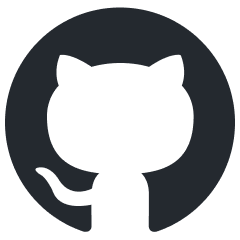
llama_ros
llama.cpp (GGUF LLMs) and llava.cpp (GGUF VLMs) for ROS 2
Stars: 195
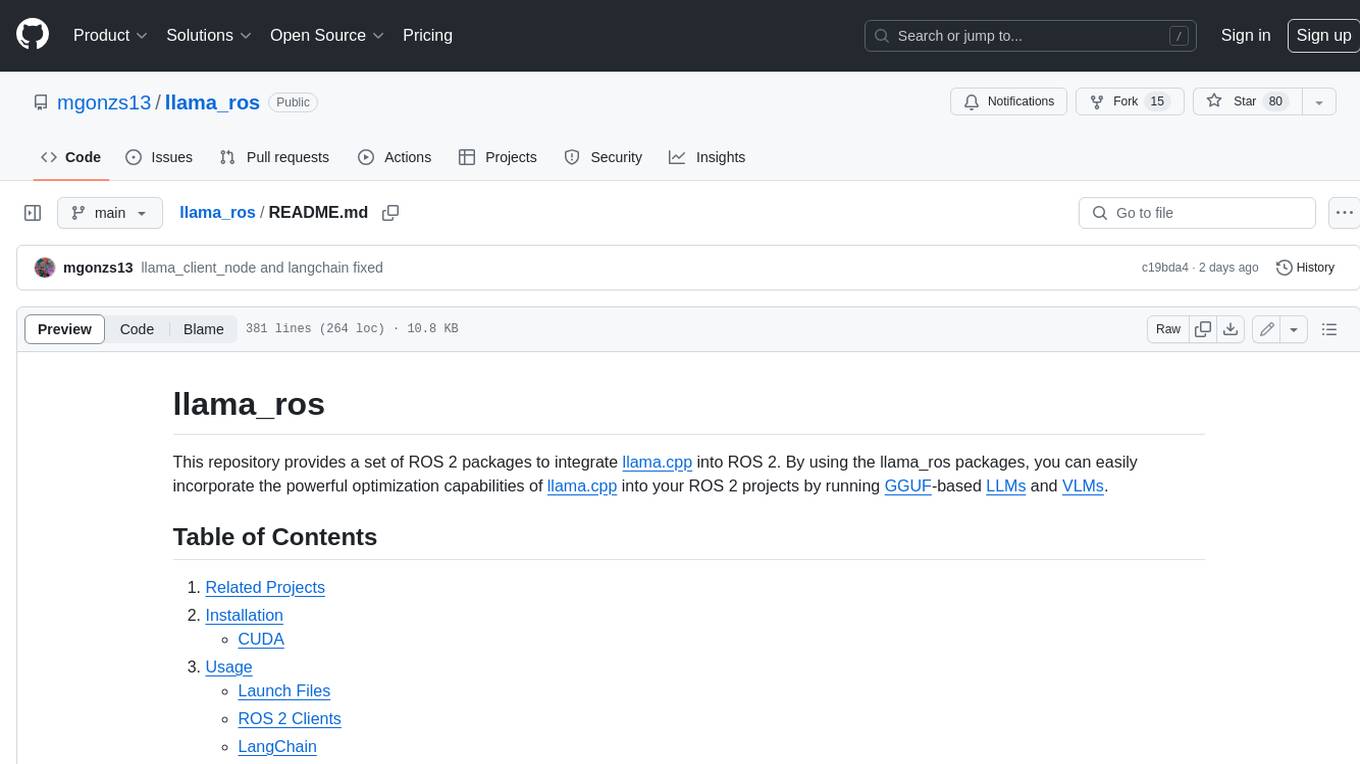
This repository provides a set of ROS 2 packages to integrate llama.cpp into ROS 2. By using the llama_ros packages, you can easily incorporate the powerful optimization capabilities of llama.cpp into your ROS 2 projects by running GGUF-based LLMs and VLMs.
README:
This repository provides a set of ROS 2 packages to integrate llama.cpp into ROS 2. Using the llama_ros packages, you can easily incorporate the powerful optimization capabilities of llama.cpp into your ROS 2 projects by running GGUF-based LLMs and VLMs. You can also use features from llama.cpp such as GBNF grammars and modify LoRAs in real-time.
- chatbot_ros → This chatbot, integrated into ROS 2, uses whisper_ros, to listen to people speech; and llama_ros, to generate responses. The chatbot is controlled by a state machine created with YASMIN.
- explainable_ros → A ROS 2 tool to explain the behavior of a robot. Using the integration of LangChain, logs are stored in a vector database. Then, RAG is applied to retrieve relevant logs for user questions answered with llama_ros.
To run llama_ros with CUDA, first, you must install the CUDA Toolkit. Then, you can compile llama_ros with --cmake-args -DGGML_CUDA=ON
to enable CUDA support.
cd ~/ros2_ws/src
git clone https://github.com/mgonzs13/llama_ros.git
pip3 install -r llama_ros/requirements.txt
cd ~/ros2_ws
rosdep install --from-paths src --ignore-src -r -y
colcon build --cmake-args -DGGML_CUDA=ON # add this for CUDA
Build the llama_ros docker or download an image from DockerHub. You can choose to build llama_ros with CUDA (USE_CUDA
) and choose the CUDA version (CUDA_VERSION
). Remember that you have to use DOCKER_BUILDKIT=0
to compile llama_ros with CUDA when building the image.
DOCKER_BUILDKIT=0 docker build -t llama_ros --build-arg USE_CUDA=1 --build-arg CUDA_VERSION=12-6 .
Run the docker container. If you want to use CUDA, you have to install the NVIDIA Container Tollkit and add --gpus all
.
docker run -it --rm --gpus all llama_ros
Commands are included in llama_ros to speed up the test of GGUF-based LLMs within the ROS 2 ecosystem. This way, the following commands are integrating into the ROS 2 commands:
Using this command launch a LLM from a YAML file. The configuration of the YAML is used to launch the LLM in the same way as using a regular launch file. Here is an example of how to use it:
ros2 llama launch ~/ros2_ws/src/llama_ros/llama_bringup/models/StableLM-Zephyr.yaml
Using this command send a prompt to a launched LLM. The command uses a string, which is the prompt and has the following arguments:
- (
-r
,--reset
): Whether to reset the LLM before prompting - (
-t
,--temp
): The temperature value - (
--image-url
): Image url to sent to a VLM
Here is an example of how to use it:
ros2 llama prompt "Do you know ROS 2?" -t 0.0
First of all, you need to create a launch file to use llama_ros or llava_ros. This launch file will contain the main parameters to download the model from HuggingFace and configure it. Take a look at the following examples and the predefined launch files.
Click to expand
from launch import LaunchDescription
from llama_bringup.utils import create_llama_launch
def generate_launch_description():
return LaunchDescription([
create_llama_launch(
n_ctx=2048, # context of the LLM in tokens
n_batch=8, # batch size in tokens
n_gpu_layers=0, # layers to load in GPU
n_threads=1, # threads
n_predict=2048, # max tokens, -1 == inf
model_repo="TheBloke/Marcoroni-7B-v3-GGUF", # Hugging Face repo
model_filename="marcoroni-7b-v3.Q4_K_M.gguf", # model file in repo
system_prompt_type="Alpaca" # system prompt type
)
])
ros2 launch llama_bringup marcoroni.launch.py
Click to expand
n_ctx: 2048 # context of the LLM in tokens
n_batch: 8 # batch size in tokens
n_gpu_layers: 0 # layers to load in GPU
n_threads: 1 # threads
n_predict: 2048 # max tokens, -1 == inf
model_repo: "cstr/Spaetzle-v60-7b-GGUF" # Hugging Face repo
model_filename: "Spaetzle-v60-7b-q4-k-m.gguf" # model file in repo
system_prompt_type: "Alpaca" # system prompt type
import os
from launch import LaunchDescription
from llama_bringup.utils import create_llama_launch_from_yaml
from ament_index_python.packages import get_package_share_directory
def generate_launch_description():
return LaunchDescription([
create_llama_launch_from_yaml(os.path.join(
get_package_share_directory("llama_bringup"), "models", "Spaetzle.yaml"))
])
ros2 launch llama_bringup spaetzle.launch.py
Click to expand
n_ctx: 2048 # context of the LLM in tokens
n_batch: 8 # batch size in tokens
n_gpu_layers: 0 # layers to load in GPU
n_threads: 1 # threads
n_predict: 2048 # max tokens, -1 == inf
model_repo: "Qwen/Qwen2.5-Coder-7B-Instruct-GGUF" # Hugging Face repo
model_filename: "qwen2.5-coder-7b-instruct-q4_k_m-00001-of-00002.gguf" # model shard file in repo
system_prompt_type: "ChatML" # system prompt type
ros2 llama launch Qwen2.yaml
Click to expand
from launch import LaunchDescription
from llama_bringup.utils import create_llama_launch
def generate_launch_description():
return LaunchDescription([
create_llama_launch(
use_llava=True, # enable llava
n_ctx=8192, # context of the LLM in tokens, use a huge context size to load images
n_batch=512, # batch size in tokens
n_gpu_layers=33, # layers to load in GPU
n_threads=1, # threads
n_predict=8192, # max tokens, -1 == inf
model_repo="cjpais/llava-1.6-mistral-7b-gguf", # Hugging Face repo
model_filename="llava-v1.6-mistral-7b.Q4_K_M.gguf", # model file in repo
mmproj_repo="cjpais/llava-1.6-mistral-7b-gguf", # Hugging Face repo
mmproj_filename="mmproj-model-f16.gguf", # mmproj file in repo
system_prompt_type="Mistral" # system prompt type
)
])
ros2 launch llama_bringup llava.launch.py
Click to expand
use_llava: True # enable llava
n_ctx: 8192 # context of the LLM in tokens use a huge context size to load images
n_batch: 512 # batch size in tokens
n_gpu_layers: 33 # layers to load in GPU
n_threads: 1 # threads
n_predict: 8192 # max tokens -1 : : inf
model_repo: "cjpais/llava-1.6-mistral-7b-gguf" # Hugging Face repo
model_filename: "llava-v1.6-mistral-7b.Q4_K_M.gguf" # model file in repo
mmproj_repo: "cjpais/llava-1.6-mistral-7b-gguf" # Hugging Face repo
mmproj_filename: "mmproj-model-f16.gguf" # mmproj file in repo
system_prompt_type: "mistral" # system prompt type
def generate_launch_description():
return LaunchDescription([
create_llama_launch_from_yaml(os.path.join(
get_package_share_directory("llama_bringup"),
"models", "llava-1.6-mistral-7b-gguf.yaml"))
])
ros2 launch llama_bringup llava.launch.py
You can use LoRA adapters when launching LLMs. Using llama.cpp features, you can load multiple adapters choosing the scale to apply for each adapter. Here you have an example of using LoRA adapters with Phi-3. You can lis the
LoRAs using the /llama/list_loras
service and modify their scales values by using the /llama/update_loras
service. A scale value of 0.0 means not using that LoRA.
Click to expand
n_ctx: 2048
n_batch: 8
n_gpu_layers: 0
n_threads: 1
n_predict: 2048
model_repo: "bartowski/Phi-3.5-mini-instruct-GGUF"
model_filename: "Phi-3.5-mini-instruct-Q4_K_M.gguf"
lora_adapters:
- repo: "zhhan/adapter-Phi-3-mini-4k-instruct_code_writing"
filename: "Phi-3-mini-4k-instruct-adaptor-f16-code_writer.gguf"
scale: 0.5
- repo: "zhhan/adapter-Phi-3-mini-4k-instruct_summarization"
filename: "Phi-3-mini-4k-instruct-adaptor-f16-summarization.gguf"
scale: 0.5
system_prompt_type: "Phi-3"
Both llama_ros and llava_ros provide ROS 2 interfaces to access the main functionalities of the models. Here you have some examples of how to use them inside ROS 2 nodes. Moreover, take a look to the llama_demo_node.py and llava_demo_node.py demos.
Click to expand
from rclpy.node import Node
from llama_msgs.srv import Tokenize
class ExampleNode(Node):
def __init__(self) -> None:
super().__init__("example_node")
# create the client
self.srv_client = self.create_client(Tokenize, "/llama/tokenize")
# create the request
req = Tokenize.Request()
req.text = "Example text"
# call the tokenize service
self.srv_client.wait_for_service()
tokens = self.srv_client.call(req).tokens
Click to expand
from rclpy.node import Node
from llama_msgs.srv import Detokenize
class ExampleNode(Node):
def __init__(self) -> None:
super().__init__("example_node")
# create the client
self.srv_client = self.create_client(Detokenize, "/llama/detokenize")
# create the request
req = Detokenize.Request()
req.tokens = [123, 123]
# call the tokenize service
self.srv_client.wait_for_service()
text = self.srv_client.call(req).text
Click to expand
Remember to launch llama_ros with embedding set to true to be able of generating embeddings with your LLM.
from rclpy.node import Node
from llama_msgs.srv import Embeddings
class ExampleNode(Node):
def __init__(self) -> None:
super().__init__("example_node")
# create the client
self.srv_client = self.create_client(Embeddings, "/llama/generate_embeddings")
# create the request
req = Embeddings.Request()
req.prompt = "Example text"
req.normalize = True
# call the embedding service
self.srv_client.wait_for_service()
embeddings = self.srv_client.call(req).embeddings
Click to expand
import rclpy
from rclpy.node import Node
from rclpy.action import ActionClient
from llama_msgs.action import GenerateResponse
class ExampleNode(Node):
def __init__(self) -> None:
super().__init__("example_node")
# create the client
self.action_client = ActionClient(
self, GenerateResponse, "/llama/generate_response")
# create the goal and set the sampling config
goal = GenerateResponse.Goal()
goal.prompt = self.prompt
goal.sampling_config.temp = 0.2
# wait for the server and send the goal
self.action_client.wait_for_server()
send_goal_future = self.action_client.send_goal_async(
goal)
# wait for the server
rclpy.spin_until_future_complete(self, send_goal_future)
get_result_future = send_goal_future.result().get_result_async()
# wait again and take the result
rclpy.spin_until_future_complete(self, get_result_future)
result: GenerateResponse.Result = get_result_future.result().result
Click to expand
import cv2
from cv_bridge import CvBridge
import rclpy
from rclpy.node import Node
from rclpy.action import ActionClient
from llama_msgs.action import GenerateResponse
class ExampleNode(Node):
def __init__(self) -> None:
super().__init__("example_node")
# create a cv bridge for the image
self.cv_bridge = CvBridge()
# create the client
self.action_client = ActionClient(
self, GenerateResponse, "/llama/generate_response")
# create the goal and set the sampling config
goal = GenerateResponse.Goal()
goal.prompt = self.prompt
goal.sampling_config.temp = 0.2
# add your image to the goal
image = cv2.imread("/path/to/your/image", cv2.IMREAD_COLOR)
goal.image = self.cv_bridge.cv2_to_imgmsg(image)
# wait for the server and send the goal
self.action_client.wait_for_server()
send_goal_future = self.action_client.send_goal_async(
goal)
# wait for the server
rclpy.spin_until_future_complete(self, send_goal_future)
get_result_future = send_goal_future.result().get_result_async()
# wait again and take the result
rclpy.spin_until_future_complete(self, get_result_future)
result: GenerateResponse.Result = get_result_future.result().result
There is a llama_ros integration for LangChain. Thus, prompt engineering techniques could be applied. Here you have an example to use it.
Click to expand
import rclpy
from llama_ros.langchain import LlamaROS
from langchain.prompts import PromptTemplate
from langchain_core.output_parsers import StrOutputParser
rclpy.init()
# create the llama_ros llm for langchain
llm = LlamaROS()
# create a prompt template
prompt_template = "tell me a joke about {topic}"
prompt = PromptTemplate(
input_variables=["topic"],
template=prompt_template
)
# create a chain with the llm and the prompt template
chain = prompt | llm | StrOutputParser()
# run the chain
text = chain.invoke({"topic": "bears"})
print(text)
rclpy.shutdown()
Click to expand
import rclpy
from llama_ros.langchain import LlamaROS
from langchain.prompts import PromptTemplate
from langchain_core.output_parsers import StrOutputParser
rclpy.init()
# create the llama_ros llm for langchain
llm = LlamaROS()
# create a prompt template
prompt_template = "tell me a joke about {topic}"
prompt = PromptTemplate(
input_variables=["topic"],
template=prompt_template
)
# create a chain with the llm and the prompt template
chain = prompt | llm | StrOutputParser()
# run the chain
for c in chain.stream({"topic": "bears"}):
print(c, flush=True, end="")
rclpy.shutdown()
Click to expand
import rclpy
from llama_ros.langchain import LlamaROS
rclpy.init()
# create the llama_ros llm for langchain
llm = LlamaROS()
# bind the url_image
image_url = "https://upload.wikimedia.org/wikipedia/commons/thumb/d/dd/Gfp-wisconsin-madison-the-nature-boardwalk.jpg/2560px-Gfp-wisconsin-madison-the-nature-boardwalk.jpg"
llm = llm.bind(image_url=image_url).stream("Describe the image")
# run the llm
for c in llm:
print(c, flush=True, end="")
rclpy.shutdown()
Click to expand
import rclpy
from langchain_chroma import Chroma
from llama_ros.langchain import LlamaROSEmbeddings
rclpy.init()
# create the llama_ros embeddings for langchain
embeddings = LlamaROSEmbeddings()
# create a vector database and assign it
db = Chroma(embedding_function=embeddings)
# create the retriever
retriever = db.as_retriever(search_kwargs={"k": 5})
# add your texts
db.add_texts(texts=["your_texts"])
# retrieve documents
documents = retriever.invoke("your_query")
print(documents)
rclpy.shutdown()
Click to expand
import rclpy
from llama_ros.langchain import LlamaROSReranker
from llama_ros.langchain import LlamaROSEmbeddings
from langchain_community.vectorstores import FAISS
from langchain_community.document_loaders import TextLoader
from langchain_text_splitters import RecursiveCharacterTextSplitter
from langchain.retrievers import ContextualCompressionRetriever
rclpy.init()
# load the documents
documents = TextLoader("../state_of_the_union.txt",).load()
text_splitter = RecursiveCharacterTextSplitter(
chunk_size=500, chunk_overlap=100)
texts = text_splitter.split_documents(documents)
# create the llama_ros embeddings
embeddings = LlamaROSEmbeddings()
# create the VD and the retriever
retriever = FAISS.from_documents(
texts, embeddings).as_retriever(search_kwargs={"k": 20})
# create the compressor using the llama_ros reranker
compressor = LlamaROSReranker()
compression_retriever = ContextualCompressionRetriever(
base_compressor=compressor, base_retriever=retriever
)
# retrieve the documents
compressed_docs = compression_retriever.invoke(
"What did the president say about Ketanji Jackson Brown"
)
for doc in compressed_docs:
print("-" * 50)
print(doc.page_content)
print("\n")
rclpy.shutdown()
Click to expand
import bs4
import rclpy
from langchain_chroma import Chroma
from langchain_community.document_loaders import WebBaseLoader
from langchain_core.output_parsers import StrOutputParser
from langchain_core.runnables import RunnablePassthrough
from langchain_core.messages import SystemMessage
from langchain_core.prompts import ChatPromptTemplate, HumanMessagePromptTemplate
from langchain_text_splitters import RecursiveCharacterTextSplitter
from langchain.retrievers import ContextualCompressionRetriever
from llama_ros.langchain import ChatLlamaROS, LlamaROSEmbeddings, LlamaROSReranker
rclpy.init()
# load, chunk and index the contents of the blog
loader = WebBaseLoader(
web_paths=("https://lilianweng.github.io/posts/2023-06-23-agent/",),
bs_kwargs=dict(
parse_only=bs4.SoupStrainer(class_=("post-content", "post-title", "post-header"))
),
)
docs = loader.load()
text_splitter = RecursiveCharacterTextSplitter(chunk_size=1000, chunk_overlap=200)
splits = text_splitter.split_documents(docs)
vectorstore = Chroma.from_documents(documents=splits, embedding=LlamaROSEmbeddings())
# retrieve and generate using the relevant snippets of the blog
retriever = vectorstore.as_retriever(search_kwargs={"k": 20})
# create prompt
prompt = ChatPromptTemplate.from_messages(
[
SystemMessage("You are an AI assistant that answer questions briefly."),
HumanMessagePromptTemplate.from_template(
"Taking into account the followin information:{context}\n\n{question}"
),
]
)
# create rerank compression retriever
compressor = LlamaROSReranker(top_n=3)
compression_retriever = ContextualCompressionRetriever(
base_compressor=compressor, base_retriever=retriever
)
def format_docs(docs):
formated_docs = ""
for d in docs:
formated_docs += f"\n\n\t- {d.page_content}"
return formated_docs
# create and use the chain
rag_chain = (
{"context": compression_retriever | format_docs, "question": RunnablePassthrough()}
| prompt
| ChatLlamaROS(temp=0.0)
| StrOutputParser()
)
for c in rag_chain.stream("What is Task Decomposition?"):
print(c, flush=True, end="")
rclpy.shutdown()
Click to expand
import rclpy
from llama_ros.langchain import ChatLlamaROS
from langchain_core.messages import SystemMessage
from langchain_core.prompts import ChatPromptTemplate, HumanMessagePromptTemplate
from langchain_core.output_parsers import StrOutputParser
rclpy.init()
# create chat
chat = ChatLlamaROS(
temp=0.2,
penalty_last_n=8
)
# create prompt template with messages
prompt = ChatPromptTemplate.from_messages([
SystemMessage("You are a IA that just answer with a single word."),
HumanMessagePromptTemplate.from_template(template=[
{"type": "text", "text": "<image>Who is the character in the middle of the image?"},
{"type": "image_url", "image_url": "{image_url}"}
])
])
# create the chain
chain = prompt | chat | StrOutputParser()
# stream and print the LLM output
for text in chain.stream({"image_url": "https://pics.filmaffinity.com/Dragon_Ball_Bola_de_Dragaon_Serie_de_TV-973171538-large.jpg"}):
print(text, end="", flush=True)
print("", end="\n", flush=True)
rclpy.shutdown()
Click to expand
import rclpy
from langchain_core.messages import HumanMessage
from llama_ros.langchain import ChatLlamaROS
from pydantic import BaseModel, Field
rclpy.init()
class Joke(BaseModel):
"""Joke to tell user."""
setup: str = Field(description="The setup of the joke")
punchline: str = Field(description="The punchline to the joke")
rating: Optional[int] = Field(
default=None, description="How funny the joke is, from 1 to 10"
)
chat = ChatLlamaROS(temp=0.6, penalty_last_n=8)
structured_chat = chat.with_structured_output(
Joke, method="function_calling"
)
prompt = ChatPromptTemplate.from_messages(
[
HumanMessagePromptTemplate.from_template(
template=[
{"type": "text", "text": "{prompt}"},
]
),
]
)
chain = prompt | structured_chat
res = chain.invoke({"prompt": "Tell me a joke about cats"})
print(f"Response: {response.content.strip()}")
rclpy.shutdown()
Click to expand
The current implementation of Tools allows executing tools without requiring a model trained for that task.
from random import randint
import rclpy
from langchain.tools import tool
from langchain_core.messages import HumanMessage
from llama_ros.langchain import ChatLlamaROS
rclpy.init()
@tool
def get_inhabitants(city: str) -> int:
"""Get the current temperature of a city"""
return randint(4_000_000, 8_000_000)
@tool
def get_curr_temperature(city: str) -> int:
"""Get the current temperature of a city"""
return randint(20, 30)
chat = ChatLlamaROS(temp=0.6, penalty_last_n=8)
messages = [
HumanMessage(
"What is the current temperature in Madrid? And its inhabitants?"
)
]
llm_tools = chat.bind_tools(
[get_inhabitants, get_curr_temperature], tool_choice='any'
)
all_tools_res = llm_tools.invoke(messages)
messages.append(all_tools_res)
for tool in all_tools_res.tool_calls:
selected_tool = {
"get_inhabitants": get_inhabitants, "get_curr_temperature": get_curr_temperature
}[tool['name']]
tool_msg = selected_tool.invoke(tool)
formatted_output = f"{tool['name']}({''.join(tool['args'].values())}) = {tool_msg.content}"
tool_msg.additional_kwargs = {'args': tool['args']}
messages.append(tool_msg)
res = llm_tools.invoke(messages)
print(f"Response: {res.content}")
rclpy.shutdown()
Click to expand
A reasoning model is required, such as Deepseek R1
import time
from random import randint
import rclpy
from langchain_core.messages import HumanMessage
from llama_ros.langchain import ChatLlamaROS
rclpy.init()
chat = ChatLlamaROS(temp=0.6, penalty_last_n=8)
messages = [
HumanMessage(
"Here we have a book, a laptop, 9 eggs and a nail. Please tell me how to stack them onto each other in a stable manner."
)
]
res = chat.invoke(messages)
print(f"Response: {res.content.strip()}")
print(f"Reasoning: {res.additional_kwargs["reasoning_content"]}")
rclpy.shutdown()
Click to expand
import time
from random import randint
import rclpy
from langchain.tools import tool
from langchain_core.messages import HumanMessage
from langgraph.prebuilt import create_react_agent
from llama_ros.langchain import ChatLlamaROS
rclpy.init()
@tool
def get_inhabitants(city: str) -> int:
"""Get the current temperature of a city"""
return randint(4_000_000, 8_000_000)
@tool
def get_curr_temperature(city: str) -> int:
"""Get the current temperature of a city"""
return randint(20, 30)
chat = ChatLlamaROS(temp=0.0)
agent_executor = create_react_agent(
self.chat, [get_inhabitants, get_curr_temperature]
)
response = self.agent_executor.invoke(
{
"messages": [
HumanMessage(
content="What is the current temperature in Madrid? And its inhabitants?"
)
]
}
)
print(f"Response: {response['messages'][-1].content}")
rclpy.shutdown()
ros2 launch llama_bringup spaetzle.launch.py
ros2 run llama_demos llama_demo_node
https://github.com/mgonzs13/llama_ros/assets/25979134/9311761b-d900-4e58-b9f8-11c8efefdac4
ros2 llama launch ~/ros2_ws/src/llama_ros/llama_bringup/models/bge-base-en-v1.5.yaml
ros2 run llama_demos llama_embeddings_demo_node
https://github.com/user-attachments/assets/7d722017-27dc-417c-ace7-bf6b747e4ced
ros2 llama launch ~/ros2_ws/src/llama_ros/llama_bringup/models/jina-reranker.yaml
ros2 run llama_demos llama_rerank_demo_node
https://github.com/user-attachments/assets/4b4adb4d-7c70-43ea-a2c1-9be57d211484
ros2 launch llama_bringup minicpm-2.6.launch.py
ros2 run llama_demos llava_demo_node --ros-args -p prompt:="your prompt" -p image_url:="url of the image" -p use_image:="whether to send the image"
https://github.com/mgonzs13/llama_ros/assets/25979134/4a9ef92f-9099-41b4-8350-765336e3503c
ros2 llama launch MiniCPM-2.6.yaml
Click to expand MiniCPM-2.6.yaml
use_llava: True
n_ctx: 8192
n_batch: 512
n_gpu_layers: 20
n_threads: -1
n_predict: 8192
image_prefix: "<image>"
image_suffix: "</image>"
model_repo: "openbmb/MiniCPM-V-2_6-gguf"
model_filename: "ggml-model-Q4_K_M.gguf"
mmproj_repo: "openbmb/MiniCPM-V-2_6-gguf"
mmproj_filename: "mmproj-model-f16.gguf"
ros2 run llama_demos chatllama_demo_node
ros2 llama launch Qwen2.yaml
ros2 run llama_demos chatllama_structured_demo_node
ros2 llama launch Qwen2.yaml
ros2 run llama_demos chatllama_tools_demo_node
ros2 llama launch DeepSeek-R1.yaml
ros2 run llama_demos chatllama_reasoning_demo_node
ros2 llama launch Qwen2.yaml
Click to expand Qwen2.yaml
_ctx: 4096
n_batch: 256
n_gpu_layers: 29
n_threads: -1
n_predict: -1
model_repo: "Qwen/Qwen2.5-Coder-7B-Instruct-GGUF"
model_filename: "qwen2.5-coder-7b-instruct-q4_k_m-00001-of-00002.gguf"
ros2 run llama_demos chatllama_langgraph_demo_node
ros2 llama launch ~/ros2_ws/src/llama_ros/llama_bringup/models/bge-base-en-v1.5.yaml
ros2 llama launch ~/ros2_ws/src/llama_ros/llama_bringup/models/jina-reranker.yaml
ros2 llama launch Qwen2.yaml
Click to expand Qwen2.yaml
_ctx: 4096
n_batch: 256
n_gpu_layers: 29
n_threads: -1
n_predict: -1
model_repo: "Qwen/Qwen2.5-Coder-3B-Instruct-GGUF"
model_filename: "qwen2.5-coder-3b-instruct-q4_k_m.gguf"
ros2 run llama_demos llama_rag_demo_node
https://github.com/user-attachments/assets/b4e3957d-1f92-427b-a1a8-cfc76737c0d6
For Tasks:
Click tags to check more tools for each tasksFor Jobs:
Alternative AI tools for llama_ros
Similar Open Source Tools
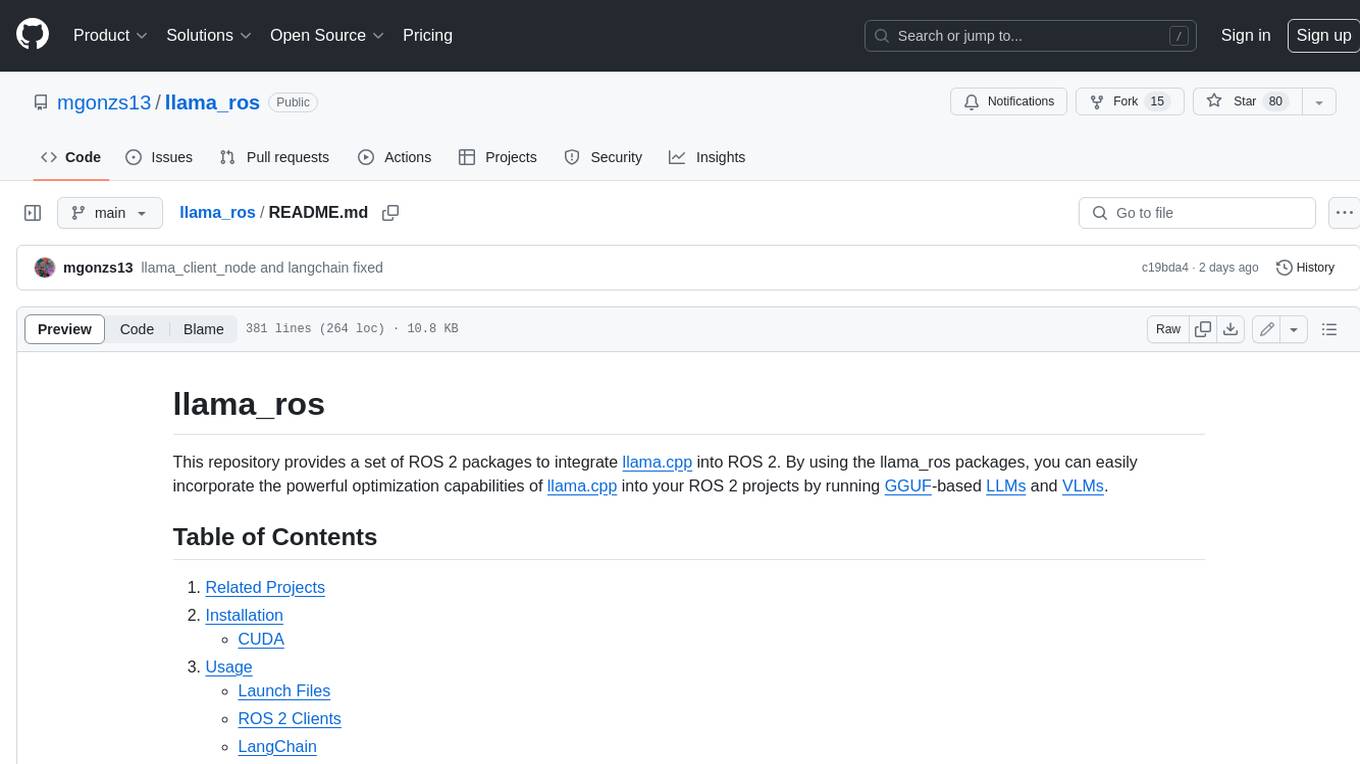
llama_ros
This repository provides a set of ROS 2 packages to integrate llama.cpp into ROS 2. By using the llama_ros packages, you can easily incorporate the powerful optimization capabilities of llama.cpp into your ROS 2 projects by running GGUF-based LLMs and VLMs.
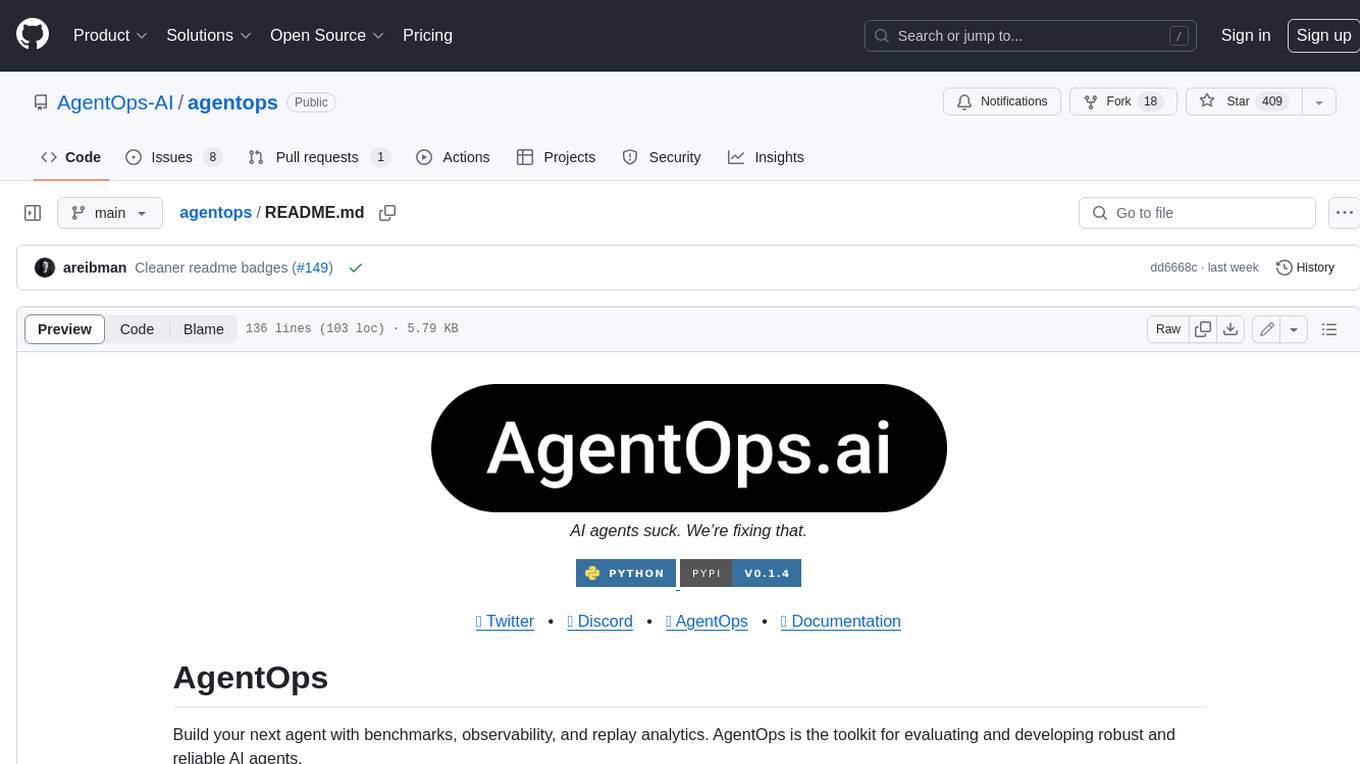
agentops
AgentOps is a toolkit for evaluating and developing robust and reliable AI agents. It provides benchmarks, observability, and replay analytics to help developers build better agents. AgentOps is open beta and can be signed up for here. Key features of AgentOps include: - Session replays in 3 lines of code: Initialize the AgentOps client and automatically get analytics on every LLM call. - Time travel debugging: (coming soon!) - Agent Arena: (coming soon!) - Callback handlers: AgentOps works seamlessly with applications built using Langchain and LlamaIndex.
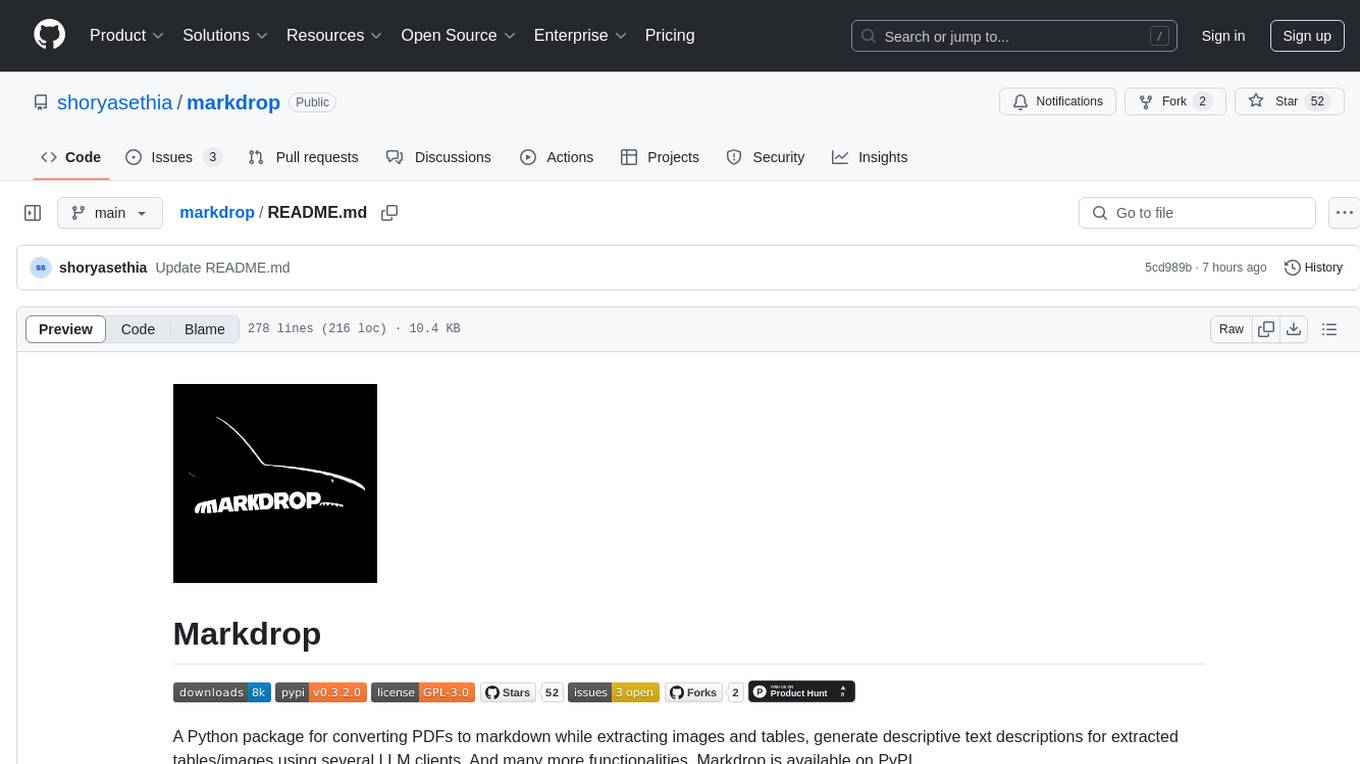
markdrop
Markdrop is a Python package that facilitates the conversion of PDFs to markdown format while extracting images and tables. It also generates descriptive text descriptions for extracted tables and images using various LLM clients. The tool offers additional functionalities such as PDF URL support, AI-powered image and table descriptions, interactive HTML output with downloadable Excel tables, customizable image resolution and UI elements, and a comprehensive logging system. Markdrop aims to simplify the process of handling PDF documents and enhancing their content with AI-generated descriptions.
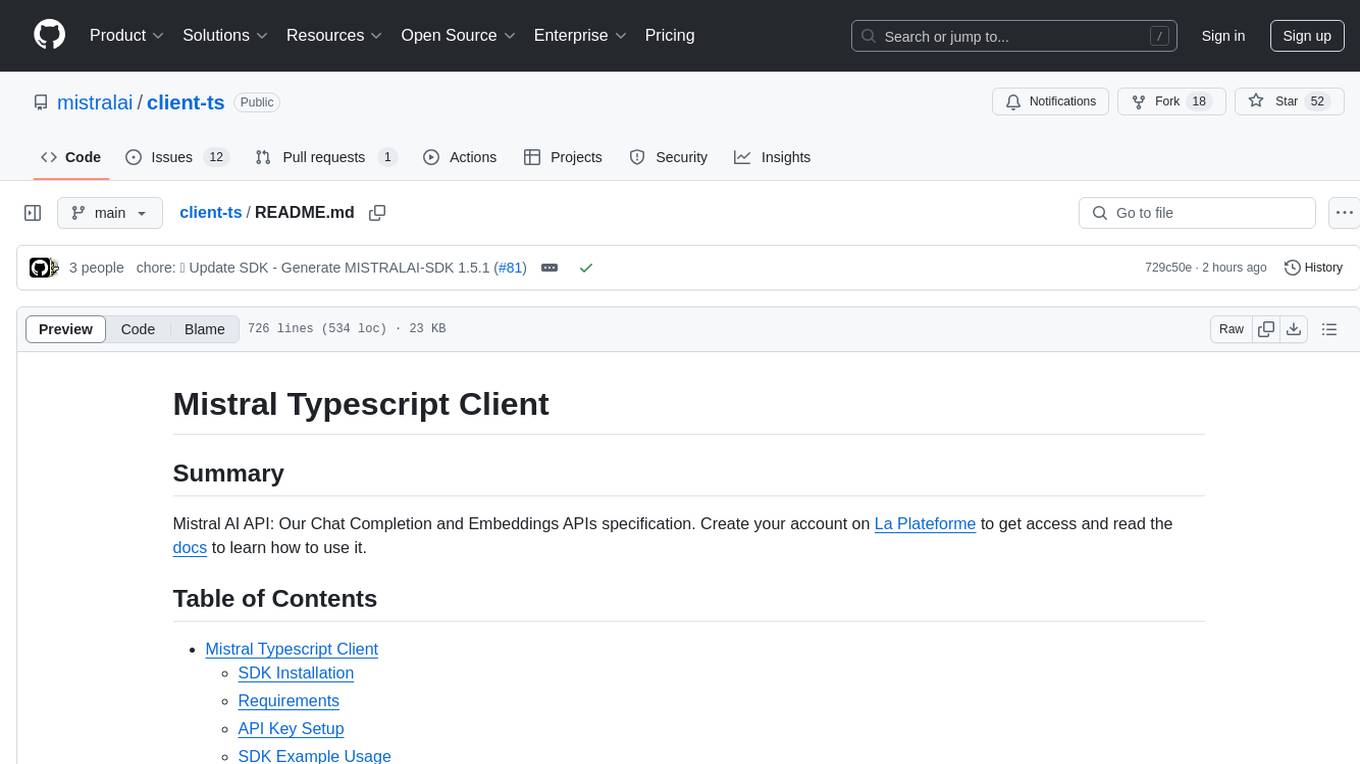
client-ts
Mistral Typescript Client is an SDK for Mistral AI API, providing Chat Completion and Embeddings APIs. It allows users to create chat completions, upload files, create agent completions, create embedding requests, and more. The SDK supports various JavaScript runtimes and provides detailed documentation on installation, requirements, API key setup, example usage, error handling, server selection, custom HTTP client, authentication, providers support, standalone functions, debugging, and contributions.
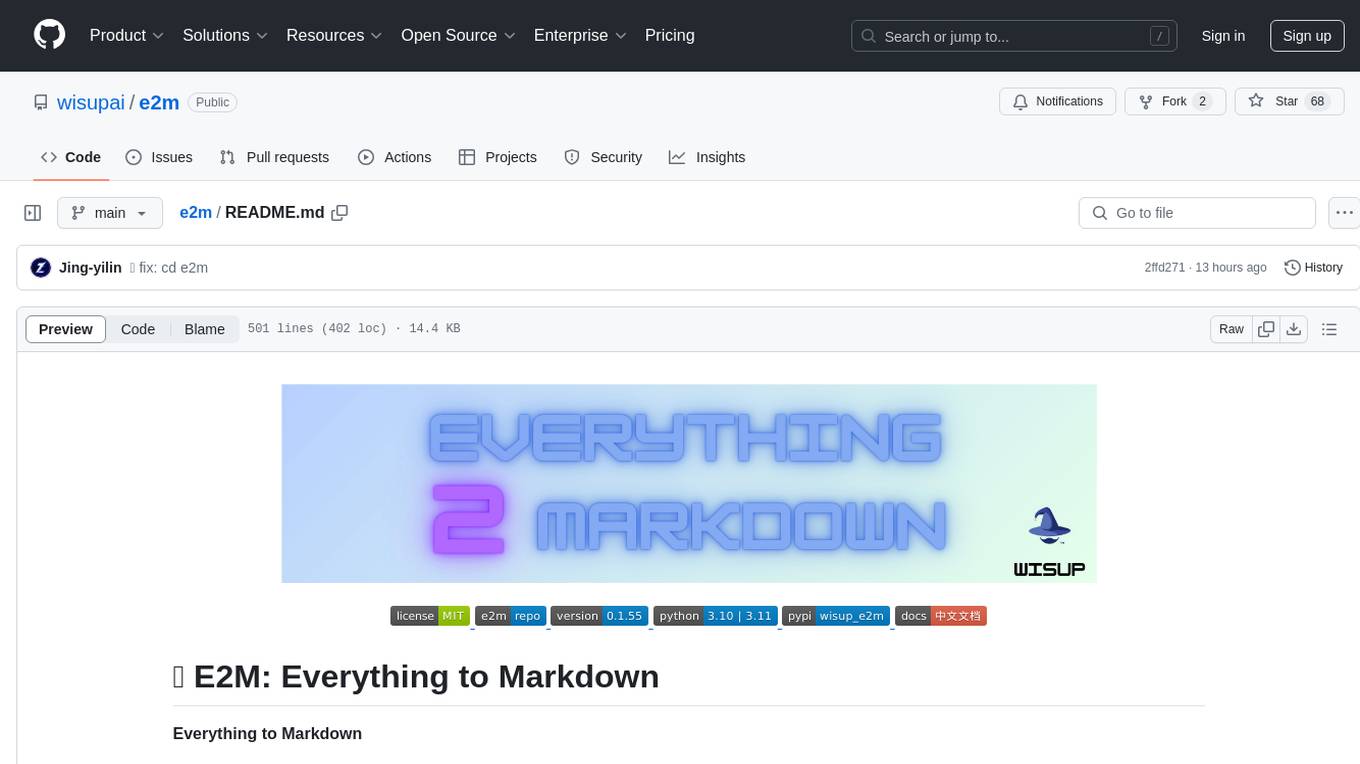
e2m
E2M is a Python library that can parse and convert various file types into Markdown format. It supports the conversion of multiple file formats, including doc, docx, epub, html, htm, url, pdf, ppt, pptx, mp3, and m4a. The ultimate goal of the E2M project is to provide high-quality data for Retrieval-Augmented Generation (RAG) and model training or fine-tuning. The core architecture consists of a Parser responsible for parsing various file types into text or image data, and a Converter responsible for converting text or image data into Markdown format.
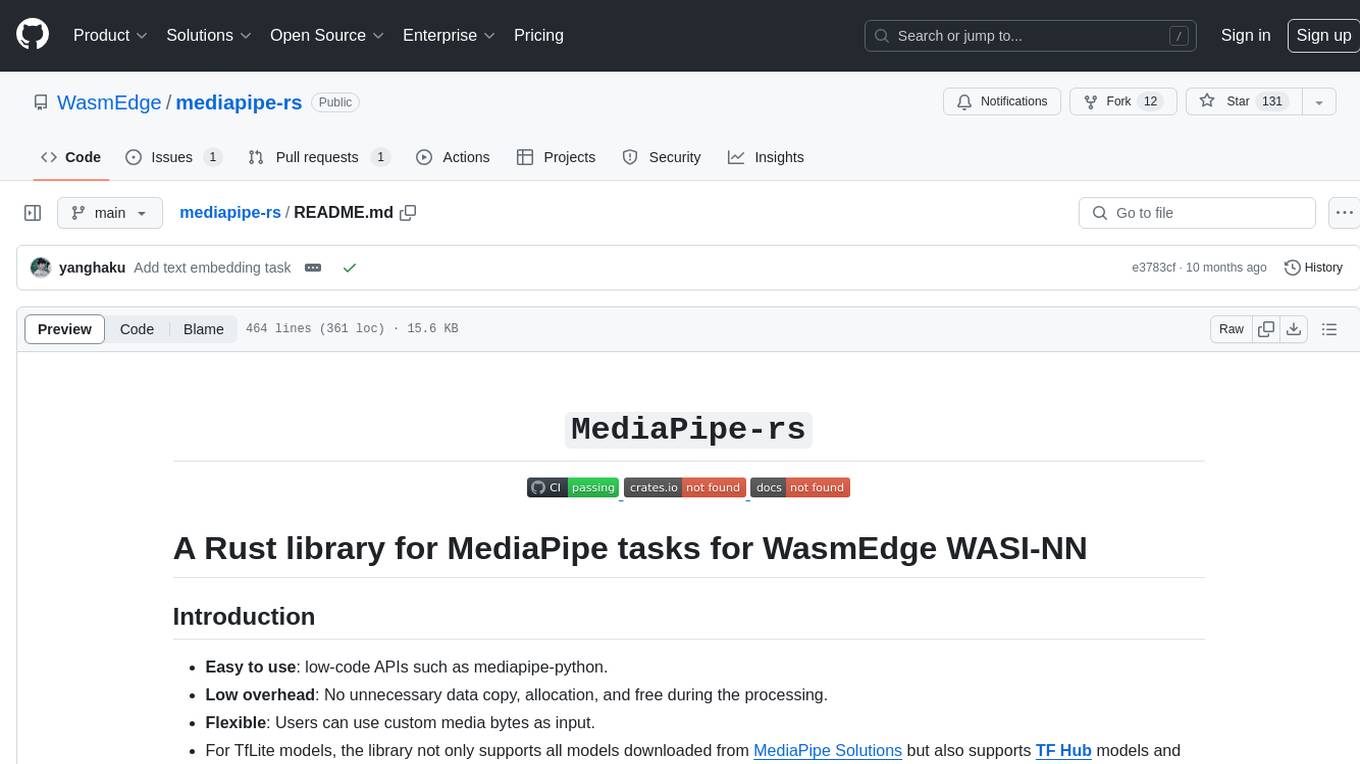
mediapipe-rs
MediaPipe-rs is a Rust library designed for MediaPipe tasks on WasmEdge WASI-NN. It offers easy-to-use low-code APIs similar to mediapipe-python, with low overhead and flexibility for custom media input. The library supports various tasks like object detection, image classification, gesture recognition, and more, including TfLite models, TF Hub models, and custom models. Users can create task instances, run sessions for pre-processing, inference, and post-processing, and speed up processing by reusing sessions. The library also provides support for audio tasks using audio data from symphonia, ffmpeg, or raw audio. Users can choose between CPU, GPU, or TPU devices for processing.
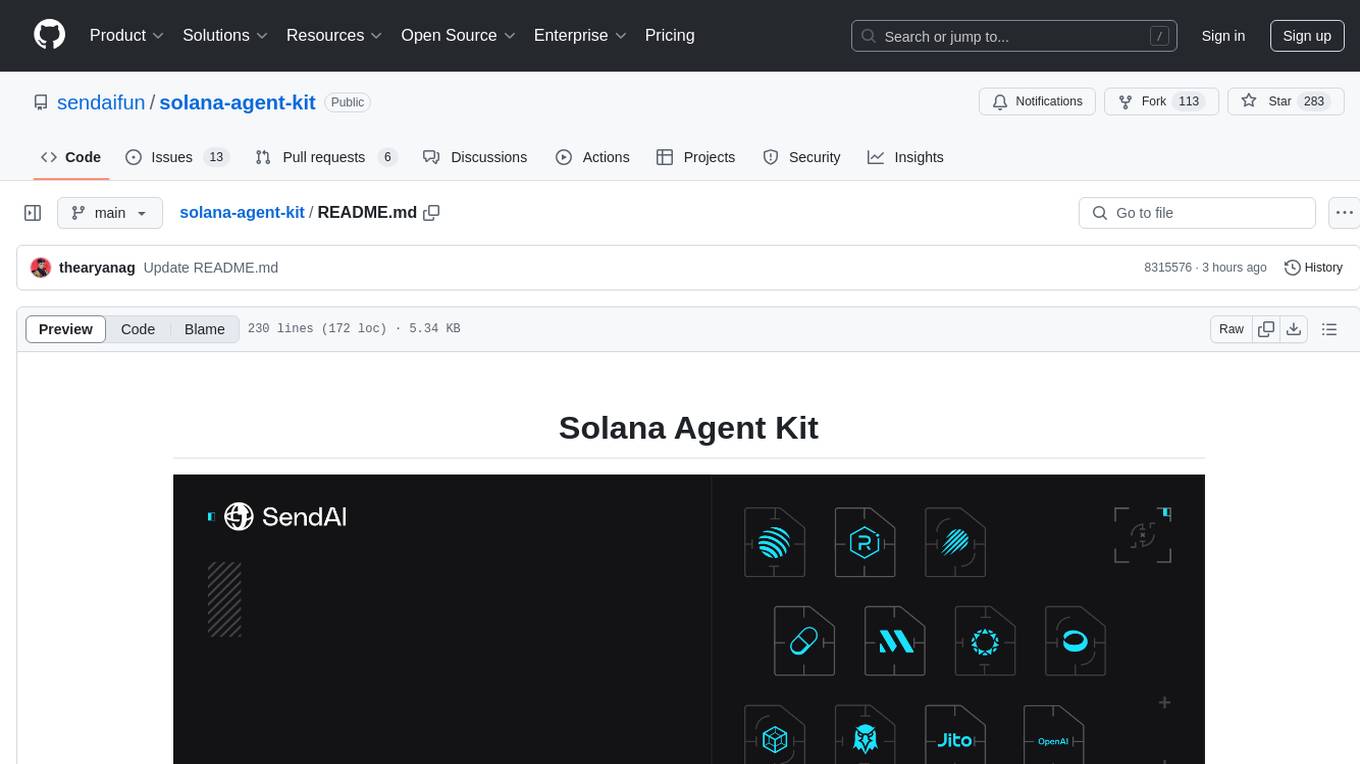
solana-agent-kit
Solana Agent Kit is an open-source toolkit designed for connecting AI agents to Solana protocols. It enables agents, regardless of the model used, to autonomously perform various Solana actions such as trading tokens, launching new tokens, lending assets, sending compressed airdrops, executing blinks, and more. The toolkit integrates core blockchain features like token operations, NFT management via Metaplex, DeFi integration, Solana blinks, AI integration features with LangChain, autonomous modes, and AI tools. It provides ready-to-use tools for blockchain operations, supports autonomous agent actions, and offers features like memory management, real-time feedback, and error handling. Solana Agent Kit facilitates tasks such as deploying tokens, creating NFT collections, swapping tokens, lending tokens, staking SOL, and sending SPL token airdrops via ZK compression. It also includes functionalities for fetching price data from Pyth and relies on key Solana and Metaplex libraries for its operations.
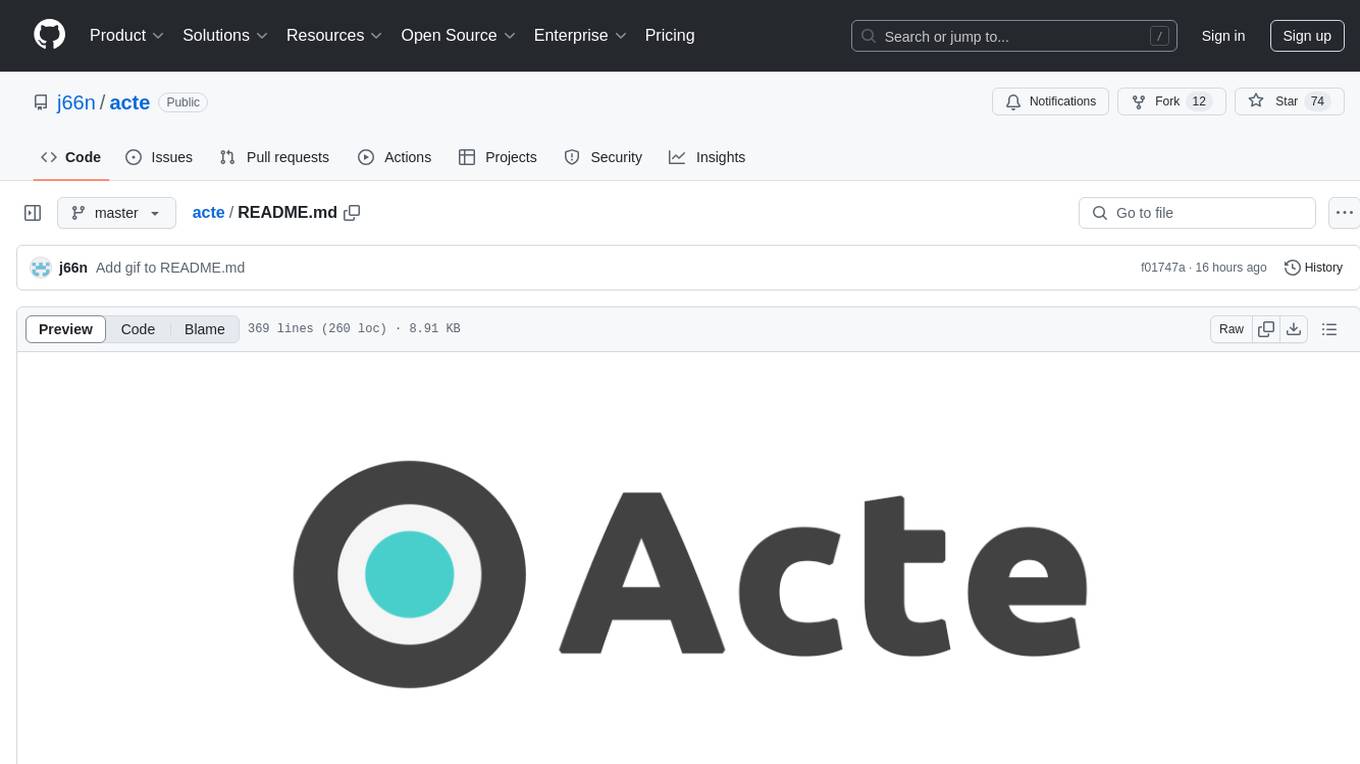
acte
Acte is a framework designed to build GUI-like tools for AI Agents. It aims to address the issues of cognitive load and freedom degrees when interacting with multiple APIs in complex scenarios. By providing a graphical user interface (GUI) for Agents, Acte helps reduce cognitive load and constraints interaction, similar to how humans interact with computers through GUIs. The tool offers APIs for starting new sessions, executing actions, and displaying screens, accessible via HTTP requests or the SessionManager class.
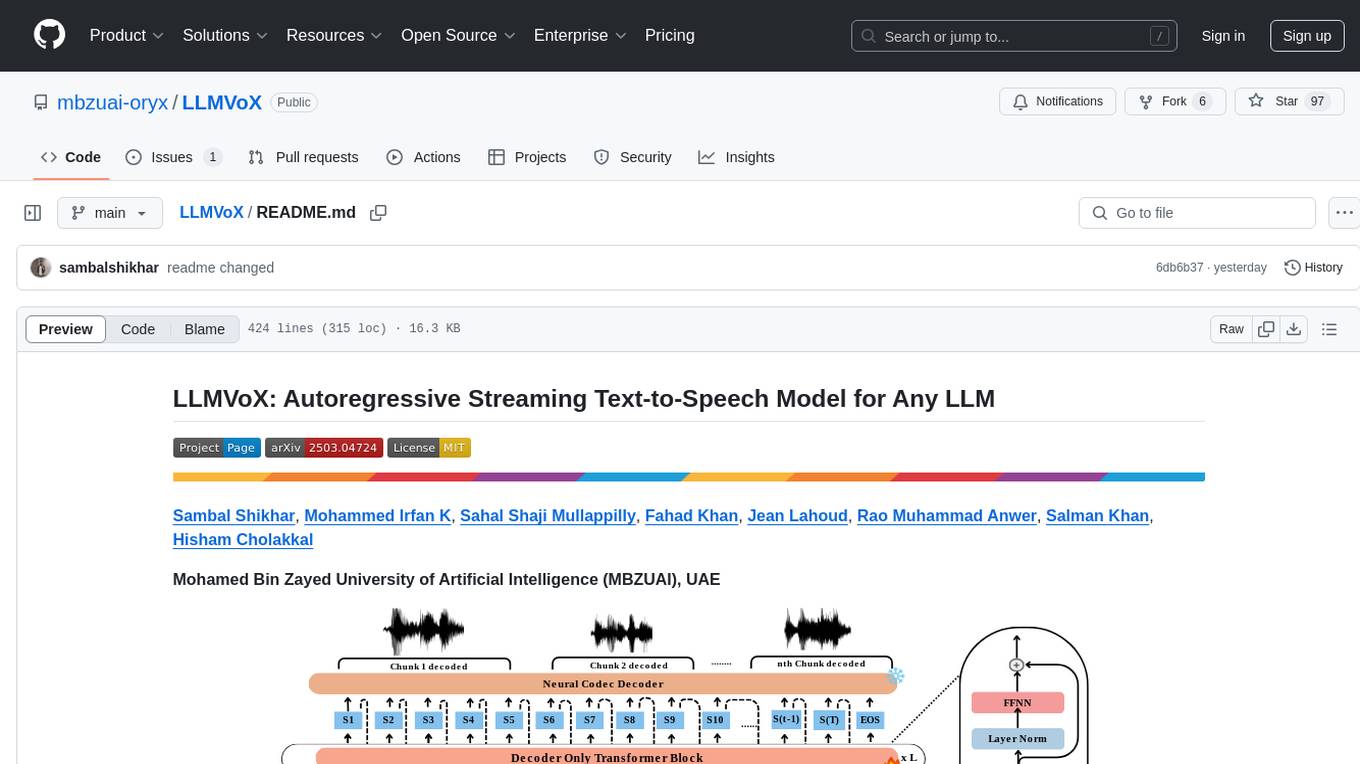
LLMVoX
LLMVoX is a lightweight 30M-parameter, LLM-agnostic, autoregressive streaming Text-to-Speech (TTS) system designed to convert text outputs from Large Language Models into high-fidelity streaming speech with low latency. It achieves significantly lower Word Error Rate compared to speech-enabled LLMs while operating at comparable latency and speech quality. Key features include being lightweight & fast with only 30M parameters, LLM-agnostic for easy integration with existing models, multi-queue streaming for continuous speech generation, and multilingual support for easy adaptation to new languages.
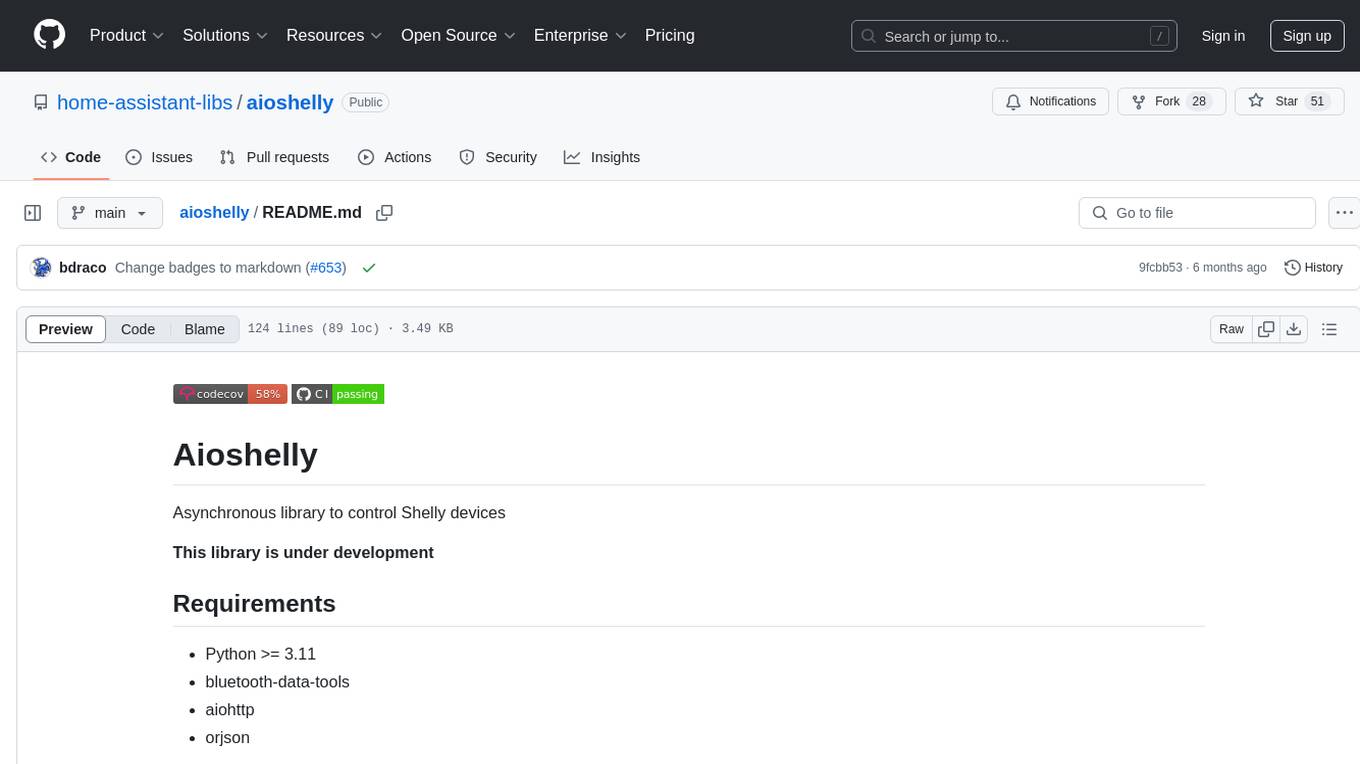
aioshelly
Aioshelly is an asynchronous library designed to control Shelly devices. It is currently under development and requires Python version 3.11 or higher, along with dependencies like bluetooth-data-tools, aiohttp, and orjson. The library provides examples for interacting with Gen1 devices using CoAP protocol and Gen2/Gen3 devices using RPC and WebSocket protocols. Users can easily connect to Shelly devices, retrieve status information, and perform various actions through the provided APIs. The repository also includes example scripts for quick testing and usage guidelines for contributors to maintain consistency with the Shelly API.
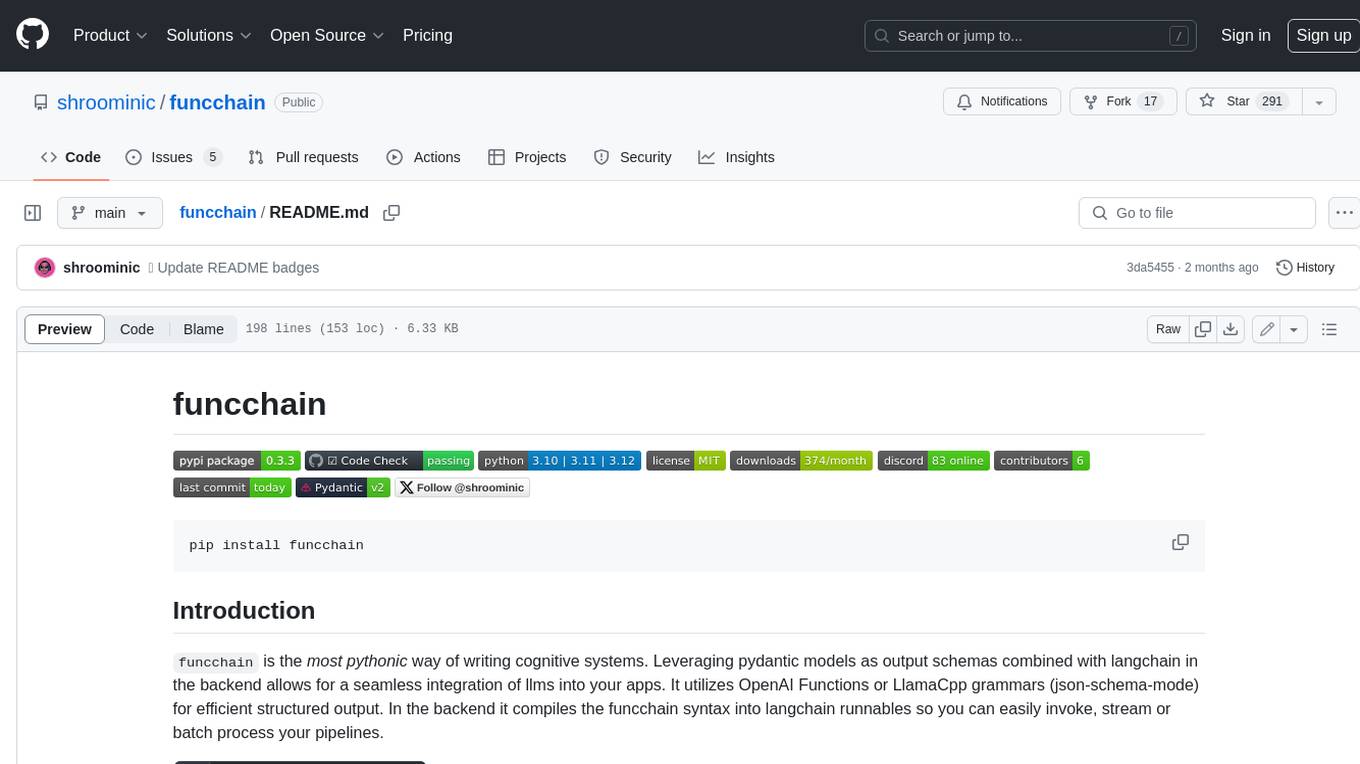
funcchain
Funcchain is a Python library that allows you to easily write cognitive systems by leveraging Pydantic models as output schemas and LangChain in the backend. It provides a seamless integration of LLMs into your apps, utilizing OpenAI Functions or LlamaCpp grammars (json-schema-mode) for efficient structured output. Funcchain compiles the Funcchain syntax into LangChain runnables, enabling you to invoke, stream, or batch process your pipelines effortlessly.
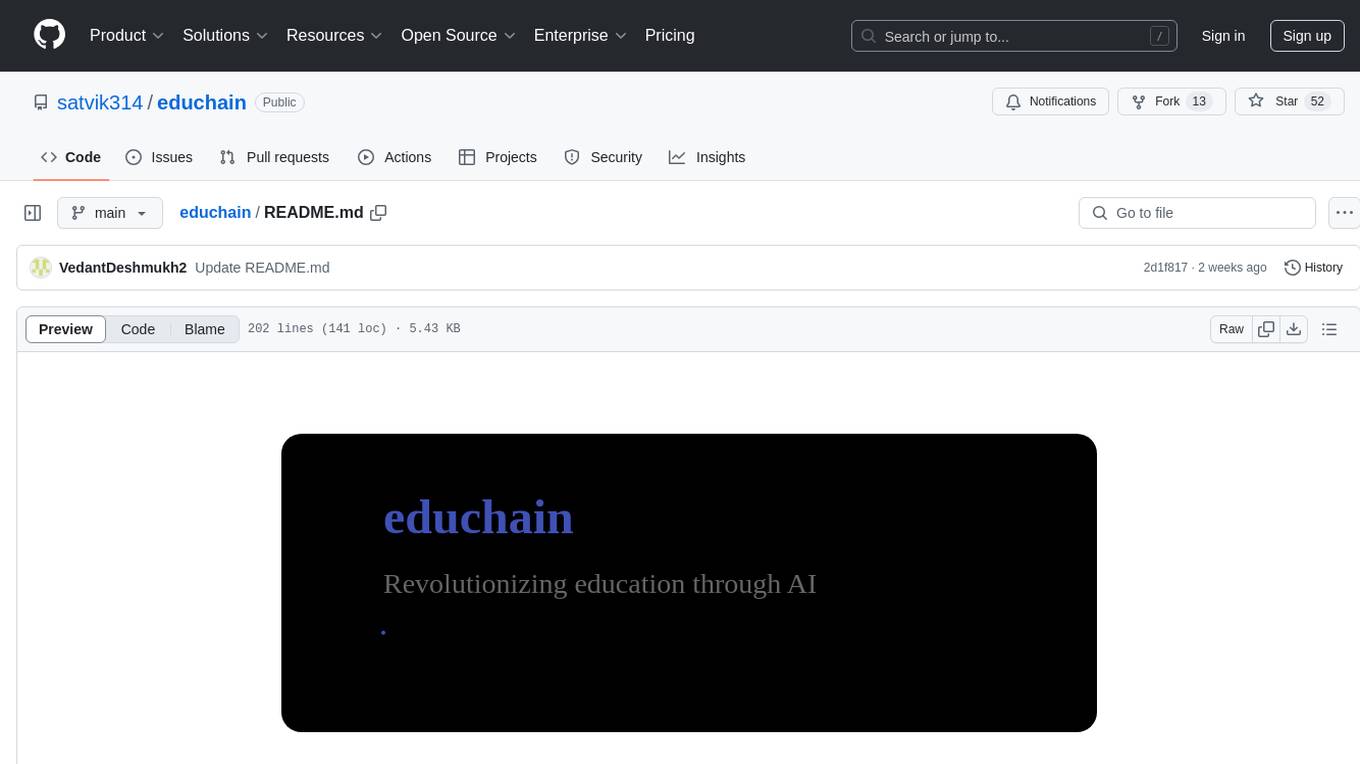
educhain
Educhain is a powerful Python package that leverages Generative AI to create engaging and personalized educational content. It enables users to generate multiple-choice questions, create lesson plans, and support various LLM models. Users can export questions to JSON, PDF, and CSV formats, customize prompt templates, and generate questions from text, PDF, URL files, youtube videos, and images. Educhain outperforms traditional methods in content generation speed and quality. It offers advanced configuration options and has a roadmap for future enhancements, including integration with popular Learning Management Systems and a mobile app for content generation on-the-go.
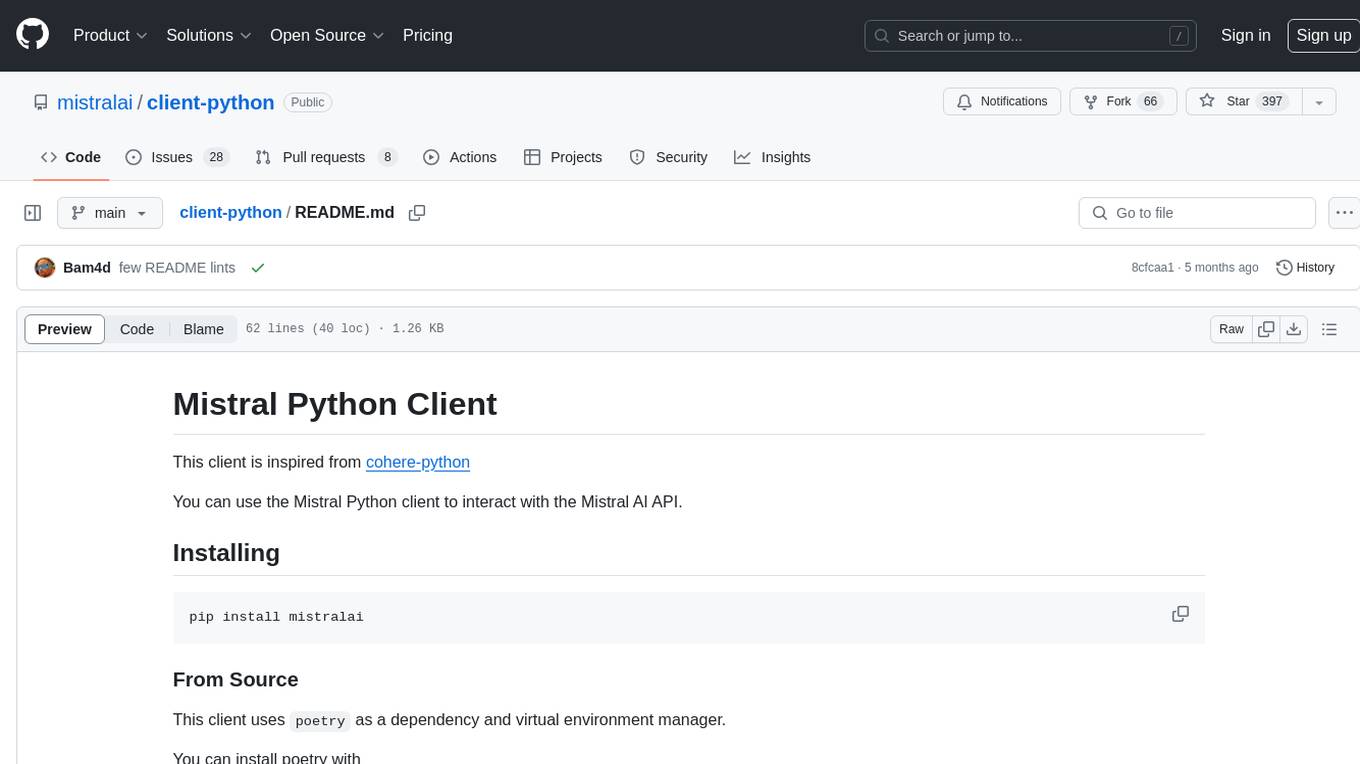
client-python
The Mistral Python Client is a tool inspired by cohere-python that allows users to interact with the Mistral AI API. It provides functionalities to access and utilize the AI capabilities offered by Mistral. Users can easily install the client using pip and manage dependencies using poetry. The client includes examples demonstrating how to use the API for various tasks, such as chat interactions. To get started, users need to obtain a Mistral API Key and set it as an environment variable. Overall, the Mistral Python Client simplifies the integration of Mistral AI services into Python applications.
For similar tasks
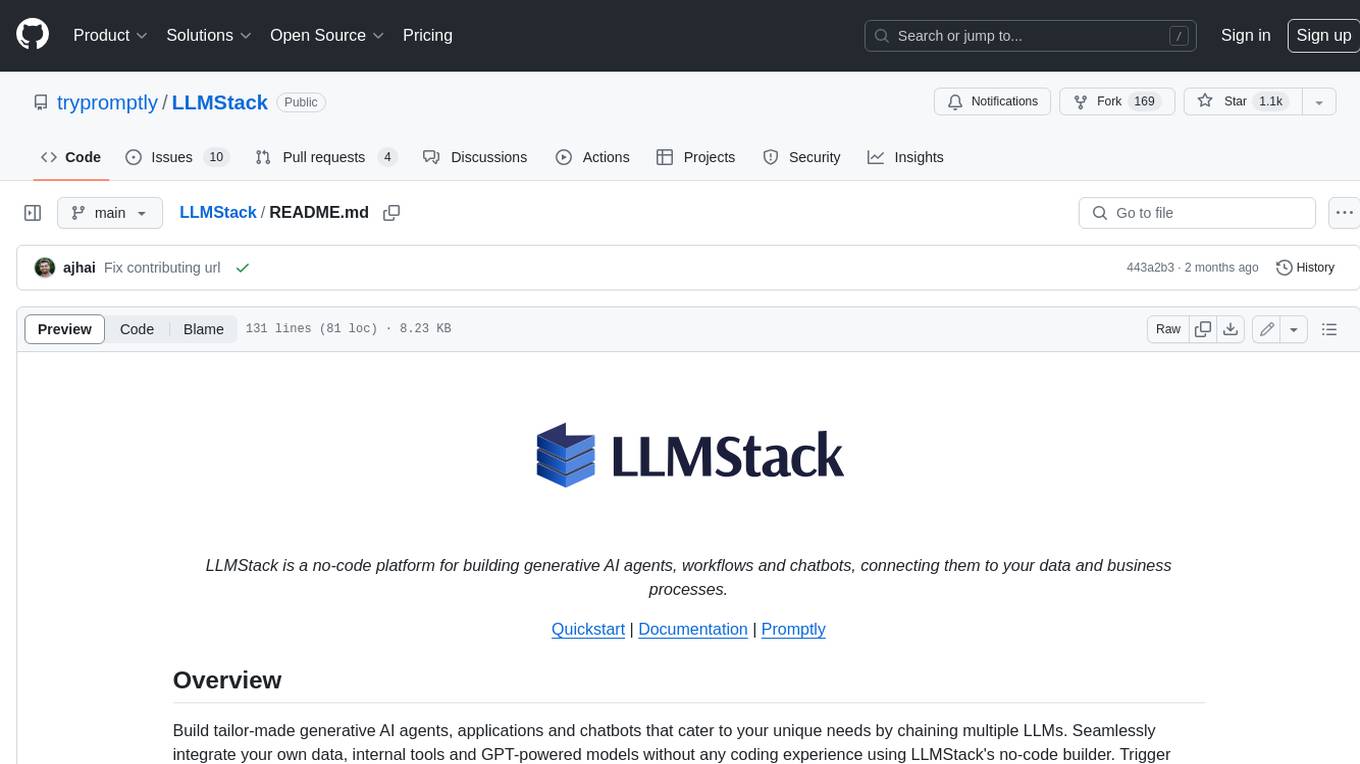
LLMStack
LLMStack is a no-code platform for building generative AI agents, workflows, and chatbots. It allows users to connect their own data, internal tools, and GPT-powered models without any coding experience. LLMStack can be deployed to the cloud or on-premise and can be accessed via HTTP API or triggered from Slack or Discord.
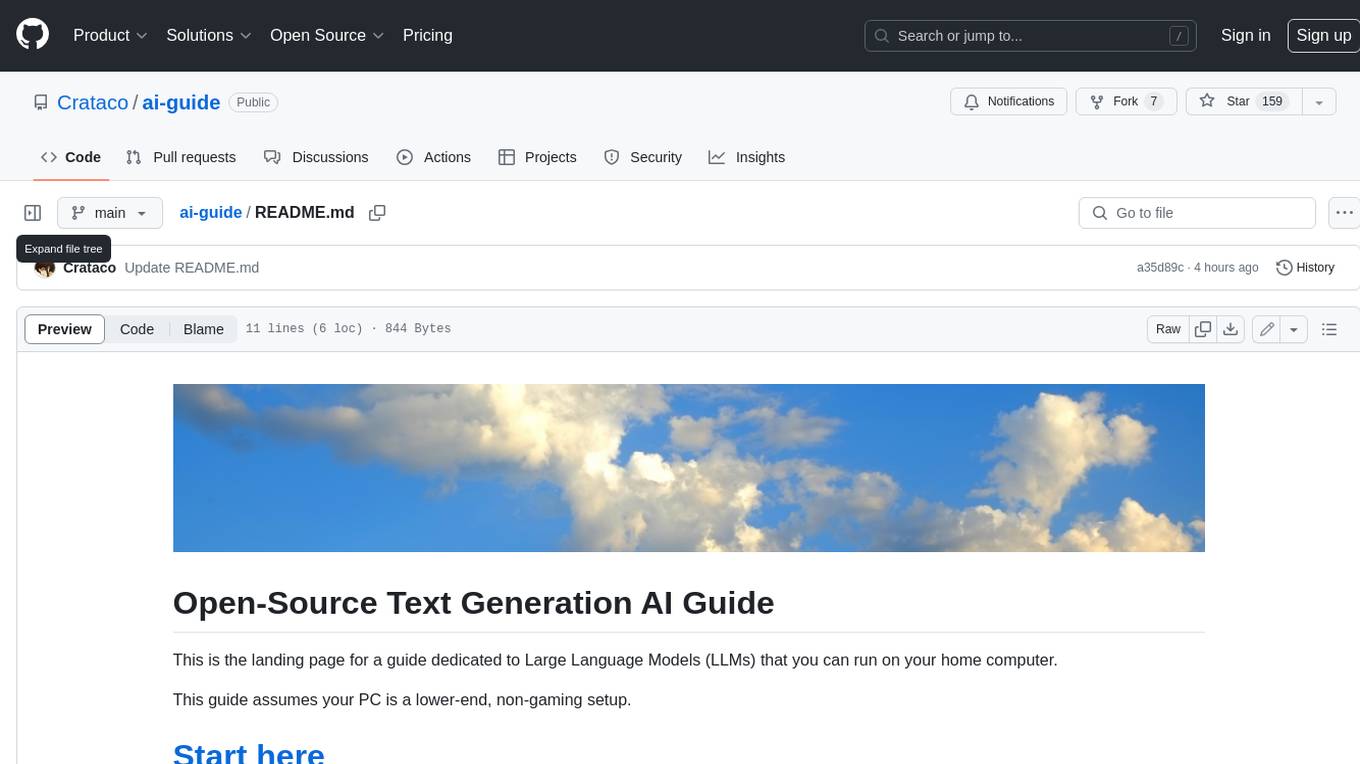
ai-guide
This guide is dedicated to Large Language Models (LLMs) that you can run on your home computer. It assumes your PC is a lower-end, non-gaming setup.
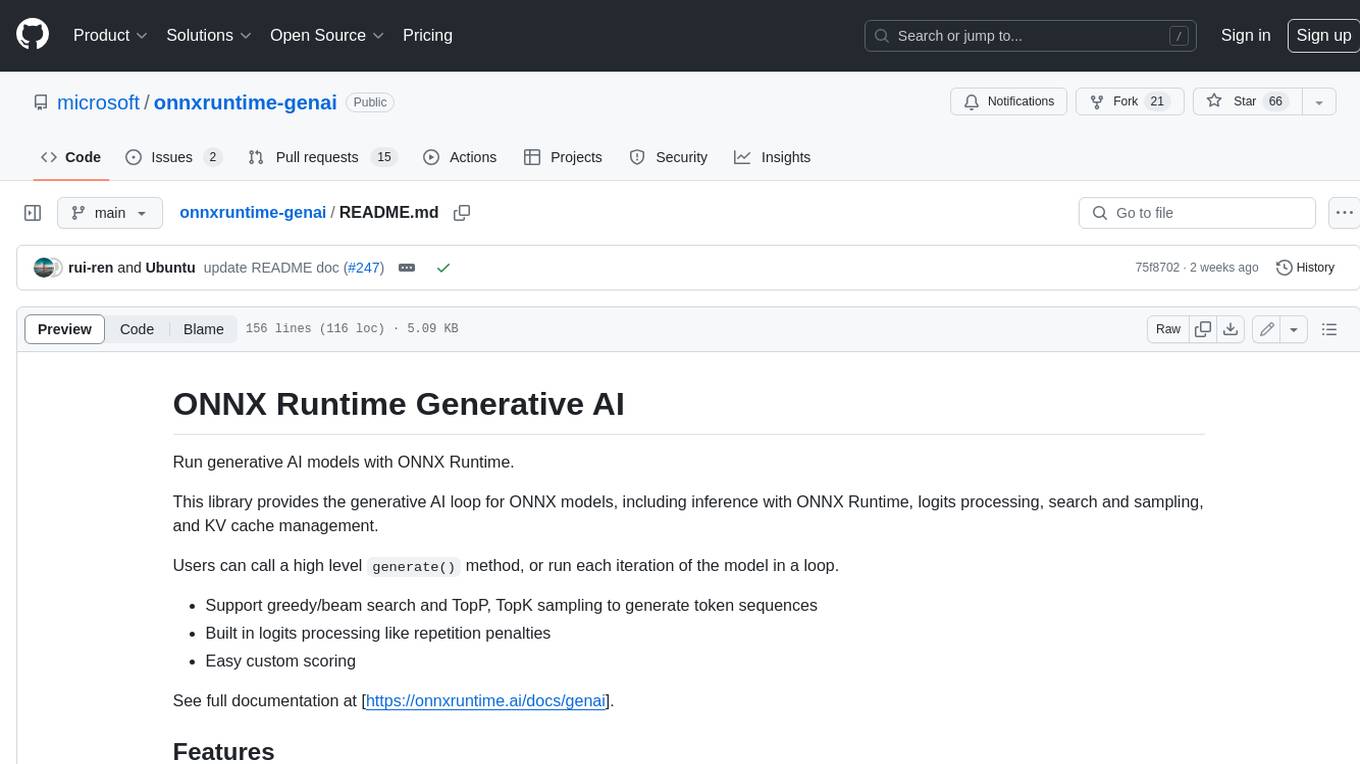
onnxruntime-genai
ONNX Runtime Generative AI is a library that provides the generative AI loop for ONNX models, including inference with ONNX Runtime, logits processing, search and sampling, and KV cache management. Users can call a high level `generate()` method, or run each iteration of the model in a loop. It supports greedy/beam search and TopP, TopK sampling to generate token sequences, has built in logits processing like repetition penalties, and allows for easy custom scoring.
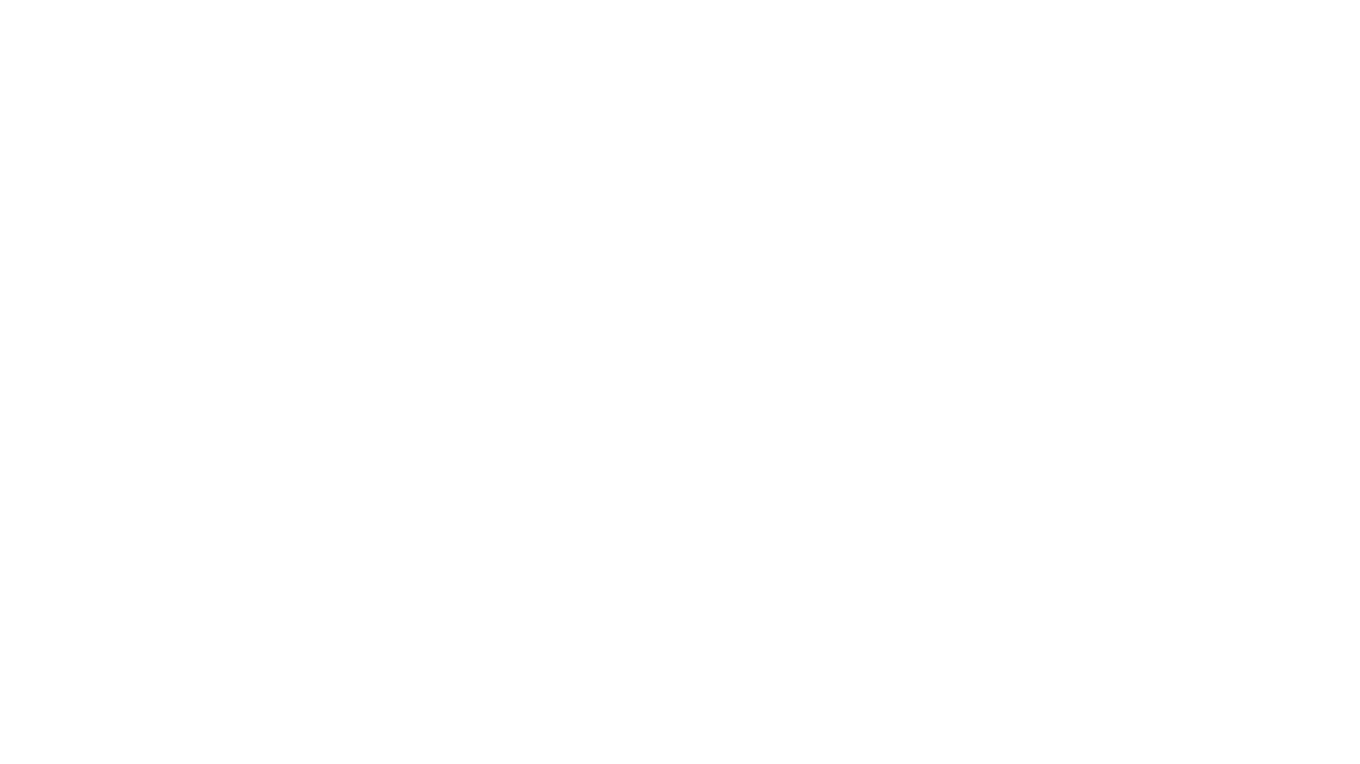
jupyter-ai
Jupyter AI connects generative AI with Jupyter notebooks. It provides a user-friendly and powerful way to explore generative AI models in notebooks and improve your productivity in JupyterLab and the Jupyter Notebook. Specifically, Jupyter AI offers: * An `%%ai` magic that turns the Jupyter notebook into a reproducible generative AI playground. This works anywhere the IPython kernel runs (JupyterLab, Jupyter Notebook, Google Colab, Kaggle, VSCode, etc.). * A native chat UI in JupyterLab that enables you to work with generative AI as a conversational assistant. * Support for a wide range of generative model providers, including AI21, Anthropic, AWS, Cohere, Gemini, Hugging Face, NVIDIA, and OpenAI. * Local model support through GPT4All, enabling use of generative AI models on consumer grade machines with ease and privacy.
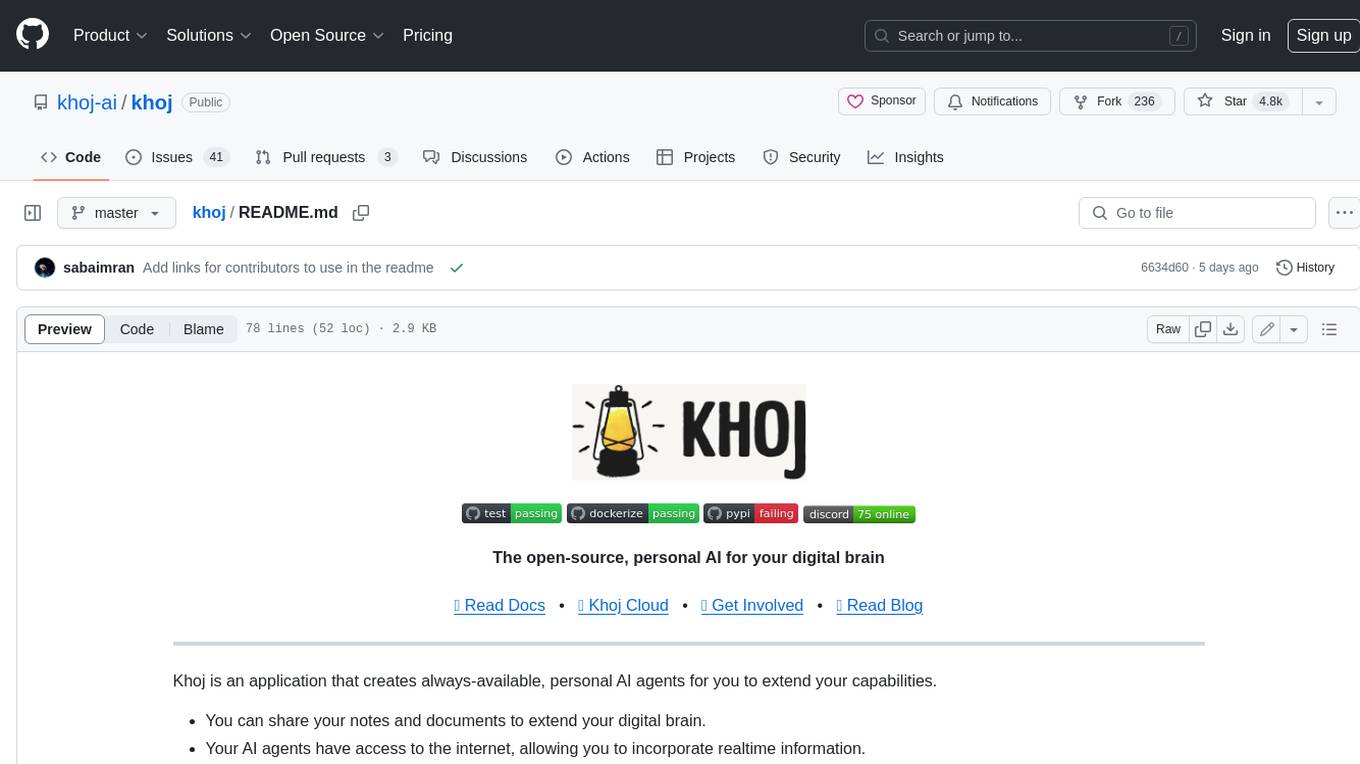
khoj
Khoj is an open-source, personal AI assistant that extends your capabilities by creating always-available AI agents. You can share your notes and documents to extend your digital brain, and your AI agents have access to the internet, allowing you to incorporate real-time information. Khoj is accessible on Desktop, Emacs, Obsidian, Web, and Whatsapp, and you can share PDF, markdown, org-mode, notion files, and GitHub repositories. You'll get fast, accurate semantic search on top of your docs, and your agents can create deeply personal images and understand your speech. Khoj is self-hostable and always will be.
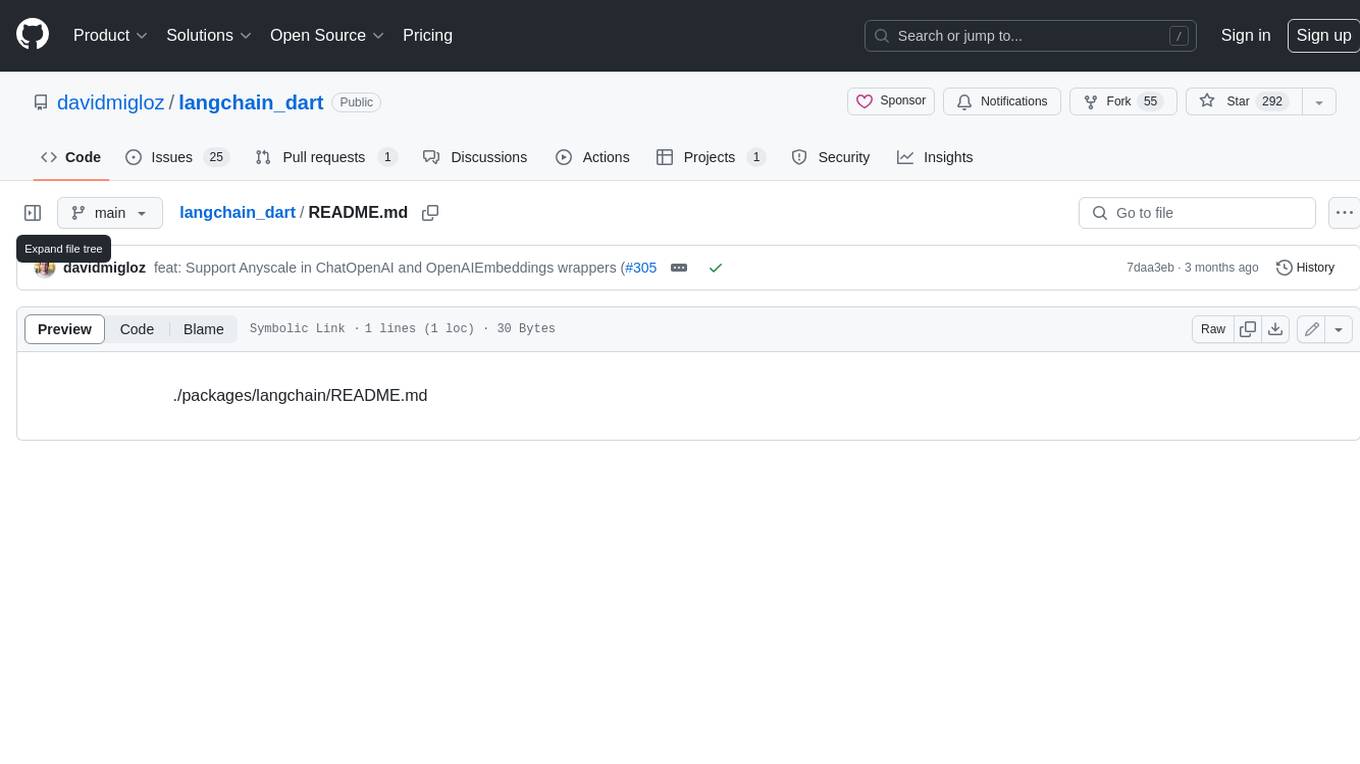
langchain_dart
LangChain.dart is a Dart port of the popular LangChain Python framework created by Harrison Chase. LangChain provides a set of ready-to-use components for working with language models and a standard interface for chaining them together to formulate more advanced use cases (e.g. chatbots, Q&A with RAG, agents, summarization, extraction, etc.). The components can be grouped into a few core modules: * **Model I/O:** LangChain offers a unified API for interacting with various LLM providers (e.g. OpenAI, Google, Mistral, Ollama, etc.), allowing developers to switch between them with ease. Additionally, it provides tools for managing model inputs (prompt templates and example selectors) and parsing the resulting model outputs (output parsers). * **Retrieval:** assists in loading user data (via document loaders), transforming it (with text splitters), extracting its meaning (using embedding models), storing (in vector stores) and retrieving it (through retrievers) so that it can be used to ground the model's responses (i.e. Retrieval-Augmented Generation or RAG). * **Agents:** "bots" that leverage LLMs to make informed decisions about which available tools (such as web search, calculators, database lookup, etc.) to use to accomplish the designated task. The different components can be composed together using the LangChain Expression Language (LCEL).
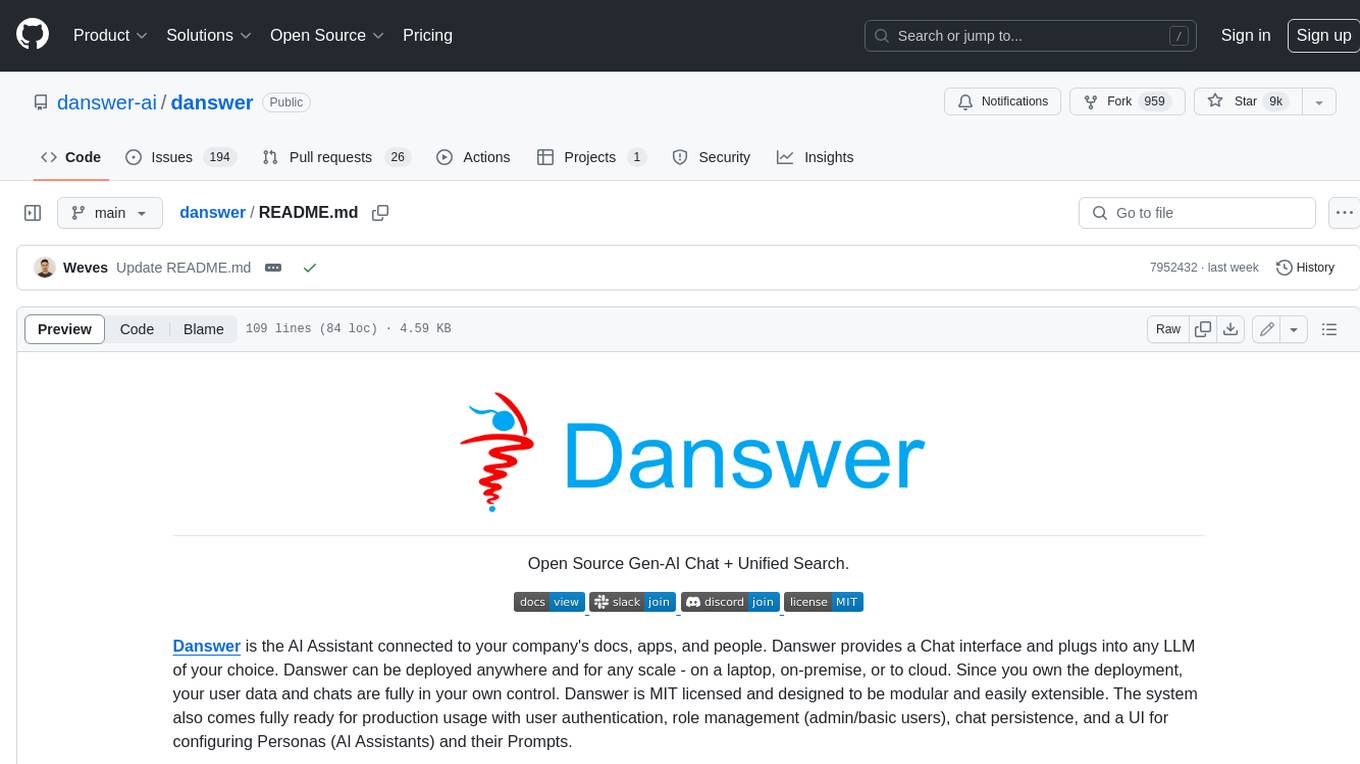
danswer
Danswer is an open-source Gen-AI Chat and Unified Search tool that connects to your company's docs, apps, and people. It provides a Chat interface and plugs into any LLM of your choice. Danswer can be deployed anywhere and for any scale - on a laptop, on-premise, or to cloud. Since you own the deployment, your user data and chats are fully in your own control. Danswer is MIT licensed and designed to be modular and easily extensible. The system also comes fully ready for production usage with user authentication, role management (admin/basic users), chat persistence, and a UI for configuring Personas (AI Assistants) and their Prompts. Danswer also serves as a Unified Search across all common workplace tools such as Slack, Google Drive, Confluence, etc. By combining LLMs and team specific knowledge, Danswer becomes a subject matter expert for the team. Imagine ChatGPT if it had access to your team's unique knowledge! It enables questions such as "A customer wants feature X, is this already supported?" or "Where's the pull request for feature Y?"
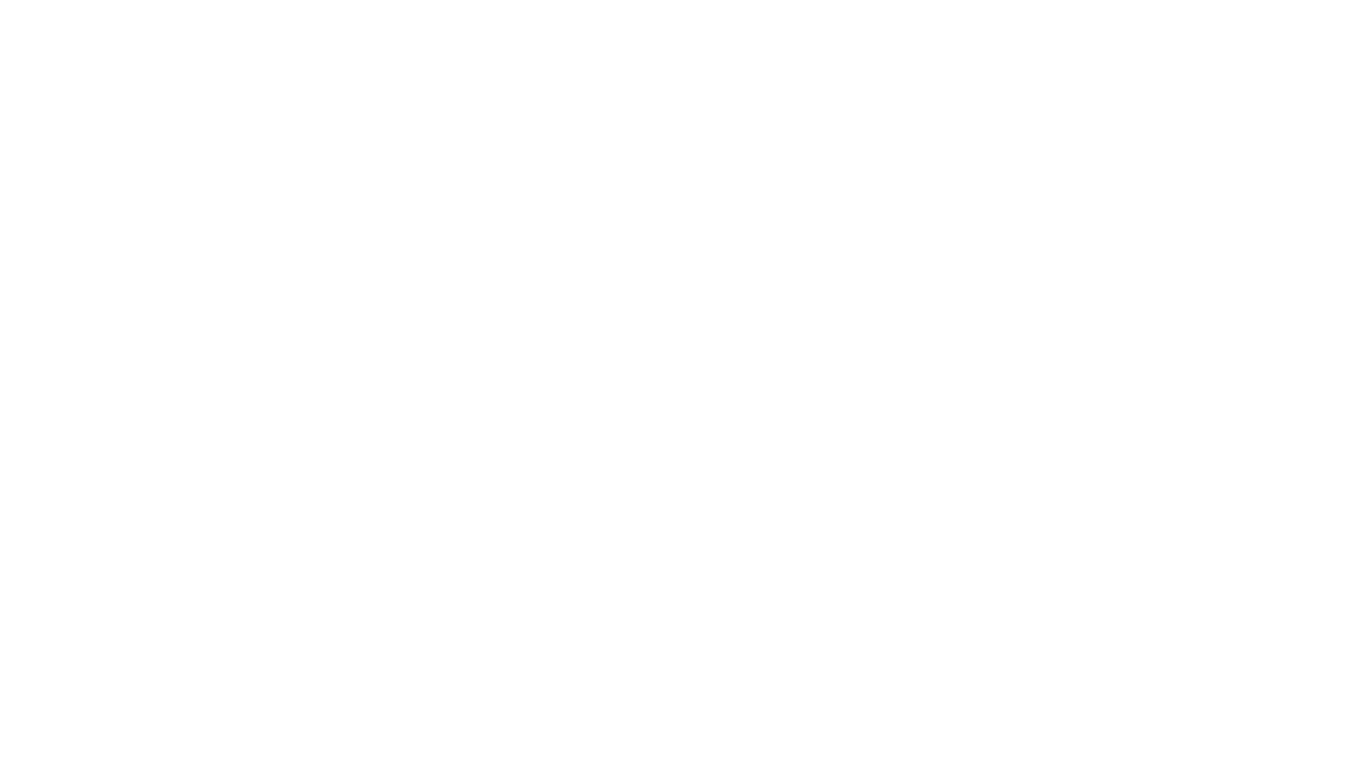
infinity
Infinity is an AI-native database designed for LLM applications, providing incredibly fast full-text and vector search capabilities. It supports a wide range of data types, including vectors, full-text, and structured data, and offers a fused search feature that combines multiple embeddings and full text. Infinity is easy to use, with an intuitive Python API and a single-binary architecture that simplifies deployment. It achieves high performance, with 0.1 milliseconds query latency on million-scale vector datasets and up to 15K QPS.
For similar jobs
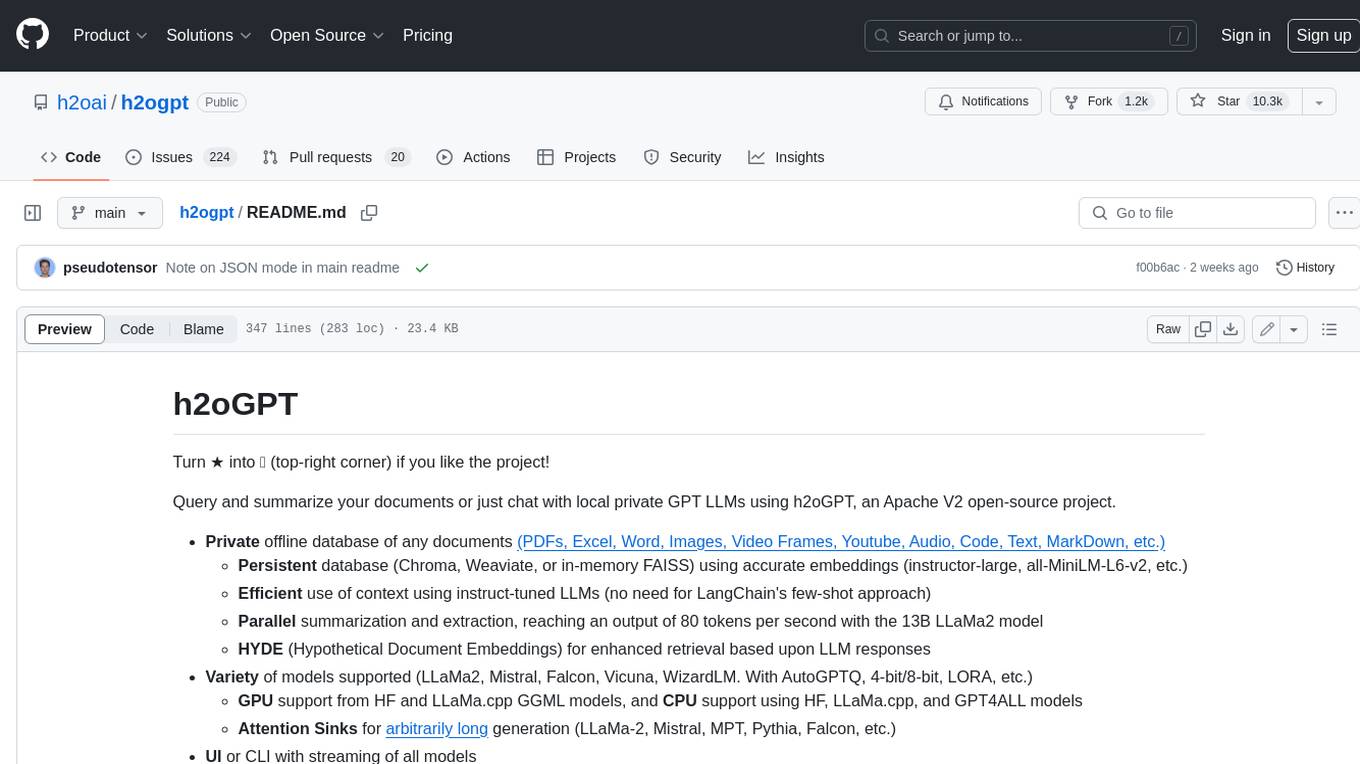
h2ogpt
h2oGPT is an Apache V2 open-source project that allows users to query and summarize documents or chat with local private GPT LLMs. It features a private offline database of any documents (PDFs, Excel, Word, Images, Video Frames, Youtube, Audio, Code, Text, MarkDown, etc.), a persistent database (Chroma, Weaviate, or in-memory FAISS) using accurate embeddings (instructor-large, all-MiniLM-L6-v2, etc.), and efficient use of context using instruct-tuned LLMs (no need for LangChain's few-shot approach). h2oGPT also offers parallel summarization and extraction, reaching an output of 80 tokens per second with the 13B LLaMa2 model, HYDE (Hypothetical Document Embeddings) for enhanced retrieval based upon LLM responses, a variety of models supported (LLaMa2, Mistral, Falcon, Vicuna, WizardLM. With AutoGPTQ, 4-bit/8-bit, LORA, etc.), GPU support from HF and LLaMa.cpp GGML models, and CPU support using HF, LLaMa.cpp, and GPT4ALL models. Additionally, h2oGPT provides Attention Sinks for arbitrarily long generation (LLaMa-2, Mistral, MPT, Pythia, Falcon, etc.), a UI or CLI with streaming of all models, the ability to upload and view documents through the UI (control multiple collaborative or personal collections), Vision Models LLaVa, Claude-3, Gemini-Pro-Vision, GPT-4-Vision, Image Generation Stable Diffusion (sdxl-turbo, sdxl) and PlaygroundAI (playv2), Voice STT using Whisper with streaming audio conversion, Voice TTS using MIT-Licensed Microsoft Speech T5 with multiple voices and Streaming audio conversion, Voice TTS using MPL2-Licensed TTS including Voice Cloning and Streaming audio conversion, AI Assistant Voice Control Mode for hands-free control of h2oGPT chat, Bake-off UI mode against many models at the same time, Easy Download of model artifacts and control over models like LLaMa.cpp through the UI, Authentication in the UI by user/password via Native or Google OAuth, State Preservation in the UI by user/password, Linux, Docker, macOS, and Windows support, Easy Windows Installer for Windows 10 64-bit (CPU/CUDA), Easy macOS Installer for macOS (CPU/M1/M2), Inference Servers support (oLLaMa, HF TGI server, vLLM, Gradio, ExLLaMa, Replicate, OpenAI, Azure OpenAI, Anthropic), OpenAI-compliant, Server Proxy API (h2oGPT acts as drop-in-replacement to OpenAI server), Python client API (to talk to Gradio server), JSON Mode with any model via code block extraction. Also supports MistralAI JSON mode, Claude-3 via function calling with strict Schema, OpenAI via JSON mode, and vLLM via guided_json with strict Schema, Web-Search integration with Chat and Document Q/A, Agents for Search, Document Q/A, Python Code, CSV frames (Experimental, best with OpenAI currently), Evaluate performance using reward models, and Quality maintained with over 1000 unit and integration tests taking over 4 GPU-hours.
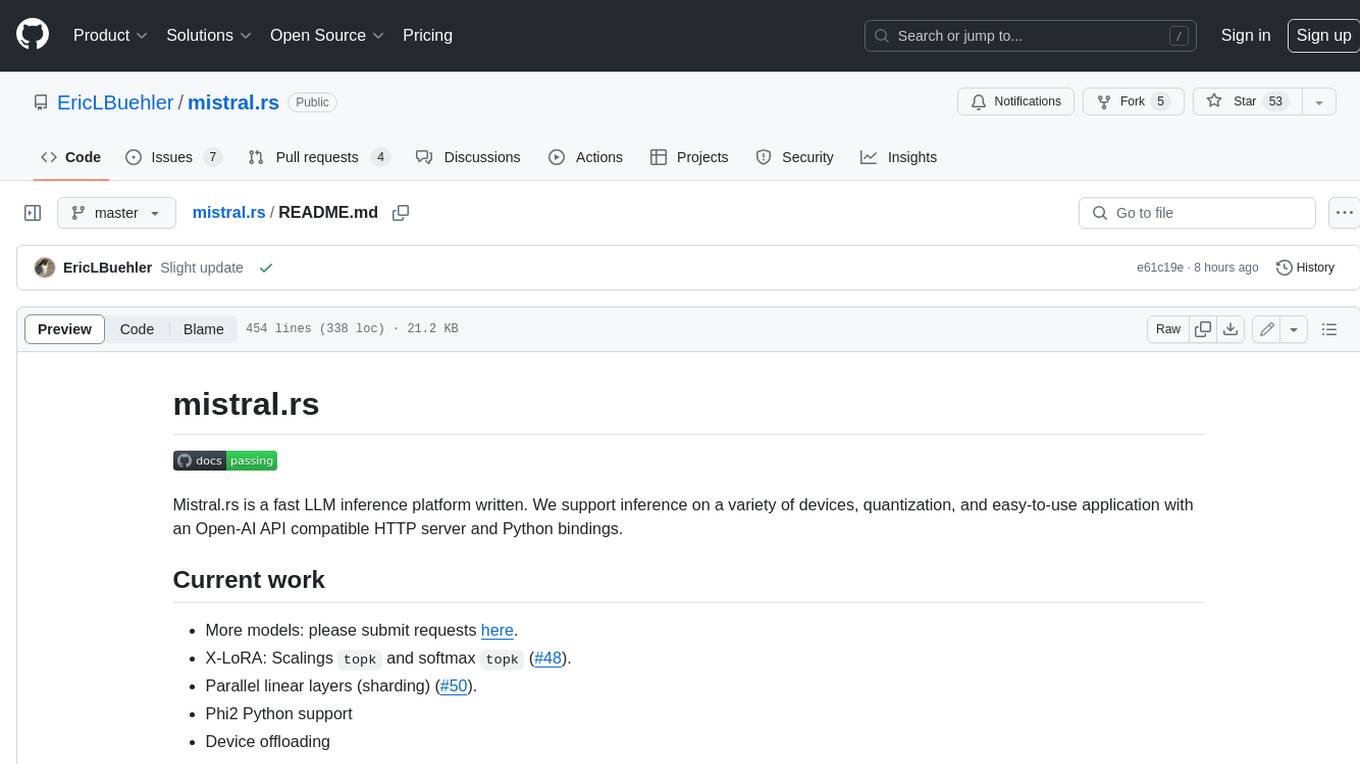
mistral.rs
Mistral.rs is a fast LLM inference platform written in Rust. We support inference on a variety of devices, quantization, and easy-to-use application with an Open-AI API compatible HTTP server and Python bindings.
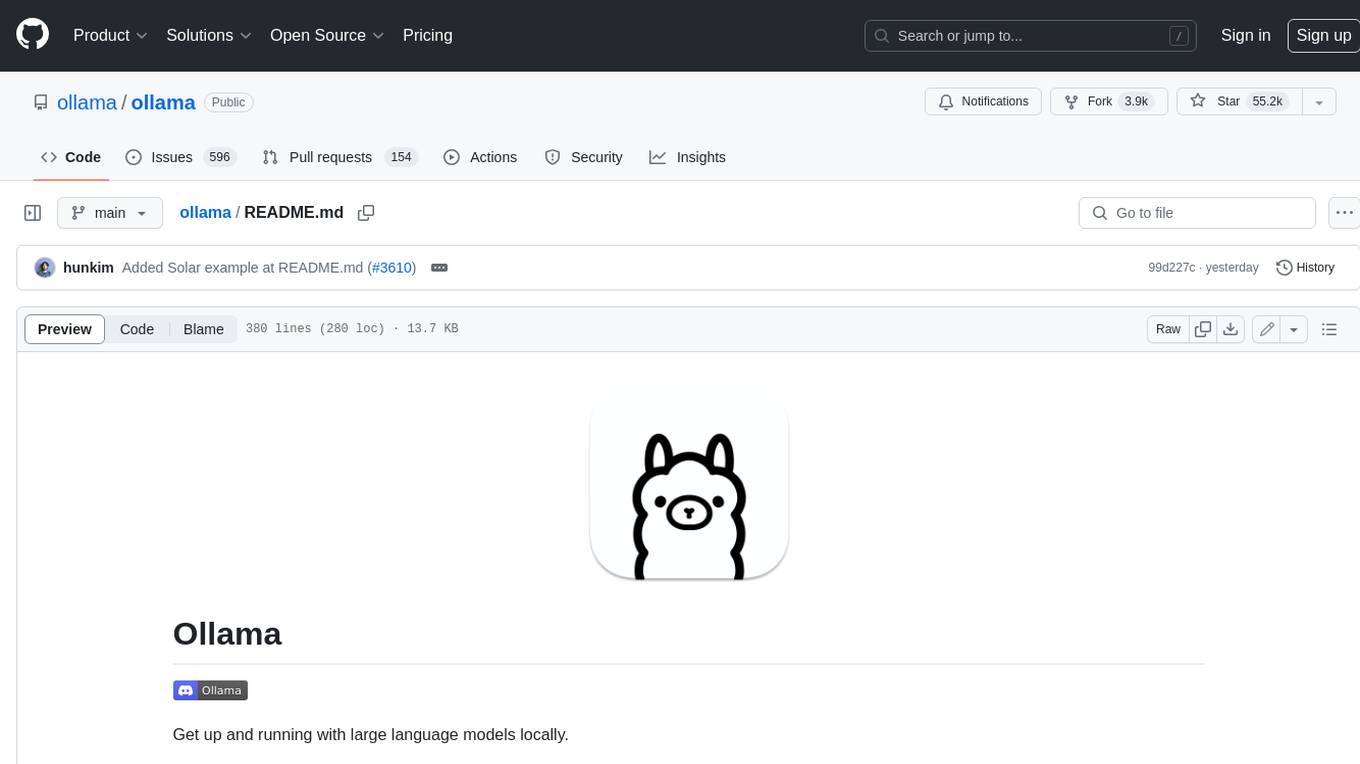
ollama
Ollama is a lightweight, extensible framework for building and running language models on the local machine. It provides a simple API for creating, running, and managing models, as well as a library of pre-built models that can be easily used in a variety of applications. Ollama is designed to be easy to use and accessible to developers of all levels. It is open source and available for free on GitHub.
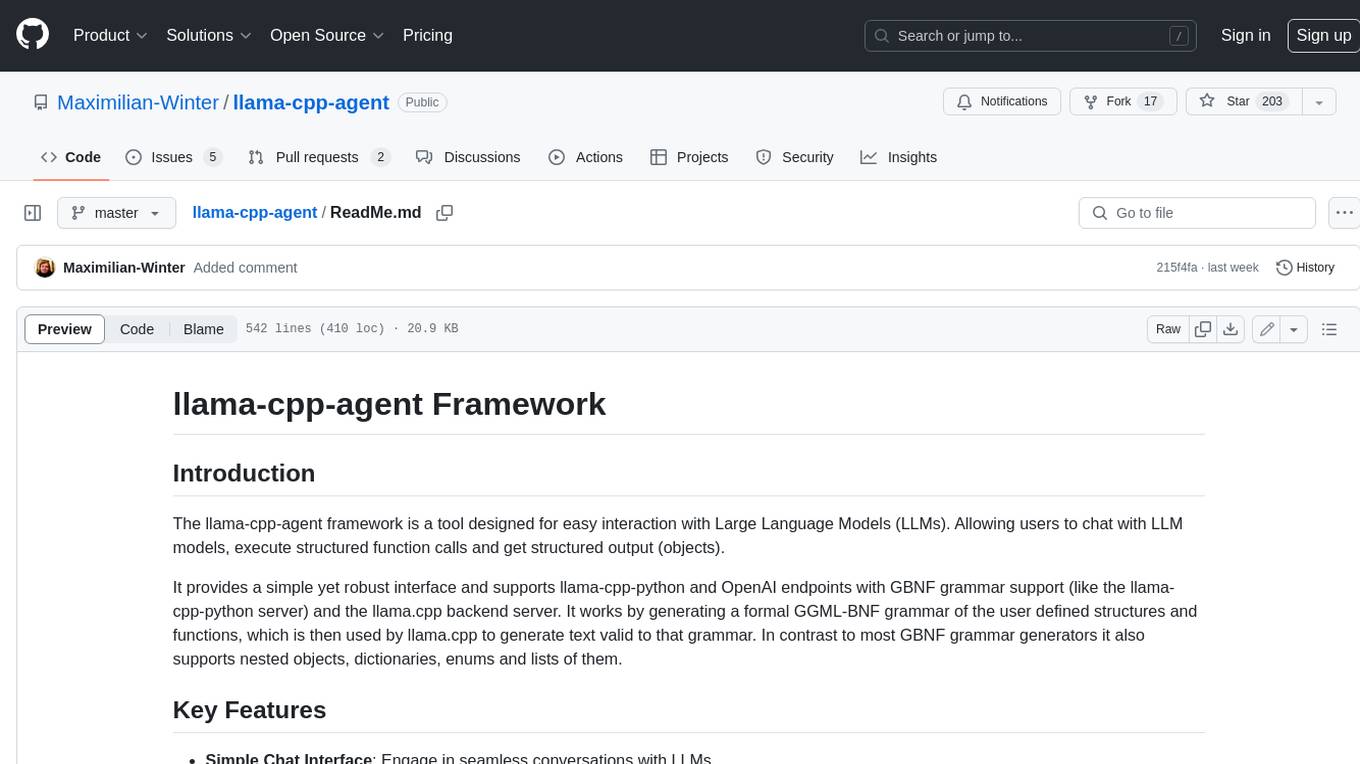
llama-cpp-agent
The llama-cpp-agent framework is a tool designed for easy interaction with Large Language Models (LLMs). Allowing users to chat with LLM models, execute structured function calls and get structured output (objects). It provides a simple yet robust interface and supports llama-cpp-python and OpenAI endpoints with GBNF grammar support (like the llama-cpp-python server) and the llama.cpp backend server. It works by generating a formal GGML-BNF grammar of the user defined structures and functions, which is then used by llama.cpp to generate text valid to that grammar. In contrast to most GBNF grammar generators it also supports nested objects, dictionaries, enums and lists of them.
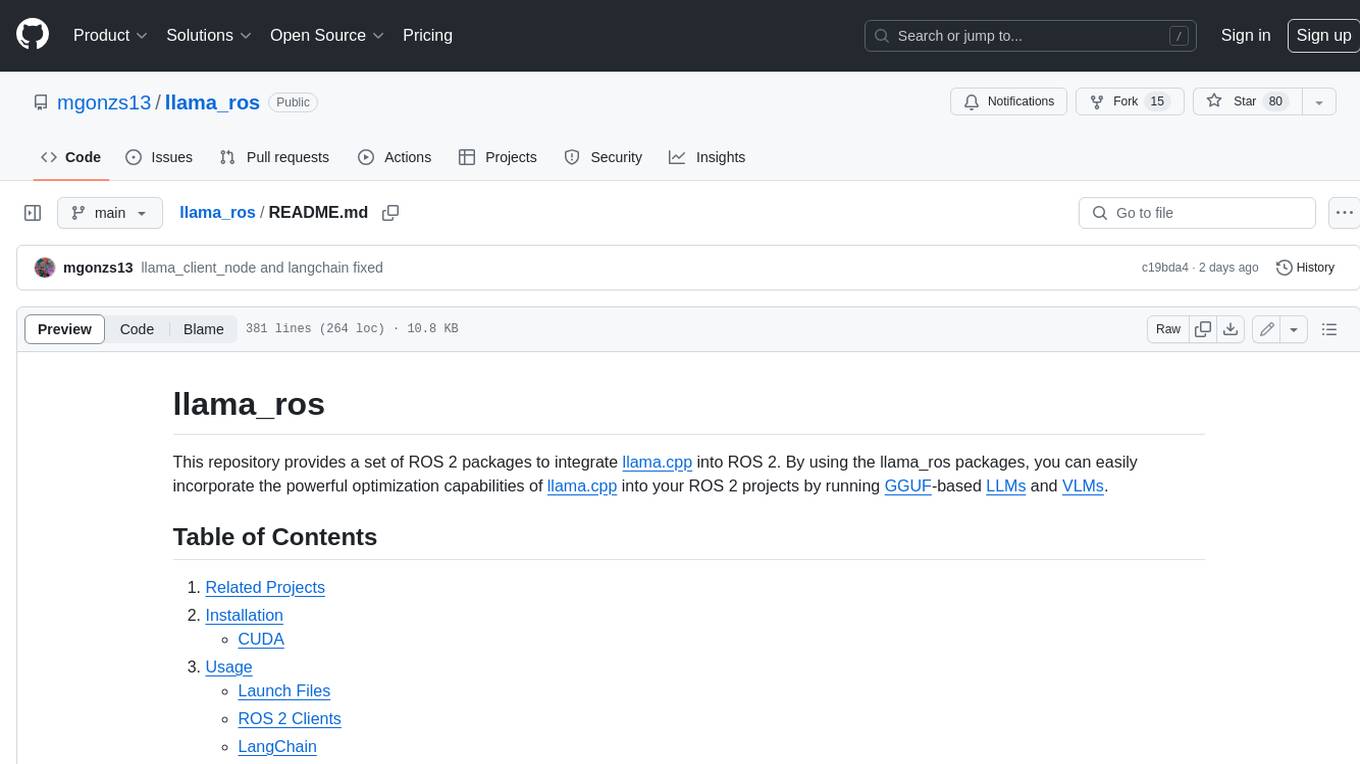
llama_ros
This repository provides a set of ROS 2 packages to integrate llama.cpp into ROS 2. By using the llama_ros packages, you can easily incorporate the powerful optimization capabilities of llama.cpp into your ROS 2 projects by running GGUF-based LLMs and VLMs.
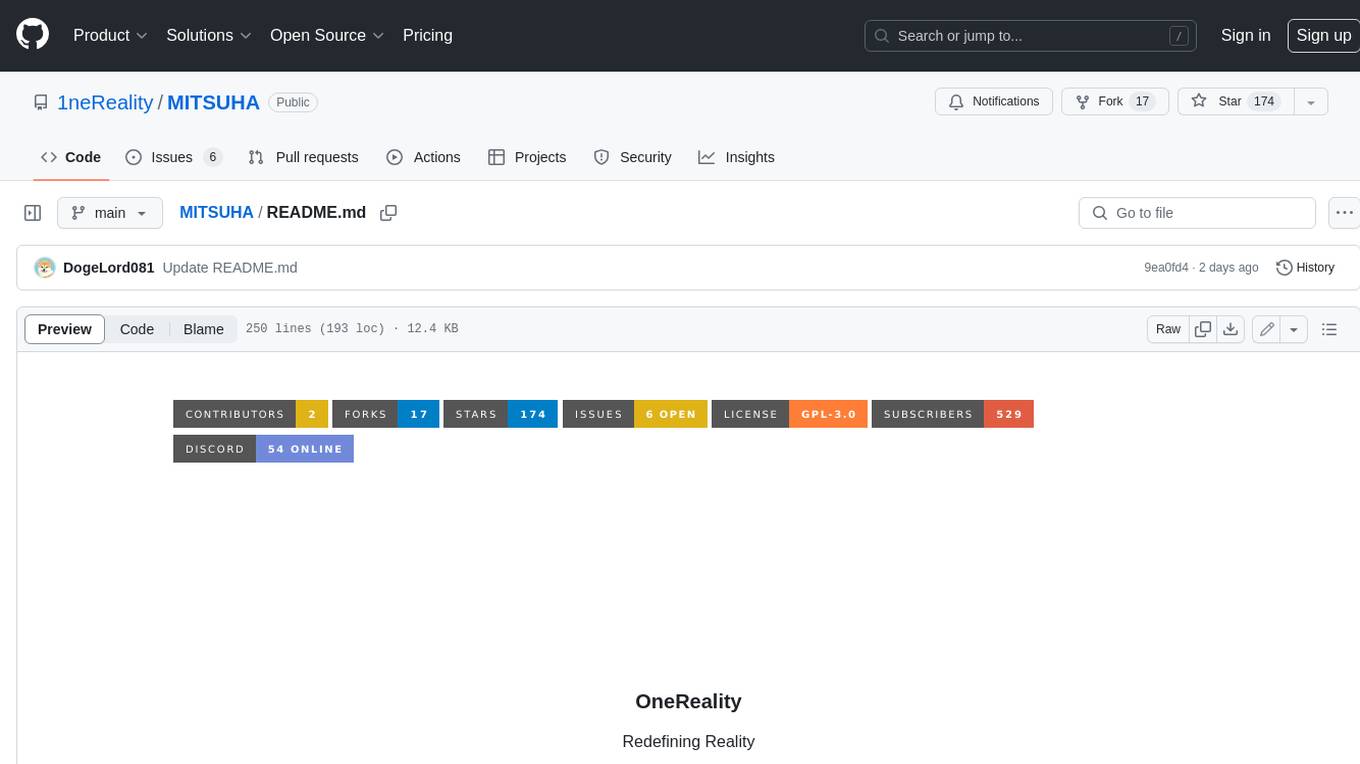
MITSUHA
OneReality is a virtual waifu/assistant that you can speak to through your mic and it'll speak back to you! It has many features such as: * You can speak to her with a mic * It can speak back to you * Has short-term memory and long-term memory * Can open apps * Smarter than you * Fluent in English, Japanese, Korean, and Chinese * Can control your smart home like Alexa if you set up Tuya (more info in Prerequisites) It is built with Python, Llama-cpp-python, Whisper, SpeechRecognition, PocketSphinx, VITS-fast-fine-tuning, VITS-simple-api, HyperDB, Sentence Transformers, and Tuya Cloud IoT.
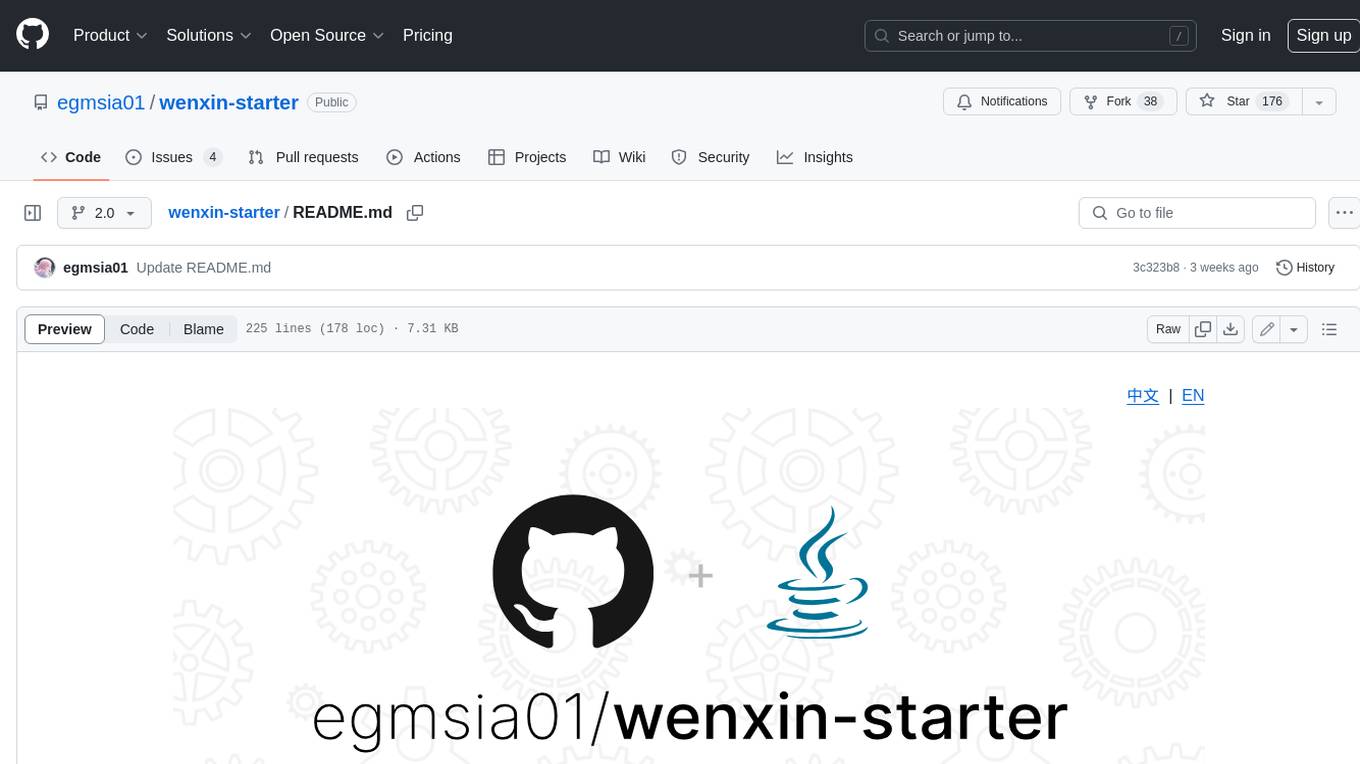
wenxin-starter
WenXin-Starter is a spring-boot-starter for Baidu's "Wenxin Qianfan WENXINWORKSHOP" large model, which can help you quickly access Baidu's AI capabilities. It fully integrates the official API documentation of Wenxin Qianfan. Supports text-to-image generation, built-in dialogue memory, and supports streaming return of dialogue. Supports QPS control of a single model and supports queuing mechanism. Plugins will be added soon.
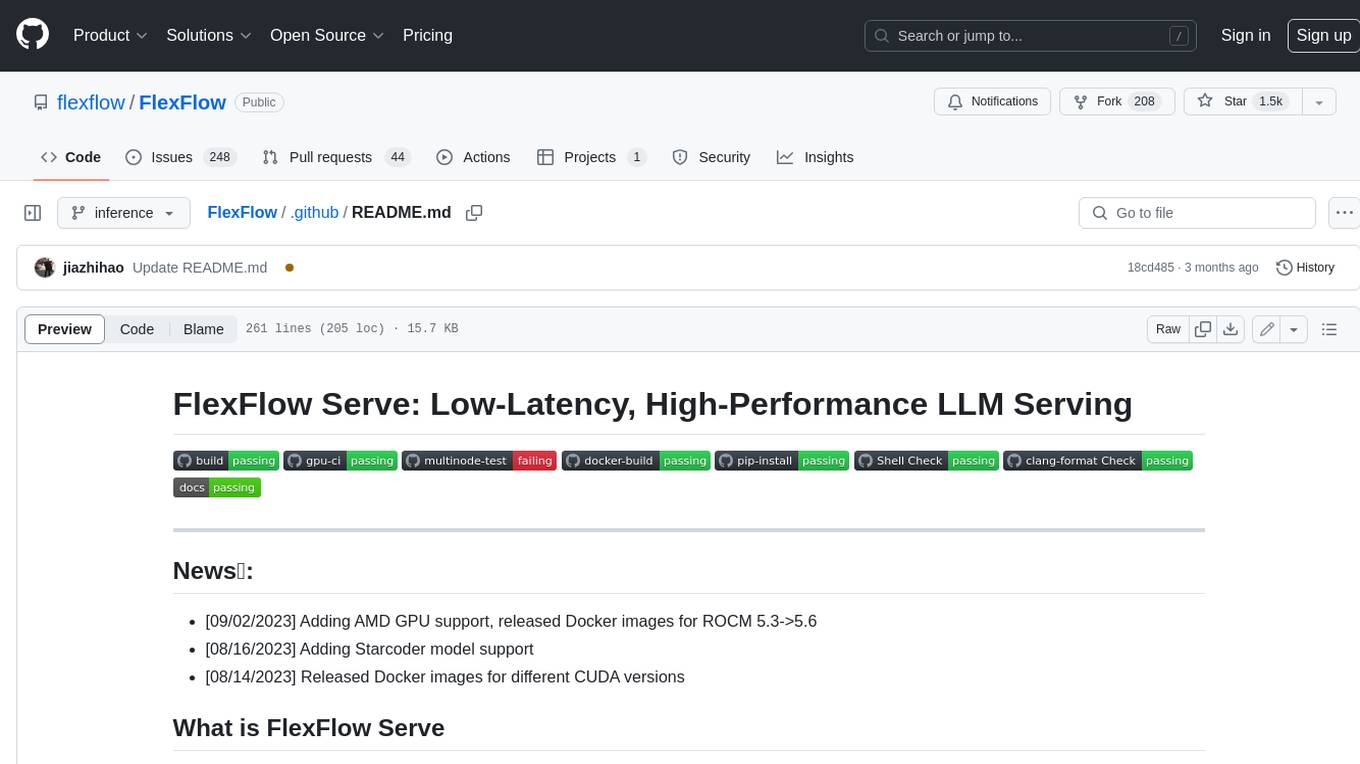
FlexFlow
FlexFlow Serve is an open-source compiler and distributed system for **low latency**, **high performance** LLM serving. FlexFlow Serve outperforms existing systems by 1.3-2.0x for single-node, multi-GPU inference and by 1.4-2.4x for multi-node, multi-GPU inference.