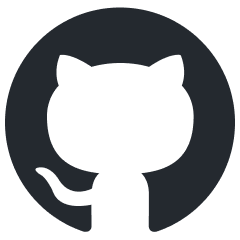
Webscout
Webscout is the all-in-one search and AI toolkit you need. Discover insights with Yep.com, DuckDuckGo, and Phind; access cutting-edge AI models; transcribe YouTube videos; generate temporary emails and phone numbers; perform text-to-speech conversions; and much more!
Stars: 210
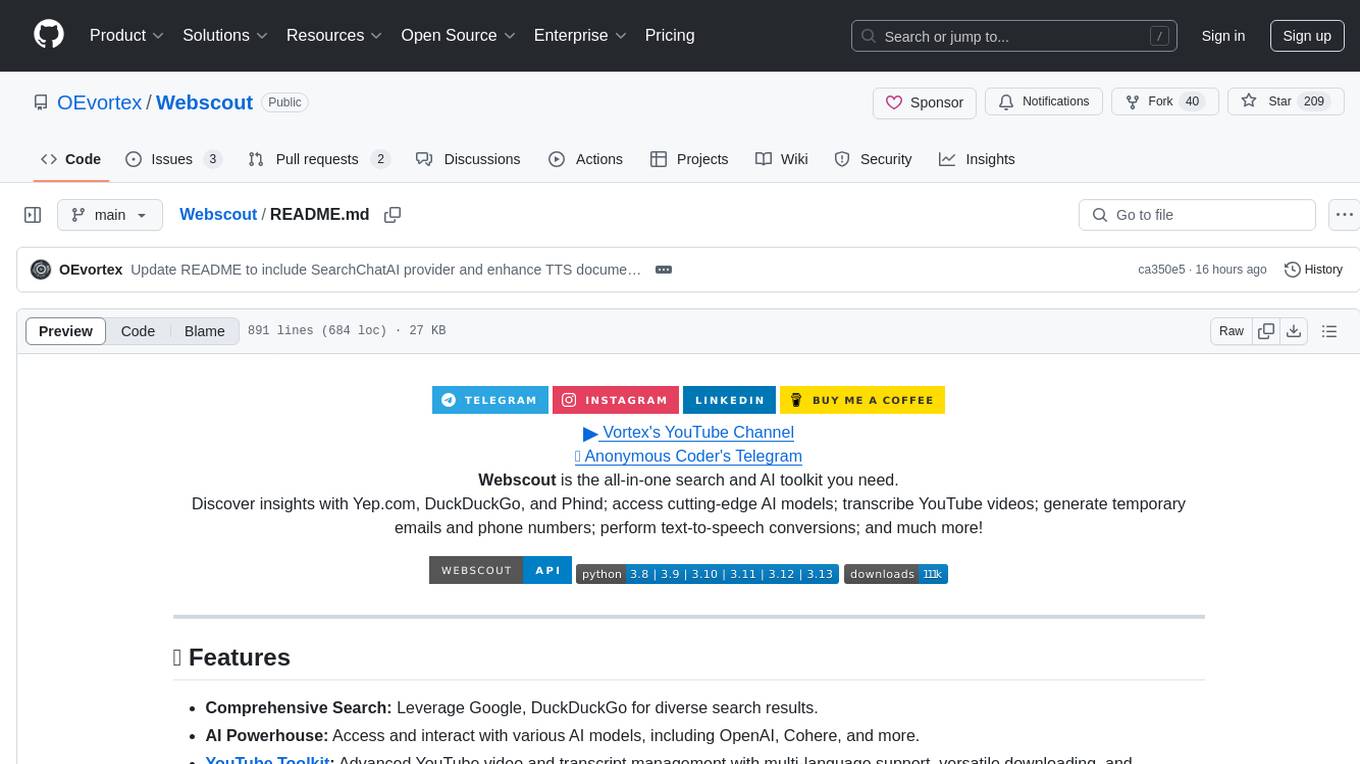
README:
Your All-in-One Python Toolkit for Web Search, AI Interaction, Digital Utilities, and More.
Access diverse search engines, cutting-edge AI models, temporary communication tools, media utilities, developer helpers, and powerful CLI interfaces – all through one unified library.
- Comprehensive Search: Leverage Google, DuckDuckGo for diverse search results.
- AI Powerhouse: Access and interact with various AI models, including OpenAI, Cohere, and more.
- YouTube Toolkit: Advanced YouTube video and transcript management with multi-language support, versatile downloading, and intelligent data extraction
- GitAPI: Powerful GitHub data extraction toolkit for seamless repository and user information retrieval, featuring commit tracking, issue management, and comprehensive user analytics - all without authentication requirements for public data
- Tempmail & Temp Number: Generate temporary email addresses and phone numbers for enhanced privacy.
- Text-to-Speech (TTS): Convert text into natural-sounding speech using multiple AI-powered providers like ElevenLabs, StreamElements, and Voicepods.
- GGUF Conversion & Quantization: Convert and quantize Hugging Face models to GGUF format.
- SwiftCLI: A powerful and elegant CLI framework that makes it easy to create beautiful command-line interfaces.
- LitPrinter: Provides beautiful, styled console output with rich formatting and colors
- LitLogger: Simplifies logging with customizable formats and color schemes
- LitAgent: Powerful and modern user agent generator that keeps your requests fresh and undetectable
- Text-to-Image: Generate high-quality images using a wide range of AI art providers
- Scout: Advanced web parsing and crawling library with intelligent HTML/XML parsing, web crawling, and Markdown conversion
- Awesome Prompts (Act): A curated collection of system prompts designed to transform Webscout into specialized personas, enhancing its ability to assist with specific tasks. Simply prefix your request with the act name or index number to leverage these tailored capabilities.
- Weather Tool kit Webscout provides tools to retrieve weather information.
- AIsearch AI Search Providers offer powerful and flexible AI-powered search Search Engine
pip install -U webscout
python -m webscout --help
Command | Description |
---|---|
python -m webscout answers -k Text | CLI function to perform an answers search using Webscout. |
python -m webscout chat | Interactive AI chat using DuckDuckGo's AI. |
python -m webscout images -k Text | CLI function to perform an images search using Webscout. |
python -m webscout maps -k Text | CLI function to perform a maps search using Webscout. |
python -m webscout news -k Text | CLI function to perform a news search using Webscout. |
python -m webscout suggestions -k Text | CLI function to perform a suggestions search using Webscout. |
python -m webscout text -k Text | CLI function to perform a text search using Webscout. |
python -m webscout translate -k Text | CLI function to perform translate using Webscout. |
python -m webscout version | A command-line interface command that prints and returns the version of the program. |
python -m webscout videos -k Text | CLI function to perform a videos search using DuckDuckGo API. |
python -m webscout weather -l qazigund | CLI function to get weather information for a location using Webscout. |
import json
import asyncio
from webscout import VNEngine
from webscout import TempMail
async def main():
vn = VNEngine()
countries = vn.get_online_countries()
if countries:
country = countries[0]['country']
numbers = vn.get_country_numbers(country)
if numbers:
number = numbers[0]['full_number']
inbox = vn.get_number_inbox(country, number)
# Serialize inbox data to JSON string
json_data = json.dumps(inbox, ensure_ascii=False, indent=4)
# Print with UTF-8 encoding
print(json_data)
async with TempMail() as client:
domains = await client.get_domains()
print("Available Domains:", domains)
email_response = await client.create_email(alias="testuser")
print("Created Email:", email_response)
messages = await client.get_messages(email_response.email)
print("Messages:", messages)
await client.delete_email(email_response.email, email_response.token)
print("Email Deleted")
if __name__ == "__main__":
asyncio.run(main())
...
from webscout import YepSearch
# Initialize YepSearch
yep = YepSearch(
timeout=20, # Optional: Set custom timeout
proxies=None, # Optional: Use proxies
verify=True # Optional: SSL verification
)
# Text Search
text_results = yep.text(
keywords="artificial intelligence",
region="all", # Optional: Region for results
safesearch="moderate", # Optional: "on", "moderate", "off"
max_results=10 # Optional: Limit number of results
)
print(text_results)
# Image Search
image_results = yep.images(
keywords="nature photography",
region="all",
safesearch="moderate",
max_results=10
)
print(image_results)
# Suggestions
suggestions = yep.suggestions("hist")
print(suggestions)
from webscout import GoogleSearch
# Initialize GoogleSearch
google = GoogleSearch(
timeout=10, # Optional: Set custom timeout
proxies=None, # Optional: Use proxies
verify=True # Optional: SSL verification
)
# Text Search
text_results = google.text(
keywords="artificial intelligence",
region="us", # Optional: Region for results
safesearch="moderate", # Optional: "on", "moderate", "off"
max_results=10 # Optional: Limit number of results
)
for result in text_results:
print(f"Title: {result.title}")
print(f"URL: {result.url}")
print(f"Description: {result.description}")
print("---")
# News Search
news_results = google.news(
keywords="technology trends",
region="us",
safesearch="moderate",
max_results=5
)
for result in news_results:
print(f"Title: {result.title}")
print(f"URL: {result.url}")
print(f"Description: {result.description}")
print("---")
# Get search suggestions
suggestions = google.suggestions("how to")
print(suggestions)
# Legacy usage is still supported
from webscout import search
results = search("Python programming", num_results=5)
for url in results:
print(url)
The WEBS
and AsyncWEBS
classes are used to retrieve search results from DuckDuckGo.com.
To use the AsyncWEBS
class, you can perform asynchronous operations using Python's asyncio
library.
To initialize an instance of the WEBS
or AsyncWEBS
classes, you can provide the following optional arguments:
Example - WEBS:
from webscout import WEBS
R = WEBS().text("python programming", max_results=5)
print(R)
Example - AsyncWEBS:
import asyncio
import logging
import sys
from itertools import chain
from random import shuffle
import requests
from webscout import AsyncWEBS
# If you have proxies, define them here
proxies = None
if sys.platform.lower().startswith("win"):
asyncio.set_event_loop_policy(asyncio.WindowsSelectorEventLoopPolicy())
def get_words():
word_site = "https://www.mit.edu/~ecprice/wordlist.10000"
resp = requests.get(word_site)
words = resp.text.splitlines()
return words
async def aget_results(word):
async with AsyncWEBS(proxies=proxies) as WEBS:
results = await WEBS.text(word, max_results=None)
return results
async def main():
words = get_words()
shuffle(words)
tasks = [aget_results(word) for word in words[:10]]
results = await asyncio.gather(*tasks)
print(f"Done")
for r in chain.from_iterable(results):
print(r)
logging.basicConfig(level=logging.DEBUG)
await main()
Important Note: The WEBS
and AsyncWEBS
classes should always be used as a context manager (with statement). This ensures proper resource management and cleanup, as the context manager will automatically handle opening and closing the HTTP client connection.
Exceptions:
-
WebscoutE
: Raised when there is a generic exception during the API request.
from webscout import WEBS
# Text search for 'live free or die' using DuckDuckGo.com
with WEBS() as WEBS:
for r in WEBS.text('live free or die', region='wt-wt', safesearch='off', timelimit='y', max_results=10):
print(r)
for r in WEBS.text('live free or die', region='wt-wt', safesearch='off', timelimit='y', max_results=10):
print(r)
from webscout import WEBS
# Instant answers for the query "sun" using DuckDuckGo.com
with WEBS() as WEBS:
for r in WEBS.answers("sun"):
print(r)
from webscout import WEBS
# Image search for the keyword 'butterfly' using DuckDuckGo.com
with WEBS() as WEBS:
keywords = 'butterfly'
WEBS_images_gen = WEBS.images(
keywords,
region="wt-wt",
safesearch="off",
size=None,
type_image=None,
layout=None,
license_image=None,
max_results=10,
)
for r in WEBS_images_gen:
print(r)
from webscout import WEBS
# Video search for the keyword 'tesla' using DuckDuckGo.com
with WEBS() as WEBS:
keywords = 'tesla'
WEBS_videos_gen = WEBS.videos(
keywords,
region="wt-wt",
safesearch="off",
timelimit="w",
resolution="high",
duration="medium",
max_results=10,
)
for r in WEBS_videos_gen:
print(r)
from webscout import WEBS
import datetime
def fetch_news(keywords, timelimit):
news_list = []
with WEBS() as webs_instance:
WEBS_news_gen = webs_instance.news(
keywords,
region="wt-wt",
safesearch="off",
timelimit=timelimit,
max_results=20
)
for r in WEBS_news_gen:
# Convert the date to a human-readable format using datetime
r['date'] = datetime.datetime.fromisoformat(r['date']).strftime('%B %d, %Y')
news_list.append(r)
return news_list
def _format_headlines(news_list, max_headlines: int = 100):
headlines = []
for idx, news_item in enumerate(news_list):
if idx >= max_headlines:
break
new_headline = f"{idx + 1}. {news_item['title'].strip()} "
new_headline += f"(URL: {news_item['url'].strip()}) "
new_headline += f"{news_item['body'].strip()}"
new_headline += "\n"
headlines.append(new_headline)
headlines = "\n".join(headlines)
return headlines
# Example usage
keywords = 'latest AI news'
timelimit = 'd'
news_list = fetch_news(keywords, timelimit)
# Format and print the headlines
formatted_headlines = _format_headlines(news_list)
print(formatted_headlines)
from webscout import WEBS
# Map search for the keyword 'school' in 'anantnag' using DuckDuckGo.com
with WEBS() as WEBS:
for r in WEBS.maps("school", place="anantnag", max_results=50):
print(r)
from webscout import WEBS
# Translation of the keyword 'school' to German ('hi') using DuckDuckGo.com
with WEBS() as WEBS:
keywords = 'school'
r = WEBS.translate(keywords, to="hi")
print(r)
from webscout import WEBS
# Suggestions for the keyword 'fly' using DuckDuckGo.com
with WEBS() as WEBS:
for r in WEBS.suggestions("fly"):
print(r)
from webscout import WEBS
# Get weather information for a location using DuckDuckGo.com
with WEBS() as webs:
weather_data = webs.weather("New York")
print(weather_data)
Retrieve a comprehensive list of all supported LLMs.
from webscout import model
from rich import print
all_models = model.llm.list()
print("Available models:")
print(all_models)
Obtain a summary of the available LLMs, including provider details.
from webscout import model
from rich import print
summary = model.llm.summary()
print("Summary of models:")
print(summary)
Filter and display LLMs available from a specific provider.
from webscout import model
from rich import print
provider_name = "PerplexityLabs" # Example provider
available_models = model.llm.get(provider_name)
if isinstance(available_models, list):
print(f"Available models for {provider_name}: {', '.join(available_models)}")
else:
print(f"Available models for {provider_name}: {available_models}")
Retrieve a comprehensive list of all supported TTS voices.
from webscout import model
from rich import print
all_voices = model.tts.list()
print("Available TTS voices:")
print(all_voices)
Obtain a summary of the available TTS voices, including provider details.
from webscout import model
from rich import print
summary = model.tts.summary()
print("Summary of TTS voices:")
print(summary)
Filter and display TTS voices available from a specific provider.
from webscout import model
from rich import print
provider_name = "ElevenlabsTTS" # Example provider
available_voices = model.tts.get(provider_name)
if isinstance(available_voices, list):
print(f"Available voices for {provider_name}: {', '.join(available_voices)}")
elif isinstance(available_voices, dict):
print(f"Available voices for {provider_name}:")
for voice_name, voice_id in available_voices.items():
print(f" - {voice_name}: {voice_id}")
else:
print(f"Available voices for {provider_name}: {available_voices}")
from webscout import WEBS as w
R = w().chat("Who are you", model='gpt-4o-mini') # mixtral-8x7b, llama-3.1-70b, claude-3-haiku, gpt-4o-mini
print(R)
from webscout import PhindSearch
# Create an instance of the PHIND class
ph = PhindSearch()
# Define a prompt to send to the AI
prompt = "write a essay on phind"
# Use the 'ask' method to send the prompt and receive a response
response = ph.ask(prompt)
# Extract and print the message from the response
message = ph.get_message(response)
print(message)
Using phindv2:
from webscout import Phindv2
# Create an instance of the PHIND class
ph = Phindv2()
# Define a prompt to send to the AI
prompt = ""
# Use the 'ask' method to send the prompt and receive a response
response = ph.ask(prompt)
# Extract and print the message from the response
message = ph.get_message(response)
print(message)
import webscout
from webscout import GEMINI
from rich import print
COOKIE_FILE = "cookies.json"
# Optional: Provide proxy details if needed
PROXIES = {}
# Initialize GEMINI with cookie file and optional proxies
gemini = GEMINI(cookie_file=COOKIE_FILE, proxy=PROXIES)
# Ask a question and print the response
response = gemini.chat("websearch about HelpingAI and who is its developer")
print(response)
from webscout import YEPCHAT
ai = YEPCHAT()
response = ai.chat(input(">>> "))
for chunk in response:
print(chunk, end="", flush=True)
from webscout import BLACKBOXAI
from rich import print
ai = BLACKBOXAI(
is_conversation=True,
max_tokens=800,
timeout=30,
intro=None,
filepath=None,
update_file=True,
proxies={},
history_offset=10250,
act=None,
model=None # You can specify a model if needed
)
# Define a prompt to send to the AI
prompt = "Tell me about india"
# Use the 'chat' method to send the prompt and receive a response
r = ai.chat(prompt)
print(r)
from webscout import Meta
from rich import print
# **For unauthenticated usage**
meta_ai = Meta()
# Simple text prompt
response = meta_ai.chat("What is the capital of France?")
print(response)
# Streaming response
for chunk in meta_ai.chat("Tell me a story about a cat."):
print(chunk, end="", flush=True)
# **For authenticated usage (including image generation)**
fb_email = "[email protected]"
fb_password = "qwertfdsa"
meta_ai = Meta(fb_email=fb_email, fb_password=fb_password)
# Text prompt with web search
response = meta_ai.ask("what is currently happning in bangladesh in aug 2024")
print(response["message"]) # Access the text message
print("Sources:", response["sources"]) # Access sources (if any)
# Image generation
response = meta_ai.ask("Create an image of a cat wearing a hat.")
print(response["message"]) # Print the text message from the response
for media in response["media"]:
print(media["url"]) # Access image URLs
from webscout import KOBOLDAI
# Instantiate the KOBOLDAI class with default parameters
koboldai = KOBOLDAI()
# Define a prompt to send to the AI
prompt = "What is the capital of France?"
# Use the 'ask' method to get a response from the AI
response = koboldai.ask(prompt)
# Extract and print the message from the response
message = koboldai.get_message(response)
print(message)
from webscout import REKA
a = REKA(is_conversation=True, max_tokens=8000, timeout=30,api_key="")
prompt = "tell me about india"
response_str = a.chat(prompt)
print(response_str)
from webscout import Cohere
a = Cohere(is_conversation=True, max_tokens=8000, timeout=30,api_key="")
prompt = "tell me about india"
response_str = a.chat(prompt)
print(response_str)
from webscout import DeepInfra
ai = DeepInfra(
is_conversation=True,
model= "Qwen/Qwen2-72B-Instruct",
max_tokens=800,
timeout=30,
intro=None,
filepath=None,
update_file=True,
proxies={},
history_offset=10250,
act=None,
)
prompt = "what is meaning of life"
response = ai.ask(prompt)
# Extract and print the message from the response
message = ai.get_message(response)
print(message)
from webscout import GROQ
ai = GROQ(api_key="")
response = ai.chat("What is the meaning of life?")
print(response)
#----------------------TOOL CALL------------------
from webscout import GROQ # Adjust import based on your project structure
from webscout import WEBS
import json
# Initialize the GROQ client
client = GROQ(api_key="")
MODEL = 'llama3-groq-70b-8192-tool-use-preview'
# Function to evaluate a mathematical expression
def calculate(expression):
"""Evaluate a mathematical expression"""
try:
result = eval(expression)
return json.dumps({"result": result})
except Exception as e:
return json.dumps({"error": str(e)})
# Function to perform a text search using DuckDuckGo.com
def search(query):
"""Perform a text search using DuckDuckGo.com"""
try:
results = WEBS().text(query, max_results=5)
return json.dumps({"results": results})
except Exception as e:
return json.dumps({"error": str(e)})
# Add the functions to the provider
client.add_function("calculate", calculate)
client.add_function("search", search)
# Define the tools
tools = [
{
"type": "function",
"function": {
"name": "calculate",
"description": "Evaluate a mathematical expression",
"parameters": {
"type": "object",
"properties": {
"expression": {
"type": "string",
"description": "The mathematical expression to evaluate",
}
},
"required": ["expression"],
},
}
},
{
"type": "function",
"function": {
"name": "search",
"description": "Perform a text search using DuckDuckGo.com and Yep.com",
"parameters": {
"type": "object",
"properties": {
"query": {
"type": "string",
"description": "The search query to execute",
}
},
"required": ["query"],
},
}
}
]
user_prompt_calculate = "What is 25 * 4 + 10?"
response_calculate = client.chat(user_prompt_calculate, tools=tools)
print(response_calculate)
user_prompt_search = "Find information on HelpingAI and who is its developer"
response_search = client.chat(user_prompt_search, tools=tools)
print(response_search)
from webscout import LLAMA
llama = LLAMA()
r = llama.chat("What is the meaning of life?")
print(r)
from webscout import AndiSearch
a = AndiSearch()
print(a.chat("HelpingAI-9B"))
LLAMA
, C4ai
, Venice
, Copilot
, HuggingFaceChat
, TwoAI
, HeckAI
, AllenAI
, PerplexityLabs
, AkashGPT
, DeepSeek
, WiseCat
, IBMGranite
, QwenLM
, ChatGPTGratis
, TextPollinationsAI
, GliderAI
, Cohere
, REKA
, GROQ
, AsyncGROQ
, OPENAI
, AsyncOPENAI
, KOBOLDAI
, AsyncKOBOLDAI
, BLACKBOXAI
, PhindSearch
, GEMINI
, DeepInfra
, AI4Chat
, Phindv2
, OLLAMA
, AndiSearch
, PIZZAGPT
, Sambanova
, DARKAI
, KOALA
, Meta
, AskMyAI
, PiAI
, Julius
, YouChat
, YEPCHAT
, Cloudflare
, TurboSeek
, Editee
, TeachAnything
, AI21
, Chatify
, X0GPT
, Cerebras
, Lepton
, GEMINIAPI
, Cleeai
, Elmo
, Free2GPT
, GPTWeb
, Netwrck
, LlamaTutor
, PromptRefine
, TutorAI
, ChatGPTES
, Bagoodex
, AIMathGPT
, GaurishCerebras
, GeminiPro
, LLMChat
, Talkai
, Llama3Mitril
, Marcus
, TypeGPT
, Netwrck
, MultiChatAI
, JadveOpenAI
, ChatGLM
, NousHermes
, FreeAIChat
, ElectronHub
, GithubChat
, Flowith
, SonusAI
, UncovrAI
, LabyrinthAI
, WebSim
, LambdaChat
, ChatGPTClone
, VercelAI
, ExaChat
, AskSteve
, Aitopia
, SearchChatAI
Code is similar to other providers.
from webscout.LLM import LLM, VLM
# Chat with text
llm = LLM("meta-llama/Meta-Llama-3-70B-Instruct")
response = llm.chat([{"role": "user", "content": "What's good?"}])
# Chat with images
vlm = VLM("cogvlm-grounding-generalist")
response = vlm.chat([{
"role": "user",
"content": [
{"type": "image", "image_url": "cool_pic.jpg"},
{"type": "text", "text": "What's in this image?"}
]
}])
Webscout provides tools to convert and quantize Hugging Face models into the GGUF format for use with offline LLMs.
Example:
from webscout.Extra.gguf import ModelConverter
"""
Valid quantization methods:
"q2_k", "q3_k_l", "q3_k_m", "q3_k_s",
"q4_0", "q4_1", "q4_k_m", "q4_k_s",
"q5_0", "q5_1", "q5_k_m", "q5_k_s",
"q6_k", "q8_0"
"""
# Create a converter instance
converter = ModelConverter(
model_id="prithivMLmods/QWQ-500M",
quantization_methods="q2_k"
)
# Run the conversion
converter.convert()
Command Line Usage:
-
GGUF Conversion:
python -m webscout.Extra.gguf convert -m "prithivMLmods/QWQ-500M" -q "q2_k"
Note:
- Replace
"your_username"
and"your_hf_token"
with your actual Hugging Face credentials. - The
model_path
inautollama
is the Hugging Face model ID, andgguf_file
is the GGUF file ID.
Contributions are welcome! If you'd like to contribute to Webscout, please follow these steps:
- Fork the repository.
- Create a new branch for your feature or bug fix.
- Make your changes and commit them with descriptive messages.
- Push your branch to your forked repository.
- Submit a pull request to the main repository.
- All the amazing developers who have contributed to the project!
- The open-source community for their support and inspiration.
For Tasks:
Click tags to check more tools for each tasksFor Jobs:
Alternative AI tools for Webscout
Similar Open Source Tools
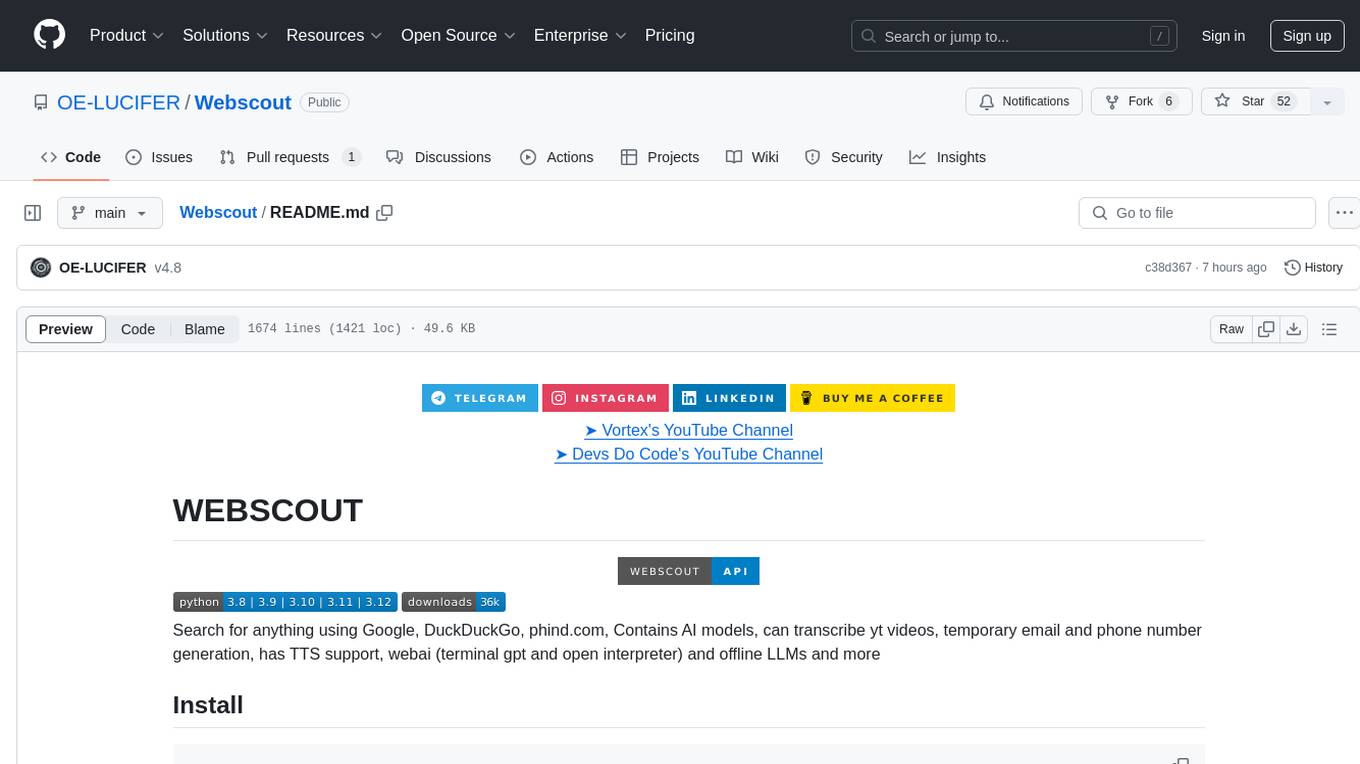
Webscout
WebScout is a versatile tool that allows users to search for anything using Google, DuckDuckGo, and phind.com. It contains AI models, can transcribe YouTube videos, generate temporary email and phone numbers, has TTS support, webai (terminal GPT and open interpreter), and offline LLMs. It also supports features like weather forecasting, YT video downloading, temp mail and number generation, text-to-speech, advanced web searches, and more.
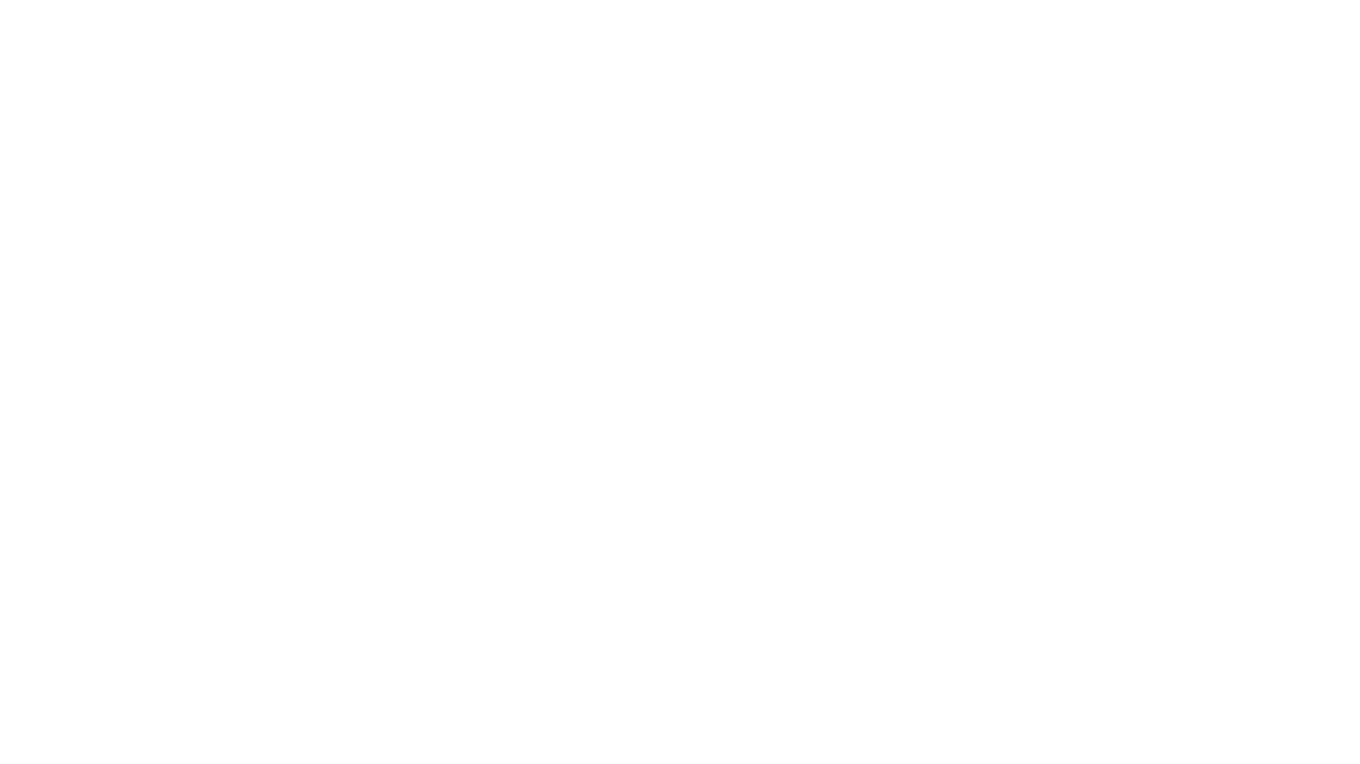
generative-ai-python
The Google AI Python SDK is the easiest way for Python developers to build with the Gemini API. The Gemini API gives you access to Gemini models created by Google DeepMind. Gemini models are built from the ground up to be multimodal, so you can reason seamlessly across text, images, and code.
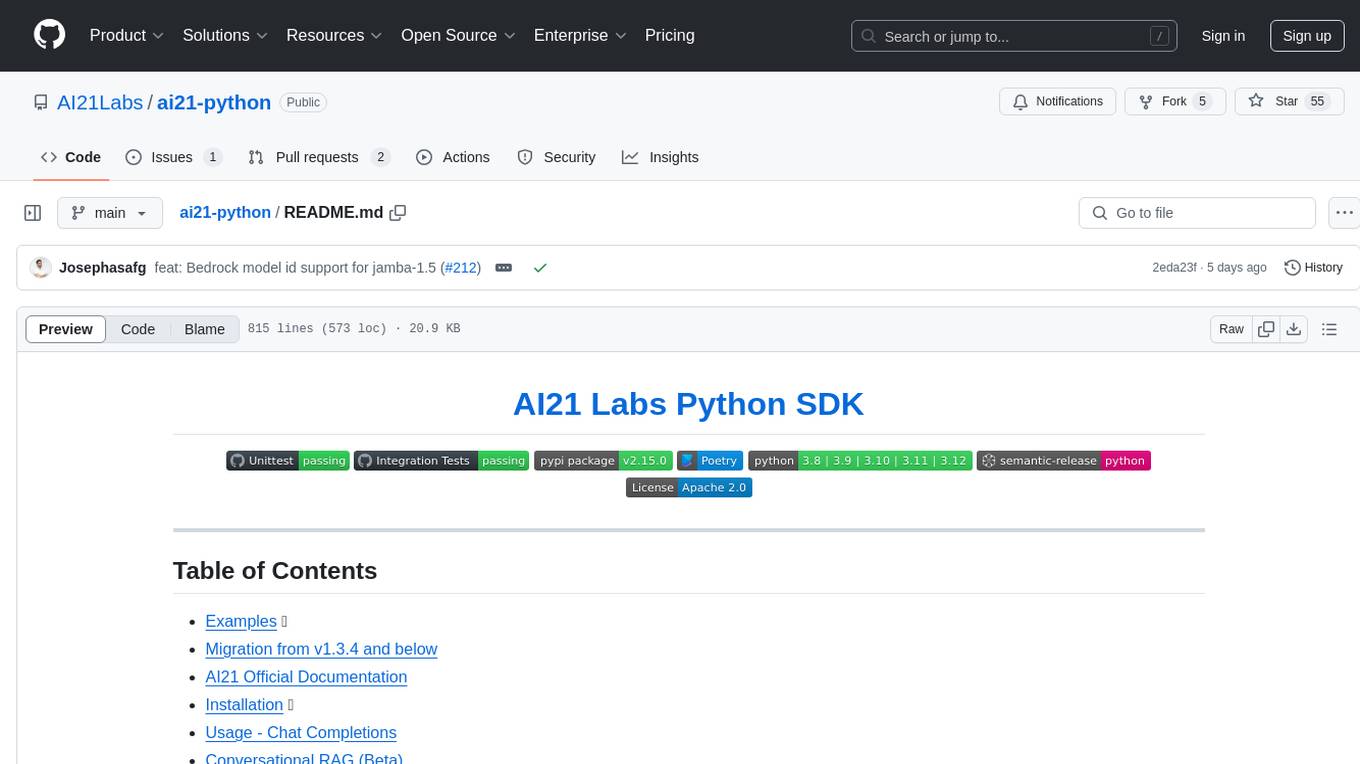
ai21-python
The AI21 Labs Python SDK is a comprehensive tool for interacting with the AI21 API. It provides functionalities for chat completions, conversational RAG, token counting, error handling, and support for various cloud providers like AWS, Azure, and Vertex. The SDK offers both synchronous and asynchronous usage, along with detailed examples and documentation. Users can quickly get started with the SDK to leverage AI21's powerful models for various natural language processing tasks.
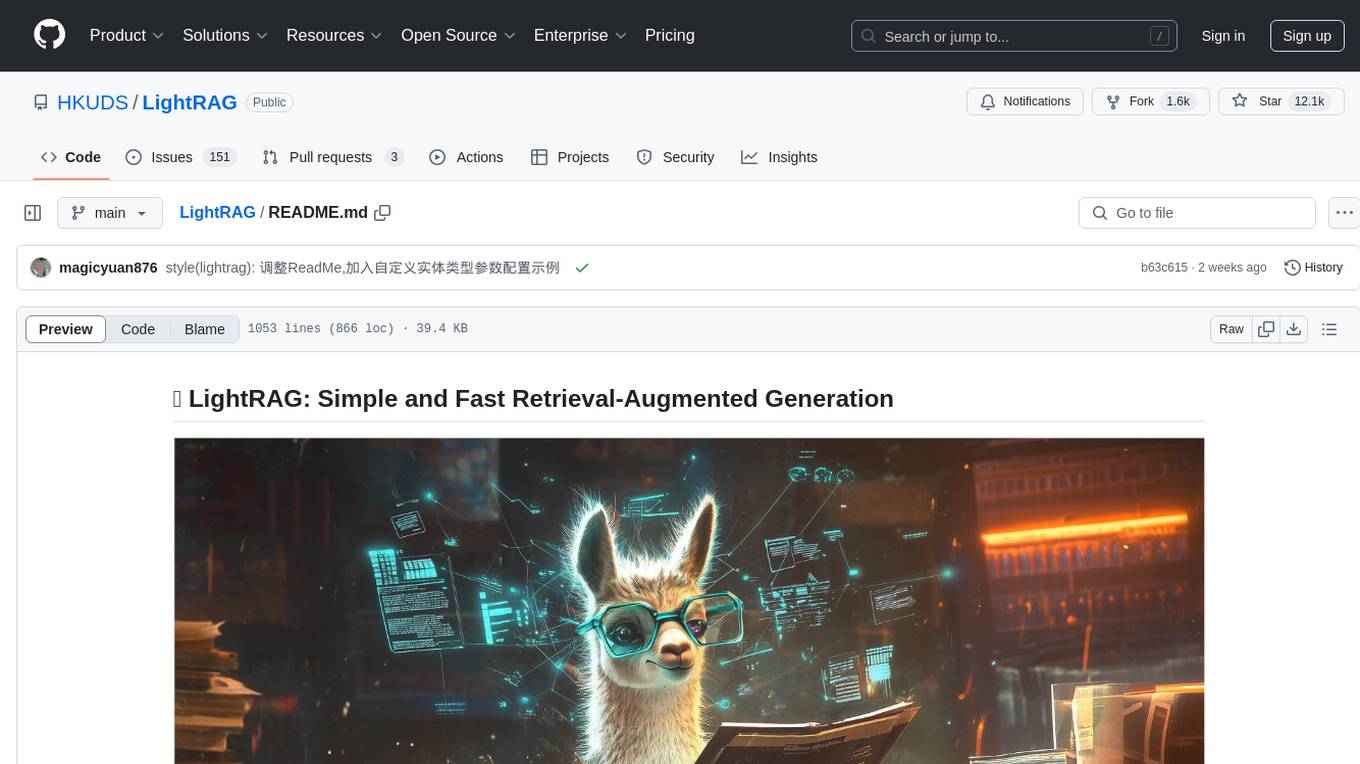
LightRAG
LightRAG is a repository hosting the code for LightRAG, a system that supports seamless integration of custom knowledge graphs, Oracle Database 23ai, Neo4J for storage, and multiple file types. It includes features like entity deletion, batch insert, incremental insert, and graph visualization. LightRAG provides an API server implementation for RESTful API access to RAG operations, allowing users to interact with it through HTTP requests. The repository also includes evaluation scripts, code for reproducing results, and a comprehensive code structure.
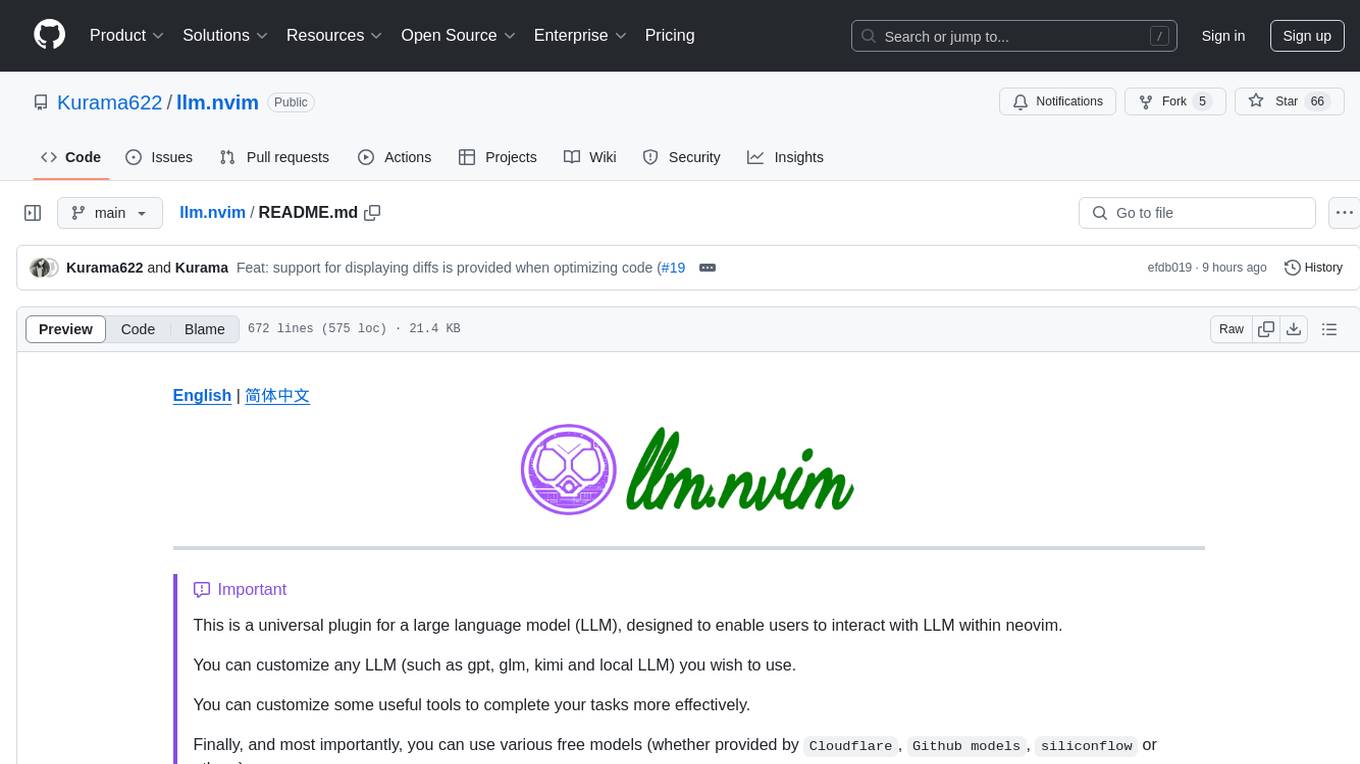
llm.nvim
llm.nvim is a universal plugin for a large language model (LLM) designed to enable users to interact with LLM within neovim. Users can customize various LLMs such as gpt, glm, kimi, and local LLM. The plugin provides tools for optimizing code, comparing code, translating text, and more. It also supports integration with free models from Cloudflare, Github models, siliconflow, and others. Users can customize tools, chat with LLM, quickly translate text, and explain code snippets. The plugin offers a flexible window interface for easy interaction and customization.
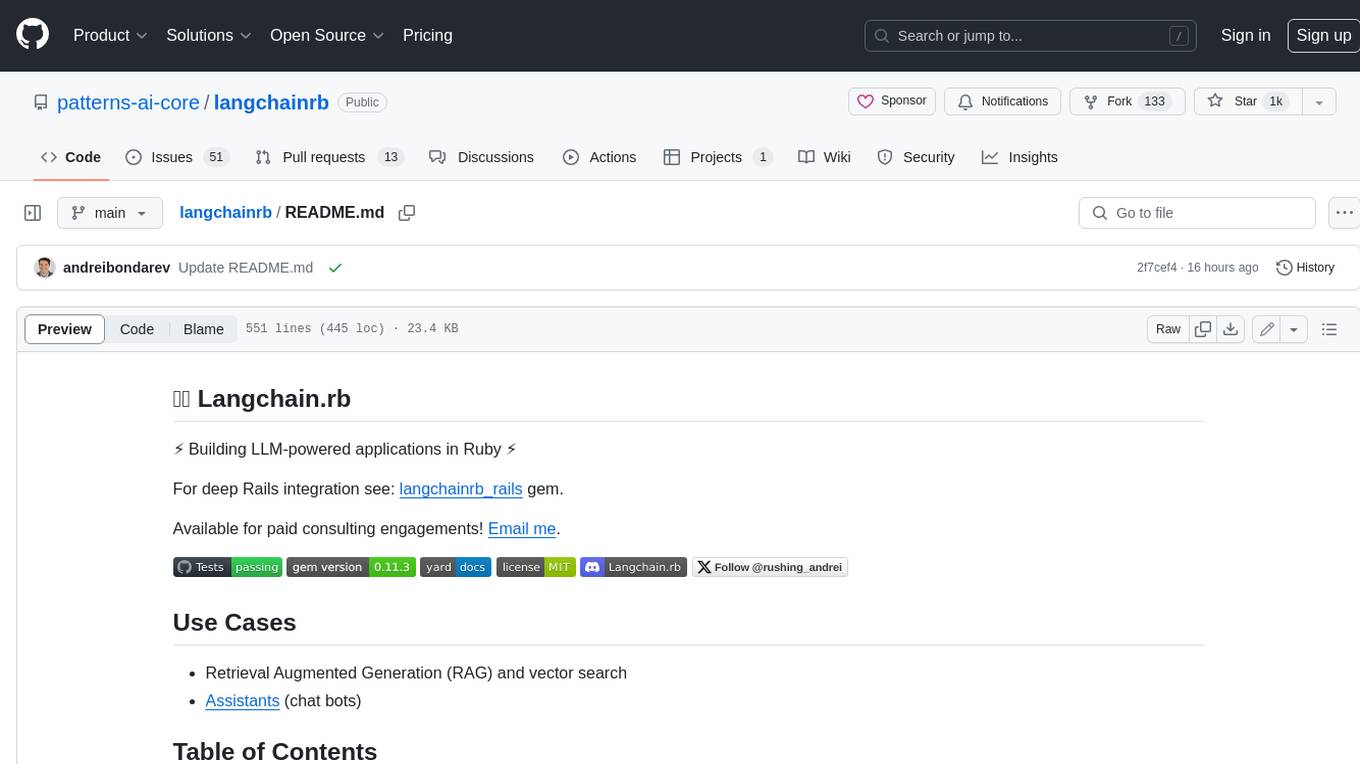
langchainrb
Langchain.rb is a Ruby library that makes it easy to build LLM-powered applications. It provides a unified interface to a variety of LLMs, vector search databases, and other tools, making it easy to build and deploy RAG (Retrieval Augmented Generation) systems and assistants. Langchain.rb is open source and available under the MIT License.
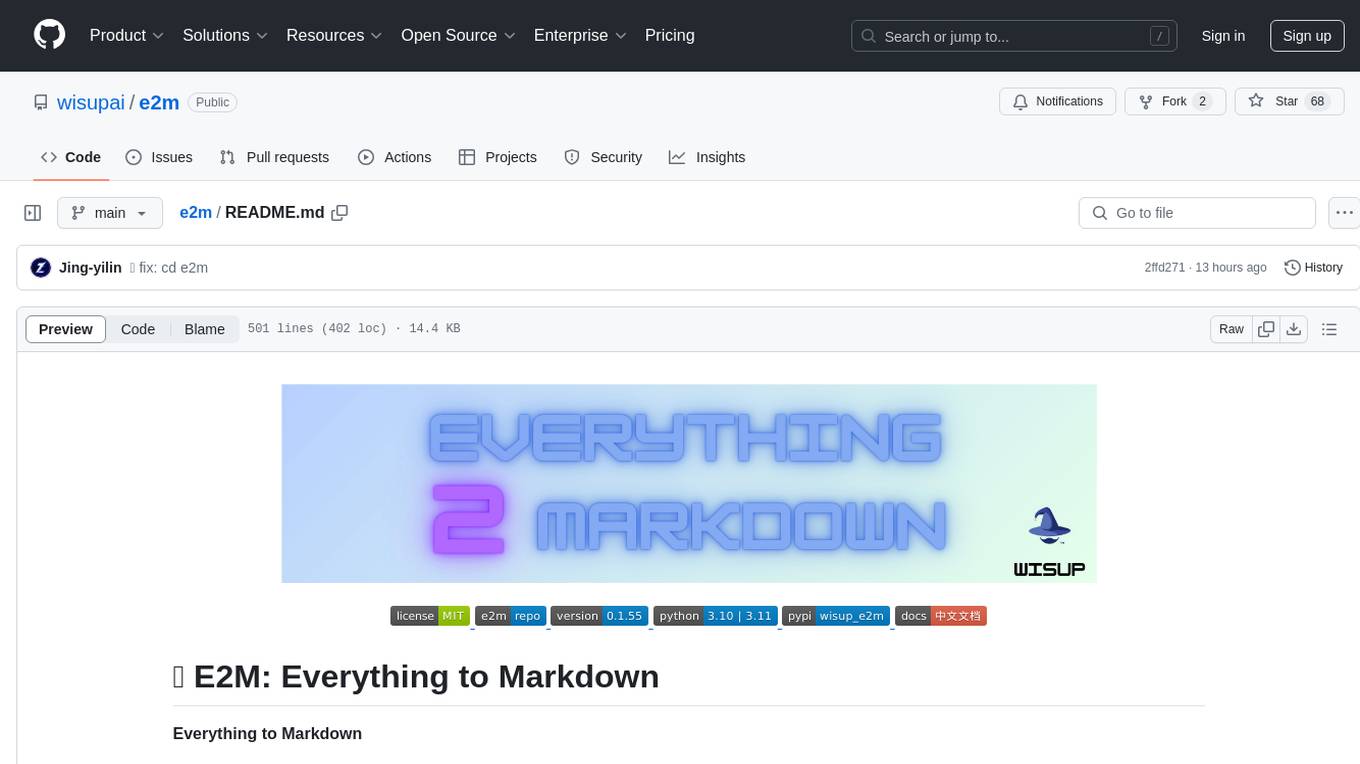
e2m
E2M is a Python library that can parse and convert various file types into Markdown format. It supports the conversion of multiple file formats, including doc, docx, epub, html, htm, url, pdf, ppt, pptx, mp3, and m4a. The ultimate goal of the E2M project is to provide high-quality data for Retrieval-Augmented Generation (RAG) and model training or fine-tuning. The core architecture consists of a Parser responsible for parsing various file types into text or image data, and a Converter responsible for converting text or image data into Markdown format.
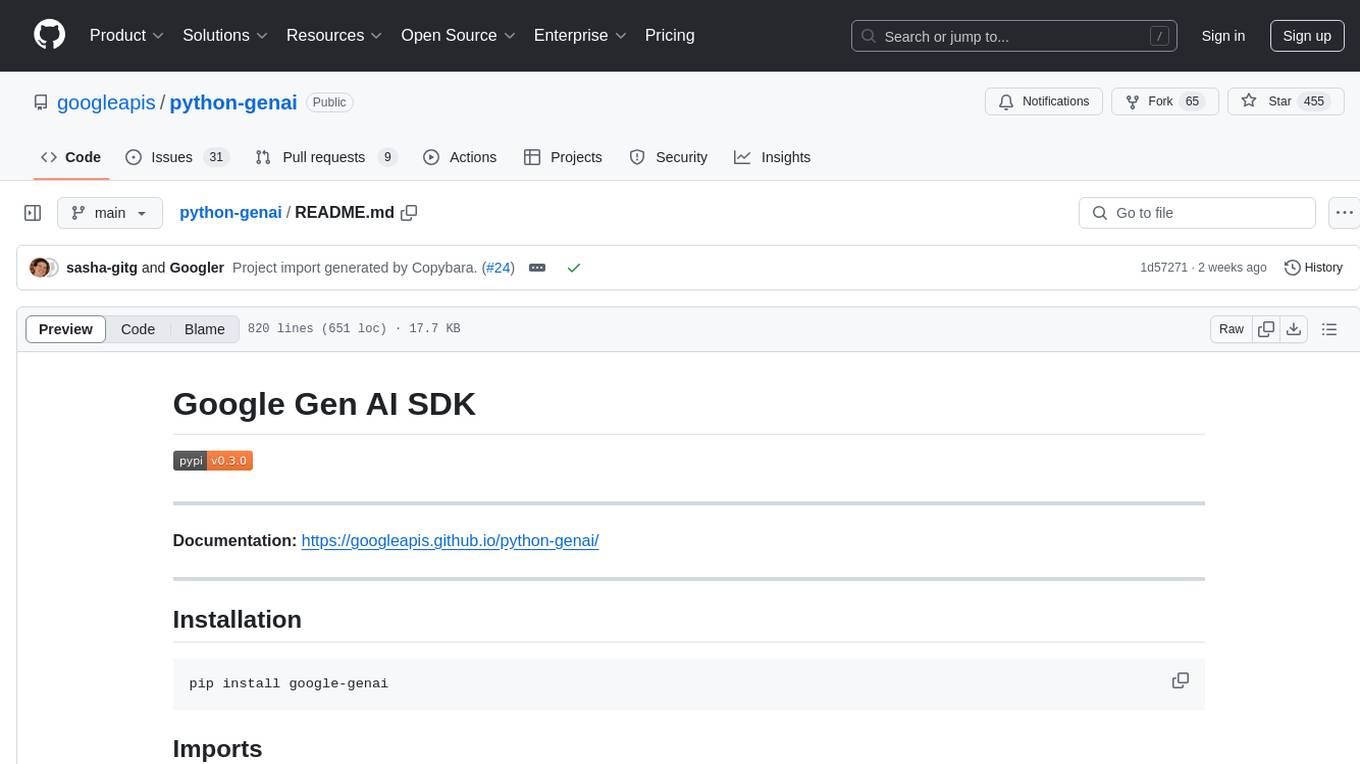
python-genai
The Google Gen AI SDK is a Python library that provides access to Google AI and Vertex AI services. It allows users to create clients for different services, work with parameter types, models, generate content, call functions, handle JSON response schemas, stream text and image content, perform async operations, count and compute tokens, embed content, generate and upscale images, edit images, work with files, create and get cached content, tune models, distill models, perform batch predictions, and more. The SDK supports various features like automatic function support, manual function declaration, JSON response schema support, streaming for text and image content, async methods, tuning job APIs, distillation, batch prediction, and more.
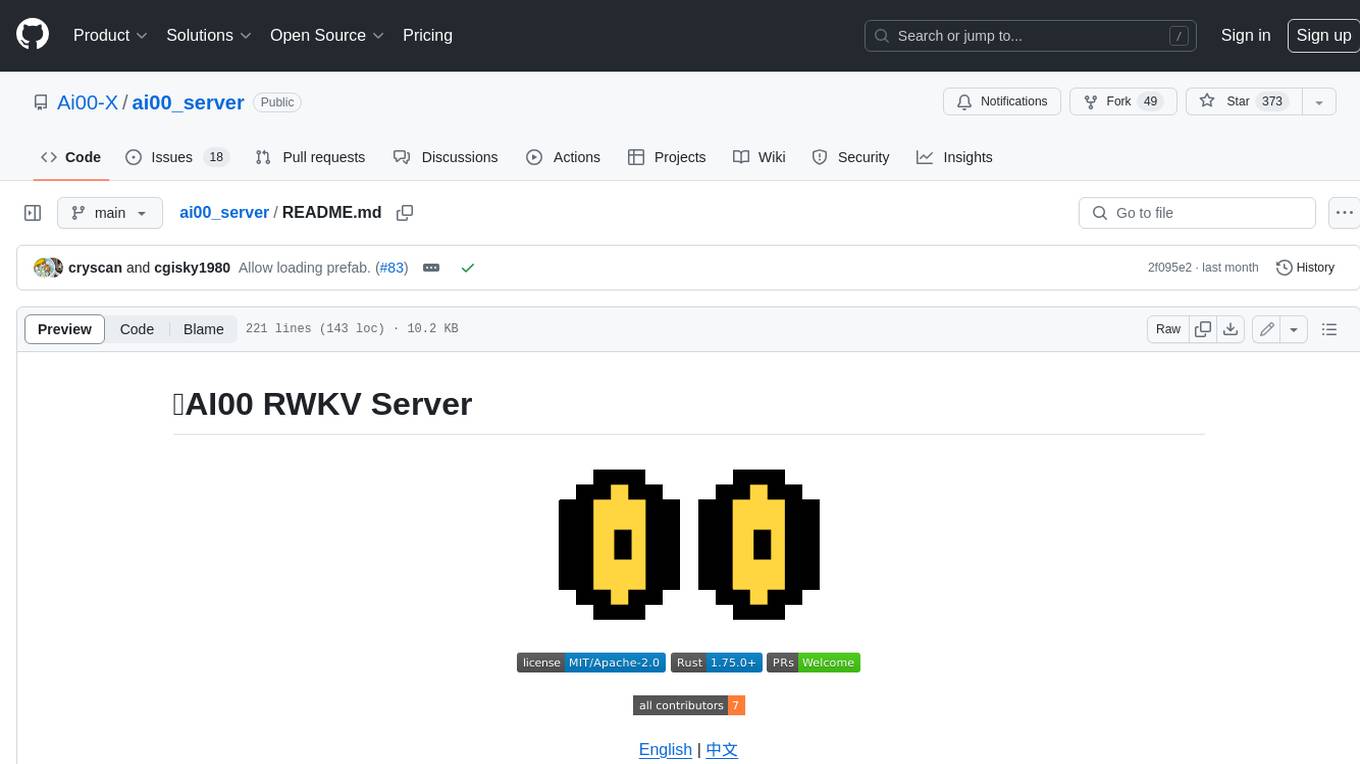
ai00_server
AI00 RWKV Server is an inference API server for the RWKV language model based upon the web-rwkv inference engine. It supports VULKAN parallel and concurrent batched inference and can run on all GPUs that support VULKAN. No need for Nvidia cards!!! AMD cards and even integrated graphics can be accelerated!!! No need for bulky pytorch, CUDA and other runtime environments, it's compact and ready to use out of the box! Compatible with OpenAI's ChatGPT API interface. 100% open source and commercially usable, under the MIT license. If you are looking for a fast, efficient, and easy-to-use LLM API server, then AI00 RWKV Server is your best choice. It can be used for various tasks, including chatbots, text generation, translation, and Q&A.
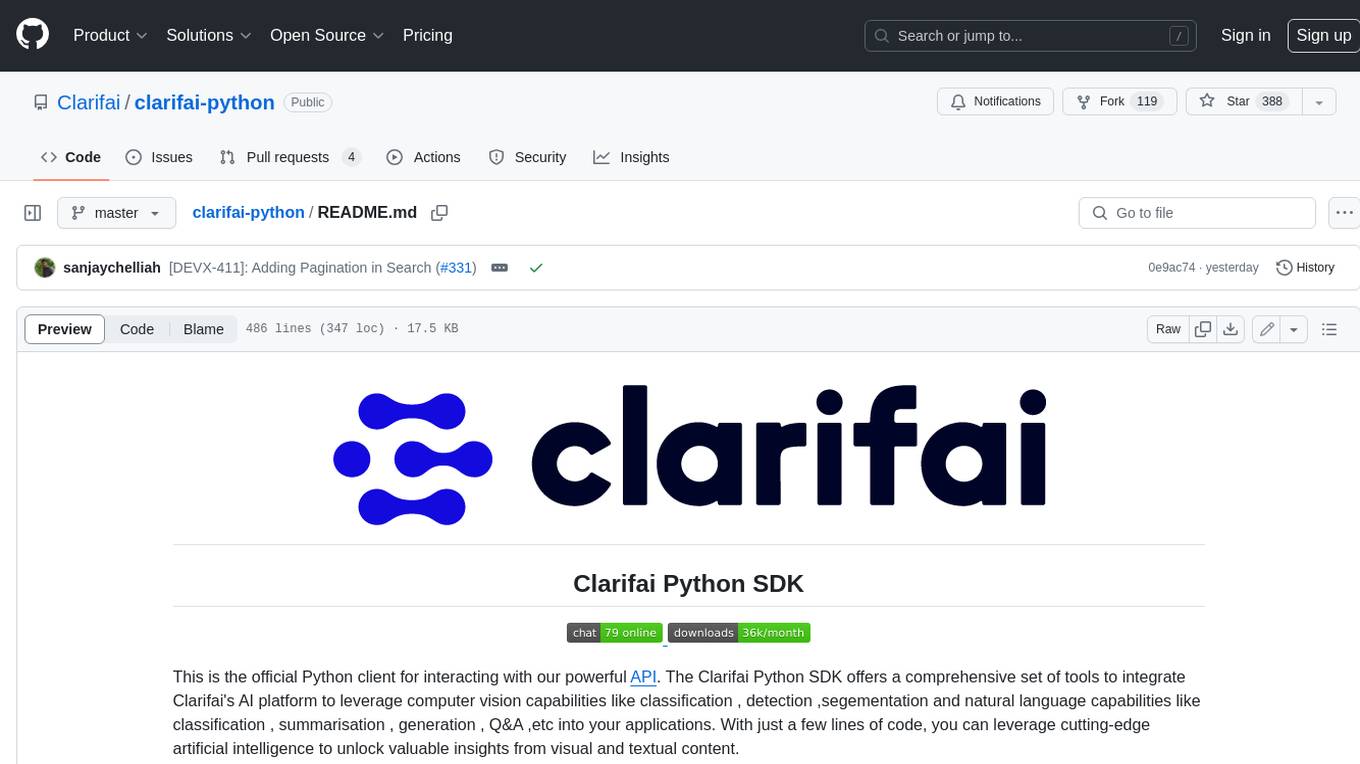
clarifai-python
The Clarifai Python SDK offers a comprehensive set of tools to integrate Clarifai's AI platform to leverage computer vision capabilities like classification , detection ,segementation and natural language capabilities like classification , summarisation , generation , Q&A ,etc into your applications. With just a few lines of code, you can leverage cutting-edge artificial intelligence to unlock valuable insights from visual and textual content.
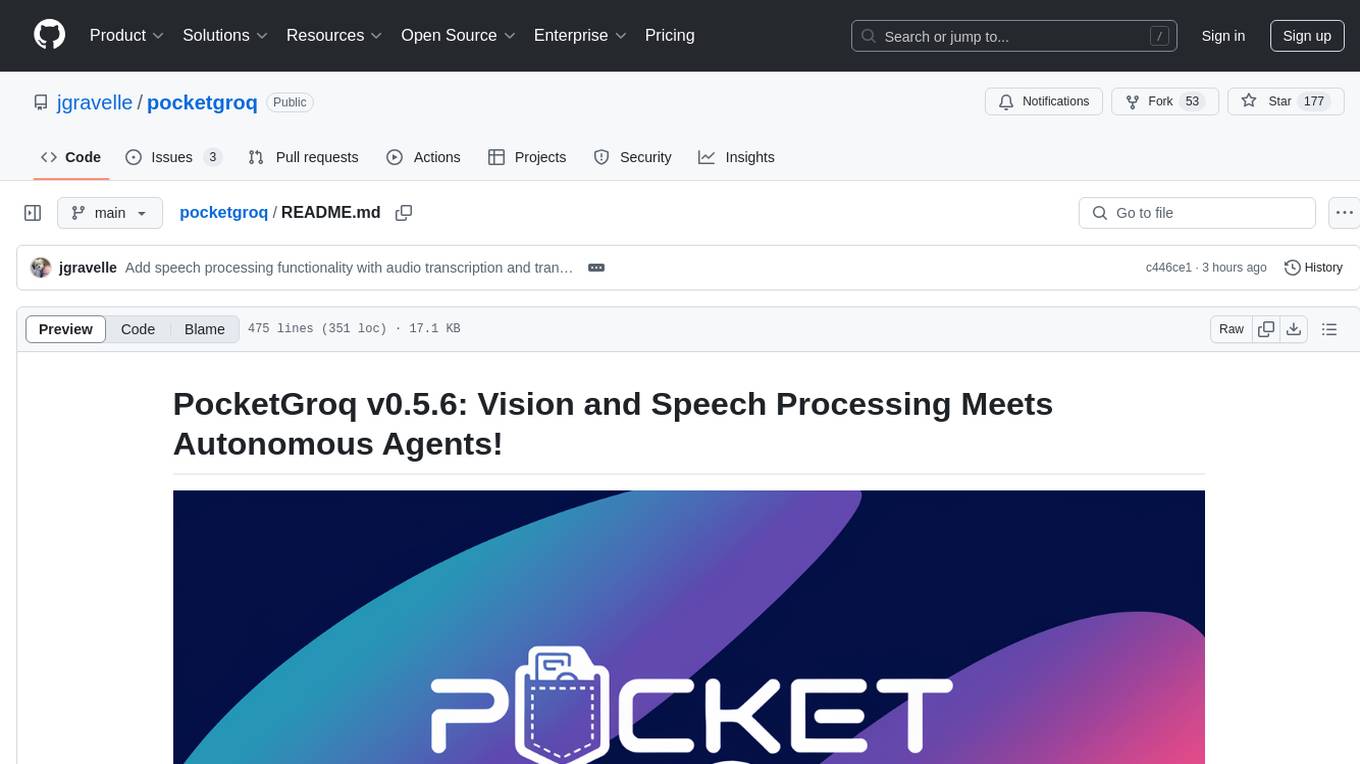
pocketgroq
PocketGroq is a tool that provides advanced functionalities for text generation, web scraping, web search, and AI response evaluation. It includes features like an Autonomous Agent for answering questions, web crawling and scraping capabilities, enhanced web search functionality, and flexible integration with Ollama server. Users can customize the agent's behavior, evaluate responses using AI, and utilize various methods for text generation, conversation management, and Chain of Thought reasoning. The tool offers comprehensive methods for different tasks, such as initializing RAG, error handling, and tool management. PocketGroq is designed to enhance development processes and enable the creation of AI-powered applications with ease.
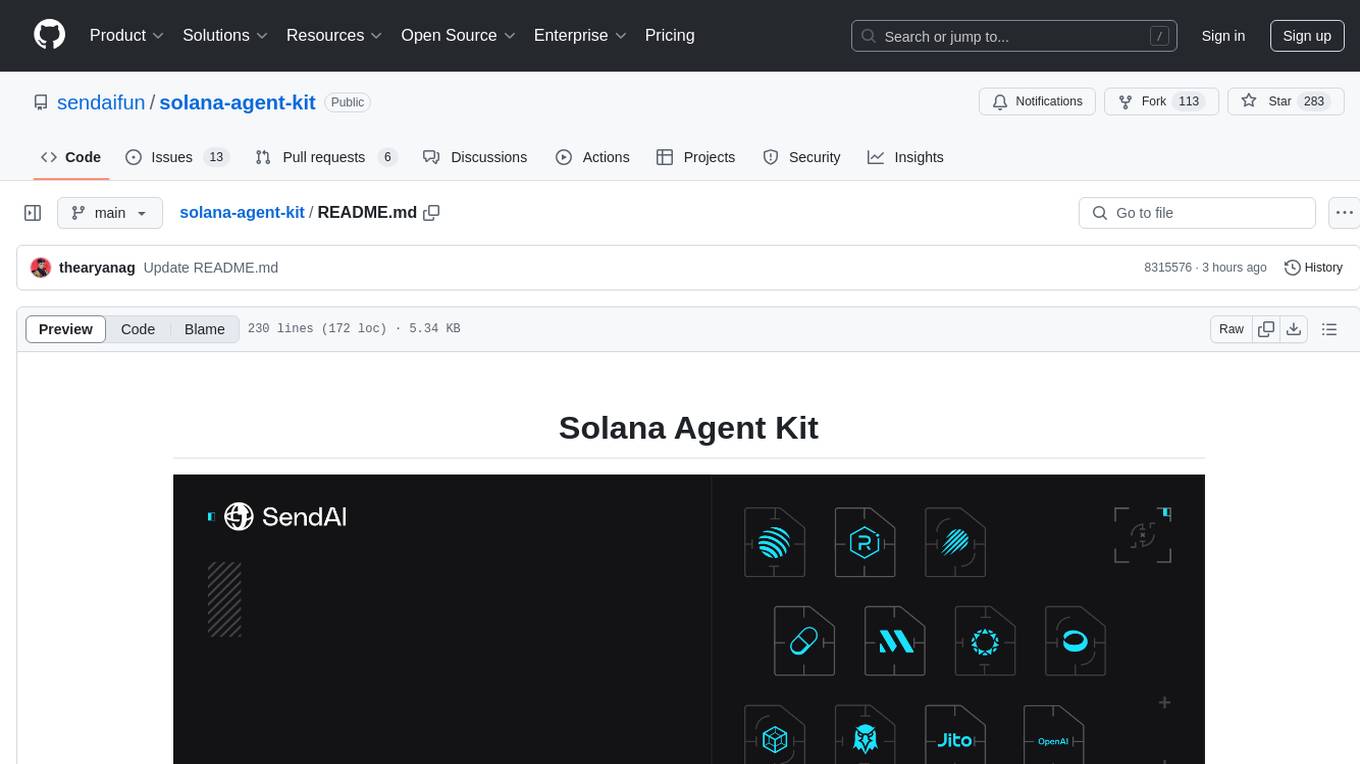
solana-agent-kit
Solana Agent Kit is an open-source toolkit designed for connecting AI agents to Solana protocols. It enables agents, regardless of the model used, to autonomously perform various Solana actions such as trading tokens, launching new tokens, lending assets, sending compressed airdrops, executing blinks, and more. The toolkit integrates core blockchain features like token operations, NFT management via Metaplex, DeFi integration, Solana blinks, AI integration features with LangChain, autonomous modes, and AI tools. It provides ready-to-use tools for blockchain operations, supports autonomous agent actions, and offers features like memory management, real-time feedback, and error handling. Solana Agent Kit facilitates tasks such as deploying tokens, creating NFT collections, swapping tokens, lending tokens, staking SOL, and sending SPL token airdrops via ZK compression. It also includes functionalities for fetching price data from Pyth and relies on key Solana and Metaplex libraries for its operations.
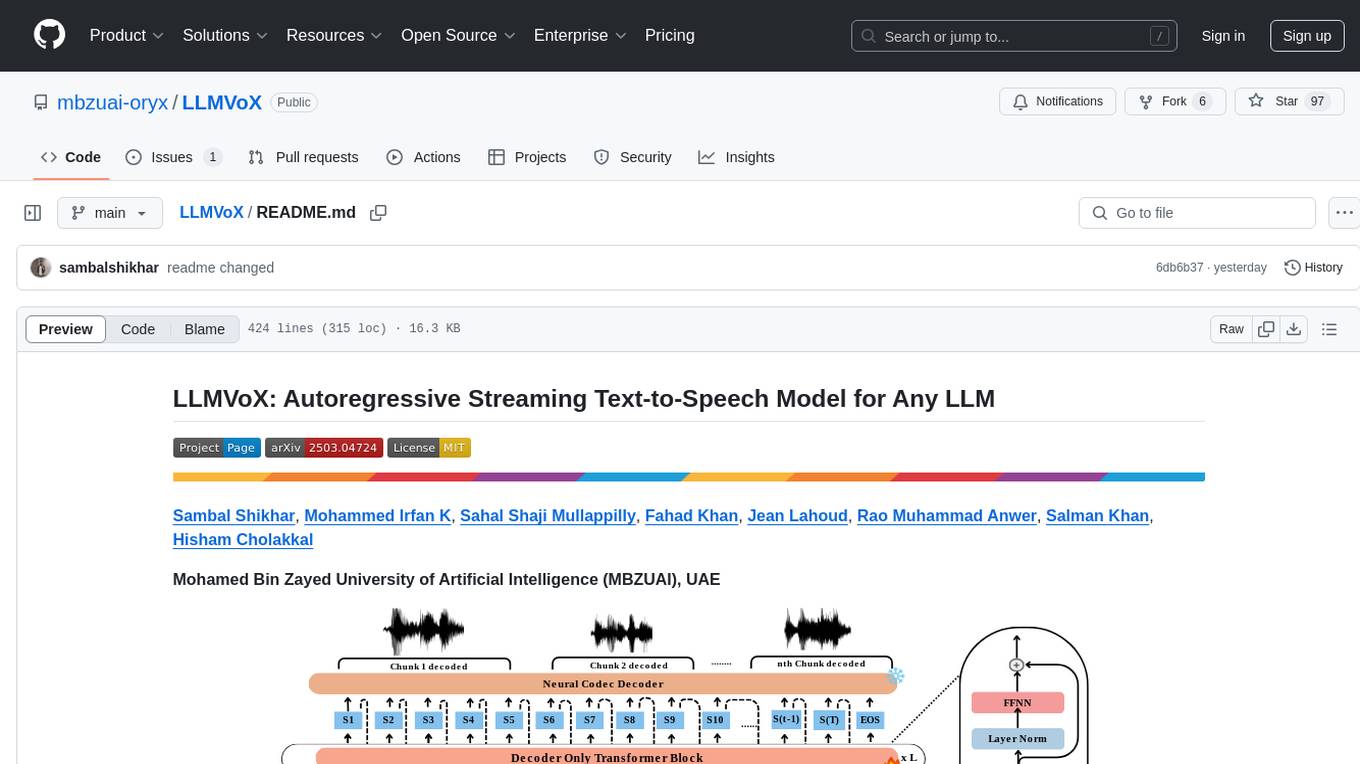
LLMVoX
LLMVoX is a lightweight 30M-parameter, LLM-agnostic, autoregressive streaming Text-to-Speech (TTS) system designed to convert text outputs from Large Language Models into high-fidelity streaming speech with low latency. It achieves significantly lower Word Error Rate compared to speech-enabled LLMs while operating at comparable latency and speech quality. Key features include being lightweight & fast with only 30M parameters, LLM-agnostic for easy integration with existing models, multi-queue streaming for continuous speech generation, and multilingual support for easy adaptation to new languages.
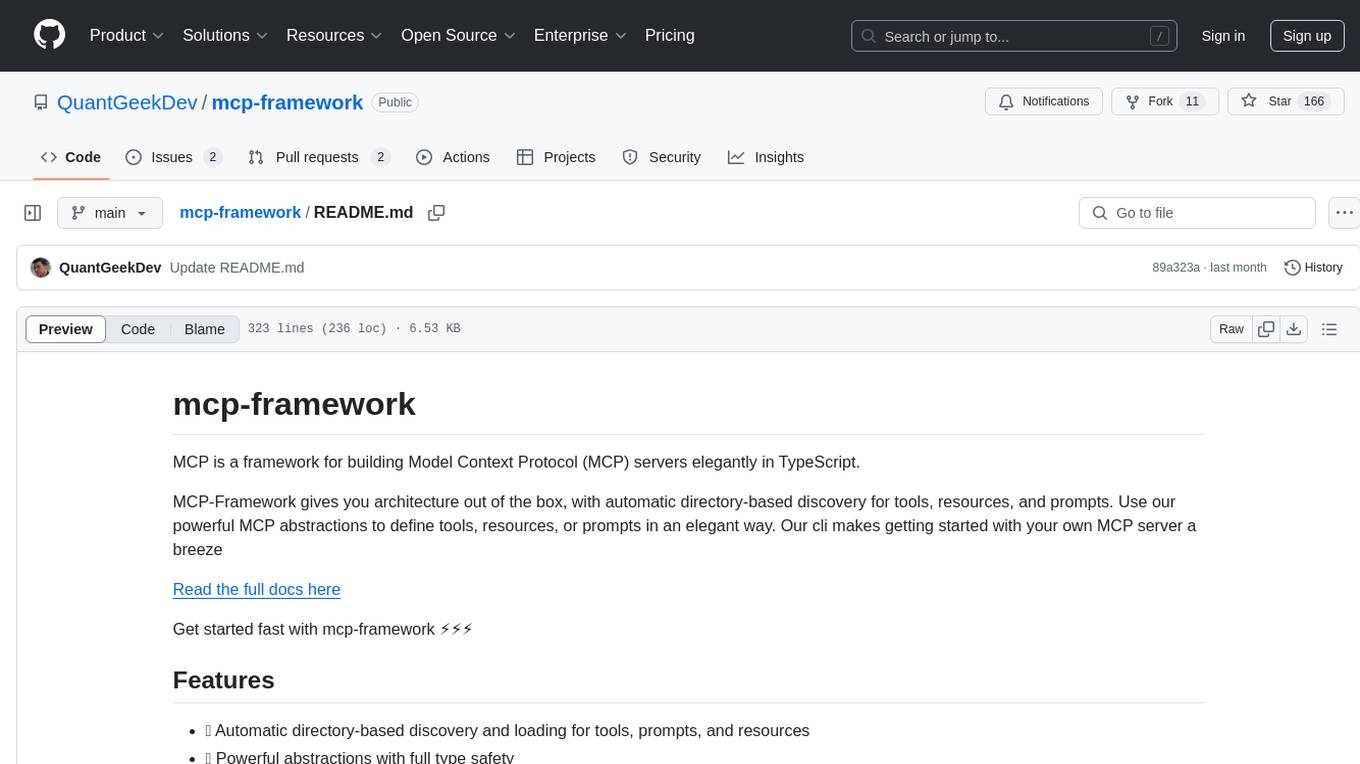
mcp-framework
MCP-Framework is a TypeScript framework for building Model Context Protocol (MCP) servers with automatic directory-based discovery for tools, resources, and prompts. It provides powerful abstractions, simple server setup, and a CLI for rapid development and project scaffolding.