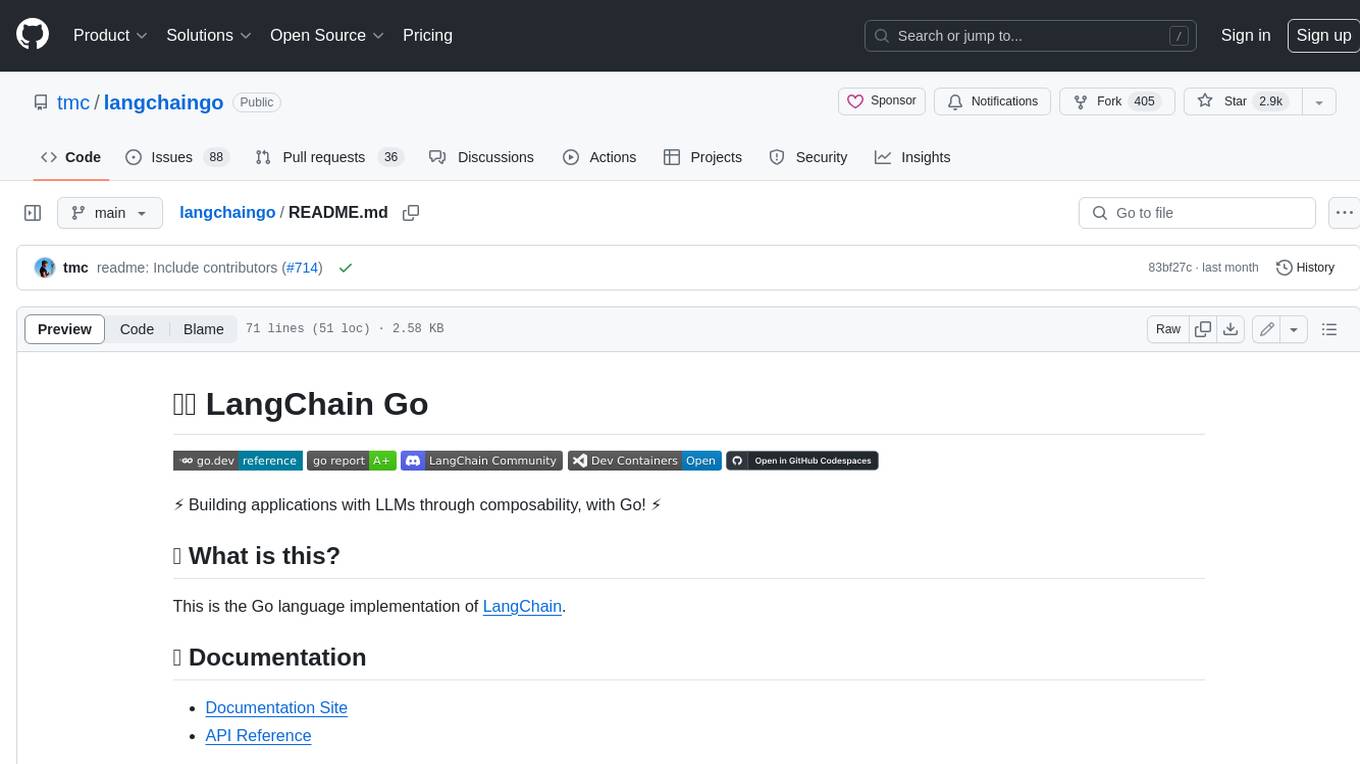
langchaingo
LangChain Go is a Go language implementation of LangChain, a framework for building applications with LLMs through composability. It provides a simple and easy-to-use API for interacting with LLMs, making it easy to add language-based features to your applications.
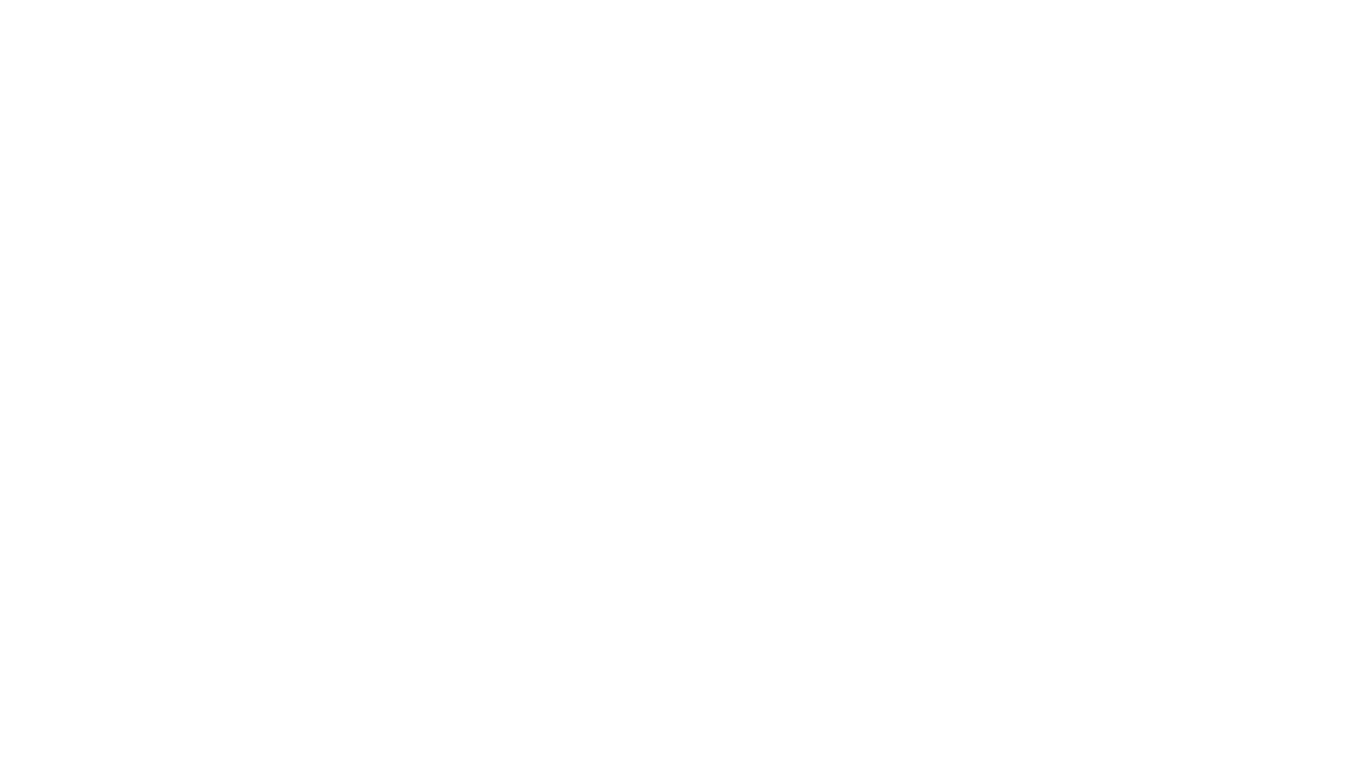
rl
TorchRL is an open-source Reinforcement Learning (RL) library for PyTorch. It provides pytorch and **python-first** , low and high level abstractions for RL that are intended to be **efficient** , **modular** , **documented** and properly **tested**. The code is aimed at supporting research in RL. Most of it is written in python in a highly modular way, such that researchers can easily swap components, transform them or write new ones with little effort.
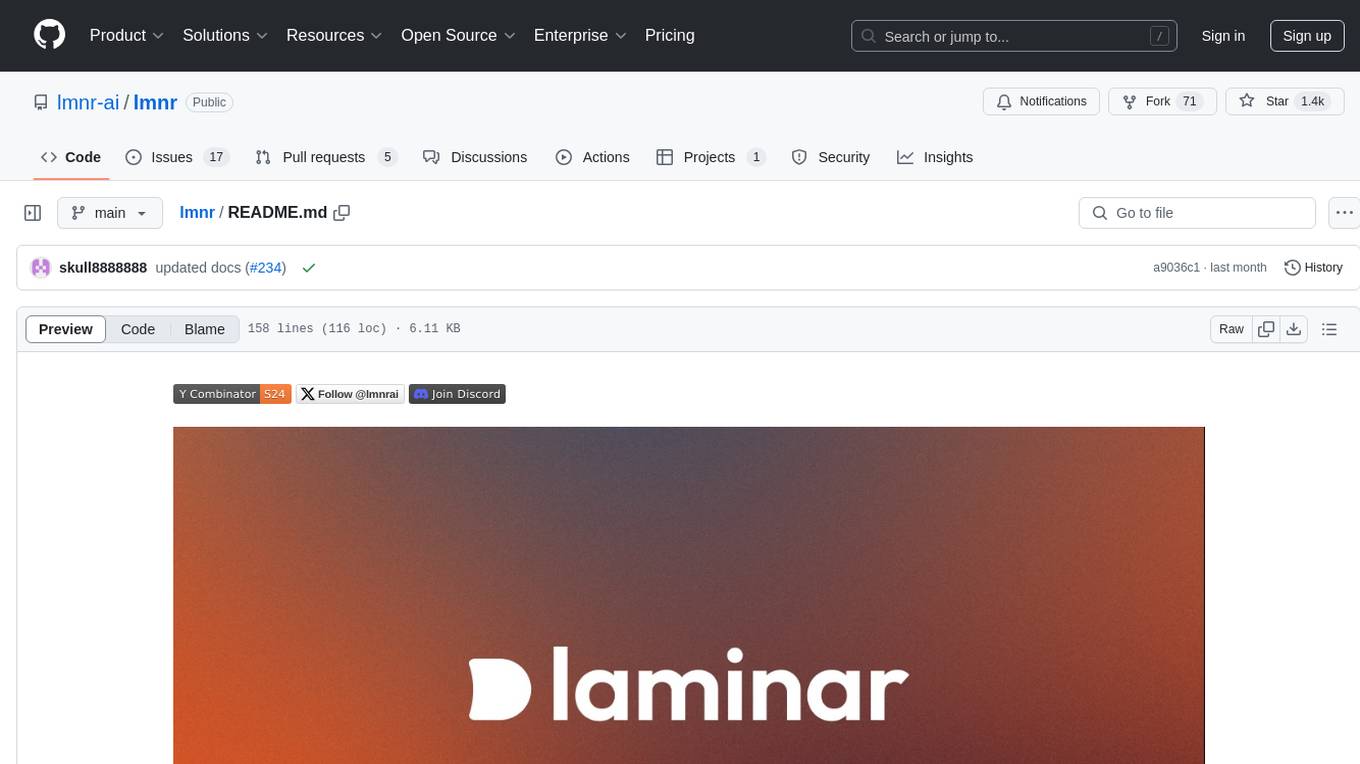
lmnr
Laminar is an all-in-one open-source platform designed for engineering AI products. It allows users to trace, evaluate, label, and analyze LLM data efficiently. The platform offers features such as automatic tracing of common AI frameworks and SDKs, local and online evaluations, simple UI for data labeling, dataset management, and scalability with gRPC communication. Laminar is built with a modern open-source stack including RabbitMQ, Postgres, Clickhouse, and Qdrant for semantic similarity search. It provides fast and beautiful dashboards for traces, evaluations, and labels, making it a comprehensive tool for AI product development.
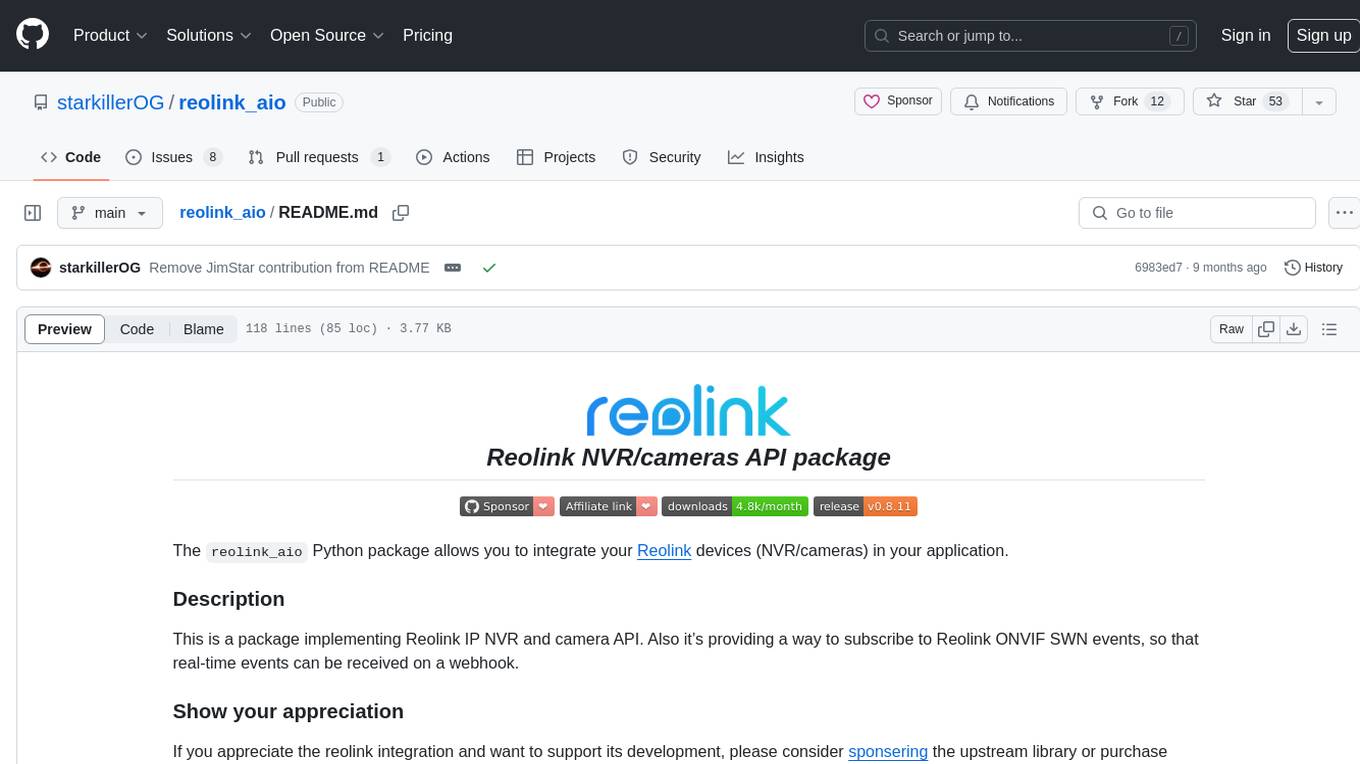
reolink_aio
The 'reolink_aio' Python package is designed to integrate Reolink devices (NVR/cameras) into your application. It implements Reolink IP NVR and camera API, allowing users to subscribe to Reolink ONVIF SWN events for real-time event notifications via webhook. The package provides functionalities to obtain and cache NVR or camera settings, capabilities, and states, as well as enable features like infrared lights, spotlight, and siren. Users can also subscribe to events, renew timers, and disconnect from the host device.