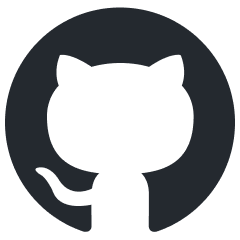
Chinese-Tiny-LLM
None
Stars: 168
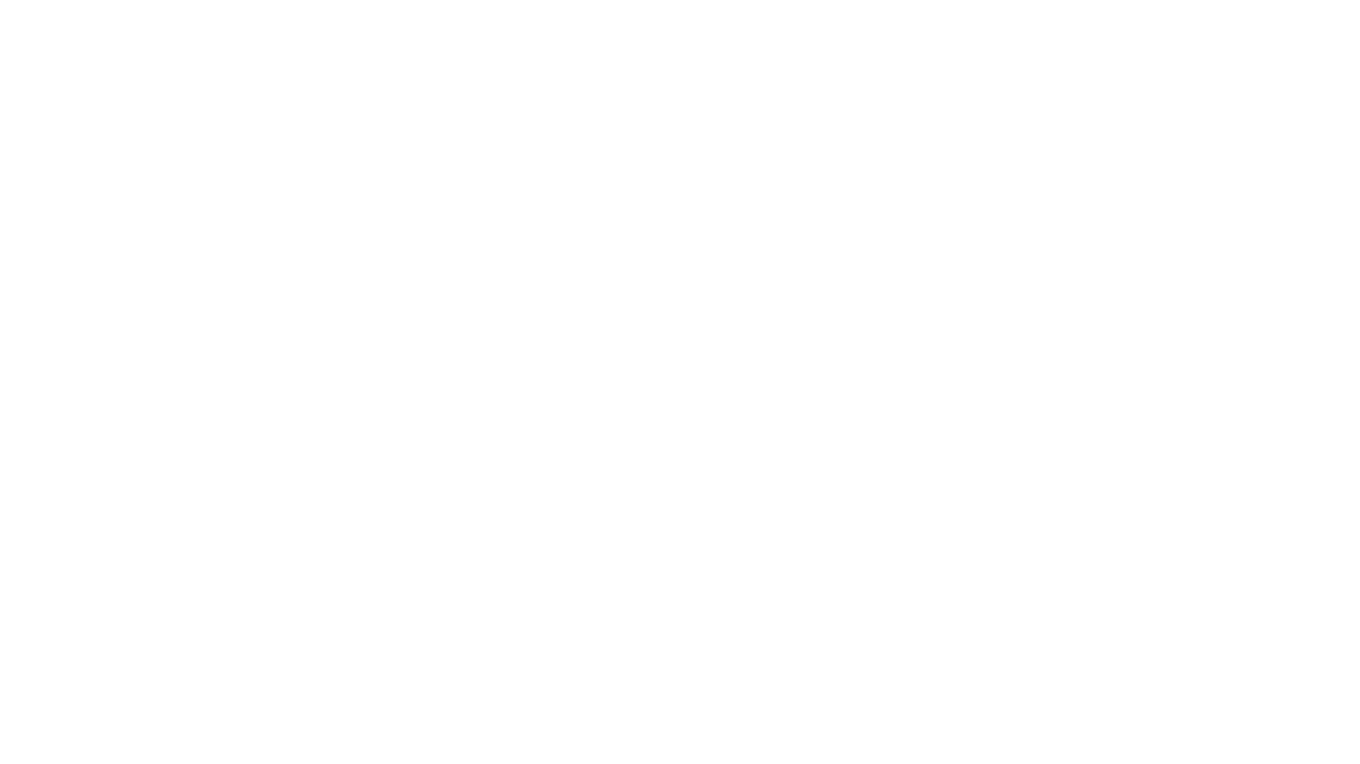
Chinese-Tiny-LLM is a repository containing procedures for cleaning Chinese web corpora and pre-training code. It introduces CT-LLM, a 2B parameter language model focused on the Chinese language. The model primarily uses Chinese data from a 1,200 billion token corpus, showing excellent performance in Chinese language tasks. The repository includes tools for filtering, deduplication, and pre-training, aiming to encourage further research and innovation in language model development.
README:
π Homepage | π€ MAP-CC | π€ CHC-Bench | π€ CT-LLM | π arXiv | GitHub
This repository contains our suite of procedures for cleaning Chinese web corpora and pre-training code.
π₯[2024/5/10]: The pretraining code has been released, and we also invite you to follow Neo. π
We introduce CT-LLM, a 2B parameter language model, marking a shift towards focusing on the Chinese language for LLM development. Starting from scratch, CT-LLM primarily uses Chinese data from a 1,200 billion token corpus, including 800 billion Chinese, 300 billion English, and 100 billion code tokens. This mix enhances its Chinese processing abilities, further improved by alignment techniques. CT-LLM shows excellent performance in Chinese language tasks on the CHC-Bench and is also adept in English through SFT. This approach challenges the norm of relying on English corpora for LLM training, expanding training methodologies. By open-sourcing CT-LLM's training process, including data processing and the Massive Appropriate Pretraining Chinese Corpus (MAP-CC), and introducing the Chinese Hard Case Benchmark (CHC-Bench), we encourage further research and innovation, aiming for more inclusive and adaptable language models.
-
MAP-CC An open-source Chinese pretraining dataset with a scale of 800 billion tokens, along with a detailed suite of procedures for cleaning Chinese web corpora, offering the NLP community high-quality Chinese pretraining data and an effective methodology for data preparation.
-
CHC-Bench A well-chosen multidisciplinary Chinese hard cases instruction understanding and following benchmark.
-
CT-LLM The first Chinese-centric large language model, both pre-training and fine-tuned primarily on Chinese corpora, offers significant insights into potential biases, Chinese language ability, and multilingual adaptability.
You first need to download fasttext model in the filter directory. The download link. The command example is
wget -P filter/ https://dl.fbaipublicfiles.com/fasttext/supervised-models/lid.176.bin
Once you download the model bin of fasttext, then you use command like follows to filter text.
python3 filter/filter.py --input_data input_dir --output_dir output_dir --success_dir success_dir --log_dir log_dir --worker num_worker
where input_dir is the direcotry containing jsonl files, output_dir is the directory to store the filtered jsonl files, success_dir is dirtory to save files about whether files are processed successfully, log_dir is the directory to save logs and num_worker is the total number of process to execute the job in parallel.
Here is an example command to run full text deduplication
python3 deduplication/full_text_dedup/full_text_dedup.py --input_dir input_dir --output_dir output_dir --content_field_name content_field_name
where input_dir is the directory to story the jsonl files, output_dir is the dirtory to save the deduplicated jsonl file and content_field_name is the field name of content in jsonl file.
To reduce the total processing time, you can partition jsonl files to multiple partitions, and then run multiple job for each partition in parallel.
Here is an example command to run Generate Minhash
python3 deduplication/minhash_lsh/generate_minhash.py --input_dir input_dir --output_dir output_dir --workers num_workers --content_field_name content_field_name
where input_dir is dirtory to save jsonl files, output_dir is the directory to save minhash value, num_worker is the total number of processes executing the job and content_field_name is the field name of content in jsonl file.
Here is an example command to run Gereate Duplicate Pairs
python3 deduplication/minhash_lsh/generate_dup_pairs.py --input_dir input_dir --output_dir output_dir
where input_dir is the directory to storay the minhash values, which is the same with the output_dir in Generate Minhash. Output_dir is the directory to save the duplicate paris files.
Here is an example command to run Genereate Connected Componenets
python3 deduplication/minhash_lsh/generate_connected_components.py --input_dir input_dir --output_file output_file --num_workers num_workers
where input_dir is the directory containing the duplicate lines file which is the same as the output_dir in Generate Duplicate Pairs. Output_file is file path to save information about connected components. Num_workers is the total number of processes executing the job.
Here is an example command to run Genereate Connected Componenets
python3 deduplication/minhash_lsh/generate_dup_line_id_for_each_file.py --input_file input_file --output_dir output_dir
where input_file is the file containing nformation about connected components which is the same as output_file in Genereate Connected Componenets. Output_dir is the directory to save duplicate line id information for each file.
Here is an example command to run Remove Duplicates
python3 deduplication/minhash_lsh/remove_dup.py --input_dir input_dir --output_dir output_dir --dup_line_id_dir dup_line_id_dir --worker num_worker
where input_dir is the directory containing the original jsonl files which is the same as the input_dir in Generate Minhash. Output_dir is the directory to save the dedupliacted jsonl files. Dup_line_id_dir is the directory containing duplicate line ids of all jsonl files which is the same as output_dir in Generate Duplicated Line ID for Each File.
Here is an example command to run Remove Duplicates
python3 deduplication/simlar_line_dedup/similar_line_dedup.py --input_dir input_dir --output_dir output_dir --wokers num_worker --content_field_name content_field_name
where input_dir is the directory containing the jsonl files, output_dir is the direcotry to save deduplicated jsonl files, num_worker is the total number of processes executing the job and content_field_name is the field name of content in jsonl file.
For detailed information and access to the pre-training code used in this project, please refer to Neo. This repo contains all the necessary code and configurations needed to replicate the pre-training process.
Welcome to follow Neo.
This model, developed for academic purposes, employs rigorously compliance-checked training data to uphold the highest standards of integrity and compliance. Despite our efforts, the inherent complexities of data and the broad spectrum of model applications prevent us from ensuring absolute accuracy or appropriateness of the model outputs in every scenario.
It is essential to highlight that our model and its associated training data are intended solely for scholarly research. We explicitly disclaim any liability for problems that may arise from improper use, interpretation errors, unlawful activities, the dissemination of false information, or any data security issues related to the utilization of our model or its training data.
We strongly encourage users to report any concerns related to data misuse, security breaches, or potential infringement issues directly to us for immediate investigation and resolution.
Contact: [email protected]; [email protected]
Our commitment to responsible data sharing and the security of our academic tools is paramount. We thank you for your cooperation in maintaining the ethical use of this technology.
The MAP-CC Dataset is made available under the terms of the Creative Commons Attribution-NonCommercial-NoDerivatives 4.0 International License (CC BY-NC-ND 4.0).
By using the MAP-CC Dataset, you accept and agree to be bound by the terms and conditions of the CC BY-NC-ND 4.0 License. This license allows users to share (copy and redistribute the material in any medium or format) the MAP-CC Dataset for non-commercial purposes only, and with no modifications or derivatives, as long as proper attribution is given to the creators. For further details, please refer to the LICENSE file.
We chose the CC BY-NC-ND 4.0 License for the MAP-CC Dataset to facilitate academic and educational use, promoting the spread of knowledge while protecting the work of the creators from unauthorized commercial use or modification.
@misc{du2024chinese,
title={Chinese Tiny LLM: Pretraining a Chinese-Centric Large Language Model},
author={Xinrun Du and Zhouliang Yu and Songyang Gao and Ding Pan and Yuyang Cheng and Ziyang Ma and Ruibin Yuan and Xingwei Qu and Jiaheng Liu and Tianyu Zheng and Xinchen Luo and Guorui Zhou and Binhang Yuan and Wenhu Chen and Jie Fu and Ge Zhang},
year={2024},
eprint={2404.04167},
archivePrefix={arXiv},
primaryClass={cs.CL}
}
For Tasks:
Click tags to check more tools for each tasksFor Jobs:
Alternative AI tools for Chinese-Tiny-LLM
Similar Open Source Tools
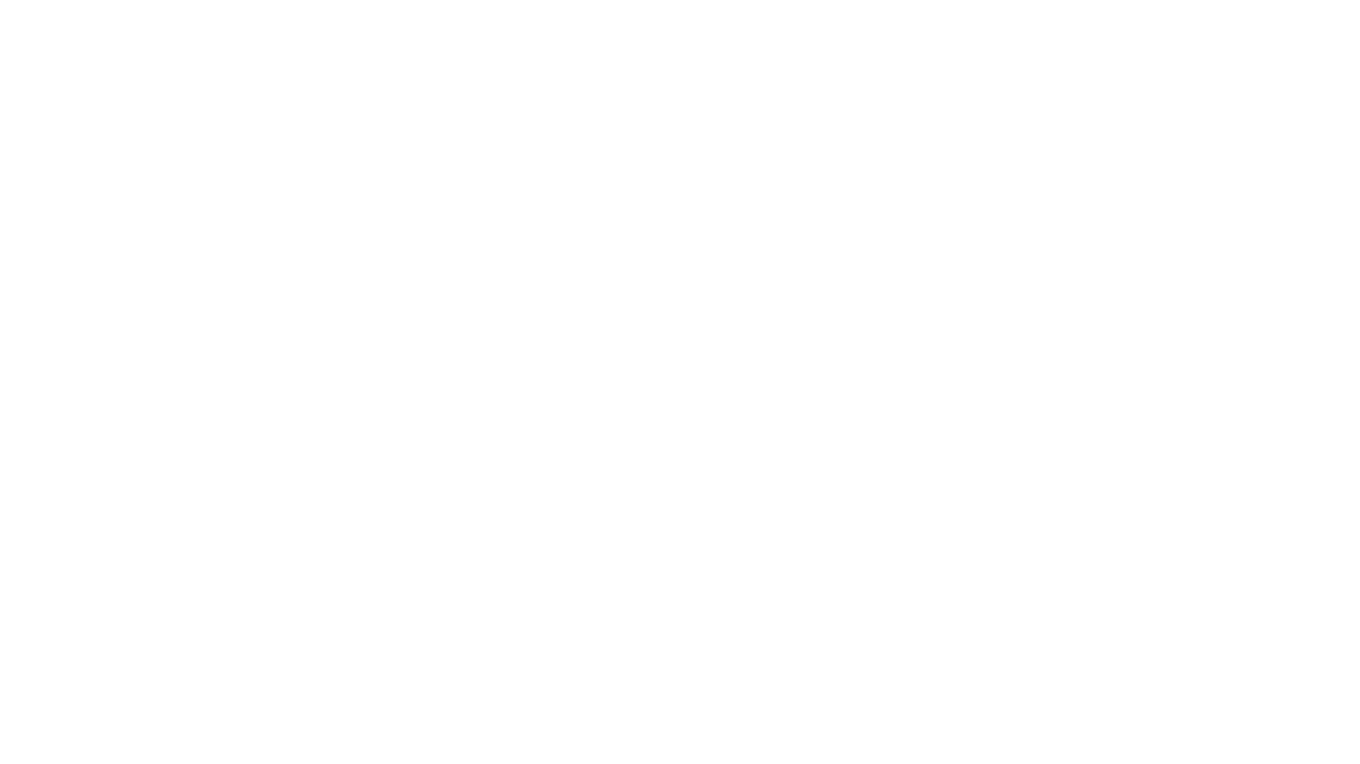
Chinese-Tiny-LLM
Chinese-Tiny-LLM is a repository containing procedures for cleaning Chinese web corpora and pre-training code. It introduces CT-LLM, a 2B parameter language model focused on the Chinese language. The model primarily uses Chinese data from a 1,200 billion token corpus, showing excellent performance in Chinese language tasks. The repository includes tools for filtering, deduplication, and pre-training, aiming to encourage further research and innovation in language model development.
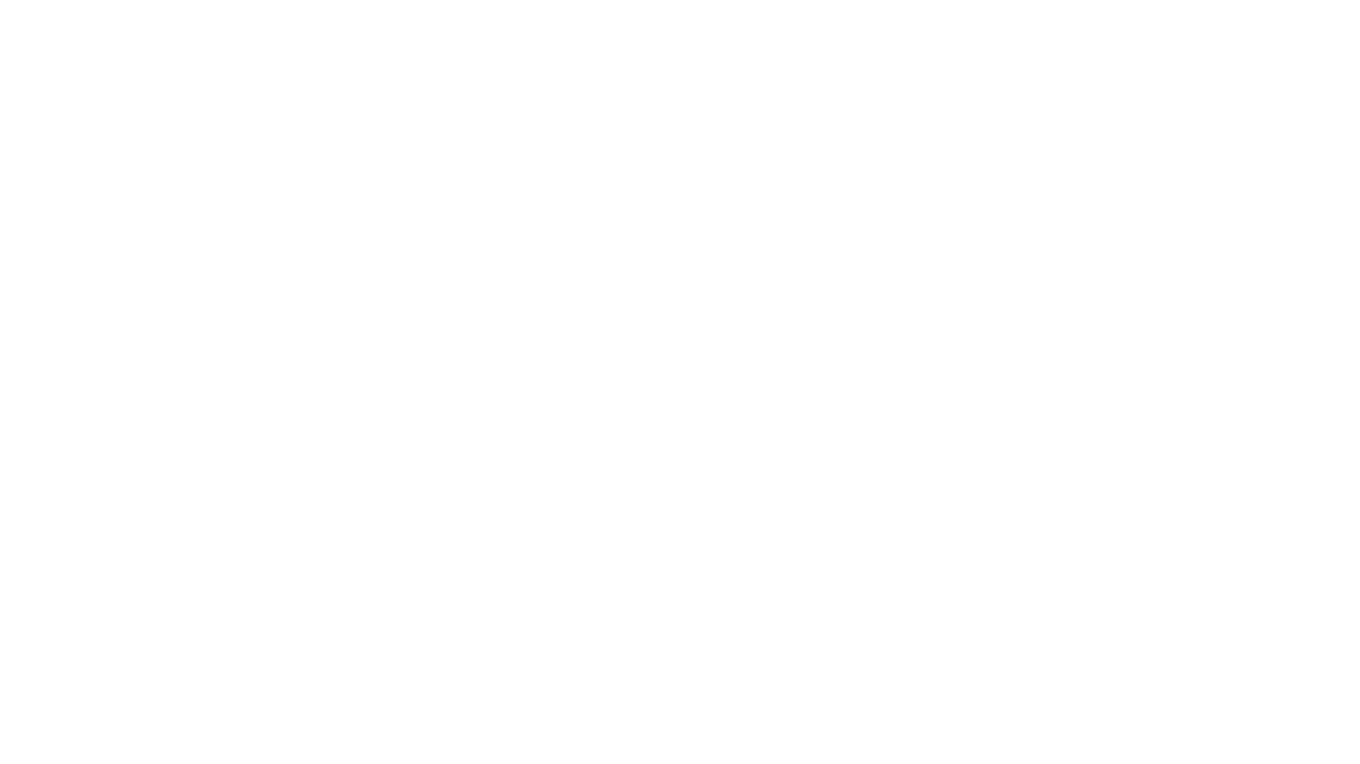
Me-LLaMA
Me LLaMA introduces a suite of open-source medical Large Language Models (LLMs), including Me LLaMA 13B/70B and their chat-enhanced versions. Developed through innovative continual pre-training and instruction tuning, these models leverage a vast medical corpus comprising PubMed papers, medical guidelines, and general domain data. Me LLaMA sets new benchmarks on medical reasoning tasks, making it a significant asset for medical NLP applications and research. The models are intended for computational linguistics and medical research, not for clinical decision-making without validation and regulatory approval.
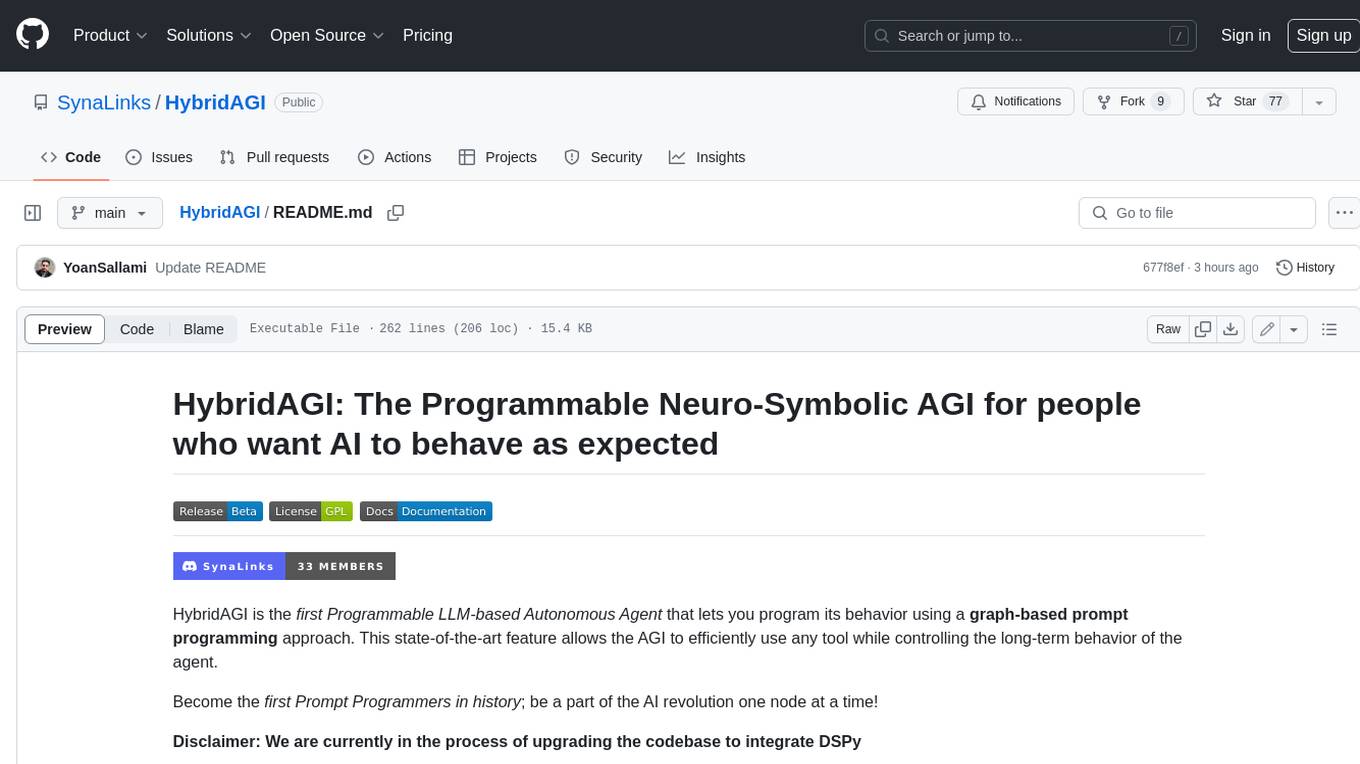
HybridAGI
HybridAGI is the first Programmable LLM-based Autonomous Agent that lets you program its behavior using a **graph-based prompt programming** approach. This state-of-the-art feature allows the AGI to efficiently use any tool while controlling the long-term behavior of the agent. Become the _first Prompt Programmers in history_ ; be a part of the AI revolution one node at a time! **Disclaimer: We are currently in the process of upgrading the codebase to integrate DSPy**
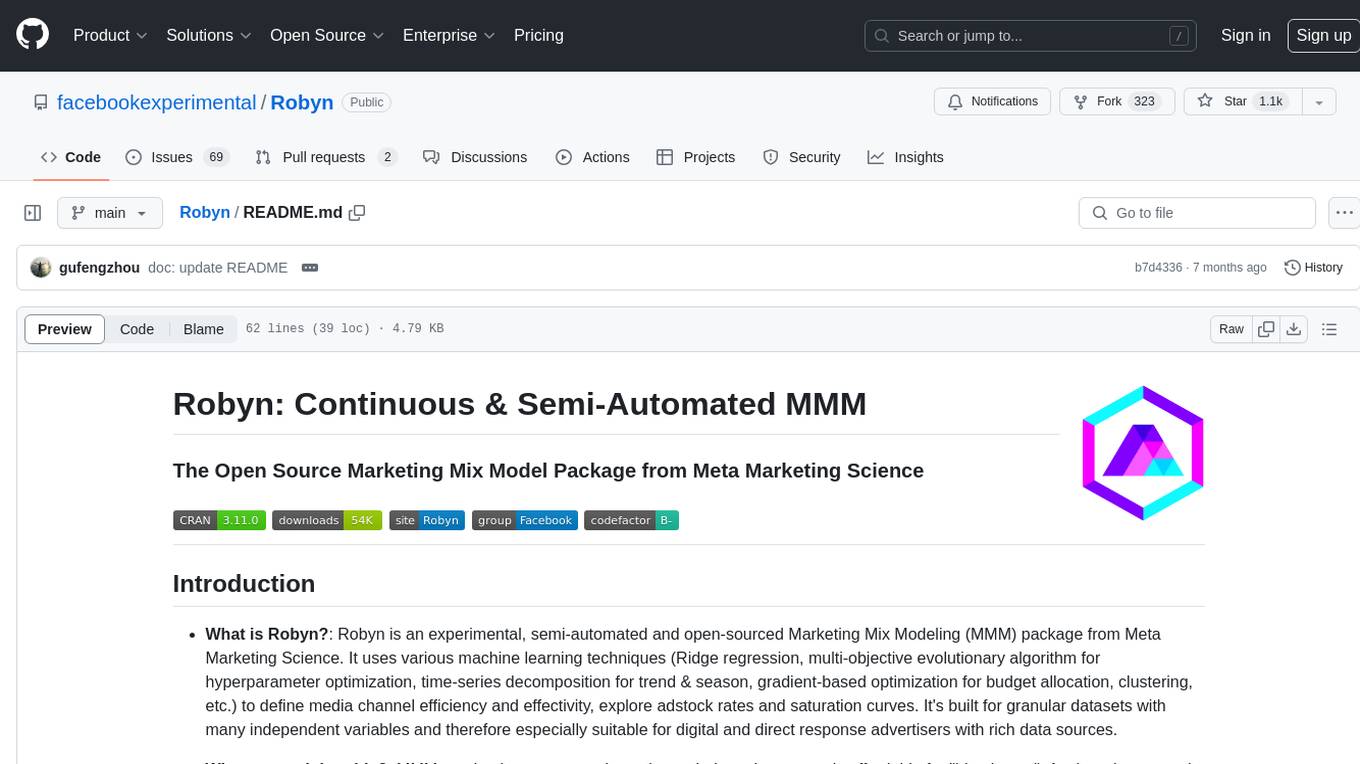
Robyn
Robyn is an experimental, semi-automated and open-sourced Marketing Mix Modeling (MMM) package from Meta Marketing Science. It uses various machine learning techniques to define media channel efficiency and effectivity, explore adstock rates and saturation curves. Built for granular datasets with many independent variables, especially suitable for digital and direct response advertisers with rich data sources. Aiming to democratize MMM, make it accessible for advertisers of all sizes, and contribute to the measurement landscape.
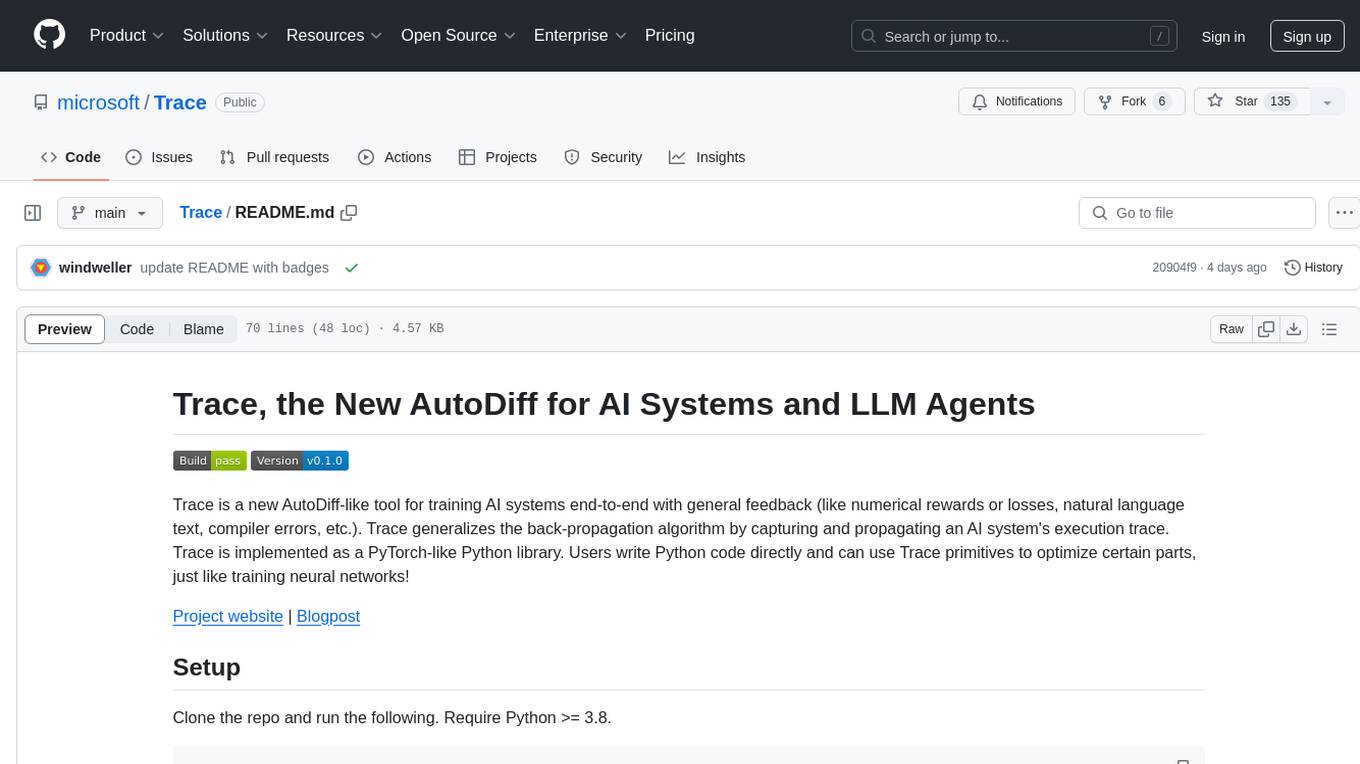
Trace
Trace is a new AutoDiff-like tool for training AI systems end-to-end with general feedback. It generalizes the back-propagation algorithm by capturing and propagating an AI system's execution trace. Implemented as a PyTorch-like Python library, users can write Python code directly and use Trace primitives to optimize certain parts, similar to training neural networks.
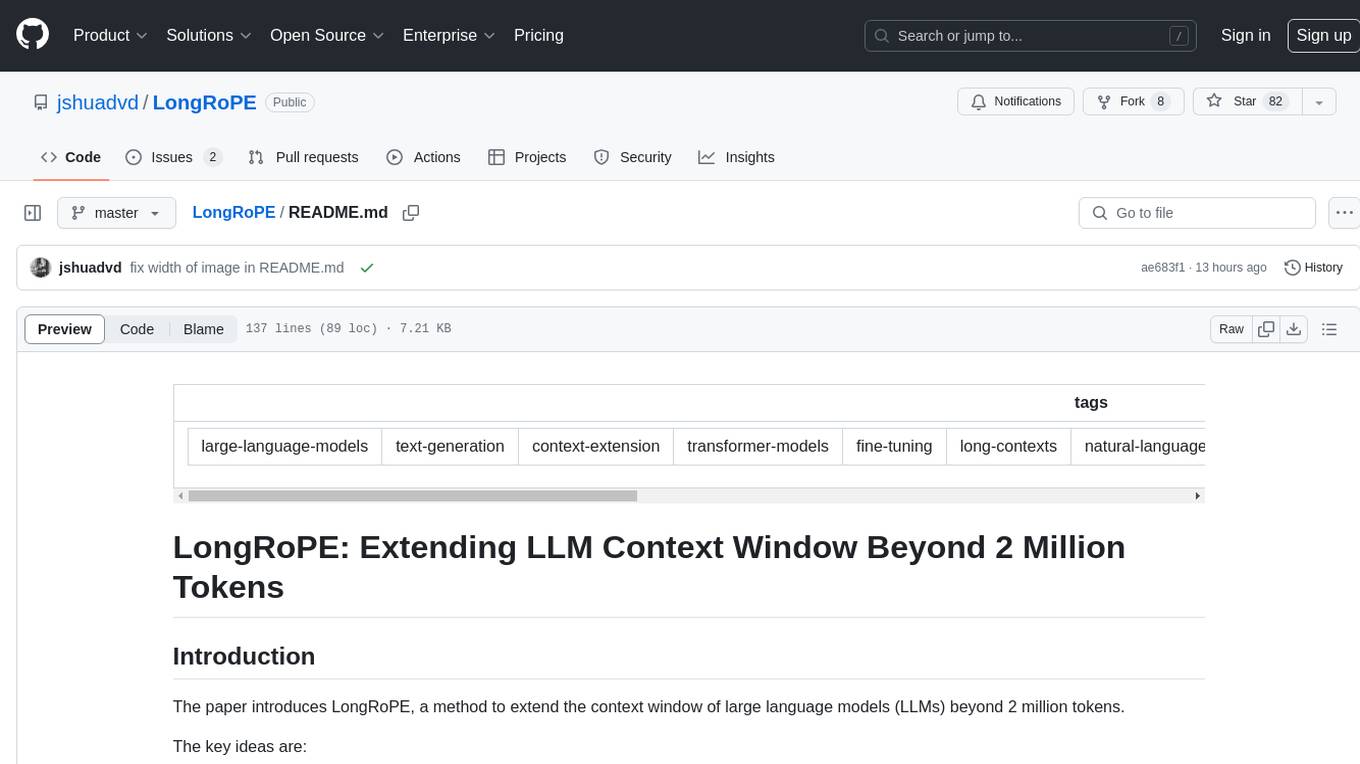
LongRoPE
LongRoPE is a method to extend the context window of large language models (LLMs) beyond 2 million tokens. It identifies and exploits non-uniformities in positional embeddings to enable 8x context extension without fine-tuning. The method utilizes a progressive extension strategy with 256k fine-tuning to reach a 2048k context. It adjusts embeddings for shorter contexts to maintain performance within the original window size. LongRoPE has been shown to be effective in maintaining performance across various tasks from 4k to 2048k context lengths.
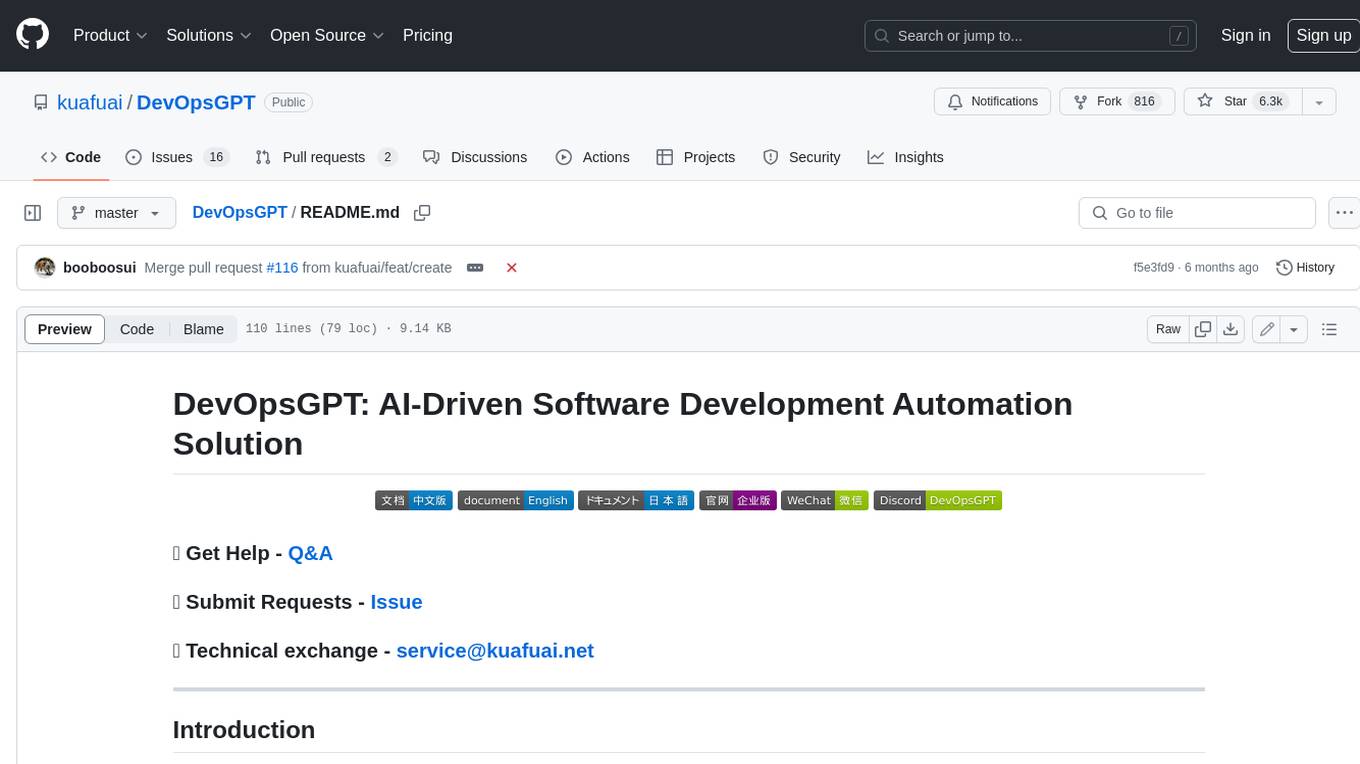
DevOpsGPT
DevOpsGPT is an AI-driven software development automation solution that combines Large Language Models (LLM) with DevOps tools to convert natural language requirements into working software. It improves development efficiency by eliminating the need for tedious requirement documentation, shortens development cycles, reduces communication costs, and ensures high-quality deliverables. The Enterprise Edition offers features like existing project analysis, professional model selection, and support for more DevOps platforms. The tool automates requirement development, generates interface documentation, provides pseudocode based on existing projects, facilitates code refinement, enables continuous integration, and supports software version release. Users can run DevOpsGPT with source code or Docker, and the tool comes with limitations in precise documentation generation and understanding existing project code. The product roadmap includes accurate requirement decomposition, rapid import of development requirements, and integration of more software engineering and professional tools for efficient software development tasks under AI planning and execution.
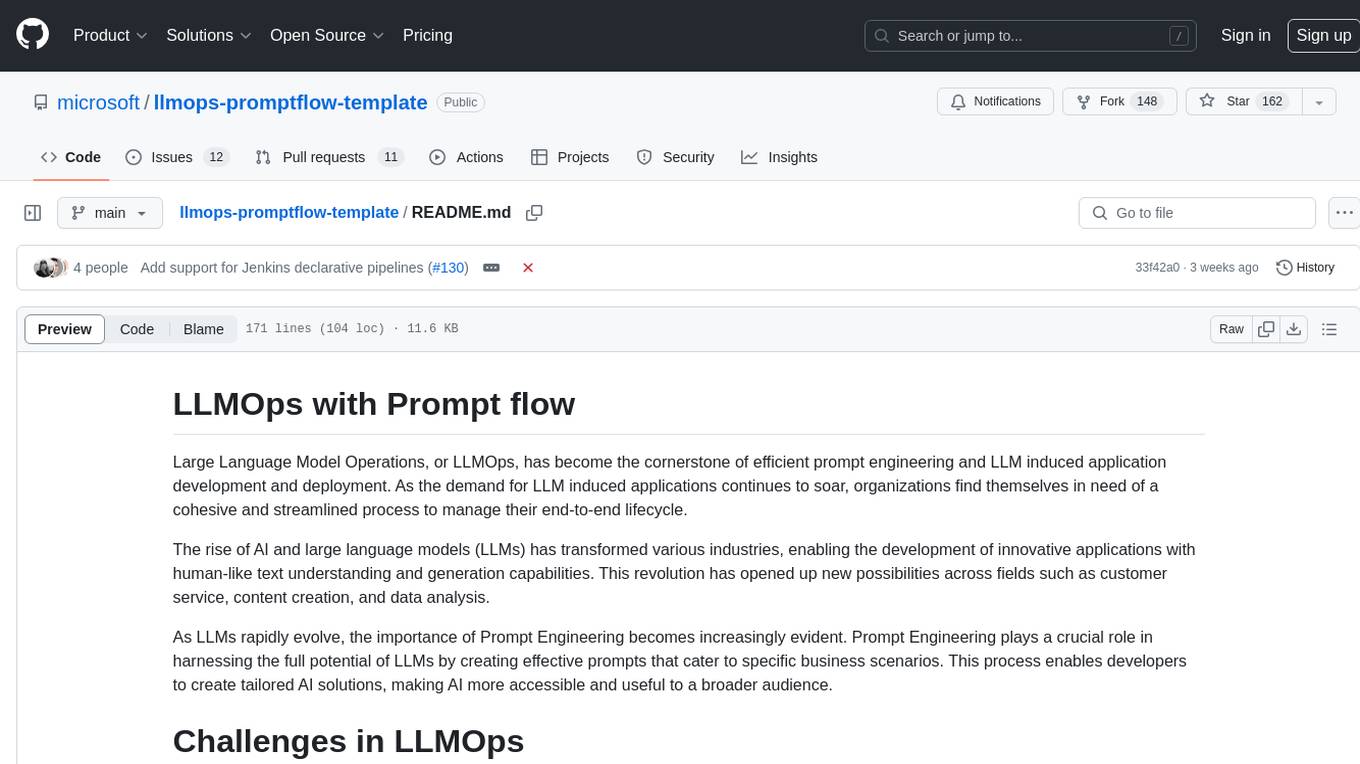
llmops-promptflow-template
LLMOps with Prompt flow is a template and guidance for building LLM-infused apps using Prompt flow. It provides centralized code hosting, lifecycle management, variant and hyperparameter experimentation, A/B deployment, many-to-many dataset/flow relationships, multiple deployment targets, comprehensive reporting, BYOF capabilities, configuration-based development, local prompt experimentation and evaluation, endpoint testing, and optional Human-in-loop validation. The tool is customizable to suit various application needs.
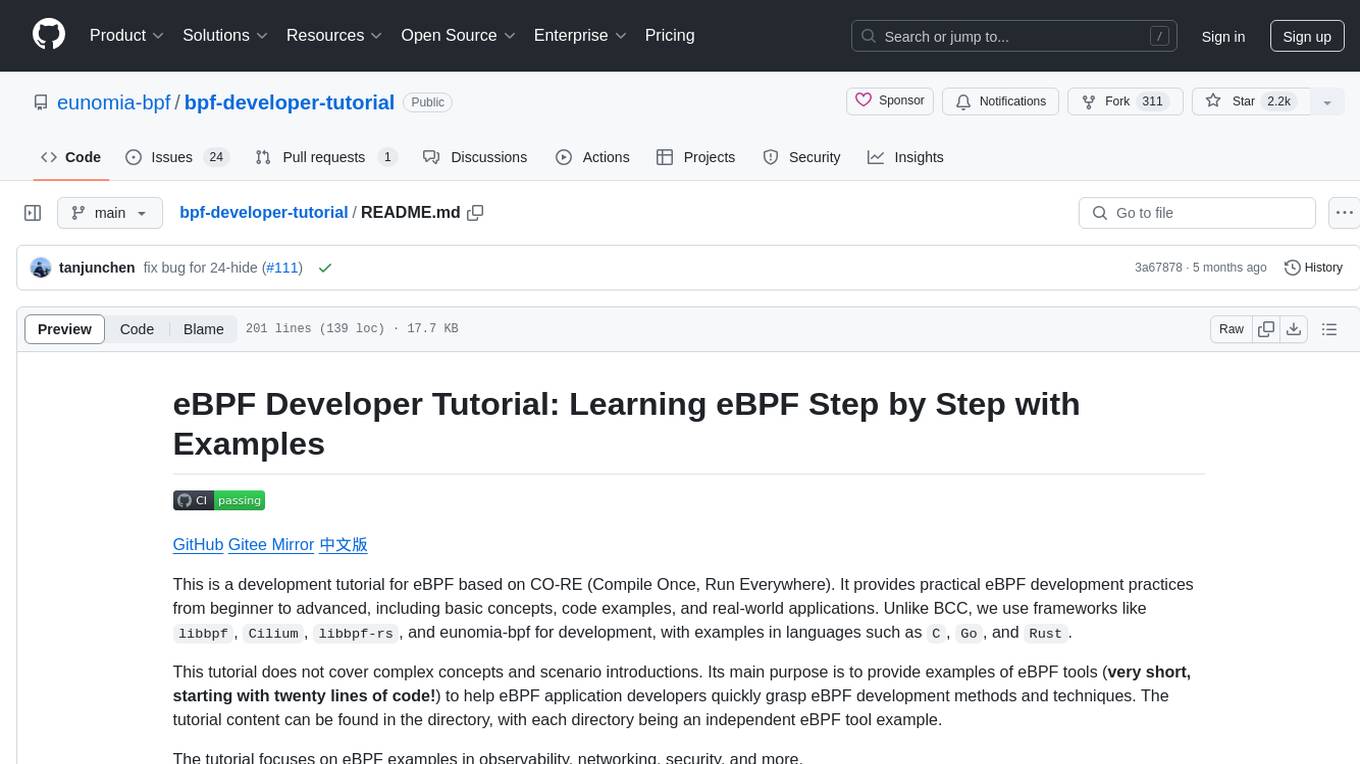
bpf-developer-tutorial
This is a development tutorial for eBPF based on CO-RE (Compile Once, Run Everywhere). It provides practical eBPF development practices from beginner to advanced, including basic concepts, code examples, and real-world applications. The tutorial focuses on eBPF examples in observability, networking, security, and more. It aims to help eBPF application developers quickly grasp eBPF development methods and techniques through examples in languages such as C, Go, and Rust. The tutorial is structured with independent eBPF tool examples in each directory, covering topics like kprobes, fentry, opensnoop, uprobe, sigsnoop, execsnoop, exitsnoop, runqlat, hardirqs, and more. The project is based on libbpf and frameworks like libbpf, Cilium, libbpf-rs, and eunomia-bpf for development.
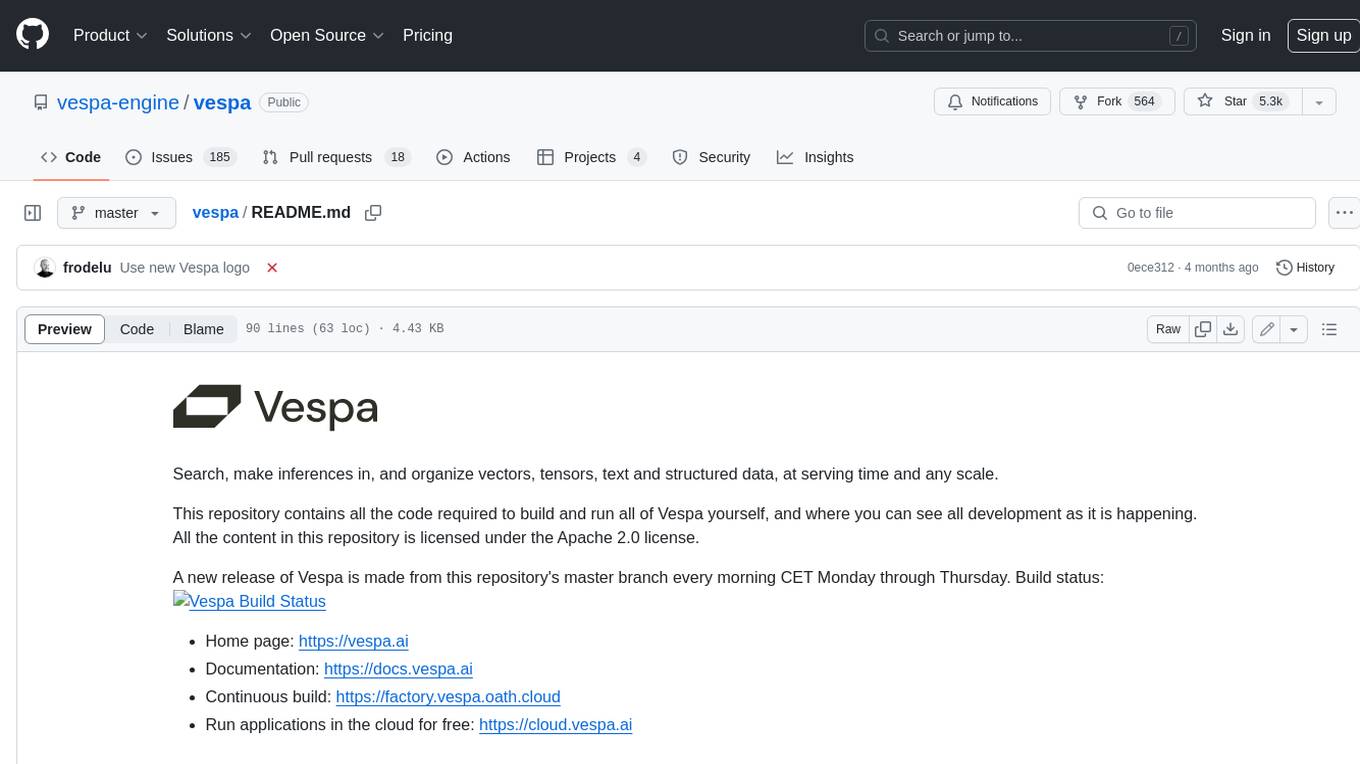
vespa
Vespa is a platform that performs operations such as selecting a subset of data in a large corpus, evaluating machine-learned models over the selected data, organizing and aggregating it, and returning it, typically in less than 100 milliseconds, all while the data corpus is continuously changing. It has been in development for many years and is used on a number of large internet services and apps which serve hundreds of thousands of queries from Vespa per second.
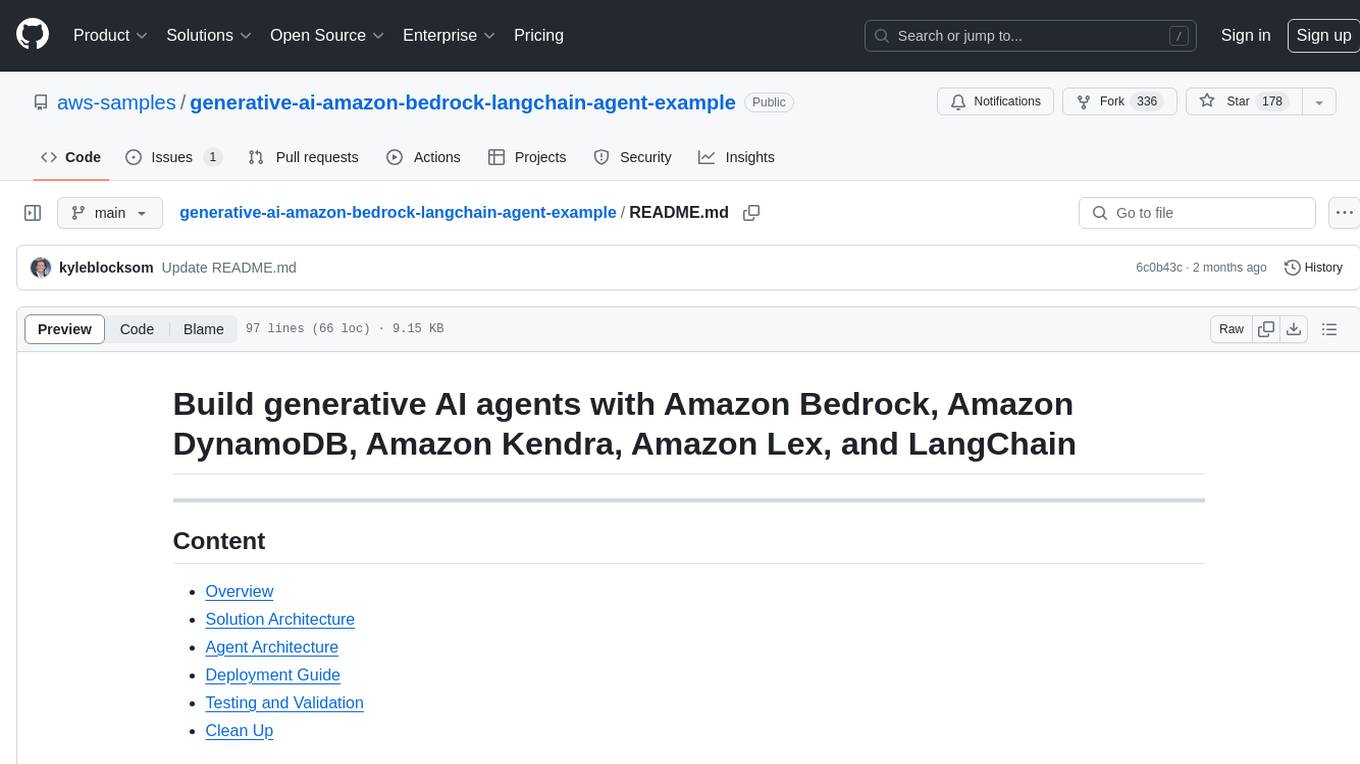
generative-ai-amazon-bedrock-langchain-agent-example
This repository provides a sample solution for building generative AI agents using Amazon Bedrock, Amazon DynamoDB, Amazon Kendra, Amazon Lex, and LangChain. The solution creates a generative AI financial services agent capable of assisting users with account information, loan applications, and answering natural language questions. It serves as a launchpad for developers to create personalized conversational agents for applications like chatbots and virtual assistants.
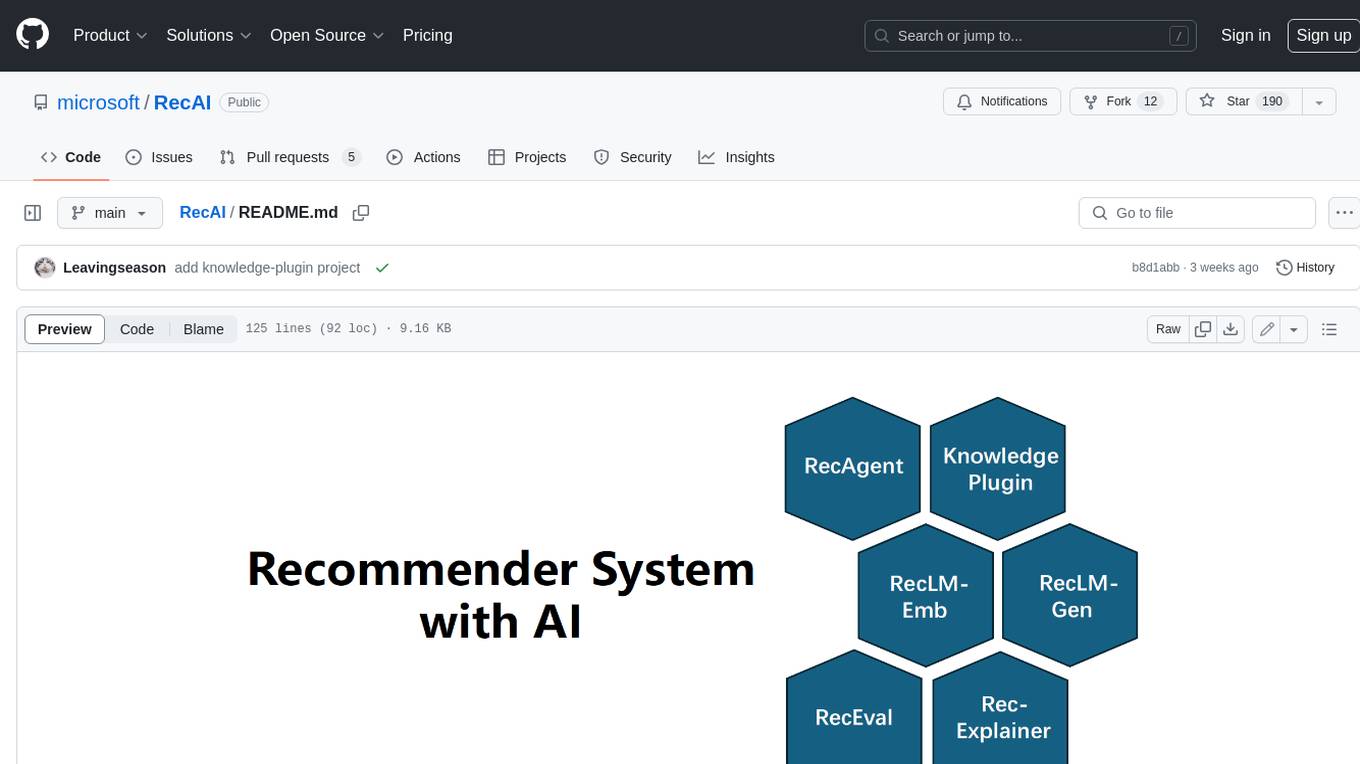
RecAI
RecAI is a project that explores the integration of Large Language Models (LLMs) into recommender systems, addressing the challenges of interactivity, explainability, and controllability. It aims to bridge the gap between general-purpose LLMs and domain-specific recommender systems, providing a holistic perspective on the practical requirements of LLM4Rec. The project investigates various techniques, including Recommender AI agents, selective knowledge injection, fine-tuning language models, evaluation, and LLMs as model explainers, to create more sophisticated, interactive, and user-centric recommender systems.
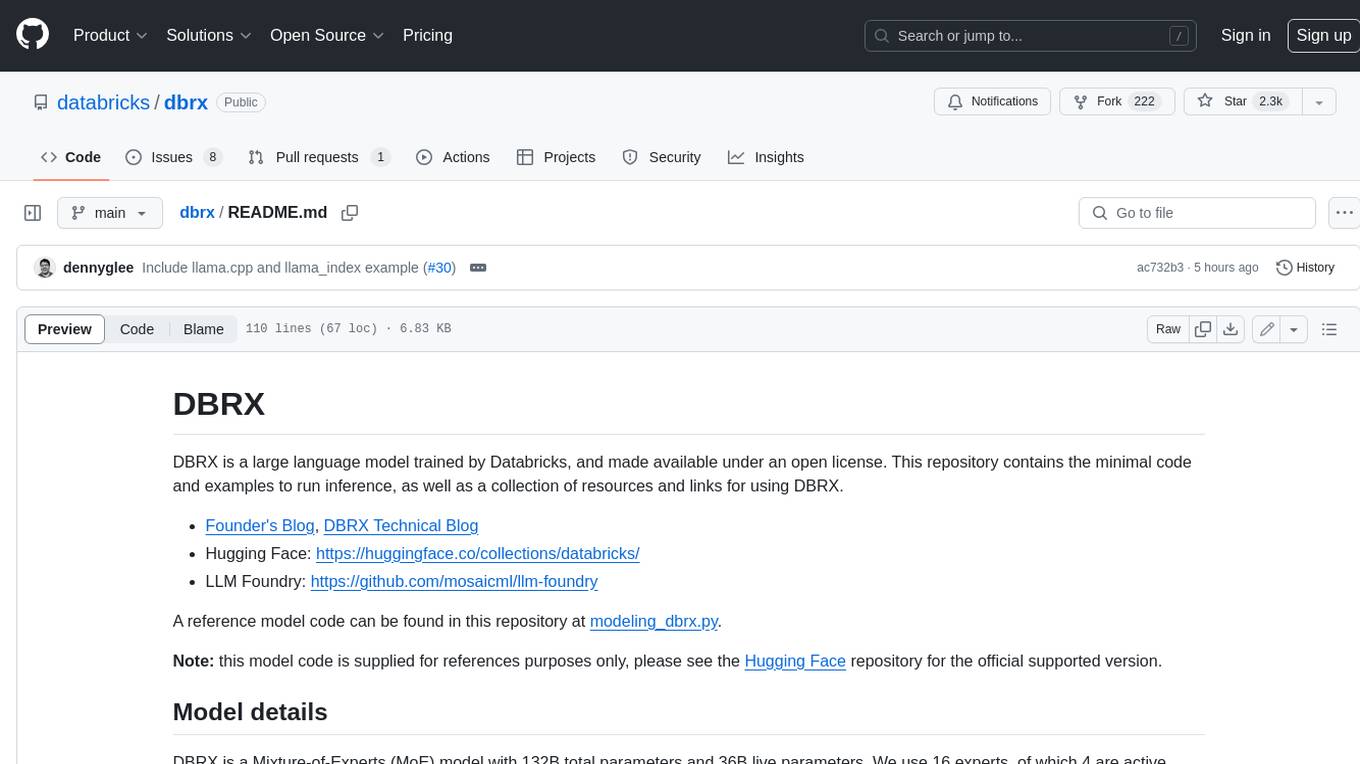
dbrx
DBRX is a large language model trained by Databricks and made available under an open license. It is a Mixture-of-Experts (MoE) model with 132B total parameters and 36B live parameters, using 16 experts, of which 4 are active during training or inference. DBRX was pre-trained for 12T tokens of text and has a context length of 32K tokens. The model is available in two versions: a base model and an Instruct model, which is finetuned for instruction following. DBRX can be used for a variety of tasks, including text generation, question answering, summarization, and translation.
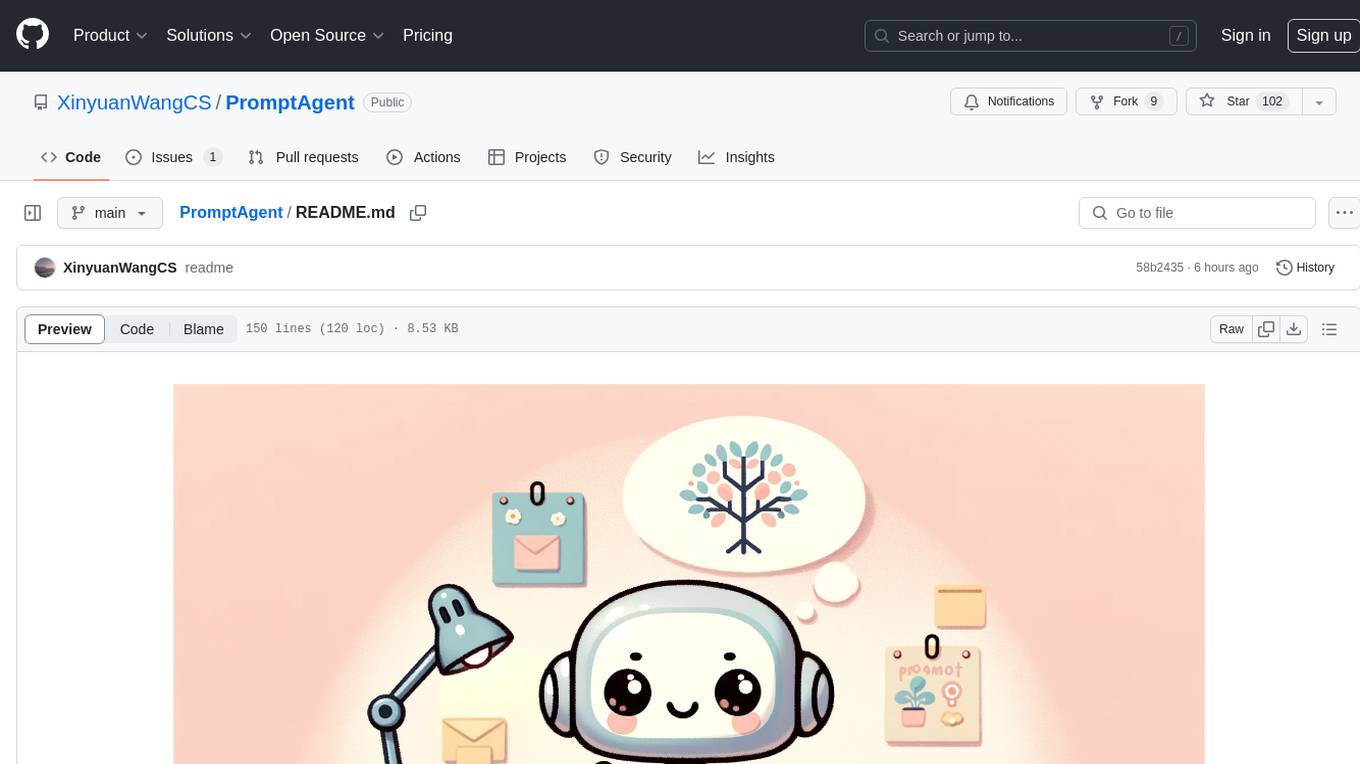
PromptAgent
PromptAgent is a repository for a novel automatic prompt optimization method that crafts expert-level prompts using language models. It provides a principled framework for prompt optimization by unifying prompt sampling and rewarding using MCTS algorithm. The tool supports different models like openai, palm, and huggingface models. Users can run PromptAgent to optimize prompts for specific tasks by strategically sampling model errors, generating error feedbacks, simulating future rewards, and searching for high-reward paths leading to expert prompts.
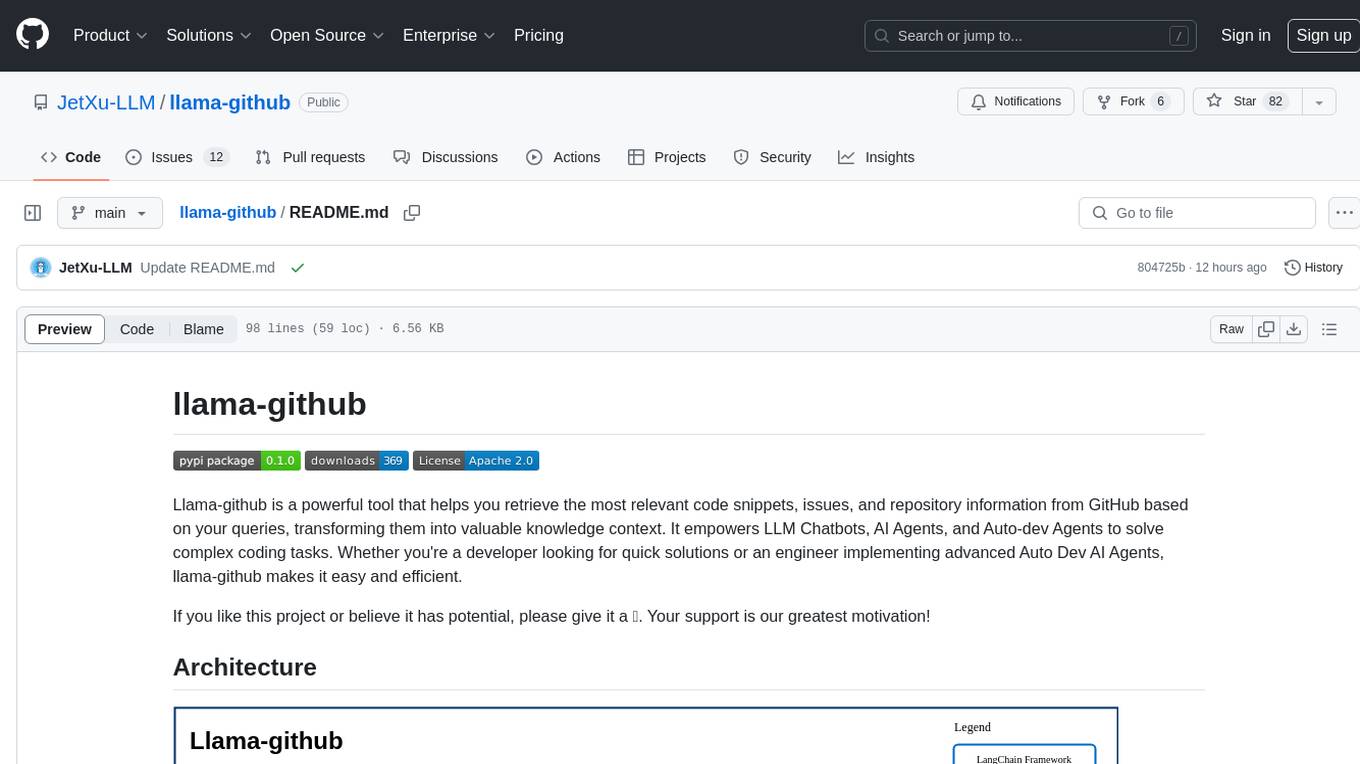
llama-github
Llama-github is a powerful tool that helps retrieve relevant code snippets, issues, and repository information from GitHub based on queries. It empowers AI agents and developers to solve coding tasks efficiently. With features like intelligent GitHub retrieval, repository pool caching, LLM-powered question analysis, and comprehensive context generation, llama-github excels at providing valuable knowledge context for development needs. It supports asynchronous processing, flexible LLM integration, robust authentication options, and logging/error handling for smooth operations and troubleshooting. The vision is to seamlessly integrate with GitHub for AI-driven development solutions, while the roadmap focuses on empowering LLMs to automatically resolve complex coding tasks.
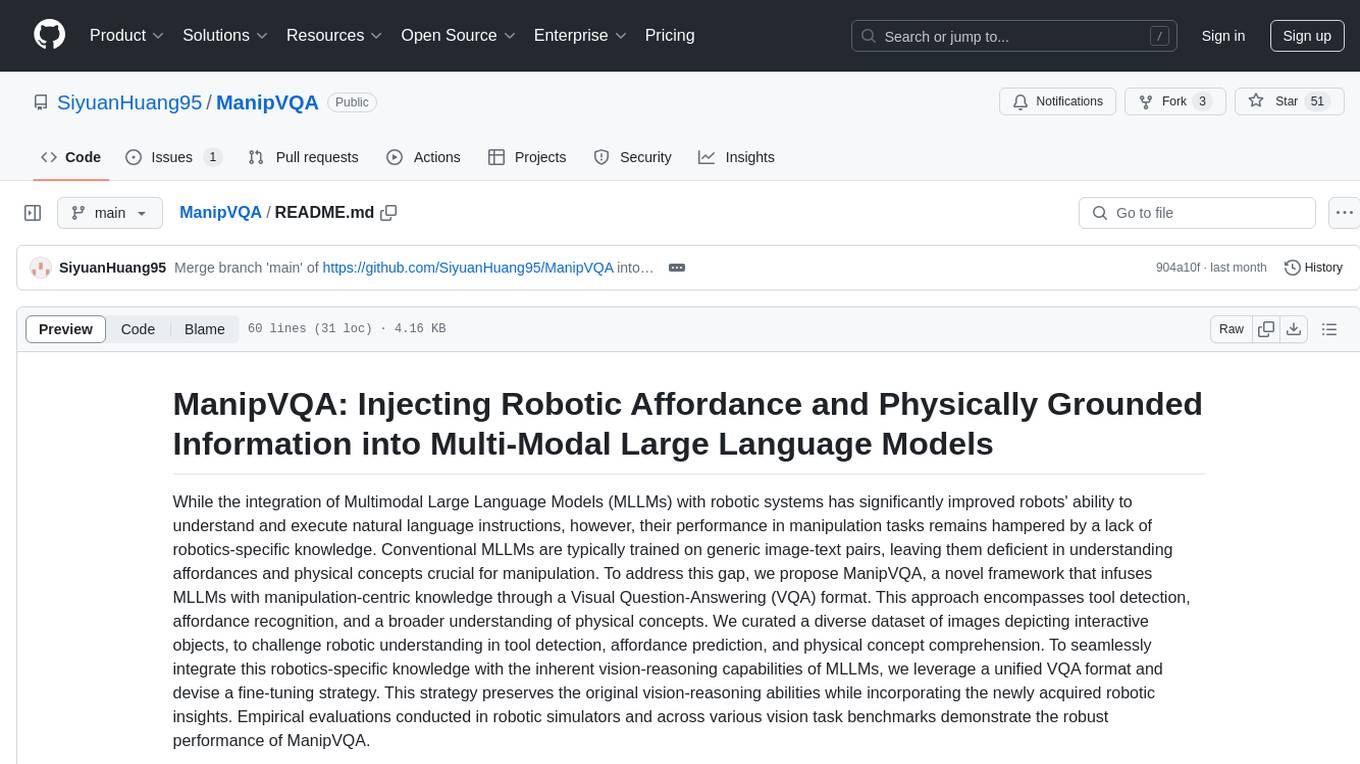
ManipVQA
ManipVQA is a framework that enhances Multimodal Large Language Models (MLLMs) with manipulation-centric knowledge through a Visual Question-Answering (VQA) format. It addresses the deficiency of conventional MLLMs in understanding affordances and physical concepts crucial for manipulation tasks. By infusing robotics-specific knowledge, including tool detection, affordance recognition, and physical concept comprehension, ManipVQA improves the performance of robots in manipulation tasks. The framework involves fine-tuning MLLMs with a curated dataset of interactive objects, enabling robots to understand and execute natural language instructions more effectively.
For similar tasks
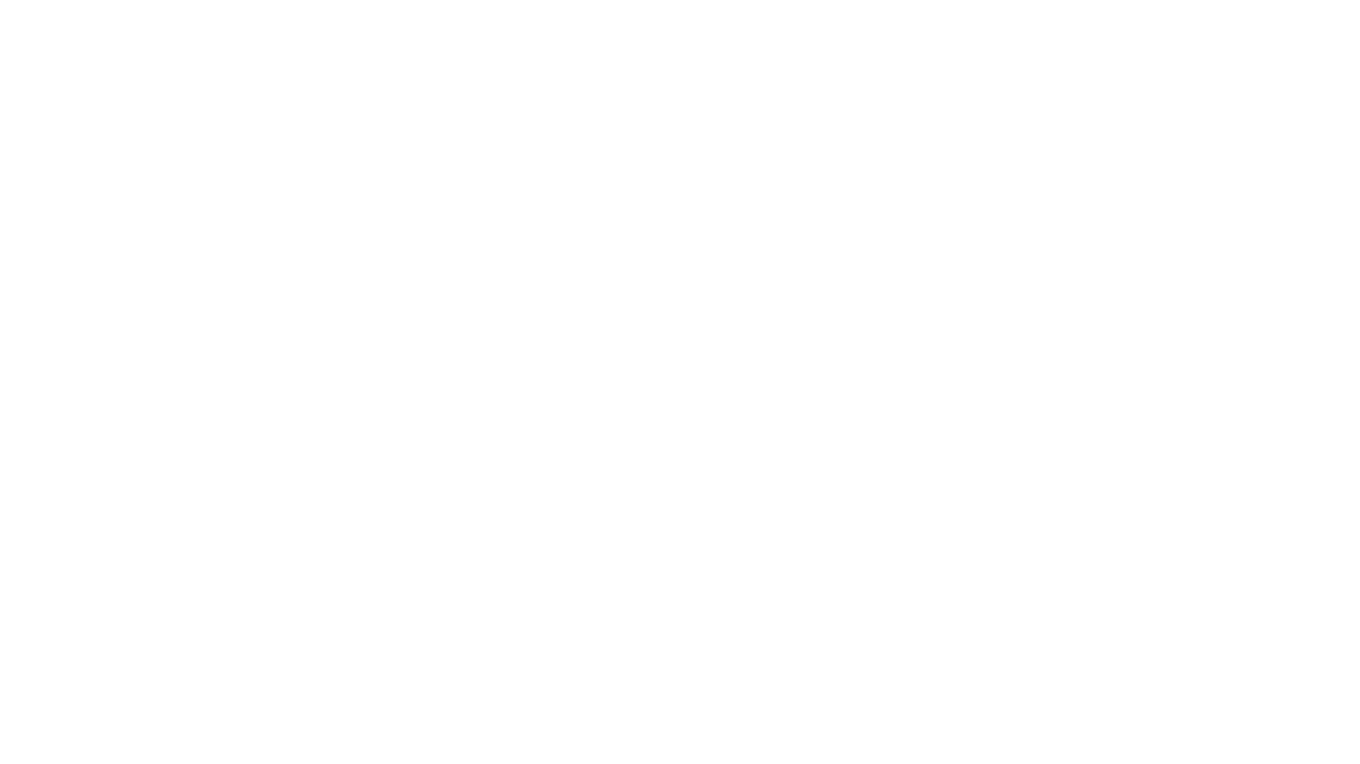
Chinese-Tiny-LLM
Chinese-Tiny-LLM is a repository containing procedures for cleaning Chinese web corpora and pre-training code. It introduces CT-LLM, a 2B parameter language model focused on the Chinese language. The model primarily uses Chinese data from a 1,200 billion token corpus, showing excellent performance in Chinese language tasks. The repository includes tools for filtering, deduplication, and pre-training, aiming to encourage further research and innovation in language model development.
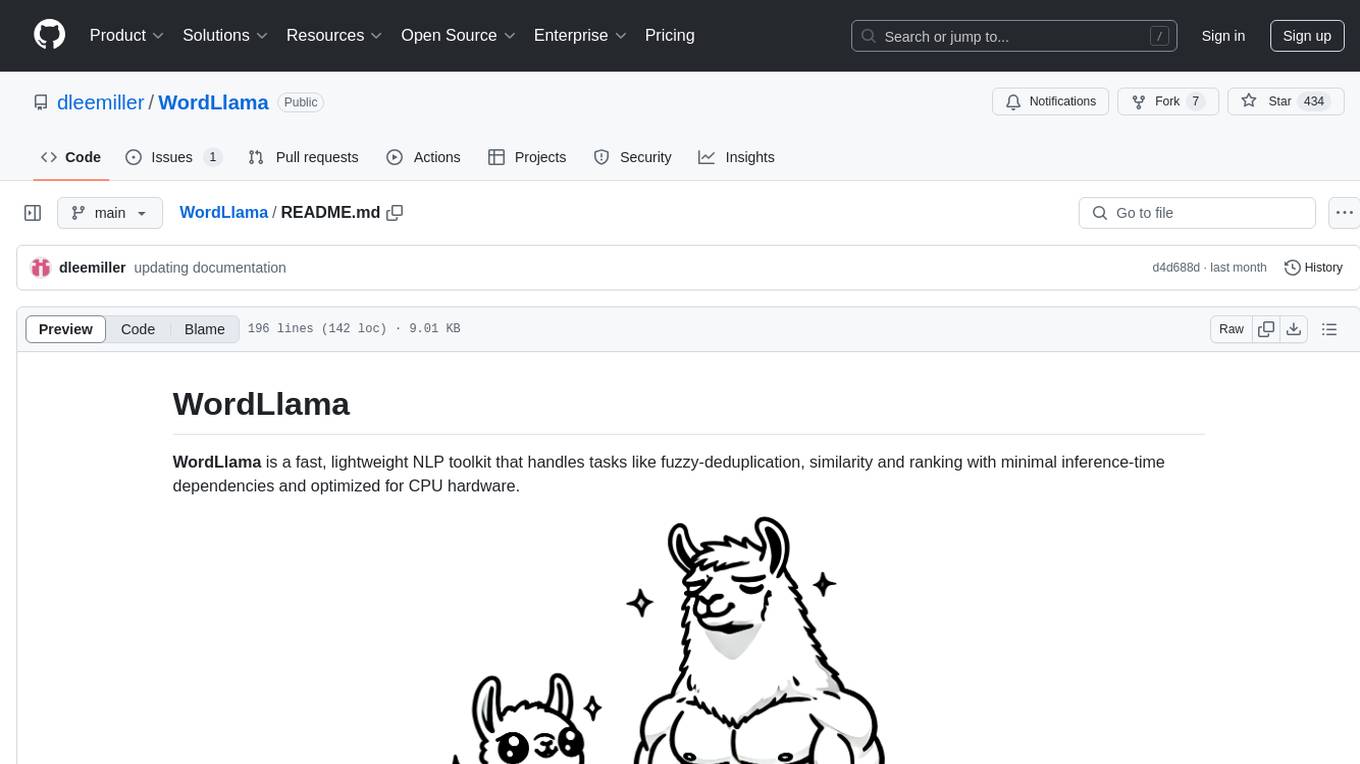
WordLlama
WordLlama is a fast, lightweight NLP toolkit optimized for CPU hardware. It recycles components from large language models to create efficient word representations. It offers features like Matryoshka Representations, low resource requirements, binarization, and numpy-only inference. The tool is suitable for tasks like semantic matching, fuzzy deduplication, ranking, and clustering, making it a good option for NLP-lite tasks and exploratory analysis.
For similar jobs
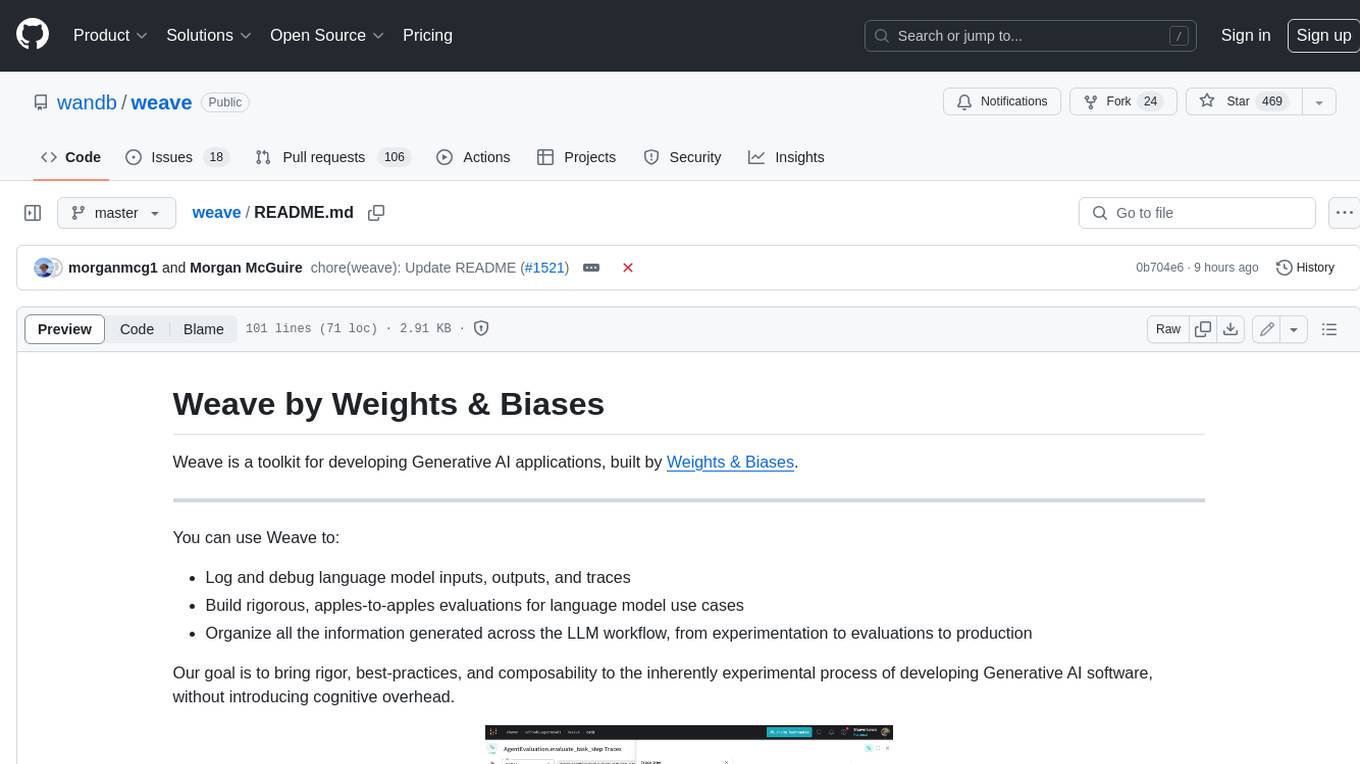
weave
Weave is a toolkit for developing Generative AI applications, built by Weights & Biases. With Weave, you can log and debug language model inputs, outputs, and traces; build rigorous, apples-to-apples evaluations for language model use cases; and organize all the information generated across the LLM workflow, from experimentation to evaluations to production. Weave aims to bring rigor, best-practices, and composability to the inherently experimental process of developing Generative AI software, without introducing cognitive overhead.
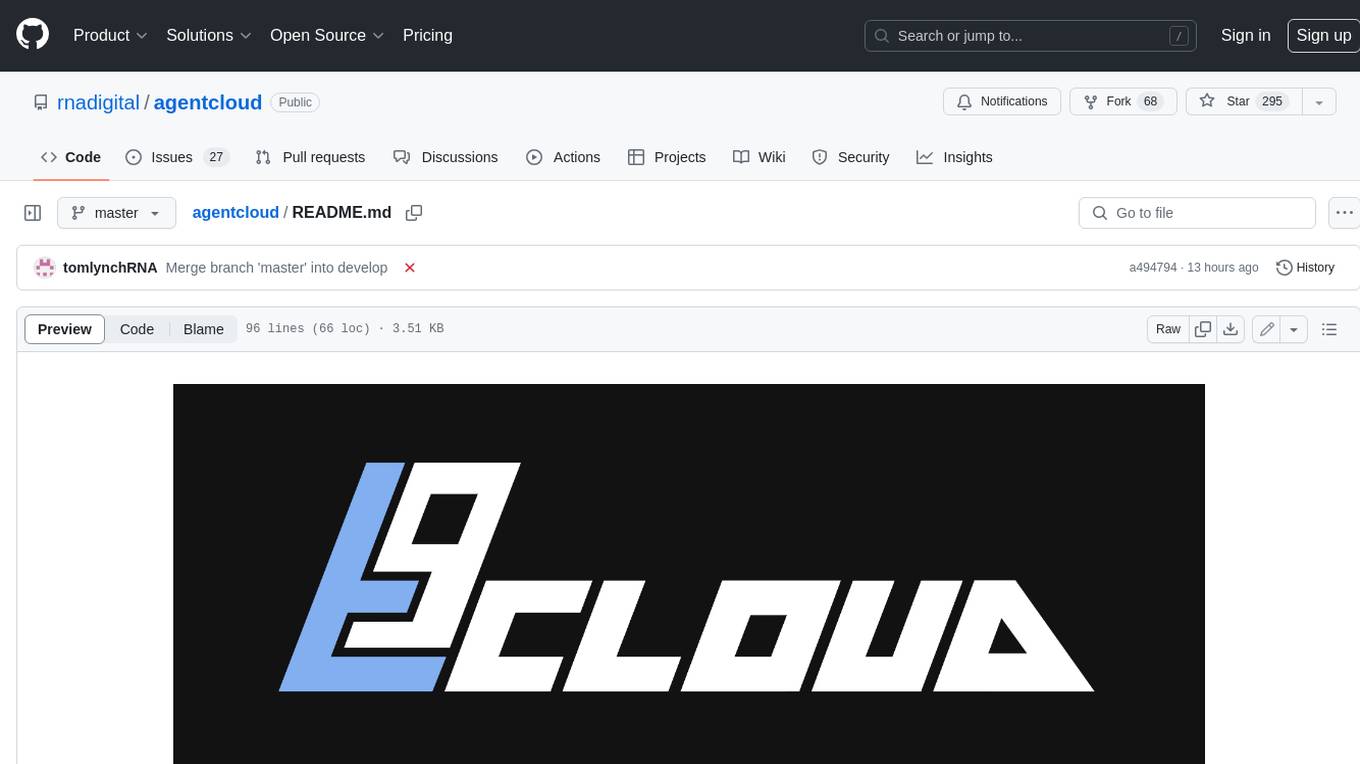
agentcloud
AgentCloud is an open-source platform that enables companies to build and deploy private LLM chat apps, empowering teams to securely interact with their data. It comprises three main components: Agent Backend, Webapp, and Vector Proxy. To run this project locally, clone the repository, install Docker, and start the services. The project is licensed under the GNU Affero General Public License, version 3 only. Contributions and feedback are welcome from the community.
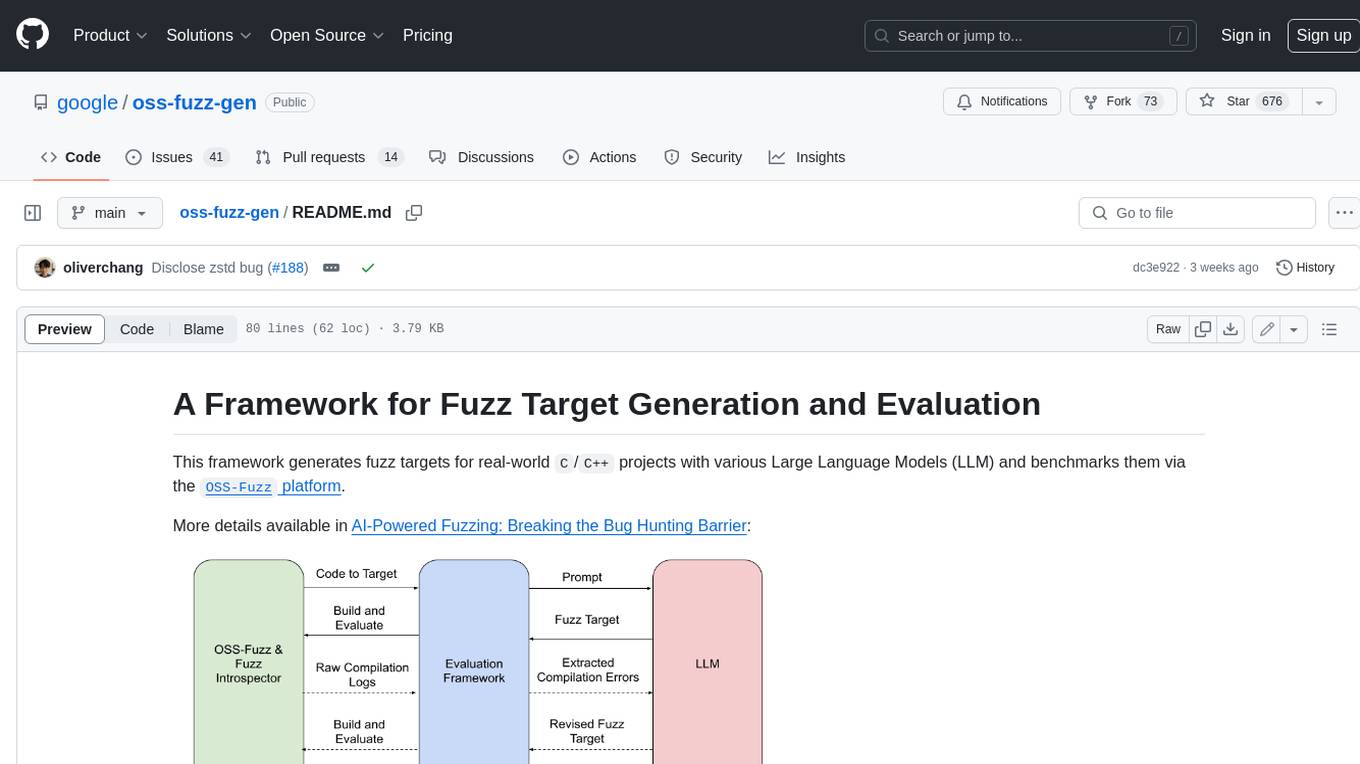
oss-fuzz-gen
This framework generates fuzz targets for real-world `C`/`C++` projects with various Large Language Models (LLM) and benchmarks them via the `OSS-Fuzz` platform. It manages to successfully leverage LLMs to generate valid fuzz targets (which generate non-zero coverage increase) for 160 C/C++ projects. The maximum line coverage increase is 29% from the existing human-written targets.
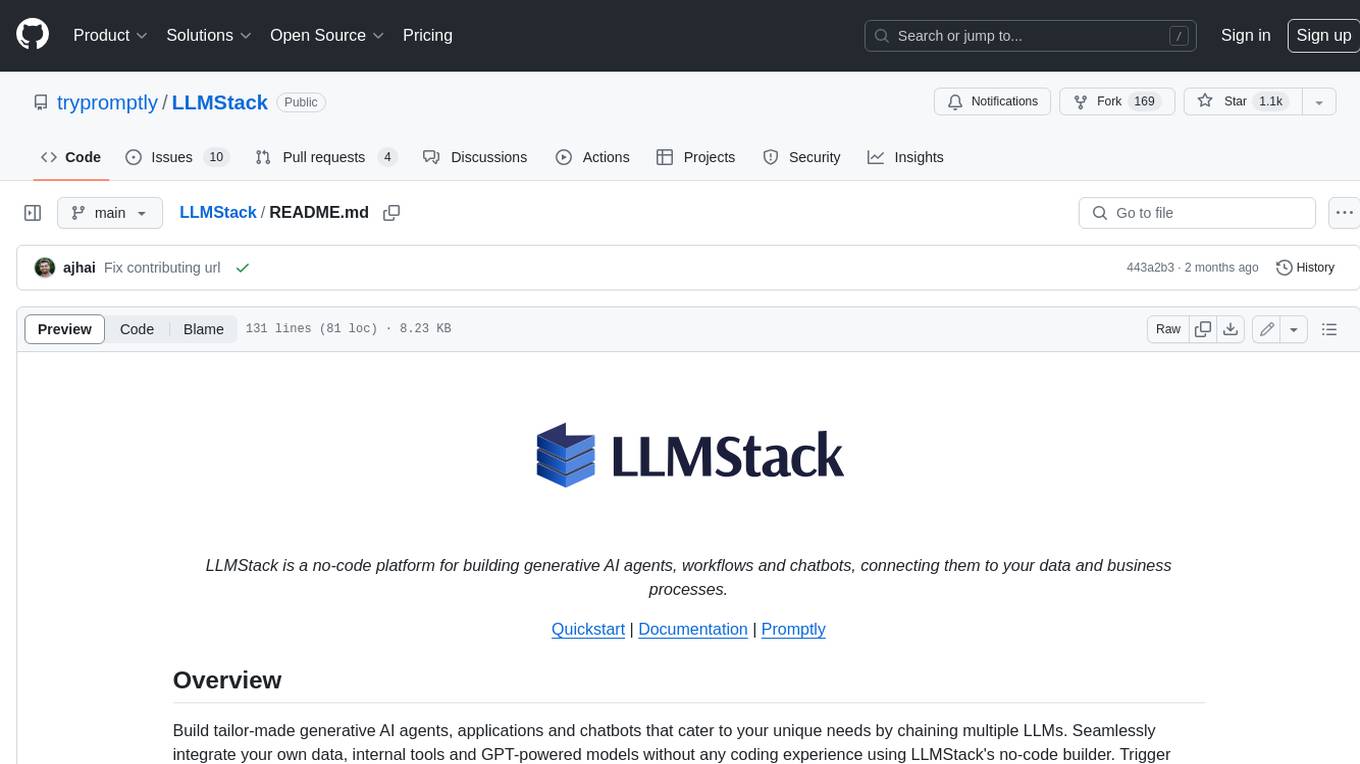
LLMStack
LLMStack is a no-code platform for building generative AI agents, workflows, and chatbots. It allows users to connect their own data, internal tools, and GPT-powered models without any coding experience. LLMStack can be deployed to the cloud or on-premise and can be accessed via HTTP API or triggered from Slack or Discord.
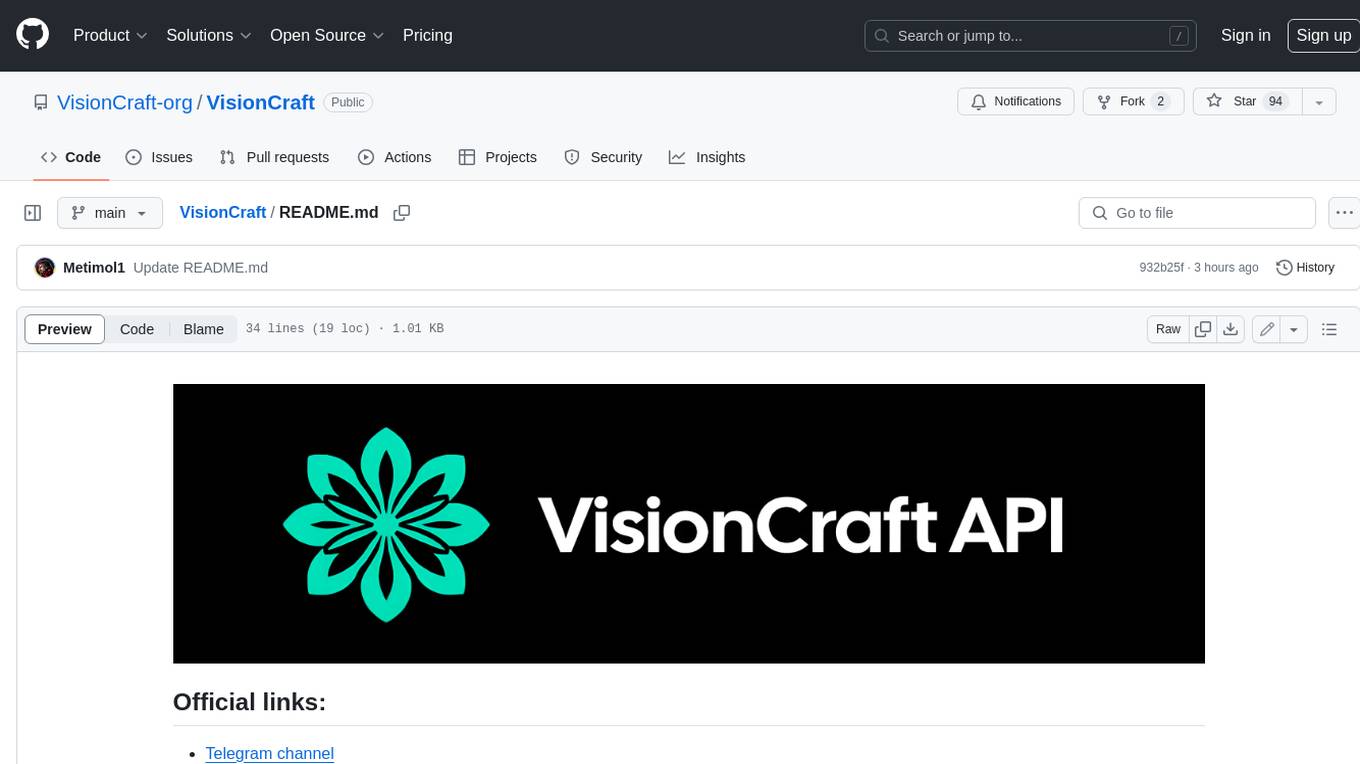
VisionCraft
The VisionCraft API is a free API for using over 100 different AI models. From images to sound.
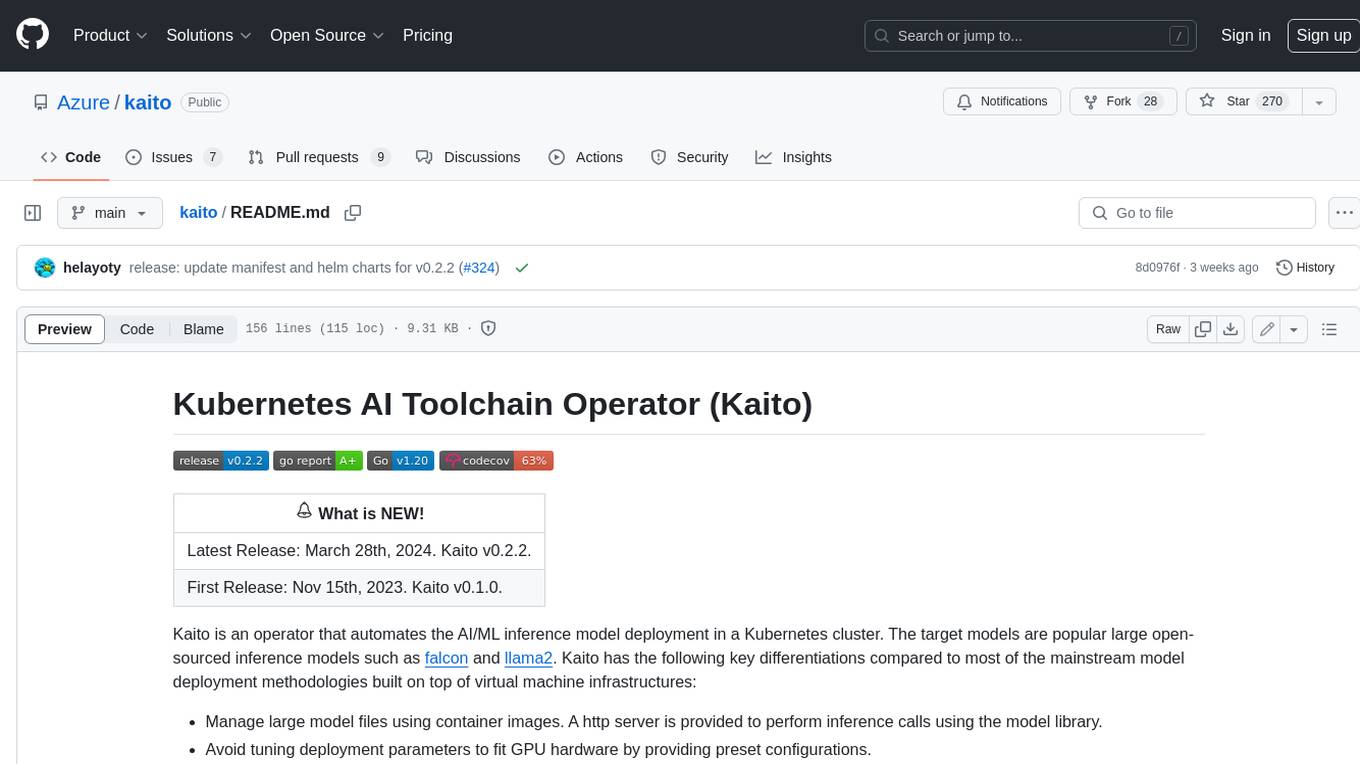
kaito
Kaito is an operator that automates the AI/ML inference model deployment in a Kubernetes cluster. It manages large model files using container images, avoids tuning deployment parameters to fit GPU hardware by providing preset configurations, auto-provisions GPU nodes based on model requirements, and hosts large model images in the public Microsoft Container Registry (MCR) if the license allows. Using Kaito, the workflow of onboarding large AI inference models in Kubernetes is largely simplified.
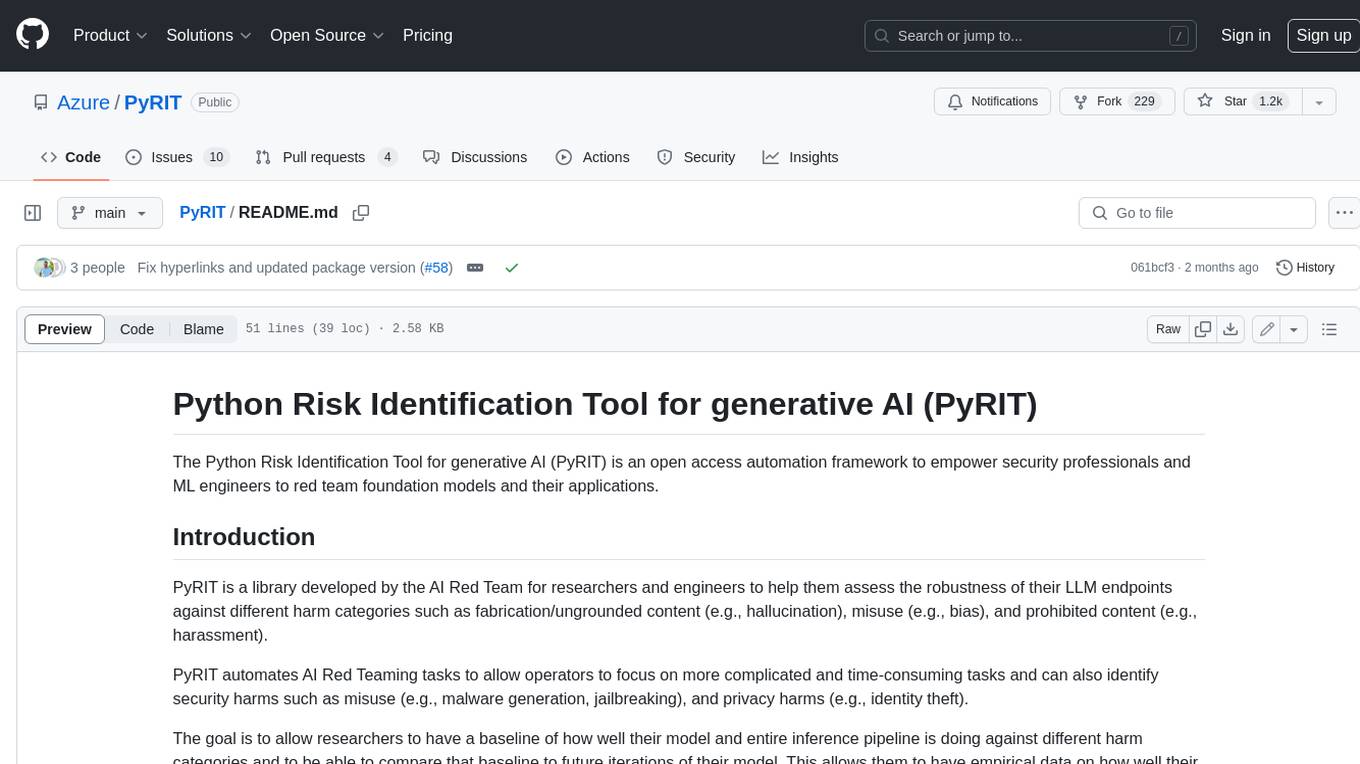
PyRIT
PyRIT is an open access automation framework designed to empower security professionals and ML engineers to red team foundation models and their applications. It automates AI Red Teaming tasks to allow operators to focus on more complicated and time-consuming tasks and can also identify security harms such as misuse (e.g., malware generation, jailbreaking), and privacy harms (e.g., identity theft). The goal is to allow researchers to have a baseline of how well their model and entire inference pipeline is doing against different harm categories and to be able to compare that baseline to future iterations of their model. This allows them to have empirical data on how well their model is doing today, and detect any degradation of performance based on future improvements.
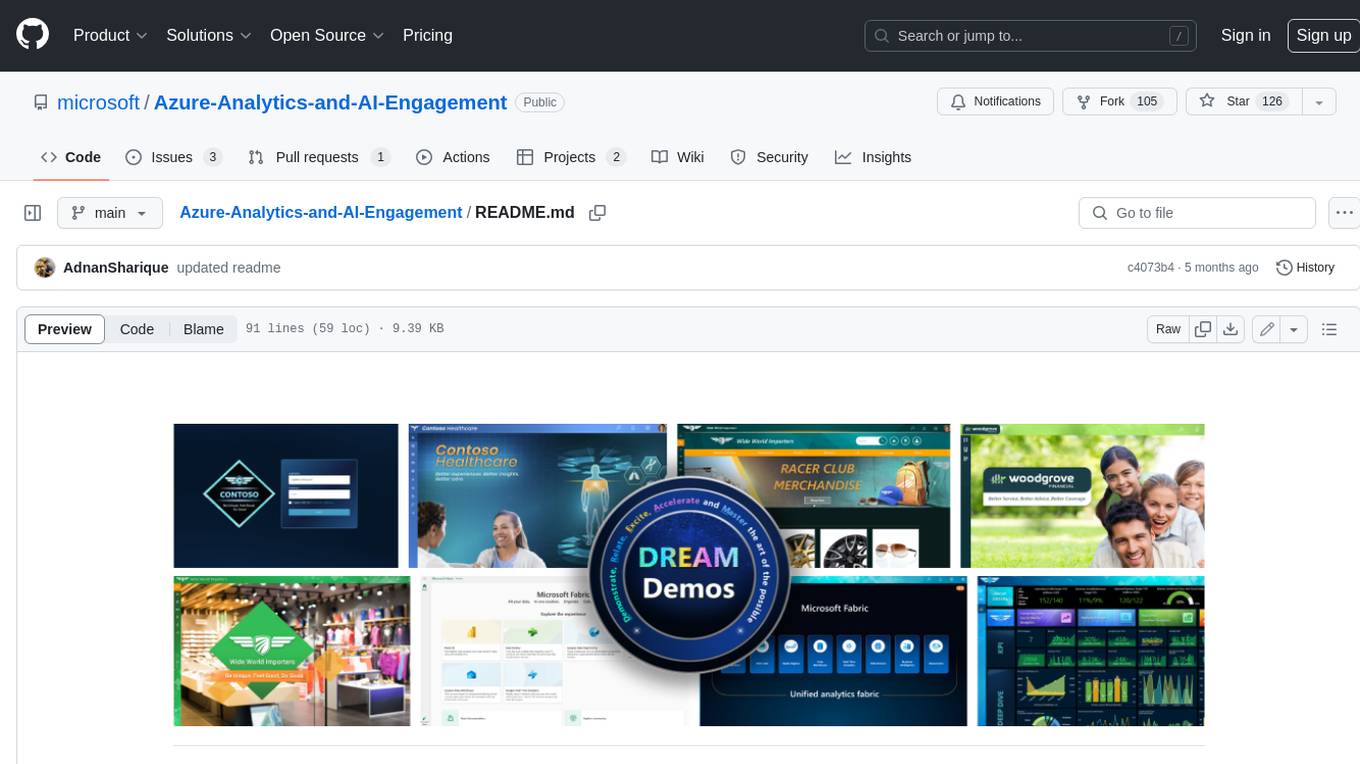
Azure-Analytics-and-AI-Engagement
The Azure-Analytics-and-AI-Engagement repository provides packaged Industry Scenario DREAM Demos with ARM templates (Containing a demo web application, Power BI reports, Synapse resources, AML Notebooks etc.) that can be deployed in a customerβs subscription using the CAPE tool within a matter of few hours. Partners can also deploy DREAM Demos in their own subscriptions using DPoC.