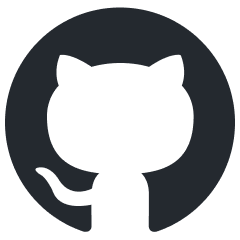
lego-ai-parser
Lego AI Parser is an open-source application that uses OpenAI to parse visible text of HTML elements.
Stars: 223
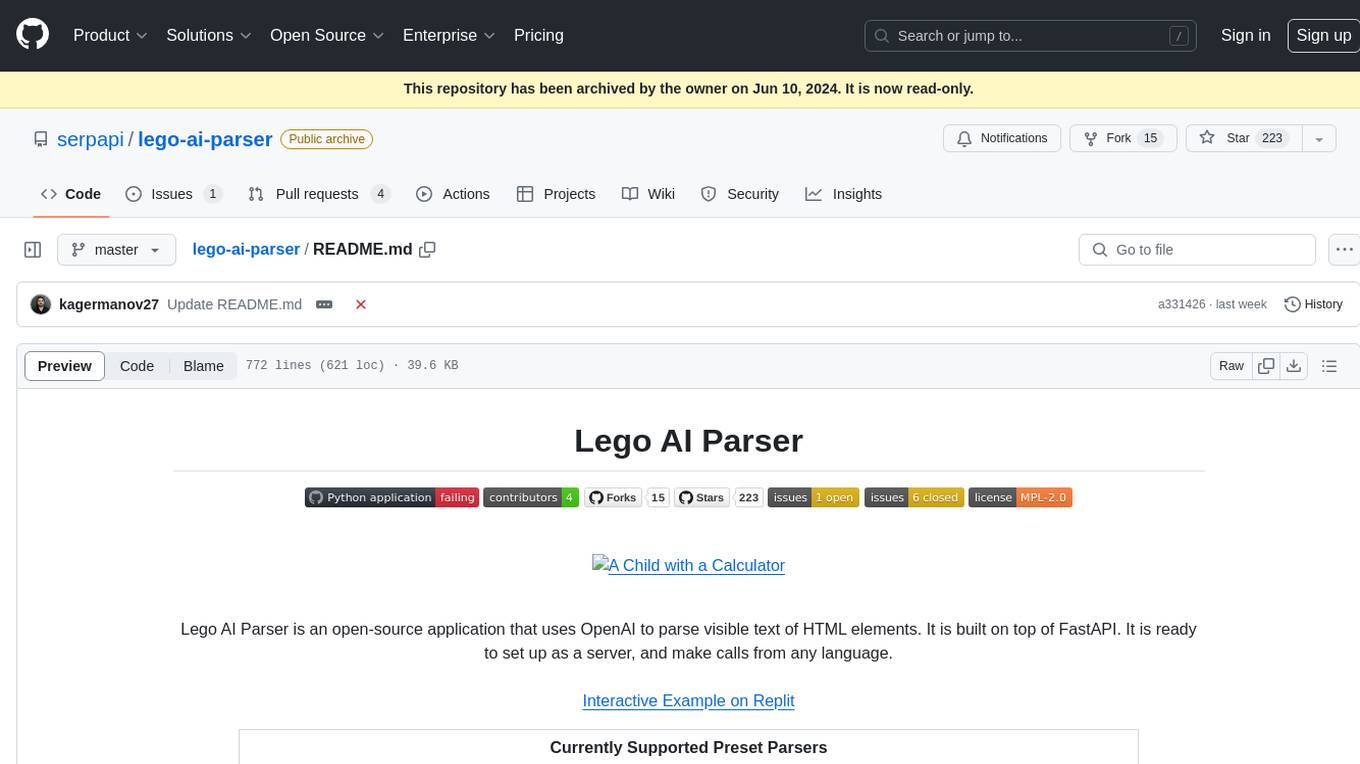
Lego AI Parser is an open-source application that uses OpenAI to parse visible text of HTML elements. It is built on top of FastAPI, ready to set up as a server, and make calls from any language. It supports preset parsers for Google Local Results, Amazon Listings, Etsy Listings, Wayfair Listings, BestBuy Listings, Costco Listings, Macy's Listings, and Nordstrom Listings. Users can also design custom parsers by providing prompts, examples, and details about the OpenAI model under the classifier key.
README:
Lego AI Parser is an open-source application that uses OpenAI to parse visible text of HTML elements. It is built on top of FastAPI. It is ready to set up as a server, and make calls from any language.
Interactive Example on Replit
Currently Supported Preset Parsers | |||
---|---|---|---|
Google Local Results Parser | Amazon Listings Parser | Etsy Listings Parser | Wayfair Listings Parser |
BestBuy Listings Parser | Costco Listings Parser | Macy's Listings Parser | Nordstrom Listings Parser |
- Lego AI Parser
You need to register a free account first. You may find your API Key here.
import requests
uri = "https://yourserver.com/classify"
headers = {"Content-Type": "application/json"}
data = {
"path": "google.google_local_results",
"targets": [
"<div jscontroller=\"AtSb\" class=\"w7Dbne\" data-record-click-time=\"false\" id=\"tsuid_25\" jsdata=\"zt2wNd;_;BvbRxs V6f1Id;_;BvbRxw\" jsaction=\"rcuQ6b:npT2md;e3EWke:kN9HDb\" data-hveid=\"CBUQAA\"><div jsname=\"jXK9ad\" class=\"uMdZh tIxNaf\" jsaction=\"mouseover:UI3Kjd\"><div class=\"VkpGBb\"><div class=\"cXedhc\"><a class=\"vwVdIc wzN8Ac rllt__link a-no-hover-decoration\" jsname=\"kj0dLd\" data-cid=\"12176489206865957637\" jsaction=\"click:h5M12e;\" role=\"link\" tabindex=\"0\" data-ved=\"2ahUKEwiS1P3_j-P7AhXnVPEDHa0oAiAQvS56BAgVEAE\"><div><div class=\"rllt__details\"><div class=\"dbg0pd\" aria-level=\"3\" role=\"heading\"><span class=\"OSrXXb\">Y Coffee</span></div><div><span class=\"Y0A0hc\"><span class=\"yi40Hd YrbPuc\" aria-hidden=\"true\">4.0</span><span class=\"z3HNkc\" aria-label=\"Rated 4.0 out of 5,\" role=\"img\"><span style=\"width:56px\"></span></span><span class=\"RDApEe YrbPuc\">(418)</span></span> · <span aria-label=\"Moderately expensive\" role=\"img\">€€</span> · Coffee shop</div><div>Nicosia</div><div class=\"pJ3Ci\"><span>Iconic Seattle-based coffeehouse chain</span></div></div></div></a><a class=\"uQ4NLd b9tNq wzN8Ac rllt__link a-no-hover-decoration\" aria-hidden=\"true\" tabindex=\"-1\" jsname=\"kj0dLd\" data-cid=\"12176489206865957637\" jsaction=\"click:h5M12e;\" role=\"link\" data-ved=\"2ahUKEwiS1P3_j-P7AhXnVPEDHa0oAiAQvS56BAgVEA4\"><g-img class=\"gTrj3e\"><img id=\"pimg_3\" src=\"https://lh5.googleusercontent.com/p/AF1QipPaihclGQYWEJpMpBnBY8Nl8QWQVqZ6tF--MlwD=w184-h184-n-k-no\" class=\"YQ4gaf zr758c wA1Bge\" alt=\"\" data-atf=\"4\" data-frt=\"0\" width=\"92\" height=\"92\"></g-img></a></div></div></div></div>"
],
"openai_key": "<OPENAI KEY>"
}
r = requests.post(url=uri, headers=headers, json=data)
print(r.json()["results"])
{
"results": [
{
"Address": "Nicosia",
"Description Or Review": "Iconic Seattle-based coffeehouse chain",
"Expensiveness": "€€",
"Number Of Reviews": "418",
"Rating": "4.0",
"Title": "Y Coffee",
"Type": "Coffee shop"
}
]
}
These instructions are for basic usage. Sharing API Keys with third-party applications is not recommended. It is recommended that you set up your own server, or use a throwaway API key to check out this fuctionality. Making the calls on server-side without sharing credentials are explained in the next sections.
In addition to using HTML of the element, using text you copy from the element is also accepted. You can pass a mixbag of HTML and Text in the same list. If all the elements exceed the token size of the model, Lego AI Parser
will separate the prompts for you and return the results in the same order. Please note that duplicate items will result in bad parsing.
import requests
uri = "https://yourserver.com/classify"
headers = {"Content-Type": "application/json"}
data = {
"path": "google.google_local_results",
"targets": [
"X Coffee 4.1(23) · €€ · Coffee shop Nicosia Counter-serve chain for coffee & snacks",
"<div jscontroller=\"AtSb\" class=\"w7Dbne\" data-record-click-time=\"false\" id=\"tsuid_25\" jsdata=\"zt2wNd;_;BvbRxs V6f1Id;_;BvbRxw\" jsaction=\"rcuQ6b:npT2md;e3EWke:kN9HDb\" data-hveid=\"CBUQAA\"><div jsname=\"jXK9ad\" class=\"uMdZh tIxNaf\" jsaction=\"mouseover:UI3Kjd\"><div class=\"VkpGBb\"><div class=\"cXedhc\"><a class=\"vwVdIc wzN8Ac rllt__link a-no-hover-decoration\" jsname=\"kj0dLd\" data-cid=\"12176489206865957637\" jsaction=\"click:h5M12e;\" role=\"link\" tabindex=\"0\" data-ved=\"2ahUKEwiS1P3_j-P7AhXnVPEDHa0oAiAQvS56BAgVEAE\"><div><div class=\"rllt__details\"><div class=\"dbg0pd\" aria-level=\"3\" role=\"heading\"><span class=\"OSrXXb\">Y Coffee</span></div><div><span class=\"Y0A0hc\"><span class=\"yi40Hd YrbPuc\" aria-hidden=\"true\">4.0</span><span class=\"z3HNkc\" aria-label=\"Rated 4.0 out of 5,\" role=\"img\"><span style=\"width:56px\"></span></span><span class=\"RDApEe YrbPuc\">(418)</span></span> · <span aria-label=\"Moderately expensive\" role=\"img\">€€</span> · Coffee shop</div><div>Nicosia</div><div class=\"pJ3Ci\"><span>Iconic Seattle-based coffeehouse chain</span></div></div></div></a><a class=\"uQ4NLd b9tNq wzN8Ac rllt__link a-no-hover-decoration\" aria-hidden=\"true\" tabindex=\"-1\" jsname=\"kj0dLd\" data-cid=\"12176489206865957637\" jsaction=\"click:h5M12e;\" role=\"link\" data-ved=\"2ahUKEwiS1P3_j-P7AhXnVPEDHa0oAiAQvS56BAgVEA4\"><g-img class=\"gTrj3e\"><img id=\"pimg_3\" src=\"https://lh5.googleusercontent.com/p/AF1QipPaihclGQYWEJpMpBnBY8Nl8QWQVqZ6tF--MlwD=w184-h184-n-k-no\" class=\"YQ4gaf zr758c wA1Bge\" alt=\"\" data-atf=\"4\" data-frt=\"0\" width=\"92\" height=\"92\"></g-img></a></div></div></div></div>",
# Some other elements in between ...
"Z Coffee 4.6(13) · € · Cafe Nicosia Takeaway"
],
"openai_key": "<OPENAI KEY>"
}
r = requests.post(url=uri, headers=headers, json=data)
print(r.json()["results"])
{
"results": [
{
"Address": "Nicosia",
"Description Or Review": "Counter-serve chain for coffee & snacks",
"Expensiveness": "€€",
"Number Of Reviews": "23",
"Rating": "4.1",
"Title": "X Coffee",
"Type": "Coffee shop"
},
{
"Address": "Nicosia",
"Description Or Review": "Iconic Seattle-based coffeehouse chain",
"Expensiveness": "€€",
"Number Of Reviews": "418",
"Rating": "4.0",
"Title": "Y Coffee",
"Type": "Coffee shop"
},
# Some Other Results in between ...
{
"Address": "Nicosia",
"Description Or Review": "Takeaway",
"Expensiveness": "€",
"Number Of Reviews": "13",
"Rating": "4.6",
"Title": "Z Coffee",
"Type": "Cafe"
}
]
}
In addition to preset parsers, designing your own parsers are also allowed in Lego AI Parser
. All that is needed is to provide a prompt
, examples
, and details about the OpenAI model
under classifier
key. Here is a breakdown of such custom parser:
{
"classifier": {
"main_prompt": "String, A prompt commanding the model to classify each item you desire. `NUMBER_OF_LABELS` is used to automatically determine the size of all unique labels in each example by `Lego AI Parser`."
"data": "Dictionary, Details of the model you want to employ. Same data field you would use in a normal OpenAI API call, excluding `max_tokens`",
"model_specific_token_size": "Integer, The maximum number of tokens allowed for the model. This is used to determine where to split multiple prompt calls in a given command. It is wise to set it just below the maximum number of tokens allowed by the model. For example, if the model allows 4000 tokens, you can set it to 3800. This is because the token count made by `Lego AI Parser` is determined by GPT-2 standards, and it might be higher than the actual token count of the model.",
"openai_endpoint": "String, Endpoint you want to call the model from. For example: `https://api.openai.com/v1/completions`",
"explicitly_excluded_strings": "List, A list of strings that you want to exclude from the results. For example, if you want to exclude new lines, you may add \"\n\" to the list.",
"examples_for_prompt": [
{
"text": "String, The text you want to classify.",
"classifications": {
"label_1": "String, The value of the label_1 for the given text.",
"label_2": "String, The value of the label_2 for the given text.",
# More Labels
}
},
# More examples
]
}
}
Here is an example script with a Custom Parser:
import requests
uri = "https://yourserver.com/classify"
headers = {"Content-Type": "application/json"}
data = {
"targets": [
"<div jscontroller=\"AtSb\" class=\"w7Dbne\" data-record-click-time=\"false\" id=\"tsuid_25\" jsdata=\"zt2wNd;_;BvbRxs V6f1Id;_;BvbRxw\" jsaction=\"rcuQ6b:npT2md;e3EWke:kN9HDb\" data-hveid=\"CBUQAA\"><div jsname=\"jXK9ad\" class=\"uMdZh tIxNaf\" jsaction=\"mouseover:UI3Kjd\"><div class=\"VkpGBb\"><div class=\"cXedhc\"><a class=\"vwVdIc wzN8Ac rllt__link a-no-hover-decoration\" jsname=\"kj0dLd\" data-cid=\"12176489206865957637\" jsaction=\"click:h5M12e;\" role=\"link\" tabindex=\"0\" data-ved=\"2ahUKEwiS1P3_j-P7AhXnVPEDHa0oAiAQvS56BAgVEAE\"><div><div class=\"rllt__details\"><div class=\"dbg0pd\" aria-level=\"3\" role=\"heading\"><span class=\"OSrXXb\">Y Coffee</span></div><div><span class=\"Y0A0hc\"><span class=\"yi40Hd YrbPuc\" aria-hidden=\"true\">4.0</span><span class=\"z3HNkc\" aria-label=\"Rated 4.0 out of 5,\" role=\"img\"><span style=\"width:56px\"></span></span><span class=\"RDApEe YrbPuc\">(418)</span></span> · <span aria-label=\"Moderately expensive\" role=\"img\">€€</span> · Coffee shop</div><div>Nicosia</div><div class=\"pJ3Ci\"><span>Iconic Seattle-based coffeehouse chain</span></div></div></div></a><a class=\"uQ4NLd b9tNq wzN8Ac rllt__link a-no-hover-decoration\" aria-hidden=\"true\" tabindex=\"-1\" jsname=\"kj0dLd\" data-cid=\"12176489206865957637\" jsaction=\"click:h5M12e;\" role=\"link\" data-ved=\"2ahUKEwiS1P3_j-P7AhXnVPEDHa0oAiAQvS56BAgVEA4\"><g-img class=\"gTrj3e\"><img id=\"pimg_3\" src=\"https://lh5.googleusercontent.com/p/AF1QipPaihclGQYWEJpMpBnBY8Nl8QWQVqZ6tF--MlwD=w184-h184-n-k-no\" class=\"YQ4gaf zr758c wA1Bge\" alt=\"\" data-atf=\"4\" data-frt=\"0\" width=\"92\" height=\"92\"></g-img></a></div></div></div></div>"
],
"openai_key": "<OPENAI KEY>",
"classifier": {
"main_prompt": "A table with NUMBER_OF_LABELS cells in each row summarizing the different parts of the text at each line even if they are not unique:\n\n",
"data": {
"model": "text-davinci-003",
"temperature": 0.001,
"top_p": 0.9,
"best_of": 2,
"frequency_penalty": 0,
"presence_penalty": 0
},
"model_specific_token_size": 3800,
"openai_endpoint": "https://api.openai.com/v1/completions",
"explicitly_excluded_strings": [
"Order",
"Website",
"Directions",
"\n"
],
"examples_for_prompt": [
{
"text": "Houndstooth Coffee 4.6(824) · $$ · Coffee shop 401 Congress Ave. #100c · In Frost Bank Tower Closed ⋅ Opens 7AM Cozy hangout for carefully sourced brews",
"classifications": {
"line": "1",
"title": "Houndstooth Coffee",
"rating": "4.1",
"number_of_reviews": "824",
"expensiveness": "$$",
"type": "Coffee Shop",
"address": "401 Congress Ave. #100c · In Frost Bank Tower",
"open_hours": "Opens 7AM",
"description_or_review": "Cozy hangout for carefully sourced brews"
}
},
# More examples ...
]
}
}
r = requests.post(url=uri, headers=headers, json=data)
print(r.json()["results"])
Custom Parser Result will be the same as the preset one:
{
"results": [
{
"Address": "Nicosia",
"Description Or Review": "Iconic Seattle-based coffeehouse chain",
"Expensiveness": "€€",
"Number Of Reviews": "418",
"Rating": "4.0",
"Title": "Y Coffee",
"Type": "Coffee shop"
}
]
}
You may also get arrays from your prompts by separating your results with a special double character, #$
. Here is an representation of such utility in product_options
key proivded in the example below:
{
# ...
"examples_for_prompt": [
{
"text": "Stumptown Coffee Roasters, Medium Roast Organic Whole Bean Coffee Gifts - Holler Mountain 12 Ounce Bag with Flavor Notes of Citrus Zest, Caramel and Hazelnut 12 Ounce 4.3 4.3 out of 5 stars (8,311) Options: 2 sizes, 6 flavors 2 sizes, 6 flavors Climate Pledge Friendly uses sustainability certifications to highlight products that support our commitment to help preserve the natural world. Time is fleeting. Learn more Product Certification (1) USDA Organic",
"classifications": {
"line": "3",
"title": "Stumptown Coffee Roasters, Medium Roast Organic Whole Bean Coffee Gifts - Holler Mountain 12 Ounce Bag with Flavor Notes of Citrus Zest, Caramel and Hazelnut",
"scale": "12 Ounce",
"rating": "4.3",
"reviews": "8,311",
"product_options": "2 sizes#$6 flavors#$",
"tags": "Climate Pledge Friendly#$USDA Organic#$"
}
},
#...
]
#...
}
Constructing a custom parser with such example will result in the following structure:
{
"results": [
{
"Line": "X",
"Product Options": [
"X",
"X"
],
"Rating": "X",
"Reviews": "X",
"Scale": "X",
"Tags": [
"X",
"X"
],
"Title": "X"
}
]
}
You can get only the prompts you need to call the OpenAI endpoint with prompts_only
key.
import requests
uri = "https://yourserver.com/classify"
headers = {"Content-Type": "application/json"}
data = {
"prompts_only": True,
"path": "google.google_local_results",
"targets": [
"<div jscontroller=\"AtSb\" class=\"w7Dbne\" data-record-click-time=\"false\" id=\"tsuid_25\" jsdata=\"zt2wNd;_;BvbRxs V6f1Id;_;BvbRxw\" jsaction=\"rcuQ6b:npT2md;e3EWke:kN9HDb\" data-hveid=\"CBUQAA\"><div jsname=\"jXK9ad\" class=\"uMdZh tIxNaf\" jsaction=\"mouseover:UI3Kjd\"><div class=\"VkpGBb\"><div class=\"cXedhc\"><a class=\"vwVdIc wzN8Ac rllt__link a-no-hover-decoration\" jsname=\"kj0dLd\" data-cid=\"12176489206865957637\" jsaction=\"click:h5M12e;\" role=\"link\" tabindex=\"0\" data-ved=\"2ahUKEwiS1P3_j-P7AhXnVPEDHa0oAiAQvS56BAgVEAE\"><div><div class=\"rllt__details\"><div class=\"dbg0pd\" aria-level=\"3\" role=\"heading\"><span class=\"OSrXXb\">Y Coffee</span></div><div><span class=\"Y0A0hc\"><span class=\"yi40Hd YrbPuc\" aria-hidden=\"true\">4.0</span><span class=\"z3HNkc\" aria-label=\"Rated 4.0 out of 5,\" role=\"img\"><span style=\"width:56px\"></span></span><span class=\"RDApEe YrbPuc\">(418)</span></span> · <span aria-label=\"Moderately expensive\" role=\"img\">€€</span> · Coffee shop</div><div>Nicosia</div><div class=\"pJ3Ci\"><span>Iconic Seattle-based coffeehouse chain</span></div></div></div></a><a class=\"uQ4NLd b9tNq wzN8Ac rllt__link a-no-hover-decoration\" aria-hidden=\"true\" tabindex=\"-1\" jsname=\"kj0dLd\" data-cid=\"12176489206865957637\" jsaction=\"click:h5M12e;\" role=\"link\" data-ved=\"2ahUKEwiS1P3_j-P7AhXnVPEDHa0oAiAQvS56BAgVEA4\"><g-img class=\"gTrj3e\"><img id=\"pimg_3\" src=\"https://lh5.googleusercontent.com/p/AF1QipPaihclGQYWEJpMpBnBY8Nl8QWQVqZ6tF--MlwD=w184-h184-n-k-no\" class=\"YQ4gaf zr758c wA1Bge\" alt=\"\" data-atf=\"4\" data-frt=\"0\" width=\"92\" height=\"92\"></g-img></a></div></div></div></div>"
]
}
r = requests.post(url=uri, headers=headers, json=data)
print(r.json())
Here is the breakdown of the response of such a call:
{
"prompts": [
"String, Individual Prompts You need to call OpenAI endpoint with. Separated into multiple calls if the calls exceed the maximum number of tokens allowed by the endpoint."
],
"prompt_objects": {
"invalid_lines_indexes": "List, An array of elements that their texts are already exceeding the allowed threshold. These results will be skipped and will be returned with an error in the final response.",
"desired_lines": "List, An array of text contents of HTML elements.",
"labels": "List, An array of labels the user wants to classify from."
}
}
Here is an example response:
{
"prompts": [
"A table with 12 cells in each row summarizing the different parts of the text at each line:\n\nHoundstooth Coffee 4.6(824) · $$ · Coffee shop 401 Congress Ave. #100c · In Frost Bank Tower Closed ⋅ Opens 7AM Cozy hangout for carefully sourced brews\nStarbucks 4.4(471) · $$ · Coffee shop 301 W 3rd St Opens soon ⋅ 5:30AM Iconic Seattle-based coffeehouse chain\nProgress Coffee Bank of America Building 5.0(1) · Cafe 515 Congress Ave. Closed ⋅ Opens 7AM Dine-in·Takeout·No delivery\nCoffee Cantata Nicosia 5.0(3) · Tea store Nicosia Closed ⋅ Opens 10AM Mon In-store shopping\nLa Bella Bakery - Gloria Jean's Coffees K. Kaymaklı 4.4(251) · €€ · Coffee shop Şehit mustafa Ruso Caddesi no:148 - Küçük Kaymaklı - Lefkoşa - KKTC Mersin 10 Turkey Lefkoşa · In Aydın Oto Camları & Döşeme Ltd. On the menu: tea\nA.D.A. Auto Repair Center 4.8(26) · Auto repair shop 30+ years in business · Nicosia · 99 639471 Closes soon ⋅ 3PM \"I strongly recommend this repair shop.\"\nEvolution GYM No reviews · Gym Nicosia · +90 533 821 10 02 Open ⋅ Closes 6PM\nA McDonald's 420 Fulton St · (929) 431-6994 Open ⋅ Closes 1AM Dine-in · Curbside pickup · No-contact delivery\nY Coffee 4.0 (418) · €€ · Coffee shop Nicosia Iconic Seattle-based coffeehouse chain\n| Address | Description Or Review | Expensiveness | Line | Number Of Reviews | Open Hours | Rating | Title | Type | Delivery Options | Phone | Years Of Business |\n| --- | --- | --- | --- | --- | --- | --- | --- | --- | --- | --- | --- |\n| 401 Congress Ave. #100c · In Frost Bank Tower | Cozy hangout for carefully sourced brews | $$ | 1 | 824 | Opens 7AM | 4.1 | Houndstooth Coffee | Coffee Shop | - | - | - |\n| 301 W 3rd St | Iconic Seattle-based coffeehouse chain | $$ | 2 | 471 | Opens soon ⋅ 5:30AM | 4.1 | Starbucks | Coffee Shop | - | - | - |\n| 515 Congress Ave. | - | - | 3 | 1 | Closed ⋅ Opens 7AM | 5.0 | Progress Coffee Bank of America Building | Cafe | Dine-in·Takeout·No delivery | - | - |\n| Nicosia | - | - | 4 | 3 | Closed ⋅ Opens 10AM Mon | 5.0 | Coffee Cantata Nicosia | Tea store | In-store shopping | - | - |\n| Şehit mustafa Ruso Caddesi no:148 - Küçük Kaymaklı - Lefkoşa - KKTC Mersin 10 Turkey Lefkoşa · In Aydın Oto Camları & Döşeme Ltd. | On the menu: tea | €€ | 5 | 251 | - | 4.4 | La Bella Bakery - Gloria Jean's Coffees K. Kaymaklı | Coffee shop | - | - | - |\n| Nicosia | \"I strongly recommend this repair shop.\" | - | 6 | 26 | Closes soon ⋅ 3PM | 4.8 | A.D.A. Auto Repair Center | Auto repair shop | - | 99 648261 | 30+ years in business |\n| Nicosia | - | - | 7 | - | Closes 6PM | - | Evolution GYM | Gym | - | +90 555 827 11 12 | - |\n| 420 Fulton St | - | - | 8 | - | Open ⋅ Closes 1AM | A | McDonald's | - | Dine-in · Curbside pickup · No-contact delivery | (959) 451-6894 | - |"
],
"prompt_objects": {
"invalid_lines_indexes": [],
"desired_lines": [
"Y Coffee 4.0 (418) · €€ · Coffee shop Nicosia Iconic Seattle-based coffeehouse chain"
],
"labels": [
"Address",
"Description Or Review",
"Expensiveness",
"Line",
"Number Of Reviews",
"Open Hours",
"Rating",
"Title",
"Type",
"Delivery Options",
"Phone",
"Years Of Business"
]
},
}
You can make the calls to OpenAI from your server-side code. The adjustment of parameters to a model should be taken as the same with preset parser you use, or the custom parser you have provided. The max_tokens
needs to be calculated on server-side per each-call. Here is an example on making a server-side call:
import os
import openai
import requests
uri = "https://yourserver.com/classify"
headers = {"Content-Type": "application/json"}
data = {
"prompts_only": True,
"path": "google.google_local_results",
"targets": [
"<div jscontroller=\"AtSb\" class=\"w7Dbne\" data-record-click-time=\"false\" id=\"tsuid_25\" jsdata=\"zt2wNd;_;BvbRxs V6f1Id;_;BvbRxw\" jsaction=\"rcuQ6b:npT2md;e3EWke:kN9HDb\" data-hveid=\"CBUQAA\"><div jsname=\"jXK9ad\" class=\"uMdZh tIxNaf\" jsaction=\"mouseover:UI3Kjd\"><div class=\"VkpGBb\"><div class=\"cXedhc\"><a class=\"vwVdIc wzN8Ac rllt__link a-no-hover-decoration\" jsname=\"kj0dLd\" data-cid=\"12176489206865957637\" jsaction=\"click:h5M12e;\" role=\"link\" tabindex=\"0\" data-ved=\"2ahUKEwiS1P3_j-P7AhXnVPEDHa0oAiAQvS56BAgVEAE\"><div><div class=\"rllt__details\"><div class=\"dbg0pd\" aria-level=\"3\" role=\"heading\"><span class=\"OSrXXb\">Y Coffee</span></div><div><span class=\"Y0A0hc\"><span class=\"yi40Hd YrbPuc\" aria-hidden=\"true\">4.0</span><span class=\"z3HNkc\" aria-label=\"Rated 4.0 out of 5,\" role=\"img\"><span style=\"width:56px\"></span></span><span class=\"RDApEe YrbPuc\">(418)</span></span> · <span aria-label=\"Moderately expensive\" role=\"img\">€€</span> · Coffee shop</div><div>Nicosia</div><div class=\"pJ3Ci\"><span>Iconic Seattle-based coffeehouse chain</span></div></div></div></a><a class=\"uQ4NLd b9tNq wzN8Ac rllt__link a-no-hover-decoration\" aria-hidden=\"true\" tabindex=\"-1\" jsname=\"kj0dLd\" data-cid=\"12176489206865957637\" jsaction=\"click:h5M12e;\" role=\"link\" data-ved=\"2ahUKEwiS1P3_j-P7AhXnVPEDHa0oAiAQvS56BAgVEA4\"><g-img class=\"gTrj3e\"><img id=\"pimg_3\" src=\"https://lh5.googleusercontent.com/p/AF1QipPaihclGQYWEJpMpBnBY8Nl8QWQVqZ6tF--MlwD=w184-h184-n-k-no\" class=\"YQ4gaf zr758c wA1Bge\" alt=\"\" data-atf=\"4\" data-frt=\"0\" width=\"92\" height=\"92\"></g-img></a></div></div></div></div>"
]
}
response_from_lego_ai_parser = requests.post(url=uri, headers=headers, json=data)
openai.api_key = os.getenv("OPENAI_API_KEY")
prompts = response_from_lego_ai_parser["prompts"]
responses = []
for prompt in prompts:
response = openai.Completion.create(
model="text-davinci-003",
prompt=prompt,
temperature=0.001,
max_tokens=400,
top_p=0.9,
best_of=2,
frequency_penalty=0,
presence_penalty=0
)
responses.append(response)
print(responses)
You can gather the responses on the server-side and then make a call with parse_only
to get the parsed results. Here is an example on making a parse_only
call:
import os
import openai
import requests
# Prompts Only Call
uri = "https://yourserver.com/classify"
headers = {"Content-Type": "application/json"}
data = {
"prompts_only": True,
"path": "google.google_local_results",
"targets": [
"<div jscontroller=\"AtSb\" class=\"w7Dbne\" data-record-click-time=\"false\" id=\"tsuid_25\" jsdata=\"zt2wNd;_;BvbRxs V6f1Id;_;BvbRxw\" jsaction=\"rcuQ6b:npT2md;e3EWke:kN9HDb\" data-hveid=\"CBUQAA\"><div jsname=\"jXK9ad\" class=\"uMdZh tIxNaf\" jsaction=\"mouseover:UI3Kjd\"><div class=\"VkpGBb\"><div class=\"cXedhc\"><a class=\"vwVdIc wzN8Ac rllt__link a-no-hover-decoration\" jsname=\"kj0dLd\" data-cid=\"12176489206865957637\" jsaction=\"click:h5M12e;\" role=\"link\" tabindex=\"0\" data-ved=\"2ahUKEwiS1P3_j-P7AhXnVPEDHa0oAiAQvS56BAgVEAE\"><div><div class=\"rllt__details\"><div class=\"dbg0pd\" aria-level=\"3\" role=\"heading\"><span class=\"OSrXXb\">Y Coffee</span></div><div><span class=\"Y0A0hc\"><span class=\"yi40Hd YrbPuc\" aria-hidden=\"true\">4.0</span><span class=\"z3HNkc\" aria-label=\"Rated 4.0 out of 5,\" role=\"img\"><span style=\"width:56px\"></span></span><span class=\"RDApEe YrbPuc\">(418)</span></span> · <span aria-label=\"Moderately expensive\" role=\"img\">€€</span> · Coffee shop</div><div>Nicosia</div><div class=\"pJ3Ci\"><span>Iconic Seattle-based coffeehouse chain</span></div></div></div></a><a class=\"uQ4NLd b9tNq wzN8Ac rllt__link a-no-hover-decoration\" aria-hidden=\"true\" tabindex=\"-1\" jsname=\"kj0dLd\" data-cid=\"12176489206865957637\" jsaction=\"click:h5M12e;\" role=\"link\" data-ved=\"2ahUKEwiS1P3_j-P7AhXnVPEDHa0oAiAQvS56BAgVEA4\"><g-img class=\"gTrj3e\"><img id=\"pimg_3\" src=\"https://lh5.googleusercontent.com/p/AF1QipPaihclGQYWEJpMpBnBY8Nl8QWQVqZ6tF--MlwD=w184-h184-n-k-no\" class=\"YQ4gaf zr758c wA1Bge\" alt=\"\" data-atf=\"4\" data-frt=\"0\" width=\"92\" height=\"92\"></g-img></a></div></div></div></div>"
]
}
response_from_lego_ai_parser = requests.post(url=uri, headers=headers, json=data)
openai.api_key = os.getenv("OPENAI_API_KEY")
prompts = response_from_lego_ai_parser["prompts"]
responses = []
# Server-Side Call to OpenAI
for prompt in prompts:
response = openai.Completion.create(
model="text-davinci-003",
prompt=prompt,
temperature=0.001,
max_tokens=400,
top_p=0.9,
best_of=2,
frequency_penalty=0,
presence_penalty=0
)
responses.append(response)
# Parse Only Call
data = {
"path": "google.google_local_results",
"parse_only": {
"responses": responses
"prompt_objects": response_from_lego_ai_parser["prompt_objects"]
}
}
response_from_lego_ai_parser = requests.post(url=uri, headers=headers, json=data)
print(response_from_lego_ai_parser.json())
Here is an example response with parse only:
{
"results": [
{
"Address": "Nicosia",
"Description Or Review": "Iconic Seattle-based coffeehouse chain",
"Expensiveness": "€€",
"Number Of Reviews": "418",
"Rating": "4.0",
"Title": "Y Coffee",
"Type": "Coffee shop"
}
]
}
Different OpenAI Errors are served in the response to save the user trouble of looking back and forth:
{
"results": [
{
"message": "Incorrect API key provided: <Your Op*****Key>. You can find your API key at https://beta.openai.com.",
"type": "invalid_request_error",
"param": null,
"code": "invalid_api_key"
}
]
}
{
"results": [
{
"message": "You exceeded your current quota, please check your plan and billing details.",
"type": "insufficient_quota",
"param": null,
"code": null
}
]
}
If there is a communication error in hosted endpoint for one or more of the concurrent requests, it will results in the following form:
{
"results": [
{"error": "Error from Local Machine"}
]
}
If the element you have passed already exceeds the maximum token size, the error will be in the following form:
{
"results": [
{"error": "Maximum Token Size is reached for this prompt. This is skipped."}
]
}
If there are any other errors you encounter, feel free to create an issue about them.
You can adjust the number of allowed concurrency for the client-side calls with allowed_concurrency
key. The maximum number of calls you can make per minute is still need to be configured by you. You may put sleep time between calls to Lego AI Parser
to avoid exceeding the limit imposed by OpenAI. Here is an example script where allowed concurrency is 2:
import requests
uri = "https://yourserver.com/classify"
headers = {"Content-Type": "application/json"}
data = {
"allowed_concurrency": 2,
"path": "google.google_local_results",
"targets": [
"<div jscontroller=\"AtSb\" class=\"w7Dbne\" data-record-click-time=\"false\" id=\"tsuid_25\" jsdata=\"zt2wNd;_;BvbRxs V6f1Id;_;BvbRxw\" jsaction=\"rcuQ6b:npT2md;e3EWke:kN9HDb\" data-hveid=\"CBUQAA\"><div jsname=\"jXK9ad\" class=\"uMdZh tIxNaf\" jsaction=\"mouseover:UI3Kjd\"><div class=\"VkpGBb\"><div class=\"cXedhc\"><a class=\"vwVdIc wzN8Ac rllt__link a-no-hover-decoration\" jsname=\"kj0dLd\" data-cid=\"12176489206865957637\" jsaction=\"click:h5M12e;\" role=\"link\" tabindex=\"0\" data-ved=\"2ahUKEwiS1P3_j-P7AhXnVPEDHa0oAiAQvS56BAgVEAE\"><div><div class=\"rllt__details\"><div class=\"dbg0pd\" aria-level=\"3\" role=\"heading\"><span class=\"OSrXXb\">Y Coffee</span></div><div><span class=\"Y0A0hc\"><span class=\"yi40Hd YrbPuc\" aria-hidden=\"true\">4.0</span><span class=\"z3HNkc\" aria-label=\"Rated 4.0 out of 5,\" role=\"img\"><span style=\"width:56px\"></span></span><span class=\"RDApEe YrbPuc\">(418)</span></span> · <span aria-label=\"Moderately expensive\" role=\"img\">€€</span> · Coffee shop</div><div>Nicosia</div><div class=\"pJ3Ci\"><span>Iconic Seattle-based coffeehouse chain</span></div></div></div></a><a class=\"uQ4NLd b9tNq wzN8Ac rllt__link a-no-hover-decoration\" aria-hidden=\"true\" tabindex=\"-1\" jsname=\"kj0dLd\" data-cid=\"12176489206865957637\" jsaction=\"click:h5M12e;\" role=\"link\" data-ved=\"2ahUKEwiS1P3_j-P7AhXnVPEDHa0oAiAQvS56BAgVEA4\"><g-img class=\"gTrj3e\"><img id=\"pimg_3\" src=\"https://lh5.googleusercontent.com/p/AF1QipPaihclGQYWEJpMpBnBY8Nl8QWQVqZ6tF--MlwD=w184-h184-n-k-no\" class=\"YQ4gaf zr758c wA1Bge\" alt=\"\" data-atf=\"4\" data-frt=\"0\" width=\"92\" height=\"92\"></g-img></a></div></div></div></div>"
],
"openai_key": "<OPENAI KEY>"
}
r = requests.post(url=uri, headers=headers, json=data)
print(r.json()["results"])
By default, allowed concurrency is 1
. You can change the default allowed_concurrency
and default openai_key
from credentials.py
when you set your own server.
For recent updates, head to Contributions Guide Page
If you want to contribute to this project, you can open a pull request. You can also create an issue if you have any questions or suggestions.
All kinds of bug reports, suggestions, and feature requests are welcomed. Head to Issues to keep track of the progress, or contribute to it.
You can design a prompt in OpenAI Playground that creates a table such as this:
And then you can turn it into a dictinary form as following example:
# app/classify/parsers/google/google_local_results.py
from app.schemas import *
def commands():
return json_to_pydantic({
"main_prompt": "A table with NUMBER_OF_LABELS cells in each row summarizing the different parts of the text at each line:\n\n",
"data": {
"model": "text-davinci-003",
"temperature": 0.001,
"top_p": 0.9,
"best_of": 2,
"frequency_penalty": 0,
"presence_penalty": 0
},
"model_specific_token_size": 3800,
"openai_endpoint": "https://api.openai.com/v1/completions",
"explicitly_excluded_strings": [
"Order",
"Website",
"Directions",
"\n"
],
"examples_for_prompt": [
{
"text": "Houndstooth Coffee 4.6(824) · $$ · Coffee shop 401 Congress Ave. #100c · In Frost Bank Tower Closed ⋅ Opens 7AM Cozy hangout for carefully sourced brews",
"classifications": {
"line": "1",
"title": "Houndstooth Coffee",
"rating": "4.1",
"number_of_reviews": "824",
"expensiveness": "$$",
"type": "Coffee Shop",
"address": "401 Congress Ave. #100c · In Frost Bank Tower",
"open_hours": "Opens 7AM",
"description_or_review": "Cozy hangout for carefully sourced brews"
}
},
# More examples ...
]
})
You can add unit tests to your contribution easily with mock_name
.
Point the results to app/classify/tests/data/results
folder, or prompts to app/classify/tests/data/prompts
folder, depending on whatever end result you are getting inside the unit test.
# app/classify/tests/unit_tests/test_google_local_results.py
# ...
def test_google_local_results_successful_response():
targets = [
"app/classify/tests/data/targets/electronic-shops-successful.json"
]
for target_filename in targets:
with open(target_filename) as json_file:
target = json.load(json_file)
r = client.post("/classify", json=target)
result_filename = target['mock_name'].replace('.json','-result.json')
result_filename = result_filename.replace('/targets/', '/results/')
with open(result_filename) as json_file:
result = json.load(json_file)
assert r.status_code == 200
assert r.json() == result
assert len(r.json()['results']) > 0
assert ("message" not in r.json()['results'][0])
# ...
mock_name
should contain the path of the file itself.
# app/classify/tests/data/targets/coffee-shops-successful.json
{
"path": "google.google_local_results",
"targets": [
"<div jscontroller=\"AtSb\" class=\"w7Dbne\" data-record-click-time=\"false\" id=\"tsuid_25\" jsdata=\"zt2wNd;_;BvbRxs V6f1Id;_;BvbRxw\" jsaction=\"rcuQ6b:npT2md;e3EWke:kN9HDb\" data-hveid=\"CBUQAA\"><div jsname=\"jXK9ad\" class=\"uMdZh tIxNaf\" jsaction=\"mouseover:UI3Kjd\"><div class=\"VkpGBb\"><div class=\"cXedhc\"><a class=\"vwVdIc wzN8Ac rllt__link a-no-hover-decoration\" jsname=\"kj0dLd\" data-cid=\"12176489206865957637\" jsaction=\"click:h5M12e;\" role=\"link\" tabindex=\"0\" data-ved=\"2ahUKEwiS1P3_j-P7AhXnVPEDHa0oAiAQvS56BAgVEAE\"><div><div class=\"rllt__details\"><div class=\"dbg0pd\" aria-level=\"3\" role=\"heading\"><span class=\"OSrXXb\">Y Coffee</span></div><div><span class=\"Y0A0hc\"><span class=\"yi40Hd YrbPuc\" aria-hidden=\"true\">4.0</span><span class=\"z3HNkc\" aria-label=\"Rated 4.0 out of 5,\" role=\"img\"><span style=\"width:56px\"></span></span><span class=\"RDApEe YrbPuc\">(418)</span></span> · <span aria-label=\"Moderately expensive\" role=\"img\">€€</span> · Coffee shop</div><div>Nicosia</div><div class=\"pJ3Ci\"><span>Iconic Seattle-based coffeehouse chain</span></div></div></div></a><a class=\"uQ4NLd b9tNq wzN8Ac rllt__link a-no-hover-decoration\" aria-hidden=\"true\" tabindex=\"-1\" jsname=\"kj0dLd\" data-cid=\"12176489206865957637\" jsaction=\"click:h5M12e;\" role=\"link\" data-ved=\"2ahUKEwiS1P3_j-P7AhXnVPEDHa0oAiAQvS56BAgVEA4\"><g-img class=\"gTrj3e\"><img id=\"pimg_3\" src=\"https://lh5.googleusercontent.com/p/AF1QipPaihclGQYWEJpMpBnBY8Nl8QWQVqZ6tF--MlwD=w184-h184-n-k-no\" class=\"YQ4gaf zr758c wA1Bge\" alt=\"\" data-atf=\"4\" data-frt=\"0\" width=\"92\" height=\"92\"></g-img></a></div></div></div></div>"
],
"mock_name": "app/classify/tests/data/results/coffee-shops-successful.json"
}
They will only be created in the initial call not to exhaust credits in testing. Here is an example result:
# app/classify/tests/data/results/coffee-shops-successful-result.json
{
"results": [
{
"Address": "Nicosia",
"Description Or Review": "Iconic Seattle-based coffeehouse chain",
"Expensiveness": "€€",
"Number Of Reviews": "418",
"Rating": "4.0",
"Title": "Y Coffee",
"Type": "Coffee shop"
}
]
}
For Tasks:
Click tags to check more tools for each tasksFor Jobs:
Alternative AI tools for lego-ai-parser
Similar Open Source Tools
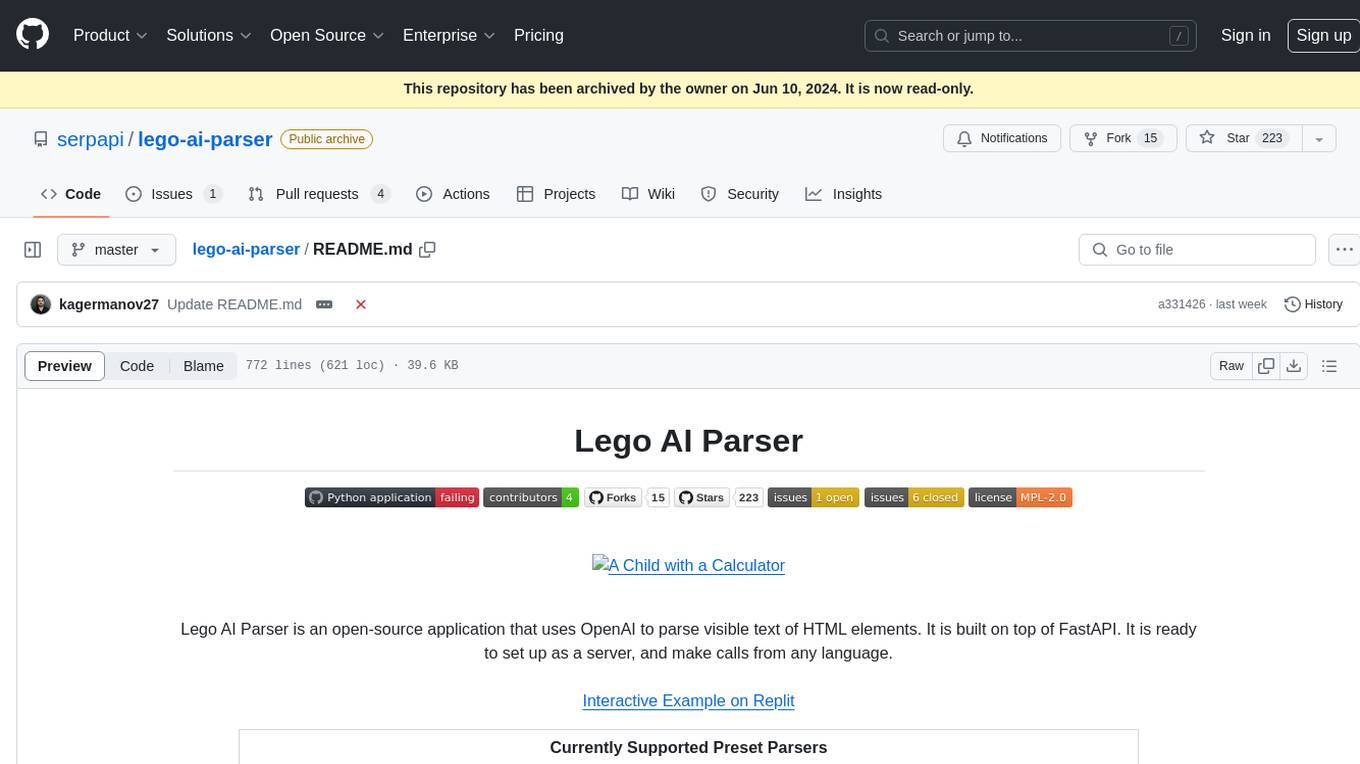
lego-ai-parser
Lego AI Parser is an open-source application that uses OpenAI to parse visible text of HTML elements. It is built on top of FastAPI, ready to set up as a server, and make calls from any language. It supports preset parsers for Google Local Results, Amazon Listings, Etsy Listings, Wayfair Listings, BestBuy Listings, Costco Listings, Macy's Listings, and Nordstrom Listings. Users can also design custom parsers by providing prompts, examples, and details about the OpenAI model under the classifier key.
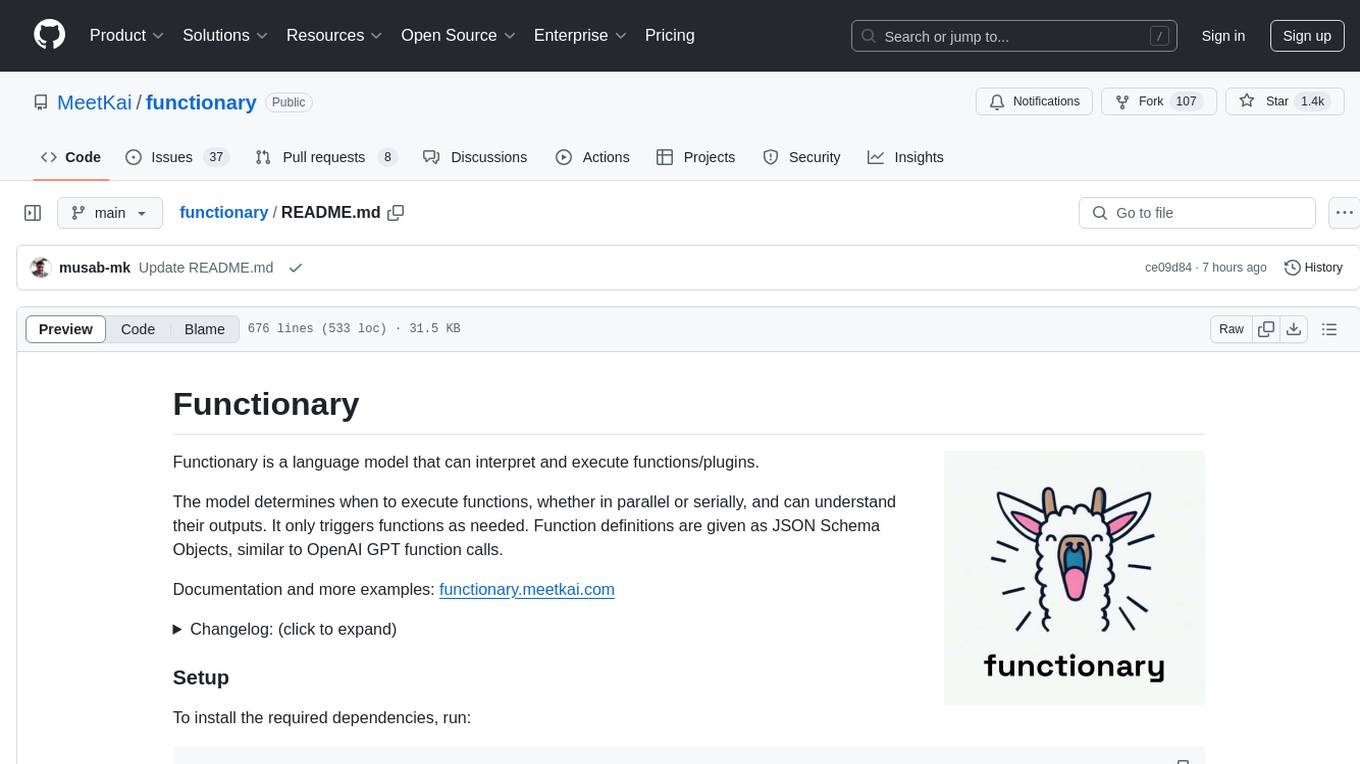
functionary
Functionary is a language model that interprets and executes functions/plugins. It determines when to execute functions, whether in parallel or serially, and understands their outputs. Function definitions are given as JSON Schema Objects, similar to OpenAI GPT function calls. It offers documentation and examples on functionary.meetkai.com. The newest model, meetkai/functionary-medium-v3.1, is ranked 2nd in the Berkeley Function-Calling Leaderboard. Functionary supports models with different context lengths and capabilities for function calling and code interpretation. It also provides grammar sampling for accurate function and parameter names. Users can deploy Functionary models serverlessly using Modal.com.
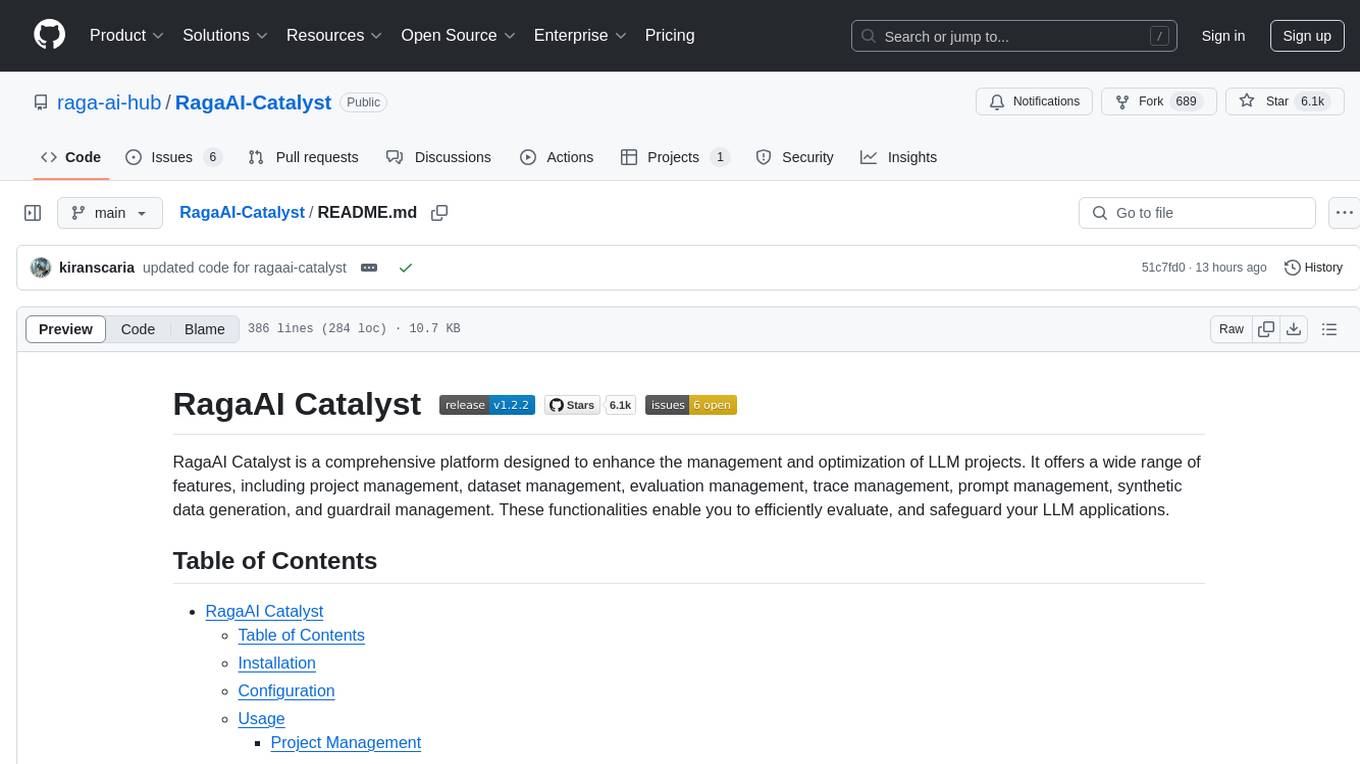
RagaAI-Catalyst
RagaAI Catalyst is a comprehensive platform designed to enhance the management and optimization of LLM projects. It offers features such as project management, dataset management, evaluation management, trace management, prompt management, synthetic data generation, and guardrail management. These functionalities enable efficient evaluation and safeguarding of LLM applications.
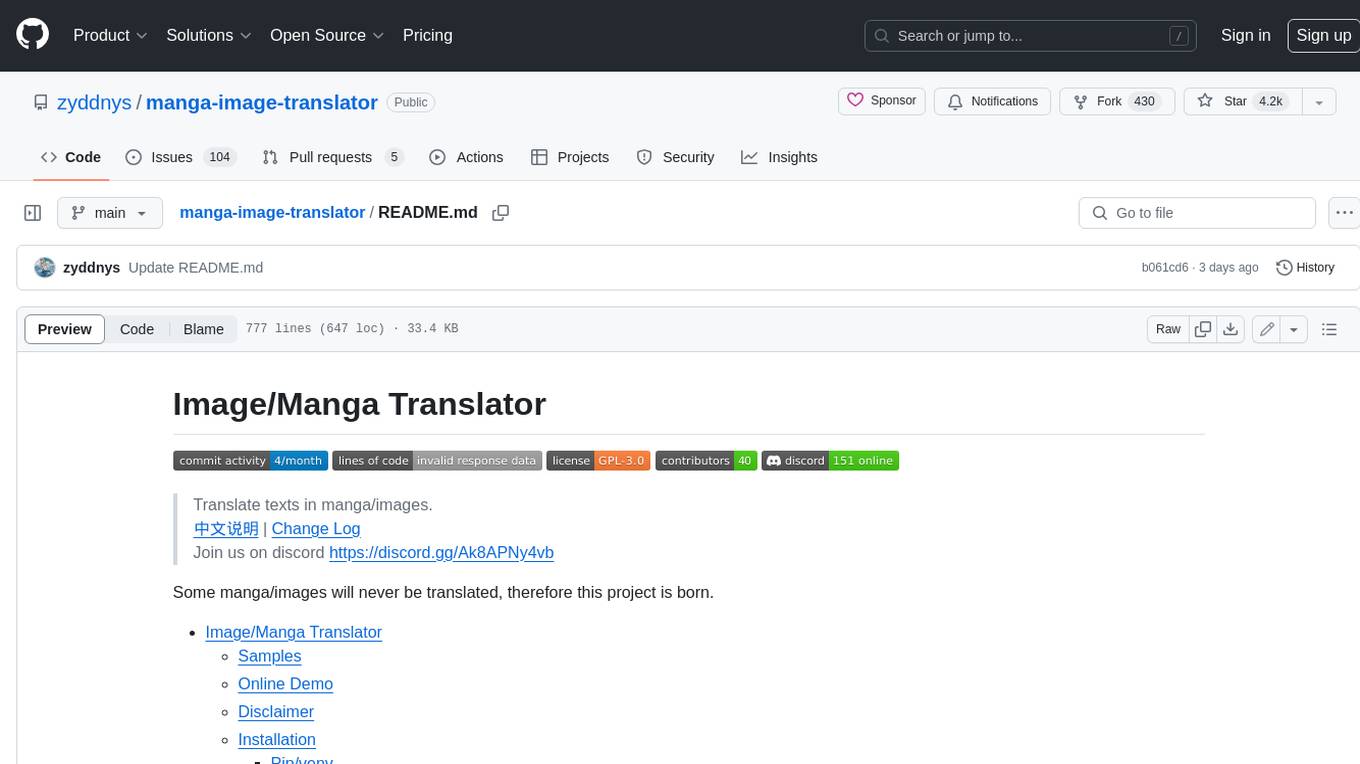
manga-image-translator
Translate texts in manga/images. Some manga/images will never be translated, therefore this project is born. * Image/Manga Translator * Samples * Online Demo * Disclaimer * Installation * Pip/venv * Poetry * Additional instructions for **Windows** * Docker * Hosting the web server * Using as CLI * Setting Translation Secrets * Using with Nvidia GPU * Building locally * Usage * Batch mode (default) * Demo mode * Web Mode * Api Mode * Related Projects * Docs * Recommended Modules * Tips to improve translation quality * Options * Language Code Reference * Translators Reference * GPT Config Reference * Using Gimp for rendering * Api Documentation * Synchronous mode * Asynchronous mode * Manual translation * Next steps * Support Us * Thanks To All Our Contributors :
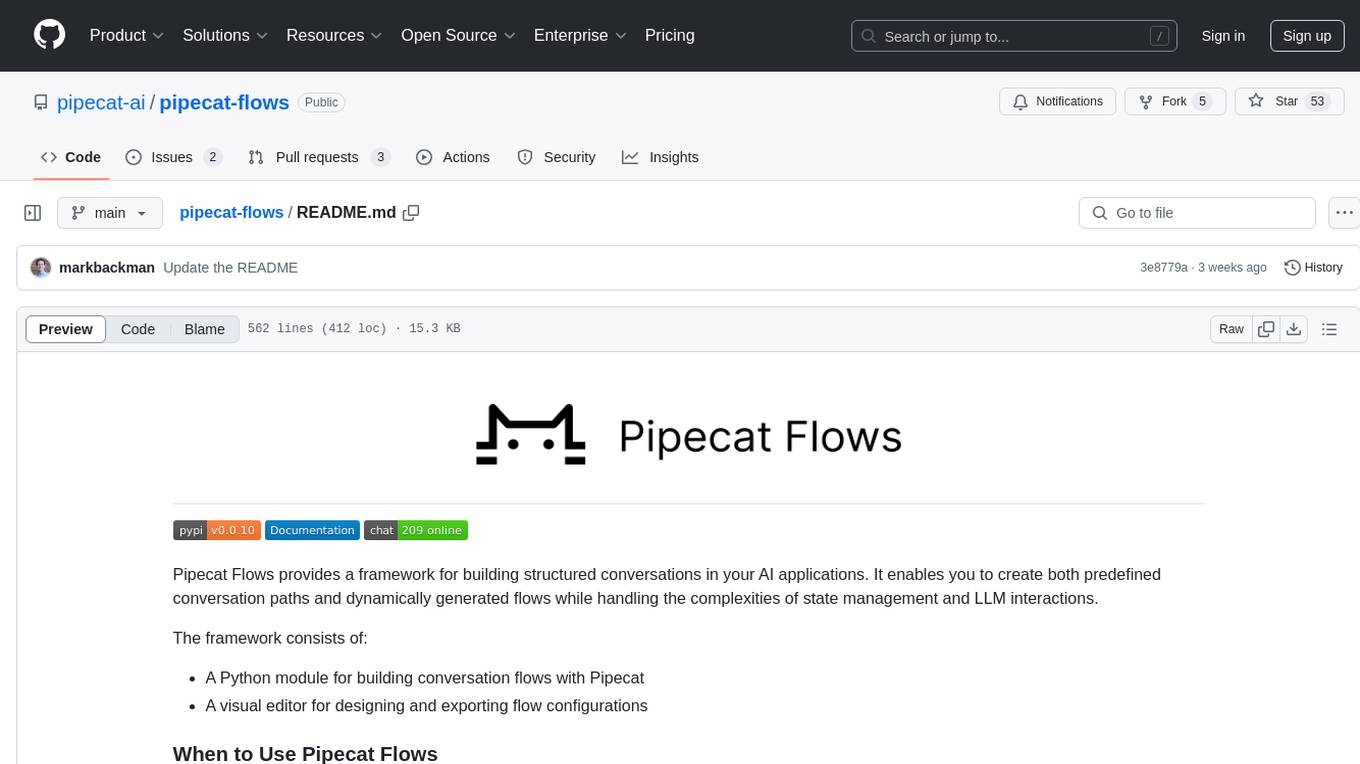
pipecat-flows
Pipecat Flows is a framework designed for building structured conversations in AI applications. It allows users to create both predefined conversation paths and dynamically generated flows, handling state management and LLM interactions. The framework includes a Python module for building conversation flows and a visual editor for designing and exporting flow configurations. Pipecat Flows is suitable for scenarios such as customer service scripts, intake forms, personalized experiences, and complex decision trees.
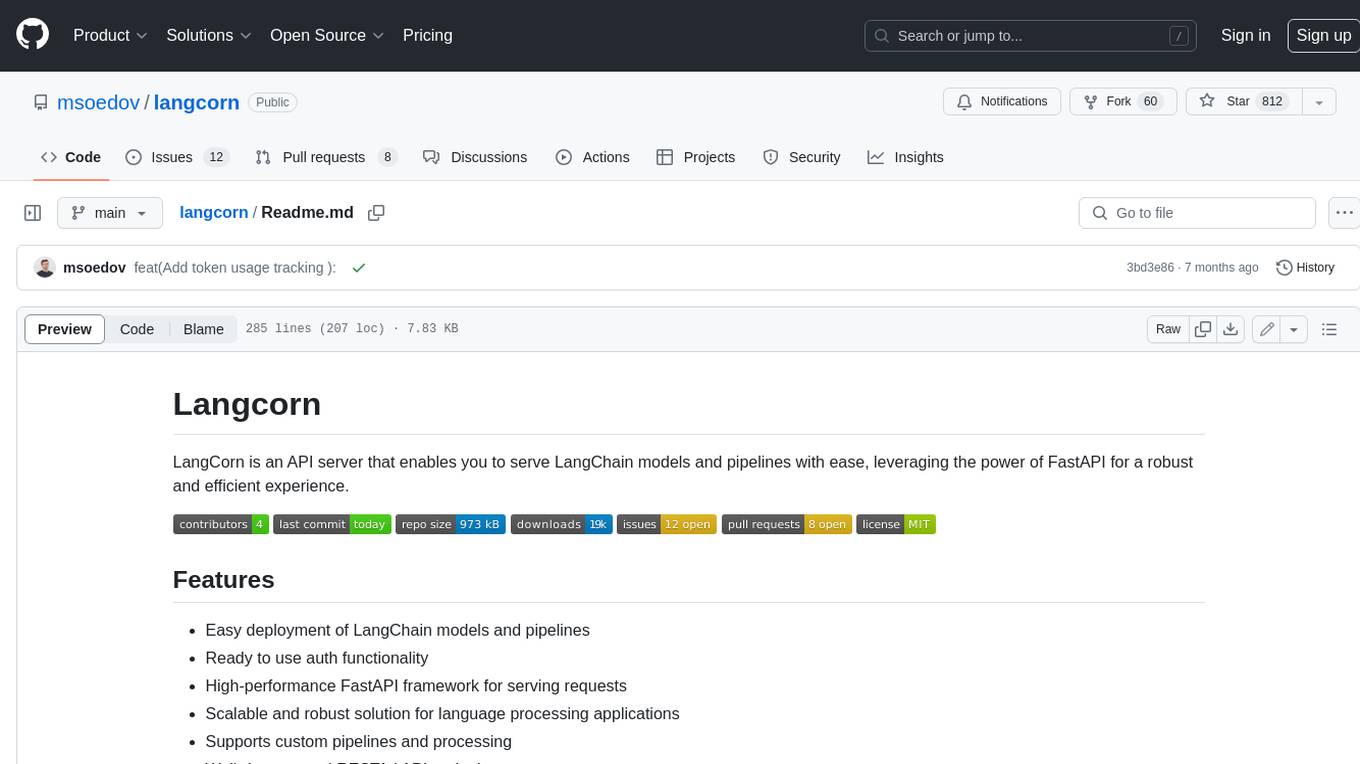
langcorn
LangCorn is an API server that enables you to serve LangChain models and pipelines with ease, leveraging the power of FastAPI for a robust and efficient experience. It offers features such as easy deployment of LangChain models and pipelines, ready-to-use authentication functionality, high-performance FastAPI framework for serving requests, scalability and robustness for language processing applications, support for custom pipelines and processing, well-documented RESTful API endpoints, and asynchronous processing for faster response times.
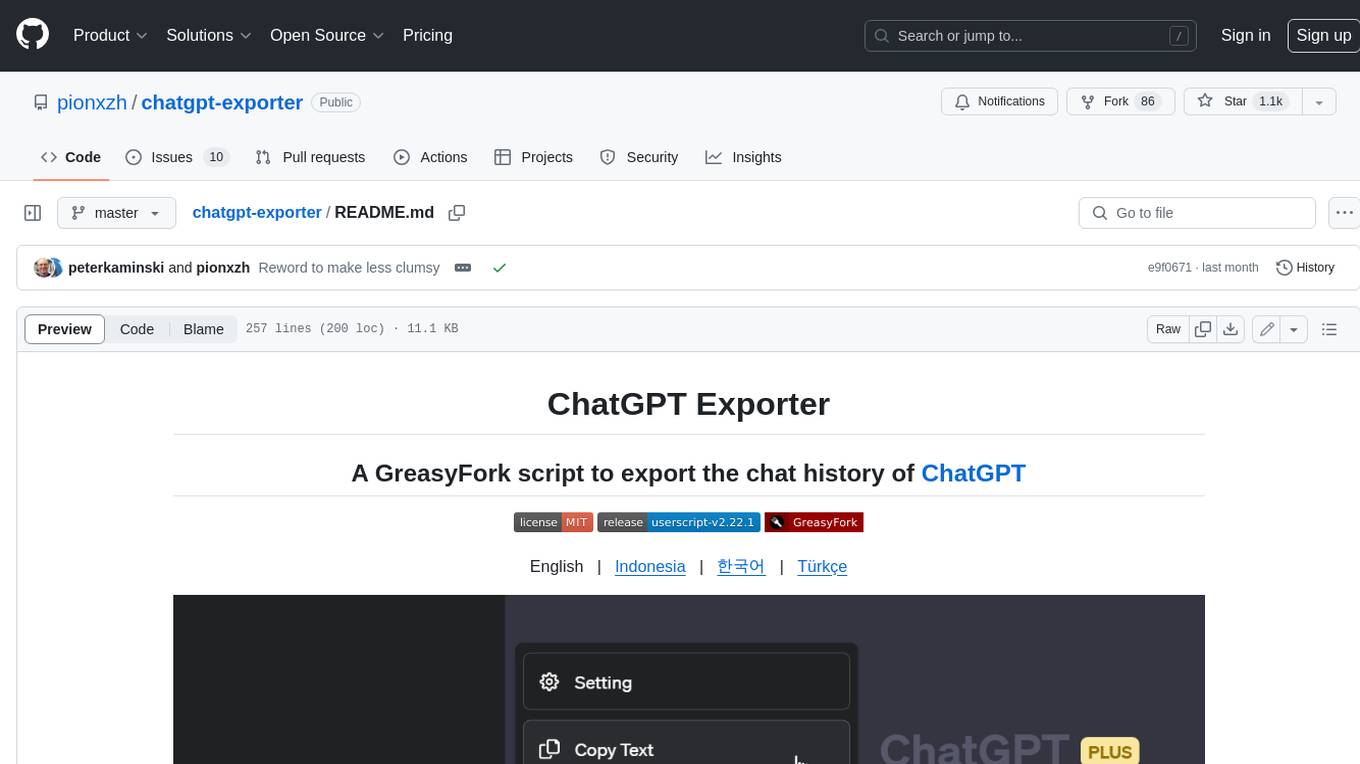
chatgpt-exporter
A script to export the chat history of ChatGPT. Supports exporting to text, HTML, Markdown, PNG, and JSON formats. Also allows for exporting multiple conversations at once.
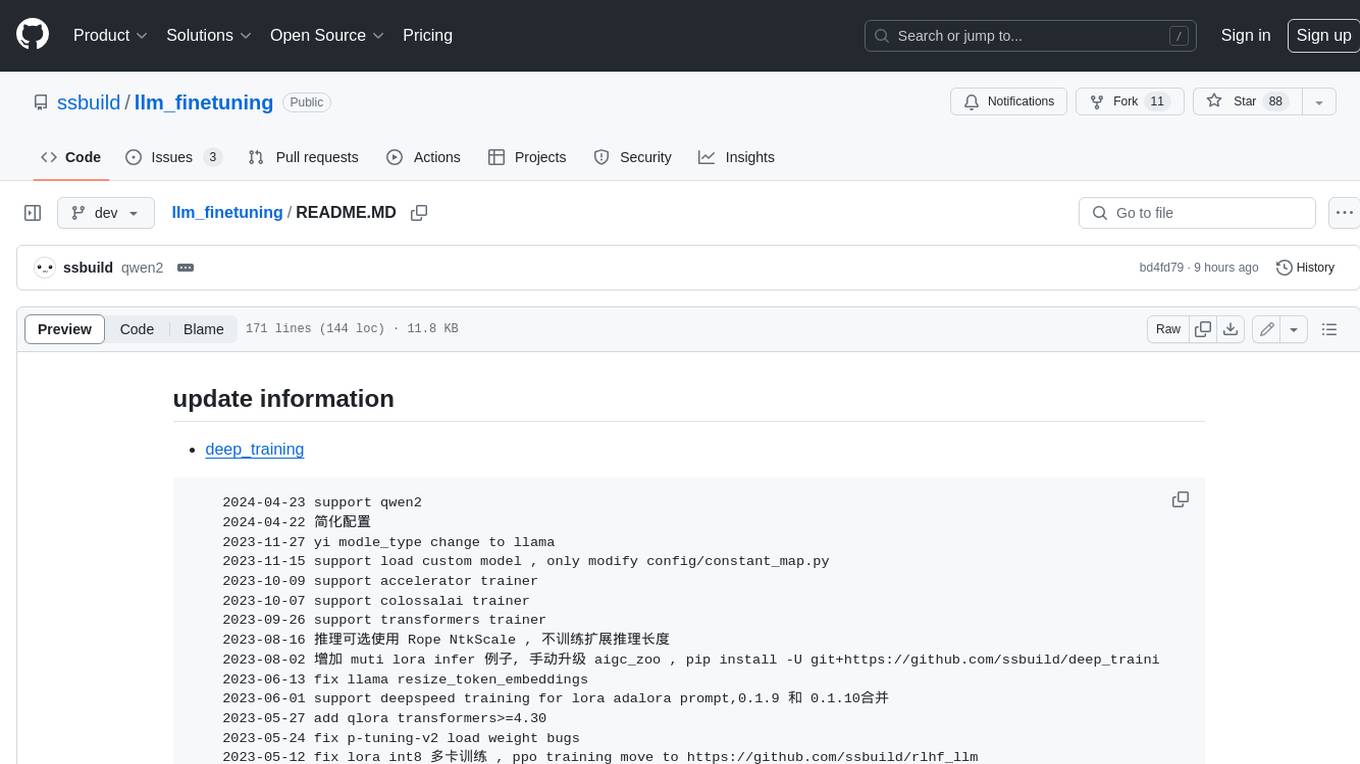
llm_finetuning
This repository provides a comprehensive set of tools for fine-tuning large language models (LLMs) using various techniques, including full parameter training, LoRA (Low-Rank Adaptation), and P-Tuning V2. It supports a wide range of LLM models, including Qwen, Yi, Llama, and others. The repository includes scripts for data preparation, training, and inference, making it easy for users to fine-tune LLMs for specific tasks. Additionally, it offers a collection of pre-trained models and provides detailed documentation and examples to guide users through the process.
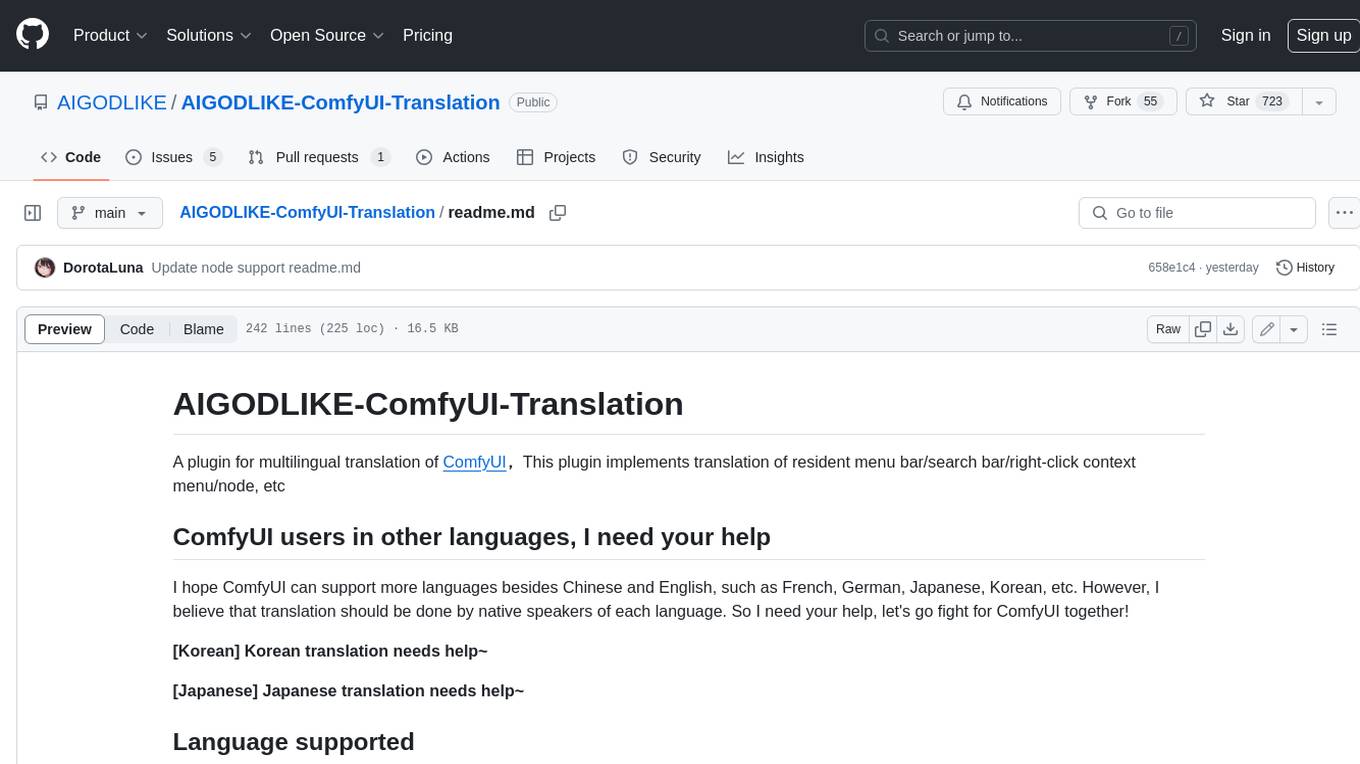
AIGODLIKE-ComfyUI-Translation
A plugin for multilingual translation of ComfyUI, This plugin implements translation of resident menu bar/search bar/right-click context menu/node, etc
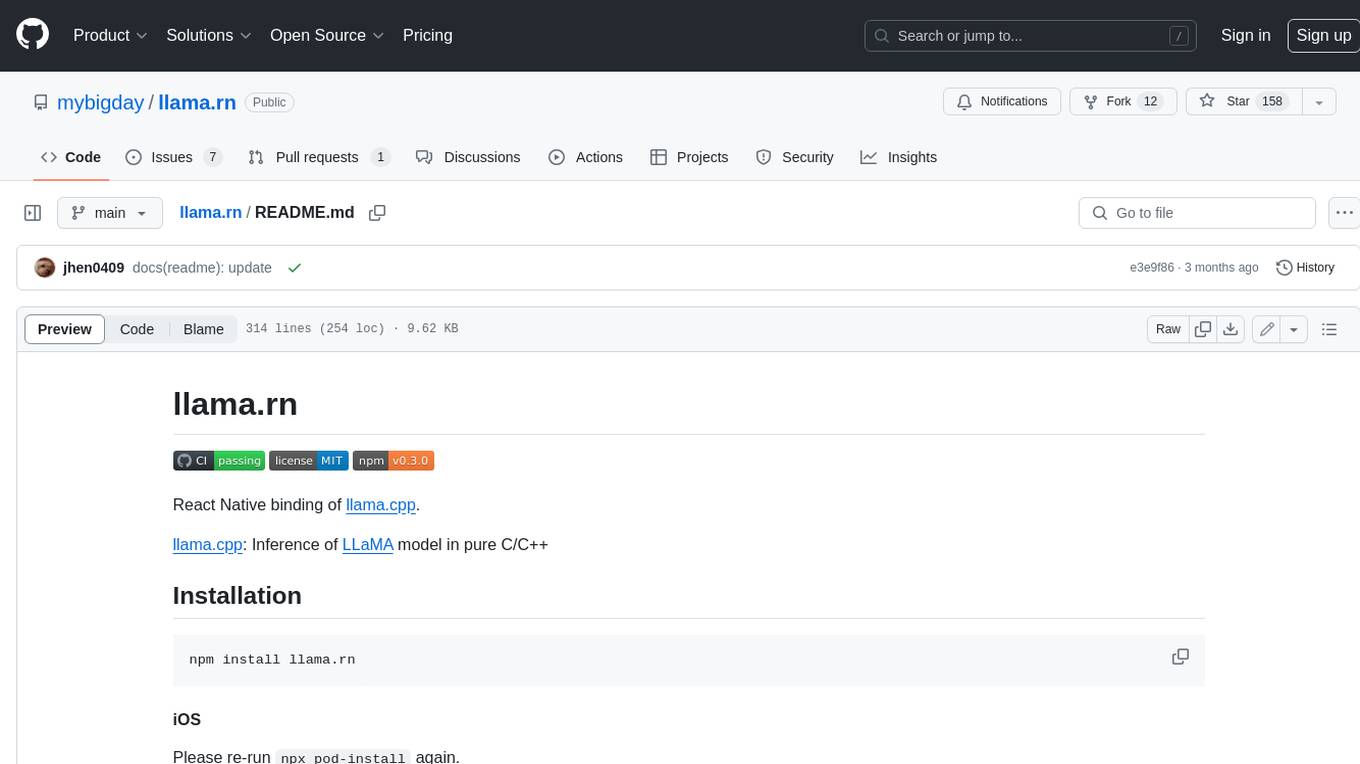
llama.rn
React Native binding of llama.cpp, which is an inference of LLaMA model in pure C/C++. This tool allows you to use the LLaMA model in your React Native applications for various tasks such as text completion, tokenization, detokenization, and embedding. It provides a convenient interface to interact with the LLaMA model and supports features like grammar sampling and mocking for testing purposes.
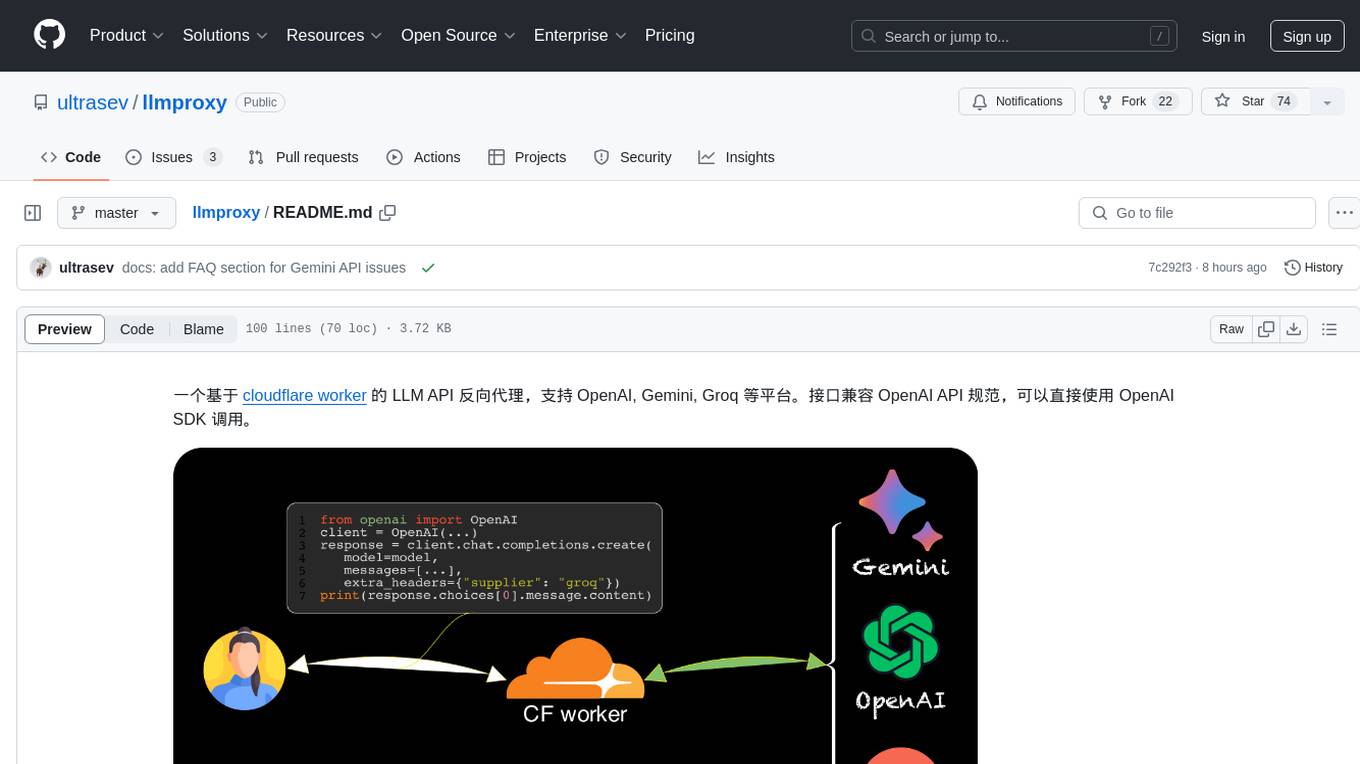
llmproxy
llmproxy is a reverse proxy for LLM API based on Cloudflare Worker, supporting platforms like OpenAI, Gemini, and Groq. The interface is compatible with the OpenAI API specification and can be directly accessed using the OpenAI SDK. It provides a convenient way to interact with various AI platforms through a unified API endpoint, enabling seamless integration and usage in different applications.
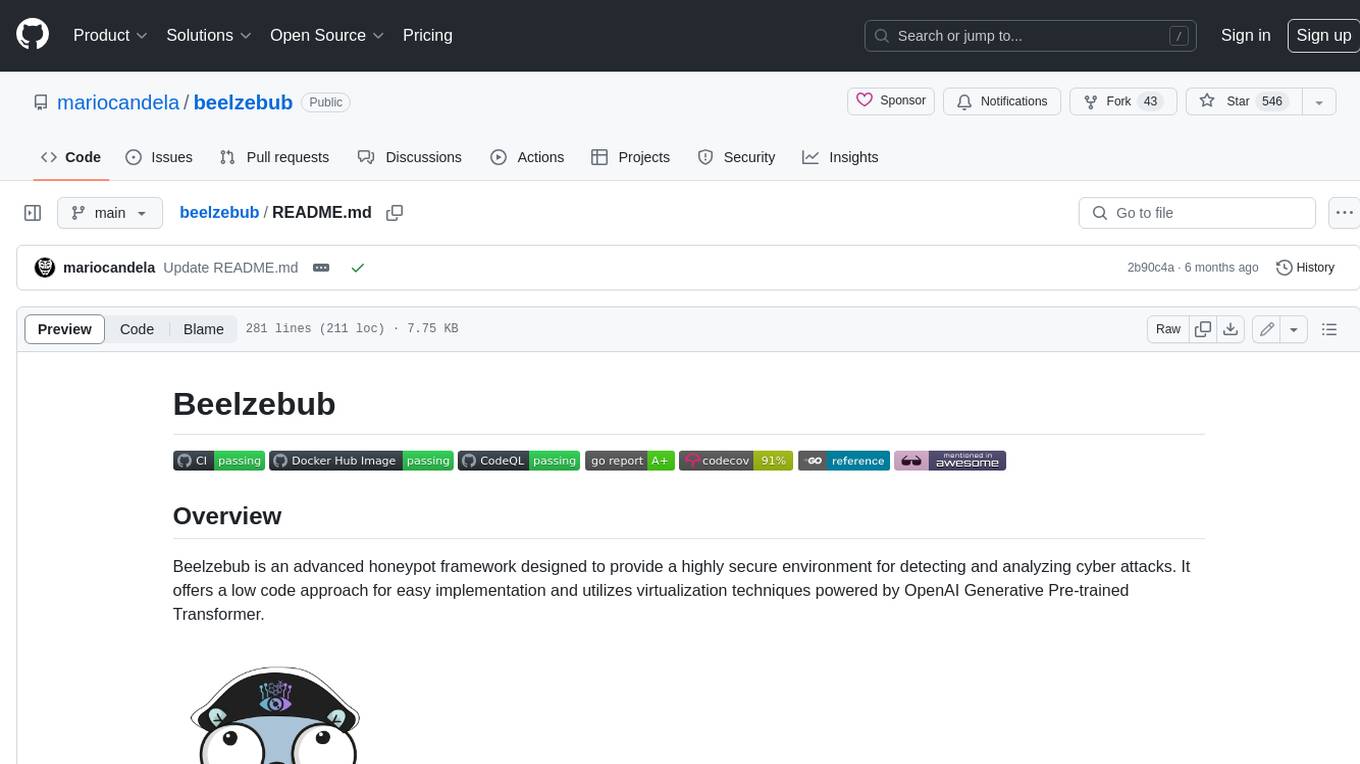
beelzebub
Beelzebub is an advanced honeypot framework designed to provide a highly secure environment for detecting and analyzing cyber attacks. It offers a low code approach for easy implementation and utilizes virtualization techniques powered by OpenAI Generative Pre-trained Transformer. Key features include OpenAI Generative Pre-trained Transformer acting as Linux virtualization, SSH Honeypot, HTTP Honeypot, TCP Honeypot, Prometheus openmetrics integration, Docker integration, RabbitMQ integration, and kubernetes support. Beelzebub allows easy configuration for different services and ports, enabling users to create custom honeypot scenarios. The roadmap includes developing Beelzebub into a robust PaaS platform. The project welcomes contributions and encourages adherence to the Code of Conduct for a supportive and respectful community.
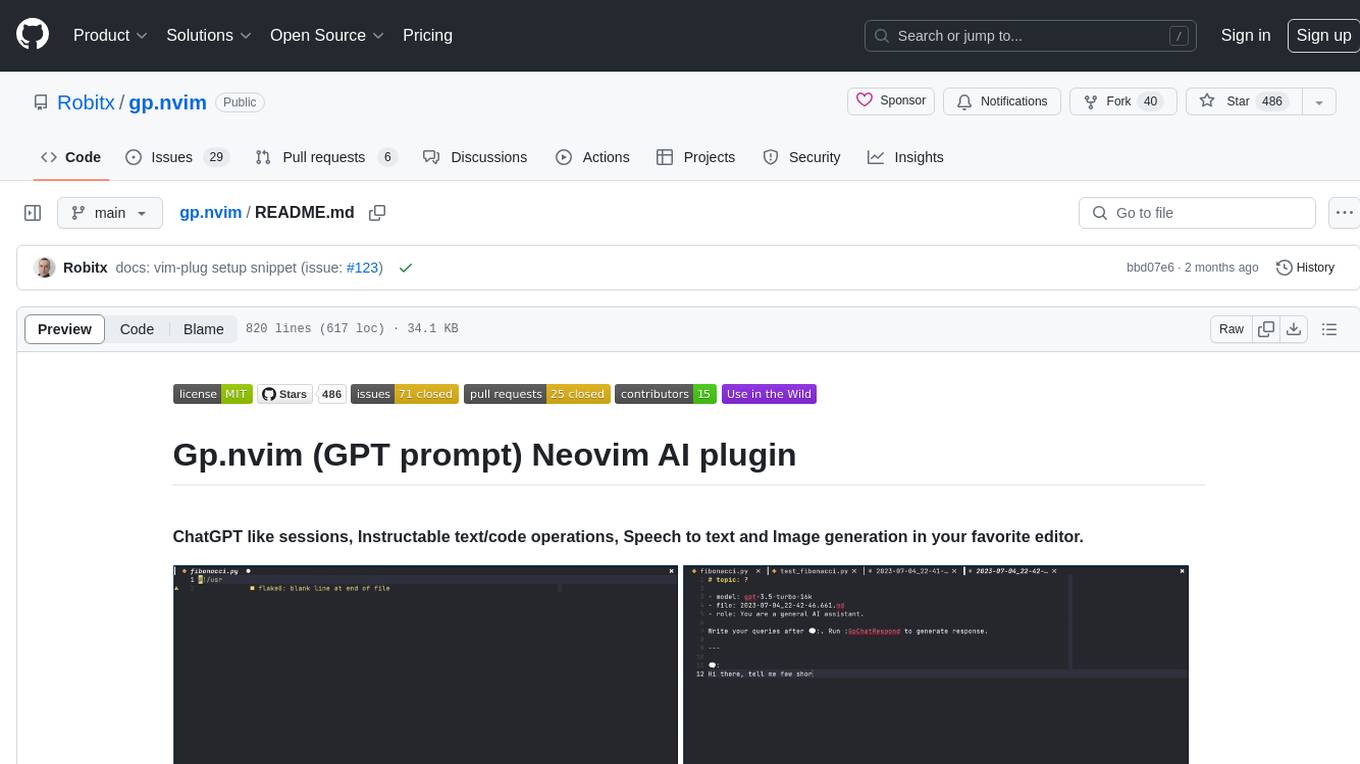
gp.nvim
Gp.nvim (GPT prompt) Neovim AI plugin provides a seamless integration of GPT models into Neovim, offering features like streaming responses, extensibility via hook functions, minimal dependencies, ChatGPT-like sessions, instructable text/code operations, speech-to-text support, and image generation directly within Neovim. The plugin aims to enhance the Neovim experience by leveraging the power of AI models in a user-friendly and native way.
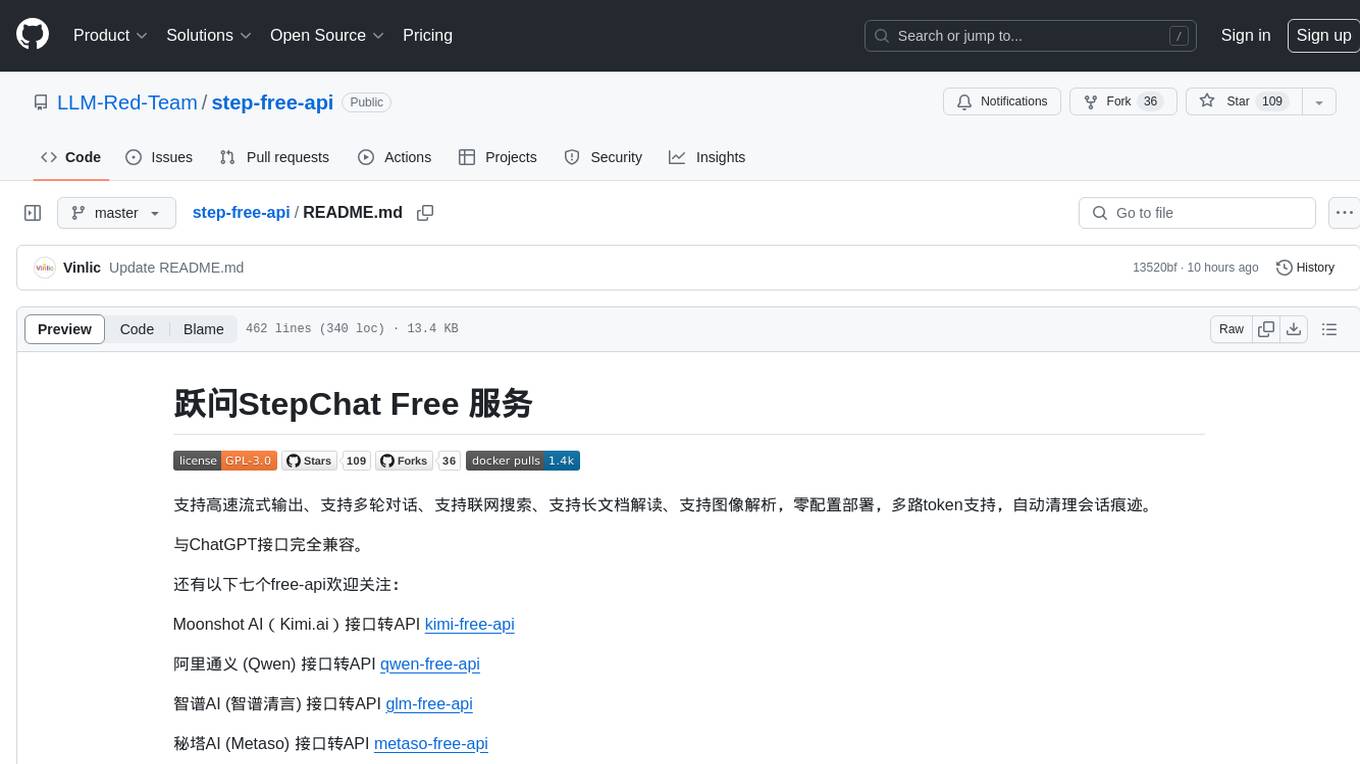
step-free-api
The StepChat Free service provides high-speed streaming output, multi-turn dialogue support, online search support, long document interpretation, and image parsing. It offers zero-configuration deployment, multi-token support, and automatic session trace cleaning. It is fully compatible with the ChatGPT interface. Additionally, it provides seven other free APIs for various services. The repository includes a disclaimer about using reverse APIs and encourages users to avoid commercial use to prevent service pressure on the official platform. It offers online testing links, showcases different demos, and provides deployment guides for Docker, Docker-compose, Render, Vercel, and native deployments. The repository also includes information on using multiple accounts, optimizing Nginx reverse proxy, and checking the liveliness of refresh tokens.
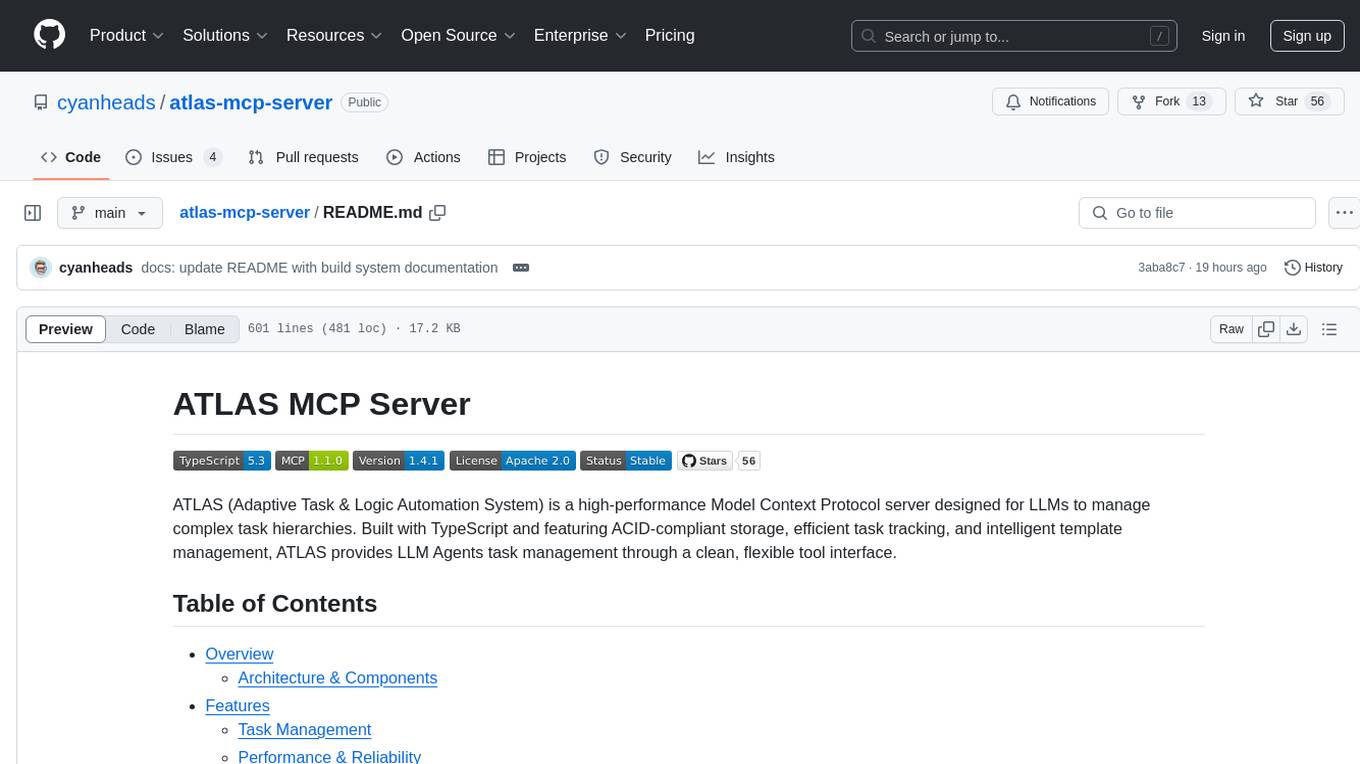
atlas-mcp-server
ATLAS (Adaptive Task & Logic Automation System) is a high-performance Model Context Protocol server designed for LLMs to manage complex task hierarchies. Built with TypeScript, it features ACID-compliant storage, efficient task tracking, and intelligent template management. ATLAS provides LLM Agents task management through a clean, flexible tool interface. The server implements the Model Context Protocol (MCP) for standardized communication between LLMs and external systems, offering hierarchical task organization, task state management, smart templates, enterprise features, and performance optimization.
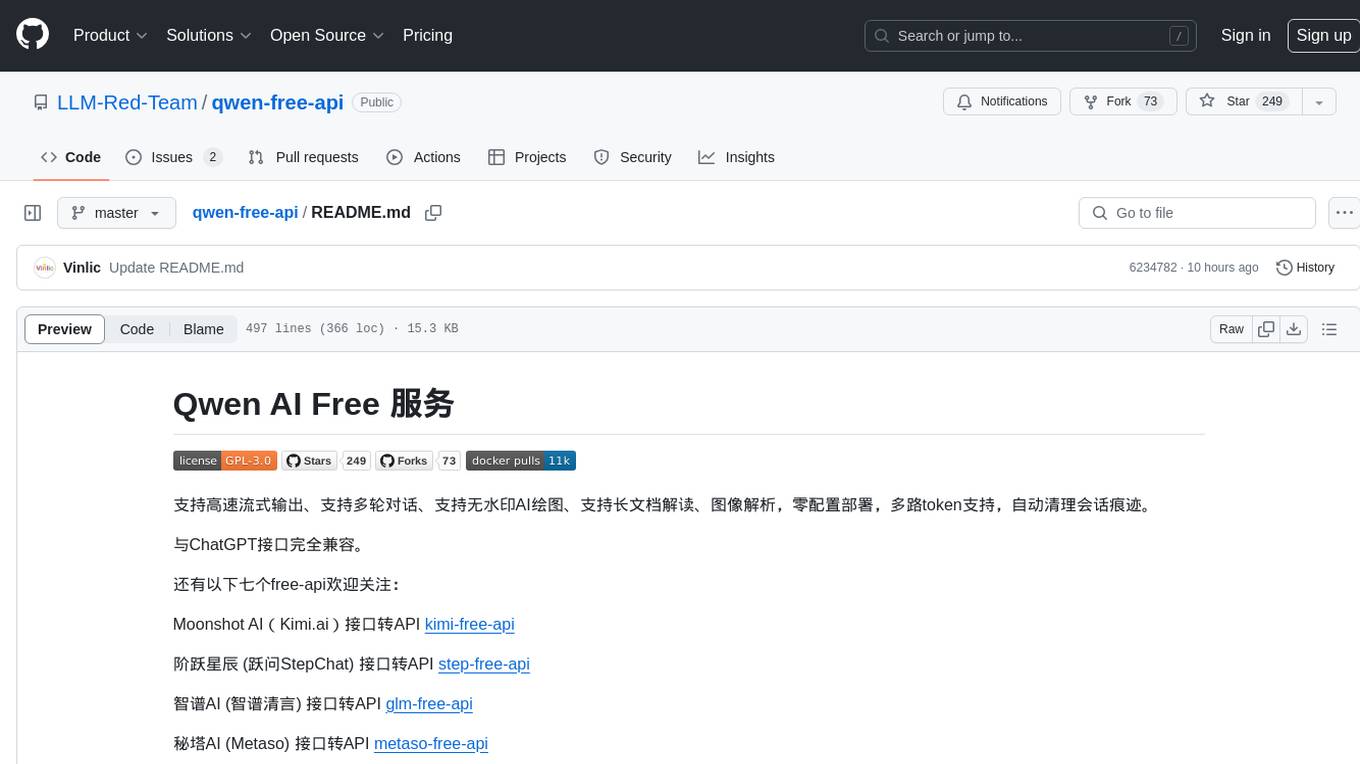
qwen-free-api
Qwen AI Free service supports high-speed streaming output, multi-turn dialogue, watermark-free AI drawing, long document interpretation, image parsing, zero-configuration deployment, multi-token support, automatic session trace cleaning. It is fully compatible with the ChatGPT interface. The repository provides various free APIs for different AI services. Users can access the service through different deployment methods like Docker, Docker-compose, Render, Vercel, and native deployment. It offers interfaces for chat completions, AI drawing, document interpretation, image parsing, and token checking. Users need to provide 'login_tongyi_ticket' for authorization. The project emphasizes research, learning, and personal use only, discouraging commercial use to avoid service pressure on the official platform.
For similar tasks
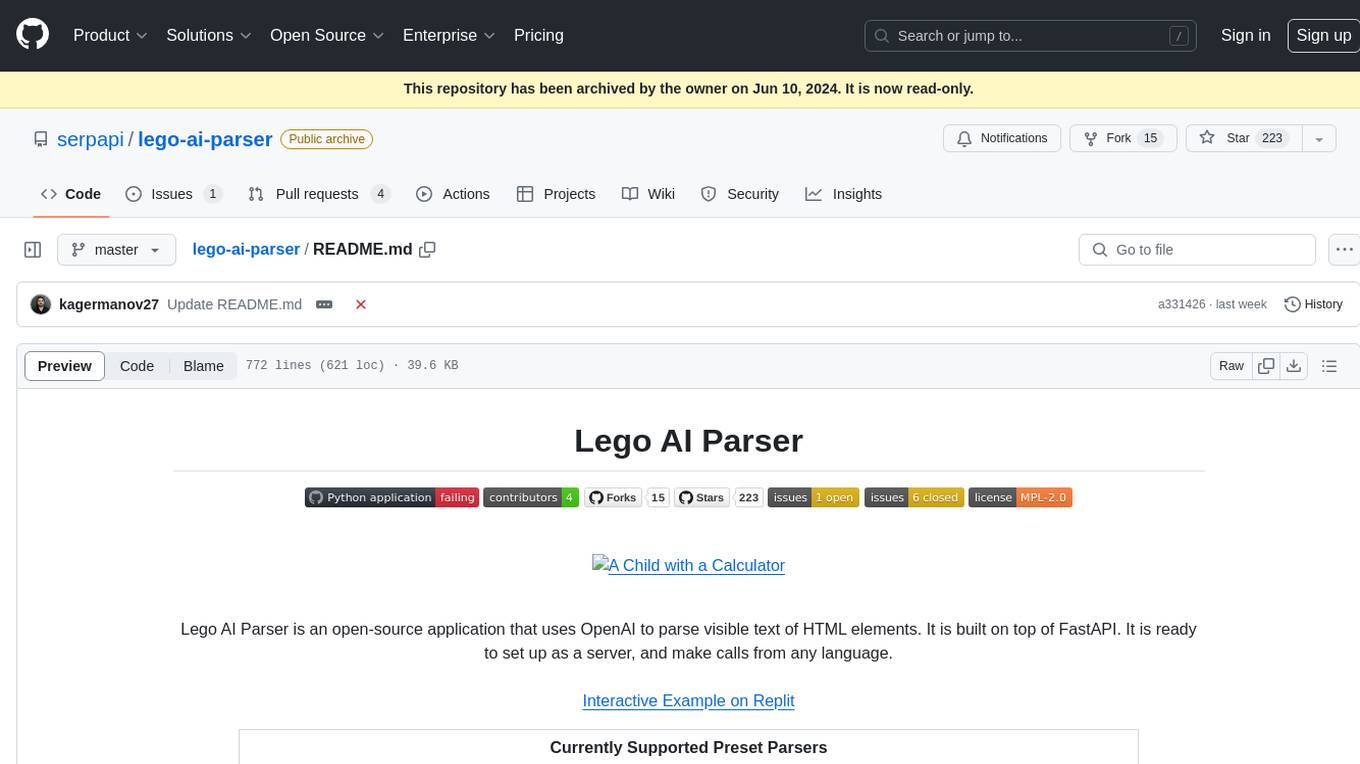
lego-ai-parser
Lego AI Parser is an open-source application that uses OpenAI to parse visible text of HTML elements. It is built on top of FastAPI, ready to set up as a server, and make calls from any language. It supports preset parsers for Google Local Results, Amazon Listings, Etsy Listings, Wayfair Listings, BestBuy Listings, Costco Listings, Macy's Listings, and Nordstrom Listings. Users can also design custom parsers by providing prompts, examples, and details about the OpenAI model under the classifier key.
For similar jobs
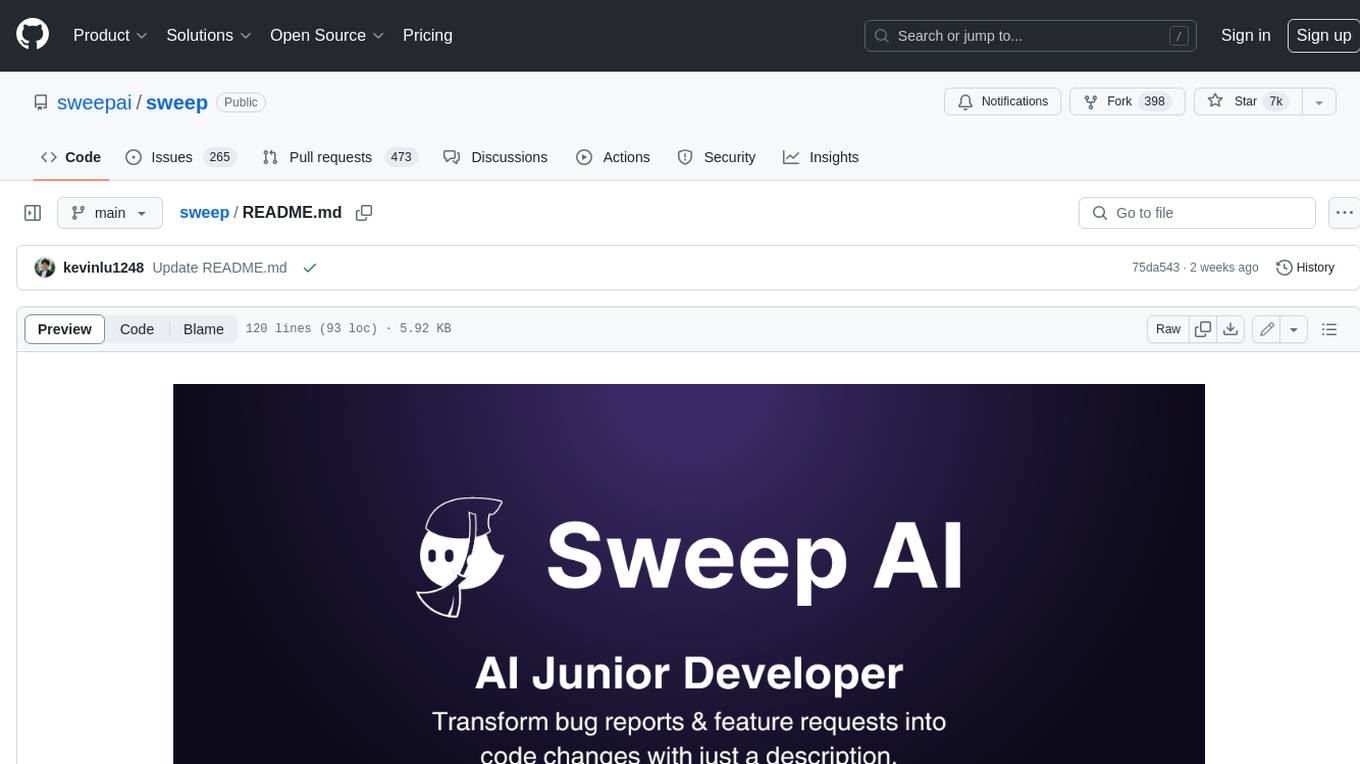
sweep
Sweep is an AI junior developer that turns bugs and feature requests into code changes. It automatically handles developer experience improvements like adding type hints and improving test coverage.
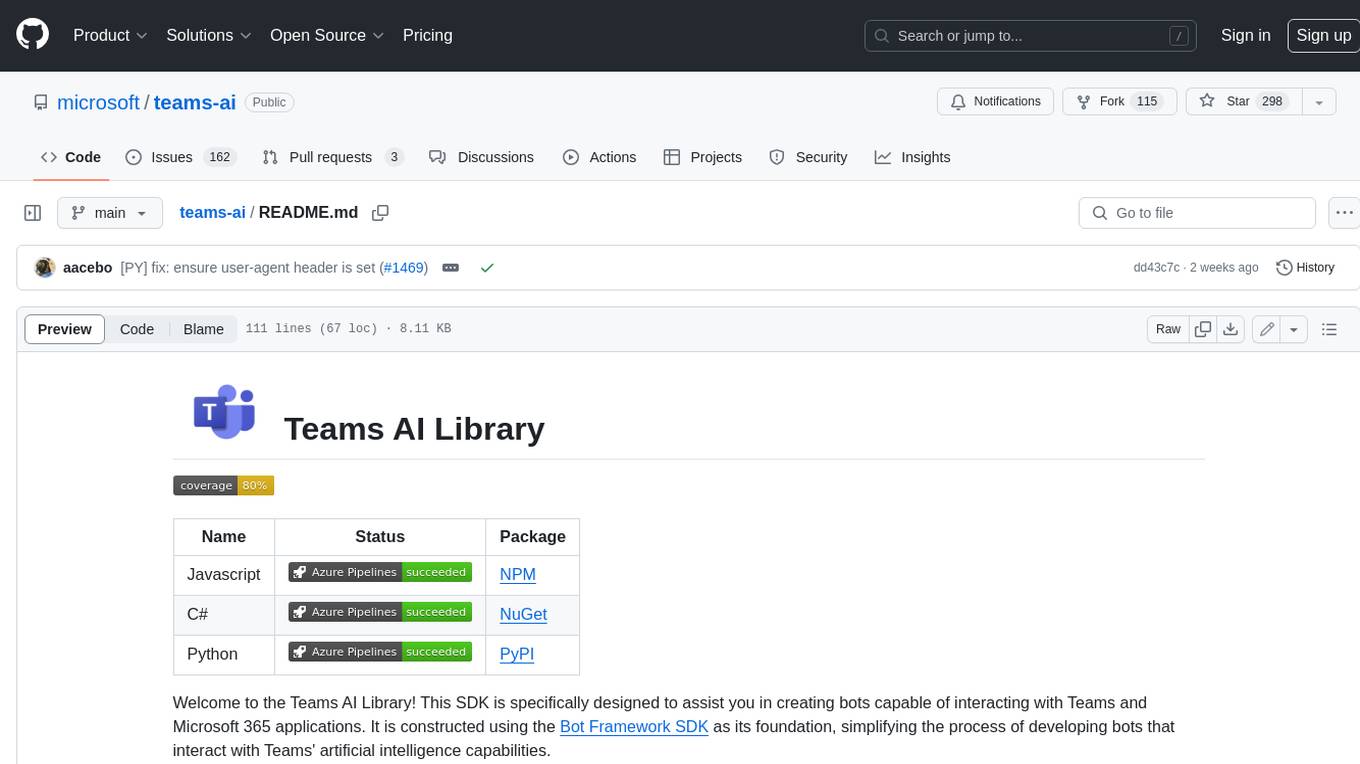
teams-ai
The Teams AI Library is a software development kit (SDK) that helps developers create bots that can interact with Teams and Microsoft 365 applications. It is built on top of the Bot Framework SDK and simplifies the process of developing bots that interact with Teams' artificial intelligence capabilities. The SDK is available for JavaScript/TypeScript, .NET, and Python.
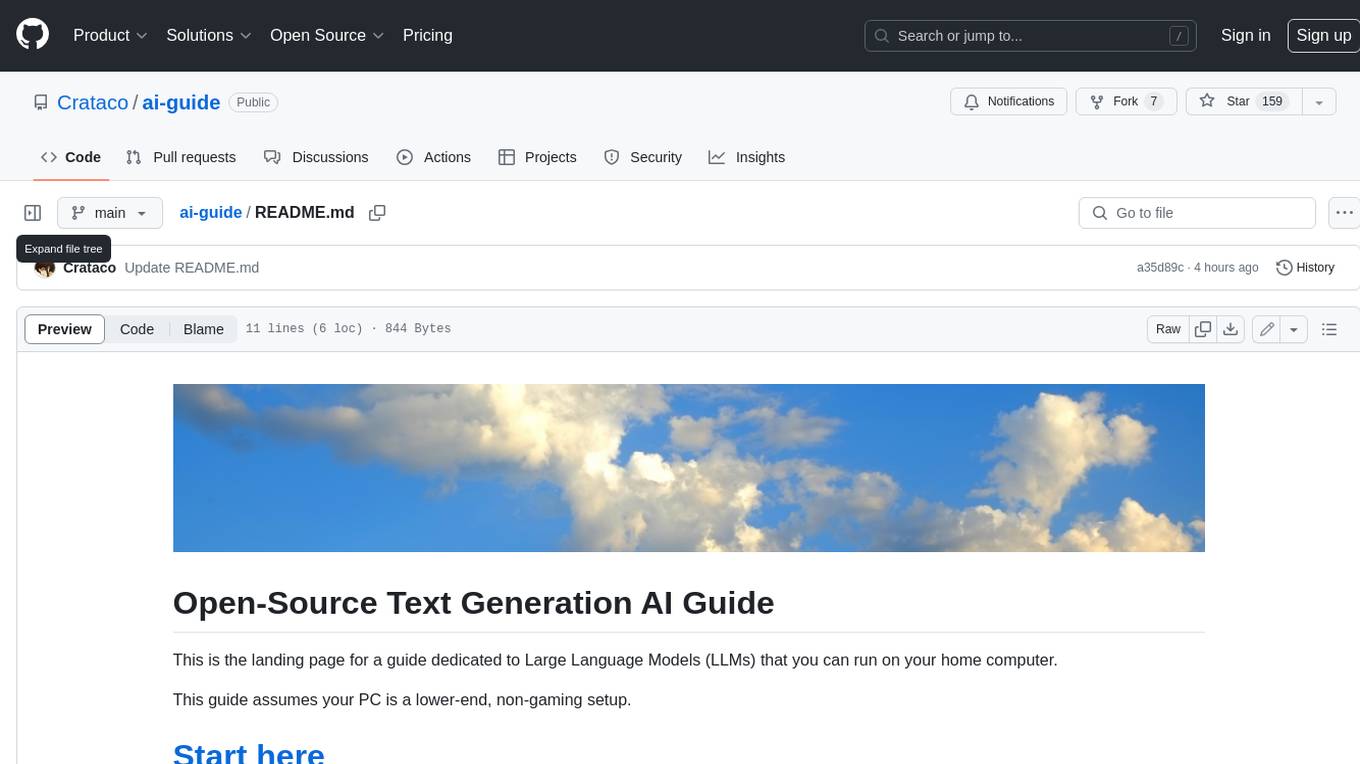
ai-guide
This guide is dedicated to Large Language Models (LLMs) that you can run on your home computer. It assumes your PC is a lower-end, non-gaming setup.
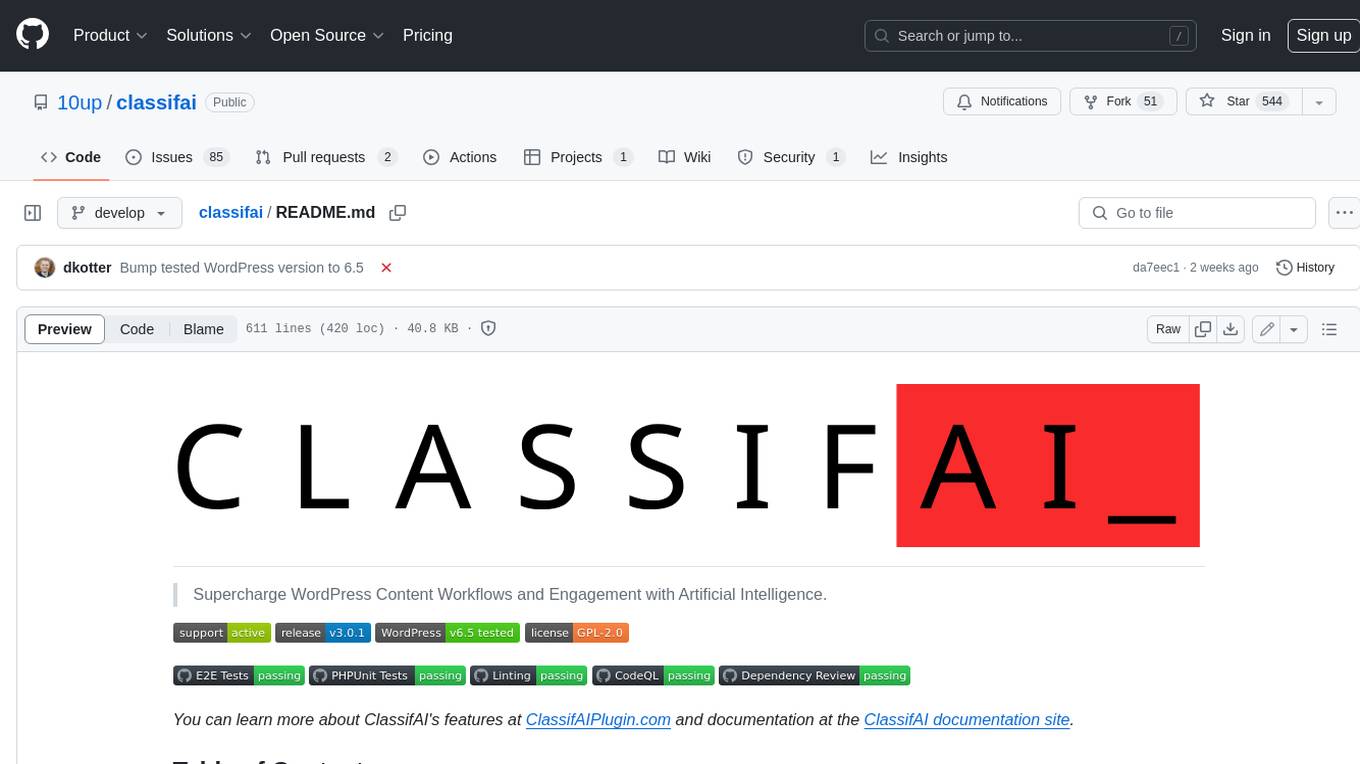
classifai
Supercharge WordPress Content Workflows and Engagement with Artificial Intelligence. Tap into leading cloud-based services like OpenAI, Microsoft Azure AI, Google Gemini and IBM Watson to augment your WordPress-powered websites. Publish content faster while improving SEO performance and increasing audience engagement. ClassifAI integrates Artificial Intelligence and Machine Learning technologies to lighten your workload and eliminate tedious tasks, giving you more time to create original content that matters.
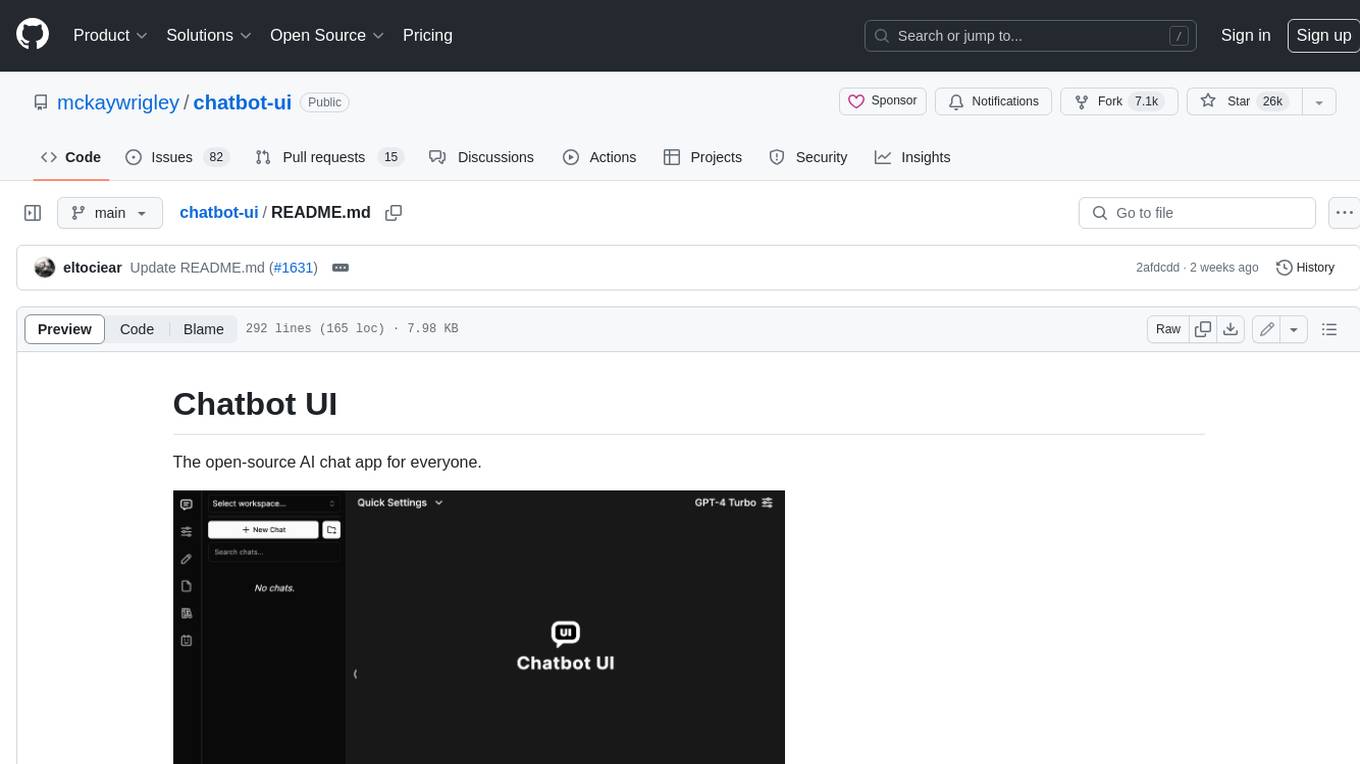
chatbot-ui
Chatbot UI is an open-source AI chat app that allows users to create and deploy their own AI chatbots. It is easy to use and can be customized to fit any need. Chatbot UI is perfect for businesses, developers, and anyone who wants to create a chatbot.
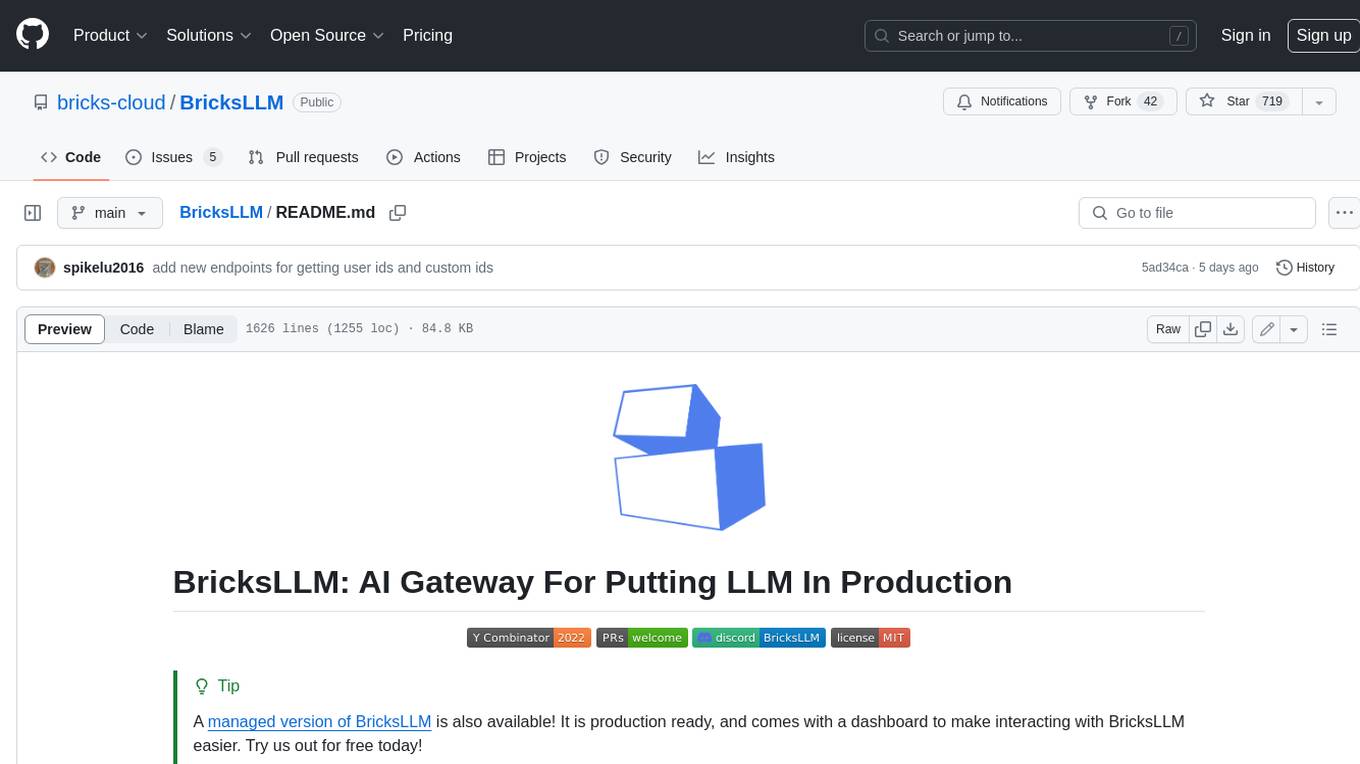
BricksLLM
BricksLLM is a cloud native AI gateway written in Go. Currently, it provides native support for OpenAI, Anthropic, Azure OpenAI and vLLM. BricksLLM aims to provide enterprise level infrastructure that can power any LLM production use cases. Here are some use cases for BricksLLM: * Set LLM usage limits for users on different pricing tiers * Track LLM usage on a per user and per organization basis * Block or redact requests containing PIIs * Improve LLM reliability with failovers, retries and caching * Distribute API keys with rate limits and cost limits for internal development/production use cases * Distribute API keys with rate limits and cost limits for students
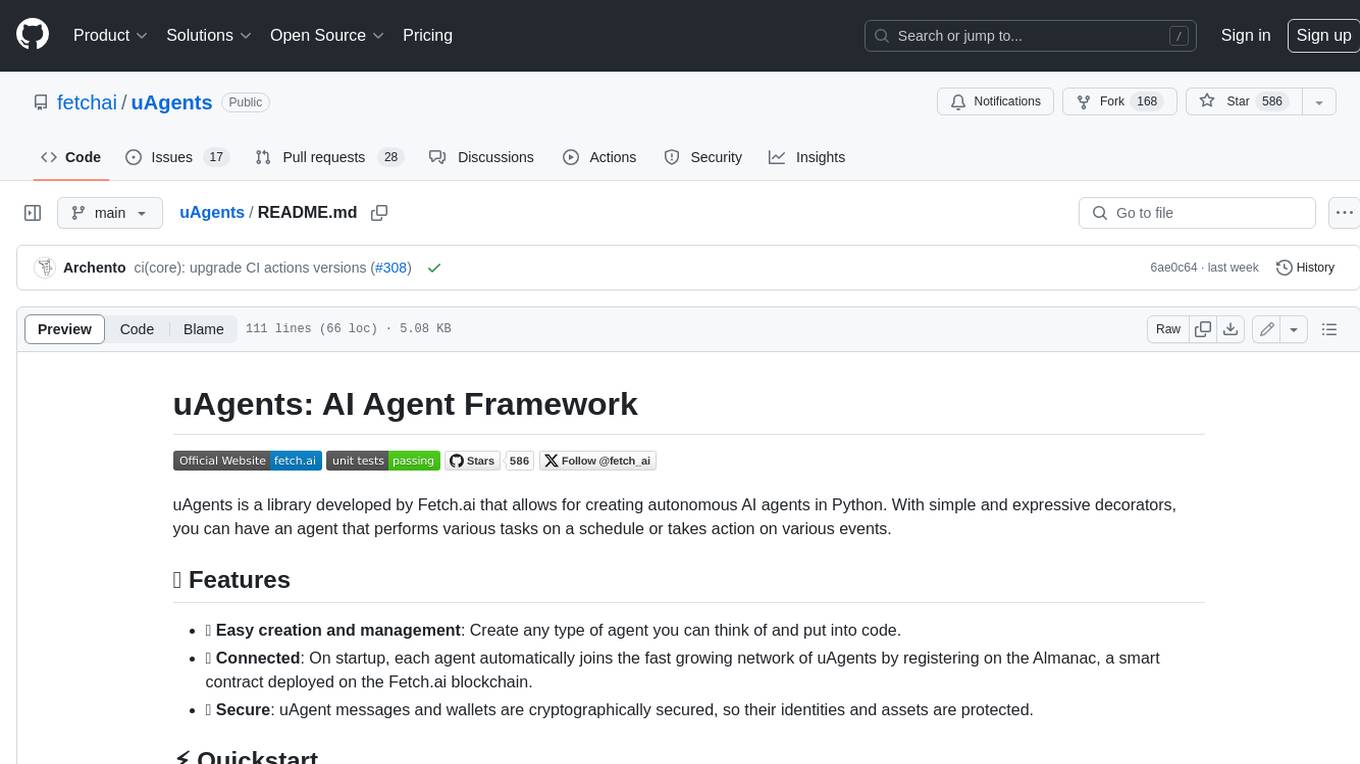
uAgents
uAgents is a Python library developed by Fetch.ai that allows for the creation of autonomous AI agents. These agents can perform various tasks on a schedule or take action on various events. uAgents are easy to create and manage, and they are connected to a fast-growing network of other uAgents. They are also secure, with cryptographically secured messages and wallets.
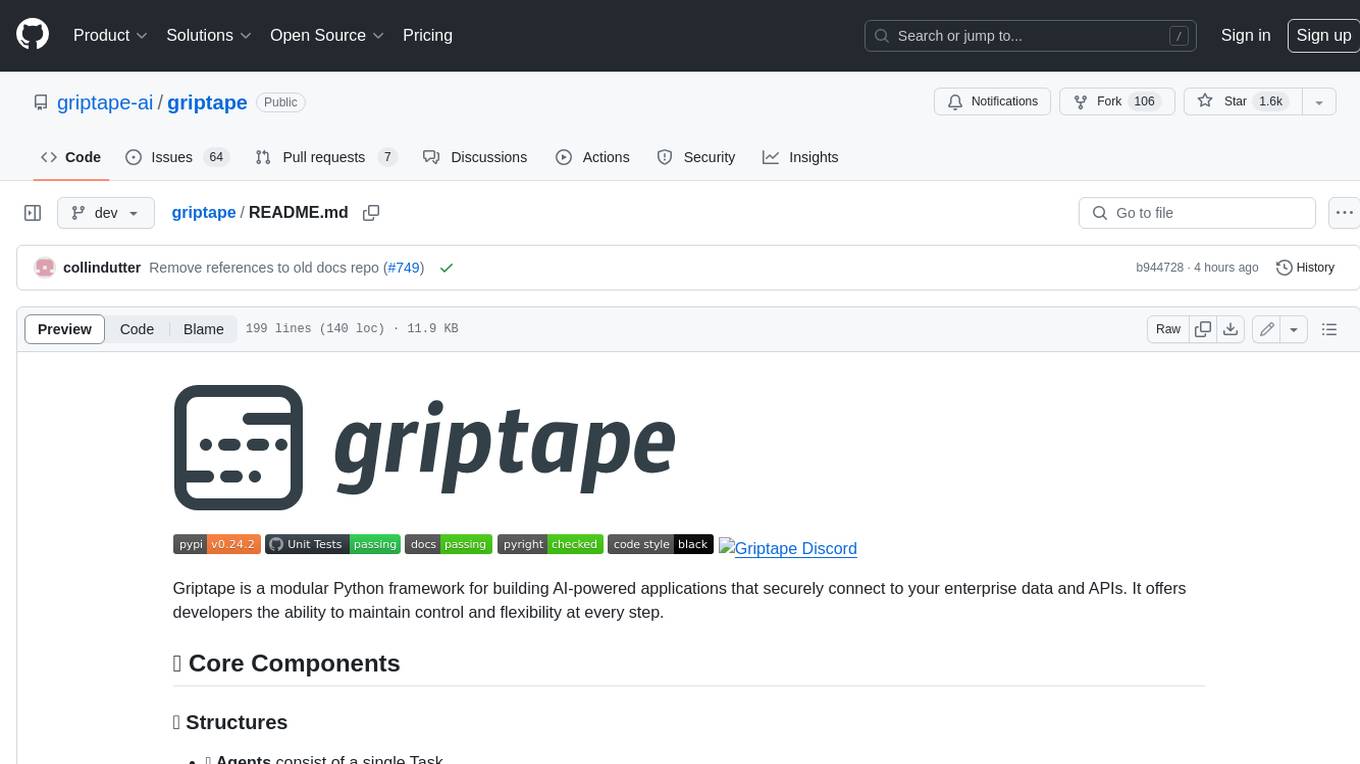
griptape
Griptape is a modular Python framework for building AI-powered applications that securely connect to your enterprise data and APIs. It offers developers the ability to maintain control and flexibility at every step. Griptape's core components include Structures (Agents, Pipelines, and Workflows), Tasks, Tools, Memory (Conversation Memory, Task Memory, and Meta Memory), Drivers (Prompt and Embedding Drivers, Vector Store Drivers, Image Generation Drivers, Image Query Drivers, SQL Drivers, Web Scraper Drivers, and Conversation Memory Drivers), Engines (Query Engines, Extraction Engines, Summary Engines, Image Generation Engines, and Image Query Engines), and additional components (Rulesets, Loaders, Artifacts, Chunkers, and Tokenizers). Griptape enables developers to create AI-powered applications with ease and efficiency.