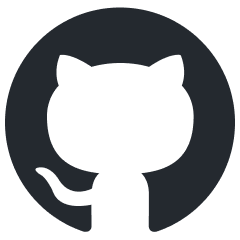
shellChatGPT
Shell wrapper for OpenAI's ChatGPT, DALL-E, Whisper, and TTS. Features LocalAI, Ollama, Gemini, Mistral, Groq, Anthropic, Novita AI, and xAI integration.
Stars: 71
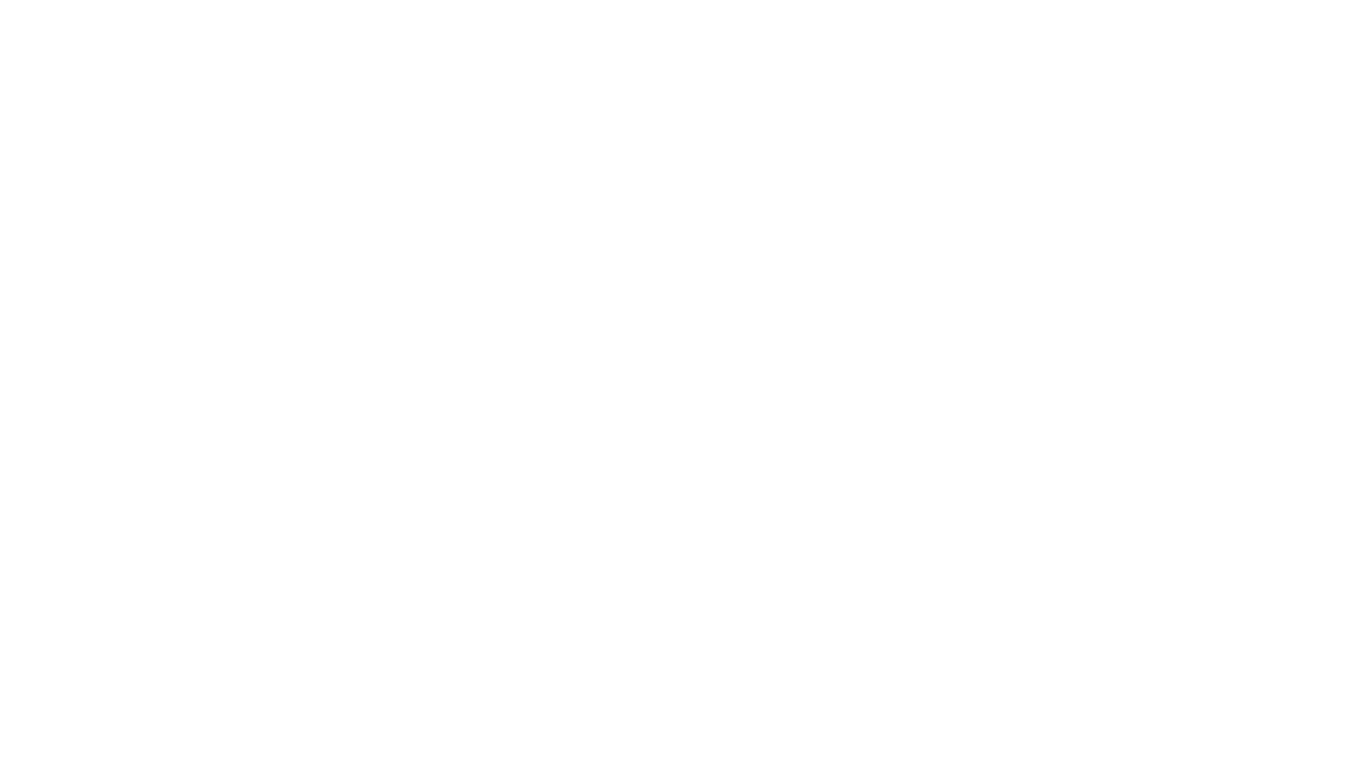
ShellChatGPT is a shell wrapper for OpenAI's ChatGPT, DALL-E, Whisper, and TTS, featuring integration with LocalAI, Ollama, Gemini, Mistral, Groq, and GitHub Models. It provides text and chat completions, vision, reasoning, and audio models, voice-in and voice-out chatting mode, text editor interface, markdown rendering support, session management, instruction prompt manager, integration with various service providers, command line completion, file picker dialogs, color scheme personalization, stdin and text file input support, and compatibility with Linux, FreeBSD, MacOS, and Termux for a responsive experience.
README:
Shell wrapper for OpenAI's ChatGPT, DALL-E, Whisper, and TTS. Features LocalAI, Ollama, Gemini, Mistral, Groq, and GitHub Models integration.
Chat completions with streaming by defaults.
Expand Markdown Processing
Markdown processing on response is triggered automatically for some time now!
Markdown rendering of chat response (optional).
Expand Text Completions
In pure text completions, start by typing some text that is going to be completed, such as news, stories, or poems.
Expand Insert Mode
Add the insert tag [insert]
where it is going to be completed.
Mistral code models
work well with the insert / fill-in-the-middel (FIM) mode!
If no suffix is provided, it works as plain text completions.
โ Click to expand! โ
-
- 3.1 Required Packages
- 3.2 Optional Packages
- 3.3 Installation
- 3.4 Usage Examples
-
- 8.1 Custom Prompts
- 8.2 Awesome Prompts
-
- 9.1 Bash
- 9.2 Zsh
- 9.3 Troubleshoot
-
- 11.1 Image Generations
- 11.2 Image Variations
- 11.3 Image Edits
- 11.3.1 Outpaint - Canvas Extension
- 11.3.2 Inpaint - Fill in the Gaps
- 11.4 Speech Transcriptions / Translations
-
- 12.1 LocalAI
- 12.1.1 LocalAI Server
- 12.1.2 Tips
- 12.1.3 Running the shell wrapper
- 12.1.4 Installing Models
- 12.1.5 Host API Configuration
- 12.2 Ollama
- 12.3 Google AI
- 12.4 Mistral AI
- 12.5 Groq
- 12.6 Anthropic
- 12.7 GitHub Models
- 12.8 Novita AI
- 12.9 xAI
- 12.1 LocalAI
-
- 14.1 Dependencies
- 14.2 TTS Chat - Removal of Markdown
- 14.3 Tiktoken
- 14.4 Troubleshoot
- Text and chat completions.
- Vision, reasoning and audio models
-
Voice-in (Whisper) plus voice out (TTS) chatting mode (
options -cczw
) - Text editor interface, Bash readline, and multiline/cat modes
- Markdown rendering support in response
- Easily regenerate responses
- Manage sessions, print out previous sessions
- Instruction prompt manager, easily create and set the initial system prompt
- Integration with various service providers and custom BaseUrl.
- Support for awesome-chatgpt-prompts & the Chinese variant
- Command line completion and file picker dialogs for a smoother experience ๐ป
- Colour scheme personalisation ๐จ and a configuration file
- Stdin and text file input support
- Shouldโข work on Linux, FreeBSD, MacOS, and Termux
- Fast shell code for a responsive experience! โก๏ธ
-
Bash
-
cURL
, andJQ
Packages required for specific features.
Click to expand!
-
Base64
- Image endpoint, multimodal models -
Python
- Modules tiktoken, markdown, bs4 -
ImageMagick
/fbida
- Image edits and variations -
SoX
/Arecord
/FFmpeg
- Record input (Whisper) -
mpv
/SoX
/Vlc
/FFplay
/afplay
- Play TTS output -
xdg-open
/open
/xsel
/xclip
/pbcopy
- Open images, set clipboard -
W3M
/Lynx
/ELinks
/Links
- Dump URL text -
bat
/Pygmentize
/Glow
/mdcat
/mdless
- Markdown support -
termux-api
/termux-tools
/play-audio
- Termux system -
poppler
/gs
/abiword
/ebook-convert
/LibreOffice
- Dump PDF or Doc as text -
dialog
/kdialog
/zenity
/osascript
/termux-dialog
- File picker
A. Download the stand-alone
chatgpt.sh
script
and make it executable:
wget https://gitlab.com/fenixdragao/shellchatgpt/-/raw/main/chatgpt.sh
chmod +x ./chatgpt.sh
B. Or clone this repo:
git clone https://gitlab.com/fenixdragao/shellchatgpt.git
C. Optionally, download and set the configuration file
~/.chatgpt.conf
:
#save configuration template:
chatgpt.sh -FF >> ~/.chatgpt.conf
#edit:
chatgpt.sh -F
# Or
vim ~/.chatgpt.conf
With command line options -cc
, some properties are set automatically to create a chat bot.
Start a new session in chat mode, and set a different temperature (gpt-3.5 and gpt-4+ models):
chatgpt.sh -cc -t0.7
Change the maximum response length to 4k tokens:
chatgpt.sh -cc -4000
chatgpt.sh -cc -M 4000
Or change a model token capacity to 200k tokens:
chatgpt.sh -cc -4000-200000
chatgpt.sh -cc -M 4000-200000
Create Marv, the sarcastic bot:
chatgpt.sh -512 -cc --frequency-penalty=0.7 --temp=0.8 --top_p=0.4 --restart-seq='\nYou: ' --start-seq='\nMarv:' --stop='You:' --stop='Marv:' -S'Marv is a factual chatbot that reluctantly answers questions with sarcastic responses.'
Load the unix instruction file ("unix.pr") for a new session. The command line syntaxes below are all aliases:
chatgpt.sh -cc .unix
chatgpt.sh -cc.unix
chatgpt.sh -cc -.unix
chatgpt.sh -cc -S .unix
To only chage the history file that the session will be recorded,
set the first positional argument in command line with the operator forward slash "/
"
and the name of the history file (defaults to the /session
command).
chatgpt.sh -cc /test
chatgpt.sh -cc /stest
chatgpt.sh -cc "/session test"
There is a shortcut to load an older session from the current history file. This opens a basic interative interface.
chatgpt.sh -cc .
Technically, this copies an old session from the target history file to the tail of it, so we can resume the session.
To load an older session from a history file that is different from the defaults, there are some options.
In order to grep for sessions with a regex, it is easier to enter chat mode
and then type in the chat command /grep [regex]
.
To only change to a defined history file name, run command !session [name]
.
Print out last session, optionally set the history name:
chatgpt.sh -P
chatgpt.sh -P /test
To send an image
/ url
to vision models, start the script and then either
set the image with the !img
chat command with one or more filepaths / URLs.
chatgpt.sh -cc -m gpt-4-vision-preview '!img path/to/image.jpg'
Alternatively, set the image paths / URLs at the end of the prompt:
chatgpt.sh -cc -m gpt-4-vision-preview
[...]
Q: In this first user prompt, what can you see? https://i.imgur.com/wpXKyRo.jpeg
TIP: Run chat command !info
to check model configuration!
DEBUG: Set option -V
to see the raw JSON request body.
To make an easy workfow, the user may add a filepath or URL to the end of the prompt. The file is then read and the text content appended to the user prompt. This is a basic text feature that works with any model.
chatgpt.sh -cc
[...]
Q: What is this page: https://example.com
Q: Help me study this paper. ~/Downloads/Prigogine\ Perspective\ on\ Nature.pdf
In the second example above, the PDF will be dumped as text (interactive mode).
For PDF text dump support, poppler/abiword
is required.
For doc and odt files, LibreOffice
is required.
See the Optional Packages section.
Also note that file paths containing white spaces must be
blackslash-escaped, or the filepath must be preceded by a pipe |
character.
My text prompt. | path/to the file.jpg
Multiple images and audio files may be appended the the prompt in this way!
The /pick
command opens a file picker (usually a command-line
file manager). The selected file's path will be appended to the
current prompt in editing mode.
The /pick
and /sh
commands may be run when typed at the end of
the current prompt, such as [PROMPT] /sh
, which opens a new
shell instance to execute commands interactively. The output of these
commands is appended to the current prompt.
When the /pick
command is run at the end of the prompt, the selected
file path is appended instead.
File paths that contain white spaces need backslash-escaping in some functions.
๐ฃ๏ธ Chat completion with speech in and out (Whisper plus TTS):
chatgpt.sh -ccwz
Chat in Portuguese with Whisper and set onyx as the TTS voice:
chatgpt.sh -ccwz -- pt -- onyx
Chat mode provides a conversational experience, prompting the user to confirm each step.
For a more automated execution, set option -v
,
and -vv
for hands-free experience (live chat with silence detection),
such as:
chatgpt.sh -cc -w -z -v
chatgpt.sh -cc -w -z -vv
Audio models, such as gpt-4o-audio
, deal with audio input and output directly, thus reducing latency in a conversation turn.
To activate the microphone recording function of the script, set command line option -w
.
Otherwise, the audio model accepts any compatible audio file (such as mp3, wav, and opus).
These files can be added to be loaded at the very end of the user prompt
or added with chat command /audio path/to/file.mp3
.
Similarly as described above, to activate the audio output mode of an audio model, do set command line option -z
to make sure to enable the speech synthesis function!
chatgpt.sh -cc -w -z -vv -m "gpt-4o-audio-preview"
Mind that this does not implement the realtime models.
When text completions is set for chatting with option -c
,
some properties are configured automatically to instruct the bot.
chatgpt.sh -c "Hello there! What is your name?"
This is the pure text completions endpoint. It is typically used to complete input text, such as for completing part of an essay.
To complete text from the command line input prompt, either
set option -d
or set a text completion model name.
chatgpt.sh -128 -m gpt-3.5-turbo-instruct "Hello there! Your name is"
chatgpt.sh -128 -d "The journalist loo"
The above examples also set maximum response value to 128 tokens.
Enter single-turn interactive mode:
chatgpt.sh -d
NOTE: For multi-turn mode with history support, set option -dd
.
A strong Instruction prompt may be needed for the language model to do what is required.
Set an instruction prompt for better results:
chatgpt.sh -d -S 'The following is a newspaper article.' "It all starts when FBI agents arrived at the governor house and"
chatgpt.sh -d -S'You are an AI assistant.' "The list below contain the 10 biggest cities in the w"
Set option -q
(or -qq
for multiturn) to enable insert mode and add the
string [insert]
where the model should insert text:
chatgpt.sh -q 'It was raining when [insert] tomorrow.'
NOTE: This example works with no instruction prompt! An instruction prompt in this mode may interfere with insert completions.
NOTE: Insert mode
works with model instruct models
.
Mistral AI has a nice FIM (fill-in-the-middle) endpoint that works
with code
models and is really good!
To enable markdown rendering of responses, set command line option --markdown
,
or run /md
in chat mode. To render last response in markdown once,
run //md
.
The markdown option uses bat
as it has line buffering on by defaults,
however other software is supported.
Set it such as --markdown=glow
or /md mdless
on chat mode.
Type in any of the following markdown software as argument to the option:
bat
, pygmentize
, glow
, mdcat
, or mdless
.
Unless the chat option -c
or -cc
are set, no instruction is
given to the language model. On chat mode, if no instruction is set,
minimal instruction is given, and some options set, such as increasing
temp and presence penalty, in order to un-lobotomise the bot.
Prompt engineering is an art on itself. Study carefully how to craft the best prompts to get the most out of text, code and chat completions models.
The model steering and capabilities require prompt engineering to even know that it should answer the questions.
Set a one-shot instruction prompt with option -S
:
chatgpt.sh -cc -S 'You are a PhD psycologist student.'
chatgpt.sh -ccS'You are a professional software programmer.'
To create or load a prompt template file, set the first positional argument
as .prompt_name
or ,prompt_name
.
In the second case, load the prompt and single-shot edit it.
chatgpt.sh -cc .psycologist
chatgpt.sh -cc ,software_programmer
Alternatively, set option -S
with the operator and the name of
the prompt as an argument:
chatgpt.sh -cc -S .psycologist
chatgpt.sh -cc -S,software_programmer
This will load the custom prompt or create it if it does not yet exist. In the second example, single-shot editing will be available after loading prompt software_programmer.
Please note and make sure to backup your important custom prompts!
They are located at "~/.cache/chatgptsh/
" with the extension ".pr".
Set a prompt from awesome-chatgpt-prompts or awesome-chatgpt-prompts-zh, (use with davinci and gpt-3.5+ models):
chatgpt.sh -cc -S /linux_terminal
chatgpt.sh -cc -S /Relationship_Coach
chatgpt.sh -cc -S '%ๆ
ไปป้
ๆๅไฝ่ๅฎ'
This project includes shell completions to enhance the user command-line experience.
Install following one of the methods below.
System-wide
sudo cp comp/bash/chatgpt.sh /usr/share/bash-completion/completions/
User-specific
mkdir -p ~/.local/share/bash-completion/completions/
cp comp/bash/chatgpt.sh ~/.local/share/bash-completion/completions/
Visit the bash-completion repository.
Install at the system location
sudo cp comp/zsh/_chatgpt.sh /usr/share/zsh/site-functions/
User-specific location
To set user-specific completion, make sure to place the completion
script under a directory in the $fpath
array.
The user may create the ~/.zfunc/
directory, for example, and
add the following lines to her ~/.zshrc
:
[[ -d ~/.zfunc ]] && fpath=(~/.zfunc $fpath)
autoload -Uz compinit
compinit
Make sure compinit
is run after setting $fpath
!
Visit the zsh-completion repository.
Bash and Zsh completions should be active in new terminal sessions.
If not, ensure your ~/.bashrc
and ~/.zshrc
source
the completion files correctly.
-
YouTube feature will get only YouTube videos heading title and its transcripts (when available).
-
PDF support feature extracts the PDF text (no images) and appends it to the user request.
-
Run chat commands with either operator
!
or/
. -
Edit live history entries with command
!hist
, for context injection.
- One can regenerate a response by typing in a new prompt a single slash
/
, or//
to have last prompt edited before the new request.
Generate image according to prompt:
chatgpt.sh -i "Dark tower in the middle of a field of red roses."
chatgpt.sh -i "512x512" "A tower."
Generate image variation:
chatgpt.sh -i path/to/image.png
chatgpt.sh -i path/to/image.png path/to/mask.png "A pink flamingo."
In this example, a mask is made from the white colour.
Adding a bat in the night sky.
Generate transcription from audio file speech. A prompt to guide the model's style is optional. The prompt should match the speech language:
chatgpt.sh -w path/to/audio.mp3
chatgpt.sh -w path/to/audio.mp3 "en" "This is a poem about X."
1. Generate transcription from voice recording, set Portuguese as the language to transcribe to:
chatgpt.sh -w pt
This also works to transcribe from one language to another.
2. Transcribe any language speech input to Japanese (prompt should be in the same language as the input audio language, preferably):
chatgpt.sh -w ja "A job interview is currently being done."
3.1 Translate English speech input to Japanese, and generate speech output from the text response.
chatgpt.sh -wz ja "Getting directions to famous places in the city."
3.2 Also doing it conversely, this gives an opportunity to (manual) conversation turns of two speakers of different languages. Below, a Japanese speaker can translate its voice and generate audio in the target language.
chatgpt.sh -wz en "Providing directions to famous places in the city."
4. Translate speech from any language to English:
chatgpt.sh -W [audio_file]
chatgpt.sh -W
To retry with the last microphone recording saved in the cache, set
audio_file as last
or retry
.
NOTE: Generate phrasal-level timestamps double setting option -ww
or option -WW
.
For word-level timestamps, set option -www
or -WWW
.
Other than Ollama and LocalAI local servers, the free service providers are GitHub Models, Google Vertex, and Groq.
Make sure you have got mudler's LocalAI, server set up and running.
The server can be run as a docker container or a binary can be downloaded. Check LocalAI tutorials Container Images, and Run Models Manually for an idea on how to install, download a model and set it up.
โโโโโโโโโโโโโโโโโโโโโโโโโโโโโโโโโโโโโโโโโโโโโโโโโโโโโ
โ Fiber v2.50.0 โ
โ http://127.0.0.1:8080 โ
โ (bound on host 0.0.0.0 and port 8080) โ
โ โ
โ Handlers ............. 1 Processes ........... 1 โ
โ Prefork ....... Disabled PID ..................1 โ
โโโโโโโโโโโโโโโโโโโโโโโโโโโโโโโโโโโโโโโโโโโโโโโโโโโโโ
1. Download a binary of localai
for your system from Mudler's release GitHub repo.
2. Run localai run --help
to check comamnd line options and environment variables.
3. Set up $GALLERIES
before starting up the server:
export GALLERIES='[{"name":"localai", "url":"github:mudler/localai/gallery/index.yaml"}]' #defaults
export GALLERIES='[{"name":"model-gallery", "url":"github:go-skynet/model-gallery/index.yaml"}]'
export GALLERIES='[{"name":"huggingface", "url": "github:go-skynet/model-gallery/huggingface.yaml"}]'
4. Install the model named phi-2-chat
from a yaml
file manually, while the server is running:
curl -L http://localhost:8080/models/apply -H "Content-Type: application/json" -d '{ "config_url": "https://raw.githubusercontent.com/mudler/LocalAI/master/embedded/models/phi-2-chat.yaml" }'
Finally, when running chatgpt.sh
, set the model name:
chatgpt.sh --localai -cc -m luna-ai-llama2
Setting some stop sequences may be needed to prevent the model from generating text past context:
chatgpt.sh --localai -cc -m luna-ai-llama2 -s'### User:' -s'### Response:'
Optionally set restart and start sequences for text completions
endpoint (option -c
), such as -s'\n### User: ' -s'\n### Response:'
(do mind setting newlines \n and whitespaces correctly).
And that's it!
Model names may be printed with chatgpt.sh -l
. A model may be
supplied as argument, so that only that model details are shown.
NOTE: Model management (downloading and setting up) must follow the LocalAI and Ollama projects guidelines and methods.
For image generation, install Stable Diffusion from the URL
github:go-skynet/model-gallery/stablediffusion.yaml
,
and for speech transcription, download Whisper from the URL
github:go-skynet/model-gallery/whisper-base.yaml
.
If the service provider Base URL is different from the defaults.
The environment varible $OPENAI_BASE_URL
is read at invocation.
export OPENAI_BASE_URL="http://127.0.0.1:8080/v1"
chatgpt.sh -c -m luna-ai-llama2
To set it a in a more permanet fashion, edit the script
configuration file .chatgpt.conf
.
Use vim:
vim ~/.chatgpt.conf
Or edit the configuration with a comamnd line option.
chatgpt.sh -F
And set the following variable:
# ~/.chatgpt.conf
OPENAI_BASE_URL="http://127.0.0.1:8080/v1"
Visit Ollama repository, and follow the instructions to install, download models, and set up the server.
After having Ollama server running, set option -O
(--ollama
),
and the name of the model in chatgpt.sh
:
chatgpt.sh -cc -O -m llama2
If Ollama server URL is not the defaults http://localhost:11434
,
edit chatgpt.sh
configuration file, and set the following variable:
# ~/.chatgpt.conf
OLLAMA_BASE_URL="http://192.168.0.3:11434"
Get a free API key for Google to be able to use Gemini and vision models. Users have a free bandwidth of 60 requests per minute, and the script offers a basic implementation of the API.
Set the enviroment variable $GOOGLE_API_KEY
and run the script
with option --google
, such as:
chatgpt.sh --google -cc -m gemini-pro-vision
OBS: Google Gemini vision models are not enabled for multiturn at the API side, so we hack it.
To list all available models, run chatgpt.sh --google -l
.
Set up a Mistral AI account,
declare the enviroment variable $MISTRAL_API_KEY
,
and run the script with option --mistral
for complete integration.
Sign in to Groq.
Create a new API key or use an existing one to set
the environmental variable $GROQ_API_KEY
.
Run the script with option --groq
.
Currently, llamma3.1 models are available at lightening speeds!
Sign in to Antropic AI.
Create a new API key or use an existing one to set
the environmental variable $ANTHROPIC_API_KEY
.
Run the script with option --anthropic
or --ant
.
Check the Claude-3 models! Run the script as:
chatgpt.sh --anthropic -cc -m claude-3-5-sonnet-20240620
The script also works on text completions with models such as
claude-2.1
, although the API documentation flags it as deprecated.
Try:
chatgpt.sh --ant -c -m claude-2.1
GitHub has partnered with Azure to use its infratructure.
As a GitHub user, join the waitlist
and then generate a personal token.
Set the environmental variable $GITHUB_TOKEN
and run the
script with option --github
or --git
.
Check the on-line model list
or list the available models and their original names with chatgpt.sh --github -l
.
chatgpt.sh --github -cc -m Phi-3-small-8k-instruct
See also the GitHub Model Catalog - Getting Started page.
Novita AI offers a range of LLM models at exceptional value, including the highly recommended Llama 3.3 model, which provides the best balance of price and performance!
For an uncensored model, consider sao10k/l3-70b-euryale-v2.1 (creative assistant and role-playing) or cognitivecomputations/dolphin-mixtral-8x22b.
Create an API key as per the
Quick Start Guide
and export your key as $NOVITA_API_KEY
to your environment.
Next, run the script such as chatgpt.sh --novita -cc
.
Check the model list web page and the price of each model.
To list all available models, run chatgpt.sh --novita -l
. Optionally set a model name with option -l
to dump model details.
Some models work with the /completions
endpoint, while others
work with the /chat/completions
endpoint, so the script does not set the endpoint automatically! Check model details and web pages to understand their capabilities, and then either run the script with option -c
(text completions) or options -cc
(chat completions).
As an exercise, instead of setting command-line option --novita
,
set Novita AI integration manually instead:
export OPENAI_API_KEY=novita-api-key
export OPENAI_BASE_URL="https://api.novita.ai/v3/openai"
chatgpt.sh -cc -m meta-llama/llama-3.1-405b-instruct
We are grateful to Novita AI for their support and collaboration. For more information, visit Novita AI.
Visit xAI Grok
to generate an API key (environment $XAI_API_KEY
).
Run the script with option --xai
and also with option -cc
(chat completions.).
Some models also work with native text completions. For that,
set command-line option -c
instead.
This project PKGBUILD is available at the Arch Linux User Repository (AUR) to install the software in Arch Linux and derivative distros.
To install the programme from the AUR, you can use an AUR helper
like yay
or paru
. For example, with yay
:
yay -S chatgpt.sh
Install the Termux
and Termux:API
apps from the F-Droid store.
Give all permissions to Termux:API
in your phone app settings.
We reccommend to also install sox
, ffmpeg
, pulseaudio
, imagemagick
, and vim
(or nano
).
Remember to execute termux-setup-storage
to set up access to the phone storage.
In Termux proper, install the termux-api
and termux-tools
packages (pkg install termux-api termux-tools
).
When recording audio (Whisper, option -w
),
if pulseaudio
is configured correctly,
the script uses sox
, ffmpeg
or other competent software,
otherwise it defaults to termux-microphone-record
Likewise, when playing audio (TTS, option -z
),
depending on pulseaudio
configuration use sox
, mpv
or
fallback to termux wrapper playback (play-audio
is optional).
To set the clipboard, it is required termux-clipboard-set
from the termux-api
package.
Markdown in TTS input may stutter the model speech generation a little.
If python
modules markdown
and bs4
are available, TTS input will
be converted to plain text. As fallback, pandoc
is used if present
(chat mode only).
Under Termux, make sure to have your system updated and installed with
python
, rust
, and rustc-dev
packages for building tiktoken
.
pkg update
pkg upgrade
pkg install python rust rustc-dev
pip install tiktoken
In order to set Termux access to recording the microphone and playing audio
(with sox
and ffmpeg
), follow the instructions below.
A. Set pulseaudio
one time only, execute:
pulseaudio -k
pulseaudio -L "module-sles-source" -D
B. To set a permanent configuration:
- Kill the process with
pulseaudio -k
. - Add
load-module module-sles-source
to one of the files:
~/.config/pulse/default.pa
/data/data/com.termux/files/usr/etc/pulse/default.pa
- Restart the server with
pulseaudio -D
.
C. To create a new user ~/.config/pulse/default.pa
, you may start with the following template:
#!/usr/bin/pulseaudio -nF
.include /data/data/com.termux/files/usr/etc/pulse/default.pa
load-module module-sles-source
To access your Termux files using Android's file manager, install a decent file manager such as FX File Explorer
from a Play Store and configure it, or run the following command in your Termux terminal:
am start -a android.intent.action.VIEW -d "content://com.android.externalstorage.documents/root/primary"
Source: https://www.reddit.com/r/termux/comments/182g7np/where_do_i_find_my_things_that_i_downloaded/
-
Implement nice features from
OpenAI API version 1
. -
Provide the closest API defaults.
-
Let the user customise defaults (as homework).
-
Premiรจre of
chatgpt.sh version 1.0
should occur at the time when OpenAI launches its next major API version update.
- We shall decrease development frequency in 2025, hopefully. LLM models in general are not really worth developer efforts sometimes, it is frustating!
- The warper is deemed finished in the sense any further updates must not change the user interface.
- We plan to gradually wind down development of new features in the near future. The project will enter a maintenance phase from 2025 onwards, focusing primarily on bug fixes and stability.
-
OpenAI API version 1 is the focus of the present project implementation. Not all features of the API will be covered.
-
This project doesn't support "Function Calling" or "Structured Outputs".
-
Probably, we will not support "Real-Time" chatting, or video modality.
-
Bash shell truncates input on
\000
(null). -
Bash "read command" may not correctly display input buffers larger than the TTY screen size during editing. However, input buffers remain unaffected. Use the text editor interface for big prompt editing.
-
Garbage in, garbage out. An idiot savant.
-
The script logic resembles a bowl of spaghetti code after a cat fight.
-
See BUGS AND LIMITS section in the man page.
Please leave bug reports at the GitHub issues page.
Read the online man page here.
Alternatively, a help page snippet can be printed with chatgpt.sh -h
.
Many Thanks to everyone who contributed to this project.
Everyone is welcome to submit issues, PRs, and new ideas!
The following projects are worth remarking. They were studied during development of this script and used as referencial code sources.
- TheR1D's shell_gpt
- xenodium's chatgpt-shell
- llm-workflow-engine
- 0xacx's chatGPT-shell-cli
- mudler's LocalAI
- Ollama
- Google Gemini
- Groq
- Antropic AI
- Novita AI
- xAI
- f's awesome-chatgpt-prompts
- PlexPt's awesome-chatgpt-prompts-zh
https://gitlab.com/fenixdragao/shellchatgpt
Mirror
https://github.com/mountaineerbr/shellChatGPT
For Tasks:
Click tags to check more tools for each tasksFor Jobs:
Alternative AI tools for shellChatGPT
Similar Open Source Tools
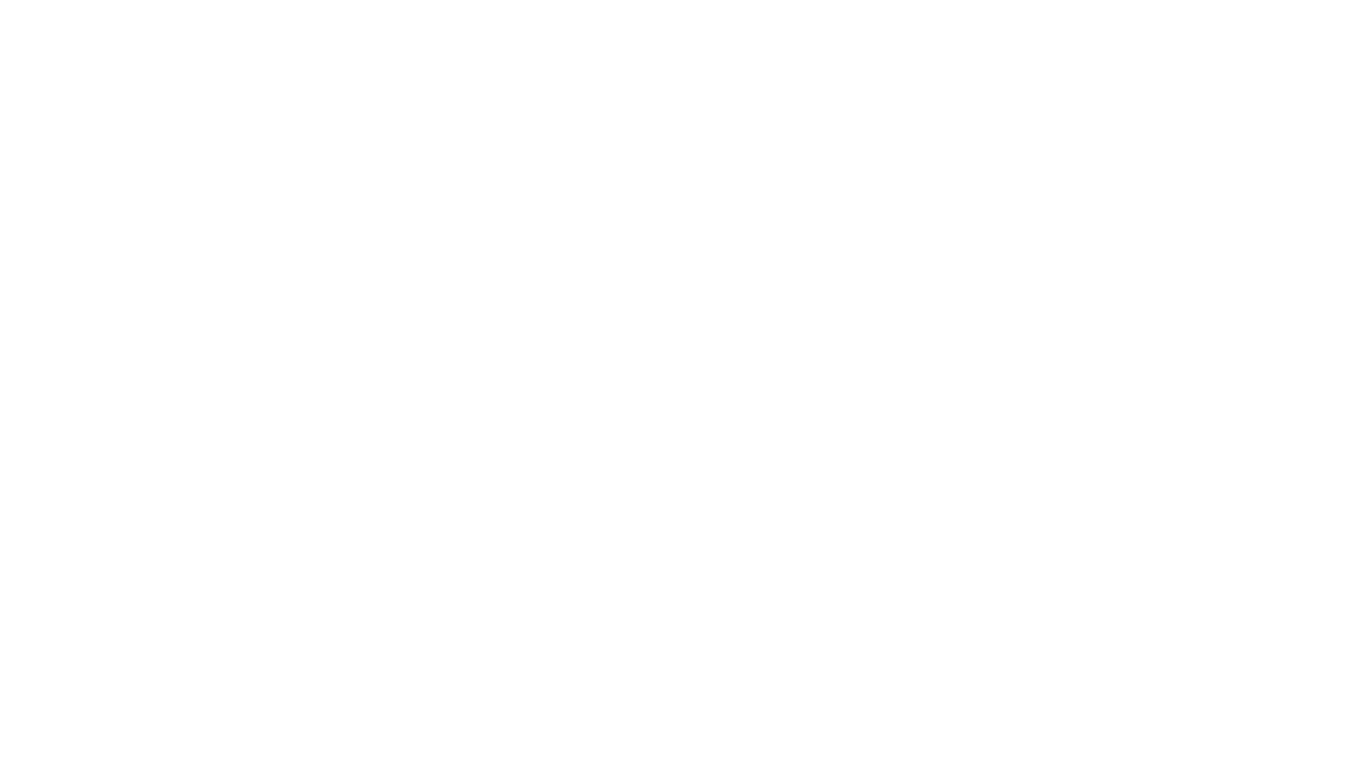
shellChatGPT
ShellChatGPT is a shell wrapper for OpenAI's ChatGPT, DALL-E, Whisper, and TTS, featuring integration with LocalAI, Ollama, Gemini, Mistral, Groq, and GitHub Models. It provides text and chat completions, vision, reasoning, and audio models, voice-in and voice-out chatting mode, text editor interface, markdown rendering support, session management, instruction prompt manager, integration with various service providers, command line completion, file picker dialogs, color scheme personalization, stdin and text file input support, and compatibility with Linux, FreeBSD, MacOS, and Termux for a responsive experience.
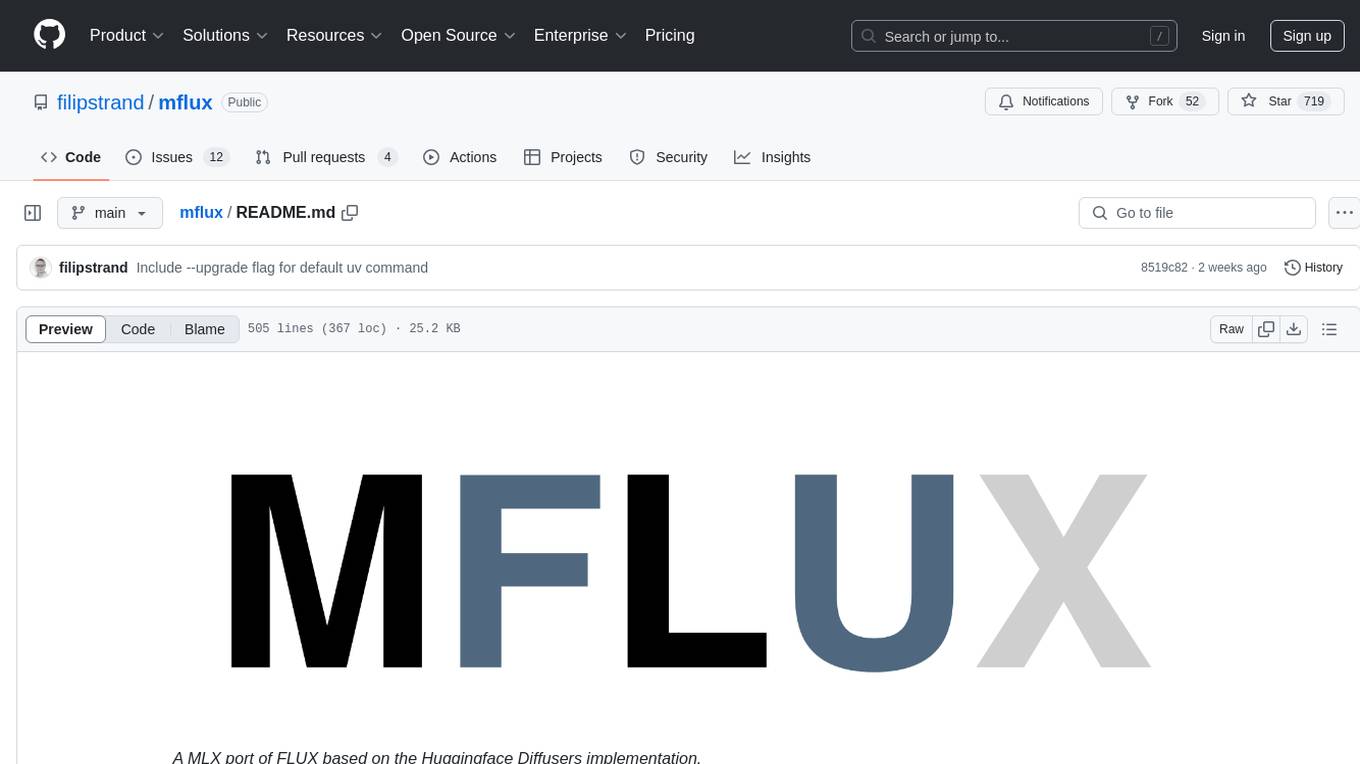
mflux
MFLUX is a line-by-line port of the FLUX implementation in the Huggingface Diffusers library to Apple MLX. It aims to run powerful FLUX models from Black Forest Labs locally on Mac machines. The codebase is minimal and explicit, prioritizing readability over generality and performance. Models are implemented from scratch in MLX, with tokenizers from the Huggingface Transformers library. Dependencies include Numpy and Pillow for image post-processing. Installation can be done using `uv tool` or classic virtual environment setup. Command-line arguments allow for image generation with specified models, prompts, and optional parameters. Quantization options for speed and memory reduction are available. LoRA adapters can be loaded for fine-tuning image generation. Controlnet support provides more control over image generation with reference images. Current limitations include generating images one by one, lack of support for negative prompts, and some LoRA adapters not working.
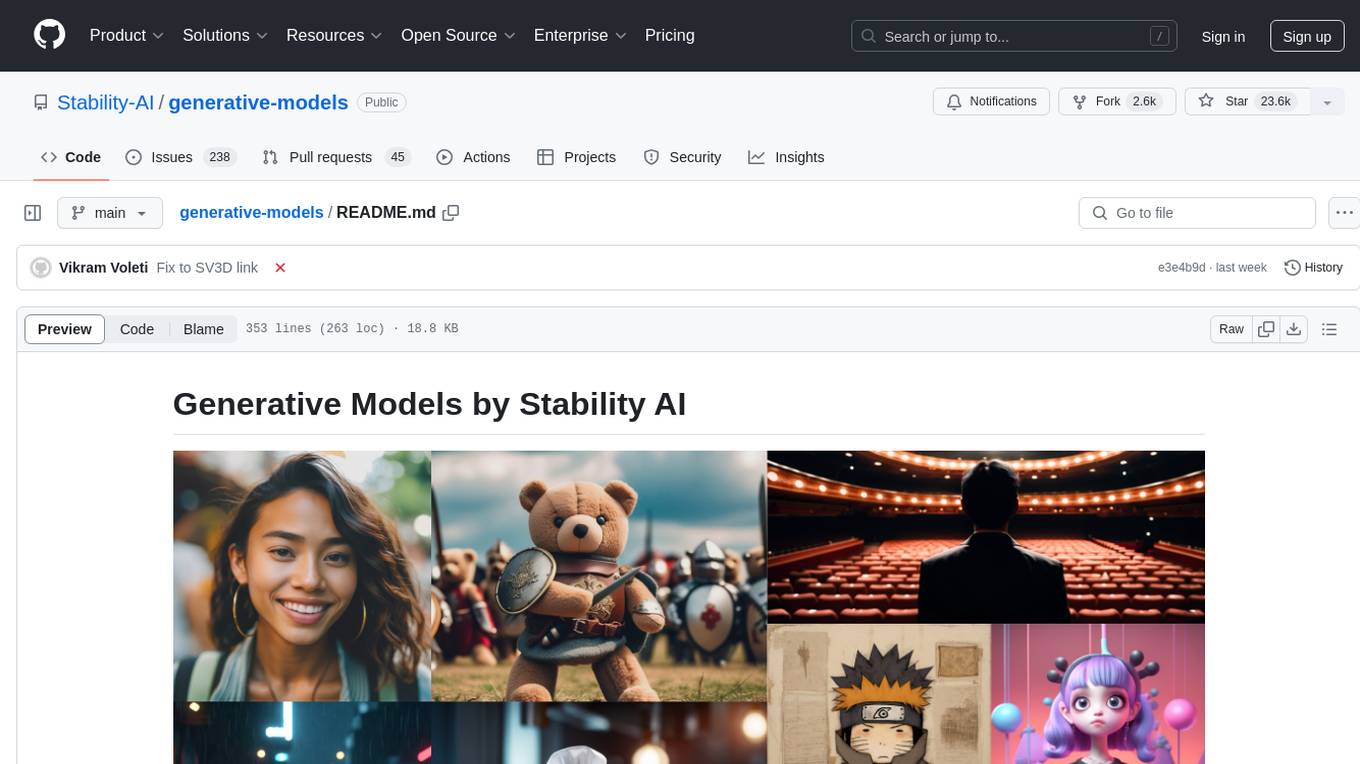
generative-models
Generative Models by Stability AI is a repository that provides various generative models for research purposes. It includes models like Stable Video 4D (SV4D) for video synthesis, Stable Video 3D (SV3D) for multi-view synthesis, SDXL-Turbo for text-to-image generation, and more. The repository focuses on modularity and implements a config-driven approach for building and combining submodules. It supports training with PyTorch Lightning and offers inference demos for different models. Users can access pre-trained models like SDXL-base-1.0 and SDXL-refiner-1.0 under a CreativeML Open RAIL++-M license. The codebase also includes tools for invisible watermark detection in generated images.
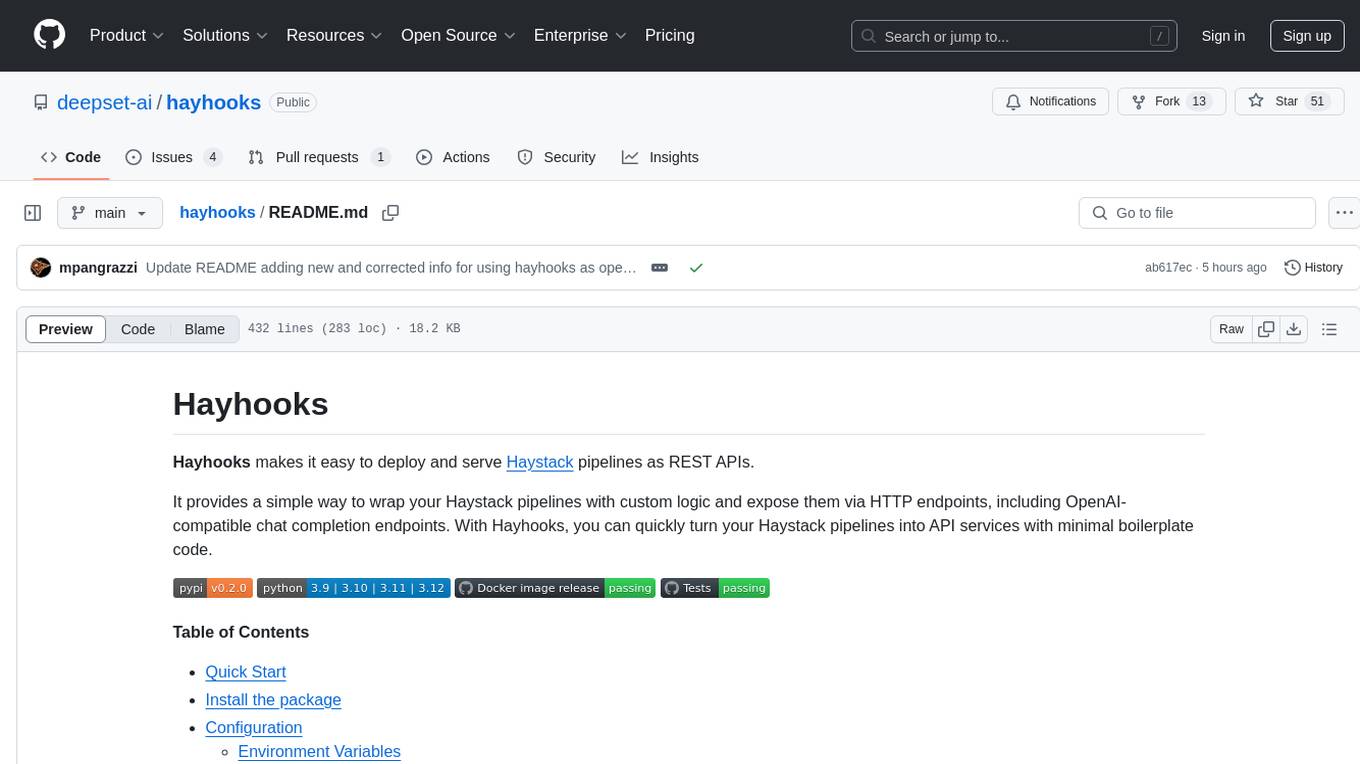
hayhooks
Hayhooks is a tool that simplifies the deployment and serving of Haystack pipelines as REST APIs. It allows users to wrap their pipelines with custom logic and expose them via HTTP endpoints, including OpenAI-compatible chat completion endpoints. With Hayhooks, users can easily convert their Haystack pipelines into API services with minimal boilerplate code.
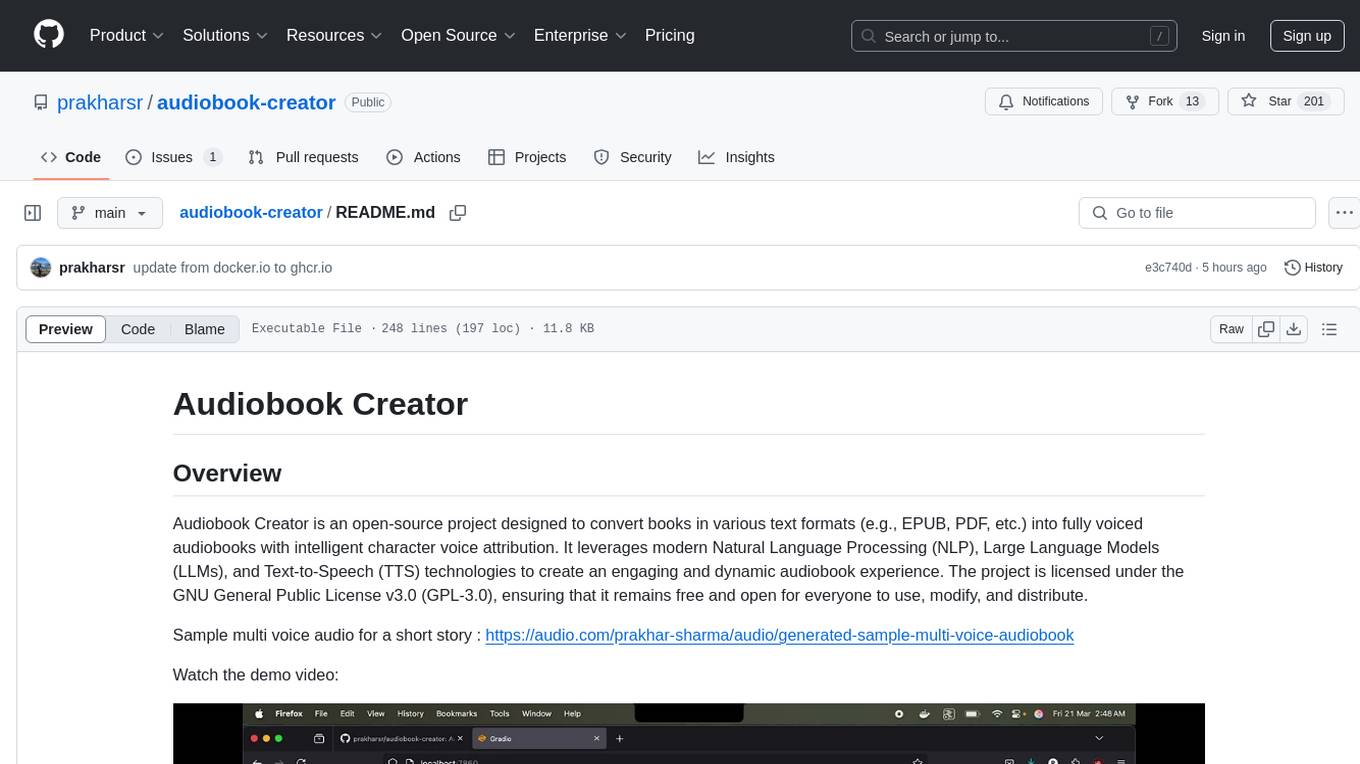
audiobook-creator
Audiobook Creator is an open-source tool that converts books in various text formats into fully voiced audiobooks with intelligent character voice attribution. It utilizes NLP, LLMs, and TTS technologies to provide an engaging audiobook experience. The project includes components for text cleaning and formatting, character identification, and audiobook generation. Key features include a Gradio UI app, M4B audiobook creation, multi-format support, Docker compatibility, customizable narration, progress tracking, and open-source licensing.
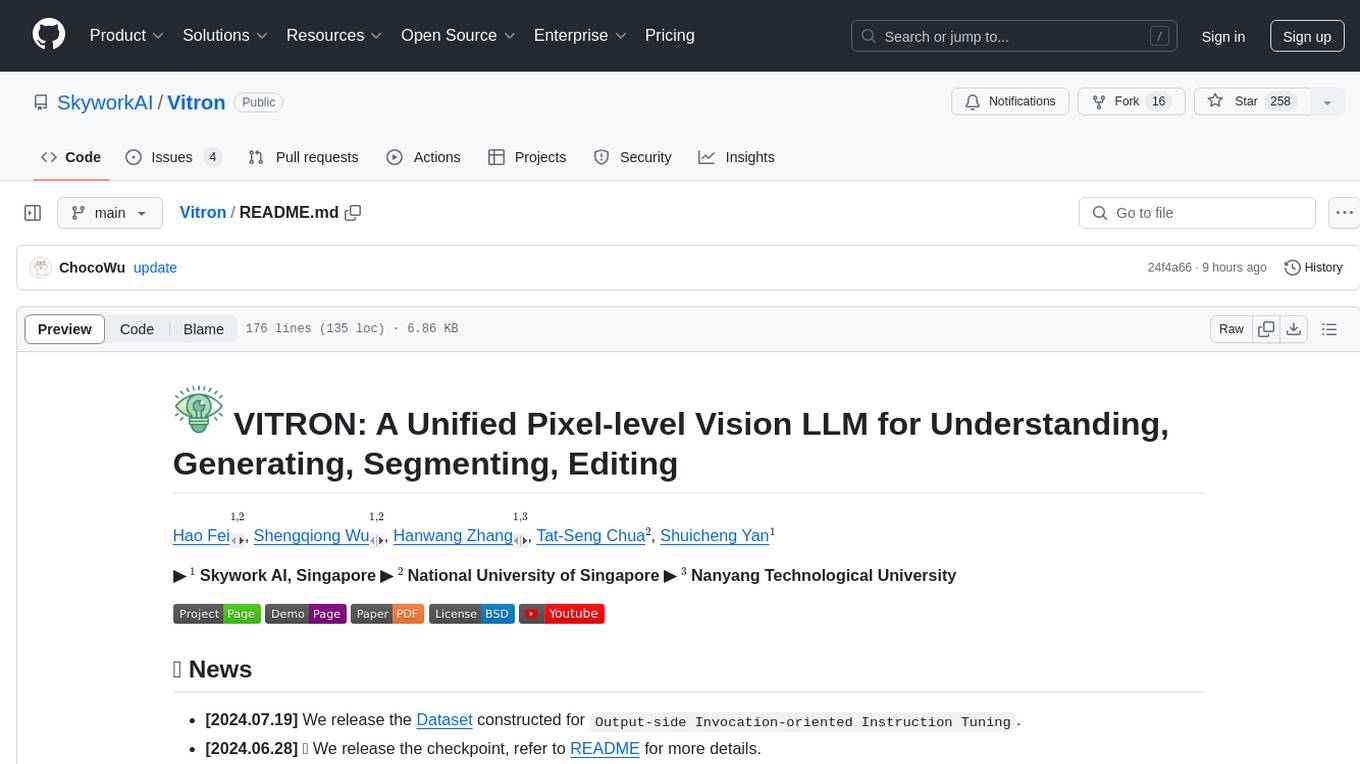
Vitron
Vitron is a unified pixel-level vision LLM designed for comprehensive understanding, generating, segmenting, and editing static images and dynamic videos. It addresses challenges in existing vision LLMs such as superficial instance-level understanding, lack of unified support for images and videos, and insufficient coverage across various vision tasks. The tool requires Python >= 3.8, Pytorch == 2.1.0, and CUDA Version >= 11.8 for installation. Users can deploy Gradio demo locally and fine-tune their models for specific tasks.
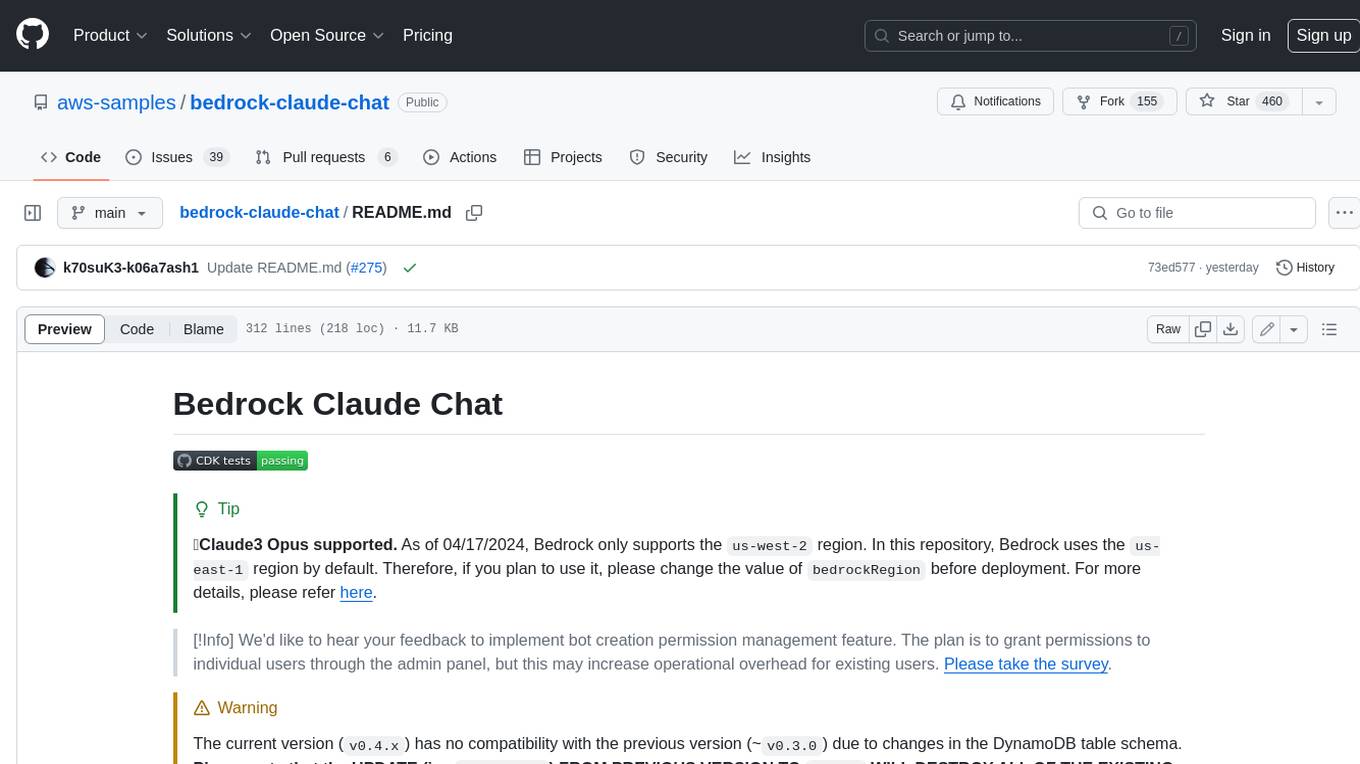
bedrock-claude-chat
This repository is a sample chatbot using the Anthropic company's LLM Claude, one of the foundational models provided by Amazon Bedrock for generative AI. It allows users to have basic conversations with the chatbot, personalize it with their own instructions and external knowledge, and analyze usage for each user/bot on the administrator dashboard. The chatbot supports various languages, including English, Japanese, Korean, Chinese, French, German, and Spanish. Deployment is straightforward and can be done via the command line or by using AWS CDK. The architecture is built on AWS managed services, eliminating the need for infrastructure management and ensuring scalability, reliability, and security.
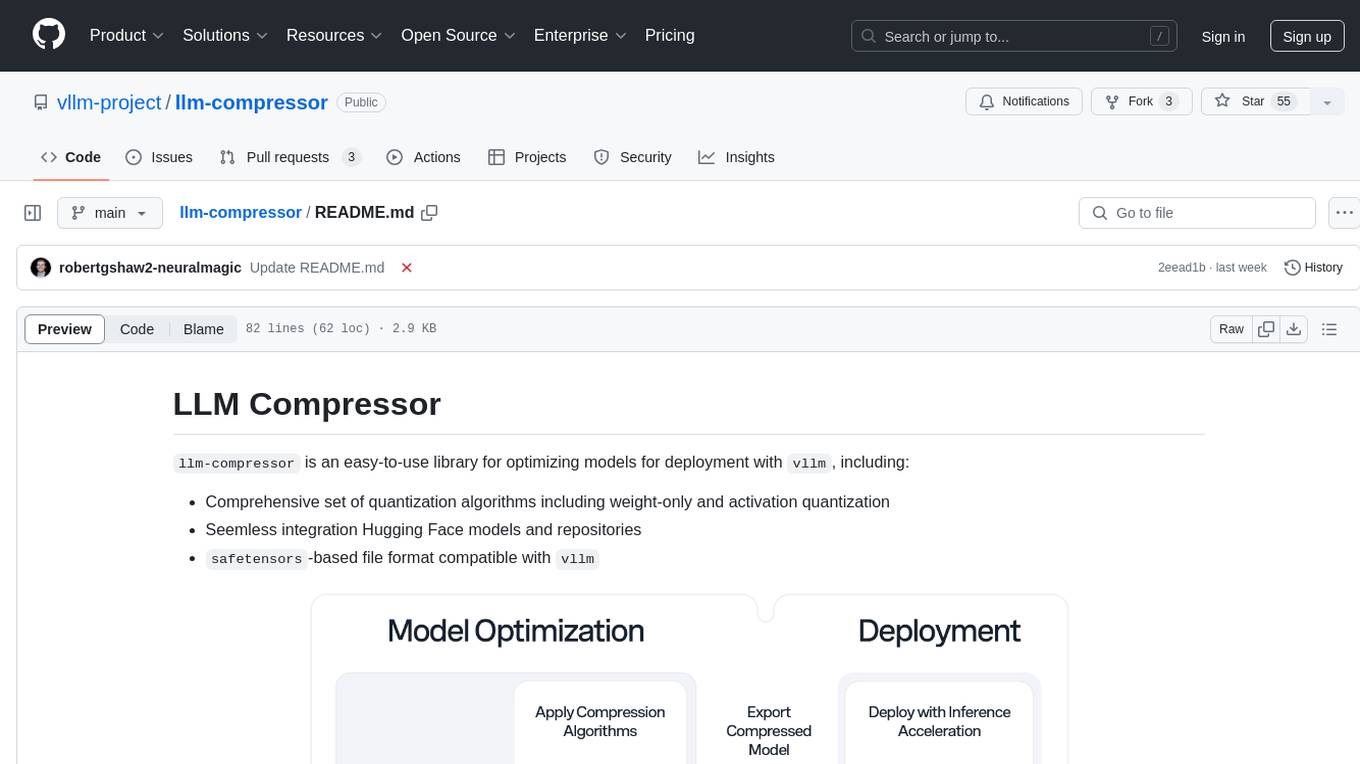
llm-compressor
llm-compressor is an easy-to-use library for optimizing models for deployment with vllm. It provides a comprehensive set of quantization algorithms, seamless integration with Hugging Face models and repositories, and supports mixed precision, activation quantization, and sparsity. Supported algorithms include PTQ, GPTQ, SmoothQuant, and SparseGPT. Installation can be done via git clone and local pip install. Compression can be easily applied by selecting an algorithm and calling the oneshot API. The library also offers end-to-end examples for model compression. Contributions to the code, examples, integrations, and documentation are appreciated.
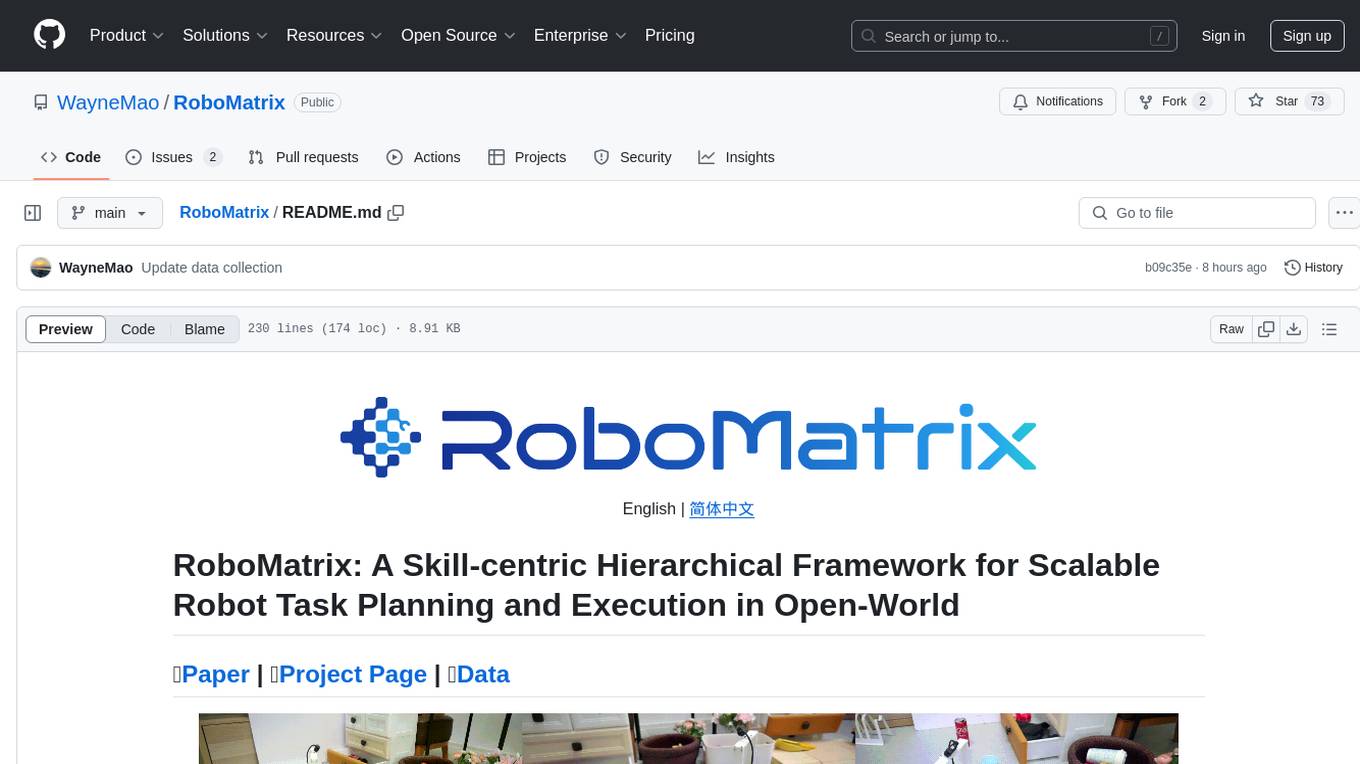
RoboMatrix
RoboMatrix is a skill-centric hierarchical framework for scalable robot task planning and execution in an open-world environment. It provides a structured approach to robot task execution using a combination of hardware components, environment configuration, installation procedures, and data collection methods. The framework is developed using the ROS2 framework on Ubuntu and supports robots from DJI's RoboMaster series. Users can follow the provided installation guidance to set up RoboMatrix and utilize it for various tasks such as data collection, task execution, and dataset construction. The framework also includes a supervised fine-tuning dataset and aims to optimize communication and release additional components in the future.
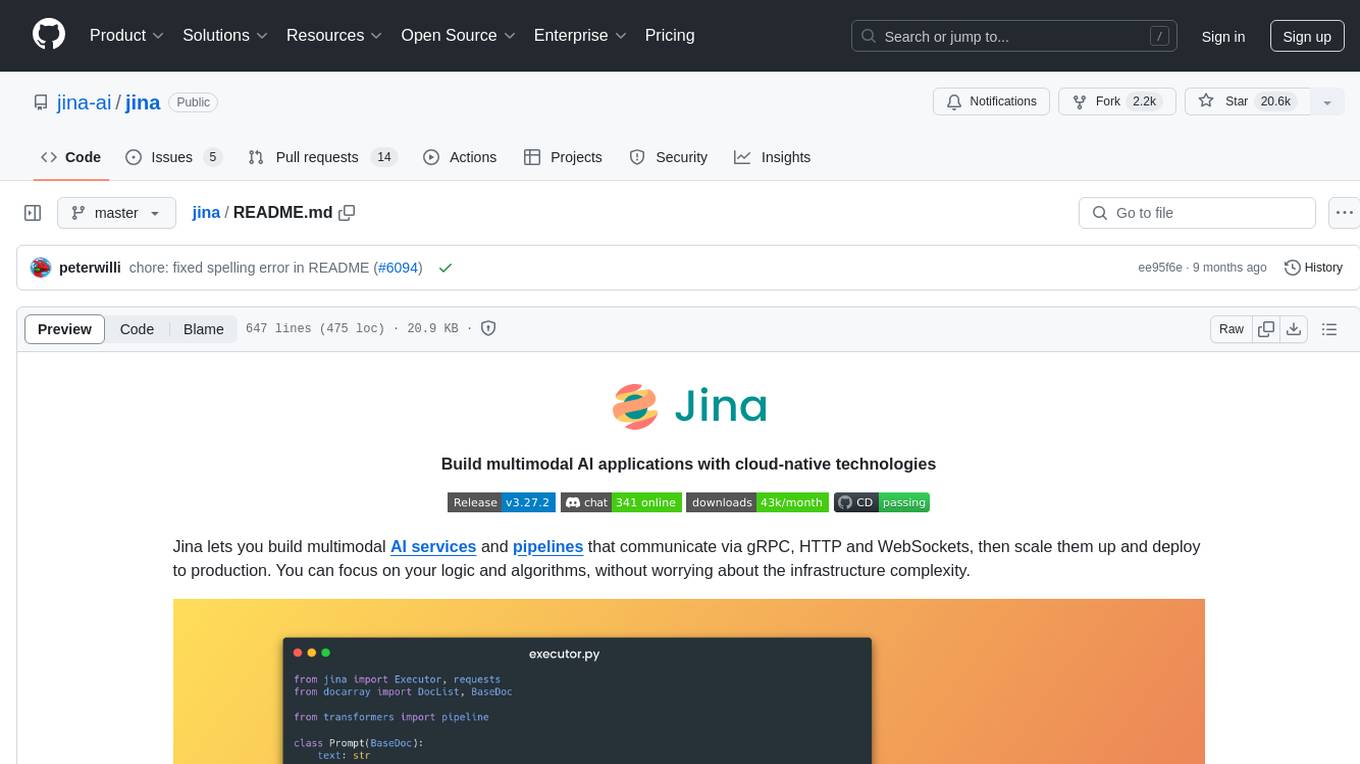
jina
Jina is a tool that allows users to build multimodal AI services and pipelines using cloud-native technologies. It provides a Pythonic experience for serving ML models and transitioning from local deployment to advanced orchestration frameworks like Docker-Compose, Kubernetes, or Jina AI Cloud. Users can build and serve models for any data type and deep learning framework, design high-performance services with easy scaling, serve LLM models while streaming their output, integrate with Docker containers via Executor Hub, and host on CPU/GPU using Jina AI Cloud. Jina also offers advanced orchestration and scaling capabilities, a smooth transition to the cloud, and easy scalability and concurrency features for applications. Users can deploy to their own cloud or system with Kubernetes and Docker Compose integration, and even deploy to JCloud for autoscaling and monitoring.
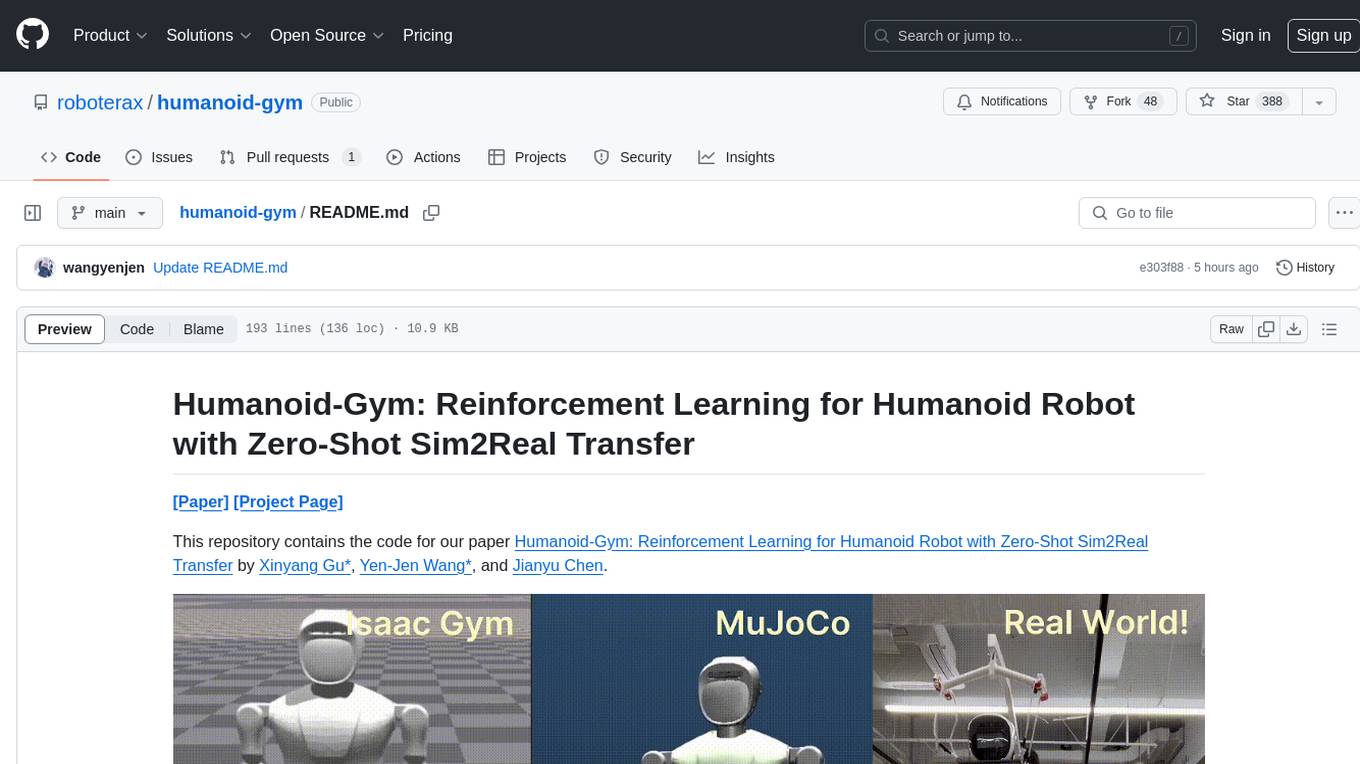
humanoid-gym
Humanoid-Gym is a reinforcement learning framework designed for training locomotion skills for humanoid robots, focusing on zero-shot transfer from simulation to real-world environments. It integrates a sim-to-sim framework from Isaac Gym to Mujoco for verifying trained policies in different physical simulations. The codebase is verified with RobotEra's XBot-S and XBot-L humanoid robots. It offers comprehensive training guidelines, step-by-step configuration instructions, and execution scripts for easy deployment. The sim2sim support allows transferring trained policies to accurate simulated environments. The upcoming features include Denoising World Model Learning and Dexterous Hand Manipulation. Installation and usage guides are provided along with examples for training PPO policies and sim-to-sim transformations. The code structure includes environment and configuration files, with instructions on adding new environments. Troubleshooting tips are provided for common issues, along with a citation and acknowledgment section.
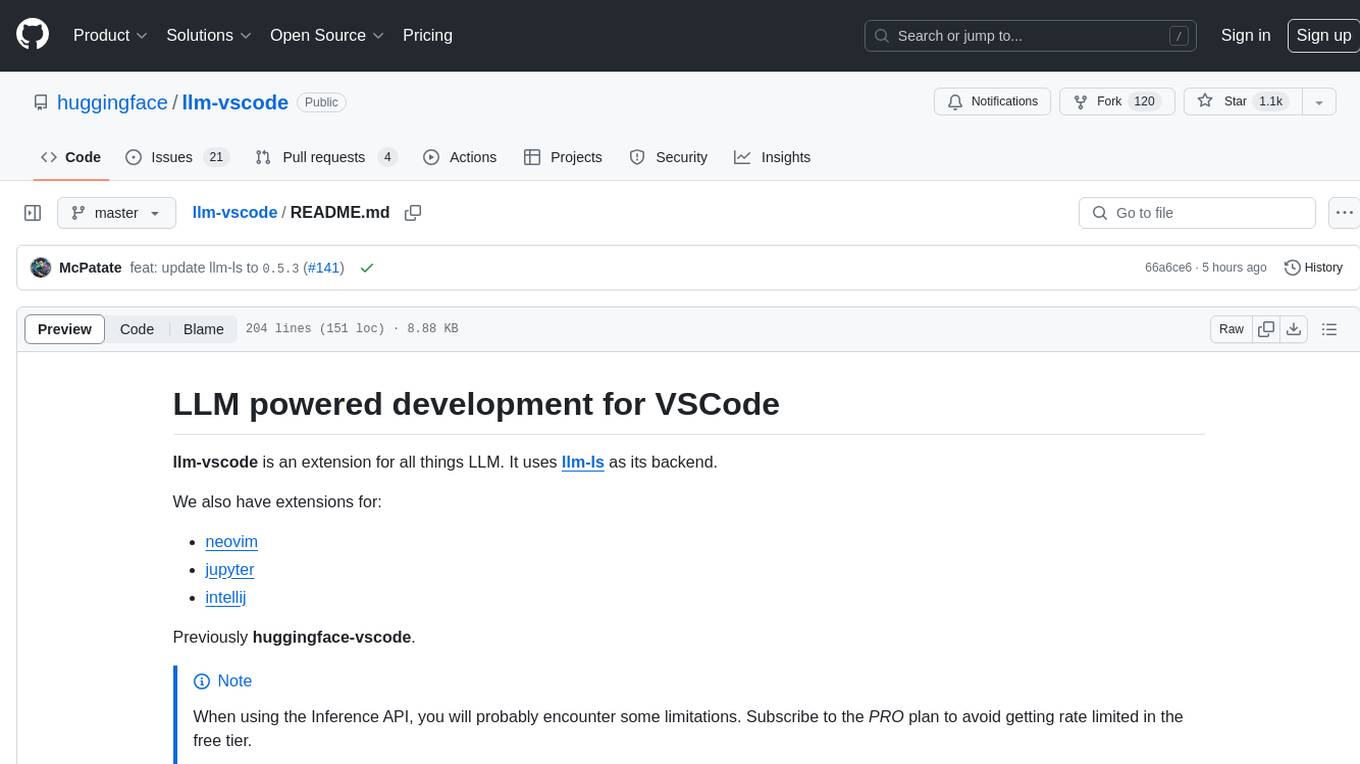
llm-vscode
llm-vscode is an extension designed for all things LLM, utilizing llm-ls as its backend. It offers features such as code completion with 'ghost-text' suggestions, the ability to choose models for code generation via HTTP requests, ensuring prompt size fits within the context window, and code attribution checks. Users can configure the backend, suggestion behavior, keybindings, llm-ls settings, and tokenization options. Additionally, the extension supports testing models like Code Llama 13B, Phind/Phind-CodeLlama-34B-v2, and WizardLM/WizardCoder-Python-34B-V1.0. Development involves cloning llm-ls, building it, and setting up the llm-vscode extension for use.
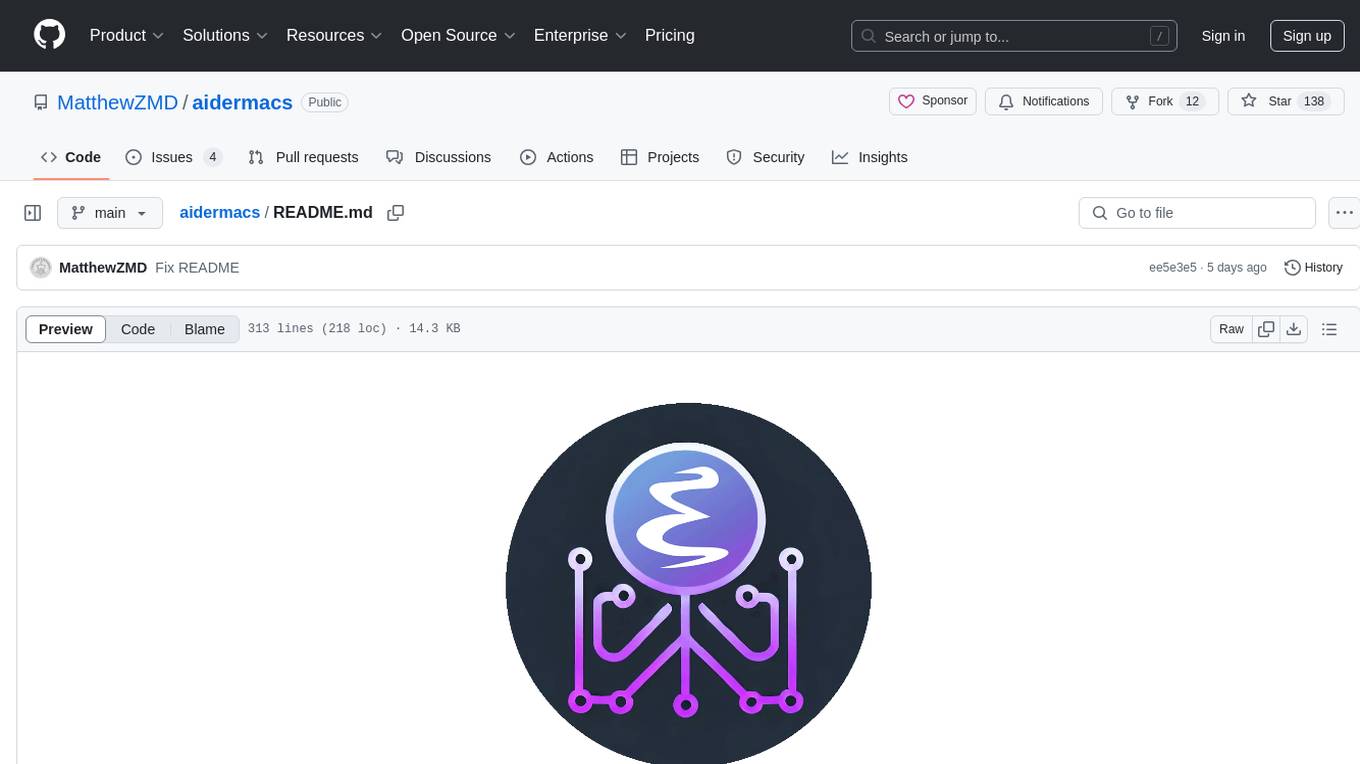
aidermacs
Aidermacs is an AI pair programming tool for Emacs that integrates Aider, a powerful open-source AI pair programming tool. It provides top performance on the SWE Bench, support for multi-file edits, real-time file synchronization, and broad language support. Aidermacs delivers an Emacs-centric experience with features like intelligent model selection, flexible terminal backend support, smarter syntax highlighting, enhanced file management, and streamlined transient menus. It thrives on community involvement, encouraging contributions, issue reporting, idea sharing, and documentation improvement.
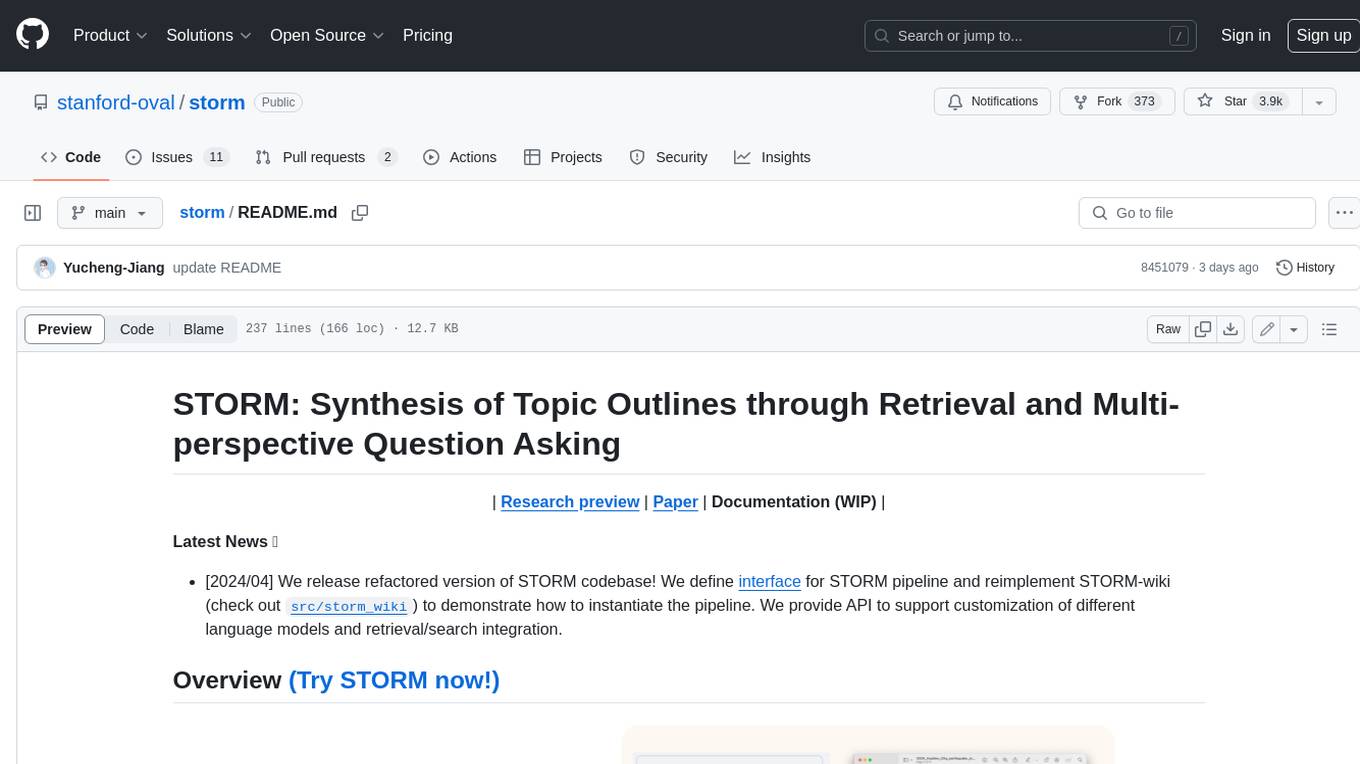
storm
STORM is a LLM system that writes Wikipedia-like articles from scratch based on Internet search. While the system cannot produce publication-ready articles that often require a significant number of edits, experienced Wikipedia editors have found it helpful in their pre-writing stage. **Try out our [live research preview](https://storm.genie.stanford.edu/) to see how STORM can help your knowledge exploration journey and please provide feedback to help us improve the system ๐!**
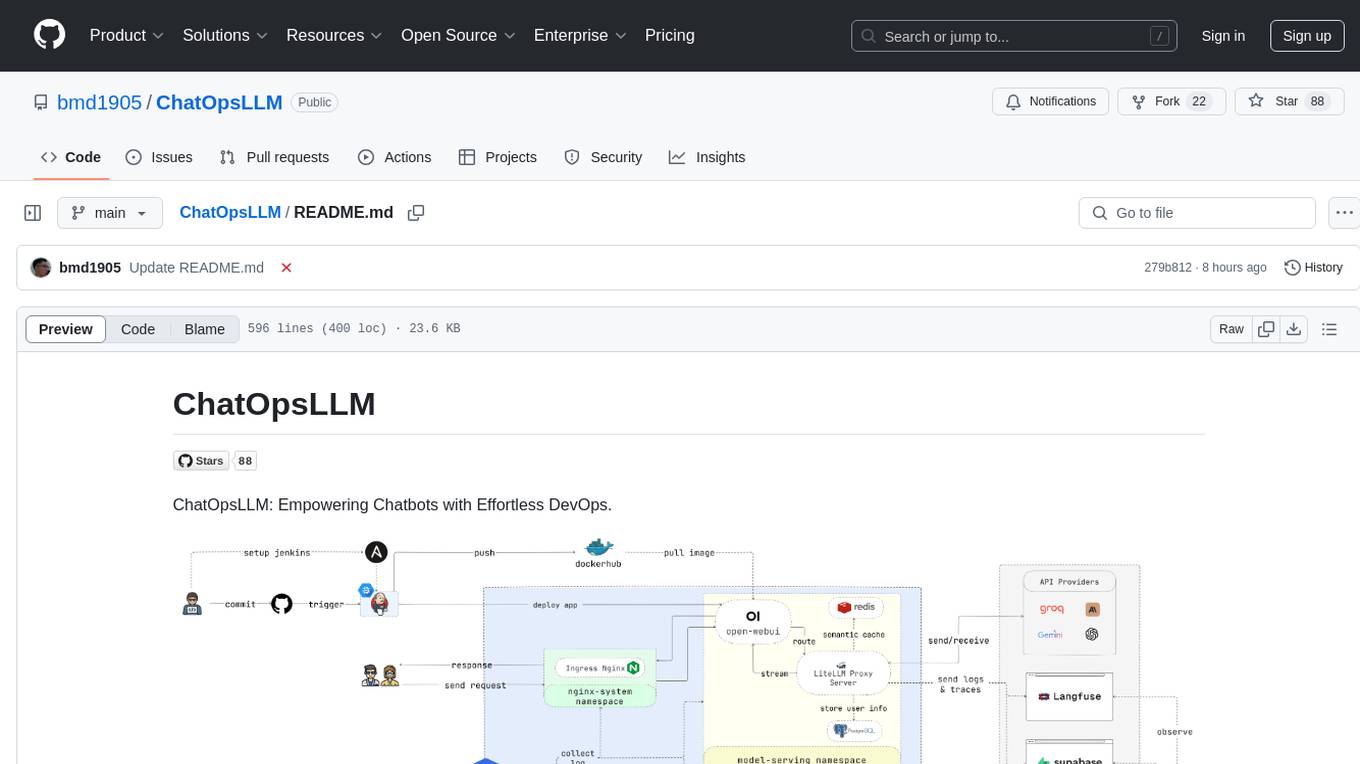
ChatOpsLLM
ChatOpsLLM is a project designed to empower chatbots with effortless DevOps capabilities. It provides an intuitive interface and streamlined workflows for managing and scaling language models. The project incorporates robust MLOps practices, including CI/CD pipelines with Jenkins and Ansible, monitoring with Prometheus and Grafana, and centralized logging with the ELK stack. Developers can find detailed documentation and instructions on the project's website.
For similar tasks
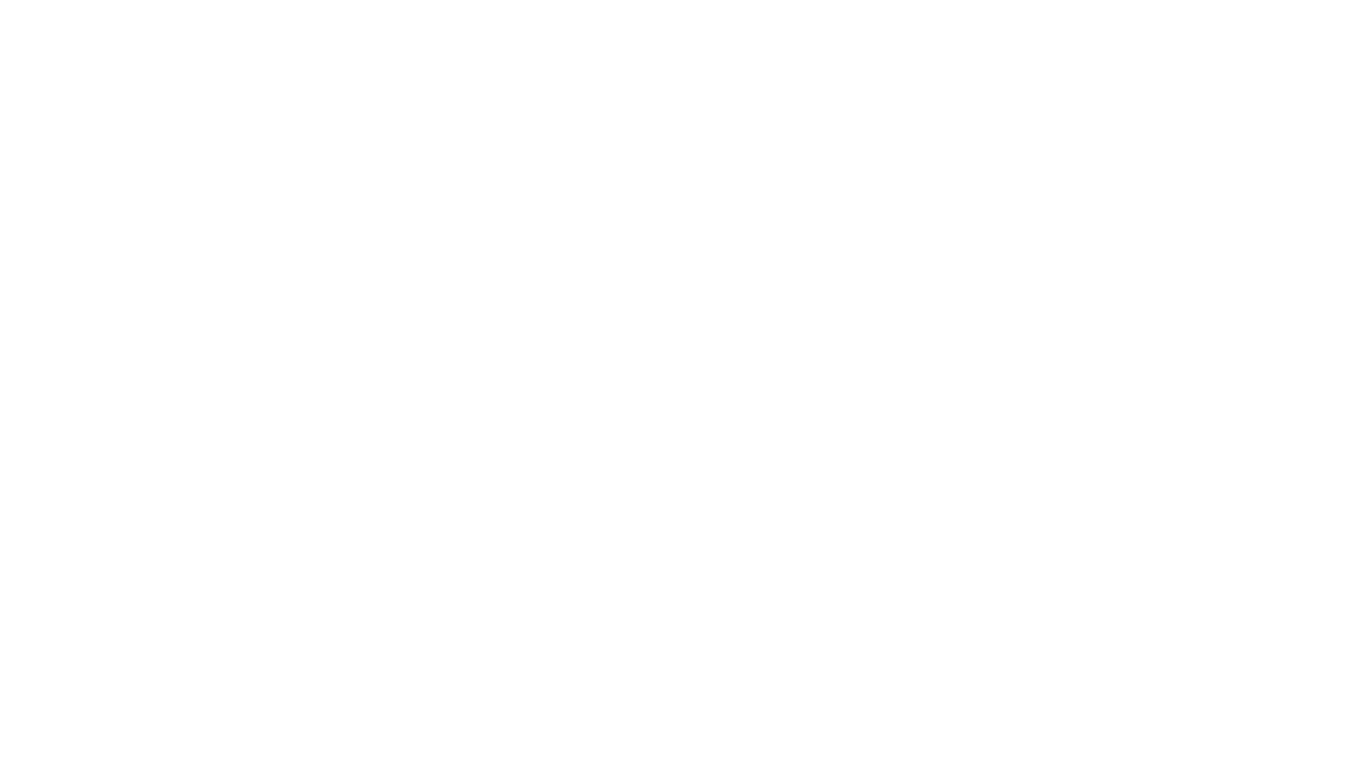
shellChatGPT
ShellChatGPT is a shell wrapper for OpenAI's ChatGPT, DALL-E, Whisper, and TTS, featuring integration with LocalAI, Ollama, Gemini, Mistral, Groq, and GitHub Models. It provides text and chat completions, vision, reasoning, and audio models, voice-in and voice-out chatting mode, text editor interface, markdown rendering support, session management, instruction prompt manager, integration with various service providers, command line completion, file picker dialogs, color scheme personalization, stdin and text file input support, and compatibility with Linux, FreeBSD, MacOS, and Termux for a responsive experience.
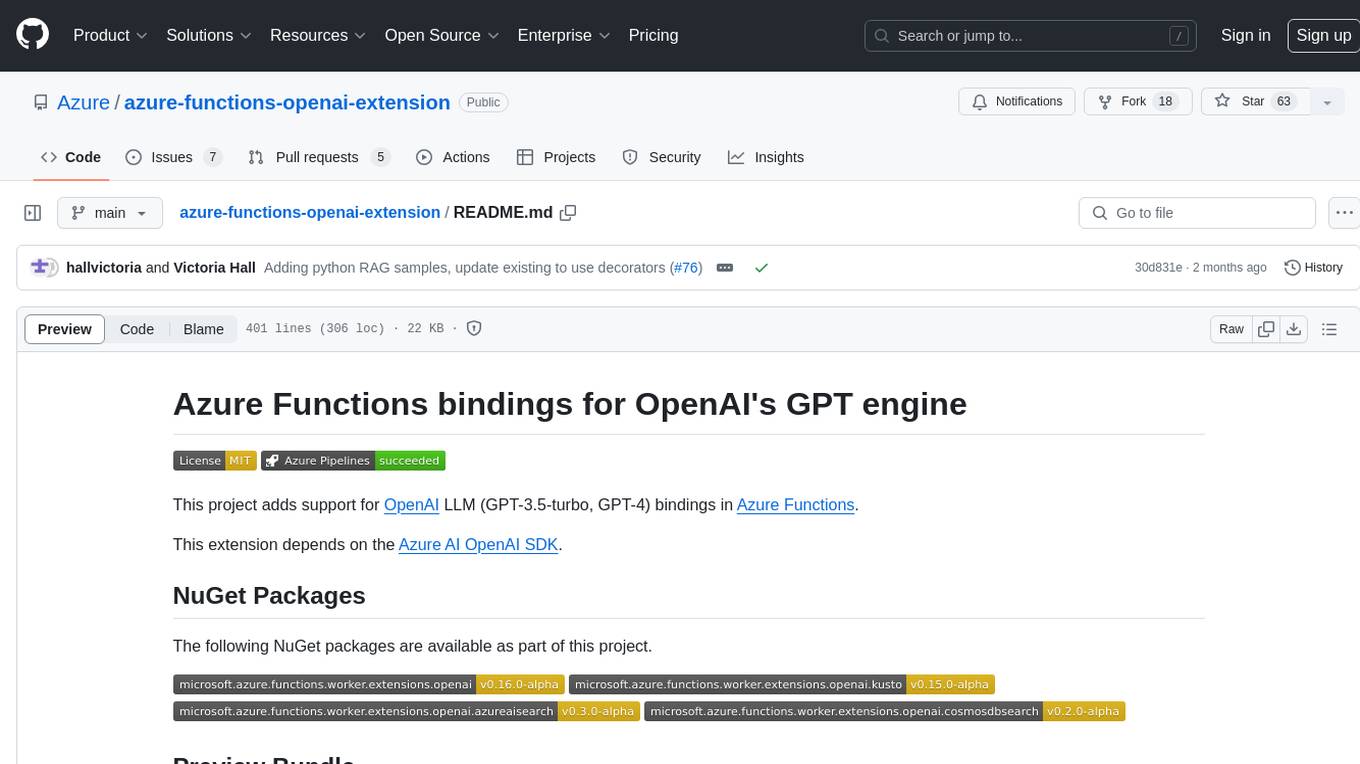
azure-functions-openai-extension
Azure Functions OpenAI Extension is a project that adds support for OpenAI LLM (GPT-3.5-turbo, GPT-4) bindings in Azure Functions. It provides NuGet packages for various functionalities like text completions, chat completions, assistants, embeddings generators, and semantic search. The project requires .NET 6 SDK or greater, Azure Functions Core Tools v4.x, and specific settings in Azure Function or local settings for development. It offers features like text completions, chat completion, assistants with custom skills, embeddings generators for text relatedness, and semantic search using vector databases. The project also includes examples in C# and Python for different functionalities.
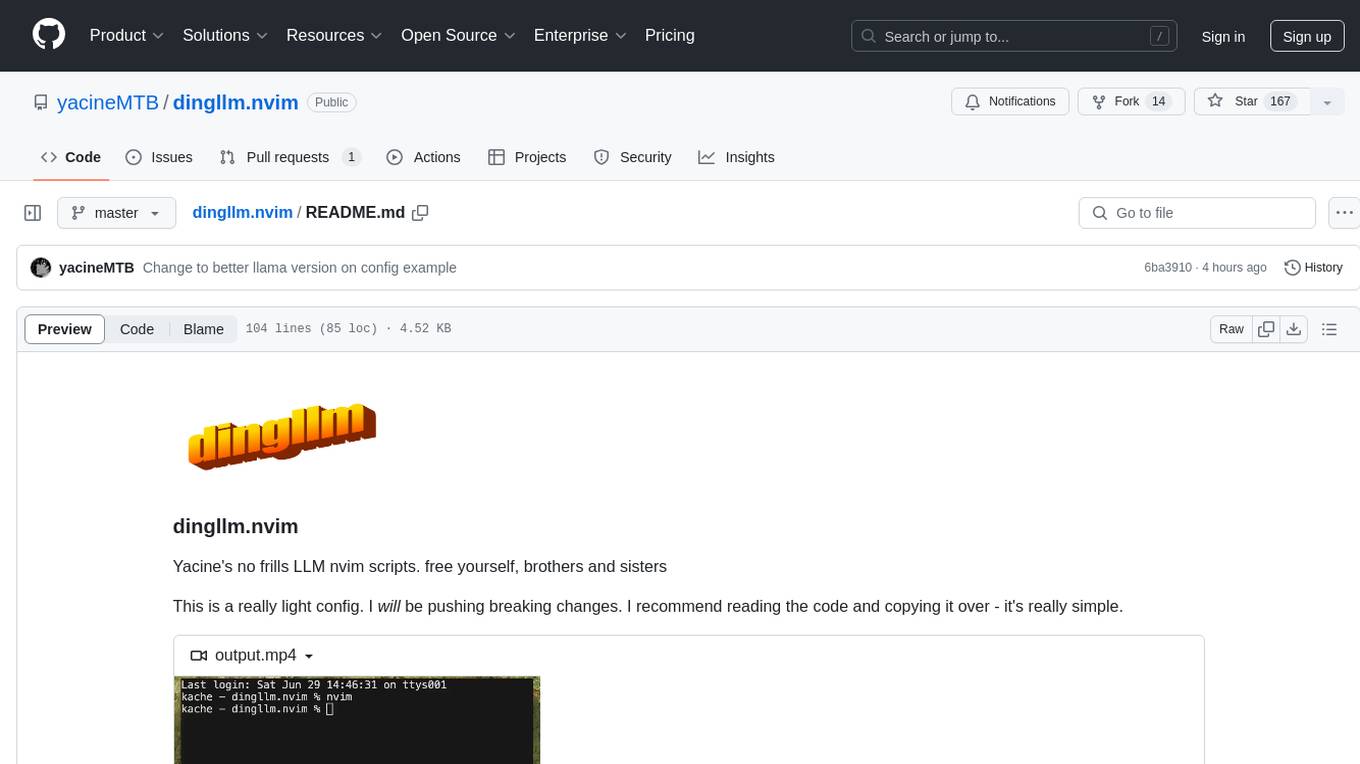
dingllm.nvim
dingllm.nvim is a lightweight configuration for Neovim that provides scripts for invoking various AI models for text generation. It offers functionalities to interact with APIs from OpenAI, Groq, and Anthropic for generating text completions. The configuration is designed to be simple and easy to understand, allowing users to quickly set up and use the provided AI models for text generation tasks.
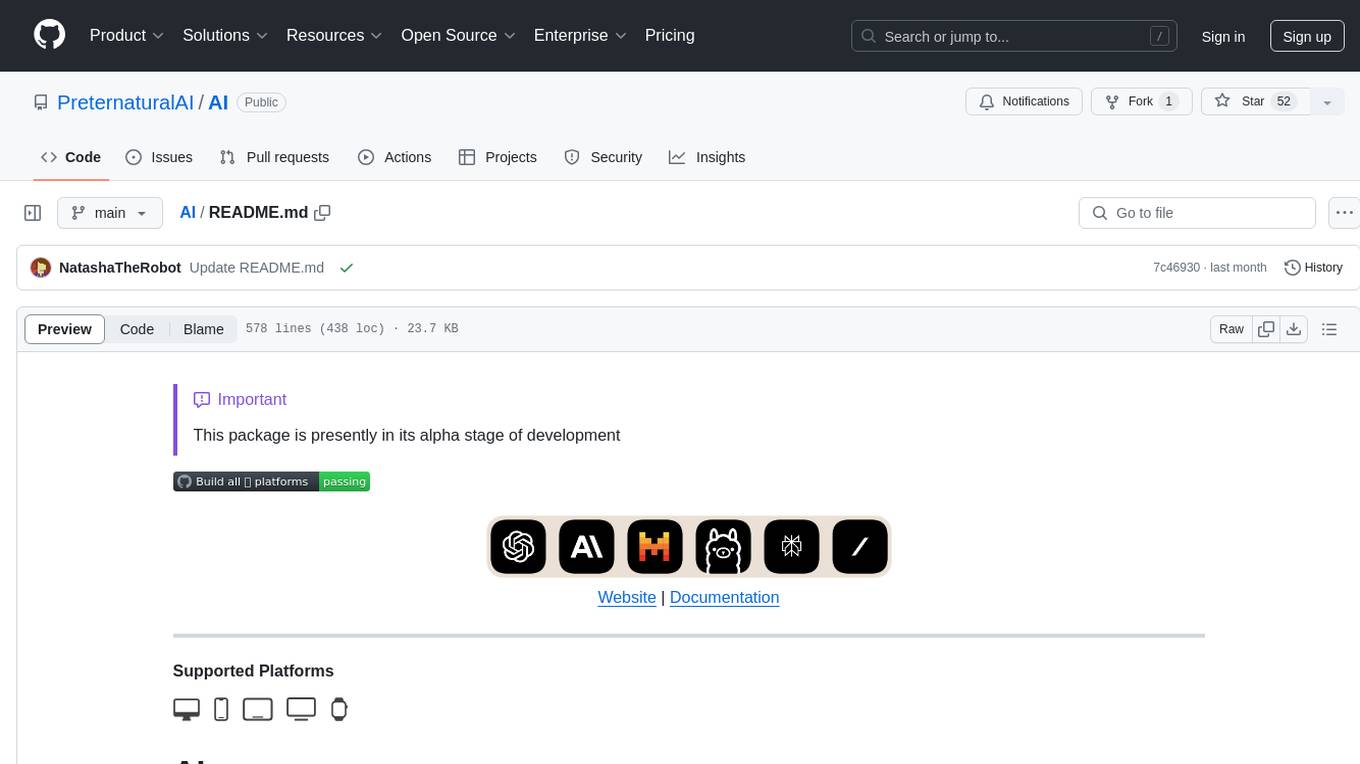
AI
AI is an open-source Swift framework for interfacing with generative AI. It provides functionalities for text completions, image-to-text vision, function calling, DALLE-3 image generation, audio transcription and generation, and text embeddings. The framework supports multiple AI models from providers like OpenAI, Anthropic, Mistral, Groq, and ElevenLabs. Users can easily integrate AI capabilities into their Swift projects using AI framework.
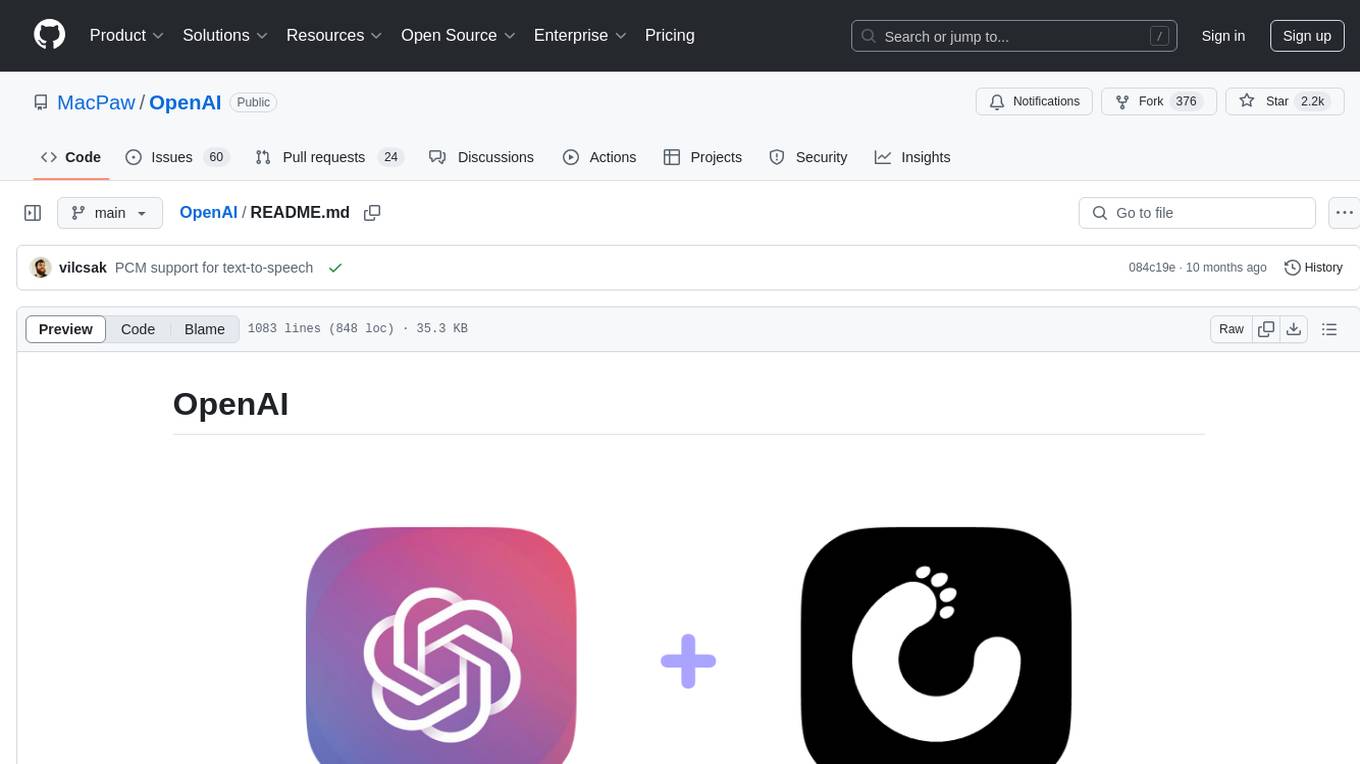
OpenAI
OpenAI is a Swift community-maintained implementation over OpenAI public API. It is a non-profit artificial intelligence research organization founded in San Francisco, California in 2015. OpenAI's mission is to ensure safe and responsible use of AI for civic good, economic growth, and other public benefits. The repository provides functionalities for text completions, chats, image generation, audio processing, edits, embeddings, models, moderations, utilities, and Combine extensions.
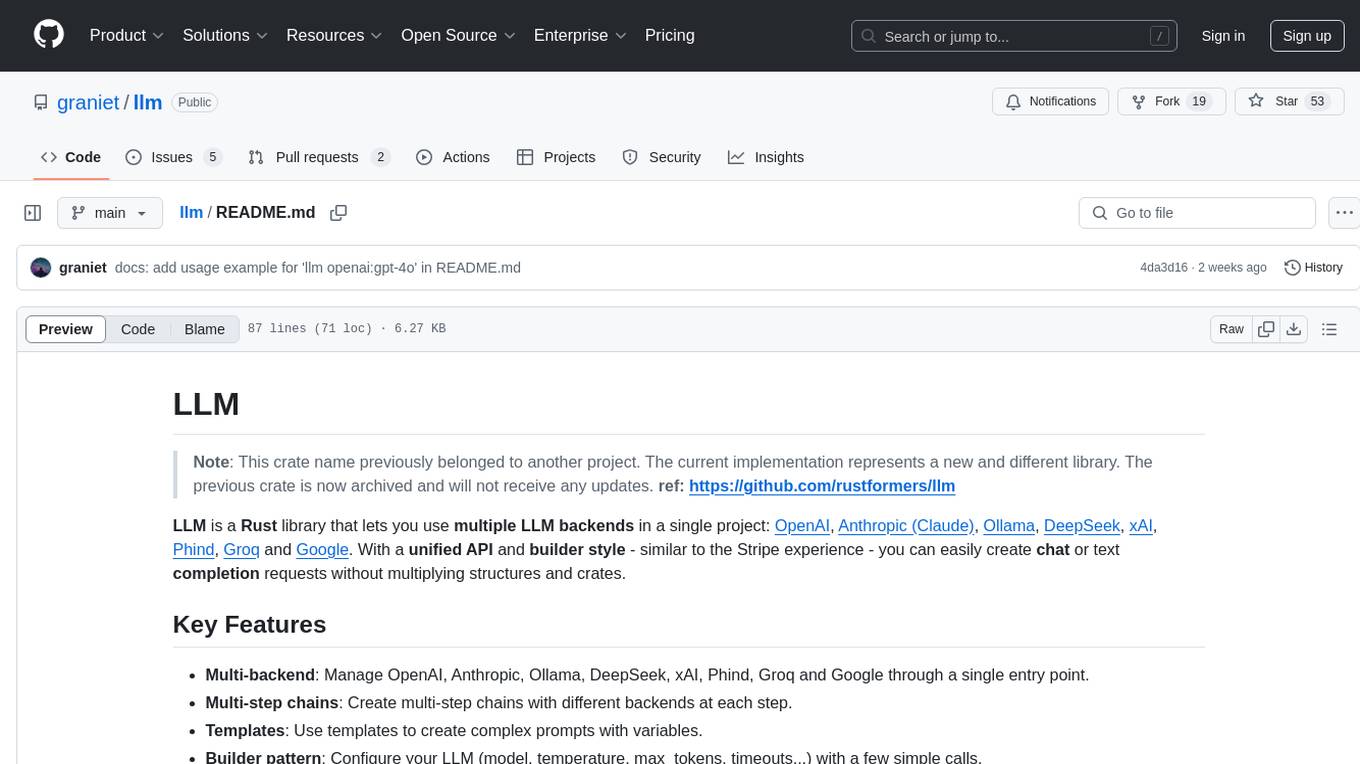
llm
LLM is a Rust library that allows users to utilize multiple LLM backends (OpenAI, Anthropic, Ollama, DeepSeek, xAI, Phind, Groq, Google) in a single project. It provides a unified API and builder style for creating chat or text completion requests without the need for multiple structures and crates. Key features include multi-backend management, multi-step chains, templates for complex prompts, builder pattern for easy configuration, extensibility, validation, evaluation, parallel evaluation, function calling, REST API support, vision integration, and reasoning capabilities.
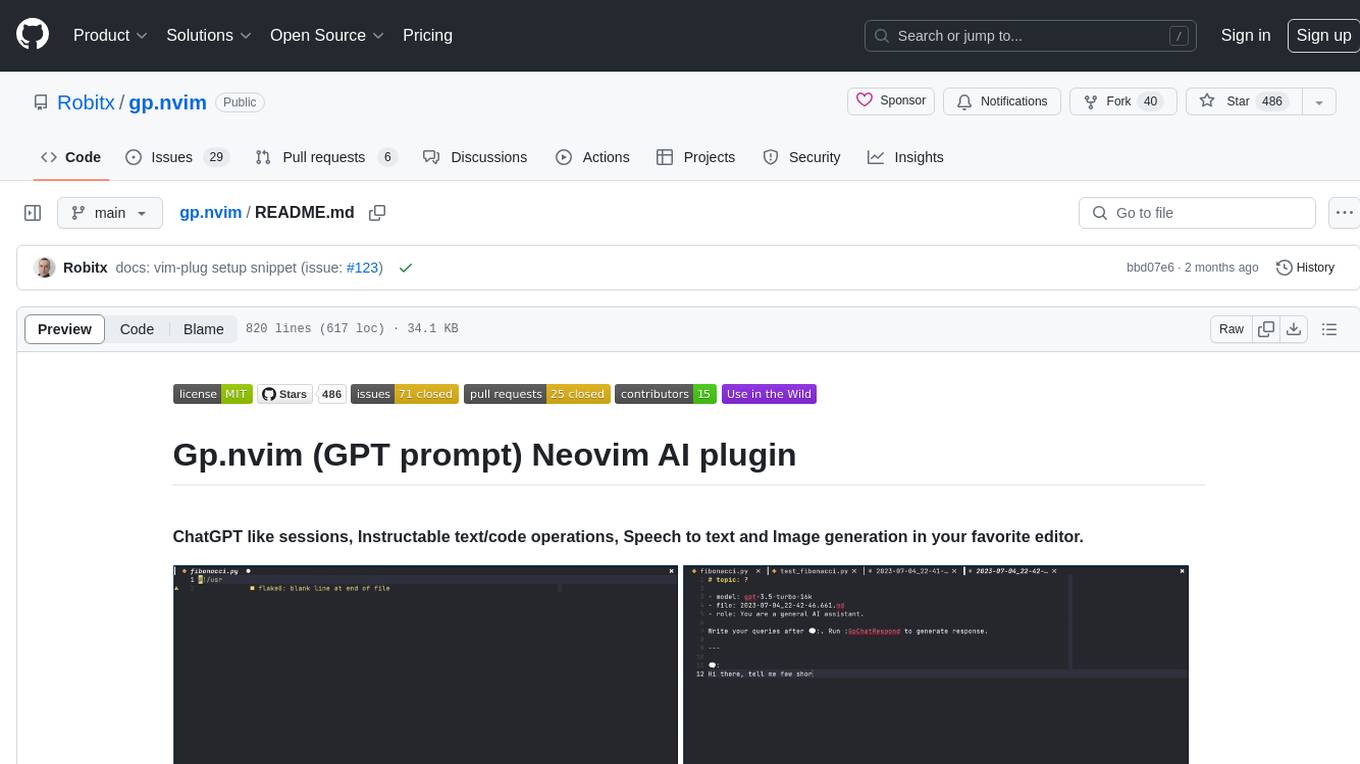
gp.nvim
Gp.nvim (GPT prompt) Neovim AI plugin provides a seamless integration of GPT models into Neovim, offering features like streaming responses, extensibility via hook functions, minimal dependencies, ChatGPT-like sessions, instructable text/code operations, speech-to-text support, and image generation directly within Neovim. The plugin aims to enhance the Neovim experience by leveraging the power of AI models in a user-friendly and native way.
For similar jobs
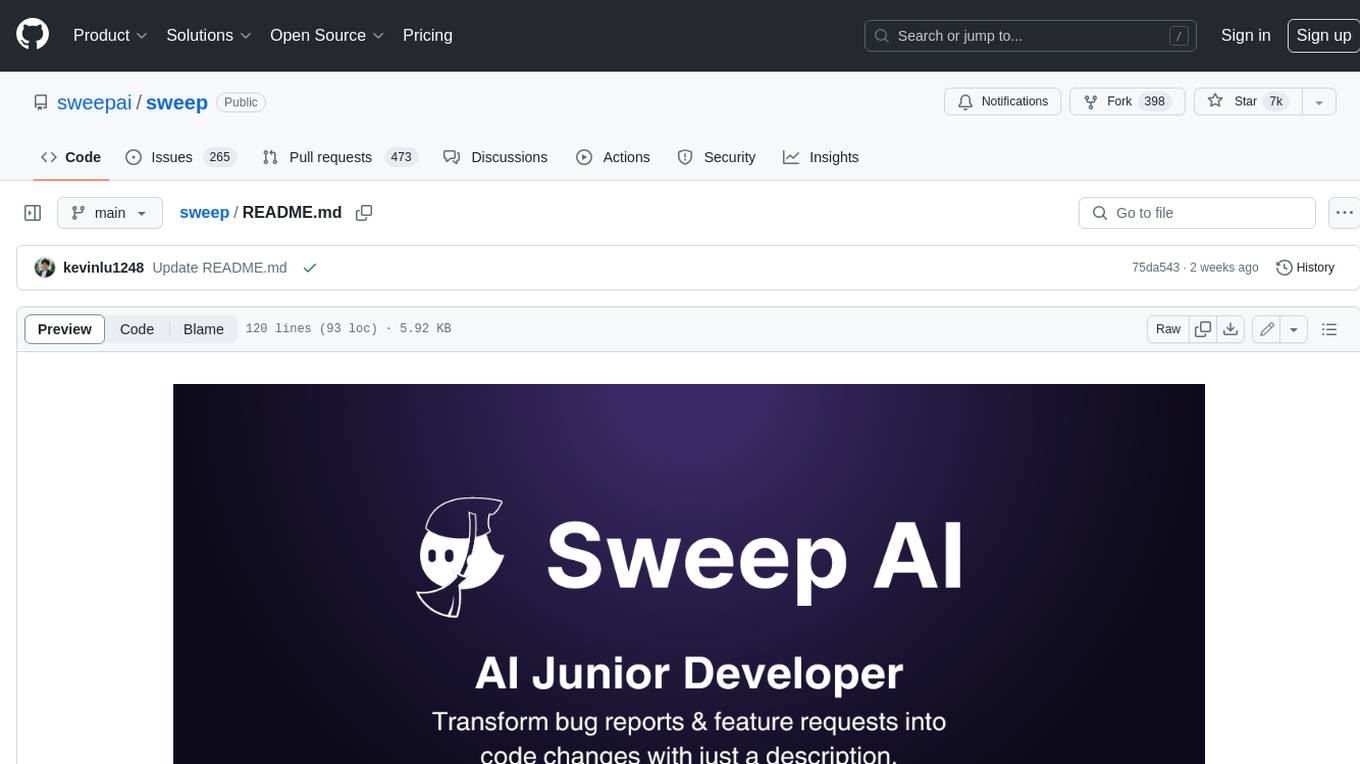
sweep
Sweep is an AI junior developer that turns bugs and feature requests into code changes. It automatically handles developer experience improvements like adding type hints and improving test coverage.
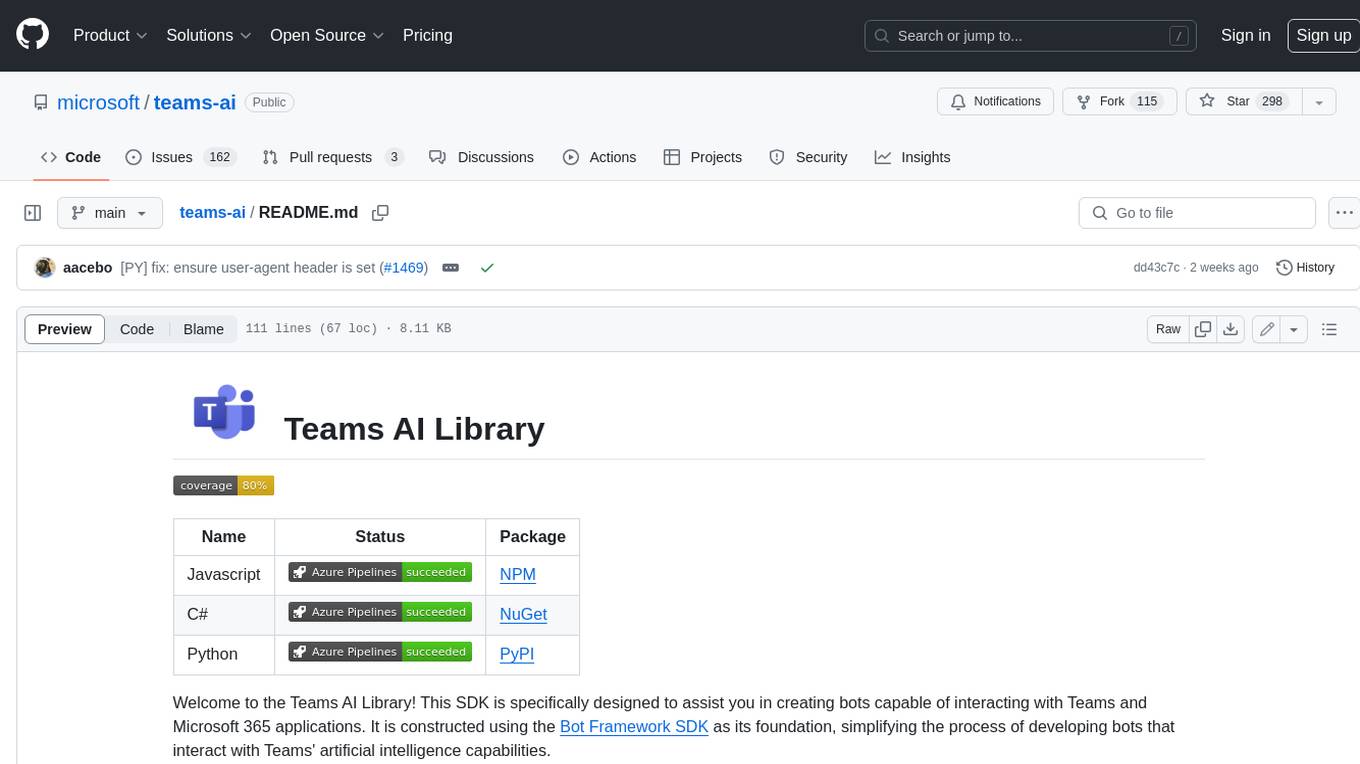
teams-ai
The Teams AI Library is a software development kit (SDK) that helps developers create bots that can interact with Teams and Microsoft 365 applications. It is built on top of the Bot Framework SDK and simplifies the process of developing bots that interact with Teams' artificial intelligence capabilities. The SDK is available for JavaScript/TypeScript, .NET, and Python.
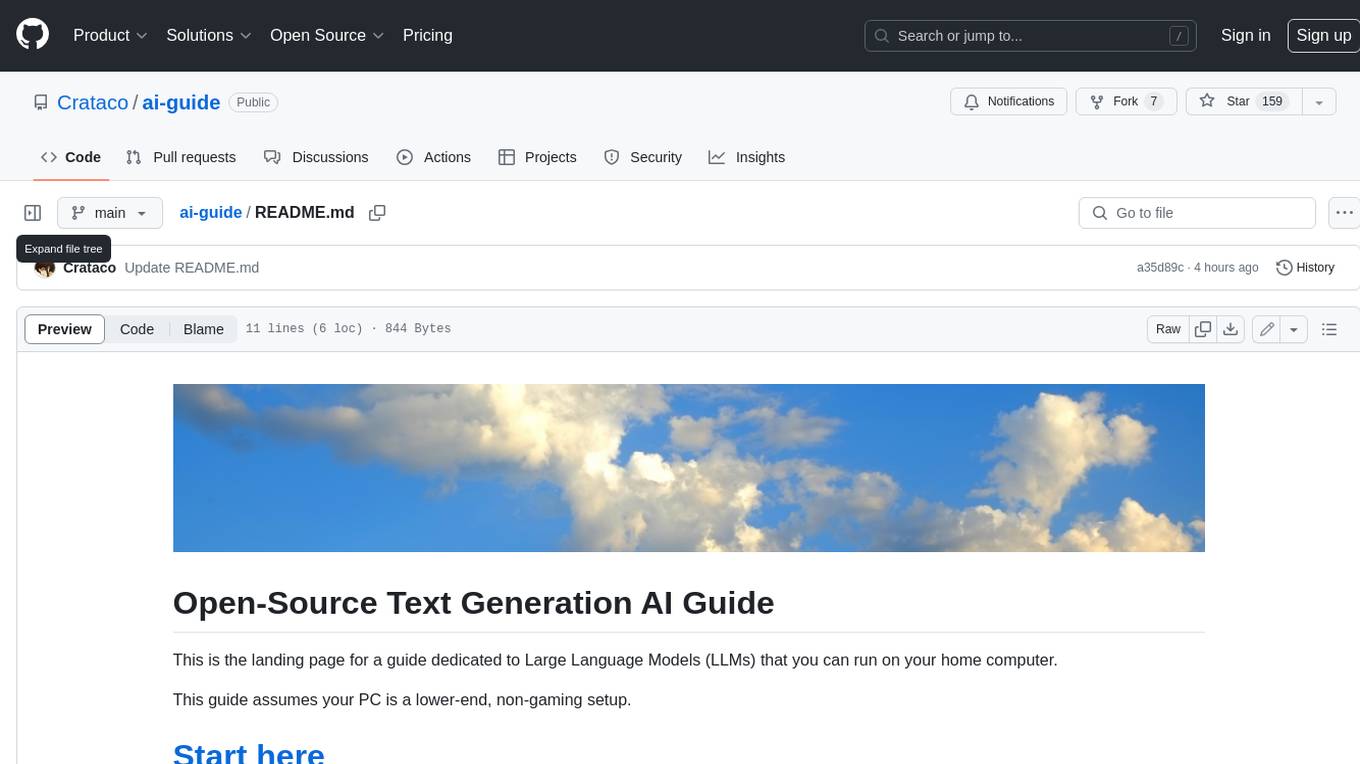
ai-guide
This guide is dedicated to Large Language Models (LLMs) that you can run on your home computer. It assumes your PC is a lower-end, non-gaming setup.
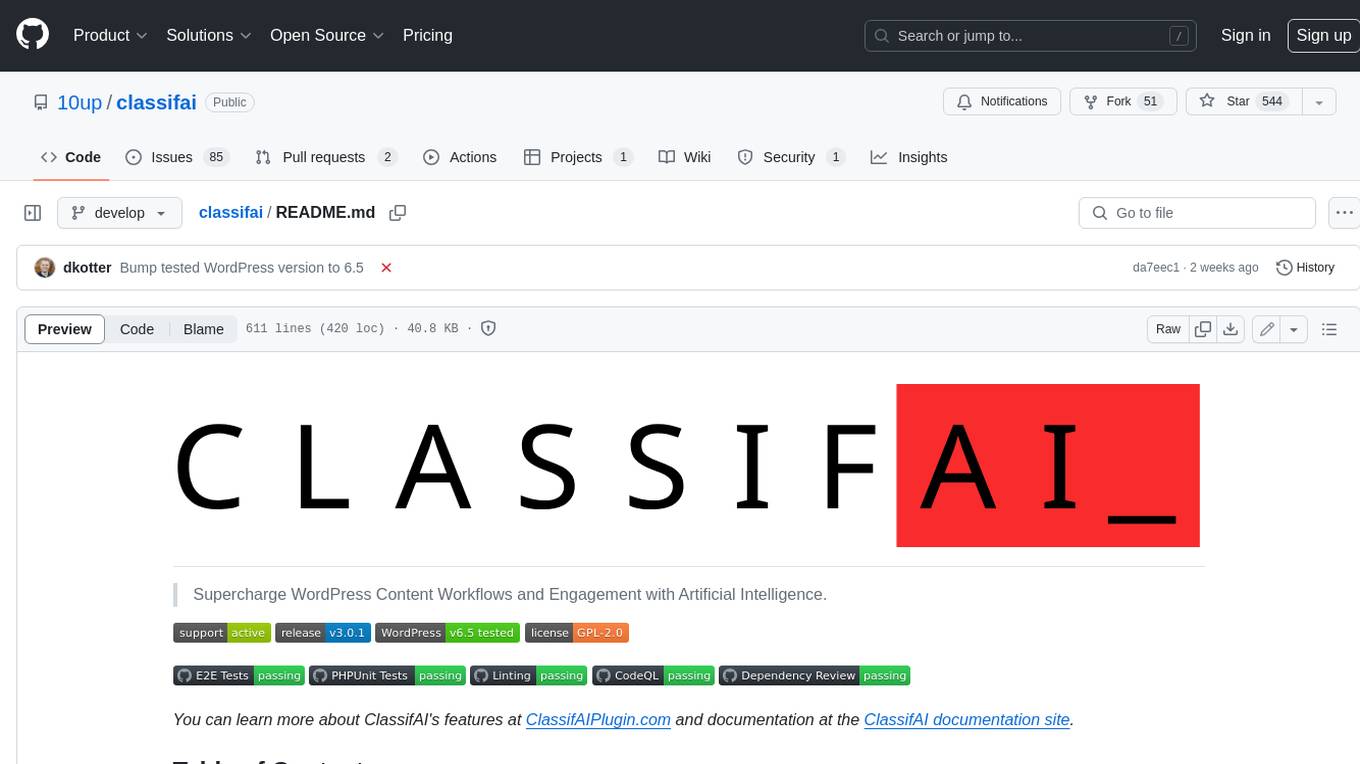
classifai
Supercharge WordPress Content Workflows and Engagement with Artificial Intelligence. Tap into leading cloud-based services like OpenAI, Microsoft Azure AI, Google Gemini and IBM Watson to augment your WordPress-powered websites. Publish content faster while improving SEO performance and increasing audience engagement. ClassifAI integrates Artificial Intelligence and Machine Learning technologies to lighten your workload and eliminate tedious tasks, giving you more time to create original content that matters.
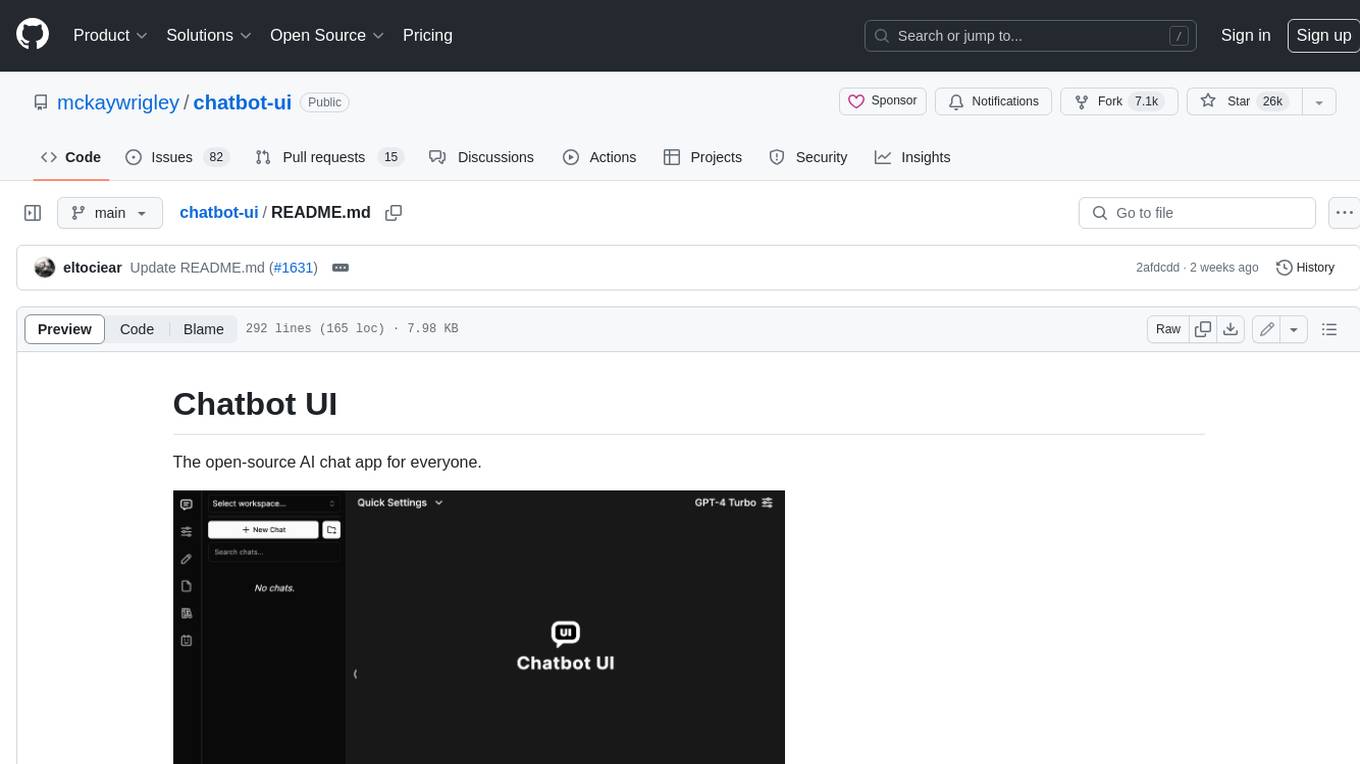
chatbot-ui
Chatbot UI is an open-source AI chat app that allows users to create and deploy their own AI chatbots. It is easy to use and can be customized to fit any need. Chatbot UI is perfect for businesses, developers, and anyone who wants to create a chatbot.
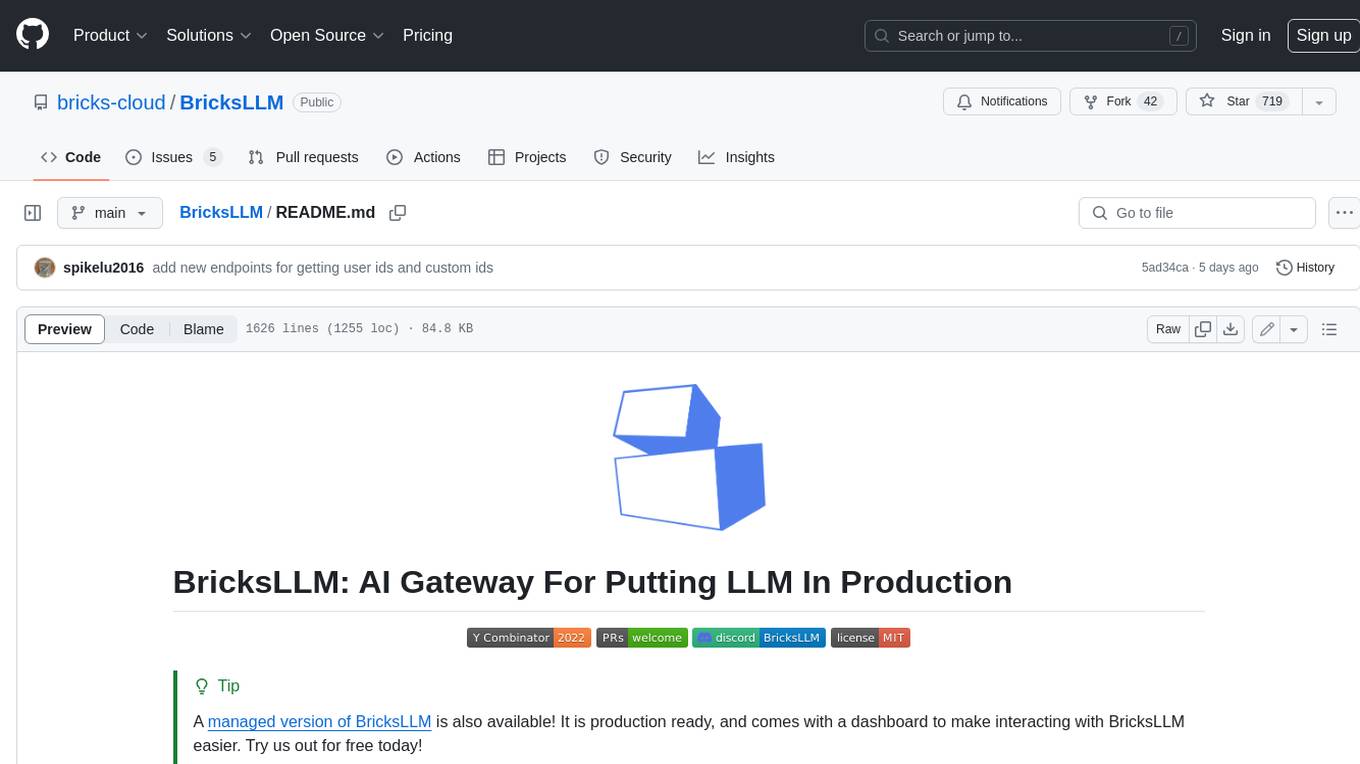
BricksLLM
BricksLLM is a cloud native AI gateway written in Go. Currently, it provides native support for OpenAI, Anthropic, Azure OpenAI and vLLM. BricksLLM aims to provide enterprise level infrastructure that can power any LLM production use cases. Here are some use cases for BricksLLM: * Set LLM usage limits for users on different pricing tiers * Track LLM usage on a per user and per organization basis * Block or redact requests containing PIIs * Improve LLM reliability with failovers, retries and caching * Distribute API keys with rate limits and cost limits for internal development/production use cases * Distribute API keys with rate limits and cost limits for students
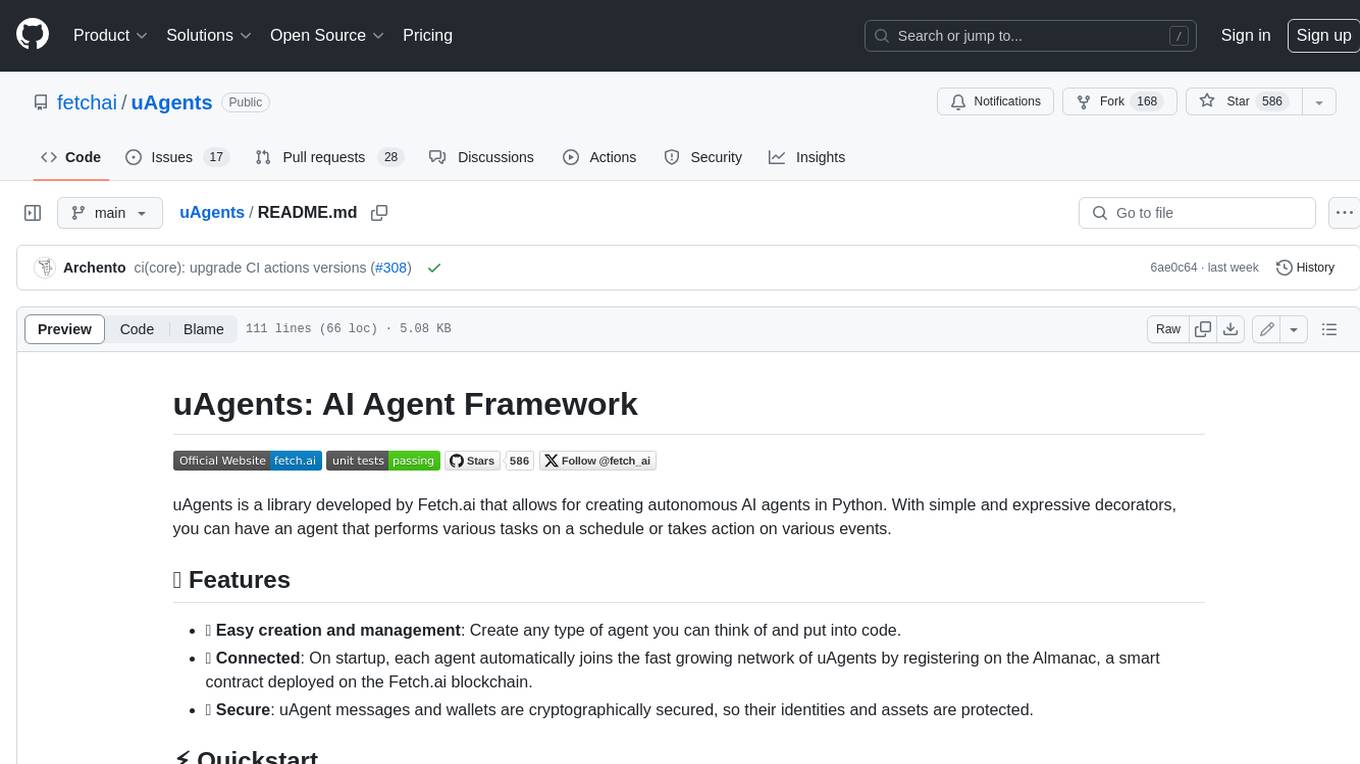
uAgents
uAgents is a Python library developed by Fetch.ai that allows for the creation of autonomous AI agents. These agents can perform various tasks on a schedule or take action on various events. uAgents are easy to create and manage, and they are connected to a fast-growing network of other uAgents. They are also secure, with cryptographically secured messages and wallets.
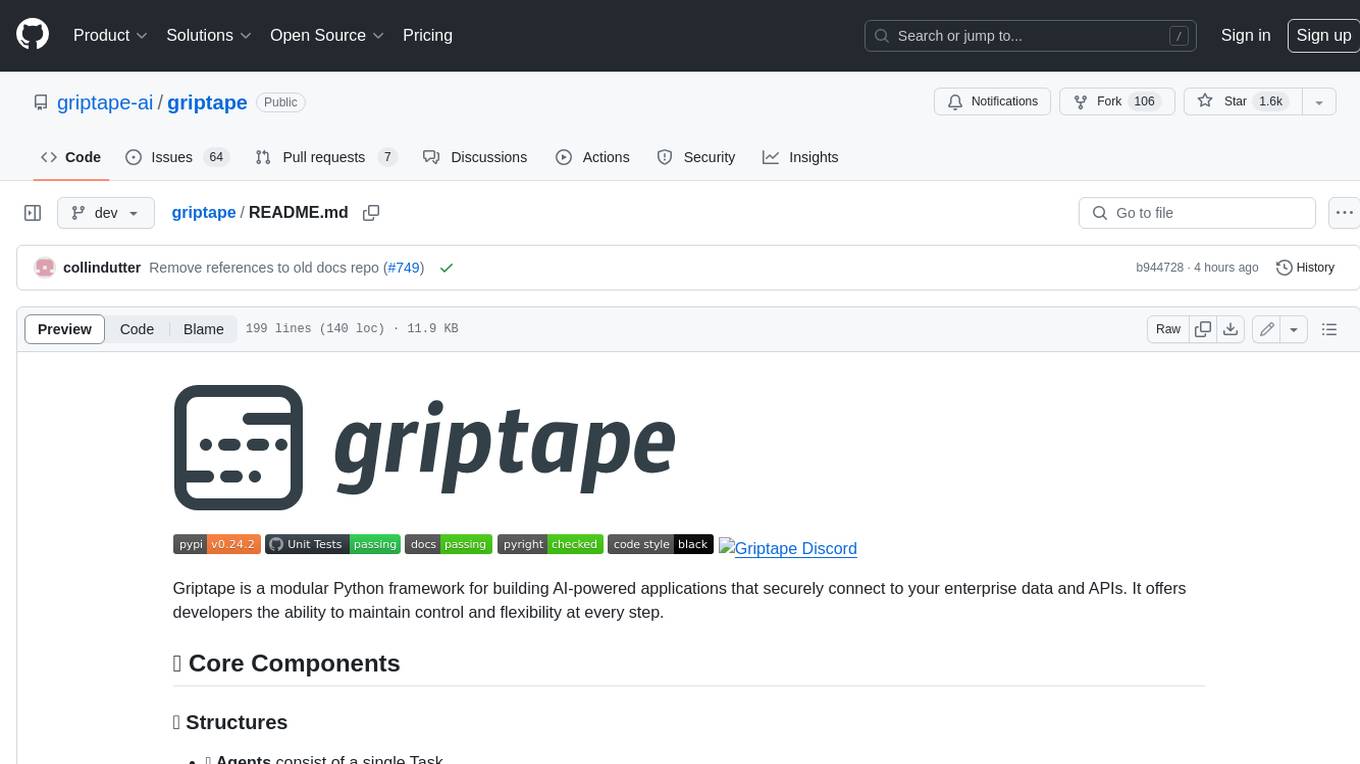
griptape
Griptape is a modular Python framework for building AI-powered applications that securely connect to your enterprise data and APIs. It offers developers the ability to maintain control and flexibility at every step. Griptape's core components include Structures (Agents, Pipelines, and Workflows), Tasks, Tools, Memory (Conversation Memory, Task Memory, and Meta Memory), Drivers (Prompt and Embedding Drivers, Vector Store Drivers, Image Generation Drivers, Image Query Drivers, SQL Drivers, Web Scraper Drivers, and Conversation Memory Drivers), Engines (Query Engines, Extraction Engines, Summary Engines, Image Generation Engines, and Image Query Engines), and additional components (Rulesets, Loaders, Artifacts, Chunkers, and Tokenizers). Griptape enables developers to create AI-powered applications with ease and efficiency.