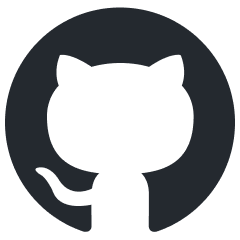
TokenPacker
The code for "TokenPacker: Efficient Visual Projector for Multimodal LLM".
Stars: 235
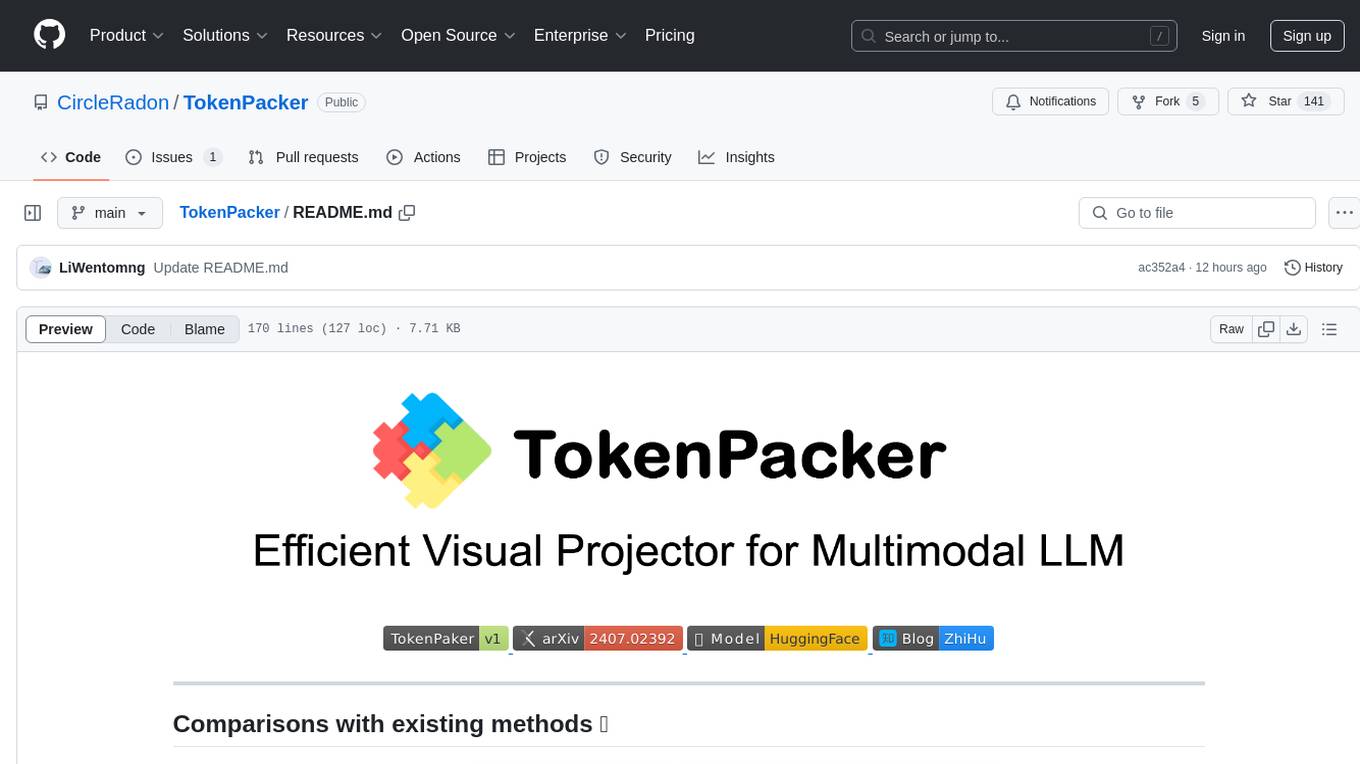
TokenPacker is a novel visual projector that compresses visual tokens by 75%βΌ89% with high efficiency. It adopts a 'coarse-to-fine' scheme to generate condensed visual tokens, achieving comparable or better performance across diverse benchmarks. The tool includes TokenPacker for general use and TokenPacker-HD for high-resolution image understanding. It provides training scripts, checkpoints, and supports various compression ratios and patch numbers.
README:
- [2024/10/22] We integrated TokenPacker-HD framework with Osprey to achieve fine-grained high-resolution pixel-level understanding with large performance gains. Please see the codes in this branch for your reference.
- [2024/7/25] We released checkpoints, please check them.
- [2024/7/3] We released the paper of our TokenPacker on Arxiv.
- [2024/7/3] We released the training and inference codes.
TokenPacker is a novel visual projector, which adopts a coarse-to-fine
scheme
to inject the enriched characteristics to generate the condensed visual tokens. Using TokenPacker, we can compress the
visual tokens by 75%βΌ89%, while achieves comparable or even better performance
across diverse benchmarks with significantly higher efficiency.
We provide the pseudo-codes to showcase the detailed processing flow.
As a visual projector, TokenPacker is implemented by a class TokenPacker
, which can be found in multimodal_projector/builder.py
To support efficient high-resolution
image understanding, we further develop an effective image
cropping method TokenPacker-HD
.
- Clone this repository and navigate to TokenPacker folder
git clone https://github.com/CircleRadon/TokenPacker.git
cd TokenPacker
- Install packages
conda create -n tokenpacker python=3.10 -y
conda activate tokenpacker
pip install --upgrade pip # enable PEP 660 support
pip install -e .
- Install additional packages for training cases
pip install -e ".[train]"
pip install flash-attn --no-build-isolation
To make a fair comparison, we use the same training data as in LLaVA-1.5, i.e., LLaVA-Pretrain-558K for stage 1, and Mix665k for stage 2.
- Stage1: Image-Text Alignment Pre-training
bash scripts/v1_5/pretrain.sh
- Stage2: Visual Instruction Tuning
bash scripts/v1_5/finetune.sh
Note: Using --scale_factor
to control compression ratio, support [2,3,4]
To obtain the competitive high-resolution performance, we use 2.7M data as organized by Mini-Gemini, i.e., 1.2M for stage 1 and 1.5M for stage 2.
- Stage1: Image-Text Alignment Pre-training
bash scripts/v1_5/pretrain_hd.sh
- Stage2: Visual Instruction Tuning
bash scripts/v1_5/finetune_hd.sh
Note:
- Using
--scale_factor
to control compression ratio, support [2,3,4]. - Using
--patch_num
to control max patch dividing number, support [9,16,25].
Model | Max Res. | Compre. Ratio | Token Num. | Max Patch Num. | Training Data | Download |
---|---|---|---|---|---|---|
TokenPacker-7b | 336x336 | 1/4 | 144 | - | 558K+665K | checkpoints |
TokenPacker-13b | 336x336 | 1/4 | 144 | - | 558K+665K | checkpoints |
TokenPacker-HD-7b | 1088x1088 | 1/4 | ~954 | 9 | 1.2M+1.5M | checkpoints |
TokenPacker-HD-13b | 1088x1088 | 1/4 | ~954 | 9 | 1.2M+1.5M | checkpoints |
TokenPacker-HD-13b | 1344x1344 | 1/4 | ~1393 | 16 | 1.2M+1.5M | checkpoints |
TokenPacker-HD-13b | 1344x1344 | 1/9 | ~619 | 16 | 1.2M+1.5M | checkpoints |
TokenPacker-HD-13b | 1344x1344 | 1/16 | ~347 | 16 | 1.2M+1.5M | checkpoints |
Note:
- The
token number
of TokenPacker-HD is theaverage
statistically across all training and test data. - The training data of
558K+665K
follows LLaVA-1.5, the one of1.2M+1.5M
follows Mini-Gemini. - All LLMs use Vicuna-7b/13b as based LLM.
We provide some visual examples.
High-resolution image understanding.
- [x] Release the training and inference codes.
- [x] Release all checkpoints.
- LLaVA-v1.5: the codebase we built upon.
- Mini-Gemini: the organized data we used for training high-resolution method.
For more recent related works, please refer to this repo of Awesome-Token-Compress.
@misc{TokenPacker,
title={TokenPacker: Efficient Visual Projector for Multimodal LLM},
author={Wentong Li, Yuqian Yuan, Jian Liu, Dongqi Tang, Song Wang, Jianke Zhu and Lei Zhang},
year={2024},
eprint={2407.02392},
archivePrefix={arXiv},
primaryClass={cs.CV}
}
For Tasks:
Click tags to check more tools for each tasksFor Jobs:
Alternative AI tools for TokenPacker
Similar Open Source Tools
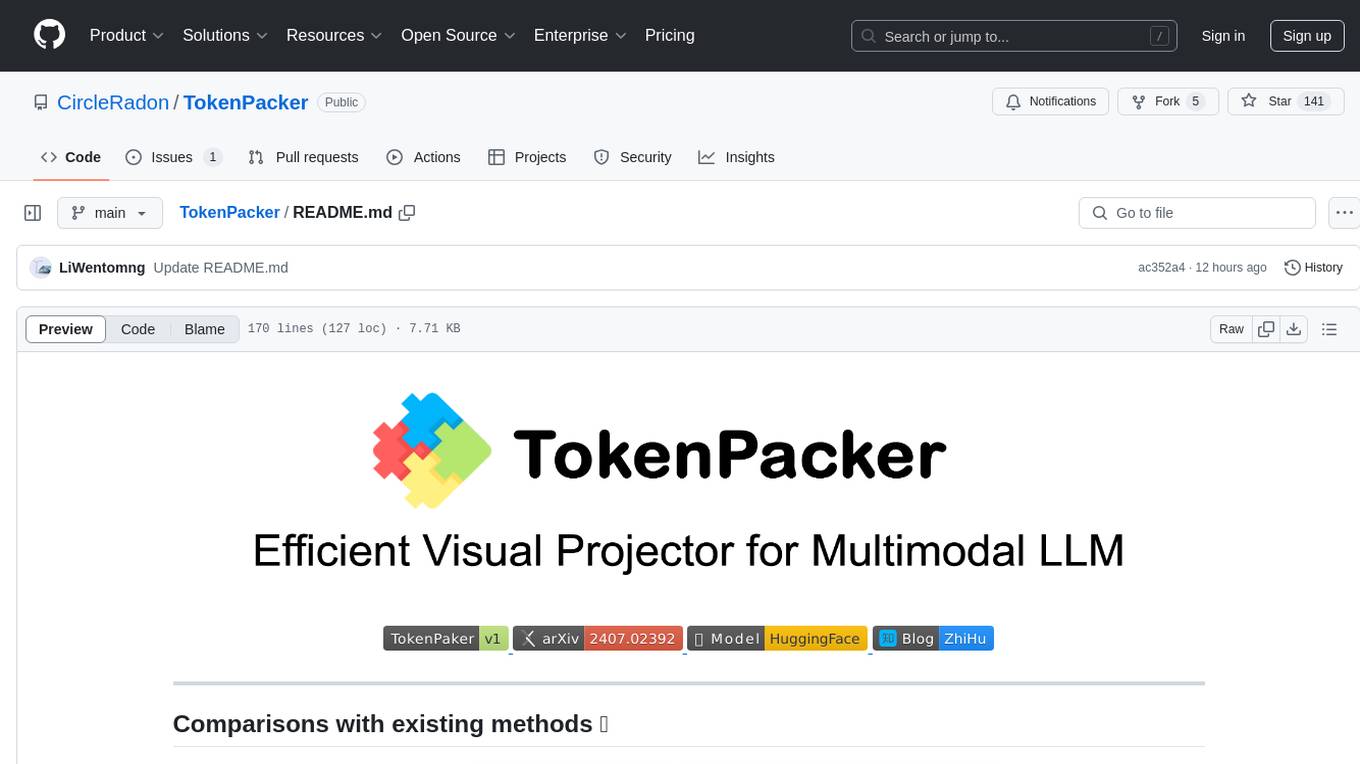
TokenPacker
TokenPacker is a novel visual projector that compresses visual tokens by 75%βΌ89% with high efficiency. It adopts a 'coarse-to-fine' scheme to generate condensed visual tokens, achieving comparable or better performance across diverse benchmarks. The tool includes TokenPacker for general use and TokenPacker-HD for high-resolution image understanding. It provides training scripts, checkpoints, and supports various compression ratios and patch numbers.
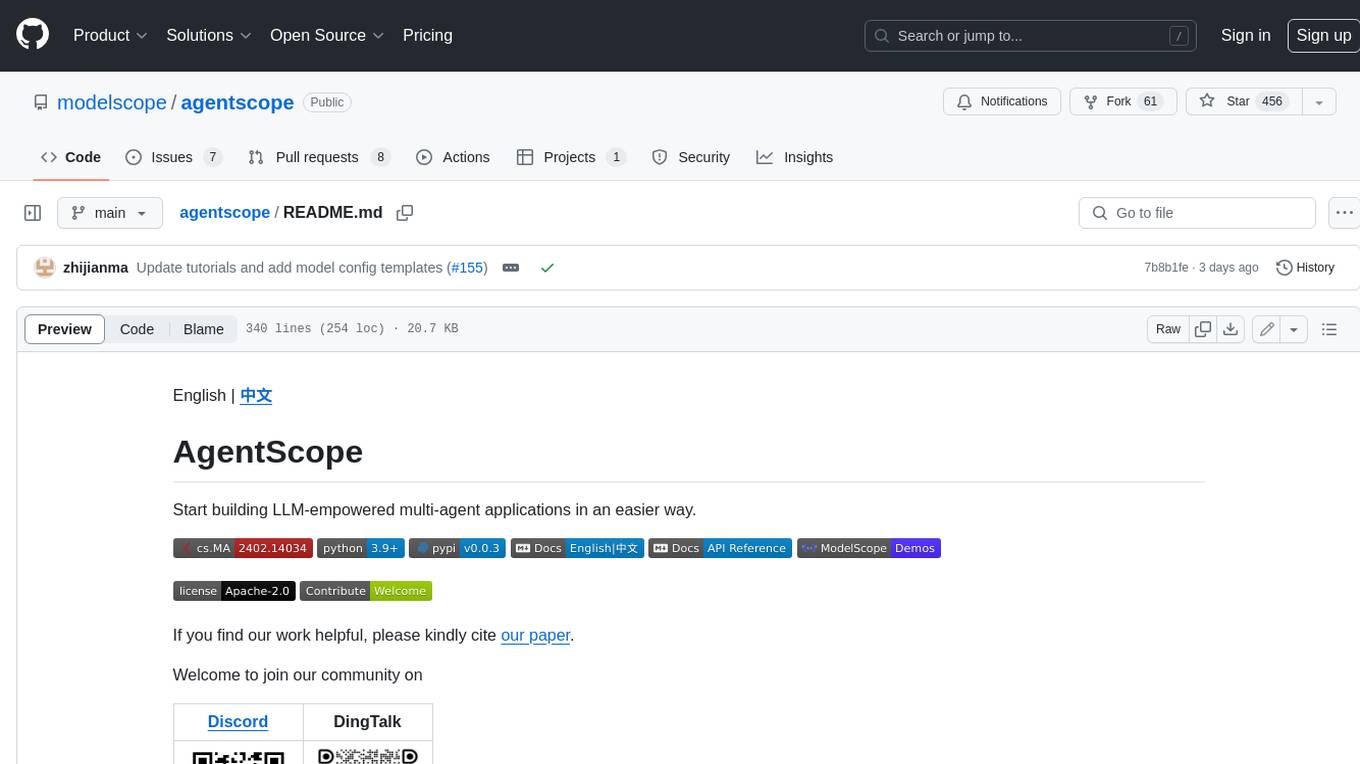
agentscope
AgentScope is a multi-agent platform designed to empower developers to build multi-agent applications with large-scale models. It features three high-level capabilities: Easy-to-Use, High Robustness, and Actor-Based Distribution. AgentScope provides a list of `ModelWrapper` to support both local model services and third-party model APIs, including OpenAI API, DashScope API, Gemini API, and ollama. It also enables developers to rapidly deploy local model services using libraries such as ollama (CPU inference), Flask + Transformers, Flask + ModelScope, FastChat, and vllm. AgentScope supports various services, including Web Search, Data Query, Retrieval, Code Execution, File Operation, and Text Processing. Example applications include Conversation, Game, and Distribution. AgentScope is released under Apache License 2.0 and welcomes contributions.
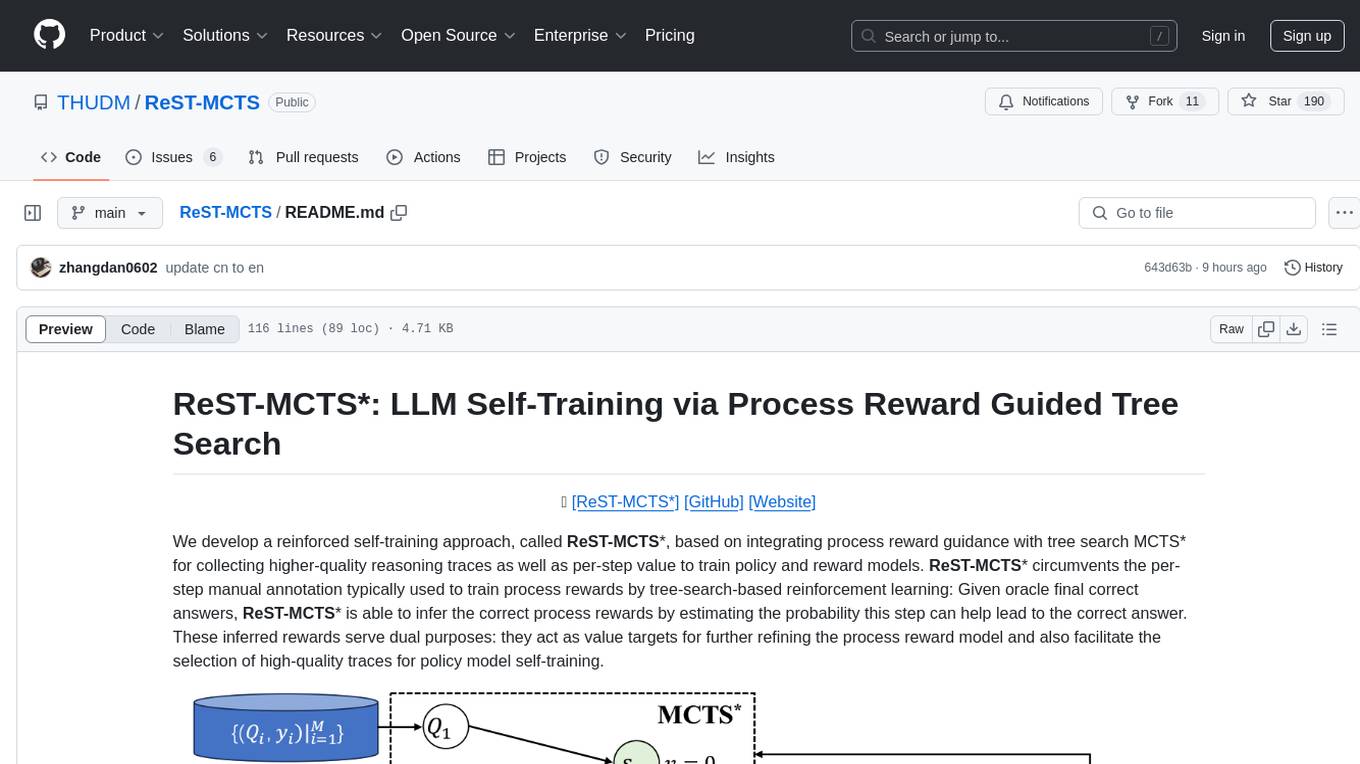
ReST-MCTS
ReST-MCTS is a reinforced self-training approach that integrates process reward guidance with tree search MCTS to collect higher-quality reasoning traces and per-step value for training policy and reward models. It eliminates the need for manual per-step annotation by estimating the probability of steps leading to correct answers. The inferred rewards refine the process reward model and aid in selecting high-quality traces for policy model self-training.
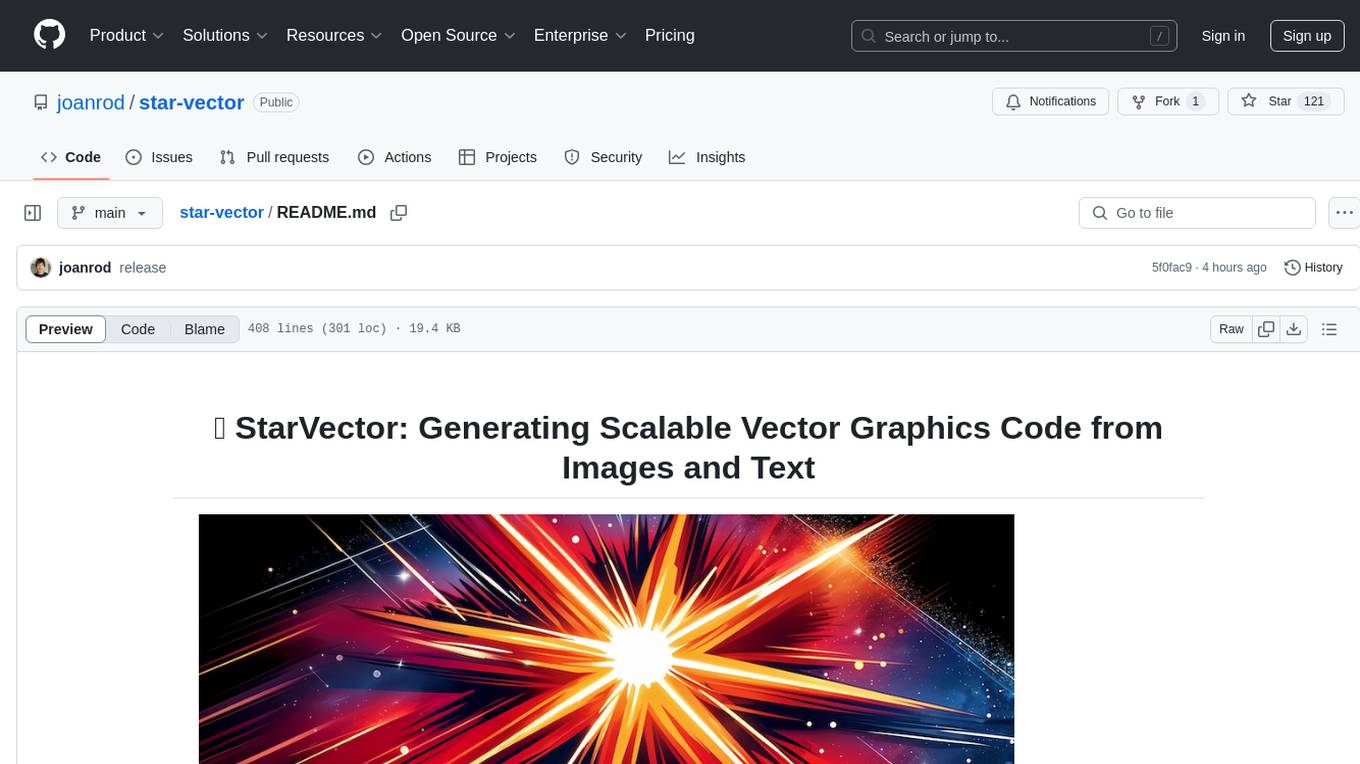
star-vector
StarVector is a multimodal vision-language model for Scalable Vector Graphics (SVG) generation. It can be used to perform image2SVG and text2SVG generation. StarVector works directly in the SVG code space, leveraging visual understanding to apply accurate SVG primitives. It achieves state-of-the-art performance in producing compact and semantically rich SVGs. The tool provides Hugging Face model checkpoints for image2SVG vectorization, with models like StarVector-8B and StarVector-1B. It also offers datasets like SVG-Stack, SVG-Fonts, SVG-Icons, SVG-Emoji, and SVG-Diagrams for evaluation. StarVector can be trained using Deepspeed or FSDP for tasks like Image2SVG and Text2SVG generation. The tool provides a demo with options for HuggingFace generation or VLLM backend for faster generation speed.
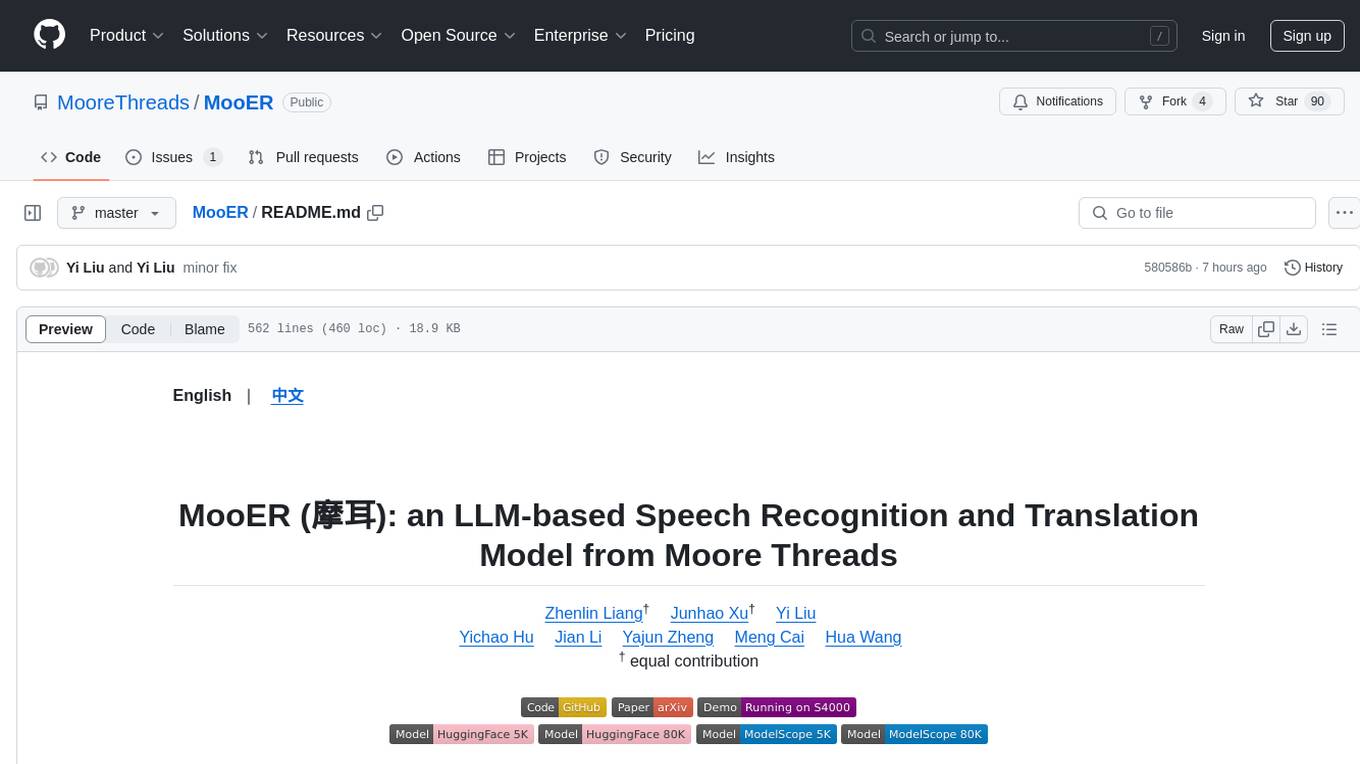
MooER
MooER (ζ©θ³) is an LLM-based speech recognition and translation model developed by Moore Threads. It allows users to transcribe speech into text (ASR) and translate speech into other languages (AST) in an end-to-end manner. The model was trained using 5K hours of data and is now also available with an 80K hours version. MooER is the first LLM-based speech model trained and inferred using domestic GPUs. The repository includes pretrained models, inference code, and a Gradio demo for a better user experience.
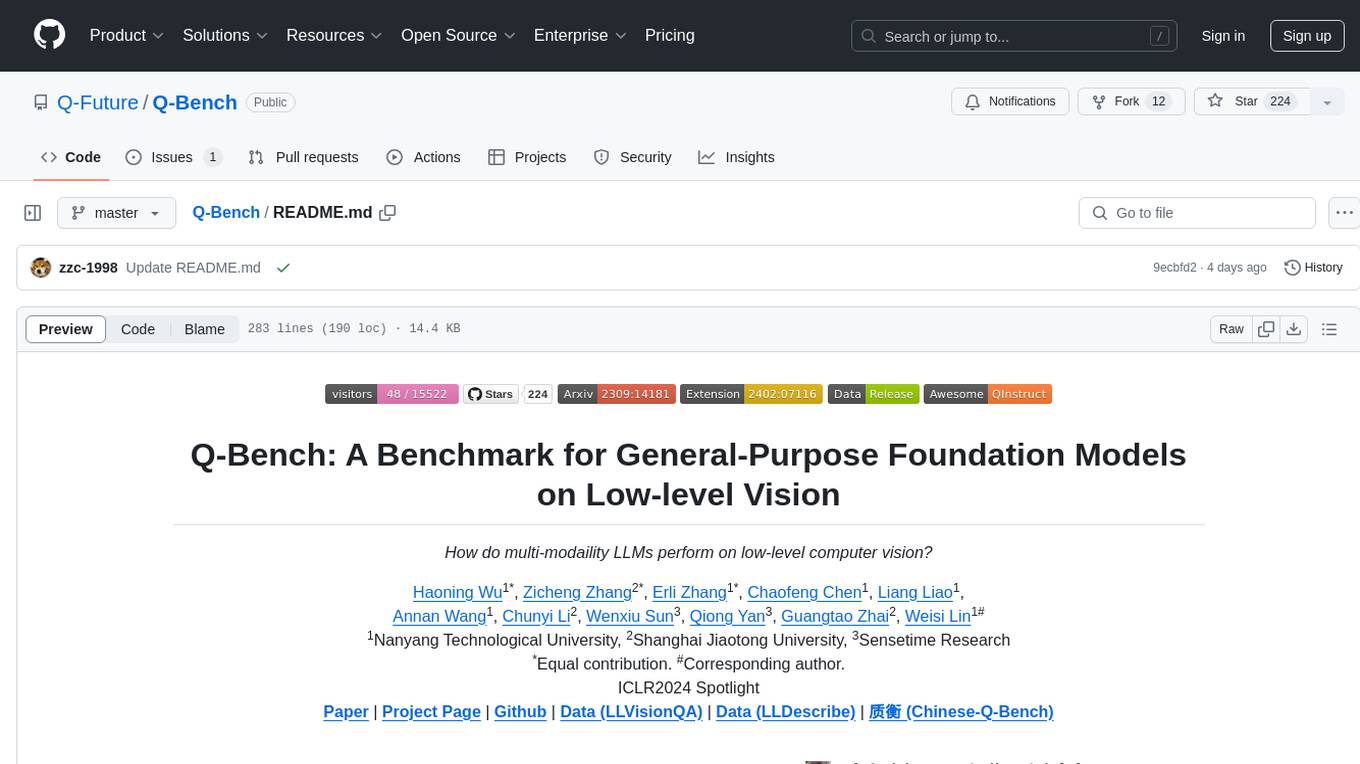
Q-Bench
Q-Bench is a benchmark for general-purpose foundation models on low-level vision, focusing on multi-modality LLMs performance. It includes three realms for low-level vision: perception, description, and assessment. The benchmark datasets LLVisionQA and LLDescribe are collected for perception and description tasks, with open submission-based evaluation. An abstract evaluation code is provided for assessment using public datasets. The tool can be used with the datasets API for single images and image pairs, allowing for automatic download and usage. Various tasks and evaluations are available for testing MLLMs on low-level vision tasks.
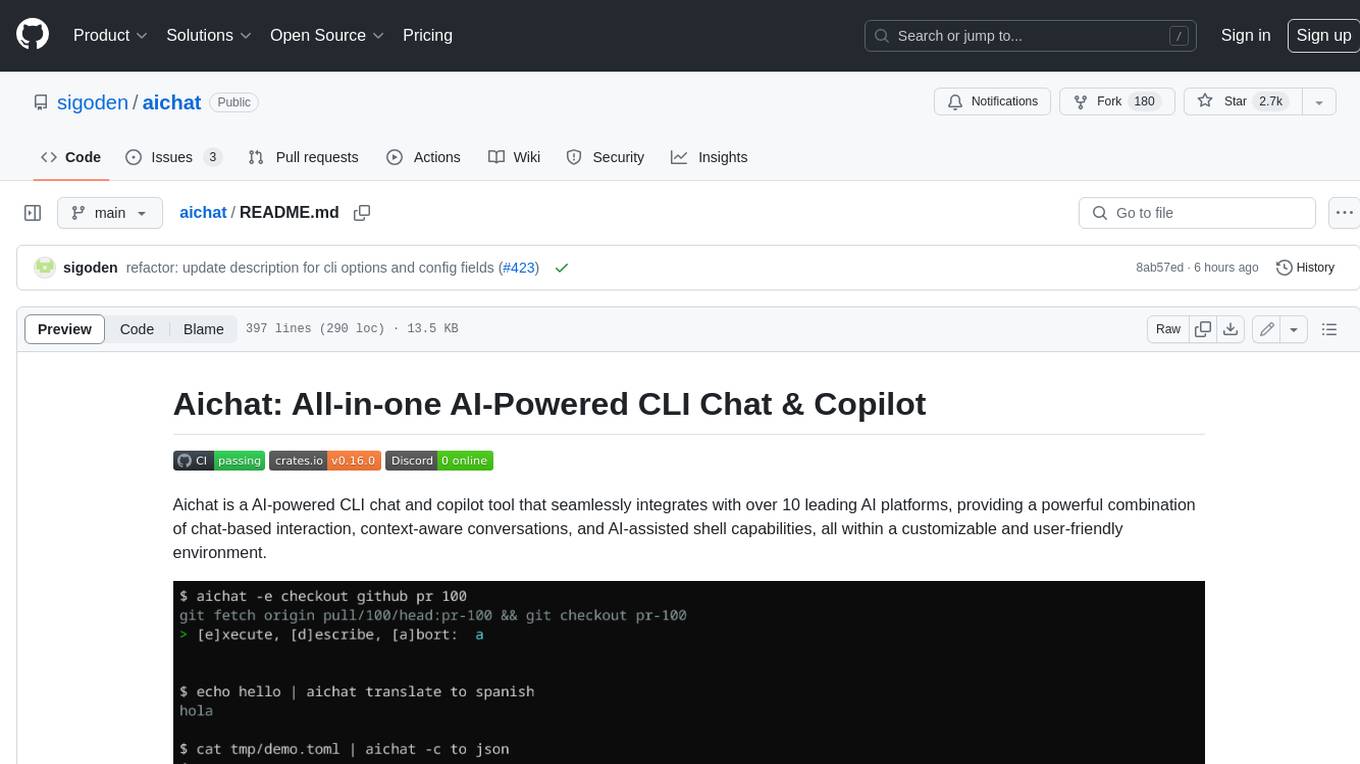
aichat
Aichat is an AI-powered CLI chat and copilot tool that seamlessly integrates with over 10 leading AI platforms, providing a powerful combination of chat-based interaction, context-aware conversations, and AI-assisted shell capabilities, all within a customizable and user-friendly environment.
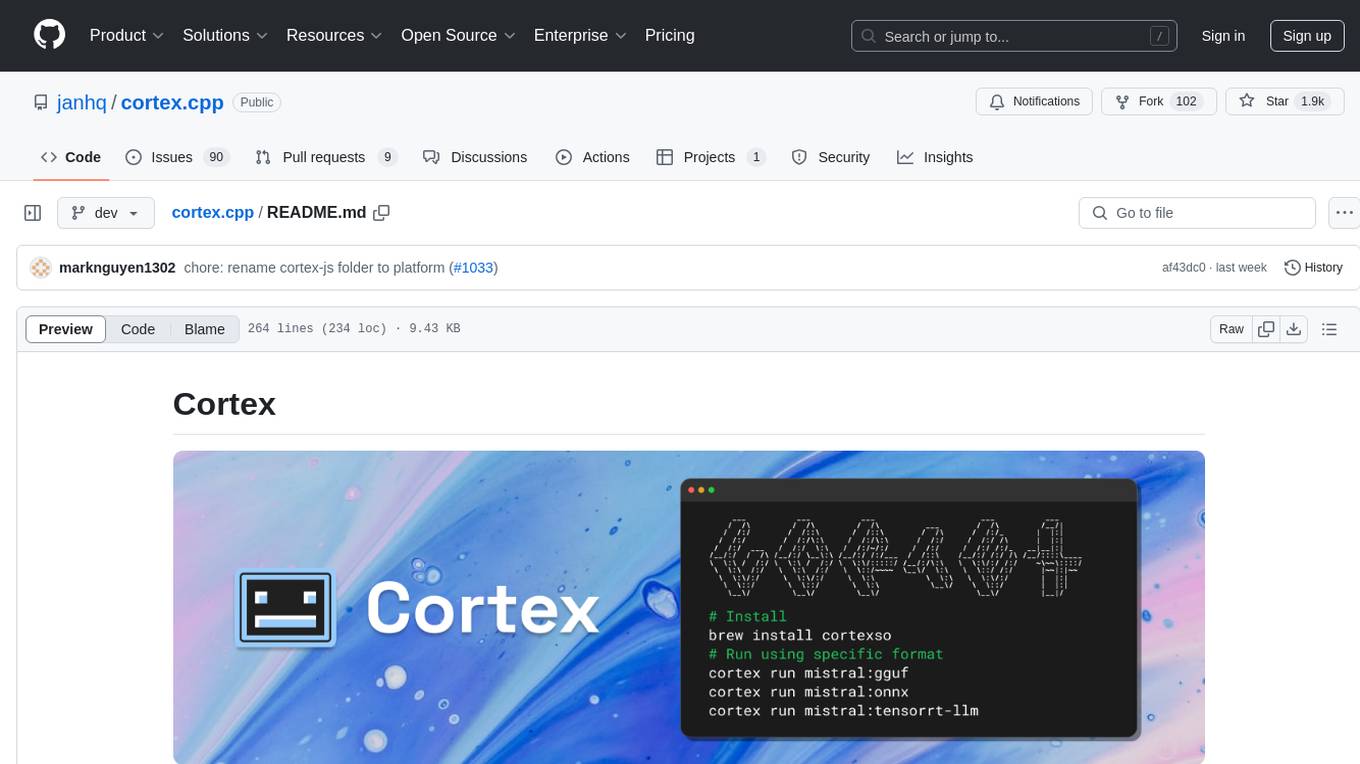
cortex.cpp
Cortex is a C++ AI engine with a Docker-like command-line interface and client libraries. It supports running AI models using ONNX, TensorRT-LLM, and llama.cpp engines. Cortex can function as a standalone server or be integrated as a library. The tool provides support for various engines and models, allowing users to easily deploy and interact with AI models. It offers a range of CLI commands for managing models, embeddings, and engines, as well as a REST API for interacting with models. Cortex is designed to simplify the deployment and usage of AI models in C++ applications.
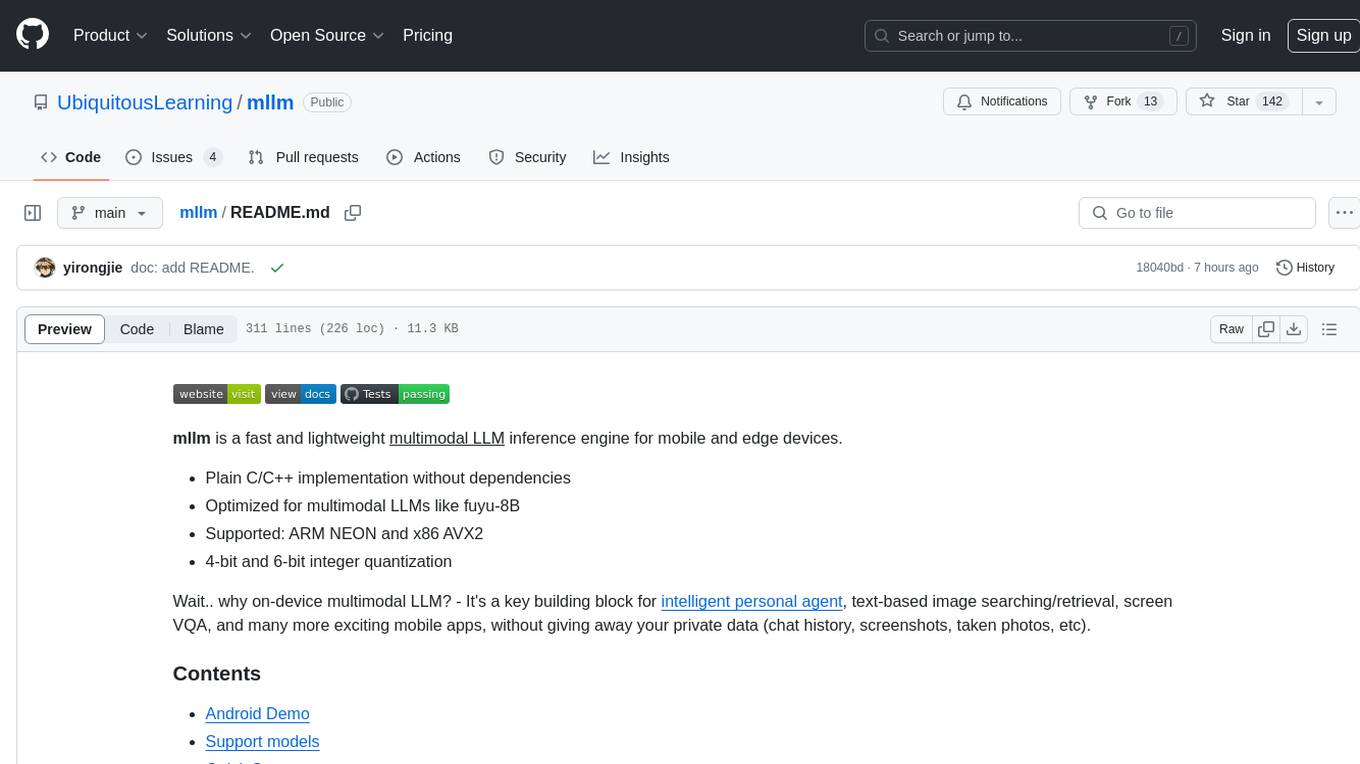
mllm
mllm is a fast and lightweight multimodal LLM inference engine for mobile and edge devices. It is a Plain C/C++ implementation without dependencies, optimized for multimodal LLMs like fuyu-8B, and supports ARM NEON and x86 AVX2. The engine offers 4-bit and 6-bit integer quantization, making it suitable for intelligent personal agents, text-based image searching/retrieval, screen VQA, and various mobile applications without compromising user privacy.
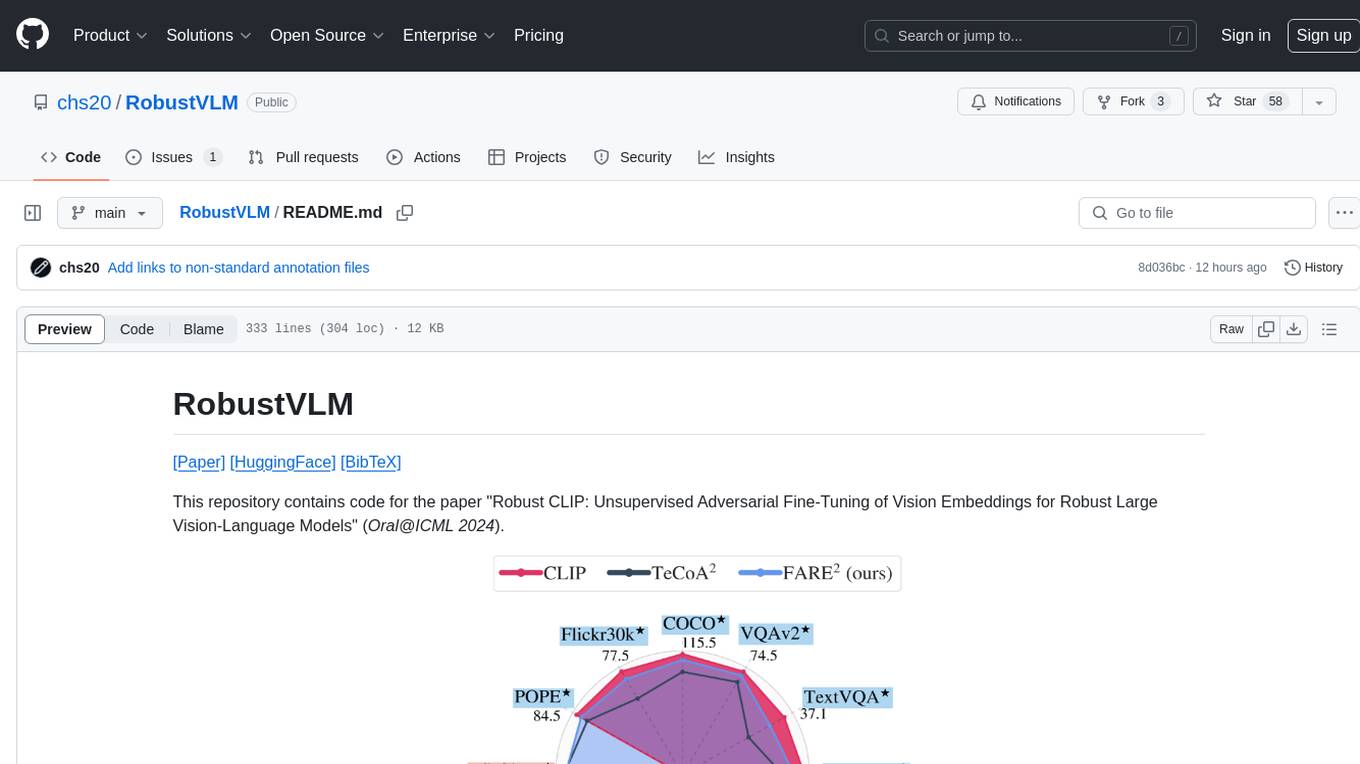
RobustVLM
This repository contains code for the paper 'Robust CLIP: Unsupervised Adversarial Fine-Tuning of Vision Embeddings for Robust Large Vision-Language Models'. It focuses on fine-tuning CLIP in an unsupervised manner to enhance its robustness against visual adversarial attacks. By replacing the vision encoder of large vision-language models with the fine-tuned CLIP models, it achieves state-of-the-art adversarial robustness on various vision-language tasks. The repository provides adversarially fine-tuned ViT-L/14 CLIP models and offers insights into zero-shot classification settings and clean accuracy improvements.
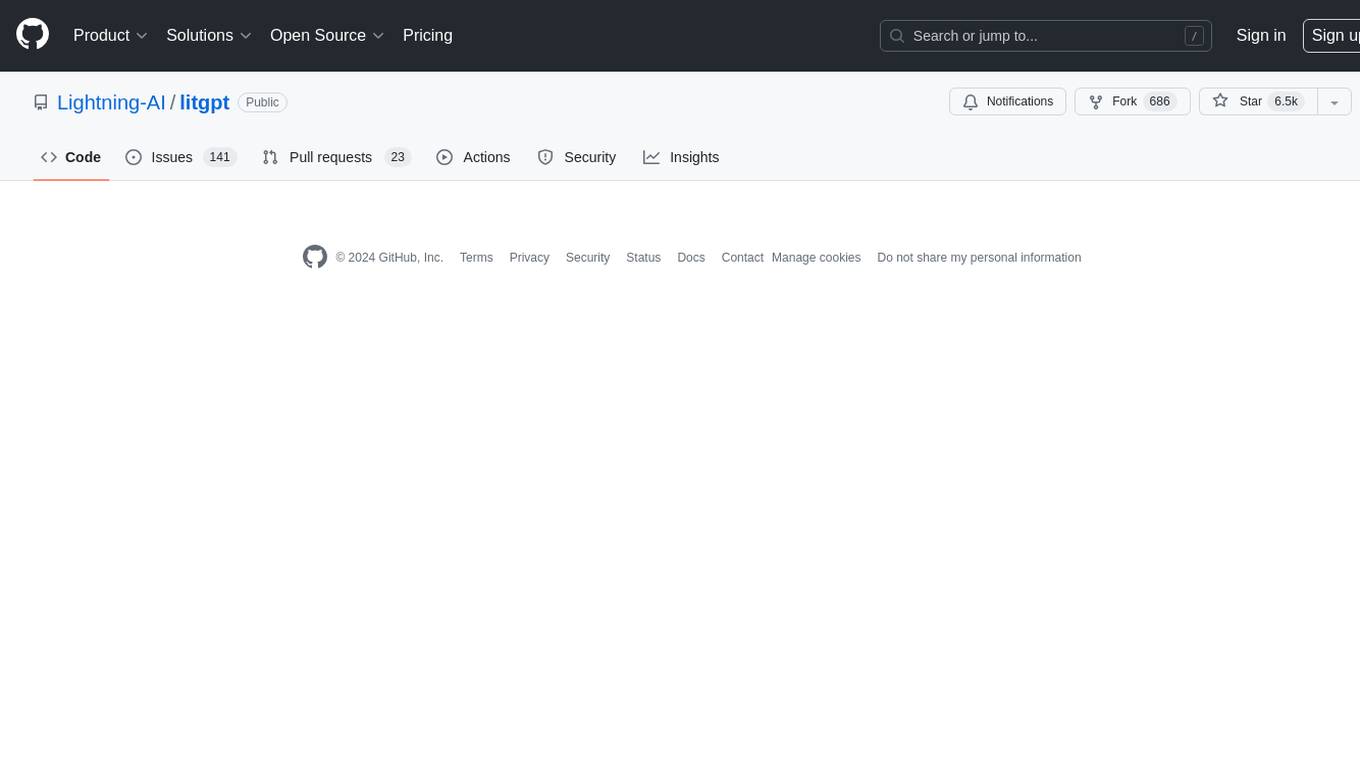
litgpt
LitGPT is a command-line tool designed to easily finetune, pretrain, evaluate, and deploy 20+ LLMs **on your own data**. It features highly-optimized training recipes for the world's most powerful open-source large-language-models (LLMs).
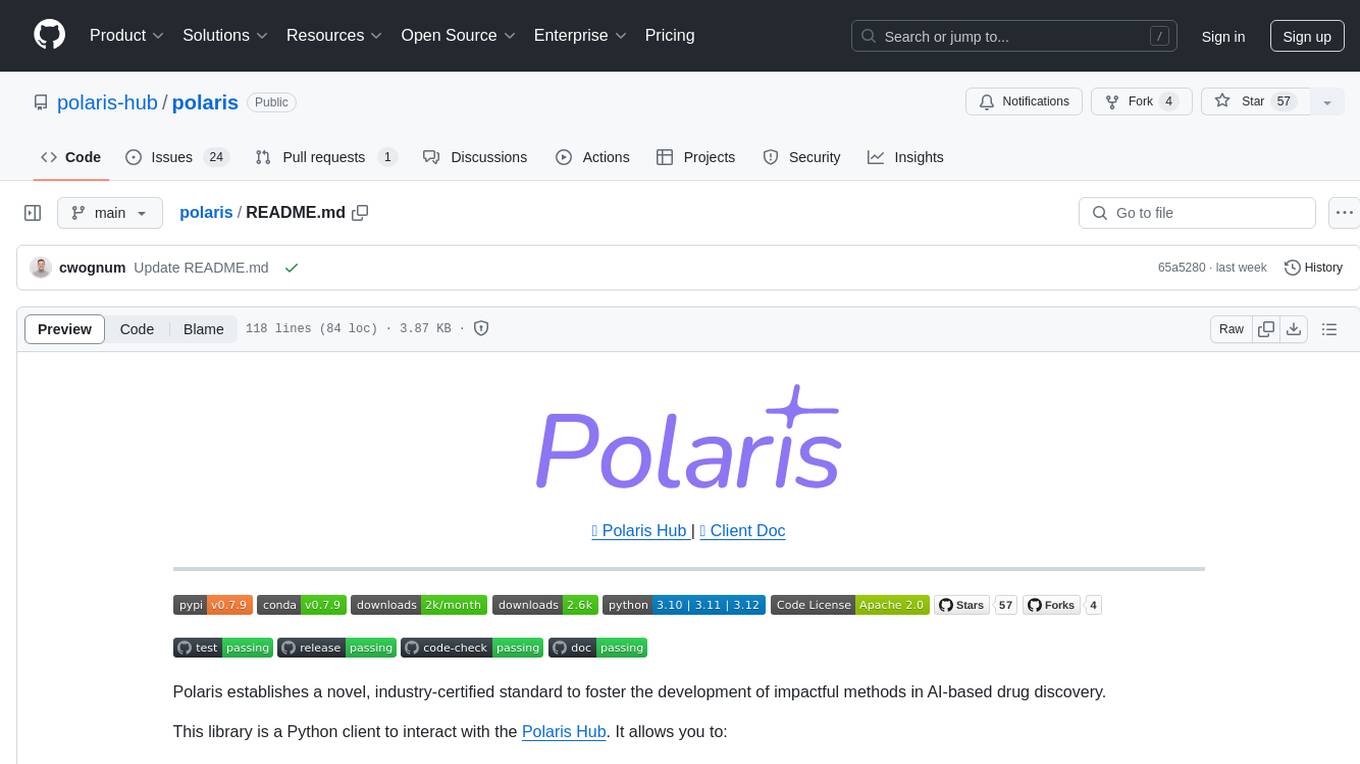
polaris
Polaris establishes a novel, industryβcertified standard to foster the development of impactful methods in AI-based drug discovery. This library is a Python client to interact with the Polaris Hub. It allows you to download Polaris datasets and benchmarks, evaluate a custom method against a Polaris benchmark, and create and upload new datasets and benchmarks.
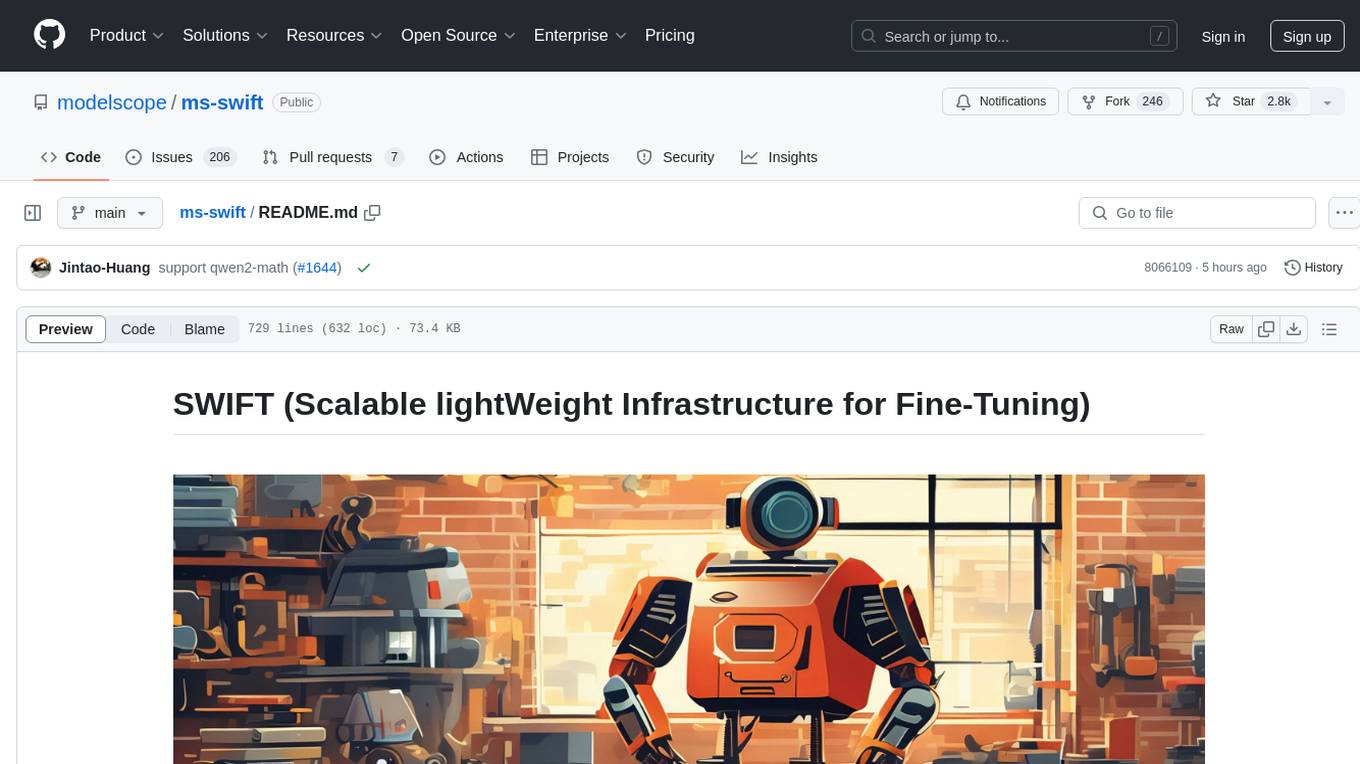
ms-swift
ms-swift is an official framework provided by the ModelScope community for fine-tuning and deploying large language models and multi-modal large models. It supports training, inference, evaluation, quantization, and deployment of over 400 large models and 100+ multi-modal large models. The framework includes various training technologies and accelerates inference, evaluation, and deployment modules. It offers a Gradio-based Web-UI interface and best practices for easy application of large models. ms-swift supports a wide range of model types, dataset types, hardware support, lightweight training methods, distributed training techniques, quantization training, RLHF training, multi-modal training, interface training, plugin and extension support, inference acceleration engines, model evaluation, and model quantization.
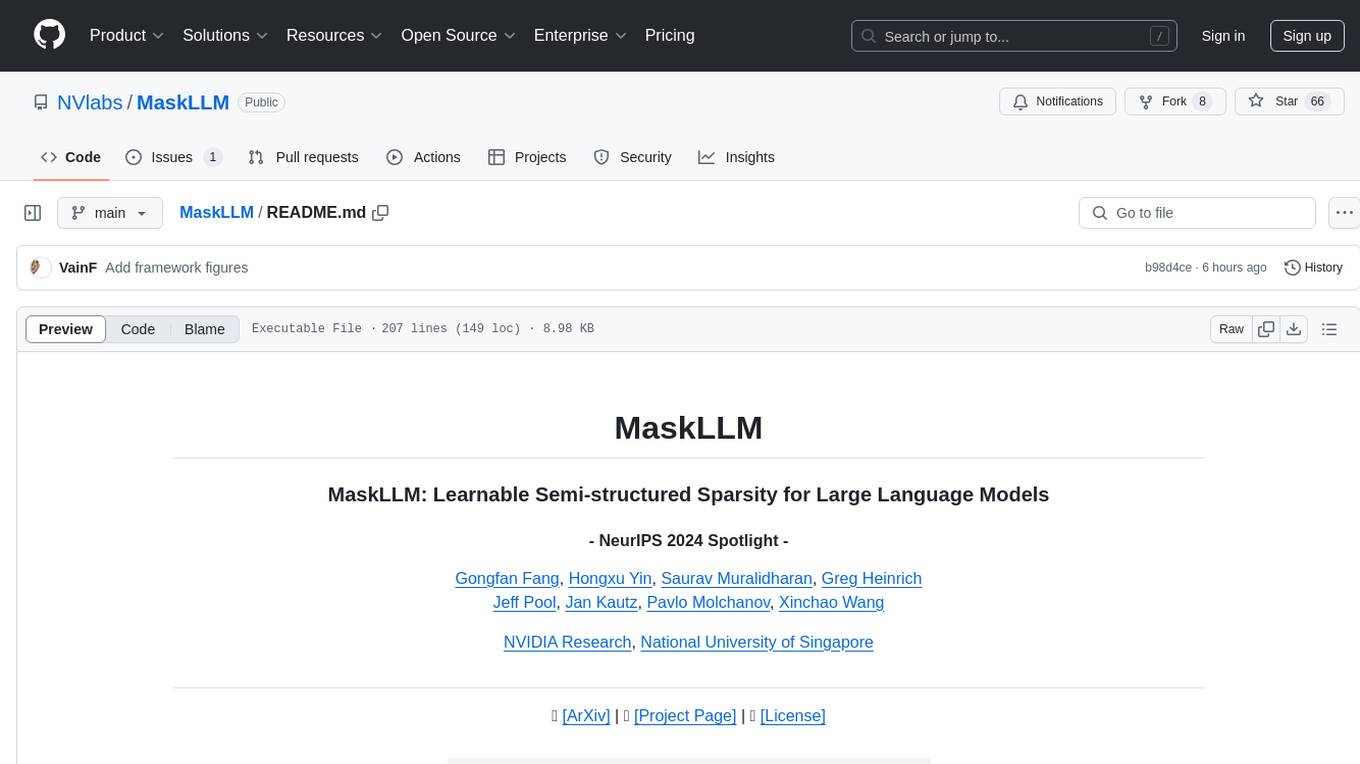
MaskLLM
MaskLLM is a learnable pruning method that establishes Semi-structured Sparsity in Large Language Models (LLMs) to reduce computational overhead during inference. It is scalable and benefits from larger training datasets. The tool provides examples for running MaskLLM with Megatron-LM, preparing LLaMA checkpoints, pre-tokenizing C4 data for Megatron, generating prior masks, training MaskLLM, and evaluating the model. It also includes instructions for exporting sparse models to Huggingface.
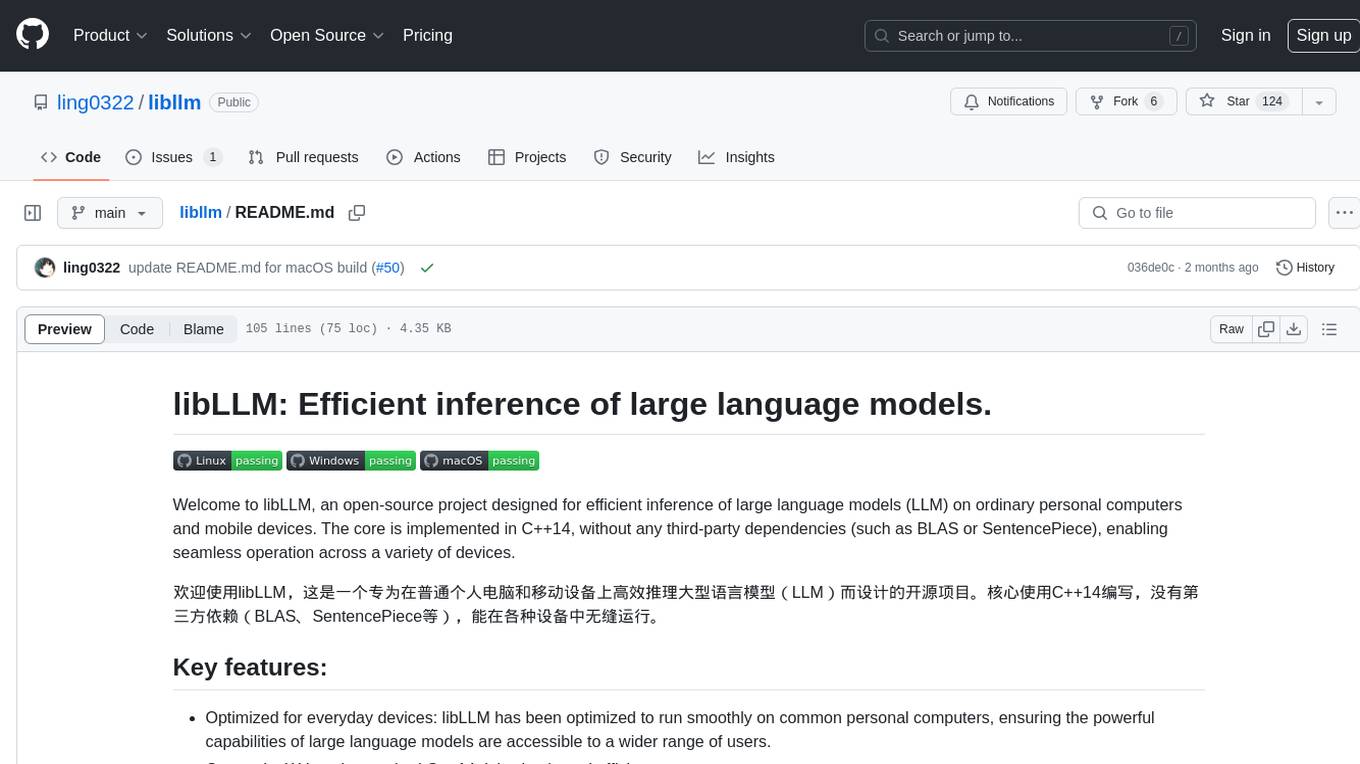
libllm
libLLM is an open-source project designed for efficient inference of large language models (LLM) on personal computers and mobile devices. It is optimized to run smoothly on common devices, written in C++14 without external dependencies, and supports CUDA for accelerated inference. Users can build the tool for CPU only or with CUDA support, and run libLLM from the command line. Additionally, there are API examples available for Python and the tool can export Huggingface models.
For similar tasks
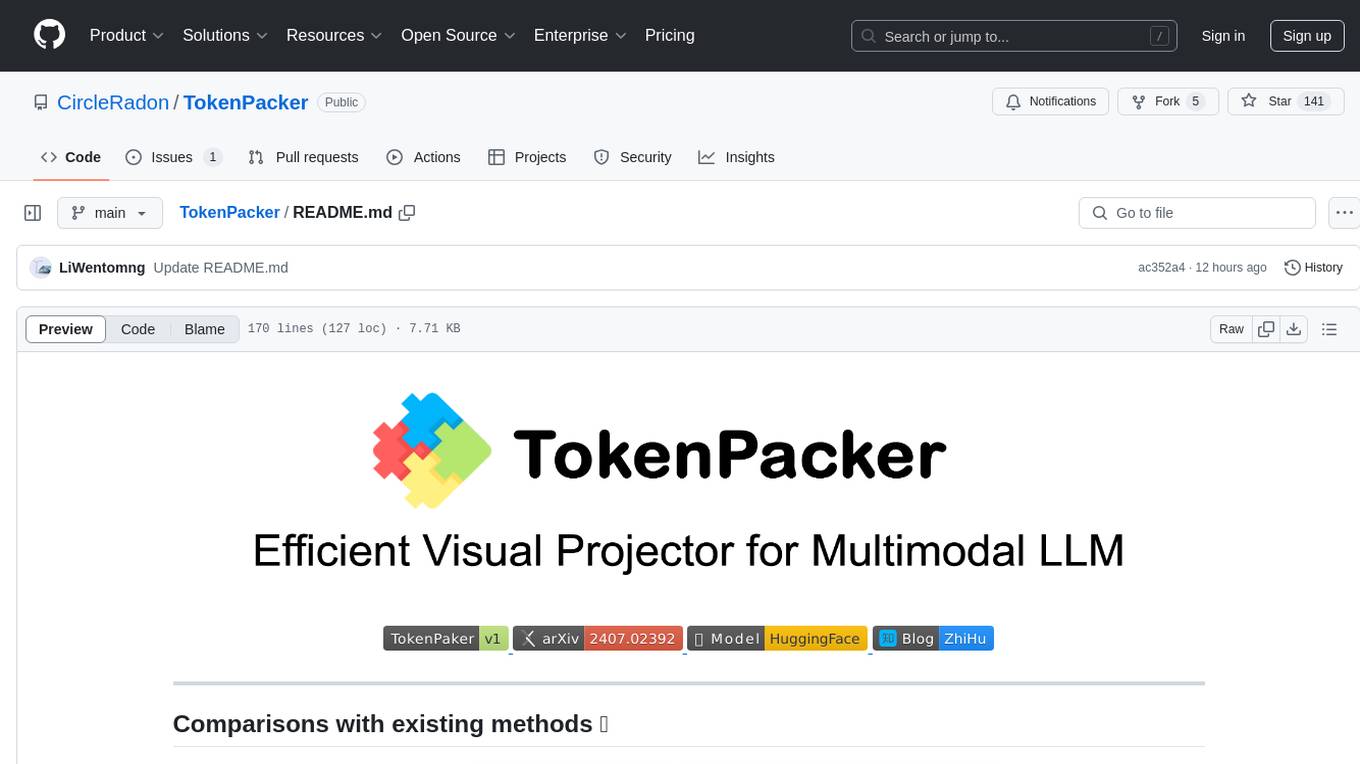
TokenPacker
TokenPacker is a novel visual projector that compresses visual tokens by 75%βΌ89% with high efficiency. It adopts a 'coarse-to-fine' scheme to generate condensed visual tokens, achieving comparable or better performance across diverse benchmarks. The tool includes TokenPacker for general use and TokenPacker-HD for high-resolution image understanding. It provides training scripts, checkpoints, and supports various compression ratios and patch numbers.
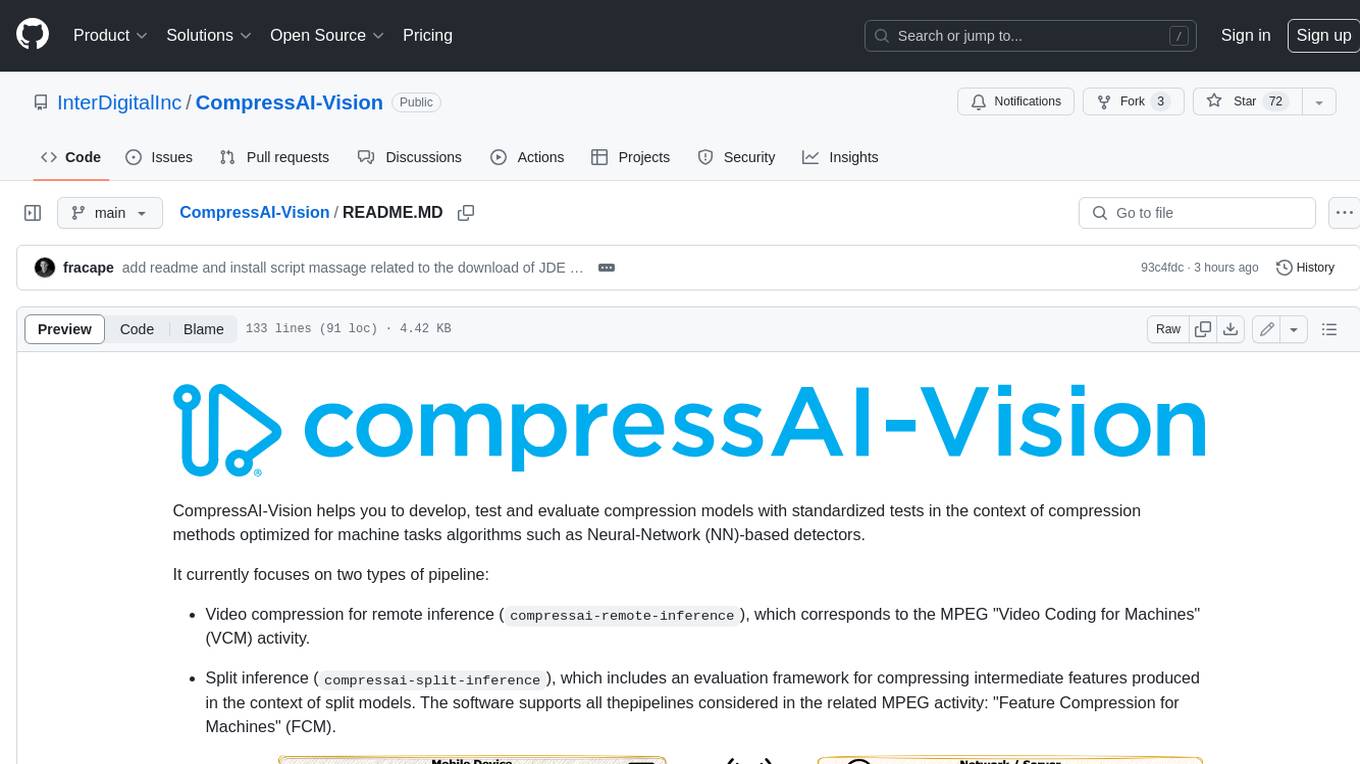
CompressAI-Vision
CompressAI-Vision is a tool that helps you develop, test, and evaluate compression models with standardized tests in the context of compression methods optimized for machine tasks algorithms such as Neural-Network (NN)-based detectors. It currently focuses on two types of pipeline: Video compression for remote inference (`compressai-remote-inference`), which corresponds to the MPEG "Video Coding for Machines" (VCM) activity. Split inference (`compressai-split-inference`), which includes an evaluation framework for compressing intermediate features produced in the context of split models. The software supports all the pipelines considered in the related MPEG activity: "Feature Compression for Machines" (FCM).
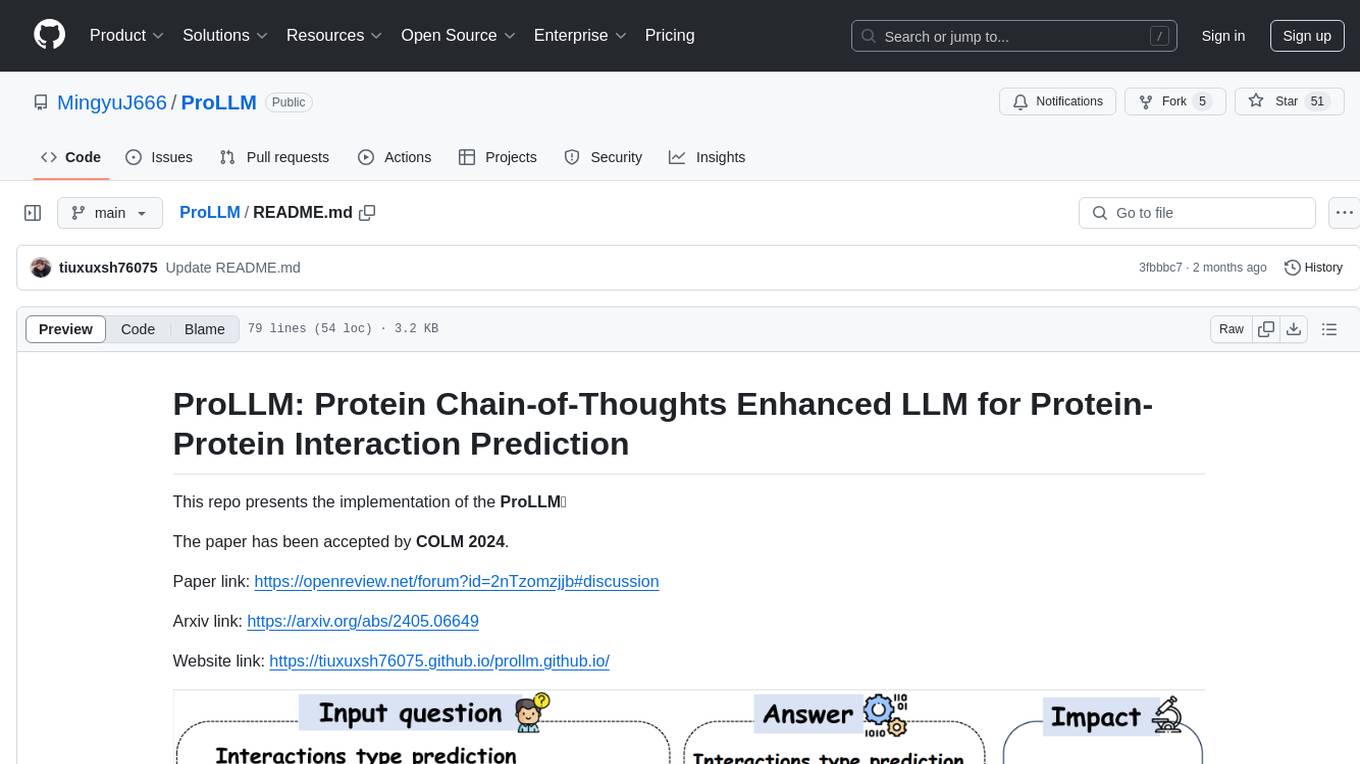
ProLLM
ProLLM is a framework that leverages Large Language Models to interpret and analyze protein sequences and interactions through natural language processing. It introduces the Protein Chain of Thought (ProCoT) method to transform complex protein interaction data into intuitive prompts, enhancing predictive accuracy by incorporating protein-specific embeddings and fine-tuning on domain-specific datasets.
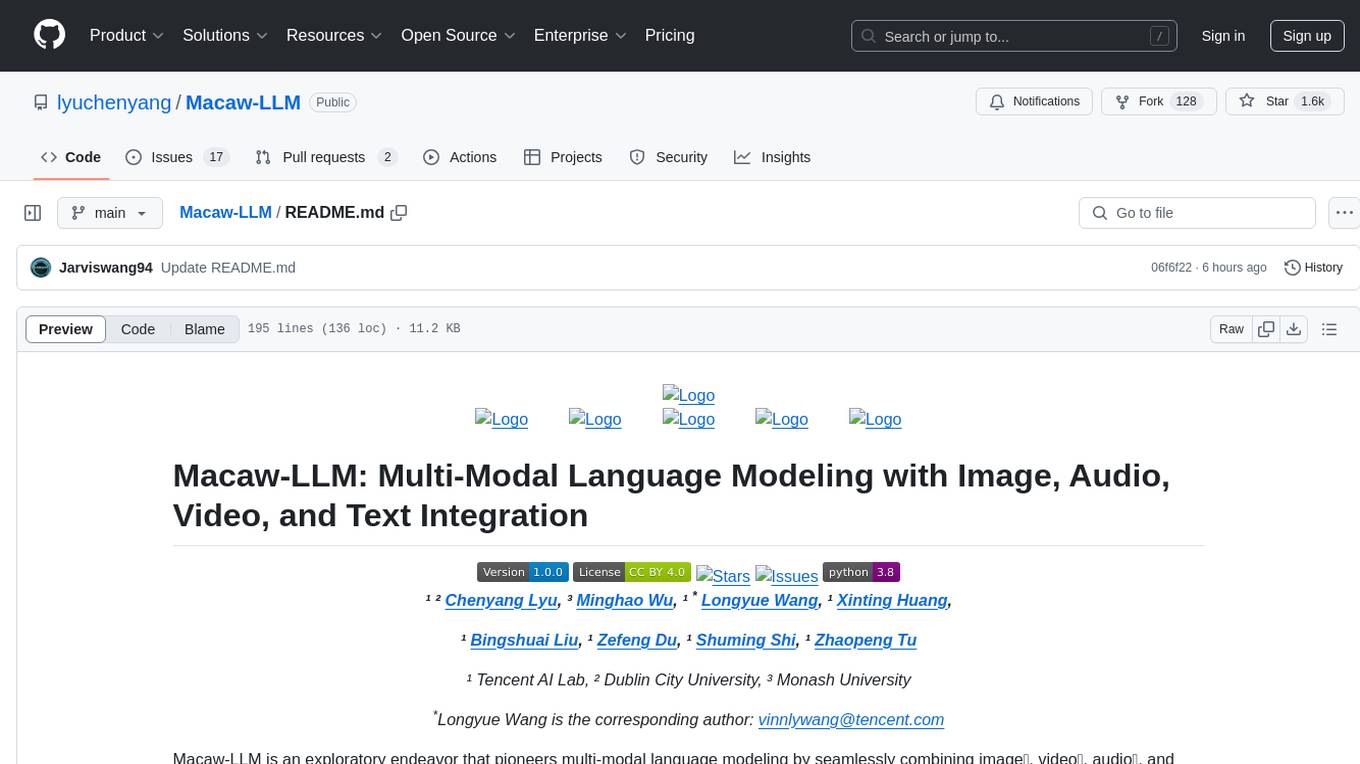
Macaw-LLM
Macaw-LLM is a pioneering multi-modal language modeling tool that seamlessly integrates image, audio, video, and text data. It builds upon CLIP, Whisper, and LLaMA models to process and analyze multi-modal information effectively. The tool boasts features like simple and fast alignment, one-stage instruction fine-tuning, and a new multi-modal instruction dataset. It enables users to align multi-modal features efficiently, encode instructions, and generate responses across different data types.
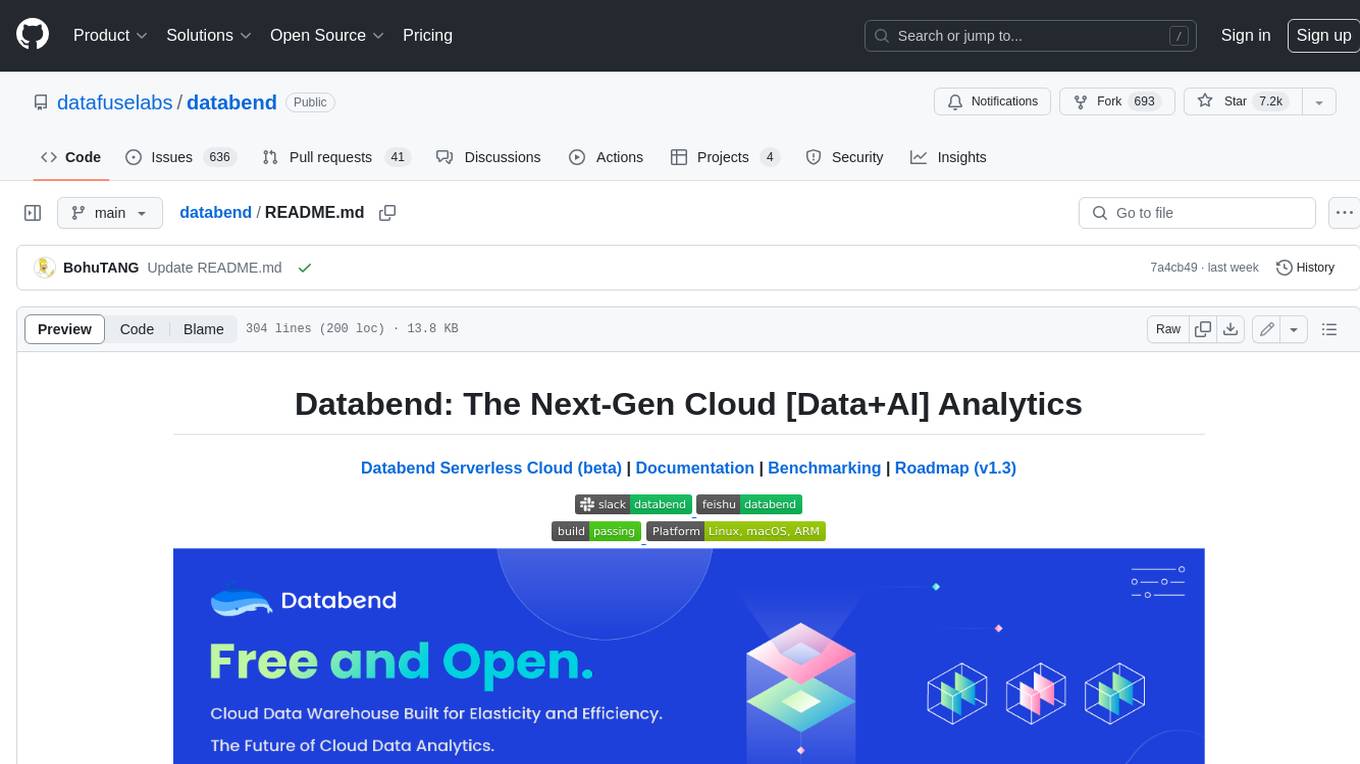
databend
Databend is an open-source cloud data warehouse that serves as a cost-effective alternative to Snowflake. With its focus on fast query execution and data ingestion, it's designed for complex analysis of the world's largest datasets.
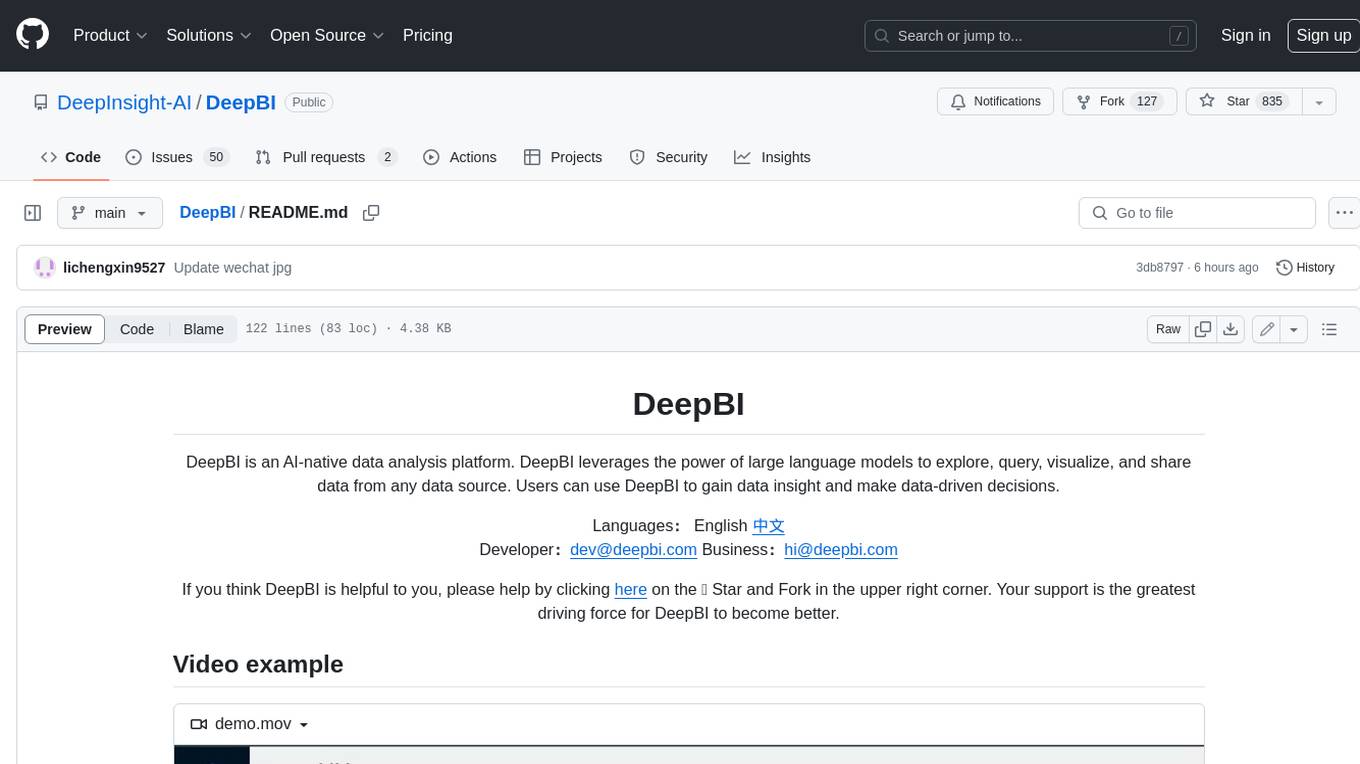
DeepBI
DeepBI is an AI-native data analysis platform that leverages the power of large language models to explore, query, visualize, and share data from any data source. Users can use DeepBI to gain data insight and make data-driven decisions.
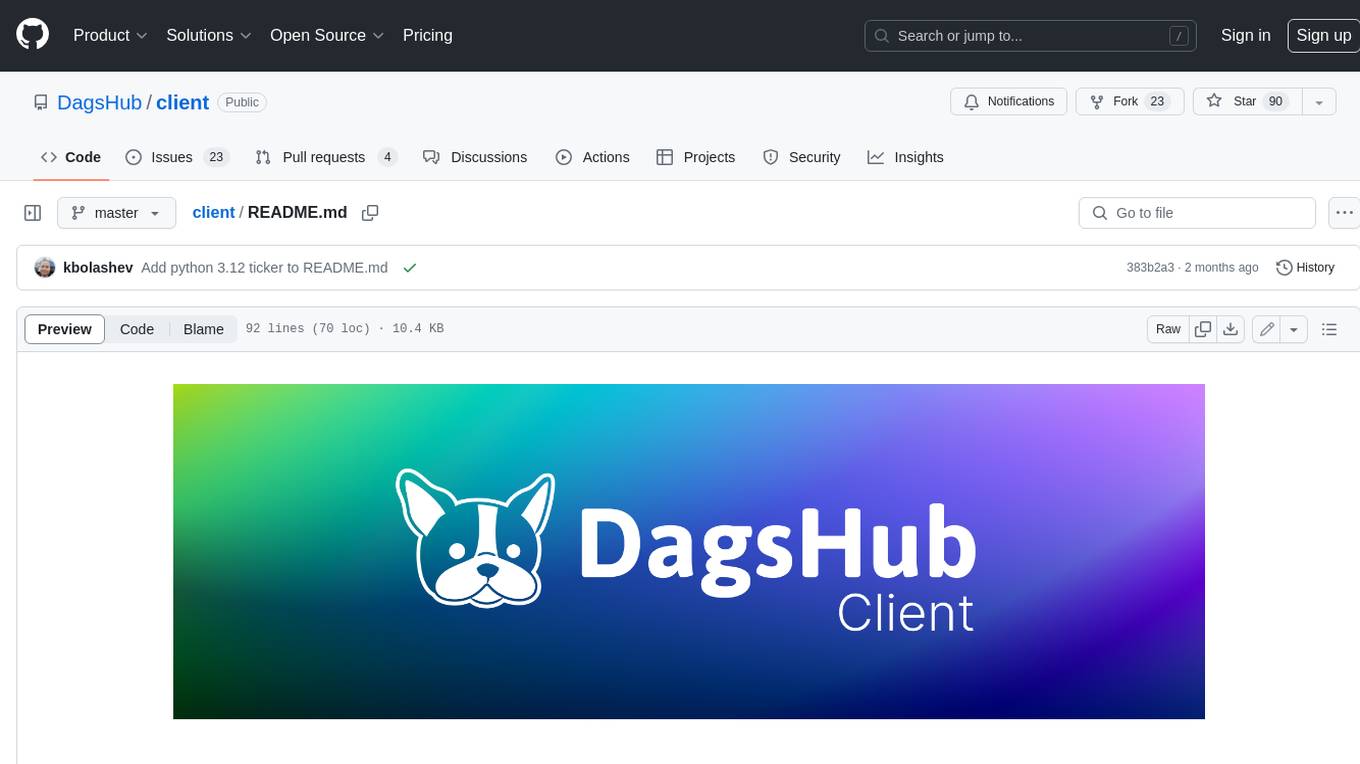
client
DagsHub is a platform for machine learning and data science teams to build, manage, and collaborate on their projects. With DagsHub you can: 1. Version code, data, and models in one place. Use the free provided DagsHub storage or connect it to your cloud storage 2. Track Experiments using Git, DVC or MLflow, to provide a fully reproducible environment 3. Visualize pipelines, data, and notebooks in and interactive, diff-able, and dynamic way 4. Label your data directly on the platform using Label Studio 5. Share your work with your team members 6. Stream and upload your data in an intuitive and easy way, while preserving versioning and structure. DagsHub is built firmly around open, standard formats for your project. In particular: * Git * DVC * MLflow * Label Studio * Standard data formats like YAML, JSON, CSV Therefore, you can work with DagsHub regardless of your chosen programming language or frameworks.
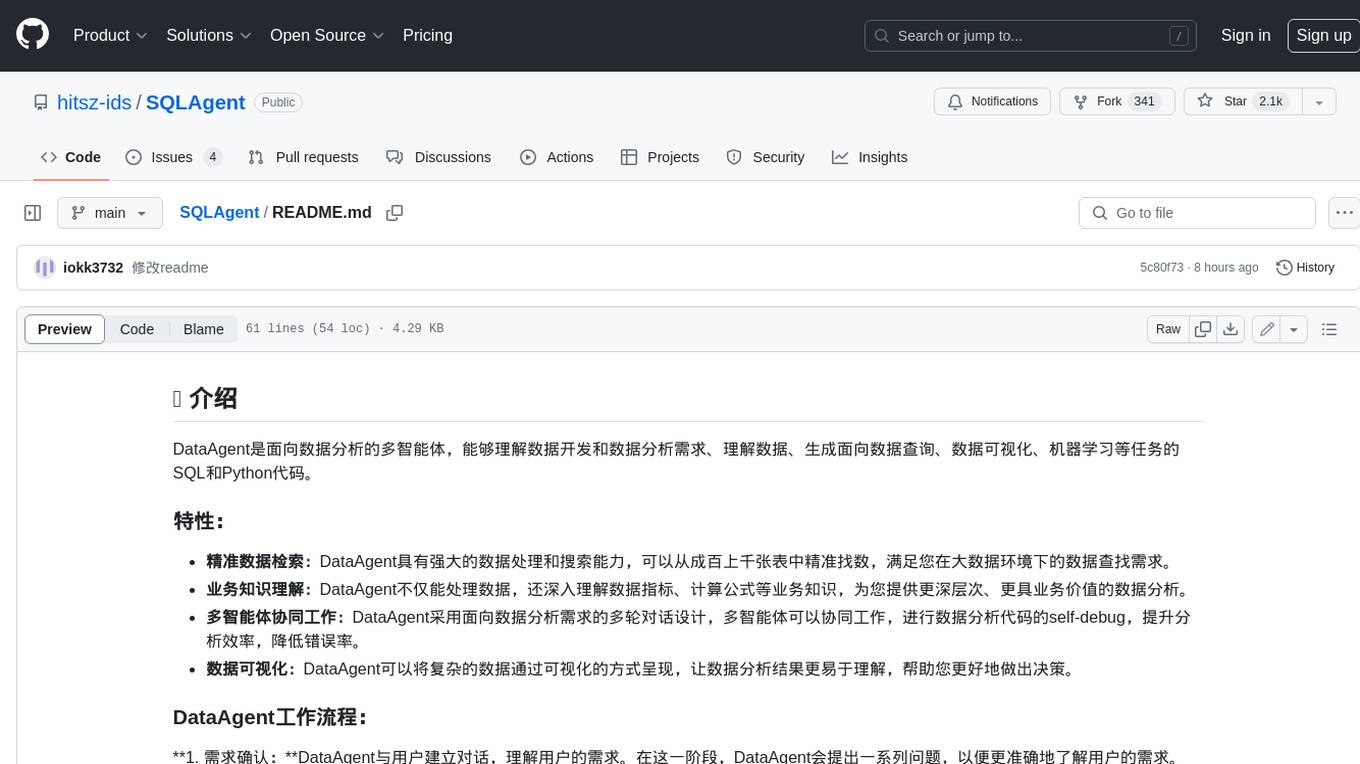
SQLAgent
DataAgent is a multi-agent system for data analysis, capable of understanding data development and data analysis requirements, understanding data, and generating SQL and Python code for tasks such as data query, data visualization, and machine learning.
For similar jobs
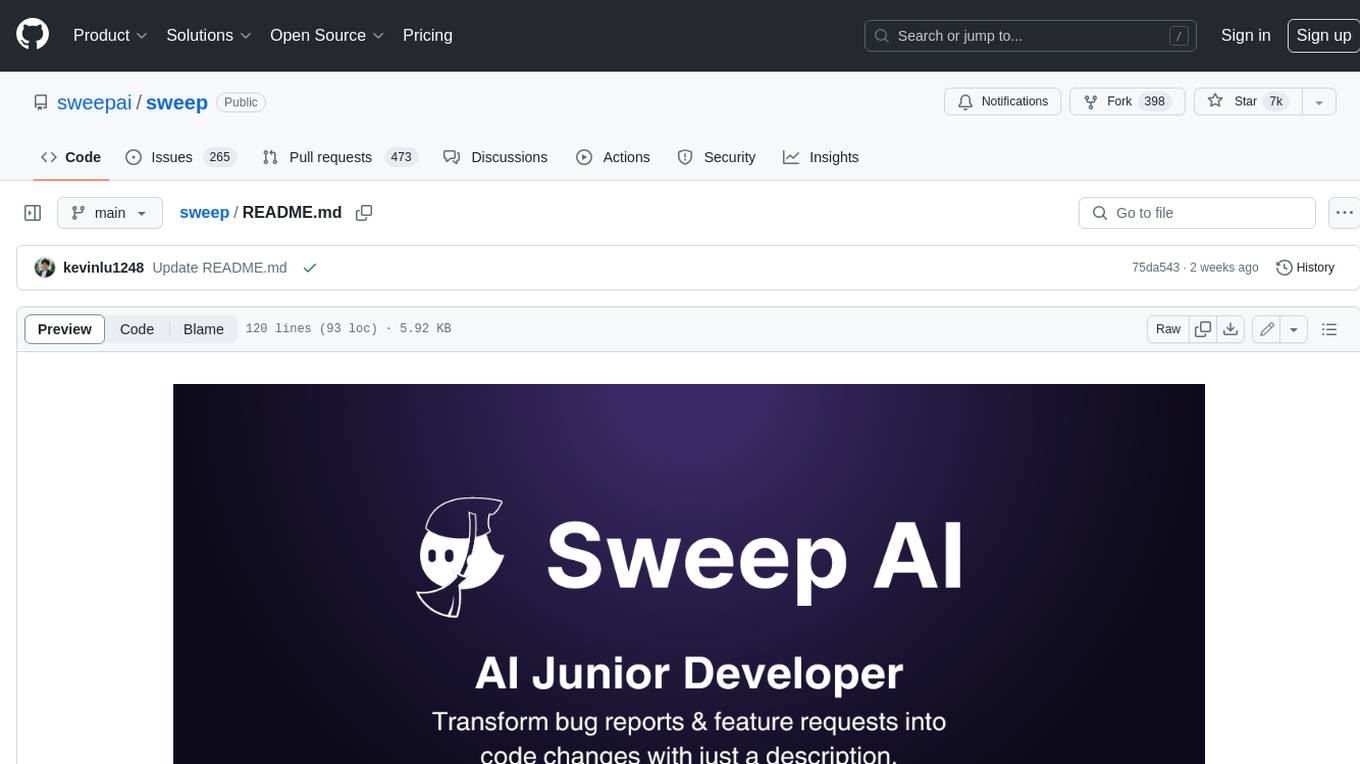
sweep
Sweep is an AI junior developer that turns bugs and feature requests into code changes. It automatically handles developer experience improvements like adding type hints and improving test coverage.
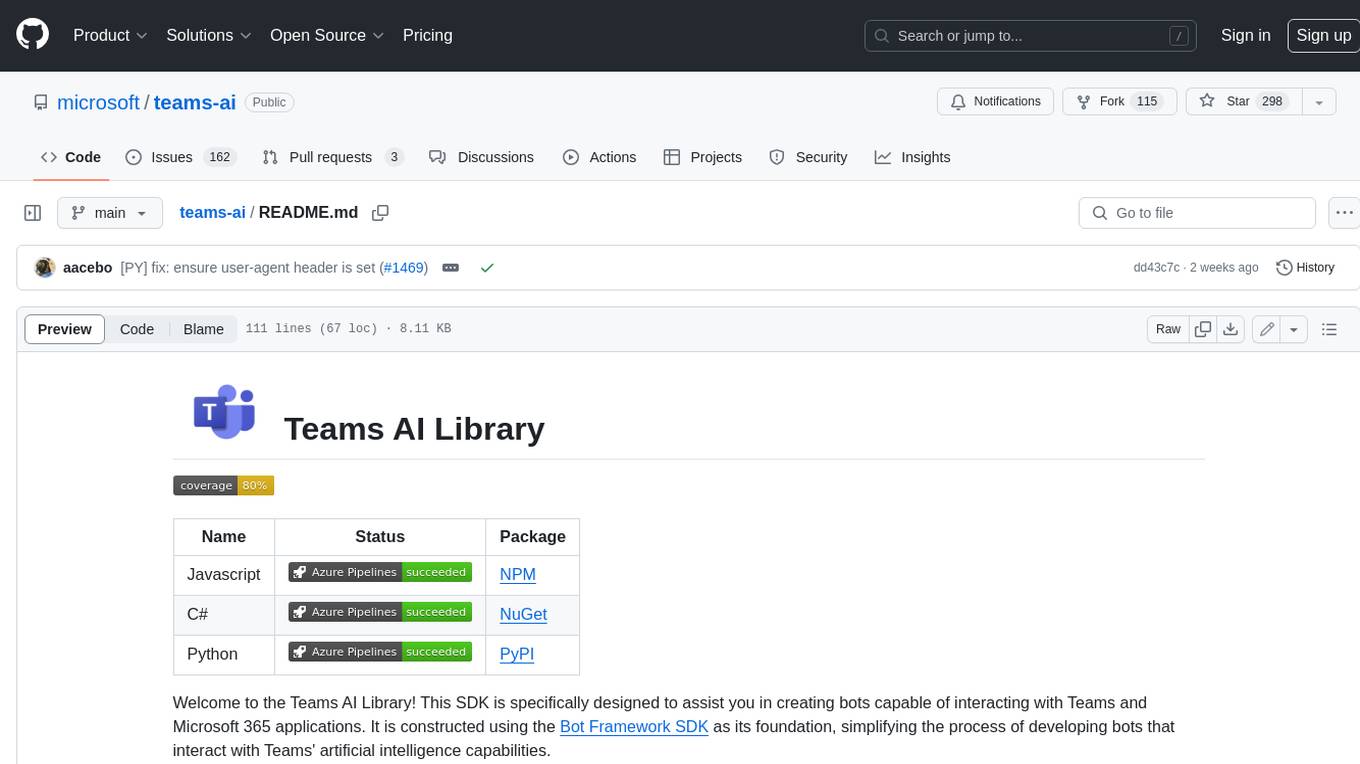
teams-ai
The Teams AI Library is a software development kit (SDK) that helps developers create bots that can interact with Teams and Microsoft 365 applications. It is built on top of the Bot Framework SDK and simplifies the process of developing bots that interact with Teams' artificial intelligence capabilities. The SDK is available for JavaScript/TypeScript, .NET, and Python.
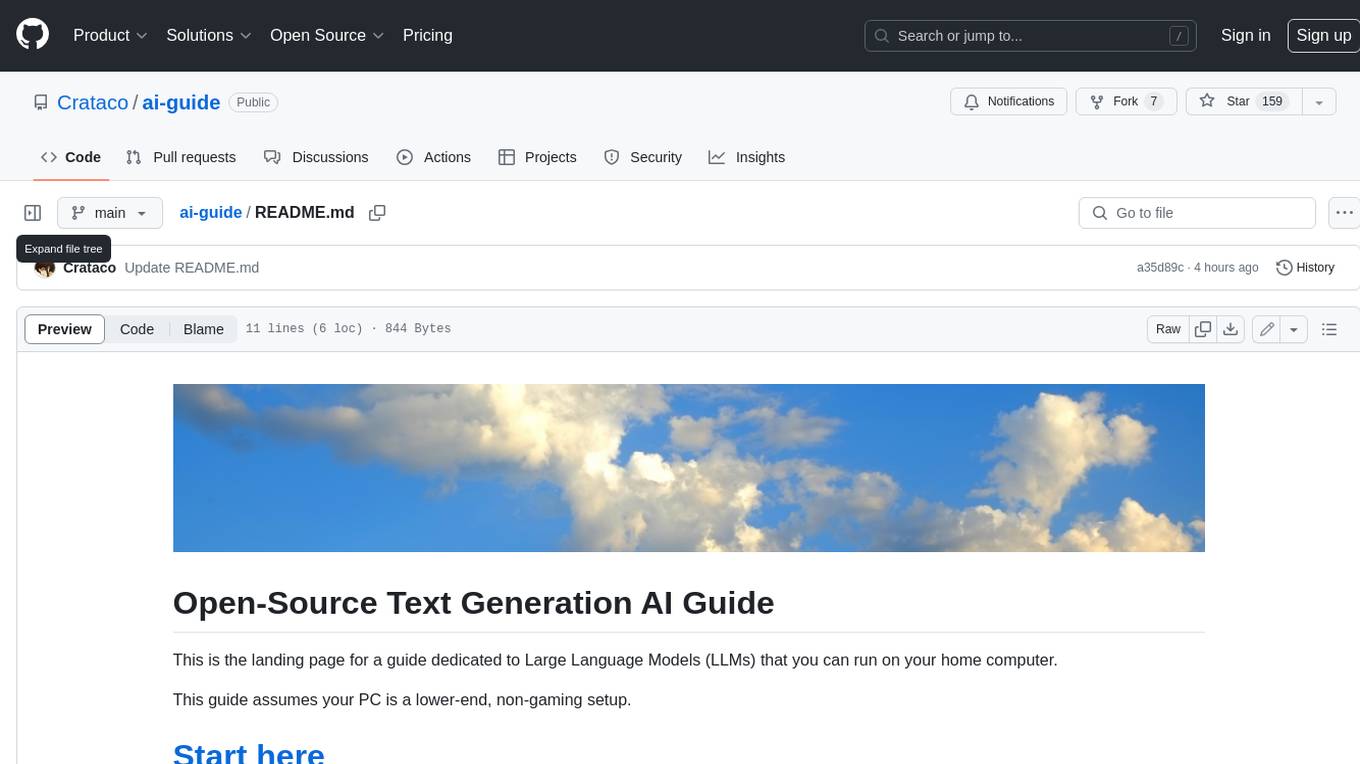
ai-guide
This guide is dedicated to Large Language Models (LLMs) that you can run on your home computer. It assumes your PC is a lower-end, non-gaming setup.
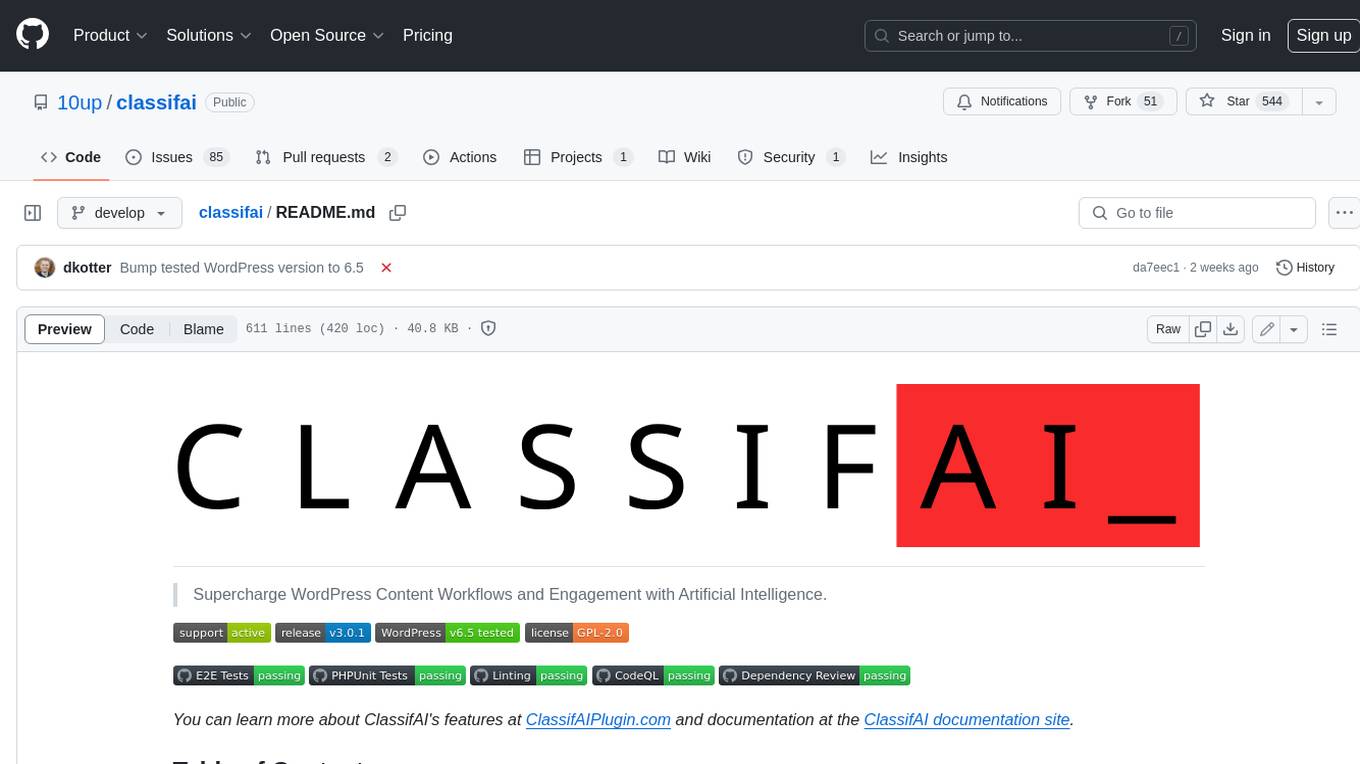
classifai
Supercharge WordPress Content Workflows and Engagement with Artificial Intelligence. Tap into leading cloud-based services like OpenAI, Microsoft Azure AI, Google Gemini and IBM Watson to augment your WordPress-powered websites. Publish content faster while improving SEO performance and increasing audience engagement. ClassifAI integrates Artificial Intelligence and Machine Learning technologies to lighten your workload and eliminate tedious tasks, giving you more time to create original content that matters.
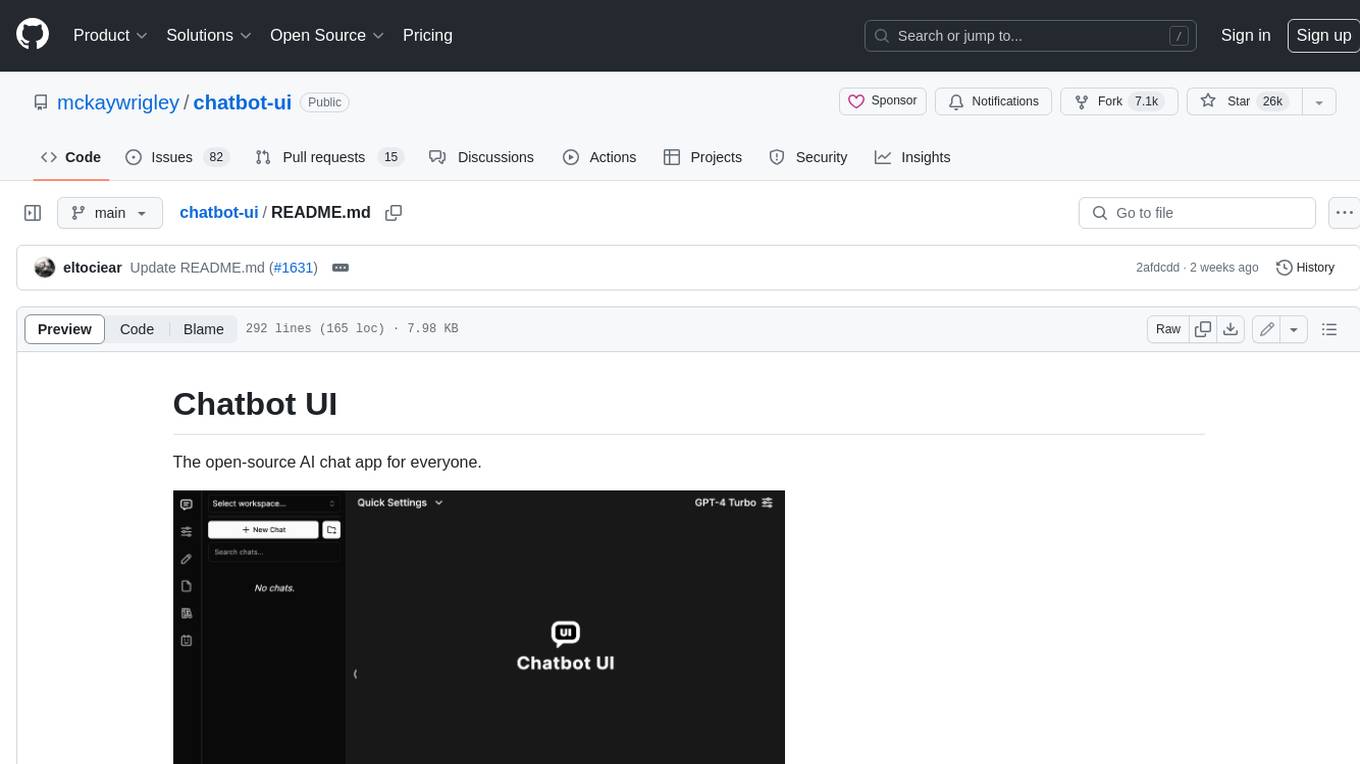
chatbot-ui
Chatbot UI is an open-source AI chat app that allows users to create and deploy their own AI chatbots. It is easy to use and can be customized to fit any need. Chatbot UI is perfect for businesses, developers, and anyone who wants to create a chatbot.
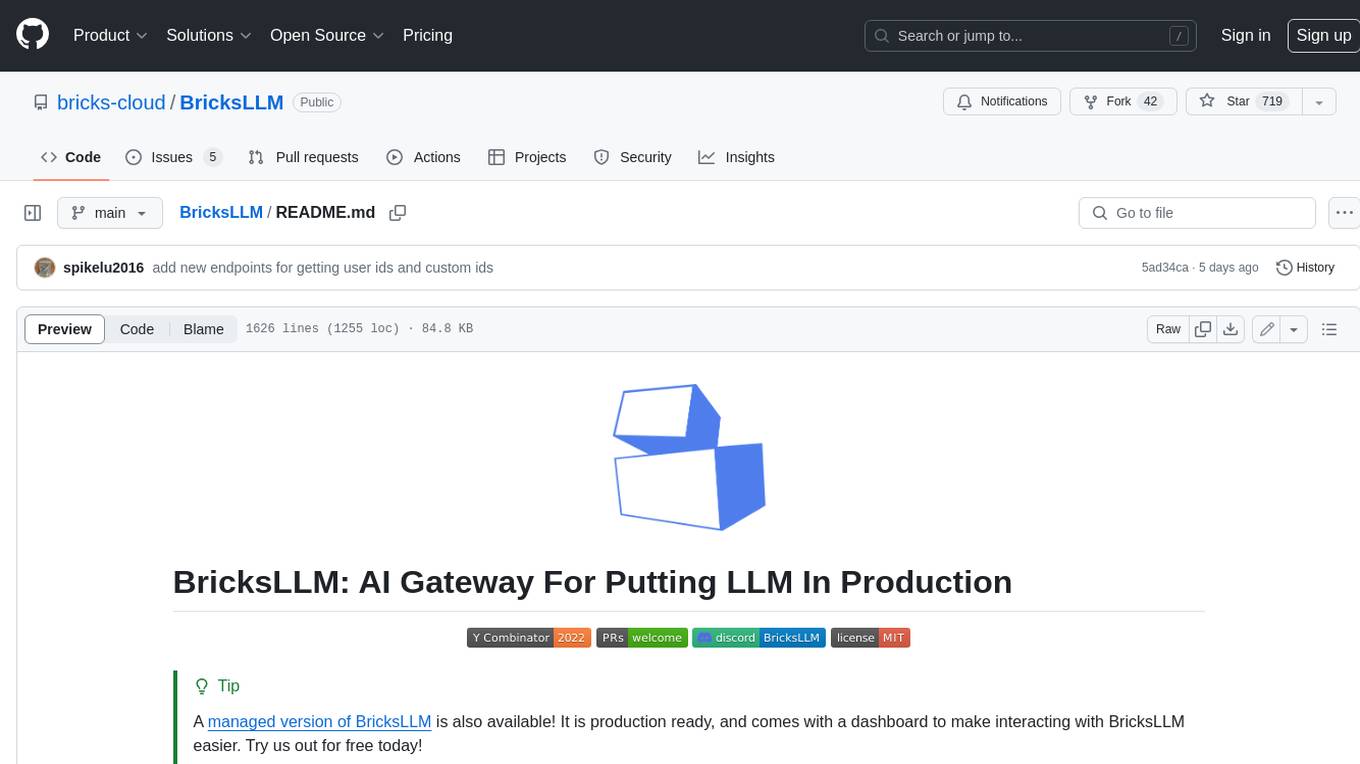
BricksLLM
BricksLLM is a cloud native AI gateway written in Go. Currently, it provides native support for OpenAI, Anthropic, Azure OpenAI and vLLM. BricksLLM aims to provide enterprise level infrastructure that can power any LLM production use cases. Here are some use cases for BricksLLM: * Set LLM usage limits for users on different pricing tiers * Track LLM usage on a per user and per organization basis * Block or redact requests containing PIIs * Improve LLM reliability with failovers, retries and caching * Distribute API keys with rate limits and cost limits for internal development/production use cases * Distribute API keys with rate limits and cost limits for students
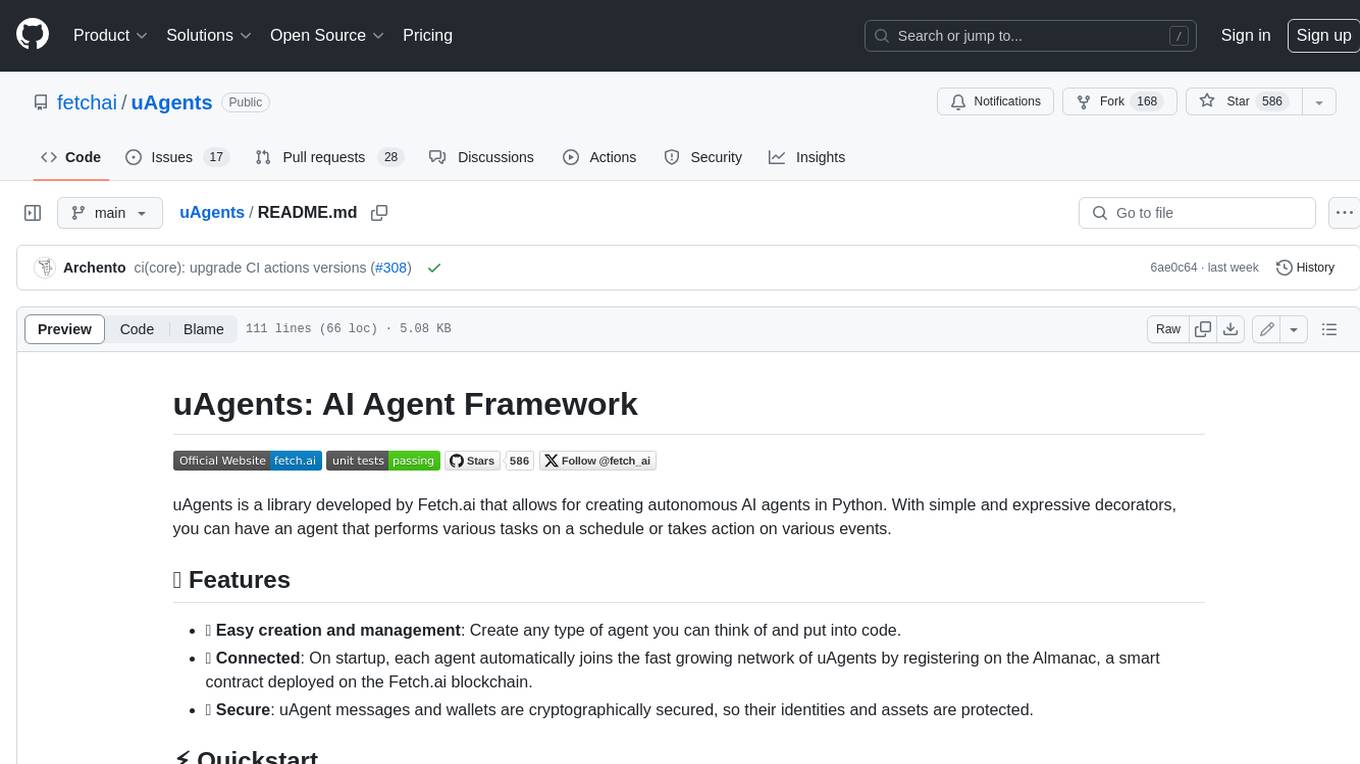
uAgents
uAgents is a Python library developed by Fetch.ai that allows for the creation of autonomous AI agents. These agents can perform various tasks on a schedule or take action on various events. uAgents are easy to create and manage, and they are connected to a fast-growing network of other uAgents. They are also secure, with cryptographically secured messages and wallets.
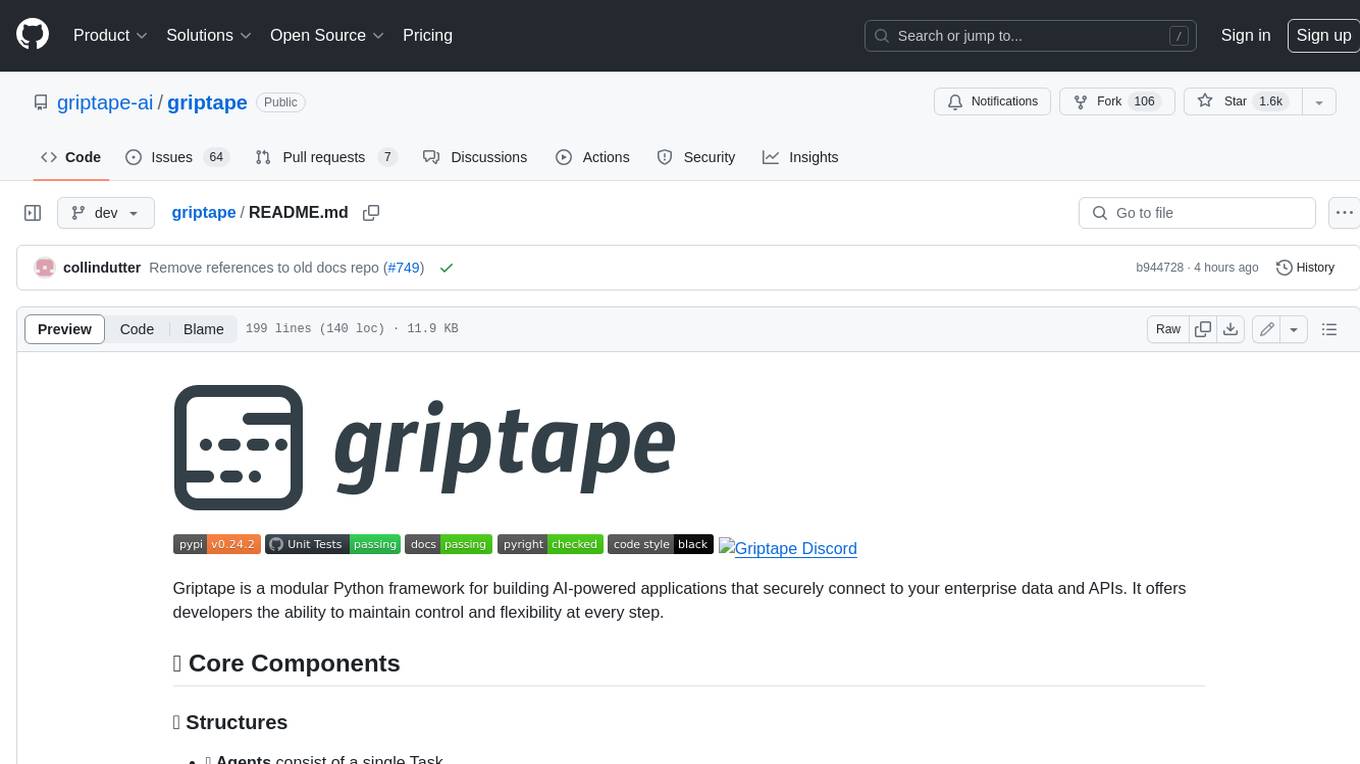
griptape
Griptape is a modular Python framework for building AI-powered applications that securely connect to your enterprise data and APIs. It offers developers the ability to maintain control and flexibility at every step. Griptape's core components include Structures (Agents, Pipelines, and Workflows), Tasks, Tools, Memory (Conversation Memory, Task Memory, and Meta Memory), Drivers (Prompt and Embedding Drivers, Vector Store Drivers, Image Generation Drivers, Image Query Drivers, SQL Drivers, Web Scraper Drivers, and Conversation Memory Drivers), Engines (Query Engines, Extraction Engines, Summary Engines, Image Generation Engines, and Image Query Engines), and additional components (Rulesets, Loaders, Artifacts, Chunkers, and Tokenizers). Griptape enables developers to create AI-powered applications with ease and efficiency.