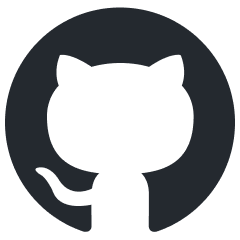
humanoid-gym
Humanoid-Gym: Reinforcement Learning for Humanoid Robot with Zero-Shot Sim2Real Transfer https://arxiv.org/abs/2404.05695
Stars: 388
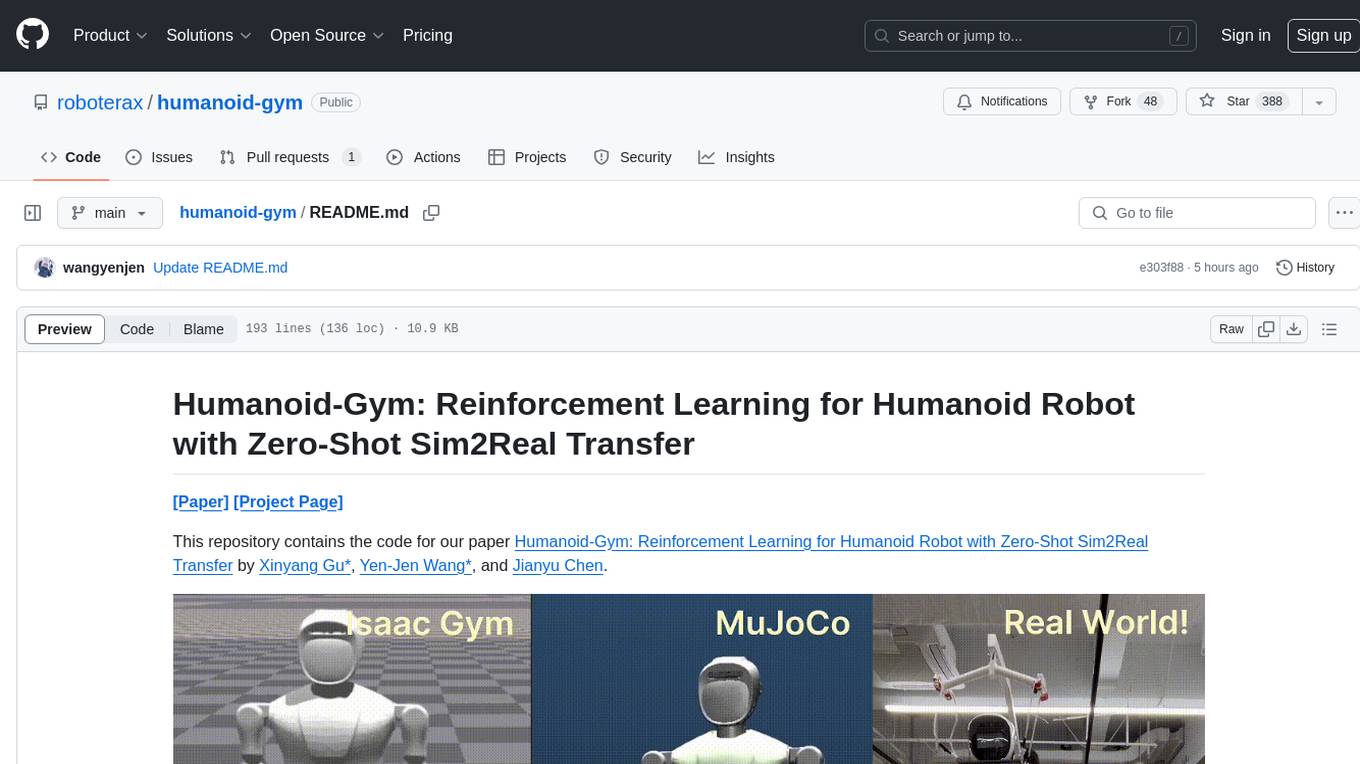
Humanoid-Gym is a reinforcement learning framework designed for training locomotion skills for humanoid robots, focusing on zero-shot transfer from simulation to real-world environments. It integrates a sim-to-sim framework from Isaac Gym to Mujoco for verifying trained policies in different physical simulations. The codebase is verified with RobotEra's XBot-S and XBot-L humanoid robots. It offers comprehensive training guidelines, step-by-step configuration instructions, and execution scripts for easy deployment. The sim2sim support allows transferring trained policies to accurate simulated environments. The upcoming features include Denoising World Model Learning and Dexterous Hand Manipulation. Installation and usage guides are provided along with examples for training PPO policies and sim-to-sim transformations. The code structure includes environment and configuration files, with instructions on adding new environments. Troubleshooting tips are provided for common issues, along with a citation and acknowledgment section.
README:
This repository contains the code for our paper Humanoid-Gym: Reinforcement Learning for Humanoid Robot with Zero-Shot Sim2Real Transfer by Xinyang Gu*, Yen-Jen Wang*, and Jianyu Chen.
Welcome to our Humanoid-Gym!
Humanoid-Gym is an easy-to-use reinforcement learning (RL) framework based on Nvidia Isaac Gym, designed to train locomotion skills for humanoid robots, emphasizing zero-shot transfer from simulation to the real-world environment. Humanoid-Gym also integrates a sim-to-sim framework from Isaac Gym to Mujoco that allows users to verify the trained policies in different physical simulations to ensure the robustness and generalization of the policies.
This codebase is verified by RobotEra's XBot-S (1.2 meter tall humanoid robot) and XBot-L (1.65 meter tall humanoid robot) in real-world environment with zero-shot sim-to-real transfer.
This repository offers comprehensive guidance and scripts for the training of humanoid robots. Humanoid-Gym features specialized rewards for humanoid robots, simplifying the difficulty of sim-to-real transfer. In this repository, we use RobotEra's XBot-L as a primary example. It can also be used for other robots with minimal adjustments. Our resources cover setup, configuration, and execution. Our goal is to fully prepare the robot for real-world locomotion by providing in-depth training and optimization.
- Comprehensive Training Guidelines: We offer thorough walkthroughs for each stage of the training process.
- Step-by-Step Configuration Instructions: Our guidance is clear and succinct, ensuring an efficient setup process.
- Execution Scripts for Easy Deployment: Utilize our pre-prepared scripts to streamline the training workflow.
We also share our sim2sim pipeline, which allows you to transfer trained policies to highly accurate and carefully designed simulated environments. Once you acquire the robot, you can confidently deploy the RL-trained policies in real-world settings.
Our simulator settings, particularly with Mujoco, are finely tuned to closely mimic real-world scenarios. This careful calibration ensures that the performances in both simulated and real-world environments are closely aligned. This improvement makes our simulations more trustworthy and enhances our confidence in their applicability to real-world scenarios.
Denoising World Model Learning(DWL) presents an advanced sim-to-real framework that integrates state estimation and system identification. This dual-method approach ensures the robot's learning and adaptation are both practical and effective in real-world contexts.
- Enhanced Sim-to-real Adaptability: Techniques to optimize the robot's transition from simulated to real environments.
- Improved State Estimation Capabilities: Advanced tools for precise and reliable state analysis.
- Generate a new Python virtual environment with Python 3.8 using
conda create -n myenv python=3.8
. - For the best performance, we recommend using NVIDIA driver version 525
sudo apt install nvidia-driver-525
. The minimal driver version supported is 515. If you're unable to install version 525, ensure that your system has at least version 515 to maintain basic functionality. - Install PyTorch 1.13 with Cuda-11.7:
conda install pytorch==1.13.1 torchvision==0.14.1 torchaudio==0.13.1 pytorch-cuda=11.7 -c pytorch -c nvidia
- Install numpy-1.23 with
conda install numpy=1.23
. - Install Isaac Gym:
- Download and install Isaac Gym Preview 4 from https://developer.nvidia.com/isaac-gym.
cd isaacgym/python && pip install -e .
- Run an example with
cd examples && python 1080_balls_of_solitude.py
. - Consult
isaacgym/docs/index.html
for troubleshooting.
- Install humanoid-gym:
- Clone this repository.
cd humanoid_gym && pip install -e .
# Launching PPO Policy Training for 'v1' Across 4096 Environments
# This command initiates the PPO algorithm-based training for the humanoid task.
python scripts/train.py --task=humanoid_ppo --run_name v1 --headless --num_envs 4096
# Evaluating the Trained PPO Policy 'v1'
# This command loads the 'v1' policy for performance assessment in its environment.
# Additionally, it automatically exports a JIT model, suitable for deployment purposes.
python scripts/play.py --task=humanoid_ppo --run_name v1
# Implementing Simulation-to-Simulation Model Transformation
# This command facilitates a sim-to-sim transformation using exported 'v1' policy.
python scripts/sim2sim.py --load_model /path/to/logs/XBot_ppo/exported/policies/policy_1.pt
# Run our trained policy
python scripts/sim2sim.py --load_model /path/to/logs/XBot_ppo/exported/policies/policy_example.pt
-
humanoid_ppo
- Purpose: Baseline, PPO policy, Multi-frame low-level control
- Observation Space: Variable $(47 \times H)$ dimensions, where $H$ is the number of frames
- $[O_{t-H} ... O_t]$
- Privileged Information: $73$ dimensions
-
humanoid_dwl (coming soon)
-
Training Command: For training the PPO policy, execute:
python humanoid/scripts/train.py --task=humanoid_ppo --load_run log_file_path --name run_name
-
Running a Trained Policy: To deploy a trained PPO policy, use:
python humanoid/scripts/play.py --task=humanoid_ppo --load_run log_file_path --name run_name
- By default, the latest model of the last run from the experiment folder is loaded. However, other run iterations/models can be selected by adjusting
load_run
andcheckpoint
in the training config.
- Please note: Before initiating the sim-to-sim process, ensure that you run
play.py
to export a JIT policy. -
Mujoco-based Sim2Sim Deployment: Utilize Mujoco for executing simulation-to-simulation (sim2sim) deployments with the command below:
python scripts/sim2sim.py --load_model /path/to/export/model.pt
-
CPU and GPU Usage: To run simulations on the CPU, set both
--sim_device=cpu
and--rl_device=cpu
. For GPU operations, specify--sim_device=cuda:{0,1,2...}
and--rl_device={0,1,2...}
accordingly. Please note thatCUDA_VISIBLE_DEVICES
is not applicable, and it's essential to match the--sim_device
and--rl_device
settings. -
Headless Operation: Include
--headless
for operations without rendering. - Rendering Control: Press 'v' to toggle rendering during training.
-
Policy Location: Trained policies are saved in
humanoid/logs/<experiment_name>/<date_time>_<run_name>/model_<iteration>.pt
.
For RL training, please refer to humanoid/utils/helpers.py#L161
.
For the sim-to-sim process, please refer to humanoid/scripts/sim2sim.py#L169
.
- Every environment hinges on an
env
file (legged_robot.py
) and aconfiguration
file (legged_robot_config.py
). The latter houses two classes:LeggedRobotCfg
(encompassing all environmental parameters) andLeggedRobotCfgPPO
(denoting all training parameters). - Both
env
andconfig
classes use inheritance. - Non-zero reward scales specified in
cfg
contribute a function of the corresponding name to the sum-total reward. - Tasks must be registered with
task_registry.register(name, EnvClass, EnvConfig, TrainConfig)
. Registration may occur withinenvs/__init__.py
, or outside of this repository.
The base environment legged_robot
constructs a rough terrain locomotion task. The corresponding configuration does not specify a robot asset (URDF/ MJCF) and no reward scales.
- If you need to add a new environment, create a new folder in the
envs/
directory with a configuration file named<your_env>_config.py
. The new configuration should inherit from existing environment configurations. - If proposing a new robot:
- Insert the corresponding assets in the
resources/
folder. - In the
cfg
file, set the path to the asset, define body names, default_joint_positions, and PD gains. Specify the desiredtrain_cfg
and the environment's name (python class). - In the
train_cfg
, set theexperiment_name
andrun_name
.
- Insert the corresponding assets in the
- If needed, create your environment in
<your_env>.py
. Inherit from existing environments, override desired functions and/or add your reward functions. - Register your environment in
humanoid/envs/__init__.py
. - Modify or tune other parameters in your
cfg
orcfg_train
as per requirements. To remove the reward, set its scale to zero. Avoid modifying the parameters of other environments! - If you want a new robot/environment to perform sim2sim, you may need to modify
humanoid/scripts/sim2sim.py
:- Check the joint mapping of the robot between MJCF and URDF.
- Change the initial joint position of the robot according to your trained policy.
Observe the following cases:
# error
ImportError: libpython3.8.so.1.0: cannot open shared object file: No such file or directory
# solution
# set the correct path
export LD_LIBRARY_PATH="~/miniconda3/envs/your_env/lib:$LD_LIBRARY_PATH"
# OR
sudo apt install libpython3.8
# error
AttributeError: module 'distutils' has no attribute 'version'
# solution
# install pytorch 1.12.0
conda install pytorch torchvision torchaudio cudatoolkit=11.3 -c pytorch
# error, results from libstdc++ version distributed with conda differing from the one used on your system to build Isaac Gym
ImportError: /home/roboterax/anaconda3/bin/../lib/libstdc++.so.6: version `GLIBCXX_3.4.20` not found (required by /home/roboterax/carbgym/python/isaacgym/_bindings/linux64/gym_36.so)
# solution
mkdir ${YOUR_CONDA_ENV}/lib/_unused
mv ${YOUR_CONDA_ENV}/lib/libstdc++* ${YOUR_CONDA_ENV}/lib/_unused
Please cite the following if you use this code or parts of it:
@article{gu2024humanoid,
title={Humanoid-Gym: Reinforcement Learning for Humanoid Robot with Zero-Shot Sim2Real Transfer},
author={Gu, Xinyang and Wang, Yen-Jen and Chen, Jianyu},
journal={arXiv preprint arXiv:2404.05695},
year={2024}
}
The implementation of Humanoid-Gym relies on resources from legged_gym and rsl_rl projects, created by the Robotic Systems Lab. We specifically utilize the LeggedRobot
implementation from their research to enhance our codebase.
If you have further questions, please feel free to contact [email protected] or create an issue in this repository.
For Tasks:
Click tags to check more tools for each tasksFor Jobs:
Alternative AI tools for humanoid-gym
Similar Open Source Tools
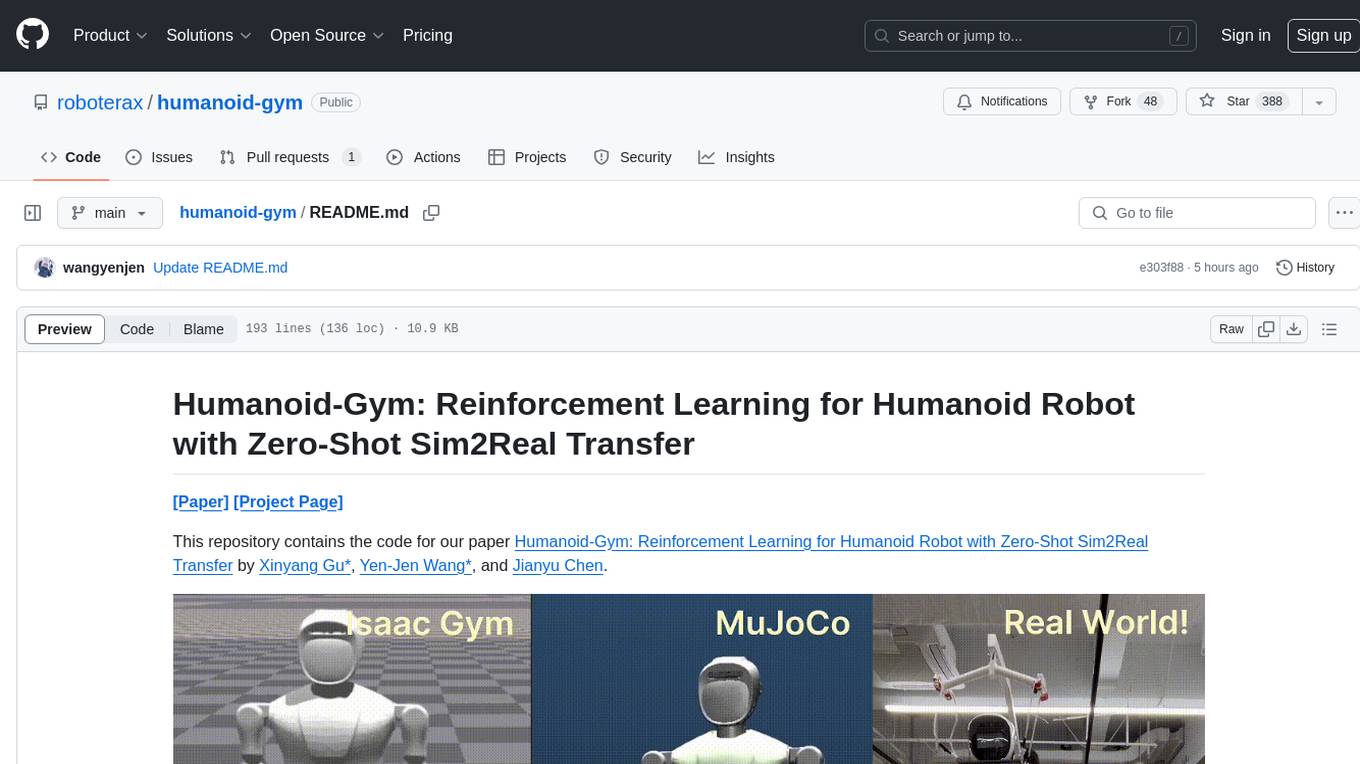
humanoid-gym
Humanoid-Gym is a reinforcement learning framework designed for training locomotion skills for humanoid robots, focusing on zero-shot transfer from simulation to real-world environments. It integrates a sim-to-sim framework from Isaac Gym to Mujoco for verifying trained policies in different physical simulations. The codebase is verified with RobotEra's XBot-S and XBot-L humanoid robots. It offers comprehensive training guidelines, step-by-step configuration instructions, and execution scripts for easy deployment. The sim2sim support allows transferring trained policies to accurate simulated environments. The upcoming features include Denoising World Model Learning and Dexterous Hand Manipulation. Installation and usage guides are provided along with examples for training PPO policies and sim-to-sim transformations. The code structure includes environment and configuration files, with instructions on adding new environments. Troubleshooting tips are provided for common issues, along with a citation and acknowledgment section.
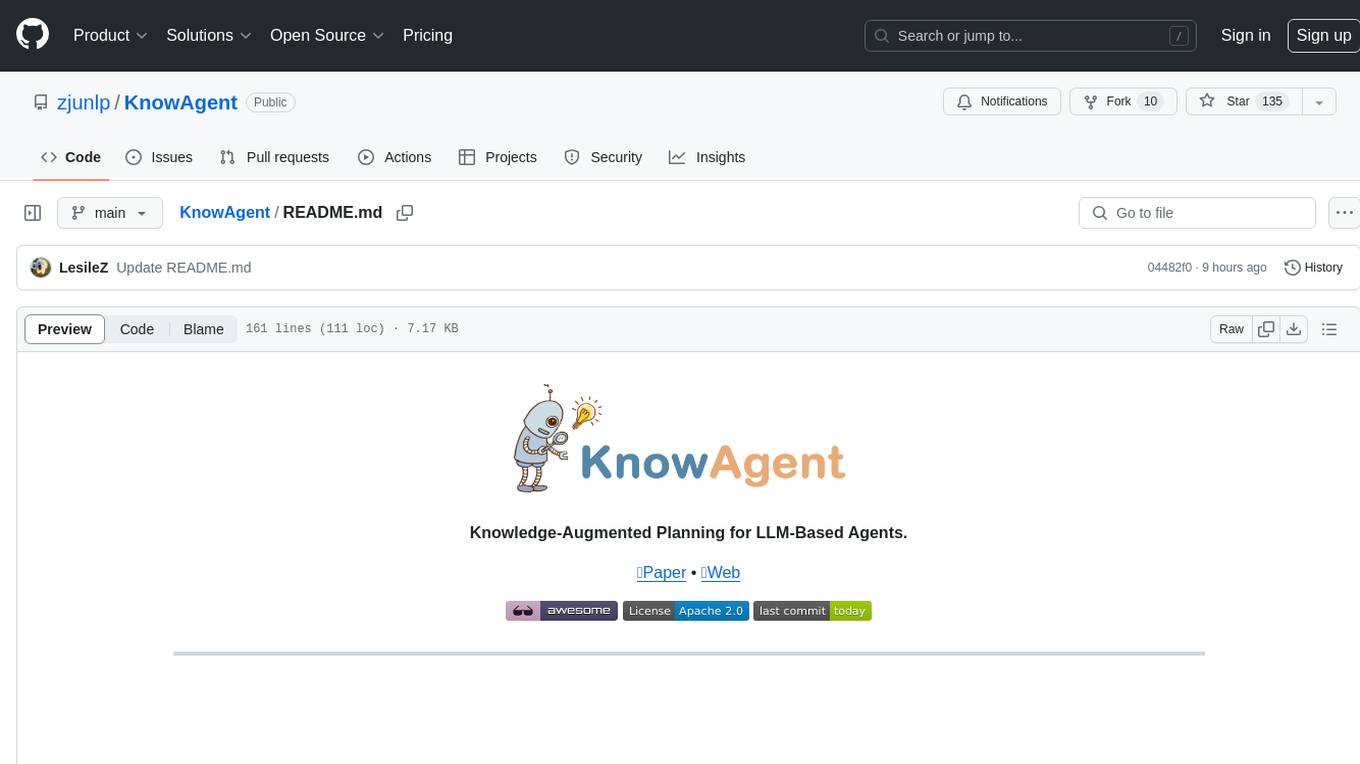
KnowAgent
KnowAgent is a tool designed for Knowledge-Augmented Planning for LLM-Based Agents. It involves creating an action knowledge base, converting action knowledge into text for model understanding, and a knowledgeable self-learning phase to continually improve the model's planning abilities. The tool aims to enhance agents' potential for application in complex situations by leveraging external reservoirs of information and iterative processes.
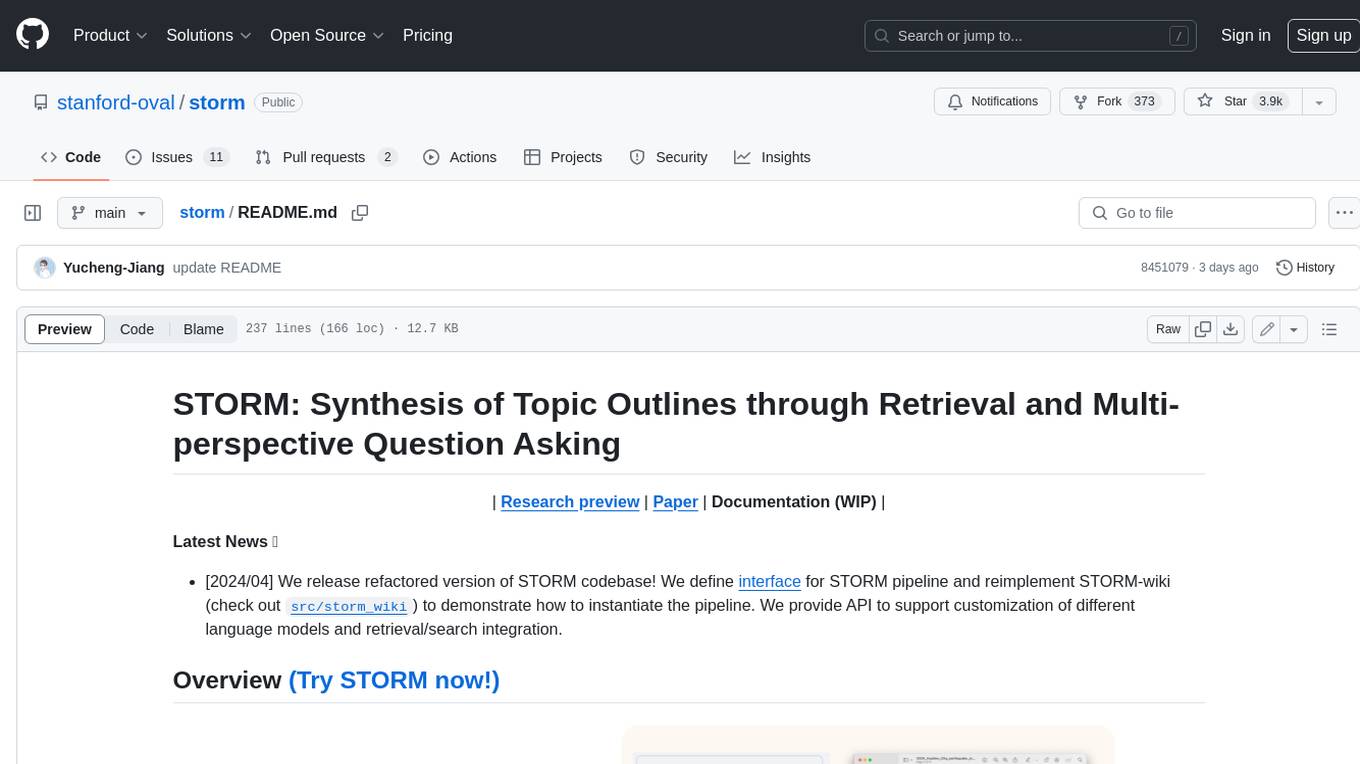
storm
STORM is a LLM system that writes Wikipedia-like articles from scratch based on Internet search. While the system cannot produce publication-ready articles that often require a significant number of edits, experienced Wikipedia editors have found it helpful in their pre-writing stage. **Try out our [live research preview](https://storm.genie.stanford.edu/) to see how STORM can help your knowledge exploration journey and please provide feedback to help us improve the system 🙏!**
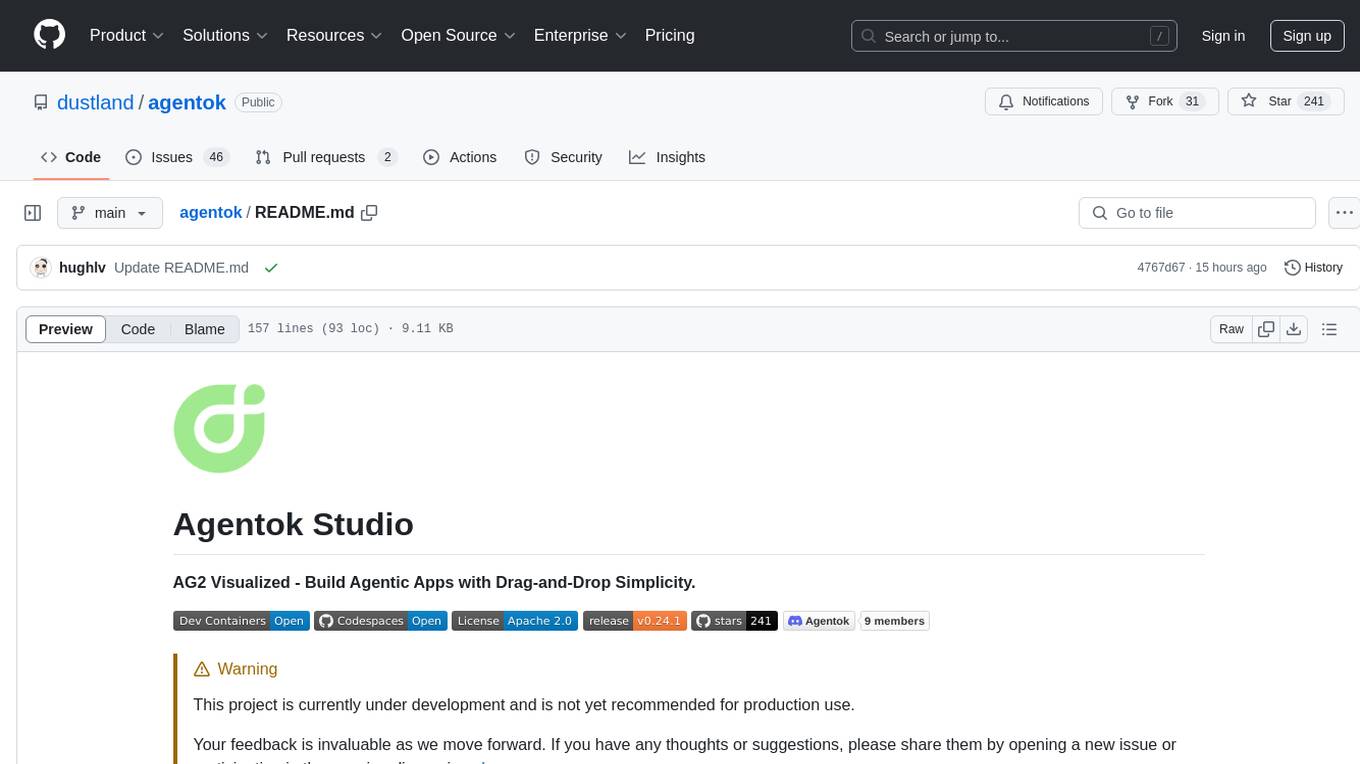
agentok
Agentok Studio is a tool built upon AG2, a powerful agent framework from Microsoft, offering intuitive visual tools to streamline the creation and management of complex agent-based workflows. It simplifies the process for creators and developers by generating native Python code with minimal dependencies, enabling users to create self-contained code that can be executed anywhere. The tool is currently under development and not recommended for production use, but contributions are welcome from the community to enhance its capabilities and functionalities.
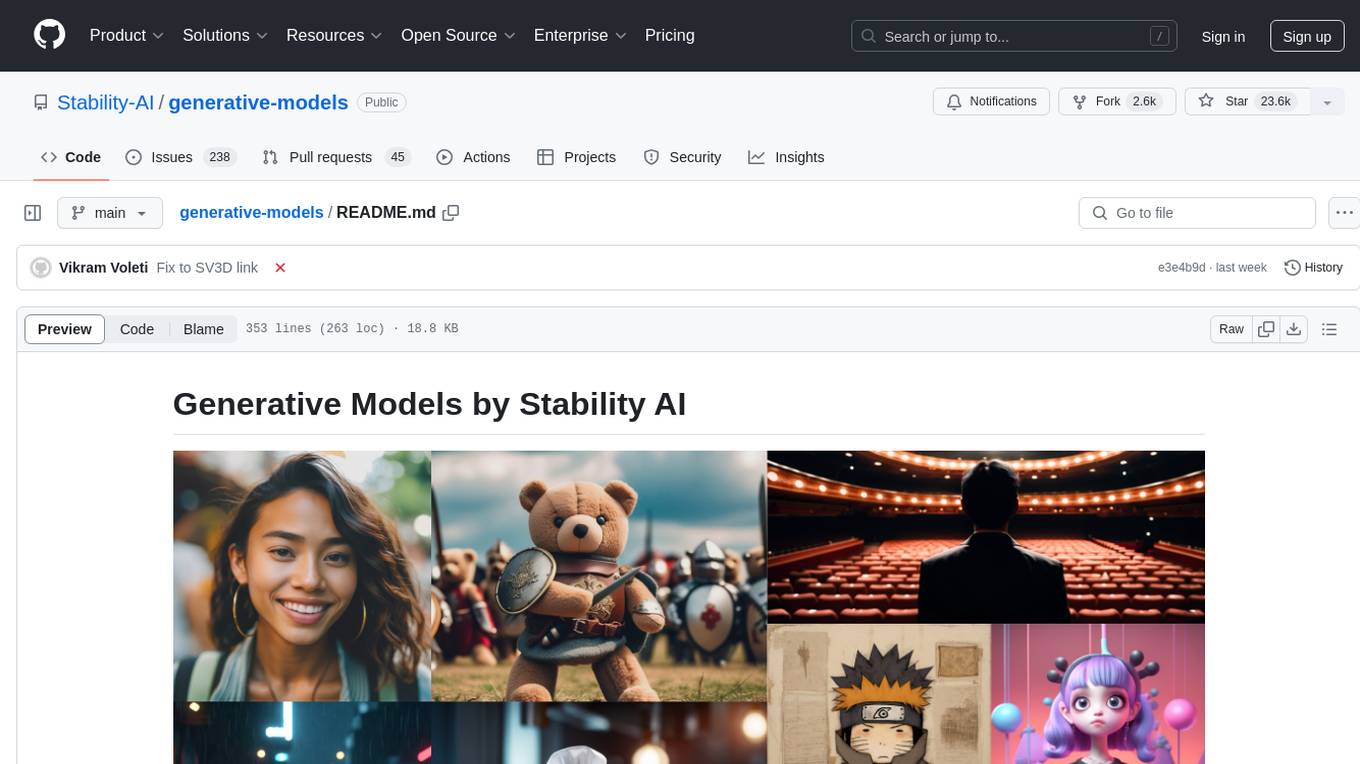
generative-models
Generative Models by Stability AI is a repository that provides various generative models for research purposes. It includes models like Stable Video 4D (SV4D) for video synthesis, Stable Video 3D (SV3D) for multi-view synthesis, SDXL-Turbo for text-to-image generation, and more. The repository focuses on modularity and implements a config-driven approach for building and combining submodules. It supports training with PyTorch Lightning and offers inference demos for different models. Users can access pre-trained models like SDXL-base-1.0 and SDXL-refiner-1.0 under a CreativeML Open RAIL++-M license. The codebase also includes tools for invisible watermark detection in generated images.
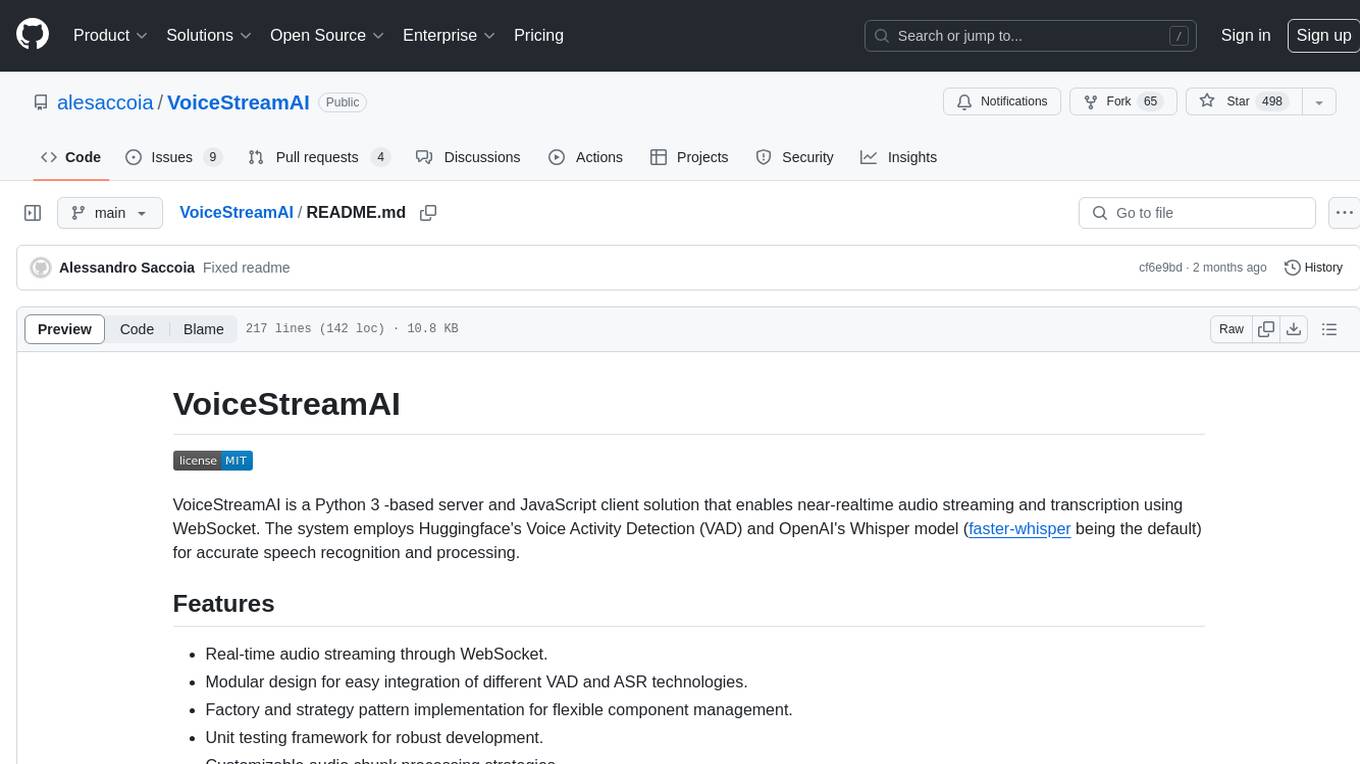
VoiceStreamAI
VoiceStreamAI is a Python 3-based server and JavaScript client solution for near-realtime audio streaming and transcription using WebSocket. It employs Huggingface's Voice Activity Detection (VAD) and OpenAI's Whisper model for accurate speech recognition. The system features real-time audio streaming, modular design for easy integration of VAD and ASR technologies, customizable audio chunk processing strategies, support for multilingual transcription, and secure sockets support. It uses a factory and strategy pattern implementation for flexible component management and provides a unit testing framework for robust development.
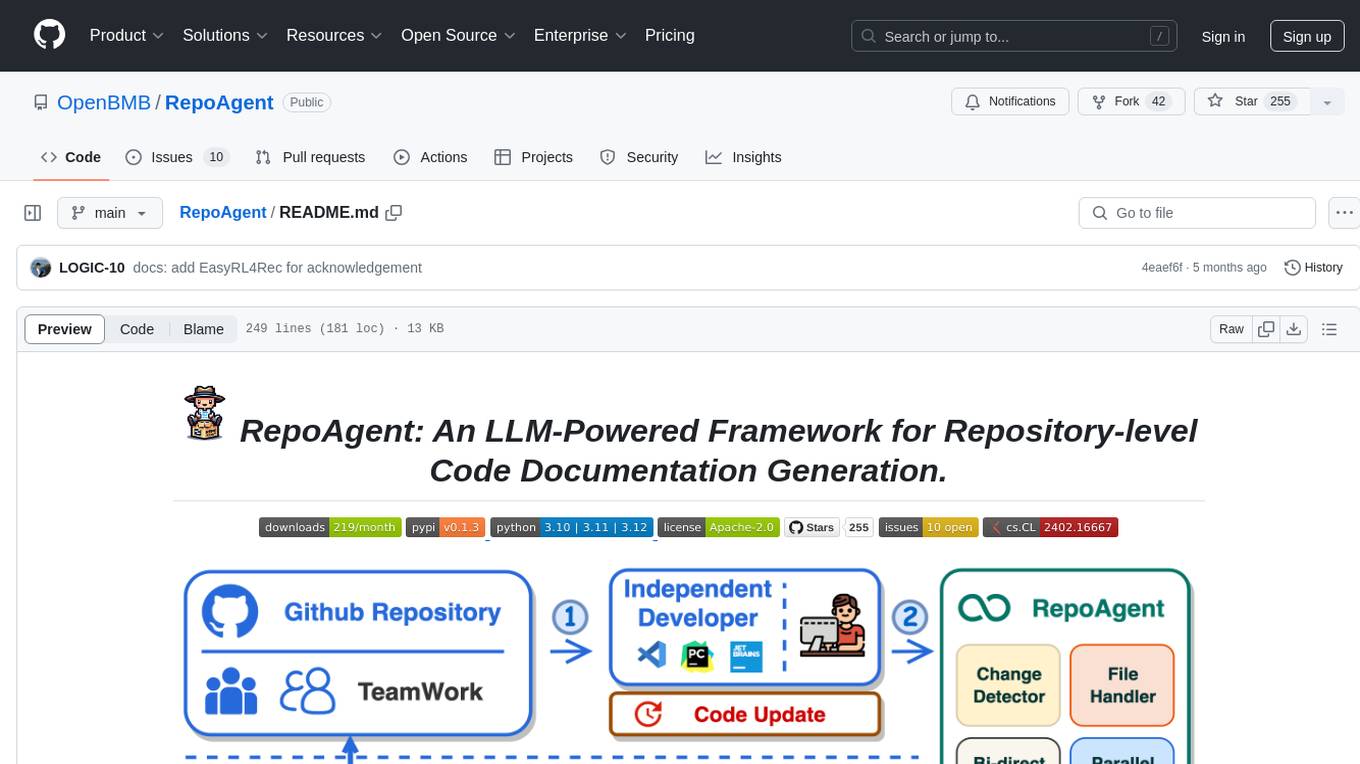
RepoAgent
RepoAgent is an LLM-powered framework designed for repository-level code documentation generation. It automates the process of detecting changes in Git repositories, analyzing code structure through AST, identifying inter-object relationships, replacing Markdown content, and executing multi-threaded operations. The tool aims to assist developers in understanding and maintaining codebases by providing comprehensive documentation, ultimately improving efficiency and saving time.
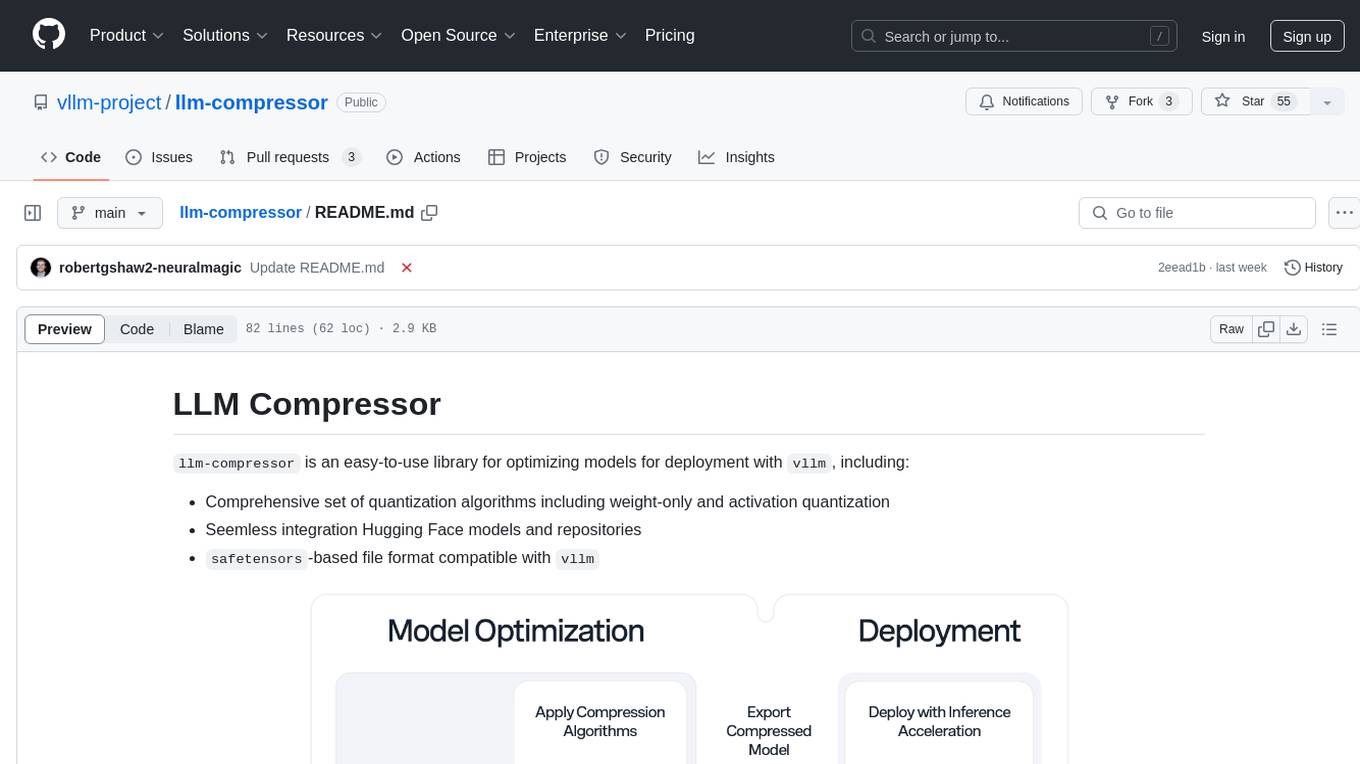
llm-compressor
llm-compressor is an easy-to-use library for optimizing models for deployment with vllm. It provides a comprehensive set of quantization algorithms, seamless integration with Hugging Face models and repositories, and supports mixed precision, activation quantization, and sparsity. Supported algorithms include PTQ, GPTQ, SmoothQuant, and SparseGPT. Installation can be done via git clone and local pip install. Compression can be easily applied by selecting an algorithm and calling the oneshot API. The library also offers end-to-end examples for model compression. Contributions to the code, examples, integrations, and documentation are appreciated.
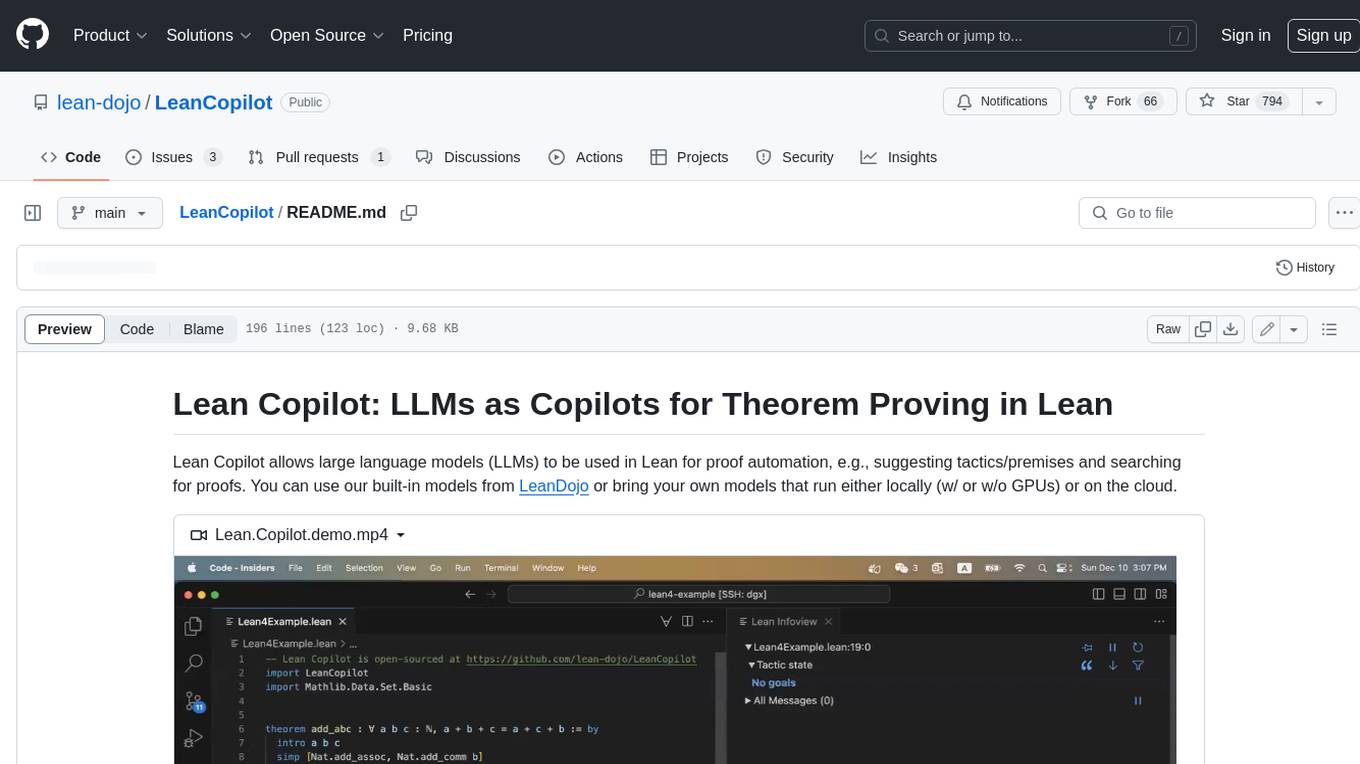
LeanCopilot
Lean Copilot is a tool that enables the use of large language models (LLMs) in Lean for proof automation. It provides features such as suggesting tactics/premises, searching for proofs, and running inference of LLMs. Users can utilize built-in models from LeanDojo or bring their own models to run locally or on the cloud. The tool supports platforms like Linux, macOS, and Windows WSL, with optional CUDA and cuDNN for GPU acceleration. Advanced users can customize behavior using Tactic APIs and Model APIs. Lean Copilot also allows users to bring their own models through ExternalGenerator or ExternalEncoder. The tool comes with caveats such as occasional crashes and issues with premise selection and proof search. Users can get in touch through GitHub Discussions for questions, bug reports, feature requests, and suggestions. The tool is designed to enhance theorem proving in Lean using LLMs.
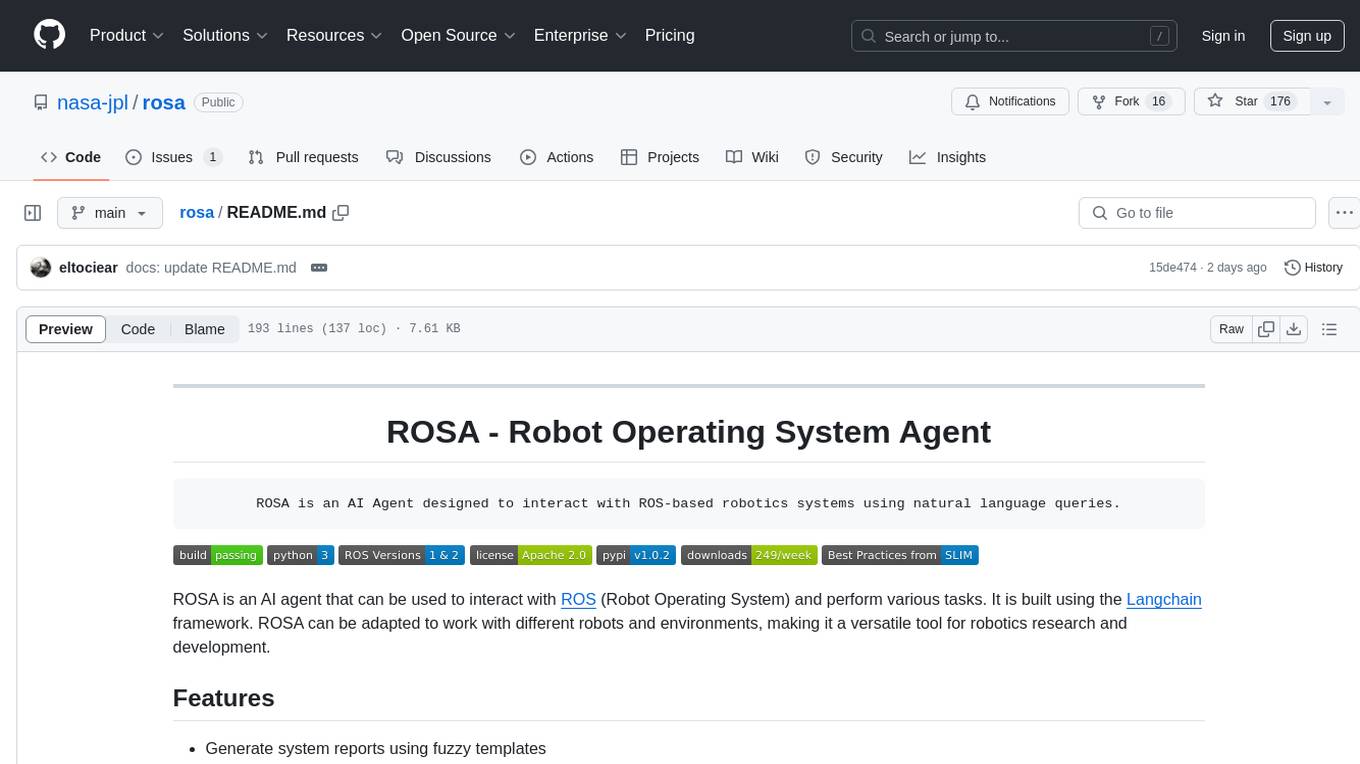
rosa
ROSA is an AI Agent designed to interact with ROS-based robotics systems using natural language queries. It can generate system reports, read and parse ROS log files, adapt to new robots, and run various ROS commands using natural language. The tool is versatile for robotics research and development, providing an easy way to interact with robots and the ROS environment.
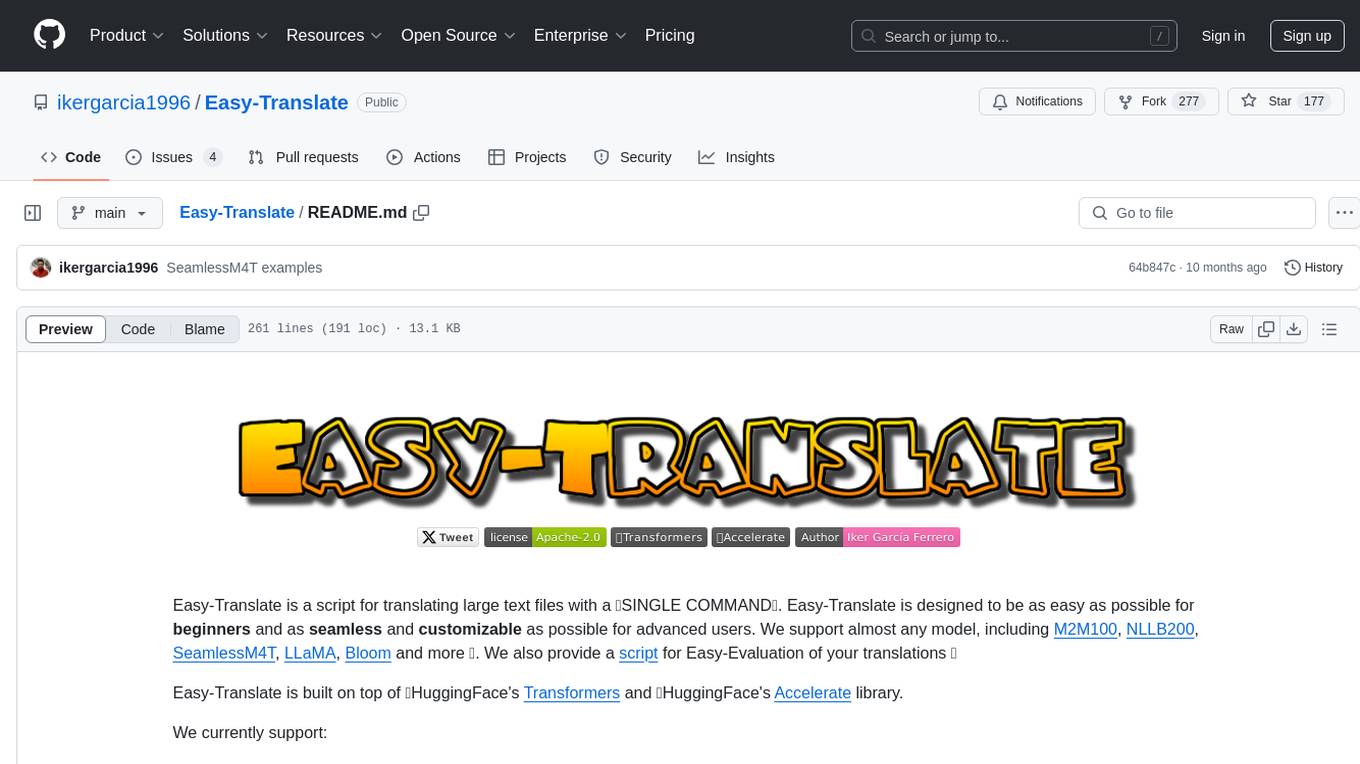
Easy-Translate
Easy-Translate is a script designed for translating large text files with a single command. It supports various models like M2M100, NLLB200, SeamlessM4T, LLaMA, and Bloom. The tool is beginner-friendly and offers seamless and customizable features for advanced users. It allows acceleration on CPU, multi-CPU, GPU, multi-GPU, and TPU, with support for different precisions and decoding strategies. Easy-Translate also provides an evaluation script for translations. Built on HuggingFace's Transformers and Accelerate library, it supports prompt usage and loading huge models efficiently.
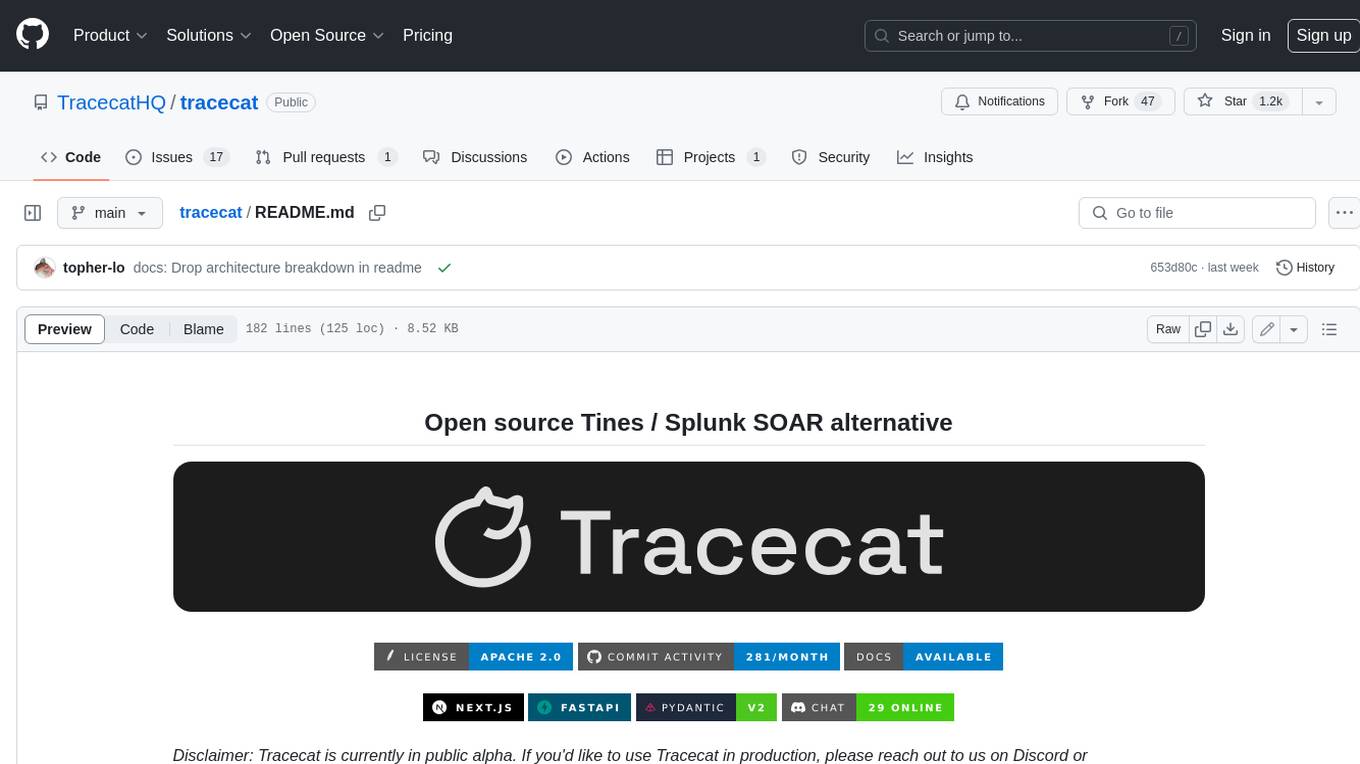
tracecat
Tracecat is an open-source automation platform for security teams. It's designed to be simple but powerful, with a focus on AI features and a practitioner-obsessed UI/UX. Tracecat can be used to automate a variety of tasks, including phishing email investigation, evidence collection, and remediation plan generation.
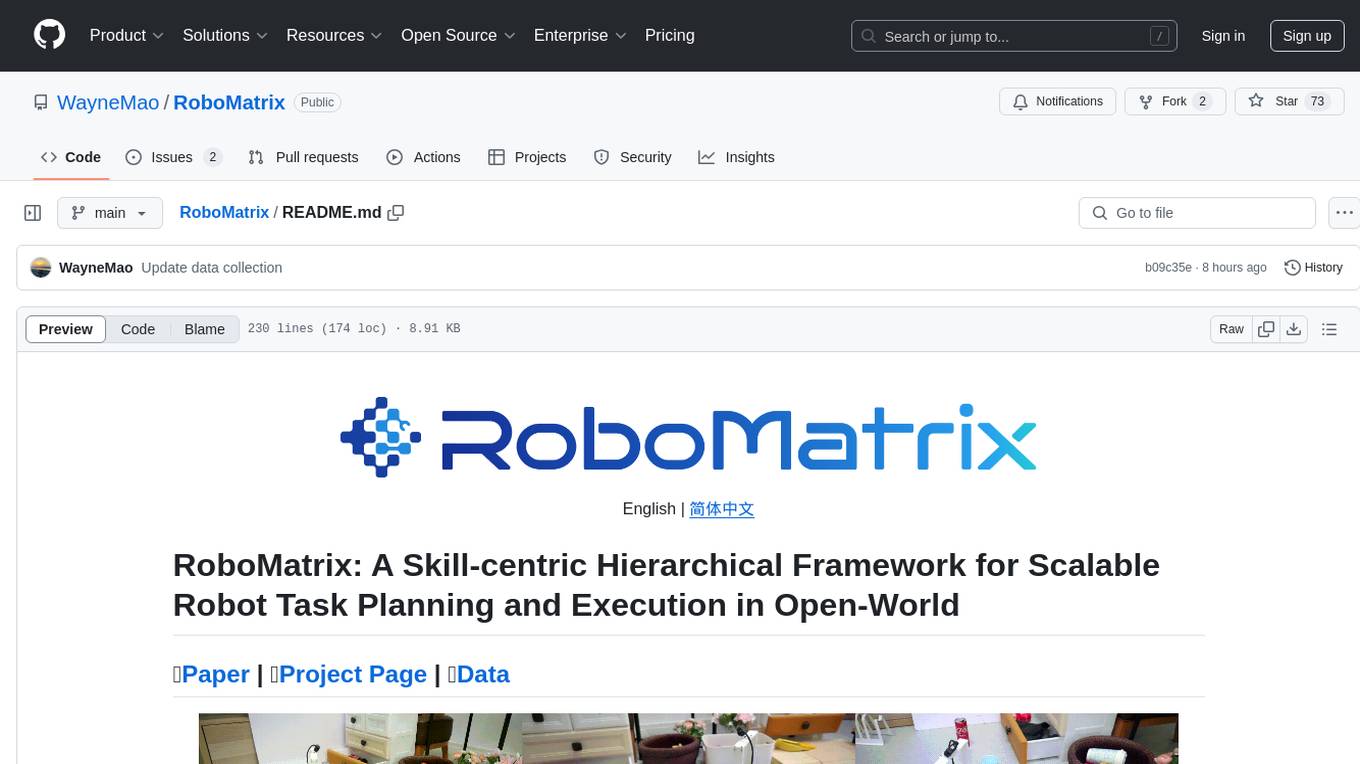
RoboMatrix
RoboMatrix is a skill-centric hierarchical framework for scalable robot task planning and execution in an open-world environment. It provides a structured approach to robot task execution using a combination of hardware components, environment configuration, installation procedures, and data collection methods. The framework is developed using the ROS2 framework on Ubuntu and supports robots from DJI's RoboMaster series. Users can follow the provided installation guidance to set up RoboMatrix and utilize it for various tasks such as data collection, task execution, and dataset construction. The framework also includes a supervised fine-tuning dataset and aims to optimize communication and release additional components in the future.
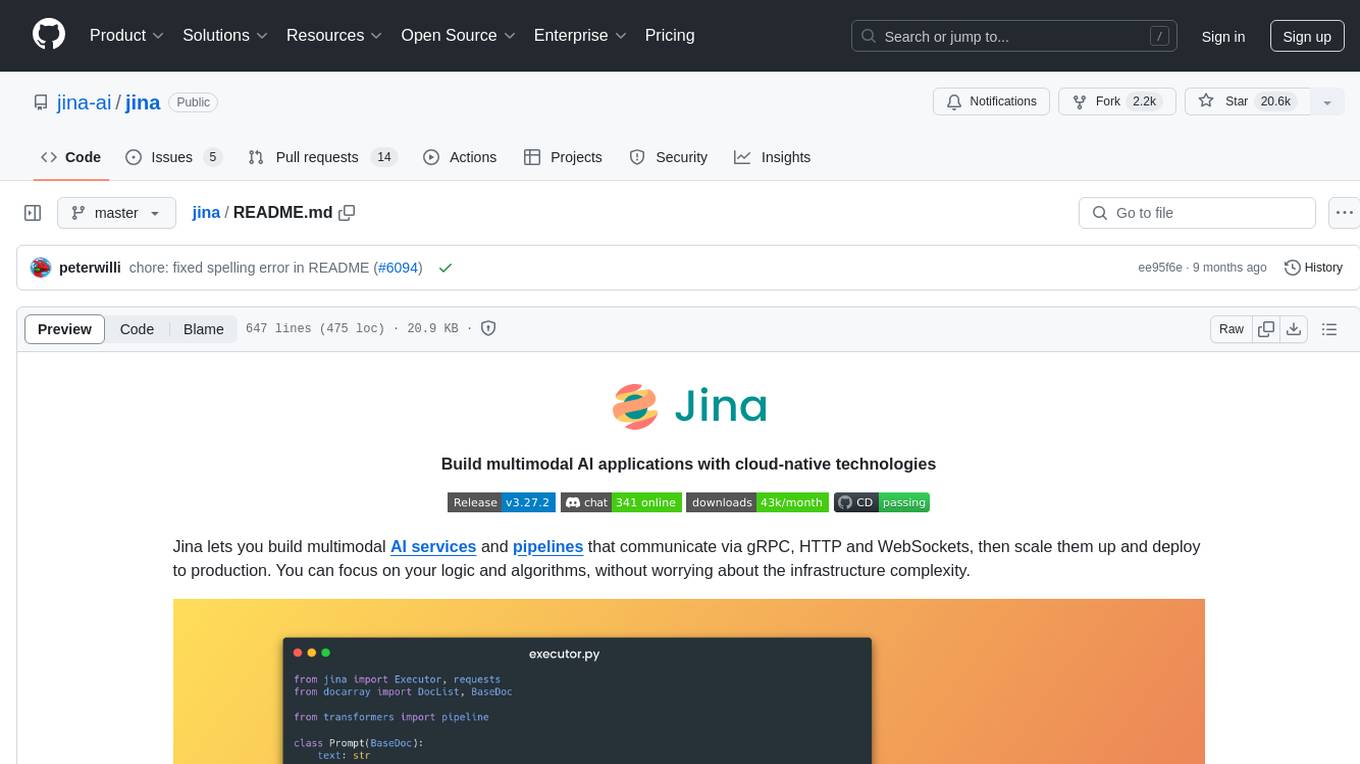
jina
Jina is a tool that allows users to build multimodal AI services and pipelines using cloud-native technologies. It provides a Pythonic experience for serving ML models and transitioning from local deployment to advanced orchestration frameworks like Docker-Compose, Kubernetes, or Jina AI Cloud. Users can build and serve models for any data type and deep learning framework, design high-performance services with easy scaling, serve LLM models while streaming their output, integrate with Docker containers via Executor Hub, and host on CPU/GPU using Jina AI Cloud. Jina also offers advanced orchestration and scaling capabilities, a smooth transition to the cloud, and easy scalability and concurrency features for applications. Users can deploy to their own cloud or system with Kubernetes and Docker Compose integration, and even deploy to JCloud for autoscaling and monitoring.
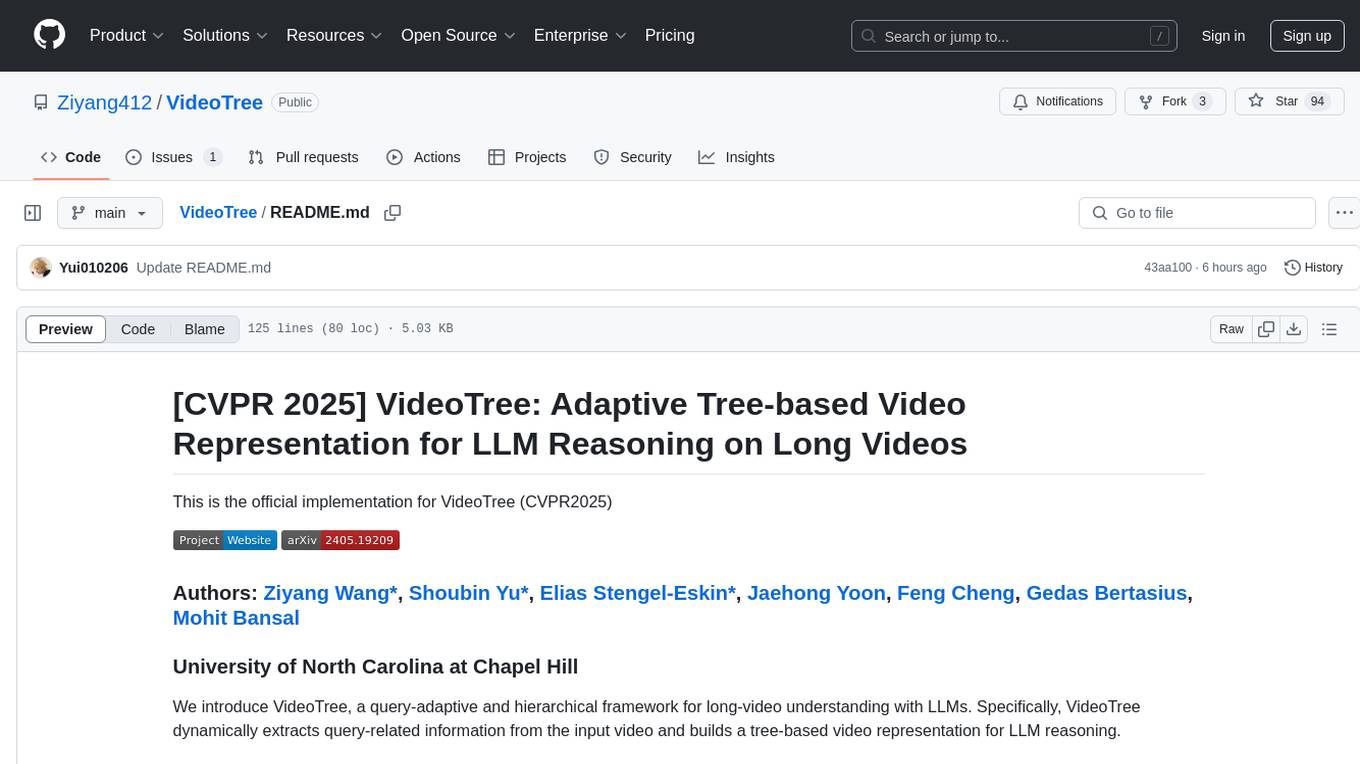
VideoTree
VideoTree is an official implementation for a query-adaptive and hierarchical framework for understanding long videos with LLMs. It dynamically extracts query-related information from input videos and builds a tree-based video representation for LLM reasoning. The tool requires Python 3.8 or above and leverages models like LaViLa and EVA-CLIP-8B for feature extraction. It also provides scripts for tasks like Adaptive Breath Expansion, Relevance-based Depth Expansion, and LLM Reasoning. The codebase is being updated to incorporate scripts/captions for NeXT-QA and IntentQA in the future.
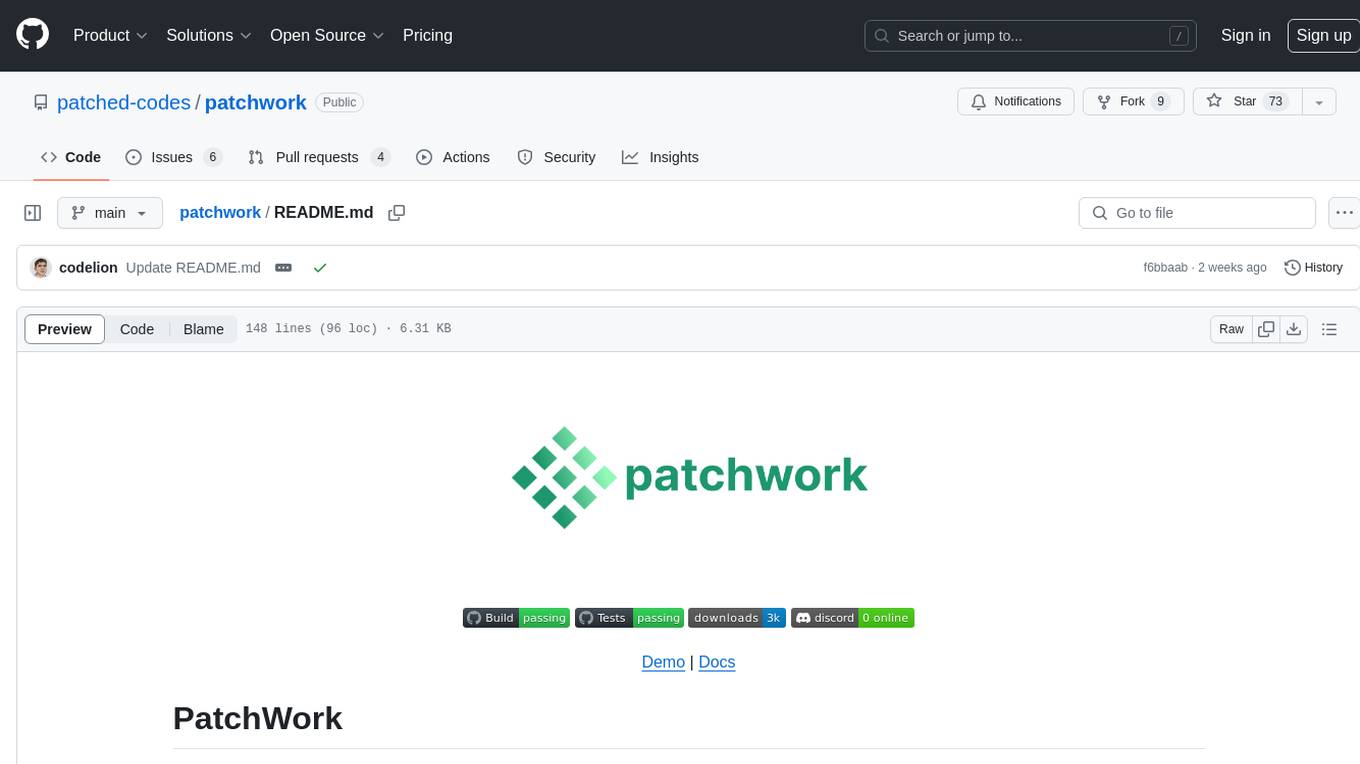
patchwork
PatchWork is an open-source framework designed for automating development tasks using large language models. It enables users to automate workflows such as PR reviews, bug fixing, security patching, and more through a self-hosted CLI agent and preferred LLMs. The framework consists of reusable atomic actions called Steps, customizable LLM prompts known as Prompt Templates, and LLM-assisted automations called Patchflows. Users can run Patchflows locally in their CLI/IDE or as part of CI/CD pipelines. PatchWork offers predefined patchflows like AutoFix, PRReview, GenerateREADME, DependencyUpgrade, and ResolveIssue, with the flexibility to create custom patchflows. Prompt templates are used to pass queries to LLMs and can be customized. Contributions to new patchflows, steps, and the core framework are encouraged, with chat assistants available to aid in the process. The roadmap includes expanding the patchflow library, introducing a debugger and validation module, supporting large-scale code embeddings, parallelization, fine-tuned models, and an open-source GUI. PatchWork is licensed under AGPL-3.0 terms, while custom patchflows and steps can be shared using the Apache-2.0 licensed patchwork template repository.
For similar tasks
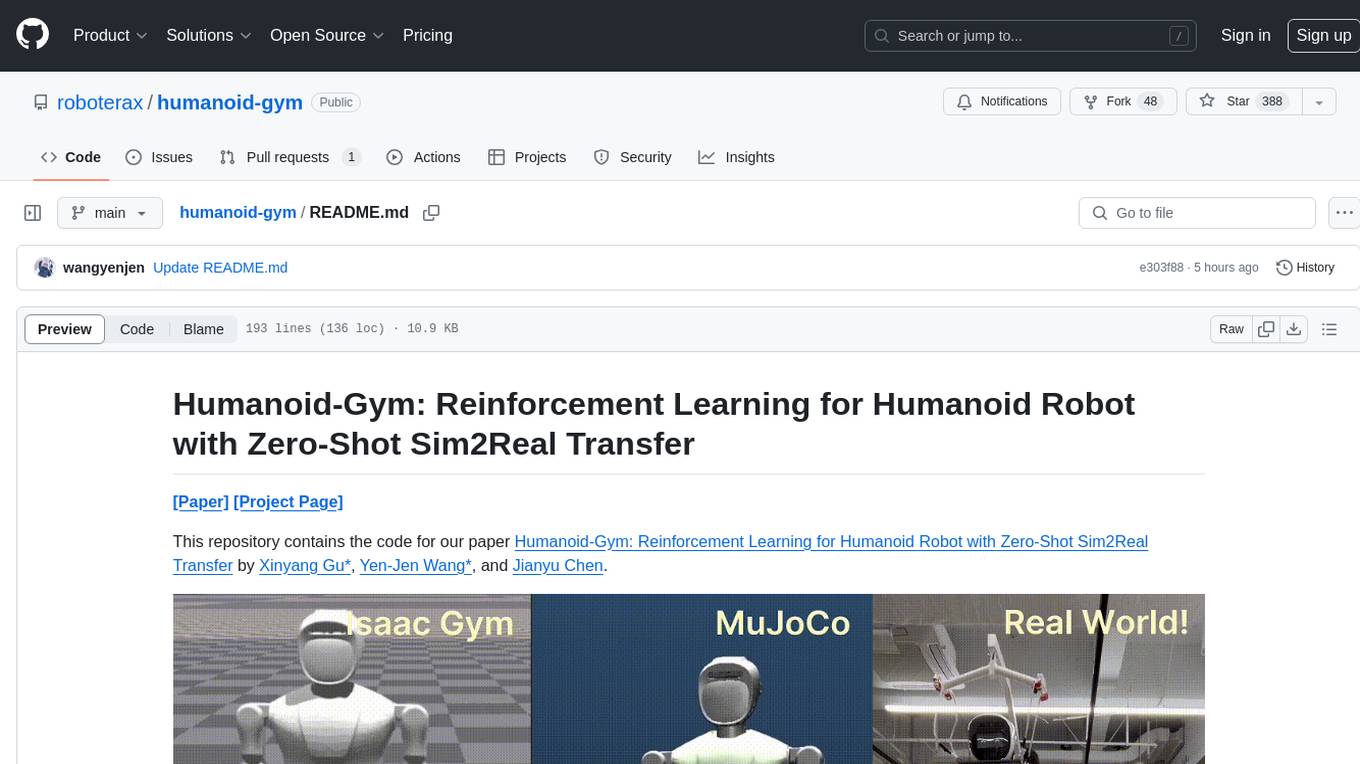
humanoid-gym
Humanoid-Gym is a reinforcement learning framework designed for training locomotion skills for humanoid robots, focusing on zero-shot transfer from simulation to real-world environments. It integrates a sim-to-sim framework from Isaac Gym to Mujoco for verifying trained policies in different physical simulations. The codebase is verified with RobotEra's XBot-S and XBot-L humanoid robots. It offers comprehensive training guidelines, step-by-step configuration instructions, and execution scripts for easy deployment. The sim2sim support allows transferring trained policies to accurate simulated environments. The upcoming features include Denoising World Model Learning and Dexterous Hand Manipulation. Installation and usage guides are provided along with examples for training PPO policies and sim-to-sim transformations. The code structure includes environment and configuration files, with instructions on adding new environments. Troubleshooting tips are provided for common issues, along with a citation and acknowledgment section.
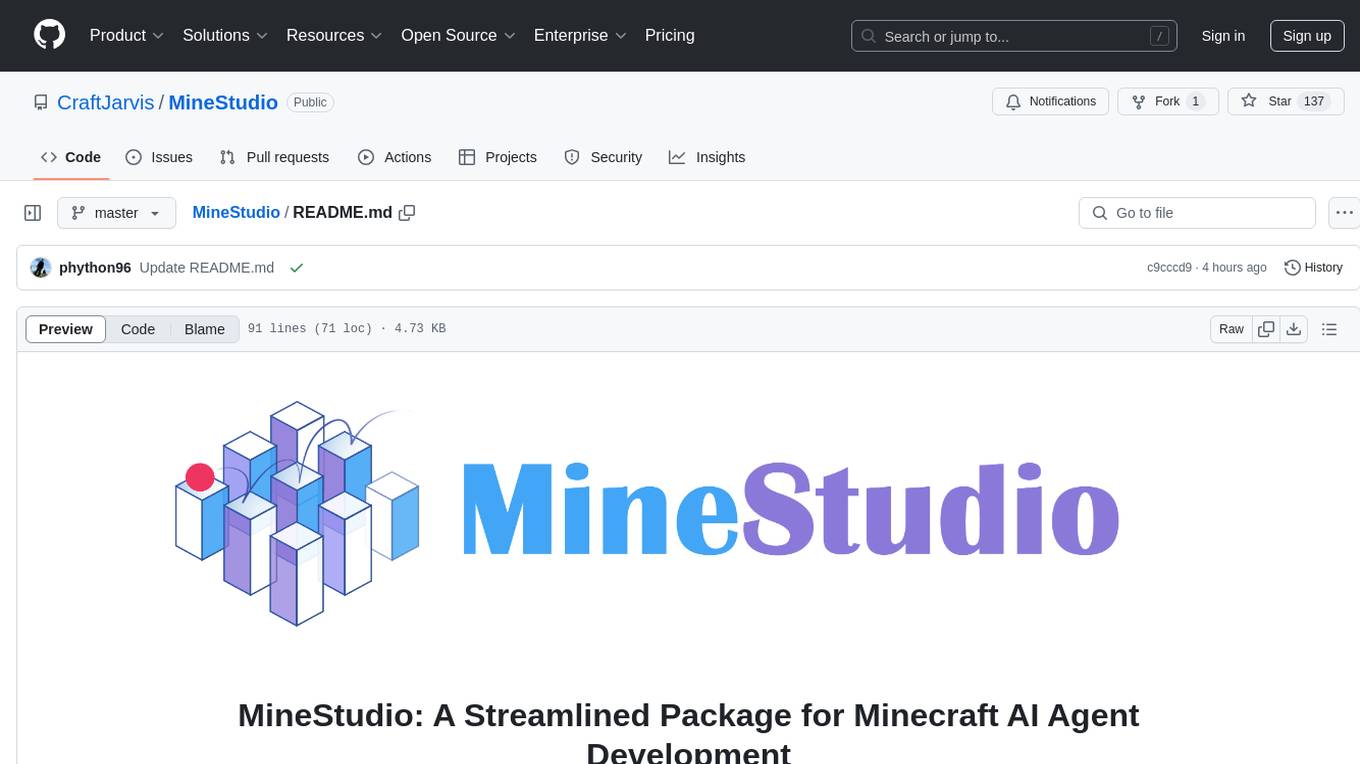
MineStudio
MineStudio is a simple and efficient Minecraft development kit for AI research. It contains tools and APIs for developing Minecraft AI agents, including a customizable simulator, trajectory data structure, policy models, offline and online training pipelines, inference framework, and benchmarking automation. The repository is under development and welcomes contributions and suggestions.
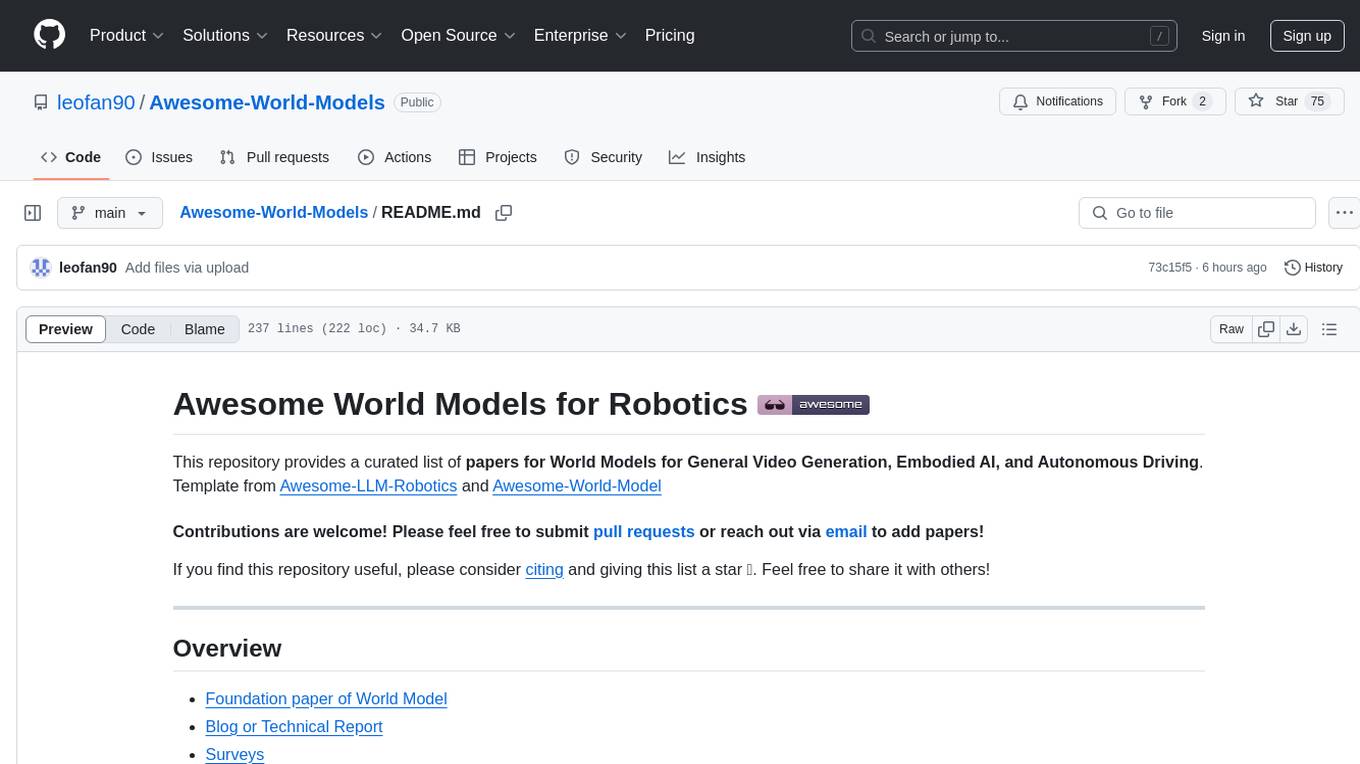
Awesome-World-Models
This repository is a curated list of papers related to World Models for General Video Generation, Embodied AI, and Autonomous Driving. It includes foundation papers, blog posts, technical reports, surveys, benchmarks, and specific world models for different applications. The repository serves as a valuable resource for researchers and practitioners interested in world models and their applications in robotics and AI.
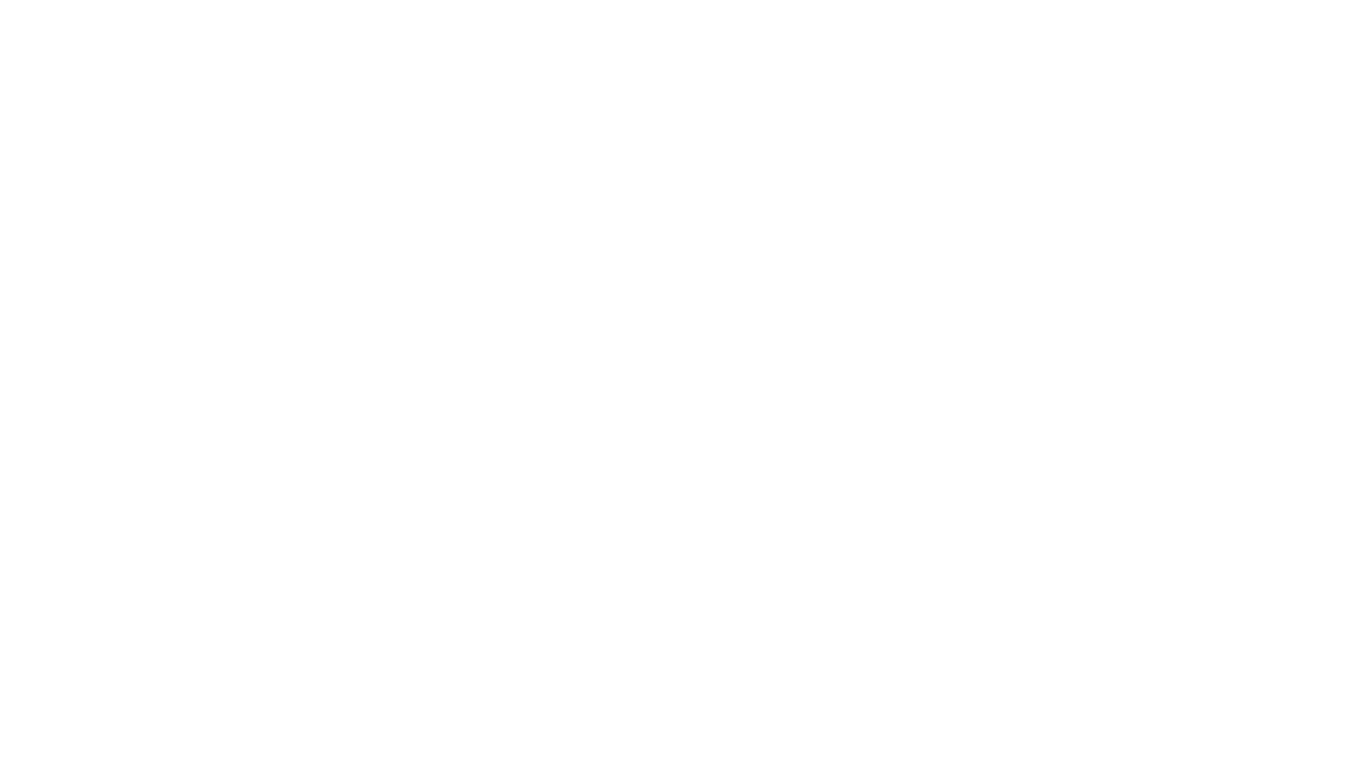
airflow
Apache Airflow (or simply Airflow) is a platform to programmatically author, schedule, and monitor workflows. When workflows are defined as code, they become more maintainable, versionable, testable, and collaborative. Use Airflow to author workflows as directed acyclic graphs (DAGs) of tasks. The Airflow scheduler executes your tasks on an array of workers while following the specified dependencies. Rich command line utilities make performing complex surgeries on DAGs a snap. The rich user interface makes it easy to visualize pipelines running in production, monitor progress, and troubleshoot issues when needed.
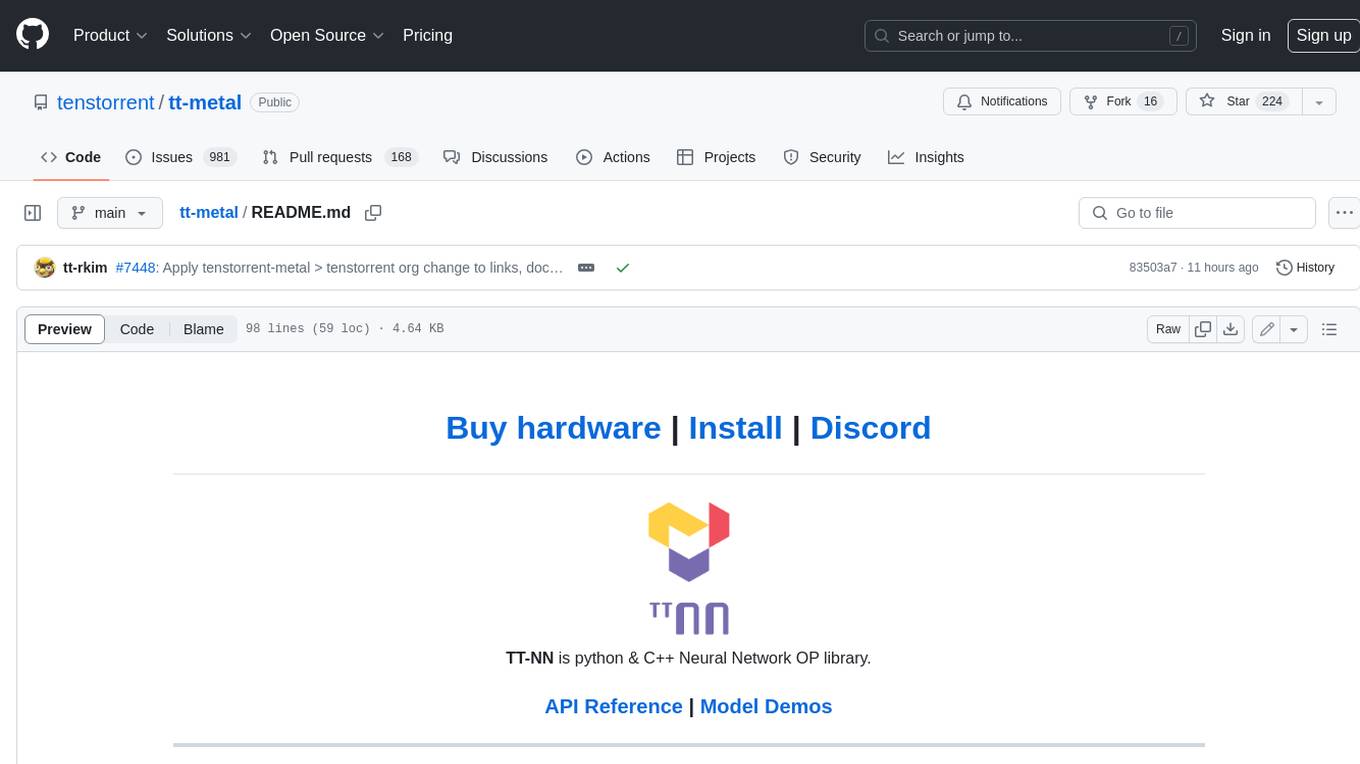
tt-metal
TT-NN is a python & C++ Neural Network OP library. It provides a low-level programming model, TT-Metalium, enabling kernel development for Tenstorrent hardware.
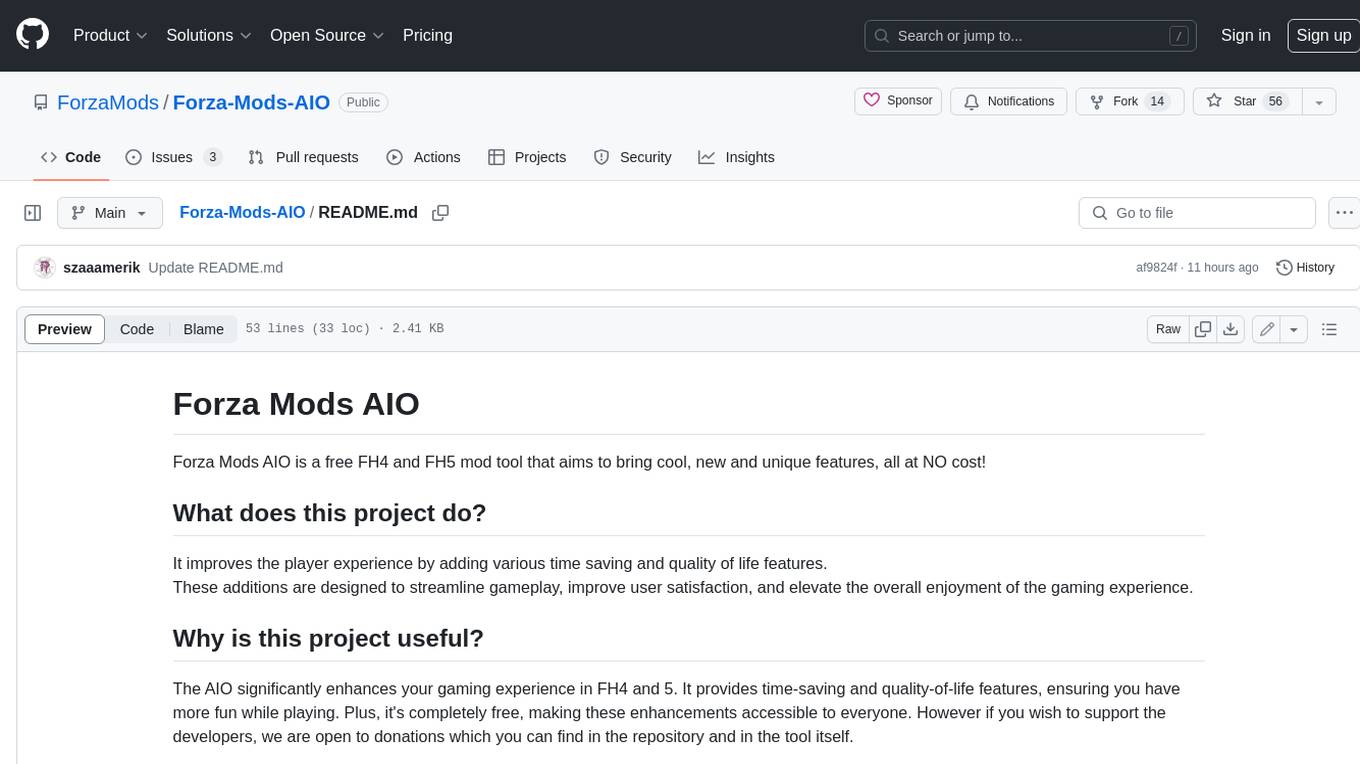
Forza-Mods-AIO
Forza Mods AIO is a free and open-source tool that enhances the gaming experience in Forza Horizon 4 and 5. It offers a range of time-saving and quality-of-life features, making gameplay more enjoyable and efficient. The tool is designed to streamline various aspects of the game, improving user satisfaction and overall enjoyment.
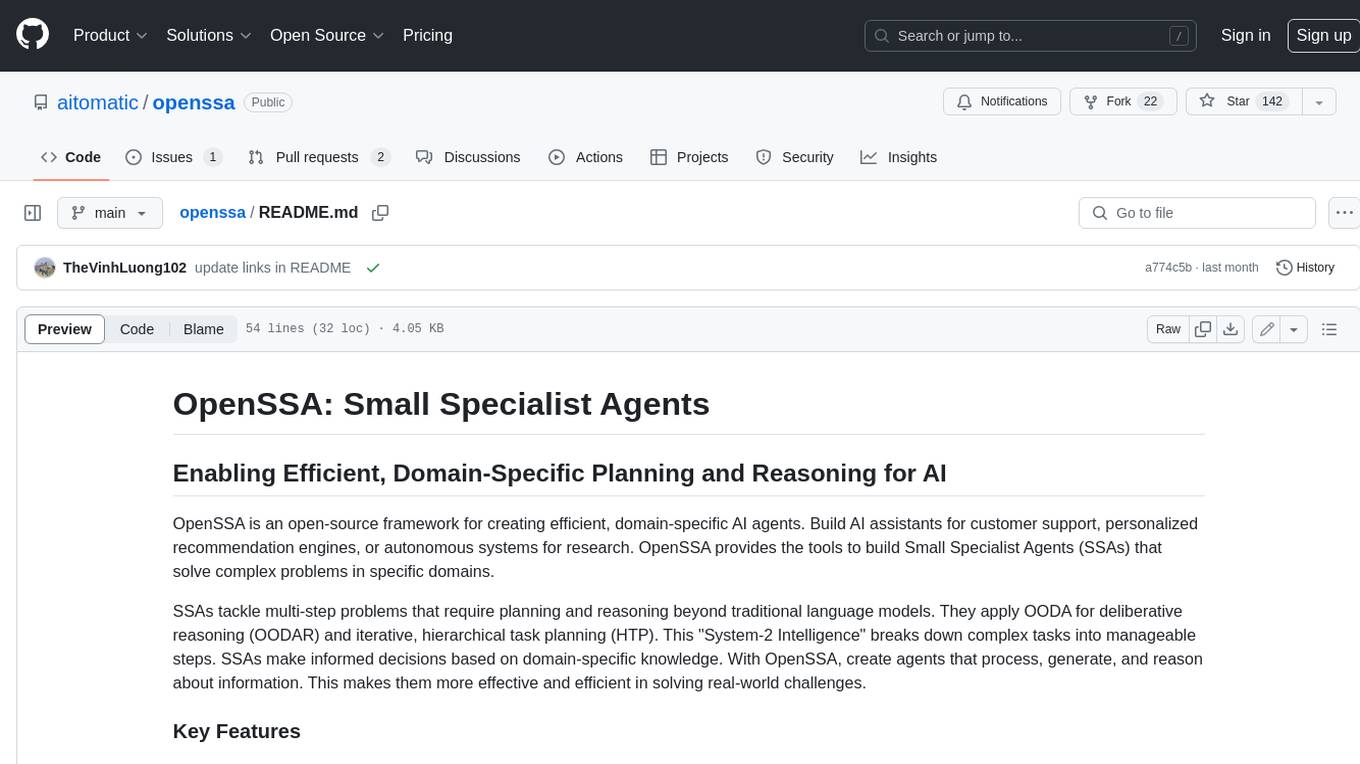
openssa
OpenSSA is an open-source framework for creating efficient, domain-specific AI agents. It enables the development of Small Specialist Agents (SSAs) that solve complex problems in specific domains. SSAs tackle multi-step problems that require planning and reasoning beyond traditional language models. They apply OODA for deliberative reasoning (OODAR) and iterative, hierarchical task planning (HTP). This "System-2 Intelligence" breaks down complex tasks into manageable steps. SSAs make informed decisions based on domain-specific knowledge. With OpenSSA, users can create agents that process, generate, and reason about information, making them more effective and efficient in solving real-world challenges.
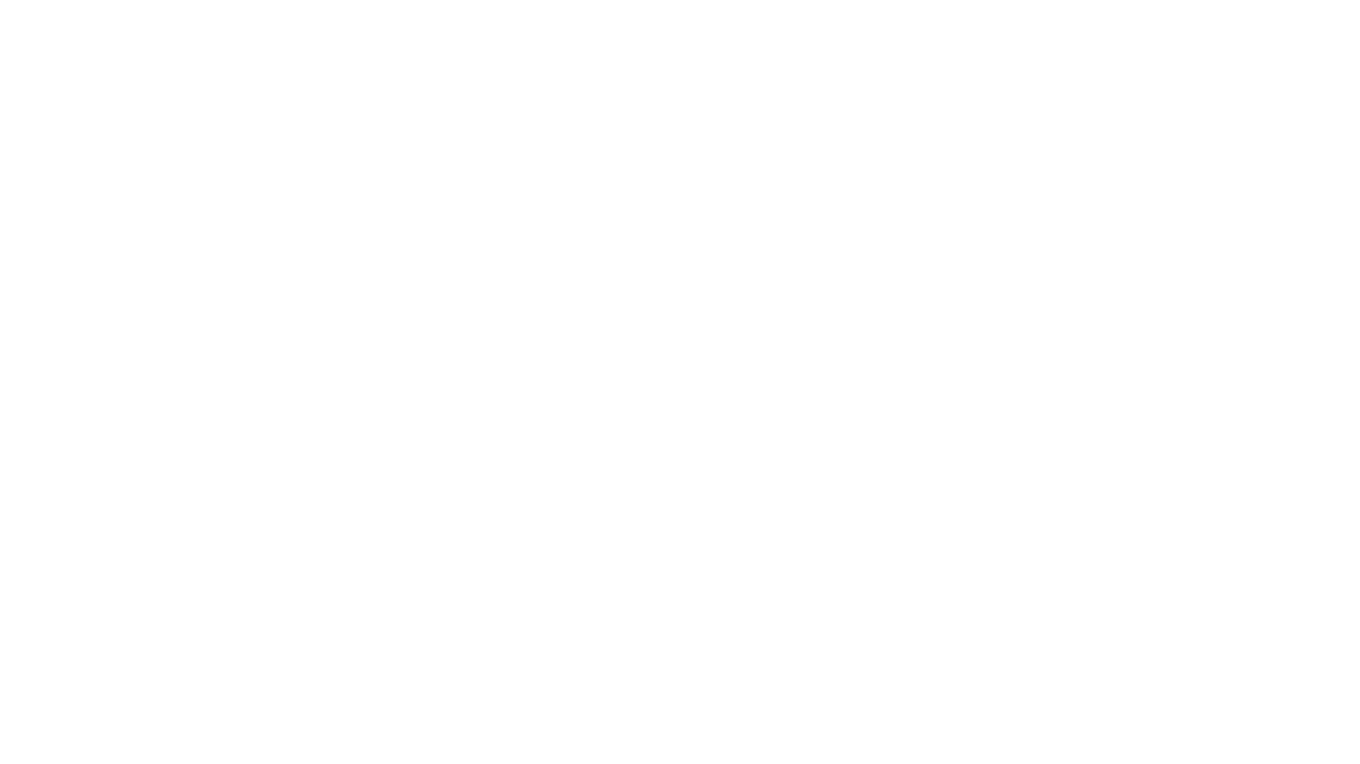
pezzo
Pezzo is a fully cloud-native and open-source LLMOps platform that allows users to observe and monitor AI operations, troubleshoot issues, save costs and latency, collaborate, manage prompts, and deliver AI changes instantly. It supports various clients for prompt management, observability, and caching. Users can run the full Pezzo stack locally using Docker Compose, with prerequisites including Node.js 18+, Docker, and a GraphQL Language Feature Support VSCode Extension. Contributions are welcome, and the source code is available under the Apache 2.0 License.
For similar jobs
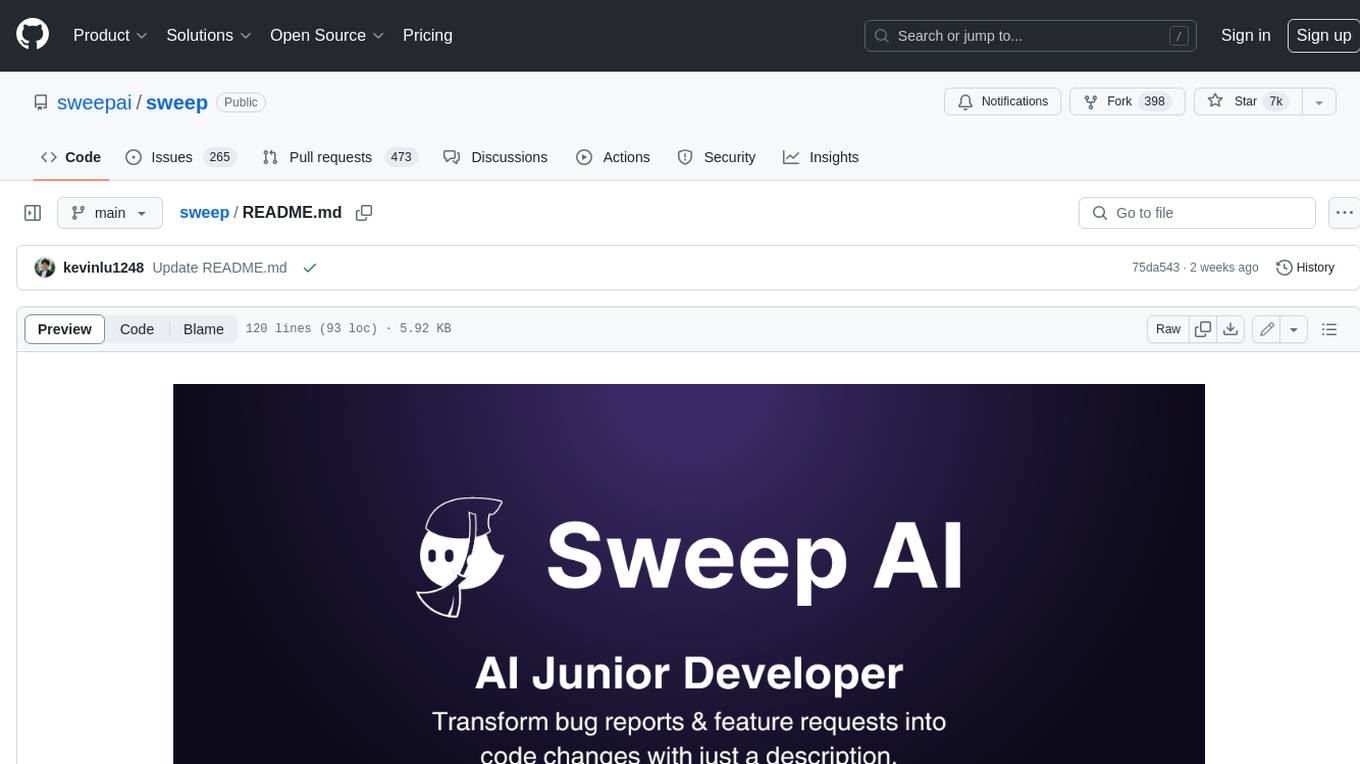
sweep
Sweep is an AI junior developer that turns bugs and feature requests into code changes. It automatically handles developer experience improvements like adding type hints and improving test coverage.
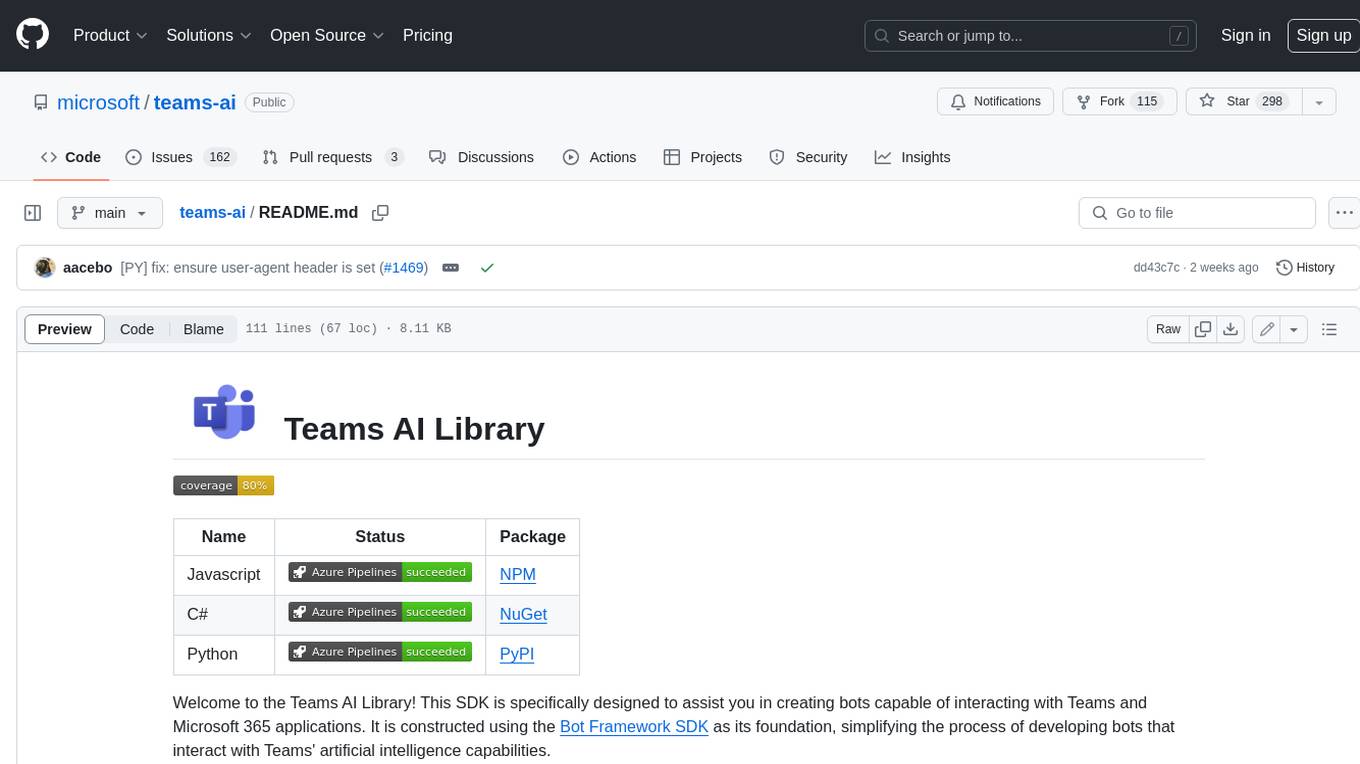
teams-ai
The Teams AI Library is a software development kit (SDK) that helps developers create bots that can interact with Teams and Microsoft 365 applications. It is built on top of the Bot Framework SDK and simplifies the process of developing bots that interact with Teams' artificial intelligence capabilities. The SDK is available for JavaScript/TypeScript, .NET, and Python.
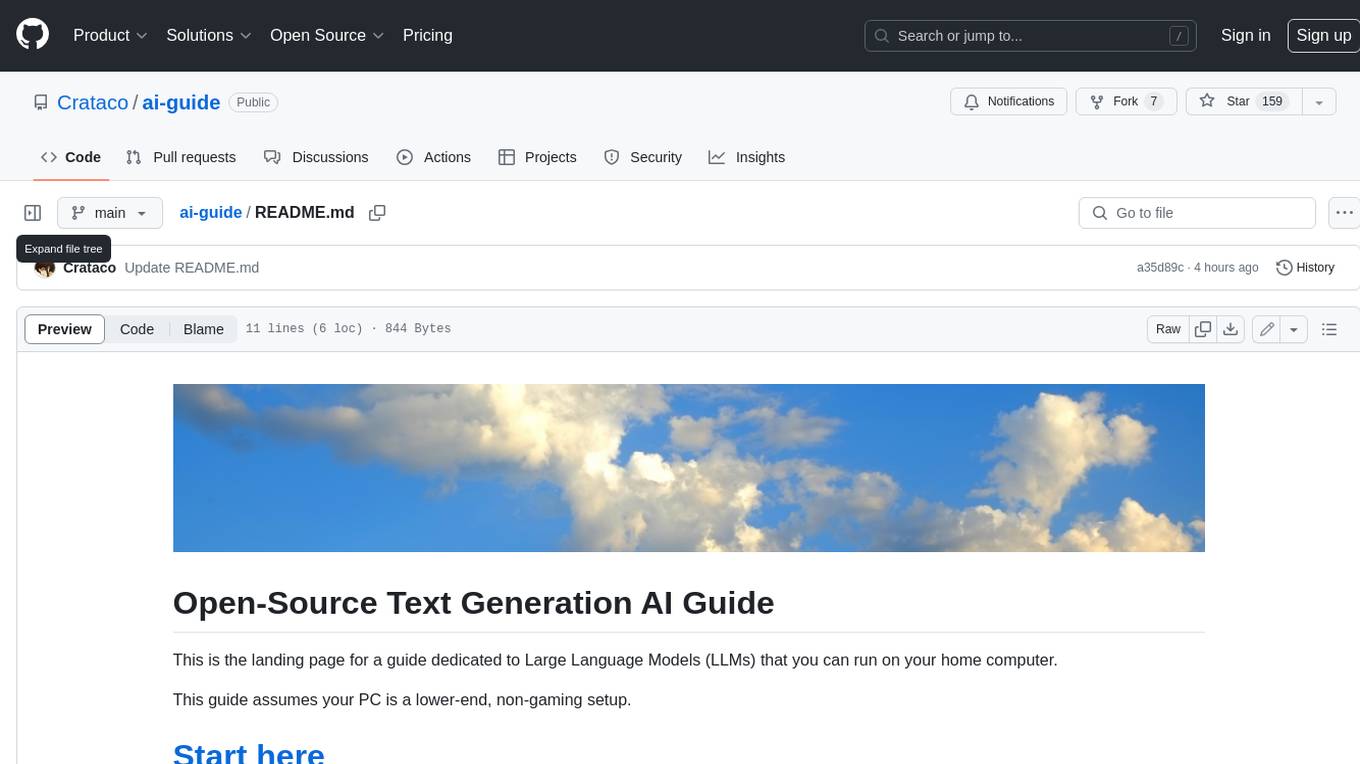
ai-guide
This guide is dedicated to Large Language Models (LLMs) that you can run on your home computer. It assumes your PC is a lower-end, non-gaming setup.
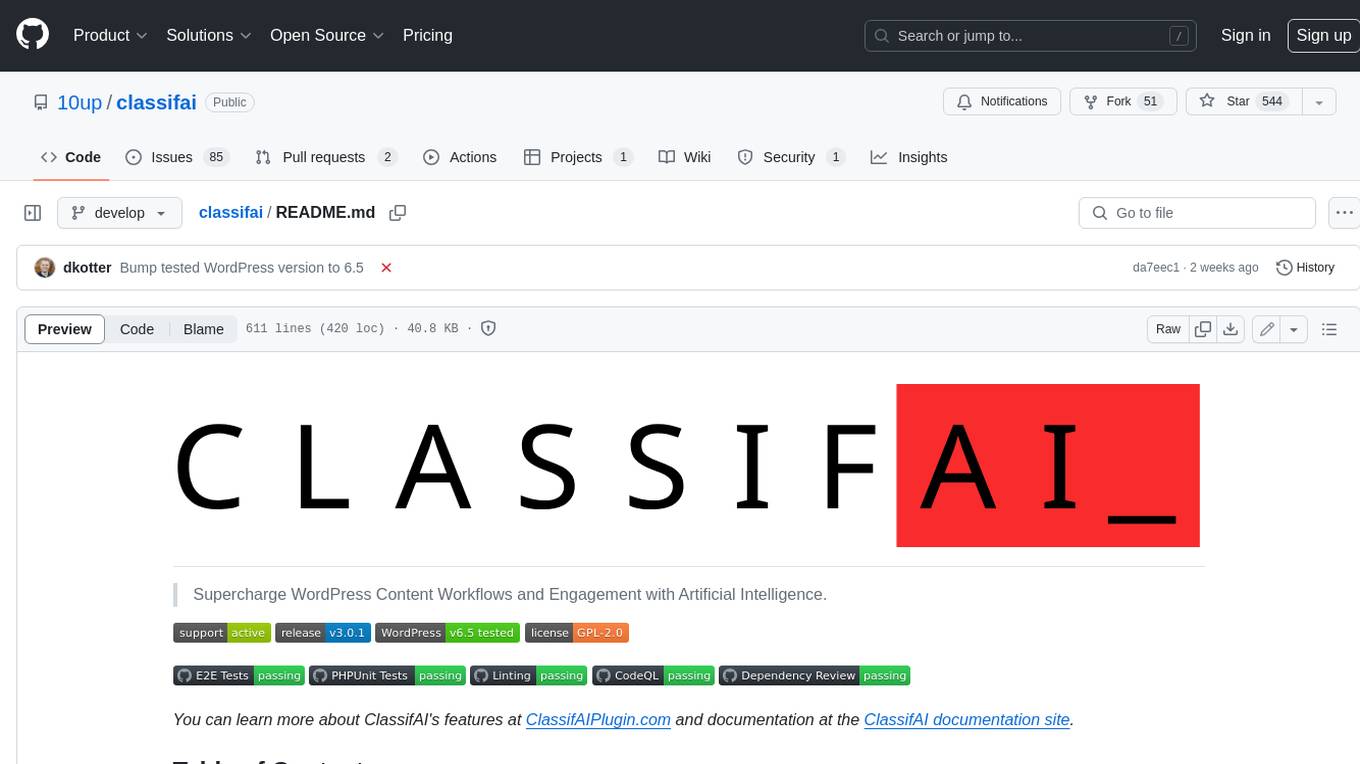
classifai
Supercharge WordPress Content Workflows and Engagement with Artificial Intelligence. Tap into leading cloud-based services like OpenAI, Microsoft Azure AI, Google Gemini and IBM Watson to augment your WordPress-powered websites. Publish content faster while improving SEO performance and increasing audience engagement. ClassifAI integrates Artificial Intelligence and Machine Learning technologies to lighten your workload and eliminate tedious tasks, giving you more time to create original content that matters.
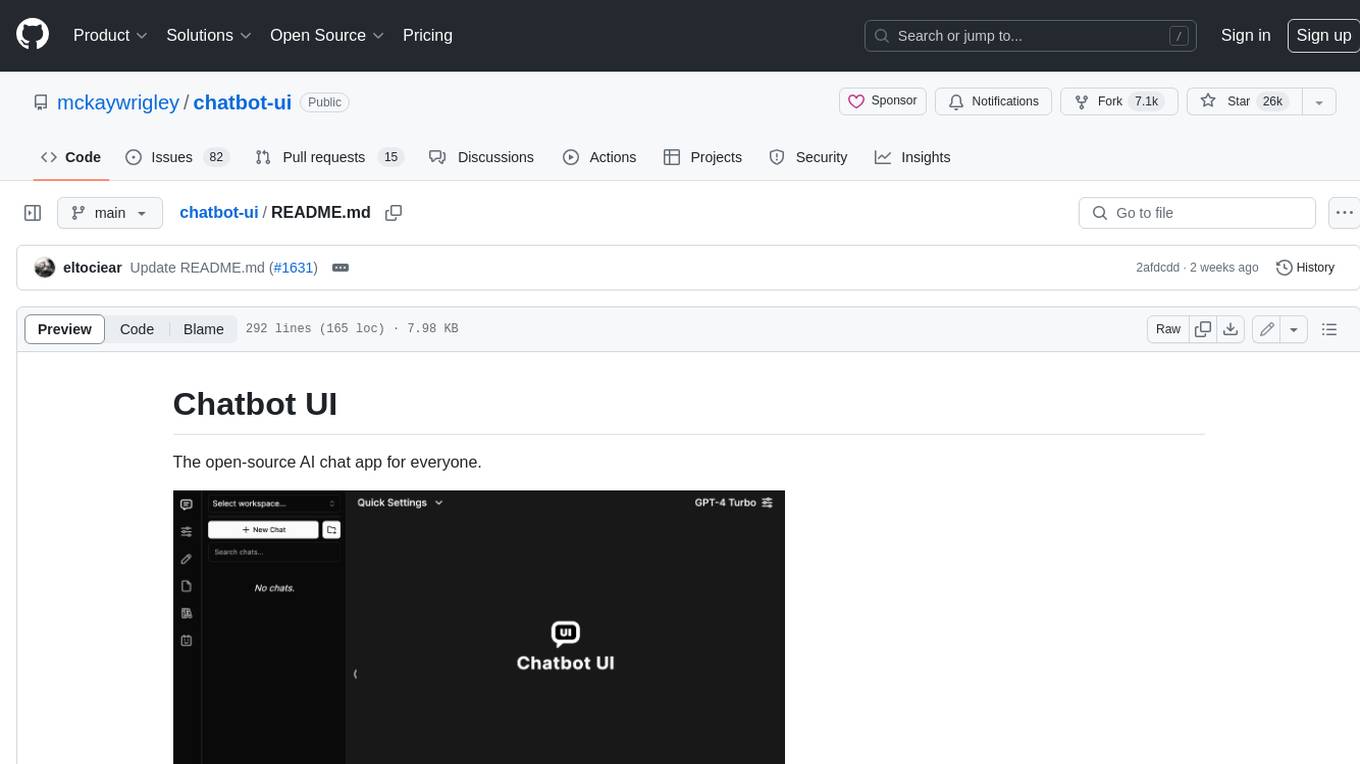
chatbot-ui
Chatbot UI is an open-source AI chat app that allows users to create and deploy their own AI chatbots. It is easy to use and can be customized to fit any need. Chatbot UI is perfect for businesses, developers, and anyone who wants to create a chatbot.
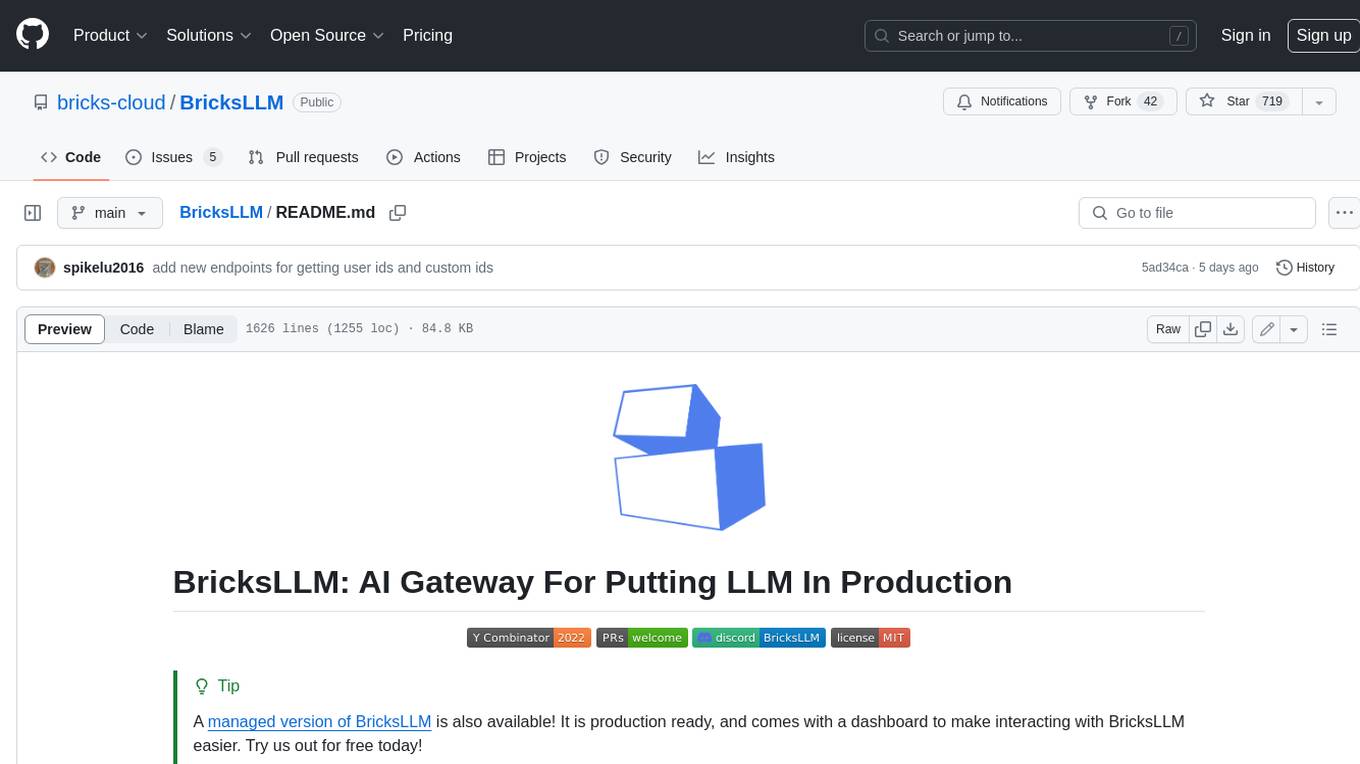
BricksLLM
BricksLLM is a cloud native AI gateway written in Go. Currently, it provides native support for OpenAI, Anthropic, Azure OpenAI and vLLM. BricksLLM aims to provide enterprise level infrastructure that can power any LLM production use cases. Here are some use cases for BricksLLM: * Set LLM usage limits for users on different pricing tiers * Track LLM usage on a per user and per organization basis * Block or redact requests containing PIIs * Improve LLM reliability with failovers, retries and caching * Distribute API keys with rate limits and cost limits for internal development/production use cases * Distribute API keys with rate limits and cost limits for students
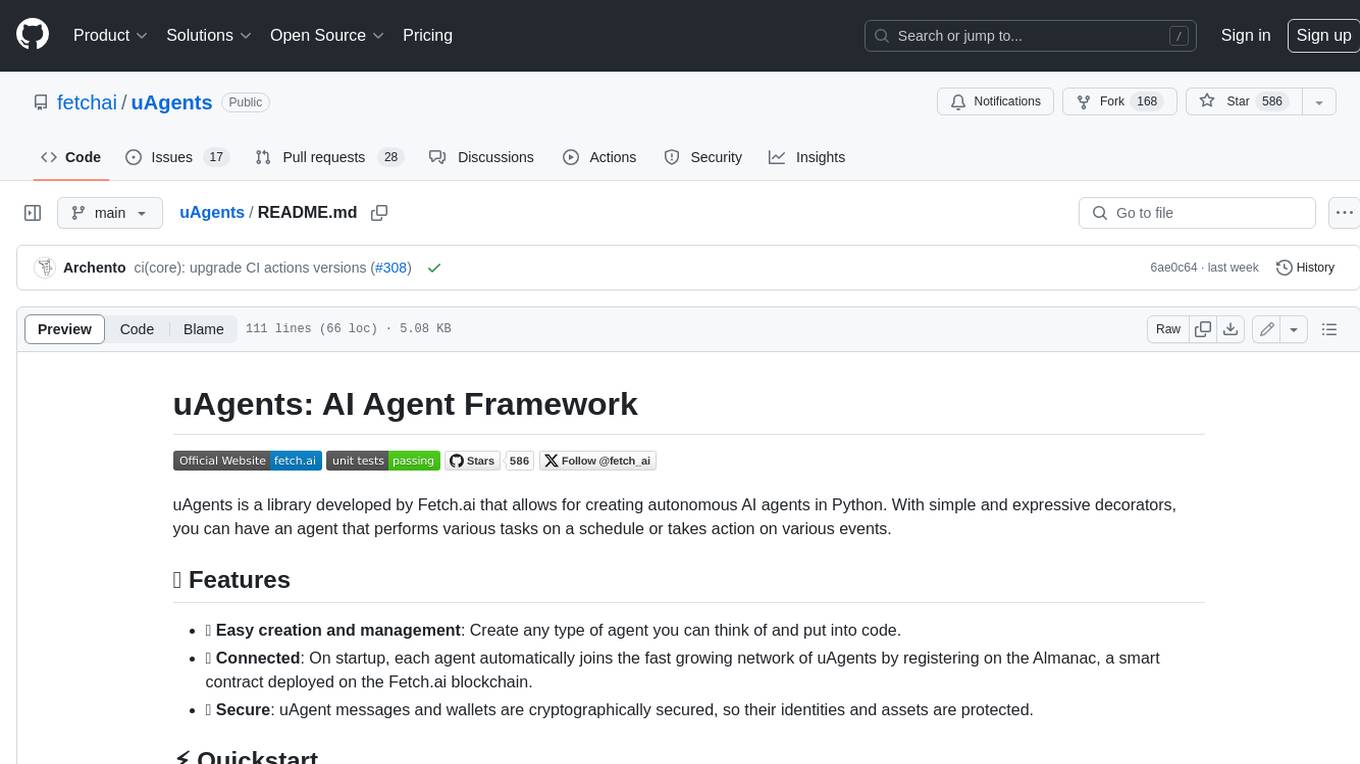
uAgents
uAgents is a Python library developed by Fetch.ai that allows for the creation of autonomous AI agents. These agents can perform various tasks on a schedule or take action on various events. uAgents are easy to create and manage, and they are connected to a fast-growing network of other uAgents. They are also secure, with cryptographically secured messages and wallets.
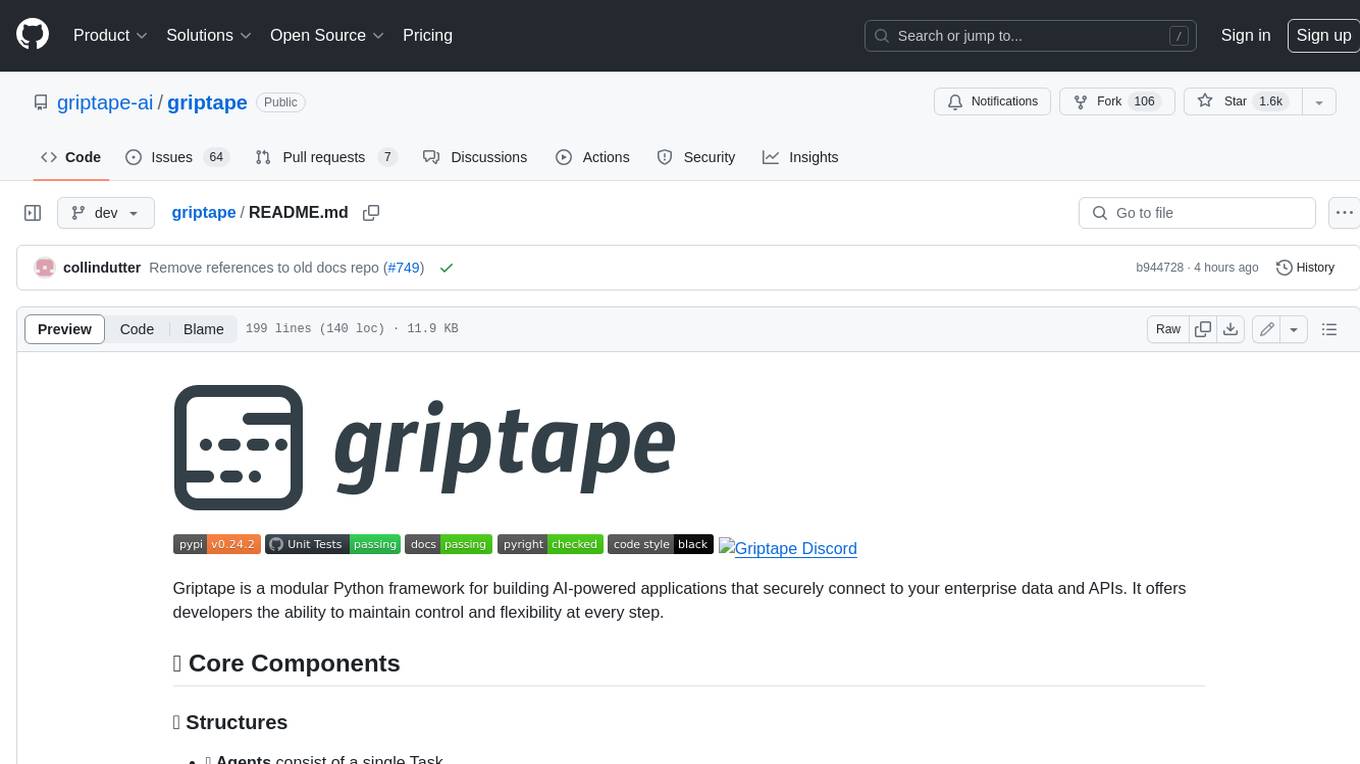
griptape
Griptape is a modular Python framework for building AI-powered applications that securely connect to your enterprise data and APIs. It offers developers the ability to maintain control and flexibility at every step. Griptape's core components include Structures (Agents, Pipelines, and Workflows), Tasks, Tools, Memory (Conversation Memory, Task Memory, and Meta Memory), Drivers (Prompt and Embedding Drivers, Vector Store Drivers, Image Generation Drivers, Image Query Drivers, SQL Drivers, Web Scraper Drivers, and Conversation Memory Drivers), Engines (Query Engines, Extraction Engines, Summary Engines, Image Generation Engines, and Image Query Engines), and additional components (Rulesets, Loaders, Artifacts, Chunkers, and Tokenizers). Griptape enables developers to create AI-powered applications with ease and efficiency.