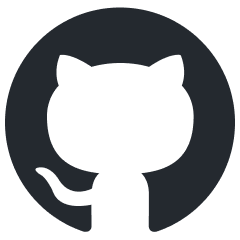
org-ai
Emacs as your personal AI assistant. Use LLMs such as ChatGPT or LLaMA for text generation or DALL-E and Stable Diffusion for image generation. Also supports speech input / output.
Stars: 648
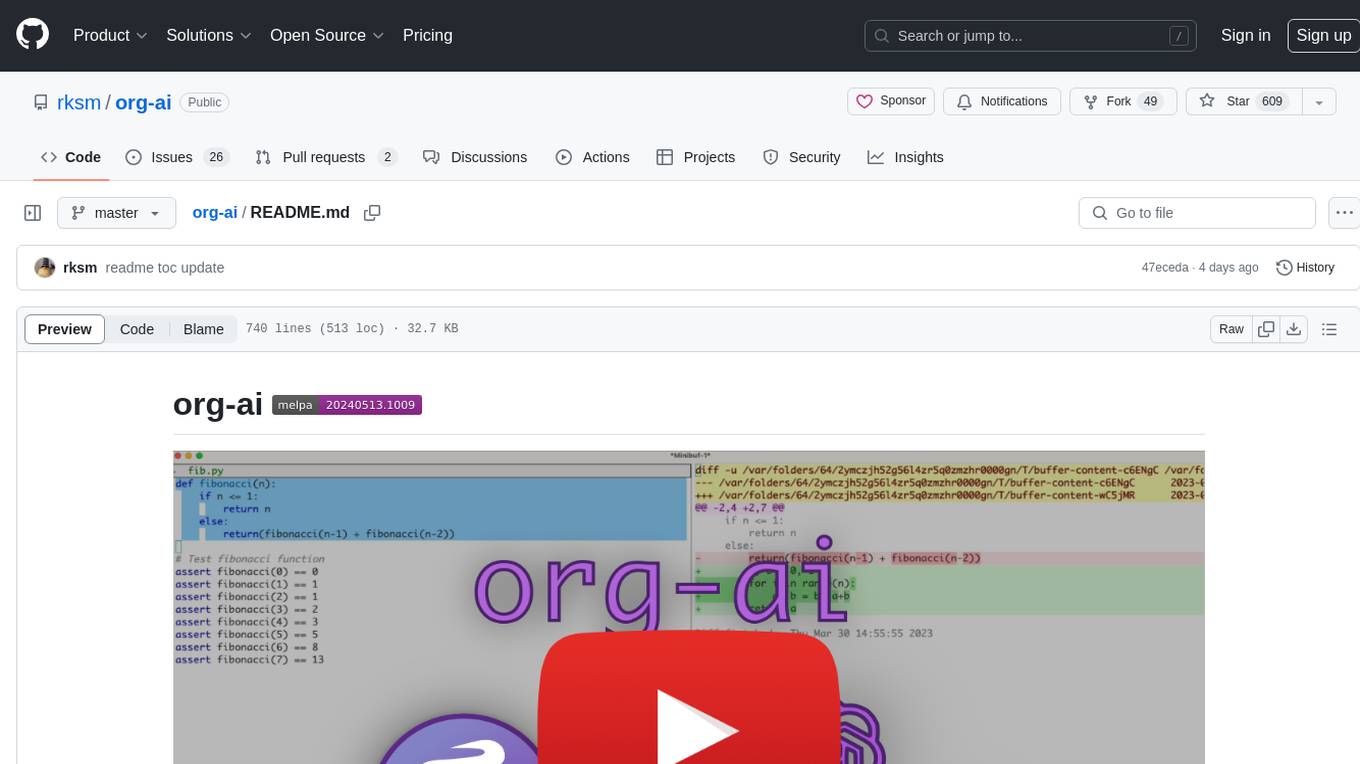
org-ai is a minor mode for Emacs org-mode that provides access to generative AI models, including OpenAI API (ChatGPT, DALL-E, other text models) and Stable Diffusion. Users can use ChatGPT to generate text, have speech input and output interactions with AI, generate images and image variations using Stable Diffusion or DALL-E, and use various commands outside org-mode for prompting using selected text or multiple files. The tool supports syntax highlighting in AI blocks, auto-fill paragraphs on insertion, and offers block options for ChatGPT, DALL-E, and other text models. Users can also generate image variations, use global commands, and benefit from Noweb support for named source blocks.
README:
Minor mode for Emacs org-mode that provides access to generative AI models. Currently supported are
- OpenAI API (ChatGPT, DALL-E, other text models), optionally run against Azure API instead of OpenAI
- Stable Diffusion through stable-diffusion-webui
Inside an org-mode buffer you can
- use ChatGPT to generate text, having full control over system and user prompts (demo)
- Speech input and output! Talk with your AI!
- generate images and image variations with a text prompt using Stable Diffusion or DALL-E (demo 1, demo 2)
- org-ai everywhere: Various commands usable outside org-mode for prompting using the selected text or multiple files.
Note: In order to use the OpenAI API you'll need an OpenAI account and you need to get an API token. As far as I can tell, the current usage limits for the free tier get you pretty far.
- Demos
- Features and Usage
- Installation
- FAQ
- Sponsoring
#+begin_ai
Is Emacs the greatest editor?
#+end_ai
You can continue to type and press C-c C-c
to create a conversation. C-g
will interrupt a running request.
Use the :image
keyword to generate an image. This uses DALL·E-3 by default.
#+begin_ai :image :size 1024x1024
Hyper realistic sci-fi rendering of super complicated technical machine.
#+end_ai
You can use the following keywords to control the image generation:
-
:size <width>x<height>
- the size of the image to generate (default: 1024x1024) -
:model <model>
- the model to use (default:"dall-e-3"
) -
:quality <quality>
- the quality of the image (choices:hd
,standard
) -
:style <style>
- the style to use (choices:vivid
,natural
) - `:n - the number of images to generate (default: 1)
(For more information about those settings see this OpenAI blog post.
You can customize the defaults for those variables with customize-variable
or by setting them in your config:
(setq org-ai-image-model "dall-e-3")
(setq org-ai-image-default-size "1792x1024")
(setq org-ai-image-default-count 2)
(setq org-ai-image-default-style 'vivid)
(setq org-ai-image-default-quality 'hd)
(setq org-ai-image-directory (expand-file-name "org-ai-images/" org-directory))
Similar to org-babel, these blocks demarcate input (and for ChatGPT also output) for the AI model. You can use it for AI chat, text completion and text -> image generation. See options below for more information.
Create a block like
#+begin_ai
Is Emacs the greatest editor?
#+end_ai
and press C-c C-c
. The Chat input will appear inline and once the response is complete, you can enter your reply and so on. See the demo below. You can press C-g
while the ai request is running to cancel it.
You can also modify the system prompt and other parameters used. The system prompt is injected before the user's input and "primes" the model to answer in a certain style. For example you can do:
#+begin_ai :max-tokens 250
[SYS]: Act as if you are a powerful medival king.
[ME]: What will you eat today?
#+end_ai
This will result in an API payload like
{
"messages": [
{
"role": "system",
"content": "Act as if you are a powerful medival king."
},
{
"role": "user",
"content": "What will you eat today?"
}
],
"model": "gpt-4o-mini",
"stream": true,
"max_tokens": 250,
"temperature": 1.2
}
For some prompt ideas see for example Awesome ChatGPT Prompts.
When generating images using the :image
flag, images will appear underneath the ai block inline. Images will be stored (together with their prompt) inside org-ai-image-directory
which defaults to ~/org/org-ai-images/
.
You can also use speech input to transcribe the input. Press C-c r
for org-ai-talk-capture-in-org
to start recording. Note that this will require you to setup speech recognition (see below). Speech output can be enabled with org-ai-talk-output-enable
.
Inside an #+begin_ai...#+end_ai
you can modify and select the parts of the chat with these commands:
- Press
C-c <backspace>
(org-ai-kill-region-at-point
) to remove the chat part under point. -
org-ai-mark-region-at-point
will mark the region at point. -
org-ai-mark-last-region
will mark the last chat part.
To apply syntax highlighted to your #+begin_ai ...
blocks just add a language major-mode name after _ai
. E.g. #+begin_ai markdown
. For markdown in particular, to then also correctly highlight code in in backticks, you can set (setq markdown-fontify-code-blocks-natively t)
. Make sure that you also have the markdown-mode package installed. Thanks @tavisrudd for this trick!
This behavior is enabled by default to so that the interaction is more similar to a chat. It can be annoying when long output is present and the buffer scrolls while you are reading. So you can disable this with:
(setq org-ai-jump-to-end-of-block nil)
Set (setq org-ai-auto-fill t)
to "fill" (automatically wrap lines according to fill-column
) the inserted text. Basically like auto-fill-mode
but for the AI.
The #+begin_ai...#+end_ai
block can take the following options.
By default, the content of ai blocks are interpreted as messages for ChatGPT. Text following [ME]:
is associated with the user, text following [AI]:
is associated as the model's response. Optionally you can start the block with a [SYS]: <behavior>
input to prime the model (see org-ai-default-chat-system-prompt
below).
-
:max-tokens number
- number of maximum tokens to generate (default: nil, use OpenAI's default) -
:temperature number
- temperature of the model (default: 1) -
:top-p number
- top_p of the model (default: 1) -
:frequency-penalty number
- frequency penalty of the model (default: 0) -
:presence-penalty
- presence penalty of the model (default: 0) -
:sys-everywhere
- repeat the system prompt for every user message (default: nil)
If you have a lot of different threads of conversation regarding the same topic and settings (system prompt, temperature, etc) and you don't want to repeat all the options, you can set org file scope properties or create a org heading with property drawer, such that all #+begin_ai...#+end_ai
blocks under that heading will inherit the settings.
Examples:
* Emacs (multiple conversations re emacs continue in this subtree)
:PROPERTIES:
:SYS: You are a emacs expert. You can help me by answering my questions. You can also ask me questions to clarify my intention.
:temperature: 0.5
:model: gpt-4o-mini
:END:
** Web programming via elisp
#+begin_ai
How to call a REST API and parse its JSON response?
#+end_ai
** Other emacs tasks
#+begin_ai...#+end_ai
* Python (multiple conversations re python continue in this subtree)
:PROPERTIES:
:SYS: You are a python programmer. Respond to the task with detailed step by step instructions and code.
:temperature: 0.1
:model: gpt-4
:END:
** Learning QUIC
#+begin_ai
How to setup a webserver with http3 support?
#+end_ai
** Other python tasks
#+begin_ai...#+end_ai
The following custom variables can be used to configure the chat:
-
org-ai-default-chat-model
(default:"gpt-4o-mini"
) -
org-ai-default-max-tokens
How long the response should be. Currently cannot exceed 4096. If this value is too small an answer might be cut off (default: nil) -
org-ai-default-chat-system-prompt
How to "prime" the model. This is a prompt that is injected before the user's input. (default:"You are a helpful assistant inside Emacs."
) -
org-ai-default-inject-sys-prompt-for-all-messages
Wether to repeat the system prompt for every user message. Sometimes the model "forgets" how it was primed. This can help remind it. (default:nil
)
When you add an :image
option to the ai block, the prompt will be used for image generation.
-
:image
- generate an image instead of text -
:size
- size of the image to generate (default: 256x256, can be 512x512 or 1024x1024) -
:n
- the number of images to generate (default: 1)
The following custom variables can be used to configure the image generation:
-
org-ai-image-directory
- where to store the generated images (default:~/org/org-ai-images/
)
Similar to DALL-E but use
#+begin_ai :sd-image
<PROMPT>
#+end_ai
You can run img2img by labeling your org-mode image with #+name and referencing it with :image-ref from your org-ai block.
#+begin_ai :sd-image :image-ref label1
forest, Gogh style
#+end_ai
M-x org-ai-sd-clip guesses the previous image's prompt on org-mode by the CLIP interrogator and saves it in the kill ring.
M-x org-ai-sd-deepdanbooru guesses the previous image's prompt on org-mode by the DeepDanbooru interrogator and saves it in the kill ring.
For requesting completions from a local model served with oobabooga/text-generation-webui, go through the setup steps described below
Then start an API server:
cd ~/.emacs.d/org-ai/text-generation-webui
conda activate org-ai
python server.py --api --model SOME-MODEL
When you add a :local
key to an org-ai block and request completions with C-c C-c
, the block will be sent to the local API server instead of the OpenAI API. For example:
#+begin_ai :local
...
#+end_ai
This will send a request to org-ai-oobabooga-websocket-url
and stream the response into the org buffer.
The older completion models can also be prompted by adding the :completion
option to the ai block.
-
:completion
- instead of using the chatgpt model, use the completion model -
:model
- which model to use, see https://platform.openai.com/docs/models for a list of models
For the detailed meaning of those parameters see the OpenAI API documentation.
The following custom variables can be used to configure the text generation:
-
org-ai-default-completion-model
(default:"text-davinci-003"
)
You can also use an existing image as input to generate more similar looking images. The org-ai-image-variation
command will prompt for a file path to an image, a size and a count and will then generate as many images and insert links to them inside the current org-mode
buffer. Images will be stored inside org-ai-image-directory
. See the demo below.
For more information see the OpenAI documentation. The input image needs to be square and its size needs to be less than 4MB. And you currently need curl available as a command line tool1.
org-ai
can be used outside of org-mode
buffers as well. When you enable org-ai-global-mode
, the prefix C-c M-a
will be bound to a number of commands:
command | keybinding | description |
---|---|---|
org-ai-on-region |
C-c M-a r |
Ask a question about the selected text or tell the AI to do something with it. The response will be opened in an org-mode buffer so that you can continue the conversation. Setting the variable org-ai-on-region-file (e.g. (setq org-ai-on-region-file (expand-file-name "org-ai-on-region.org" org-directory)) ) will associate a file with that buffer. |
org-ai-summarize |
C-c M-a s |
Summarize the selected text. |
org-ai-refactor-code |
C-c M-a c |
Tell the AI how to change the selected code, a diff buffer will appear with the changes. |
org-ai-on-project |
C-c M-a p |
Run prompts and modify / refactor multiple files at once. Will use projectile if available, falls back to the current directory if not. |
org-ai-prompt |
C-c M-a P |
Prompt the user for a text and then print the AI's response in current buffer. |
org-ai-switch-chat-model |
C-c M-a m |
Interactively change org-ai-default-chat-model
|
org-ai-open-account-usage-page |
C-c M-a $ |
Opens https://platform.openai.com/account/usage to see how much money you have burned. |
org-ai-open-request-buffer |
C-c M-a ! |
Opens the url request buffer. If something doesn't work it can be helpful to take a look. |
org-ai-talk-input-toggle |
C-c M-a t |
Generally enable speech input for the different prompt commands. |
org-ai-talk-output-toggle |
C-c M-a T |
Generally enable speech output. |
Using the org-ai-on-project buffer allows you to run commands on files in a project, alternatively also just on selected text in those files. You can e.g. select the readme of a project and ask "what is it all about?" or have code explained to you. You can also ask for code changes, which will generate a diff. If you know somehone who thinks only VS Code with Copilot enabled can do that, point them here.
Running the org-ai-on-project
command will open a separate buffer that allows you to select choose multiple files (and optionally select a sub-region inside a file) and then run a prompt on it.
If you deactivate "modify code", the effect is similar to running org-ai-on-region
just that the file contents all appear in the prompt.
With "modify code" activated, you can ask the AI to modify or refactor the code. By default ("Request diffs") deactivated, we will prompt to generate the new code for all selected files/regions and you can then see a diff per file and decide to apply it or not. With "Request diffs" active, the AI will be asked to directly create a unified diff that can then be applied.
Given a named source block
#+name: sayhi
#+begin_src shell
echo "Hello there"
#+end_src
We can try to reference it by name, but it doesn't work.
#+begin_ai
[SYS]: You are a mimic. Whenever I say something, repeat back what I say to you. Say exactly what I said, do not add anything.
[ME]: <<sayhi()>>
[AI]: <<sayhi()>>
[ME]:
#+end_ai
With :noweb yes
#+begin_ai :noweb yes
[SYS]: You are a mimic. Whenever I say something, repeat back what I say to you. Say exactly what I said, do not add anything.
[ME]: <<sayhi()>>
[AI]: Hello there.
[ME]:
#+end_ai
You can also trigger noweb expansion with an org-ai-noweb: yes
heading proprty anywhere in the parent headings (header args takes precedence).
To see what your document will expand to when sent to the api, run org-ai-expand-block
.
This is a hack but it works really well.
Create a block
#+name: identity
#+begin_src emacs-lisp :var x="fill me in"
(format "%s" x)
#+end_src
We can invoke it and let noweb parameters (which support lisp) evaluate as code
#+begin_ai :noweb yes
Tell me some 3, simple ways to improve this dockerfile
<<identity(x=(quelpa-slurp-file "~/code/ibr-api/Dockerfile"))>>
[AI]: 1. Use a more specific version of Python, such as "python:3.9.6-buster" instead of "python:3.9-buster", to ensure compatibility with future updates.
2. Add a cleanup step after installing poetry to remove any unnecessary files or dependencies, thus reducing the size of the final image.
3. Use multi-stage builds to separate the build environment from the production environment, thus reducing the size of the final image and increasing security. For example, the first stage can be used to install dependencies and build the code, while the second stage can contain only the final artifacts and be used for deployment.
[ME]:
#+end_ai
org-ai is on Melpa: https://melpa.org/#/org-ai. If you have added Melpa to your package archives with
(require 'package)
(add-to-list 'package-archives '("melpa" . "http://melpa.org/packages/") t)
(package-initialize)
you can install it with:
(use-package org-ai
:ensure t
:commands (org-ai-mode
org-ai-global-mode)
:init
(add-hook 'org-mode-hook #'org-ai-mode) ; enable org-ai in org-mode
(org-ai-global-mode) ; installs global keybindings on C-c M-a
:config
(setq org-ai-default-chat-model "gpt-4") ; if you are on the gpt-4 beta:
(org-ai-install-yasnippets)) ; if you are using yasnippet and want `ai` snippets
(straight-use-package
'(org-ai :type git :host github :repo "rksm/org-ai"
:local-repo "org-ai"
:files ("*.el" "README.md" "snippets")))
Checkout this repository.
git clone
https://github.com/rksm/org-ai
Then, if you use use-package
:
(use-package org-ai
:ensure t
:load-path (lambda () "path/to/org-ai"))
;; ...rest as above...
or just with require
:
(package-install 'websocket)
(add-to-list 'load-path "path/to/org-ai")
(require 'org)
(require 'org-ai)
(add-hook 'org-mode-hook #'org-ai-mode)
(org-ai-global-mode)
(setq org-ai-default-chat-model "gpt-4") ; if you are on the gpt-4 beta:
(org-ai-install-yasnippets) ; if you are using yasnippet and want `ai` snippets
You can either directly set your api token in your config:
(setq org-ai-openai-api-token "<ENTER YOUR API TOKEN HERE>")
Alternatively, org-ai
supports auth-source
for retrieving your API key. You can store a secret in the format
machine api.openai.com login org-ai password <your-api-key>
in your ~/authinfo.gpg
file. If this is present, org-ai will use this mechanism to retrieve the token when a request is made. If you do not want org-ai
to try to retrieve the key from auth-source
, you can set org-ai-use-auth-source
to nil
before loading org-ai
.
You can switch to Azure by customizing these variables, either interactively with M-x customize-variable
or by adding them to your config:
(setq org-ai-service 'azure-openai
org-ai-azure-openai-api-base "https://your-instance.openai.azure.com"
org-ai-azure-openai-deployment "azure-openai-deployment-name"
org-ai-azure-openai-api-version "2023-07-01-preview")
To store the API credentials, follow the authinfo instructions above but use org-ai-azure-openai-api-base
as the machine name.
For a list of available models see the perplexity.ai documentation.
Either switch the default service in your config:
(setq org-ai-service 'perplexity.ai)
(setq org-ai-default-chat-model "llama-3-sonar-large-32k-online")
or per block:
#+begin_ai :service perplexity.ai :model llama-3-sonar-large-32k-online
[ME]: Tell me fun facts about Emacs.
#+end_ai
For the authentication have an entry like machine api.perplexity.ai login org-ai password pplx-***
in your authinfo.gpg
or set org-ai-openai-api-token
.
Note: Currently the perplexity.ai does not give access to references/links via the API so Emacs will not be able to display references. They have a beta program for that running and I sure hope that this will be available generally soon.
Similar to the above. E.g.
#+begin_ai :service anthropic :model claude-3-opus-20240229
[ME]: Tell me fun facts about Emacs.
#+end_ai
Anthropic models are here.
There is currently only one API version that is set via org-ai-anthropic-api-version
. If other version come out you can find them here.
For the API token use machine api.anthropic.com login org-ai password sk-ant-***
in your authinfo.gpg
.
These setup steps are optional. If you don't want to use speech input / output, you can skip this section.
Note: My personal config for org-ai can be found in this gist. It contains a working whisper setup.
This has been tested on macOS and Linux. Someone with a Windows computer, please test this and let me know what needs to be done to make it work (Thank You!).
The speech input uses whisper.el and ffmpeg
. You need to clone the repo directly or use straight.el to install it.
- install ffmpeg (e.g.
brew install ffmpeg
on macOS) orsudo apt install ffmpeg
on Linux. - Clone whisper.el:
git clone https://github.com/natrys/whisper.el path/to/whisper.el
You should now be able to load it inside Emacs:
(use-package whisper
:load-path "path/to/whisper.el"
:bind ("M-s-r" . whisper-run))
Now also load:
(use-package greader :ensure)
(require 'whisper)
(require 'org-ai-talk)
;; macOS speech settings, optional
(setq org-ai-talk-say-words-per-minute 210)
(setq org-ai-talk-say-voice "Karen")
On macOS you will need to do two more things:
- Allow Emacs to record audio
- Tell whisper.el what microphone to use
You can use the tccutil helper:
git clone https://github.com/DocSystem/tccutil
cd tccutil
sudo python ./tccutil.py -p /Applications/Emacs.app -e --microphone
When you now run ffmpeg -f avfoundation -i :0 output.mp3
from within an Emacs shell, there should be no abort trap: 6
error.
(As an alternative to tccutil.py see the method mentioned in this issue.)
You can use the output of ffmpeg -f avfoundation -list_devices true -i ""
to list the audio input devices and then tell whisper.el about it: (setq whisper--ffmpeg-input-device ":0")
. :0
is the microphone index, see the output of the command above to use another one.
I've created an emacs helper that let's you select the microphone interactively. See this gist.
My full speech enabled config then looks like:
(use-package whisper
:load-path (lambda () (expand-file-name "lisp/other-libs/whisper.el" user-emacs-directory))
:config
(setq whisper-model "base"
whisper-language "en"
whisper-translate nil)
(when *is-a-mac*
(rk/select-default-audio-device "Macbook Pro Microphone")
(when rk/default-audio-device)
(setq whisper--ffmpeg-input-device (format ":%s" rk/default-audio-device))))
On macOS, instead of whisper, you can also use the built-in Siri dictation. To enable that, go to Preferences -> Keyboard -> Dictation
, enable it and set up a shortcut. The default is ctrl-ctrl.
The way (defun whisper--check-install-and-run) is implemented does not work on Win10 (see https://github.com/rksm/org-ai/issues/66).
A workaround is to install whisper.cpp and model manually and patch:
(defun whisper--check-install-and-run (buffer status)
(whisper--record-audio))
Speech output on non-macOS systems defaults to using the greader package which uses espeak underneath to synthesize speech. You will need to install greader manually (e.g. via M-x package-install
). From that point on it should "just work". You can test it by selecting some text and calling M-x org-ai-talk-read-region
.
An API for Stable Diffusion can be hosted with the stable-diffusion-webui project. Go through the install steps for your platform, then start an API-only server:
cd path/to/stable-diffusion-webui
./webui.sh --nowebui
This will start a server on http://127.0.0.1:7861 by default. In order to use it with org-ai, you need to set org-ai-sd-endpoint-base
:
(setq org-ai-sd-endpoint-base "http://localhost:7861/sdapi/v1/")
If you use a server hosted elsewhere, change that URL accordingly.
Since version 0.4 org-ai supports local models served with oobabooga/text-generation-webui. See the installation instructions to set it up for your system.
Here is a setup walk-through that was tested on Ubuntu 22.04. It assumes miniconda or Anaconda as well as git-lfs to be installed.
conda create -n org-ai python=3.10.9
conda activate org-ai
pip3 install torch torchvision torchaudio
mkdir -p ~/.emacs.d/org-ai/
cd ~/.emacs.d/org-ai/
git clone https://github.com/oobabooga/text-generation-webui
cd text-generation-webui
pip install -r requirements.txt
oobabooga/text-generation-webui supports a number of language models. Normally, you would install them from huggingface. For example, to install the CodeLlama-7b-Instruct
model:
cd ~/.emacs.d/org-ai/text-generation-webui/models
git clone [email protected]:codellama/CodeLlama-7b-Instruct-hf
cd ~/.emacs.d/org-ai/text-generation-webui
conda activate org-ai
python server.py --api --model CodeLlama-7b-Instruct-hf
Depending on your hardware and the model used you might need to adjust the server parameters, e.g. use --load-in-8bit
to reduce memory usage or --cpu
if you don't have a suitable GPU.
You should now be able to use the local model with org-ai by adding the :local
option to the #+begin_ai
block:
#+begin_ai :local
Hello CodeLlama!
#+end_ai
No, OpenAI is the easiest to setup (you only need an API key) but you can use local models as well. See how to use Stable Diffusion and local LLMs with oobabooga/text-generation-webui above. Anthropic Claude and perplexity.ai are also supported. Please open an issue or PR for other services you'd like to see supported. I can be slow to respond but will add support if there is enough interest.
The gptel package provides an alternative interface to the OpenAI ChatGPT API: https://github.com/karthink/gptel
If you find this project useful please consider sponsoring. Thank you!
-
Note: Currenly the image variation implementation requires a command line curl to be installed. Reason for that is that the OpenAI API expects multipart/form-data requests and the emacs built-in
url-retrieve
does not support that (At least I haven't figured out how). Switching torequest.el
might be a better alternative. If you're interested in contributing, PRs are very welcome! ↩
For Tasks:
Click tags to check more tools for each tasksFor Jobs:
Alternative AI tools for org-ai
Similar Open Source Tools
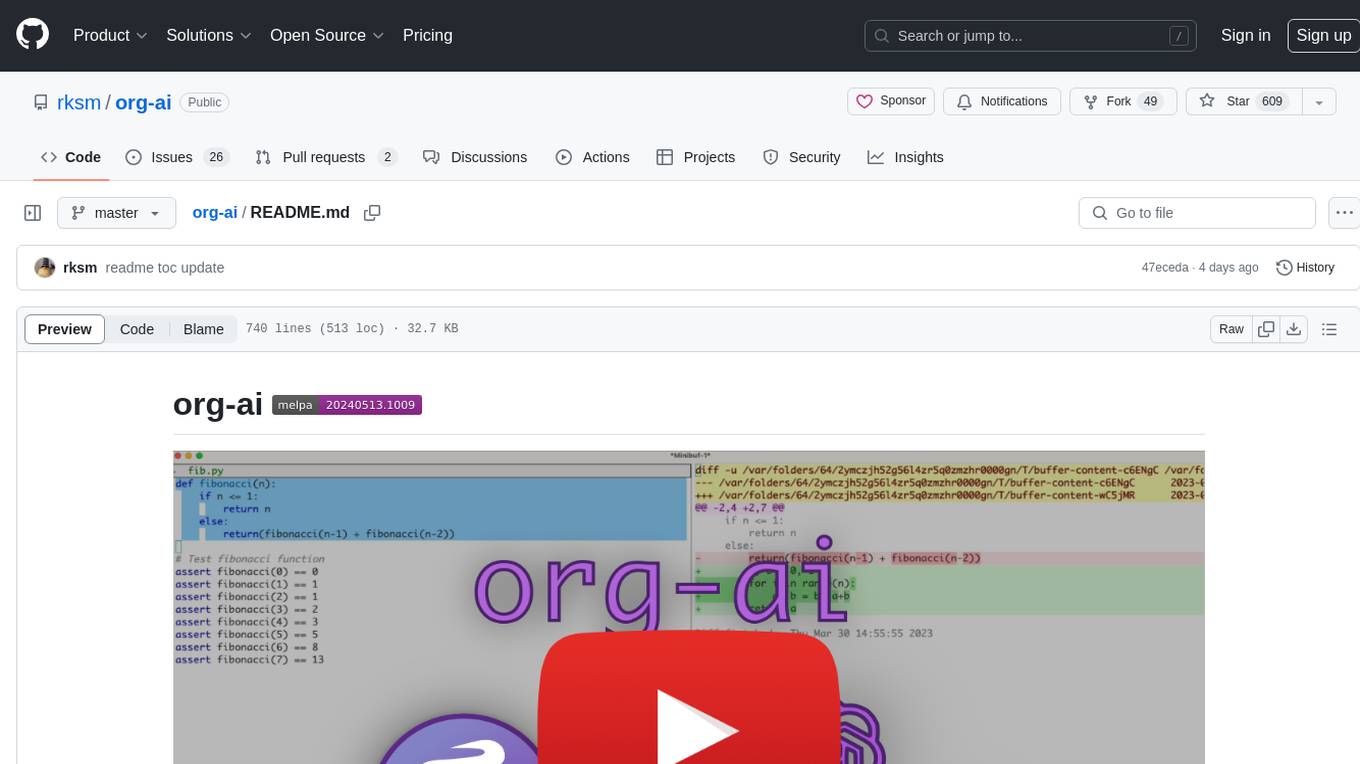
org-ai
org-ai is a minor mode for Emacs org-mode that provides access to generative AI models, including OpenAI API (ChatGPT, DALL-E, other text models) and Stable Diffusion. Users can use ChatGPT to generate text, have speech input and output interactions with AI, generate images and image variations using Stable Diffusion or DALL-E, and use various commands outside org-mode for prompting using selected text or multiple files. The tool supports syntax highlighting in AI blocks, auto-fill paragraphs on insertion, and offers block options for ChatGPT, DALL-E, and other text models. Users can also generate image variations, use global commands, and benefit from Noweb support for named source blocks.
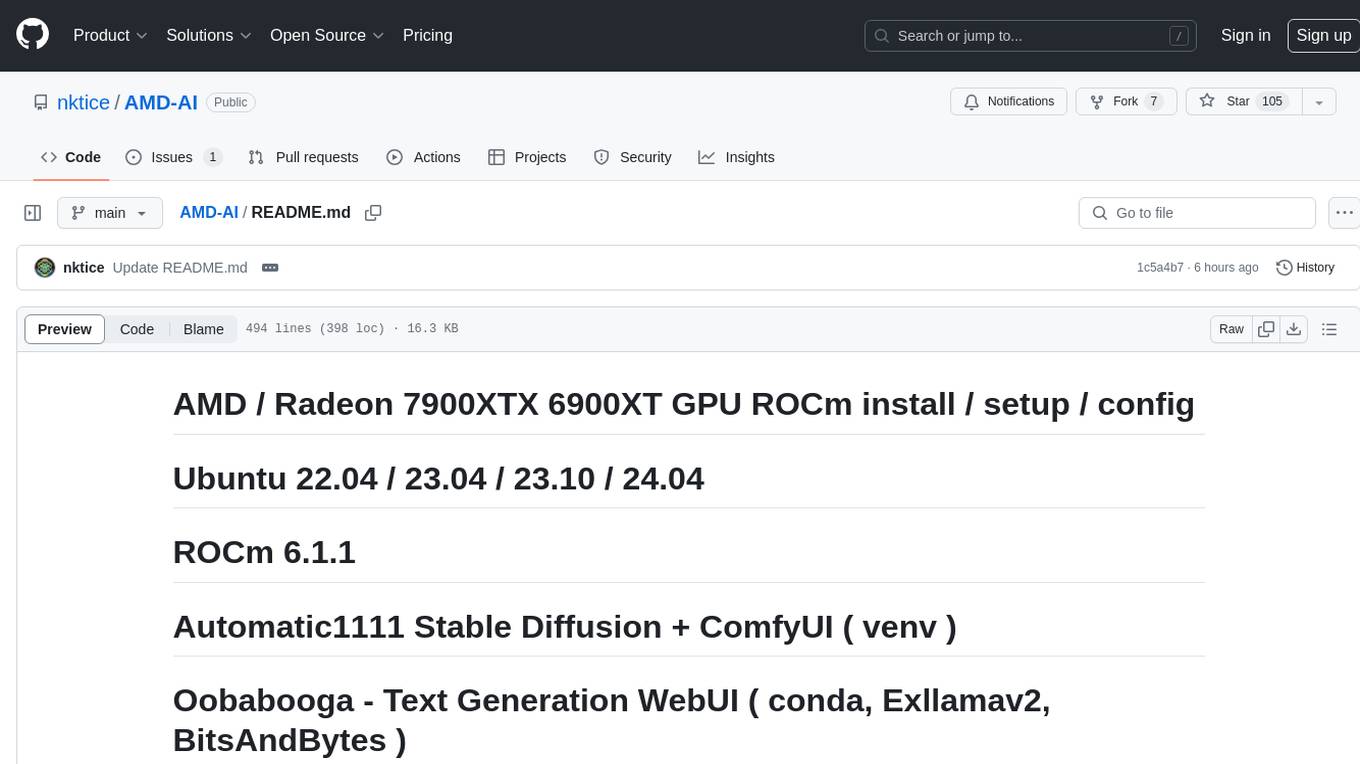
AMD-AI
AMD-AI is a repository containing detailed instructions for installing, setting up, and configuring ROCm on Ubuntu systems with AMD GPUs. The repository includes information on installing various tools like Stable Diffusion, ComfyUI, and Oobabooga for tasks like text generation and performance tuning. It provides guidance on adding AMD GPU package sources, installing ROCm-related packages, updating system packages, and finding graphics devices. The instructions are aimed at users with AMD hardware looking to set up their Linux systems for AI-related tasks.
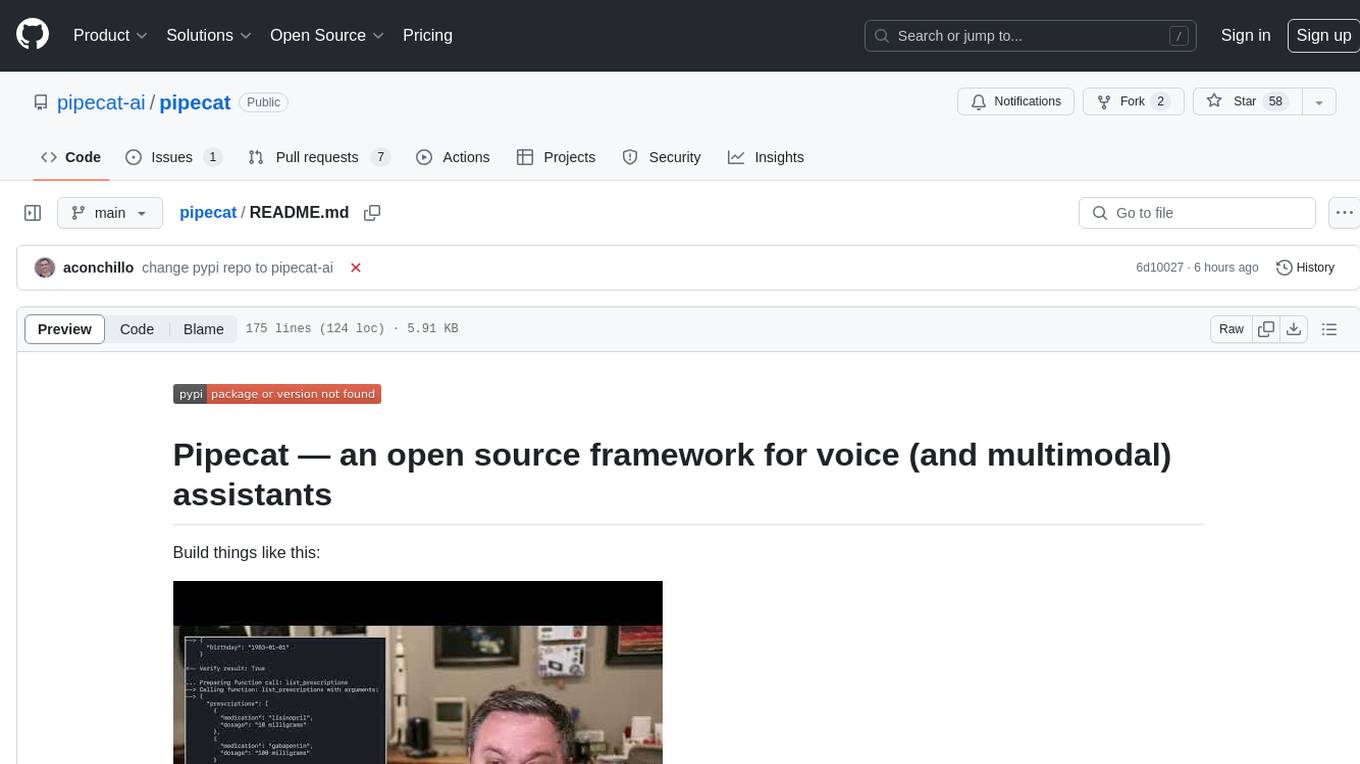
pipecat
Pipecat is an open-source framework designed for building generative AI voice bots and multimodal assistants. It provides code building blocks for interacting with AI services, creating low-latency data pipelines, and transporting audio, video, and events over the Internet. Pipecat supports various AI services like speech-to-text, text-to-speech, image generation, and vision models. Users can implement new services and contribute to the framework. Pipecat aims to simplify the development of applications like personal coaches, meeting assistants, customer support bots, and more by providing a complete framework for integrating AI services.
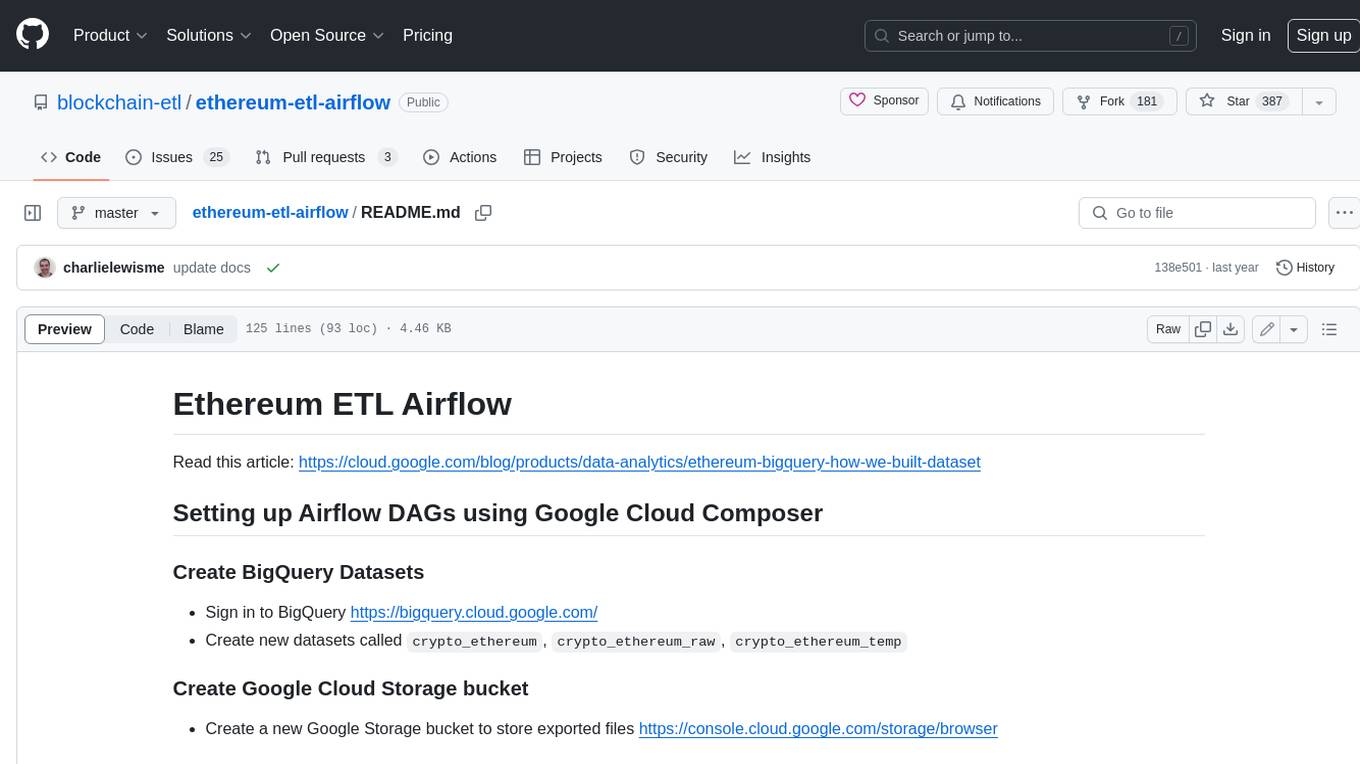
ethereum-etl-airflow
This repository contains Airflow DAGs for extracting, transforming, and loading (ETL) data from the Ethereum blockchain into BigQuery. The DAGs use the Google Cloud Platform (GCP) services, including BigQuery, Cloud Storage, and Cloud Composer, to automate the ETL process. The repository also includes scripts for setting up the GCP environment and running the DAGs locally.
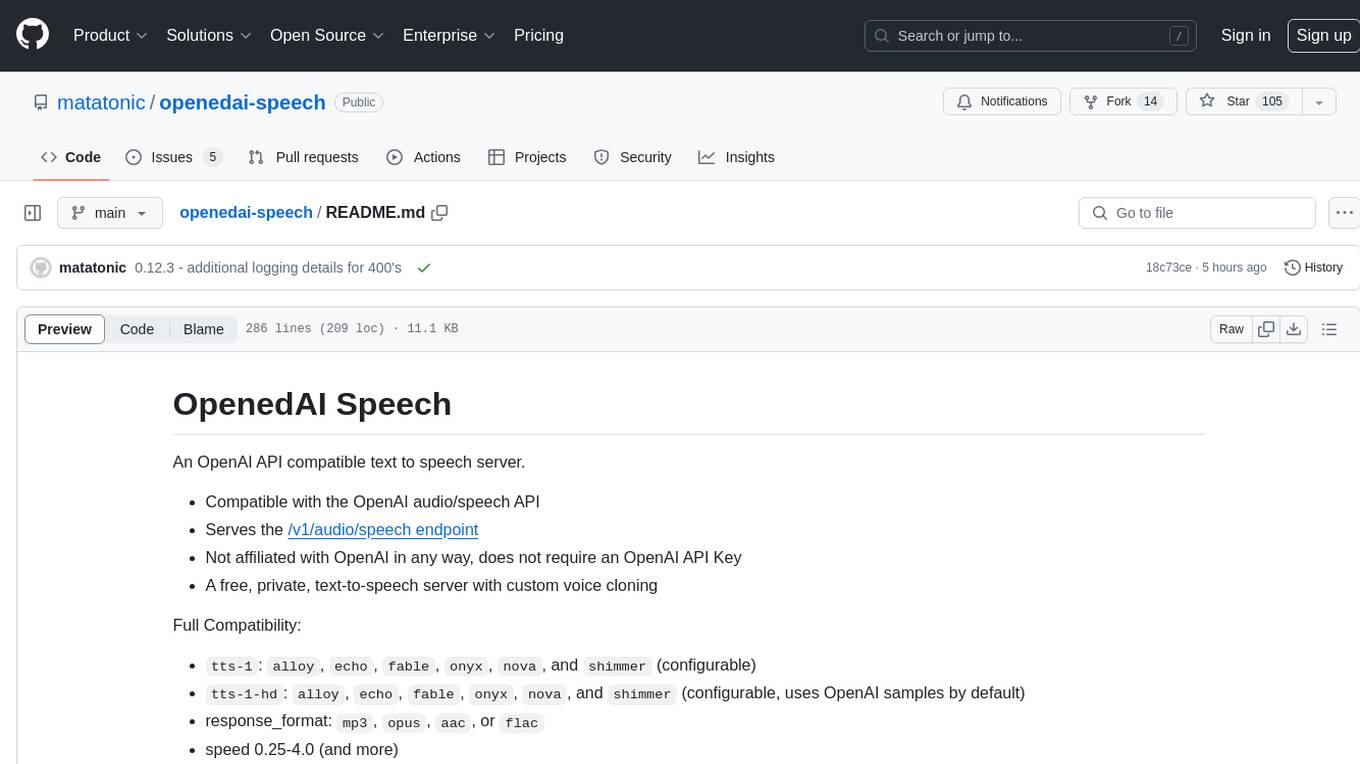
openedai-speech
OpenedAI Speech is a free, private text-to-speech server compatible with the OpenAI audio/speech API. It offers custom voice cloning and supports various models like tts-1 and tts-1-hd. Users can map their own piper voices and create custom cloned voices. The server provides multilingual support with XTTS voices and allows fixing incorrect sounds with regex. Recent changes include bug fixes, improved error handling, and updates for multilingual support. Installation can be done via Docker or manual setup, with usage instructions provided. Custom voices can be created using Piper or Coqui XTTS v2, with guidelines for preparing audio files. The tool is suitable for tasks like generating speech from text, creating custom voices, and multilingual text-to-speech applications.
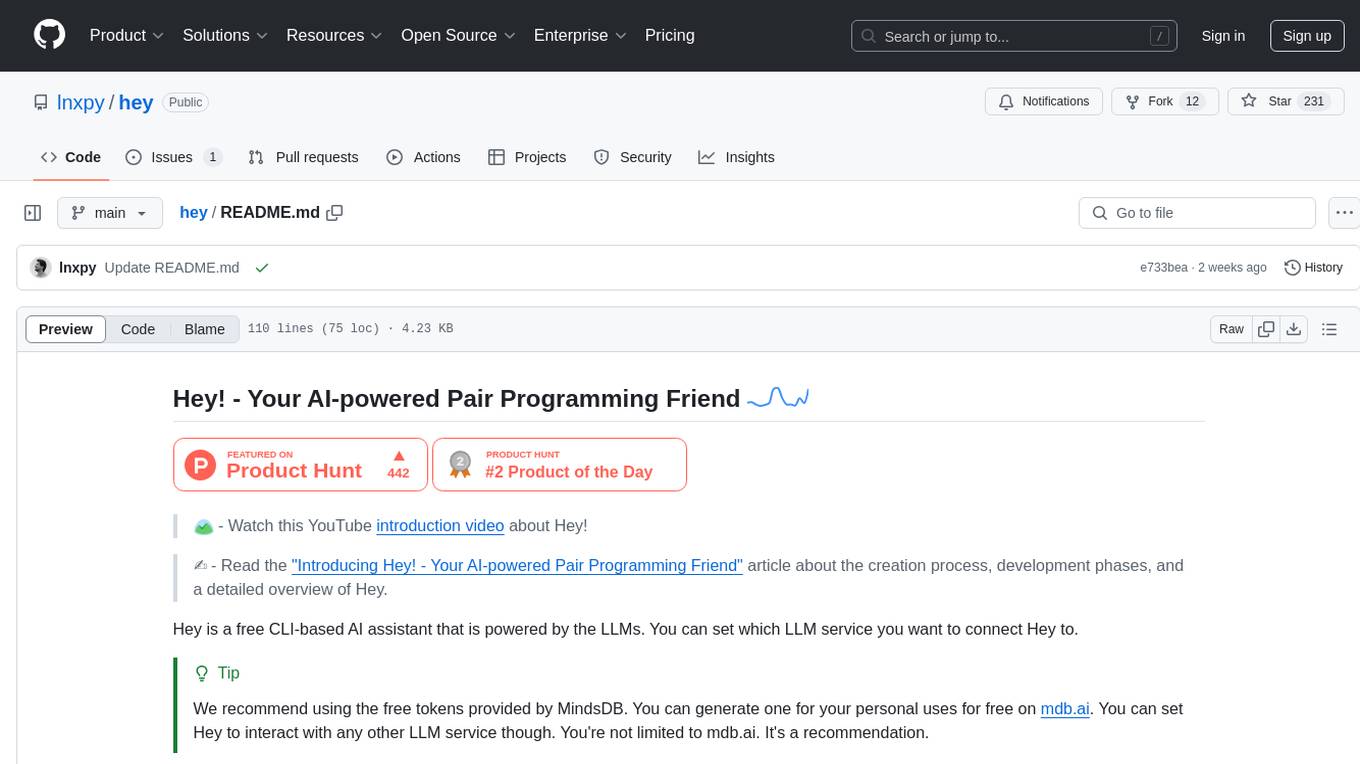
hey
Hey is a free CLI-based AI assistant powered by LLMs, allowing users to connect Hey to different LLM services. It provides commands for quick usage, customization options, and integration with code editors. Hey was created for a hackathon and is licensed under the MIT License.
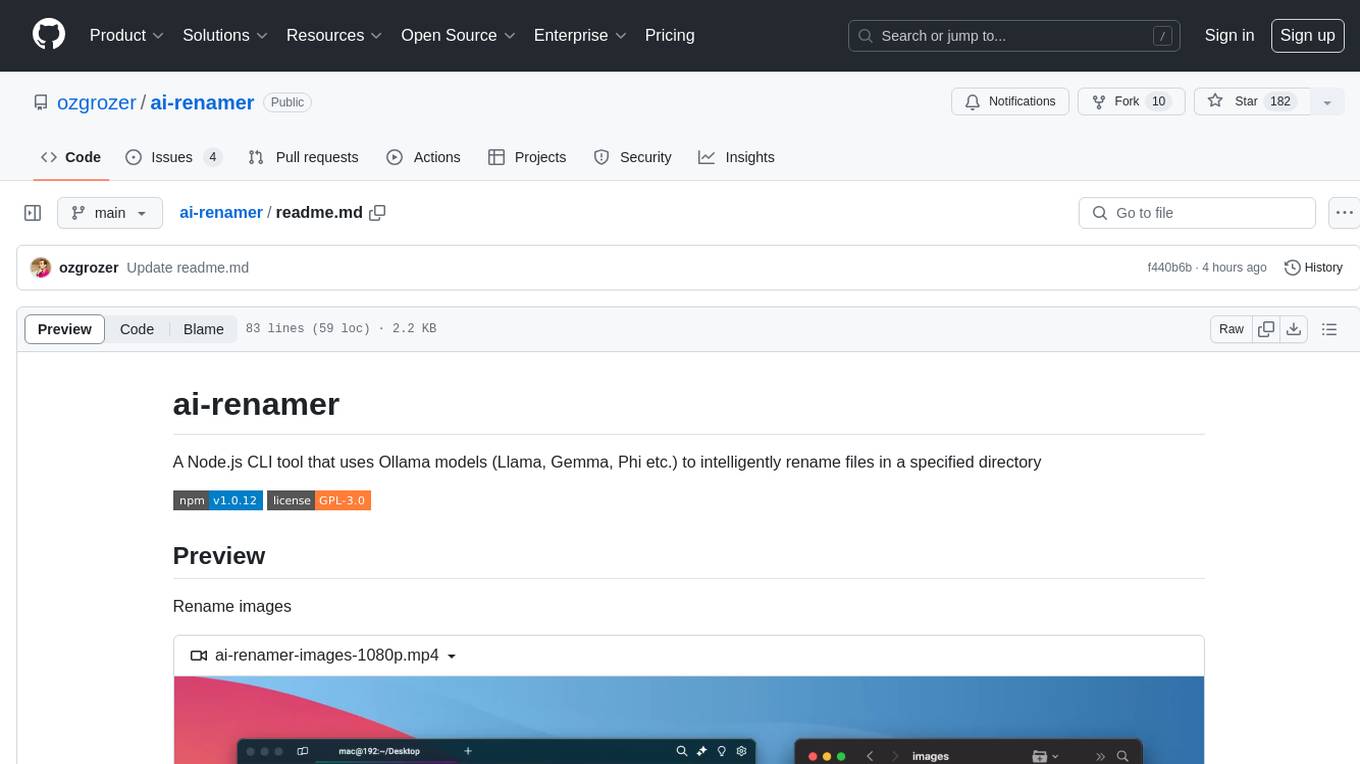
ai-renamer
ai-renamer is a Node.js CLI tool that intelligently renames files in a specified directory using Ollama models like Llama, Gemma, Phi, etc. It allows users to set case style, model, maximum characters in the filename, and output language. The tool utilizes the change-case library for case styling and requires Ollama and at least one LLM to be installed on the system. Users can contribute by opening new issues or making pull requests. Licensed under GPL-3.0.
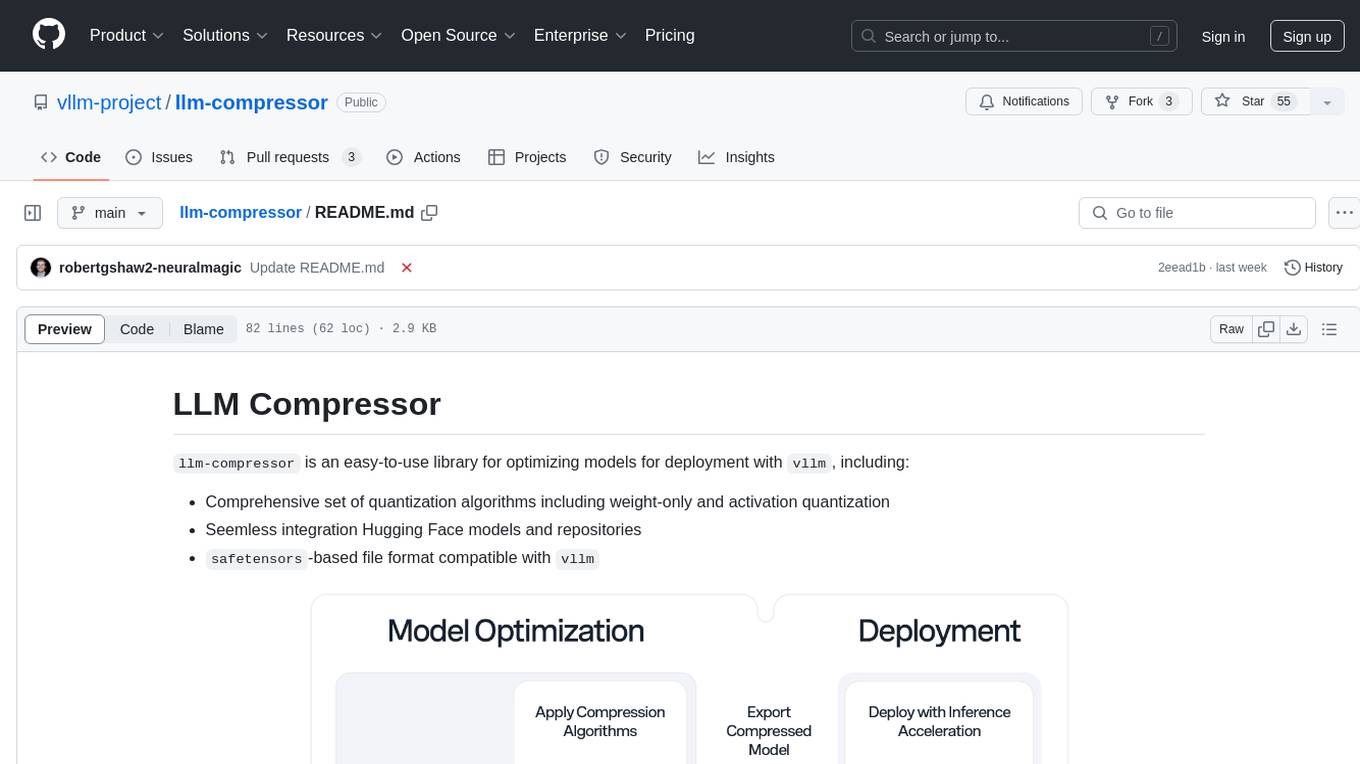
llm-compressor
llm-compressor is an easy-to-use library for optimizing models for deployment with vllm. It provides a comprehensive set of quantization algorithms, seamless integration with Hugging Face models and repositories, and supports mixed precision, activation quantization, and sparsity. Supported algorithms include PTQ, GPTQ, SmoothQuant, and SparseGPT. Installation can be done via git clone and local pip install. Compression can be easily applied by selecting an algorithm and calling the oneshot API. The library also offers end-to-end examples for model compression. Contributions to the code, examples, integrations, and documentation are appreciated.
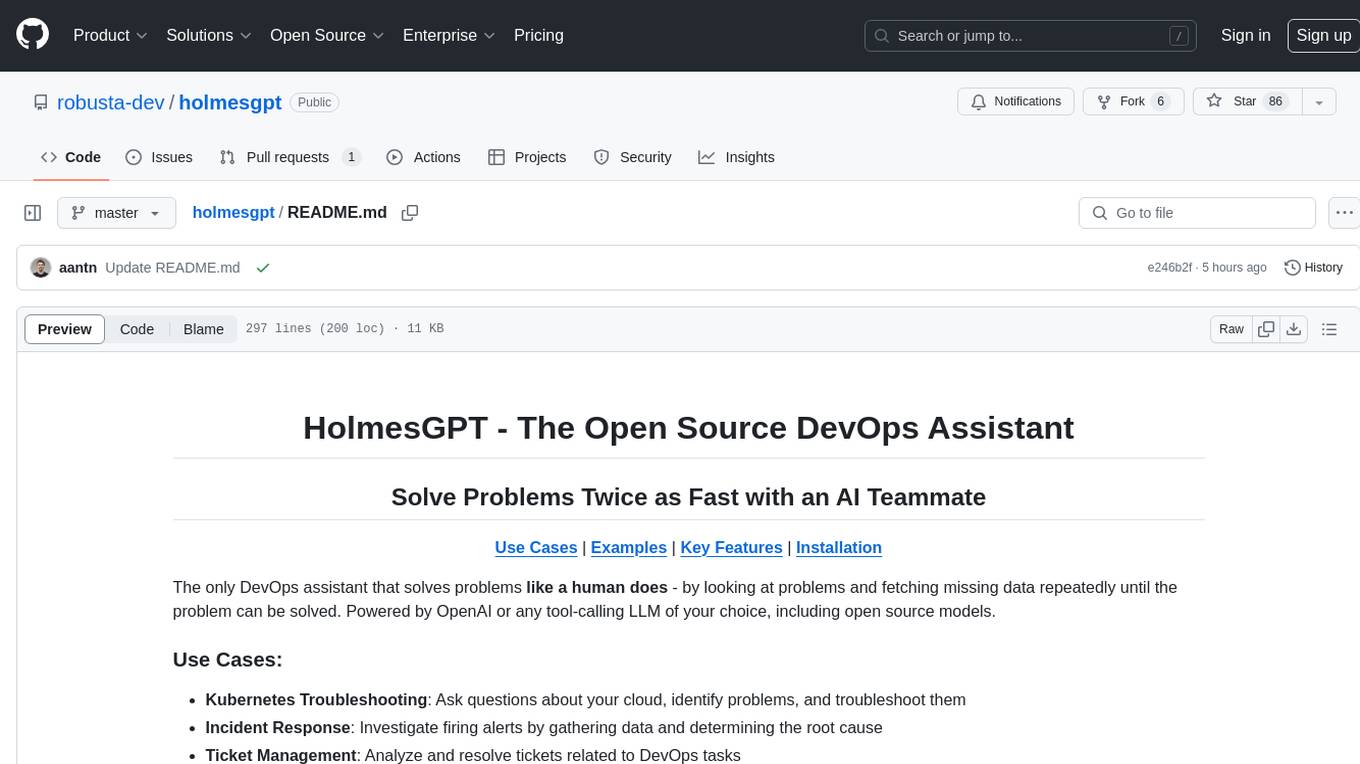
holmesgpt
HolmesGPT is an open-source DevOps assistant powered by OpenAI or any tool-calling LLM of your choice. It helps in troubleshooting Kubernetes, incident response, ticket management, automated investigation, and runbook automation in plain English. The tool connects to existing observability data, is compliance-friendly, provides transparent results, supports extensible data sources, runbook automation, and integrates with existing workflows. Users can install HolmesGPT using Brew, prebuilt Docker container, Python Poetry, or Docker. The tool requires an API key for functioning and supports OpenAI, Azure AI, and self-hosted LLMs.
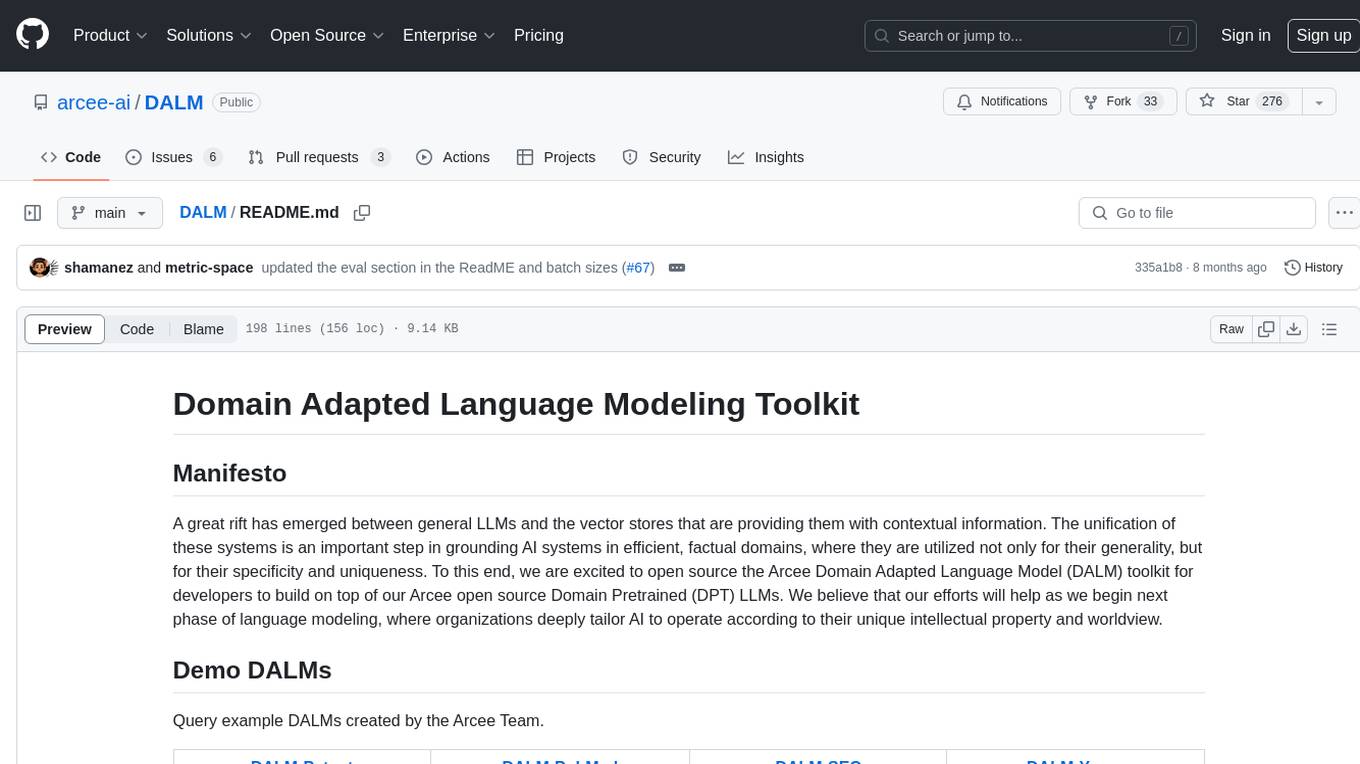
DALM
The DALM (Domain Adapted Language Modeling) toolkit is designed to unify general LLMs with vector stores to ground AI systems in efficient, factual domains. It provides developers with tools to build on top of Arcee's open source Domain Pretrained LLMs, enabling organizations to deeply tailor AI according to their unique intellectual property and worldview. The toolkit contains code for fine-tuning a fully differential Retrieval Augmented Generation (RAG-end2end) architecture, incorporating in-batch negative concept alongside RAG's marginalization for efficiency. It includes training scripts for both retriever and generator models, evaluation scripts, data processing codes, and synthetic data generation code.
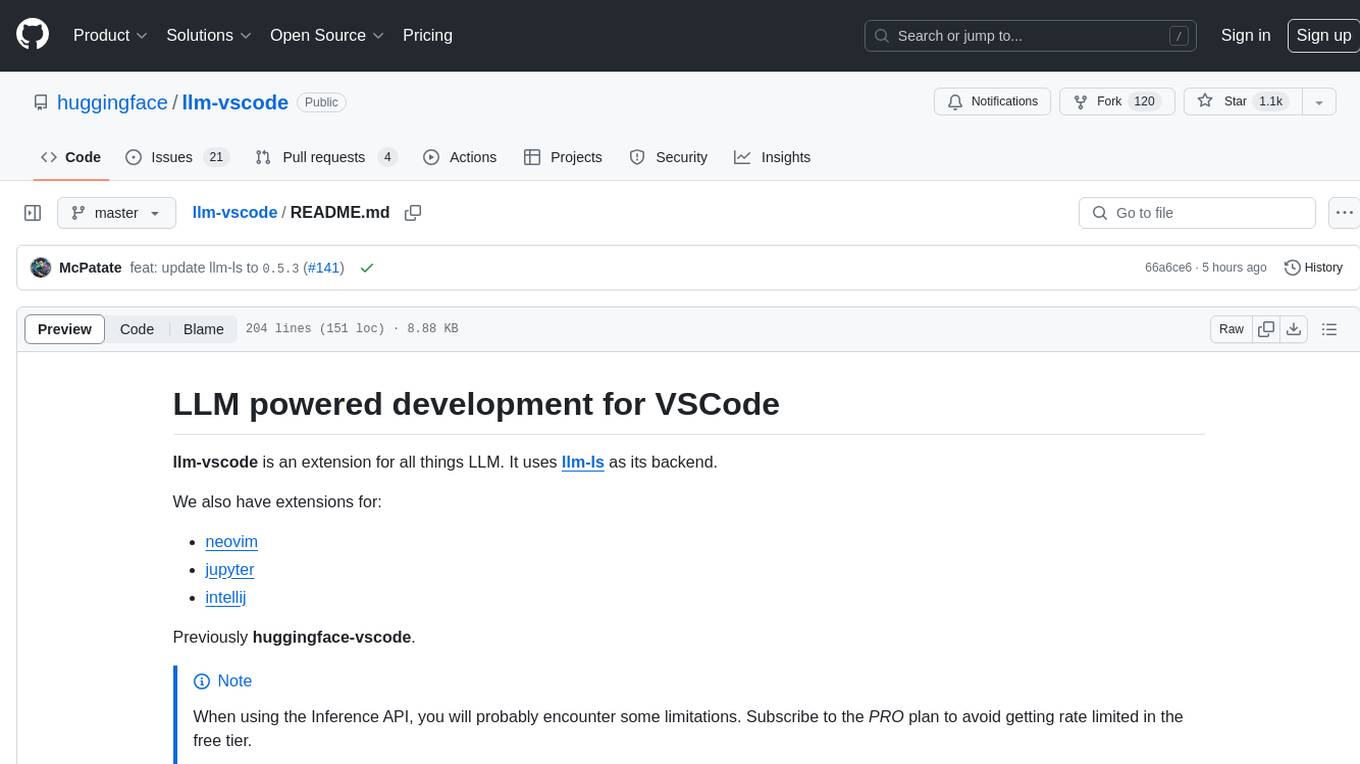
llm-vscode
llm-vscode is an extension designed for all things LLM, utilizing llm-ls as its backend. It offers features such as code completion with 'ghost-text' suggestions, the ability to choose models for code generation via HTTP requests, ensuring prompt size fits within the context window, and code attribution checks. Users can configure the backend, suggestion behavior, keybindings, llm-ls settings, and tokenization options. Additionally, the extension supports testing models like Code Llama 13B, Phind/Phind-CodeLlama-34B-v2, and WizardLM/WizardCoder-Python-34B-V1.0. Development involves cloning llm-ls, building it, and setting up the llm-vscode extension for use.
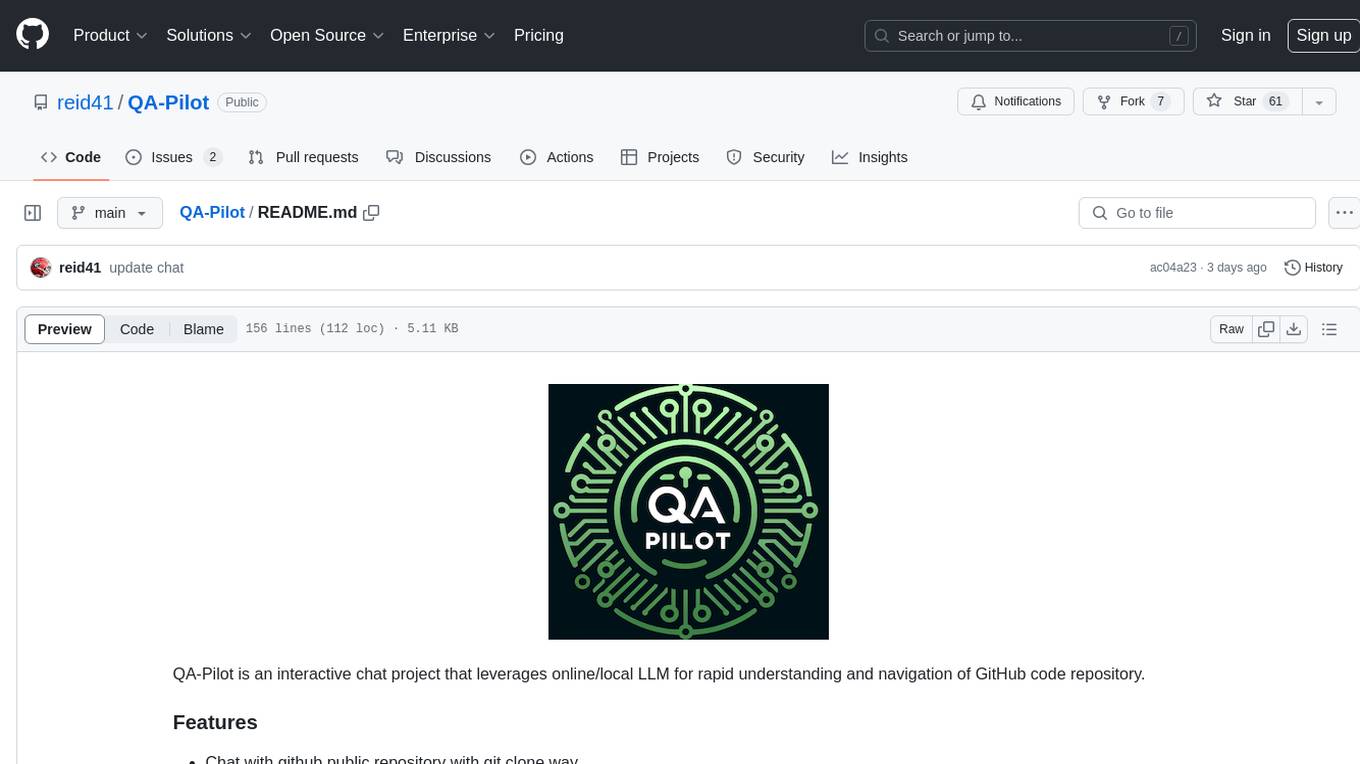
QA-Pilot
QA-Pilot is an interactive chat project that leverages online/local LLM for rapid understanding and navigation of GitHub code repository. It allows users to chat with GitHub public repositories using a git clone approach, store chat history, configure settings easily, manage multiple chat sessions, and quickly locate sessions with a search function. The tool integrates with `codegraph` to view Python files and supports various LLM models such as ollama, openai, mistralai, and localai. The project is continuously updated with new features and improvements, such as converting from `flask` to `fastapi`, adding `localai` API support, and upgrading dependencies like `langchain` and `Streamlit` to enhance performance.
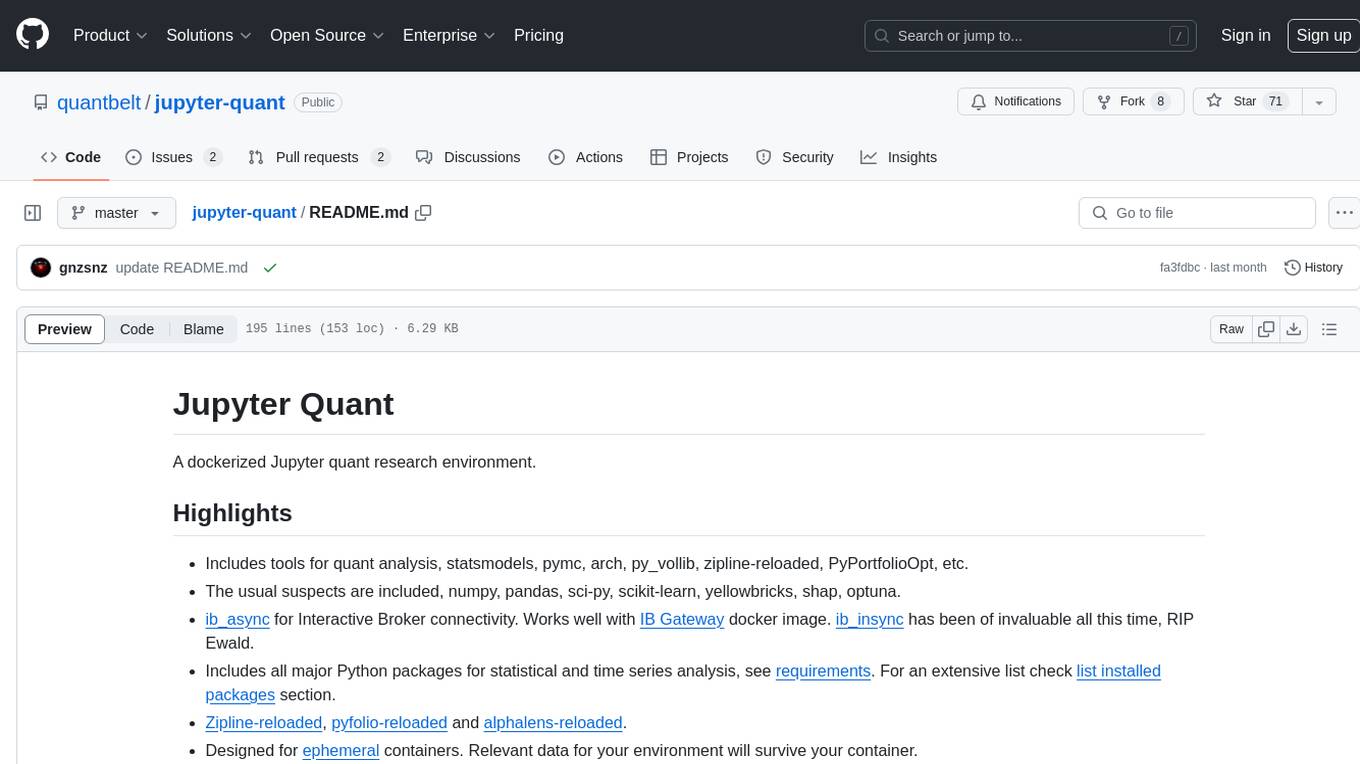
jupyter-quant
Jupyter Quant is a dockerized environment tailored for quantitative research, equipped with essential tools like statsmodels, pymc, arch, py_vollib, zipline-reloaded, PyPortfolioOpt, numpy, pandas, sci-py, scikit-learn, yellowbricks, shap, optuna, and more. It provides Interactive Broker connectivity via ib_async and includes major Python packages for statistical and time series analysis. The image is optimized for size, includes jedi language server, jupyterlab-lsp, and common command line utilities. Users can install new packages with sudo, leverage apt cache, and bring their own dot files and SSH keys. The tool is designed for ephemeral containers, ensuring data persistence and flexibility for quantitative analysis tasks.
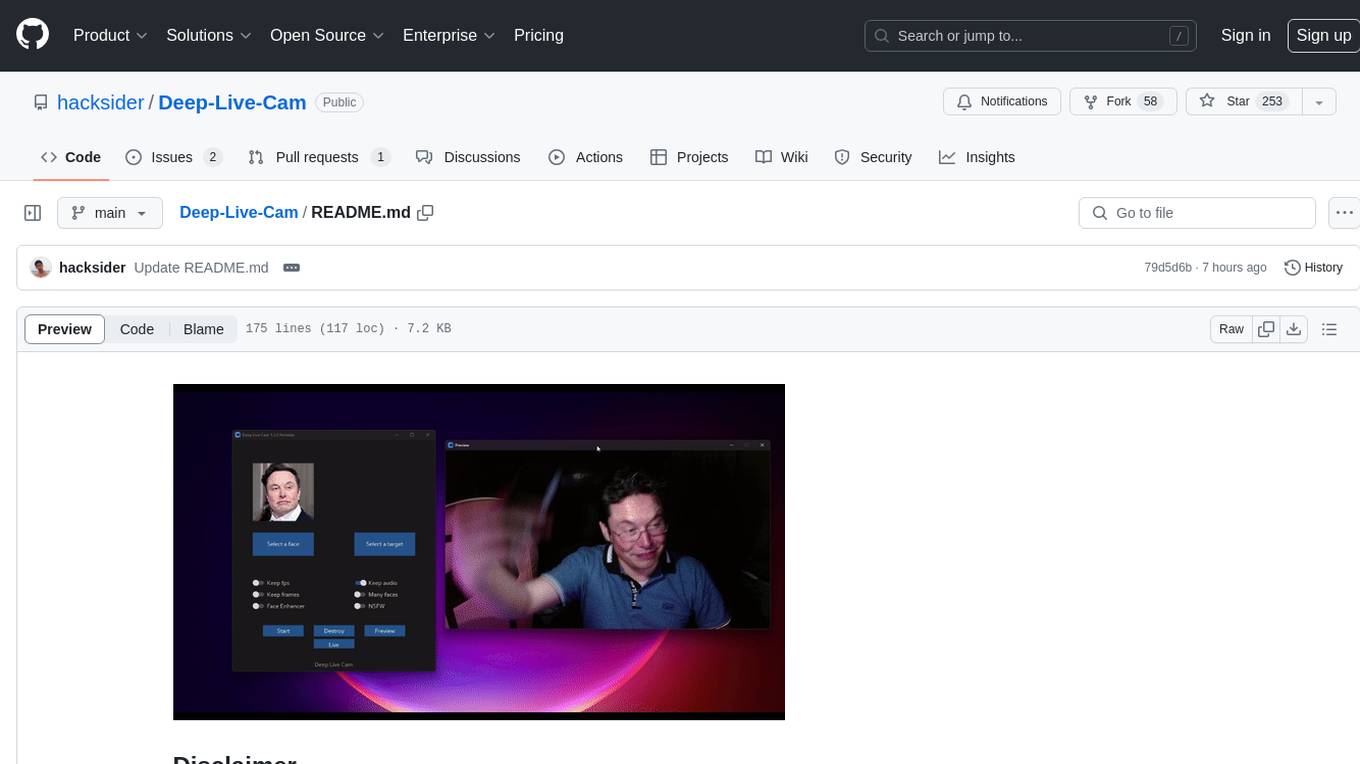
Deep-Live-Cam
Deep-Live-Cam is a software tool designed to assist artists in tasks such as animating custom characters or using characters as models for clothing. The tool includes built-in checks to prevent unethical applications, such as working on inappropriate media. Users are expected to use the tool responsibly and adhere to local laws, especially when using real faces for deepfake content. The tool supports both CPU and GPU acceleration for faster processing and provides a user-friendly GUI for swapping faces in images or videos.
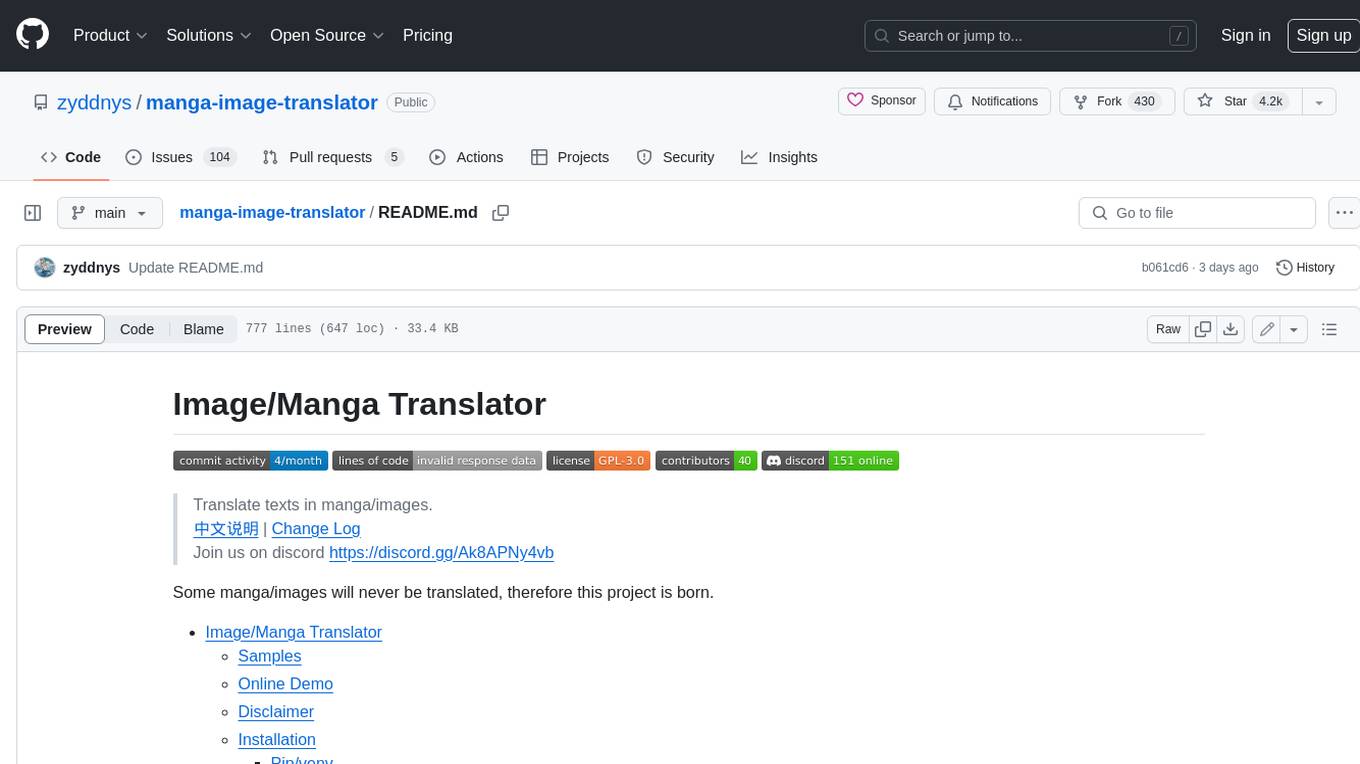
manga-image-translator
Translate texts in manga/images. Some manga/images will never be translated, therefore this project is born. * Image/Manga Translator * Samples * Online Demo * Disclaimer * Installation * Pip/venv * Poetry * Additional instructions for **Windows** * Docker * Hosting the web server * Using as CLI * Setting Translation Secrets * Using with Nvidia GPU * Building locally * Usage * Batch mode (default) * Demo mode * Web Mode * Api Mode * Related Projects * Docs * Recommended Modules * Tips to improve translation quality * Options * Language Code Reference * Translators Reference * GPT Config Reference * Using Gimp for rendering * Api Documentation * Synchronous mode * Asynchronous mode * Manual translation * Next steps * Support Us * Thanks To All Our Contributors :
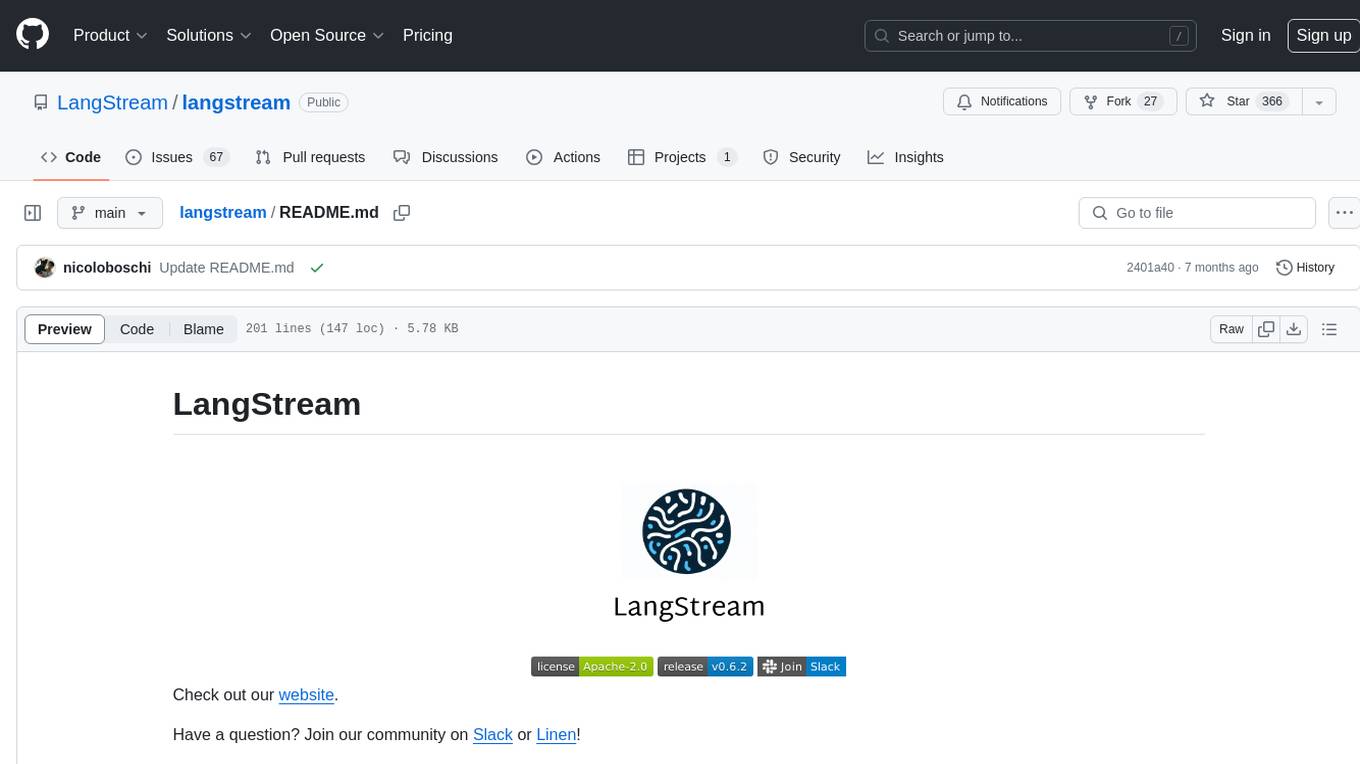
langstream
LangStream is a tool for natural language processing tasks, providing a CLI for easy installation and usage. Users can try sample applications like Chat Completions and create their own applications using the developer documentation. It supports running on Kubernetes for production-ready deployment, with support for various Kubernetes distributions and external components like Apache Kafka or Apache Pulsar cluster. Users can deploy LangStream locally using minikube and manage the cluster with mini-langstream. Development requirements include Docker, Java 17, Git, Python 3.11+, and PIP, with the option to test local code changes using mini-langstream.
For similar tasks
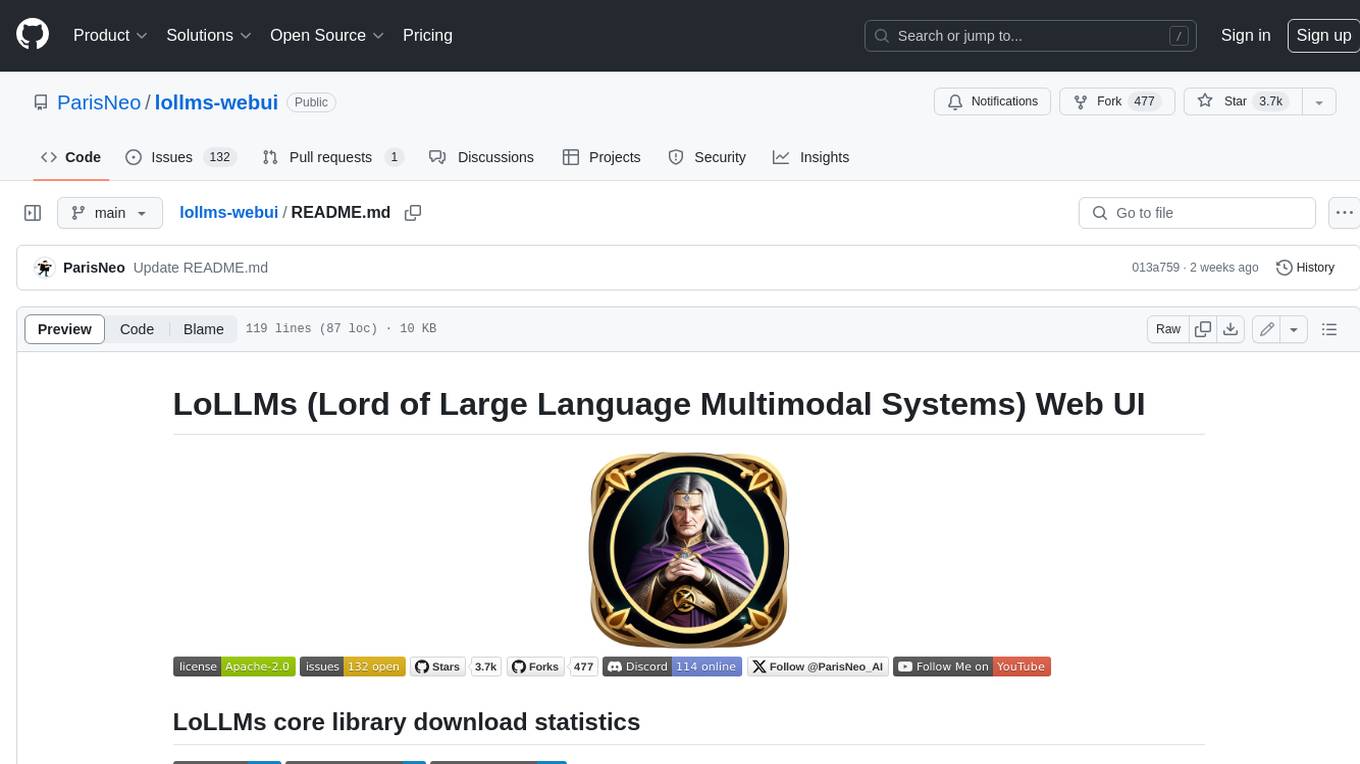
lollms-webui
LoLLMs WebUI (Lord of Large Language Multimodal Systems: One tool to rule them all) is a user-friendly interface to access and utilize various LLM (Large Language Models) and other AI models for a wide range of tasks. With over 500 AI expert conditionings across diverse domains and more than 2500 fine tuned models over multiple domains, LoLLMs WebUI provides an immediate resource for any problem, from car repair to coding assistance, legal matters, medical diagnosis, entertainment, and more. The easy-to-use UI with light and dark mode options, integration with GitHub repository, support for different personalities, and features like thumb up/down rating, copy, edit, and remove messages, local database storage, search, export, and delete multiple discussions, make LoLLMs WebUI a powerful and versatile tool.
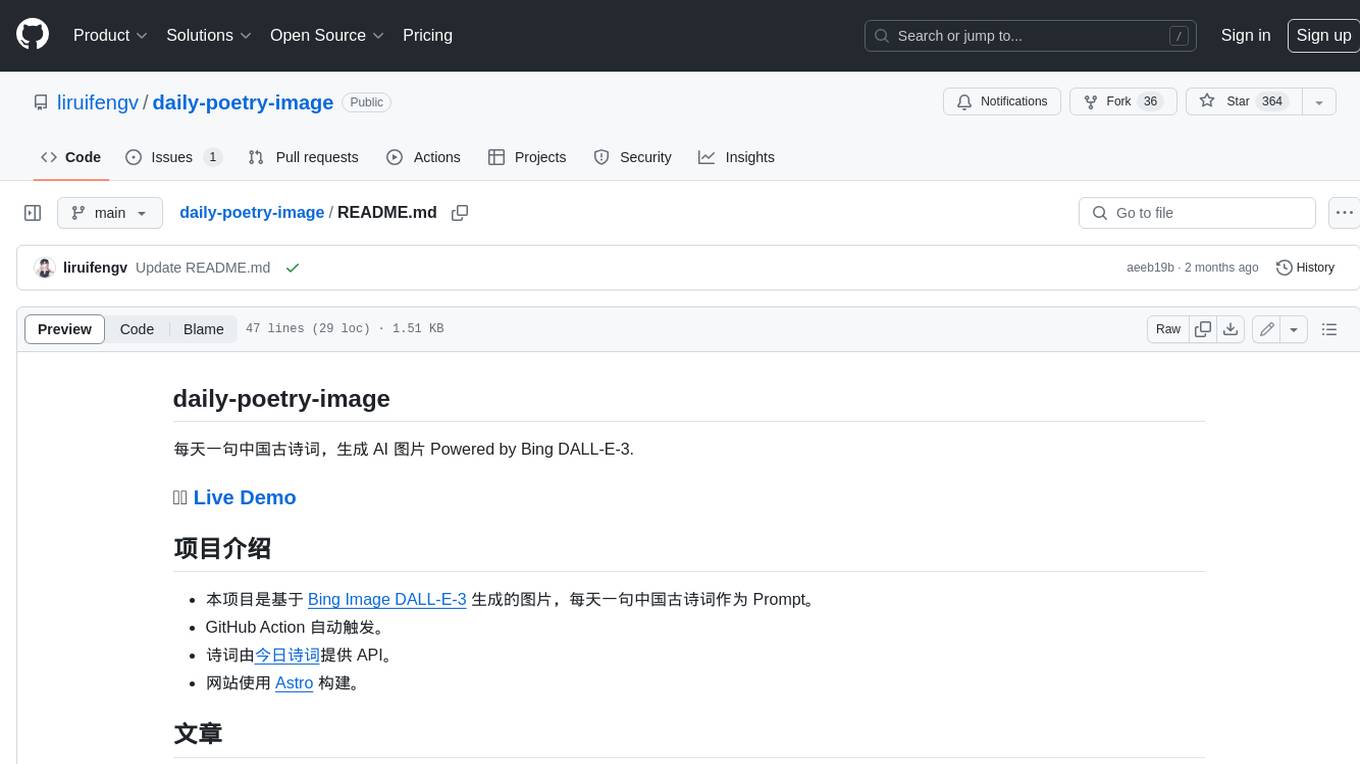
daily-poetry-image
Daily Chinese ancient poetry and AI-generated images powered by Bing DALL-E-3. GitHub Action triggers the process automatically. Poetry is provided by Today's Poem API. The website is built with Astro.
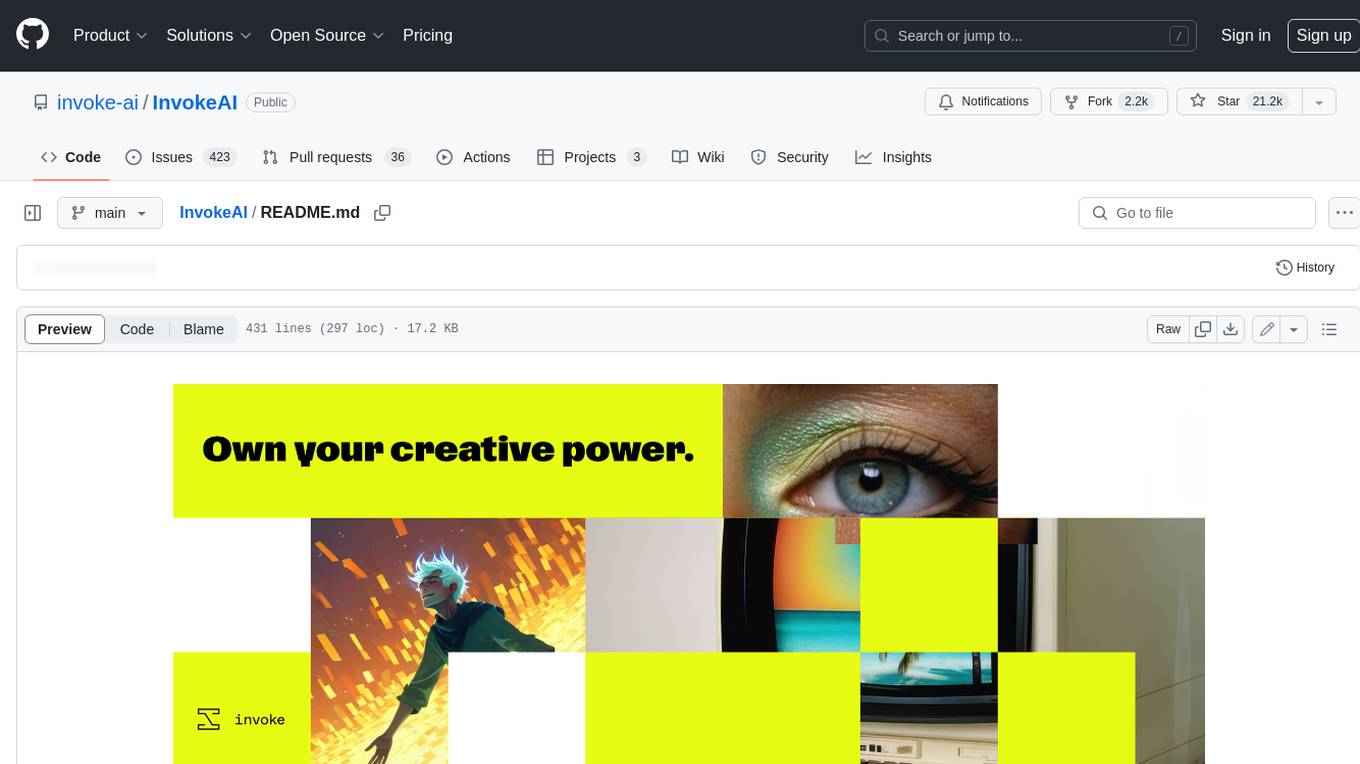
InvokeAI
InvokeAI is a leading creative engine built to empower professionals and enthusiasts alike. Generate and create stunning visual media using the latest AI-driven technologies. InvokeAI offers an industry leading Web Interface, interactive Command Line Interface, and also serves as the foundation for multiple commercial products.
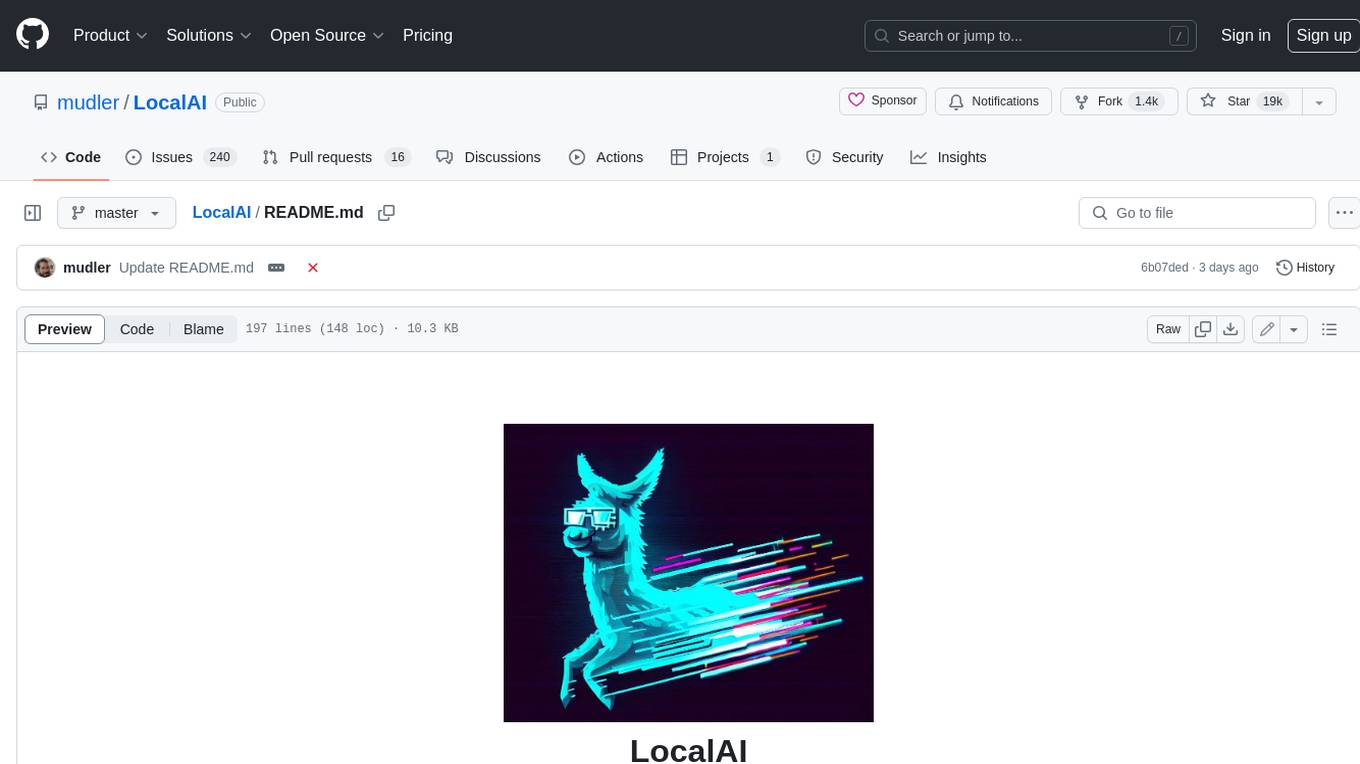
LocalAI
LocalAI is a free and open-source OpenAI alternative that acts as a drop-in replacement REST API compatible with OpenAI (Elevenlabs, Anthropic, etc.) API specifications for local AI inferencing. It allows users to run LLMs, generate images, audio, and more locally or on-premises with consumer-grade hardware, supporting multiple model families and not requiring a GPU. LocalAI offers features such as text generation with GPTs, text-to-audio, audio-to-text transcription, image generation with stable diffusion, OpenAI functions, embeddings generation for vector databases, constrained grammars, downloading models directly from Huggingface, and a Vision API. It provides a detailed step-by-step introduction in its Getting Started guide and supports community integrations such as custom containers, WebUIs, model galleries, and various bots for Discord, Slack, and Telegram. LocalAI also offers resources like an LLM fine-tuning guide, instructions for local building and Kubernetes installation, projects integrating LocalAI, and a how-tos section curated by the community. It encourages users to cite the repository when utilizing it in downstream projects and acknowledges the contributions of various software from the community.
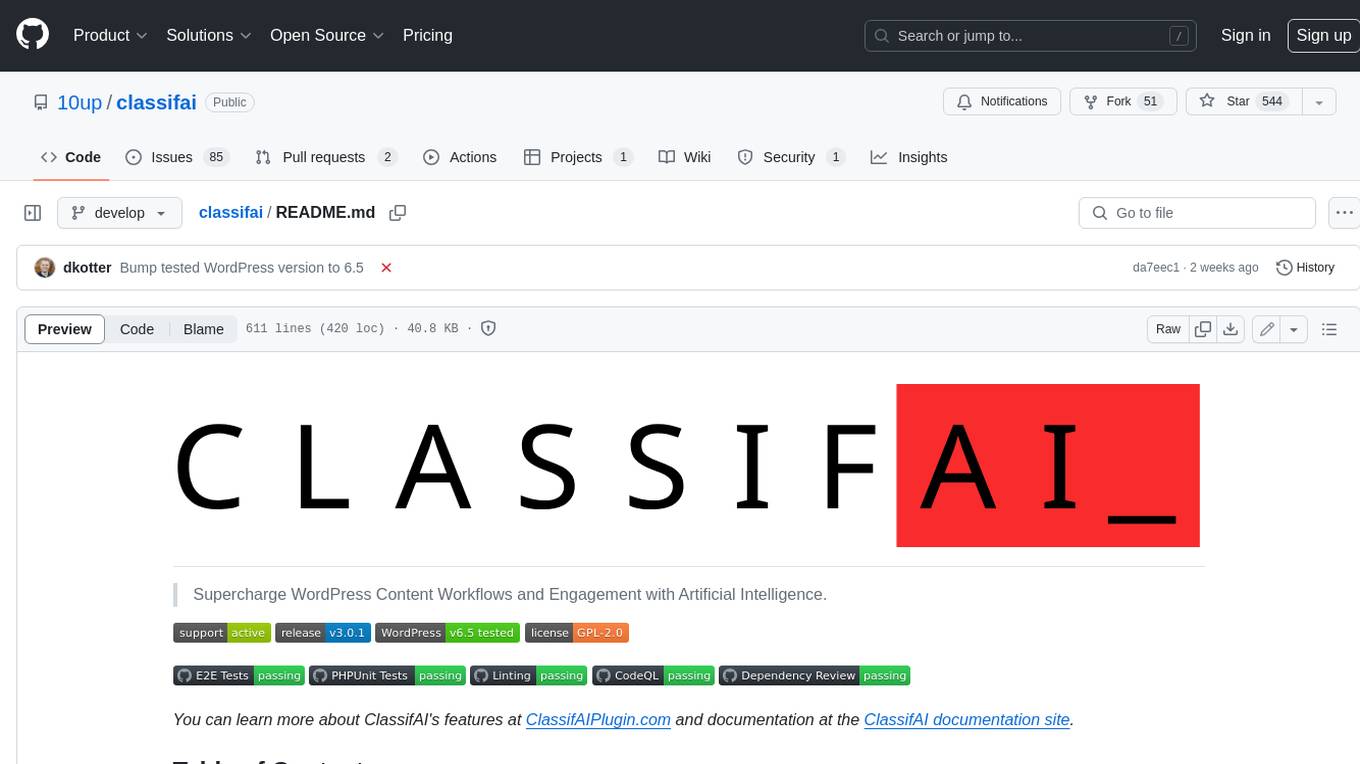
classifai
Supercharge WordPress Content Workflows and Engagement with Artificial Intelligence. Tap into leading cloud-based services like OpenAI, Microsoft Azure AI, Google Gemini and IBM Watson to augment your WordPress-powered websites. Publish content faster while improving SEO performance and increasing audience engagement. ClassifAI integrates Artificial Intelligence and Machine Learning technologies to lighten your workload and eliminate tedious tasks, giving you more time to create original content that matters.
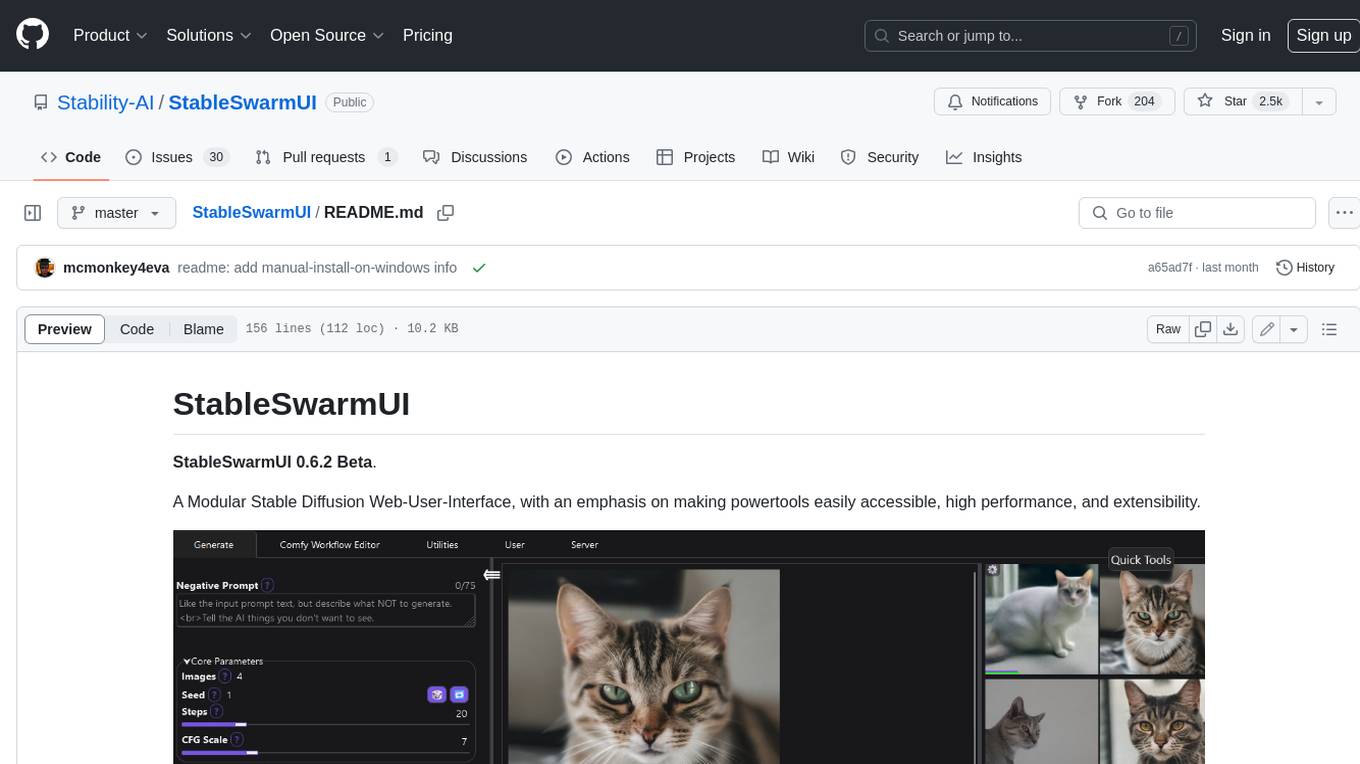
StableSwarmUI
StableSwarmUI is a modular Stable Diffusion web user interface that emphasizes making power tools easily accessible, high performance, and extensible. It is designed to be a one-stop-shop for all things Stable Diffusion, providing a wide range of features and capabilities to enhance the user experience.
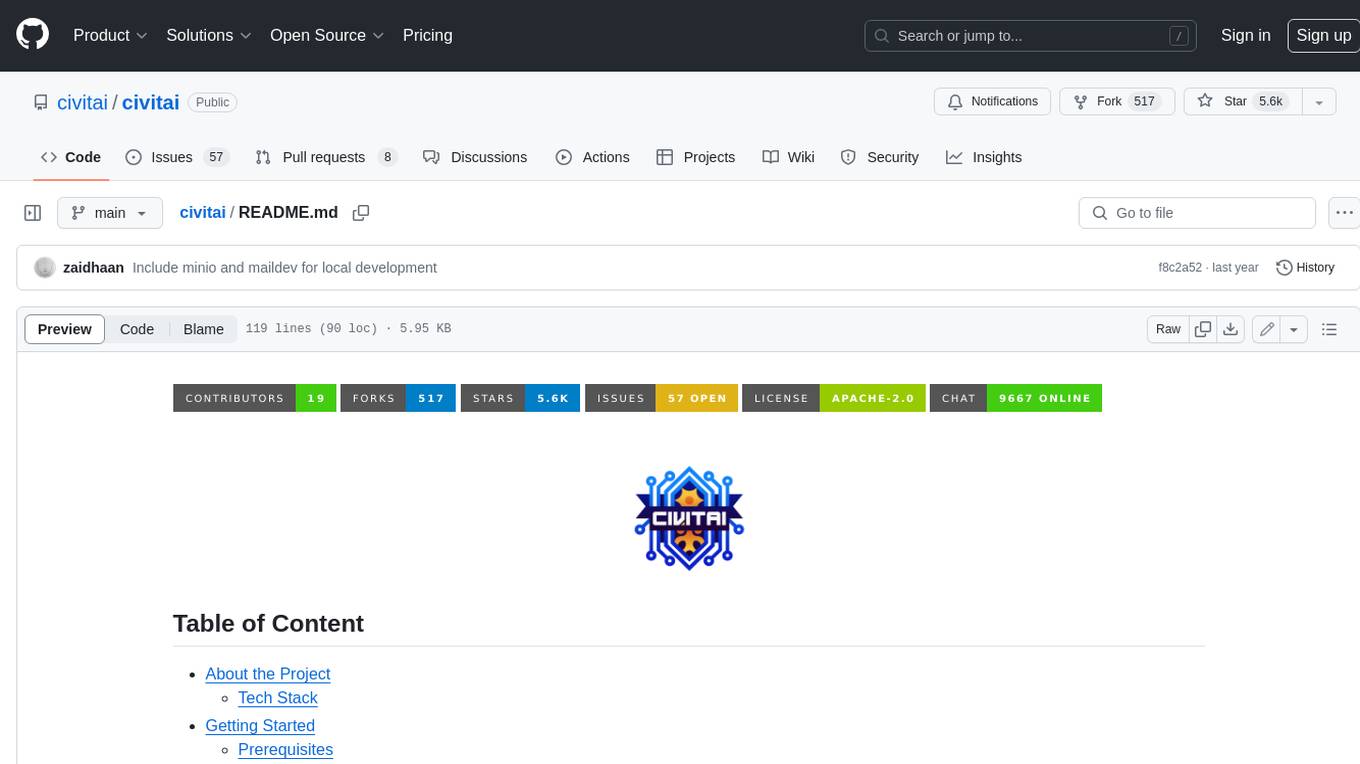
civitai
Civitai is a platform where people can share their stable diffusion models (textual inversions, hypernetworks, aesthetic gradients, VAEs, and any other crazy stuff people do to customize their AI generations), collaborate with others to improve them, and learn from each other's work. The platform allows users to create an account, upload their models, and browse models that have been shared by others. Users can also leave comments and feedback on each other's models to facilitate collaboration and knowledge sharing.
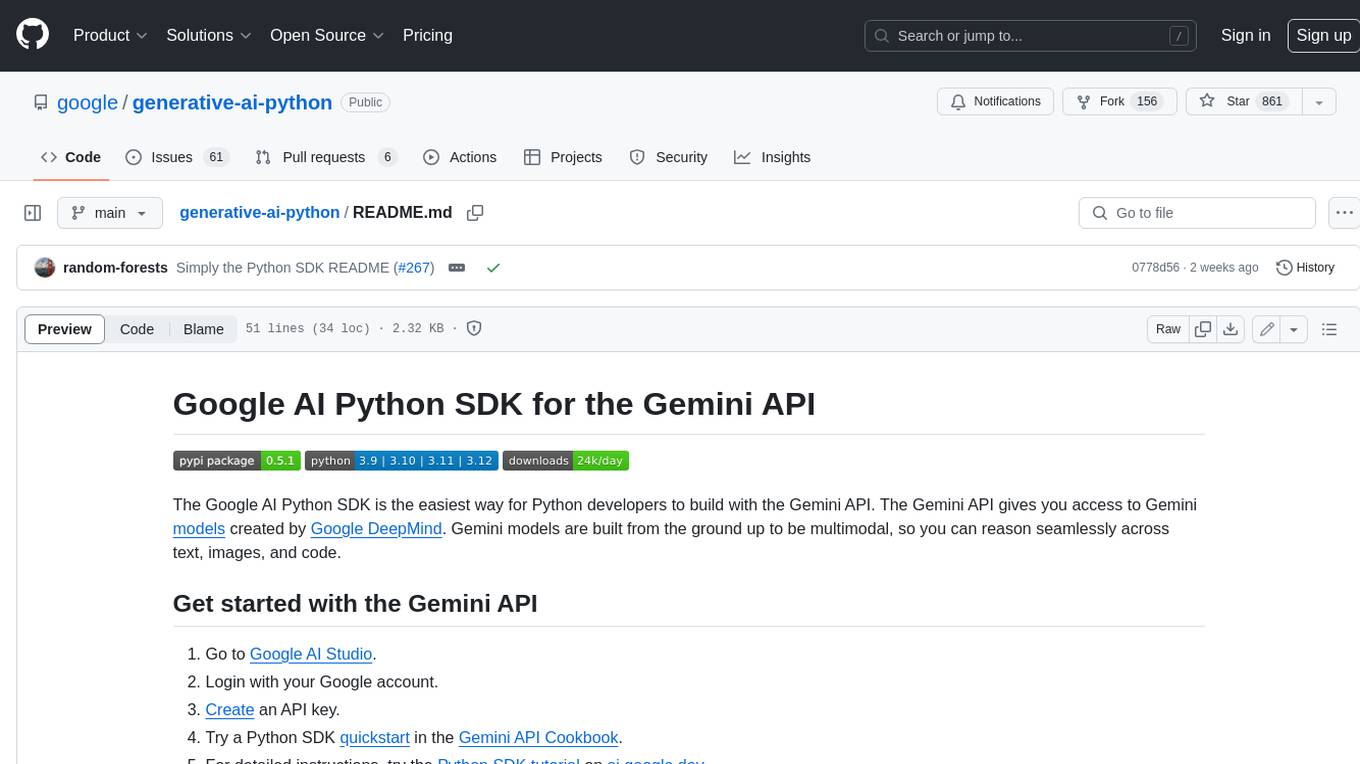
generative-ai-python
The Google AI Python SDK is the easiest way for Python developers to build with the Gemini API. The Gemini API gives you access to Gemini models created by Google DeepMind. Gemini models are built from the ground up to be multimodal, so you can reason seamlessly across text, images, and code.
For similar jobs
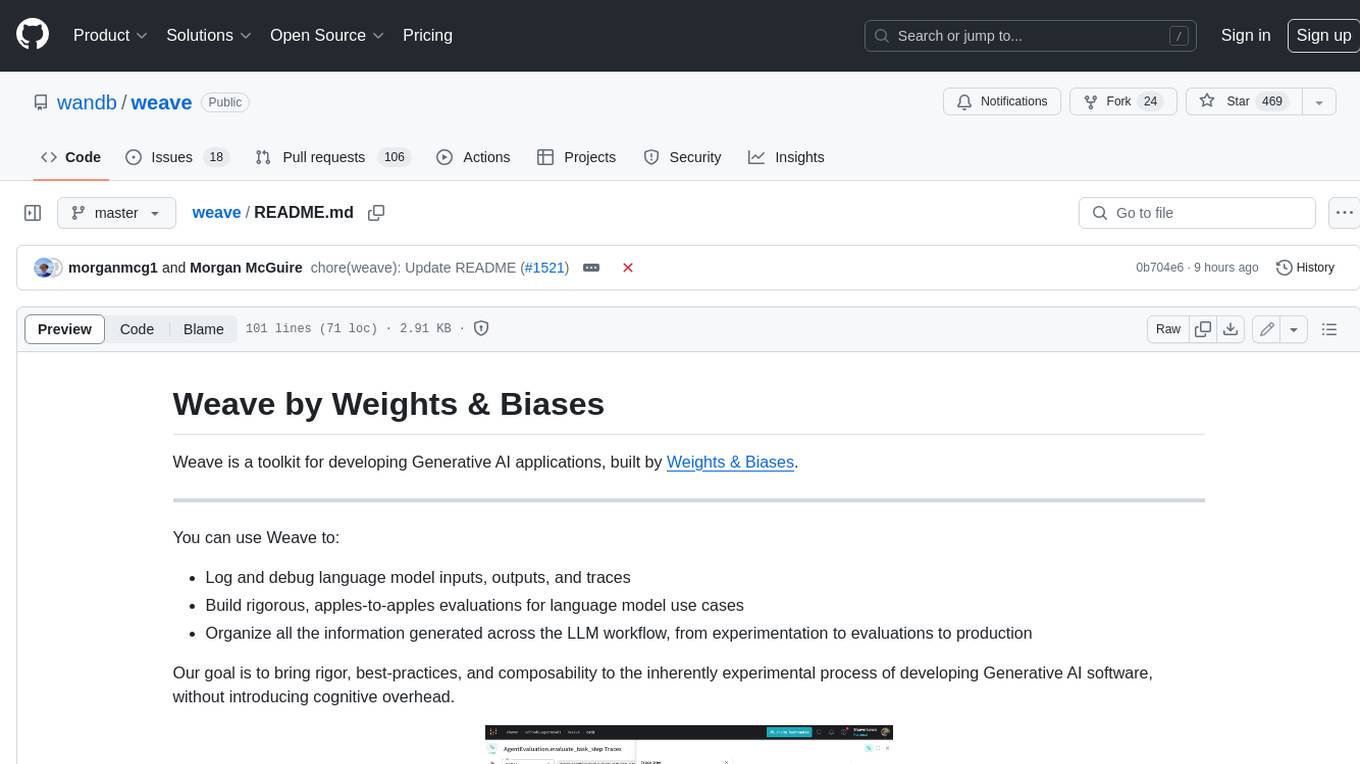
weave
Weave is a toolkit for developing Generative AI applications, built by Weights & Biases. With Weave, you can log and debug language model inputs, outputs, and traces; build rigorous, apples-to-apples evaluations for language model use cases; and organize all the information generated across the LLM workflow, from experimentation to evaluations to production. Weave aims to bring rigor, best-practices, and composability to the inherently experimental process of developing Generative AI software, without introducing cognitive overhead.
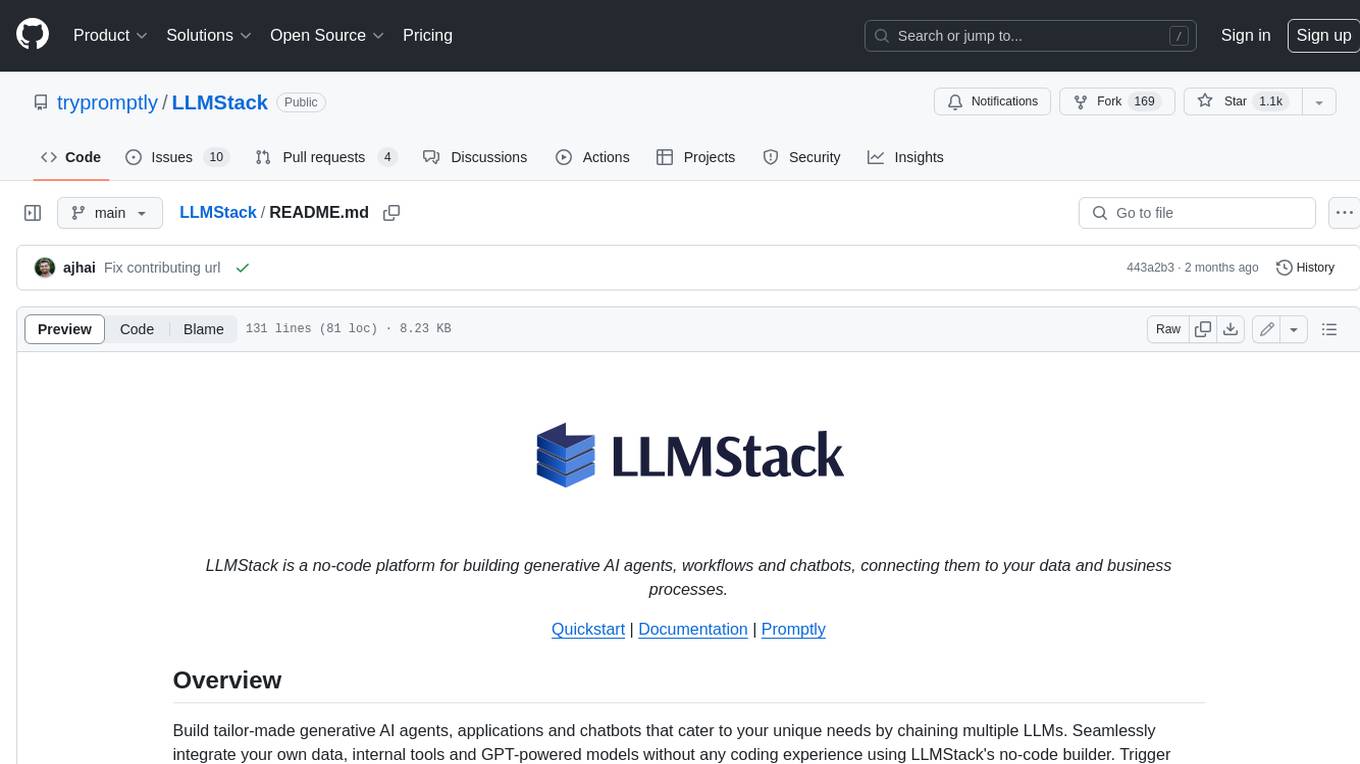
LLMStack
LLMStack is a no-code platform for building generative AI agents, workflows, and chatbots. It allows users to connect their own data, internal tools, and GPT-powered models without any coding experience. LLMStack can be deployed to the cloud or on-premise and can be accessed via HTTP API or triggered from Slack or Discord.
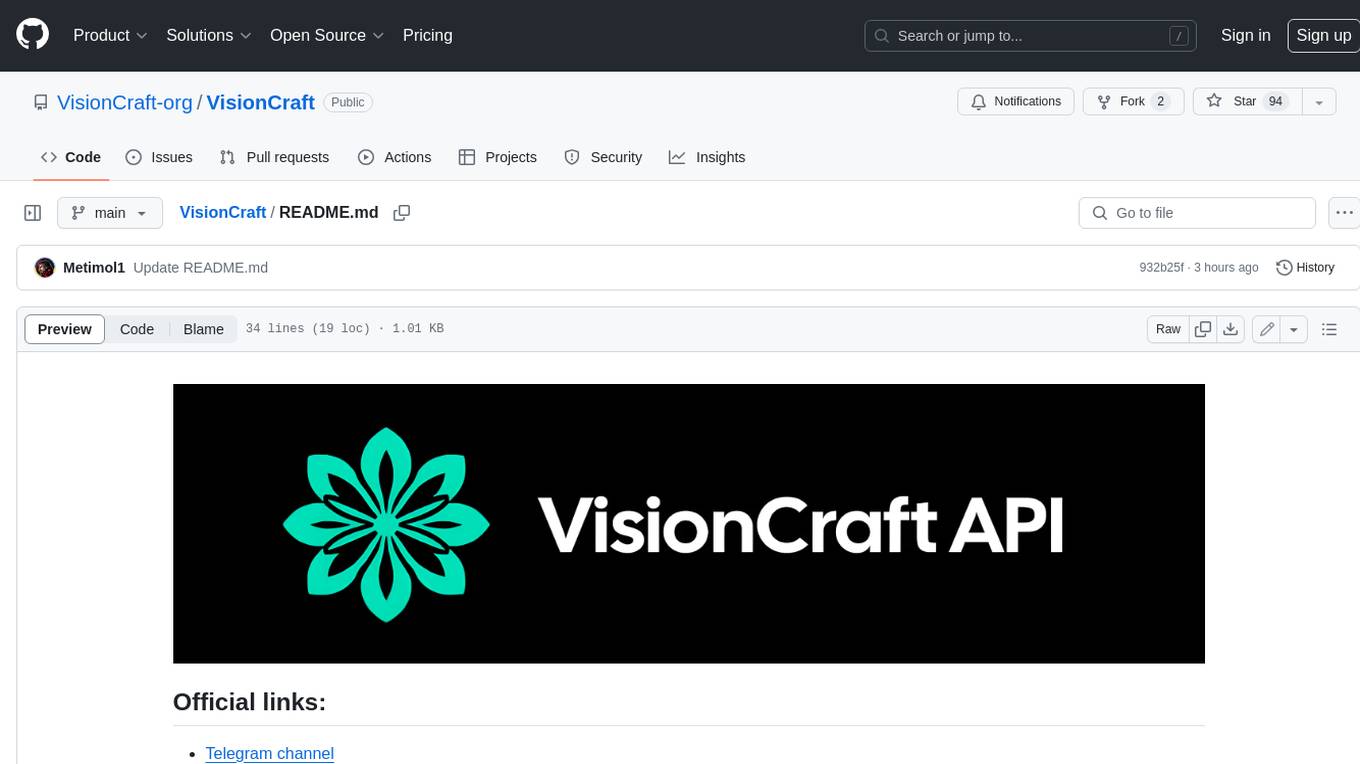
VisionCraft
The VisionCraft API is a free API for using over 100 different AI models. From images to sound.
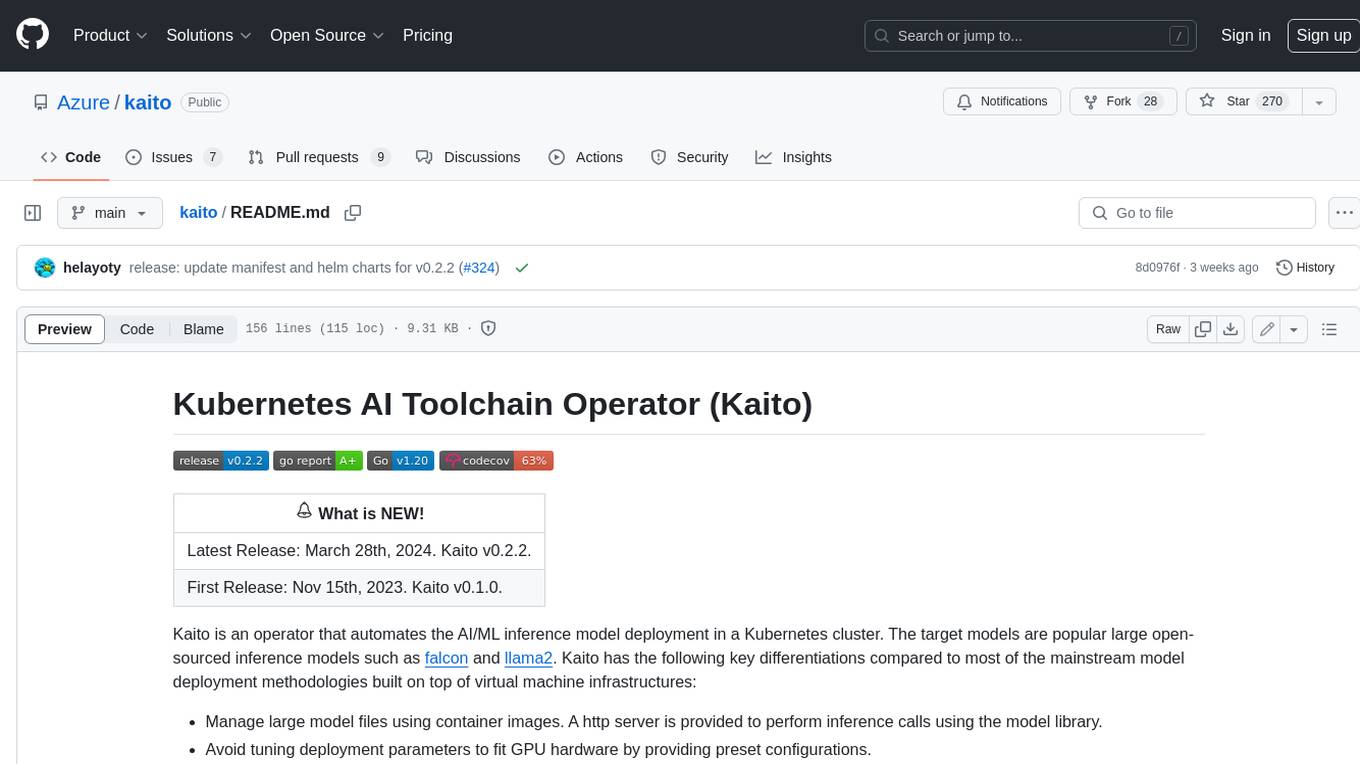
kaito
Kaito is an operator that automates the AI/ML inference model deployment in a Kubernetes cluster. It manages large model files using container images, avoids tuning deployment parameters to fit GPU hardware by providing preset configurations, auto-provisions GPU nodes based on model requirements, and hosts large model images in the public Microsoft Container Registry (MCR) if the license allows. Using Kaito, the workflow of onboarding large AI inference models in Kubernetes is largely simplified.
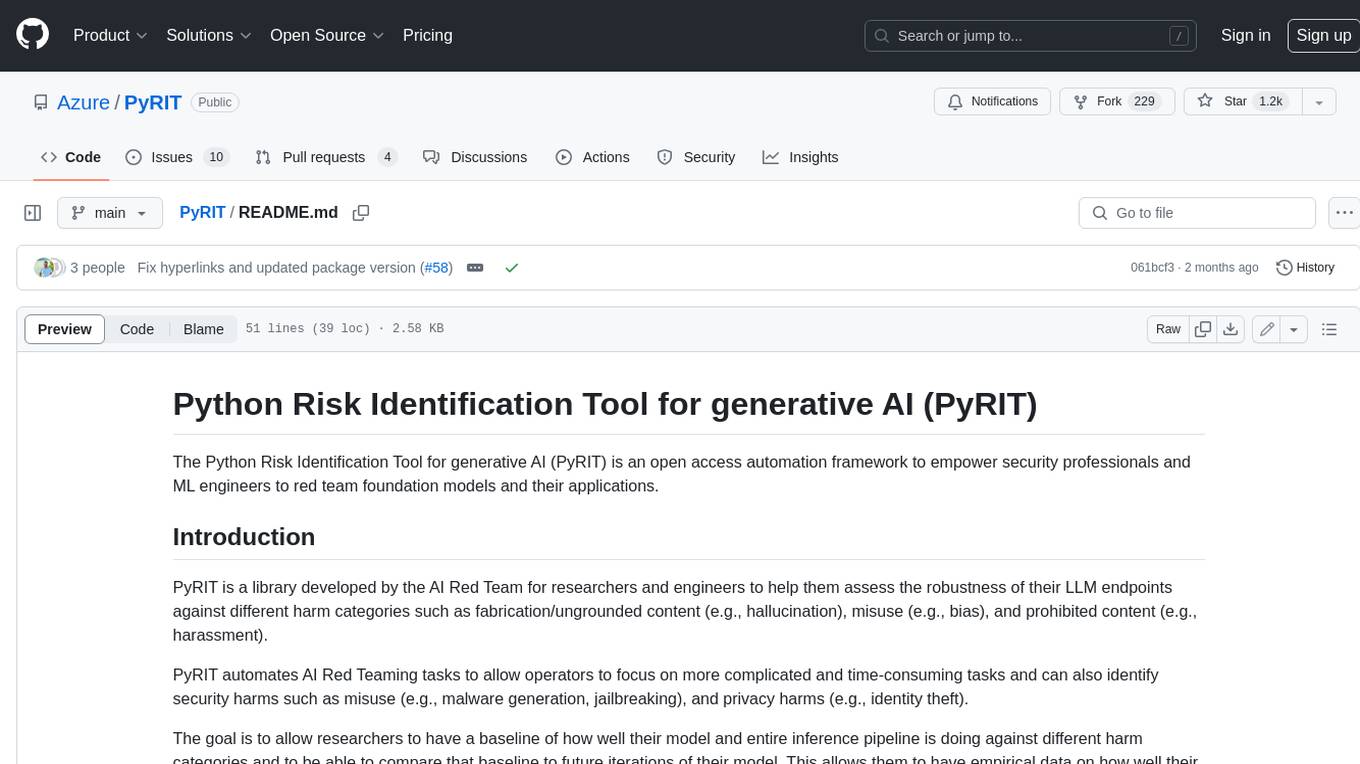
PyRIT
PyRIT is an open access automation framework designed to empower security professionals and ML engineers to red team foundation models and their applications. It automates AI Red Teaming tasks to allow operators to focus on more complicated and time-consuming tasks and can also identify security harms such as misuse (e.g., malware generation, jailbreaking), and privacy harms (e.g., identity theft). The goal is to allow researchers to have a baseline of how well their model and entire inference pipeline is doing against different harm categories and to be able to compare that baseline to future iterations of their model. This allows them to have empirical data on how well their model is doing today, and detect any degradation of performance based on future improvements.
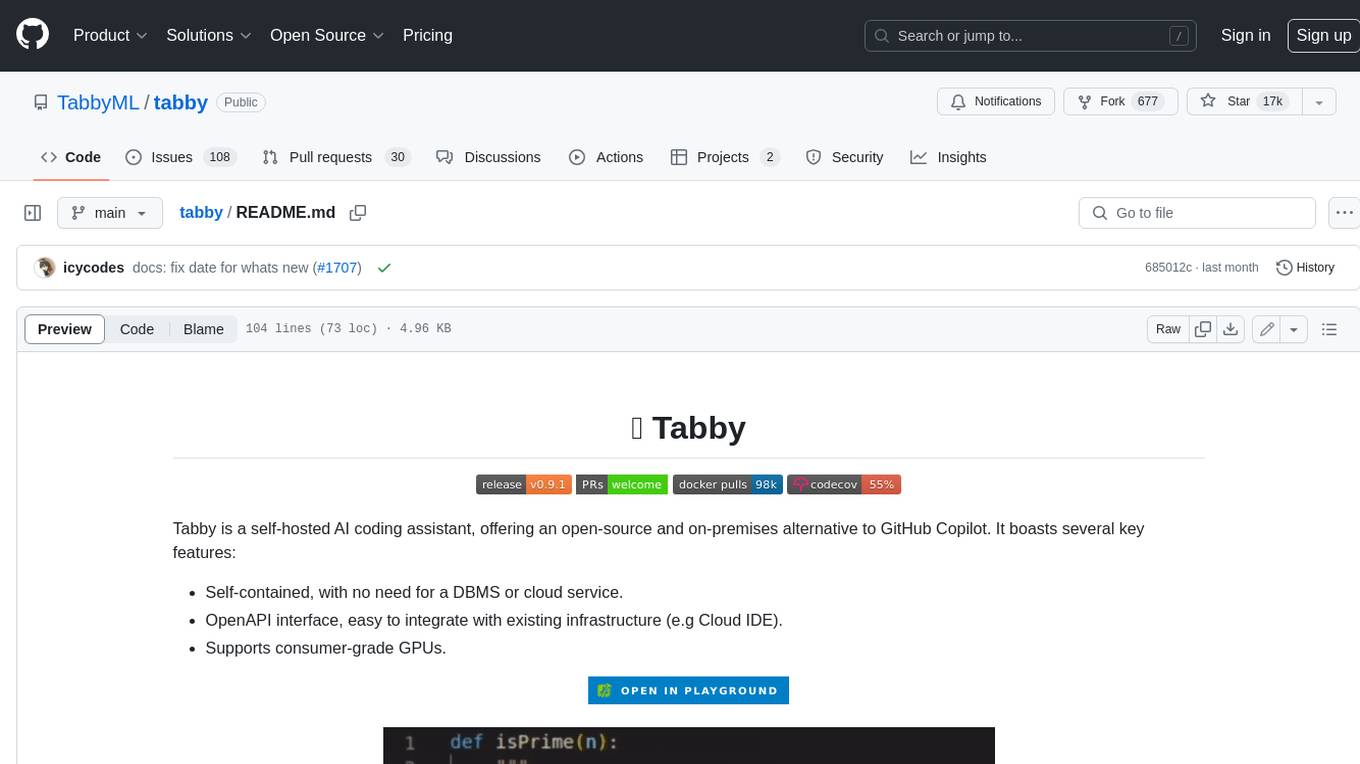
tabby
Tabby is a self-hosted AI coding assistant, offering an open-source and on-premises alternative to GitHub Copilot. It boasts several key features: * Self-contained, with no need for a DBMS or cloud service. * OpenAPI interface, easy to integrate with existing infrastructure (e.g Cloud IDE). * Supports consumer-grade GPUs.
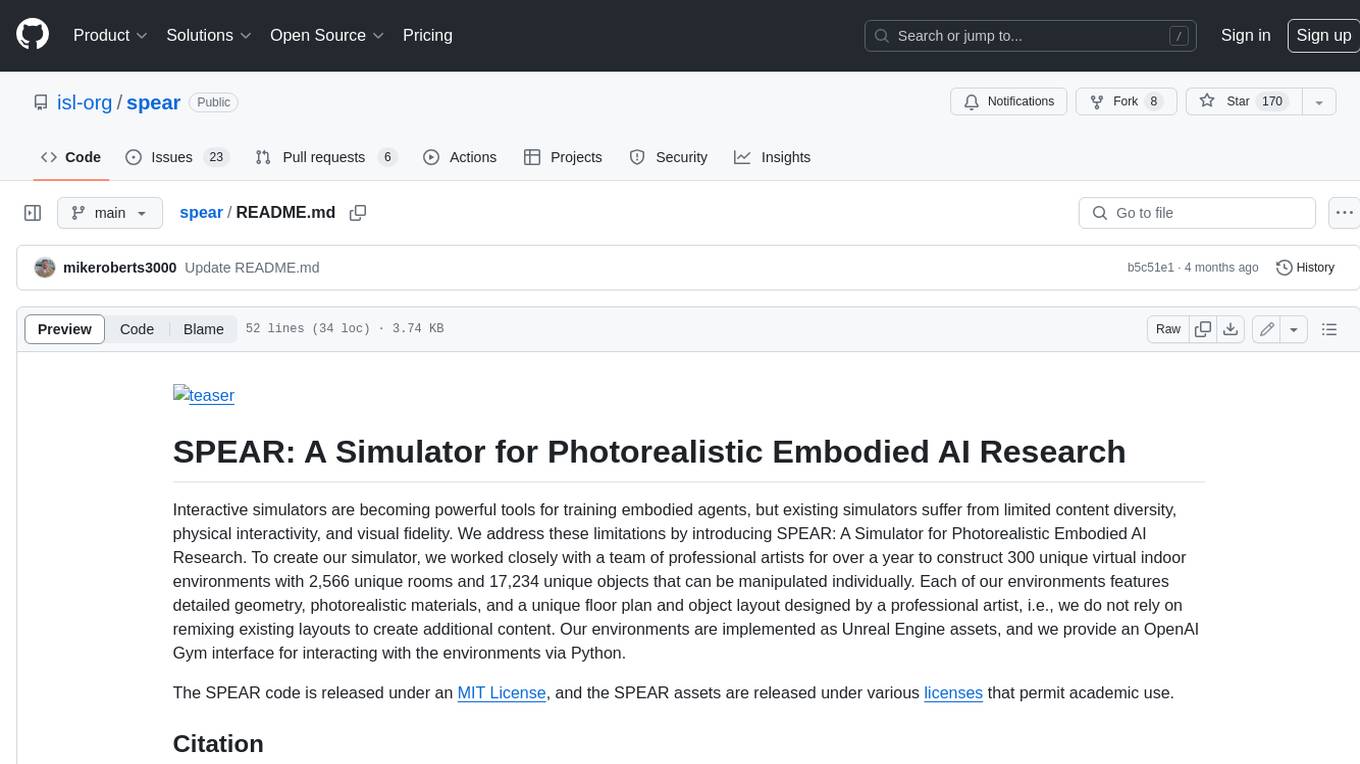
spear
SPEAR (Simulator for Photorealistic Embodied AI Research) is a powerful tool for training embodied agents. It features 300 unique virtual indoor environments with 2,566 unique rooms and 17,234 unique objects that can be manipulated individually. Each environment is designed by a professional artist and features detailed geometry, photorealistic materials, and a unique floor plan and object layout. SPEAR is implemented as Unreal Engine assets and provides an OpenAI Gym interface for interacting with the environments via Python.
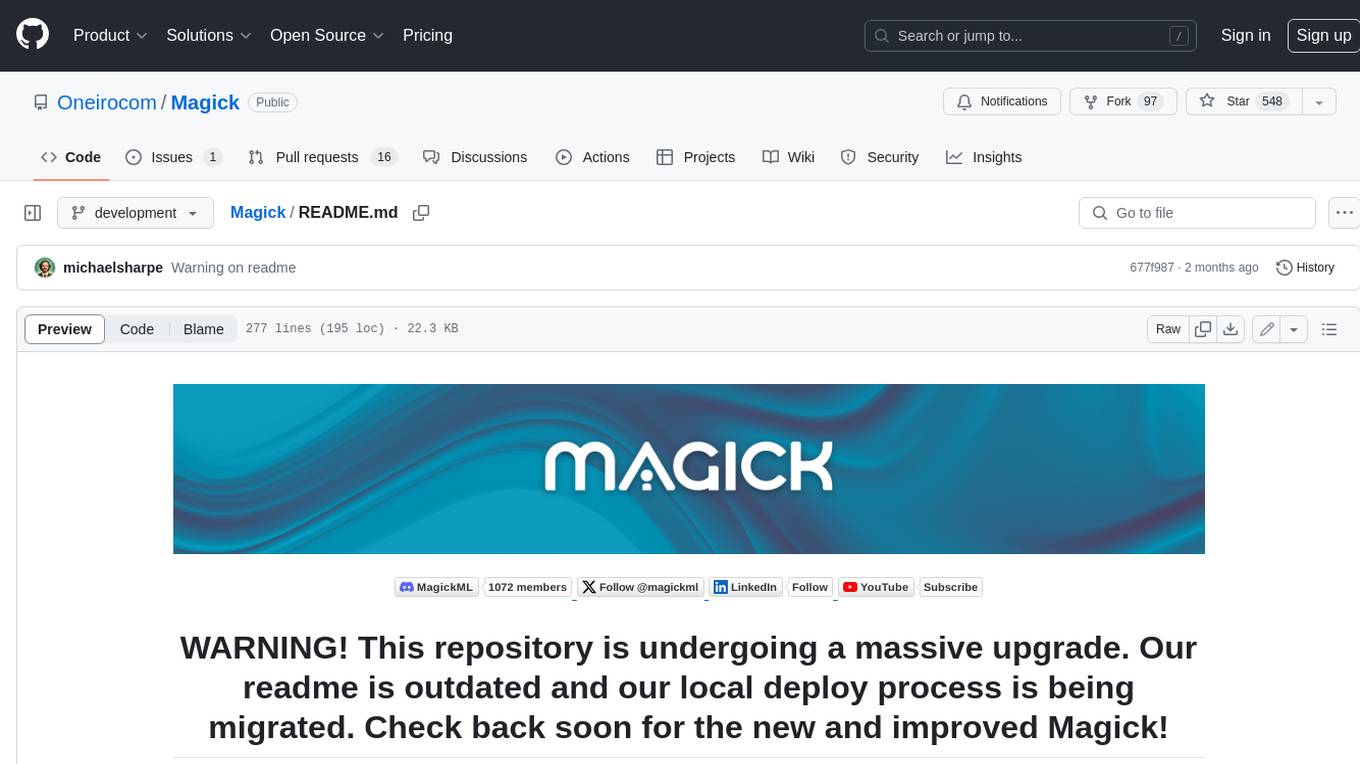
Magick
Magick is a groundbreaking visual AIDE (Artificial Intelligence Development Environment) for no-code data pipelines and multimodal agents. Magick can connect to other services and comes with nodes and templates well-suited for intelligent agents, chatbots, complex reasoning systems and realistic characters.