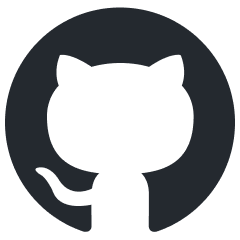
AMD-AI
AMD (Radeon GPU) ROCm based setup for popular AI tools on Ubuntu 24.04
Stars: 141
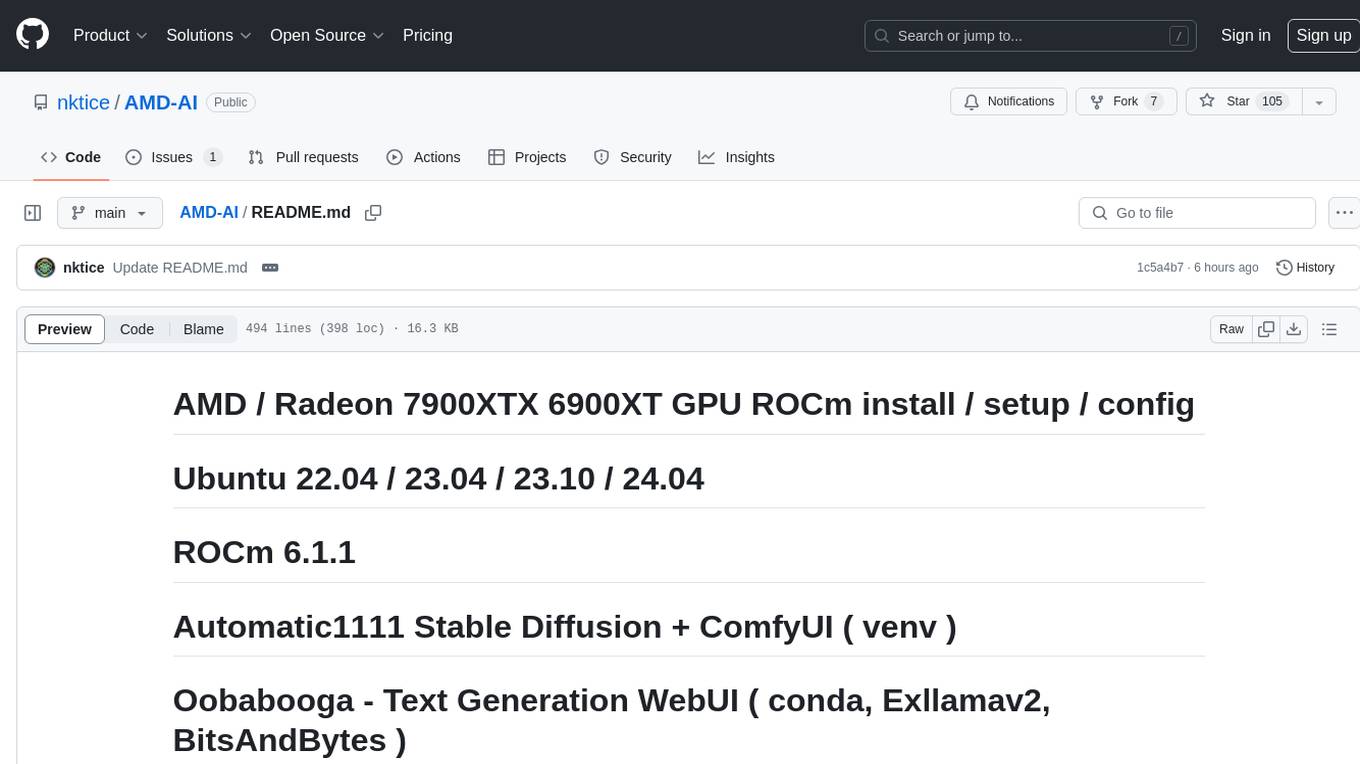
AMD-AI is a repository containing detailed instructions for installing, setting up, and configuring ROCm on Ubuntu systems with AMD GPUs. The repository includes information on installing various tools like Stable Diffusion, ComfyUI, and Oobabooga for tasks like text generation and performance tuning. It provides guidance on adding AMD GPU package sources, installing ROCm-related packages, updating system packages, and finding graphics devices. The instructions are aimed at users with AMD hardware looking to set up their Linux systems for AI-related tasks.
README:
This file is focused on the current stable version of PyTorch. There is another variation of these instructions for the development / nightly version(s) here : https://github.com/nktice/AMD-AI/blob/main/dev.md
2023-07 - I have composed this collection of instructions as they are my notes. I use this setup my own Linux system with AMD parts. I've gone over these doing many re-installs to get them all right. This is what I had hoped to find when I had search for install instructions - so I'm sharing them in the hopes that they save time for other people. There may be in here extra parts that aren't needed but this works for me. Originally text, with comments like a shell script that I cut and paste.
2023-09-09 - I had a report that this doesn't work in virtual machines (virtualbox) as the system there cannot see the hardware, it can't load drivers, etc. While this is not a guide about Windows, Windows users may find it more helpful to try DirectML - https://rocm.docs.amd.com/en/latest/deploy/windows/quick_start.html / https://github.com/lshqqytiger/stable-diffusion-webui-directml
[ ... updates abridged ... ]
2024-07-24 - PyTorch has updated with 2.4 now stable and referring to ROCm 6.1, so there's updates here to reflect those changes.
2024-08-04 - ROCm 6.2 is out, including support for the current version of Ubuntu (24.04 / Noble) so this revision includes changes to emphasize use of the new version. Previous stable has been set aside here - https://github.com/nktice/AMD-AI/blob/main/ROCm-6.1.3-Stable.md - Note I'm getting errors with the 2nd GPU with the new ROCm, bug report is filed, here is a link to that thread so you can follow : https://github.com/ROCm/ROCm/issues/3518
ROCm 6.2 includes support for Ubuntu 24.04 (noble).
At this point we assume you've done the system install and you know what that is, have a user, root, etc.
# update system packages
sudo apt update -y && sudo apt upgrade -y
#turn on devel and sources.
sudo apt-add-repository -y -s -s
sudo apt install -y "linux-headers-$(uname -r)" \
"linux-modules-extra-$(uname -r)"
This allows calls to older versions of Python by using "deadsnakes"
sudo add-apt-repository ppa:deadsnakes/ppa -y
sudo apt update -y
Make the directory if it doesn't exist yet. This location is recommended by the distribution maintainers.
sudo mkdir --parents --mode=0755 /etc/apt/keyrings
Download the key, convert the signing-key to a full Keyring required by apt and store in the keyring directory
wget https://repo.radeon.com/rocm/rocm.gpg.key -O - | \
gpg --dearmor | sudo tee /etc/apt/keyrings/rocm.gpg > /dev/null
amdgpu repository
echo 'deb [arch=amd64 signed-by=/etc/apt/keyrings/rocm.gpg] https://repo.radeon.com/amdgpu/6.2/ubuntu noble main' \
| sudo tee /etc/apt/sources.list.d/amdgpu.list
sudo apt update -y
AMDGPU DKMS
sudo apt install -y amdgpu-dkms
https://rocmdocs.amd.com/en/latest/deploy/linux/os-native/install.html
echo "deb [arch=amd64 signed-by=/etc/apt/keyrings/rocm.gpg] https://repo.radeon.com/rocm/apt/6.2 noble main" \
| sudo tee --append /etc/apt/sources.list.d/rocm.list
echo -e 'Package: *\nPin: release o=repo.radeon.com\nPin-Priority: 600' \
| sudo tee /etc/apt/preferences.d/rocm-pin-600
sudo apt update -y
This is lots of stuff, but comparatively small so worth including, as some stuff later may want as dependencies without much notice.
# ROCm...
sudo apt install -y rocm-dev rocm-libs rocm-hip-sdk rocm-libs
# ld.so.conf update
sudo tee --append /etc/ld.so.conf.d/rocm.conf <<EOF
/opt/rocm/lib
/opt/rocm/lib64
EOF
sudo ldconfig
# update path
echo "PATH=/opt/rocm/bin:/opt/rocm/opencl/bin:$PATH" >> ~/.profile
sudo /opt/rocm/bin/rocminfo | grep gfx
My 6900 reported as gfx1030, and my 7900 XTX show up as gfx1100
Of course note to change the user name to match your user.
sudo adduser `whoami` video
sudo adduser `whoami` render
# git and git-lfs (large file support
sudo apt install -y git git-lfs
# development tool may be required later...
sudo apt install -y libstdc++-12-dev
# stable diffusion likes TCMalloc...
sudo apt install -y libtcmalloc-minimal4
This section is optional, and as such has been moved to performance-tuning
nvtop Note : I have had issues with the distro version crashes with 2 GPUs, installing new version from sources works fine. Instructions for that are included at the bottom, as they depend on things installed between here and there. Project website : https://github.com/Syllo/nvtop
sudo apt install -y nvtop
sudo apt install -y radeontop rovclock
sudo reboot
This system is built to use its own venv ( rather than Conda )...
https://github.com/AUTOMATIC1111/stable-diffusion-webui Get the files...
cd
git clone https://github.com/AUTOMATIC1111/stable-diffusion-webui.git
cd stable-diffusion-webui
The 1.9.x+ release series breaks the API so that it won't work with Oobabooga's TGW - so the following resets to use the 1.8.0 relaase that does work with Oobabooga.
2024-07-04 - Oobabooga 1.9 resolves this issue - these lines are remarked out for now, but preserved in case someone wants to see how to do something similar in the future...
# git checkout bef51ae
# git reset --hard
sudo apt install -y wget git python3.10 python3.10-venv libgl1
python3.10 -m venv venv
source venv/bin/activate
python3.10 -m pip install -U pip
deactivate
tee --append webui-user.sh <<EOF
# specify compatible python version
python_cmd="python3.10"
## Torch for ROCm
# workaround for ROCm + Torch > 2.4.x - https://github.com/comfyanonymous/ComfyUI/issues/3698
export TORCH_BLAS_PREFER_HIPBLASLT=0
# generic import...
# export TORCH_COMMAND="pip install torch torchvision --index-url https://download.pytorch.org/whl/nightly/rocm6.1"
# use specific versions to avoid downloading all the nightlies... ( update dates as needed )
export TORCH_COMMAND="pip install --pre torch torchvision --index-url https://download.pytorch.org/whl/rocm6.1"
## And if you want to call this from other programs...
export COMMANDLINE_ARGS="--api"
## crashes with 2 cards, so to get it to run on the second card (only), unremark the following
# export CUDA_VISIBLE_DEVICES="1"
EOF
If you don't do this, it will install a default to get you going. Note that these start files do include things that it needs you'll want to copy into the folder where you have other models ( to avoid issues )
#mv models models.1
#ln -s /path/to/models models
Note that the first time it starts it may take it a while to go and get things it's not always good about saying what it's up to.
./webui.sh
- variation of https://raw.githubusercontent.com/ltdrdata/ComfyUI-Manager/main/scripts/install-comfyui-venv-linux.sh Includes ComfyUI-Manager
Same install of packages here as for Stable Diffusion ( included here in case you're not installed SD and just want ComfyUI... )
sudo apt install -y wget git python3 python3-venv libgl1
cd
git clone https://github.com/comfyanonymous/ComfyUI
cd ComfyUI/custom_nodes
git clone https://github.com/ltdrdata/ComfyUI-Manager
cd ..
python3 -m venv venv
source venv/bin/activate
# pre-install torch and torchvision from nightlies - note you may want to update versions...
python3 -m pip install --pre torch torchvision --index-url https://download.pytorch.org/whl/rocm6.1
python3 -m pip install -r requirements.txt --extra-index-url https://download.pytorch.org/whl/rocm6.1
python3 -m pip install -r custom_nodes/ComfyUI-Manager/requirements.txt --extra-index-url https://download.pytorch.org/whl/rocm6.1
# end vend if needed...
deactivate
Scripts for running the program...
# run_gpu.sh
tee --append run_gpu.sh <<EOF
#!/bin/bash
source venv/bin/activate
python3 main.py --preview-method auto
EOF
chmod +x run_gpu.sh
#run_cpu.sh
tee --append run_cpu.sh <<EOF
#!/bin/bash
source venv/bin/activate
python3 main.py --preview-method auto --cpu
EOF
chmod +x run_cpu.sh
Update the config file to point to Stable Diffusion (presuming it's installed...)
# config file - connecto stable-diffusion-webui
cp extra_model_paths.yaml.example extra_model_paths.yaml
sed -i "s@path/to@`echo ~`@g" extra_model_paths.yaml
# edit config file to point to your checkpoints etc
#vi extra_model_paths.yaml
Project Website : https://github.com/oobabooga/text-generation-webui.git
First we'll need Conda ... Required for pytorch... Conda provides virtual environments for python, so that programs with different dependencies can have different environments. Here is more info on managing conda : https://docs.conda.io/projects/conda/en/latest/user-guide/getting-started.html# Other notes : https://docs.conda.io/projects/conda/en/latest/user-guide/install/linux.html Download info : https://www.anaconda.com/download/
Anaconda ( if you prefer this to miniconda below )
#cd ~/Downloads/
#wget https://repo.anaconda.com/archive/Anaconda3-2023.09-0-Linux-x86_64.sh
#bash Anaconda3-2023.09-0-Linux-x86_64.sh -b
#cd ~
#ln -s anaconda3 conda
Miniconda ( if you prefer this to Anaconda above... ) [ https://docs.conda.io/projects/miniconda/en/latest/ ]
cd ~/Downloads/
wget https://repo.anaconda.com/miniconda/Miniconda3-latest-Linux-x86_64.sh
bash Miniconda3-latest-Linux-x86_64.sh -b
cd ~
ln -s miniconda3 conda
echo "PATH=~/conda/bin:$PATH" >> ~/.profile
source ~/.profile
conda update -y -n base -c defaults conda
conda install -y cmake ninja
conda init
source ~/.profile
sudo apt install -y pip
pip3 install --upgrade pip
## show outdated packages...
#pip list --outdated
## check dependencies
#pip check
## install specified bersion
#pip install <packagename>==<version>
conda create -n textgen python=3.11 -y
conda activate textgen
# pre-install
pip install --pre cmake colorama filelock lit numpy Pillow Jinja2 \
mpmath fsspec MarkupSafe certifi filelock networkx \
sympy packaging requests \
--index-url https://download.pytorch.org/whl/rocm6.1
There's version conflicts, so we specify versions that we want installed -
#pip install --pre torch torchvision torchtext torchaudio triton pytorch-triton-rocm \
#pip install --pre torch==2.3.1+rocm6.0 torchvision==0.18.1+rocm6.0 torchaudio==2.3.1 triton pytorch-triton-rocm \
# --index-url https://download.pytorch.org/whl/rocm6.0
pip install --pre torch==2.4.0+rocm6.1 torchvision==0.19.0+rocm6.1 torchaudio==2.4.0 triton pytorch-triton-rocm \
--index-url https://download.pytorch.org/whl/rocm6.1
2024-05-12 For some odd reason, torchtext isn't recognized, even though it's there... so we specify it using it's URL to be explicit.
pip install https://download.pytorch.org/whl/cpu/torchtext-0.18.0%2Bcpu-cp311-cp311-linux_x86_64.whl#sha256=c760e672265cd6f3e4a7c8d4a78afe9e9617deacda926a743479ee0418d4207d
2024-04-24 - AMD's own ROCm version of bitsandbytes has been updated! - https://github.com/ROCm/bitsandbytes ( ver 0.44.0.dev0 at time of writing )
cd
git clone https://github.com/ROCm/bitsandbytes.git
cd bitsandbytes
pip install .
cd
git clone https://github.com/oobabooga/text-generation-webui
cd text-generation-webui
cd
git clone https://github.com/oobabooga/text-generation-webui
cd text-generation-webui
2024-07-26 Oobabooga release 1.12 changed how requirements are done, including calls that refer to old versions of PyTorch which didn't work for me... So the usual command here is remarked out, and I have instead offered a replacement requirements.txt with minimal includes, that combined with what else is here gets it up and running ( for me ), using more recent versions of packages.
#pip install -r requirements_amd.txt
tee --append requirements_amdai.txt <<EOF
# alternate simplified requirements from https://github.com/nktice/AMD-AI
accelerate>=0.32
colorama
datasets
einops
gradio>=4.26
hqq>=0.1.7.post3
jinja2>=3.1.4
lm_eval>=0.3.0
markdown
numba>=0.59
numpy>=1.26
optimum>=1.17
pandas
peft>=0.8
Pillow>=9.5.0
psutil
pyyaml
requests
rich
safetensors>=0.4
scipy
sentencepiece
tensorboard
transformers>=4.43
tqdm
wandb
# API
SpeechRecognition>=3.10.0
flask_cloudflared>=0.0.14
sse-starlette>=1.6.5
tiktoken
EOF
pip install -r requirements_amdai.txt --extra-index-url https://download.pytorch.org/whl/nightly/rocm6.1
git clone https://github.com/turboderp/exllamav2 repositories/exllamav2
cd repositories/exllamav2
## Force collection back to base 0.0.11
## git reset --hard a4ecea6
pip install -r requirements.txt --extra-index-url https://download.pytorch.org/whl/rocm6.1
pip install . --index-url https://download.pytorch.org/whl/rocm6.1
cd ../..
2024-06-18 - Llama-cpp-python - Another loader, that is highly efficient in resource use, but not very fast. https://github.com/abetlen/llama-cpp-python It may need models in GGUF format ( and not other types ).
## remove old versions
pip uninstall llama_cpp_python -y
pip uninstall llama_cpp_python_cuda -y
## install llama-cpp-python
git clone --recurse-submodules https://github.com/abetlen/llama-cpp-python.git repositories/llama-cpp-python
cd repositories/llama-cpp-python
CC='/opt/rocm/llvm/bin/clang' CXX='/opt/rocm/llvm/bin/clang++' CFLAGS='-fPIC' CXXFLAGS='-fPIC' CMAKE_PREFIX_PATH='/opt/rocm' ROCM_PATH="/opt/rocm" HIP_PATH="/opt/rocm" CMAKE_ARGS="-GNinja -DLLAMA_HIPBLAS=ON -DLLAMA_AVX2=on " pip install --no-cache-dir .
cd ../..
Models : If you're new to this - new models can be downloaded from the shell via a python script, or from a form in the interface. There are lots of them - http://huggingface.co Generally the GPTQ models by TheBloke are likely to load... https://huggingface.co/TheBloke The 30B/33B models will load on 24GB of VRAM, but may error, or run out of memory depending on usage and parameters. Worthy of mention, TurboDerp ( author of the exllama loaders ) has been posting exllamav2 ( exl2 ) processed versions of models - https://huggingface.co/turboderp ( for use with exllamav2 loader ) - when downloading, note the --branch option.
To get new models note the ~/text-generation-webui directory has a program " download-model.py " that is made for downloading models from HuggingFace's collection.
If you have old models, link pre-stored models into the models
# cd ~/text-generation-webui
# mv models models.1
# ln -s /path/to/models models
Let's create a script (run.sh) to run the program...
tee --append run.sh <<EOF
#!/bin/bash
## activate conda
conda activate textgen
## command to run server...
python server.py --extensions sd_api_pictures send_pictures gallery
# if you want the server to listen on the local network so other machines can access it, add --listen.
#python server.py --listen --extensions sd_api_pictures send_pictures gallery
conda deactivate
EOF
chmod u+x run.sh
Note that to run the script :
source run.sh
Here's an example, nvtop, sd console, tgw console...
this screencap taken using ROCm 6.1.3 - under this config : https://github.com/nktice/AMD-AI/blob/main/ROCm-6.1.3-Dev.md
( As one from packages crashes on 2 GPUs, while this never version from sources works fine. ) project website : https://github.com/Syllo/nvtop optional - tool for displaying gpu / memory usage info The package for this crashes with 2 gpu's, here it is from source.
sudo apt install -y libdrm-dev libsystemd-dev libudev-dev
cd
git clone https://github.com/Syllo/nvtop.git
mkdir -p nvtop/build && cd nvtop/build
cmake .. -DNVIDIA_SUPPORT=OFF -DAMDGPU_SUPPORT=ON -DINTEL_SUPPORT=OFF
make
sudo make install
For Tasks:
Click tags to check more tools for each tasksFor Jobs:
Alternative AI tools for AMD-AI
Similar Open Source Tools
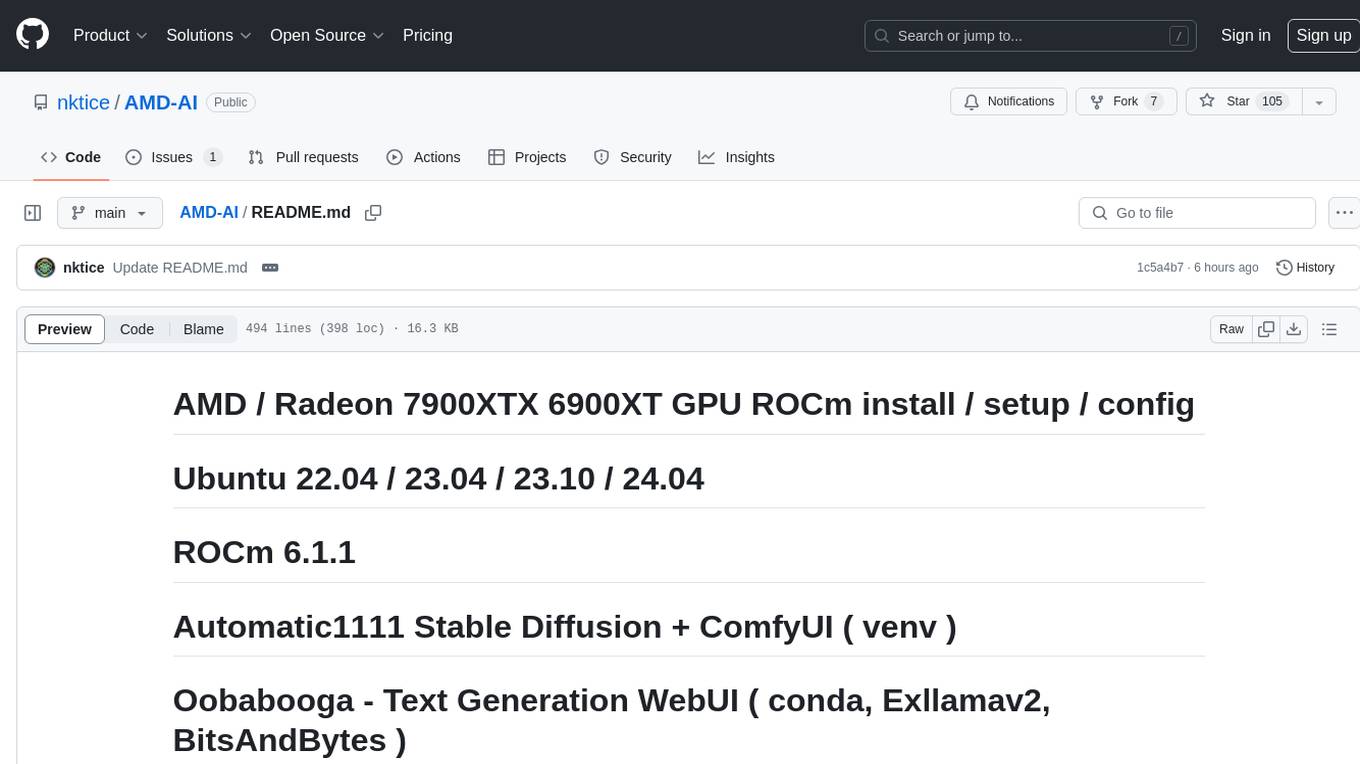
AMD-AI
AMD-AI is a repository containing detailed instructions for installing, setting up, and configuring ROCm on Ubuntu systems with AMD GPUs. The repository includes information on installing various tools like Stable Diffusion, ComfyUI, and Oobabooga for tasks like text generation and performance tuning. It provides guidance on adding AMD GPU package sources, installing ROCm-related packages, updating system packages, and finding graphics devices. The instructions are aimed at users with AMD hardware looking to set up their Linux systems for AI-related tasks.
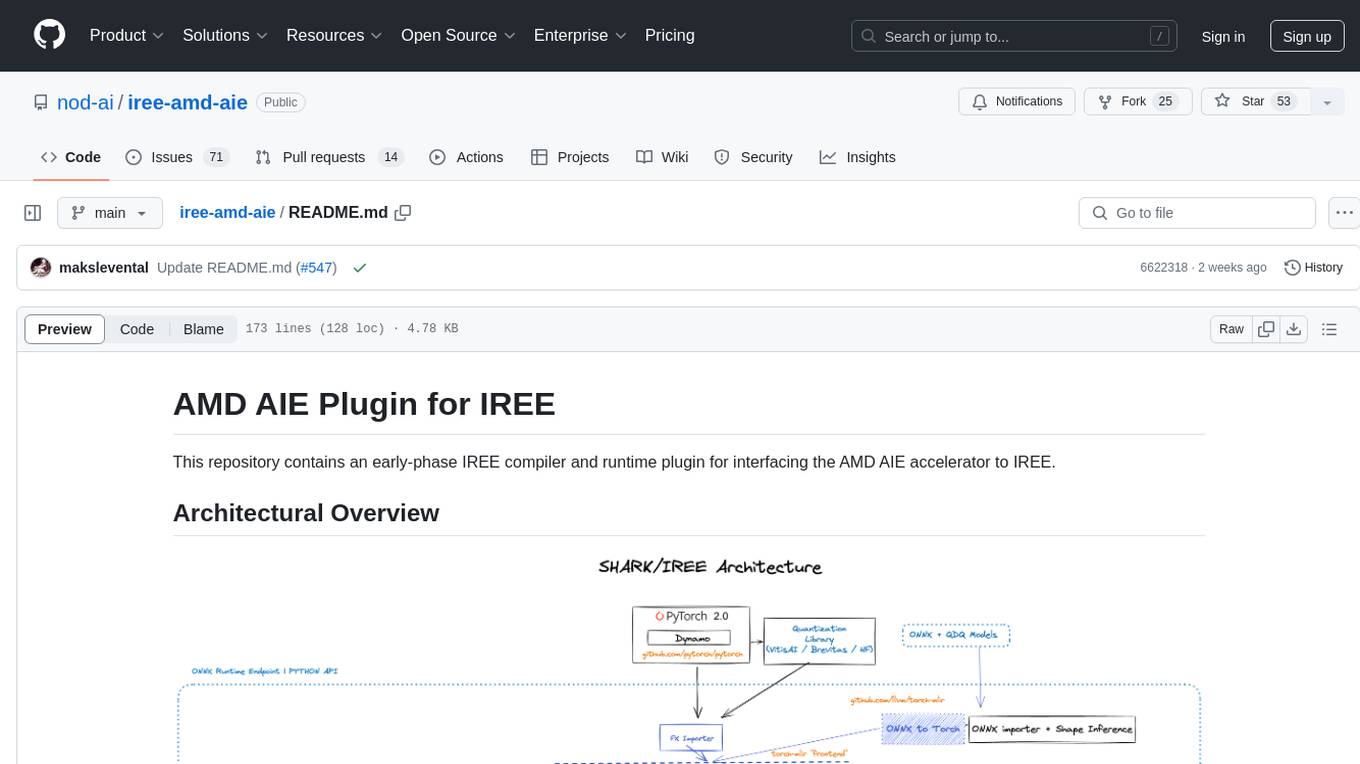
iree-amd-aie
This repository contains an early-phase IREE compiler and runtime plugin for interfacing the AMD AIE accelerator to IREE. It provides architectural overview, developer setup instructions, building guidelines, and runtime driver setup details. The repository focuses on enabling the integration of the AMD AIE accelerator with IREE, offering developers the tools and resources needed to build and run applications leveraging this technology.
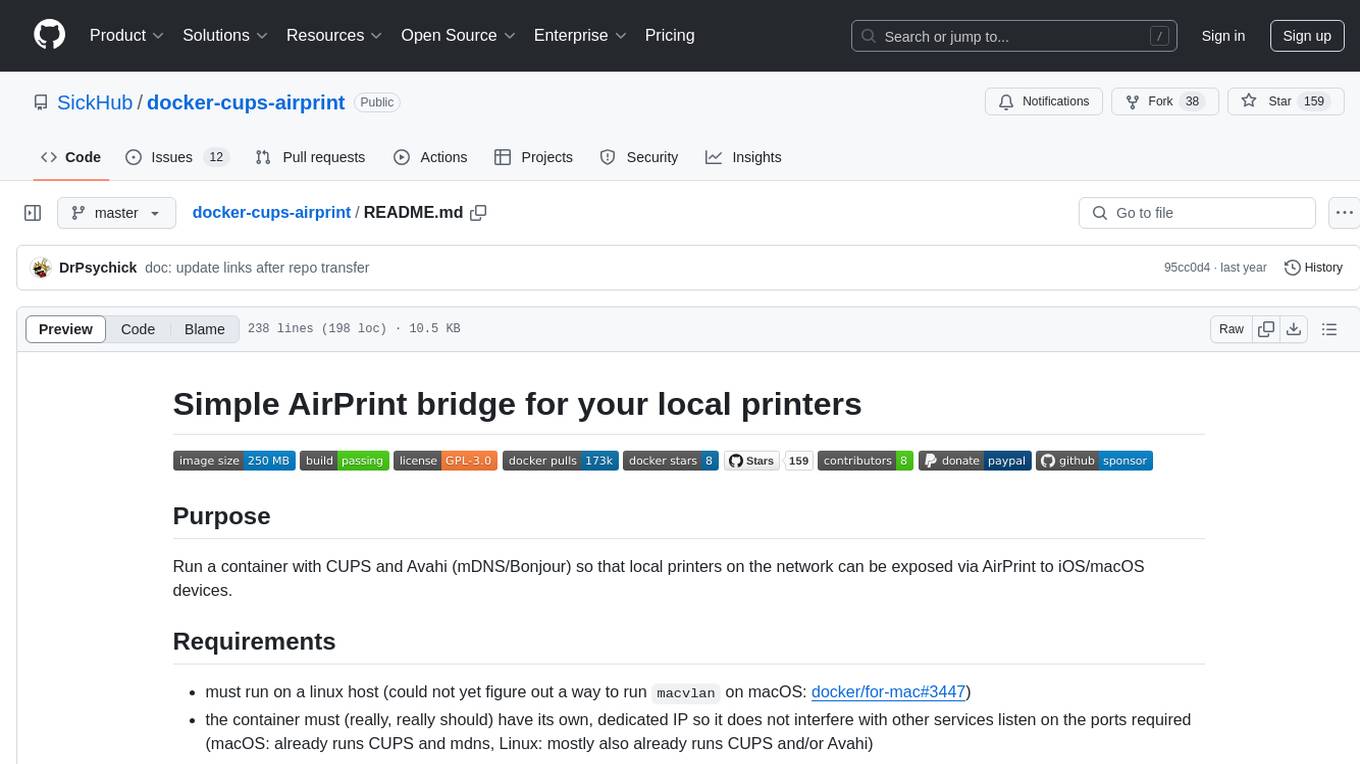
docker-cups-airprint
This repository provides a Docker image that acts as an AirPrint bridge for local printers, allowing them to be exposed to iOS/macOS devices. It runs a container with CUPS and Avahi to facilitate this functionality. Users must have CUPS drivers available for their printers. The tool requires a Linux host and a dedicated IP for the container to avoid interference with other services. It supports setting up printers through environment variables and offers options for automated configuration via command line, web interface, or files. The repository includes detailed instructions on setting up and testing the AirPrint bridge.
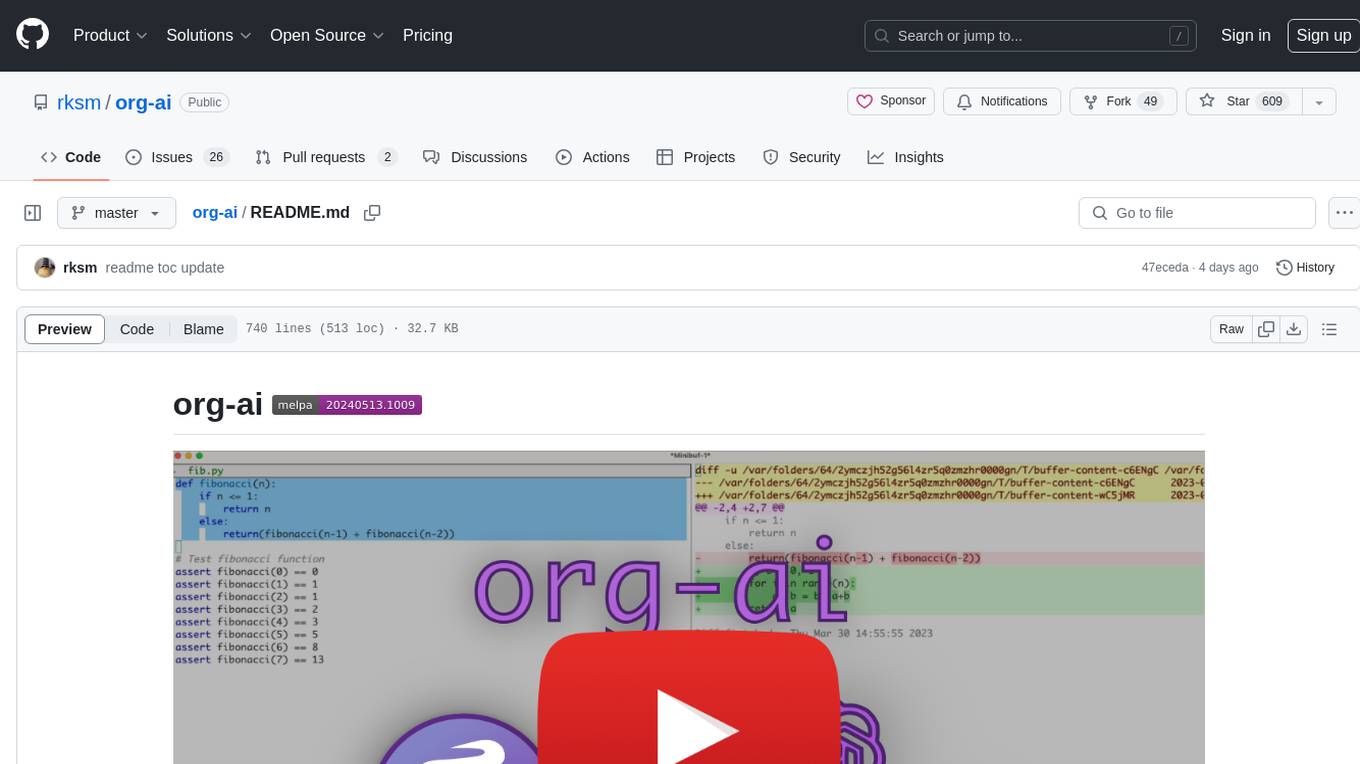
org-ai
org-ai is a minor mode for Emacs org-mode that provides access to generative AI models, including OpenAI API (ChatGPT, DALL-E, other text models) and Stable Diffusion. Users can use ChatGPT to generate text, have speech input and output interactions with AI, generate images and image variations using Stable Diffusion or DALL-E, and use various commands outside org-mode for prompting using selected text or multiple files. The tool supports syntax highlighting in AI blocks, auto-fill paragraphs on insertion, and offers block options for ChatGPT, DALL-E, and other text models. Users can also generate image variations, use global commands, and benefit from Noweb support for named source blocks.
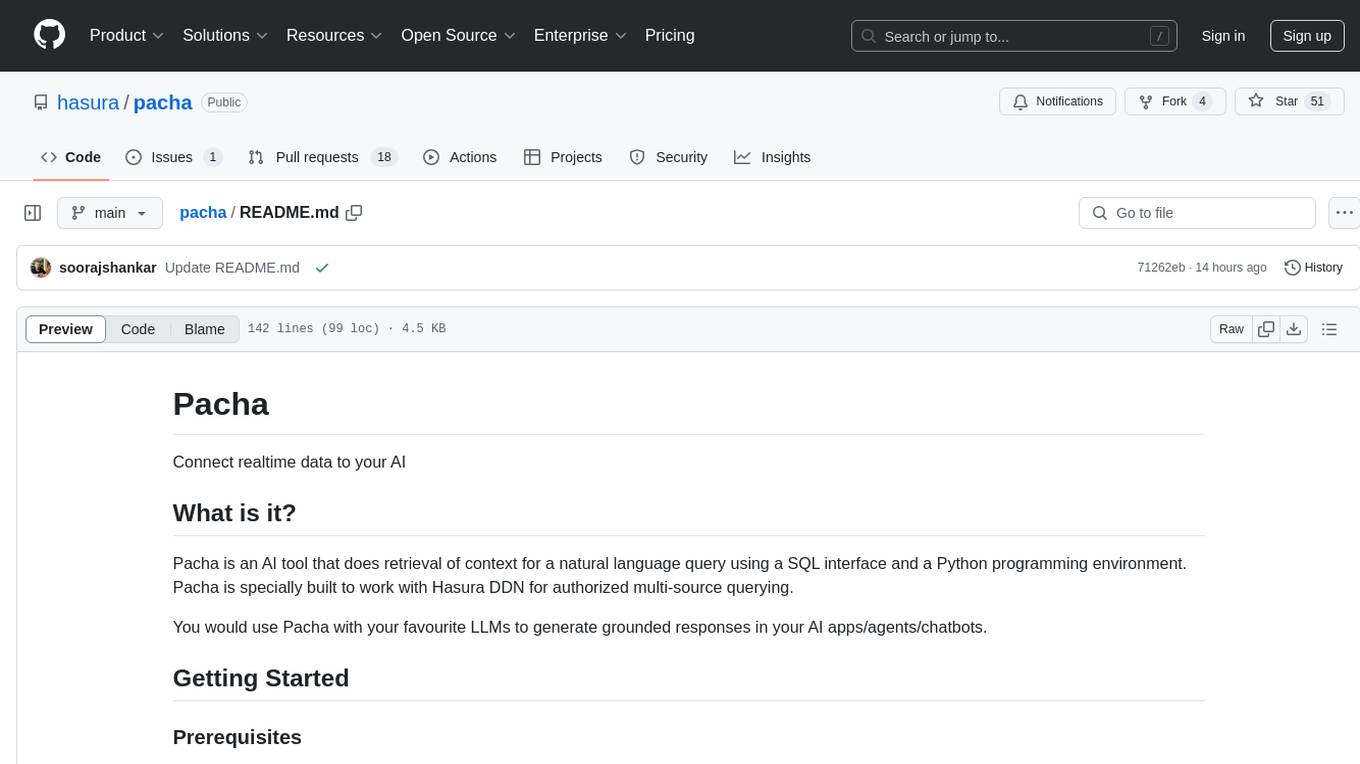
pacha
Pacha is an AI tool designed for retrieving context for natural language queries using a SQL interface and Python programming environment. It is optimized for working with Hasura DDN for multi-source querying. Pacha is used in conjunction with language models to produce informed responses in AI applications, agents, and chatbots.
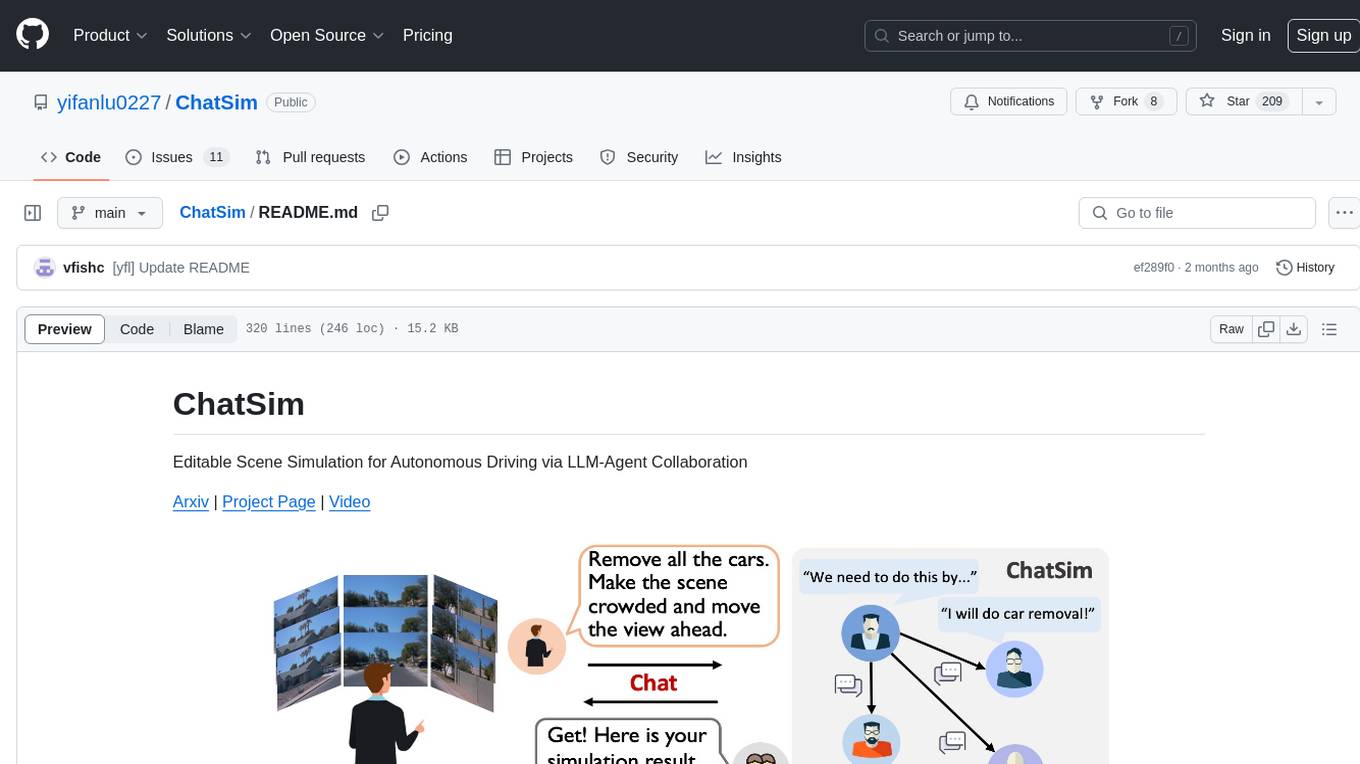
ChatSim
ChatSim is a tool designed for editable scene simulation for autonomous driving via LLM-Agent collaboration. It provides functionalities for setting up the environment, installing necessary dependencies like McNeRF and Inpainting tools, and preparing data for simulation. Users can train models, simulate scenes, and track trajectories for smoother and more realistic results. The tool integrates with Blender software and offers options for training McNeRF models and McLight's skydome estimation network. It also includes a trajectory tracking module for improved trajectory tracking. ChatSim aims to facilitate the simulation of autonomous driving scenarios with collaborative LLM-Agents.
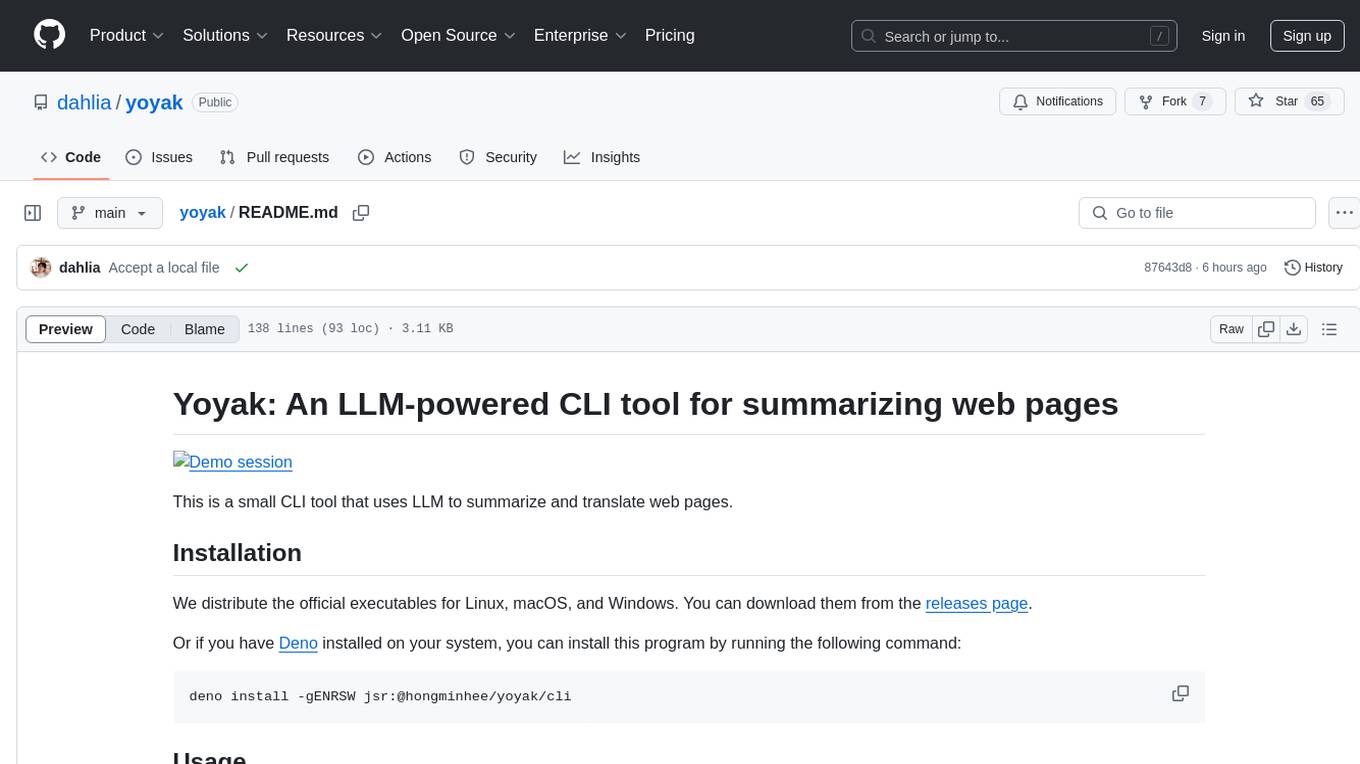
yoyak
Yoyak is a small CLI tool powered by LLM for summarizing and translating web pages. It provides shell completion scripts for bash, fish, and zsh. Users can set the model they want to use and summarize web pages with the 'yoyak summary' command. Additionally, translation to other languages is supported using the '-l' option with ISO 639-1 language codes. Yoyak supports various models for summarization and translation tasks.
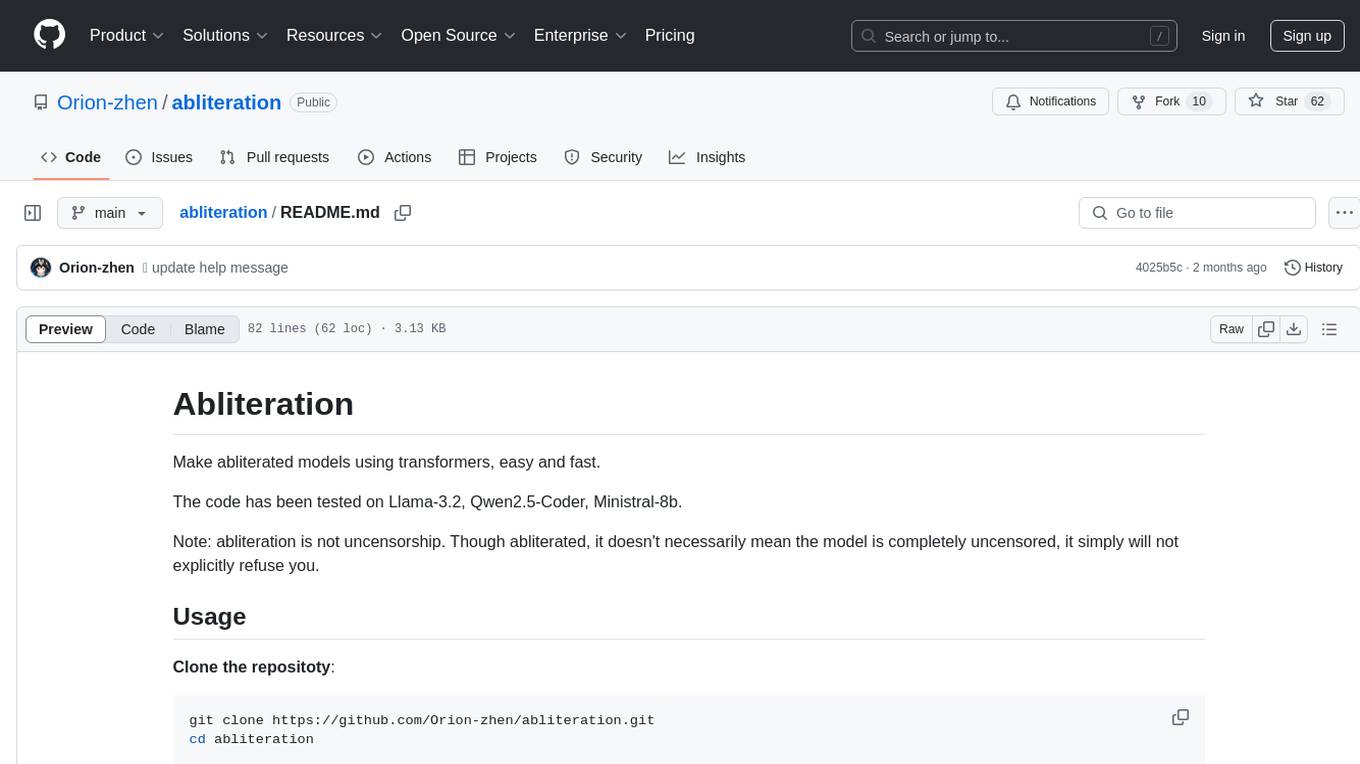
abliteration
Abliteration is a tool that allows users to create abliterated models using transformers quickly and easily. It is not a tool for uncensorship, but rather for making models that will not explicitly refuse users. Users can clone the repository, install dependencies, and make abliterations using the provided commands. The tool supports adjusting parameters for stubborn models and offers various options for customization. Abliteration can be used for creating modified models for specific tasks or topics.
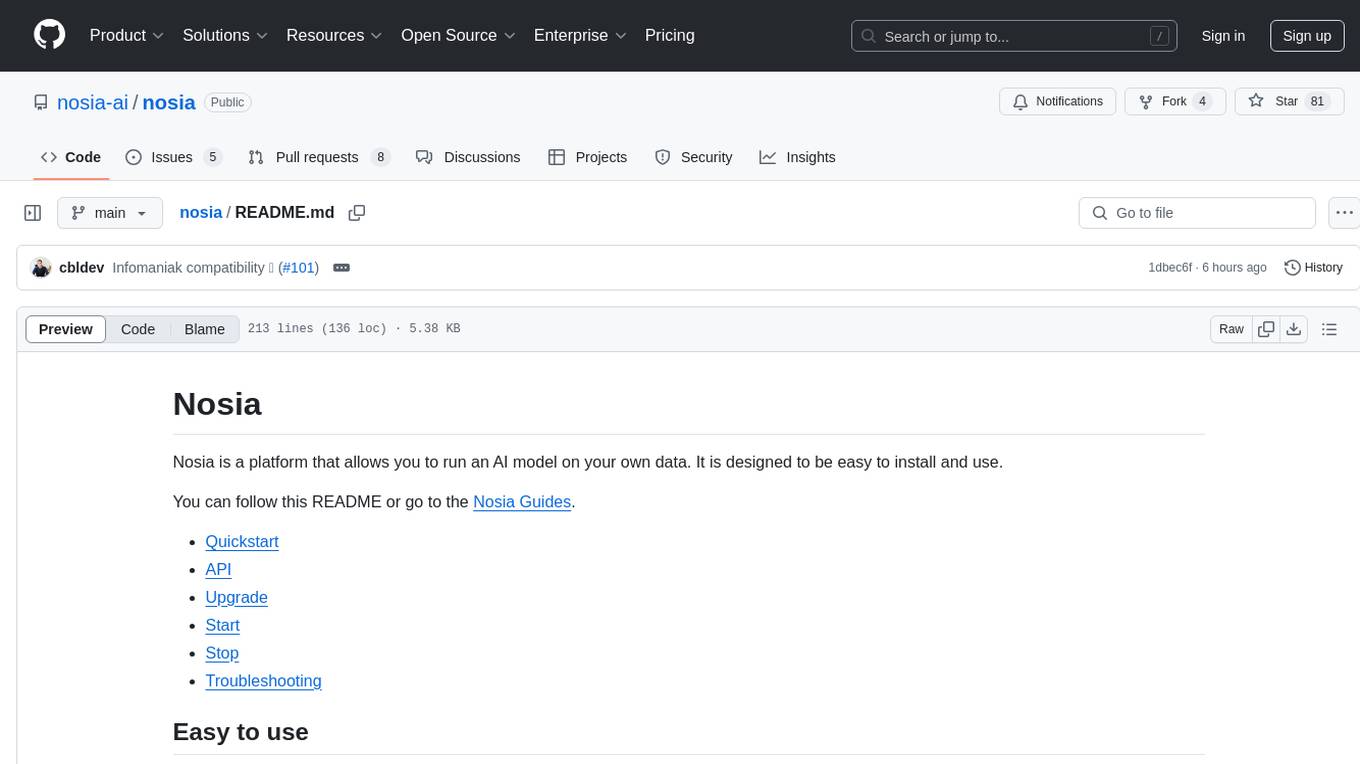
nosia
Nosia is a platform that allows users to run an AI model on their own data. It is designed to be easy to install and use. Users can follow the provided guides for quickstart, API usage, upgrading, starting, stopping, and troubleshooting. The platform supports custom installations with options for remote Ollama instances, custom completion models, and custom embeddings models. Advanced installation instructions are also available for macOS with a Debian or Ubuntu VM setup. Users can access the platform at 'https://nosia.localhost' and troubleshoot any issues by checking logs and job statuses.
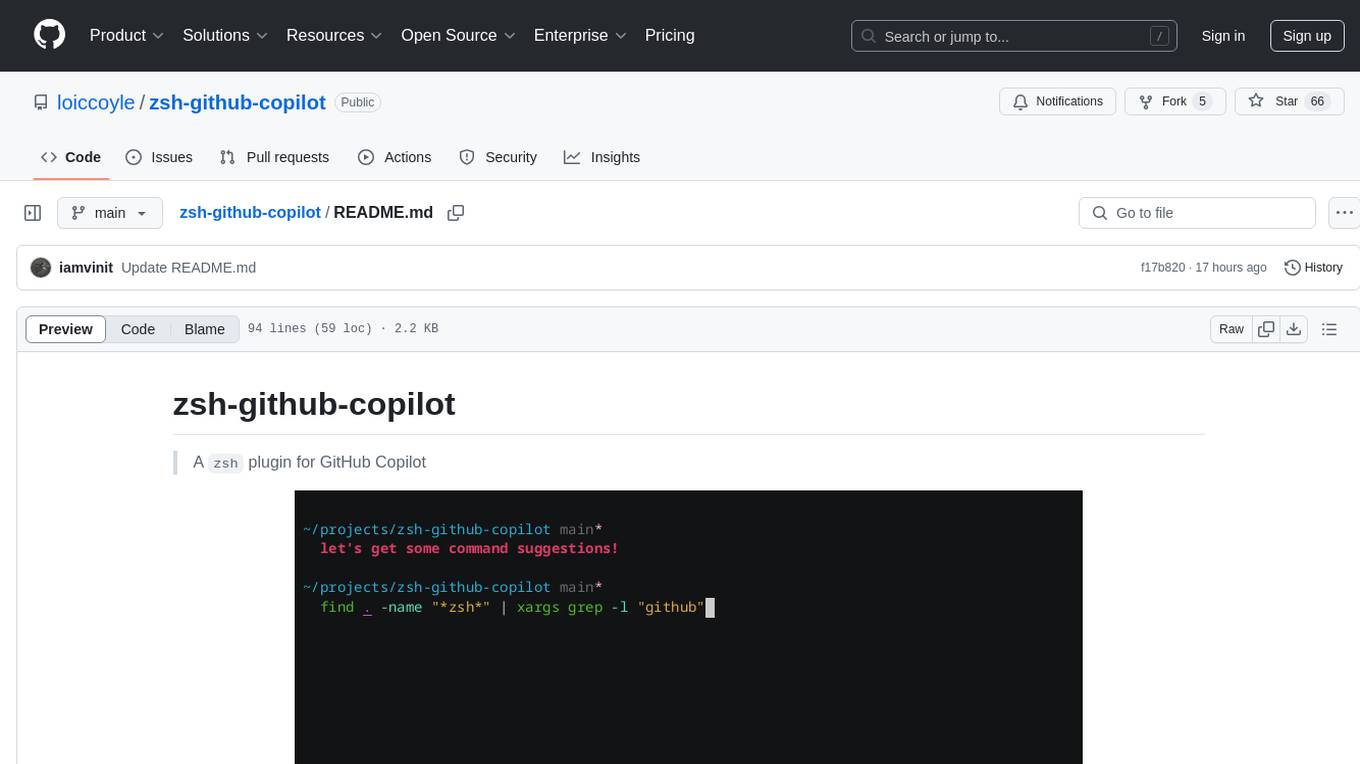
zsh-github-copilot
zsh-github-copilot is a `zsh` plugin that enhances the GitHub Copilot experience by providing keybinds to quickly access command explanations and get Copilot suggestions. It integrates seamlessly with GitHub CLI and offers a smooth setup process. Users can easily install the plugin using popular zsh plugin managers like antigen, oh-my-zsh, zinit, zplug, and zpm. By binding specific keys, users can access the 'suggest' and 'explain' functionalities to improve their coding workflow with GitHub Copilot. This plugin is designed to streamline the usage of GitHub Copilot within the zsh shell environment.
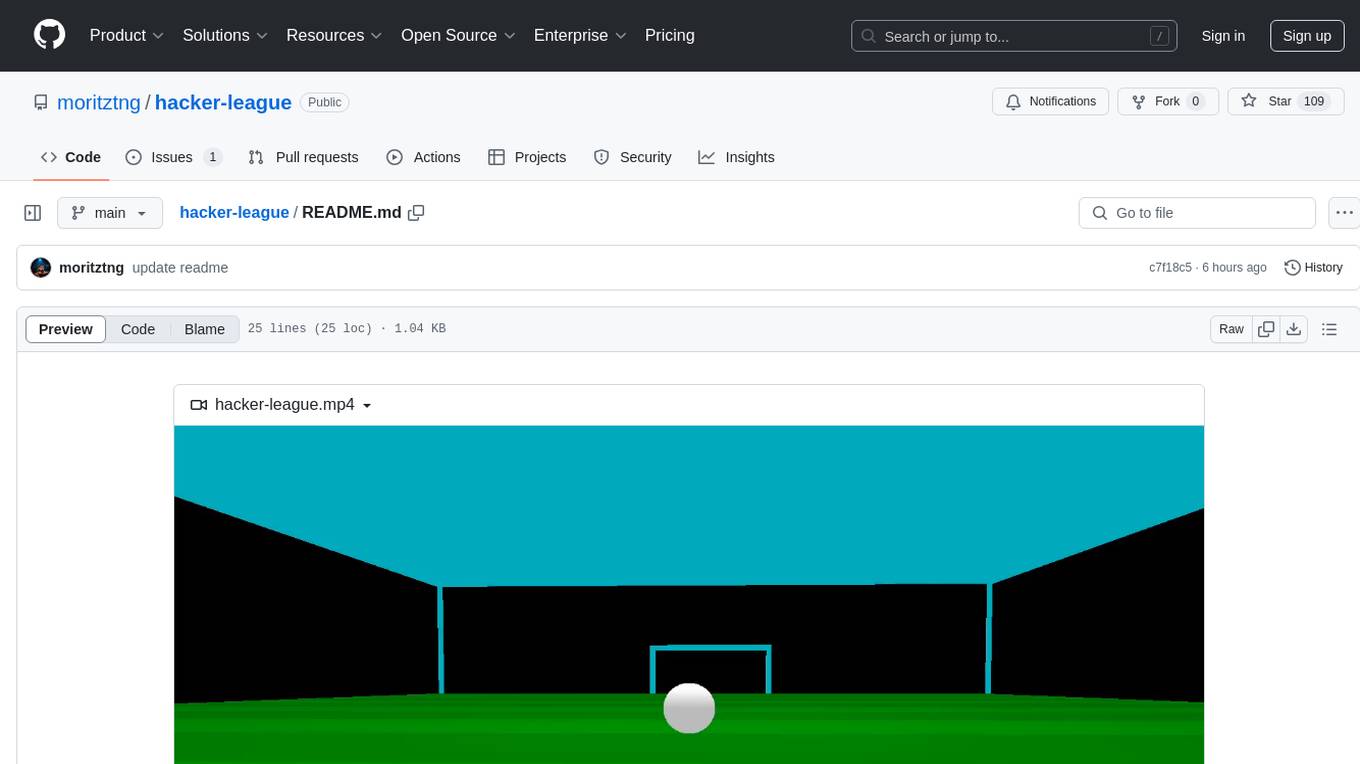
hacker-league
Hacker-league is a tool designed for gaming enthusiasts and developers to explore and play with game development. It provides a platform for users to build games, experiment with graphics, and enhance their coding skills. The tool offers features such as gamepad support, Vulkan API integration, shader compilation, and community engagement through Discord and public development showcases. Users can easily install the tool on Debian-based systems and contribute to its development for broader platform compatibility.
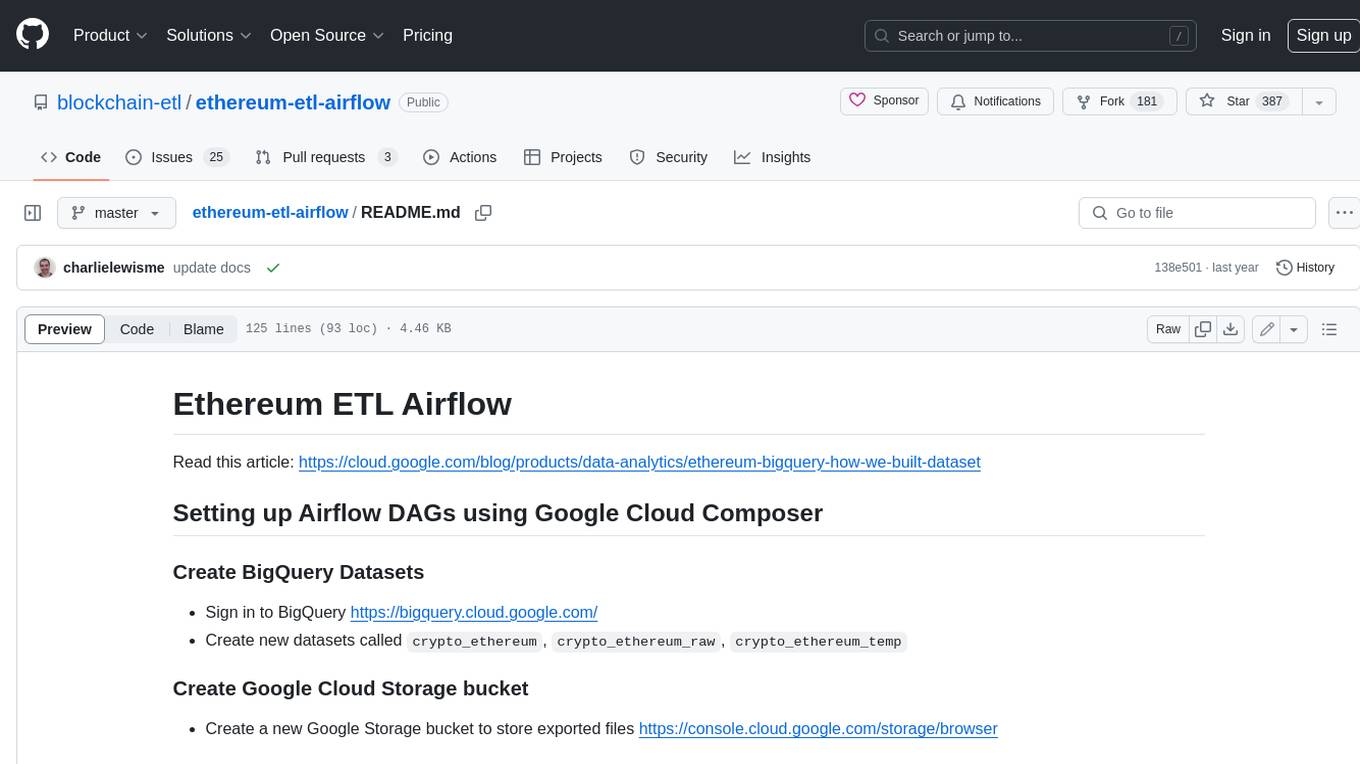
ethereum-etl-airflow
This repository contains Airflow DAGs for extracting, transforming, and loading (ETL) data from the Ethereum blockchain into BigQuery. The DAGs use the Google Cloud Platform (GCP) services, including BigQuery, Cloud Storage, and Cloud Composer, to automate the ETL process. The repository also includes scripts for setting up the GCP environment and running the DAGs locally.
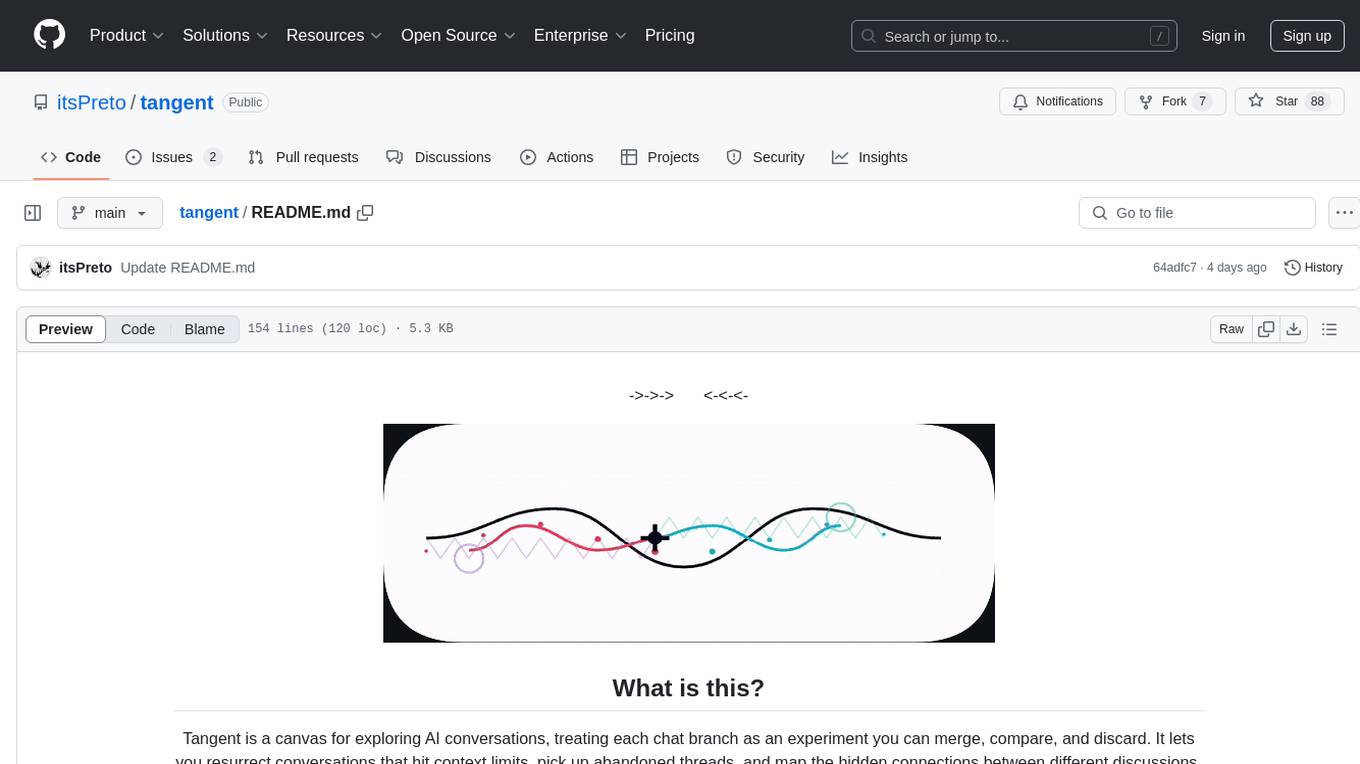
tangent
Tangent is a canvas for exploring AI conversations, allowing users to resurrect and continue conversations, branch and explore different ideas, organize conversations by topics, and support archive data exports. It aims to provide a visual/textual/audio exploration experience with AI assistants, offering a 'thoughts workbench' for experimenting freely, reviving old threads, and diving into tangents. The project structure includes a modular backend with components for API routes, background task management, data processing, and more. Prerequisites for setup include Whisper.cpp, Ollama, and exported archive data from Claude or ChatGPT. Users can initialize the environment, install Python packages, set up Ollama, configure local models, and start the backend and frontend to interact with the tool.
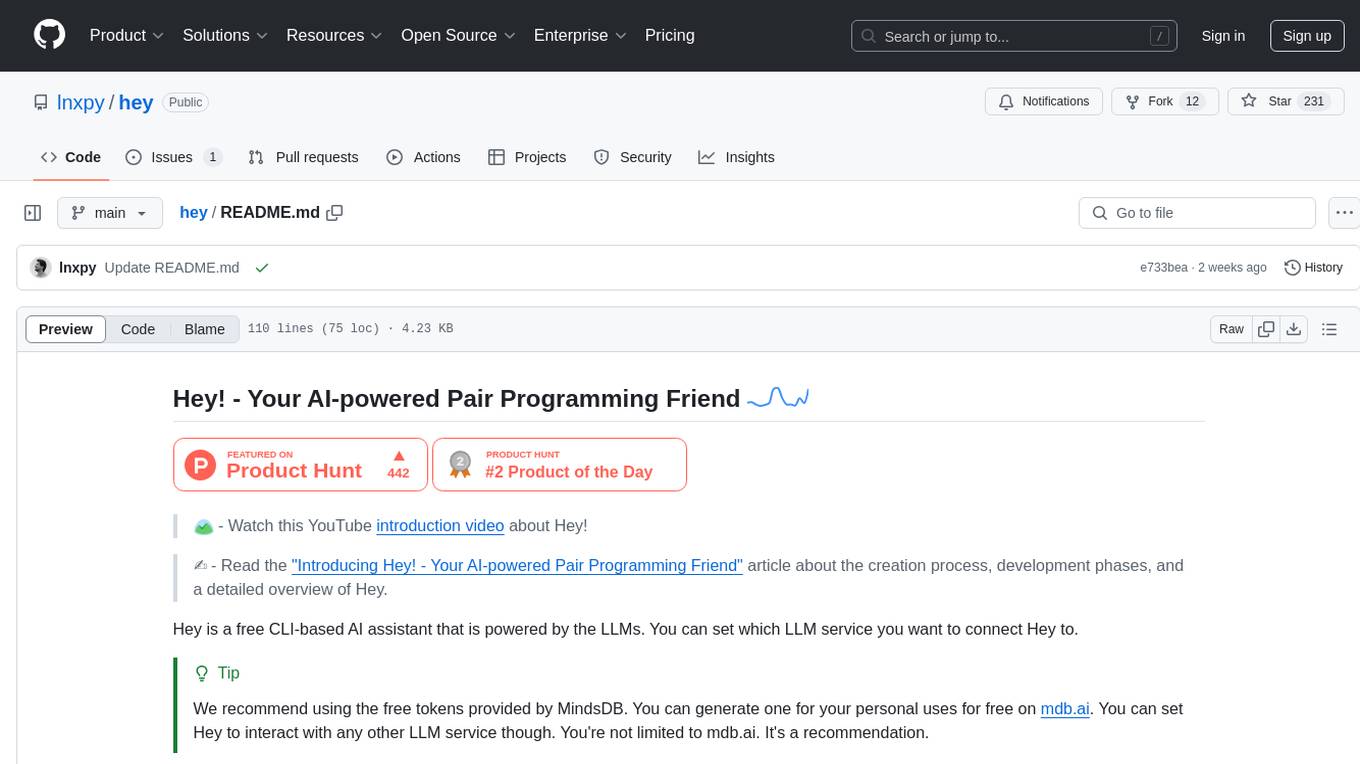
hey
Hey is a free CLI-based AI assistant powered by LLMs, allowing users to connect Hey to different LLM services. It provides commands for quick usage, customization options, and integration with code editors. Hey was created for a hackathon and is licensed under the MIT License.
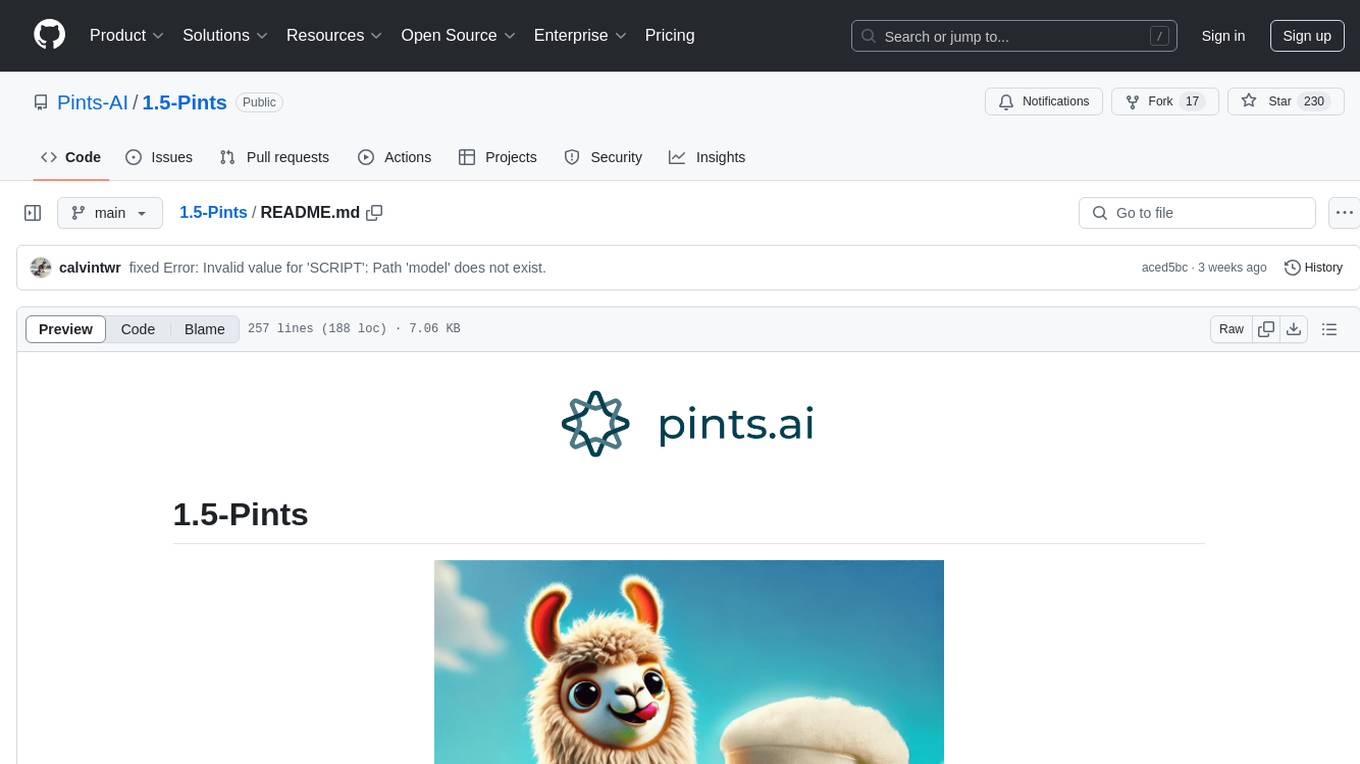
1.5-Pints
1.5-Pints is a repository that provides a recipe to pre-train models in 9 days, aiming to create AI assistants comparable to Apple OpenELM and Microsoft Phi. It includes model architecture, training scripts, and utilities for 1.5-Pints and 0.12-Pint developed by Pints.AI. The initiative encourages replication, experimentation, and open-source development of Pint by sharing the model's codebase and architecture. The repository offers installation instructions, dataset preparation scripts, model training guidelines, and tools for model evaluation and usage. Users can also find information on finetuning models, converting lit models to HuggingFace models, and running Direct Preference Optimization (DPO) post-finetuning. Additionally, the repository includes tests to ensure code modifications do not disrupt the existing functionality.
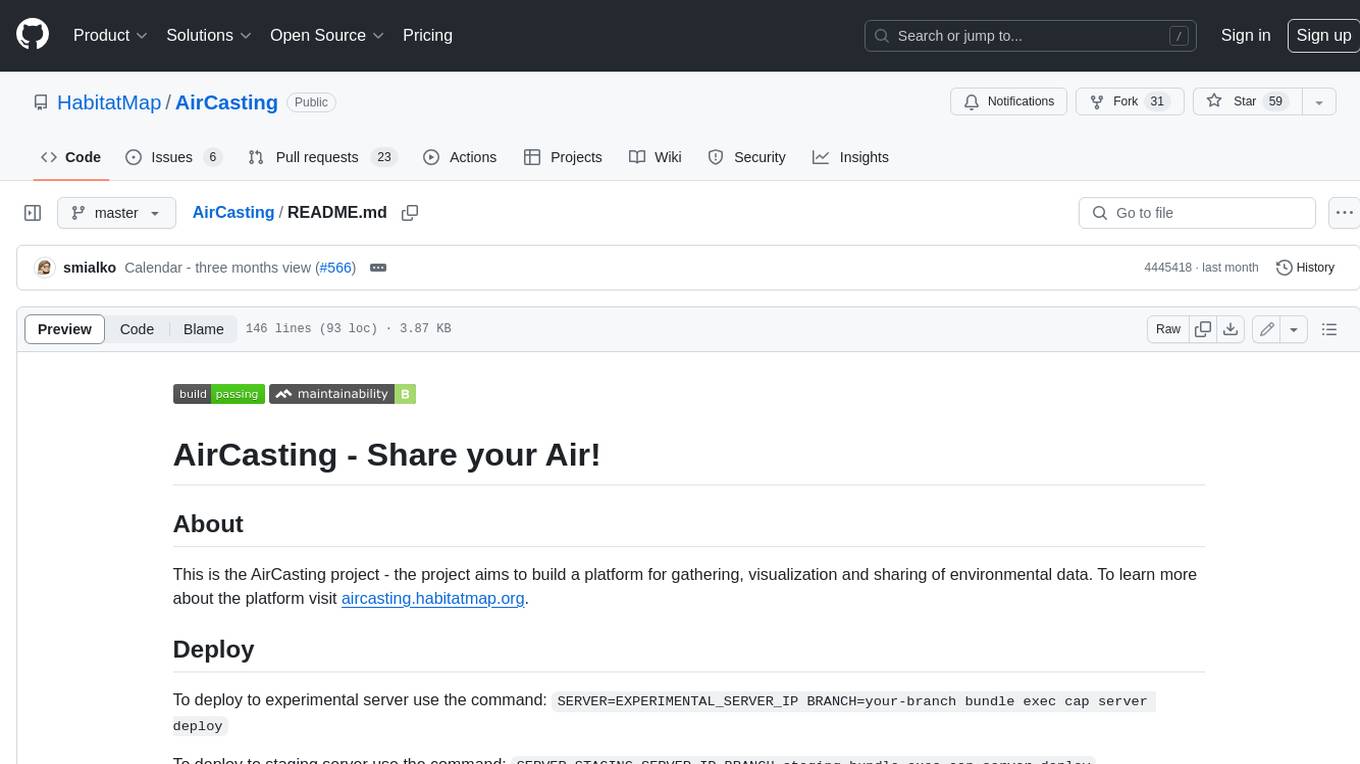
AirCasting
AirCasting is a platform for gathering, visualizing, and sharing environmental data. It aims to provide a central hub for environmental data, making it easier for people to access and use this information to make informed decisions about their environment.
For similar tasks
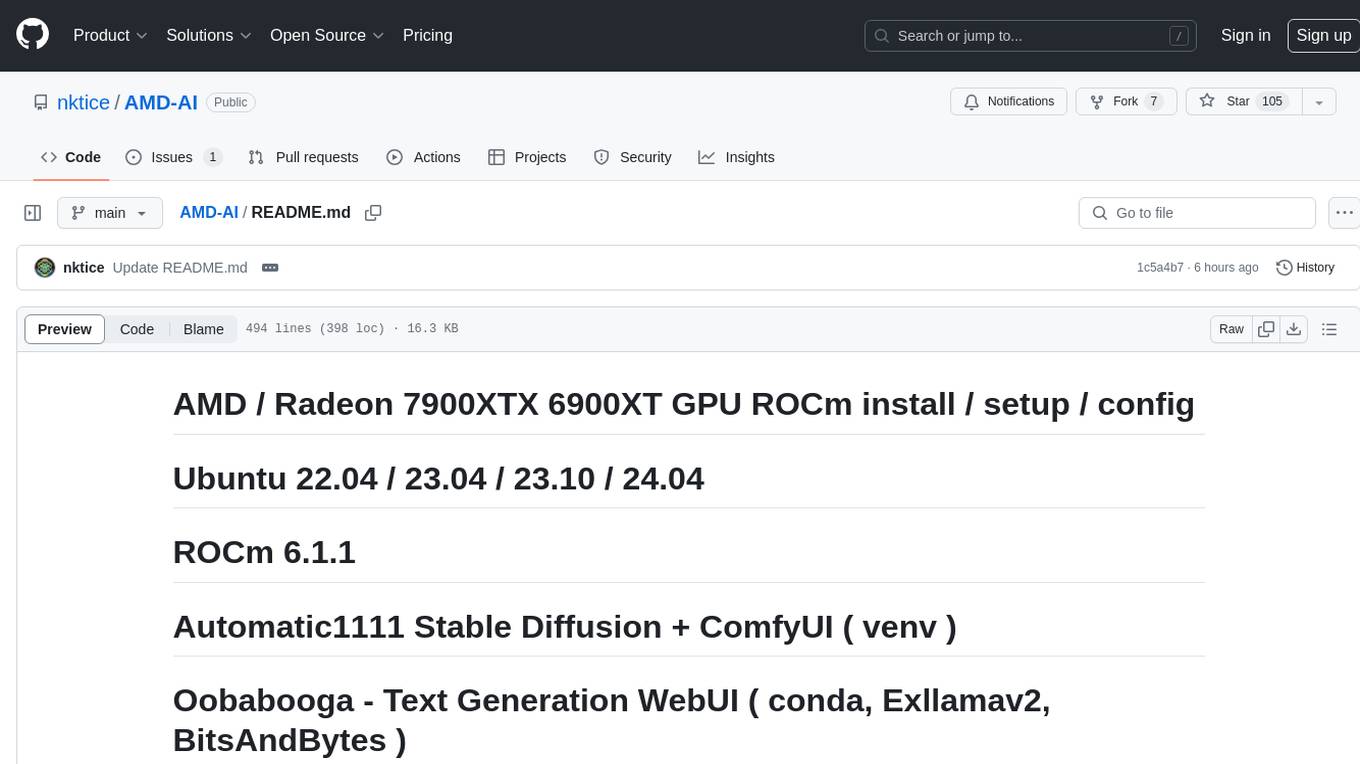
AMD-AI
AMD-AI is a repository containing detailed instructions for installing, setting up, and configuring ROCm on Ubuntu systems with AMD GPUs. The repository includes information on installing various tools like Stable Diffusion, ComfyUI, and Oobabooga for tasks like text generation and performance tuning. It provides guidance on adding AMD GPU package sources, installing ROCm-related packages, updating system packages, and finding graphics devices. The instructions are aimed at users with AMD hardware looking to set up their Linux systems for AI-related tasks.
For similar jobs
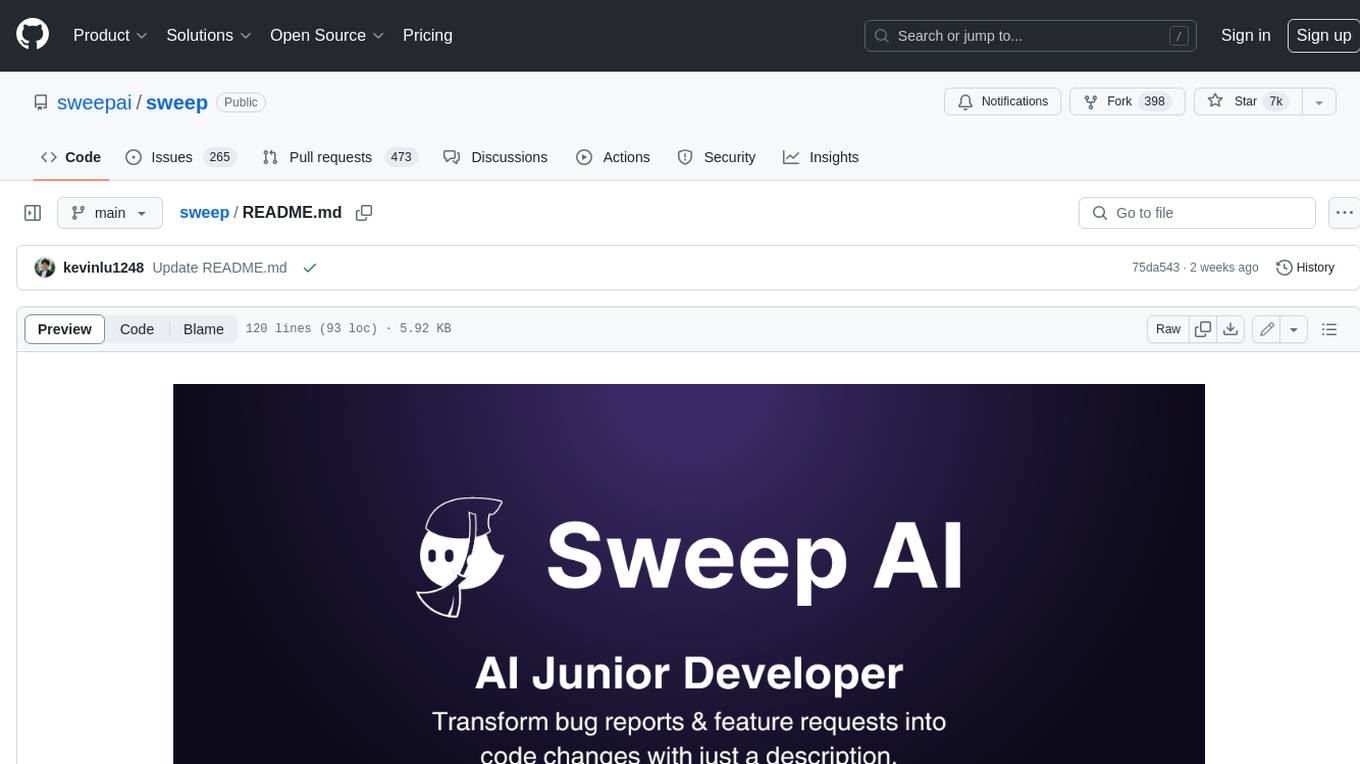
sweep
Sweep is an AI junior developer that turns bugs and feature requests into code changes. It automatically handles developer experience improvements like adding type hints and improving test coverage.
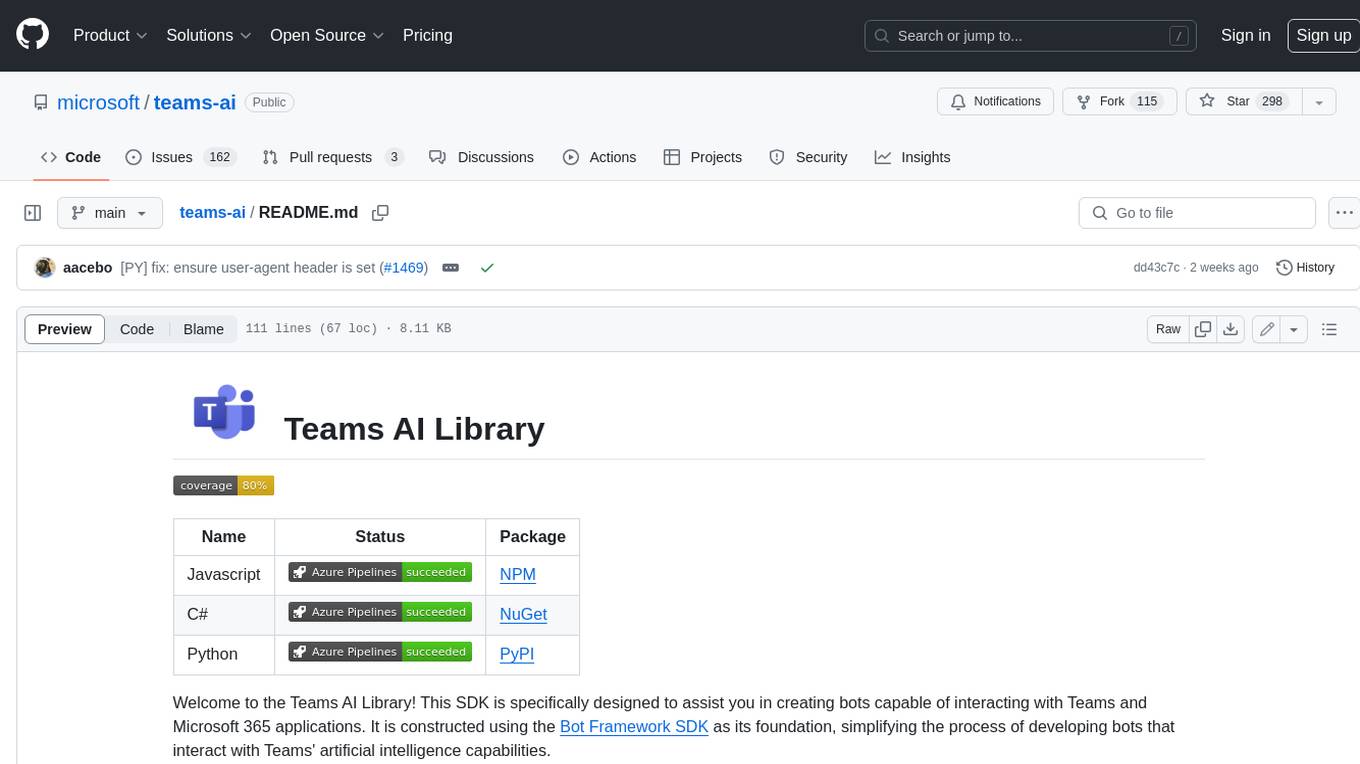
teams-ai
The Teams AI Library is a software development kit (SDK) that helps developers create bots that can interact with Teams and Microsoft 365 applications. It is built on top of the Bot Framework SDK and simplifies the process of developing bots that interact with Teams' artificial intelligence capabilities. The SDK is available for JavaScript/TypeScript, .NET, and Python.
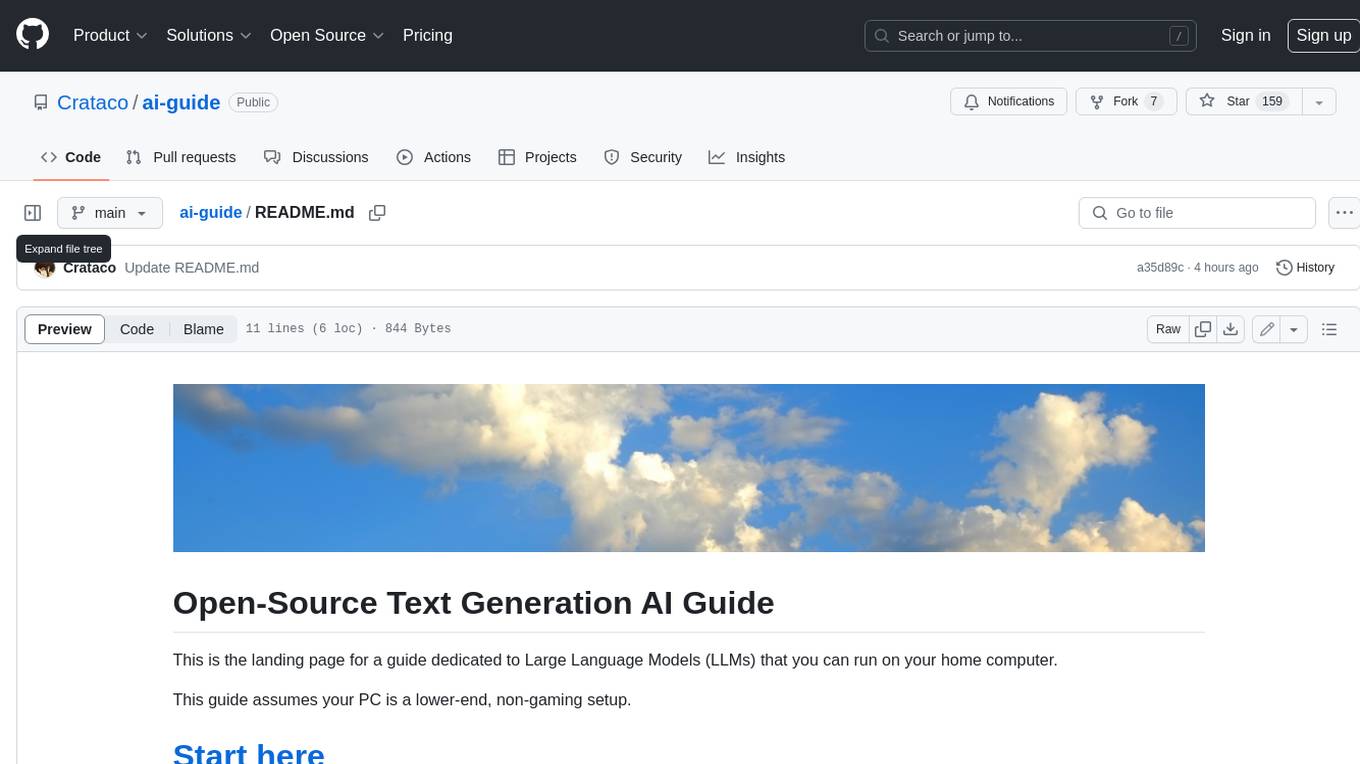
ai-guide
This guide is dedicated to Large Language Models (LLMs) that you can run on your home computer. It assumes your PC is a lower-end, non-gaming setup.
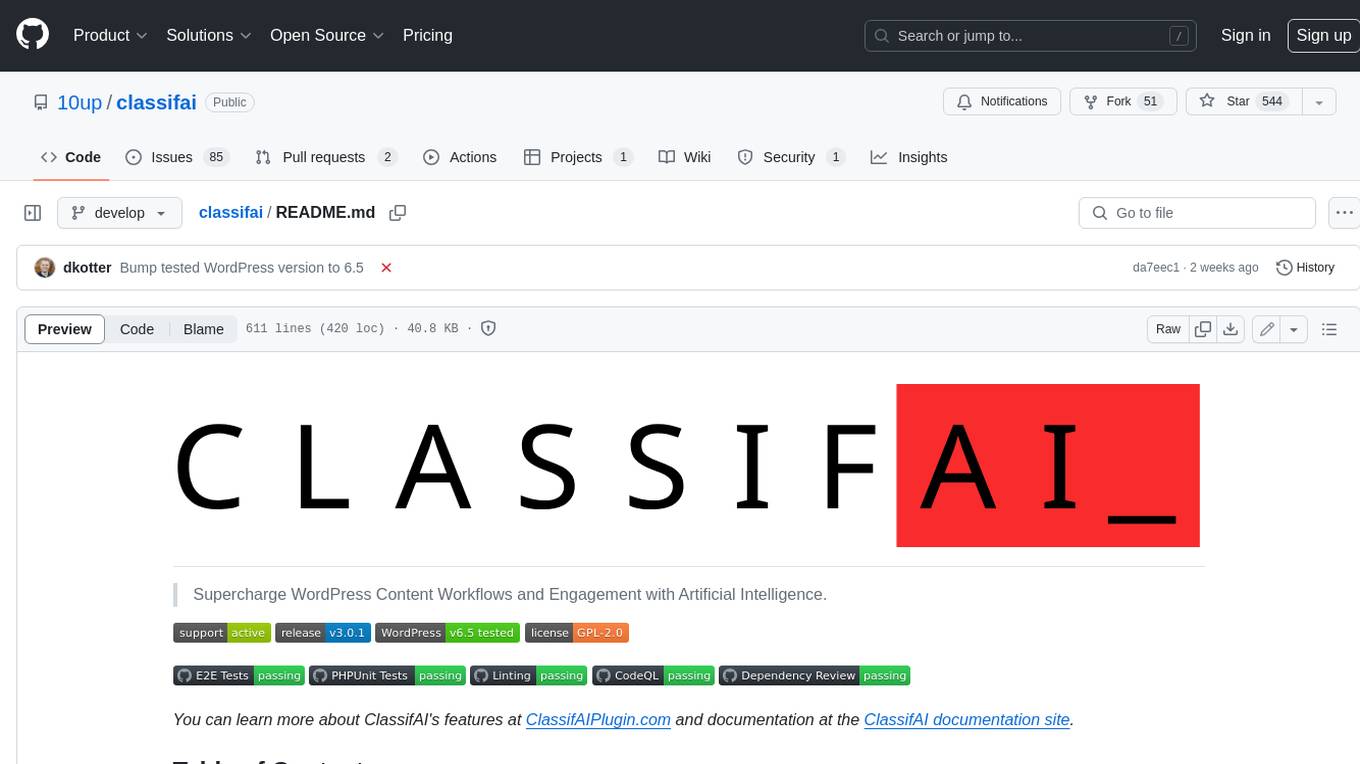
classifai
Supercharge WordPress Content Workflows and Engagement with Artificial Intelligence. Tap into leading cloud-based services like OpenAI, Microsoft Azure AI, Google Gemini and IBM Watson to augment your WordPress-powered websites. Publish content faster while improving SEO performance and increasing audience engagement. ClassifAI integrates Artificial Intelligence and Machine Learning technologies to lighten your workload and eliminate tedious tasks, giving you more time to create original content that matters.
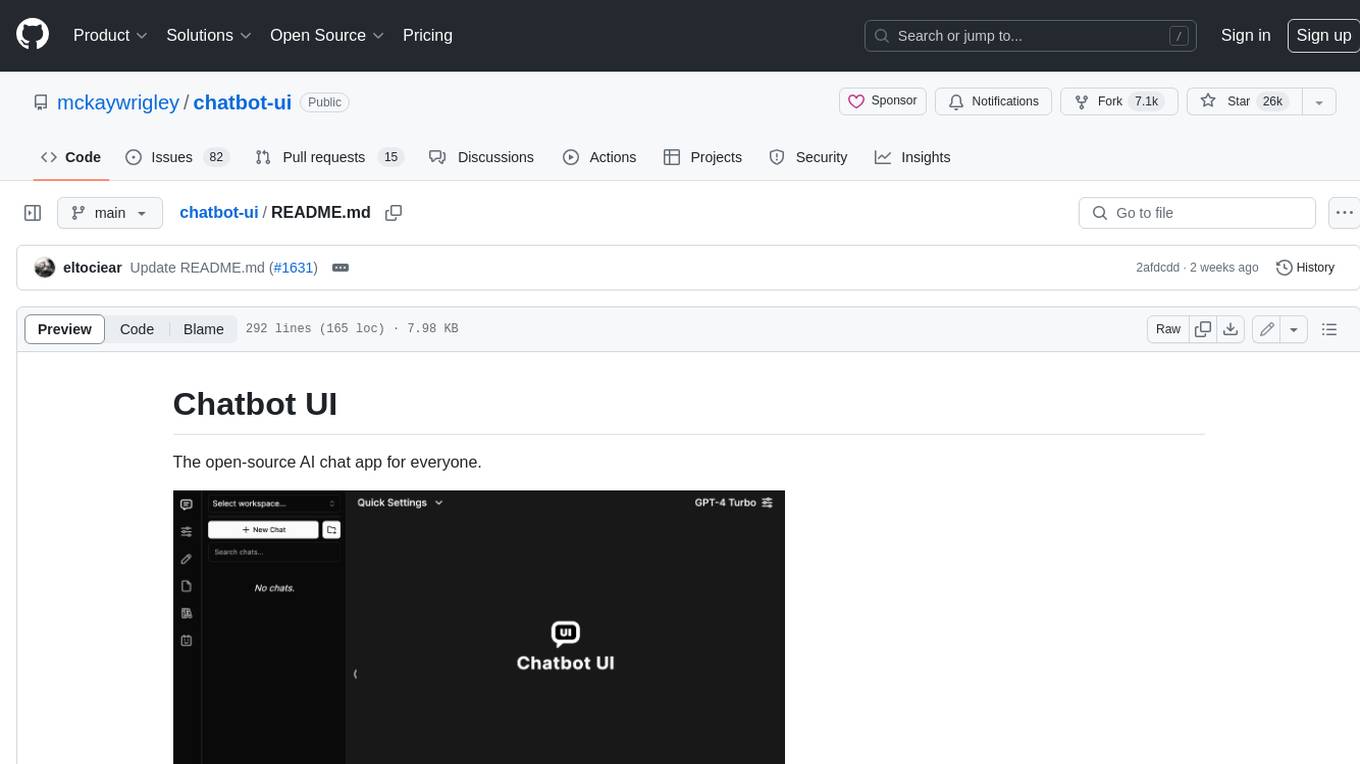
chatbot-ui
Chatbot UI is an open-source AI chat app that allows users to create and deploy their own AI chatbots. It is easy to use and can be customized to fit any need. Chatbot UI is perfect for businesses, developers, and anyone who wants to create a chatbot.
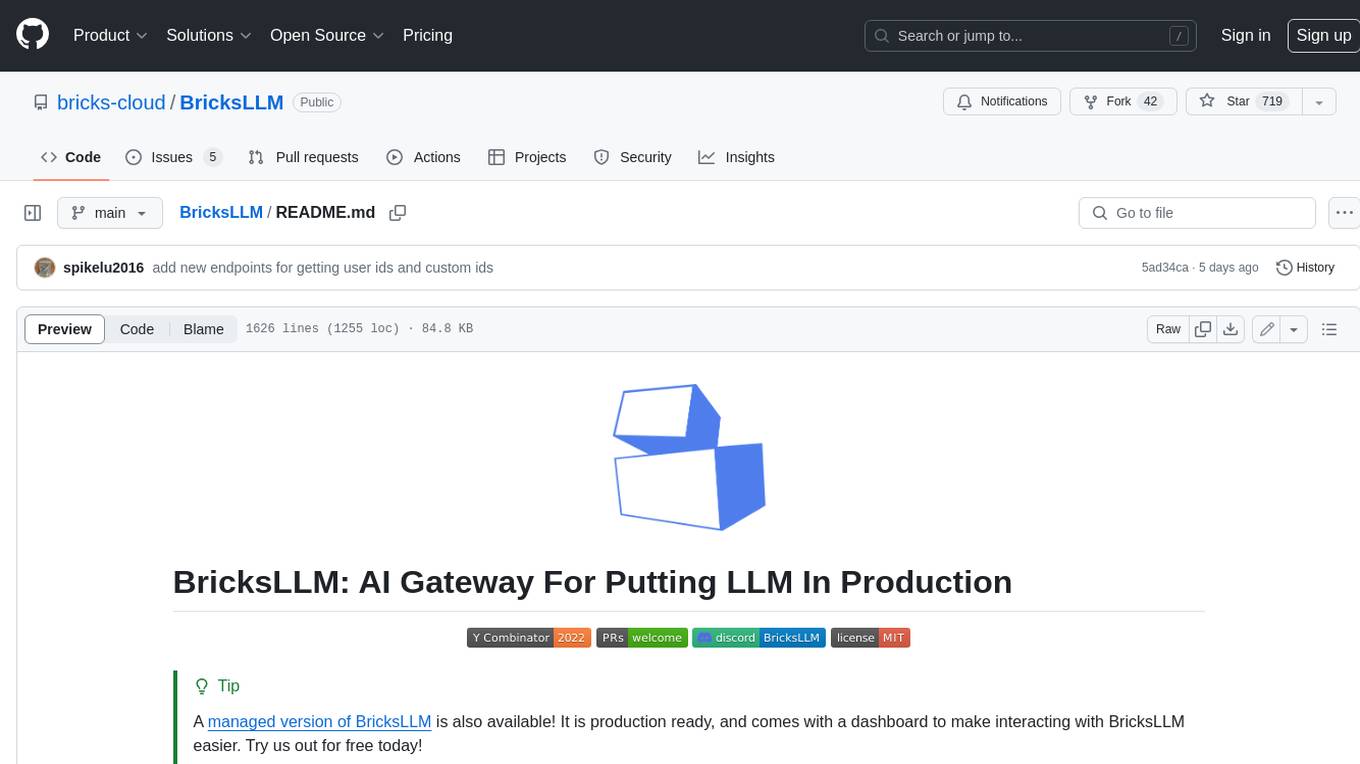
BricksLLM
BricksLLM is a cloud native AI gateway written in Go. Currently, it provides native support for OpenAI, Anthropic, Azure OpenAI and vLLM. BricksLLM aims to provide enterprise level infrastructure that can power any LLM production use cases. Here are some use cases for BricksLLM: * Set LLM usage limits for users on different pricing tiers * Track LLM usage on a per user and per organization basis * Block or redact requests containing PIIs * Improve LLM reliability with failovers, retries and caching * Distribute API keys with rate limits and cost limits for internal development/production use cases * Distribute API keys with rate limits and cost limits for students
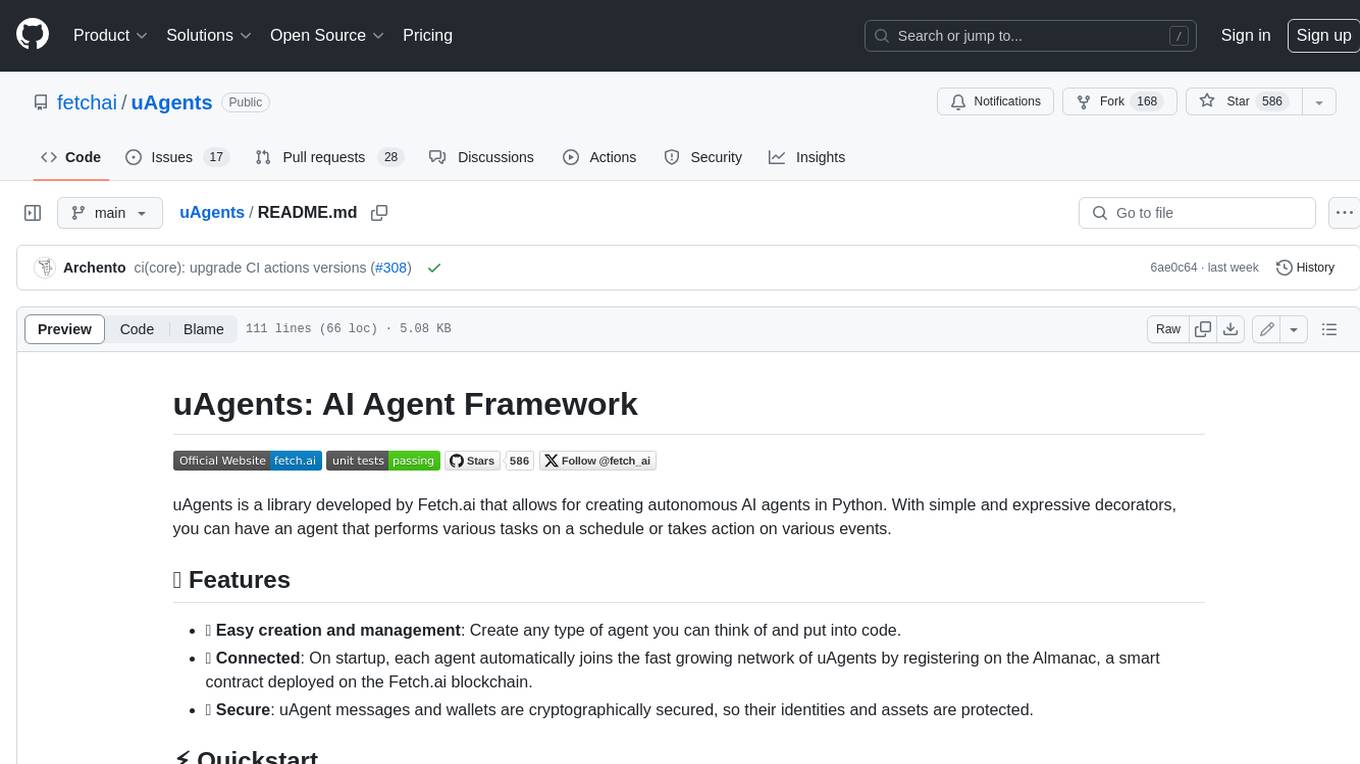
uAgents
uAgents is a Python library developed by Fetch.ai that allows for the creation of autonomous AI agents. These agents can perform various tasks on a schedule or take action on various events. uAgents are easy to create and manage, and they are connected to a fast-growing network of other uAgents. They are also secure, with cryptographically secured messages and wallets.
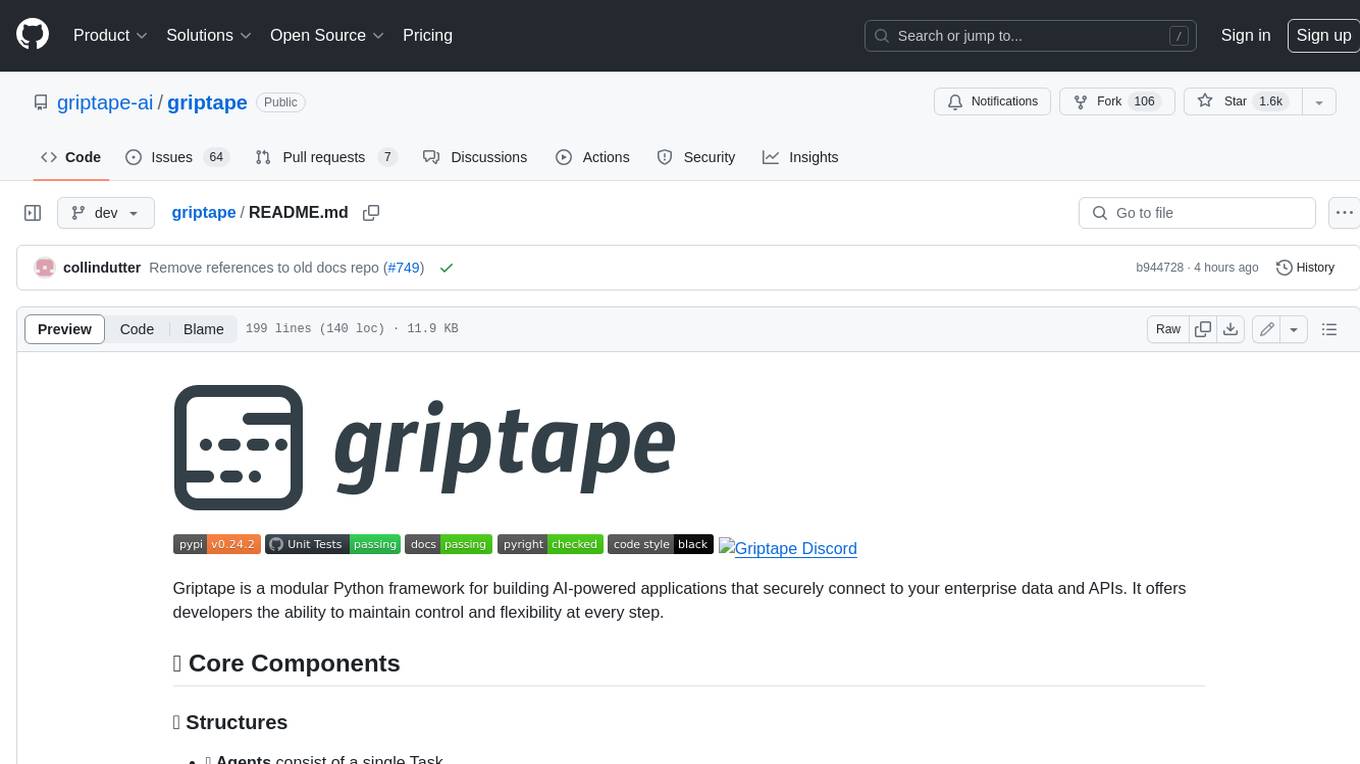
griptape
Griptape is a modular Python framework for building AI-powered applications that securely connect to your enterprise data and APIs. It offers developers the ability to maintain control and flexibility at every step. Griptape's core components include Structures (Agents, Pipelines, and Workflows), Tasks, Tools, Memory (Conversation Memory, Task Memory, and Meta Memory), Drivers (Prompt and Embedding Drivers, Vector Store Drivers, Image Generation Drivers, Image Query Drivers, SQL Drivers, Web Scraper Drivers, and Conversation Memory Drivers), Engines (Query Engines, Extraction Engines, Summary Engines, Image Generation Engines, and Image Query Engines), and additional components (Rulesets, Loaders, Artifacts, Chunkers, and Tokenizers). Griptape enables developers to create AI-powered applications with ease and efficiency.