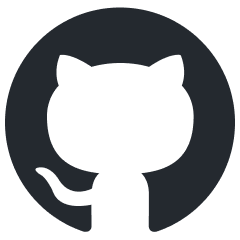
generative-ai-python
The official Python library for the Google Gemini API
Stars: 1982
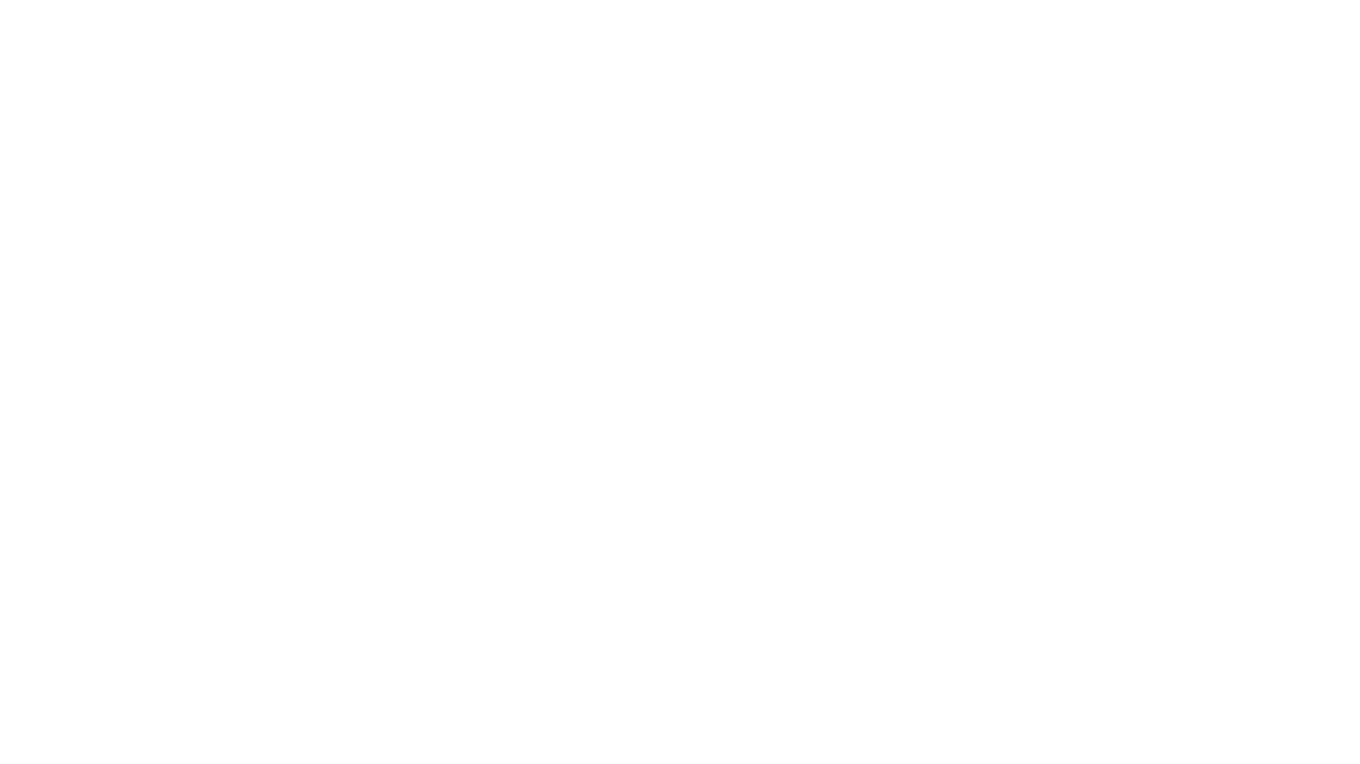
The Google AI Python SDK is the easiest way for Python developers to build with the Gemini API. The Gemini API gives you access to Gemini models created by Google DeepMind. Gemini models are built from the ground up to be multimodal, so you can reason seamlessly across text, images, and code.
README:
The Google AI Python SDK is the easiest way for Python developers to build with the Gemini API. The Gemini API gives you access to Gemini models created by Google DeepMind. Gemini models are built from the ground up to be multimodal, so you can reason seamlessly across text, images, and code.
- Go to Google AI Studio.
- Login with your Google account.
- Create an API key.
- Try a Python SDK quickstart in the Gemini API Cookbook.
- For detailed instructions, try the Python SDK tutorial on ai.google.dev.
See the Gemini API Cookbook or ai.google.dev for complete code.
- Install from PyPI.
pip install -U google-generativeai
- Import the SDK and configure your API key.
import google.generativeai as genai
import os
genai.configure(api_key=os.environ["GEMINI_API_KEY"])
- Create a model and run a prompt.
model = genai.GenerativeModel('gemini-1.5-flash')
response = model.generate_content("The opposite of hot is")
print(response.text)
See the Gemini API Cookbook or ai.google.dev for complete documentation.
See Contributing for more information on contributing to the Google AI Python SDK.
The contents of this repository are licensed under the Apache License, version 2.0.
For Tasks:
Click tags to check more tools for each tasksFor Jobs:
Alternative AI tools for generative-ai-python
Similar Open Source Tools
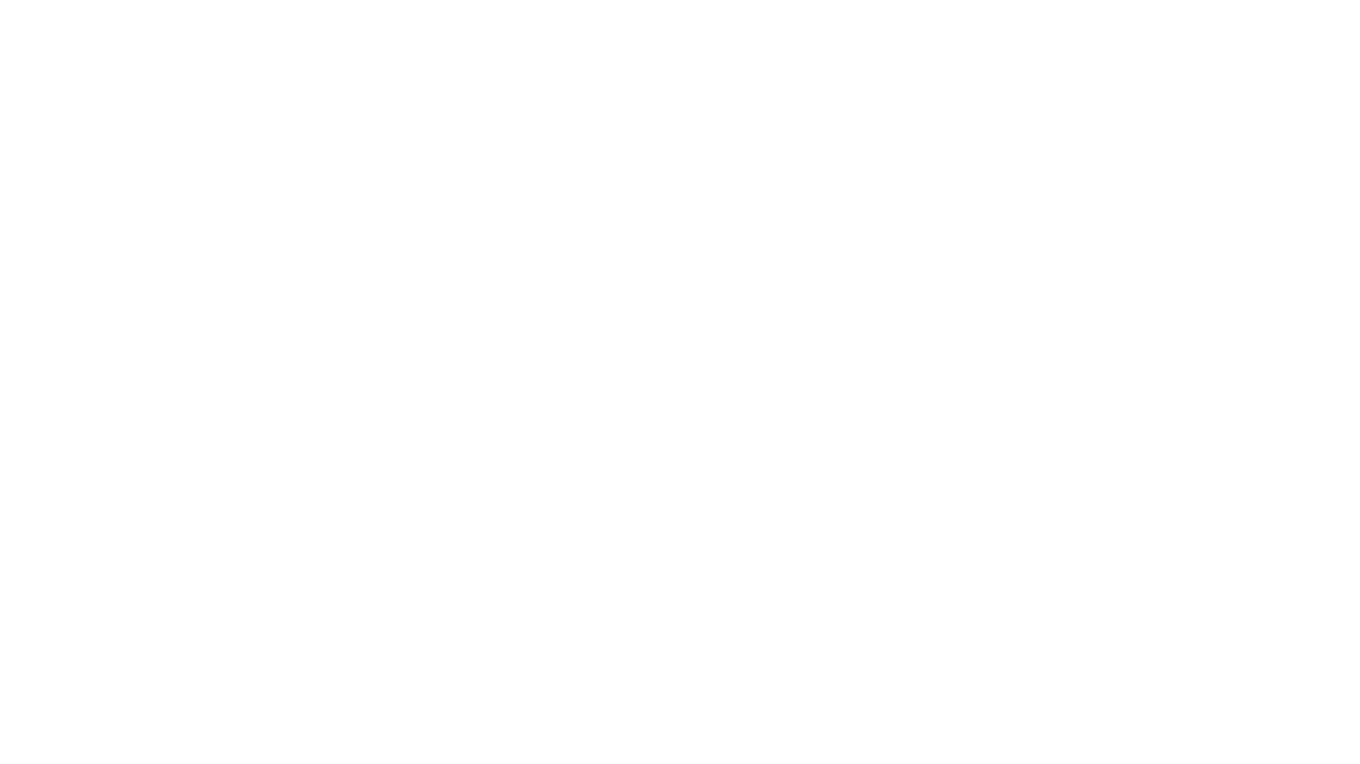
generative-ai-python
The Google AI Python SDK is the easiest way for Python developers to build with the Gemini API. The Gemini API gives you access to Gemini models created by Google DeepMind. Gemini models are built from the ground up to be multimodal, so you can reason seamlessly across text, images, and code.
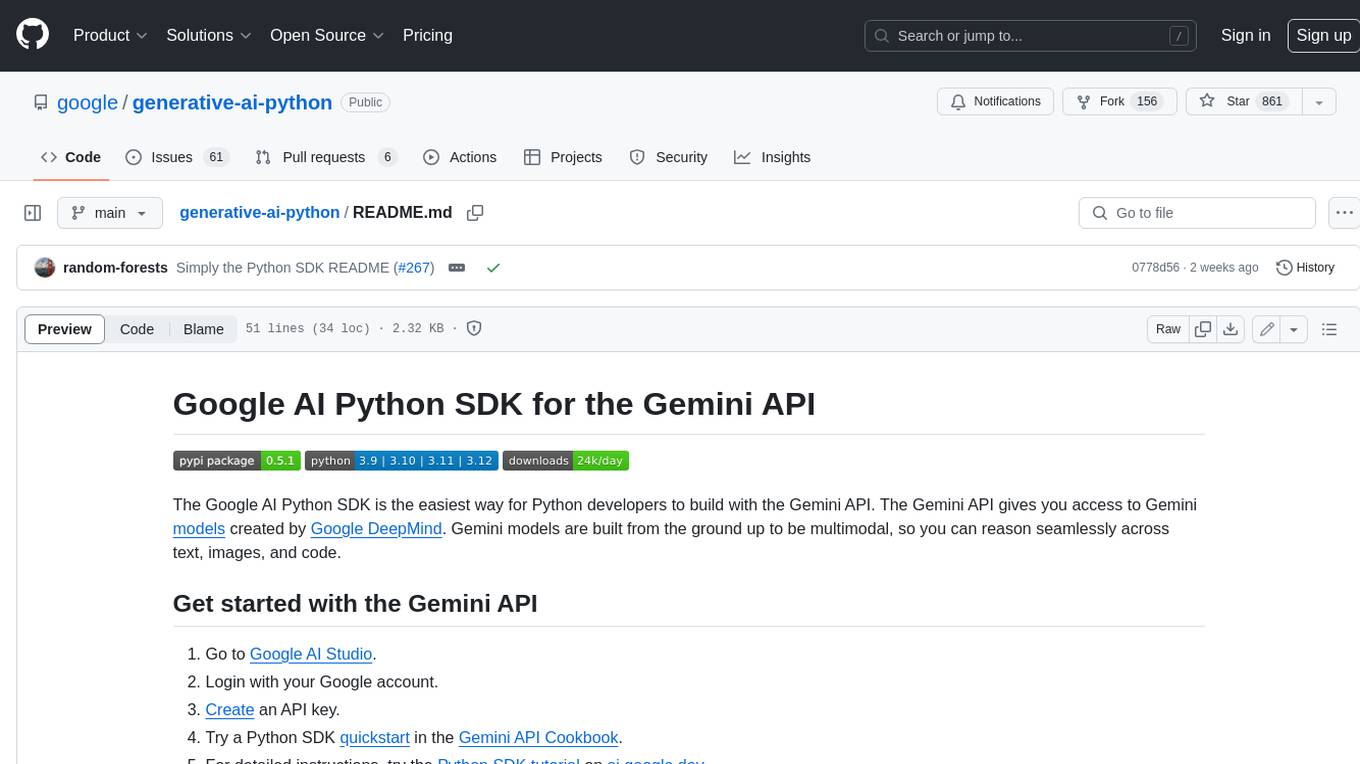
generative-ai-python
The Google AI Python SDK is the easiest way for Python developers to build with the Gemini API. The Gemini API gives you access to Gemini models created by Google DeepMind. Gemini models are built from the ground up to be multimodal, so you can reason seamlessly across text, images, and code.
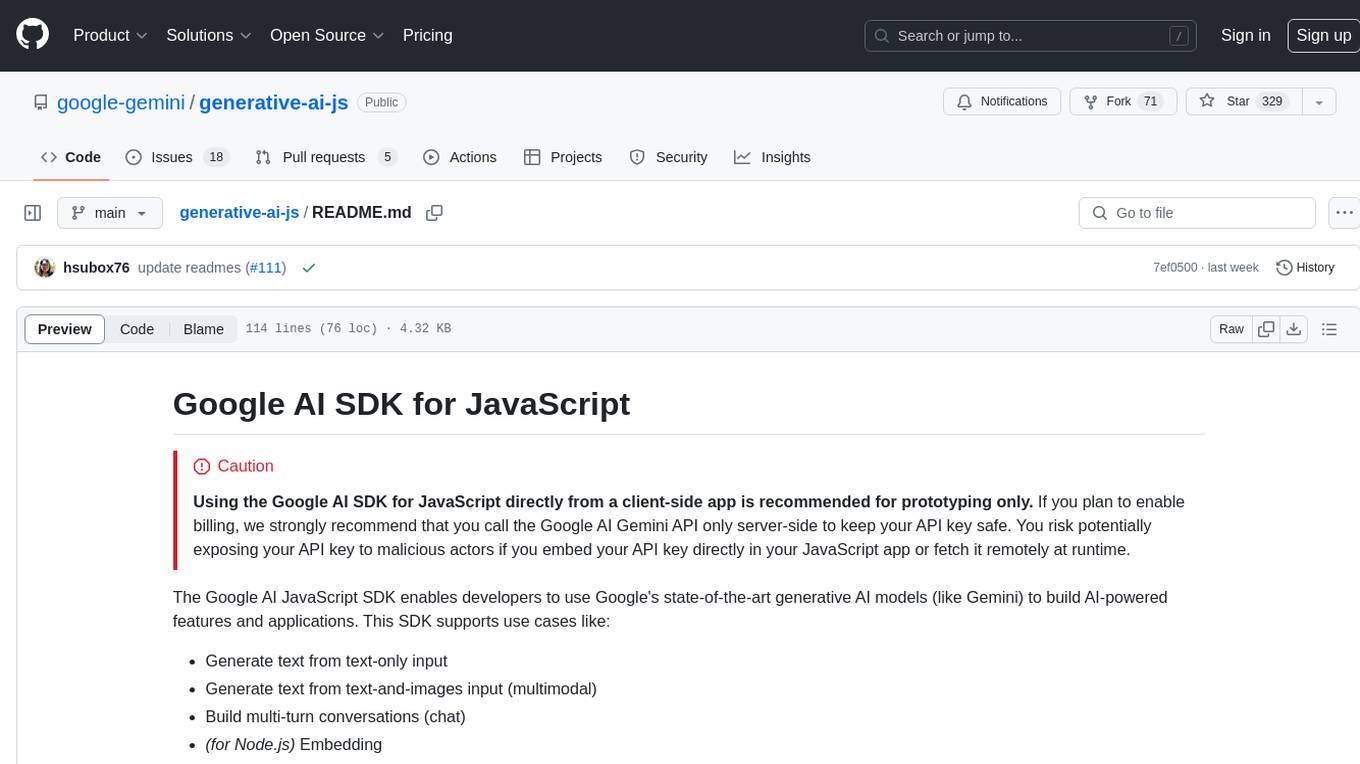
generative-ai-js
Generative AI JS is a JavaScript library that provides tools for creating generative art and music using artificial intelligence techniques. It allows users to generate unique and creative content by leveraging machine learning models. The library includes functions for generating images, music, and text based on user input and preferences. With Generative AI JS, users can explore the intersection of art and technology, experiment with different creative processes, and create dynamic and interactive content for various applications.
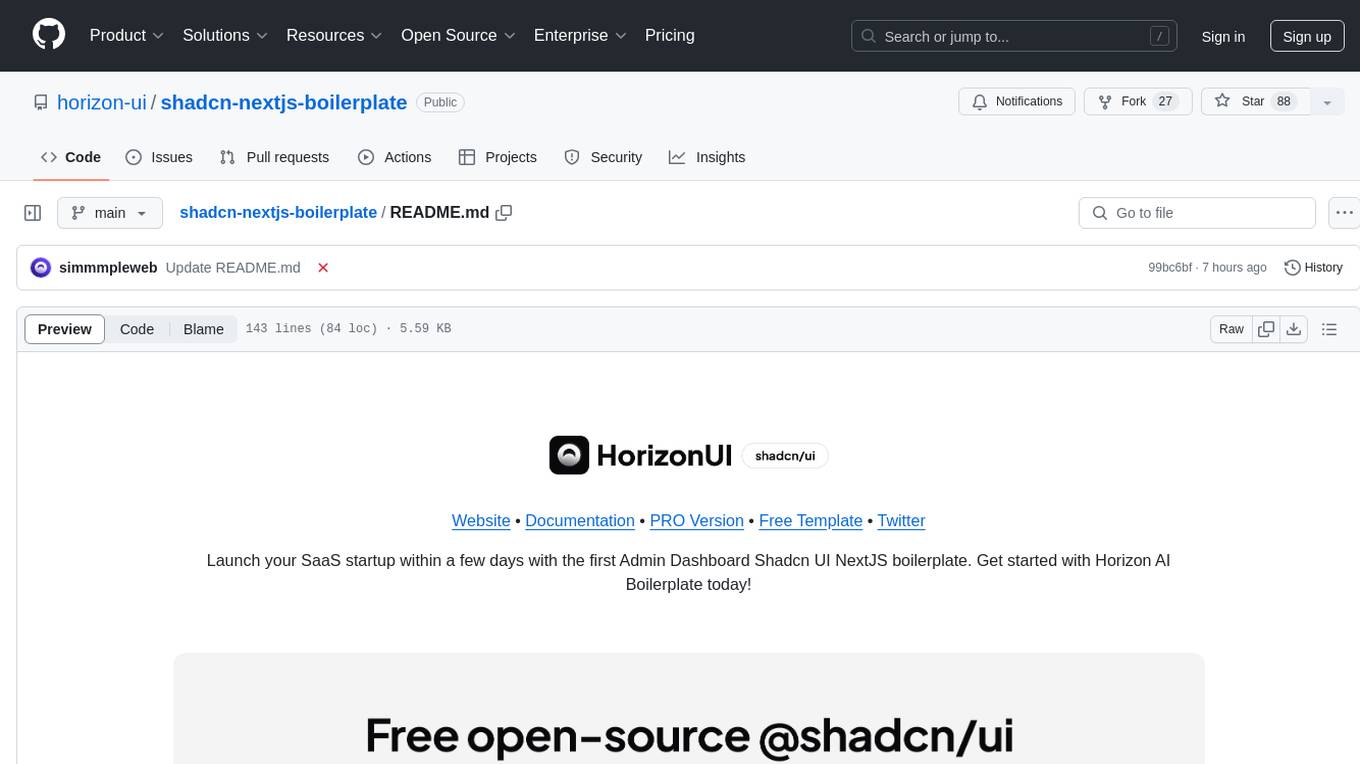
shadcn-nextjs-boilerplate
Horizon AI Boilerplate is an open-source Admin Dashboard template designed for Shadcn UI, NextJS, and Tailwind CSS. It provides over 30 dark/light frontend elements for creating Chat AI SaaS Apps quickly. The documentation is detailed and complex, guiding users through installation and usage. Users can start their local server with simple commands. The tool requires a valid OpenAI API key for ChatGPT functionality. Additionally, a Figma version is available for design purposes. The PRO version offers more components and pages. Users can report issues on GitHub and connect with the community via Discord. The tool credits open-source resources like Shadcn UI Library, NextJS Subscription Payments, and ChatBot UI by mckaywrigley.
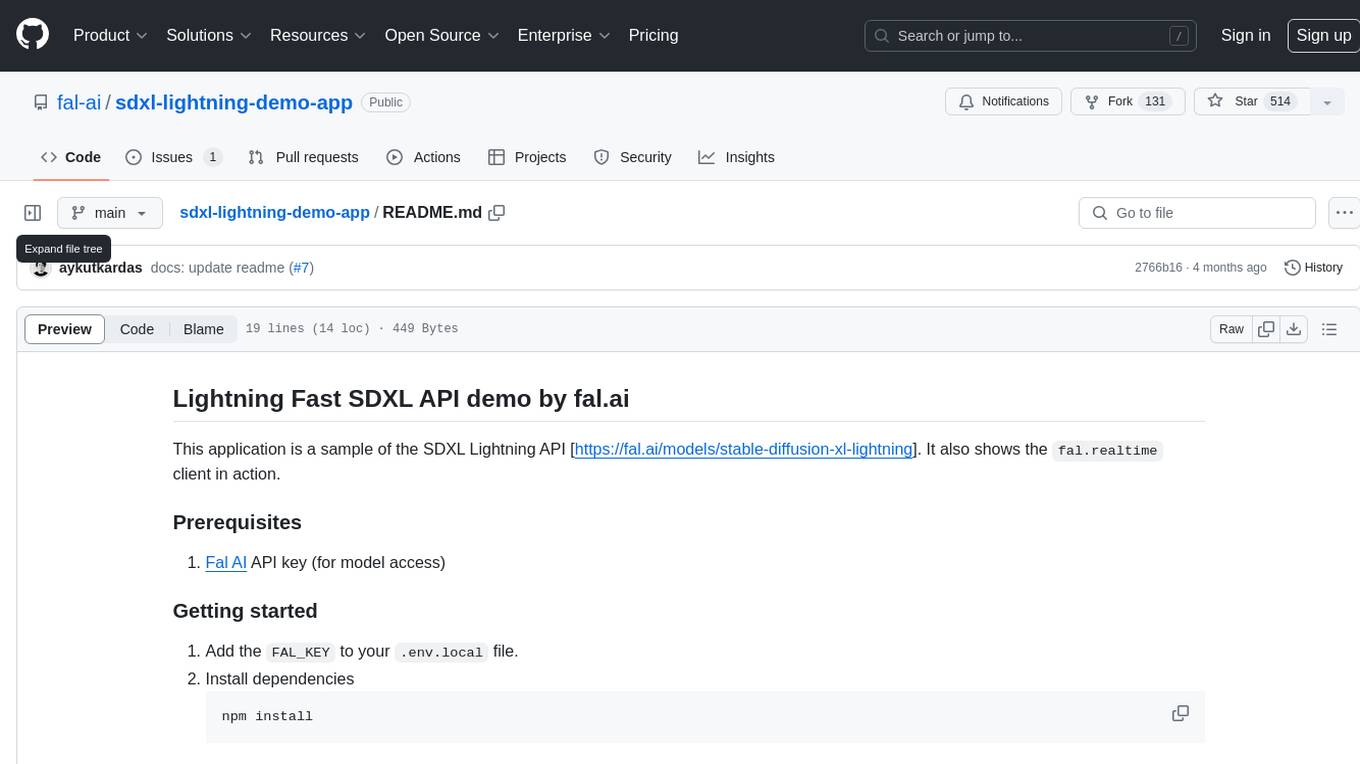
sdxl-lightning-demo-app
This repository contains a demo application showcasing the usage of the SDXL Lightning API by fal.ai. The application also demonstrates the functionality of the fal.realtime client. To get started, users need to have a Fal AI API key for model access. The setup involves adding the API key to the .env.local file, installing dependencies using 'npm install', and running the application with 'npm run dev'.
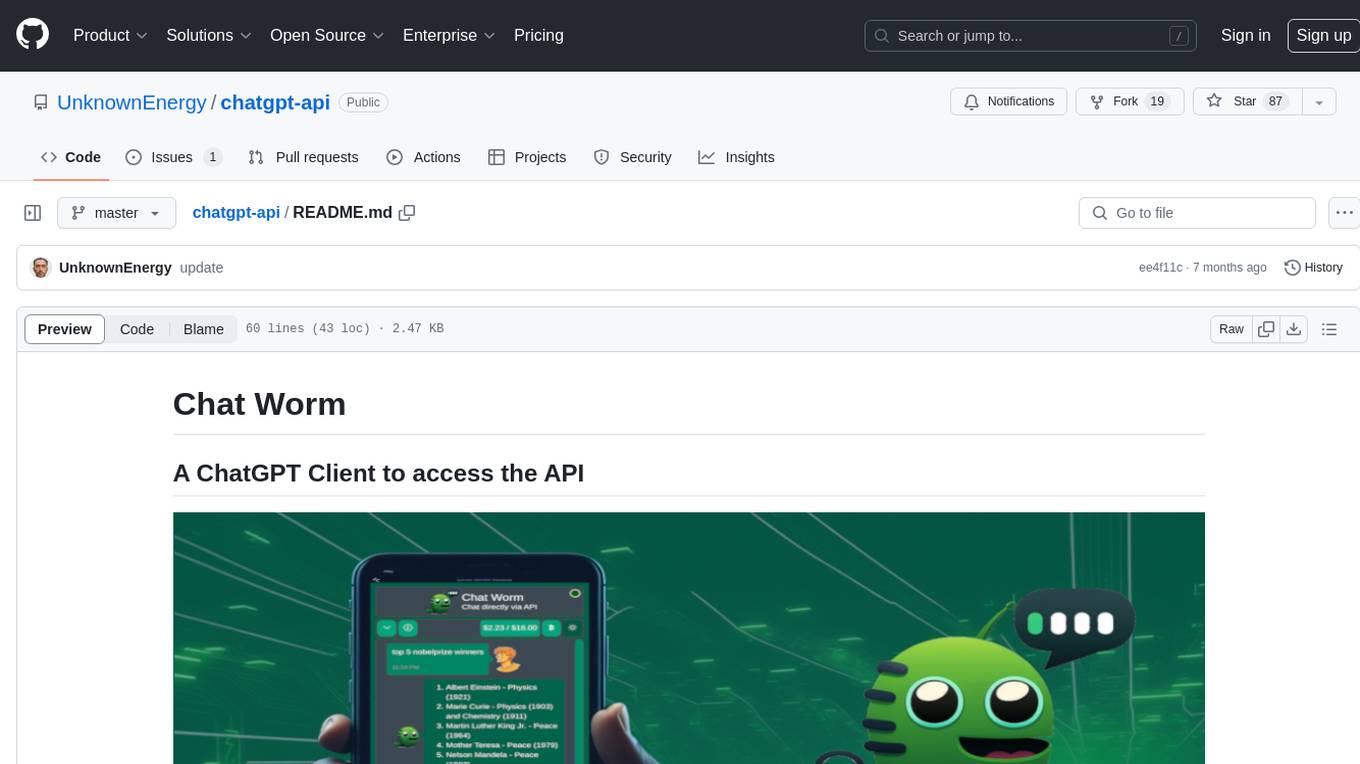
chatgpt-api
Chat Worm is a ChatGPT client that provides access to the API for generating text using OpenAI's GPT models. It works as a single-page application directly communicating with the API, allowing users to interact with the latest GPT-4 model if they have access. The project includes web, Android, and Windows apps for easy access. Users can set up local development, contribute improvements via pull requests, report bugs or request features on GitHub, deploy to production servers, and release on different app stores. The project is licensed under the MIT License.
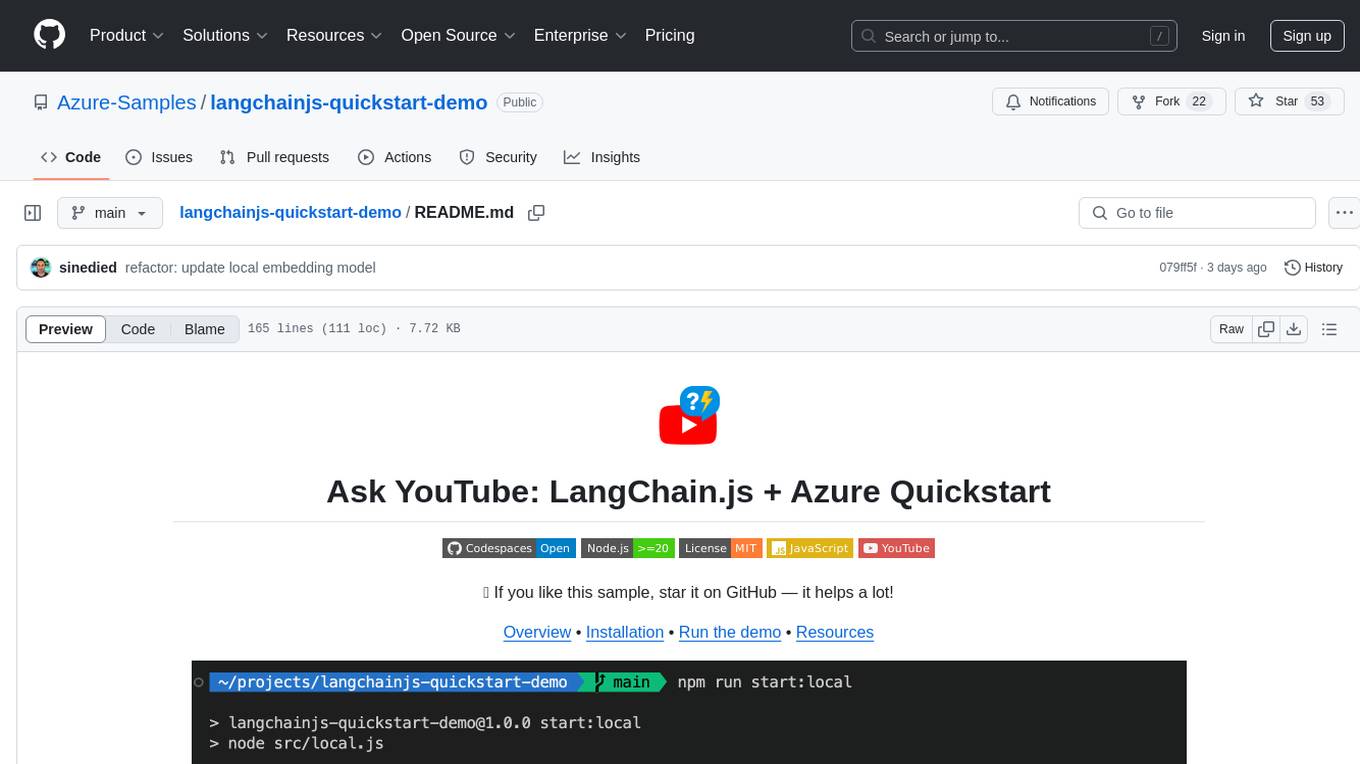
langchainjs-quickstart-demo
Discover the journey of building a generative AI application using LangChain.js and Azure. This demo explores the development process from idea to production, using a RAG-based approach for a Q&A system based on YouTube video transcripts. The application allows to ask text-based questions about a YouTube video and uses the transcript of the video to generate responses. The code comes in two versions: local prototype using FAISS and Ollama with LLaMa3 model for completion and all-minilm-l6-v2 for embeddings, and Azure cloud version using Azure AI Search and GPT-4 Turbo model for completion and text-embedding-3-large for embeddings. Either version can be run as an API using the Azure Functions runtime.
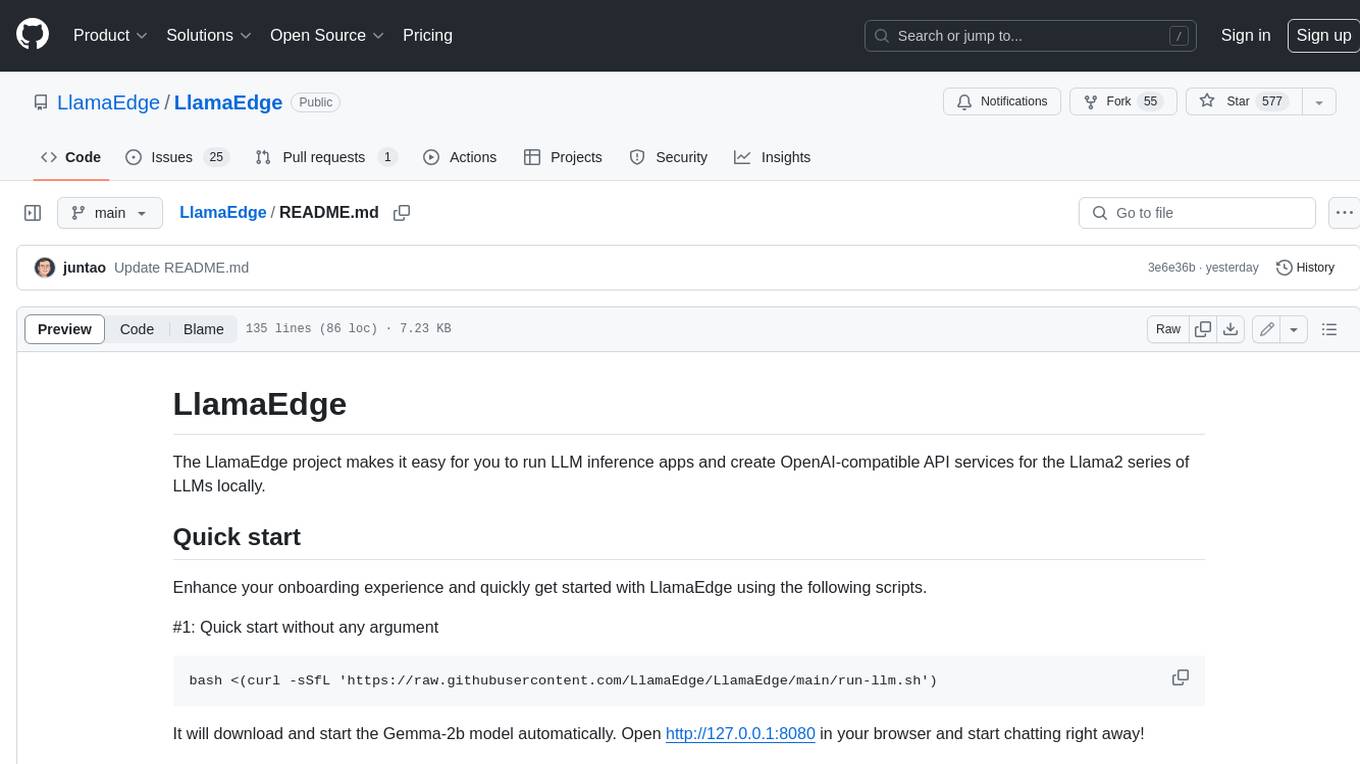
LlamaEdge
The LlamaEdge project makes it easy to run LLM inference apps and create OpenAI-compatible API services for the Llama2 series of LLMs locally. It provides a Rust+Wasm stack for fast, portable, and secure LLM inference on heterogeneous edge devices. The project includes source code for text generation, chatbot, and API server applications, supporting all LLMs based on the llama2 framework in the GGUF format. LlamaEdge is committed to continuously testing and validating new open-source models and offers a list of supported models with download links and startup commands. It is cross-platform, supporting various OSes, CPUs, and GPUs, and provides troubleshooting tips for common errors.
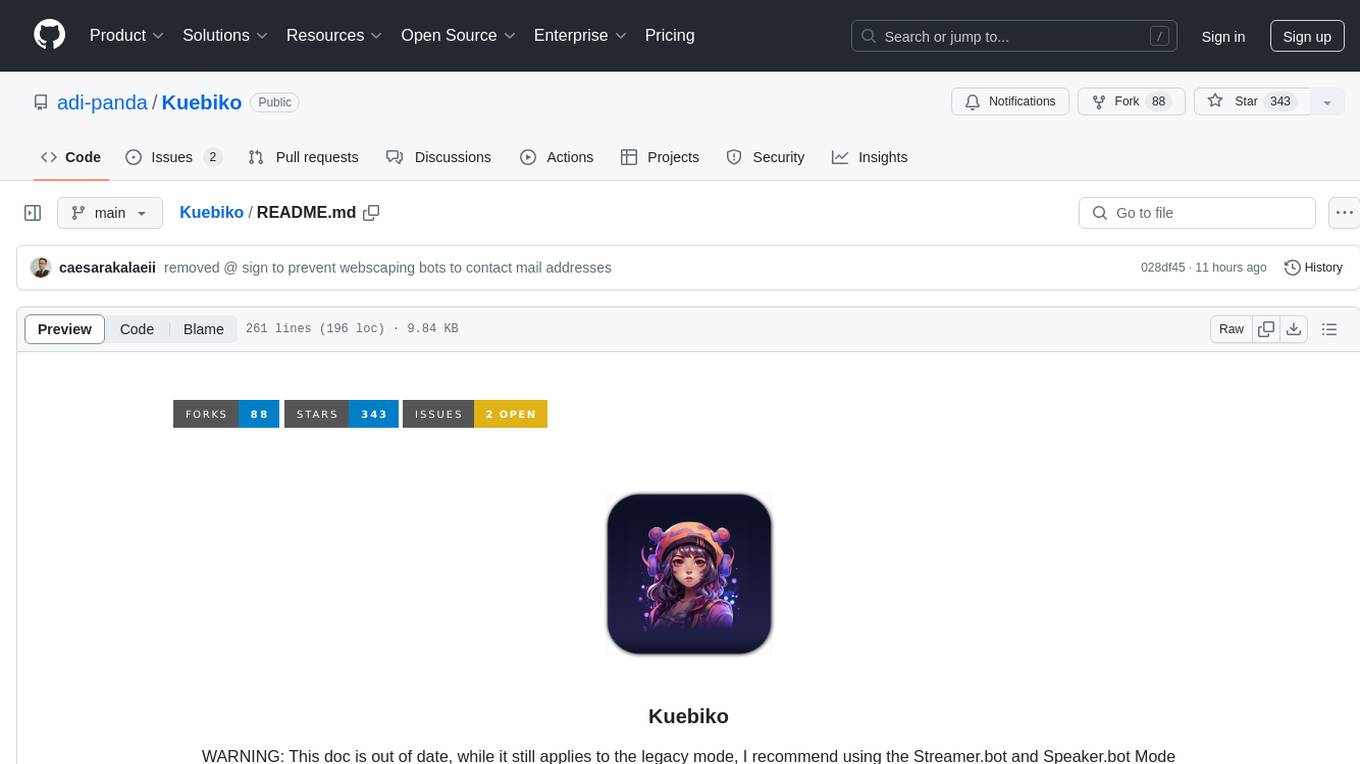
Kuebiko
Kuebiko is a Twitch Chat Bot that reads twitch chat and generates text-to-speech responses using Google Cloud API and OpenAI's GPT-3 text completion model. It allows users to set up their own VTuber AI similar to 'Neuro-Sama'. The project is built with Python and requires setting up various API keys and configurations to enable the bot functionality. Users can customize the voice of their VTuber and route audio using VBAudio Cable. Kuebiko provides a unique way to interact with viewers through chat responses and captions in OBS.
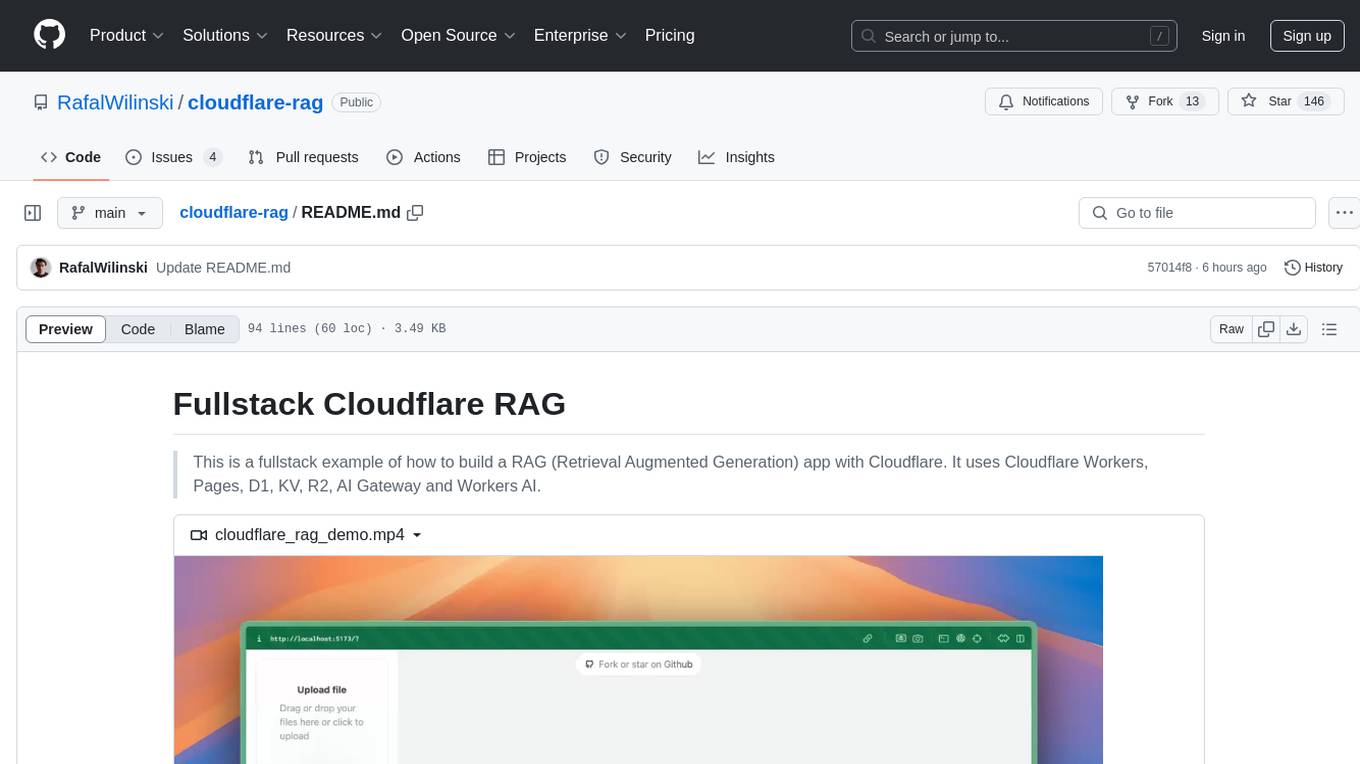
cloudflare-rag
This repository provides a fullstack example of building a Retrieval Augmented Generation (RAG) app with Cloudflare. It utilizes Cloudflare Workers, Pages, D1, KV, R2, AI Gateway, and Workers AI. The app features streaming interactions to the UI, hybrid RAG with Full-Text Search and Vector Search, switchable providers using AI Gateway, per-IP rate limiting with Cloudflare's KV, OCR within Cloudflare Worker, and Smart Placement for workload optimization. The development setup requires Node, pnpm, and wrangler CLI, along with setting up necessary primitives and API keys. Deployment involves setting up secrets and deploying the app to Cloudflare Pages. The project implements a Hybrid Search RAG approach combining Full Text Search against D1 and Hybrid Search with embeddings against Vectorize to enhance context for the LLM.
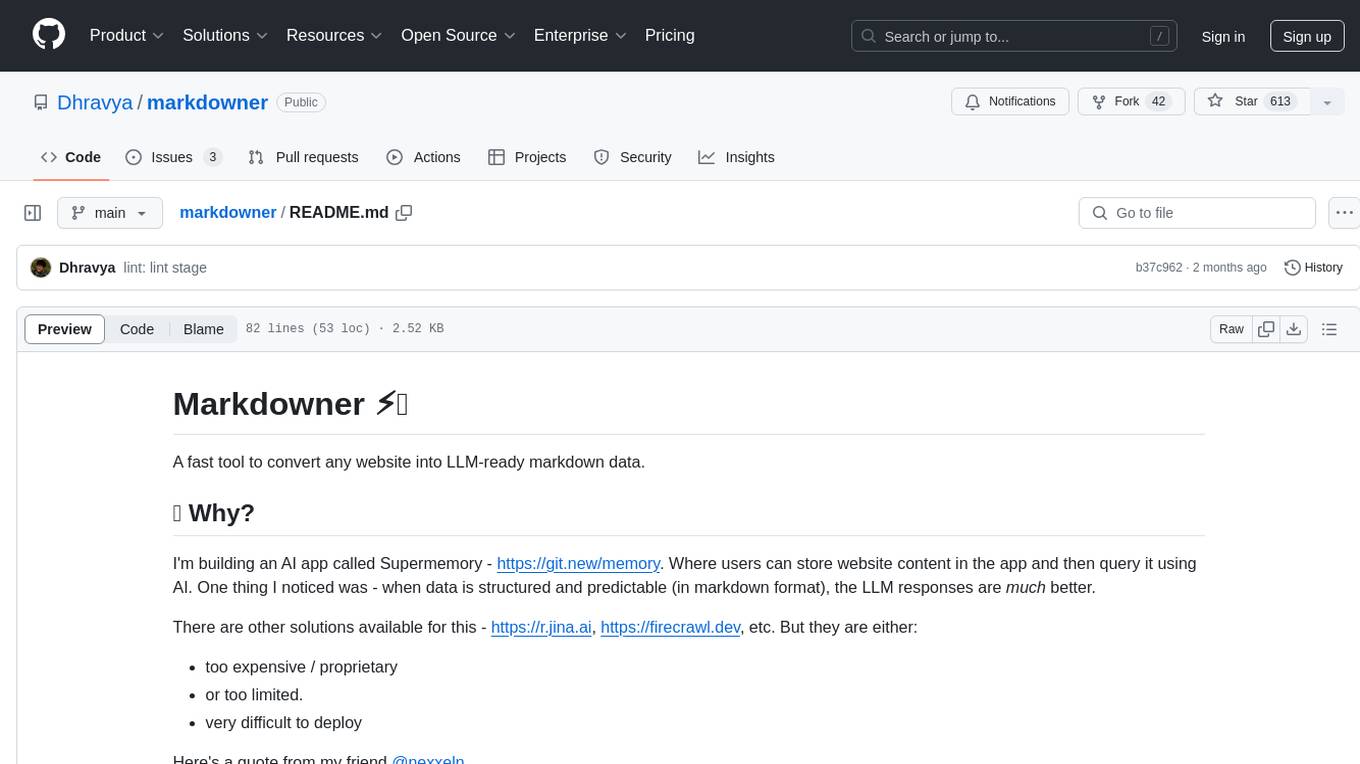
markdowner
Markdowner is a fast tool designed to convert any website into LLM-ready markdown data. It aims to improve the quality of responses in the AI app Supermemory by structuring and predicting data in markdown format. The tool offers features such as website conversion, LLM filtering, detailed markdown mode, auto crawler, text and JSON responses, and easy self-hosting. Markdowner utilizes Cloudflare's Browser rendering and Durable objects for browser instance creation and markdown conversion. Users can self-host the project with the Workers paid plan, following simple steps. Support the project by starring the repository.
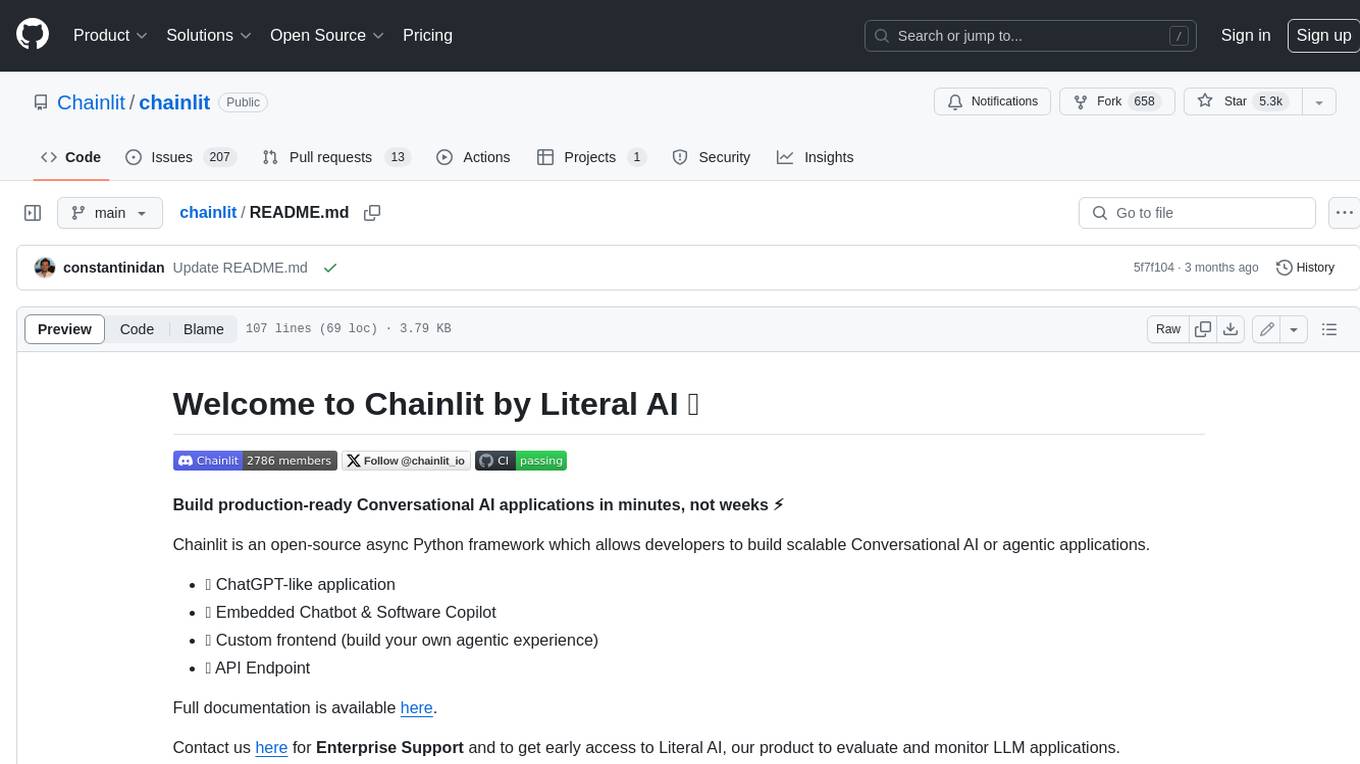
chainlit
Chainlit is an open-source async Python framework which allows developers to build scalable Conversational AI or agentic applications. It enables users to create ChatGPT-like applications, embedded chatbots, custom frontends, and API endpoints. The framework provides features such as multi-modal chats, chain of thought visualization, data persistence, human feedback, and an in-context prompt playground. Chainlit is compatible with various Python programs and libraries, including LangChain, Llama Index, Autogen, OpenAI Assistant, and Haystack. It offers a range of examples and a cookbook to showcase its capabilities and inspire users. Chainlit welcomes contributions and is licensed under the Apache 2.0 license.
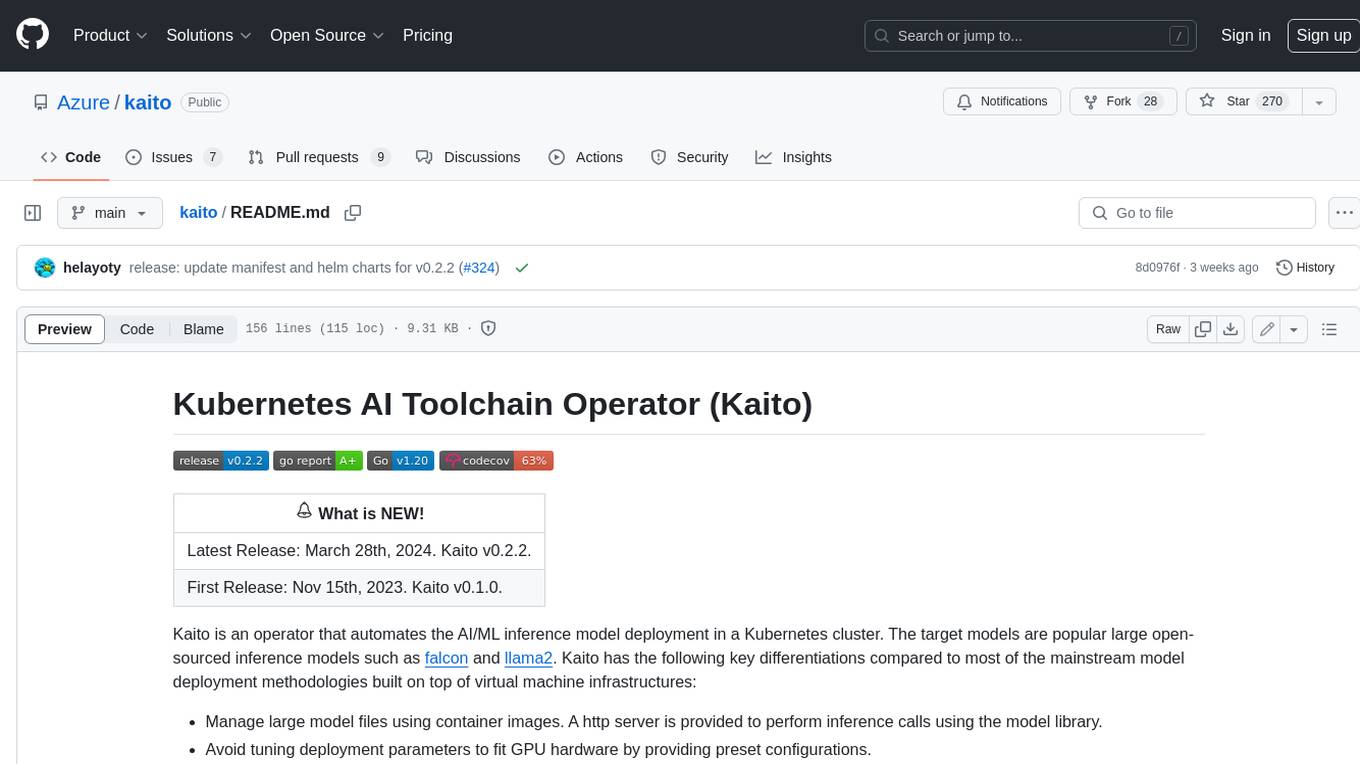
kaito
Kaito is an operator that automates the AI/ML inference model deployment in a Kubernetes cluster. It manages large model files using container images, avoids tuning deployment parameters to fit GPU hardware by providing preset configurations, auto-provisions GPU nodes based on model requirements, and hosts large model images in the public Microsoft Container Registry (MCR) if the license allows. Using Kaito, the workflow of onboarding large AI inference models in Kubernetes is largely simplified.
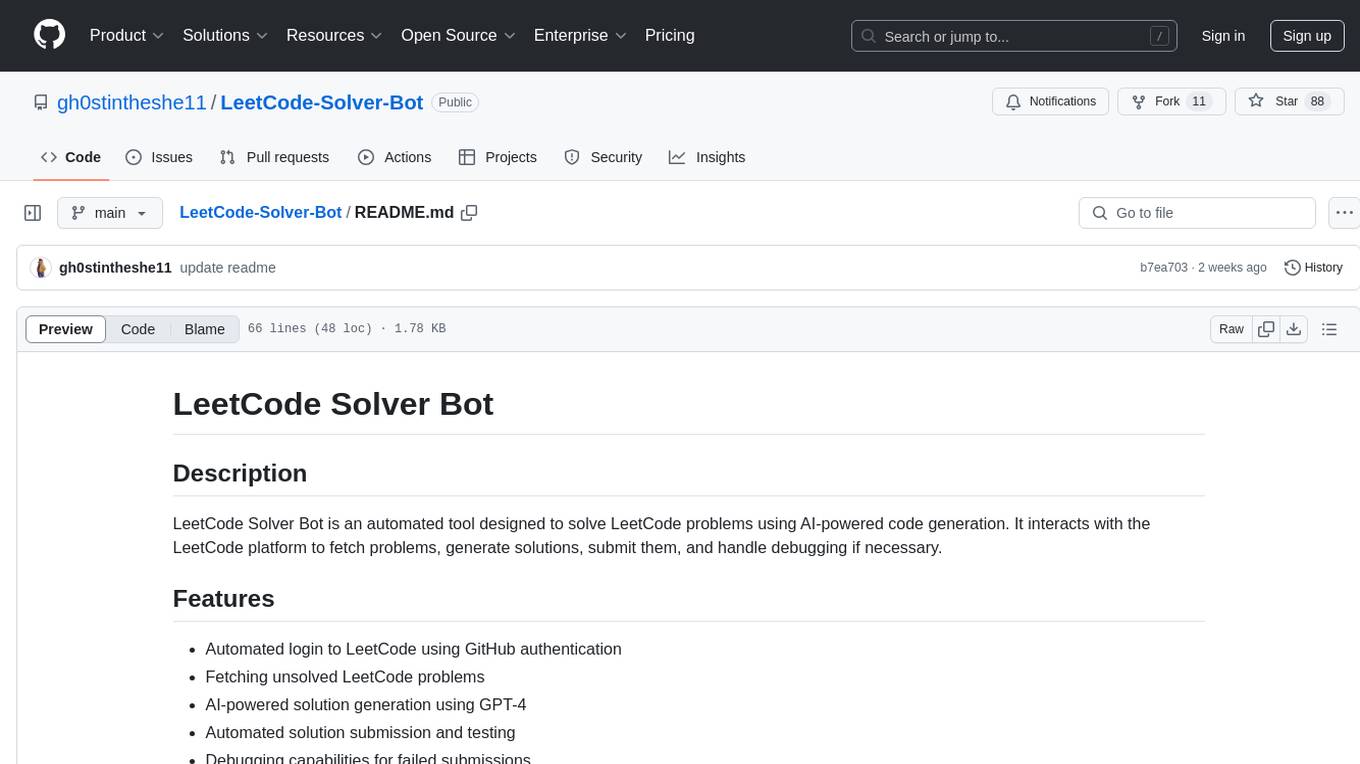
LeetCode-Solver-Bot
LeetCode Solver Bot is an automated tool designed to solve LeetCode problems using AI-powered code generation. It interacts with the LeetCode platform to fetch problems, generate solutions, submit them, and handle debugging if necessary. The tool supports automated login using GitHub authentication, fetching unsolved problems, AI-powered solution generation with GPT-4, automated solution submission and testing, debugging capabilities for failed submissions, and currently focuses on Python programming language.
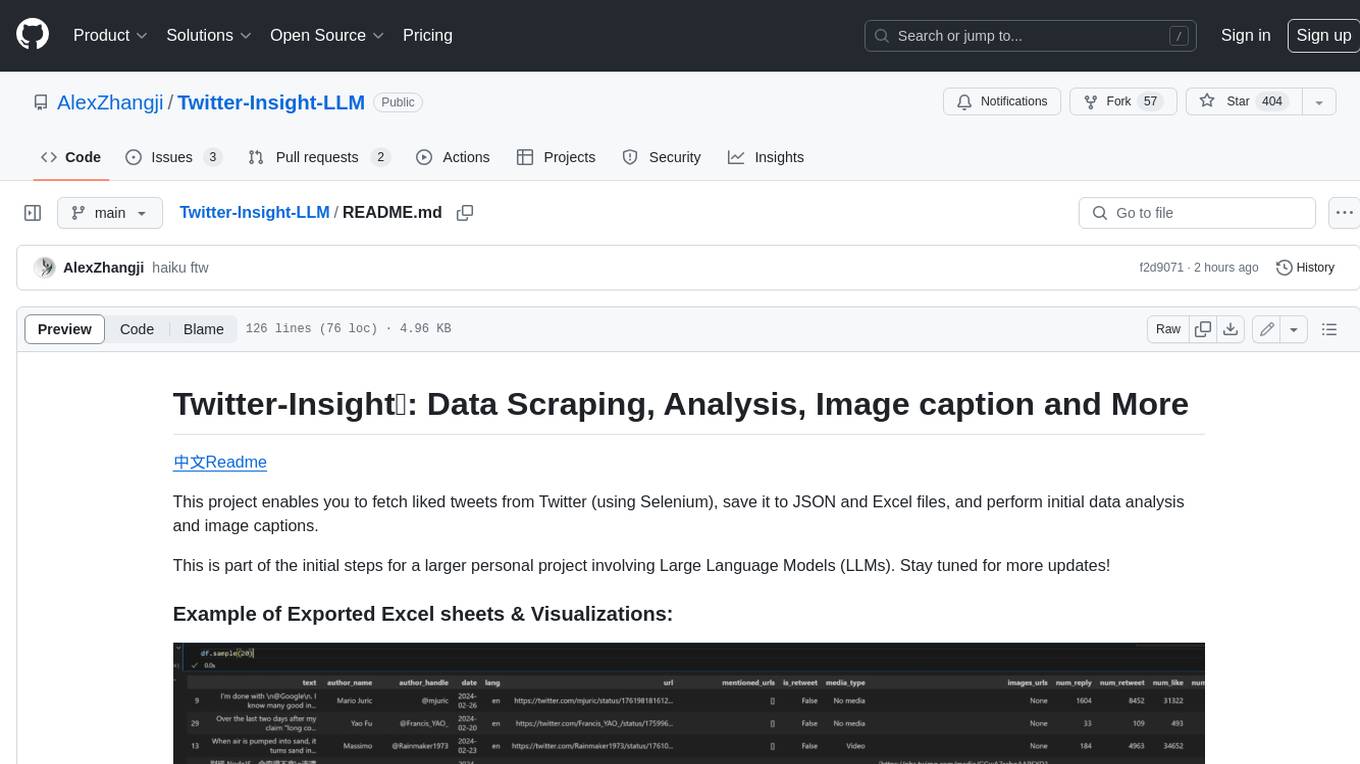
Twitter-Insight-LLM
This project enables you to fetch liked tweets from Twitter (using Selenium), save it to JSON and Excel files, and perform initial data analysis and image captions. This is part of the initial steps for a larger personal project involving Large Language Models (LLMs).
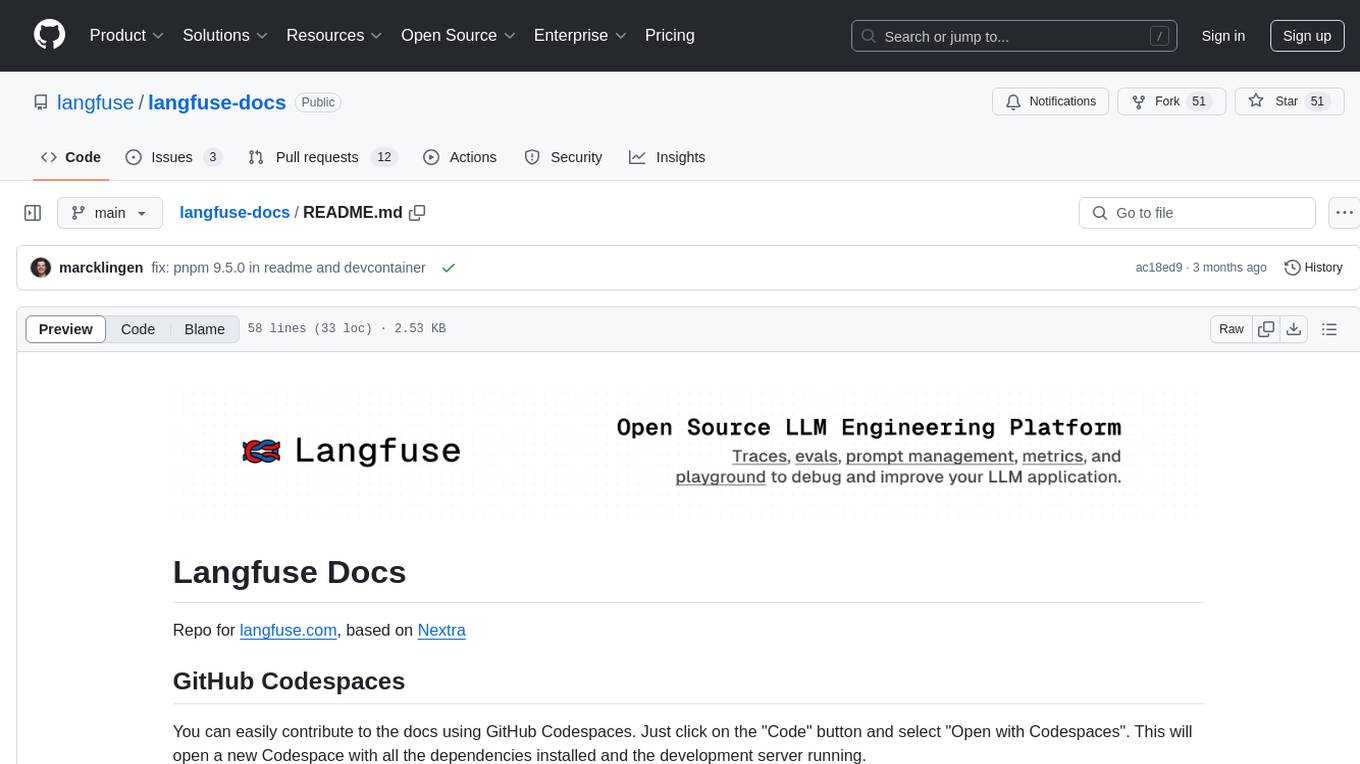
langfuse-docs
Langfuse Docs is a repository for langfuse.com, built on Nextra. It provides guidelines for contributing to the documentation using GitHub Codespaces and local development setup. The repository includes Python cookbooks in Jupyter notebooks format, which are converted to markdown for rendering on the site. It also covers media management for images, videos, and gifs. The stack includes Nextra, Next.js, shadcn/ui, and Tailwind CSS. Additionally, there is a bundle analysis feature to analyze the production build bundle size using @next/bundle-analyzer.
For similar tasks
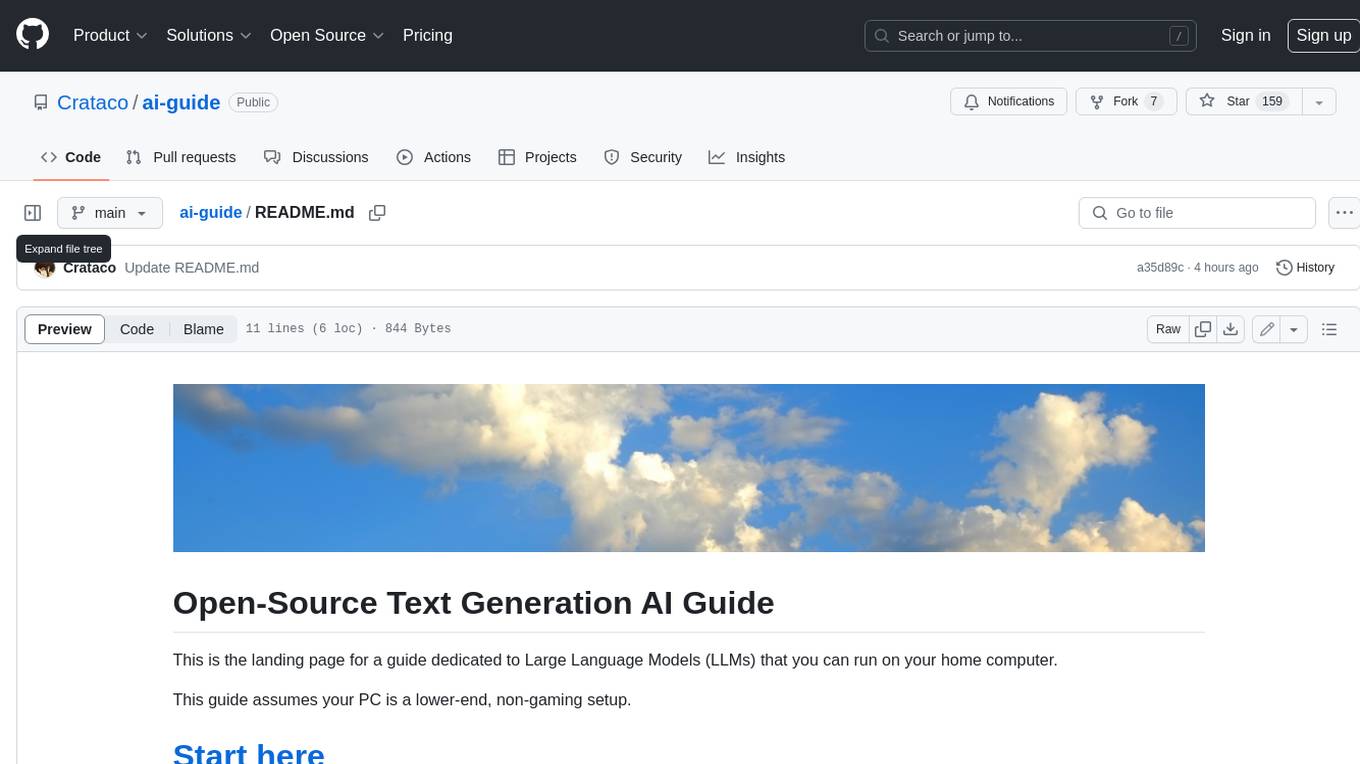
ai-guide
This guide is dedicated to Large Language Models (LLMs) that you can run on your home computer. It assumes your PC is a lower-end, non-gaming setup.
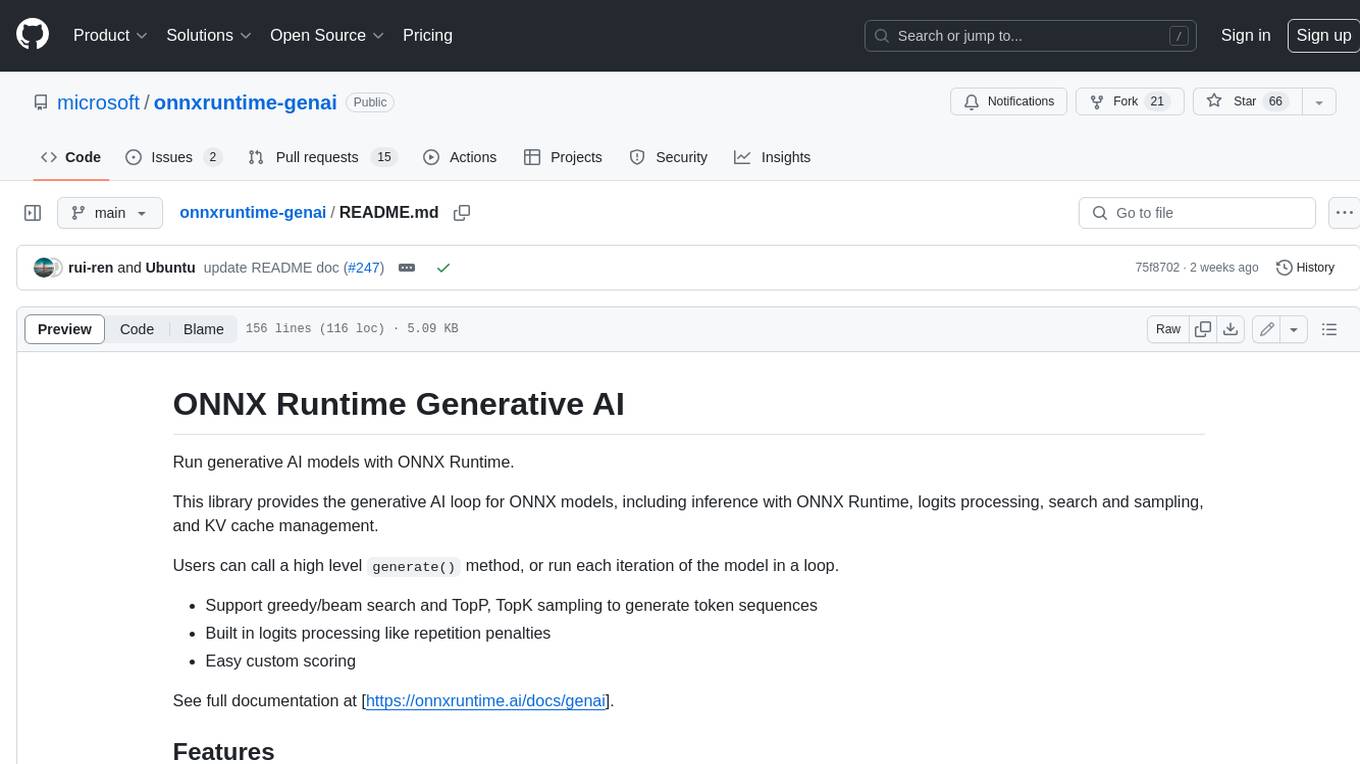
onnxruntime-genai
ONNX Runtime Generative AI is a library that provides the generative AI loop for ONNX models, including inference with ONNX Runtime, logits processing, search and sampling, and KV cache management. Users can call a high level `generate()` method, or run each iteration of the model in a loop. It supports greedy/beam search and TopP, TopK sampling to generate token sequences, has built in logits processing like repetition penalties, and allows for easy custom scoring.
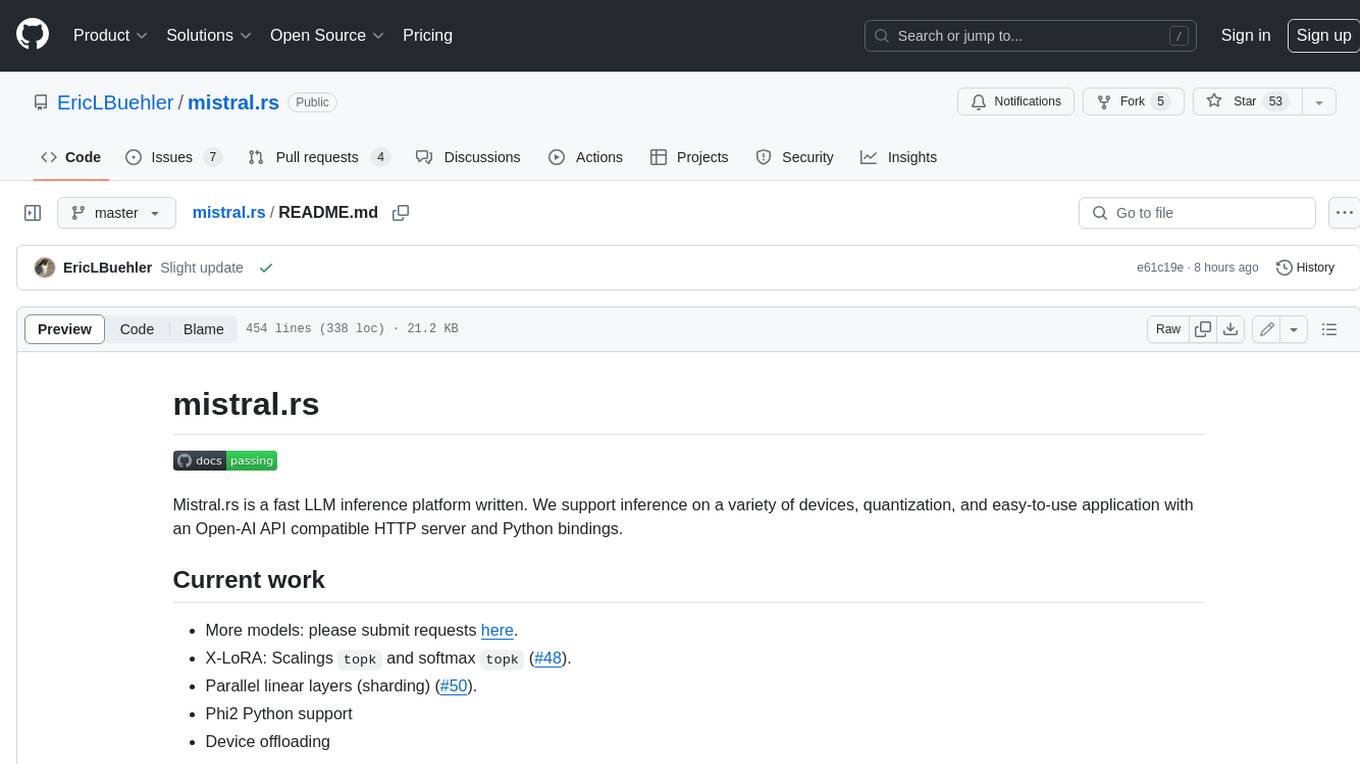
mistral.rs
Mistral.rs is a fast LLM inference platform written in Rust. We support inference on a variety of devices, quantization, and easy-to-use application with an Open-AI API compatible HTTP server and Python bindings.
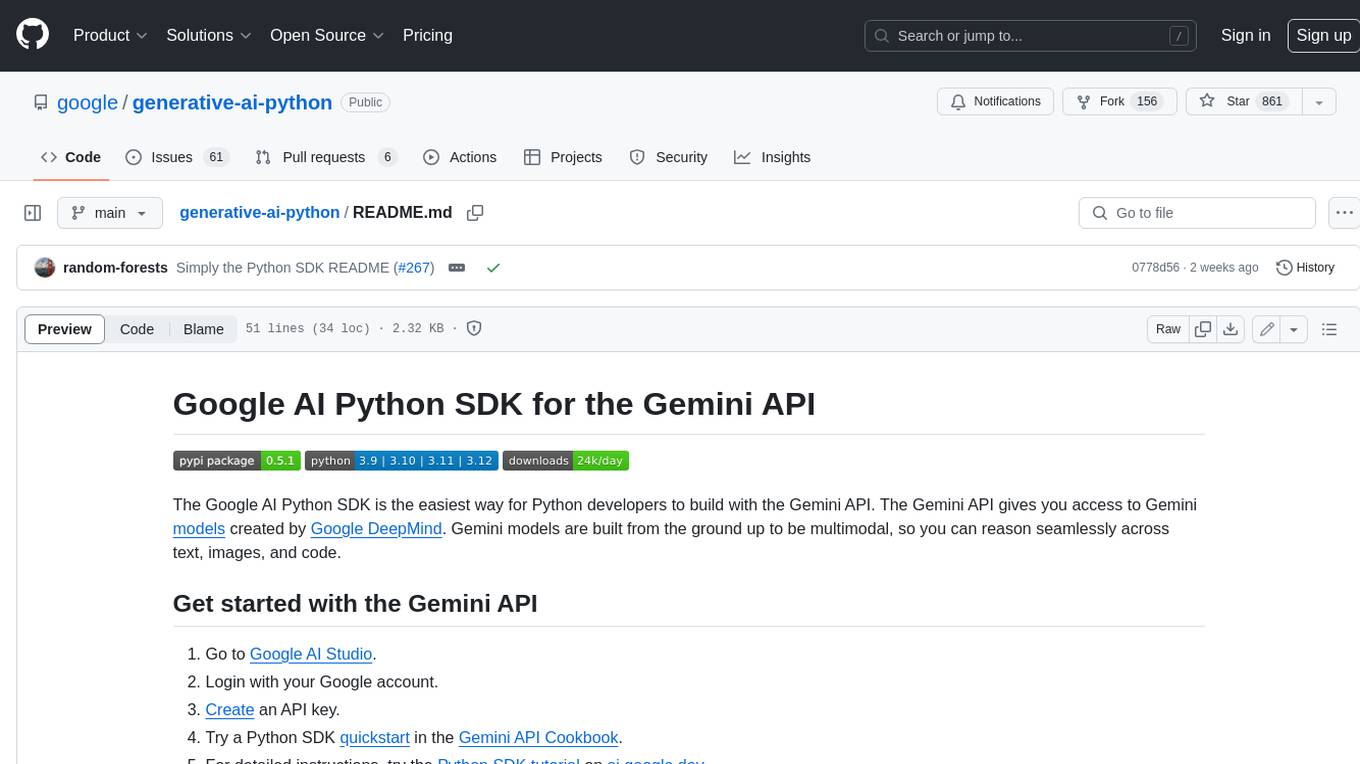
generative-ai-python
The Google AI Python SDK is the easiest way for Python developers to build with the Gemini API. The Gemini API gives you access to Gemini models created by Google DeepMind. Gemini models are built from the ground up to be multimodal, so you can reason seamlessly across text, images, and code.
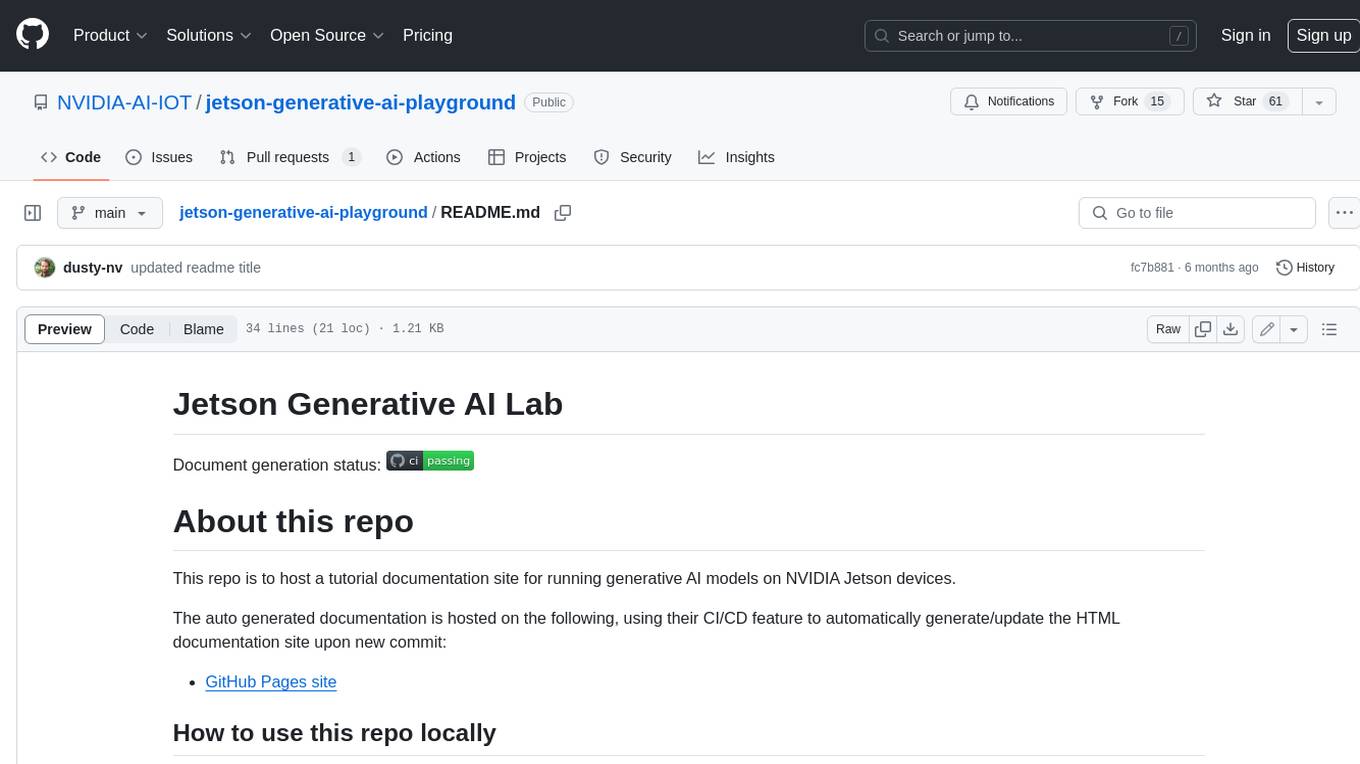
jetson-generative-ai-playground
This repo hosts tutorial documentation for running generative AI models on NVIDIA Jetson devices. The documentation is auto-generated and hosted on GitHub Pages using their CI/CD feature to automatically generate/update the HTML documentation site upon new commits.
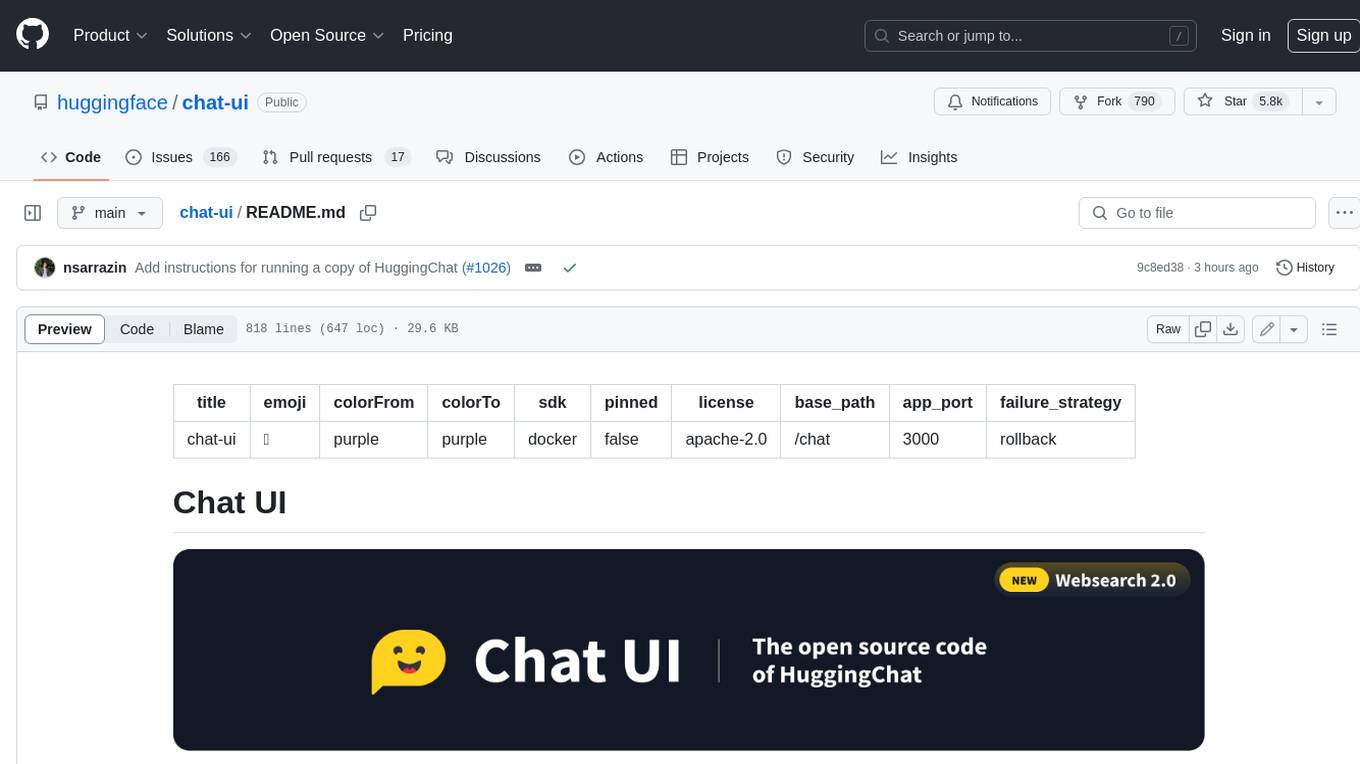
chat-ui
A chat interface using open source models, eg OpenAssistant or Llama. It is a SvelteKit app and it powers the HuggingChat app on hf.co/chat.
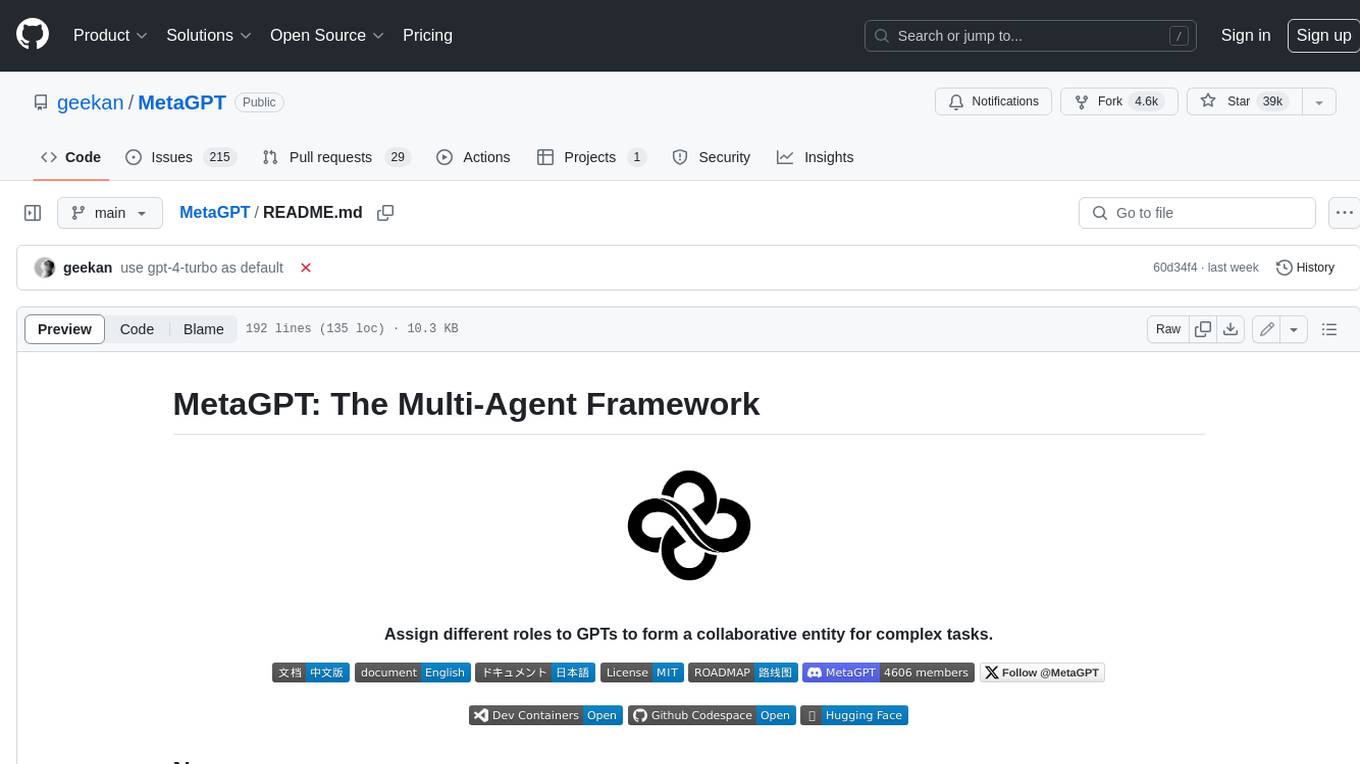
MetaGPT
MetaGPT is a multi-agent framework that enables GPT to work in a software company, collaborating to tackle more complex tasks. It assigns different roles to GPTs to form a collaborative entity for complex tasks. MetaGPT takes a one-line requirement as input and outputs user stories, competitive analysis, requirements, data structures, APIs, documents, etc. Internally, MetaGPT includes product managers, architects, project managers, and engineers. It provides the entire process of a software company along with carefully orchestrated SOPs. MetaGPT's core philosophy is "Code = SOP(Team)", materializing SOP and applying it to teams composed of LLMs.
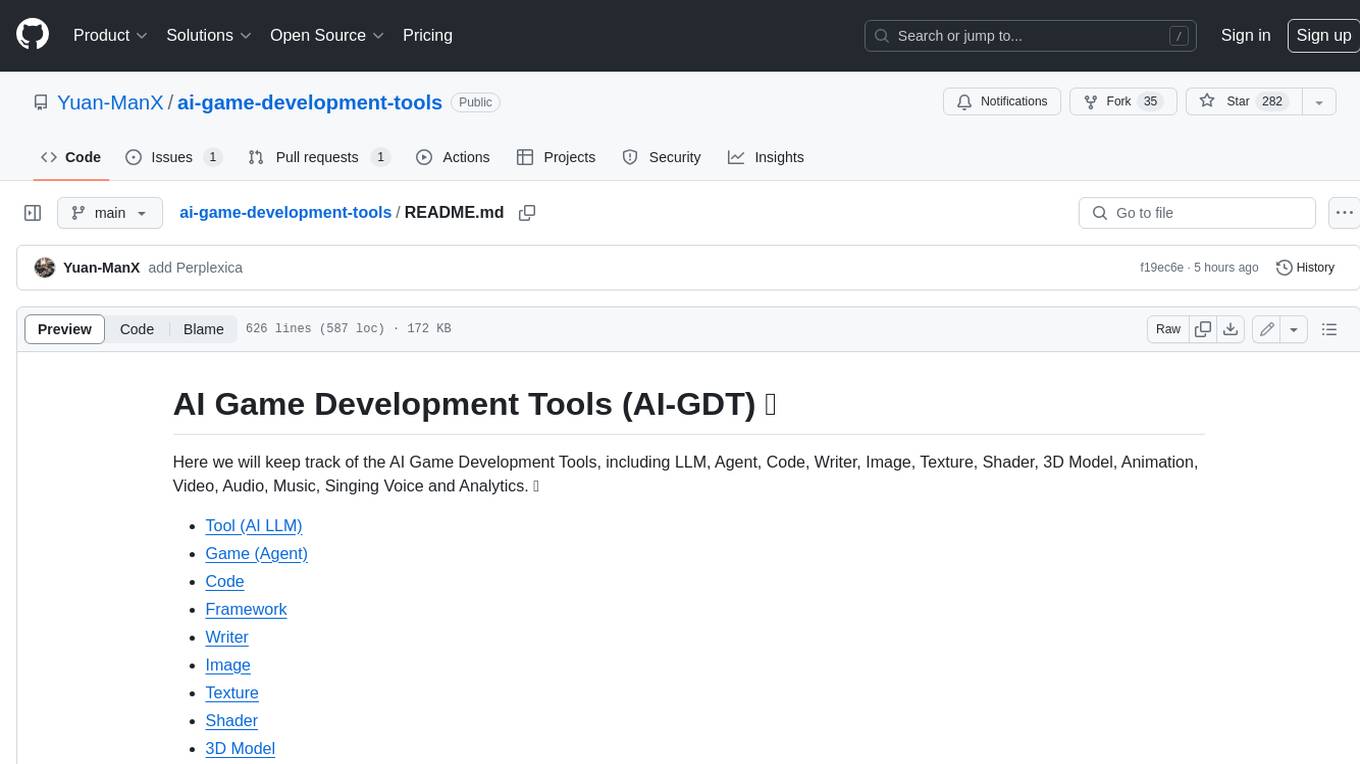
ai-game-development-tools
Here we will keep track of the AI Game Development Tools, including LLM, Agent, Code, Writer, Image, Texture, Shader, 3D Model, Animation, Video, Audio, Music, Singing Voice and Analytics. 🔥 * Tool (AI LLM) * Game (Agent) * Code * Framework * Writer * Image * Texture * Shader * 3D Model * Avatar * Animation * Video * Audio * Music * Singing Voice * Speech * Analytics * Video Tool
For similar jobs
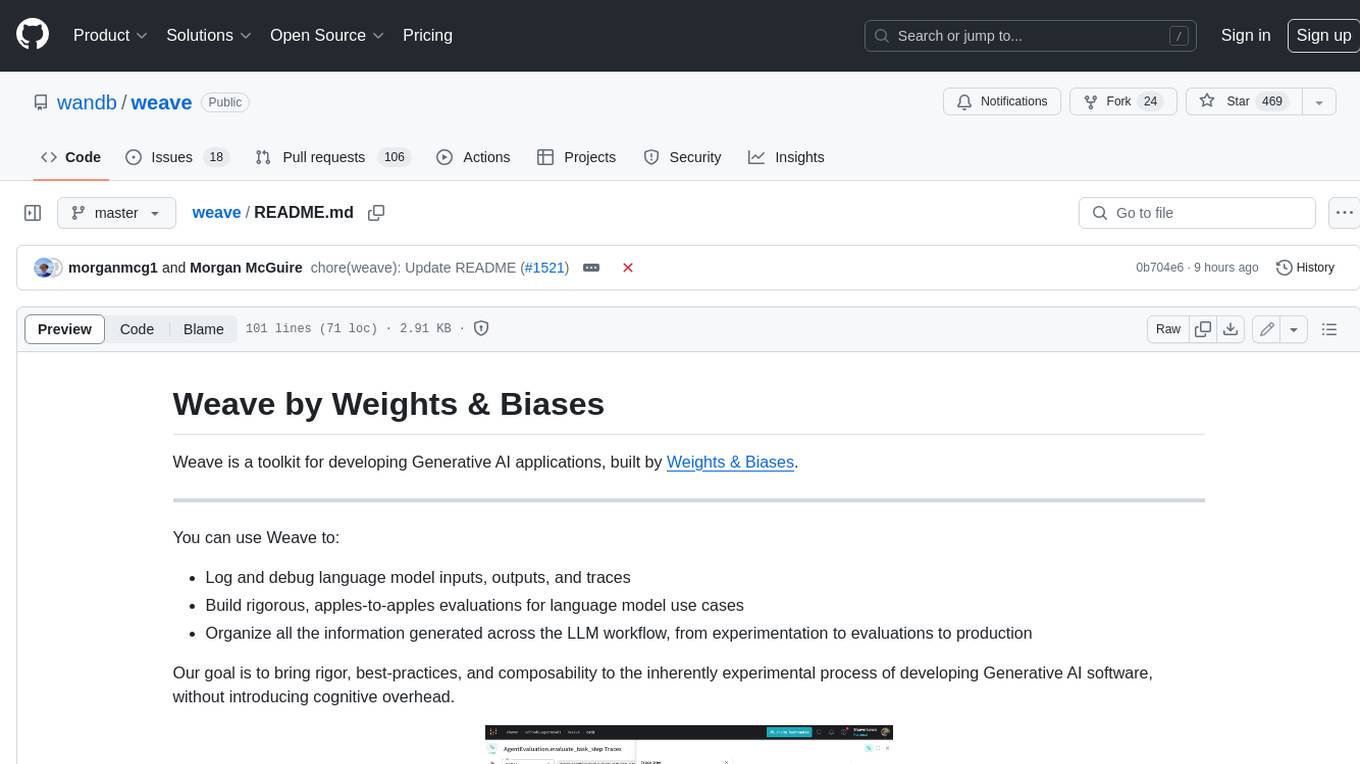
weave
Weave is a toolkit for developing Generative AI applications, built by Weights & Biases. With Weave, you can log and debug language model inputs, outputs, and traces; build rigorous, apples-to-apples evaluations for language model use cases; and organize all the information generated across the LLM workflow, from experimentation to evaluations to production. Weave aims to bring rigor, best-practices, and composability to the inherently experimental process of developing Generative AI software, without introducing cognitive overhead.
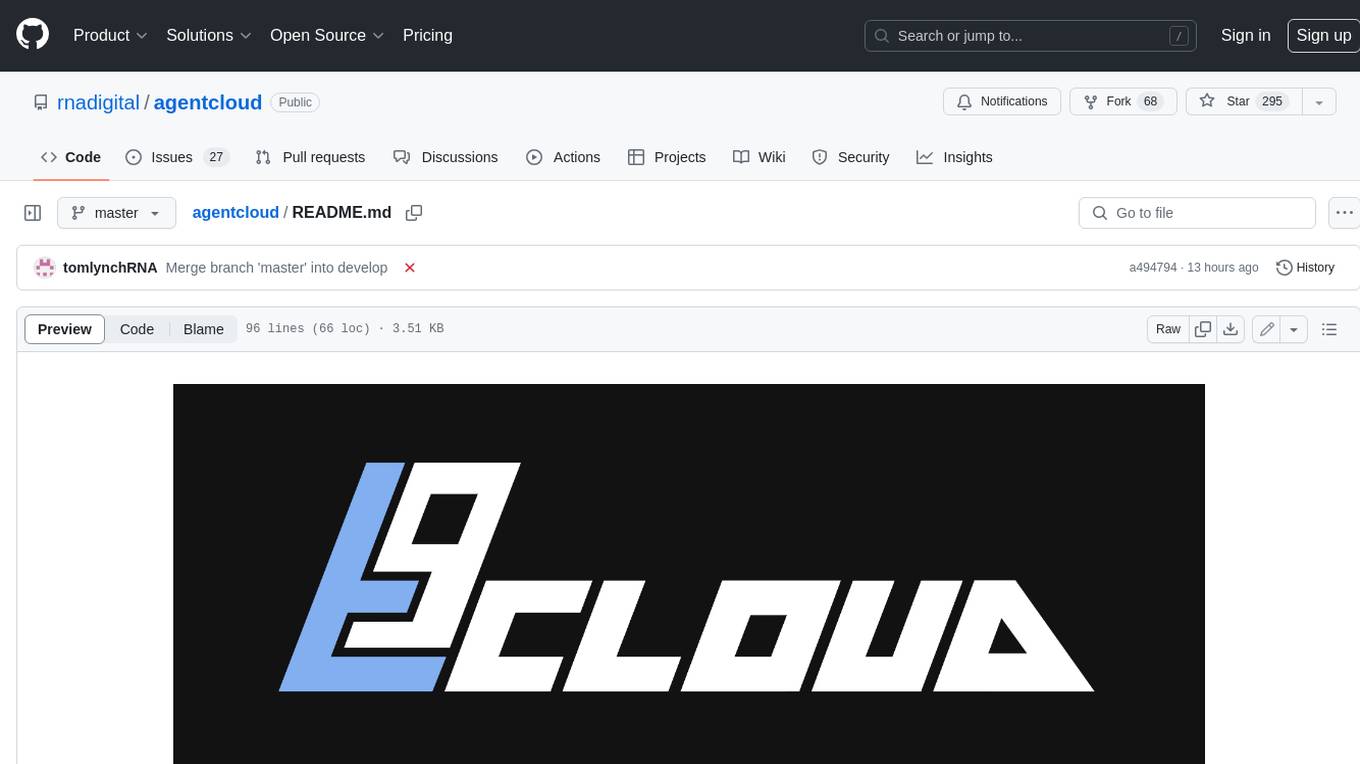
agentcloud
AgentCloud is an open-source platform that enables companies to build and deploy private LLM chat apps, empowering teams to securely interact with their data. It comprises three main components: Agent Backend, Webapp, and Vector Proxy. To run this project locally, clone the repository, install Docker, and start the services. The project is licensed under the GNU Affero General Public License, version 3 only. Contributions and feedback are welcome from the community.
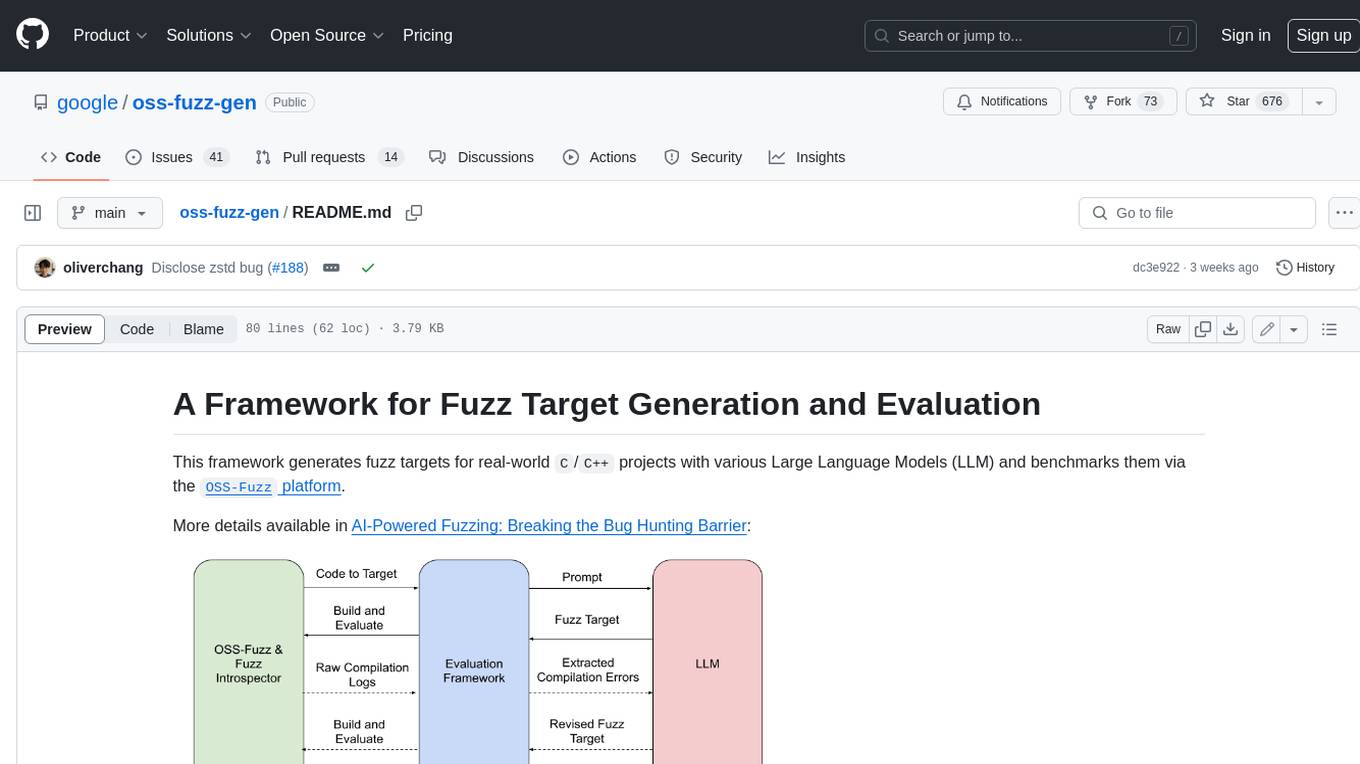
oss-fuzz-gen
This framework generates fuzz targets for real-world `C`/`C++` projects with various Large Language Models (LLM) and benchmarks them via the `OSS-Fuzz` platform. It manages to successfully leverage LLMs to generate valid fuzz targets (which generate non-zero coverage increase) for 160 C/C++ projects. The maximum line coverage increase is 29% from the existing human-written targets.
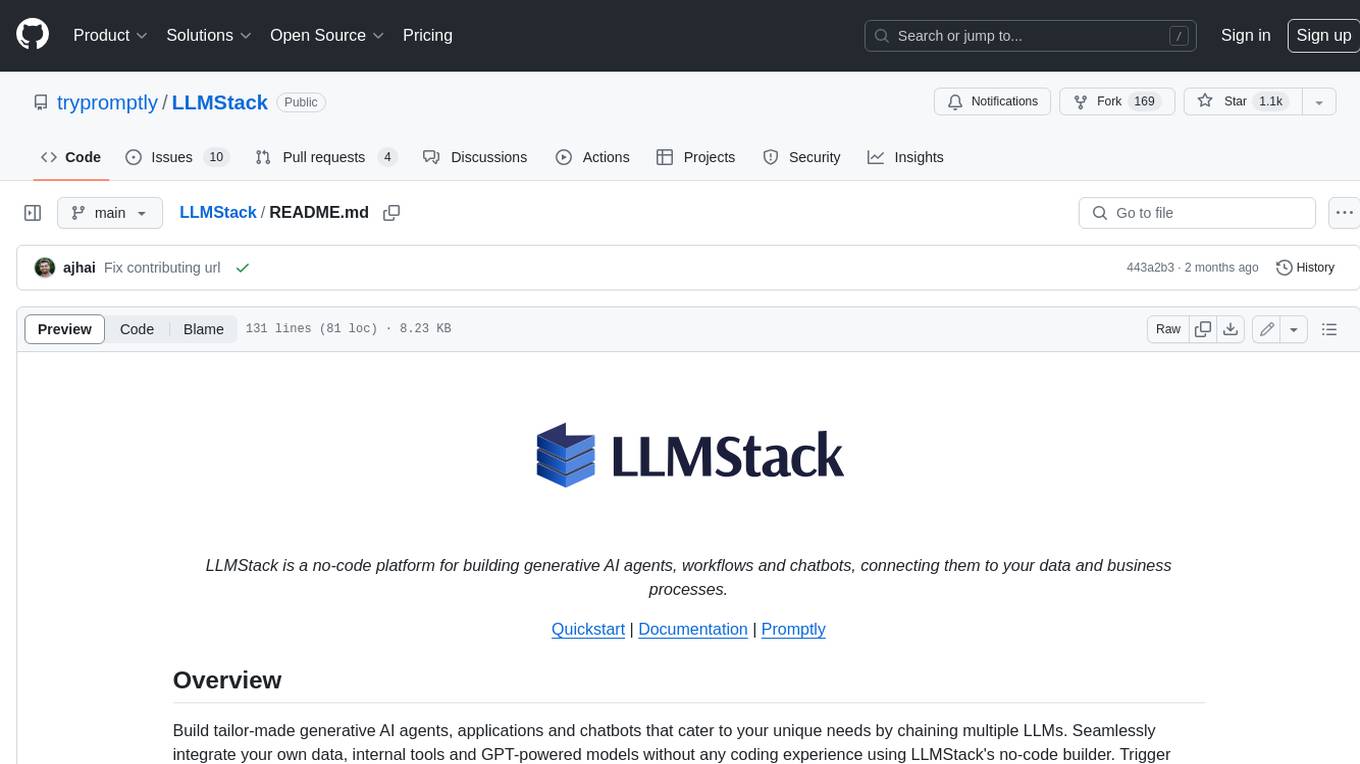
LLMStack
LLMStack is a no-code platform for building generative AI agents, workflows, and chatbots. It allows users to connect their own data, internal tools, and GPT-powered models without any coding experience. LLMStack can be deployed to the cloud or on-premise and can be accessed via HTTP API or triggered from Slack or Discord.
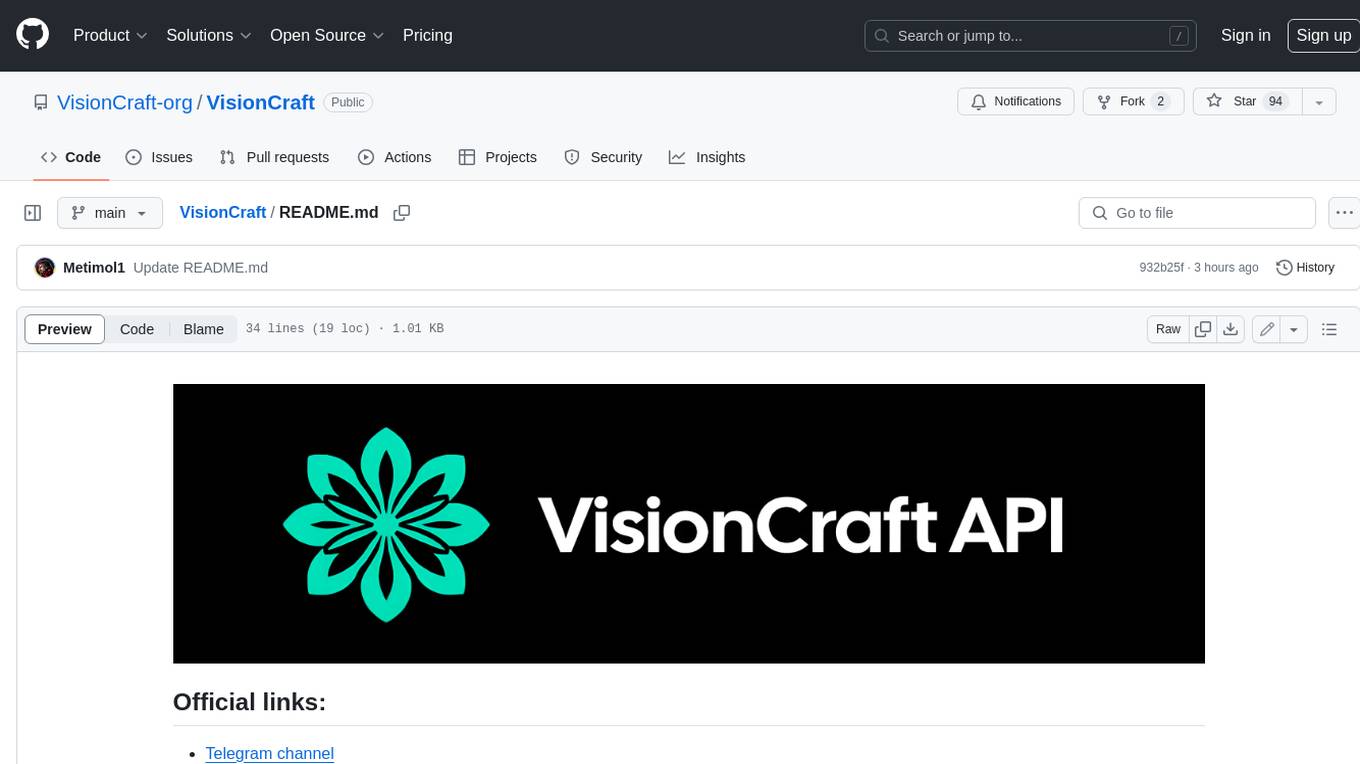
VisionCraft
The VisionCraft API is a free API for using over 100 different AI models. From images to sound.
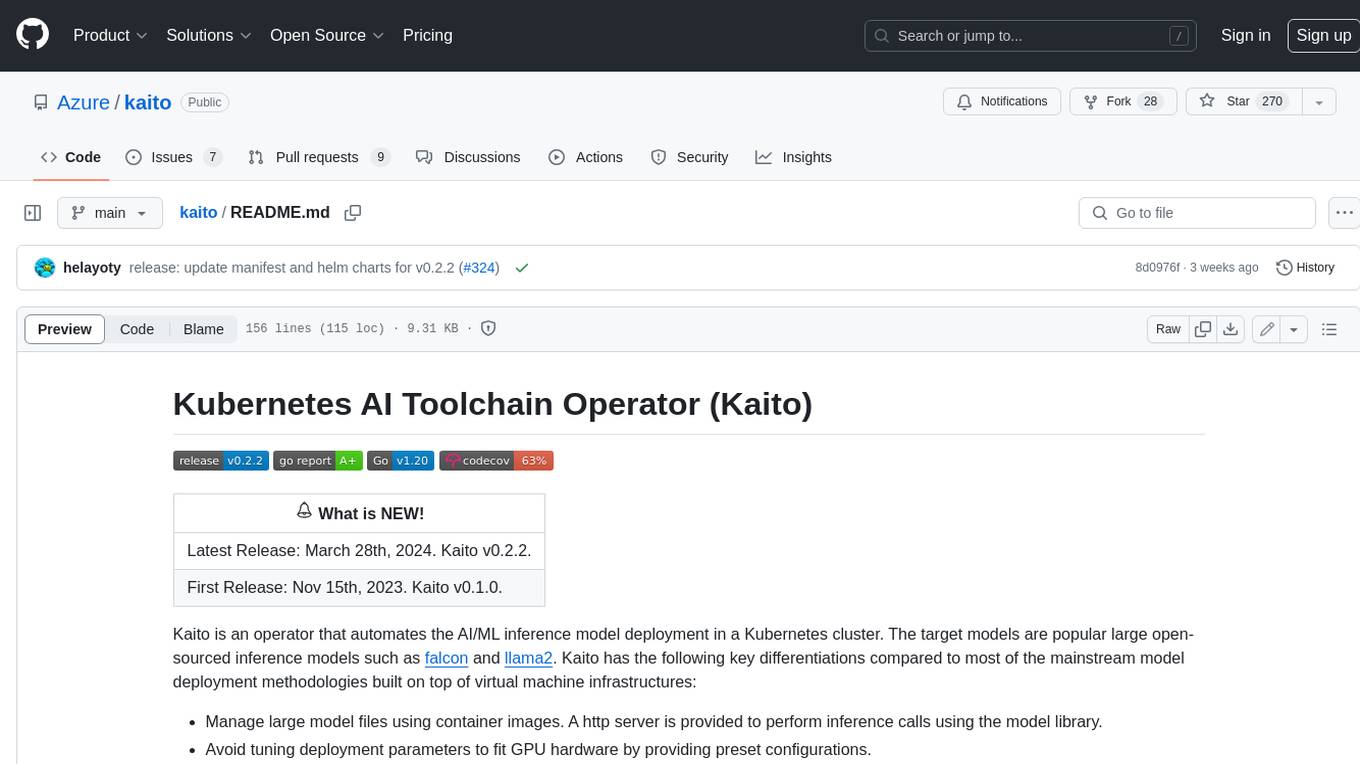
kaito
Kaito is an operator that automates the AI/ML inference model deployment in a Kubernetes cluster. It manages large model files using container images, avoids tuning deployment parameters to fit GPU hardware by providing preset configurations, auto-provisions GPU nodes based on model requirements, and hosts large model images in the public Microsoft Container Registry (MCR) if the license allows. Using Kaito, the workflow of onboarding large AI inference models in Kubernetes is largely simplified.
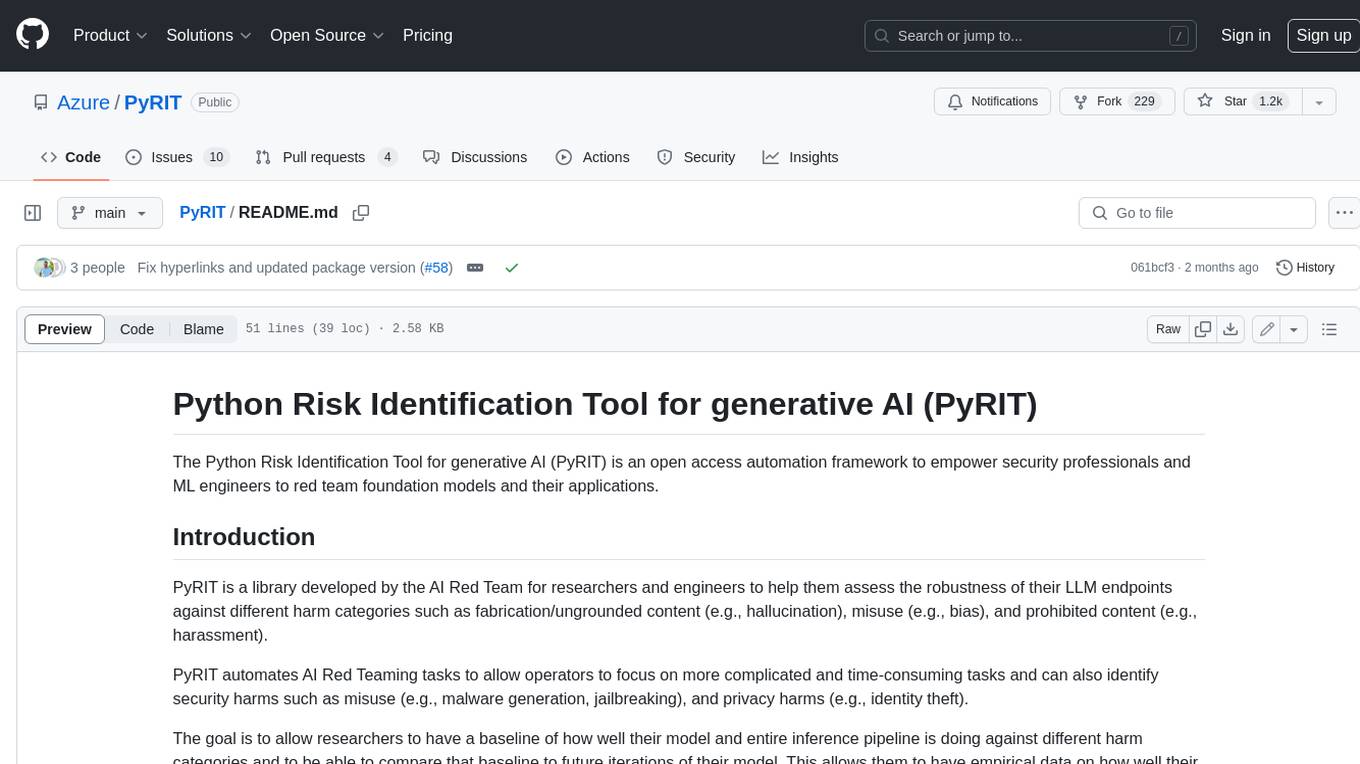
PyRIT
PyRIT is an open access automation framework designed to empower security professionals and ML engineers to red team foundation models and their applications. It automates AI Red Teaming tasks to allow operators to focus on more complicated and time-consuming tasks and can also identify security harms such as misuse (e.g., malware generation, jailbreaking), and privacy harms (e.g., identity theft). The goal is to allow researchers to have a baseline of how well their model and entire inference pipeline is doing against different harm categories and to be able to compare that baseline to future iterations of their model. This allows them to have empirical data on how well their model is doing today, and detect any degradation of performance based on future improvements.
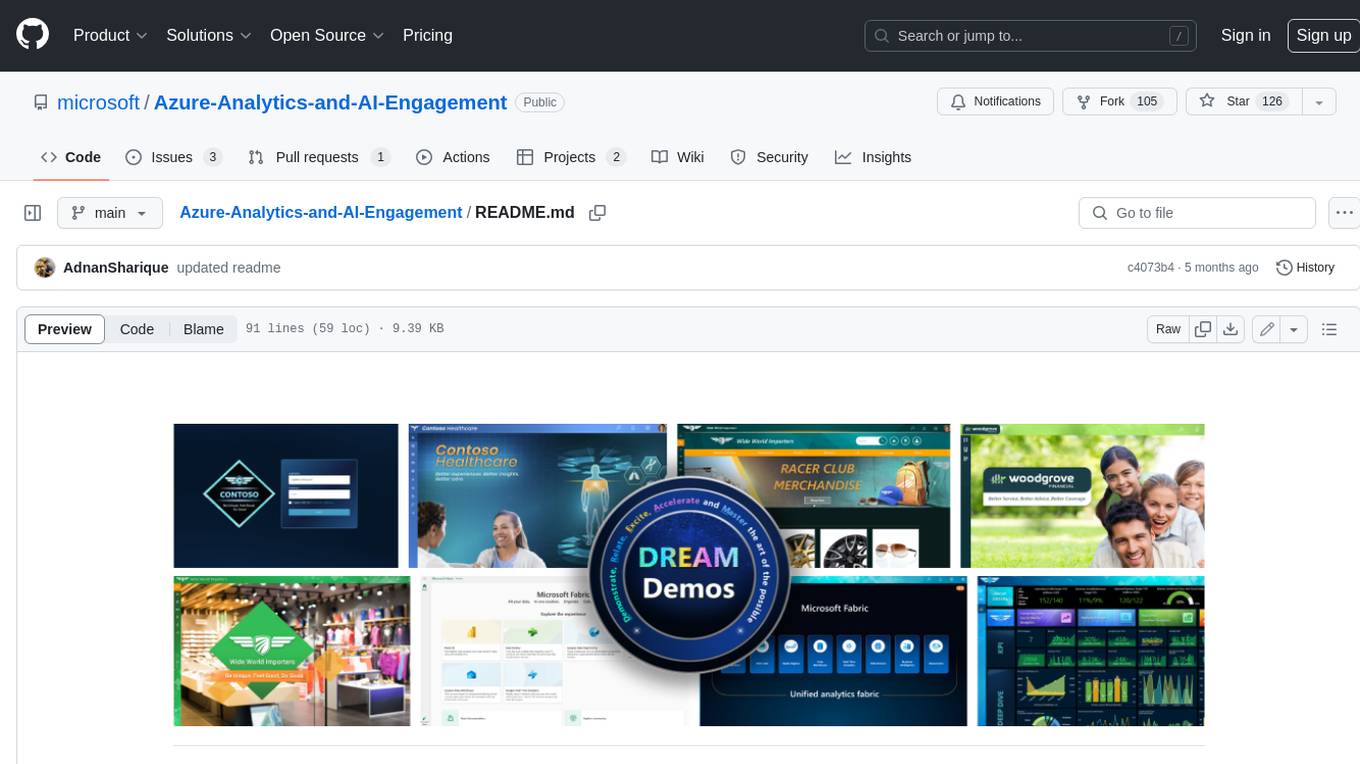
Azure-Analytics-and-AI-Engagement
The Azure-Analytics-and-AI-Engagement repository provides packaged Industry Scenario DREAM Demos with ARM templates (Containing a demo web application, Power BI reports, Synapse resources, AML Notebooks etc.) that can be deployed in a customer’s subscription using the CAPE tool within a matter of few hours. Partners can also deploy DREAM Demos in their own subscriptions using DPoC.