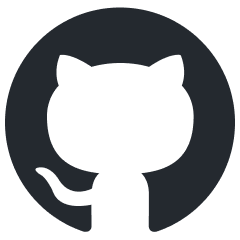
DB-GPT
An LLM Based Diagnosis System (https://arxiv.org/pdf/2312.01454.pdf)
Stars: 480
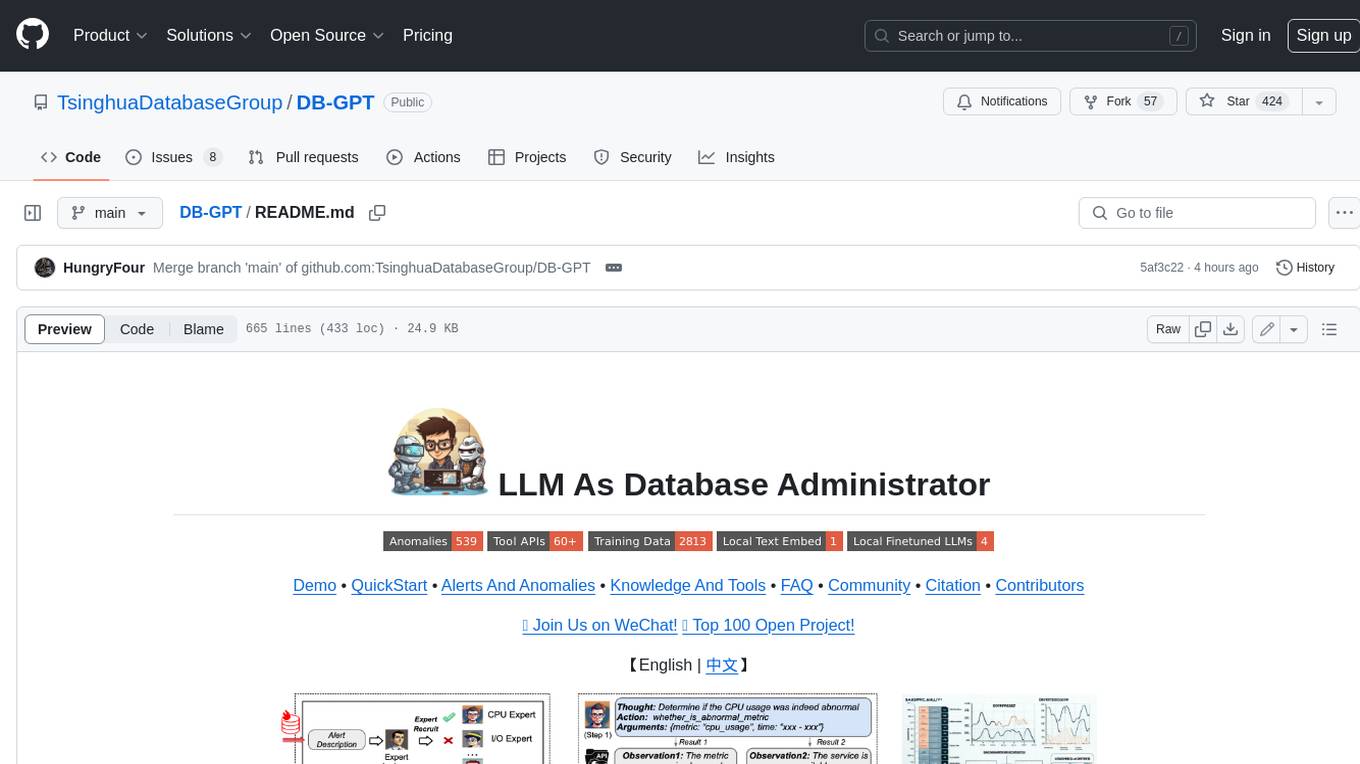
DB-GPT is a personal database administrator that can solve database problems by reading documents, using various tools, and writing analysis reports. It is currently undergoing an upgrade. **Features:** * **Online Demo:** * Import documents into the knowledge base * Utilize the knowledge base for well-founded Q&A and diagnosis analysis of abnormal alarms * Send feedbacks to refine the intermediate diagnosis results * Edit the diagnosis result * Browse all historical diagnosis results, used metrics, and detailed diagnosis processes * **Language Support:** * English (default) * Chinese (add "language: zh" in config.yaml) * **New Frontend:** * Knowledgebase + Chat Q&A + Diagnosis + Report Replay * **Extreme Speed Version for localized llms:** * 4-bit quantized LLM (reducing inference time by 1/3) * vllm for fast inference (qwen) * Tiny LLM * **Multi-path extraction of document knowledge:** * Vector database (ChromaDB) * RESTful Search Engine (Elasticsearch) * **Expert prompt generation using document knowledge** * **Upgrade the LLM-based diagnosis mechanism:** * Task Dispatching -> Concurrent Diagnosis -> Cross Review -> Report Generation * Synchronous Concurrency Mechanism during LLM inference * **Support monitoring and optimization tools in multiple levels:** * Monitoring metrics (Prometheus) * Flame graph in code level * Diagnosis knowledge retrieval (dbmind) * Logical query transformations (Calcite) * Index optimization algorithms (for PostgreSQL) * Physical operator hints (for PostgreSQL) * Backup and Point-in-time Recovery (Pigsty) * **Continuously updated papers and experimental reports** This project is constantly evolving with new features. Don't forget to star β and watch π to stay up to date.
README:
Demo β’ QuickStart β’ Alerts And Anomalies β’ Knowledge And Tools β’ Dockers β’ FAQ β’ Community β’ Citation β’ Contributors β’ OpenAI, Azure aggregated API discounted access plan.
π« Join Us on WeChat! π Top 100 Open Project! π VLDB 2024!
γEnglish | δΈζγ
π¦Ύ Build your personal database administrator (D-Bot)π§βπ», which is good at solving database problems by reading documents, using various tools, writing analysis reports! Undergoing An Upgrade!
- After launching the local service (adopting frontend and configs from Chatchat), you can easily import documents into the knowledge base, utilize the knowledge base for well-founded Q&A and diagnosis analysis of abnormal alarms.
- With the user feedback function π, you can (1) send feedbacks to make D-Bot follow and refine the intermediate diagnosis results, and (2) edit the diagnosis result by clicking the βEditβ button. D-Bot can accumulate refinement patterns from the user feedbacks (stored in vector database) and adaptively align to user's diagnosis preference.
- On the online website (http://dbgpt.dbmind.cn), you can browse all historical diagnosis results, used metrics, and detailed diagnosis processes.
Old Version 1: [Gradio for Diag Game] (no langchain)
Old Version 2: [Vue for Report Replay] (no langchain)
-
[ ] Docker for a quick and safe use of D-Bot
-
[x] Metric Monitoring (prometheus), Database (postgres_db), Alert (alertmanager) and Alert Recording (python_app).
-
[ ] D-bot (still too large, with over 12GB)
-
-
[ ] Human Feedback π₯π₯π₯
-
[x] Test-based Diagnosis Refinement with User Feedbacks
-
[x] Refinement Patterns Extraction & Management
-
-
[ ] Language Support (english / chinese)
- [x] english : default
- [x] chinese : add "language: zh" in config.yaml
-
[ ] New Frontend
- [x] Knowledgebase + Chat Q&A + Diagnosis + Report Replay
-
[x] Result Report with reference
-
[ ] Extreme Speed Version for localized llms
-
[x] 4-bit quantized LLM (reducing inference time by 1/3)
-
[x] vllm for fast inference (qwen)
-
[ ] Tiny LLM
-
-
[x] Multi-path extraction of document knowledge
-
[x] Vector database (ChromaDB)
-
[x] RESTful Search Engine (Elasticsearch)
-
-
[x] Expert prompt generation using document knowledge
-
[ ] Upgrade the LLM-based diagnosis mechanism:
-
[x] Task Dispatching -> Concurrent Diagnosis -> Cross Review -> Report Generation
-
[ ] Synchronous Concurrency Mechanism during LLM inference
-
-
[ ] Support monitoring and optimization tools in multiple levels π link
- [x] Monitoring metrics (Prometheus)
- [ ] Flame graph in code level
- [x] Diagnosis knowledge retrieval (dbmind)
- [x] Logical query transformations (Calcite)
- [x] Index optimization algorithms (for PostgreSQL)
- [x] Physical operator hints (for PostgreSQL)
- [ ] Backup and Point-in-time Recovery (Pigsty)
-
[x] Papers and experimental reports are continuously updated
This project is evolving with new features π«π«
Don't forget to star β and watch π to stay up to date :)
- First, ensure that your machine has Python (>= 3.10) installed.
$ python --version
Python 3.10.12
- Next, create a virtual environment and install the dependencies for the project within it.
# Clone the repository
$ git clone https://github.com/TsinghuaDatabaseGroup/DB-GPT.git
# Enter the directory
$ cd DB-GPT
# Install all dependencies
$ pip3 install -r requirements.txt
$ pip3 install -r requirements_api.txt # If only running the API, you can just install the API dependencies, please use requirements_api.txt
# Default dependencies include the basic runtime environment (Chroma-DB vector library). If you want to use other vector libraries, please uncomment the respective dependencies in requirements.txt before installation.
If fail to install google-colab, try conda install -c conda-forge google-colab
-
PostgreSQL v12 (We have developed and tested based on PostgreSQL v12, we do not guarantee compatibility with other versions of PostgreSQL)
Ensure your database supports remote connections (link)
Moreover, install extensions like pg_stat_statements (track frequent queries), pg_hint_plan (optimize physical operators), and hypopg (create hypothetical indexes).
Note pg_stat_statements accumulates query statistics over time. Therefore, you need to regularly clear the statistics: 1) to discard all statistics, execute "SELECT pg_stat_statements_reset();"; 2) to discard statistics for a specific query, execute "SELECT pg_stat_statements_reset(userid, dbid, queryid);".
-
(optional) If you need to run this project locally or in an offline environment, you first need to download the required models to your local machine and then correctly adapt some configurations.
- Download the model parameters of Sentence Trasformer
Create a new directory ./multiagents/localized_llms/sentence_embedding/
Place the downloaded sentence-transformer.zip in the ./multiagents/localized_llms/sentence_embedding/ directory; unzip the archive.
- Download LLM and embedding models from HuggingFace.
To download models, first install Git LFS, then run
$ git lfs install
$ git clone https://huggingface.co/moka-ai/m3e-base
$ git clone https://huggingface.co/Qwen/Qwen-1_8B-Chat
- Adapt the model configuration to the download model paths, e.g.,
EMBEDDING_MODEL = "m3e-base"
LLM_MODELS = ["Qwen-1_8B-Chat"]
MODEL_PATH = {
"embed_model": {
"m3e-base": "m3e-base", # Download path of embedding model.
},
"llm_model": {
"Qwen-1_8B-Chat": "Qwen-1_8B-Chat", # Download path of LLM.
},
}
- Download and config localized LLMs.
- Ensure that your machine has Node (>= 18.15.0)
$ node -v
v18.15.0
Install pnpm and dependencies
cd webui
# pnpm address https://pnpm.io/zh/motivation
# install dependency(Recommend use pnpm)
# you can use "npm -g i pnpm" to install pnpm
pnpm install
Copy the configuration files
$ python copy_config_example.py
# The generated configuration files are in the configs/ directory
# basic_config.py is the basic configuration file, no modification needed
# diagnose_config.py is the diagnostic configuration file, needs to be modified according to your environment.
# kb_config.py is the knowledge base configuration file, you can modify DEFAULT_VS_TYPE to specify the storage vector library of the knowledge base, or modify related paths.
# model_config.py is the model configuration file, you can modify LLM_MODELS to specify the model used, the current model configuration is mainly for knowledge base search, diagnostic related models are still hardcoded in the code, they will be unified here later.
# prompt_config.py is the prompt configuration file, mainly for LLM dialogue and knowledge base prompts.
# server_config.py is the server configuration file, mainly for server port numbers, etc.
!!! Attention, please modify the following configurations before initializing the knowledge base, otherwise, it may cause the database initialization to fail.
- model_config.py
# EMBEDDING_MODEL Vectorization model, if choosing a local model, it needs to be downloaded to the root directory as required.
# LLM_MODELS LLM, if choosing a local model, it needs to be downloaded to the root directory as required.
# ONLINE_LLM_MODEL If using an online model, you need to modify the configuration.
- server_config.py
# WEBUI_SERVER.api_base_url Pay attention to this parameter, if deploying the project on a server, then you need to modify the configuration.
- In diagnose_config.py, we set config.yaml as the default LLM expert configuration file.
DIAGNOSTIC_CONFIG_FILE = "config.yaml"
- To enable interactive diagnosis refinement with user feedbacks, you can set
DIAGNOSTIC_CONFIG_FILE = "config_feedback.yaml"
- To enable diagnosis in Chinese with Qwen, you can set
DIAGNOSTIC_CONFIG_FILE = "config_qwen.yaml"
- Initialize the knowledge base
$ python init_database.py --recreate-vs
Start the project with the following commands
$ python startup.py -a
If started correctly, you will see the following interface
- FastAPI Docs Interface
- Web UI Launch Interface Examples:
- Web UI Knowledge Base Management PageοΌ
- Web UI Conversation Interface:
- Web UI UI Diagnostic PageοΌ
Save time by trying out the docker deployment.
-
(optional) Enable slow query log in PostgreSQL (link)
(1) For "systemctl restart postgresql", the service name can be different (e.g., postgresql-12.service);
(2) Use absolute log path name like "log_directory = '/var/lib/pgsql/12/data/log'";
(3) Set "log_line_prefix = '%m [%p] [%d]'" in postgresql.conf (to record the database names of different queries).
-
(optional) Prometheus
Check prometheus.md for detailed installation guides.
We put multiple test cases under the test_case folder. You can select a case file on the front-end page for diagnosis or use the command line.
python3 run_diagnose.py --anomaly_file ./test_cases/testing_cases_5.json --config_file config.yaml
Check out how to deploy prometheus and alertmanager in prometheus_service_docker.
- You can also choose to quickly put your hands on by using our docker (docker deployment)
We provide scripts that trigger typical anomalies (anomalies directory) using highly concurrent operations (e.g., inserts, deletes, updates) in combination with specific test benches.
Single Root Cause Anomalies:
Execute the following command to trigger a single type of anomaly with customized parameters:
python anomaly_trigger/main.py --anomaly MISSING_INDEXES --threads 100 --ncolumn 20 --colsize 100 --nrow 20000
Parameters:
-
--anomaly
: Specifies the type of anomaly to trigger. -
--threads
: Sets the number of concurrent clients. -
--ncolumn
: Defines the number of columns. -
--colsize
: Determines the size of each column (in bytes). -
--nrow
: Indicates the number of rows.
Multiple Root Cause Anomalies:
To trigger anomalies caused by multiple factors, use the following command:
python anomaly_trigger/multi_anomalies.py
Modify the script as needed to simulate different types of anomalies.
Check detailed use cases at http://dbgpt.dbmind.cn.
Click to check 29 typical anomalies together with expert analysis (supported by the DBMind team)
(Basic version by Zui Chen)
(1) If you only need simple document splitting, you can directly use the document import function in the "Knowledge Base Management Page".
(2) We require the document itself to have chapter format information, and currently only support the docx format.
Step 1. Configure the ROOT_DIR_NAME path in ./doc2knowledge/doc_to_section.py and store all docx format documents in ROOT_DIR_NAME.
Step 2. Configure OPENAI_KEY.
export OPENAI_API_KEY=XXXXX
Step 3. Split the document into separate chapter files by chapter index.
cd doc2knowledge/
python doc_to_section.py
Step 4. Modify parameters in the doc2knowledge.py script and run the script:
python doc2knowledge.py
Step 5. With the extracted knowledge, you can visualize their clustering results:
python knowledge_clustering.py
-
Tool APIs (for optimization)
Module Functions index_selection (equipped) heuristic algorithm query_rewrite (equipped) 45 rules physical_hint (equipped) 15 parameters For functions within [query_rewrite, physical_hint], you can use api_test.py script to verify the effectiveness.
If the function actually works, append it to the api.py of corresponding module.
We utilize db2advis heuristic algorithm to recommend indexes for given workloads. The function api is optimize_index_selection.
You can use docker for a quick and safe use of the monitoring platform and database.
Refer to tutorials (e.g., on CentOS) for installing Docker and Docoker-Compose.
We use docker-compose to build and manage multiple dockers for metric monitoring (prometheus), alert (alertmanager), database (postgres_db), and alert recording (python_app).
cd prometheus_service_docker
docker-compose -p prometheus_service -f docker-compose.yml up --build
Next time starting the prometheus_service, you can directly execute "docker-compose -p prometheus_service -f docker-compose.yml up" without building the dockers.
Configure the settings in anomaly_trigger/utils/database.py (e.g., replace "host" with the IP address of the server) and execute an anomaly generation command, like:
cd anomaly_trigger
python3 main.py --anomaly MISSING_INDEXES --threads 100 --ncolumn 20 --colsize 100 --nrow 20000
You may need to modify the arugment values like "--threads 100" if no alert is recorded after execution.
After receiving a request sent to http://127.0.0.1:8023/alert from prometheus_service, the alert summary will be recorded in prometheus_and_db_docker/alert_history.txt, like:
This way, you can use the alert marked as `resolved' as a new anomaly (under the ./diagnostic_files directory) for diagnosis by d-bot.
π€¨ The '.sh' script command cannot be executed on windows system.
Switch the shell to *git bash* or use *git bash* to execute the '.sh' script.π€¨ "No module named 'xxx'" on windows system.
This error is caused by issues with the Python runtime environment path. You need to perform the following steps:Step 1: Check Environment Variables.
You must configure the "Scripts" in the environment variables.
Step 2: Check IDE Settings.
For VS Code, download the Python extension for code. For PyCharm, specify the Python version for the current project.
Project cleaningSupport more anomaliesSupport more knowledge sourcesQuery log option (potential to take up disk space and we need to consider it carefully)Add more communication mechanismsPrometheus-as-a-Service- Localized model that reaches D-bot(gpt4)'s capability
- Support other databases (e.g., mysql/redis)
https://github.com/OpenBMB/AgentVerse
https://github.com/Vonng/pigsty
https://github.com/UKPLab/sentence-transformers
https://github.com/chatchat-space/Langchain-Chatchat
https://github.com/shreyashankar/spade-experiments
Feel free to cite us (paper link) if you like this project.
@misc{zhou2023llm4diag,
title={D-Bot: Database Diagnosis System using Large Language Models},
author={Xuanhe Zhou, Guoliang Li, Zhaoyan Sun, Zhiyuan Liu, Weize Chen, Jianming Wu, Jiesi Liu, Ruohang Feng, Guoyang Zeng},
year={2023},
eprint={2312.01454},
archivePrefix={arXiv},
primaryClass={cs.DB}
}
@misc{zhou2023dbgpt,
title={DB-GPT: Large Language Model Meets Database},
author={Xuanhe Zhou, Zhaoyan Sun, Guoliang Li},
year={2023},
archivePrefix={Data Science and Engineering},
}
Other Collaborators: Wei Zhou, Kunyi Li.
We thank all the contributors to this project. Do not hesitate if you would like to get involved or contribute!
ππ»Welcome to our wechat group!
For Tasks:
Click tags to check more tools for each tasksFor Jobs:
Alternative AI tools for DB-GPT
Similar Open Source Tools
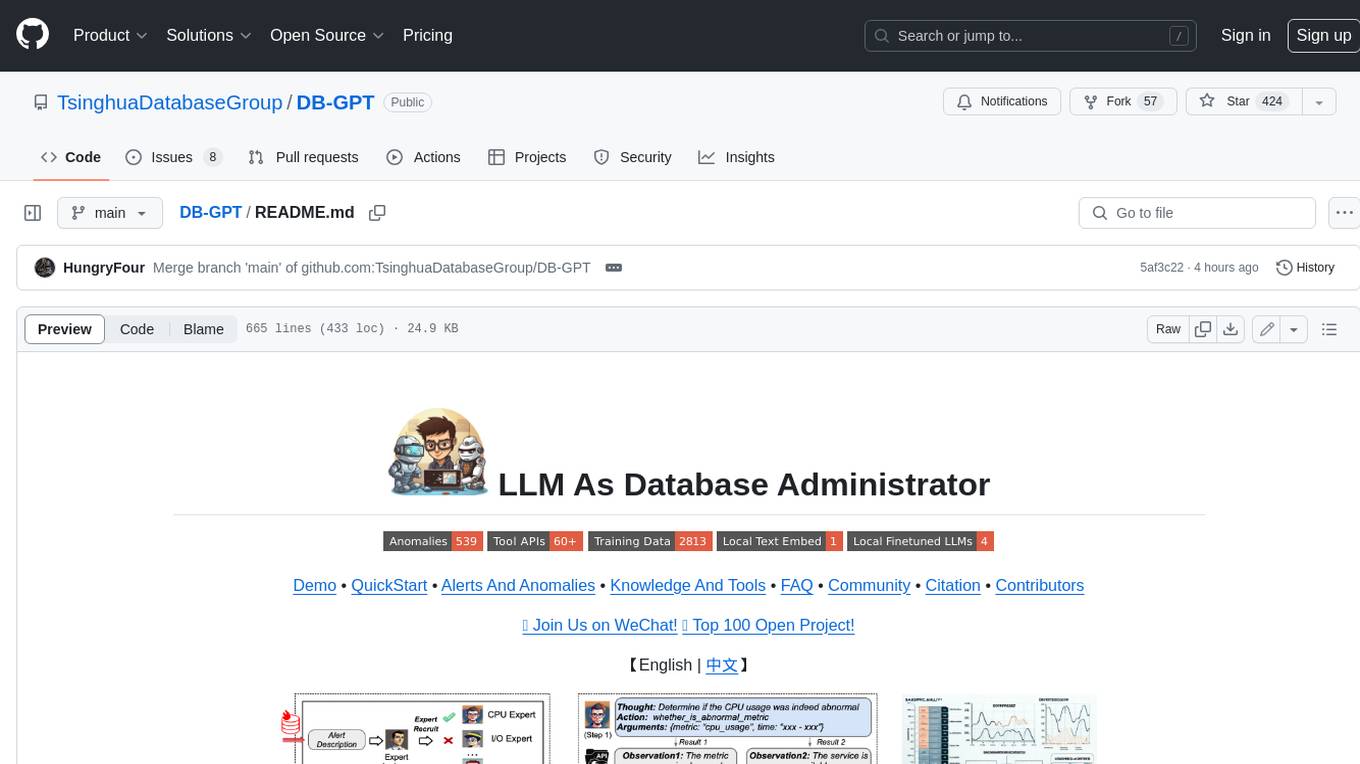
DB-GPT
DB-GPT is a personal database administrator that can solve database problems by reading documents, using various tools, and writing analysis reports. It is currently undergoing an upgrade. **Features:** * **Online Demo:** * Import documents into the knowledge base * Utilize the knowledge base for well-founded Q&A and diagnosis analysis of abnormal alarms * Send feedbacks to refine the intermediate diagnosis results * Edit the diagnosis result * Browse all historical diagnosis results, used metrics, and detailed diagnosis processes * **Language Support:** * English (default) * Chinese (add "language: zh" in config.yaml) * **New Frontend:** * Knowledgebase + Chat Q&A + Diagnosis + Report Replay * **Extreme Speed Version for localized llms:** * 4-bit quantized LLM (reducing inference time by 1/3) * vllm for fast inference (qwen) * Tiny LLM * **Multi-path extraction of document knowledge:** * Vector database (ChromaDB) * RESTful Search Engine (Elasticsearch) * **Expert prompt generation using document knowledge** * **Upgrade the LLM-based diagnosis mechanism:** * Task Dispatching -> Concurrent Diagnosis -> Cross Review -> Report Generation * Synchronous Concurrency Mechanism during LLM inference * **Support monitoring and optimization tools in multiple levels:** * Monitoring metrics (Prometheus) * Flame graph in code level * Diagnosis knowledge retrieval (dbmind) * Logical query transformations (Calcite) * Index optimization algorithms (for PostgreSQL) * Physical operator hints (for PostgreSQL) * Backup and Point-in-time Recovery (Pigsty) * **Continuously updated papers and experimental reports** This project is constantly evolving with new features. Don't forget to star β and watch π to stay up to date.
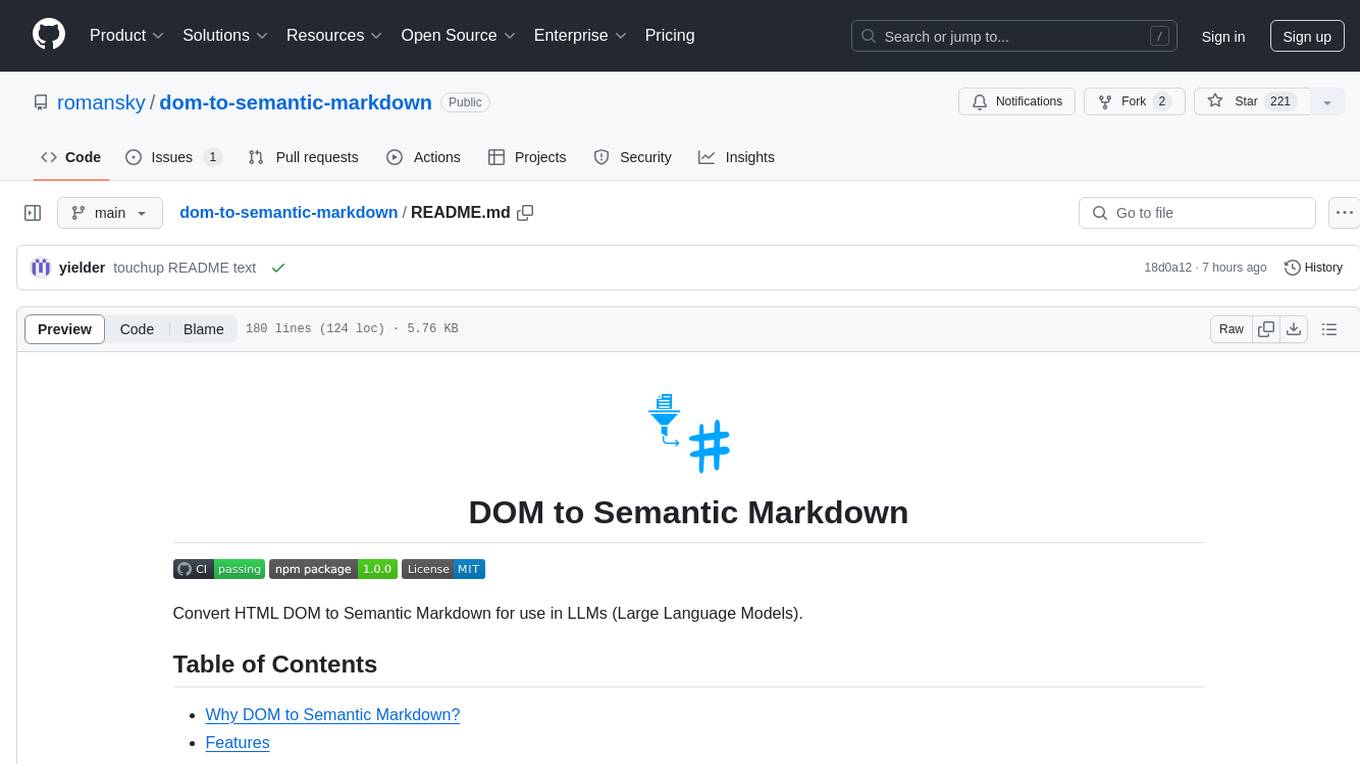
dom-to-semantic-markdown
DOM to Semantic Markdown is a tool that converts HTML DOM to Semantic Markdown for use in Large Language Models (LLMs). It maximizes semantic information, token efficiency, and preserves metadata to enhance LLMs' processing capabilities. The tool captures rich web content structure, including semantic tags, image metadata, table structures, and link destinations. It offers customizable conversion options and supports both browser and Node.js environments.
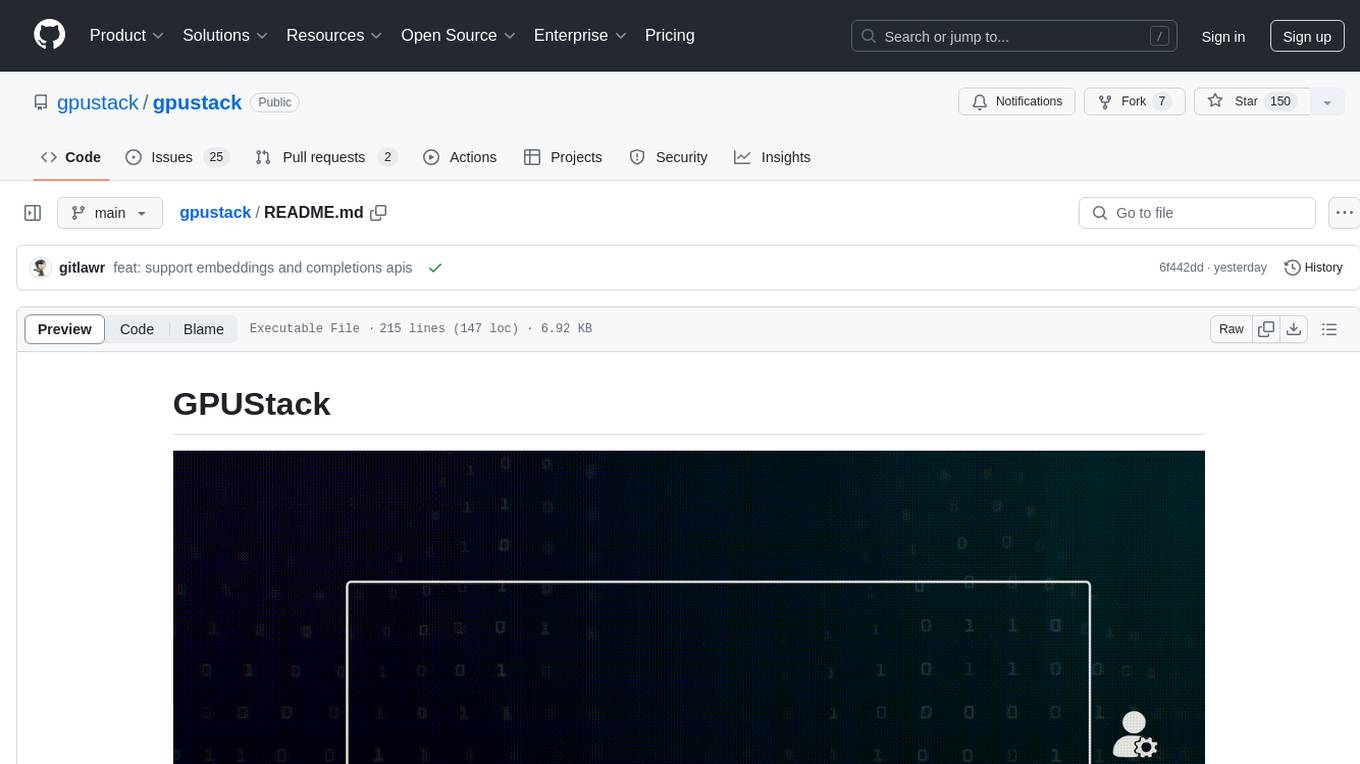
gpustack
GPUStack is an open-source GPU cluster manager designed for running large language models (LLMs). It supports a wide variety of hardware, scales with GPU inventory, offers lightweight Python package with minimal dependencies, provides OpenAI-compatible APIs, simplifies user and API key management, enables GPU metrics monitoring, and facilitates token usage and rate metrics tracking. The tool is suitable for managing GPU clusters efficiently and effectively.
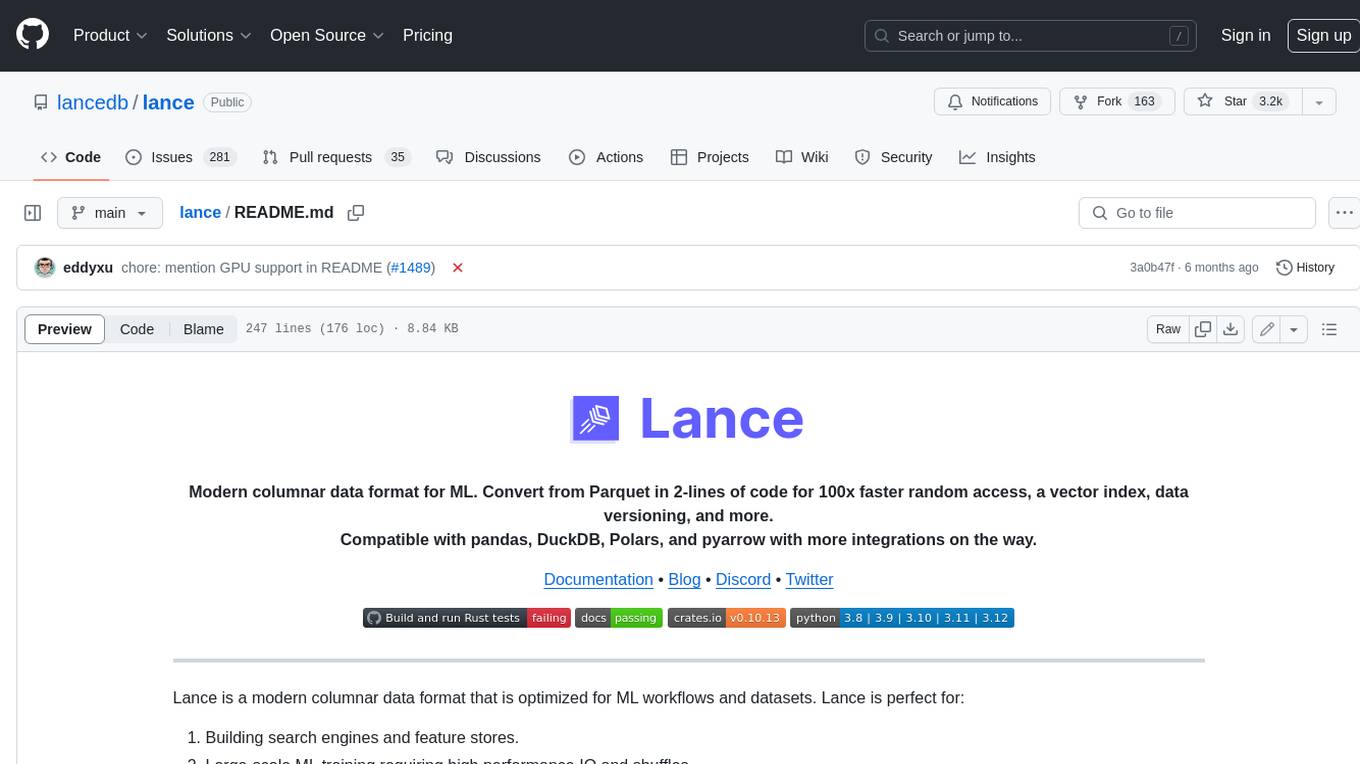
lance
Lance is a modern columnar data format optimized for ML workflows and datasets. It offers high-performance random access, vector search, zero-copy automatic versioning, and ecosystem integrations with Apache Arrow, Pandas, Polars, and DuckDB. Lance is designed to address the challenges of the ML development cycle, providing a unified data format for collection, exploration, analytics, feature engineering, training, evaluation, deployment, and monitoring. It aims to reduce data silos and streamline the ML development process.
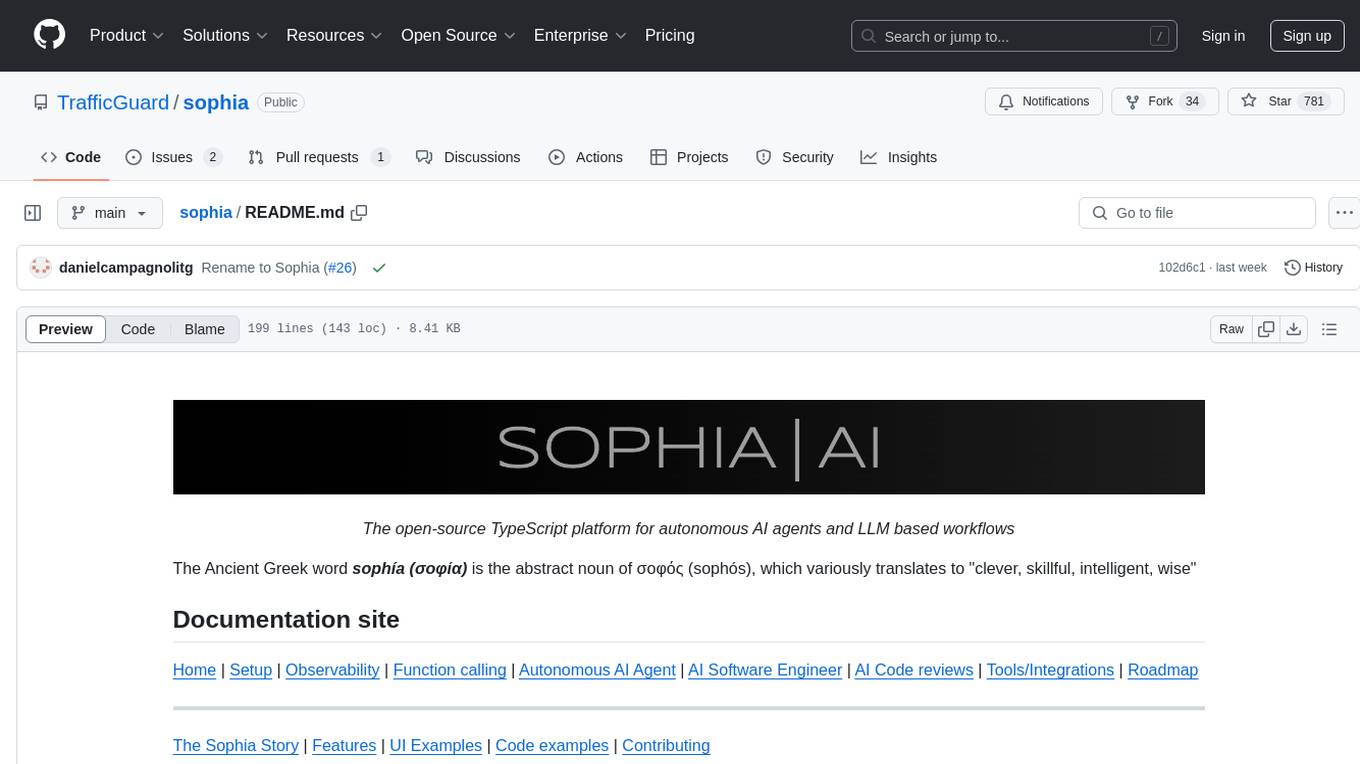
sophia
Sophia is an open-source TypeScript platform designed for autonomous AI agents and LLM based workflows. It aims to automate processes, review code, assist with refactorings, and support various integrations. The platform offers features like advanced autonomous agents, reasoning/planning inspired by Google's Self-Discover paper, memory and function call history, adaptive iterative planning, and more. Sophia supports multiple LLMs/services, CLI and web interface, human-in-the-loop interactions, flexible deployment options, observability with OpenTelemetry tracing, and specific agents for code editing, software engineering, and code review. It provides a flexible platform for the TypeScript community to expand and support various use cases and integrations.
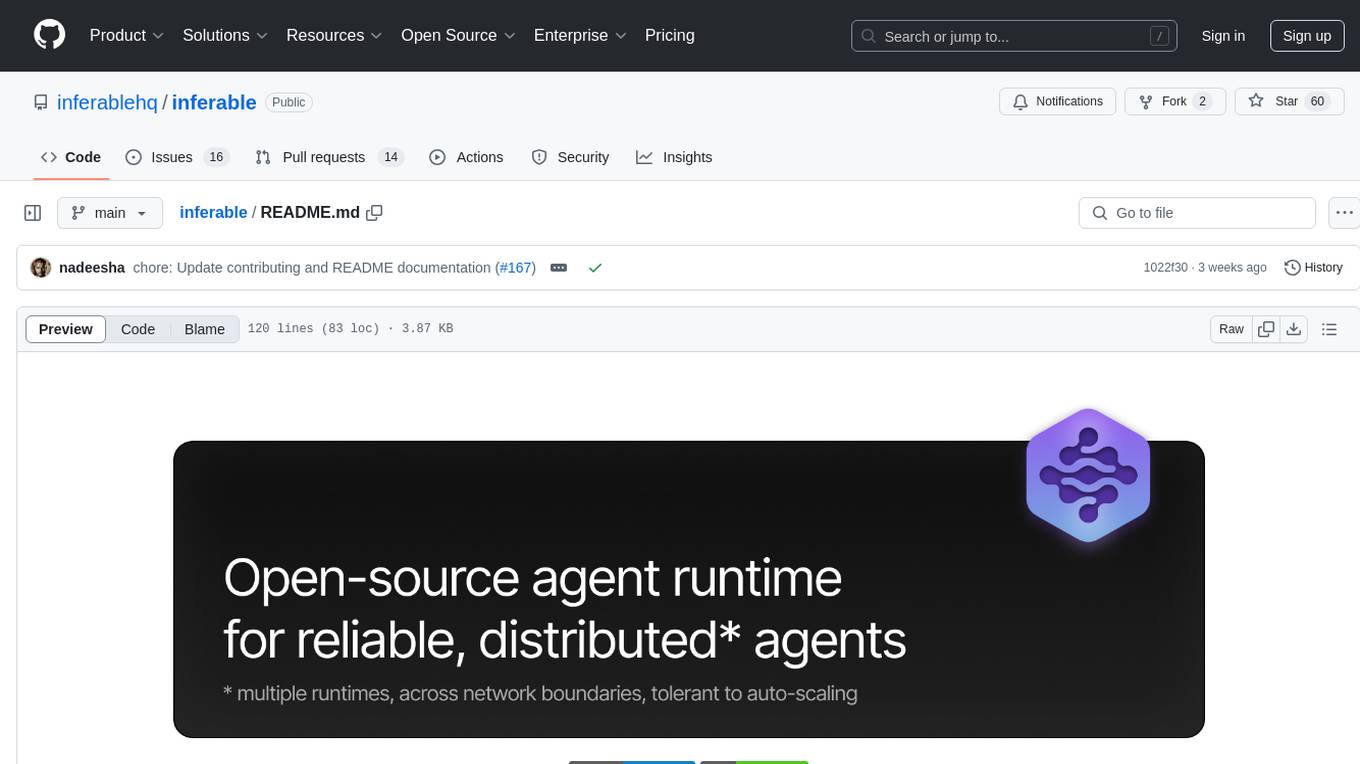
inferable
Inferable is an open source platform that helps users build reliable LLM-powered agentic automations at scale. It offers a managed agent runtime, durable tool calling, zero network configuration, multiple language support, and is fully open source under the MIT license. Users can define functions, register them with Inferable, and create runs that utilize these functions to automate tasks. The platform supports Node.js/TypeScript, Go, .NET, and React, and provides SDKs, core services, and bootstrap templates for various languages.
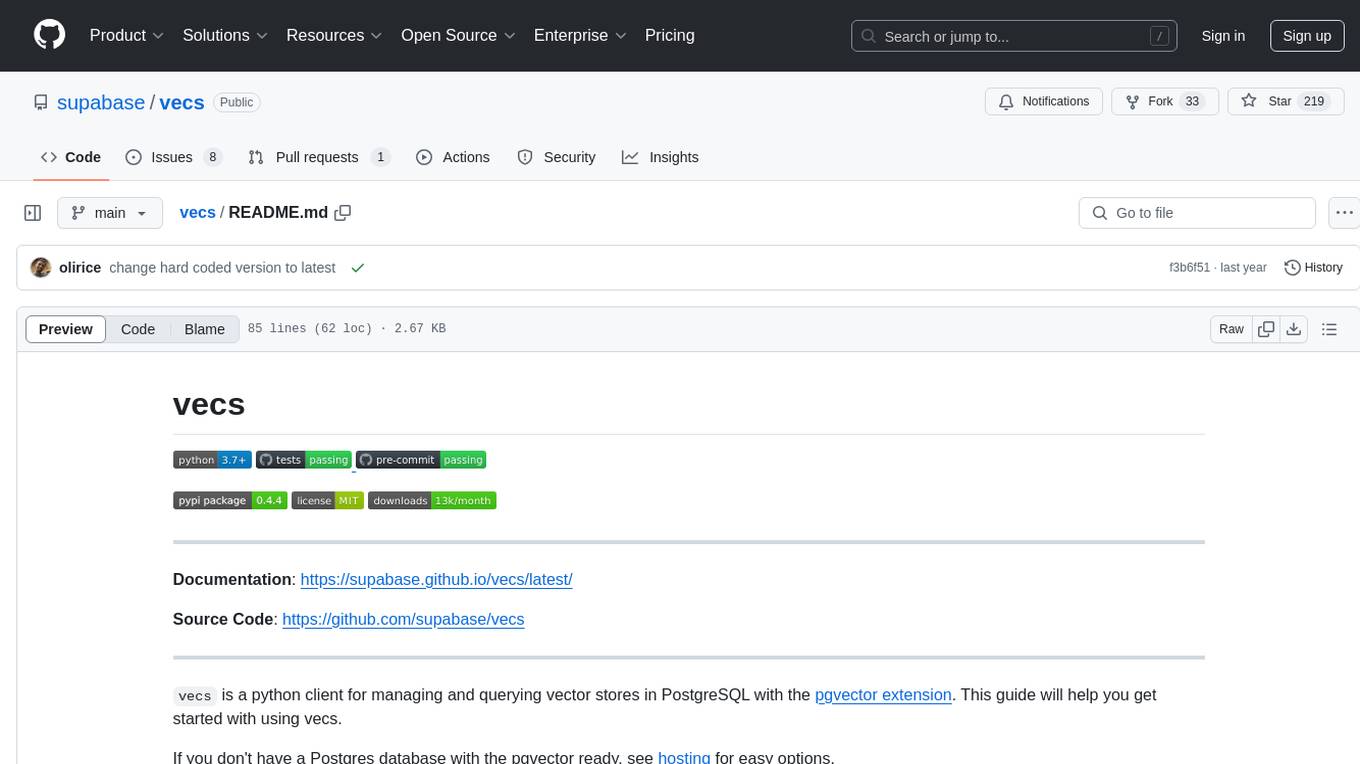
vecs
vecs is a Python client for managing and querying vector stores in PostgreSQL with the pgvector extension. It allows users to create collections of vectors with associated metadata, index the collections for fast search performance, and query the collections based on specified filters. The tool simplifies the process of working with vector data in a PostgreSQL database, making it easier to store, retrieve, and analyze vector information.
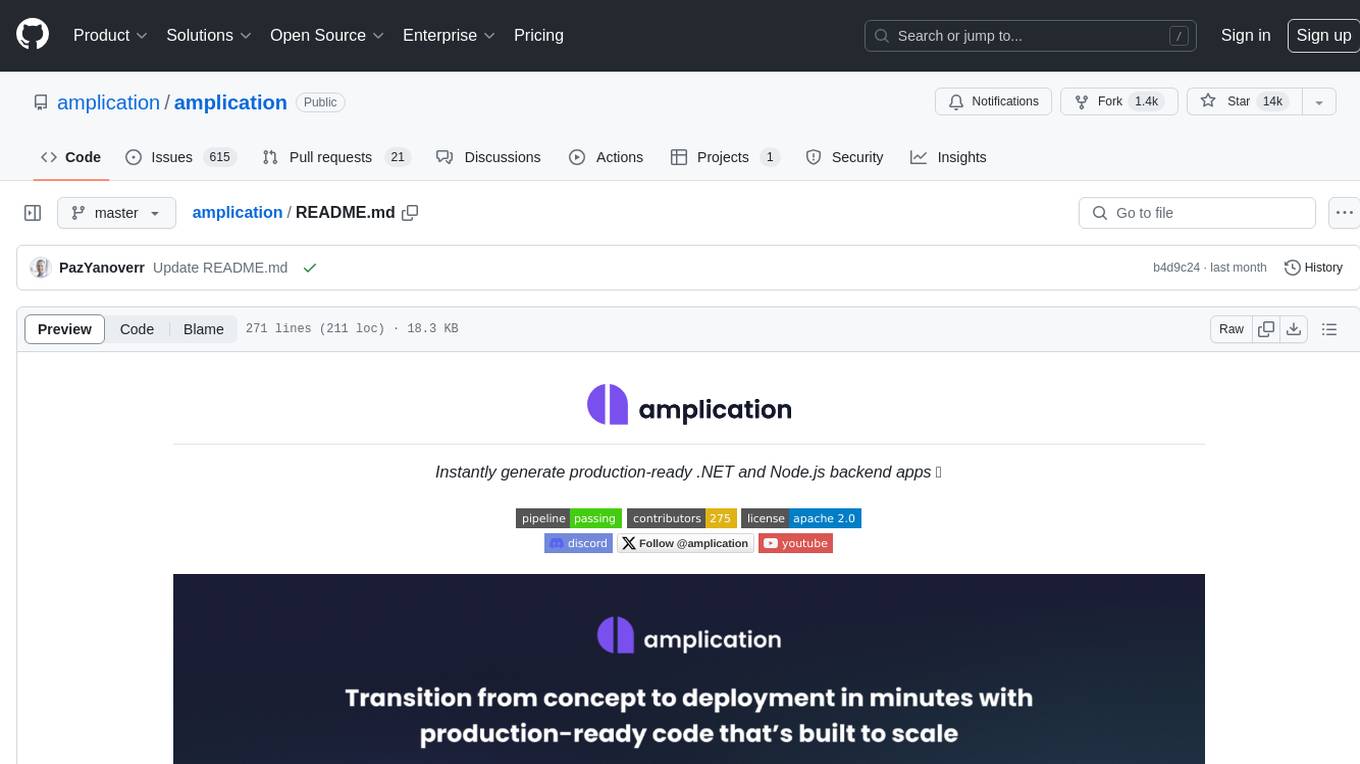
amplication
Amplication is a robust, open-source development platform designed to revolutionize the creation of scalable and secure .NET and Node.js applications. It automates backend applications development, ensuring consistency, predictability, and adherence to the highest standards with code that's built to scale. The user-friendly interface fosters seamless integration of APIs, data models, databases, authentication, and authorization. Built on a flexible, plugin-based architecture, Amplication allows effortless customization of the code and offers a diverse range of integrations. With a strong focus on collaboration, Amplication streamlines team-oriented development, making it an ideal choice for groups of all sizes, from startups to large enterprises. It enables users to concentrate on business logic while handling the heavy lifting of development. Experience the fastest way to develop .NET and Node.js applications with Amplication.
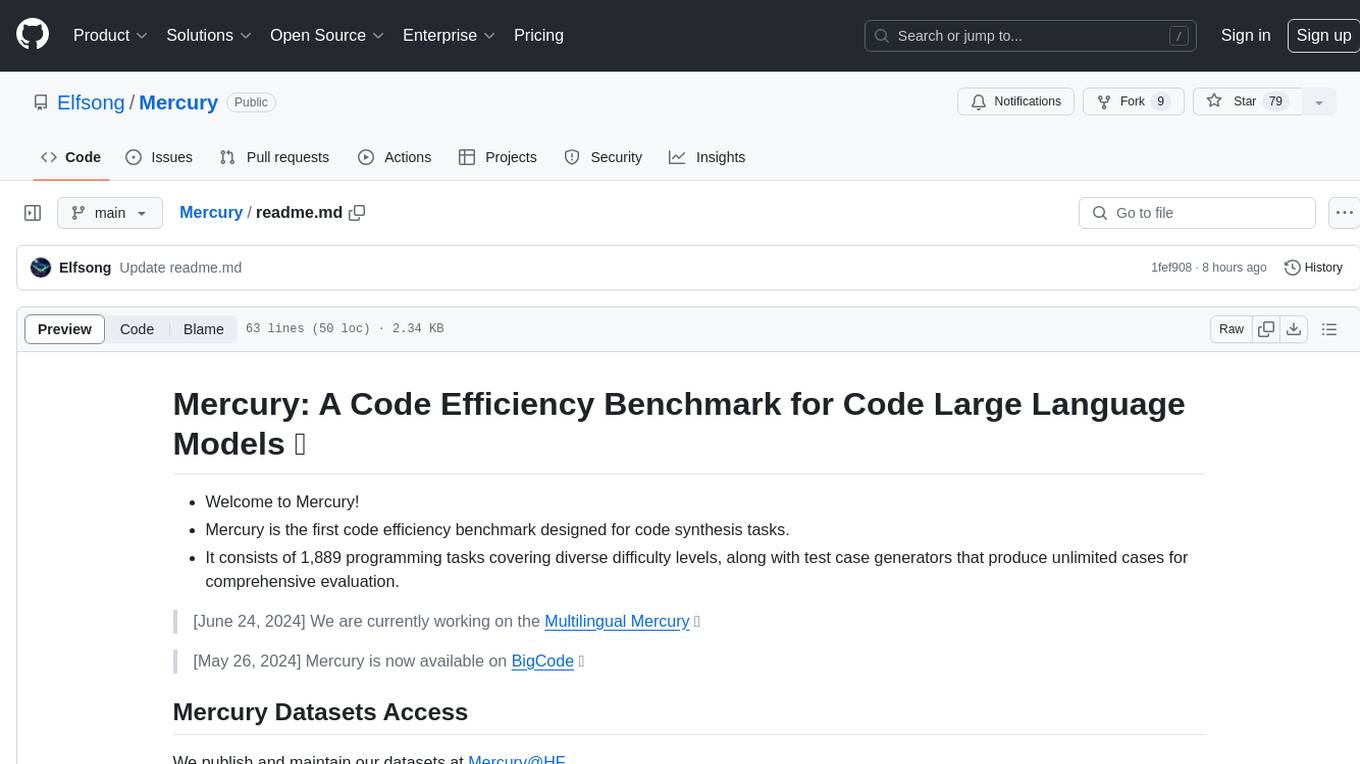
Mercury
Mercury is a code efficiency benchmark designed for code synthesis tasks. It includes 1,889 programming tasks of varying difficulty levels and provides test case generators for comprehensive evaluation. The benchmark aims to assess the efficiency of large language models in generating code solutions.
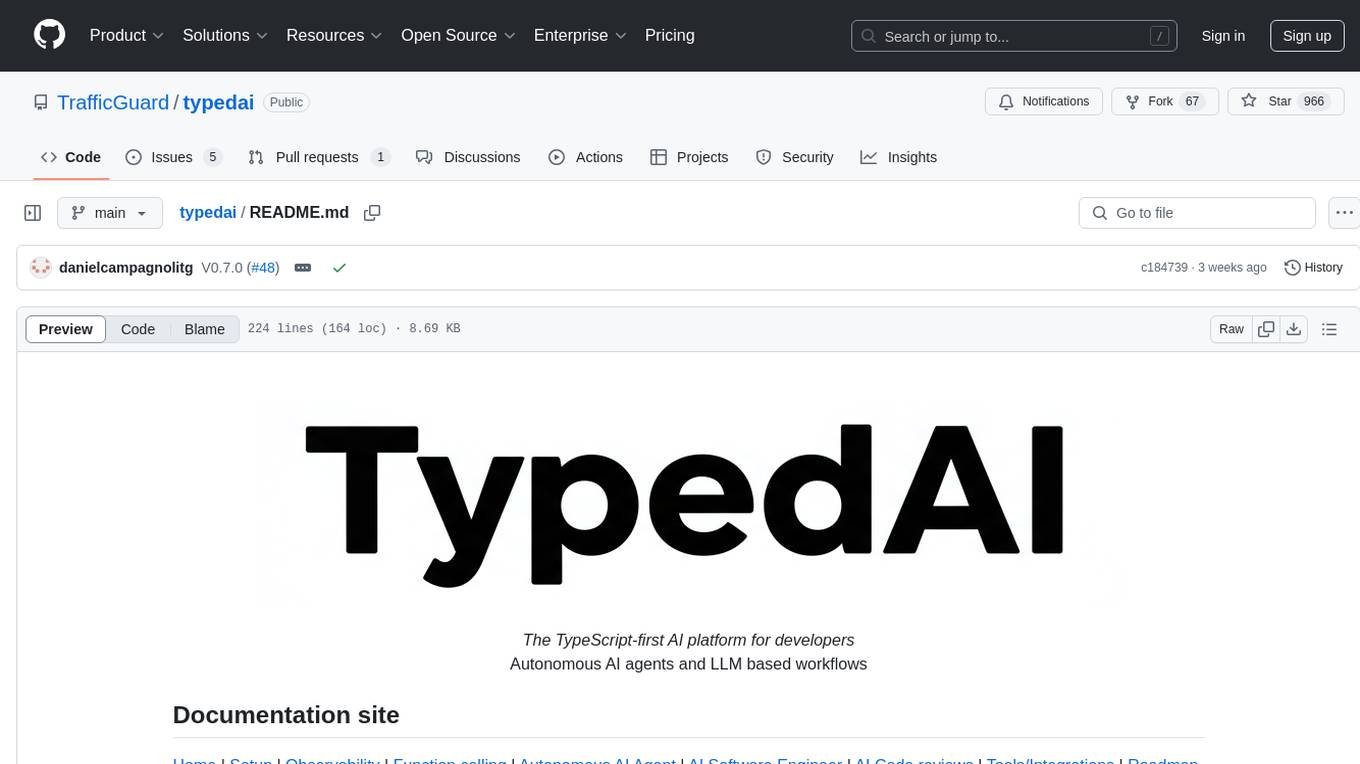
typedai
TypedAI is a TypeScript-first AI platform designed for developers to create and run autonomous AI agents, LLM based workflows, and chatbots. It offers advanced autonomous agents, software developer agents, pull request code review agent, AI chat interface, Slack chatbot, and supports various LLM services. The platform features configurable Human-in-the-loop settings, functional callable tools/integrations, CLI and Web UI interface, and can be run locally or deployed on the cloud with multi-user/SSO support. It leverages the Python AI ecosystem through executing Python scripts/packages and provides flexible run/deploy options like single user mode, Firestore & Cloud Run deployment, and multi-user SSO enterprise deployment. TypedAI also includes UI examples, code examples, and automated LLM function schemas for seamless development and execution of AI workflows.
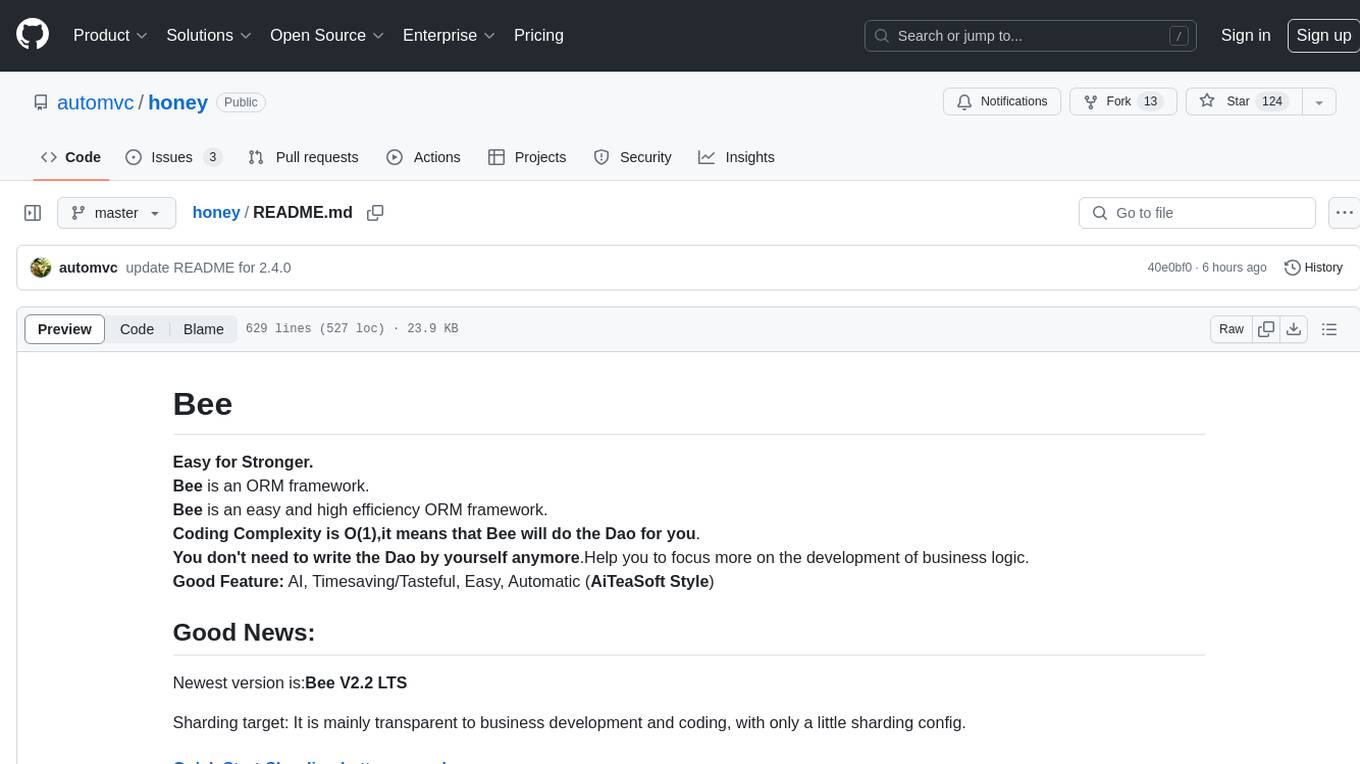
honey
Bee is an ORM framework that provides easy and high-efficiency database operations, allowing developers to focus on business logic development. It supports various databases and features like automatic filtering, partial field queries, pagination, and JSON format results. Bee also offers advanced functionalities like sharding, transactions, complex queries, and MongoDB ORM. The tool is designed for rapid application development in Java, offering faster development for Java Web and Spring Cloud microservices. The Enterprise Edition provides additional features like financial computing support, automatic value insertion, desensitization, dictionary value conversion, multi-tenancy, and more.
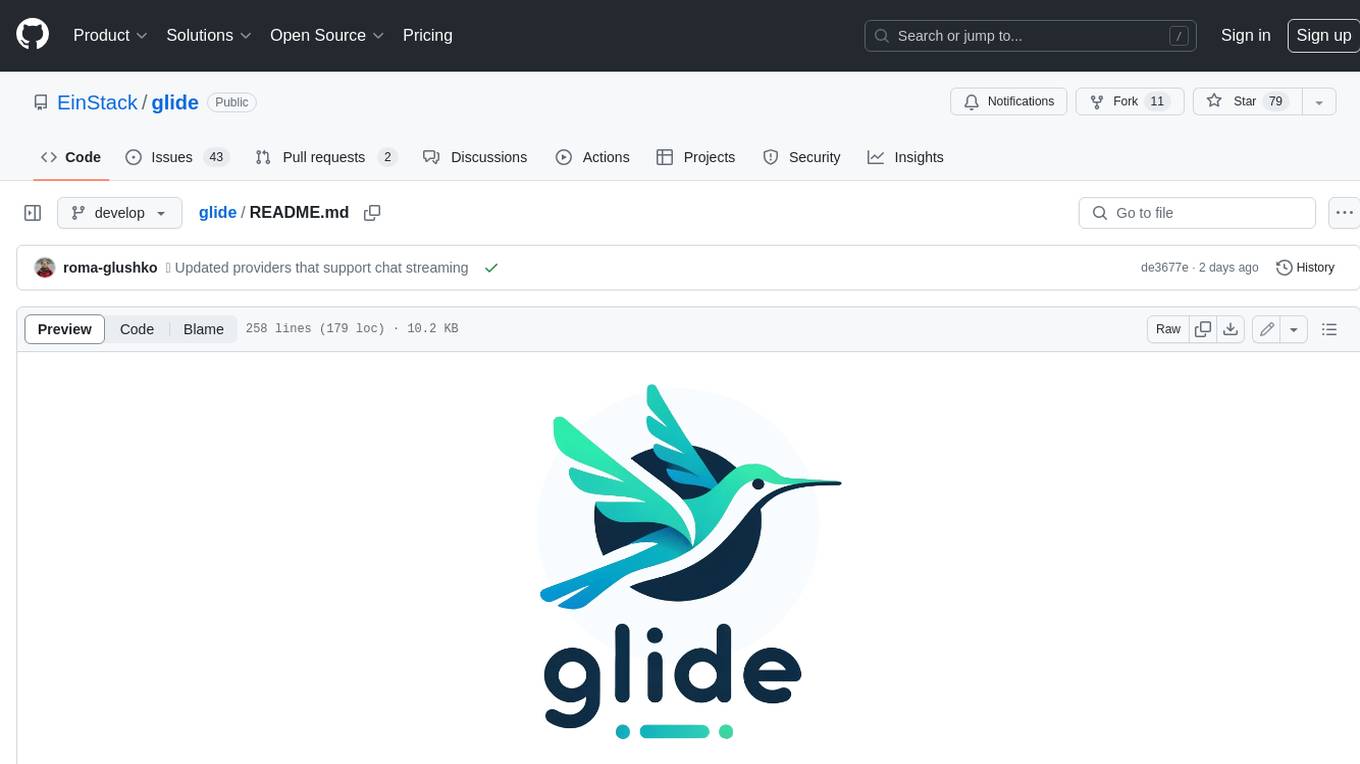
glide
Glide is a cloud-native LLM gateway that provides a unified REST API for accessing various large language models (LLMs) from different providers. It handles LLMOps tasks such as model failover, caching, key management, and more, making it easy to integrate LLMs into applications. Glide supports popular LLM providers like OpenAI, Anthropic, Azure OpenAI, AWS Bedrock (Titan), Cohere, Google Gemini, OctoML, and Ollama. It offers high availability, performance, and observability, and provides SDKs for Python and NodeJS to simplify integration.
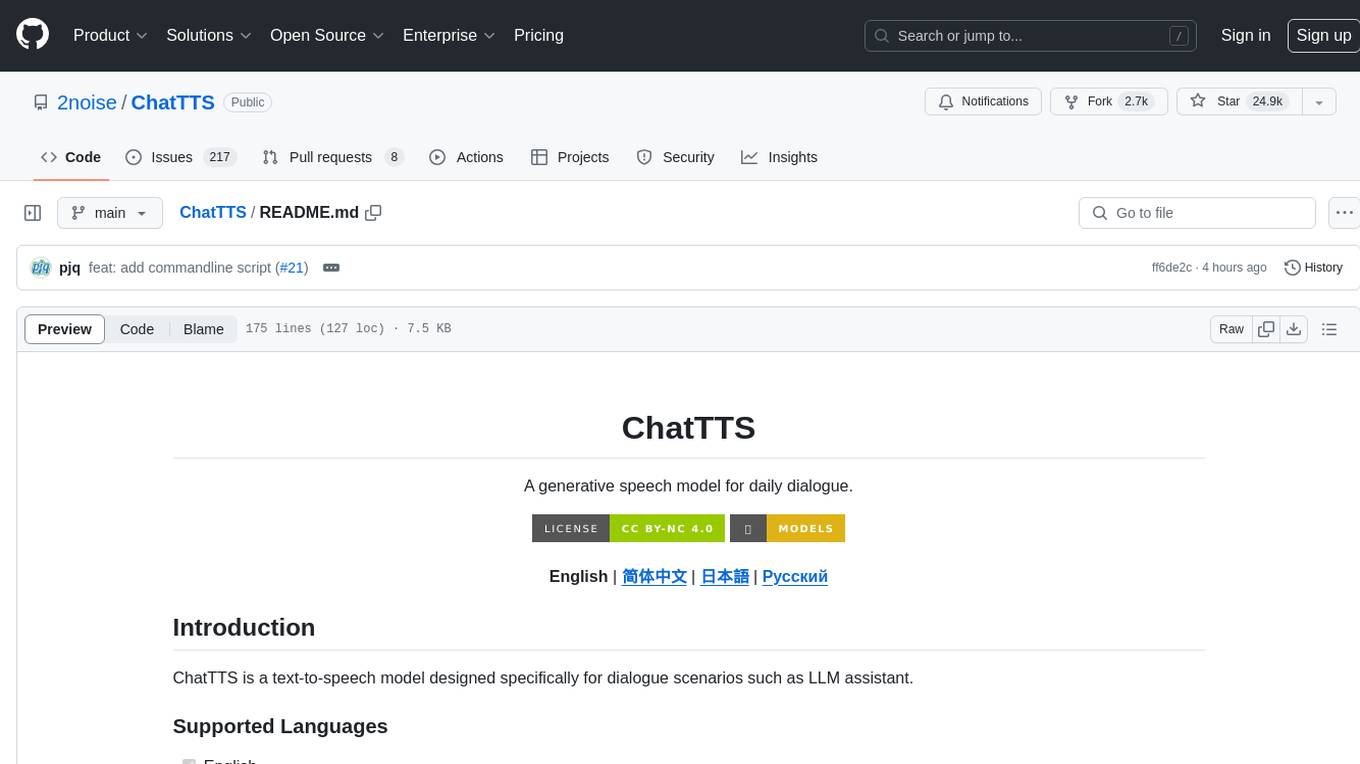
ChatTTS
ChatTTS is a generative speech model optimized for dialogue scenarios, providing natural and expressive speech synthesis with fine-grained control over prosodic features. It supports multiple speakers and surpasses most open-source TTS models in terms of prosody. The model is trained with 100,000+ hours of Chinese and English audio data, and the open-source version on HuggingFace is a 40,000-hour pre-trained model without SFT. The roadmap includes open-sourcing additional features like VQ encoder, multi-emotion control, and streaming audio generation. The tool is intended for academic and research use only, with precautions taken to limit potential misuse.
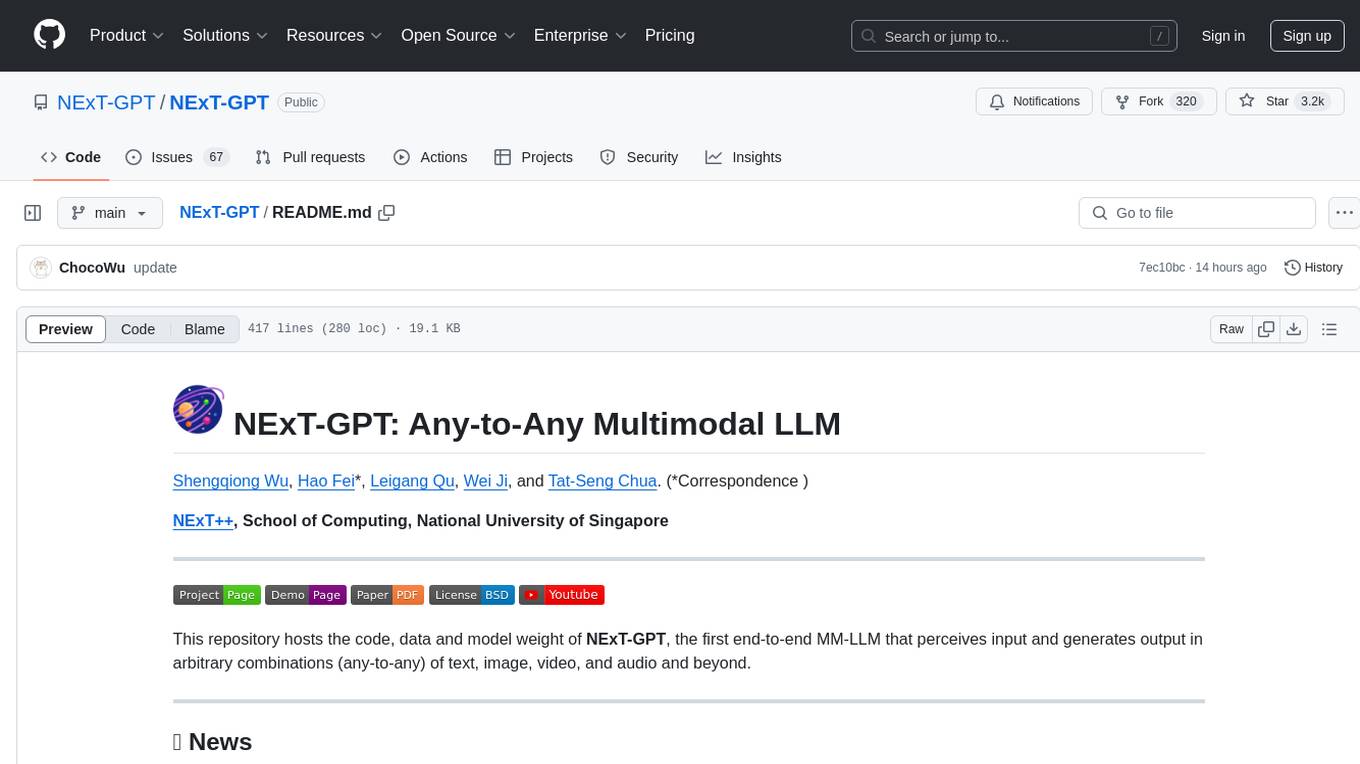
NExT-GPT
NExT-GPT is an end-to-end multimodal large language model that can process input and generate output in various combinations of text, image, video, and audio. It leverages existing pre-trained models and diffusion models with end-to-end instruction tuning. The repository contains code, data, and model weights for NExT-GPT, allowing users to work with different modalities and perform tasks like encoding, understanding, reasoning, and generating multimodal content.
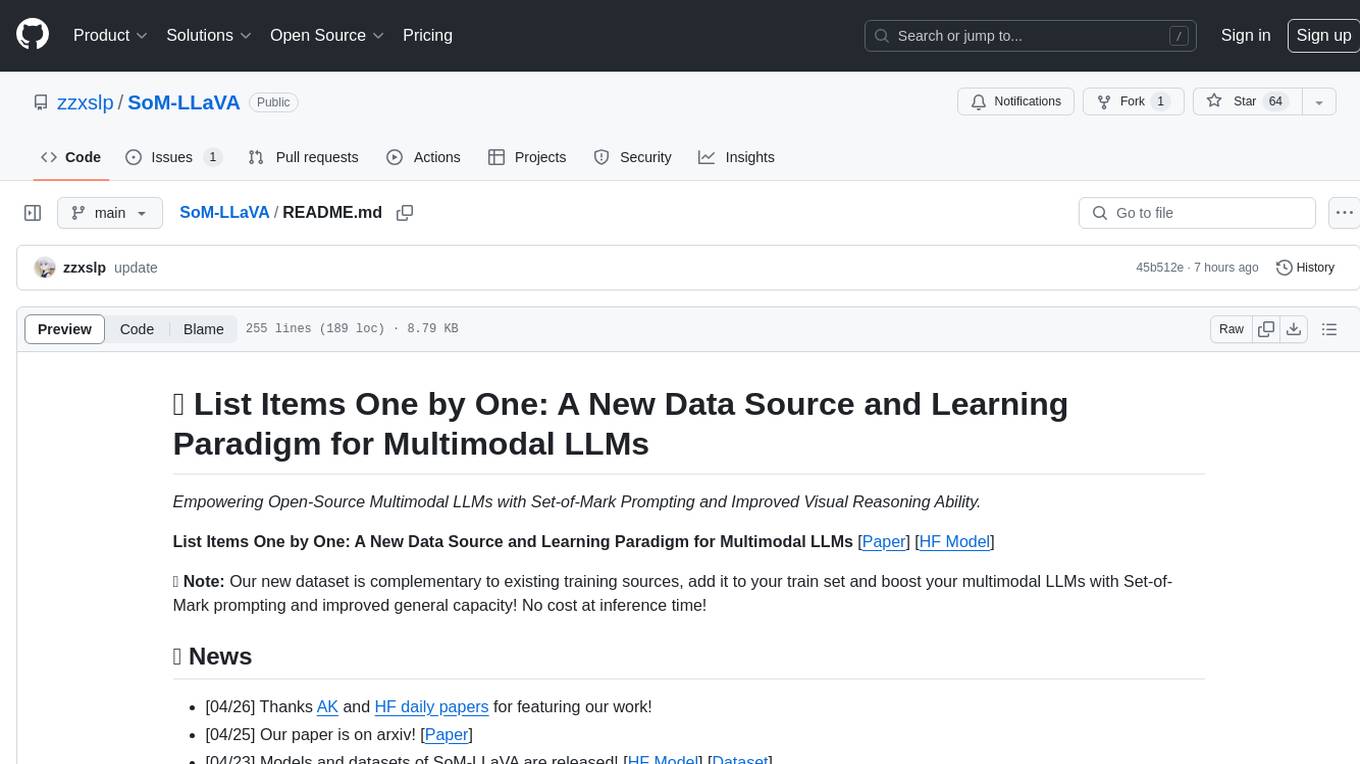
SoM-LLaVA
SoM-LLaVA is a new data source and learning paradigm for Multimodal LLMs, empowering open-source Multimodal LLMs with Set-of-Mark prompting and improved visual reasoning ability. The repository provides a new dataset that is complementary to existing training sources, enhancing multimodal LLMs with Set-of-Mark prompting and improved general capacity. By adding 30k SoM data to the visual instruction tuning stage of LLaVA, the tool achieves 1% to 6% relative improvements on all benchmarks. Users can train SoM-LLaVA via command line and utilize the implementation to annotate COCO images with SoM. Additionally, the tool can be loaded in Huggingface for further usage.
For similar tasks
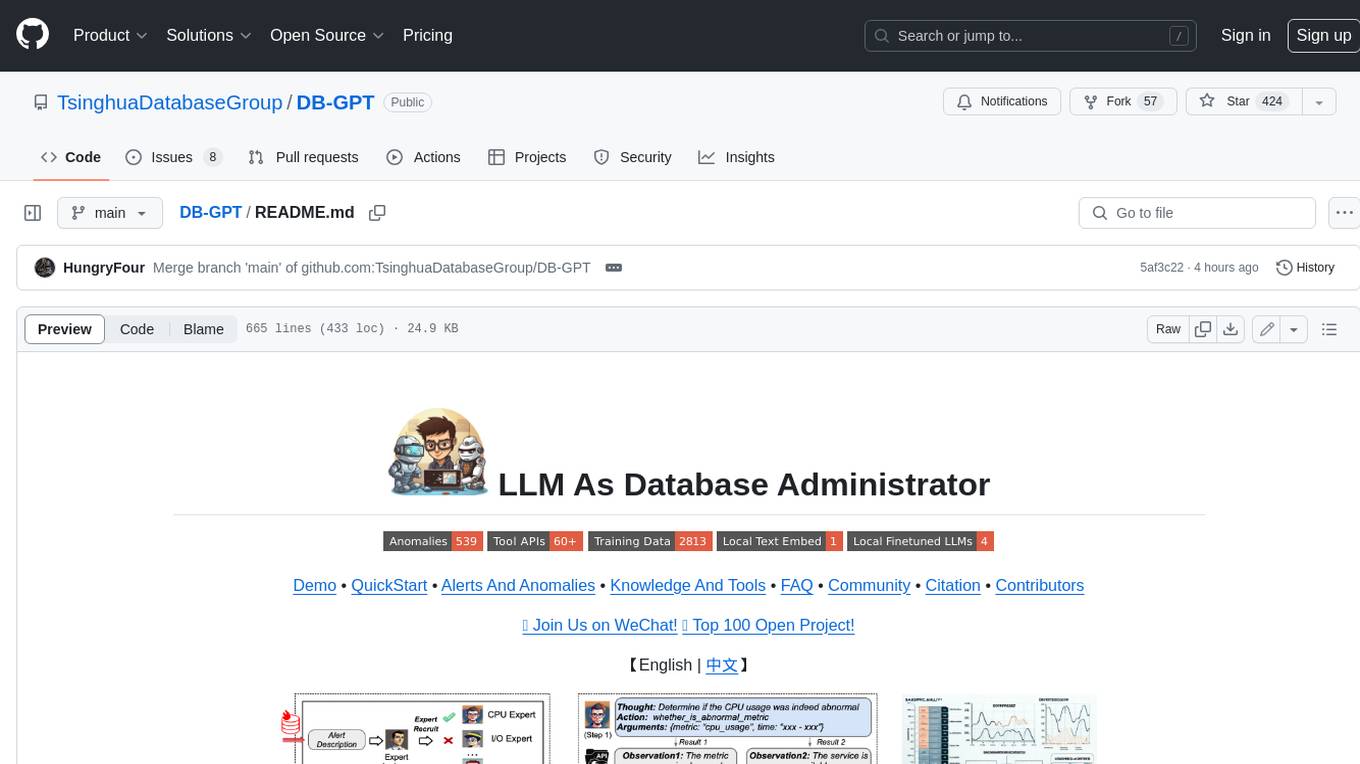
DB-GPT
DB-GPT is a personal database administrator that can solve database problems by reading documents, using various tools, and writing analysis reports. It is currently undergoing an upgrade. **Features:** * **Online Demo:** * Import documents into the knowledge base * Utilize the knowledge base for well-founded Q&A and diagnosis analysis of abnormal alarms * Send feedbacks to refine the intermediate diagnosis results * Edit the diagnosis result * Browse all historical diagnosis results, used metrics, and detailed diagnosis processes * **Language Support:** * English (default) * Chinese (add "language: zh" in config.yaml) * **New Frontend:** * Knowledgebase + Chat Q&A + Diagnosis + Report Replay * **Extreme Speed Version for localized llms:** * 4-bit quantized LLM (reducing inference time by 1/3) * vllm for fast inference (qwen) * Tiny LLM * **Multi-path extraction of document knowledge:** * Vector database (ChromaDB) * RESTful Search Engine (Elasticsearch) * **Expert prompt generation using document knowledge** * **Upgrade the LLM-based diagnosis mechanism:** * Task Dispatching -> Concurrent Diagnosis -> Cross Review -> Report Generation * Synchronous Concurrency Mechanism during LLM inference * **Support monitoring and optimization tools in multiple levels:** * Monitoring metrics (Prometheus) * Flame graph in code level * Diagnosis knowledge retrieval (dbmind) * Logical query transformations (Calcite) * Index optimization algorithms (for PostgreSQL) * Physical operator hints (for PostgreSQL) * Backup and Point-in-time Recovery (Pigsty) * **Continuously updated papers and experimental reports** This project is constantly evolving with new features. Don't forget to star β and watch π to stay up to date.
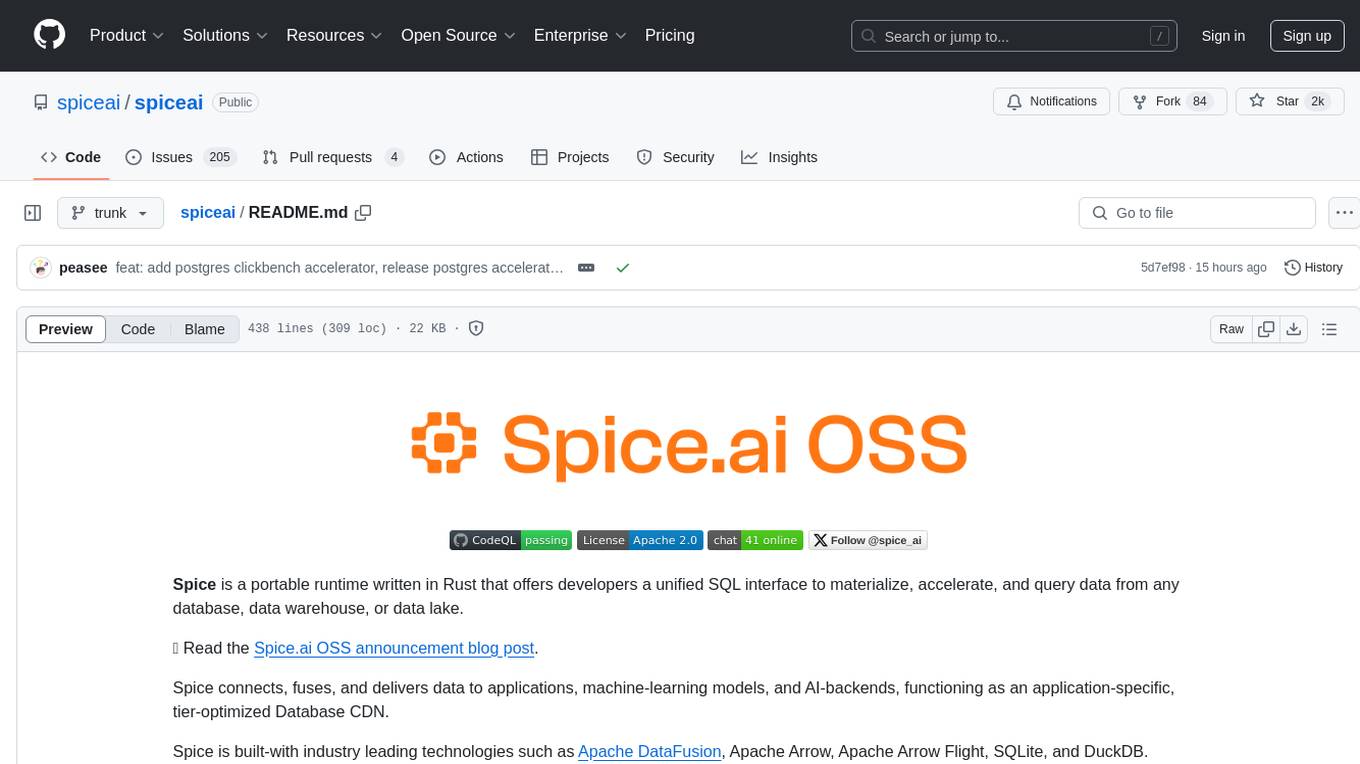
spiceai
Spice is a portable runtime written in Rust that offers developers a unified SQL interface to materialize, accelerate, and query data from any database, data warehouse, or data lake. It connects, fuses, and delivers data to applications, machine-learning models, and AI-backends, functioning as an application-specific, tier-optimized Database CDN. Built with industry-leading technologies such as Apache DataFusion, Apache Arrow, Apache Arrow Flight, SQLite, and DuckDB. Spice makes it fast and easy to query data from one or more sources using SQL, co-locating a managed dataset with applications or machine learning models, and accelerating it with Arrow in-memory, SQLite/DuckDB, or attached PostgreSQL for fast, high-concurrency, low-latency queries.
For similar jobs
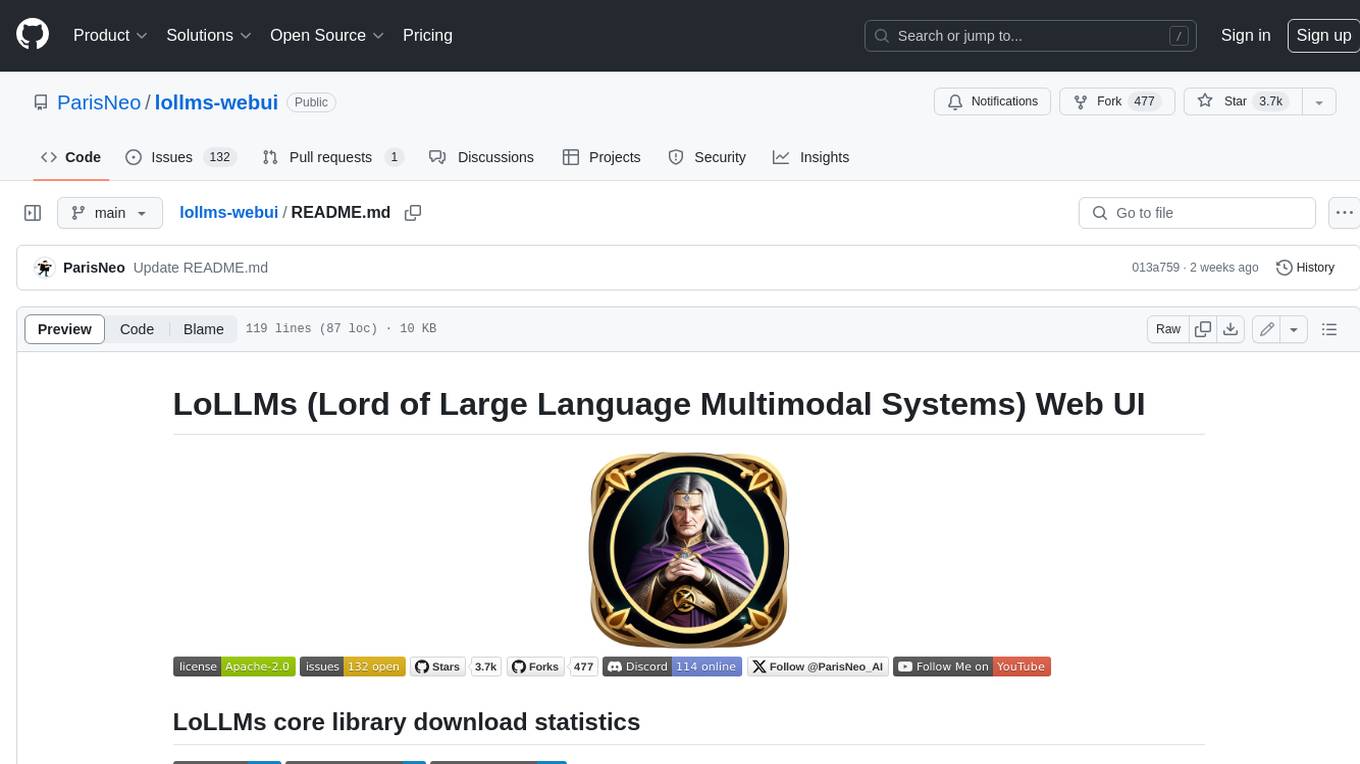
lollms-webui
LoLLMs WebUI (Lord of Large Language Multimodal Systems: One tool to rule them all) is a user-friendly interface to access and utilize various LLM (Large Language Models) and other AI models for a wide range of tasks. With over 500 AI expert conditionings across diverse domains and more than 2500 fine tuned models over multiple domains, LoLLMs WebUI provides an immediate resource for any problem, from car repair to coding assistance, legal matters, medical diagnosis, entertainment, and more. The easy-to-use UI with light and dark mode options, integration with GitHub repository, support for different personalities, and features like thumb up/down rating, copy, edit, and remove messages, local database storage, search, export, and delete multiple discussions, make LoLLMs WebUI a powerful and versatile tool.
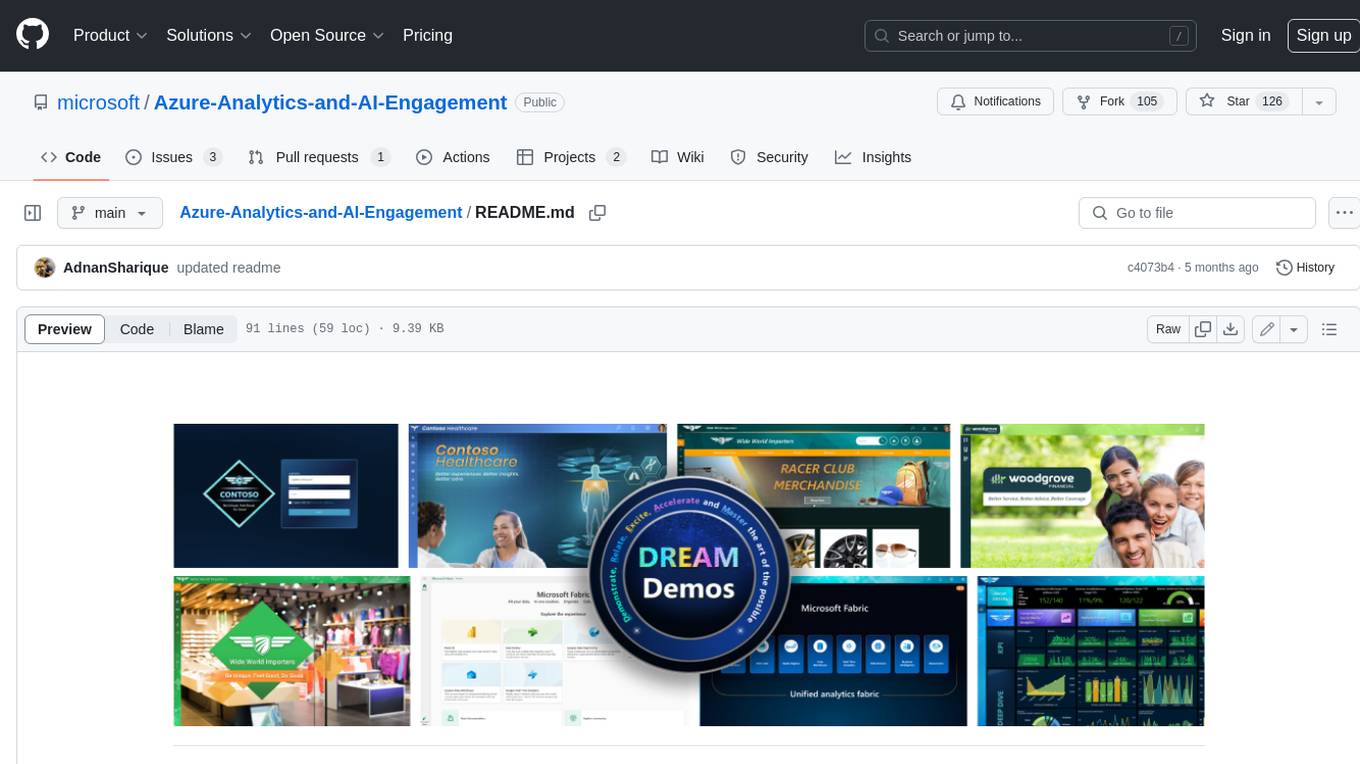
Azure-Analytics-and-AI-Engagement
The Azure-Analytics-and-AI-Engagement repository provides packaged Industry Scenario DREAM Demos with ARM templates (Containing a demo web application, Power BI reports, Synapse resources, AML Notebooks etc.) that can be deployed in a customerβs subscription using the CAPE tool within a matter of few hours. Partners can also deploy DREAM Demos in their own subscriptions using DPoC.
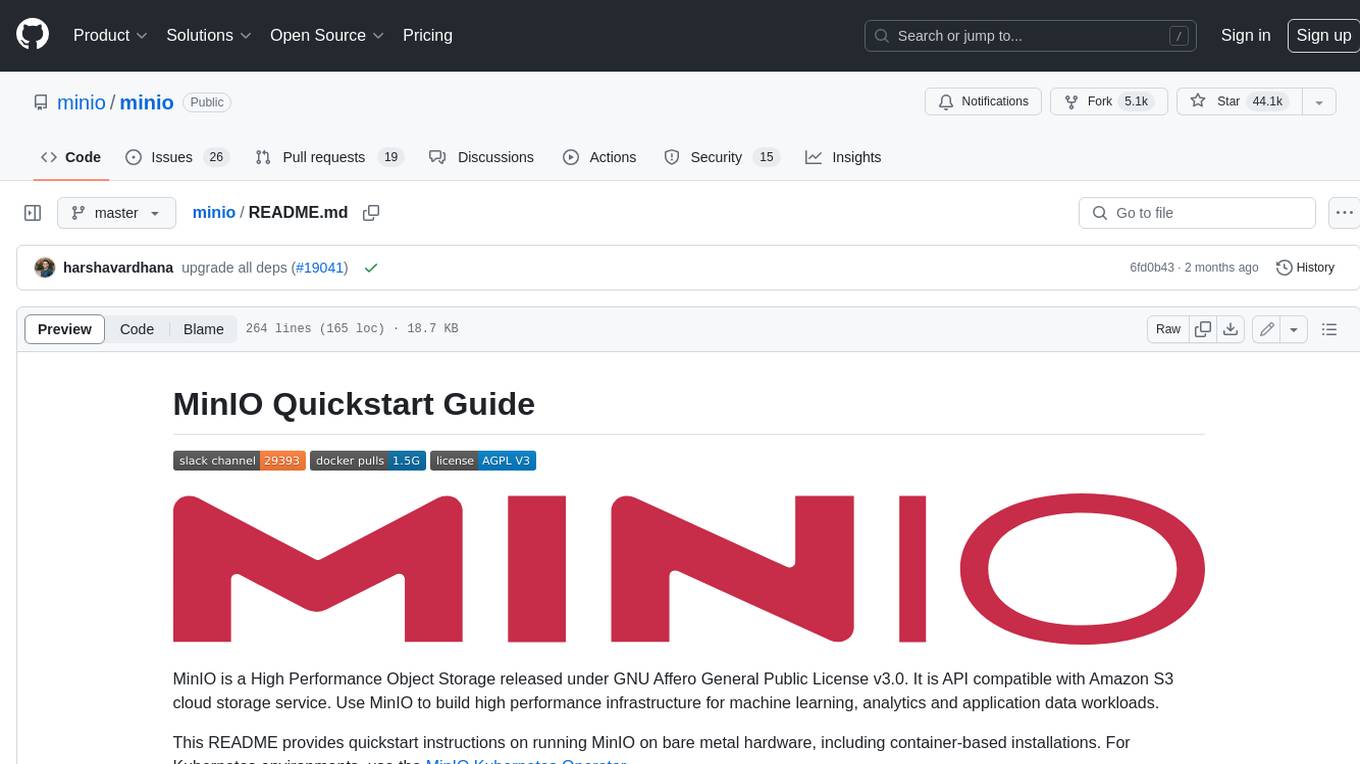
minio
MinIO is a High Performance Object Storage released under GNU Affero General Public License v3.0. It is API compatible with Amazon S3 cloud storage service. Use MinIO to build high performance infrastructure for machine learning, analytics and application data workloads.
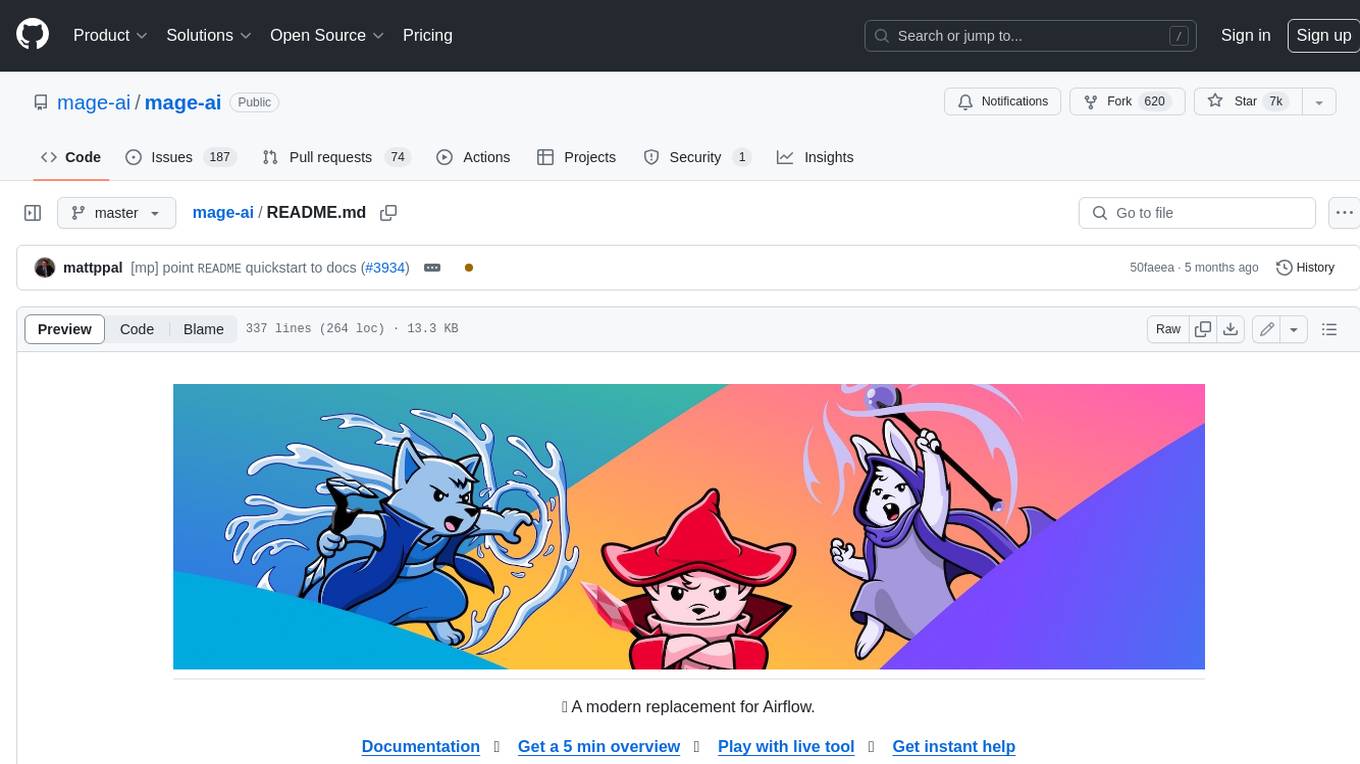
mage-ai
Mage is an open-source data pipeline tool for transforming and integrating data. It offers an easy developer experience, engineering best practices built-in, and data as a first-class citizen. Mage makes it easy to build, preview, and launch data pipelines, and provides observability and scaling capabilities. It supports data integrations, streaming pipelines, and dbt integration.
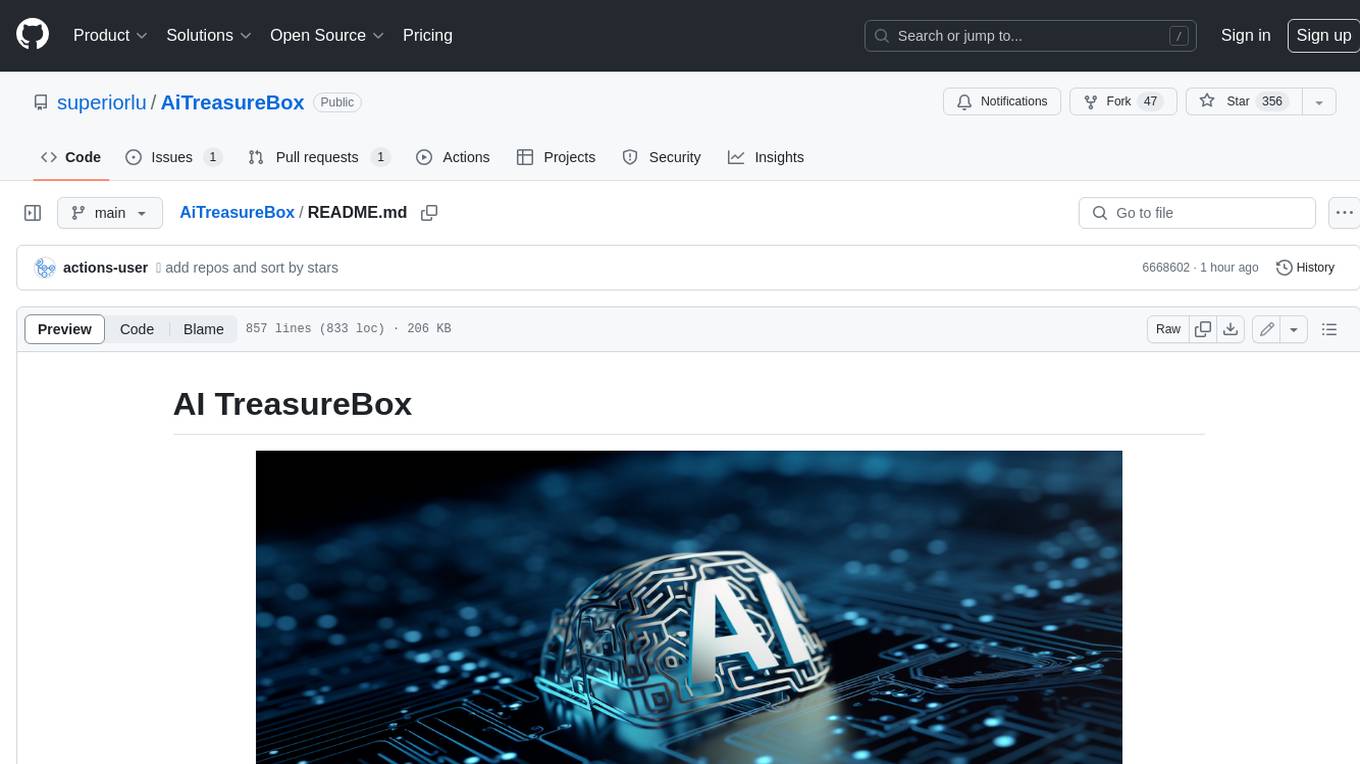
AiTreasureBox
AiTreasureBox is a versatile AI tool that provides a collection of pre-trained models and algorithms for various machine learning tasks. It simplifies the process of implementing AI solutions by offering ready-to-use components that can be easily integrated into projects. With AiTreasureBox, users can quickly prototype and deploy AI applications without the need for extensive knowledge in machine learning or deep learning. The tool covers a wide range of tasks such as image classification, text generation, sentiment analysis, object detection, and more. It is designed to be user-friendly and accessible to both beginners and experienced developers, making AI development more efficient and accessible to a wider audience.
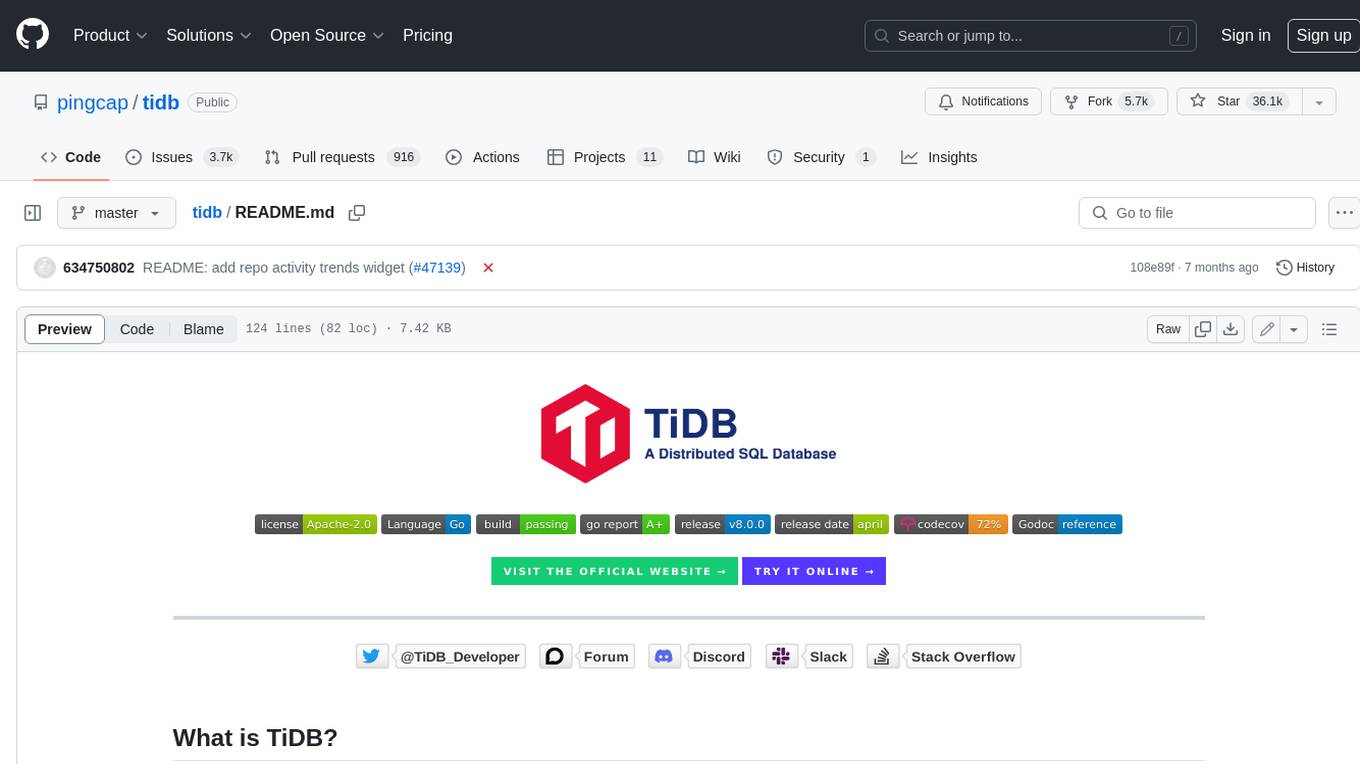
tidb
TiDB is an open-source distributed SQL database that supports Hybrid Transactional and Analytical Processing (HTAP) workloads. It is MySQL compatible and features horizontal scalability, strong consistency, and high availability.
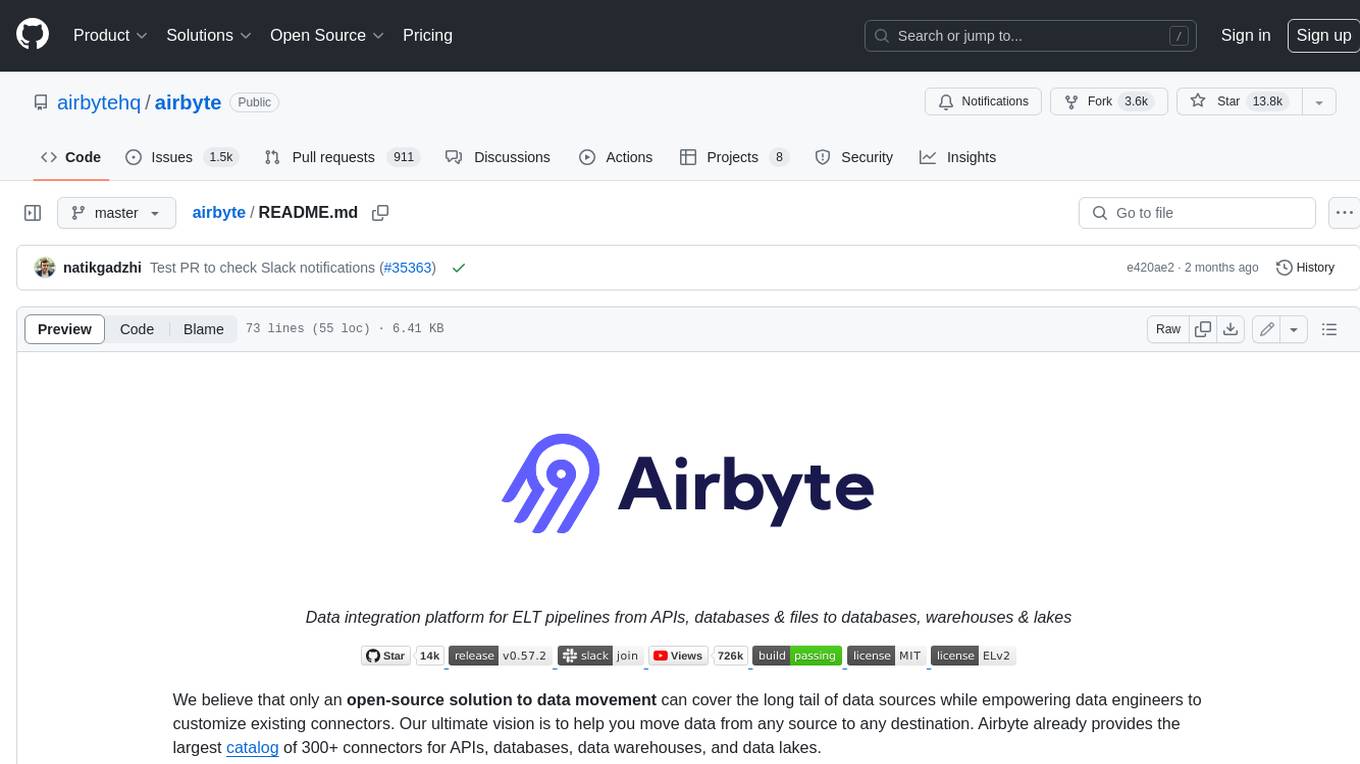
airbyte
Airbyte is an open-source data integration platform that makes it easy to move data from any source to any destination. With Airbyte, you can build and manage data pipelines without writing any code. Airbyte provides a library of pre-built connectors that make it easy to connect to popular data sources and destinations. You can also create your own connectors using Airbyte's no-code Connector Builder or low-code CDK. Airbyte is used by data engineers and analysts at companies of all sizes to build and manage their data pipelines.
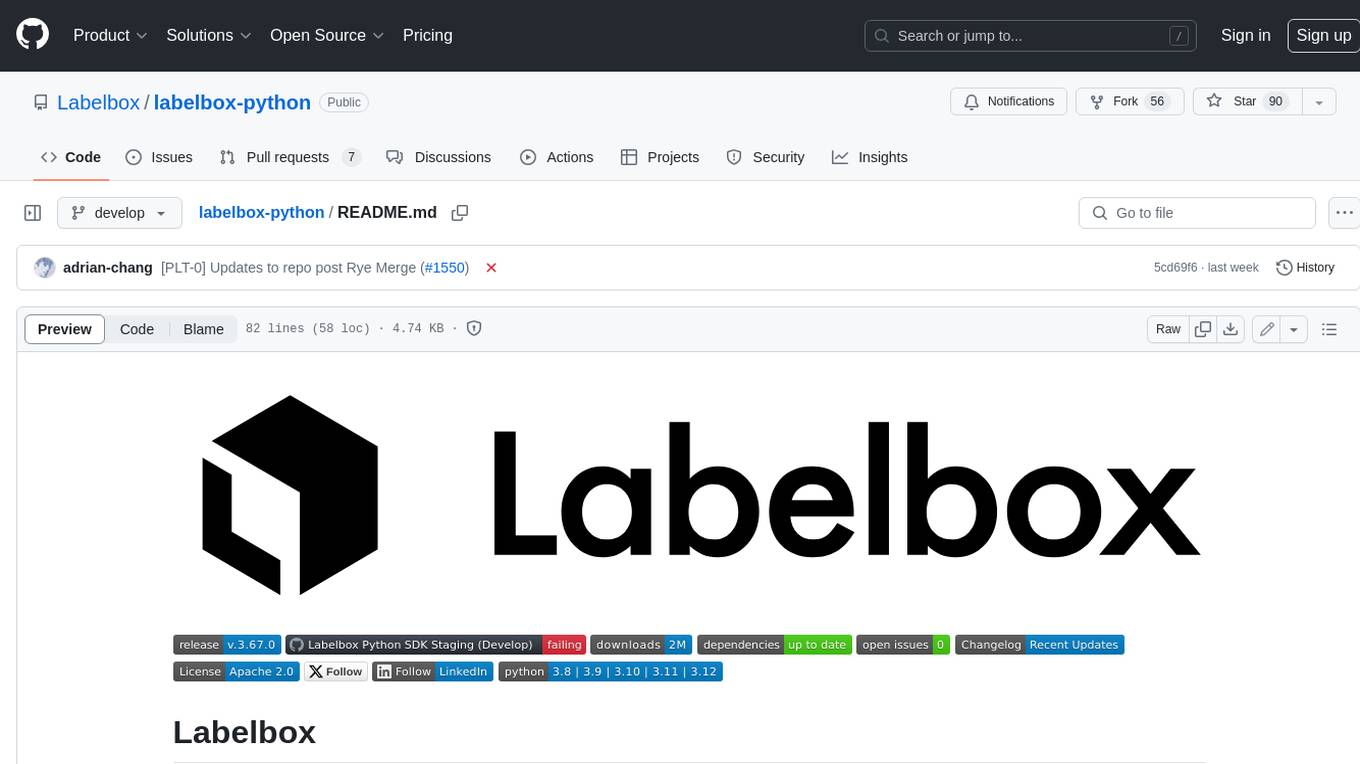
labelbox-python
Labelbox is a data-centric AI platform for enterprises to develop, optimize, and use AI to solve problems and power new products and services. Enterprises use Labelbox to curate data, generate high-quality human feedback data for computer vision and LLMs, evaluate model performance, and automate tasks by combining AI and human-centric workflows. The academic & research community uses Labelbox for cutting-edge AI research.