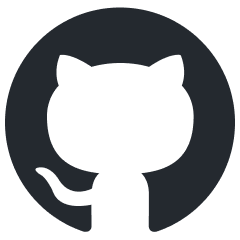
aiohttp-sse
Server-sent events support for aiohttp
Stars: 186
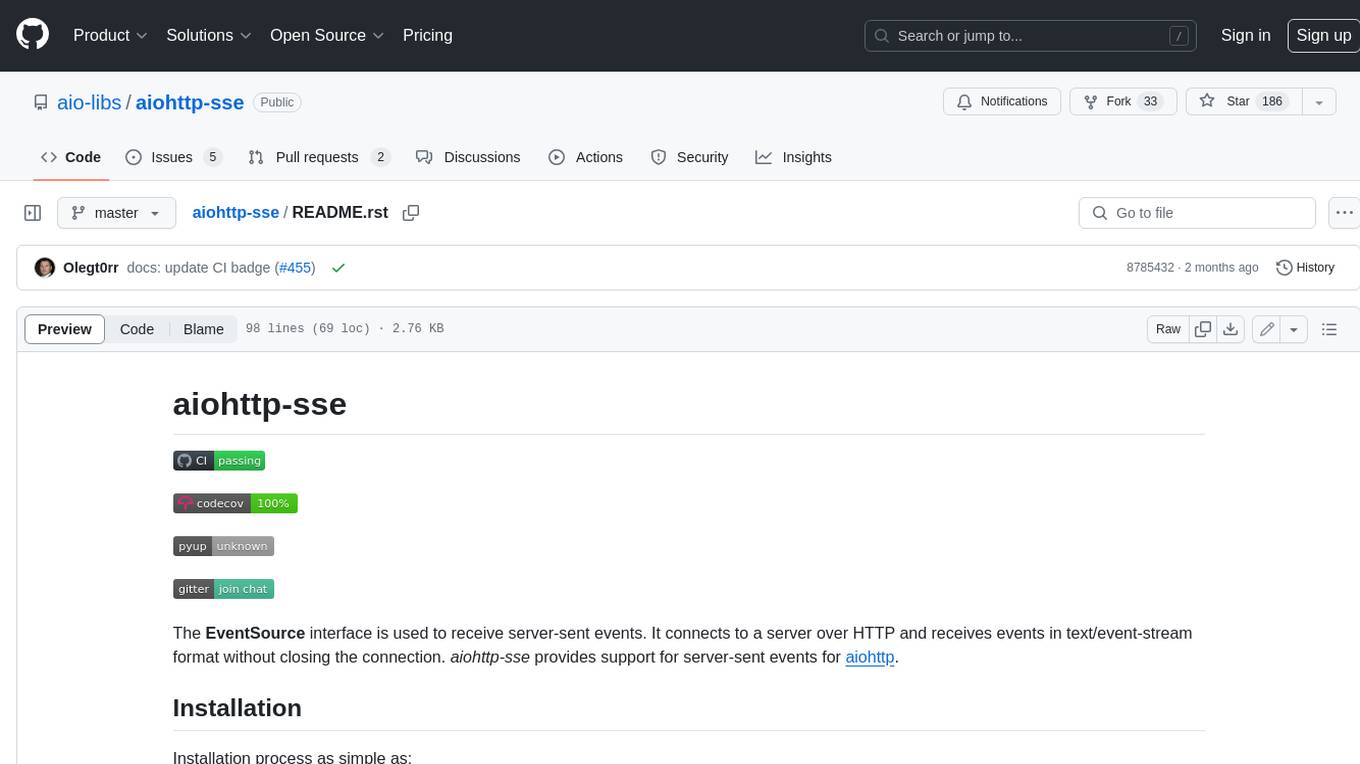
aiohttp-sse is a library that provides support for server-sent events for aiohttp. Server-sent events are a way to send real-time updates from a server to a client. This can be useful for things like live chat, stock tickers, or any other application where you need to send updates to a client without having to wait for the client to request them.
README:
.. image:: https://github.com/aio-libs/aiohttp-sse/workflows/CI/badge.svg?event=push :target: https://github.com/aio-libs/aiohttp-sse/actions?query=event%3Apush+branch%3Amaster
.. image:: https://codecov.io/gh/aio-libs/aiohttp-sse/branch/master/graph/badge.svg :target: https://codecov.io/gh/aio-libs/aiohttp-sse
.. image:: https://pyup.io/repos/github/aio-libs/aiohttp-sse/shield.svg :target: https://pyup.io/repos/github/aio-libs/aiohttp-sse/ :alt: Updates
.. image:: https://badges.gitter.im/Join%20Chat.svg :target: https://gitter.im/aio-libs/Lobby :alt: Chat on Gitter
The EventSource interface is used to receive server-sent events. It connects to a server over HTTP and receives events in text/event-stream format without closing the connection. aiohttp-sse provides support for server-sent events for aiohttp_.
Installation process as simple as::
$ pip install aiohttp-sse
.. code:: python
import asyncio
from datetime import datetime
from aiohttp import web
from aiohttp_sse import sse_response
async def hello(request: web.Request) -> web.StreamResponse:
async with sse_response(request) as resp:
while resp.is_connected():
time_dict = {"time": f"Server Time : {datetime.now()}"}
data = json.dumps(time_dict, indent=2)
print(data)
await resp.send(data)
await asyncio.sleep(1)
return resp
async def index(_request: web.Request) -> web.StreamResponse:
html = """
<html>
<body>
<script>
var eventSource = new EventSource("/hello");
eventSource.addEventListener("message", event => {
document.getElementById("response").innerText = event.data;
});
</script>
<h1>Response from server:</h1>
<div id="response"></div>
</body>
</html>
"""
return web.Response(text=html, content_type="text/html")
app = web.Application()
app.router.add_route("GET", "/hello", hello)
app.router.add_route("GET", "/", index)
web.run_app(app, host="127.0.0.1", port=8080)
- http://www.w3.org/TR/2011/WD-eventsource-20110310/
- https://developer.mozilla.org/en-US/docs/Server-sent_events/Using_server-sent_events
- aiohttp_ 3+
The aiohttp-sse is offered under Apache 2.0 license.
.. _Python: https://www.python.org .. _asyncio: http://docs.python.org/3/library/asyncio.html .. _aiohttp: https://github.com/aio-libs/aiohttp
For Tasks:
Click tags to check more tools for each tasksFor Jobs:
Alternative AI tools for aiohttp-sse
Similar Open Source Tools
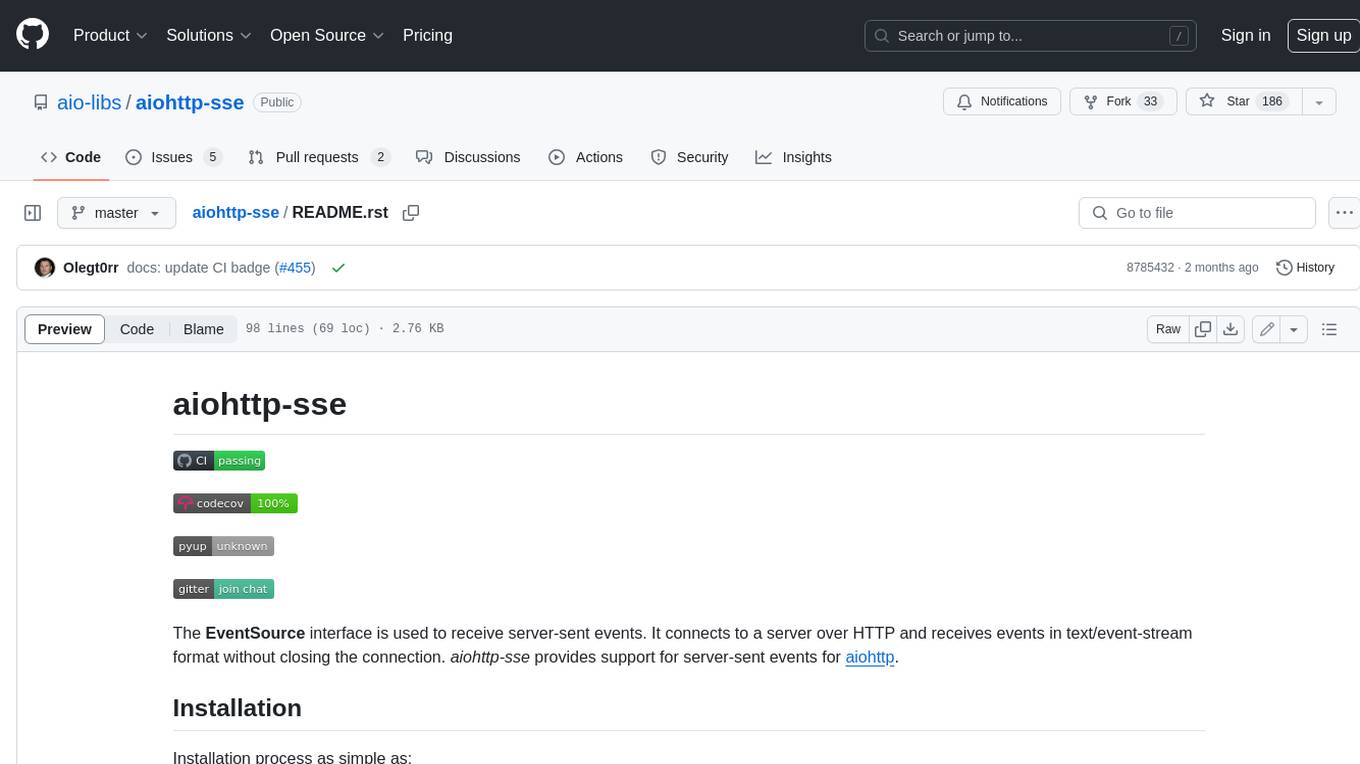
aiohttp-sse
aiohttp-sse is a library that provides support for server-sent events for aiohttp. Server-sent events are a way to send real-time updates from a server to a client. This can be useful for things like live chat, stock tickers, or any other application where you need to send updates to a client without having to wait for the client to request them.
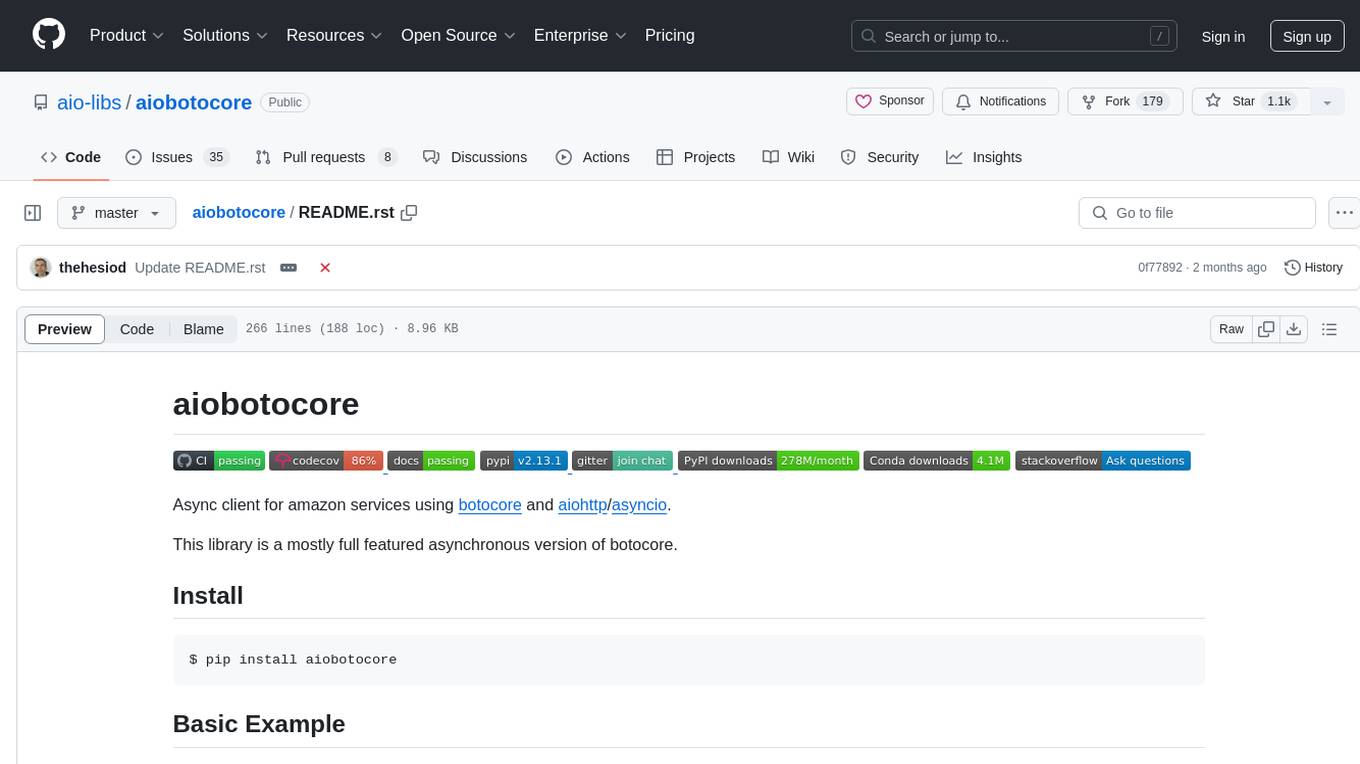
aiobotocore
aiobotocore is an async client for Amazon services using botocore and aiohttp/asyncio. It provides a mostly full-featured asynchronous version of botocore, allowing users to interact with various AWS services asynchronously. The library supports operations such as uploading objects to S3, getting object properties, listing objects, and deleting objects. It also offers context manager examples for managing resources efficiently. aiobotocore supports multiple AWS services like S3, DynamoDB, SNS, SQS, CloudFormation, and Kinesis, with basic methods tested for each service. Users can run tests using moto for mocked tests or against personal Amazon keys. Additionally, the tool enables type checking and code completion for better development experience.
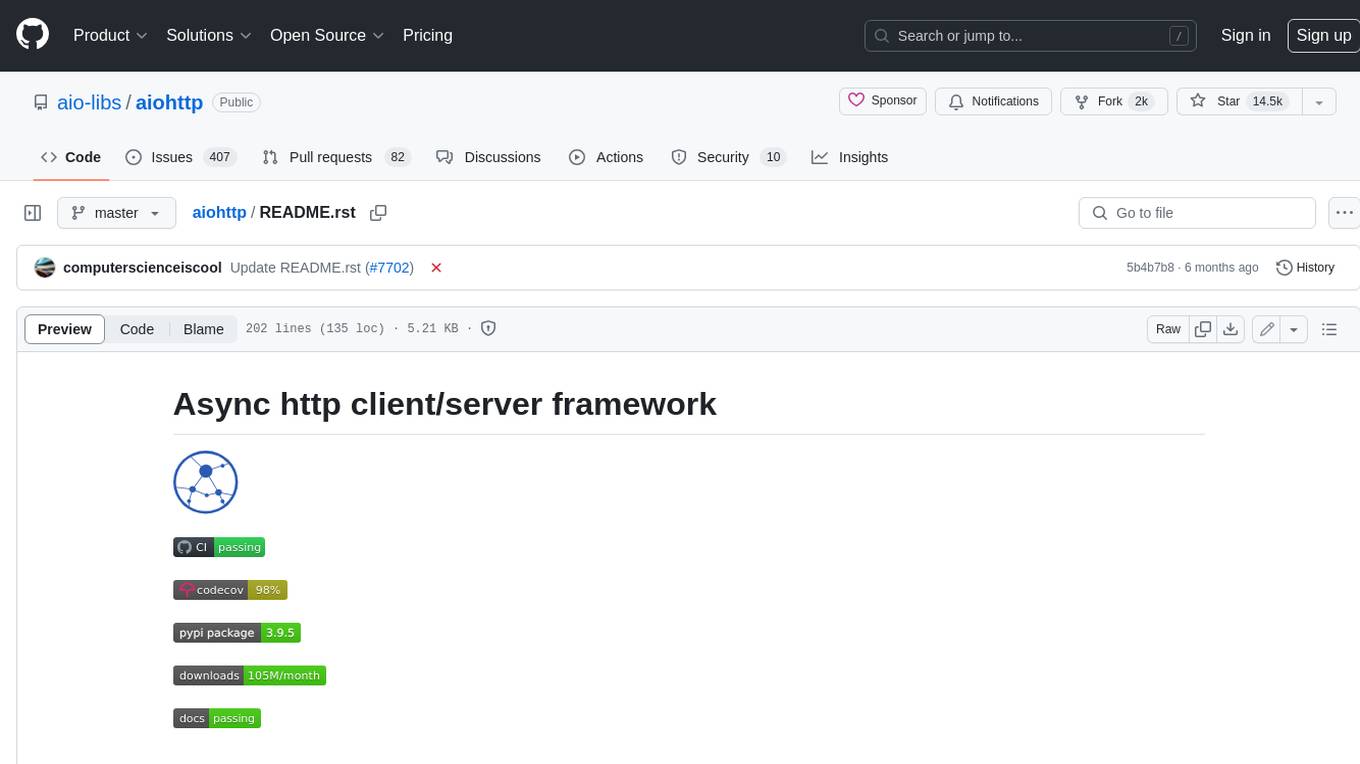
aiohttp
aiohttp is an async http client/server framework that supports both client and server side of HTTP protocol. It also supports both client and server Web-Sockets out-of-the-box and avoids Callback Hell. aiohttp provides a Web-server with middleware and pluggable routing.
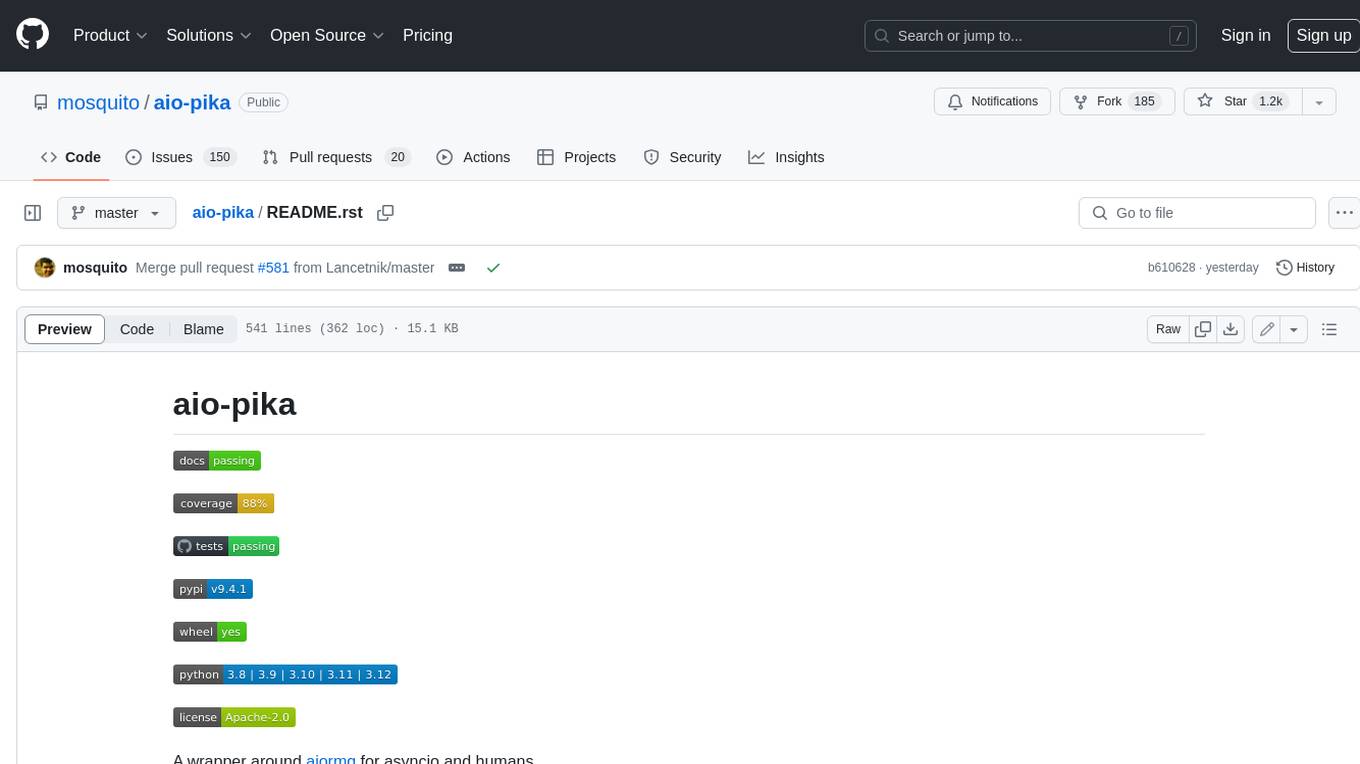
aio-pika
Aio-pika is a wrapper around aiormq for asyncio and humans. It provides a completely asynchronous API, object-oriented API, transparent auto-reconnects with complete state recovery, Python 3.7+ compatibility, transparent publisher confirms support, transactions support, and complete type-hints coverage.
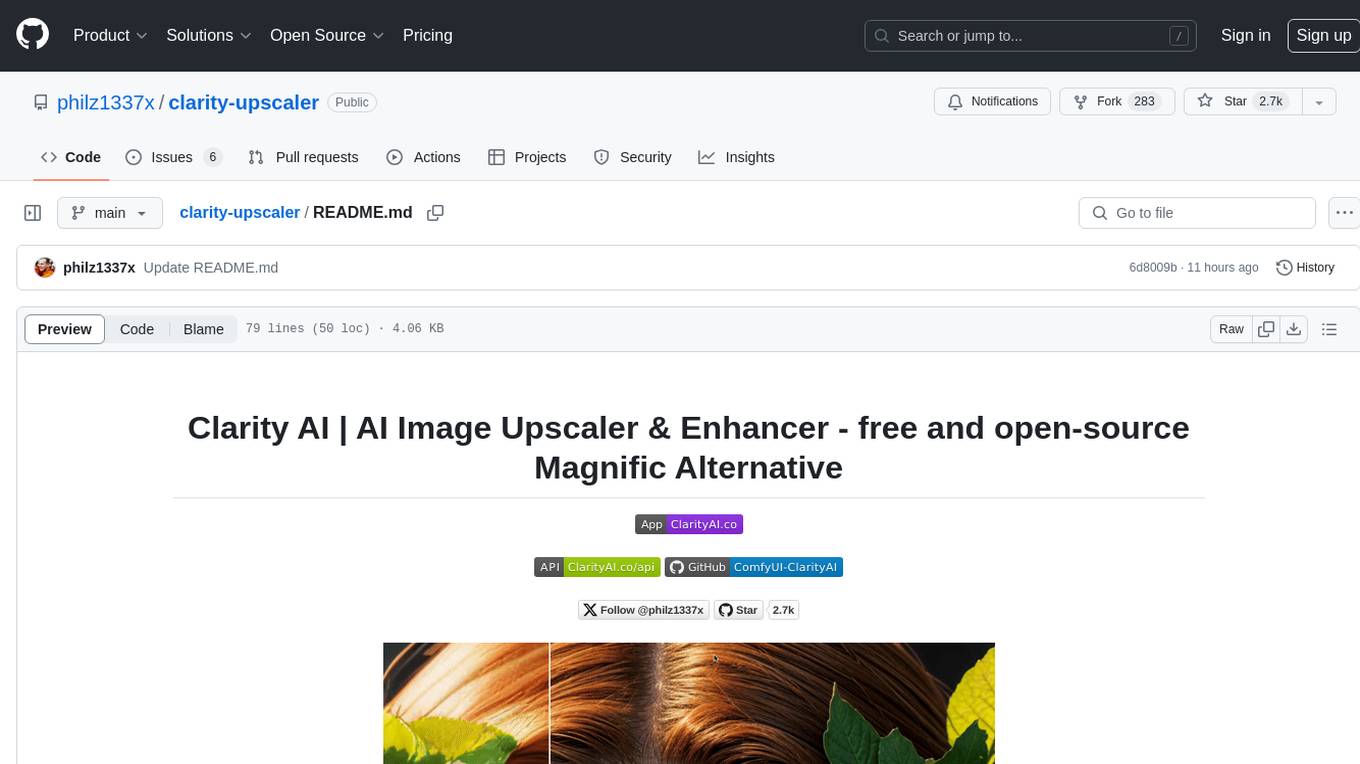
clarity-upscaler
Clarity AI is a free and open-source AI image upscaler and enhancer, providing an alternative to Magnific. It offers various features such as multi-step upscaling, resemblance fixing, speed improvements, support for custom safetensors checkpoints, anime upscaling, LoRa support, pre-downscaling, and fractality. Users can access the tool through the ClarityAI.co app, ComfyUI manager, API, or by deploying and running locally or in the cloud with cog or A1111 webUI. The tool aims to enhance image quality and resolution using advanced AI algorithms and models.
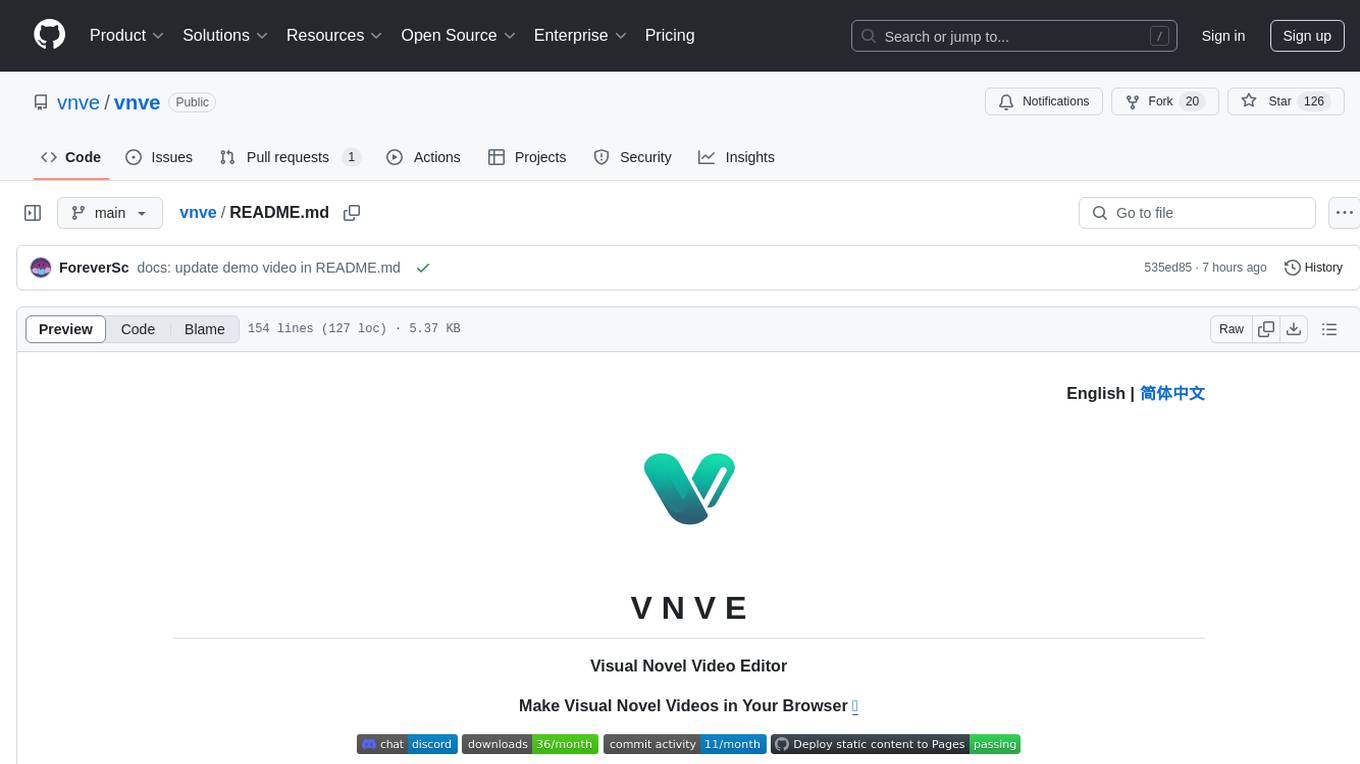
vnve
VNVE is a Visual Novel Video Editor that allows users to create visual novel videos in their browser with AI-powered rapid creation. It offers a low-cost production solution for converting textual content into videos, creating interactive videos for gaming experiences, and making video teasers for novels and short video dramas. The tool is a pure front-end Typescript implementation powered by PixiJS + WebCodecs, and users can also create videos programmatically using the npm package. VNVE is tailored specifically for visual novels, focusing on text content and simplifying the video creation process for users.
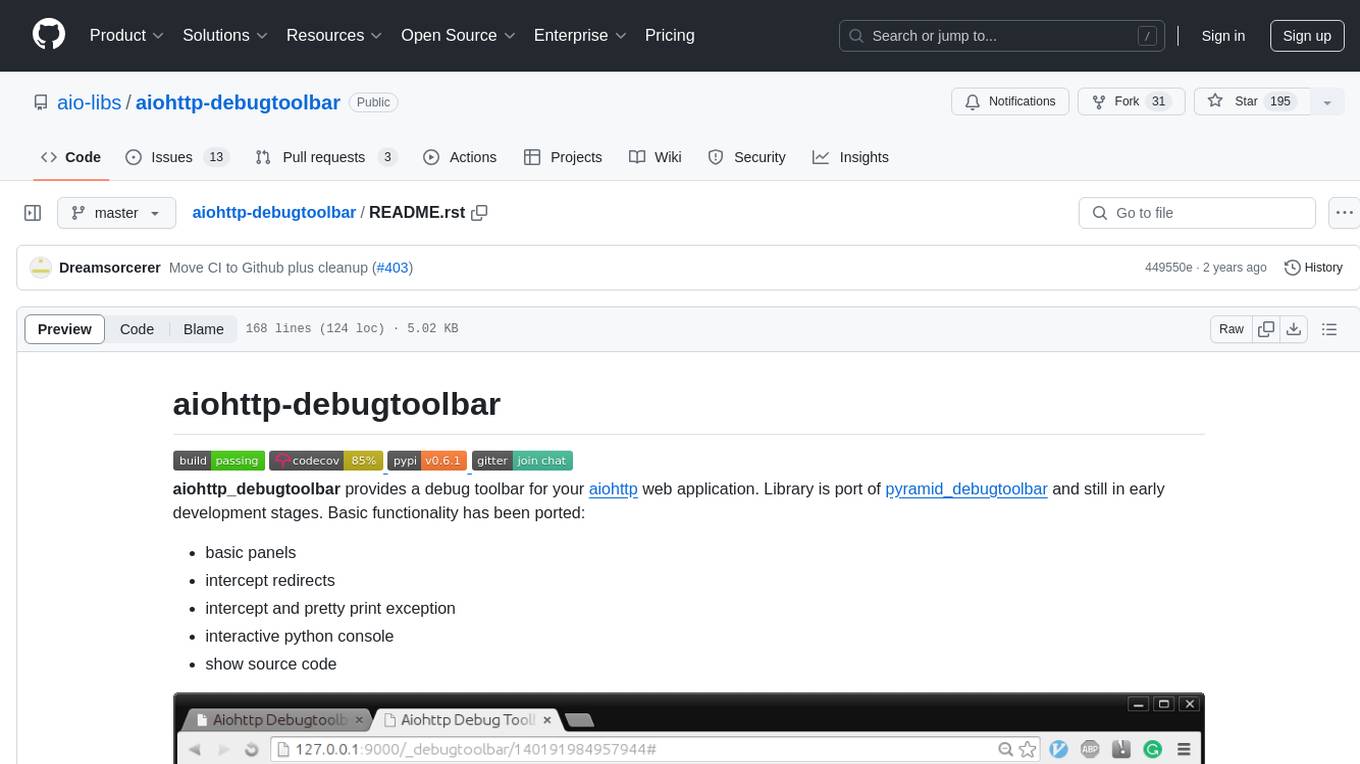
aiohttp-debugtoolbar
aiohttp_debugtoolbar provides a debug toolbar for aiohttp web applications. It is a port of pyramid_debugtoolbar and offers basic functionality such as basic panels, intercepting redirects, pretty printing exceptions, an interactive python console, and showing source code. The library is still in early development stages and offers various debug panels for monitoring different aspects of the web application. It is a useful tool for developers working with aiohttp to debug and optimize their applications.
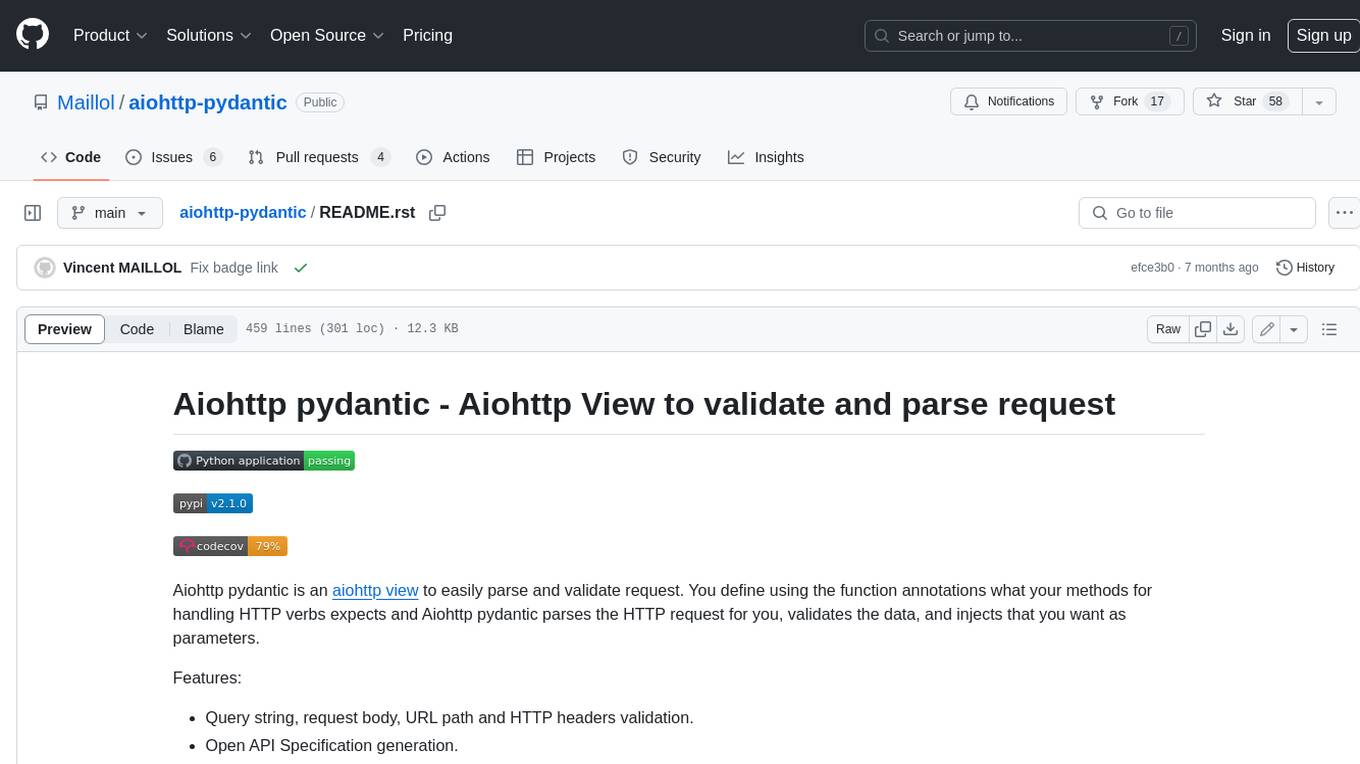
aiohttp-pydantic
Aiohttp pydantic is an aiohttp view to easily parse and validate requests. You define using function annotations what your methods for handling HTTP verbs expect, and Aiohttp pydantic parses the HTTP request for you, validates the data, and injects the parameters you want. It provides features like query string, request body, URL path, and HTTP headers validation, as well as Open API Specification generation.
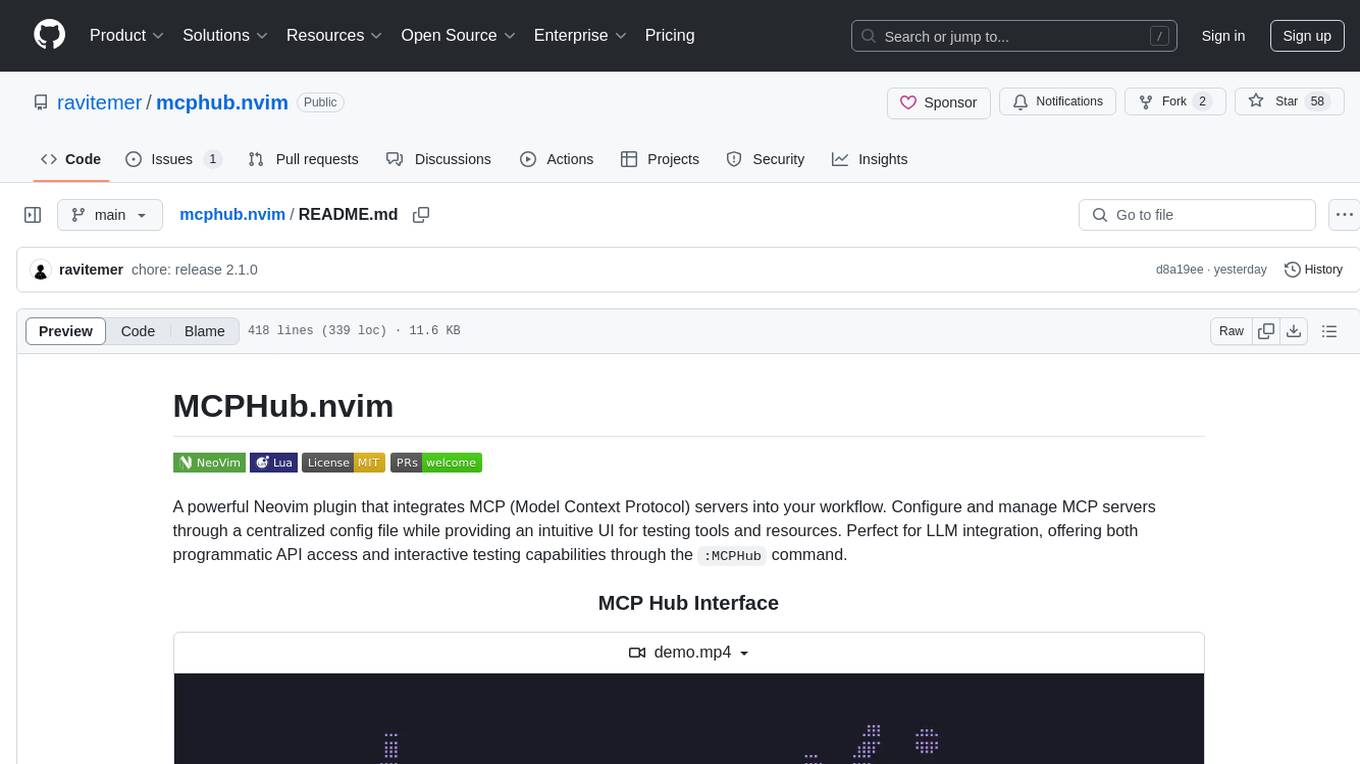
mcphub.nvim
MCPHub.nvim is a powerful Neovim plugin that integrates MCP (Model Context Protocol) servers into your workflow. It offers a centralized config file for managing servers and tools, with an intuitive UI for testing resources. Ideal for LLM integration, it provides programmatic API access and interactive testing through the `:MCPHub` command.
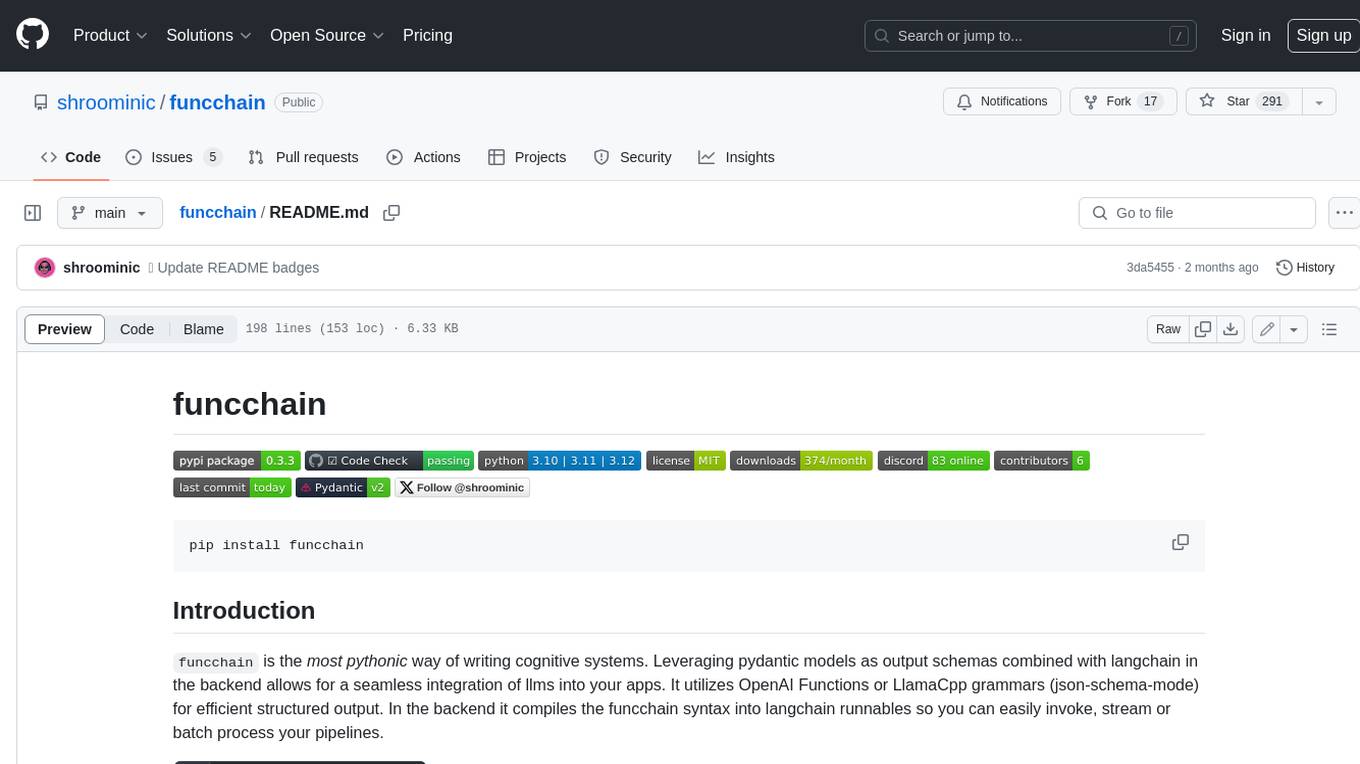
funcchain
Funcchain is a Python library that allows you to easily write cognitive systems by leveraging Pydantic models as output schemas and LangChain in the backend. It provides a seamless integration of LLMs into your apps, utilizing OpenAI Functions or LlamaCpp grammars (json-schema-mode) for efficient structured output. Funcchain compiles the Funcchain syntax into LangChain runnables, enabling you to invoke, stream, or batch process your pipelines effortlessly.
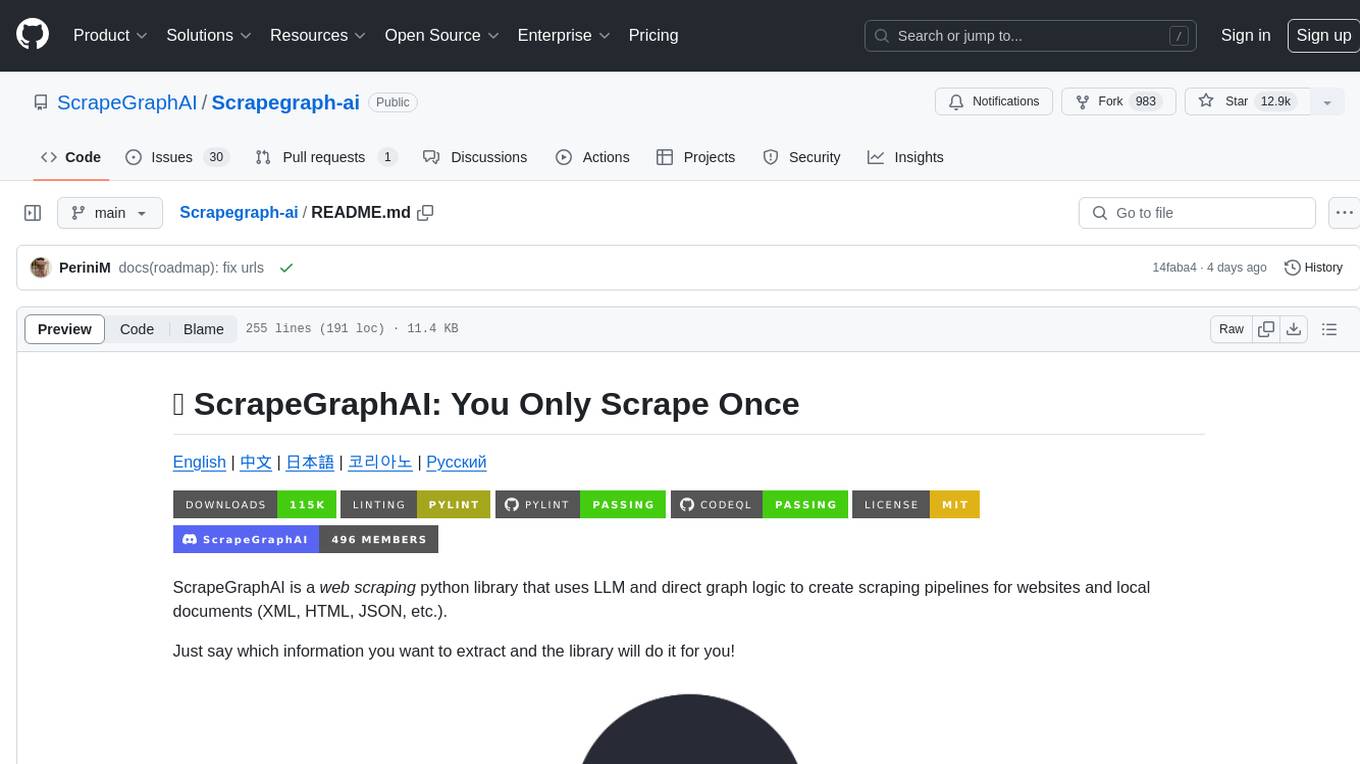
Scrapegraph-ai
ScrapeGraphAI is a web scraping Python library that utilizes LLM and direct graph logic to create scraping pipelines for websites and local documents. It offers various standard scraping pipelines like SmartScraperGraph, SearchGraph, SpeechGraph, and ScriptCreatorGraph. Users can extract information by specifying prompts and input sources. The library supports different LLM APIs such as OpenAI, Groq, Azure, and Gemini, as well as local models using Ollama. ScrapeGraphAI is designed for data exploration and research purposes, providing a versatile tool for extracting information from web pages and generating outputs like Python scripts, audio summaries, and search results.
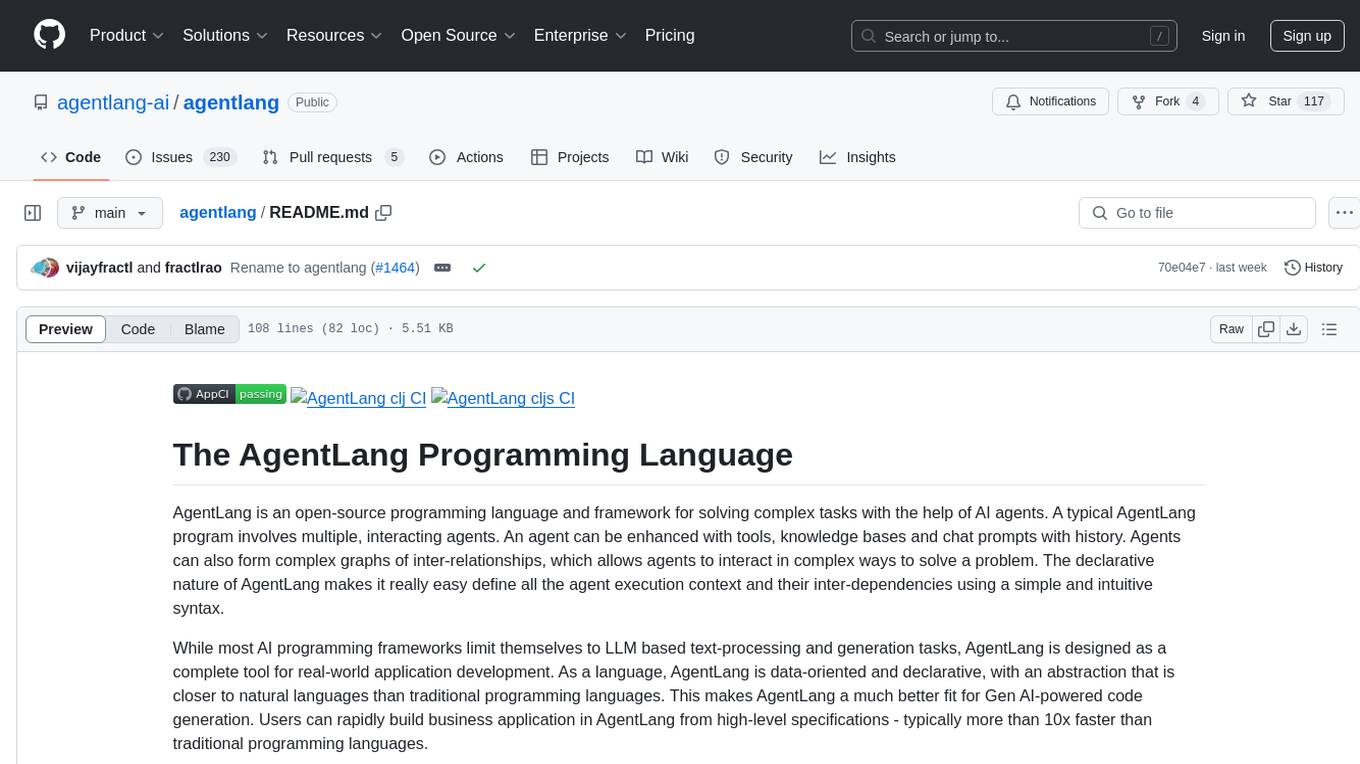
agentlang
AgentLang is an open-source programming language and framework designed for solving complex tasks with the help of AI agents. It allows users to build business applications rapidly from high-level specifications, making it more efficient than traditional programming languages. The language is data-oriented and declarative, with a syntax that is intuitive and closer to natural languages. AgentLang introduces innovative concepts such as first-class AI agents, graph-based hierarchical data model, zero-trust programming, declarative dataflow, resolvers, interceptors, and entity-graph-database mapping.
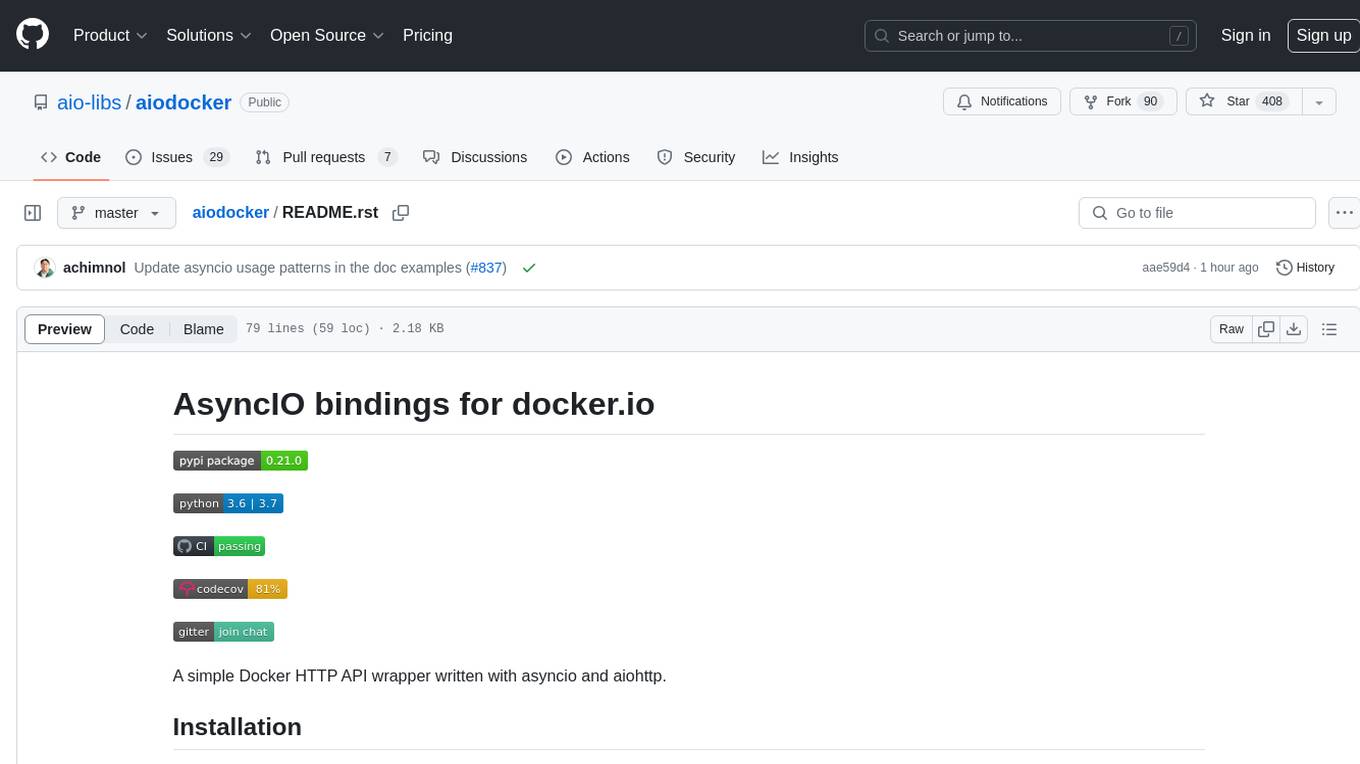
aiodocker
Aiodocker is a simple Docker HTTP API wrapper written with asyncio and aiohttp. It provides asynchronous bindings for interacting with Docker containers and images. Users can easily manage Docker resources using async functions and methods. The library offers features such as listing images and containers, creating and running containers, and accessing container logs. Aiodocker is designed to work seamlessly with Python's asyncio framework, making it suitable for building asynchronous Docker management applications.
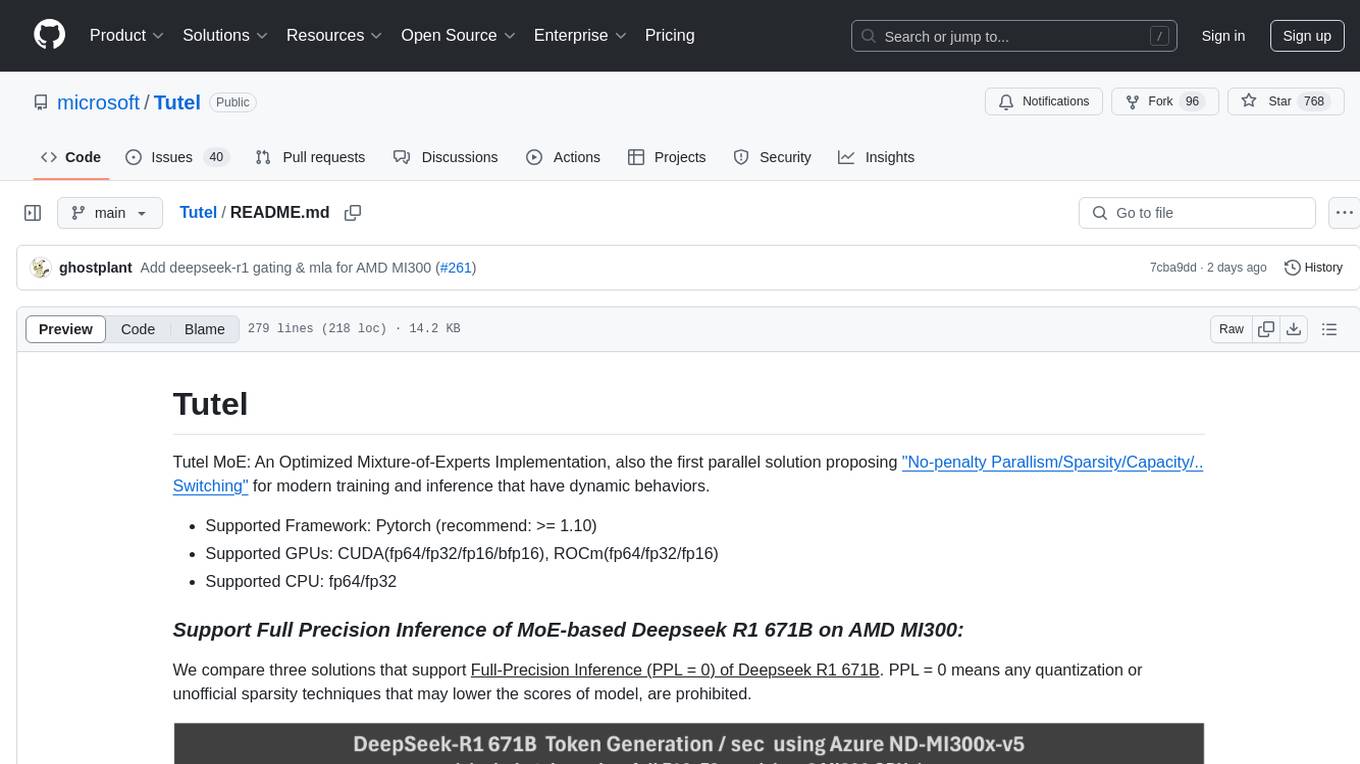
Tutel
Tutel MoE is an optimized Mixture-of-Experts implementation that offers a parallel solution with 'No-penalty Parallism/Sparsity/Capacity/Switching' for modern training and inference. It supports Pytorch framework (version >= 1.10) and various GPUs including CUDA and ROCm. The tool enables Full Precision Inference of MoE-based Deepseek R1 671B on AMD MI300. Tutel provides features like all-to-all benchmarking, tensorcore option, NCCL timeout settings, Megablocks solution, and dynamic switchable configurations. Users can run Tutel in distributed mode across multiple GPUs and machines. The tool allows for custom MoE implementations and offers detailed usage examples and reference documentation.
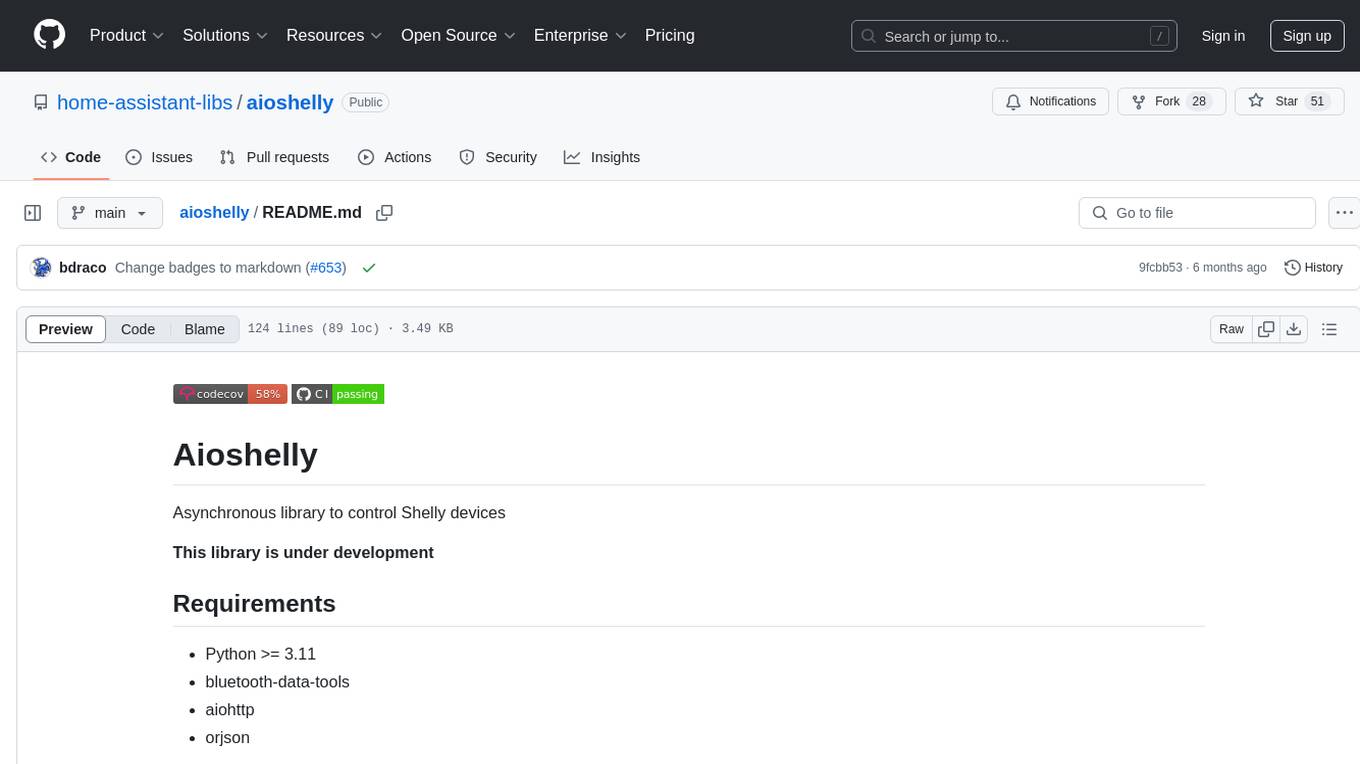
aioshelly
Aioshelly is an asynchronous library designed to control Shelly devices. It is currently under development and requires Python version 3.11 or higher, along with dependencies like bluetooth-data-tools, aiohttp, and orjson. The library provides examples for interacting with Gen1 devices using CoAP protocol and Gen2/Gen3 devices using RPC and WebSocket protocols. Users can easily connect to Shelly devices, retrieve status information, and perform various actions through the provided APIs. The repository also includes example scripts for quick testing and usage guidelines for contributors to maintain consistency with the Shelly API.
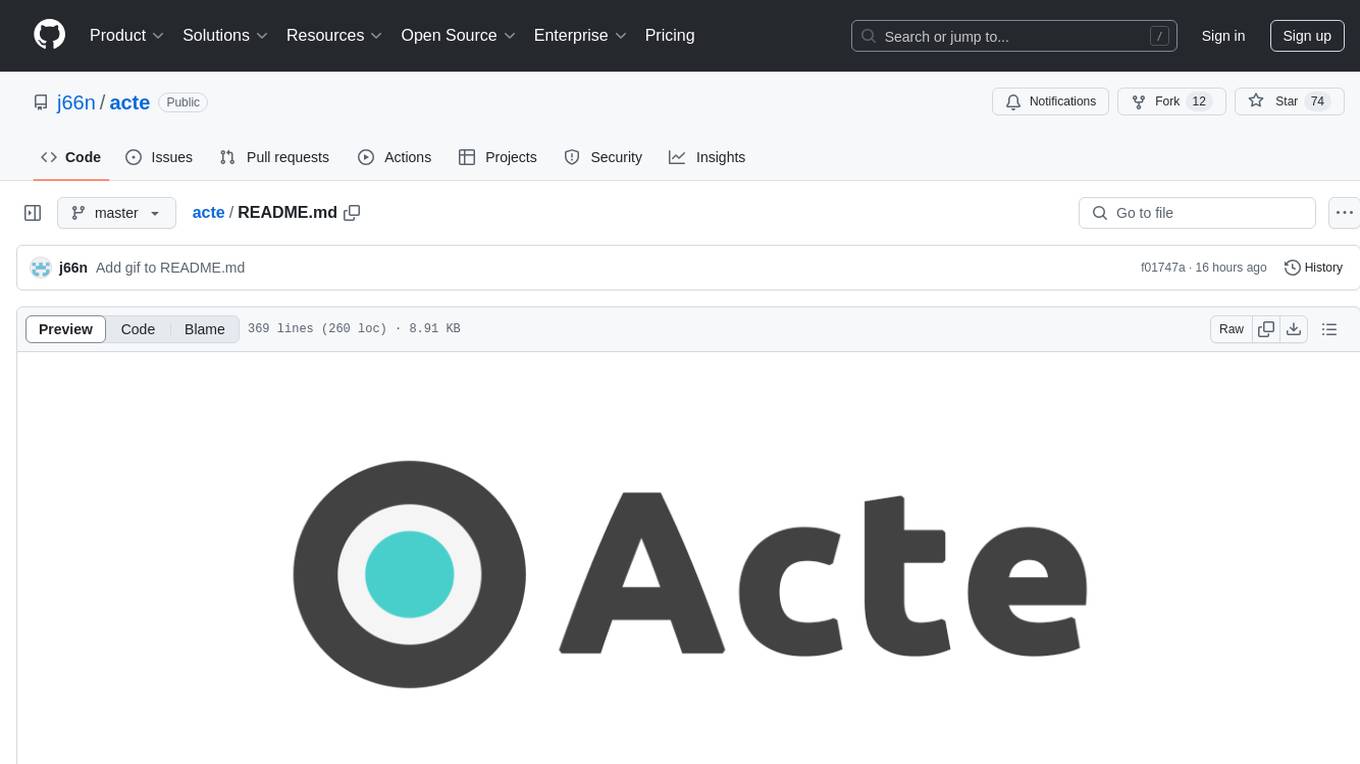
acte
Acte is a framework designed to build GUI-like tools for AI Agents. It aims to address the issues of cognitive load and freedom degrees when interacting with multiple APIs in complex scenarios. By providing a graphical user interface (GUI) for Agents, Acte helps reduce cognitive load and constraints interaction, similar to how humans interact with computers through GUIs. The tool offers APIs for starting new sessions, executing actions, and displaying screens, accessible via HTTP requests or the SessionManager class.
For similar tasks
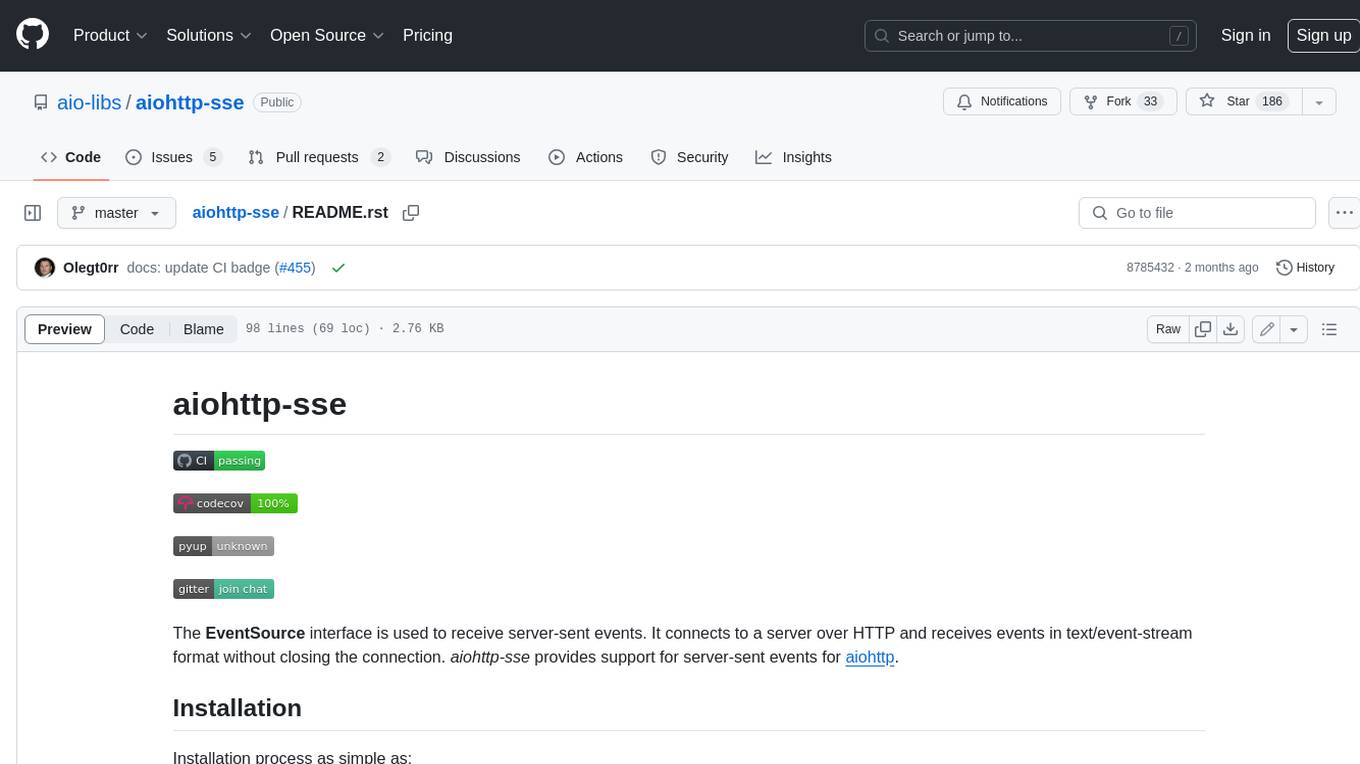
aiohttp-sse
aiohttp-sse is a library that provides support for server-sent events for aiohttp. Server-sent events are a way to send real-time updates from a server to a client. This can be useful for things like live chat, stock tickers, or any other application where you need to send updates to a client without having to wait for the client to request them.
For similar jobs
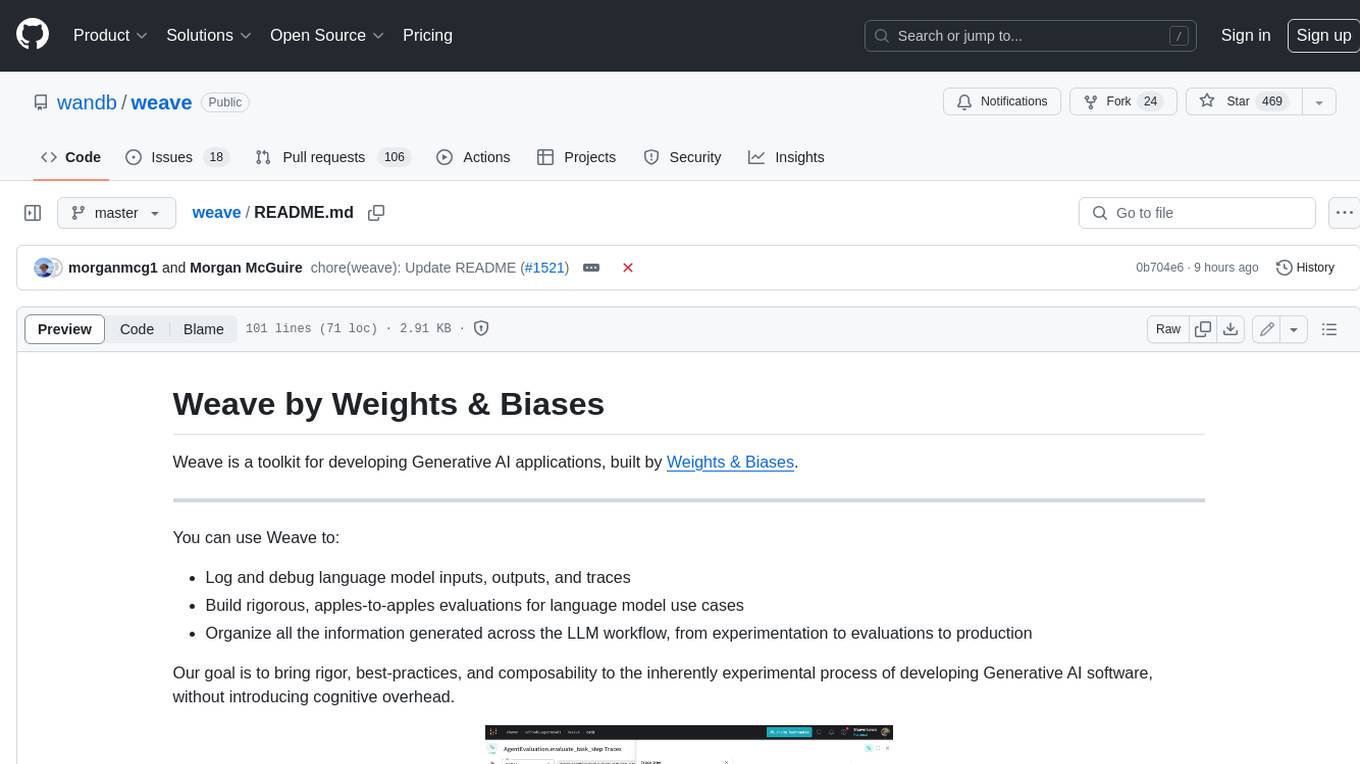
weave
Weave is a toolkit for developing Generative AI applications, built by Weights & Biases. With Weave, you can log and debug language model inputs, outputs, and traces; build rigorous, apples-to-apples evaluations for language model use cases; and organize all the information generated across the LLM workflow, from experimentation to evaluations to production. Weave aims to bring rigor, best-practices, and composability to the inherently experimental process of developing Generative AI software, without introducing cognitive overhead.
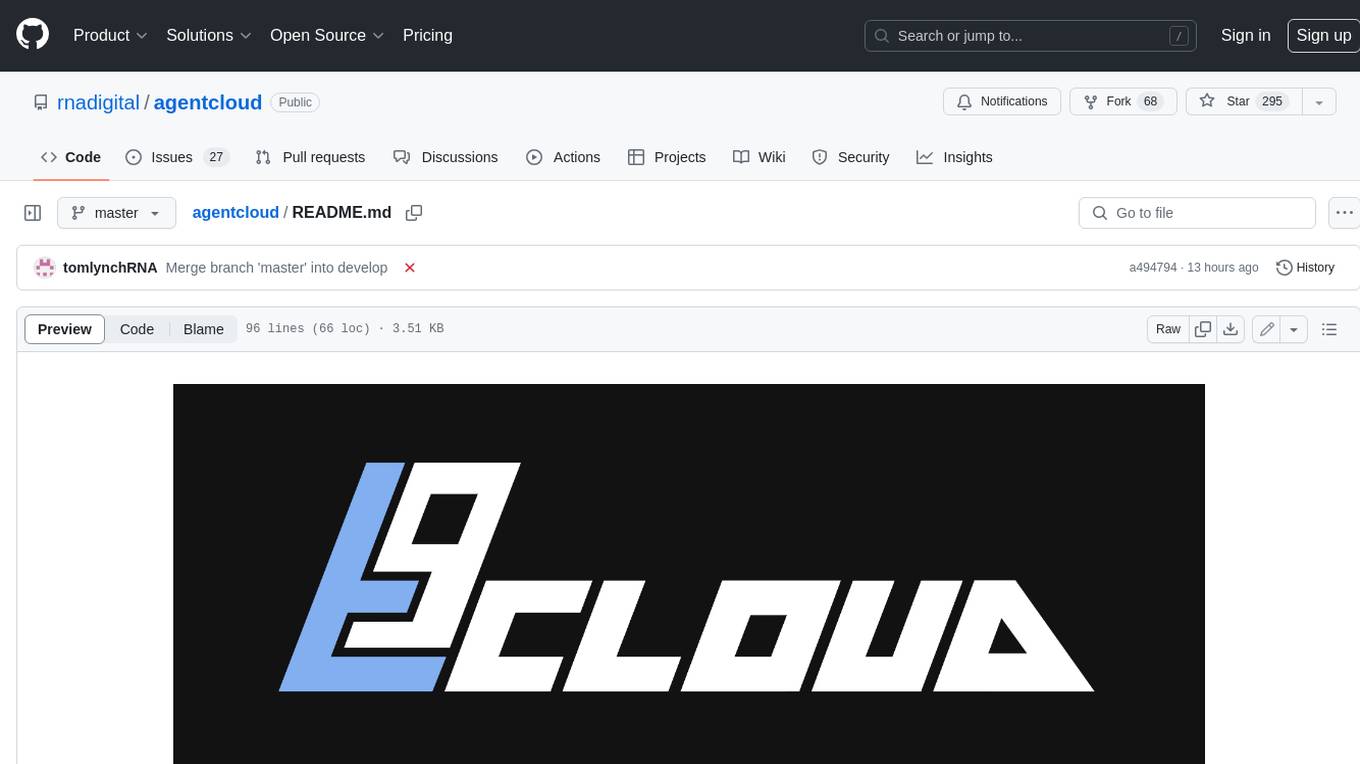
agentcloud
AgentCloud is an open-source platform that enables companies to build and deploy private LLM chat apps, empowering teams to securely interact with their data. It comprises three main components: Agent Backend, Webapp, and Vector Proxy. To run this project locally, clone the repository, install Docker, and start the services. The project is licensed under the GNU Affero General Public License, version 3 only. Contributions and feedback are welcome from the community.
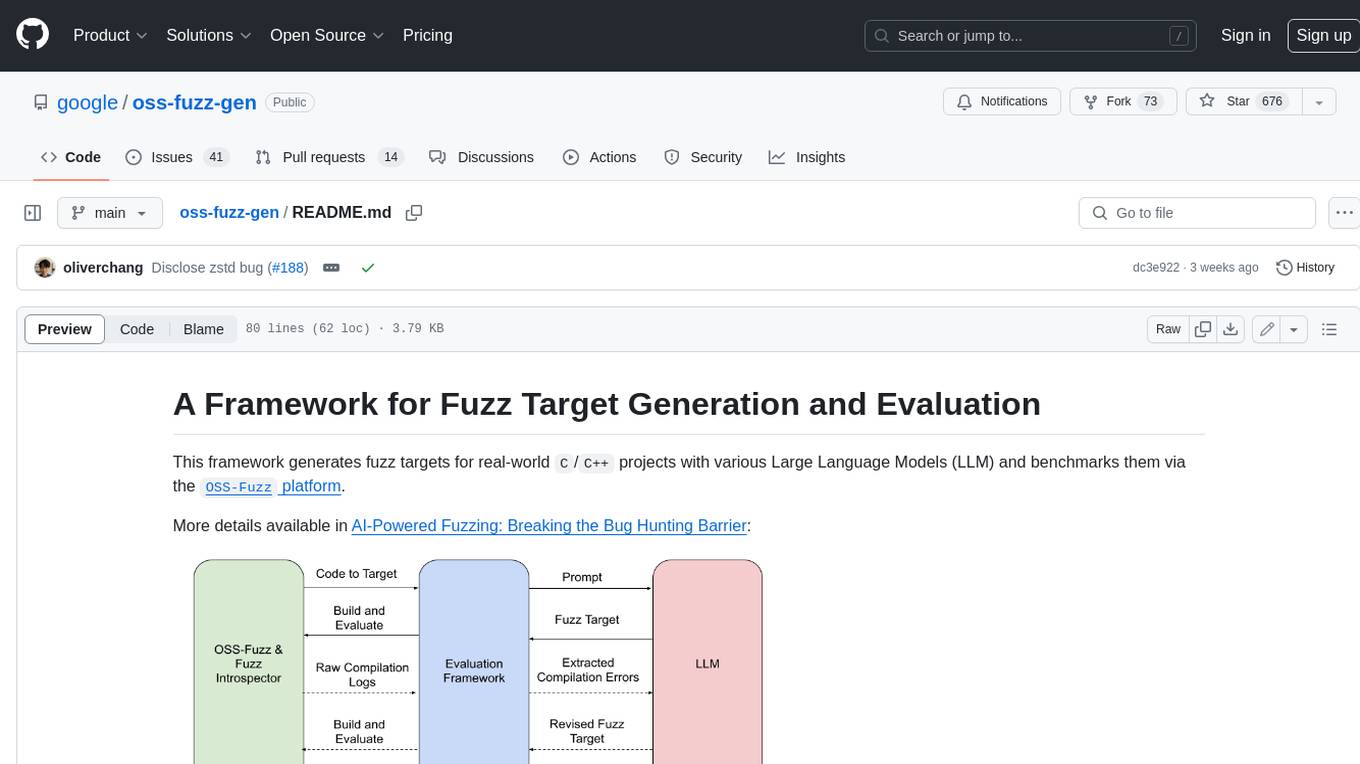
oss-fuzz-gen
This framework generates fuzz targets for real-world `C`/`C++` projects with various Large Language Models (LLM) and benchmarks them via the `OSS-Fuzz` platform. It manages to successfully leverage LLMs to generate valid fuzz targets (which generate non-zero coverage increase) for 160 C/C++ projects. The maximum line coverage increase is 29% from the existing human-written targets.
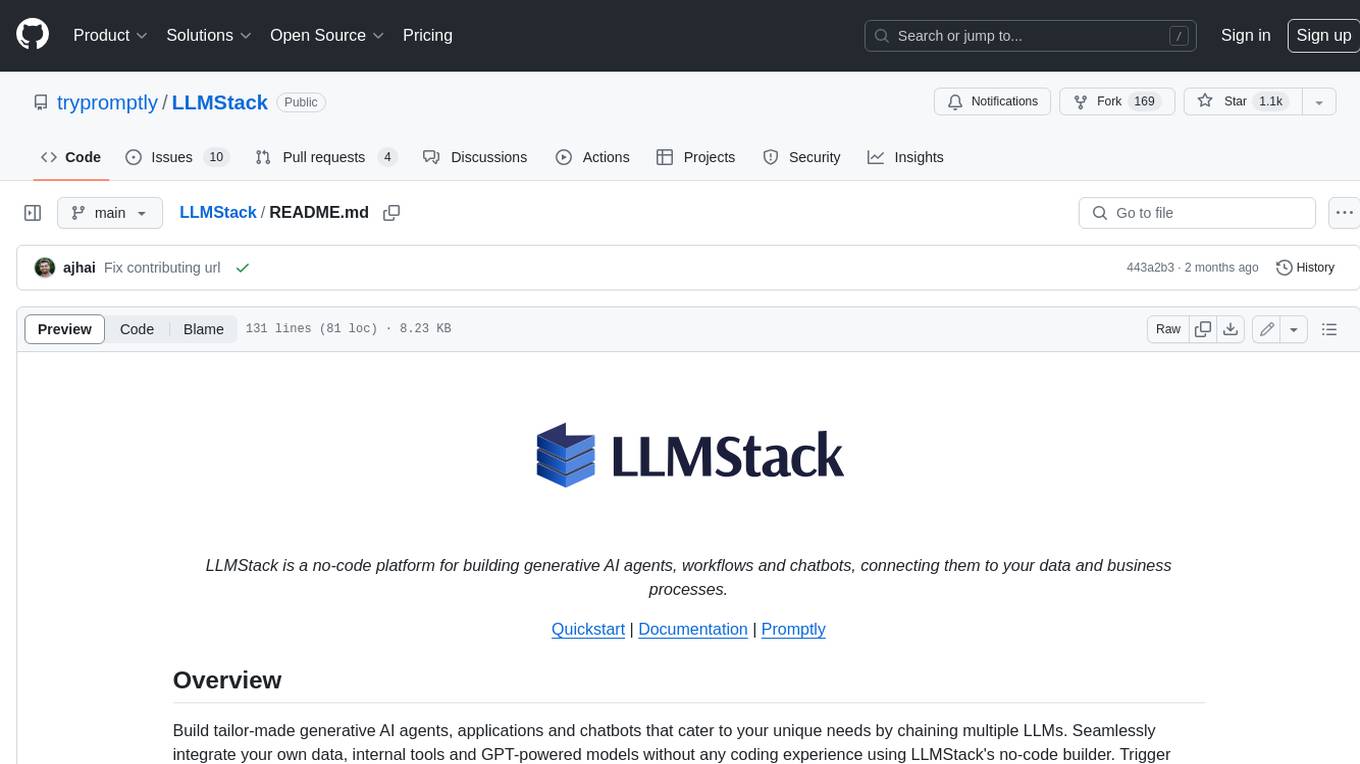
LLMStack
LLMStack is a no-code platform for building generative AI agents, workflows, and chatbots. It allows users to connect their own data, internal tools, and GPT-powered models without any coding experience. LLMStack can be deployed to the cloud or on-premise and can be accessed via HTTP API or triggered from Slack or Discord.
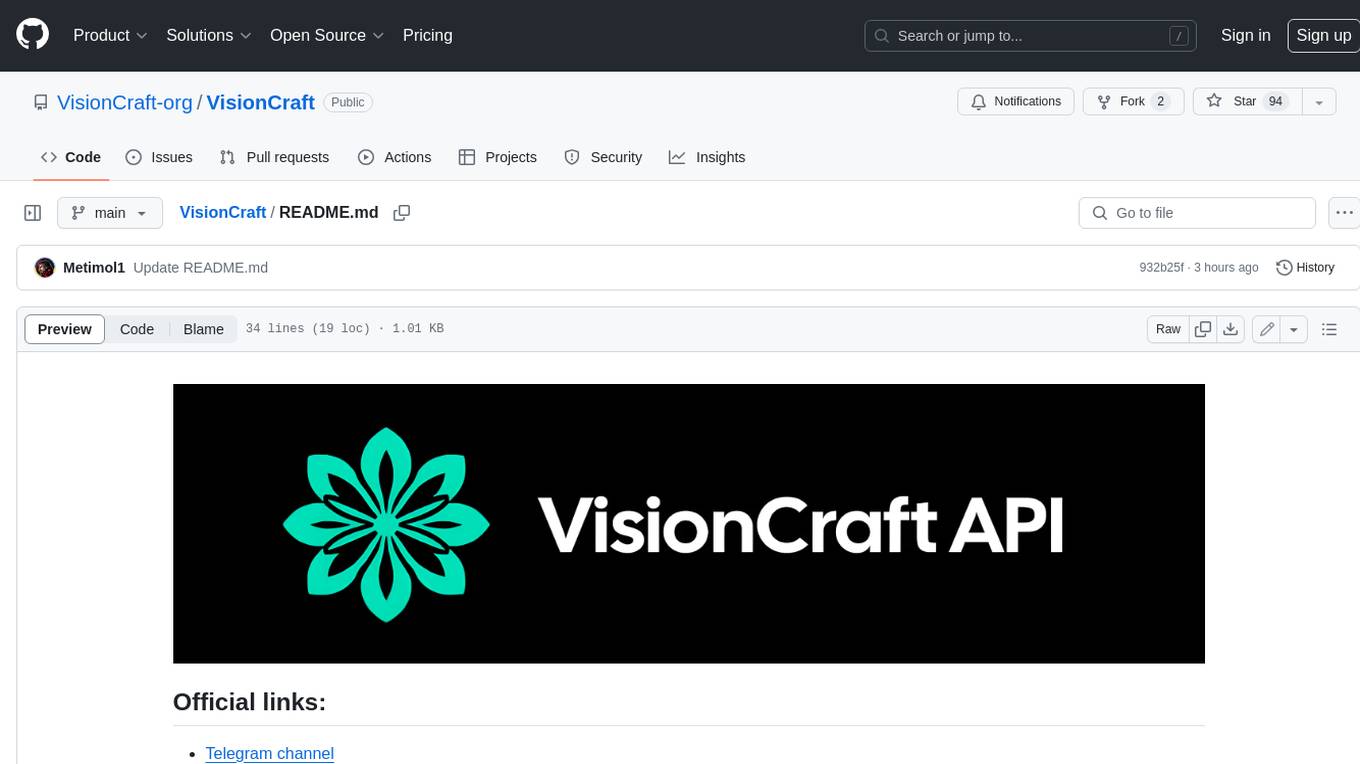
VisionCraft
The VisionCraft API is a free API for using over 100 different AI models. From images to sound.
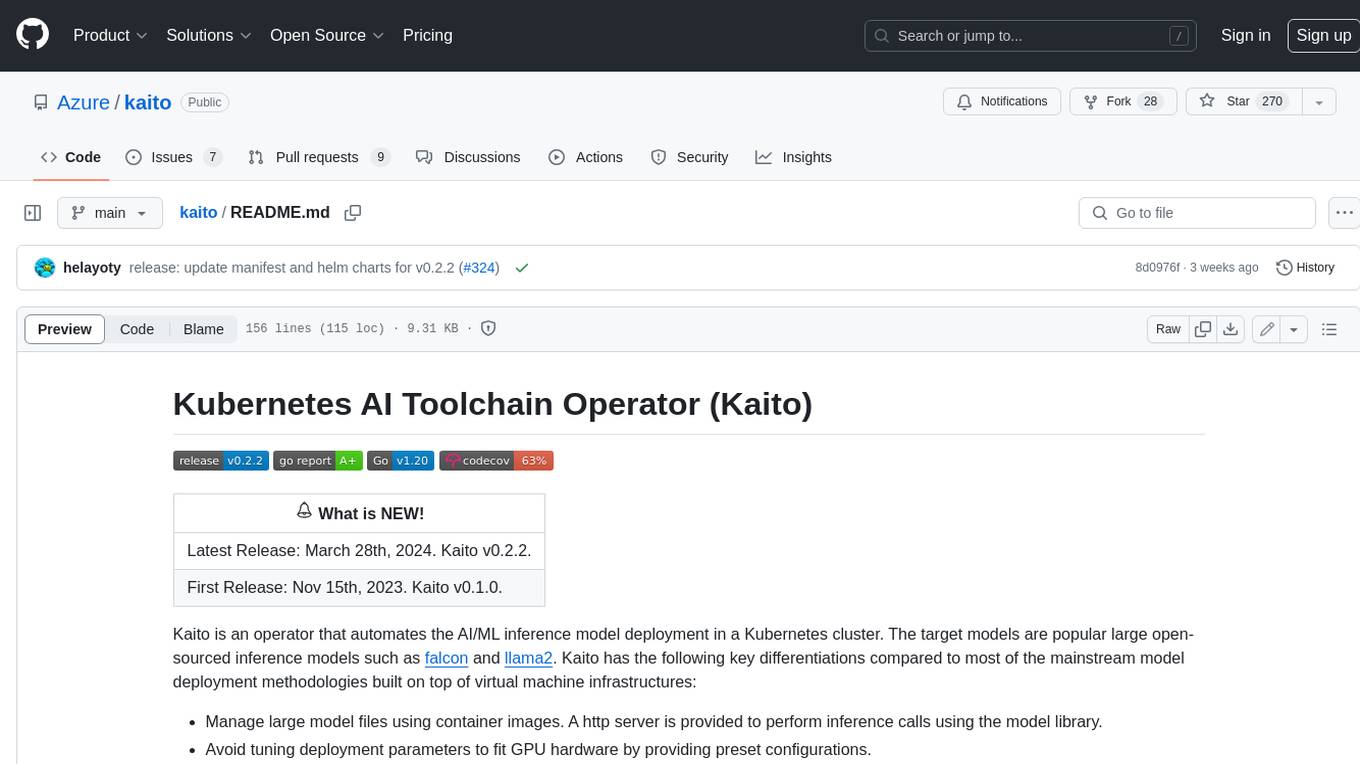
kaito
Kaito is an operator that automates the AI/ML inference model deployment in a Kubernetes cluster. It manages large model files using container images, avoids tuning deployment parameters to fit GPU hardware by providing preset configurations, auto-provisions GPU nodes based on model requirements, and hosts large model images in the public Microsoft Container Registry (MCR) if the license allows. Using Kaito, the workflow of onboarding large AI inference models in Kubernetes is largely simplified.
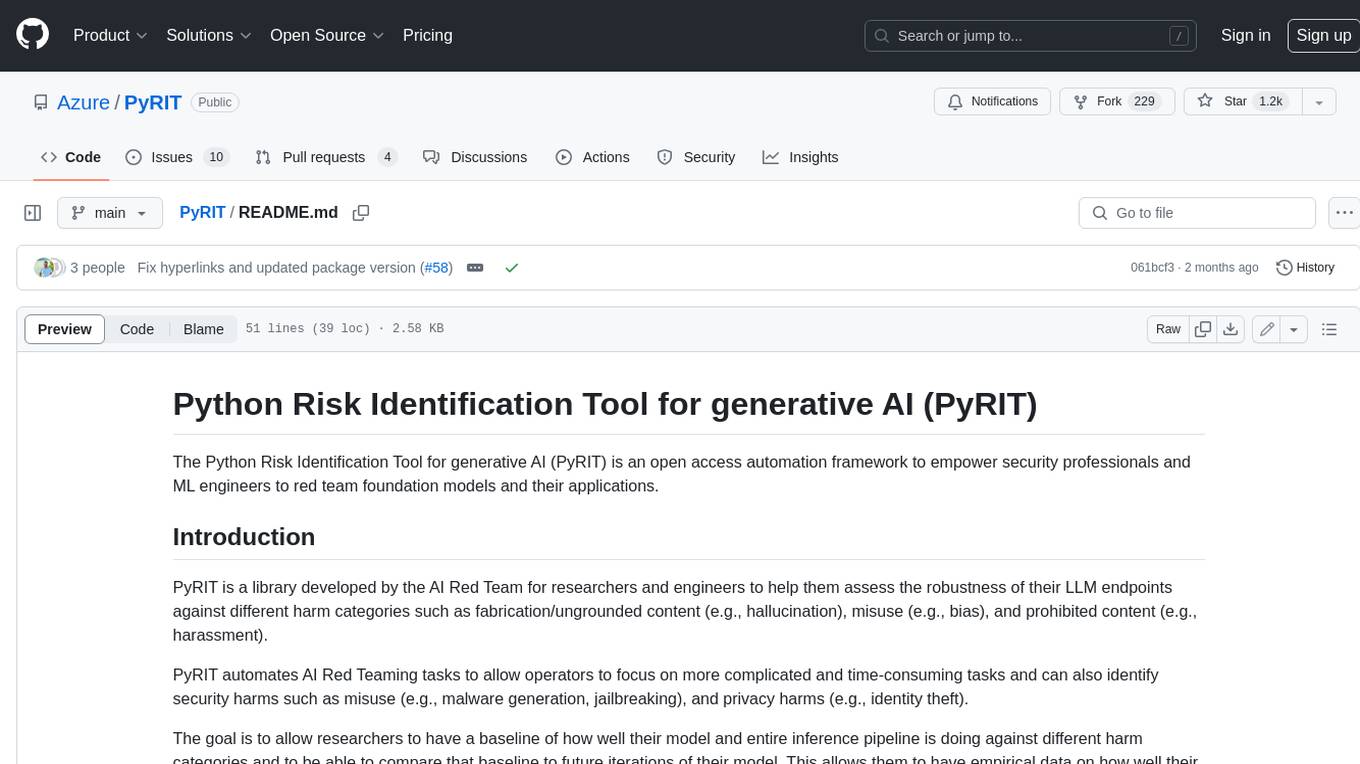
PyRIT
PyRIT is an open access automation framework designed to empower security professionals and ML engineers to red team foundation models and their applications. It automates AI Red Teaming tasks to allow operators to focus on more complicated and time-consuming tasks and can also identify security harms such as misuse (e.g., malware generation, jailbreaking), and privacy harms (e.g., identity theft). The goal is to allow researchers to have a baseline of how well their model and entire inference pipeline is doing against different harm categories and to be able to compare that baseline to future iterations of their model. This allows them to have empirical data on how well their model is doing today, and detect any degradation of performance based on future improvements.
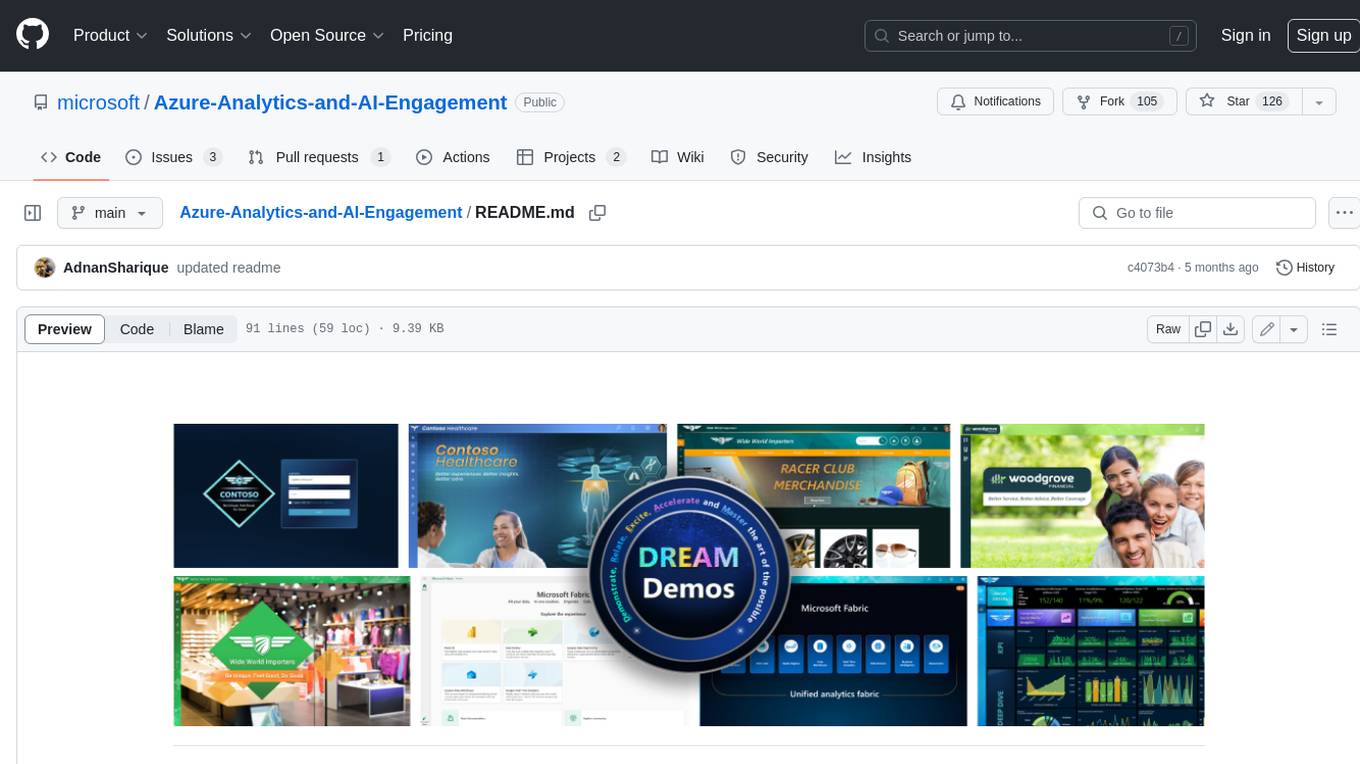
Azure-Analytics-and-AI-Engagement
The Azure-Analytics-and-AI-Engagement repository provides packaged Industry Scenario DREAM Demos with ARM templates (Containing a demo web application, Power BI reports, Synapse resources, AML Notebooks etc.) that can be deployed in a customer’s subscription using the CAPE tool within a matter of few hours. Partners can also deploy DREAM Demos in their own subscriptions using DPoC.