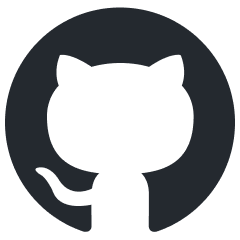
mediapipe-rs
The Google mediapipe AI library. Write AI inference applications for image recognition, text classification, audio / video processing and more, in Rust and run them in the secure WasmEdge sandbox. Zero Python dependency!
Stars: 143
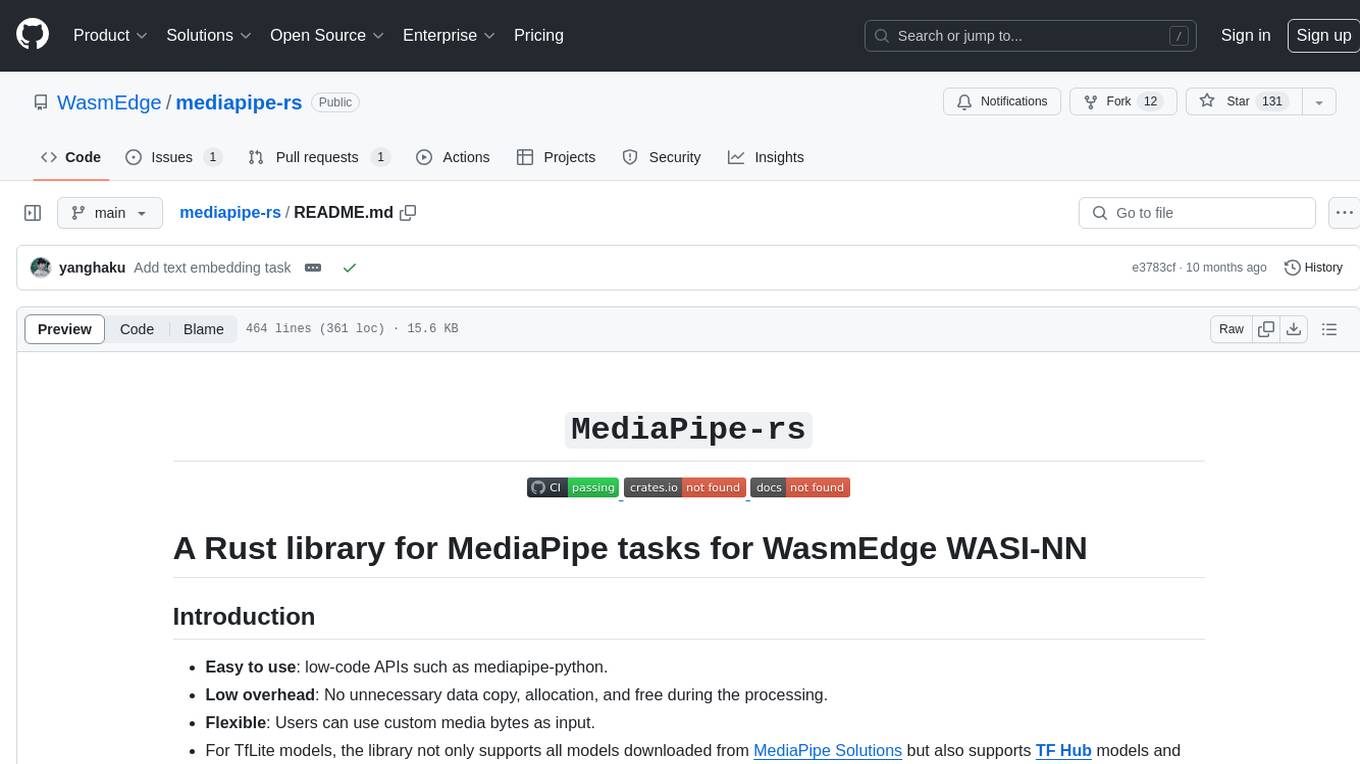
MediaPipe-rs is a Rust library designed for MediaPipe tasks on WasmEdge WASI-NN. It offers easy-to-use low-code APIs similar to mediapipe-python, with low overhead and flexibility for custom media input. The library supports various tasks like object detection, image classification, gesture recognition, and more, including TfLite models, TF Hub models, and custom models. Users can create task instances, run sessions for pre-processing, inference, and post-processing, and speed up processing by reusing sessions. The library also provides support for audio tasks using audio data from symphonia, ffmpeg, or raw audio. Users can choose between CPU, GPU, or TPU devices for processing.
README:
- Easy to use: low-code APIs such as mediapipe-python.
- Low overhead: No unnecessary data copy, allocation, and free during the processing.
- Flexible: Users can use custom media bytes as input.
- For TfLite models, the library not only supports all models downloaded from MediaPipe Solutions but also supports TF Hub models and custom models with essential information.
- [x] Object Detection
- [x] Image Classification
- [x] Image Segmentation
- [ ] Interactive Image Segmentation
- [x] Gesture Recognition
- [x] Hand Landmark Detection
- [x] Image Embedding
- [x] Face Detection
- [x] Face Landmark Detection
- [ ] Pose Landmark Detection
- [x] Audio Classification
- [x] Text Classification
- [x] Text Embedding
- [ ] Language Detection
Every task has three types: XxxBuilder
, Xxx
, XxxSession
. (Xxx
is the task name)
-
XxxBuilder
is used to create a task instanceXxx
, which has many options to set.example: use
ImageClassifierBuilder
to build aImageClassifier
task.let classifier = ImageClassifierBuilder::new() .max_results(3) // set max result .category_deny_list(vec!["denied label".into()]) // set deny list .gpu() // set running device .build_from_file(model_path)?; // create a image classifier
-
Xxx
is a task instance, which contains task information and model information.example: use
ImageClassifier
to create a newImageClassifierSession
let classifier_session = classifier.new_session()?;
-
XxxSession
is a running session to perform pre-process, inference, and post-process, which has buffers to store mid-results.example: use
ImageClassifierSession
to run the image classification task and return classification results:let classification_result = classifier_session.classify(&image::open(img_path)?)?;
Note: the session can be reused to speed up, if the code just uses the session once, it can use the task's wrapper function to simplify.
// let classifier_session = classifier.new_session()?; // let classification_result = classifier_session.classify(&image::open(img_path)?)?; // The above 2-line code is equal to: let classification_result = classifier.classify(&image::open(img_path)?)?;
- vision:
- gesture recognition:
GestureRecognizerBuilder
->GestureRecognizer
->GestureRecognizerSession
- hand detection:
HandDetectorBuilder
->HandDetector
->HandDetectorSession
- image classification:
ImageClassifierBuilder
->ImageClassifier
->ImageClassifierSession
- image embedding:
ImageEmbedderBuilder
->ImageEmbedder
->ImageEmbedderSession
- image segmentation:
ImageSegmenterBuilder
->ImageSegmenter
->ImageSegmenterSession
- object detection:
ObjectDetectorBuilder
->ObjectDetector
->ObjectDetectorSession
- face detection:
FaceDetectorBuilder
->FaceDetector
->FaceDetectorSession
- face landmark detection:
FaceLandmarkerBuilder
->FaceLandmarker
->FaceLandmarkerSession
- gesture recognition:
- audio:
- audio classification:
AudioClassifierBuilder
->AudioClassifier
->AudioClassifierSession
- audio classification:
- text:
- text classification:
TextClassifierBuilder
->TextClassifier
->TextClassifierSession
- text classification:
use mediapipe_rs::tasks::vision::ImageClassifierBuilder;
fn main() -> Result<(), Box<dyn std::error::Error>> {
let (model_path, img_path) = parse_args()?;
let classification_result = ImageClassifierBuilder::new()
.max_results(3) // set max result
.build_from_file(model_path)? // create a image classifier
.classify(&image::open(img_path)?)?; // do inference and generate results
// show formatted result message
println!("{}", classification_result);
Ok(())
}
Example input: (The image is downloaded from https://storage.googleapis.com/mediapipe-assets/burger.jpg)
Example output in console:
$ cargo run --release --example image_classification -- ./assets/models/image_classification/efficientnet_lite0_fp32.tflite ./assets/testdata/img/burger.jpg
Finished release [optimized] target(s) in 0.01s
Running `/mediapipe-rs/./scripts/wasmedge-runner.sh target/wasm32-wasi/release/examples/image_classification.wasm ./assets/models/image_classification/efficientnet_lite0_fp32.tflite ./assets/testdata/img/burger.jpg`
ClassificationResult:
Classification #0:
Category #0:
Category name: "cheeseburger"
Display name: None
Score: 0.70625573
Index: 933
use mediapipe_rs::postprocess::utils::draw_detection;
use mediapipe_rs::tasks::vision::ObjectDetectorBuilder;
fn main() -> Result<(), Box<dyn std::error::Error>> {
let (model_path, img_path, output_path) = parse_args()?;
let mut input_img = image::open(img_path)?;
let detection_result = ObjectDetectorBuilder::new()
.max_results(2) // set max result
.build_from_file(model_path)? // create a object detector
.detect(&input_img)?; // do inference and generate results
// show formatted result message
println!("{}", detection_result);
if let Some(output_path) = output_path {
// draw detection result to image
draw_detection(&mut input_img, &detection_result);
// save output image
input_img.save(output_path)?;
}
Ok(())
}
Example input: (The image is downloaded from https://storage.googleapis.com/mediapipe-tasks/object_detector/cat_and_dog.jpg)
Example output in console:
$ cargo run --release --example object_detection -- ./assets/models/object_detection/efficientdet_lite0_fp32.tflite ./assets/testdata/img/cat_and_dog.jpg
Finished release [optimized] target(s) in 0.00s
Running `/mediapipe-rs/./scripts/wasmedge-runner.sh target/wasm32-wasi/release/examples/object_detection.wasm ./assets/models/object_detection/efficientdet_lite0_fp32.tflite ./assets/testdata/img/cat_and_dog.jpg`
DetectionResult:
Detection #0:
Box: (left: 0.12283102, top: 0.38476586, right: 0.51069236, bottom: 0.851197)
Category #0:
Category name: "cat"
Display name: None
Score: 0.8460574
Index: 16
Detection #1:
Box: (left: 0.47926134, top: 0.06873521, right: 0.8711677, bottom: 0.87927735)
Category #0:
Category name: "dog"
Display name: None
Score: 0.8375256
Index: 17
fn main() -> Result<(), Box<dyn std::error::Error>> {
let model_path = parse_args()?;
let text_classifier = TextClassifierBuilder::new()
.max_results(1) // set max result
.build_from_file(model_path)?; // create a text classifier
let positive_str = "I love coding so much!";
let negative_str = "I don't like raining.";
// classify show formatted result message
let result = text_classifier.classify(&positive_str)?;
println!("`{}` -- {}", positive_str, result);
let result = text_classifier.classify(&negative_str)?;
println!("`{}` -- {}", negative_str, result);
Ok(())
}
Example output in console (use the bert model):
$ cargo run --release --example text_classification -- ./assets/models/text_classification/bert_text_classifier.tflite
Finished release [optimized] target(s) in 0.01s
Running `/mediapipe-rs/./scripts/wasmedge-runner.sh target/wasm32-wasi/release/examples/text_classification.wasm ./assets/models/text_classification/bert_text_classifier.tflite`
`I love coding so much!` -- ClassificationResult:
Classification #0:
Category #0:
Category name: "positive"
Display name: None
Score: 0.99990463
Index: 1
`I don't like raining.` -- ClassificationResult:
Classification #0:
Category #0:
Category name: "negative"
Display name: None
Score: 0.99541473
Index: 0
use mediapipe_rs::tasks::vision::GestureRecognizerBuilder;
fn main() -> Result<(), Box<dyn std::error::Error>> {
let (model_path, img_path) = parse_args()?;
let gesture_recognition_results = GestureRecognizerBuilder::new()
.num_hands(1) // set only recognition one hand
.max_results(1) // set max result
.build_from_file(model_path)? // create a task instance
.recognize(&image::open(img_path)?)?; // do inference and generate results
for g in gesture_recognition_results {
println!("{}", g.gestures.classifications[0].categories[0]);
}
Ok(())
}
Example input: (The image is download from https://storage.googleapis.com/mediapipe-tasks/gesture_recognizer/victory.jpg)
Example output in console:
$ cargo run --release --example gesture_recognition -- ./assets/models/gesture_recognition/gesture_recognizer.task ./assets/testdata/img/gesture_recognition_google_samples/victory.jpg
Finished release [optimized] target(s) in 0.02s
Running `/mediapipe-rs/./scripts/wasmedge-runner.sh target/wasm32-wasi/release/examples/gesture_recognition.wasm ./assets/models/gesture_recognition/gesture_recognizer.task ./assets/testdata/img/gesture_recognition_google_samples/victory.jpg`
Category name: "Victory"
Display name: None
Score: 0.9322255
Index: 6
use mediapipe_rs::tasks::vision::FaceLandmarkerBuilder;
use mediapipe_rs::postprocess::utils::DrawLandmarksOptions;
use mediapipe_rs::tasks::vision::FaceLandmarkConnections;
fn main() -> Result<(), Box<dyn std::error::Error>> {
let (model_path, img_path, output_path) = parse_args()?;
let mut input_img = image::open(img_path)?;
let face_landmark_results = FaceLandmarkerBuilder::new()
.num_faces(1) // set max number of faces to detect
.min_face_detection_confidence(0.5)
.min_face_presence_confidence(0.5)
.min_tracking_confidence(0.5)
.output_face_blendshapes(true)
.build_from_file(model_path)? // create a face landmarker
.detect(&input_img)?; // do inference and generate results
// show formatted result message
println!("{}", face_landmark_results);
if let Some(output_path) = output_path {
// draw face landmarks result to image
let options = DrawLandmarksOptions::default()
.connections(FaceLandmarkConnections::get_connections(
&FaceLandmarkConnections::FacemeshTesselation,
))
.landmark_radius_percent(0.003);
for result in face_landmark_results.iter() {
result.draw_with_options(&mut input_img, &options);
}
// save output image
input_img.save(output_path)?;
}
Ok(())
}
Example input: (The image is downloaded from https://storage.googleapis.com/mediapipe-assets/portrait.jpg)
Example output in console:
$ cargo run --release --example face_landmark -- ./assets/models/face_landmark/face_landmarker.task ./assets/testdata/img/face.jpg ./assets/doc/face_landmark_output.jpg
Finished release [optimized] target(s) in 4.50s
Running `./scripts/wasmedge-runner.sh target/wasm32-wasi/release/examples/face_landmark.wasm ./assets/models/face_landmark/face_landmarker.task ./assets/testdata/img/face.jpg ./assets/doc/face_landmark_output.jpg`
FaceLandmarkResult #0
Landmarks:
Normalized Landmark #0:
x: 0.49687287
y: 0.24964334
z: -0.029807145
Normalized Landmark #1:
x: 0.49801534
y: 0.22689381
z: -0.05928771
Normalized Landmark #2:
x: 0.49707597
y: 0.23421054
z: -0.03364953
Every audio media which implements the trait AudioData
can be used as audio tasks input.
Now the library has builtin implementation to support symphonia
, ffmpeg
, and raw audio data as input.
Examples for Audio Classification:
use mediapipe_rs::tasks::audio::AudioClassifierBuilder;
#[cfg(feature = "ffmpeg")]
use mediapipe_rs::preprocess::audio::FFMpegAudioData;
#[cfg(not(feature = "ffmpeg"))]
use mediapipe_rs::preprocess::audio::SymphoniaAudioData;
#[cfg(not(feature = "ffmpeg"))]
fn read_audio_using_symphonia(audio_path: String) -> SymphoniaAudioData {
let file = std::fs::File::open(audio_path).unwrap();
let probed = symphonia::default::get_probe()
.format(
&Default::default(),
symphonia::core::io::MediaSourceStream::new(Box::new(file), Default::default()),
&Default::default(),
&Default::default(),
)
.unwrap();
let codec_params = &probed.format.default_track().unwrap().codec_params;
let decoder = symphonia::default::get_codecs()
.make(codec_params, &Default::default())
.unwrap();
SymphoniaAudioData::new(probed.format, decoder)
}
#[cfg(feature = "ffmpeg")]
fn read_video_using_ffmpeg(audio_path: String) -> FFMpegAudioData {
ffmpeg_next::init().unwrap();
FFMpegAudioData::new(ffmpeg_next::format::input(&audio_path.as_str()).unwrap()).unwrap()
}
fn main() -> Result<(), Box<dyn std::error::Error>> {
let (model_path, audio_path) = parse_args()?;
#[cfg(not(feature = "ffmpeg"))]
let audio = read_audio_using_symphonia(audio_path);
#[cfg(feature = "ffmpeg")]
let audio = read_video_using_ffmpeg(audio_path);
let classification_results = AudioClassifierBuilder::new()
.max_results(3) // set max result
.build_from_file(model_path)? // create a task instance
.classify(audio)?; // do inference and generate results
// show formatted result message
for c in classification_results {
println!("{}", c);
}
Ok(())
}
The session includes inference sessions (such as TfLite interpreter), input and output buffers, etc. Explicitly using the session can reuse these resources to speed up.
Origin :
use mediapipe_rs::tasks::text::TextClassifier;
use mediapipe_rs::postprocess::ClassificationResult;
use mediapipe_rs::Error;
fn inference(
text_classifier: &TextClassifier,
inputs: &Vec<String>
) -> Result<Vec<ClassificationResult>, Error> {
let mut res = Vec::with_capacity(inputs.len());
for input in inputs {
// text_classifier will create new session every time
res.push(text_classifier.classify(input.as_str())?);
}
Ok(res)
}
Use the session to speed up:
use mediapipe_rs::tasks::text::TextClassifier;
use mediapipe_rs::postprocess::ClassificationResult;
use mediapipe_rs::Error;
fn inference(
text_classifier: &TextClassifier,
inputs: &Vec<String>
) -> Result<Vec<ClassificationResult>, Error> {
let mut res = Vec::with_capacity(inputs.len());
// only create one session and reuse the resources in session.
let mut session = text_classifier.new_session()?;
for input in inputs {
res.push(session.classify(input.as_str())?);
}
Ok(res)
}
When building the library with ffmpeg
feature using cargo, users must set the following environment variables:
-
FFMPEG_DIR
: the pre-built FFmpeg library path. You can download it from https://github.com/yanghaku/ffmpeg-wasm32-wasi/releases. -
WASI_SDK
or (WASI_SYSROOT
andCLANG_RT
), You can download it from https://github.com/WebAssembly/wasi-sdk/releases -
BINDGEN_EXTRA_CLANG_ARGS
: set sysroot and target and function visibility for libclang. (The sysroot must be absolute path).
Example:
export FFMPEG_DIR=/path/to/ffmpeg/library
export WASI_SDK=/opt/wasi-sdk
export BINDGEN_EXTRA_CLANG_ARGS="--sysroot=/opt/wasi-sdk/share/wasi-sysroot --target=wasm32-wasi -fvisibility=default"
# Then run cargo
The default device is CPU, and user can use APIs to choose device to use:
use mediapipe_rs::tasks::vision::ObjectDetectorBuilder;
fn create_gpu(model_blob: Vec<u8>) {
let detector_gpu = ObjectDetectorBuilder::new()
.gpu()
.build_from_buffer(model_blob)
.unwrap();
}
fn create_tpu(model_blob: Vec<u8>) {
let detector_tpu = ObjectDetectorBuilder::new()
.tpu()
.build_from_buffer(model_blob)
.unwrap();
}
This work is made possible by Google's work on Mediapipe.
- LFX Workspace: A Rust library crate for mediapipe models for WasmEdge NN
- WasmEdge
- MediaPipe
- wasi-nn safe
- wasi-nn specification
- wasi-nn
This project is licensed under the Apache 2.0 license. See LICENSE for more details.
For Tasks:
Click tags to check more tools for each tasksFor Jobs:
Alternative AI tools for mediapipe-rs
Similar Open Source Tools
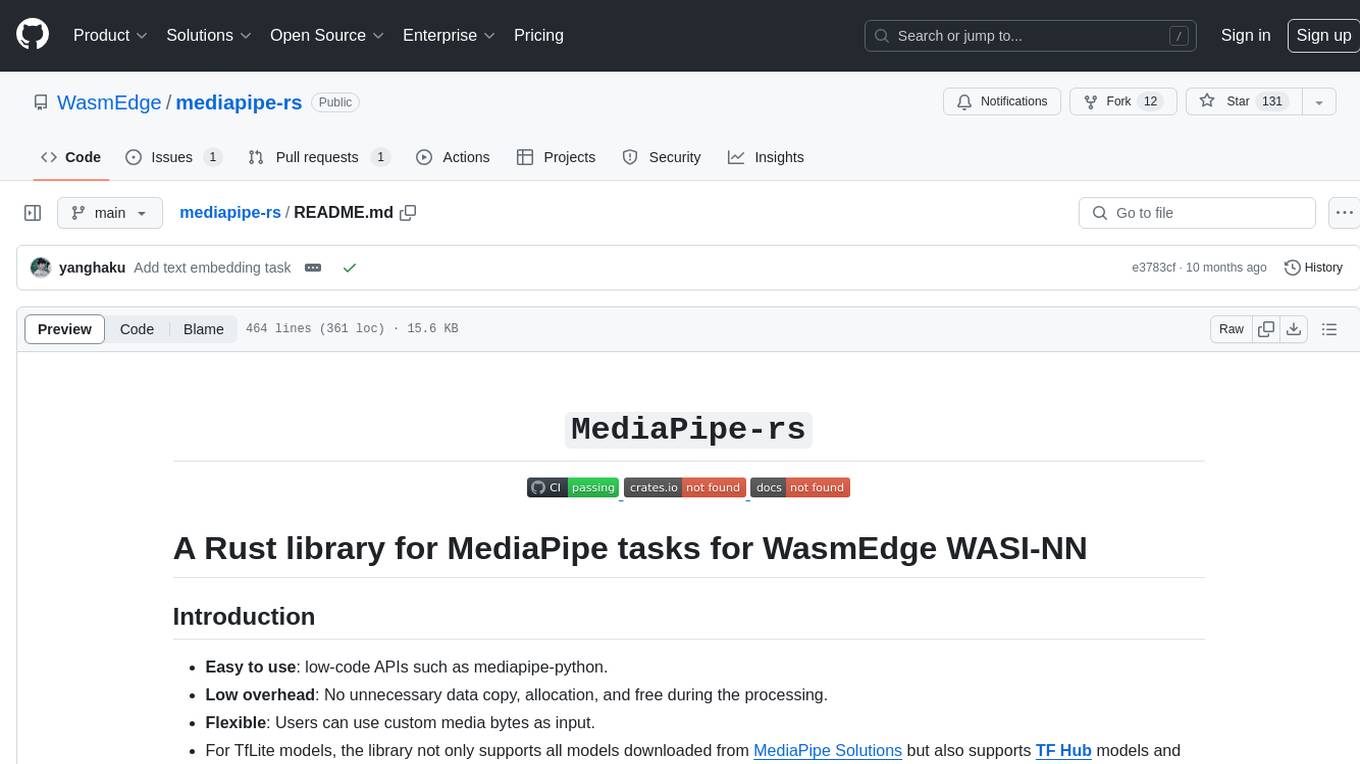
mediapipe-rs
MediaPipe-rs is a Rust library designed for MediaPipe tasks on WasmEdge WASI-NN. It offers easy-to-use low-code APIs similar to mediapipe-python, with low overhead and flexibility for custom media input. The library supports various tasks like object detection, image classification, gesture recognition, and more, including TfLite models, TF Hub models, and custom models. Users can create task instances, run sessions for pre-processing, inference, and post-processing, and speed up processing by reusing sessions. The library also provides support for audio tasks using audio data from symphonia, ffmpeg, or raw audio. Users can choose between CPU, GPU, or TPU devices for processing.
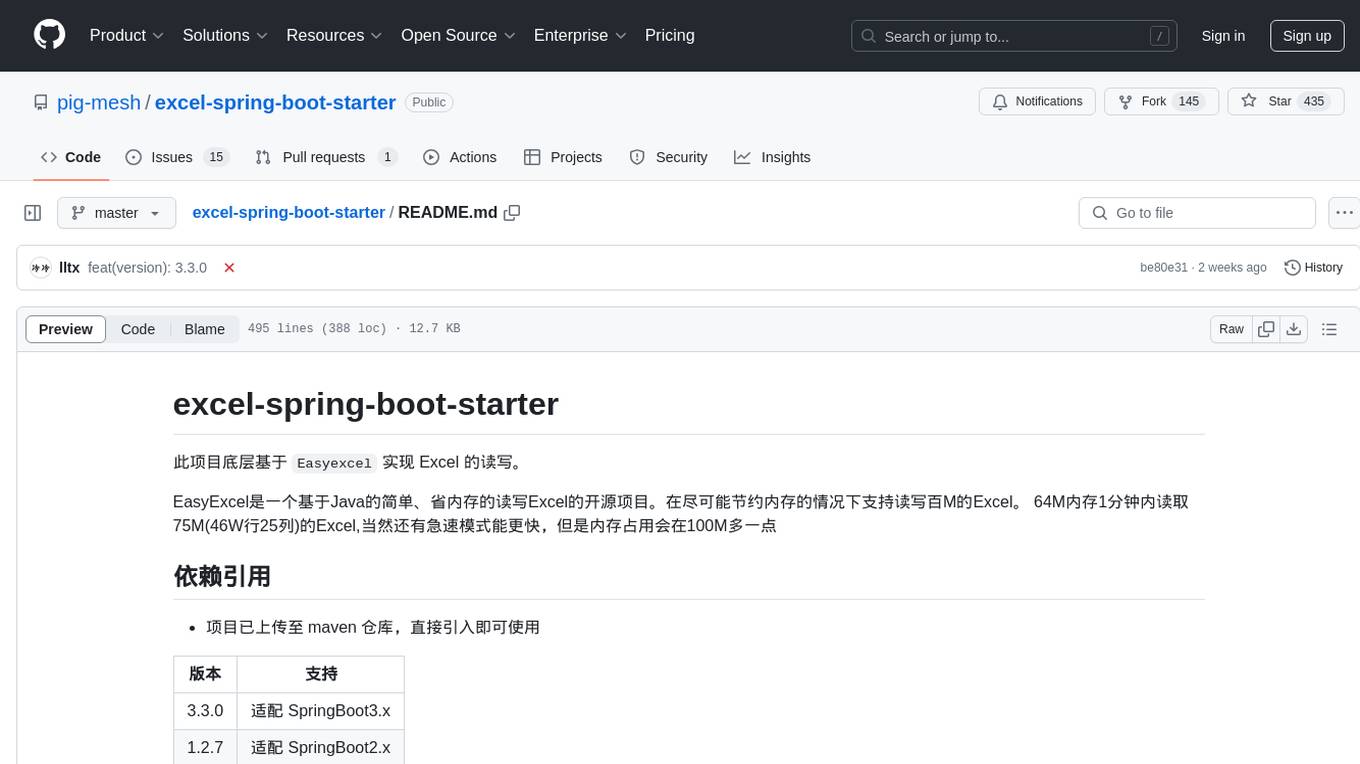
excel-spring-boot-starter
The excel-spring-boot-starter project is based on Easyexcel to implement reading and writing Excel files. EasyExcel is an open-source project for simple and memory-efficient reading and writing of Excel files in Java. It supports reading and writing Excel files up to 75M (46W rows 25 columns) in 1 minute with 64M memory, and there is a fast mode for even quicker performance but with slightly more memory consumption.
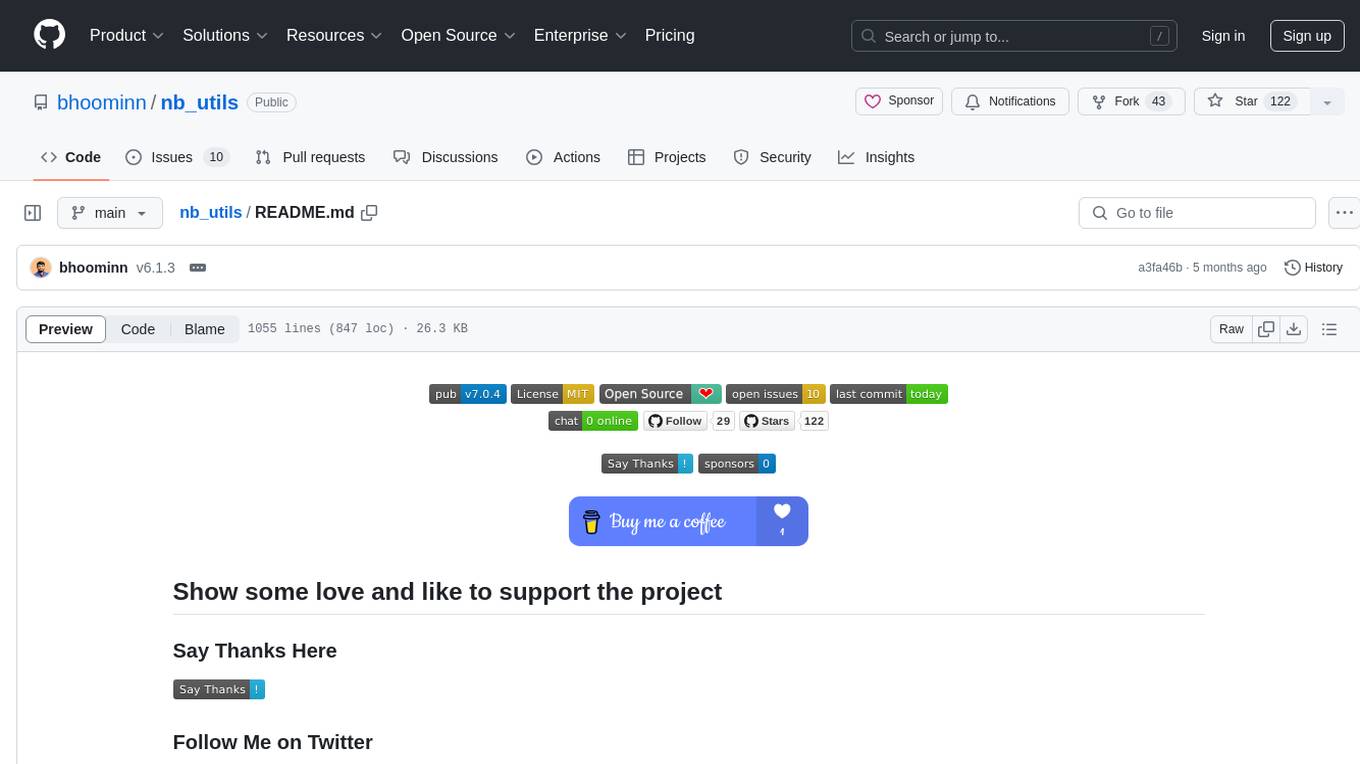
nb_utils
nb_utils is a Flutter package that provides a collection of useful methods, extensions, widgets, and utilities to simplify Flutter app development. It includes features like shared preferences, text styles, decorations, widgets, extensions for strings, colors, build context, date time, device, numbers, lists, scroll controllers, system methods, network utils, JWT decoding, and custom dialogs. The package aims to enhance productivity and streamline common tasks in Flutter development.
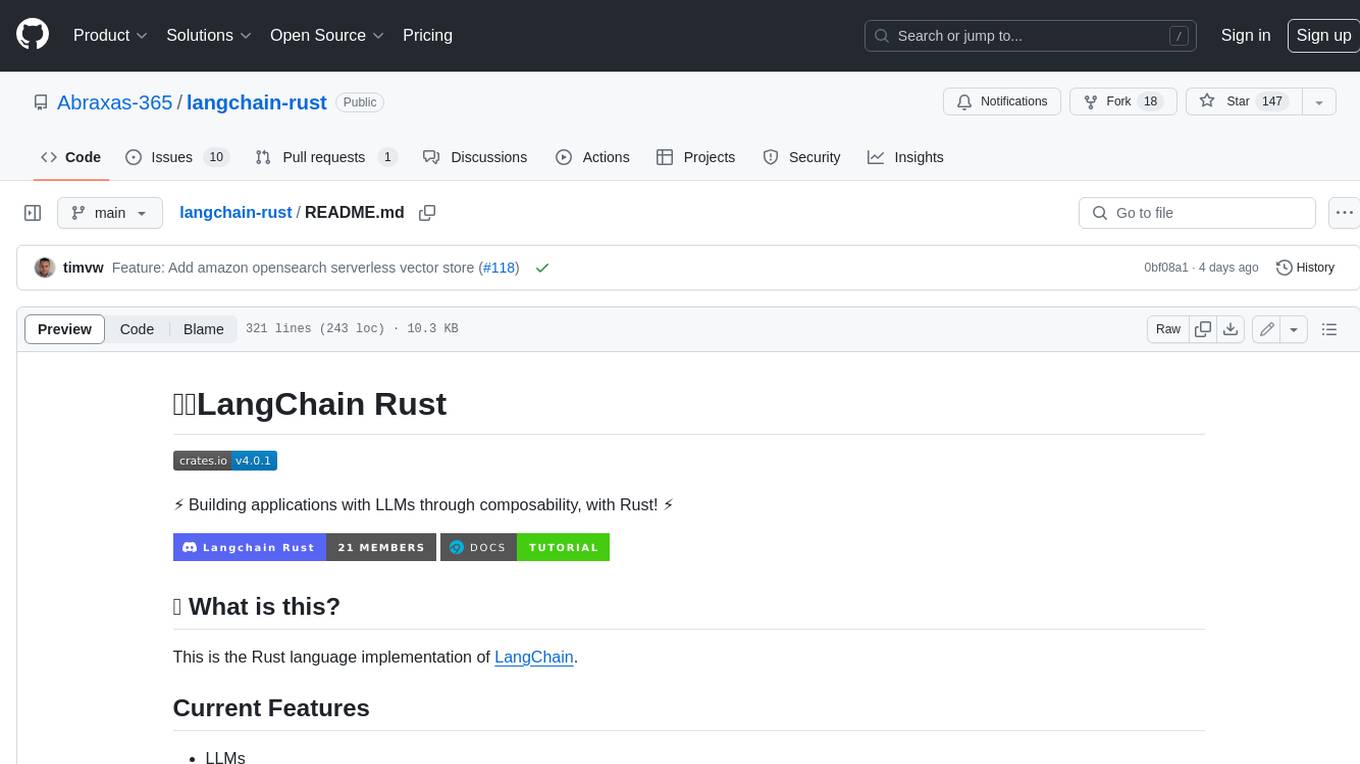
langchain-rust
LangChain Rust is a library for building applications with Large Language Models (LLMs) through composability. It provides a set of tools and components that can be used to create conversational agents, document loaders, and other applications that leverage LLMs. LangChain Rust supports a variety of LLMs, including OpenAI, Azure OpenAI, Ollama, and Anthropic Claude. It also supports a variety of embeddings, vector stores, and document loaders. LangChain Rust is designed to be easy to use and extensible, making it a great choice for developers who want to build applications with LLMs.
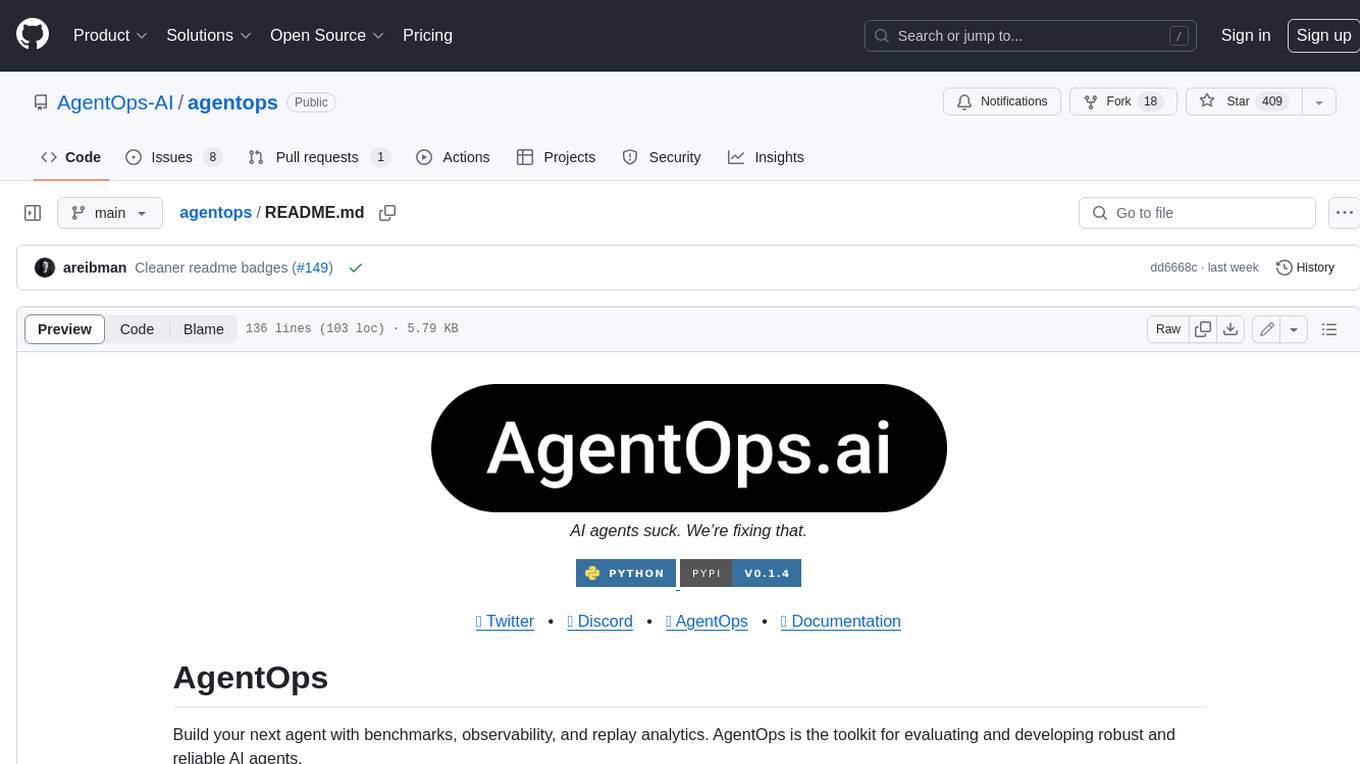
agentops
AgentOps is a toolkit for evaluating and developing robust and reliable AI agents. It provides benchmarks, observability, and replay analytics to help developers build better agents. AgentOps is open beta and can be signed up for here. Key features of AgentOps include: - Session replays in 3 lines of code: Initialize the AgentOps client and automatically get analytics on every LLM call. - Time travel debugging: (coming soon!) - Agent Arena: (coming soon!) - Callback handlers: AgentOps works seamlessly with applications built using Langchain and LlamaIndex.
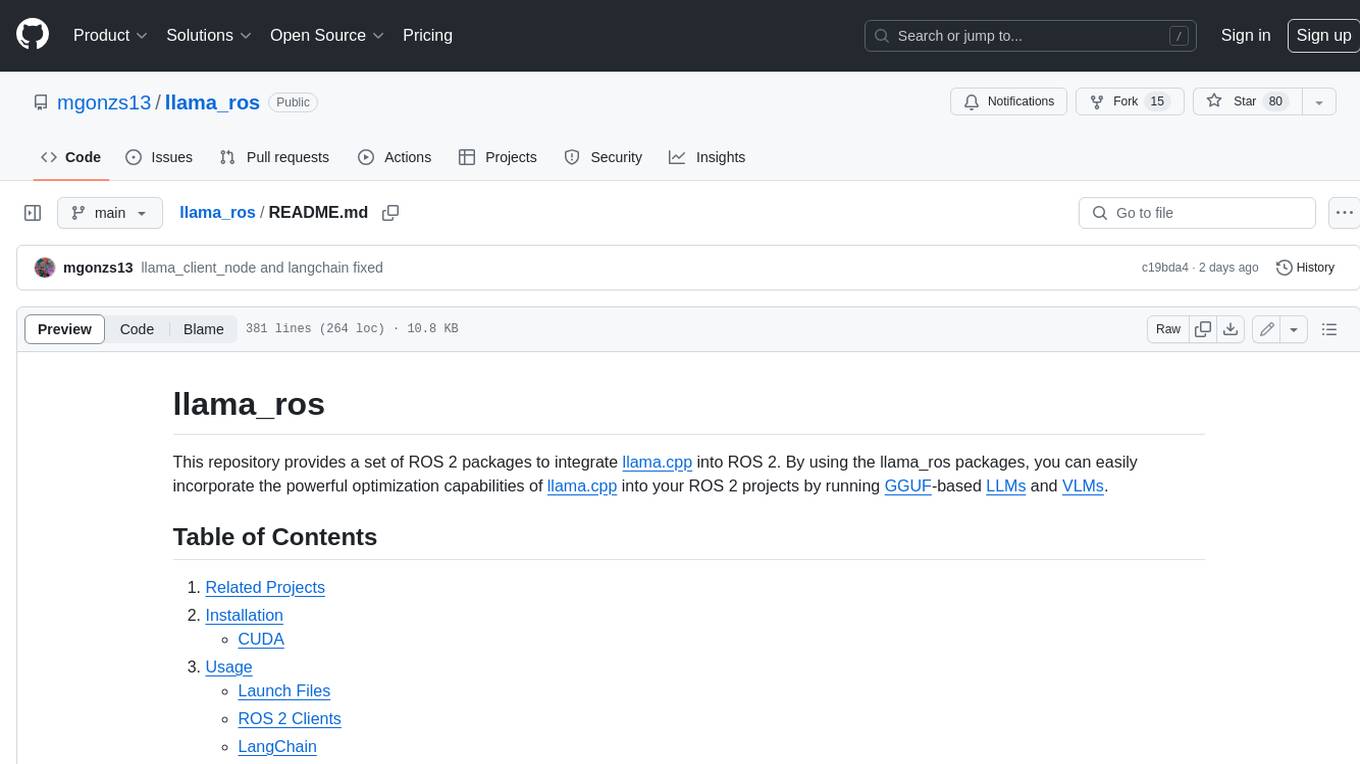
llama_ros
This repository provides a set of ROS 2 packages to integrate llama.cpp into ROS 2. By using the llama_ros packages, you can easily incorporate the powerful optimization capabilities of llama.cpp into your ROS 2 projects by running GGUF-based LLMs and VLMs.
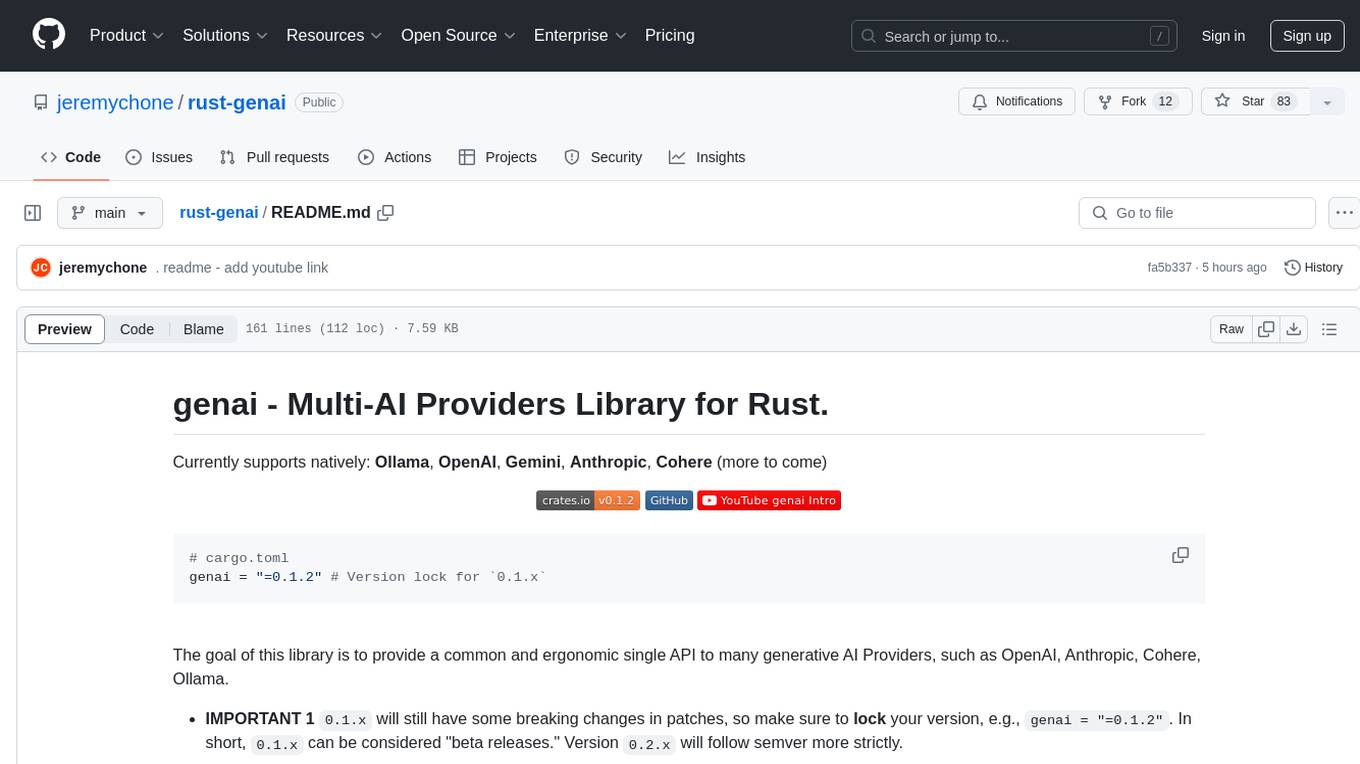
rust-genai
genai is a multi-AI providers library for Rust that aims to provide a common and ergonomic single API to various generative AI providers such as OpenAI, Anthropic, Cohere, Ollama, and Gemini. It focuses on standardizing chat completion APIs across major AI services, prioritizing ergonomics and commonality. The library initially focuses on text chat APIs and plans to expand to support images, function calling, and more in the future versions. Version 0.1.x will have breaking changes in patches, while version 0.2.x will follow semver more strictly. genai does not provide a full representation of a given AI provider but aims to simplify the differences at a lower layer for ease of use.
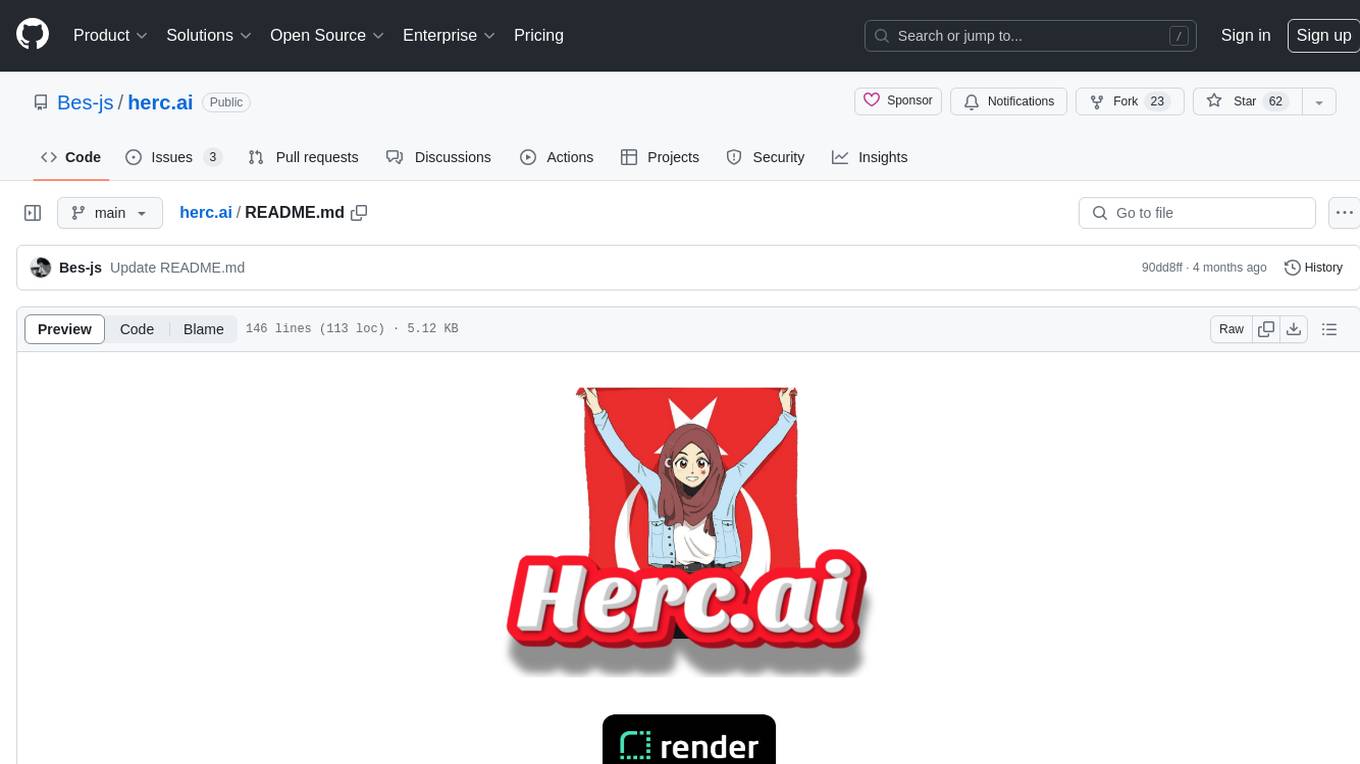
herc.ai
Herc.ai is a powerful library for interacting with the Herc.ai API. It offers free access to users and supports all languages. Users can benefit from Herc.ai's features unlimitedly with a one-time subscription and API key. The tool provides functionalities for question answering and text-to-image generation, with support for various models and customization options. Herc.ai can be easily integrated into CLI, CommonJS, TypeScript, and supports beta models for advanced usage. Developed by FiveSoBes and Luppux Development.
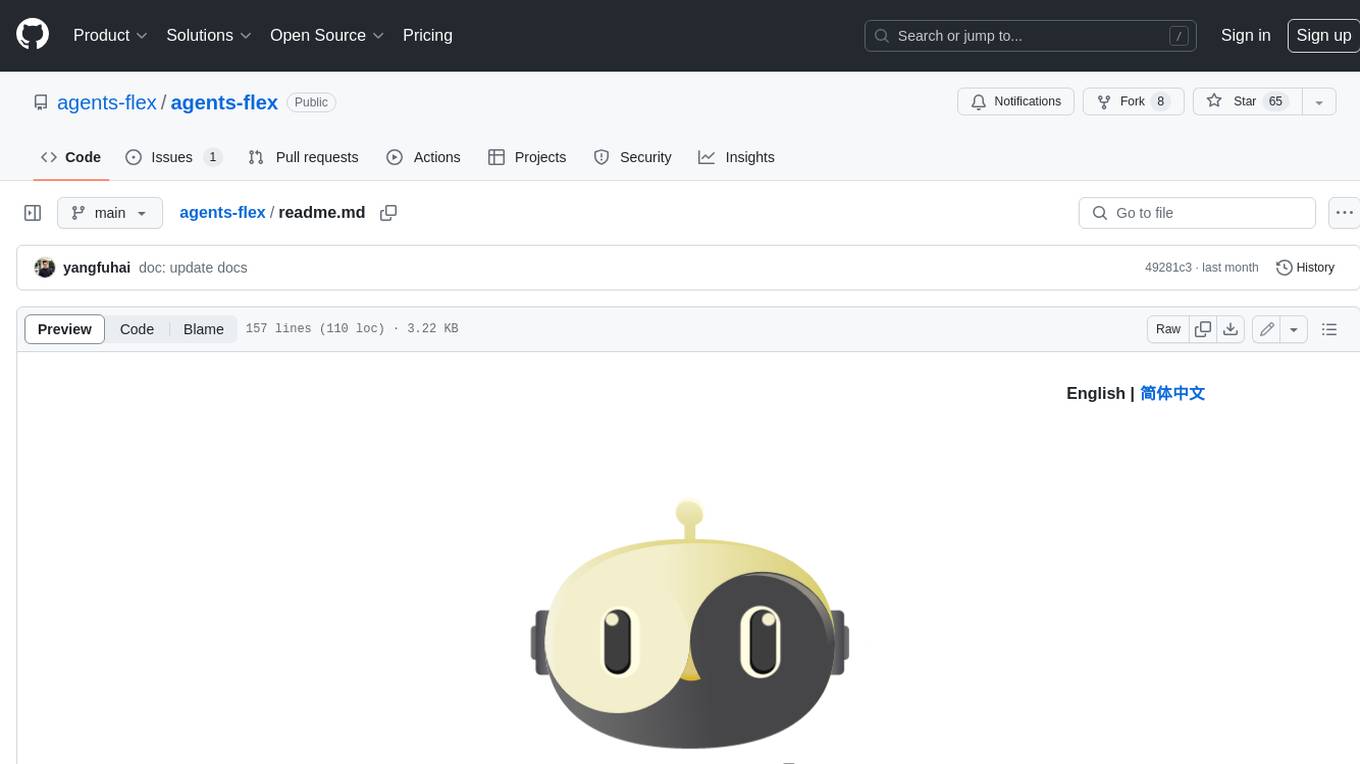
agents-flex
Agents-Flex is a LLM Application Framework like LangChain base on Java. It provides a set of tools and components for building LLM applications, including LLM Visit, Prompt and Prompt Template Loader, Function Calling Definer, Invoker and Running, Memory, Embedding, Vector Storage, Resource Loaders, Document, Splitter, Loader, Parser, LLMs Chain, and Agents Chain.
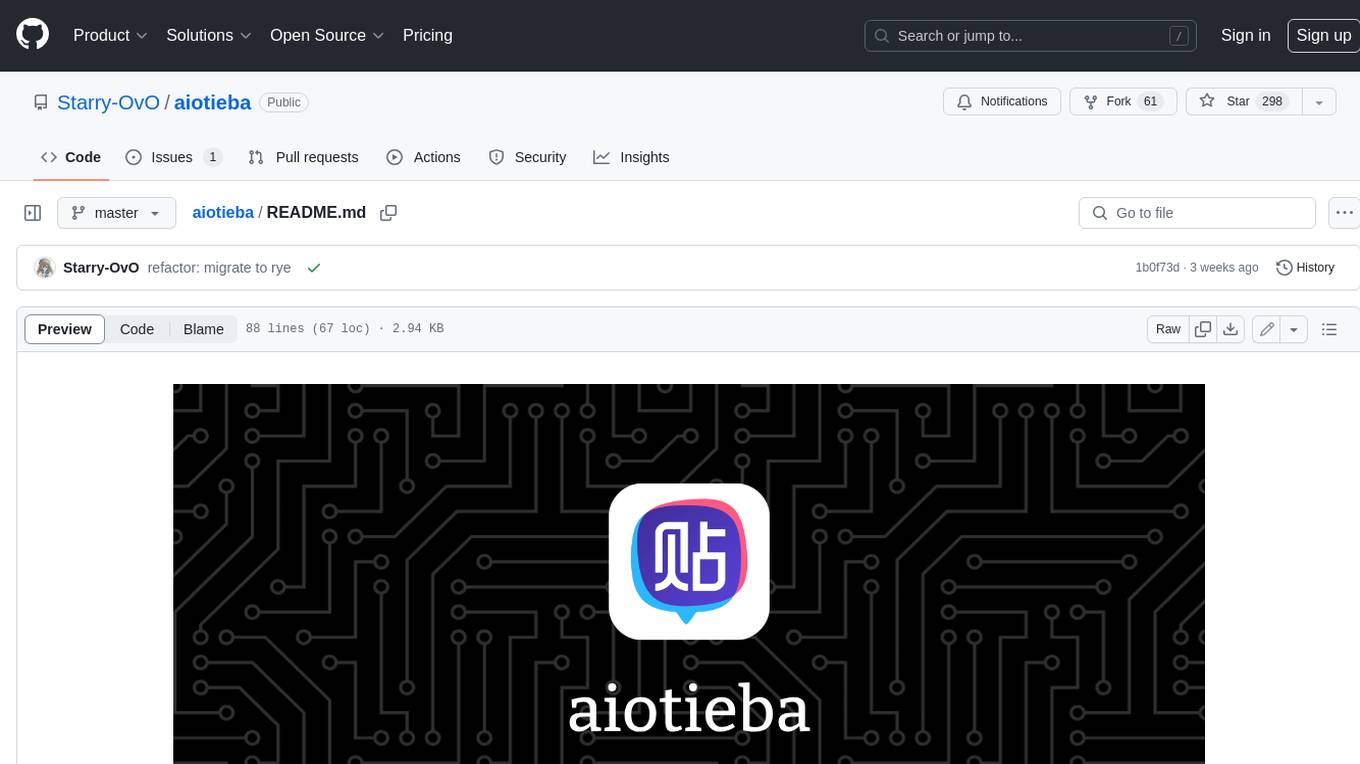
aiotieba
Aiotieba is an asynchronous Python library for interacting with the Tieba API. It provides a comprehensive set of features for working with Tieba, including support for authentication, thread and post management, and image and file uploading. Aiotieba is well-documented and easy to use, making it a great choice for developers who want to build applications that interact with Tieba.
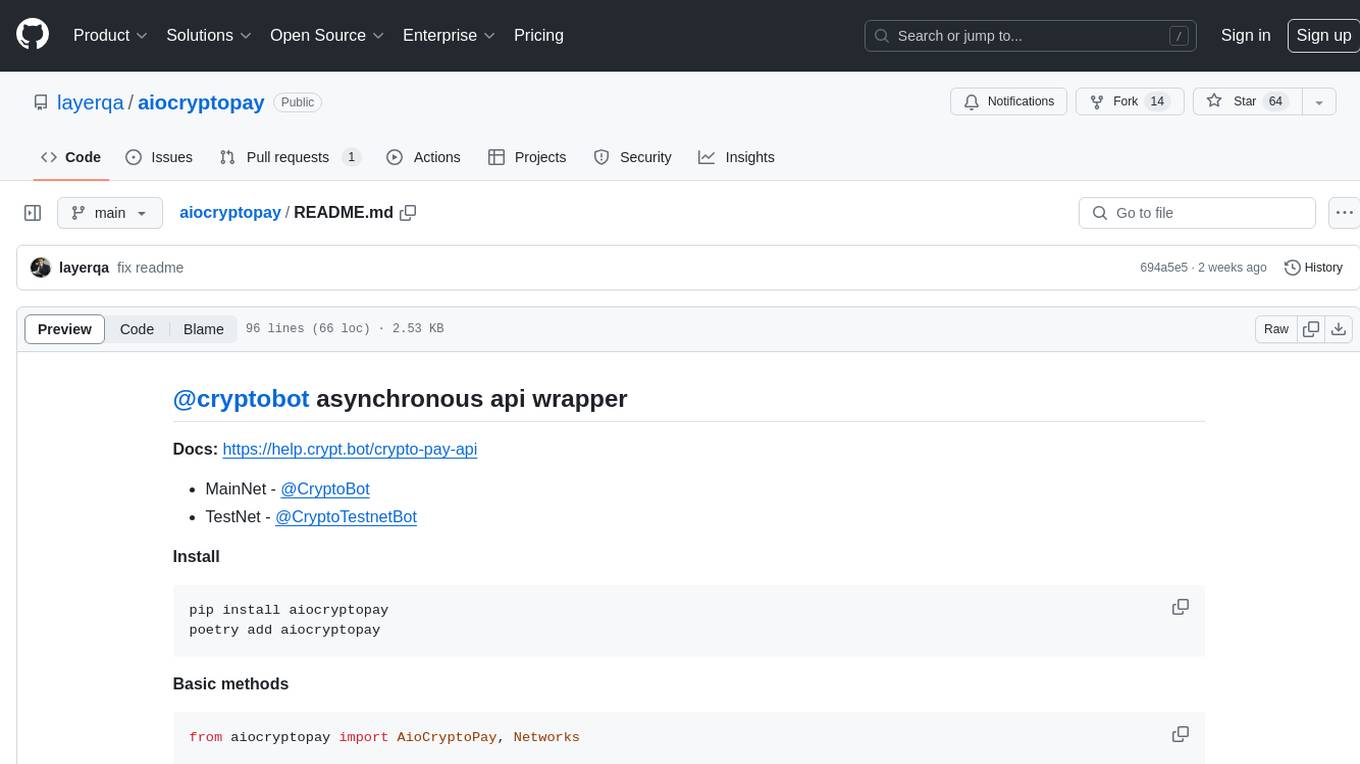
aiocryptopay
The aiocryptopay repository is an asynchronous API wrapper for interacting with the @cryptobot and @CryptoTestnetBot APIs. It provides methods for creating, getting, and deleting invoices and checks, as well as handling webhooks for invoice payments. Users can easily integrate this tool into their applications to manage cryptocurrency payments and transactions.
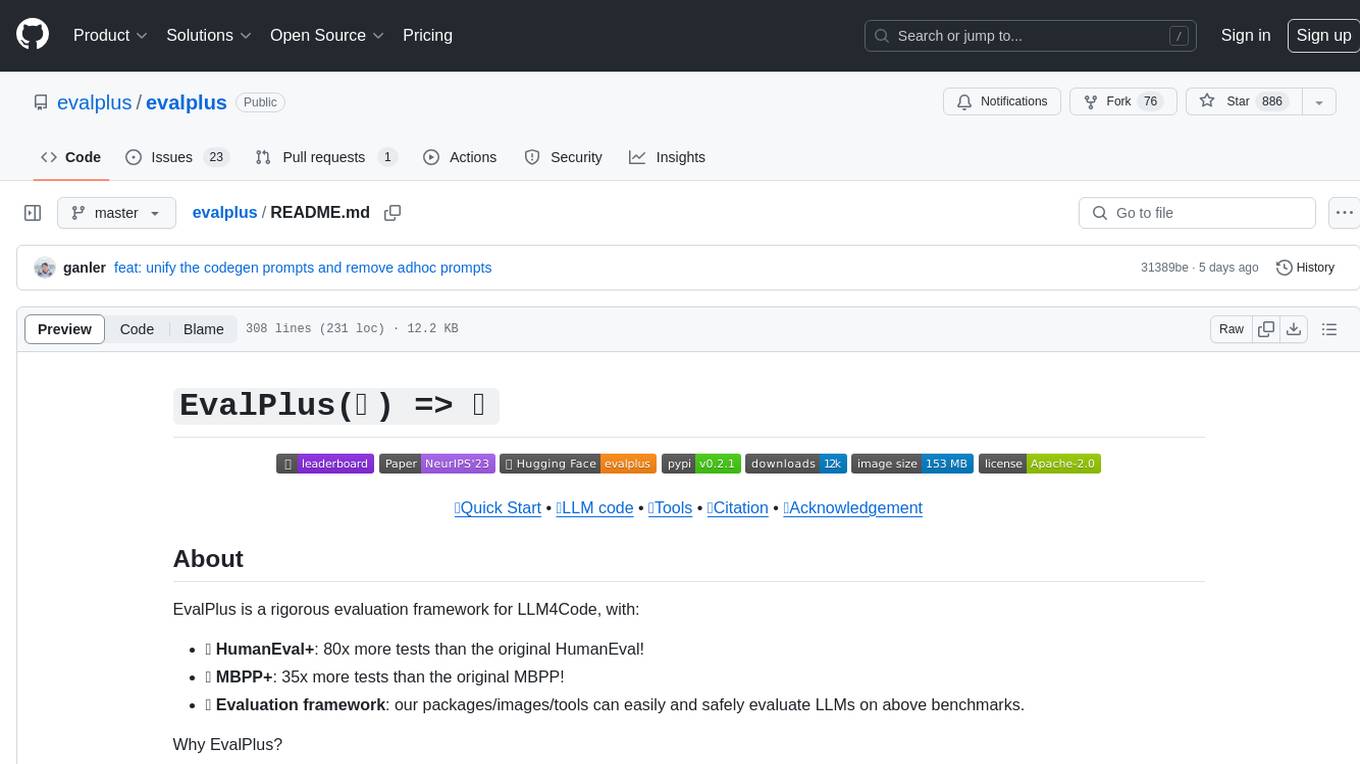
evalplus
EvalPlus is a rigorous evaluation framework for LLM4Code, providing HumanEval+ and MBPP+ tests to evaluate large language models on code generation tasks. It offers precise evaluation and ranking, coding rigorousness analysis, and pre-generated code samples. Users can use EvalPlus to generate code solutions, post-process code, and evaluate code quality. The tool includes tools for code generation and test input generation using various backends.
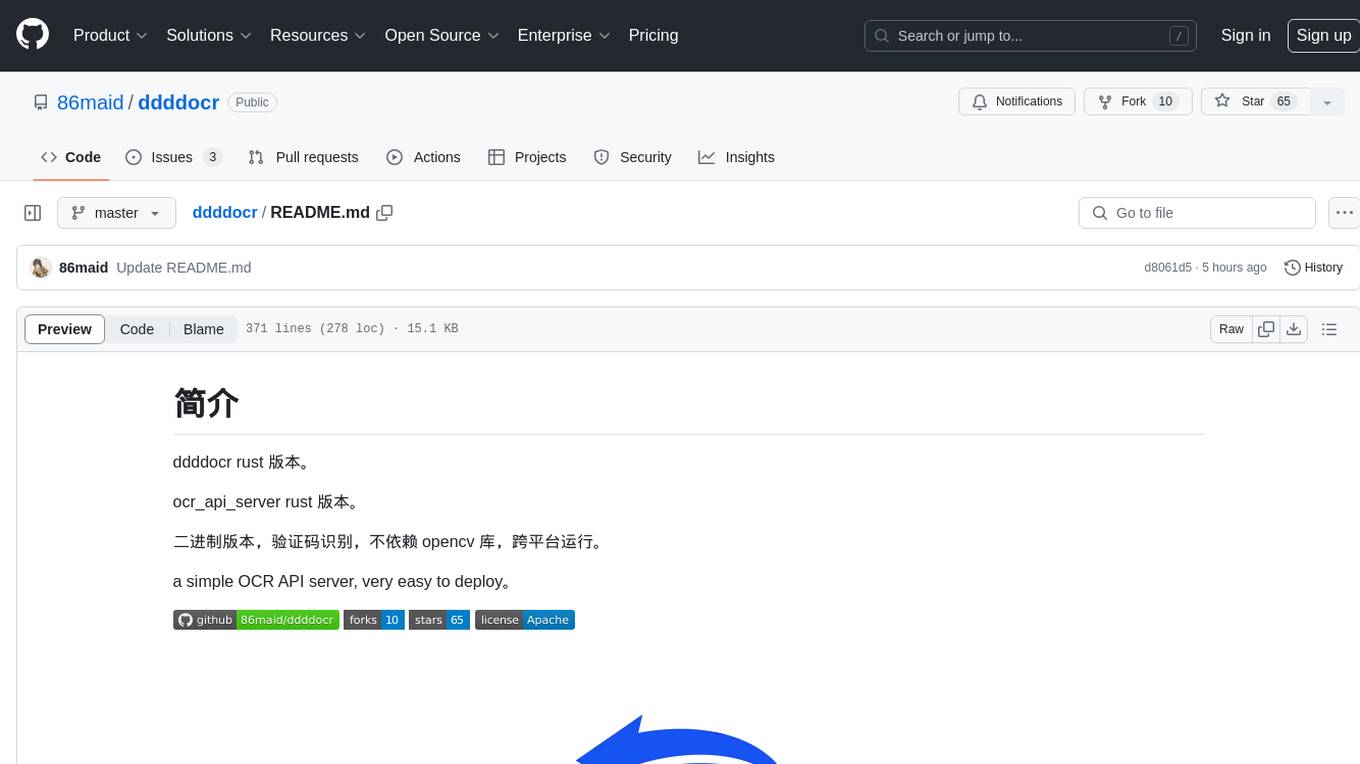
ddddocr
ddddocr is a Rust version of a simple OCR API server that provides easy deployment for captcha recognition without relying on the OpenCV library. It offers a user-friendly general-purpose captcha recognition Rust library. The tool supports recognizing various types of captchas, including single-line text, transparent black PNG images, target detection, and slider matching algorithms. Users can also import custom OCR training models and utilize the OCR API server for flexible OCR result control and range limitation. The tool is cross-platform and can be easily deployed.
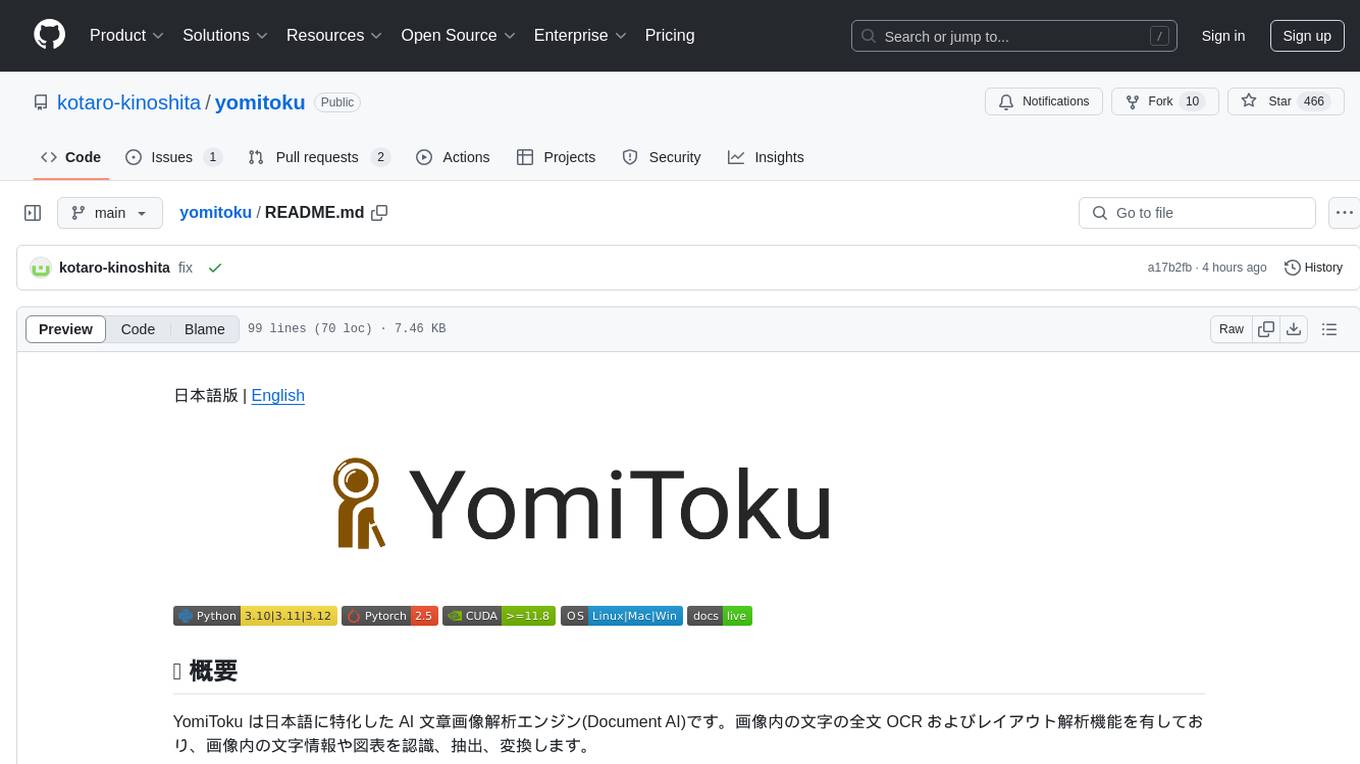
yomitoku
YomiToku is a Japanese-focused AI document image analysis engine that provides full-text OCR and layout analysis capabilities for images. It recognizes, extracts, and converts text information and figures in images. It includes 4 AI models trained on Japanese datasets for tasks such as detecting text positions, recognizing text strings, analyzing layouts, and recognizing table structures. The models are specialized for Japanese document images, supporting recognition of over 7000 Japanese characters and analyzing layout structures specific to Japanese documents. It offers features like layout analysis, table structure analysis, and reading order estimation to extract information from document images without disrupting their semantic structure. YomiToku supports various output formats such as HTML, markdown, JSON, and CSV, and can also extract figures, tables, and images from documents. It operates efficiently in GPU environments, enabling fast and effective analysis of document transcriptions without requiring high-end GPUs.
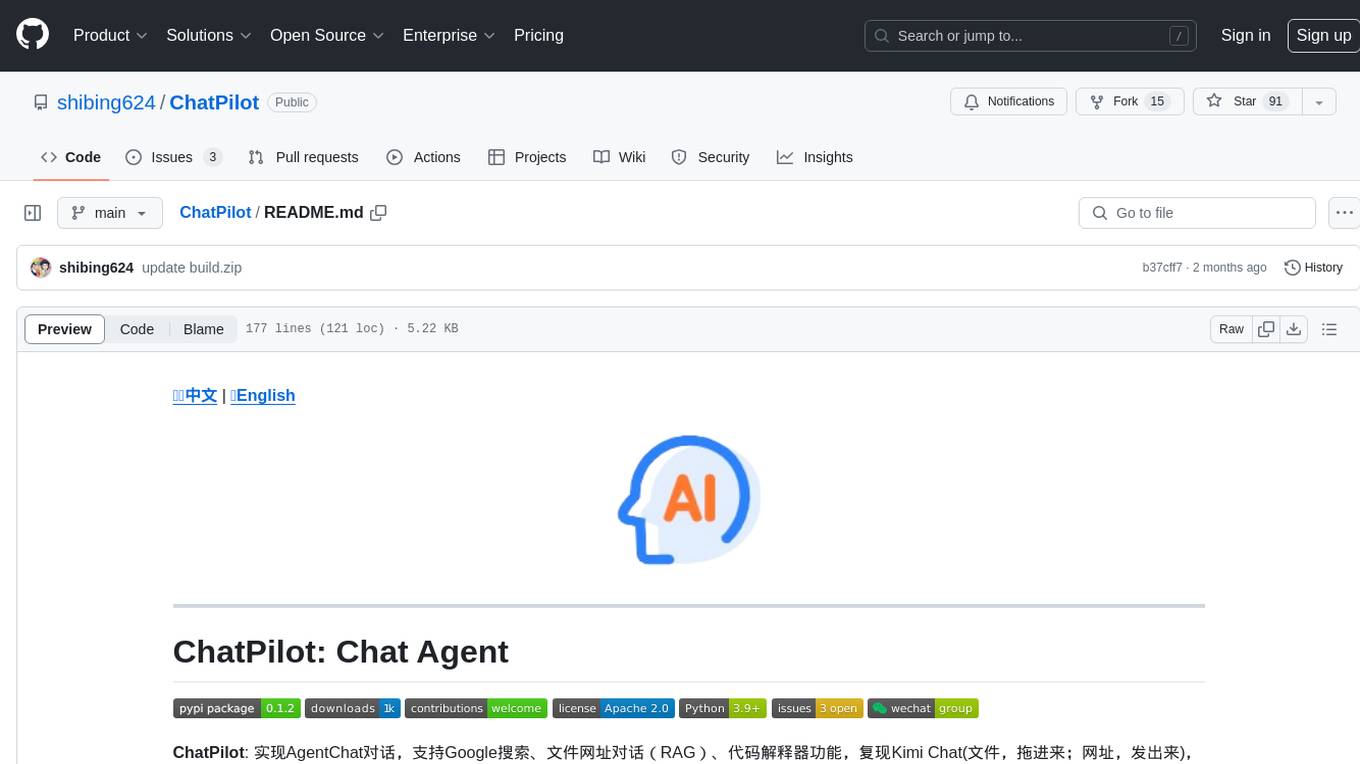
ChatPilot
ChatPilot is a chat agent tool that enables AgentChat conversations, supports Google search, URL conversation (RAG), and code interpreter functionality, replicates Kimi Chat (file, drag and drop; URL, send out), and supports OpenAI/Azure API. It is based on LangChain and implements ReAct and OpenAI Function Call for agent Q&A dialogue. The tool supports various automatic tools such as online search using Google Search API, URL parsing tool, Python code interpreter, and enhanced RAG file Q&A with query rewriting support. It also allows front-end and back-end service separation using Svelte and FastAPI, respectively. Additionally, it supports voice input/output, image generation, user management, permission control, and chat record import/export.
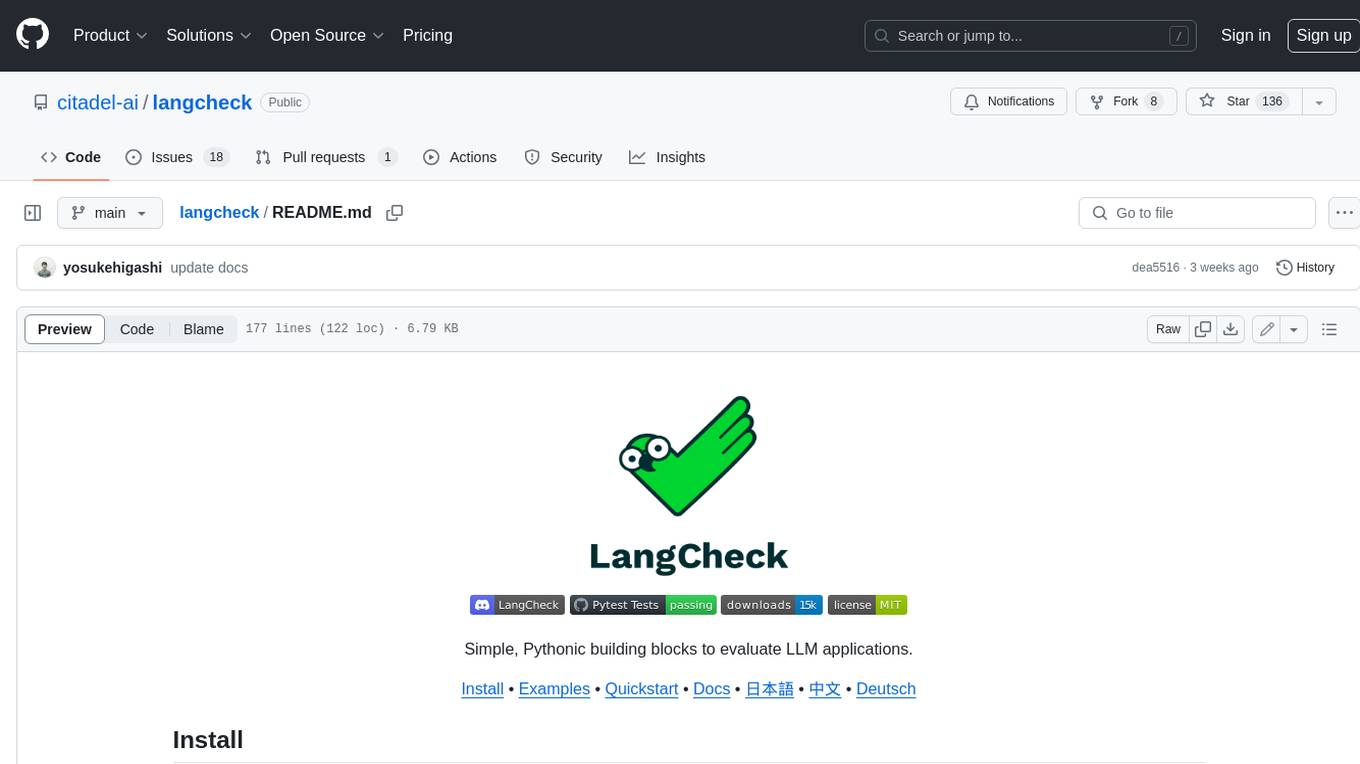
langcheck
LangCheck is a Python library that provides a suite of metrics and tools for evaluating the quality of text generated by large language models (LLMs). It includes metrics for evaluating text fluency, sentiment, toxicity, factual consistency, and more. LangCheck also provides tools for visualizing metrics, augmenting data, and writing unit tests for LLM applications. With LangCheck, you can quickly and easily assess the quality of LLM-generated text and identify areas for improvement.
For similar tasks
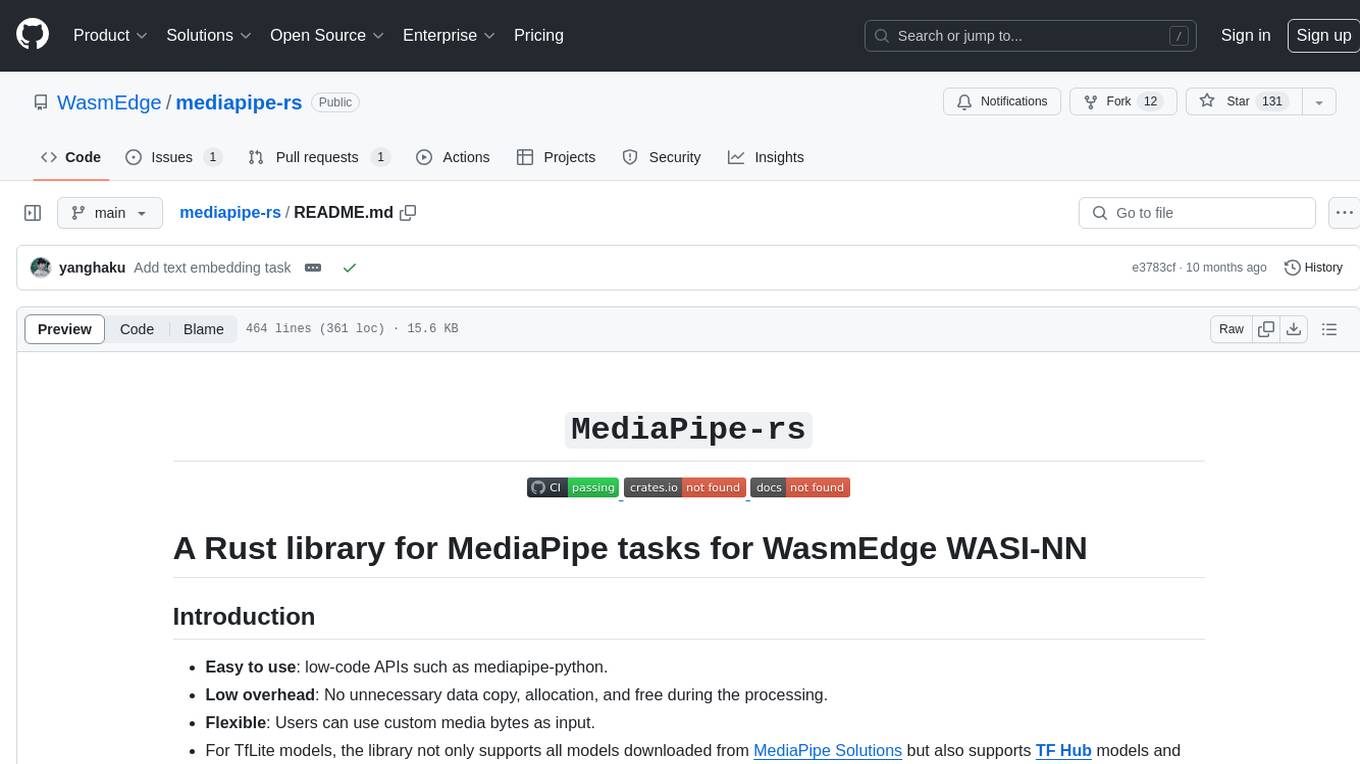
mediapipe-rs
MediaPipe-rs is a Rust library designed for MediaPipe tasks on WasmEdge WASI-NN. It offers easy-to-use low-code APIs similar to mediapipe-python, with low overhead and flexibility for custom media input. The library supports various tasks like object detection, image classification, gesture recognition, and more, including TfLite models, TF Hub models, and custom models. Users can create task instances, run sessions for pre-processing, inference, and post-processing, and speed up processing by reusing sessions. The library also provides support for audio tasks using audio data from symphonia, ffmpeg, or raw audio. Users can choose between CPU, GPU, or TPU devices for processing.
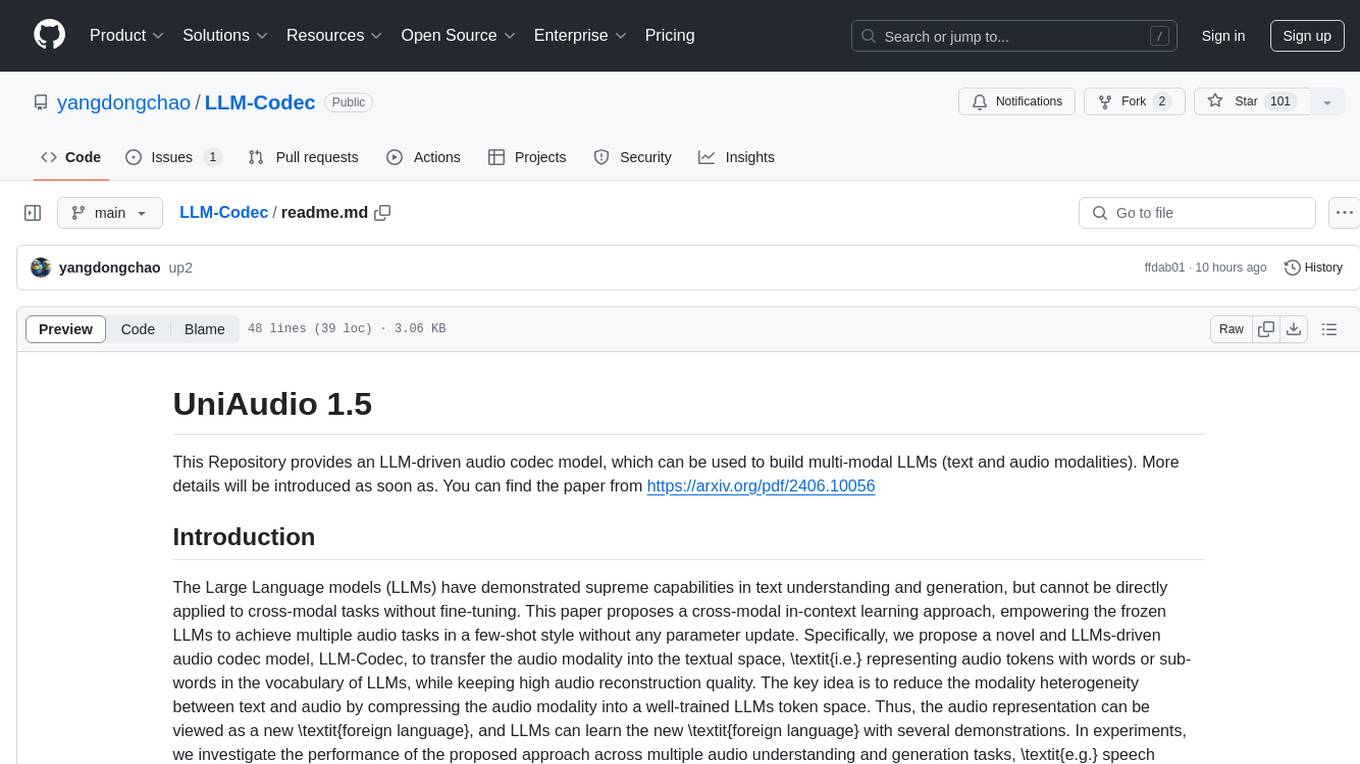
LLM-Codec
This repository provides an LLM-driven audio codec model, LLM-Codec, for building multi-modal LLMs (text and audio modalities). The model enables frozen LLMs to achieve multiple audio tasks in a few-shot style without parameter updates. It compresses the audio modality into a well-trained LLMs token space, treating audio representation as a 'foreign language' that LLMs can learn with minimal examples. The proposed approach supports tasks like speech emotion classification, audio classification, text-to-speech generation, speech enhancement, etc., demonstrating feasibility and effectiveness in simple scenarios. The LLM-Codec model is open-sourced to facilitate research on few-shot audio task learning and multi-modal LLMs.
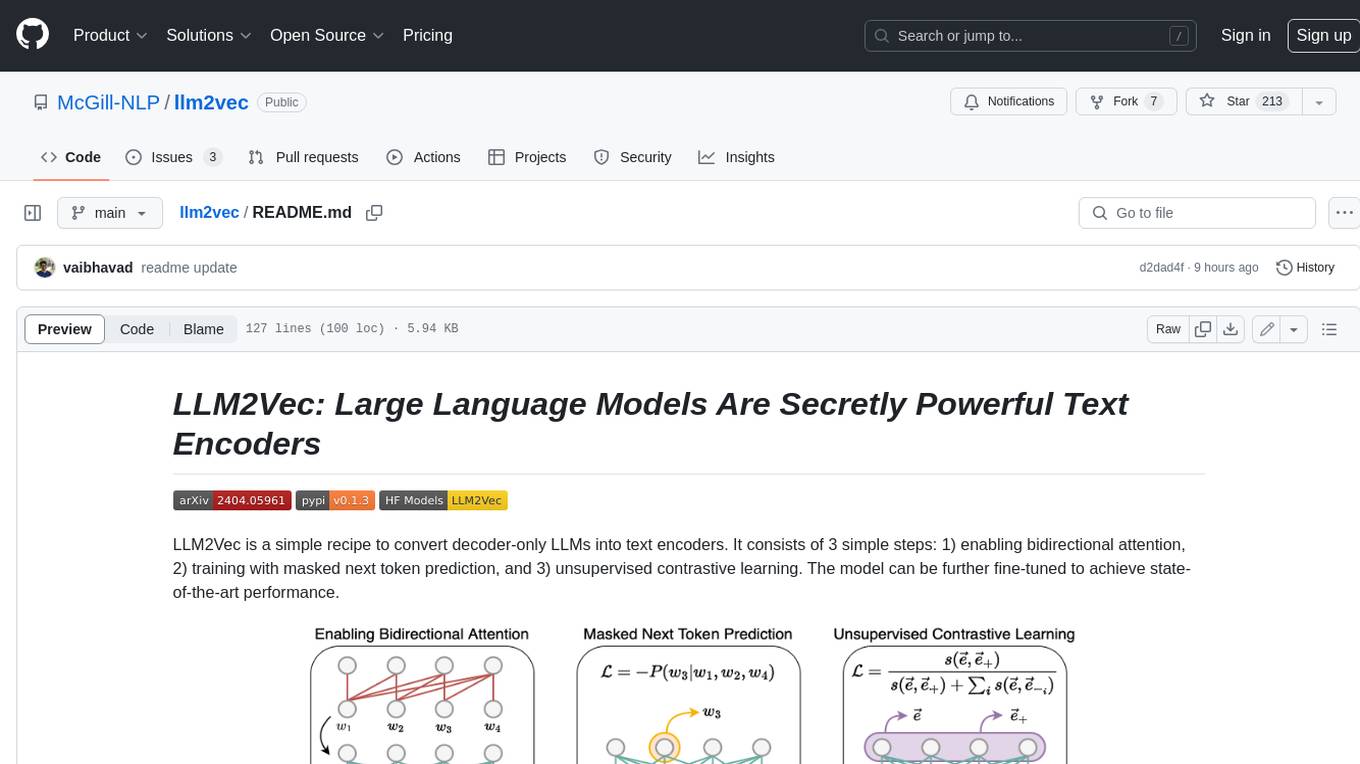
llm2vec
LLM2Vec is a simple recipe to convert decoder-only LLMs into text encoders. It consists of 3 simple steps: 1) enabling bidirectional attention, 2) training with masked next token prediction, and 3) unsupervised contrastive learning. The model can be further fine-tuned to achieve state-of-the-art performance.
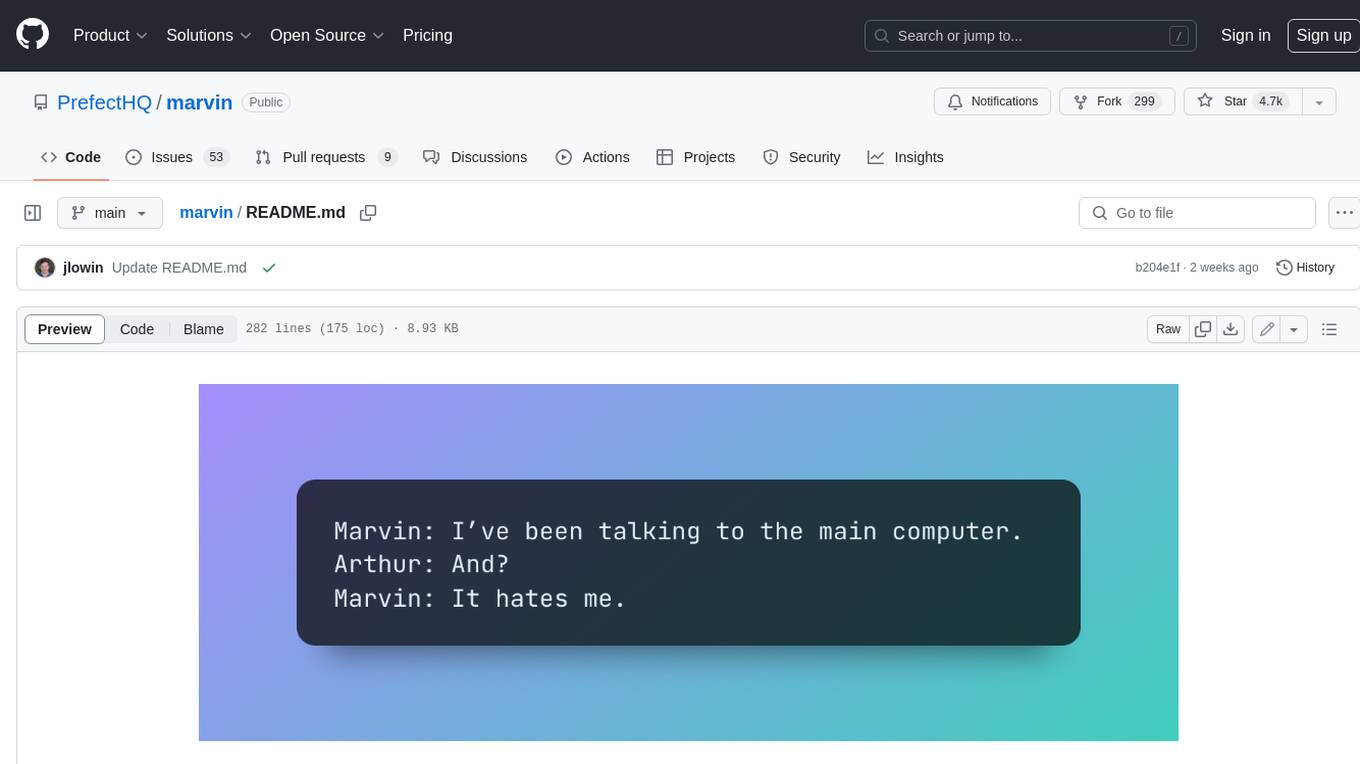
marvin
Marvin is a lightweight AI toolkit for building natural language interfaces that are reliable, scalable, and easy to trust. Each of Marvin's tools is simple and self-documenting, using AI to solve common but complex challenges like entity extraction, classification, and generating synthetic data. Each tool is independent and incrementally adoptable, so you can use them on their own or in combination with any other library. Marvin is also multi-modal, supporting both image and audio generation as well using images as inputs for extraction and classification. Marvin is for developers who care more about _using_ AI than _building_ AI, and we are focused on creating an exceptional developer experience. Marvin users should feel empowered to bring tightly-scoped "AI magic" into any traditional software project with just a few extra lines of code. Marvin aims to merge the best practices for building dependable, observable software with the best practices for building with generative AI into a single, easy-to-use library. It's a serious tool, but we hope you have fun with it. Marvin is open-source, free to use, and made with 💙 by the team at Prefect.
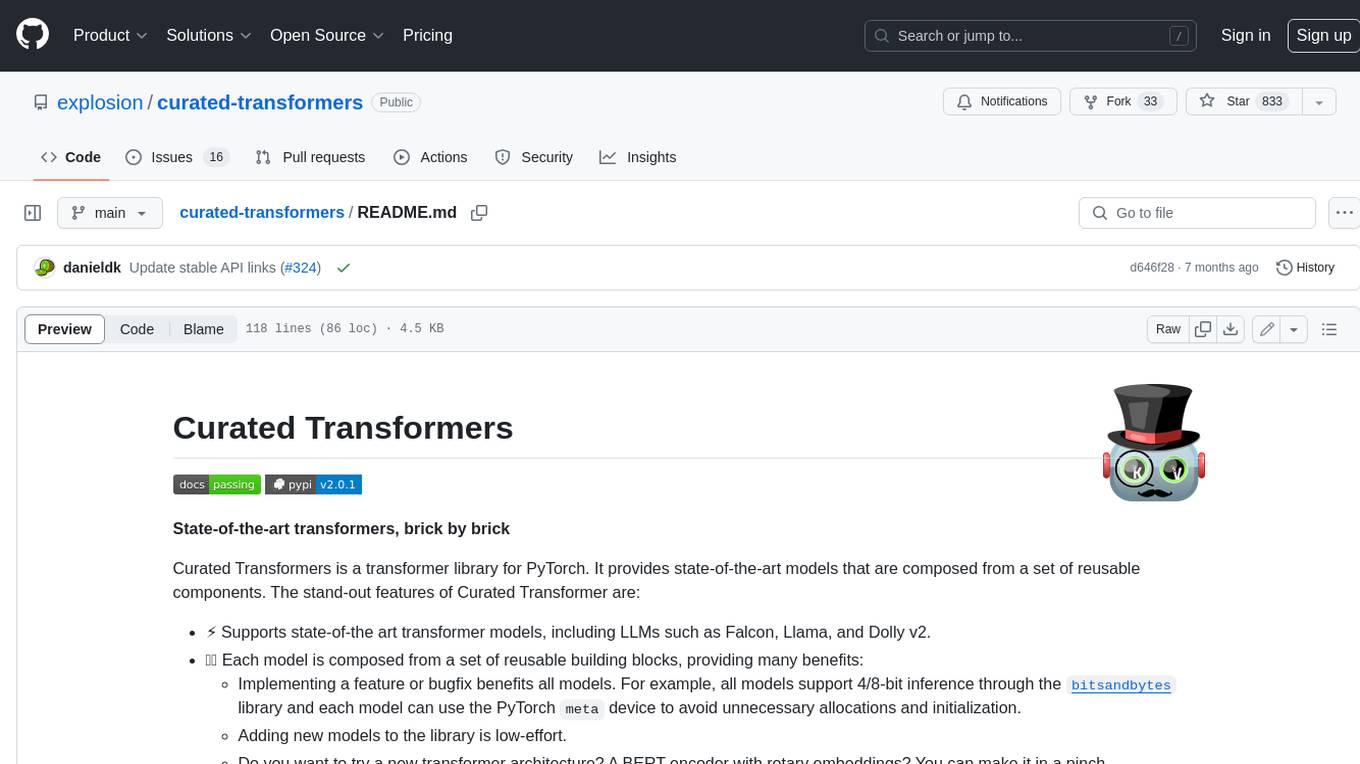
curated-transformers
Curated Transformers is a transformer library for PyTorch that provides state-of-the-art models composed of reusable components. It supports various transformer architectures, including encoders like ALBERT, BERT, and RoBERTa, and decoders like Falcon, Llama, and MPT. The library emphasizes consistent type annotations, minimal dependencies, and ease of use for education and research. It has been production-tested by Explosion and will be the default transformer implementation in spaCy 3.7.
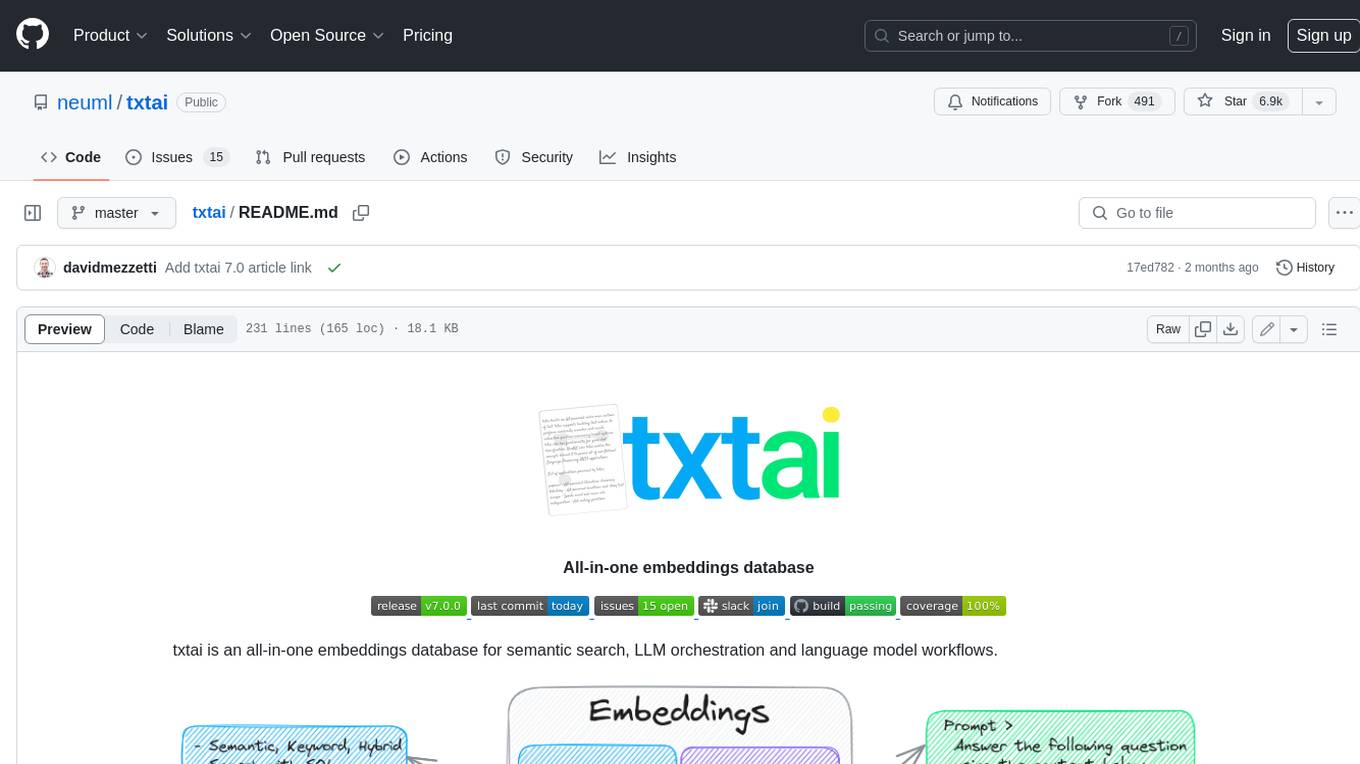
txtai
Txtai is an all-in-one embeddings database for semantic search, LLM orchestration, and language model workflows. It combines vector indexes, graph networks, and relational databases to enable vector search with SQL, topic modeling, retrieval augmented generation, and more. Txtai can stand alone or serve as a knowledge source for large language models (LLMs). Key features include vector search with SQL, object storage, topic modeling, graph analysis, multimodal indexing, embedding creation for various data types, pipelines powered by language models, workflows to connect pipelines, and support for Python, JavaScript, Java, Rust, and Go. Txtai is open-source under the Apache 2.0 license.
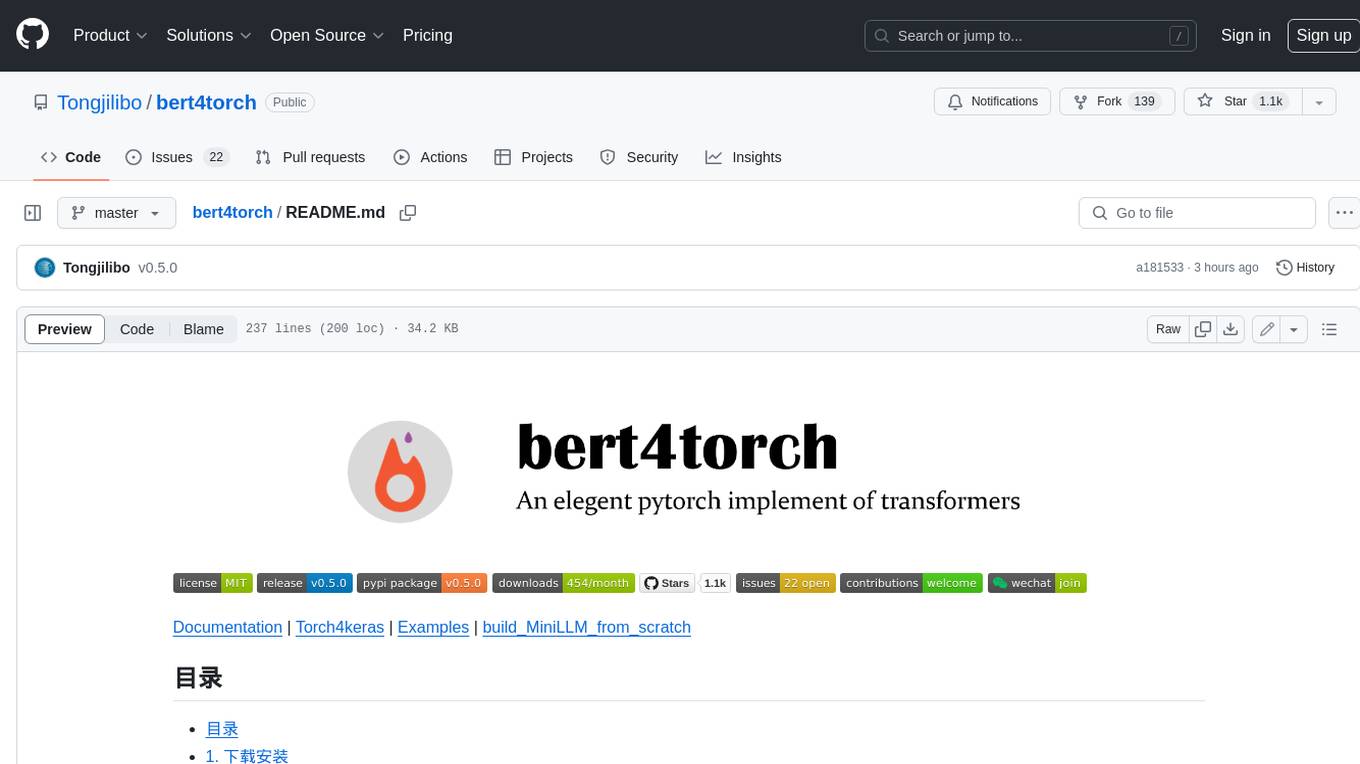
bert4torch
**bert4torch** is a high-level framework for training and deploying transformer models in PyTorch. It provides a simple and efficient API for building, training, and evaluating transformer models, and supports a wide range of pre-trained models, including BERT, RoBERTa, ALBERT, XLNet, and GPT-2. bert4torch also includes a number of useful features, such as data loading, tokenization, and model evaluation. It is a powerful and versatile tool for natural language processing tasks.
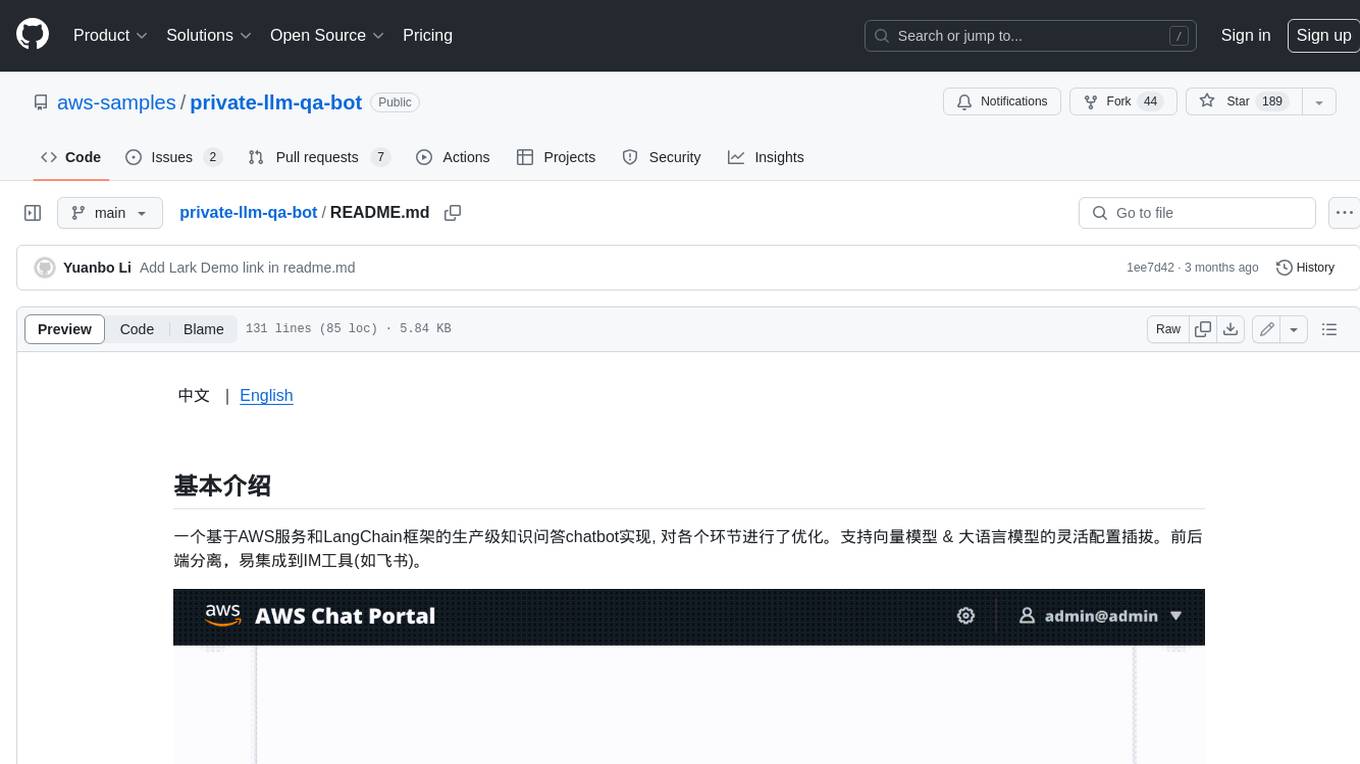
private-llm-qa-bot
This is a production-grade knowledge Q&A chatbot implementation based on AWS services and the LangChain framework, with optimizations at various stages. It supports flexible configuration and plugging of vector models and large language models. The front and back ends are separated, making it easy to integrate with IM tools (such as Feishu).
For similar jobs
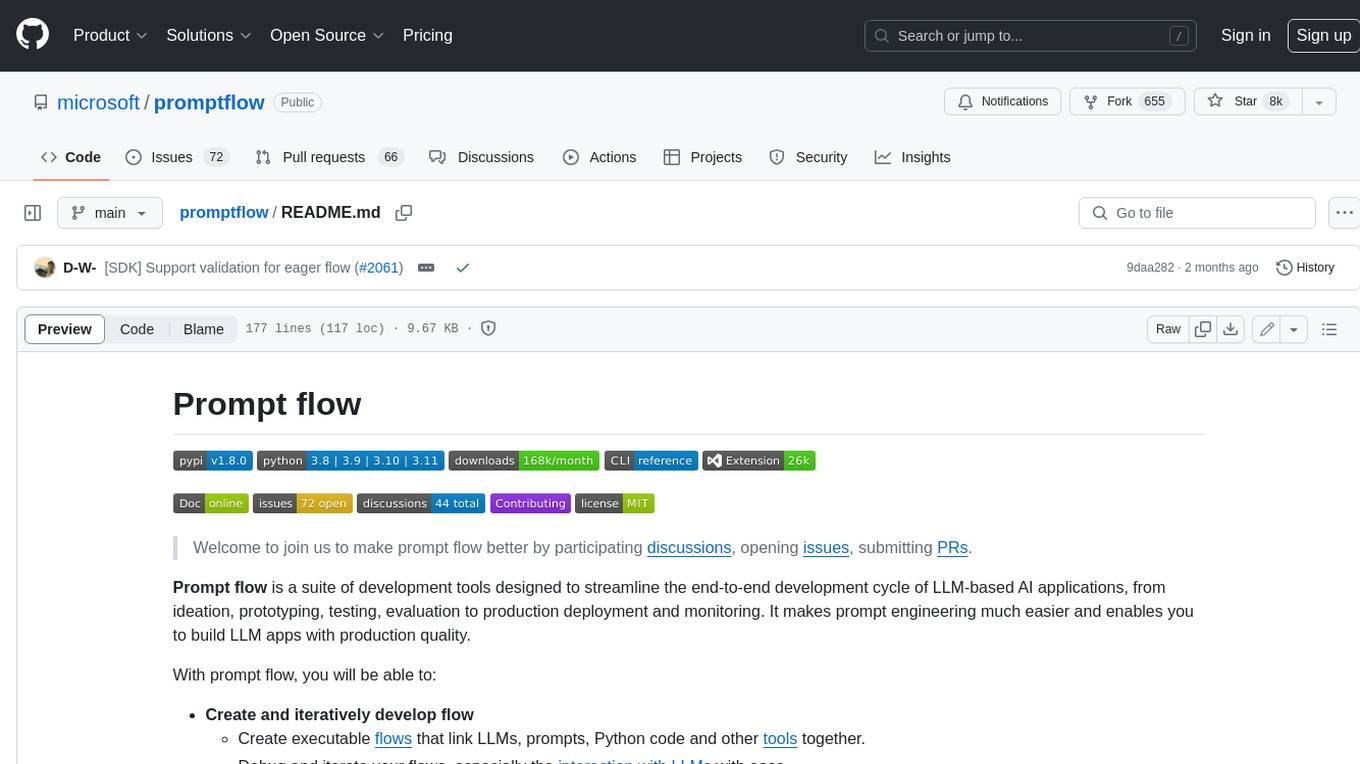
promptflow
**Prompt flow** is a suite of development tools designed to streamline the end-to-end development cycle of LLM-based AI applications, from ideation, prototyping, testing, evaluation to production deployment and monitoring. It makes prompt engineering much easier and enables you to build LLM apps with production quality.
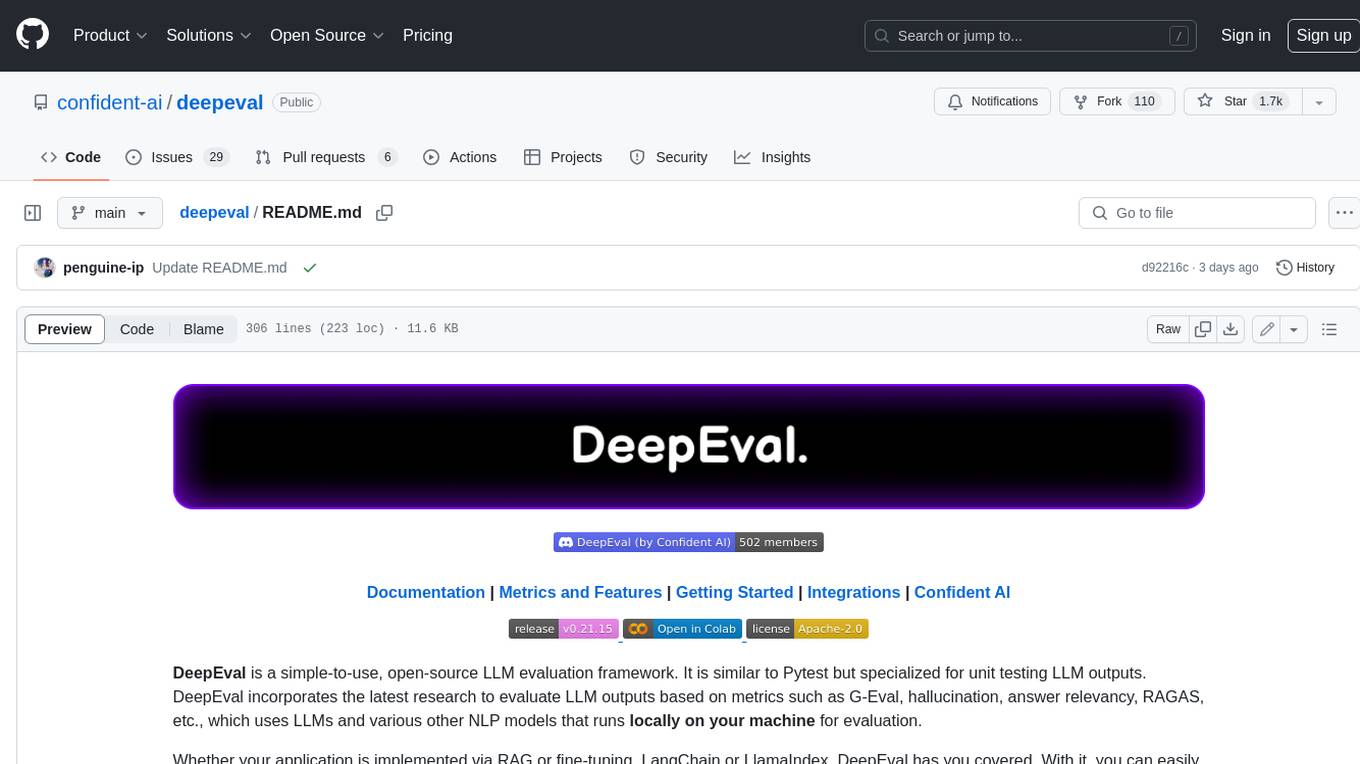
deepeval
DeepEval is a simple-to-use, open-source LLM evaluation framework specialized for unit testing LLM outputs. It incorporates various metrics such as G-Eval, hallucination, answer relevancy, RAGAS, etc., and runs locally on your machine for evaluation. It provides a wide range of ready-to-use evaluation metrics, allows for creating custom metrics, integrates with any CI/CD environment, and enables benchmarking LLMs on popular benchmarks. DeepEval is designed for evaluating RAG and fine-tuning applications, helping users optimize hyperparameters, prevent prompt drifting, and transition from OpenAI to hosting their own Llama2 with confidence.
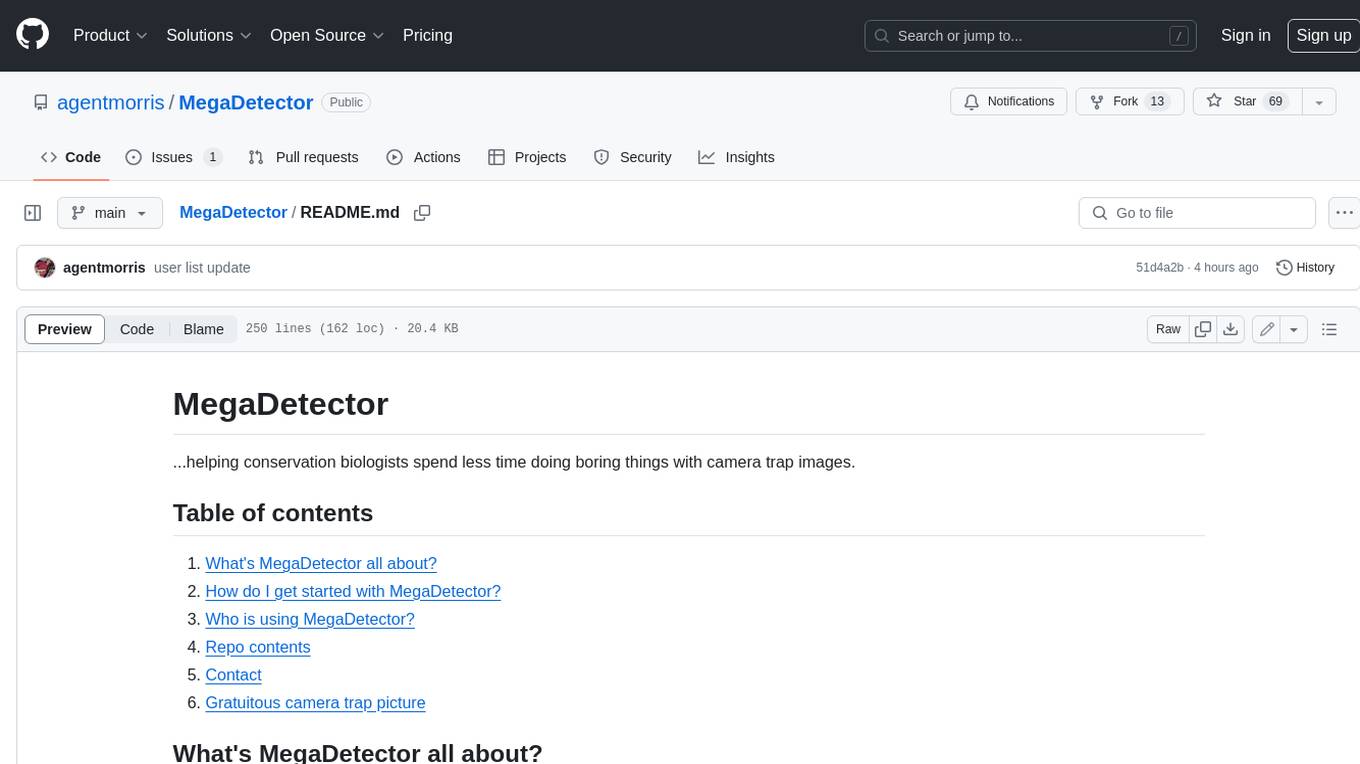
MegaDetector
MegaDetector is an AI model that identifies animals, people, and vehicles in camera trap images (which also makes it useful for eliminating blank images). This model is trained on several million images from a variety of ecosystems. MegaDetector is just one of many tools that aims to make conservation biologists more efficient with AI. If you want to learn about other ways to use AI to accelerate camera trap workflows, check out our of the field, affectionately titled "Everything I know about machine learning and camera traps".
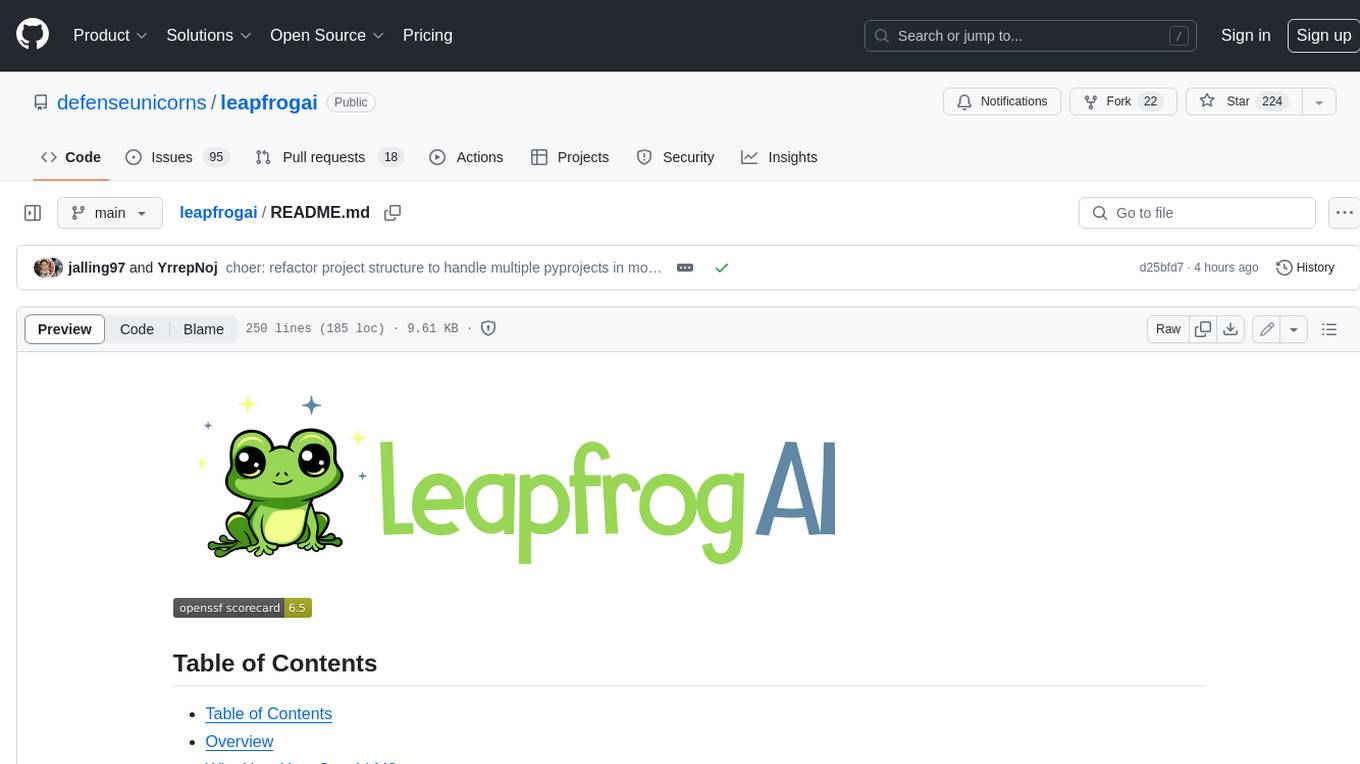
leapfrogai
LeapfrogAI is a self-hosted AI platform designed to be deployed in air-gapped resource-constrained environments. It brings sophisticated AI solutions to these environments by hosting all the necessary components of an AI stack, including vector databases, model backends, API, and UI. LeapfrogAI's API closely matches that of OpenAI, allowing tools built for OpenAI/ChatGPT to function seamlessly with a LeapfrogAI backend. It provides several backends for various use cases, including llama-cpp-python, whisper, text-embeddings, and vllm. LeapfrogAI leverages Chainguard's apko to harden base python images, ensuring the latest supported Python versions are used by the other components of the stack. The LeapfrogAI SDK provides a standard set of protobuffs and python utilities for implementing backends and gRPC. LeapfrogAI offers UI options for common use-cases like chat, summarization, and transcription. It can be deployed and run locally via UDS and Kubernetes, built out using Zarf packages. LeapfrogAI is supported by a community of users and contributors, including Defense Unicorns, Beast Code, Chainguard, Exovera, Hypergiant, Pulze, SOSi, United States Navy, United States Air Force, and United States Space Force.
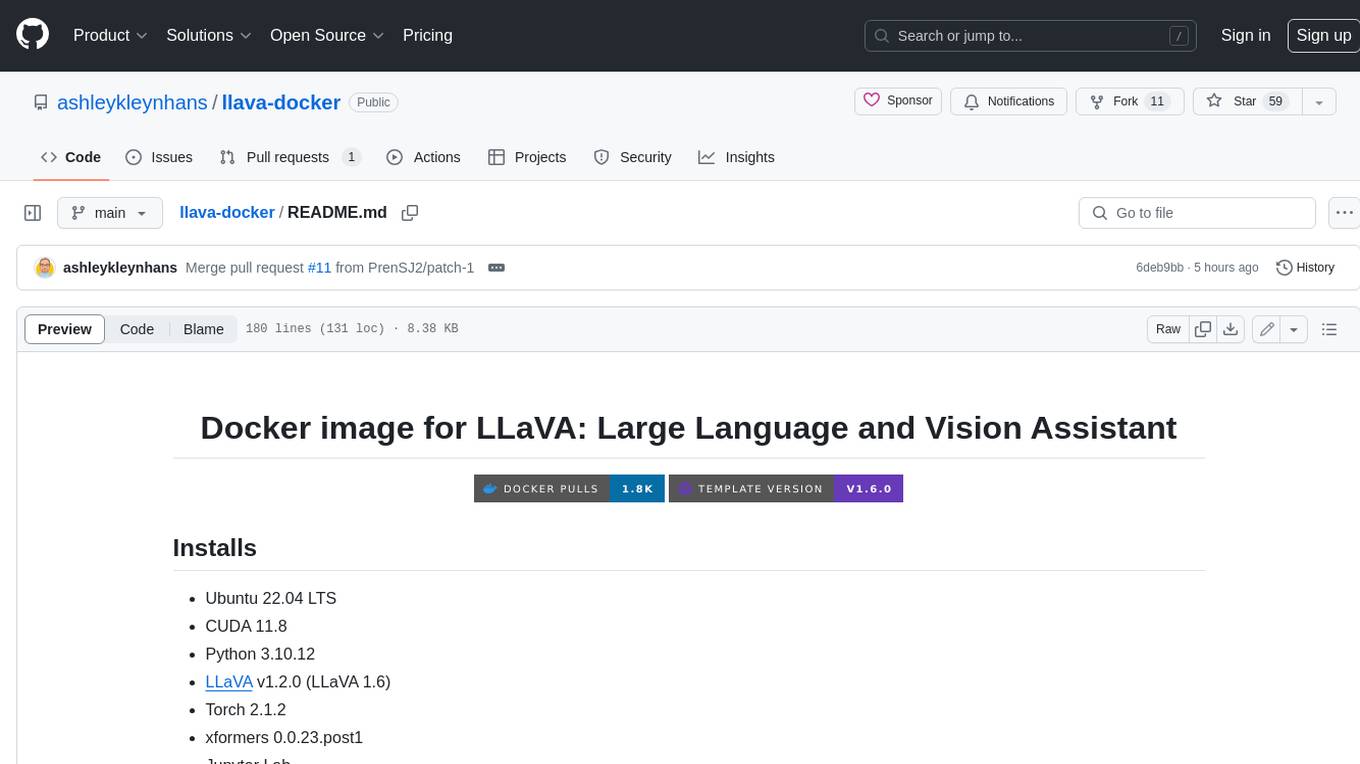
llava-docker
This Docker image for LLaVA (Large Language and Vision Assistant) provides a convenient way to run LLaVA locally or on RunPod. LLaVA is a powerful AI tool that combines natural language processing and computer vision capabilities. With this Docker image, you can easily access LLaVA's functionalities for various tasks, including image captioning, visual question answering, text summarization, and more. The image comes pre-installed with LLaVA v1.2.0, Torch 2.1.2, xformers 0.0.23.post1, and other necessary dependencies. You can customize the model used by setting the MODEL environment variable. The image also includes a Jupyter Lab environment for interactive development and exploration. Overall, this Docker image offers a comprehensive and user-friendly platform for leveraging LLaVA's capabilities.
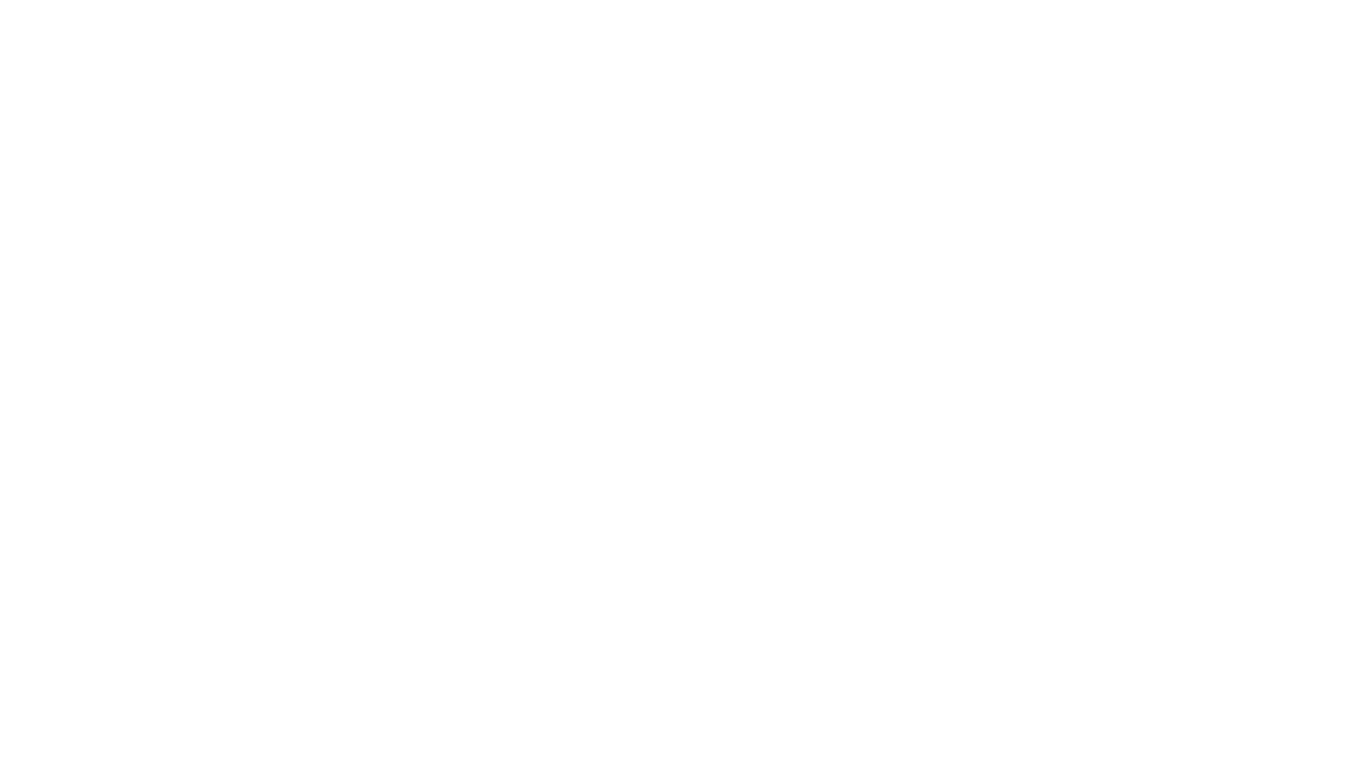
carrot
The 'carrot' repository on GitHub provides a list of free and user-friendly ChatGPT mirror sites for easy access. The repository includes sponsored sites offering various GPT models and services. Users can find and share sites, report errors, and access stable and recommended sites for ChatGPT usage. The repository also includes a detailed list of ChatGPT sites, their features, and accessibility options, making it a valuable resource for ChatGPT users seeking free and unlimited GPT services.
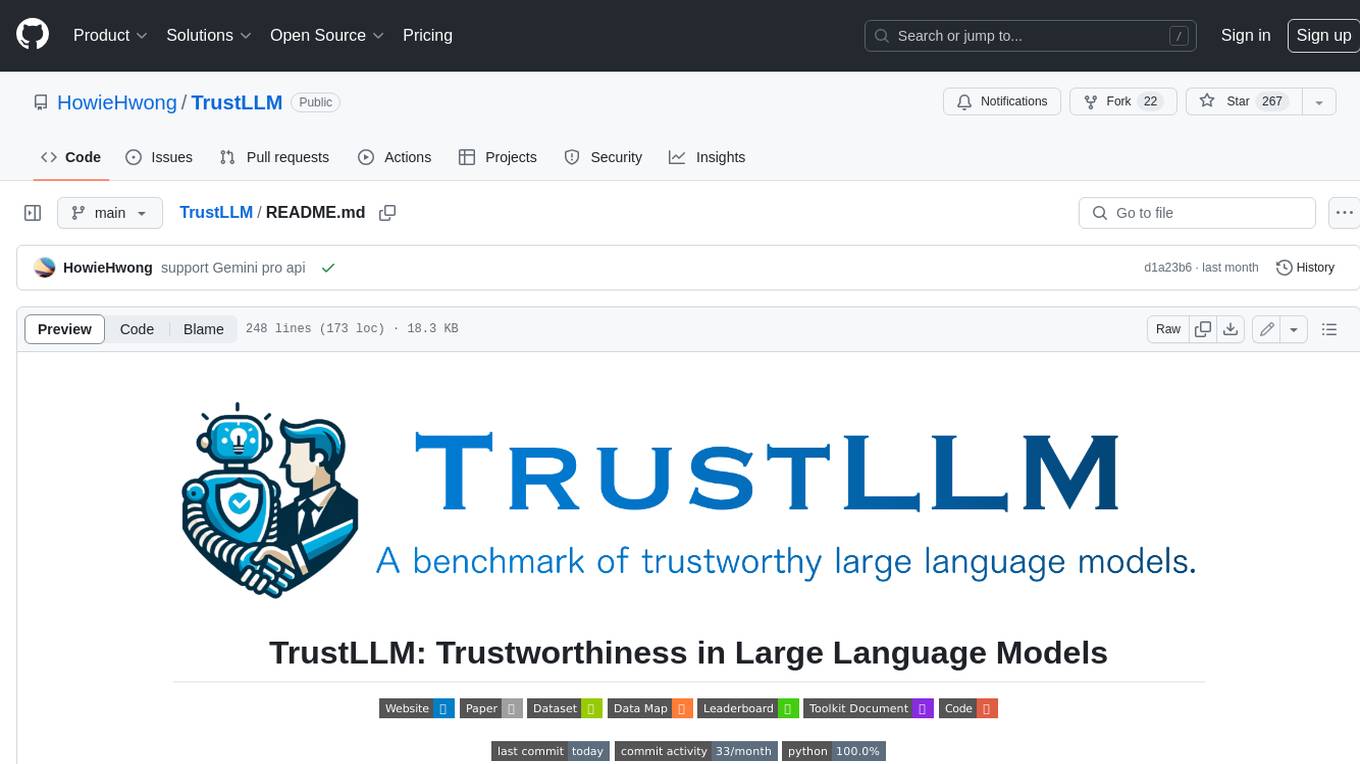
TrustLLM
TrustLLM is a comprehensive study of trustworthiness in LLMs, including principles for different dimensions of trustworthiness, established benchmark, evaluation, and analysis of trustworthiness for mainstream LLMs, and discussion of open challenges and future directions. Specifically, we first propose a set of principles for trustworthy LLMs that span eight different dimensions. Based on these principles, we further establish a benchmark across six dimensions including truthfulness, safety, fairness, robustness, privacy, and machine ethics. We then present a study evaluating 16 mainstream LLMs in TrustLLM, consisting of over 30 datasets. The document explains how to use the trustllm python package to help you assess the performance of your LLM in trustworthiness more quickly. For more details about TrustLLM, please refer to project website.
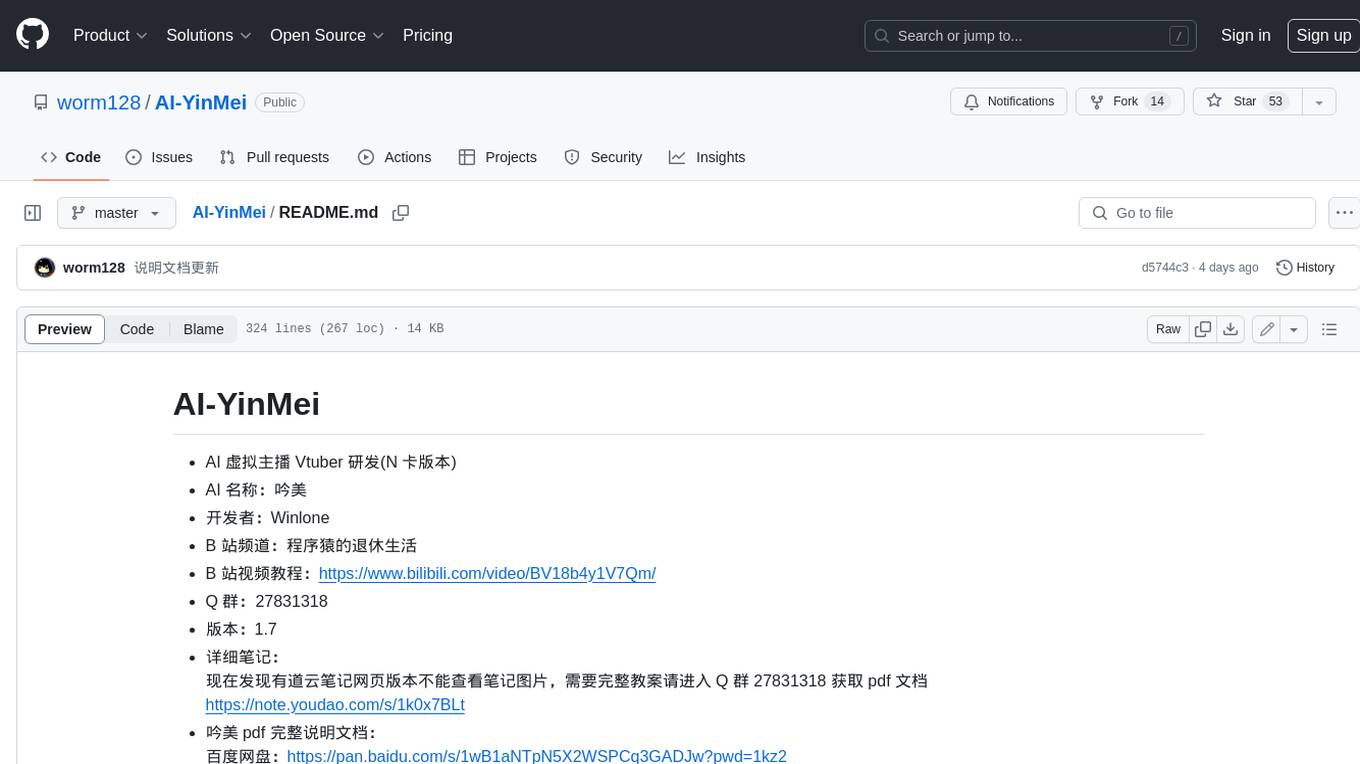
AI-YinMei
AI-YinMei is an AI virtual anchor Vtuber development tool (N card version). It supports fastgpt knowledge base chat dialogue, a complete set of solutions for LLM large language models: [fastgpt] + [one-api] + [Xinference], supports docking bilibili live broadcast barrage reply and entering live broadcast welcome speech, supports Microsoft edge-tts speech synthesis, supports Bert-VITS2 speech synthesis, supports GPT-SoVITS speech synthesis, supports expression control Vtuber Studio, supports painting stable-diffusion-webui output OBS live broadcast room, supports painting picture pornography public-NSFW-y-distinguish, supports search and image search service duckduckgo (requires magic Internet access), supports image search service Baidu image search (no magic Internet access), supports AI reply chat box [html plug-in], supports AI singing Auto-Convert-Music, supports playlist [html plug-in], supports dancing function, supports expression video playback, supports head touching action, supports gift smashing action, supports singing automatic start dancing function, chat and singing automatic cycle swing action, supports multi scene switching, background music switching, day and night automatic switching scene, supports open singing and painting, let AI automatically judge the content.