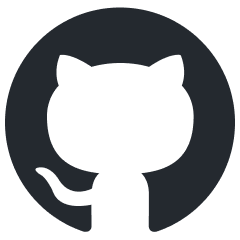
llm-compressor
Transformers-compatible library for applying various compression algorithms to LLMs for optimized deployment with vLLM
Stars: 1164
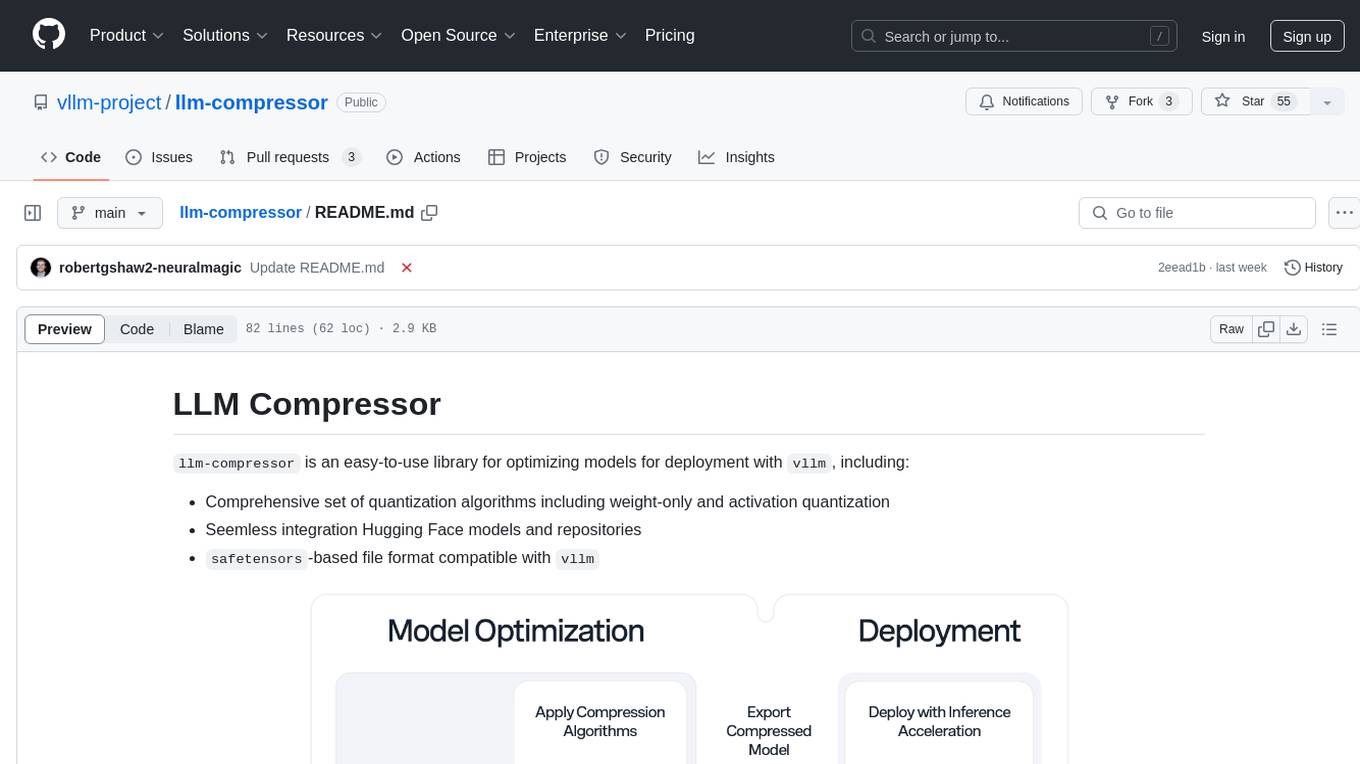
llm-compressor is an easy-to-use library for optimizing models for deployment with vllm. It provides a comprehensive set of quantization algorithms, seamless integration with Hugging Face models and repositories, and supports mixed precision, activation quantization, and sparsity. Supported algorithms include PTQ, GPTQ, SmoothQuant, and SparseGPT. Installation can be done via git clone and local pip install. Compression can be easily applied by selecting an algorithm and calling the oneshot API. The library also offers end-to-end examples for model compression. Contributions to the code, examples, integrations, and documentation are appreciated.
README:
llmcompressor
is an easy-to-use library for optimizing models for deployment with vllm
, including:
- Comprehensive set of quantization algorithms for weight-only and activation quantization
- Seamless integration with Hugging Face models and repositories
-
safetensors
-based file format compatible withvllm
- Large model support via
accelerate
✨ Read the announcement blog here! ✨
- Activation Quantization: W8A8 (int8 and fp8)
- Mixed Precision: W4A16, W8A16
- 2:4 Semi-structured and Unstructured Sparsity
- Simple PTQ
- GPTQ
- SmoothQuant
- SparseGPT
Please refer to docs/schemes.md for detailed information about available optimization schemes and their use cases.
pip install llmcompressor
Applying quantization with llmcompressor
:
- Activation quantization to
int8
- Activation quantization to
fp8
- Weight only quantization to
int4
- Quantizing MoE LLMs
- Quantizing Vision-Language Models
- Quantizing Audio-Language Models
Deep dives into advanced usage of llmcompressor
:
Let's quantize TinyLlama
with 8 bit weights and activations using the GPTQ
and SmoothQuant
algorithms.
Note that the model can be swapped for a local or remote HF-compatible checkpoint and the recipe
may be changed to target different quantization algorithms or formats.
Quantization is applied by selecting an algorithm and calling the oneshot
API.
from llmcompressor.modifiers.smoothquant import SmoothQuantModifier
from llmcompressor.modifiers.quantization import GPTQModifier
from llmcompressor import oneshot
# Select quantization algorithm. In this case, we:
# * apply SmoothQuant to make the activations easier to quantize
# * quantize the weights to int8 with GPTQ (static per channel)
# * quantize the activations to int8 (dynamic per token)
recipe = [
SmoothQuantModifier(smoothing_strength=0.8),
GPTQModifier(scheme="W8A8", targets="Linear", ignore=["lm_head"]),
]
# Apply quantization using the built in open_platypus dataset.
# * See examples for demos showing how to pass a custom calibration set
oneshot(
model="TinyLlama/TinyLlama-1.1B-Chat-v1.0",
dataset="open_platypus",
recipe=recipe,
output_dir="TinyLlama-1.1B-Chat-v1.0-INT8",
max_seq_length=2048,
num_calibration_samples=512,
)
The checkpoints created by llmcompressor
can be loaded and run in vllm
:
Install:
pip install vllm
Run:
from vllm import LLM
model = LLM("TinyLlama-1.1B-Chat-v1.0-INT8")
output = model.generate("My name is")
- If you have any questions or requests open an issue and we will add an example or documentation.
- We appreciate contributions to the code, examples, integrations, and documentation as well as bug reports and feature requests! Learn how here.
For Tasks:
Click tags to check more tools for each tasksFor Jobs:
Alternative AI tools for llm-compressor
Similar Open Source Tools
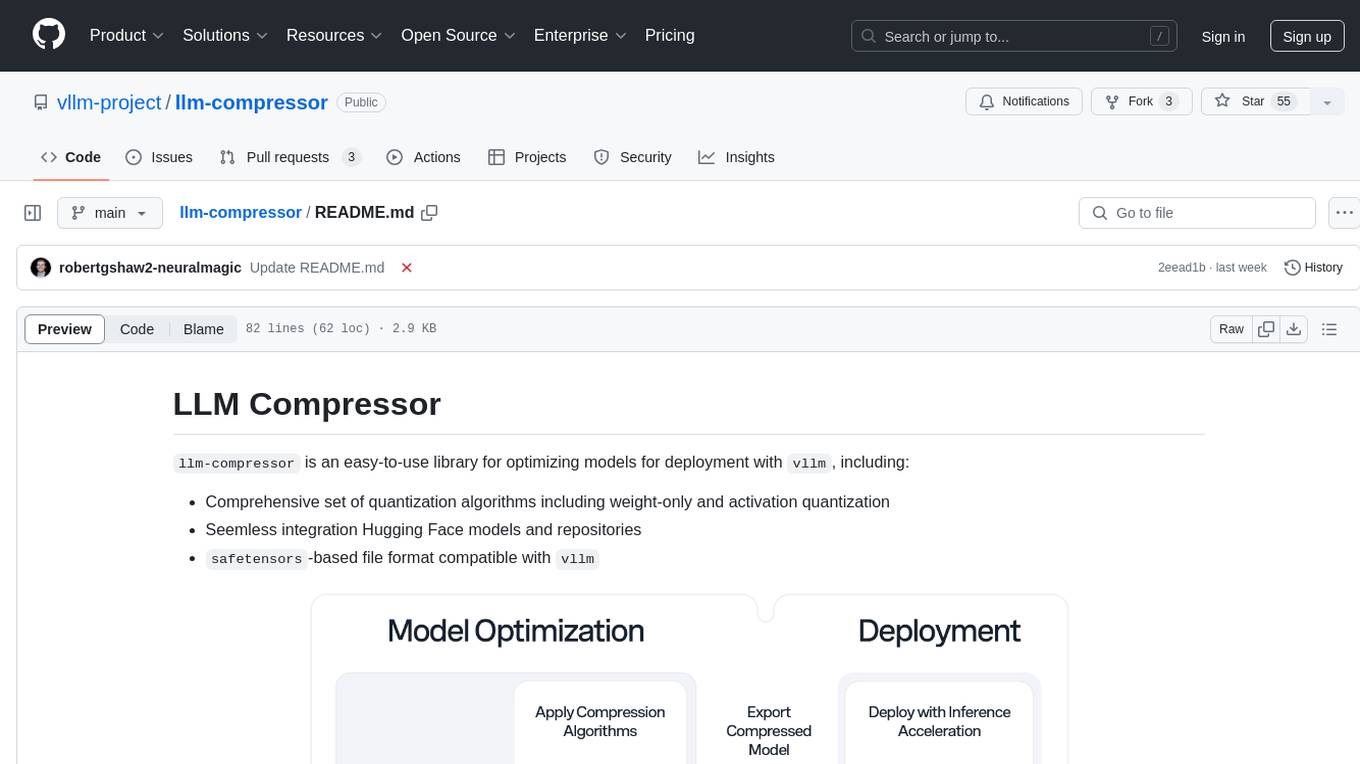
llm-compressor
llm-compressor is an easy-to-use library for optimizing models for deployment with vllm. It provides a comprehensive set of quantization algorithms, seamless integration with Hugging Face models and repositories, and supports mixed precision, activation quantization, and sparsity. Supported algorithms include PTQ, GPTQ, SmoothQuant, and SparseGPT. Installation can be done via git clone and local pip install. Compression can be easily applied by selecting an algorithm and calling the oneshot API. The library also offers end-to-end examples for model compression. Contributions to the code, examples, integrations, and documentation are appreciated.
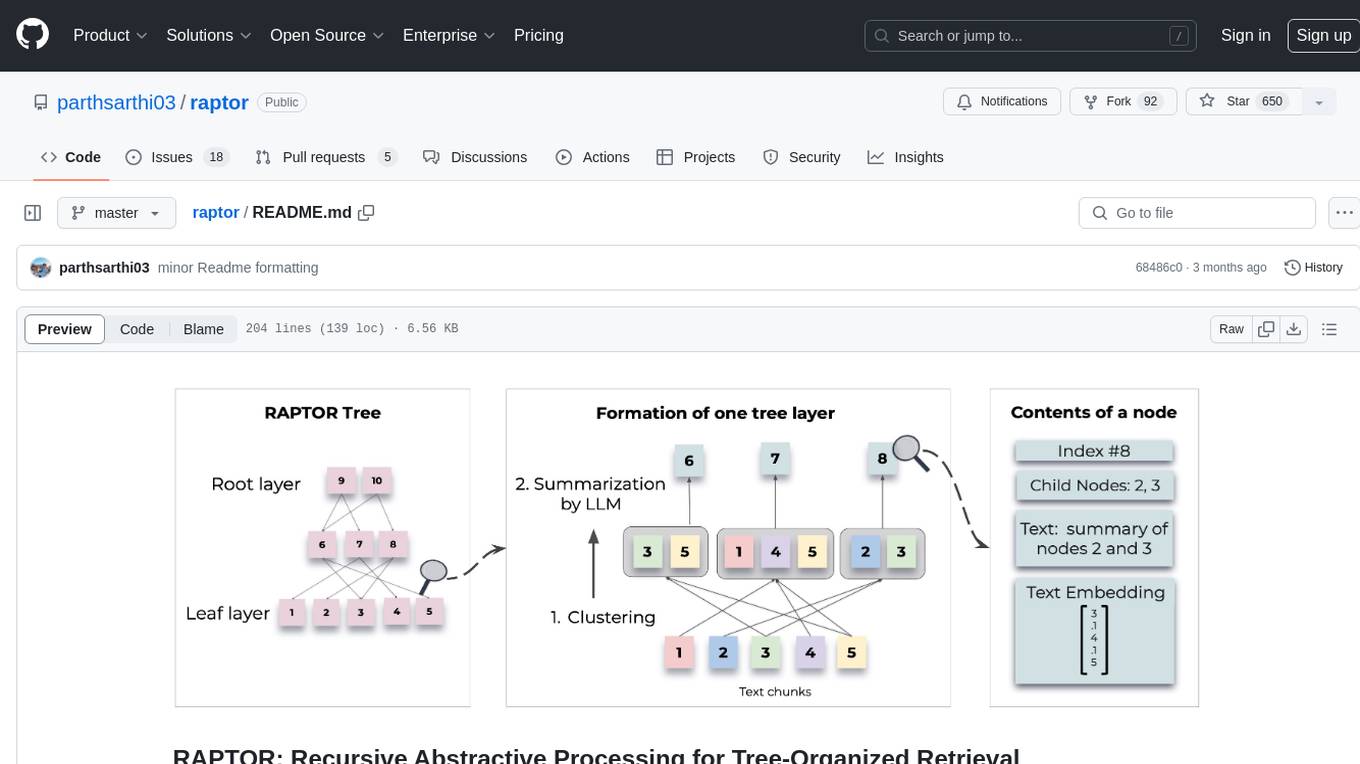
raptor
RAPTOR introduces a novel approach to retrieval-augmented language models by constructing a recursive tree structure from documents. This allows for more efficient and context-aware information retrieval across large texts, addressing common limitations in traditional language models. Users can add documents to the tree, answer questions based on indexed documents, save and load the tree, and extend RAPTOR with custom summarization, question-answering, and embedding models. The tool is designed to be flexible and customizable for various NLP tasks.
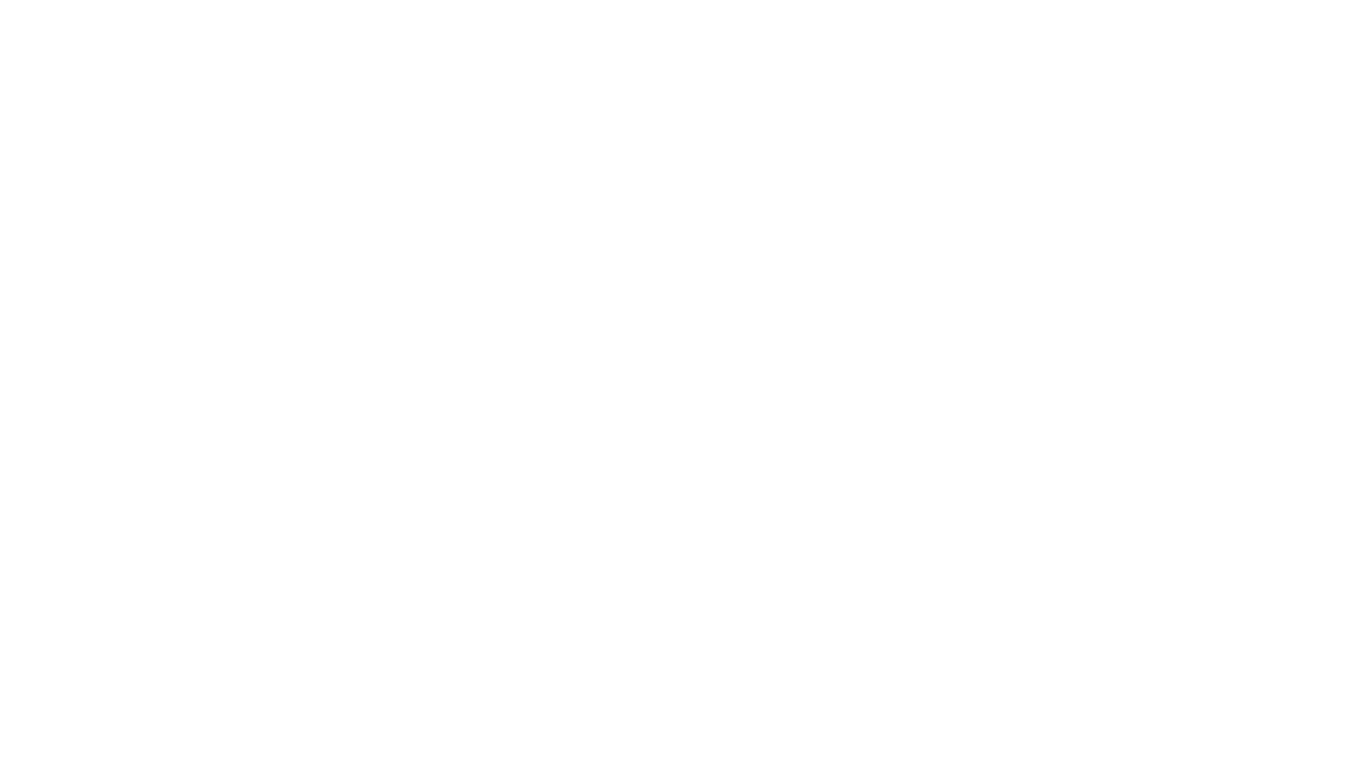
shellChatGPT
ShellChatGPT is a shell wrapper for OpenAI's ChatGPT, DALL-E, Whisper, and TTS, featuring integration with LocalAI, Ollama, Gemini, Mistral, Groq, and GitHub Models. It provides text and chat completions, vision, reasoning, and audio models, voice-in and voice-out chatting mode, text editor interface, markdown rendering support, session management, instruction prompt manager, integration with various service providers, command line completion, file picker dialogs, color scheme personalization, stdin and text file input support, and compatibility with Linux, FreeBSD, MacOS, and Termux for a responsive experience.
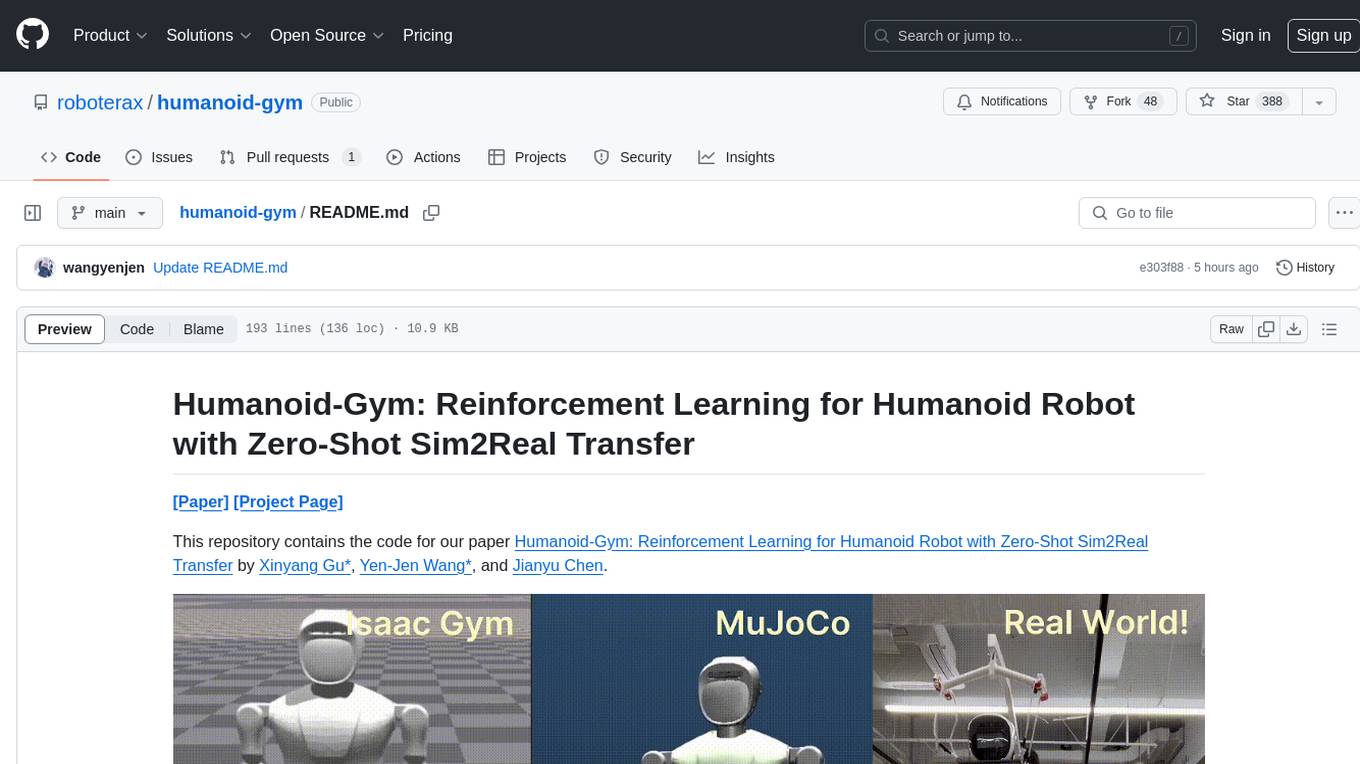
humanoid-gym
Humanoid-Gym is a reinforcement learning framework designed for training locomotion skills for humanoid robots, focusing on zero-shot transfer from simulation to real-world environments. It integrates a sim-to-sim framework from Isaac Gym to Mujoco for verifying trained policies in different physical simulations. The codebase is verified with RobotEra's XBot-S and XBot-L humanoid robots. It offers comprehensive training guidelines, step-by-step configuration instructions, and execution scripts for easy deployment. The sim2sim support allows transferring trained policies to accurate simulated environments. The upcoming features include Denoising World Model Learning and Dexterous Hand Manipulation. Installation and usage guides are provided along with examples for training PPO policies and sim-to-sim transformations. The code structure includes environment and configuration files, with instructions on adding new environments. Troubleshooting tips are provided for common issues, along with a citation and acknowledgment section.
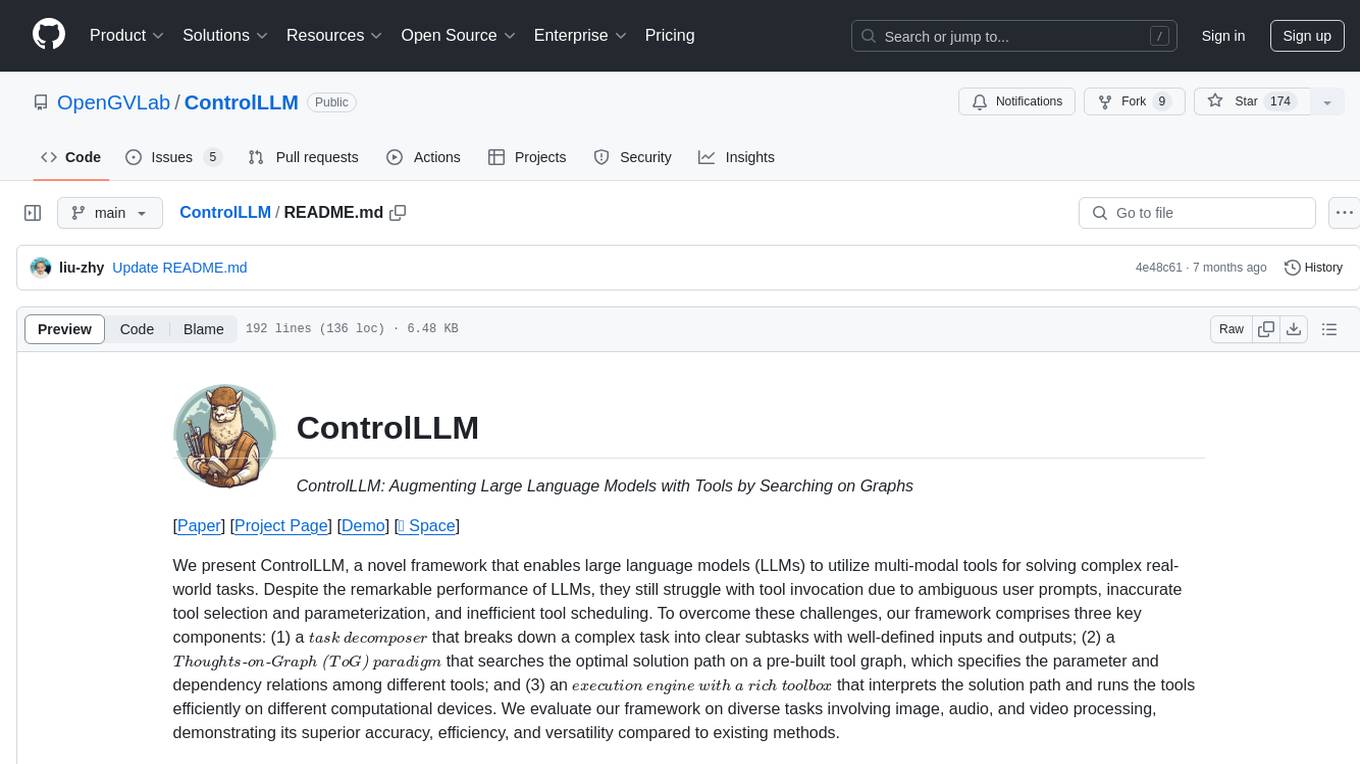
ControlLLM
ControlLLM is a framework that empowers large language models to leverage multi-modal tools for solving complex real-world tasks. It addresses challenges like ambiguous user prompts, inaccurate tool selection, and inefficient tool scheduling by utilizing a task decomposer, a Thoughts-on-Graph paradigm, and an execution engine with a rich toolbox. The framework excels in tasks involving image, audio, and video processing, showcasing superior accuracy, efficiency, and versatility compared to existing methods.
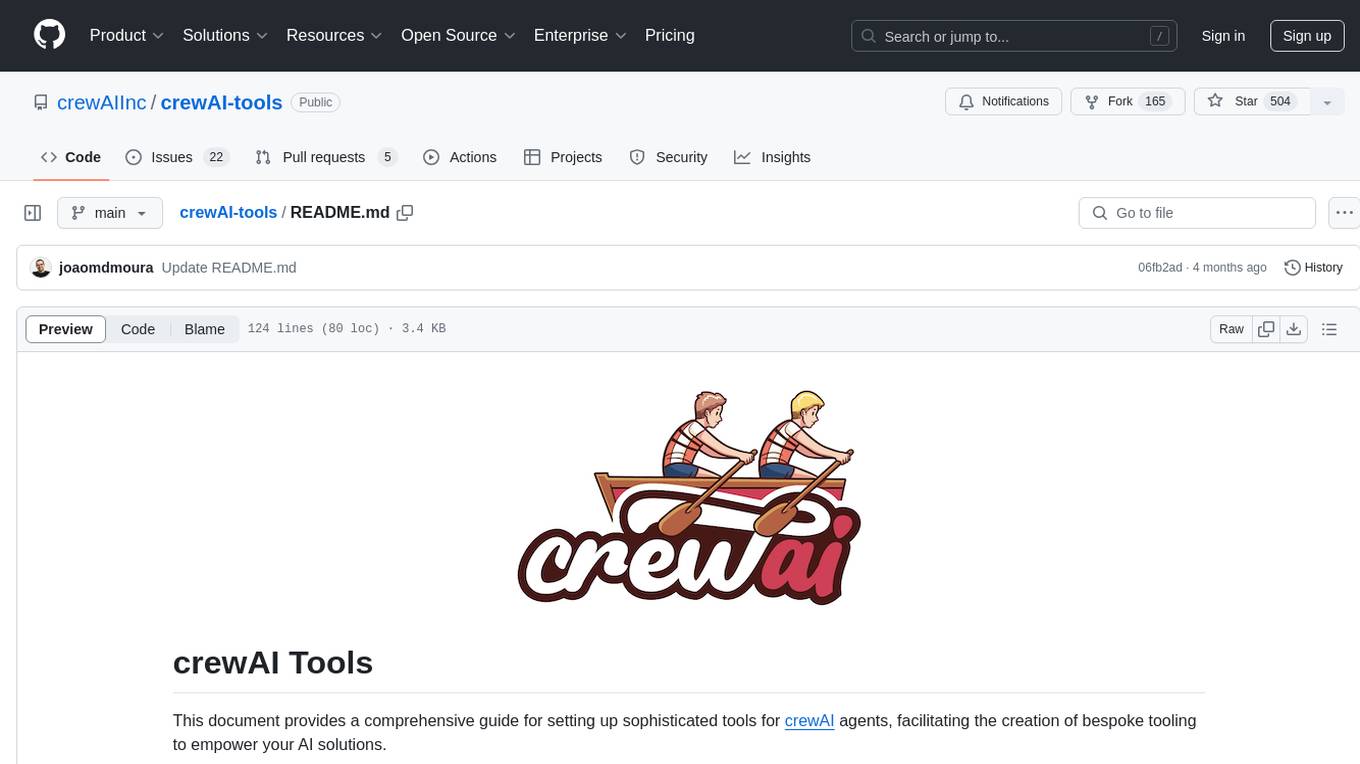
crewAI-tools
This repository provides a guide for setting up tools for crewAI agents to enhance functionality. It offers steps to equip agents with ready-to-use tools and create custom ones. Tools are expected to return strings for generating responses. Users can create tools by subclassing BaseTool or using the tool decorator. Contributions are welcome to enrich the toolset, and guidelines are provided for contributing. The development setup includes installing dependencies, activating virtual environment, setting up pre-commit hooks, running tests, static type checking, packaging, and local installation. The goal is to empower AI solutions through advanced tooling.
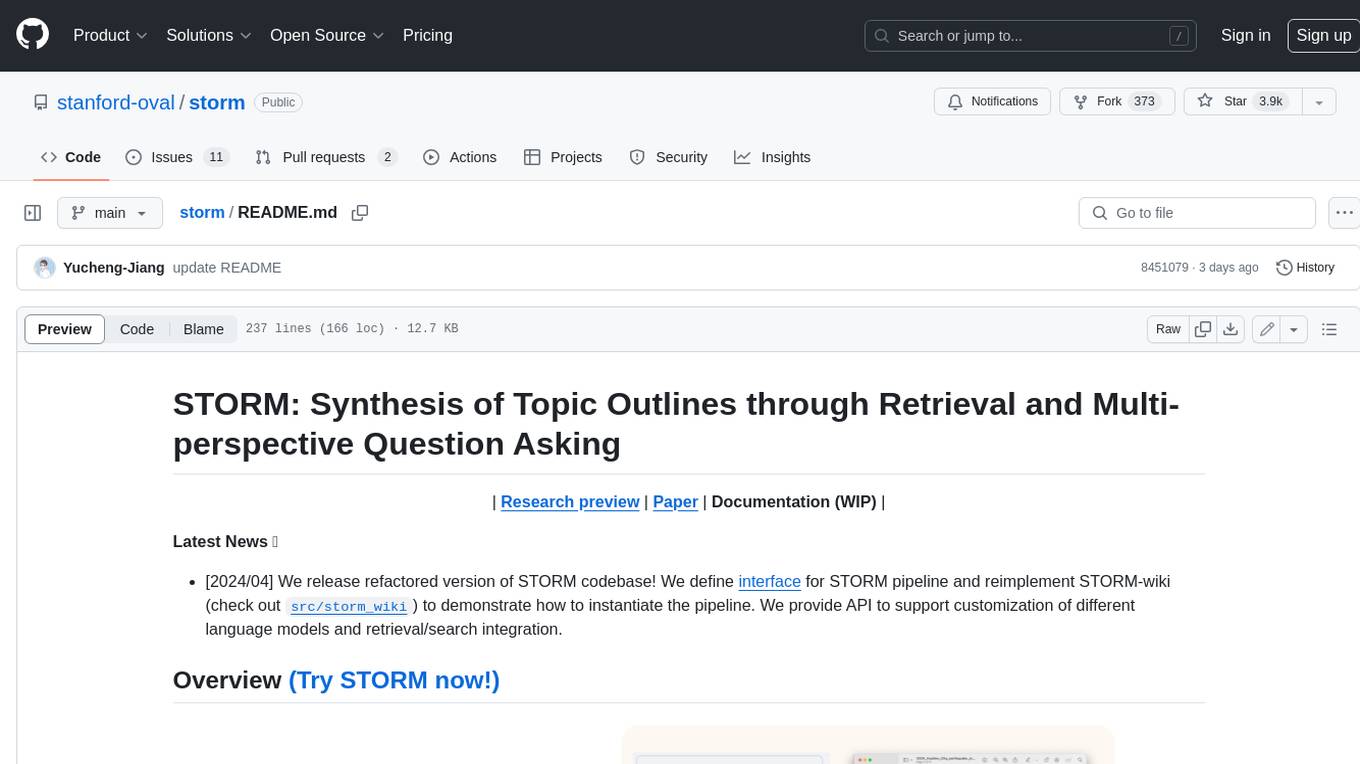
storm
STORM is a LLM system that writes Wikipedia-like articles from scratch based on Internet search. While the system cannot produce publication-ready articles that often require a significant number of edits, experienced Wikipedia editors have found it helpful in their pre-writing stage. **Try out our [live research preview](https://storm.genie.stanford.edu/) to see how STORM can help your knowledge exploration journey and please provide feedback to help us improve the system 🙏!**
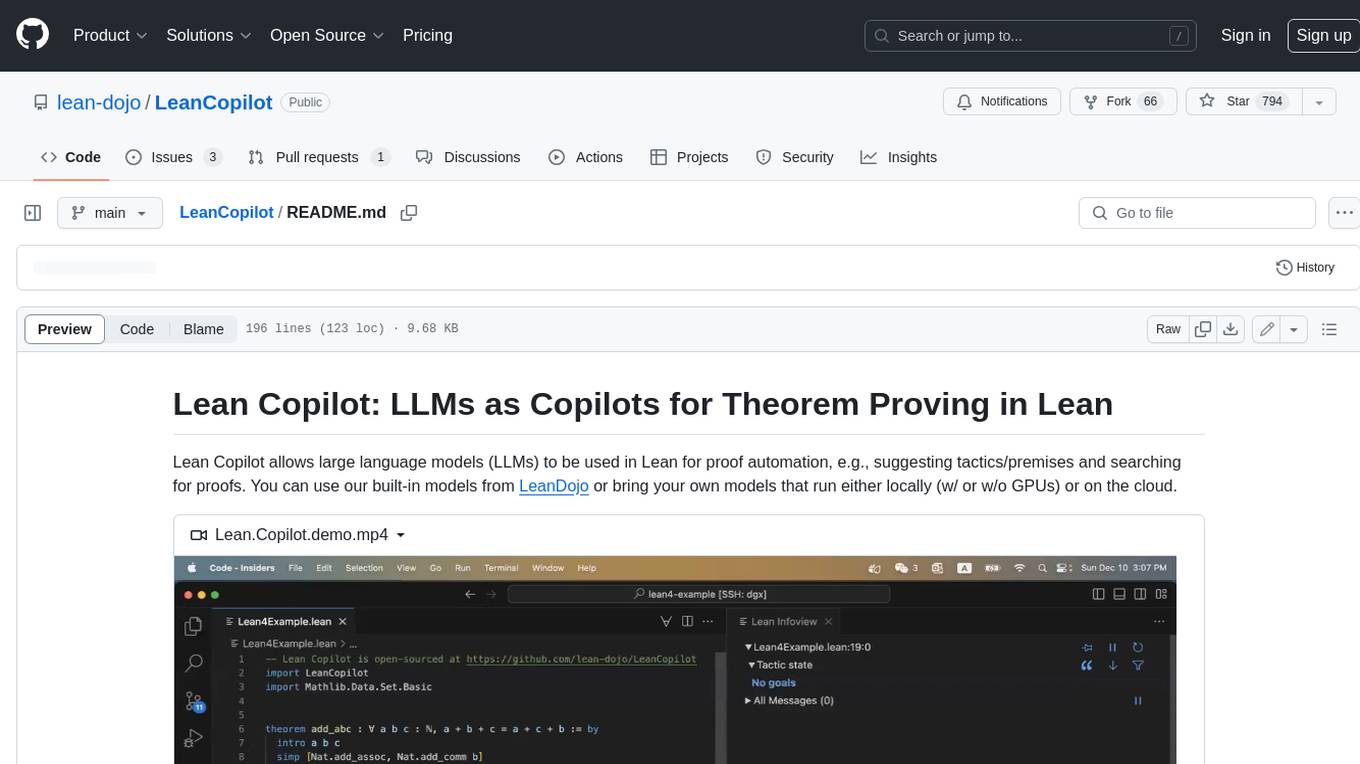
LeanCopilot
Lean Copilot is a tool that enables the use of large language models (LLMs) in Lean for proof automation. It provides features such as suggesting tactics/premises, searching for proofs, and running inference of LLMs. Users can utilize built-in models from LeanDojo or bring their own models to run locally or on the cloud. The tool supports platforms like Linux, macOS, and Windows WSL, with optional CUDA and cuDNN for GPU acceleration. Advanced users can customize behavior using Tactic APIs and Model APIs. Lean Copilot also allows users to bring their own models through ExternalGenerator or ExternalEncoder. The tool comes with caveats such as occasional crashes and issues with premise selection and proof search. Users can get in touch through GitHub Discussions for questions, bug reports, feature requests, and suggestions. The tool is designed to enhance theorem proving in Lean using LLMs.
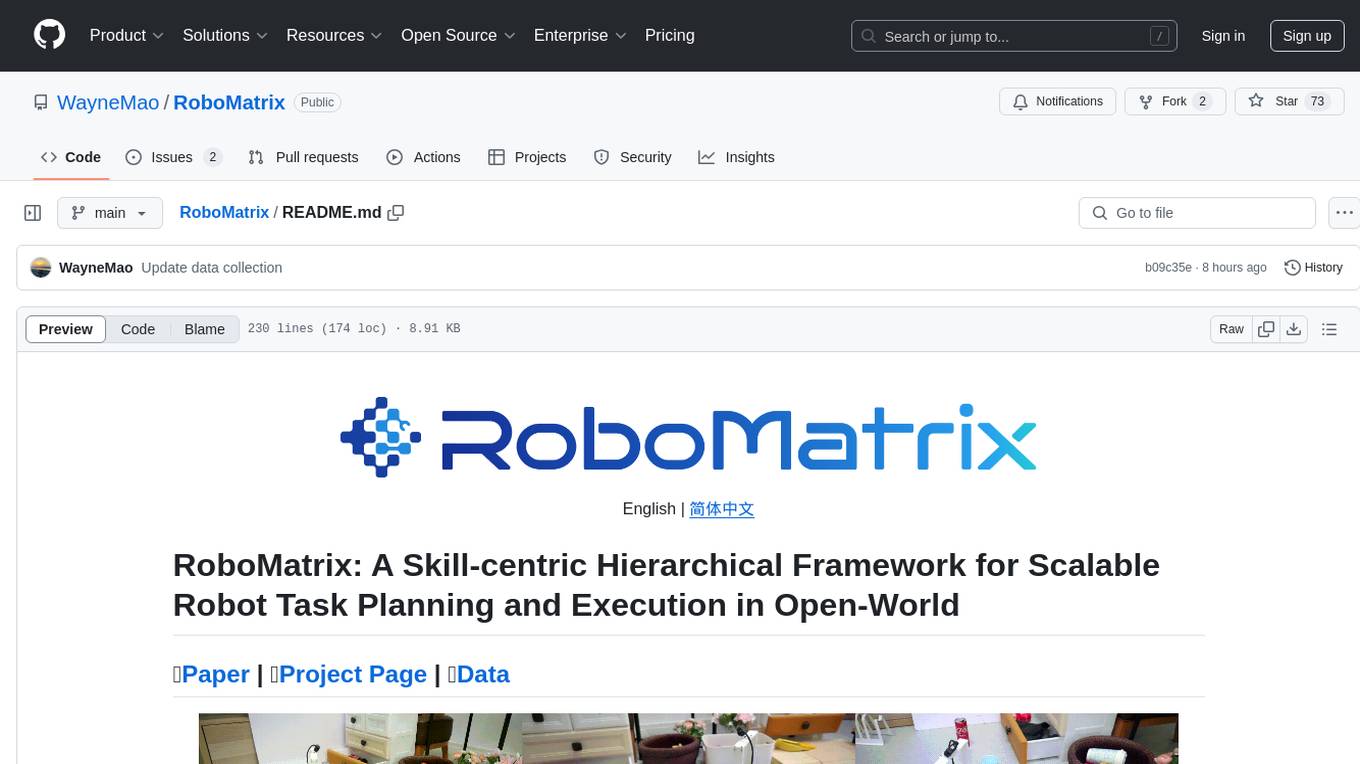
RoboMatrix
RoboMatrix is a skill-centric hierarchical framework for scalable robot task planning and execution in an open-world environment. It provides a structured approach to robot task execution using a combination of hardware components, environment configuration, installation procedures, and data collection methods. The framework is developed using the ROS2 framework on Ubuntu and supports robots from DJI's RoboMaster series. Users can follow the provided installation guidance to set up RoboMatrix and utilize it for various tasks such as data collection, task execution, and dataset construction. The framework also includes a supervised fine-tuning dataset and aims to optimize communication and release additional components in the future.
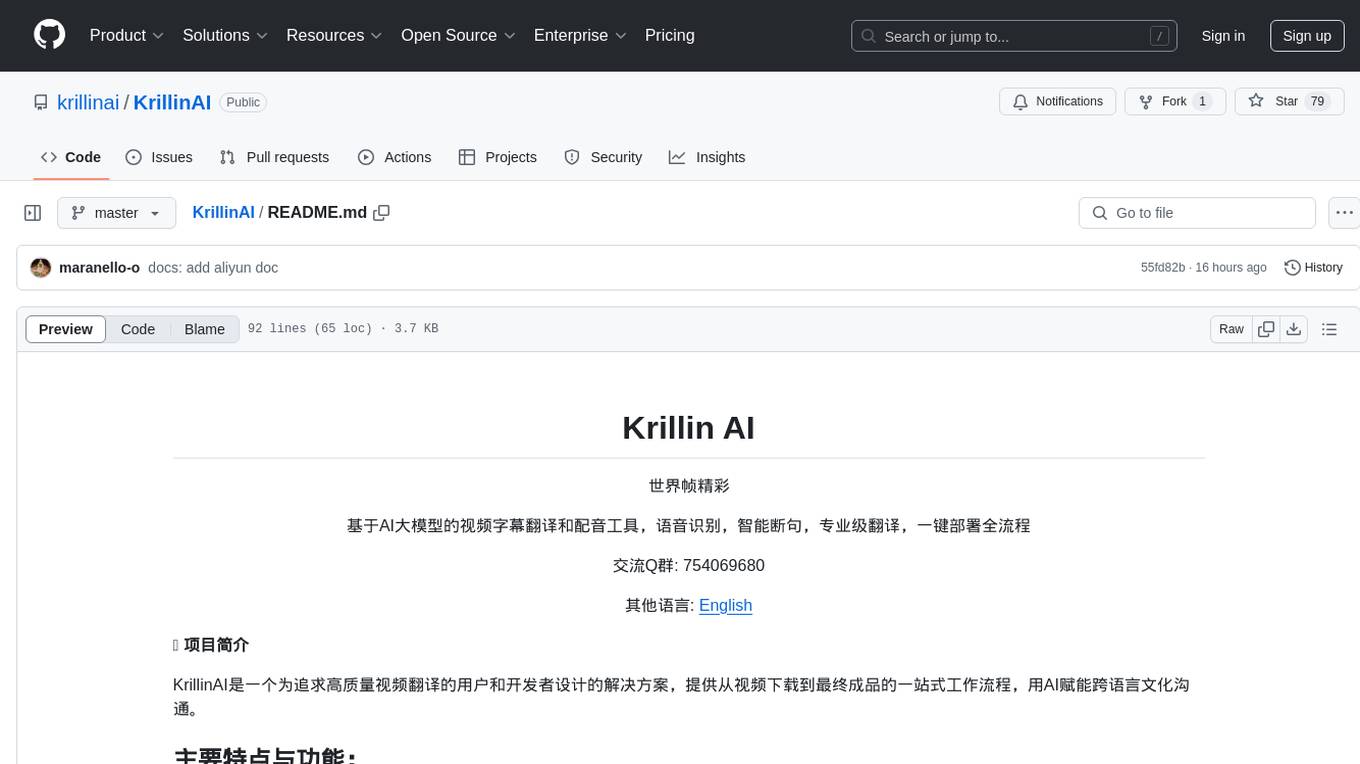
KrillinAI
KrillinAI is a video subtitle translation and dubbing tool based on AI large models, featuring speech recognition, intelligent sentence segmentation, professional translation, and one-click deployment of the entire process. It provides a one-stop workflow from video downloading to the final product, empowering cross-language cultural communication with AI. The tool supports multiple languages for input and translation, integrates features like automatic dependency installation, video downloading from platforms like YouTube and Bilibili, high-speed subtitle recognition, intelligent subtitle segmentation and alignment, custom vocabulary replacement, professional-level translation engine, and diverse external service selection for speech and large model services.
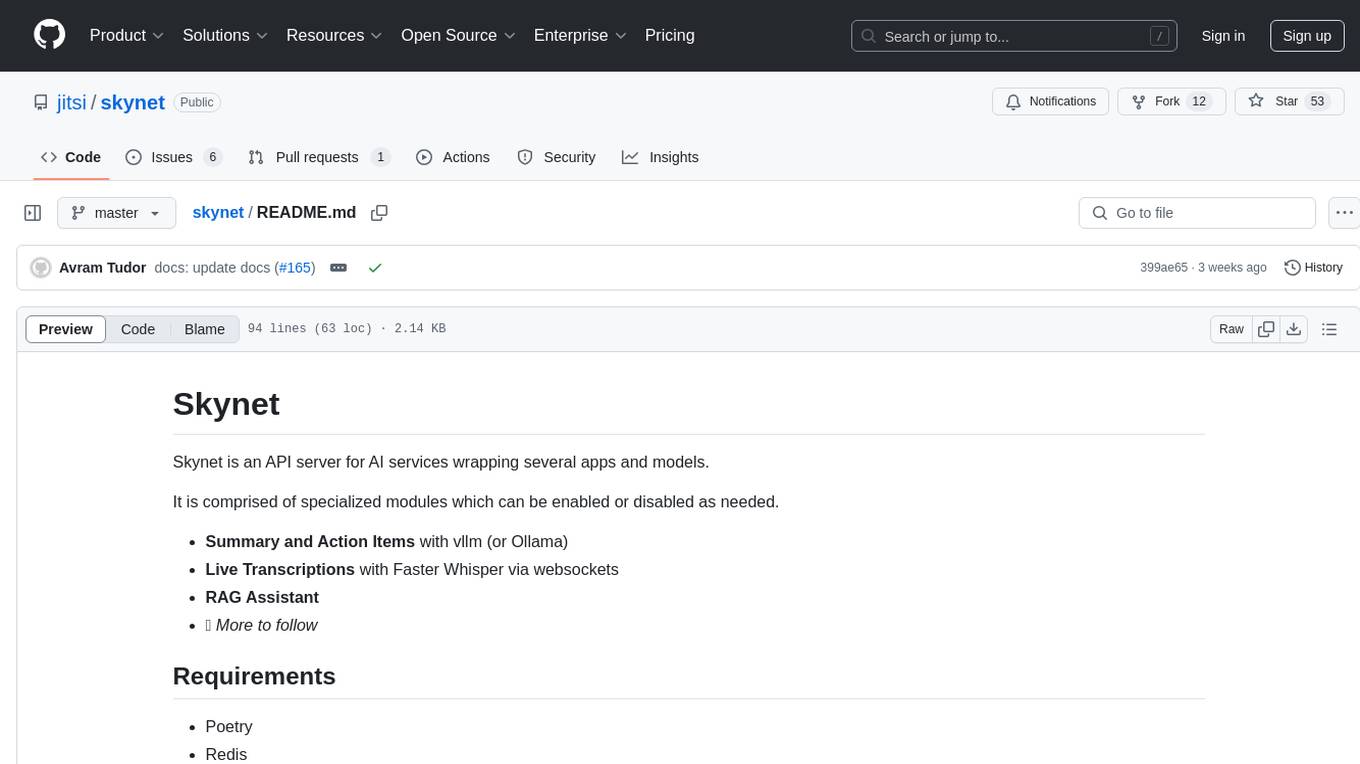
skynet
Skynet is an API server for AI services that wraps several apps and models. It consists of specialized modules that can be enabled or disabled as needed. Users can utilize Skynet for tasks such as summaries and action items with vllm or Ollama, live transcriptions with Faster Whisper via websockets, and RAG Assistant. The tool requires Poetry and Redis for operation. Skynet provides a quickstart guide for both Summaries/Assistant and Live Transcriptions, along with instructions for testing docker changes and running demos. Detailed documentation on configuration, running, building, and monitoring Skynet is available in the docs. Developers can contribute to Skynet by installing the pre-commit hook for linting. Skynet is distributed under the Apache 2.0 License.
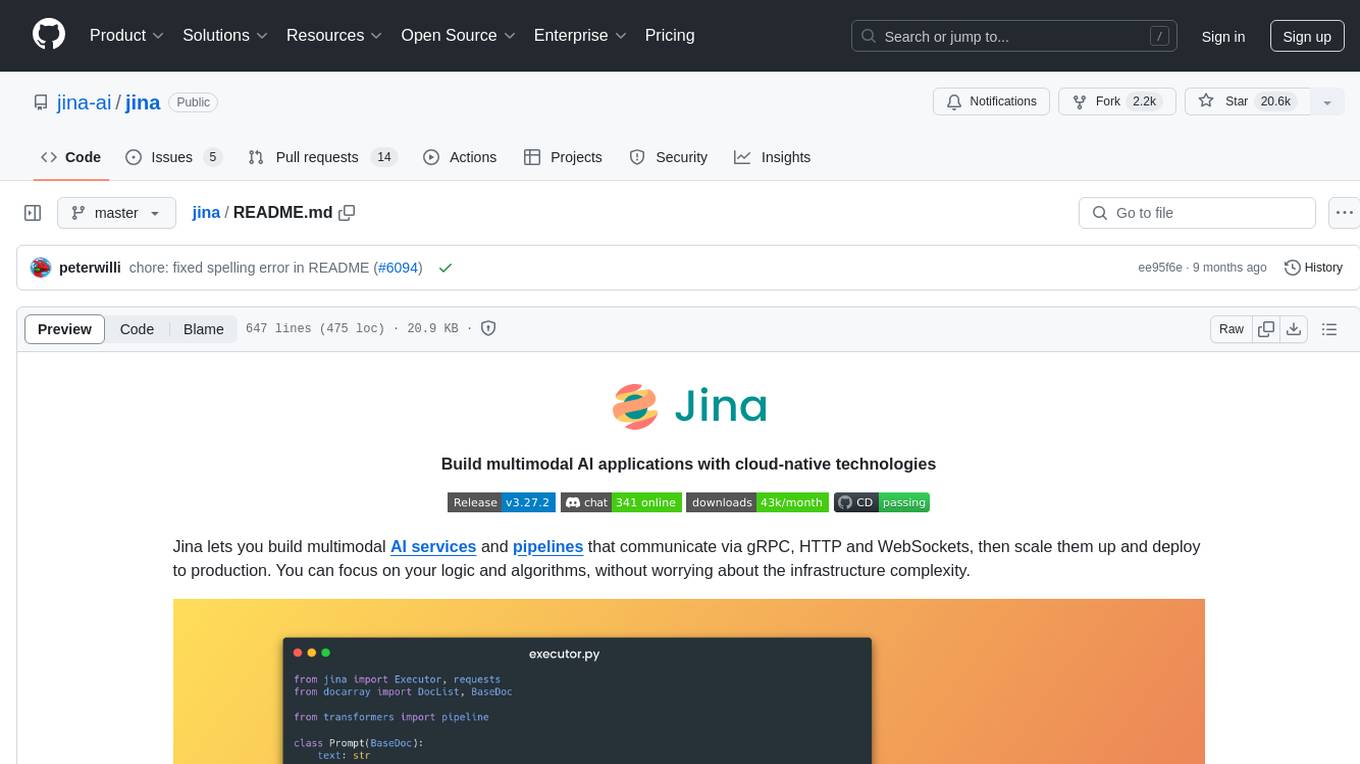
jina
Jina is a tool that allows users to build multimodal AI services and pipelines using cloud-native technologies. It provides a Pythonic experience for serving ML models and transitioning from local deployment to advanced orchestration frameworks like Docker-Compose, Kubernetes, or Jina AI Cloud. Users can build and serve models for any data type and deep learning framework, design high-performance services with easy scaling, serve LLM models while streaming their output, integrate with Docker containers via Executor Hub, and host on CPU/GPU using Jina AI Cloud. Jina also offers advanced orchestration and scaling capabilities, a smooth transition to the cloud, and easy scalability and concurrency features for applications. Users can deploy to their own cloud or system with Kubernetes and Docker Compose integration, and even deploy to JCloud for autoscaling and monitoring.
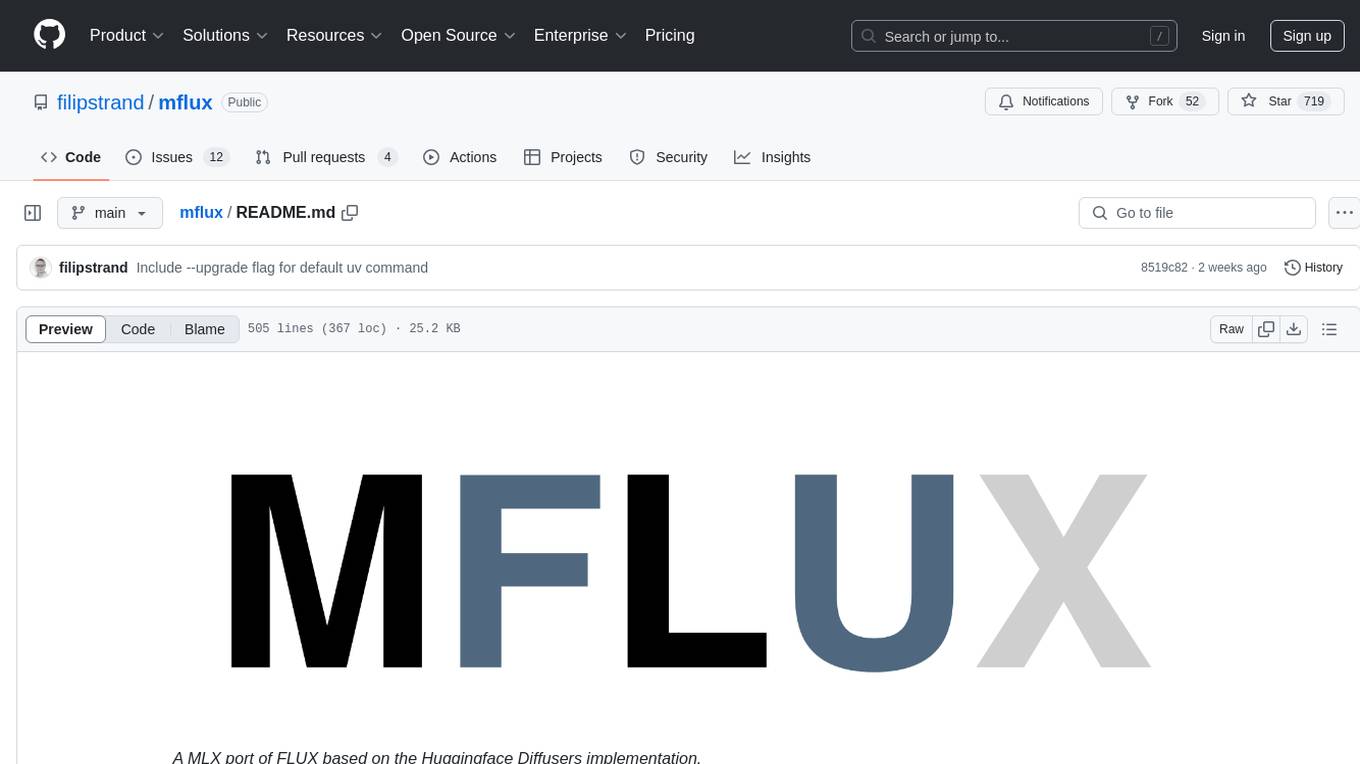
mflux
MFLUX is a line-by-line port of the FLUX implementation in the Huggingface Diffusers library to Apple MLX. It aims to run powerful FLUX models from Black Forest Labs locally on Mac machines. The codebase is minimal and explicit, prioritizing readability over generality and performance. Models are implemented from scratch in MLX, with tokenizers from the Huggingface Transformers library. Dependencies include Numpy and Pillow for image post-processing. Installation can be done using `uv tool` or classic virtual environment setup. Command-line arguments allow for image generation with specified models, prompts, and optional parameters. Quantization options for speed and memory reduction are available. LoRA adapters can be loaded for fine-tuning image generation. Controlnet support provides more control over image generation with reference images. Current limitations include generating images one by one, lack of support for negative prompts, and some LoRA adapters not working.
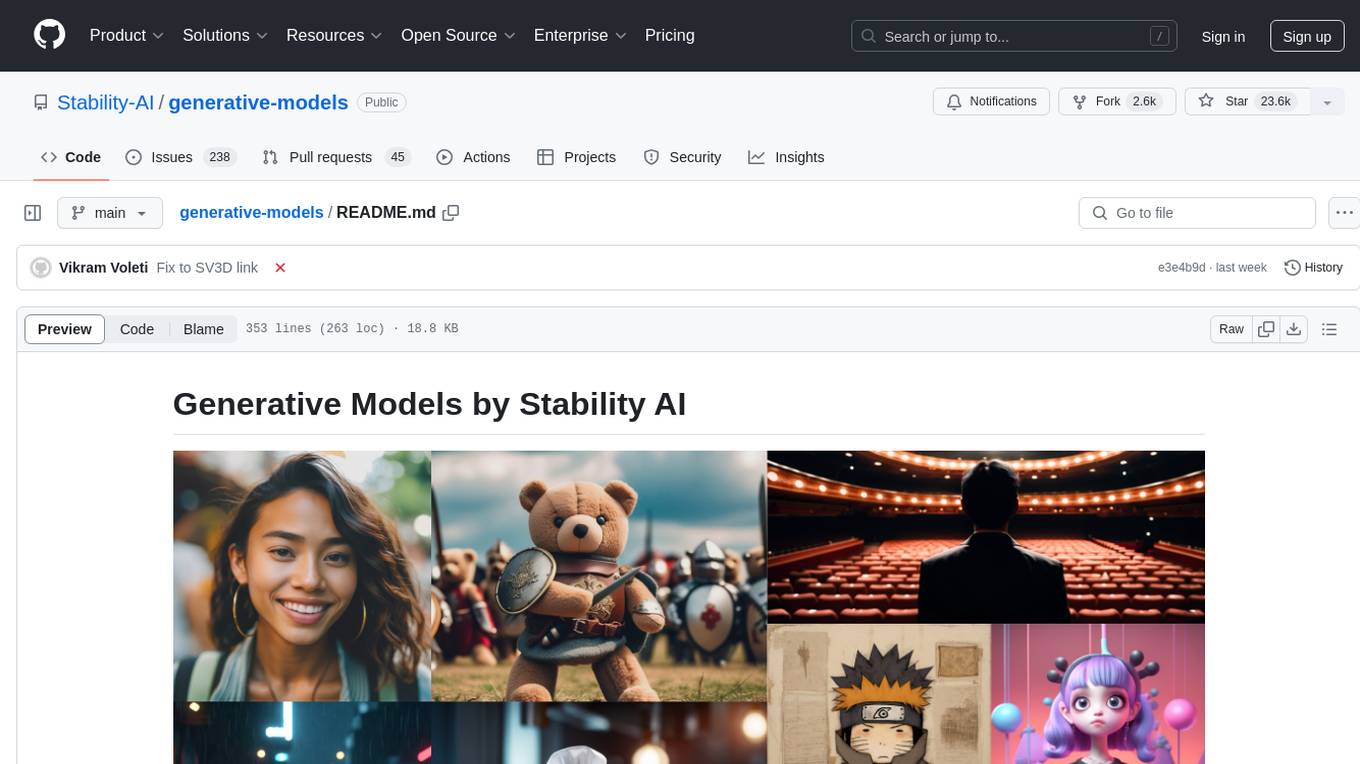
generative-models
Generative Models by Stability AI is a repository that provides various generative models for research purposes. It includes models like Stable Video 4D (SV4D) for video synthesis, Stable Video 3D (SV3D) for multi-view synthesis, SDXL-Turbo for text-to-image generation, and more. The repository focuses on modularity and implements a config-driven approach for building and combining submodules. It supports training with PyTorch Lightning and offers inference demos for different models. Users can access pre-trained models like SDXL-base-1.0 and SDXL-refiner-1.0 under a CreativeML Open RAIL++-M license. The codebase also includes tools for invisible watermark detection in generated images.
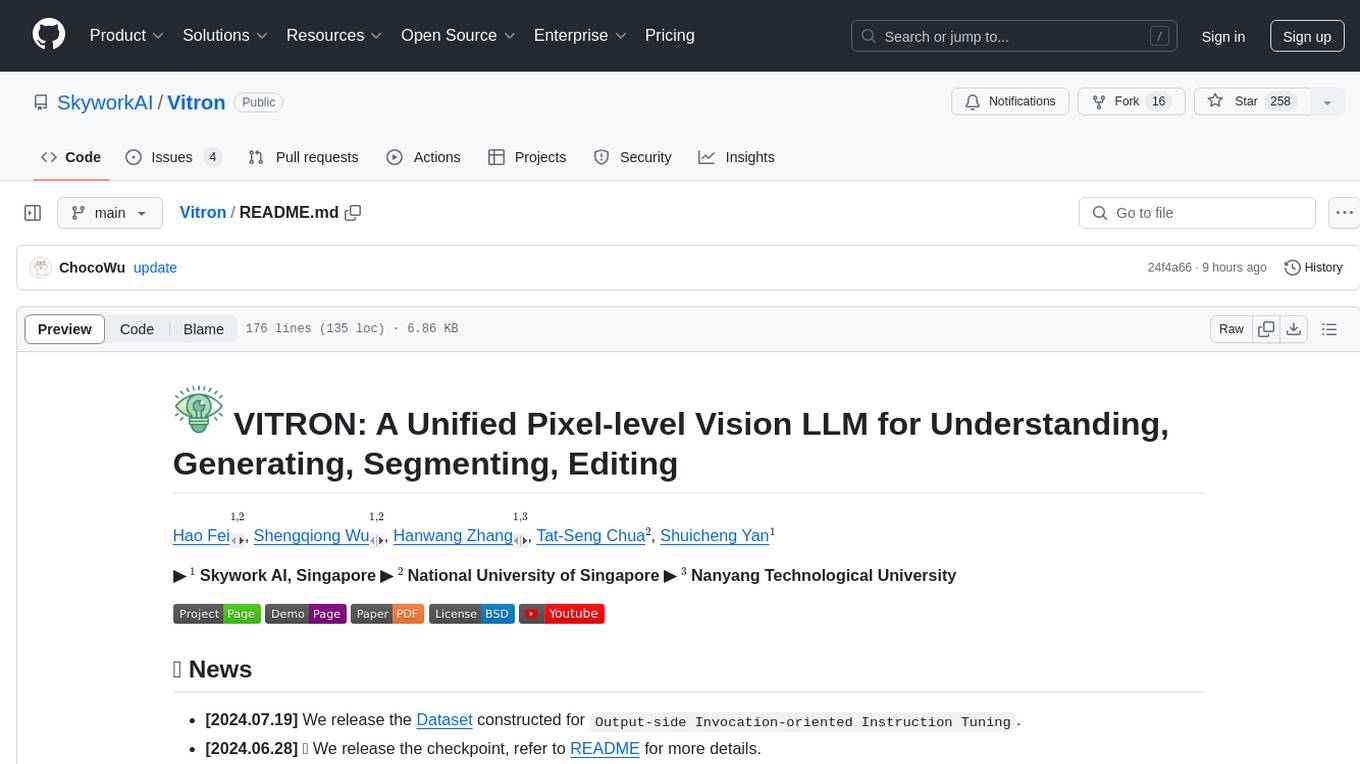
Vitron
Vitron is a unified pixel-level vision LLM designed for comprehensive understanding, generating, segmenting, and editing static images and dynamic videos. It addresses challenges in existing vision LLMs such as superficial instance-level understanding, lack of unified support for images and videos, and insufficient coverage across various vision tasks. The tool requires Python >= 3.8, Pytorch == 2.1.0, and CUDA Version >= 11.8 for installation. Users can deploy Gradio demo locally and fine-tune their models for specific tasks.
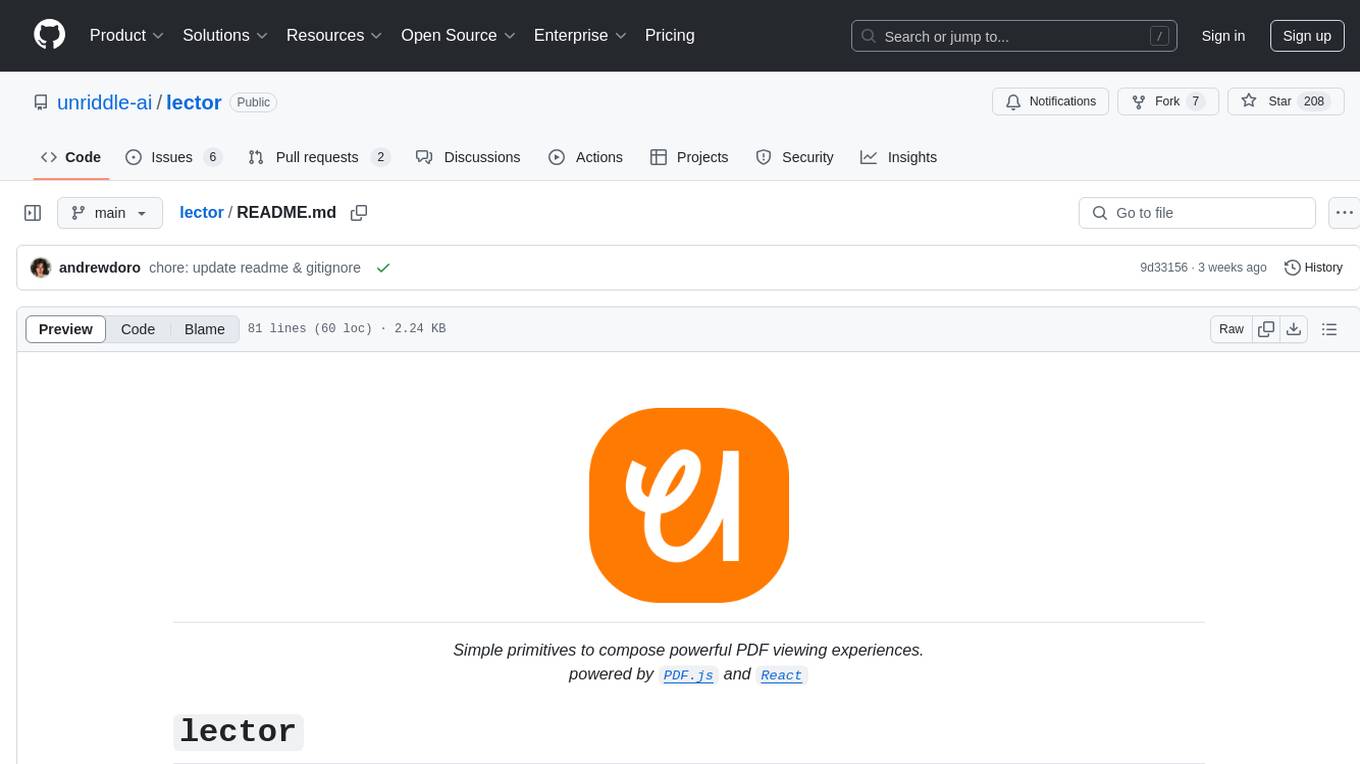
lector
Lector is a text analysis tool that helps users extract insights from unstructured text data. It provides functionalities such as sentiment analysis, keyword extraction, entity recognition, and text summarization. With Lector, users can easily analyze large volumes of text data to uncover patterns, trends, and valuable information. The tool is designed to be user-friendly and efficient, making it suitable for both beginners and experienced users in the field of natural language processing and text mining.
For similar tasks
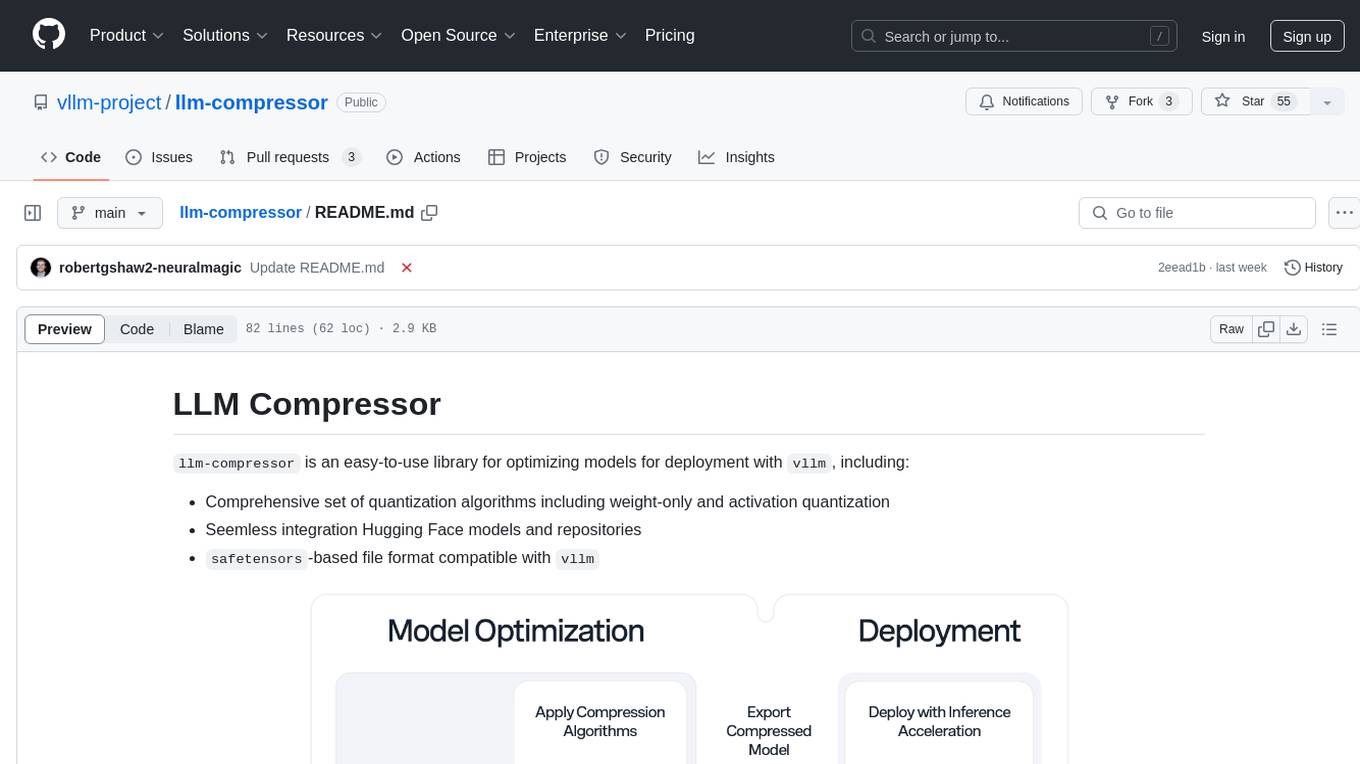
llm-compressor
llm-compressor is an easy-to-use library for optimizing models for deployment with vllm. It provides a comprehensive set of quantization algorithms, seamless integration with Hugging Face models and repositories, and supports mixed precision, activation quantization, and sparsity. Supported algorithms include PTQ, GPTQ, SmoothQuant, and SparseGPT. Installation can be done via git clone and local pip install. Compression can be easily applied by selecting an algorithm and calling the oneshot API. The library also offers end-to-end examples for model compression. Contributions to the code, examples, integrations, and documentation are appreciated.
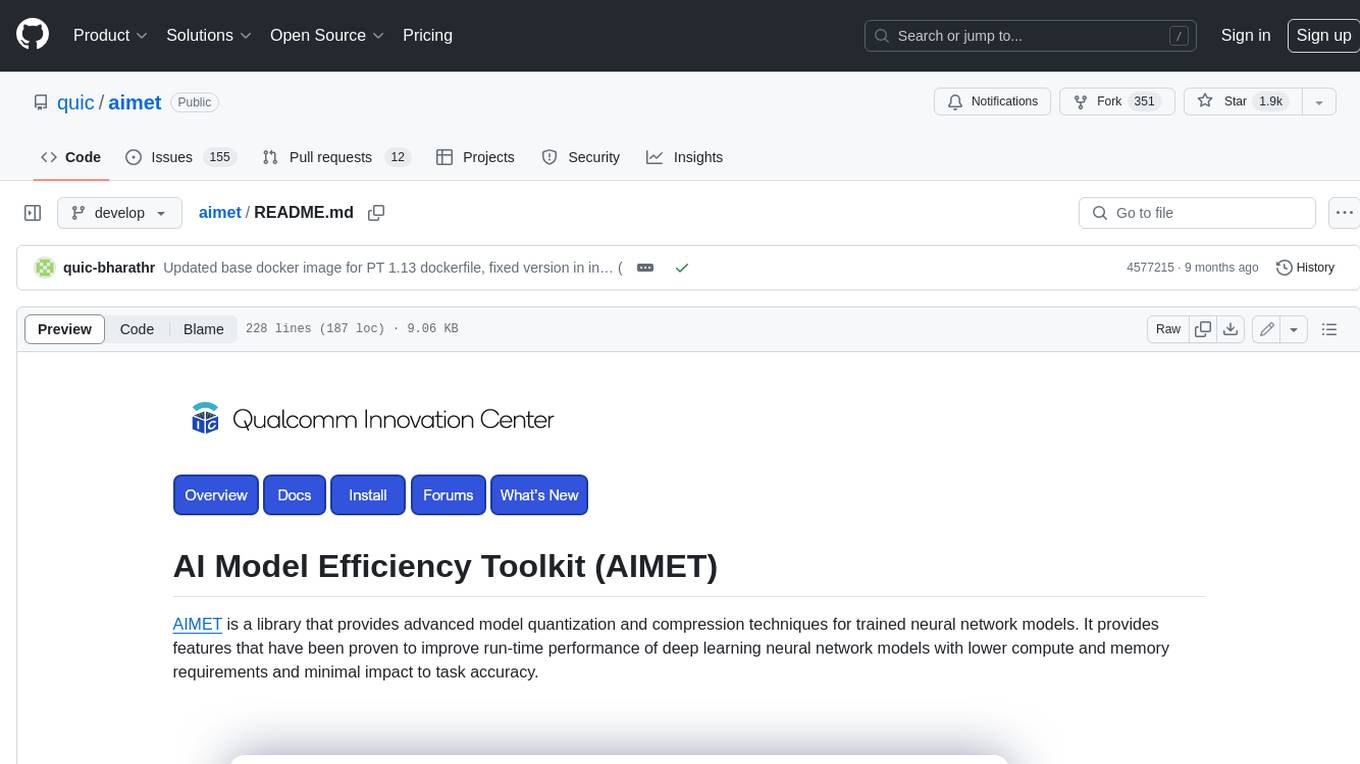
aimet
AIMET is a library that provides advanced model quantization and compression techniques for trained neural network models. It provides features that have been proven to improve run-time performance of deep learning neural network models with lower compute and memory requirements and minimal impact to task accuracy. AIMET is designed to work with PyTorch, TensorFlow and ONNX models. We also host the AIMET Model Zoo - a collection of popular neural network models optimized for 8-bit inference. We also provide recipes for users to quantize floating point models using AIMET.
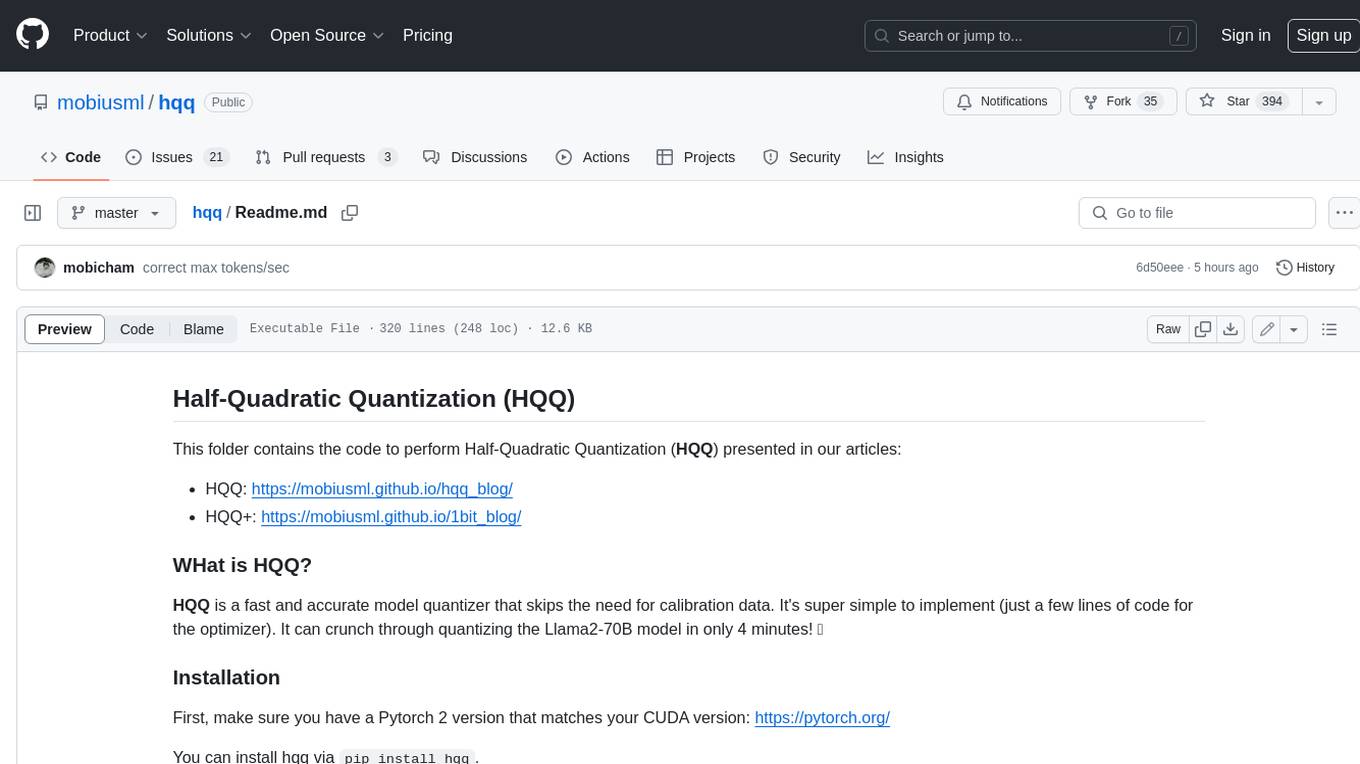
hqq
HQQ is a fast and accurate model quantizer that skips the need for calibration data. It's super simple to implement (just a few lines of code for the optimizer). It can crunch through quantizing the Llama2-70B model in only 4 minutes! 🚀
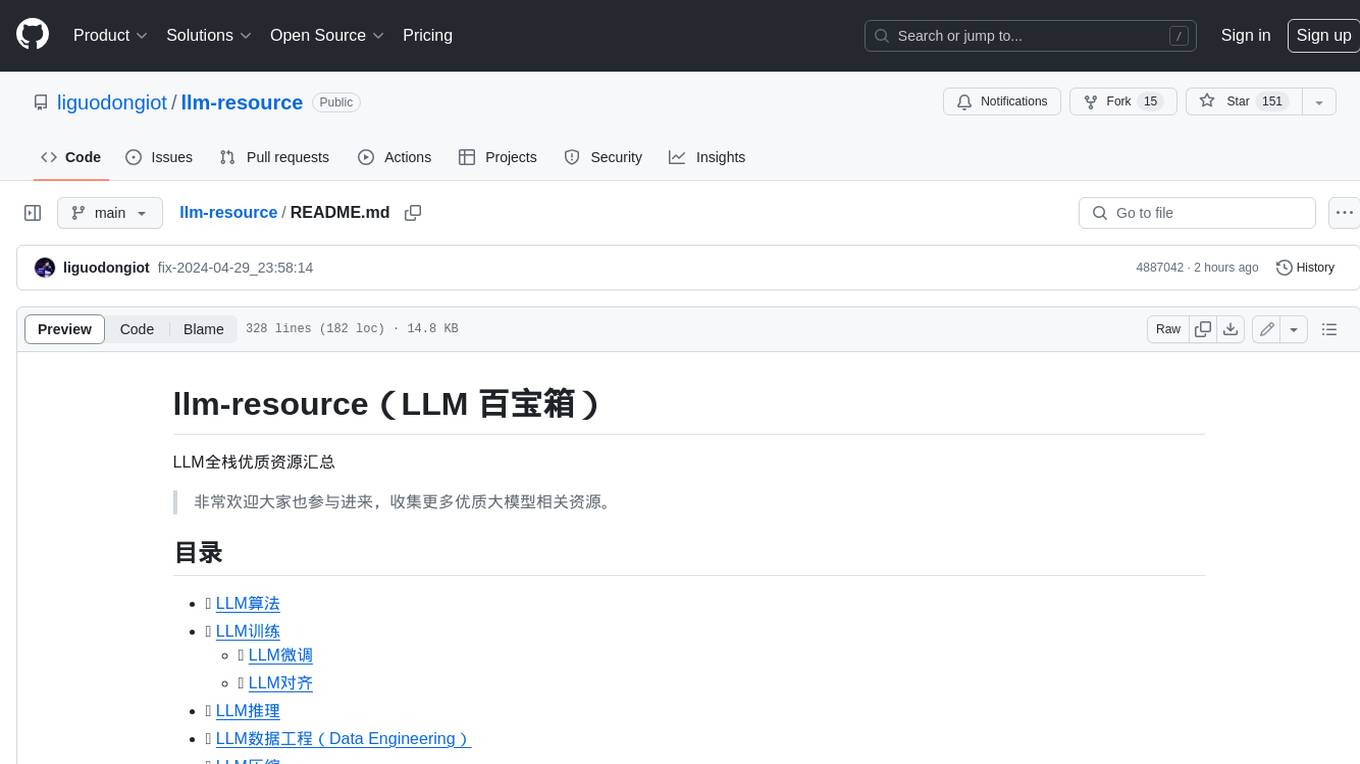
llm-resource
llm-resource is a comprehensive collection of high-quality resources for Large Language Models (LLM). It covers various aspects of LLM including algorithms, training, fine-tuning, alignment, inference, data engineering, compression, evaluation, prompt engineering, AI frameworks, AI basics, AI infrastructure, AI compilers, LLM application development, LLM operations, AI systems, and practical implementations. The repository aims to gather and share valuable resources related to LLM for the community to benefit from.
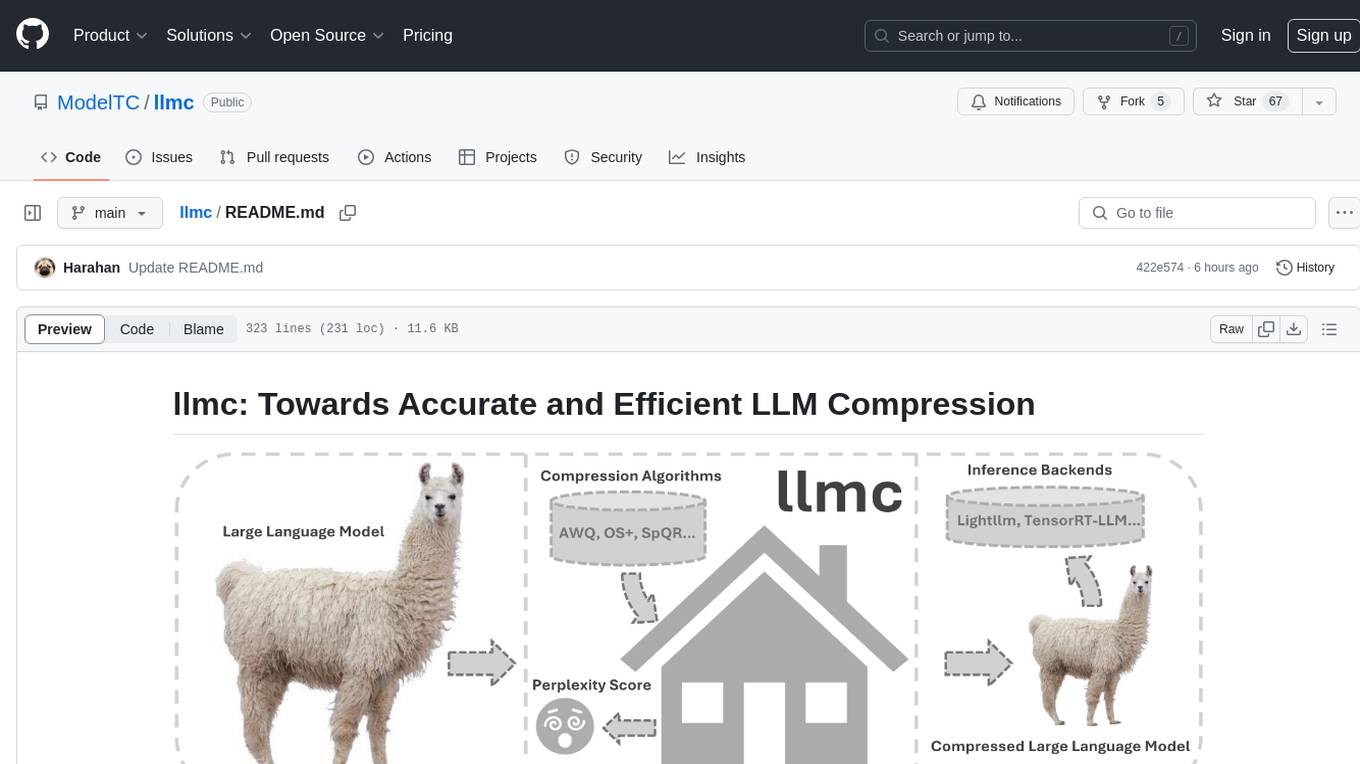
llmc
llmc is an off-the-shell tool designed for compressing LLM, leveraging state-of-the-art compression algorithms to enhance efficiency and reduce model size without compromising performance. It provides users with the ability to quantize LLMs, choose from various compression algorithms, export transformed models for further optimization, and directly infer compressed models with a shallow memory footprint. The tool supports a range of model types and quantization algorithms, with ongoing development to include pruning techniques. Users can design their configurations for quantization and evaluation, with documentation and examples planned for future updates. llmc is a valuable resource for researchers working on post-training quantization of large language models.
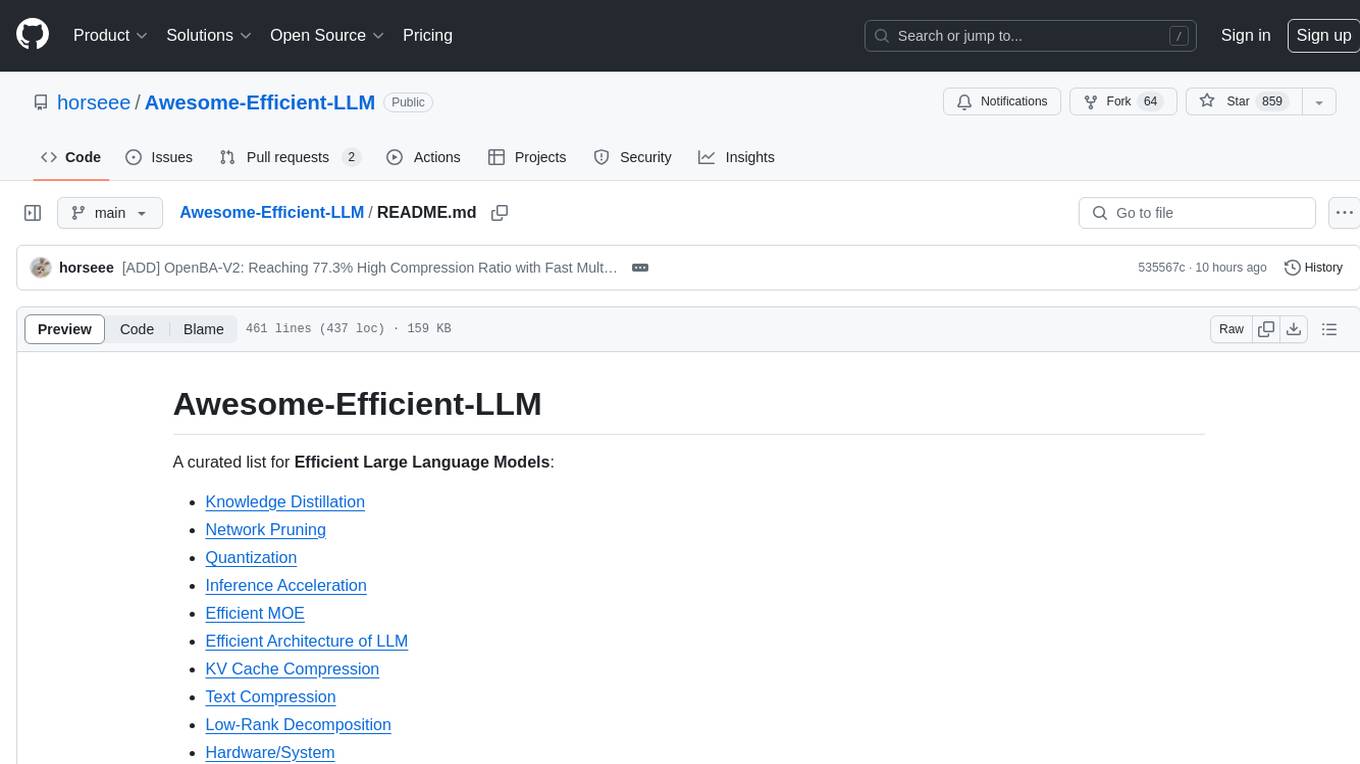
Awesome-Efficient-LLM
Awesome-Efficient-LLM is a curated list focusing on efficient large language models. It includes topics such as knowledge distillation, network pruning, quantization, inference acceleration, efficient MOE, efficient architecture of LLM, KV cache compression, text compression, low-rank decomposition, hardware/system, tuning, and survey. The repository provides a collection of papers and projects related to improving the efficiency of large language models through various techniques like sparsity, quantization, and compression.
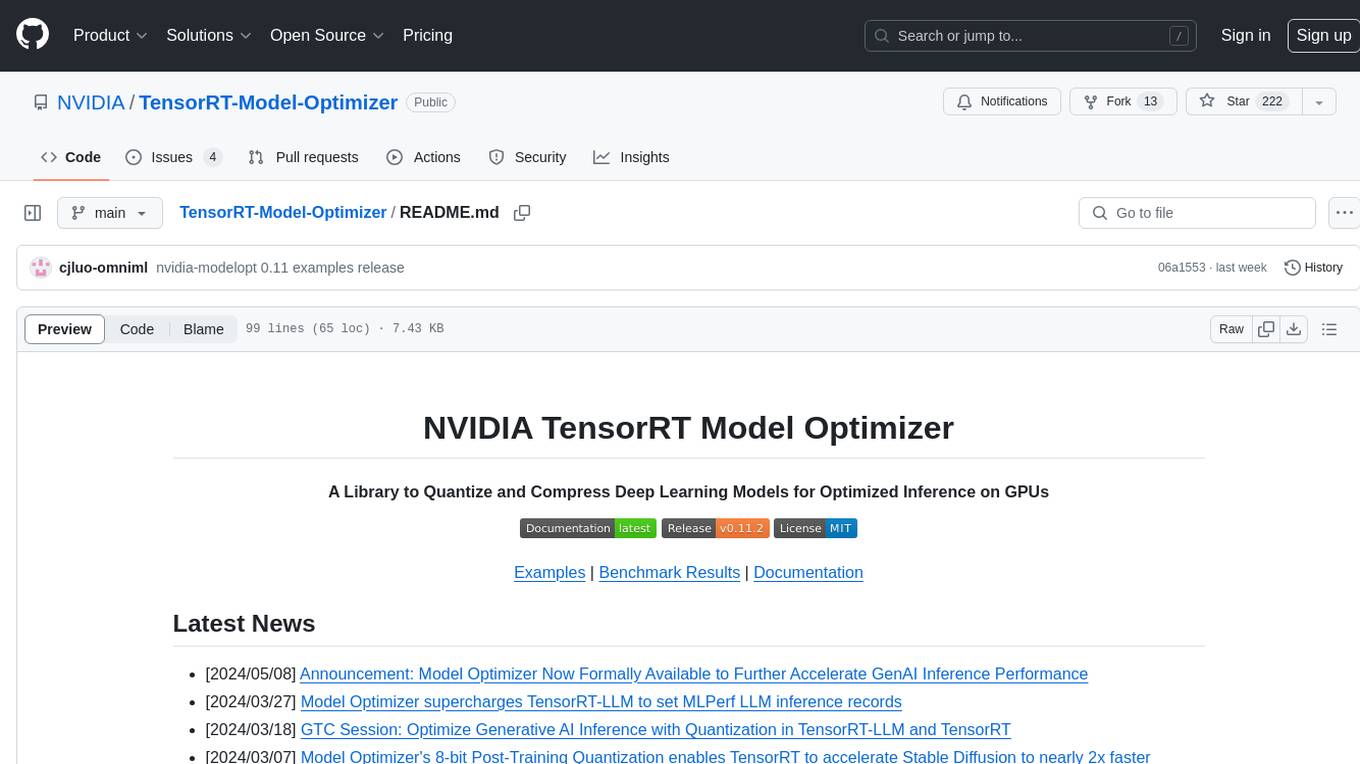
TensorRT-Model-Optimizer
The NVIDIA TensorRT Model Optimizer is a library designed to quantize and compress deep learning models for optimized inference on GPUs. It offers state-of-the-art model optimization techniques including quantization and sparsity to reduce inference costs for generative AI models. Users can easily stack different optimization techniques to produce quantized checkpoints from torch or ONNX models. The quantized checkpoints are ready for deployment in inference frameworks like TensorRT-LLM or TensorRT, with planned integrations for NVIDIA NeMo and Megatron-LM. The tool also supports 8-bit quantization with Stable Diffusion for enterprise users on NVIDIA NIM. Model Optimizer is available for free on NVIDIA PyPI, and this repository serves as a platform for sharing examples, GPU-optimized recipes, and collecting community feedback.
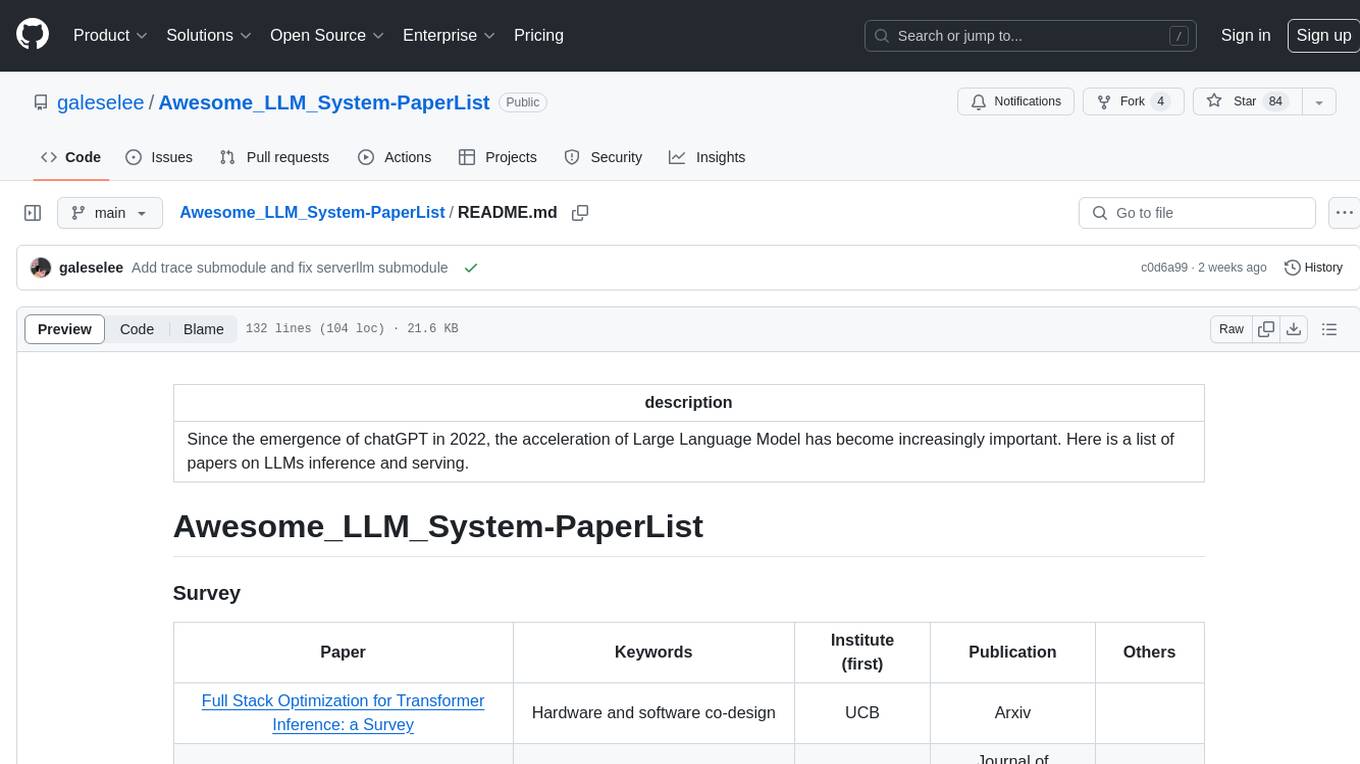
Awesome_LLM_System-PaperList
Since the emergence of chatGPT in 2022, the acceleration of Large Language Model has become increasingly important. Here is a list of papers on LLMs inference and serving.
For similar jobs
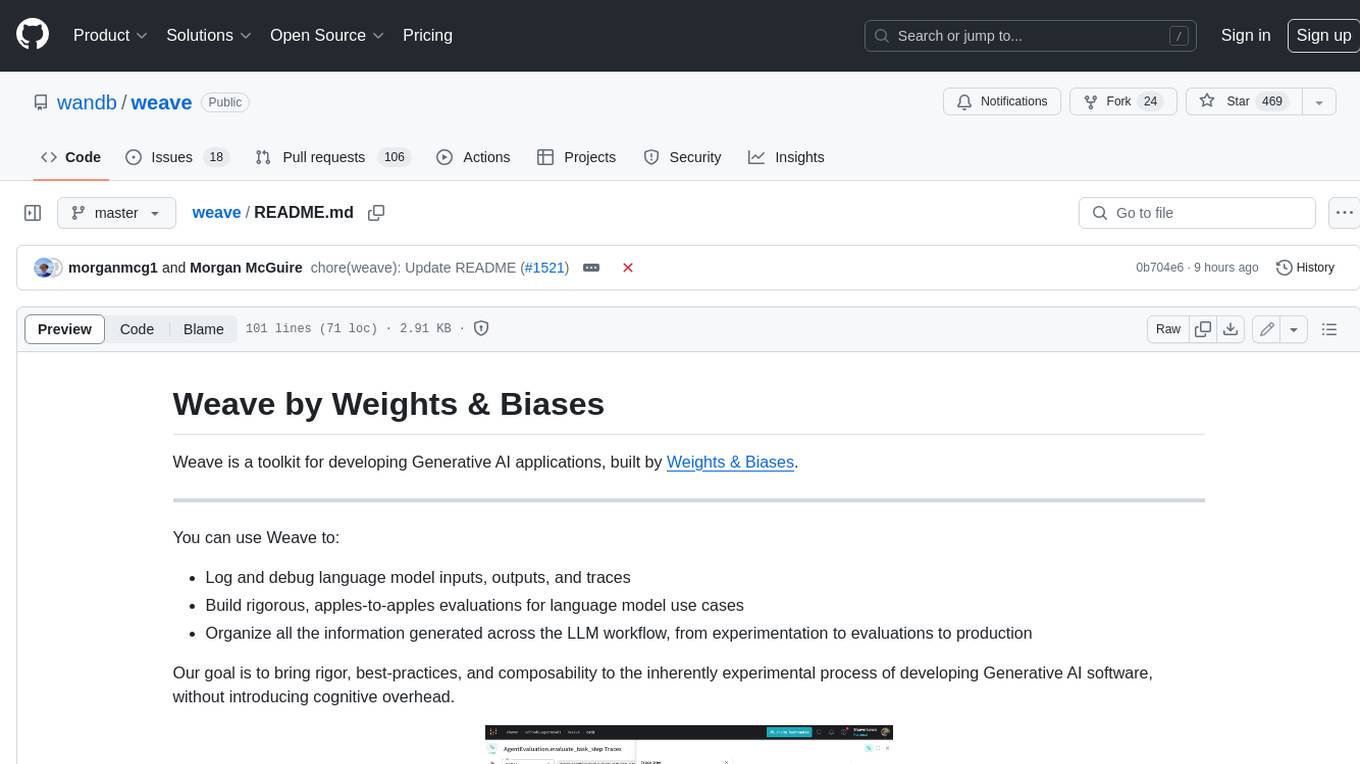
weave
Weave is a toolkit for developing Generative AI applications, built by Weights & Biases. With Weave, you can log and debug language model inputs, outputs, and traces; build rigorous, apples-to-apples evaluations for language model use cases; and organize all the information generated across the LLM workflow, from experimentation to evaluations to production. Weave aims to bring rigor, best-practices, and composability to the inherently experimental process of developing Generative AI software, without introducing cognitive overhead.
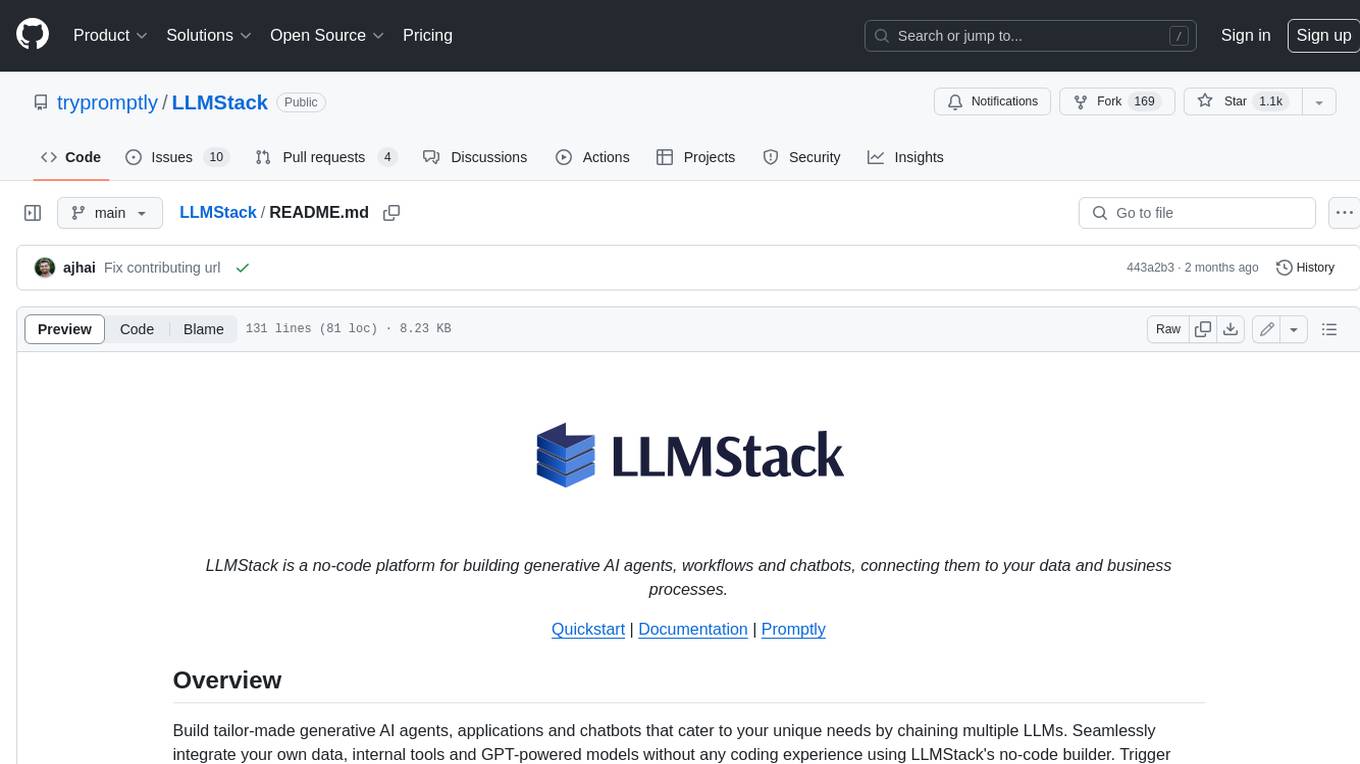
LLMStack
LLMStack is a no-code platform for building generative AI agents, workflows, and chatbots. It allows users to connect their own data, internal tools, and GPT-powered models without any coding experience. LLMStack can be deployed to the cloud or on-premise and can be accessed via HTTP API or triggered from Slack or Discord.
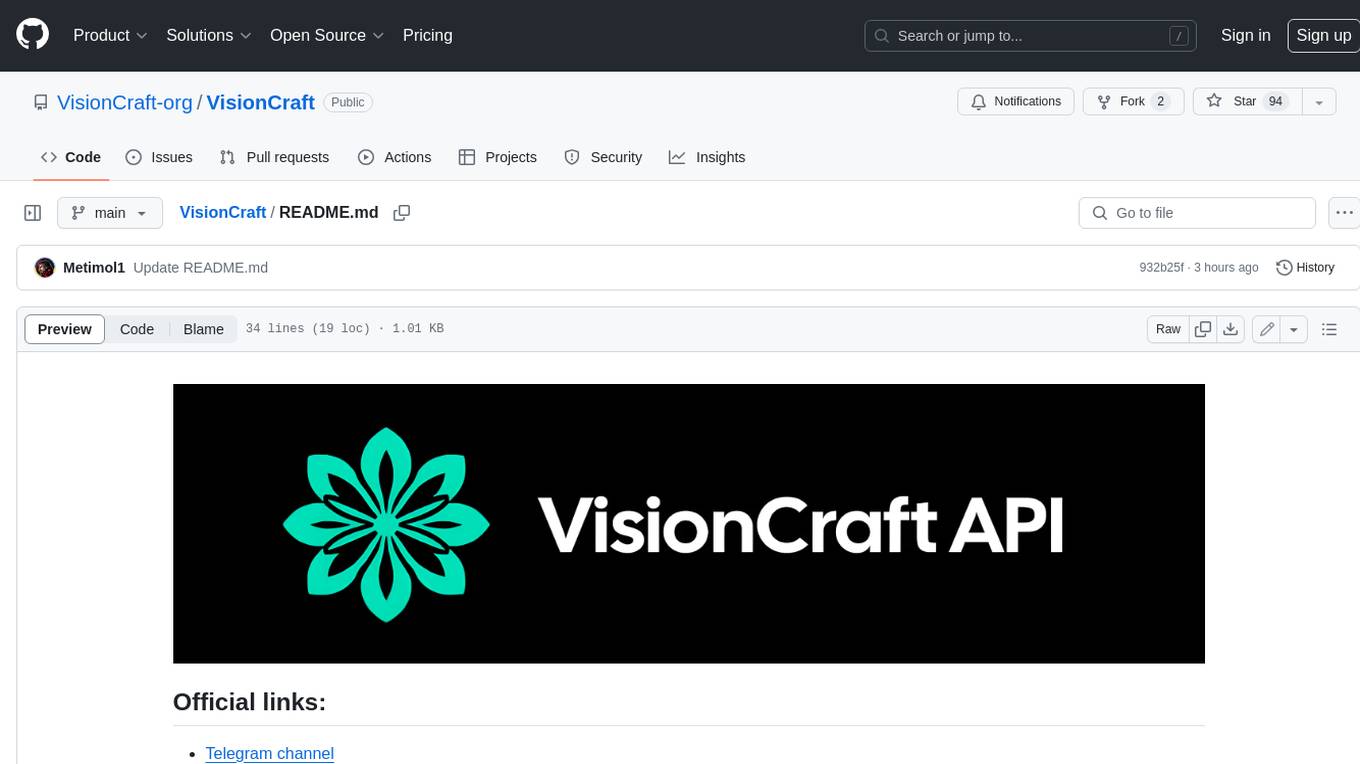
VisionCraft
The VisionCraft API is a free API for using over 100 different AI models. From images to sound.
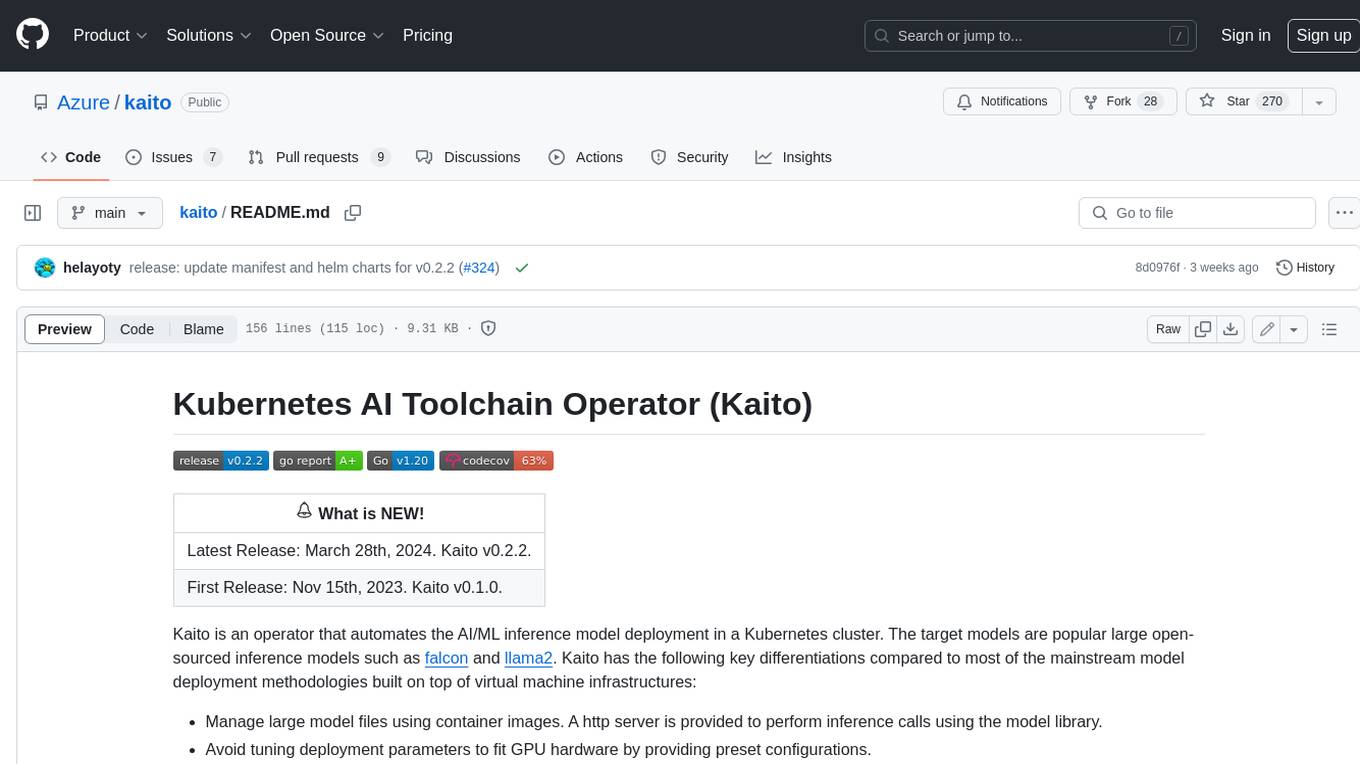
kaito
Kaito is an operator that automates the AI/ML inference model deployment in a Kubernetes cluster. It manages large model files using container images, avoids tuning deployment parameters to fit GPU hardware by providing preset configurations, auto-provisions GPU nodes based on model requirements, and hosts large model images in the public Microsoft Container Registry (MCR) if the license allows. Using Kaito, the workflow of onboarding large AI inference models in Kubernetes is largely simplified.
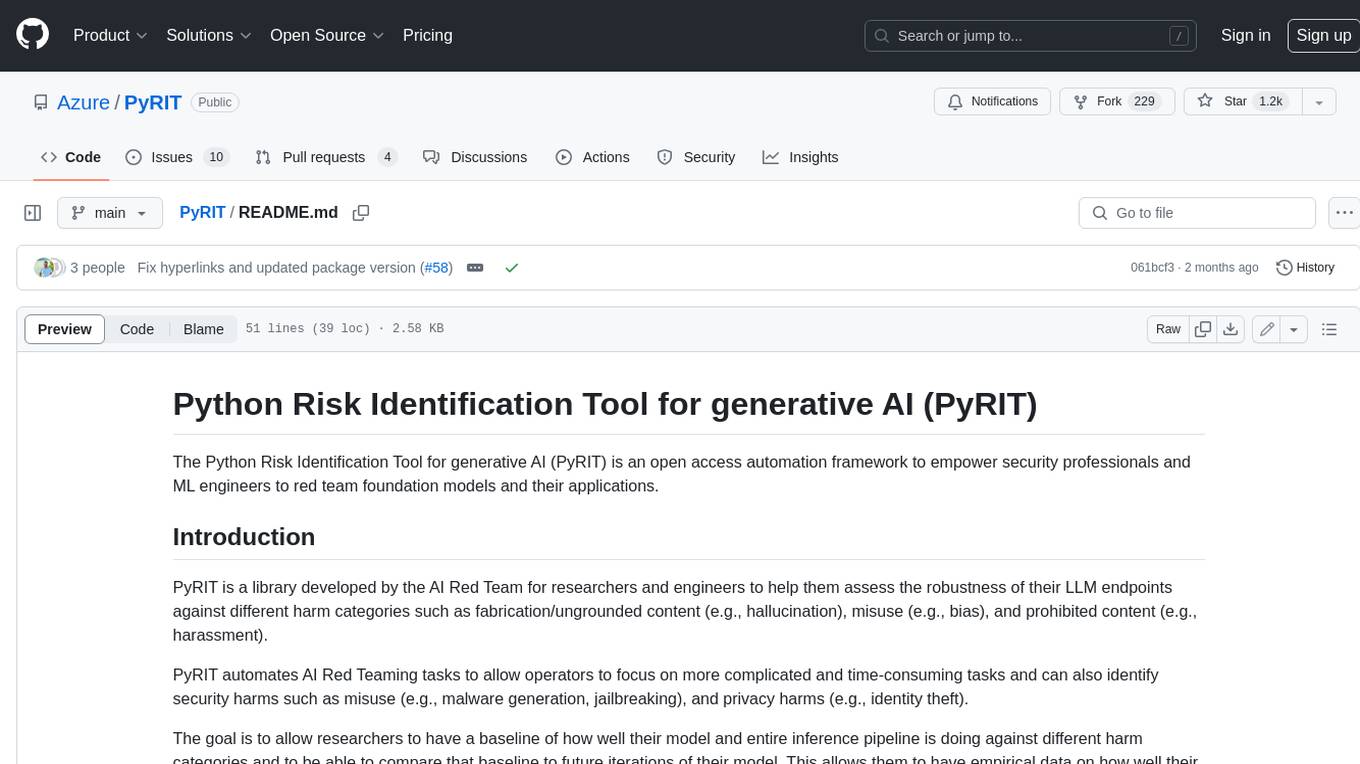
PyRIT
PyRIT is an open access automation framework designed to empower security professionals and ML engineers to red team foundation models and their applications. It automates AI Red Teaming tasks to allow operators to focus on more complicated and time-consuming tasks and can also identify security harms such as misuse (e.g., malware generation, jailbreaking), and privacy harms (e.g., identity theft). The goal is to allow researchers to have a baseline of how well their model and entire inference pipeline is doing against different harm categories and to be able to compare that baseline to future iterations of their model. This allows them to have empirical data on how well their model is doing today, and detect any degradation of performance based on future improvements.
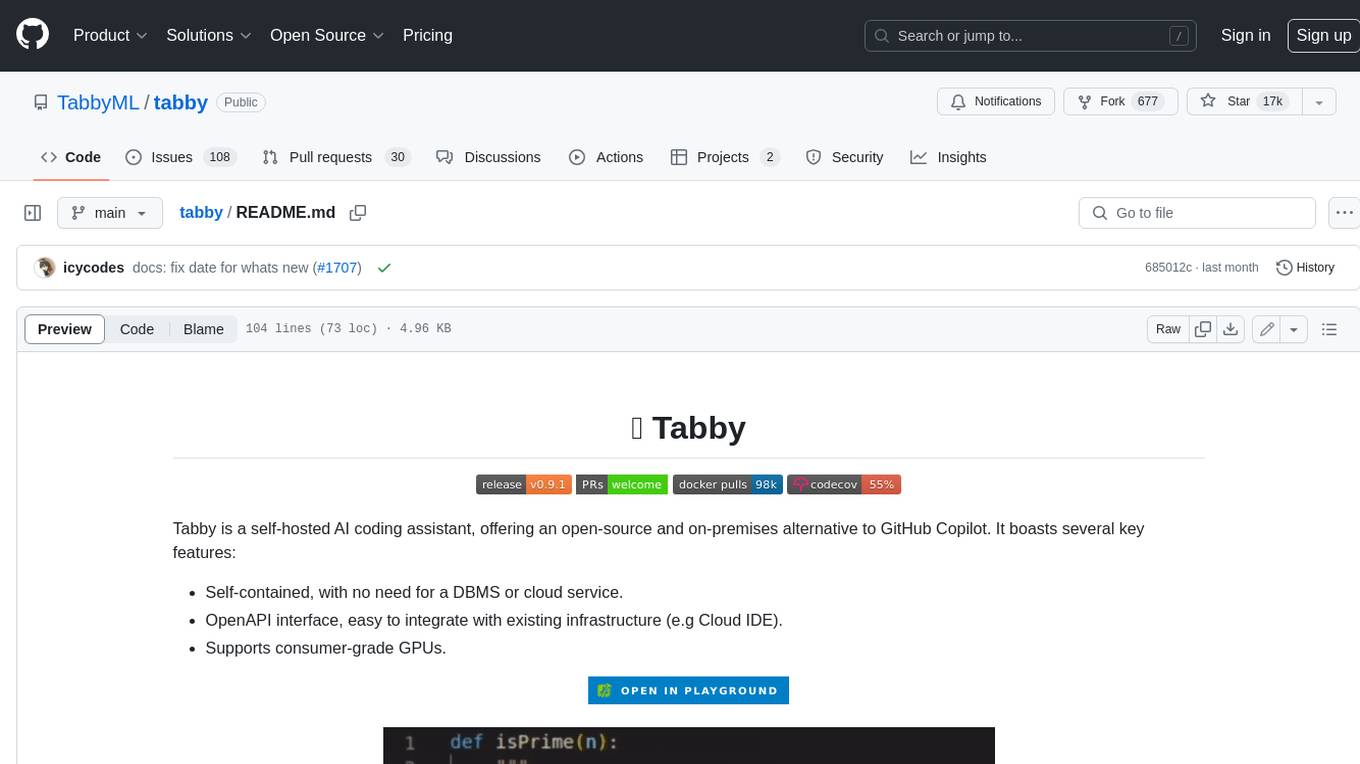
tabby
Tabby is a self-hosted AI coding assistant, offering an open-source and on-premises alternative to GitHub Copilot. It boasts several key features: * Self-contained, with no need for a DBMS or cloud service. * OpenAPI interface, easy to integrate with existing infrastructure (e.g Cloud IDE). * Supports consumer-grade GPUs.
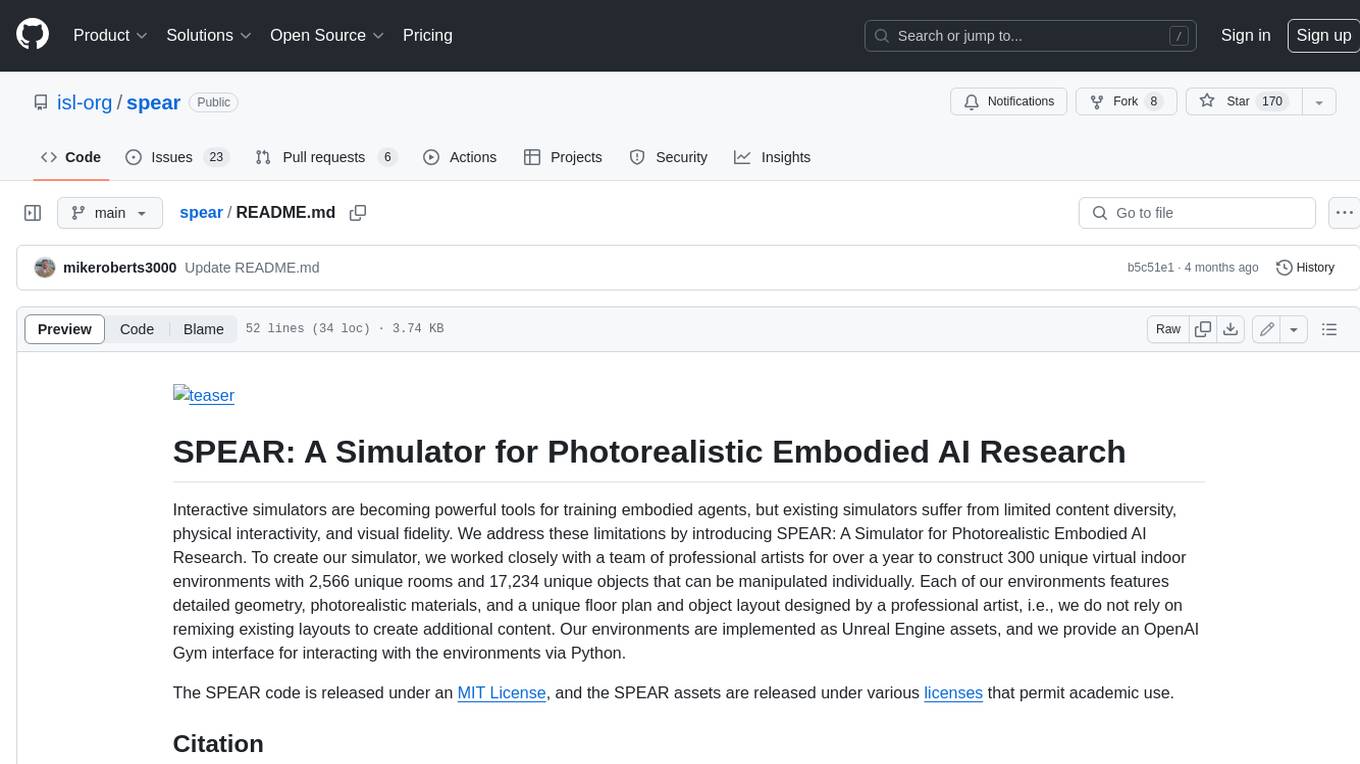
spear
SPEAR (Simulator for Photorealistic Embodied AI Research) is a powerful tool for training embodied agents. It features 300 unique virtual indoor environments with 2,566 unique rooms and 17,234 unique objects that can be manipulated individually. Each environment is designed by a professional artist and features detailed geometry, photorealistic materials, and a unique floor plan and object layout. SPEAR is implemented as Unreal Engine assets and provides an OpenAI Gym interface for interacting with the environments via Python.
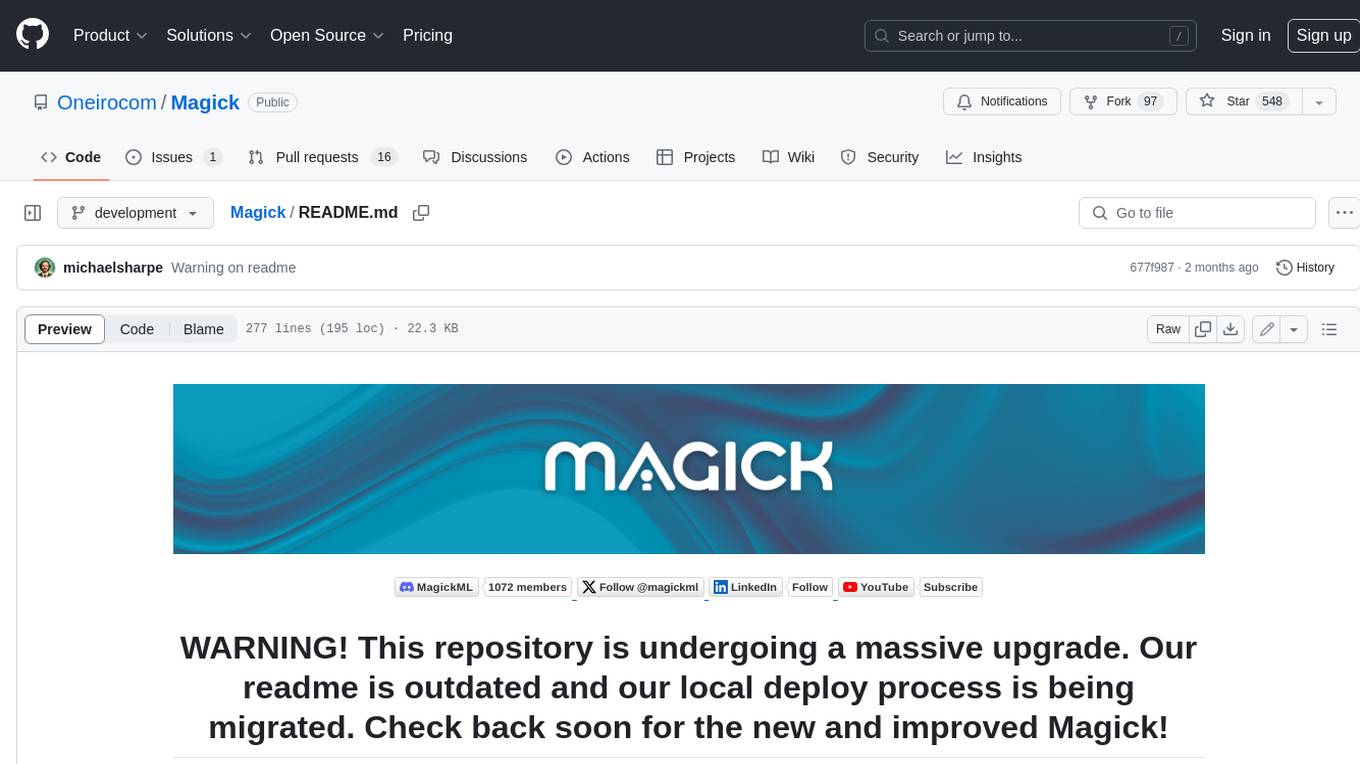
Magick
Magick is a groundbreaking visual AIDE (Artificial Intelligence Development Environment) for no-code data pipelines and multimodal agents. Magick can connect to other services and comes with nodes and templates well-suited for intelligent agents, chatbots, complex reasoning systems and realistic characters.