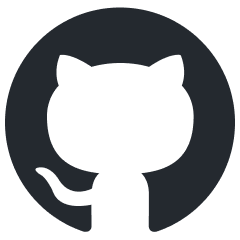
langgraph4j
🚀 LangGraph for Java. A library for building stateful, multi-actor applications with LLMs, built for work jointly with langchain4j
Stars: 365
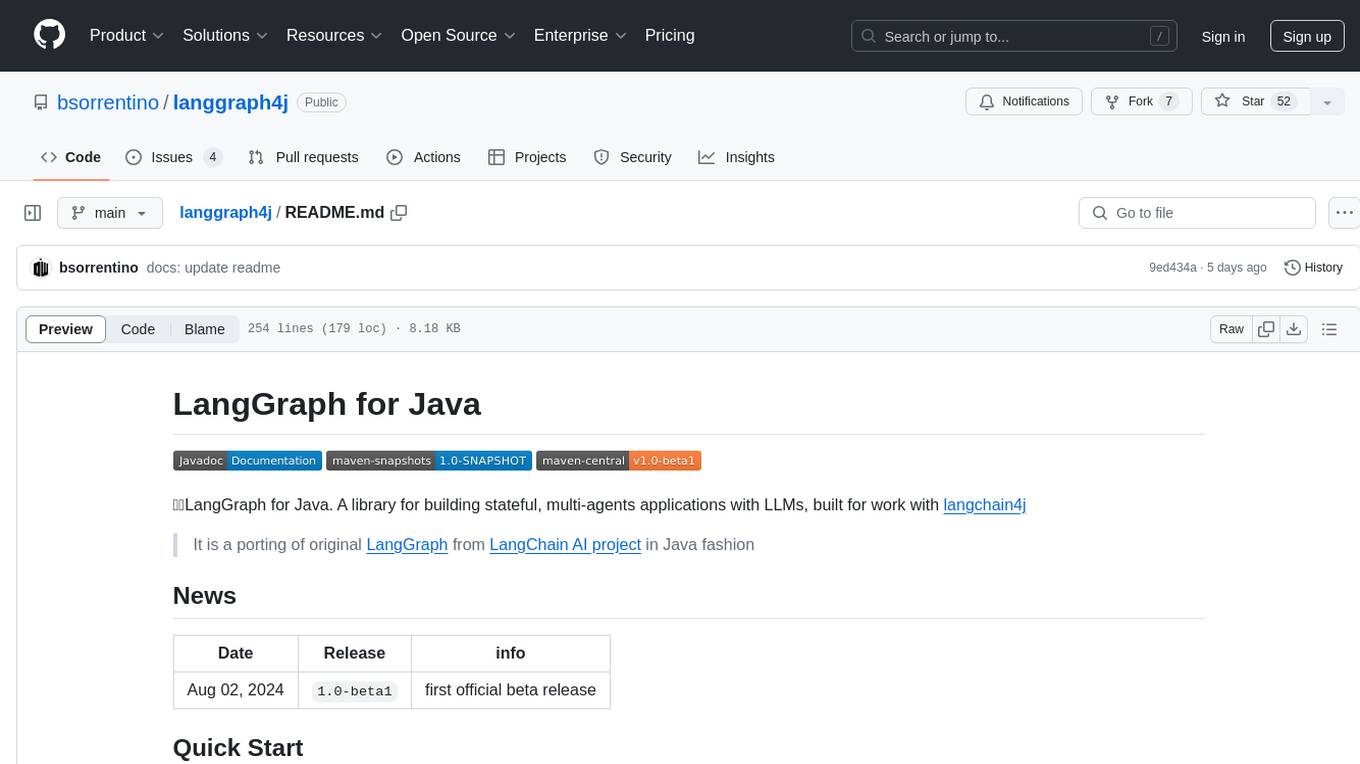
LangGraph for Java is a library designed for building stateful, multi-agent applications with LLMs. It is a porting of the original LangGraph from the LangChain AI project to Java. The library allows users to define agent states, nodes, and edges in a graph structure to create complex workflows. It integrates with LangChain4j and provides tools for executing actions based on agent decisions. LangGraph for Java enables users to create asynchronous node actions, conditional edges, and normal edges to model decision-making processes in applications.
README:
LangGraph for Java. A library for building stateful, multi-agents applications with LLMs, built for work with langchain4j
It is a porting of original LangGraph from LangChain AI project in Java fashion
- [x] StateGraph
- [x] Nodes
- [x] Edges
- [x] Conditional Edges
- [x] Entry Points
- [x] Conditional Entry Points
- [x] State
- [x] Schema (a series of Channels)
- [x] Reducer (how apply updates to the state attributes)
- [x] Default provider
- [x] Appender channel (values accumulator)
- [x] delete messages
- [x] Schema (a series of Channels)
- [x] Compiling graph
- [x] Async support (throught CompletableFuture)
- [x] Streaming support (throught java-async-generator)
- [x] Checkpoints (save and replay feature)
- [x] Graph visualization
- [x] Playground (Embeddable Webapp that plays with LangGraph4j)
- [x] Threads (checkpointing of multiple different runs)
- [x] Update state (interact with the state directly and update it)
- [x] Breakpoints (pause and resume feature)
- [x] Studio (Playground Webapp)
- [x] Spring Boot
- [x] Jetty
- [x] Quarkus
- [X] Streaming response from LLM results
- [X] Child Graphs
- [X] Parallel Node Execution
- With some constraints
- [X] Generator (Visual Builder)
Note:
From release 1.2.x the miminum supported Java version is the
Java 17
and the artifactlanggraph4j-core-jdk8
is replaced bylanggraph4j-core
Date | Release | info |
---|---|---|
Apr 06, 2025 | 1.5.4 |
official release |
- How to add persistence ("memory") to your graph
- How to view and update past graph state
- How to parallel branch
- How to wait for user input
- How to sub-graph
- Use Case
Project | Integrated With |
---|---|
Agent Executor | SpringAI |
Agent Executor | Langchain4j |
Image To PlantUML Diagram | Langchain4j |
Adaptive RAG | Langchain4j |
Maven
<dependency>
<groupId>org.bsc.langgraph4j</groupId>
<artifactId>langgraph4j-core</artifactId>
<version>1.5.4</version>
</dependency>
Maven
<dependency>
<groupId>org.bsc.langgraph4j</groupId>
<artifactId>langgraph4j-core</artifactId>
<version>1.5-SNAPSHOT</version>
</dependency>
The main type of graph in langgraph
is the StatefulGraph
. This graph is parameterized by a state object that it passes around to each node.
Each node then returns operations to update that state. These operations can either SET specific attributes on the state (e.g. overwrite the existing values) or ADD to the existing attribute.
Whether to set or add is described in the state's schema provided to the graph. The schema is a Map of Channels, each Channel represent an attribute in the state. If an attribute is described with an AppendeChannel
it will be a List and each element referring the attribute will be automaically added by graph during processing. The State must inherit from AgentState
base class (that essentially is a Map
wrapper).
public class AgentState {
public AgentState( Map<String,Object> initData ) { ... }
public final java.util.Map<String,Object> data() { ... }
public final <T> Optional<T> value(String key) { ... }
public final <T> T value(String key, T defaultValue ) { ... }
public final <T> T value(String key, Supplier<T> defaultProvider ) { ... }
}
We now need to define a few different nodes in our graph. In langgraph
, a node is an async/sync function that accept an AgentState
as argument and returns a (partial) state update. There are two main nodes we need for this:
- The agent: responsible for deciding what (if any) actions to take.
- A function to invoke tools: if the agent decides to take an action, this node will then execute that action.
/**
* Represents an asynchronous node action that operates on an agent state and returns state update.
*
* @param <S> the type of the agent state
*/
@FunctionalInterface
public interface AsyncNodeAction<S extends AgentState> extends Function<S, CompletableFuture<Map<String, Object>>> {
CompletableFuture<Map<String, Object>> apply(S t);
/**
* Creates an asynchronous node action from a synchronous node action.
*/
static <S extends AgentState> AsyncNodeAction<S> node_async(NodeAction<S> syncAction) { ... }
}
We will also need to define some edges. Some of these edges may be conditional. The reason they are conditional is that based on the output of a node, one of several paths may be taken. The path that is taken is not known until that node is run (the LLM decides).
-
Conditional Edge: after the agent is called, we should either:
- If the agent said to take an action, then the function to invoke tools should be called
- If the agent said that it was finished, then it should finish
- Normal Edge: after the tools are invoked, it should always go back to the agent to decide what to do next
/**
* Represents an asynchronous edge action that operates on an agent state and returns a new route.
*
* @param <S> the type of the agent state
*/
public interface AsyncEdgeAction<S extends AgentState> extends Function<S, CompletableFuture<String>> {
CompletableFuture<String> apply(S t);
/**
* Creates an asynchronous edge action from a synchronous edge action.
*/
static <S extends AgentState> AsyncEdgeAction<S> edge_async(EdgeAction<S> syncAction ) { ... }
}
We can now put it all together and define the graph! (see example below)
Like default use case proposed in LangGraph blog, We have converted AgentExecutor implementation from langchain using LangGraph4j. In the agent-executor project's sample, there is the complete working code with tests. Feel free to checkout and use it as a reference.
Below you can find a piece of code of the AgentExecutor
to give you an idea of how is has built in langgraph style.
/**
* Represents the state of an agent.
*/
class State extends MessagesState<ChatMessage> {
public State(Map<String, Object> initData) {
super(initData);
}
public Optional<String> finalResponse() {
return value("agent_response");
}
}
var toolNode = ToolNode.builder()
.toolSpecification( tools )
.build();
var agent = Agent.builder()
.chatLanguageModel(chatLanguageModel)
.tools(toolNode.toolSpecifications())
.build();
var callAgent = new CallAgent(agent);
var executeTools = new ExecuteTools(agent, toolNode);
// Fluent Interface
var app = new StateGraph<>(State.SCHEMA, State::new)
.addEdge(START,"agent")
.addNode("agent", node_async(callAgent))
.addNode("action", node_async(executeTools))
.addConditionalEdges(
"agent",
edge_async( state ->
state.finalResponse()
.map(res -> "end")
.orElse("continue");
),
Map.of("continue", "action", "end", END)
)
.addEdge("action", "agent")
.compile();
return app.stream( inputs );
For Tasks:
Click tags to check more tools for each tasksFor Jobs:
Alternative AI tools for langgraph4j
Similar Open Source Tools
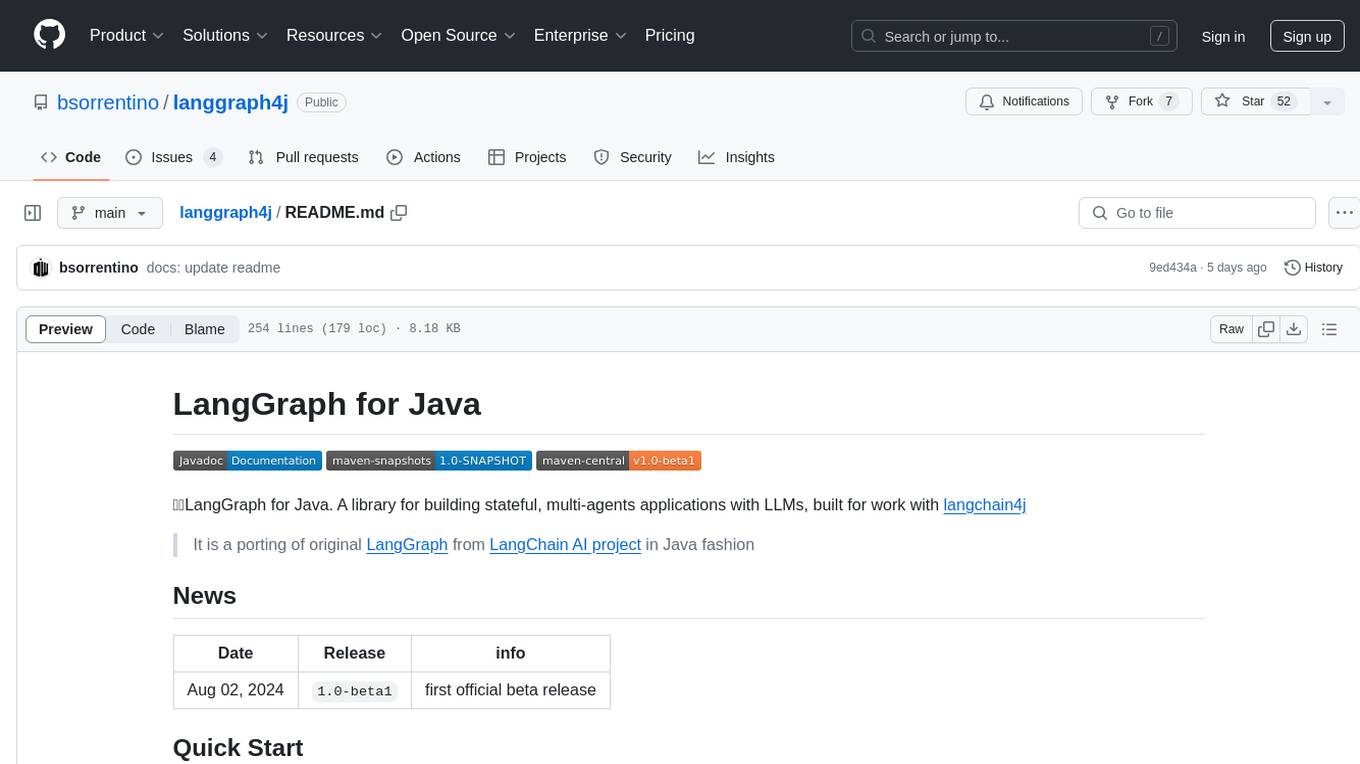
langgraph4j
LangGraph for Java is a library designed for building stateful, multi-agent applications with LLMs. It is a porting of the original LangGraph from the LangChain AI project to Java. The library allows users to define agent states, nodes, and edges in a graph structure to create complex workflows. It integrates with LangChain4j and provides tools for executing actions based on agent decisions. LangGraph for Java enables users to create asynchronous node actions, conditional edges, and normal edges to model decision-making processes in applications.
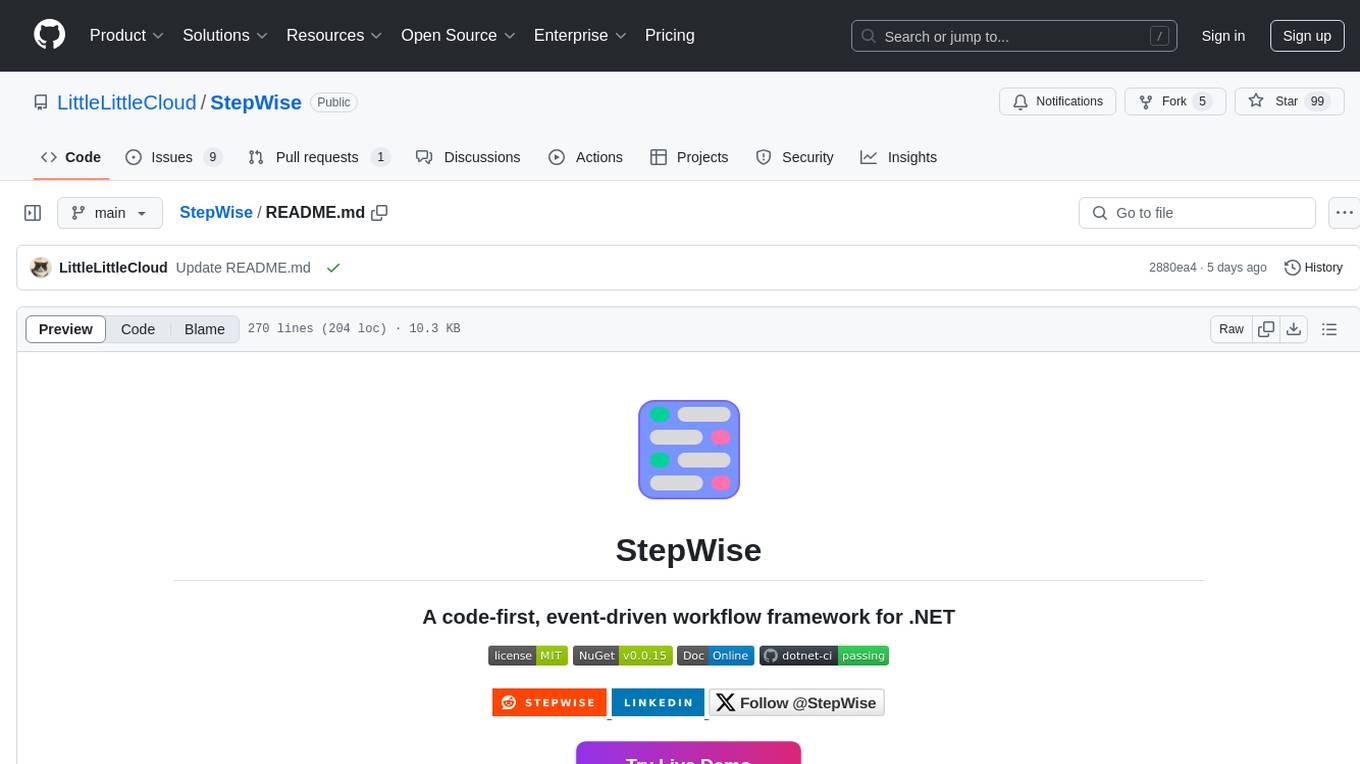
StepWise
StepWise is a code-first, event-driven workflow framework for .NET designed to help users build complex workflows in a simple and efficient way. It allows users to define workflows using C# code, visualize and execute workflows from a browser, execute steps in parallel, and resolve dependencies automatically. StepWise also features an AI assistant called `Geeno` in its WebUI to help users run and analyze workflows with ease.
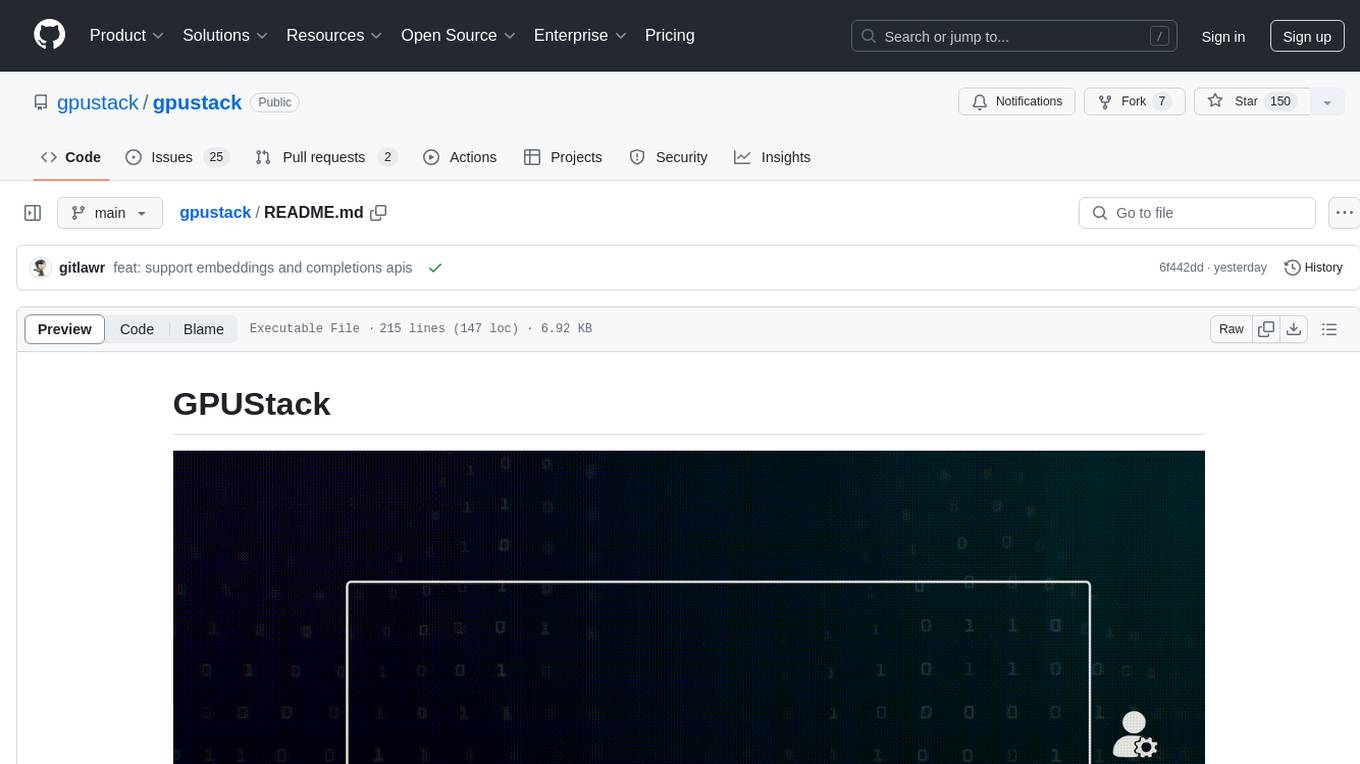
gpustack
GPUStack is an open-source GPU cluster manager designed for running large language models (LLMs). It supports a wide variety of hardware, scales with GPU inventory, offers lightweight Python package with minimal dependencies, provides OpenAI-compatible APIs, simplifies user and API key management, enables GPU metrics monitoring, and facilitates token usage and rate metrics tracking. The tool is suitable for managing GPU clusters efficiently and effectively.
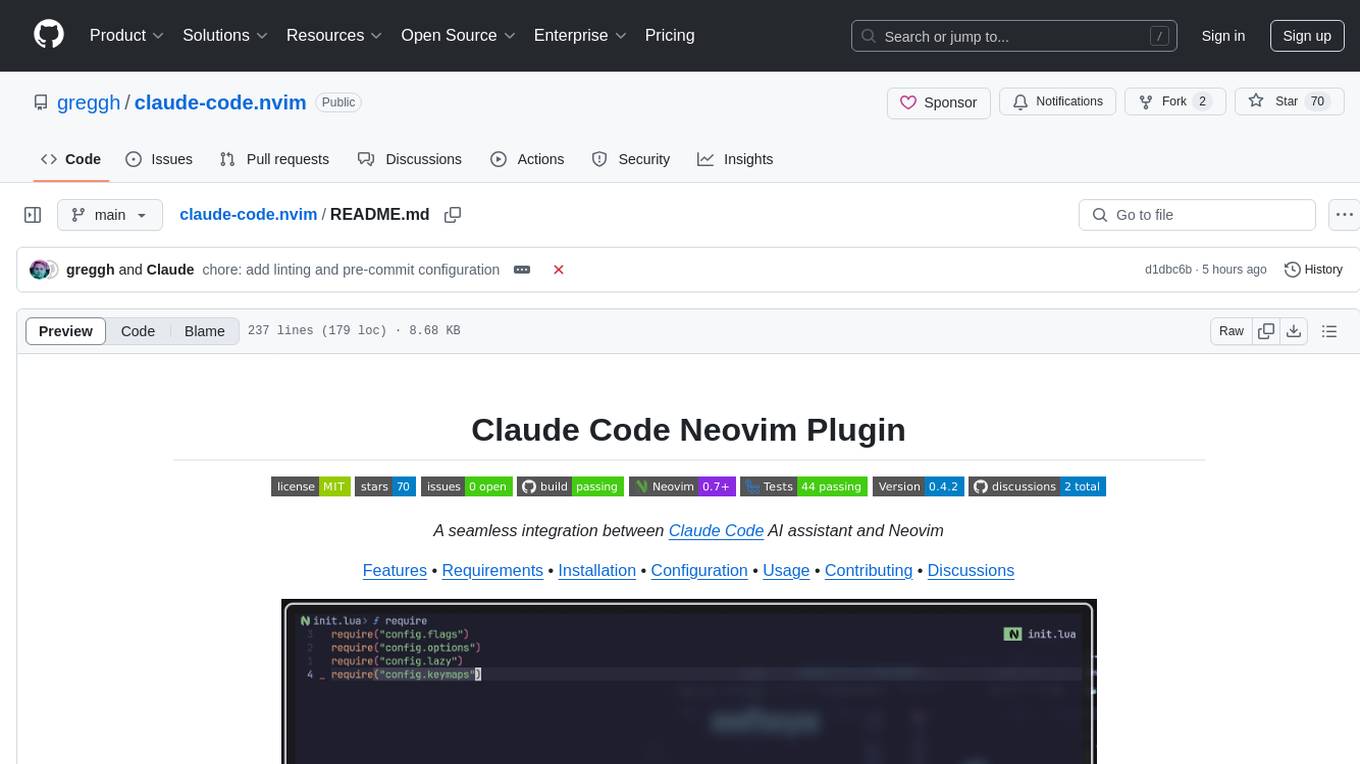
claude-code.nvim
Claude Code Neovim Plugin is a seamless integration between Claude Code AI assistant and Neovim. It allows users to toggle Claude Code in a terminal window with a single key press, automatically detect and reload files modified by Claude Code, provide real-time buffer updates when files are changed externally, offer customizable window position and size, integrate with which-key, use git project root as working directory, maintain a modular code structure, provide type annotations with LuaCATS for better IDE support, offer configuration validation, and include a testing framework for reliability. The plugin creates a terminal buffer running the Claude Code CLI, sets up autocommands to detect file changes on disk, automatically reloads files modified by Claude Code, provides keymaps and commands for toggling the terminal, and detects git repositories to set the working directory to the git root.
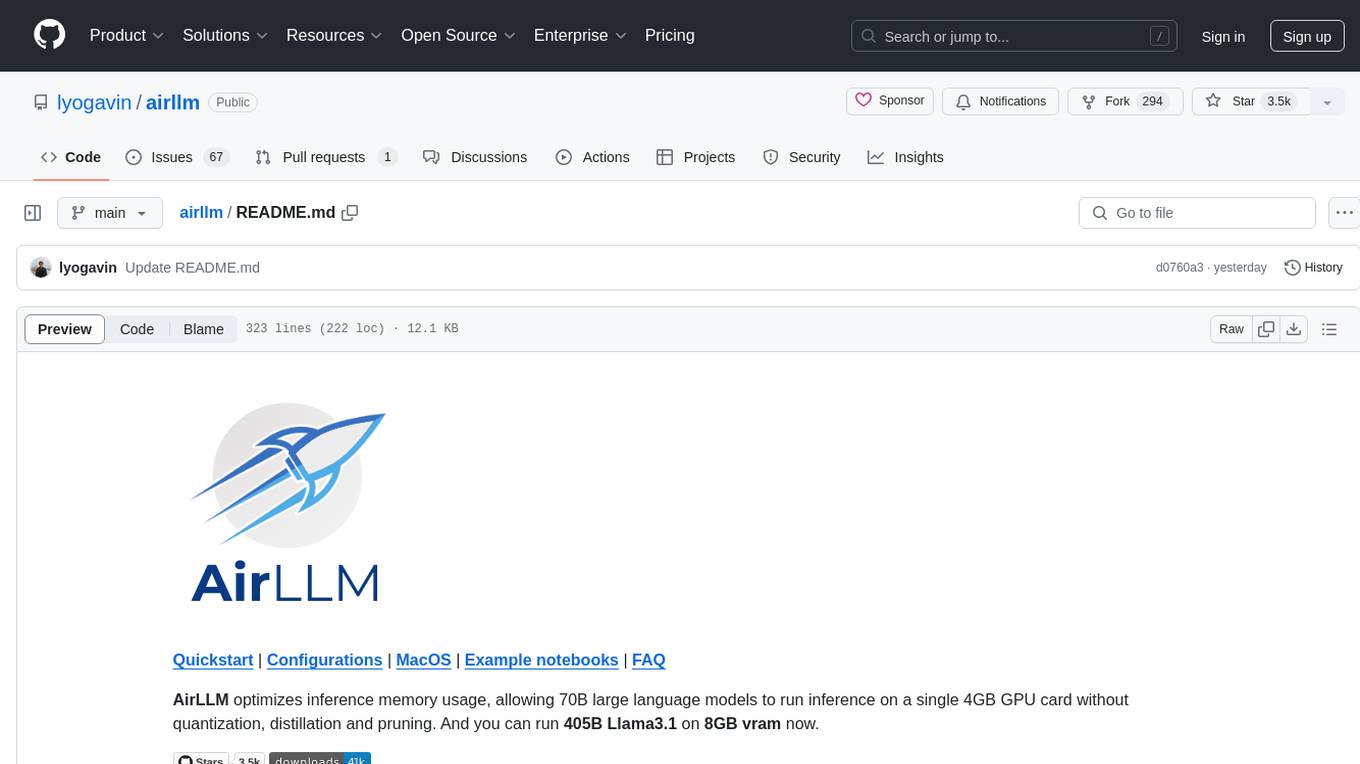
airllm
AirLLM is a tool that optimizes inference memory usage, enabling large language models to run on low-end GPUs without quantization, distillation, or pruning. It supports models like Llama3.1 on 8GB VRAM. The tool offers model compression for up to 3x inference speedup with minimal accuracy loss. Users can specify compression levels, profiling modes, and other configurations when initializing models. AirLLM also supports prefetching and disk space management. It provides examples and notebooks for easy implementation and usage.
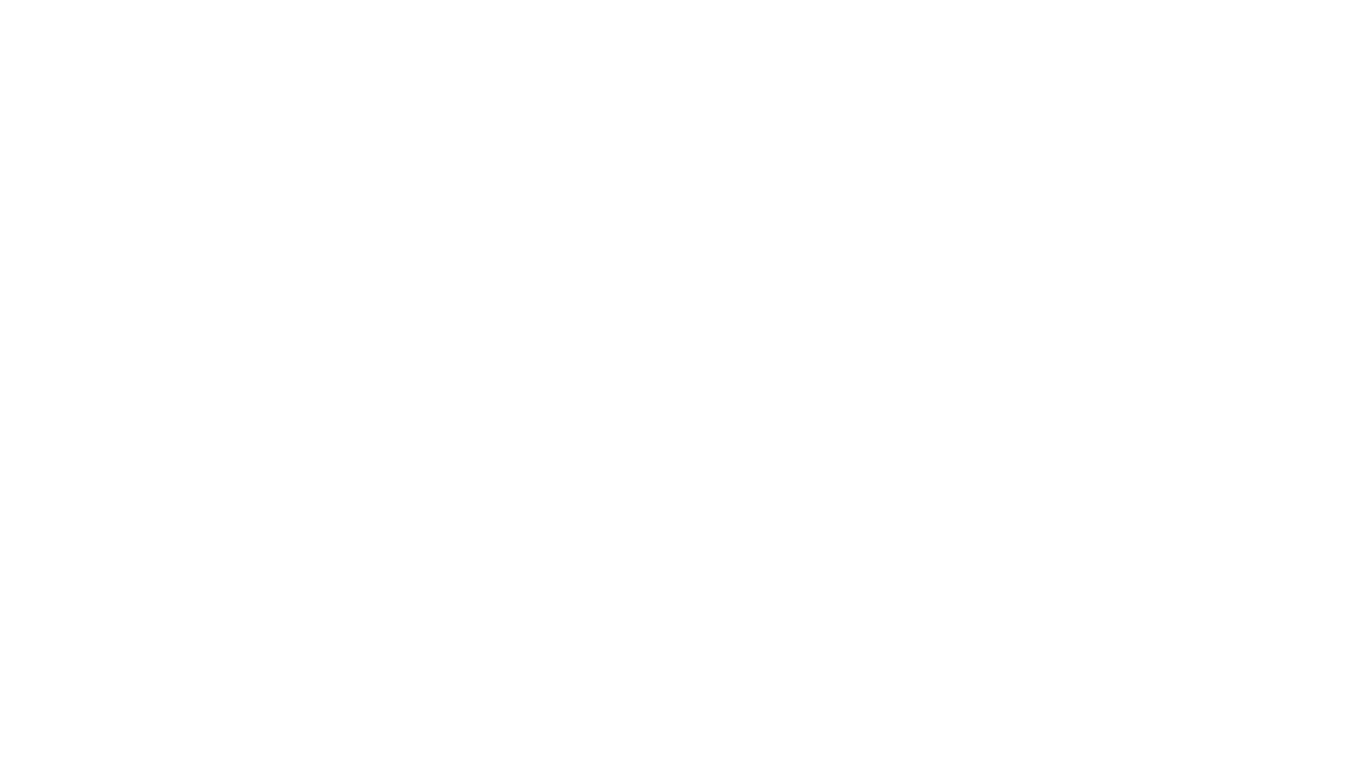
rl
TorchRL is an open-source Reinforcement Learning (RL) library for PyTorch. It provides pytorch and **python-first** , low and high level abstractions for RL that are intended to be **efficient** , **modular** , **documented** and properly **tested**. The code is aimed at supporting research in RL. Most of it is written in python in a highly modular way, such that researchers can easily swap components, transform them or write new ones with little effort.
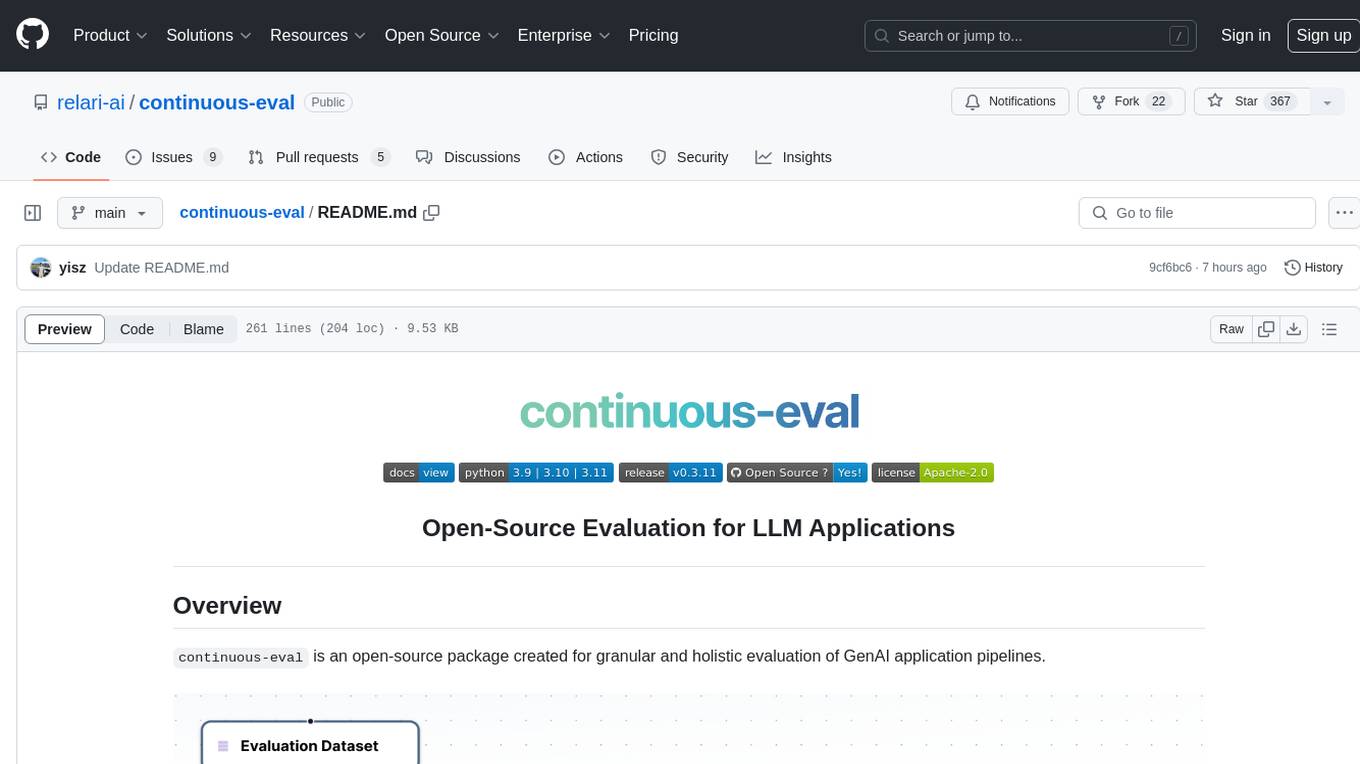
continuous-eval
Open-Source Evaluation for LLM Applications. `continuous-eval` is an open-source package created for granular and holistic evaluation of GenAI application pipelines. It offers modularized evaluation, a comprehensive metric library covering various LLM use cases, the ability to leverage user feedback in evaluation, and synthetic dataset generation for testing pipelines. Users can define their own metrics by extending the Metric class. The tool allows running evaluation on a pipeline defined with modules and corresponding metrics. Additionally, it provides synthetic data generation capabilities to create user interaction data for evaluation or training purposes.
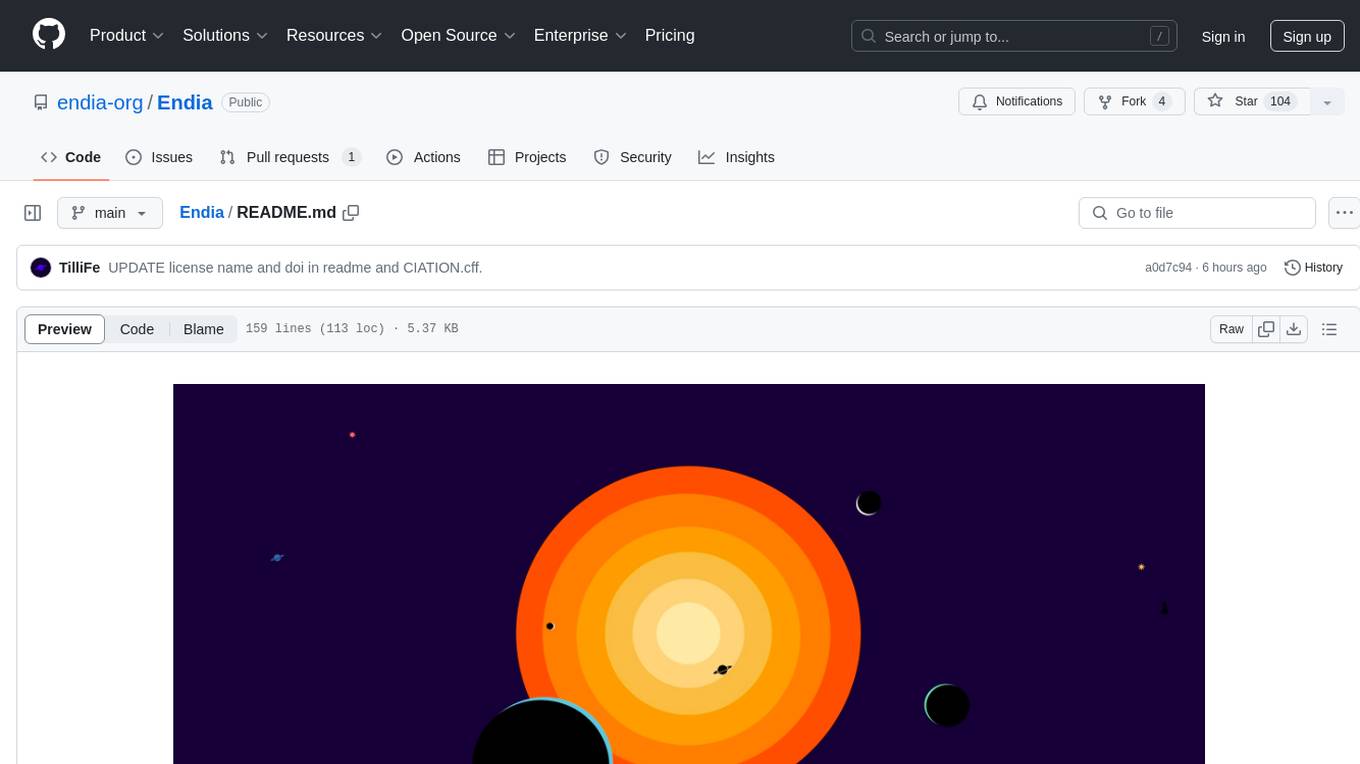
Endia
Endia is a dynamic Array library for Scientific Computing, offering automatic differentiation of arbitrary order, complex number support, dual API with PyTorch-like imperative or JAX-like functional interface, and JIT Compilation for speeding up training and inference. It can handle complex valued functions, perform both forward and reverse-mode automatic differentiation, and has a builtin JIT compiler. Endia aims to advance AI & Scientific Computing by pushing boundaries with clear algorithms, providing high-performance open-source code that remains readable and pythonic, and prioritizing clarity and educational value over exhaustive features.
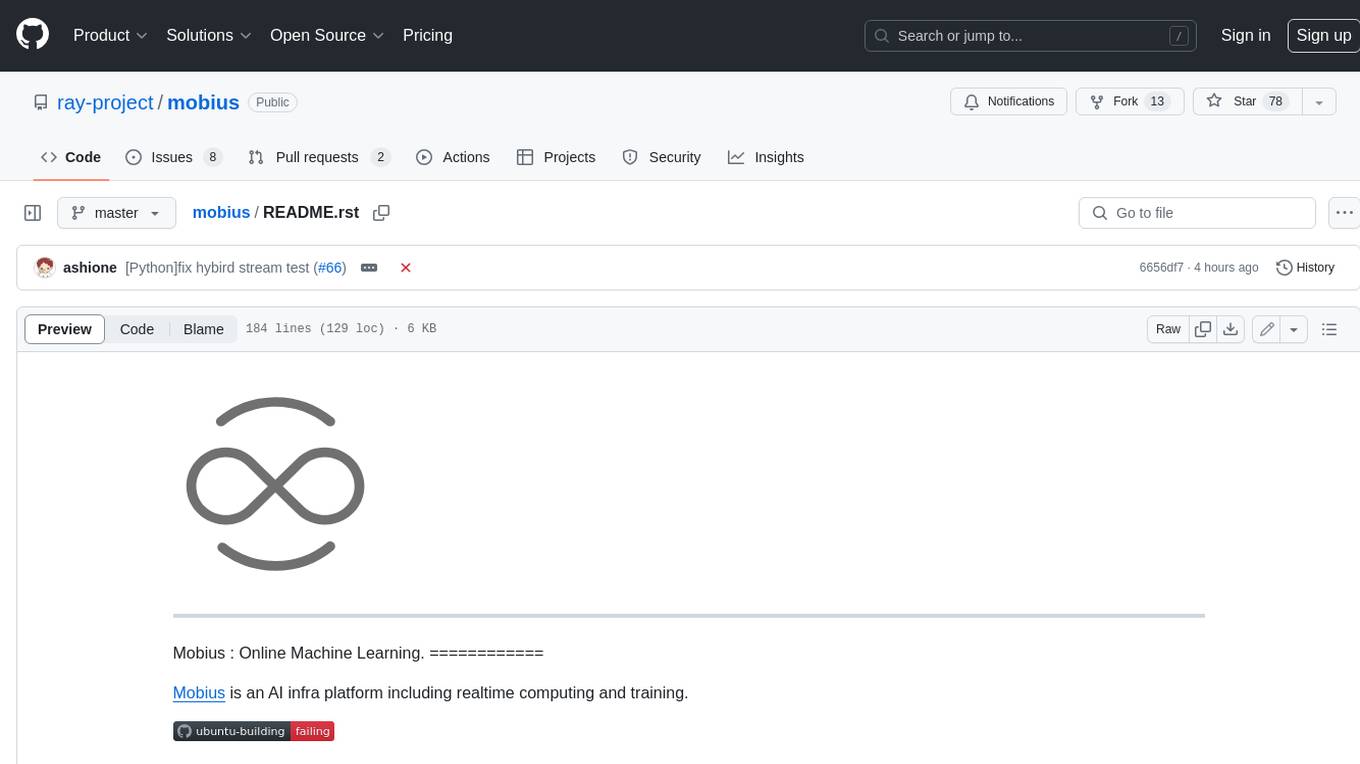
mobius
Mobius is an AI infra platform including realtime computing and training. It is built on Ray, a distributed computing framework, and provides a number of features that make it well-suited for online machine learning tasks. These features include: * **Cross Language**: Mobius can run in multiple languages (only Python and Java are supported currently) with high efficiency. You can implement your operator in different languages and run them in one job. * **Single Node Failover**: Mobius has a special failover mechanism that only needs to rollback the failed node itself, in most cases, to recover the job. This is a huge benefit if your job is sensitive about failure recovery time. * **AutoScaling**: Mobius can generate a new graph with different configurations in runtime without stopping the job. * **Fusion Training**: Mobius can combine TensorFlow/Pytorch and streaming, then building an e2e online machine learning pipeline. Mobius is still under development, but it has already been used to power a number of real-world applications, including: * A real-time recommendation system for a major e-commerce company * A fraud detection system for a large financial institution * A personalized news feed for a major news organization If you are interested in using Mobius for your own online machine learning projects, you can find more information in the documentation.
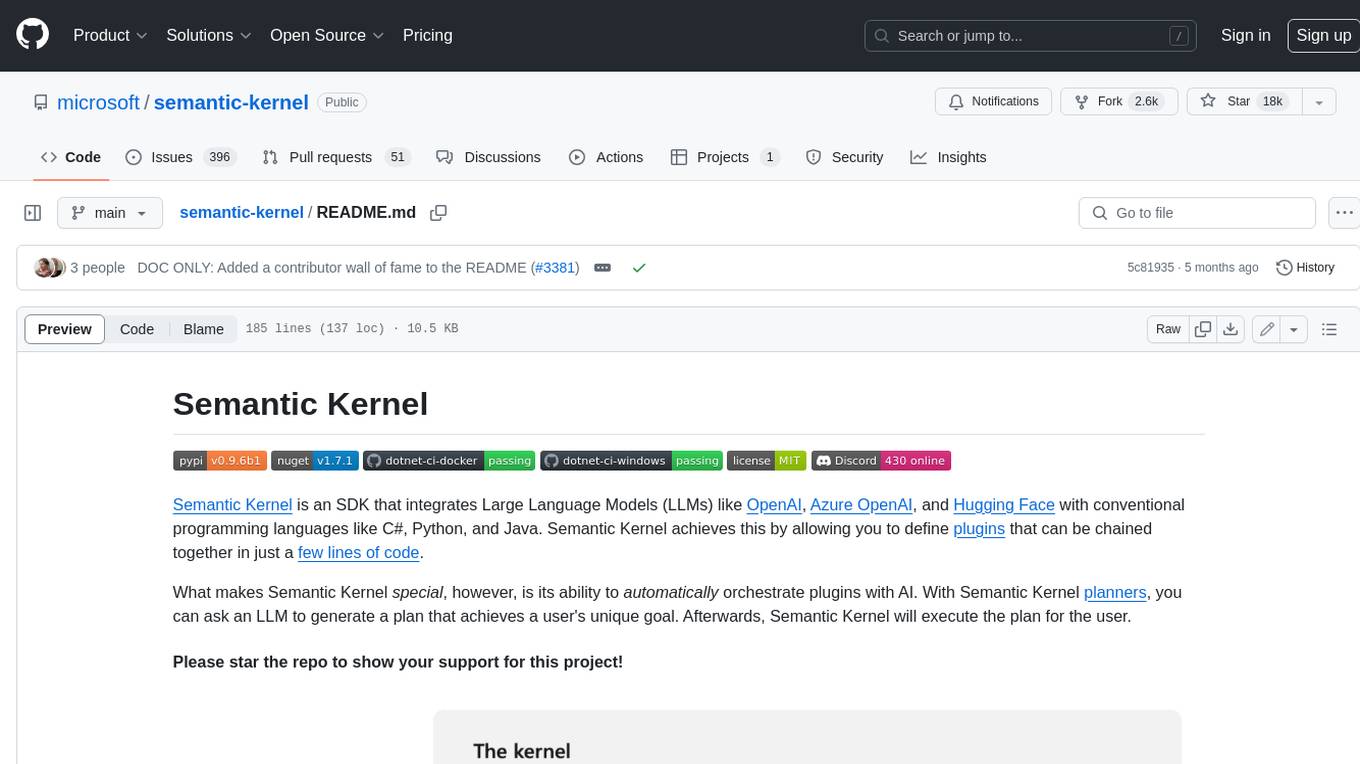
semantic-kernel
Semantic Kernel is an SDK that integrates Large Language Models (LLMs) like OpenAI, Azure OpenAI, and Hugging Face with conventional programming languages like C#, Python, and Java. Semantic Kernel achieves this by allowing you to define plugins that can be chained together in just a few lines of code. What makes Semantic Kernel _special_ , however, is its ability to _automatically_ orchestrate plugins with AI. With Semantic Kernel planners, you can ask an LLM to generate a plan that achieves a user's unique goal. Afterwards, Semantic Kernel will execute the plan for the user.
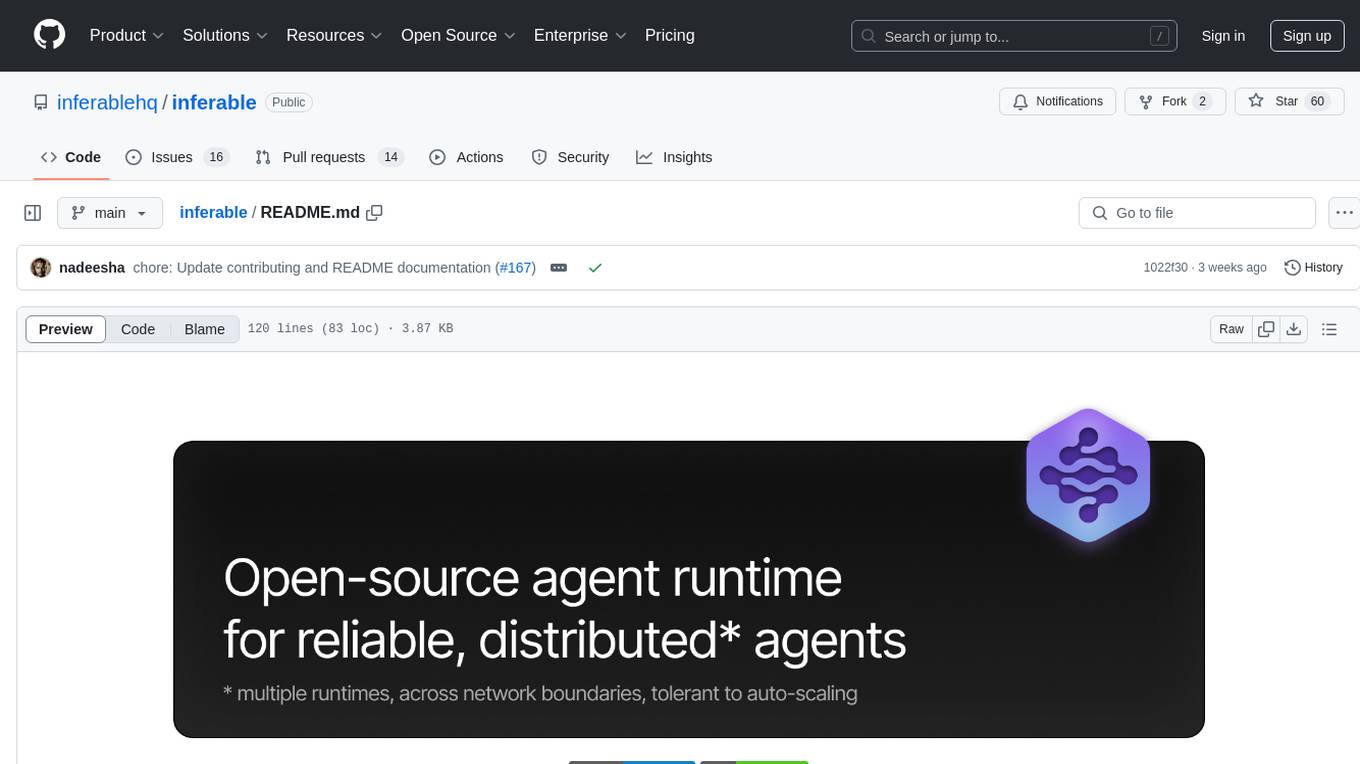
inferable
Inferable is an open source platform that helps users build reliable LLM-powered agentic automations at scale. It offers a managed agent runtime, durable tool calling, zero network configuration, multiple language support, and is fully open source under the MIT license. Users can define functions, register them with Inferable, and create runs that utilize these functions to automate tasks. The platform supports Node.js/TypeScript, Go, .NET, and React, and provides SDKs, core services, and bootstrap templates for various languages.
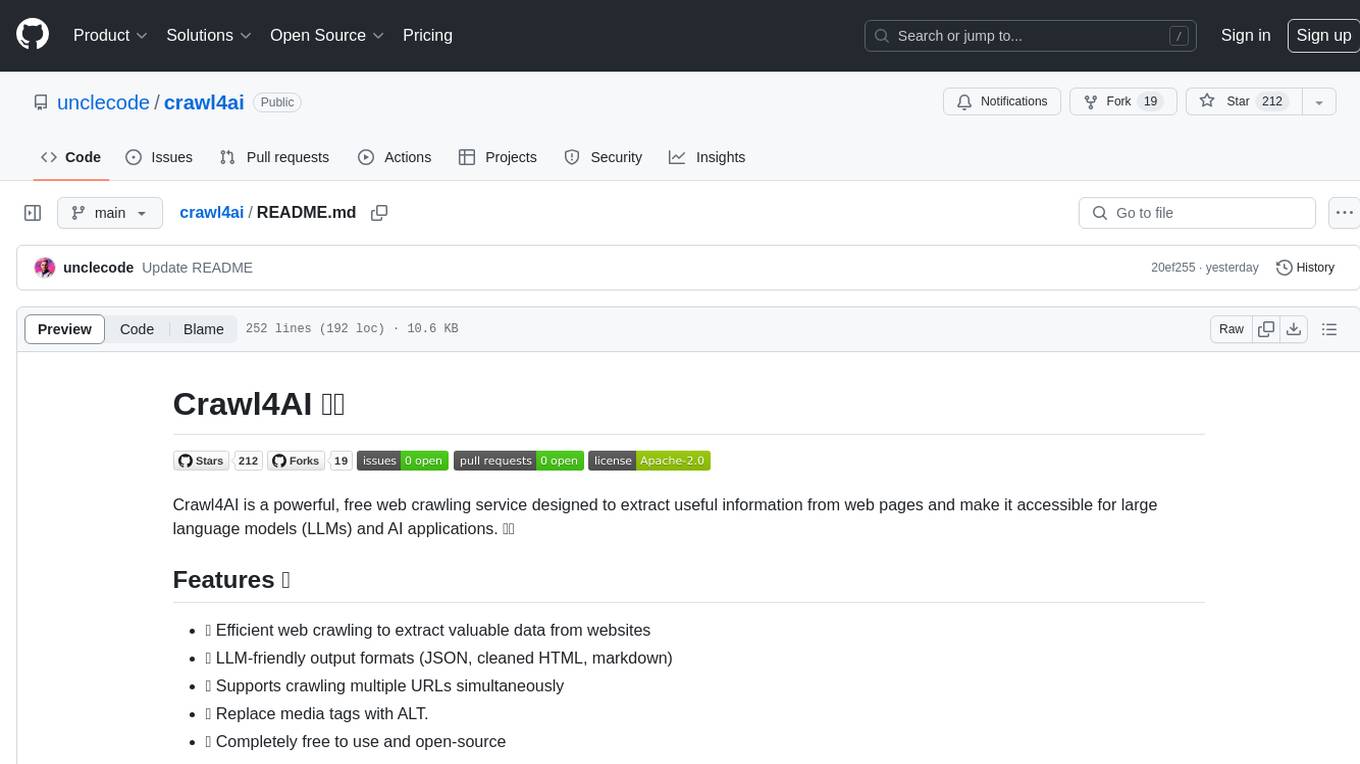
crawl4ai
Crawl4AI is a powerful and free web crawling service that extracts valuable data from websites and provides LLM-friendly output formats. It supports crawling multiple URLs simultaneously, replaces media tags with ALT, and is completely free to use and open-source. Users can integrate Crawl4AI into Python projects as a library or run it as a standalone local server. The tool allows users to crawl and extract data from specified URLs using different providers and models, with options to include raw HTML content, force fresh crawls, and extract meaningful text blocks. Configuration settings can be adjusted in the `crawler/config.py` file to customize providers, API keys, chunk processing, and word thresholds. Contributions to Crawl4AI are welcome from the open-source community to enhance its value for AI enthusiasts and developers.
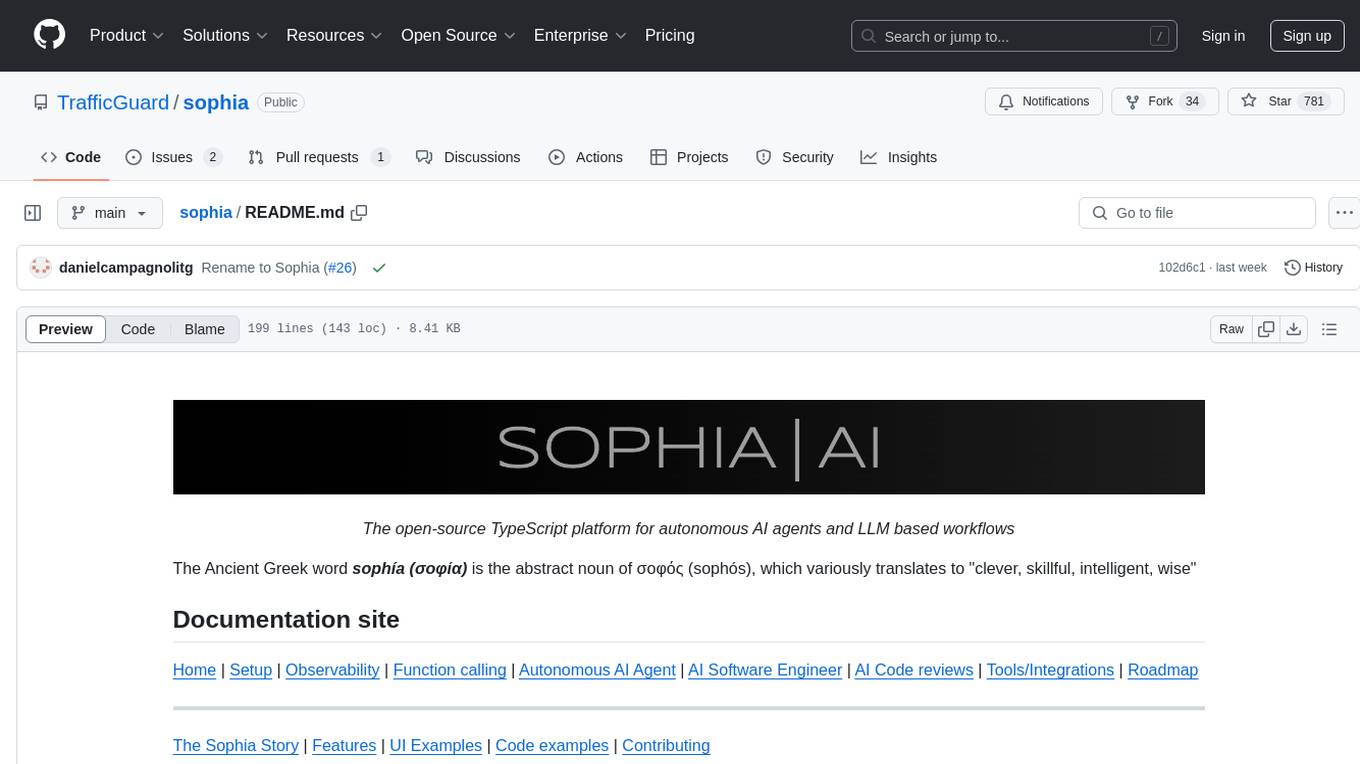
sophia
Sophia is an open-source TypeScript platform designed for autonomous AI agents and LLM based workflows. It aims to automate processes, review code, assist with refactorings, and support various integrations. The platform offers features like advanced autonomous agents, reasoning/planning inspired by Google's Self-Discover paper, memory and function call history, adaptive iterative planning, and more. Sophia supports multiple LLMs/services, CLI and web interface, human-in-the-loop interactions, flexible deployment options, observability with OpenTelemetry tracing, and specific agents for code editing, software engineering, and code review. It provides a flexible platform for the TypeScript community to expand and support various use cases and integrations.
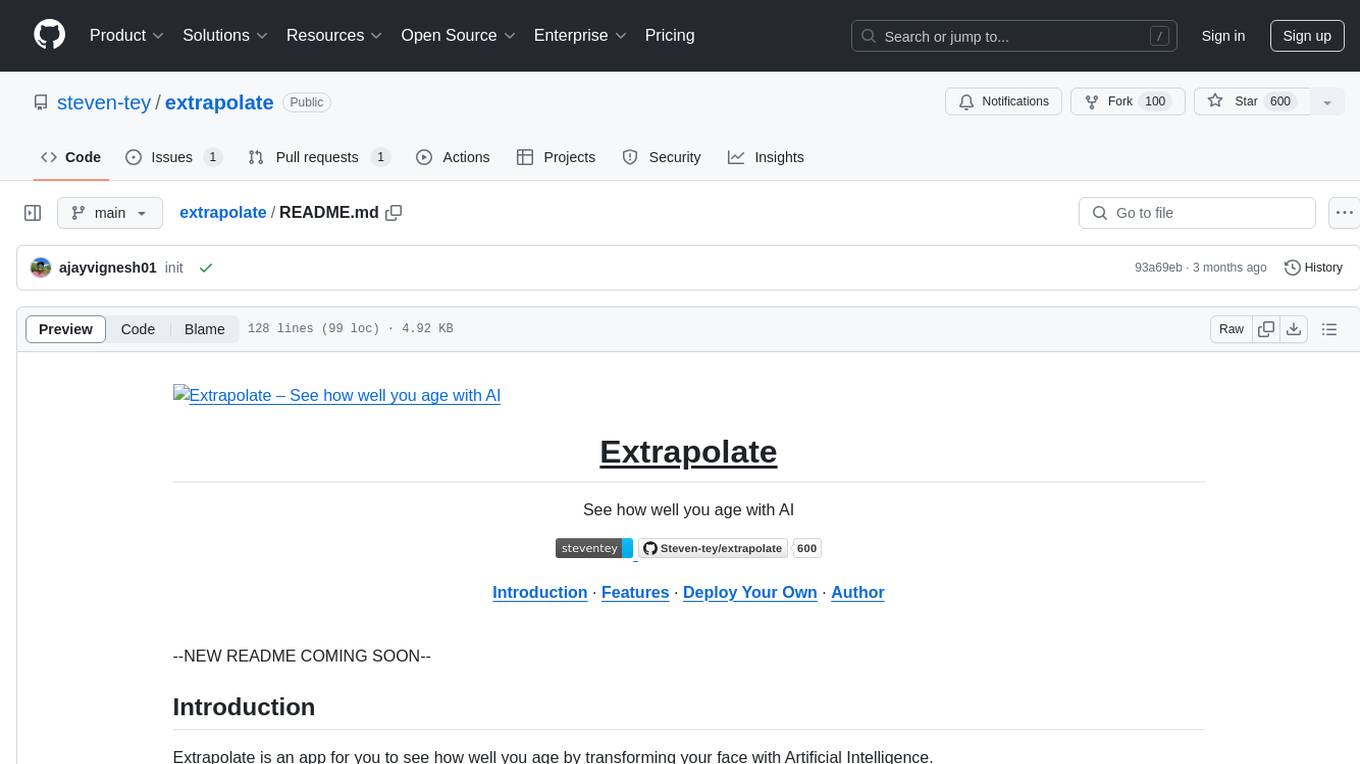
extrapolate
Extrapolate is an app that uses Artificial Intelligence to show you how your face ages over time. It generates a 3-second GIF of your aging face and allows you to store and retrieve photos from Cloudflare R2 using Workers. Users can deploy their own version of Extrapolate on Vercel by setting up ReplicateHQ and Upstash accounts, as well as creating a Cloudflare R2 instance with a Cloudflare Worker to handle uploads and reads. The tool provides a fun and interactive way to visualize the aging process through AI technology.
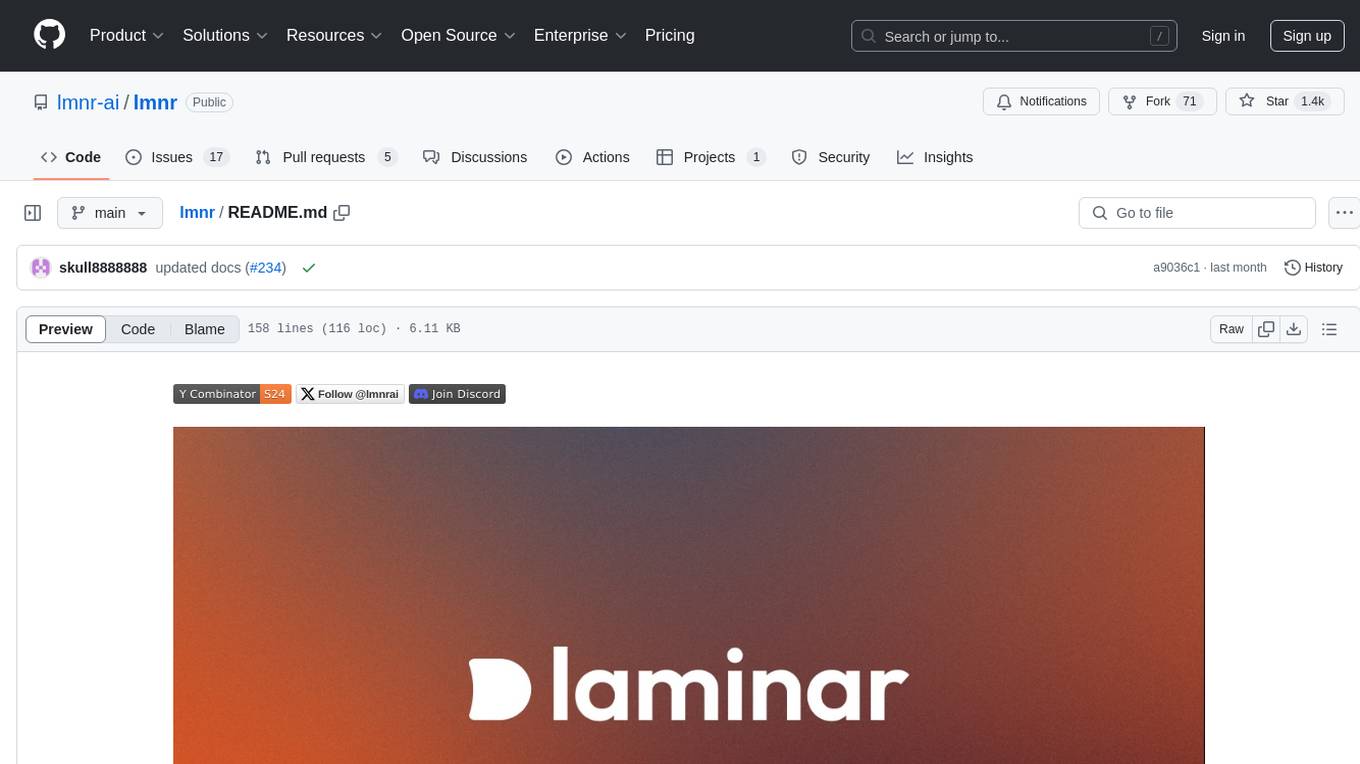
lmnr
Laminar is an all-in-one open-source platform designed for engineering AI products. It allows users to trace, evaluate, label, and analyze LLM data efficiently. The platform offers features such as automatic tracing of common AI frameworks and SDKs, local and online evaluations, simple UI for data labeling, dataset management, and scalability with gRPC communication. Laminar is built with a modern open-source stack including RabbitMQ, Postgres, Clickhouse, and Qdrant for semantic similarity search. It provides fast and beautiful dashboards for traces, evaluations, and labels, making it a comprehensive tool for AI product development.
For similar tasks
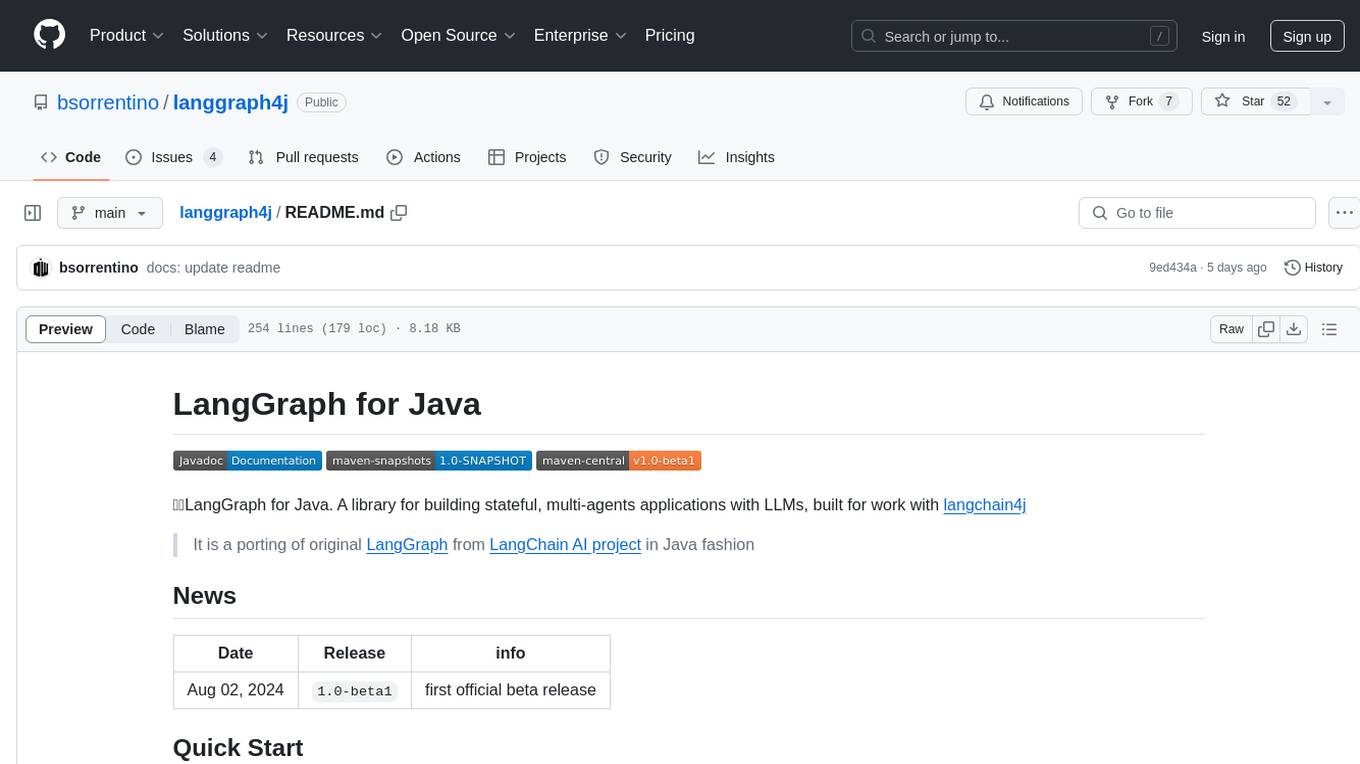
langgraph4j
LangGraph for Java is a library designed for building stateful, multi-agent applications with LLMs. It is a porting of the original LangGraph from the LangChain AI project to Java. The library allows users to define agent states, nodes, and edges in a graph structure to create complex workflows. It integrates with LangChain4j and provides tools for executing actions based on agent decisions. LangGraph for Java enables users to create asynchronous node actions, conditional edges, and normal edges to model decision-making processes in applications.
For similar jobs
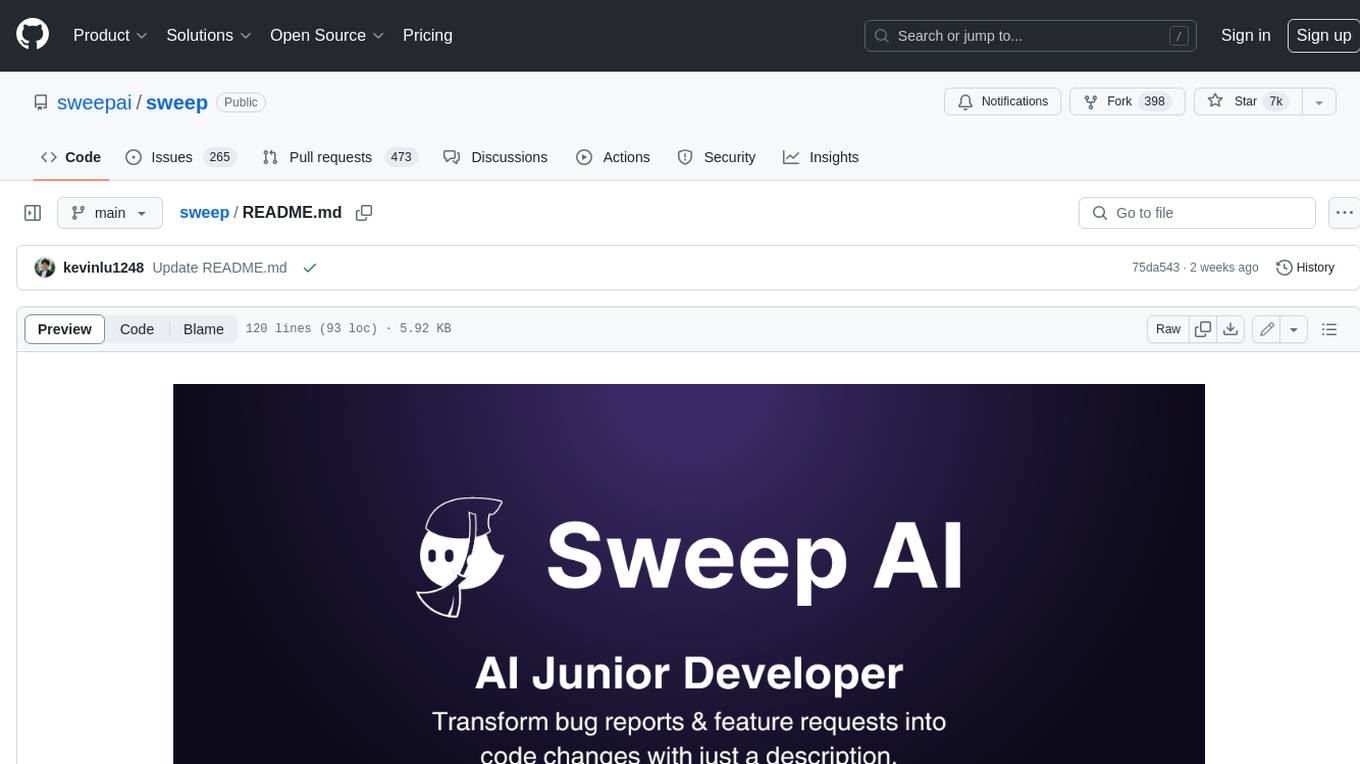
sweep
Sweep is an AI junior developer that turns bugs and feature requests into code changes. It automatically handles developer experience improvements like adding type hints and improving test coverage.
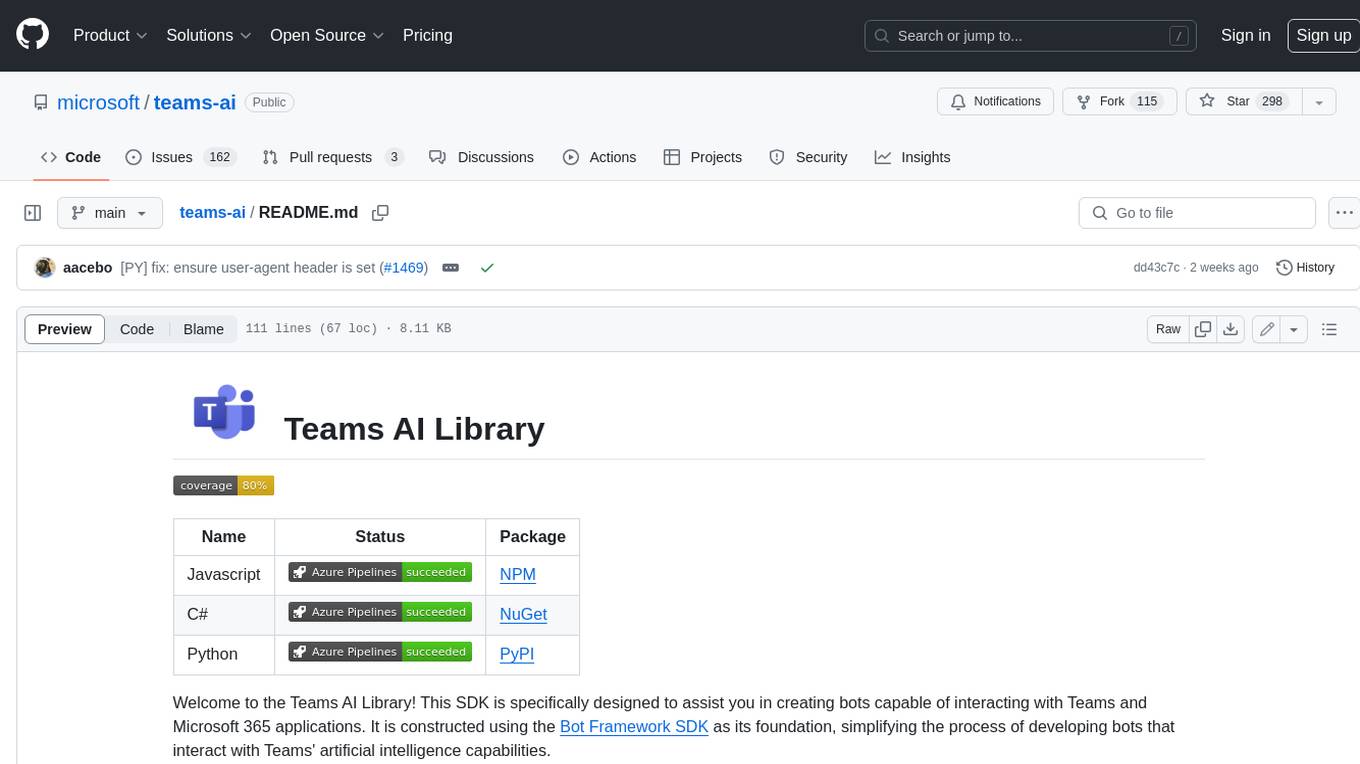
teams-ai
The Teams AI Library is a software development kit (SDK) that helps developers create bots that can interact with Teams and Microsoft 365 applications. It is built on top of the Bot Framework SDK and simplifies the process of developing bots that interact with Teams' artificial intelligence capabilities. The SDK is available for JavaScript/TypeScript, .NET, and Python.
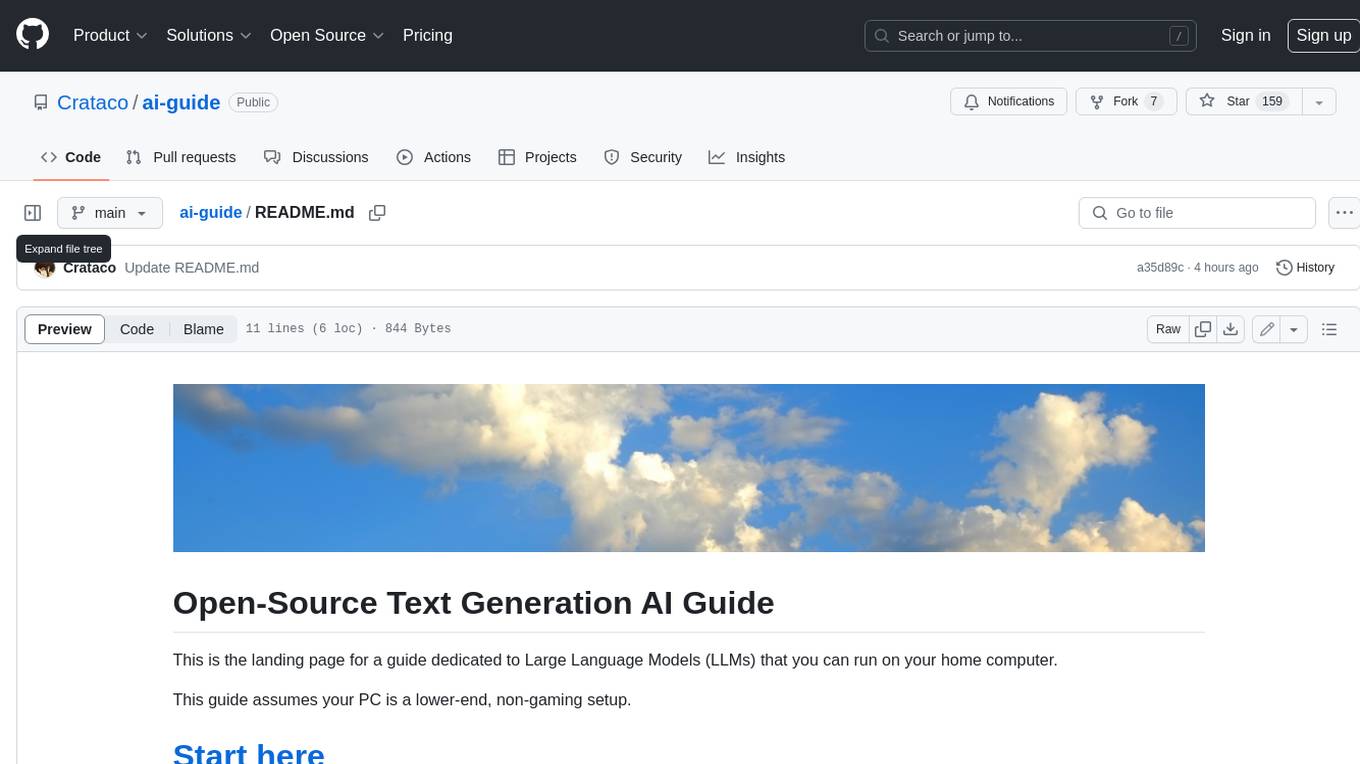
ai-guide
This guide is dedicated to Large Language Models (LLMs) that you can run on your home computer. It assumes your PC is a lower-end, non-gaming setup.
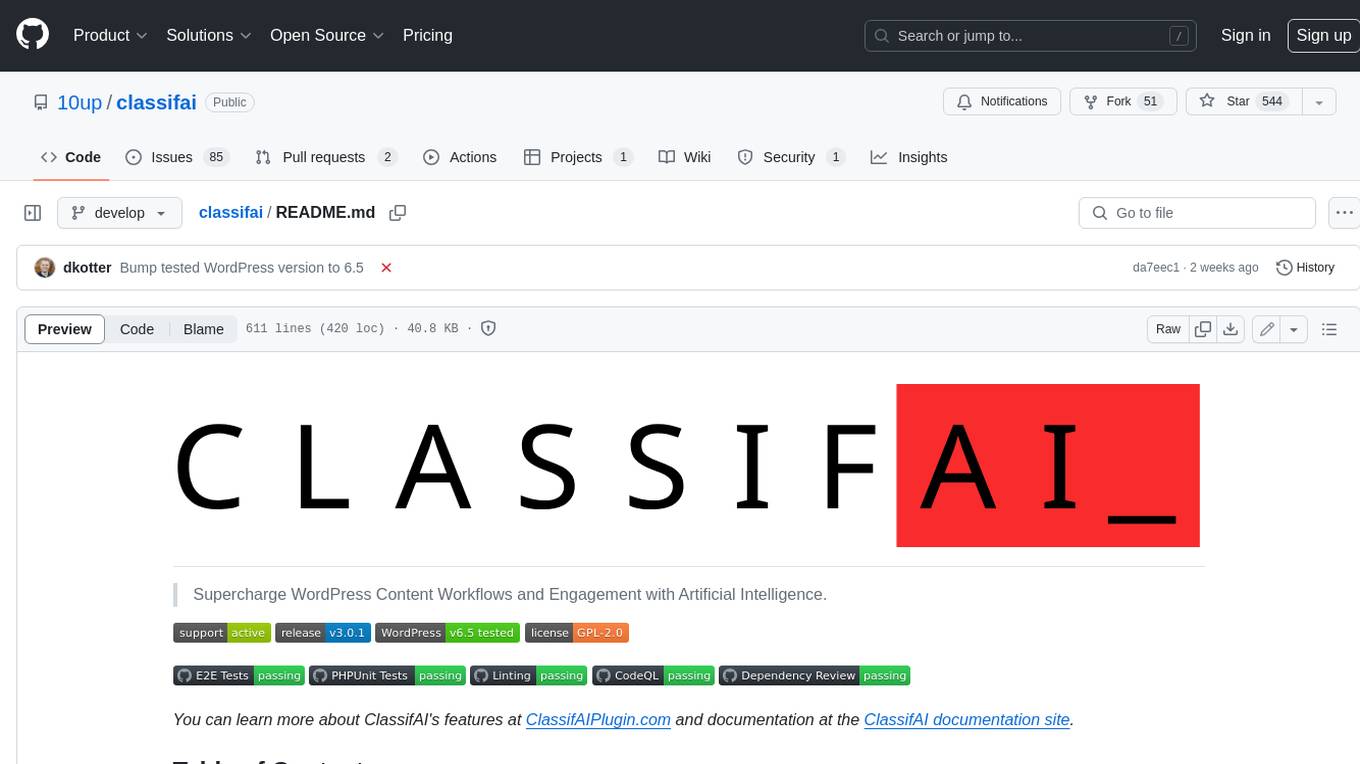
classifai
Supercharge WordPress Content Workflows and Engagement with Artificial Intelligence. Tap into leading cloud-based services like OpenAI, Microsoft Azure AI, Google Gemini and IBM Watson to augment your WordPress-powered websites. Publish content faster while improving SEO performance and increasing audience engagement. ClassifAI integrates Artificial Intelligence and Machine Learning technologies to lighten your workload and eliminate tedious tasks, giving you more time to create original content that matters.
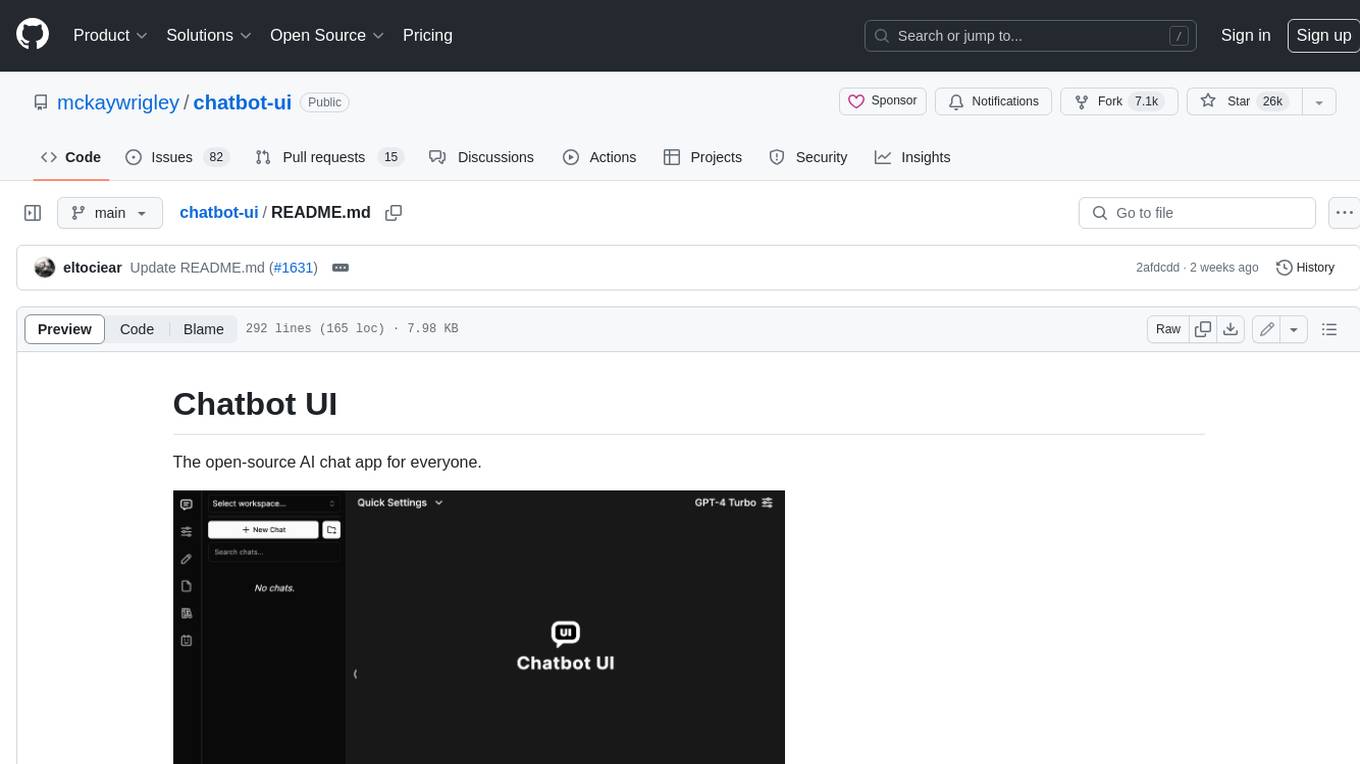
chatbot-ui
Chatbot UI is an open-source AI chat app that allows users to create and deploy their own AI chatbots. It is easy to use and can be customized to fit any need. Chatbot UI is perfect for businesses, developers, and anyone who wants to create a chatbot.
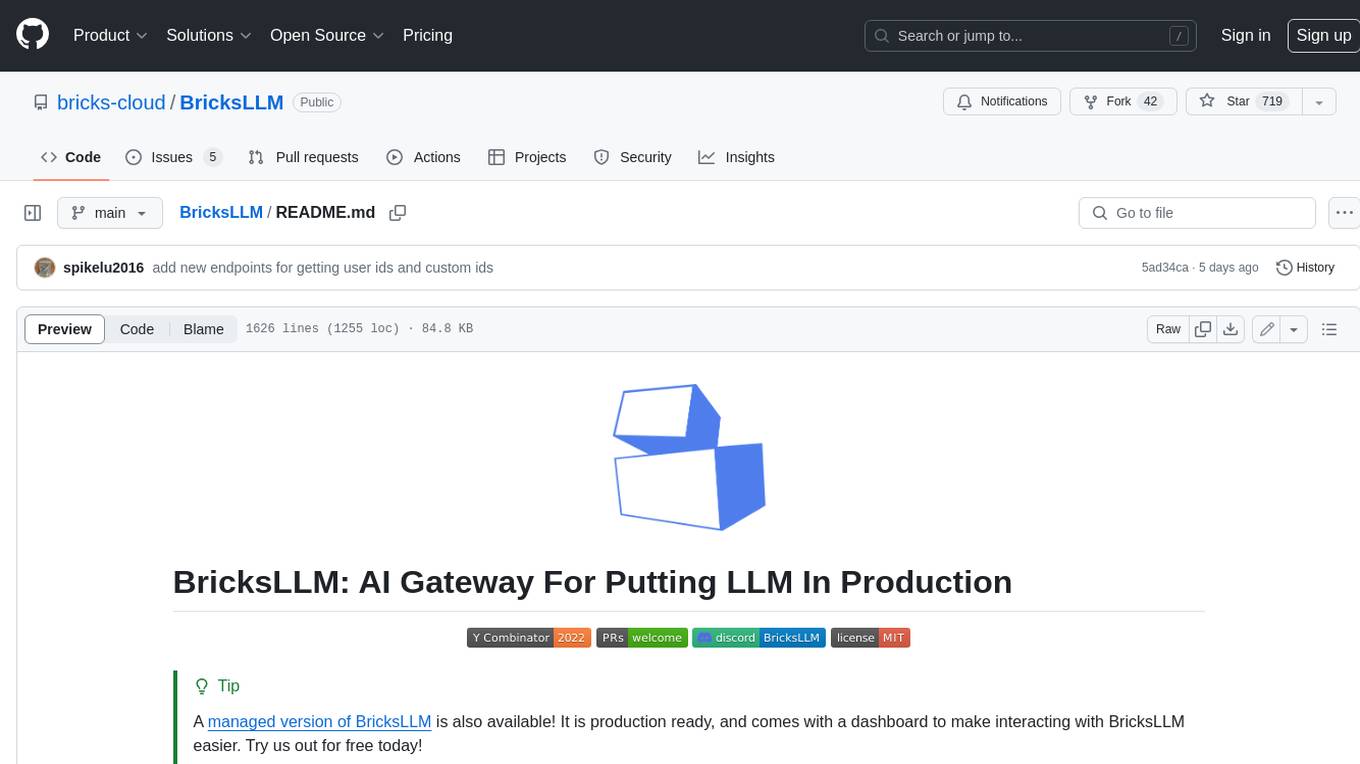
BricksLLM
BricksLLM is a cloud native AI gateway written in Go. Currently, it provides native support for OpenAI, Anthropic, Azure OpenAI and vLLM. BricksLLM aims to provide enterprise level infrastructure that can power any LLM production use cases. Here are some use cases for BricksLLM: * Set LLM usage limits for users on different pricing tiers * Track LLM usage on a per user and per organization basis * Block or redact requests containing PIIs * Improve LLM reliability with failovers, retries and caching * Distribute API keys with rate limits and cost limits for internal development/production use cases * Distribute API keys with rate limits and cost limits for students
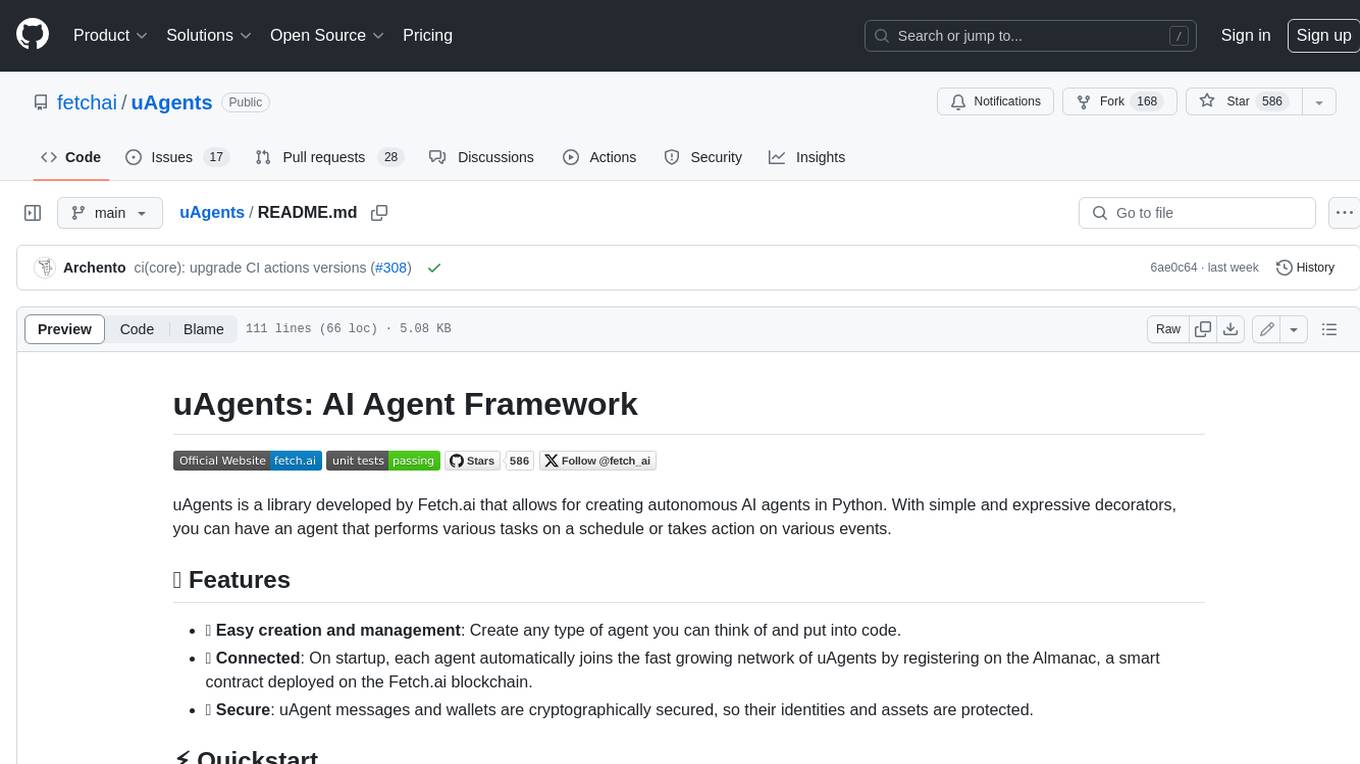
uAgents
uAgents is a Python library developed by Fetch.ai that allows for the creation of autonomous AI agents. These agents can perform various tasks on a schedule or take action on various events. uAgents are easy to create and manage, and they are connected to a fast-growing network of other uAgents. They are also secure, with cryptographically secured messages and wallets.
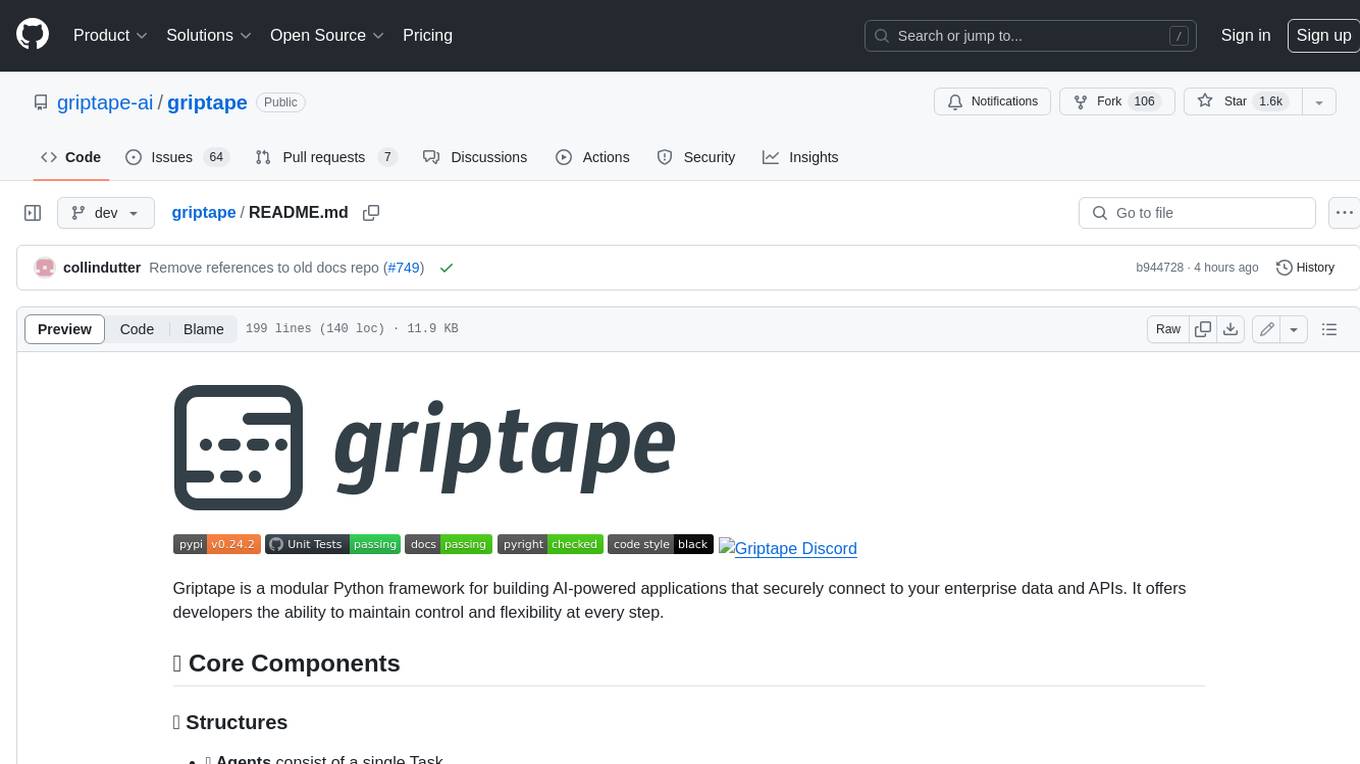
griptape
Griptape is a modular Python framework for building AI-powered applications that securely connect to your enterprise data and APIs. It offers developers the ability to maintain control and flexibility at every step. Griptape's core components include Structures (Agents, Pipelines, and Workflows), Tasks, Tools, Memory (Conversation Memory, Task Memory, and Meta Memory), Drivers (Prompt and Embedding Drivers, Vector Store Drivers, Image Generation Drivers, Image Query Drivers, SQL Drivers, Web Scraper Drivers, and Conversation Memory Drivers), Engines (Query Engines, Extraction Engines, Summary Engines, Image Generation Engines, and Image Query Engines), and additional components (Rulesets, Loaders, Artifacts, Chunkers, and Tokenizers). Griptape enables developers to create AI-powered applications with ease and efficiency.