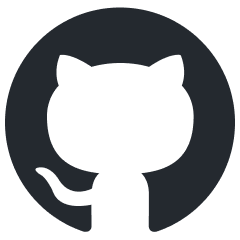
generative-ai-amazon-bedrock-langchain-agent-example
None
Stars: 199
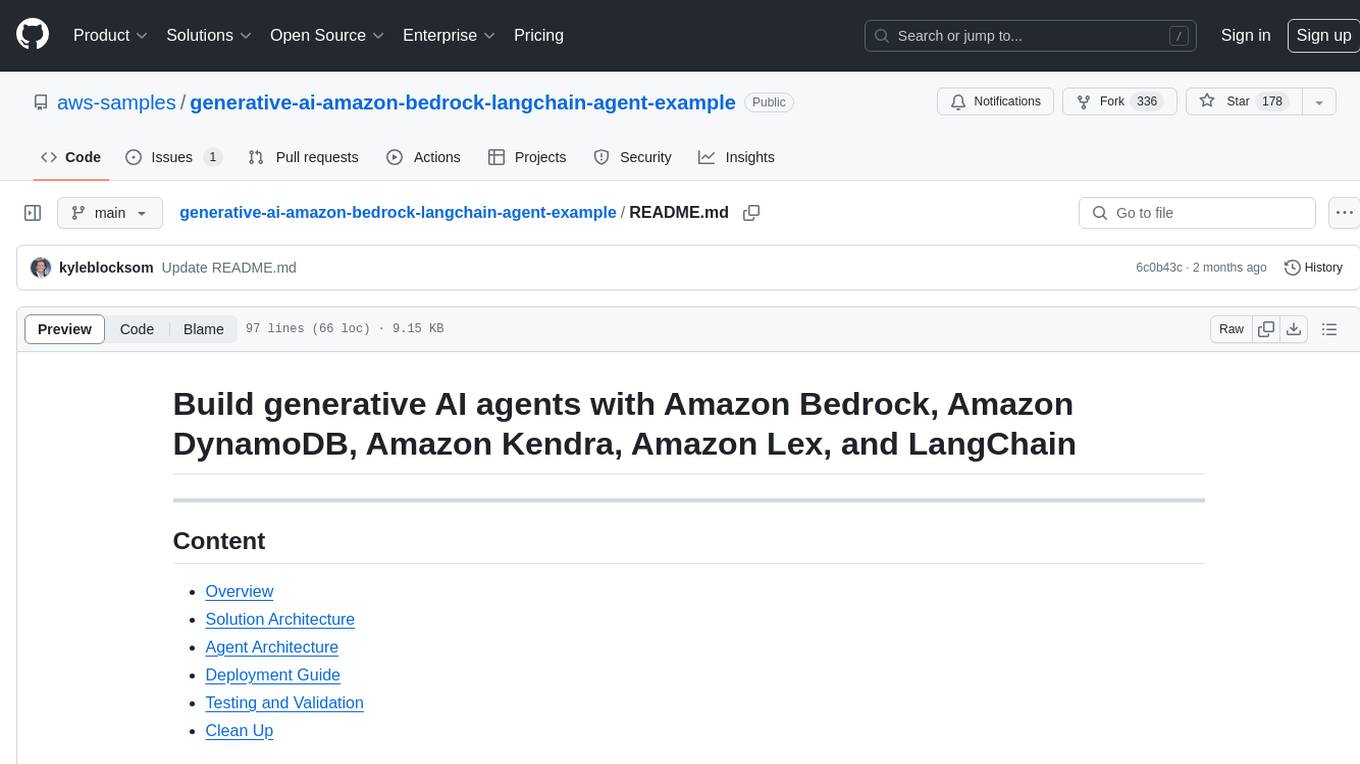
This repository provides a sample solution for building generative AI agents using Amazon Bedrock, Amazon DynamoDB, Amazon Kendra, Amazon Lex, and LangChain. The solution creates a generative AI financial services agent capable of assisting users with account information, loan applications, and answering natural language questions. It serves as a launchpad for developers to create personalized conversational agents for applications like chatbots and virtual assistants.
README:
Build generative AI agents with Amazon Bedrock, Amazon DynamoDB, Amazon Kendra, Amazon Lex, and LangChain
Generative AI agents are capable of producing human-like responses and engaging in natural language conversations by orchestrating a chain of calls to foundation models (FMs) and other augmenting tools based on user input. Instead of only fulfilling pre-defined intents through a static decision tree, agents are autonomous within the context of their suite of available tools. Amazon Bedrock is a fully managed service that makes leading foundation models from AI companies available through an API along with developer tooling to help build and scale generative AI applications.
This sample solution creates a generative AI financial services agent powered by Amazon Bedrock. The agent can assist users with finding their account information, completing a loan application, or answering natural language questions while also citing sources for the provided answers. This solution is intended to act as a launchpad for developers to create their own personalized conversational agents for various applications, such as chatbots, virtual assistants, and customer support systems.
Amazon Lex supplies the natural language understanding (NLU) and natural language processing (NLP) interface for the open source LangChain conversational agent within an AWS Amplify website. The agent is equipped with tools that include an Anthropic Claude 3 Sonnet FM hosted on Amazon Bedrock and synthetic customer data stored on Amazon DynamoDB and Amazon Kendra.
- Provide Personalized Responses - Query DynamoDB for customer account information, such as mortgage summary details, due balance, and next payment date.
- Access General Knowledge - Harness the agent’s reasoning logic in tandem with the vast amounts of data used to pretrain the different FMs provided through Bedrock to produce replies for any customer prompt.
- Curate Opinionated Answers - Inform agent responses using a Kendra Index configured with authoritative data sources: customer documents stored in Amazon Simple Storage Service (S3) and Web Crawler configured for the customer's website.
Diagram 1: Solution Architecture Overview
-
Users perform natural dialog with the Agent through their choice of Web, SMS, or Voice channels. The Web channel includes an AWS Amplify hosted website with an Amazon Lex embedded chatbot for an example customer, Octank Financial. Each user request is processed by Lex which invokes an AWS Lambda handler for intent fulfillment. SMS and Voice channels can be optionally configured using Amazon Connect and messaging integrations for Amazon Lex.
Each user request is processed by Lex to determine user intent through a process called intent recognition, which involves analyzing and interpreting the user's input (text or speech) to understand the user's intended action or purpose.
-
Lex then invokes an AWS Lambda handler for user intent fulfillment. The Lambda function associated with the Lex chatbot contains the logic and business rules required to process the user's intent. Lambda performs specific actions or retrieves information based on the user's input, making decisions and generating appropriate responses.
-
Lambda instruments the Financial Services agent logic as a LangChain Conversational Agent that can access customer-specific data stored on DynamoDB, curate opinionated responses using your documents and webpages indexed by Kendra, and provide general knowledge answers through the FM on Bedrock.
Responses generated by Kendra will include source attribution, demonstrating how you can provide additional contextual information to the agent through Retrieval-Augmented Generation (RAG). RAG allows you to enhance your agent’s ability to generate more accurate and contextually relevant responses using your own data.
Diagram 2: LangChain Conversational Agent Architecture
-
The LangChain Conversational Agent incorporates conversation memory so it can respond to multiple queries with contextual generation. This memory allows the agent to provide responses that take into account the context of the ongoing conversation. This is achieved through contextual generation, where the agent generates responses that are relevant and contextually appropriate based on the information it has remembered from the conversation.
In simpler terms, the agent remembers what was said earlier and uses that information to respond to multiple questions in a way that makes sense in the ongoing discussion. Our agent leverages LangChain's DynamoDB Chat Message History class as a conversation memory buffer so it can recall past interactions and enhance the user experience with more meaningful, context-aware responses.
-
The agent uses Anthropic Claude 3 Sonnet on Amazon Bedrock to complete the desired task through a series of carefully self-generated text inputs known as prompts. The primary objective of prompt engineering is to elicit specific and accurate responses from the FM. Different prompt engineering techniques include:
- Zero-Shot - A single question is presented to the model without any additional clues. The model is expected to generate a response based solely on the given question.
- Few-Shot - A set of sample questions and their corresponding answers are included before the actual question. By exposing the model to these examples, it learns to respond in a similar manner.
- Chain-of-Thought - A specific style of few-shot prompting where the prompt is designed to contain a series of intermediate reasoning steps, guiding the model through a logical thought process, ultimately leading to the desired answer.
Our Agent utilizes chain-of-thought reasoning by executing a set of Actions upon receiving a request. Following each Action, the Agent enters the Observation step, where it expresses a Thought. If a Final Answer is not yet achieved, the Agent iterates, selecting different Actions to progress towards reaching the Final Answer.
Thought: Do I need to use a tool? Yes
Action: The action to take
Action Input: The input to the action
Observation: The result of the action
Thought: Do I need to use a tool? No
FSI Agent: [answer and source documents]
-
As part of the agent's different reasoning paths and self-evaluating choices to decide the next course of action, it has the ability to access customer authoritative data sources using an Amazon Kendra index. Using Kendra, the agent performs a semantic similarity search across a wide range of content types, including documents, FAQs, knowledge bases, manuals, and websites - Please refer to the list of Kendra supported Data Sources.
The agent has the power to use this tool to provide opinionated responses to user prompts that should be answered using an authoritative, customer-provided knowledge library, instead of the more general knowledge corpus used to pretrain the Bedrock FM.
Sample Prompts:
- Why should I use Octank Financial?
- How competitive are their rates?
- Which type of mortgage should I use?
- What are current mortgage trends?
- How much do I need saved for a down payment?
- What other costs will I pay at closing?
see Deployment Guide
see Clean Up
Copyright Amazon.com, Inc. or its affiliates. All Rights Reserved. SPDX-License-Identifier: MIT-0
For Tasks:
Click tags to check more tools for each tasksFor Jobs:
Alternative AI tools for generative-ai-amazon-bedrock-langchain-agent-example
Similar Open Source Tools
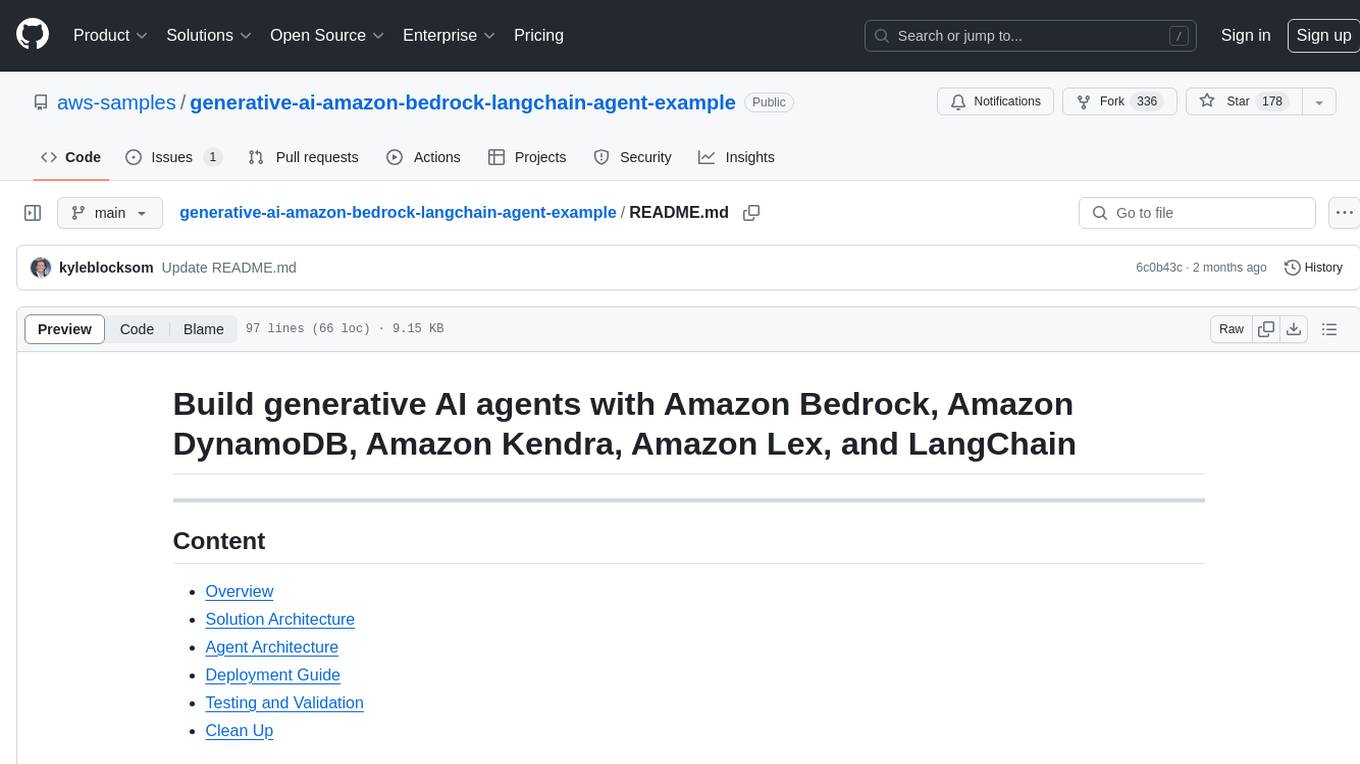
generative-ai-amazon-bedrock-langchain-agent-example
This repository provides a sample solution for building generative AI agents using Amazon Bedrock, Amazon DynamoDB, Amazon Kendra, Amazon Lex, and LangChain. The solution creates a generative AI financial services agent capable of assisting users with account information, loan applications, and answering natural language questions. It serves as a launchpad for developers to create personalized conversational agents for applications like chatbots and virtual assistants.
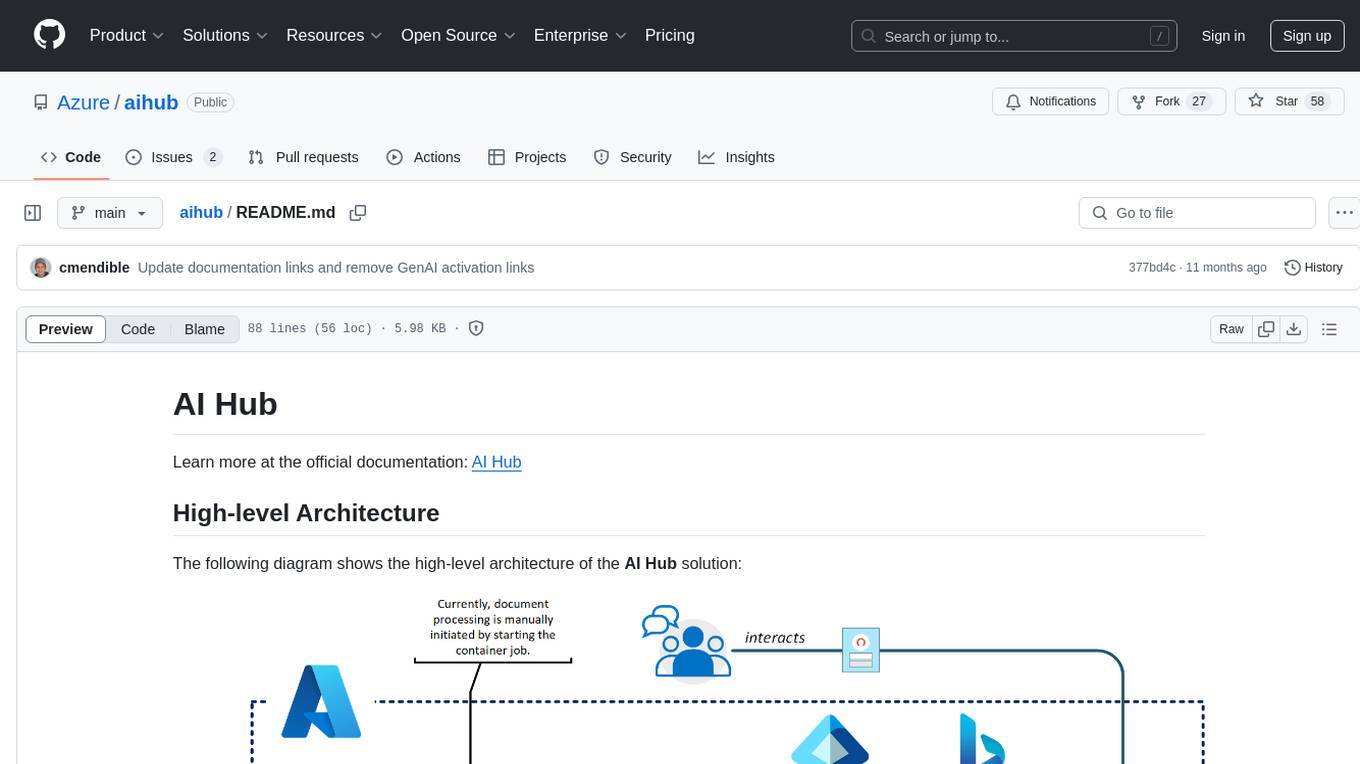
aihub
AI Hub is a comprehensive solution that leverages artificial intelligence and cloud computing to provide functionalities such as document search and retrieval, call center analytics, image analysis, brand reputation analysis, form analysis, document comparison, and content safety moderation. It integrates various Azure services like Cognitive Search, ChatGPT, Azure Vision Services, and Azure Document Intelligence to offer scalable, extensible, and secure AI-powered capabilities for different use cases and scenarios.
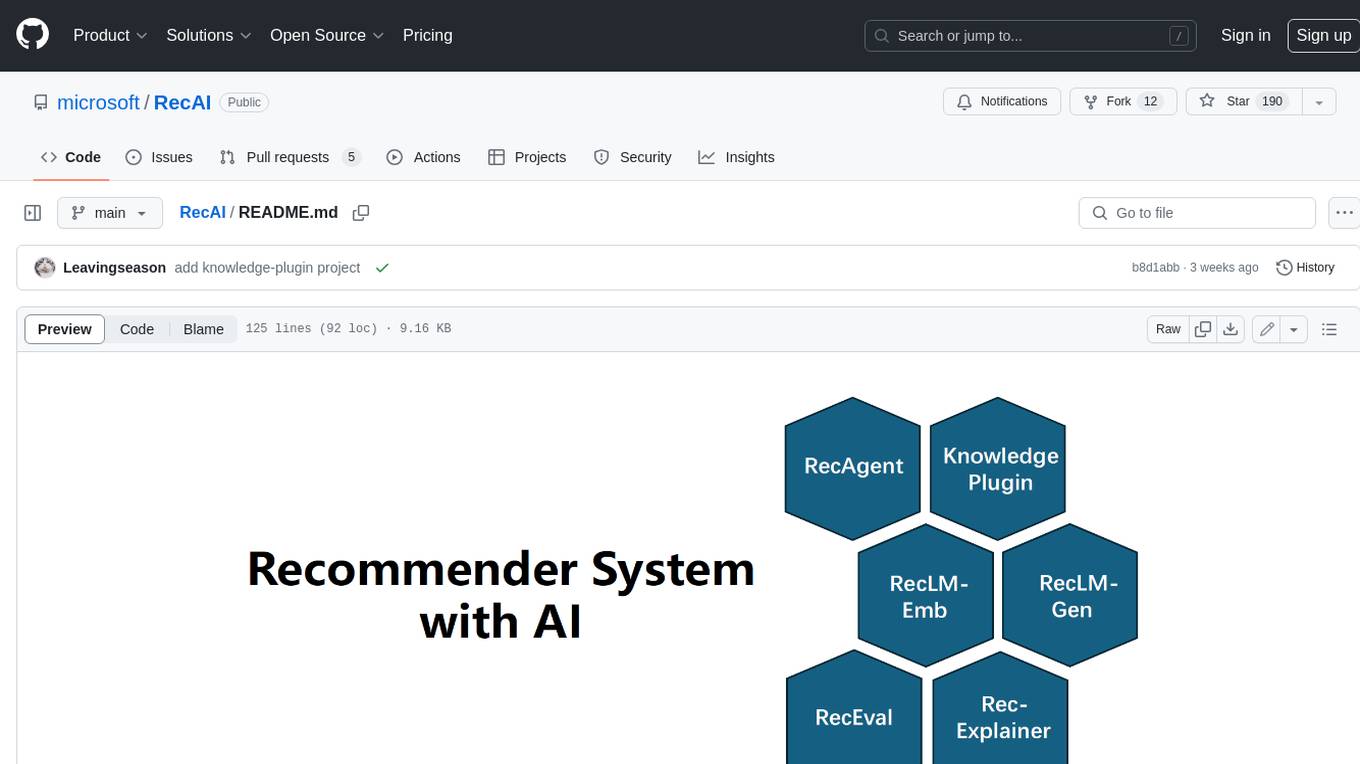
RecAI
RecAI is a project that explores the integration of Large Language Models (LLMs) into recommender systems, addressing the challenges of interactivity, explainability, and controllability. It aims to bridge the gap between general-purpose LLMs and domain-specific recommender systems, providing a holistic perspective on the practical requirements of LLM4Rec. The project investigates various techniques, including Recommender AI agents, selective knowledge injection, fine-tuning language models, evaluation, and LLMs as model explainers, to create more sophisticated, interactive, and user-centric recommender systems.
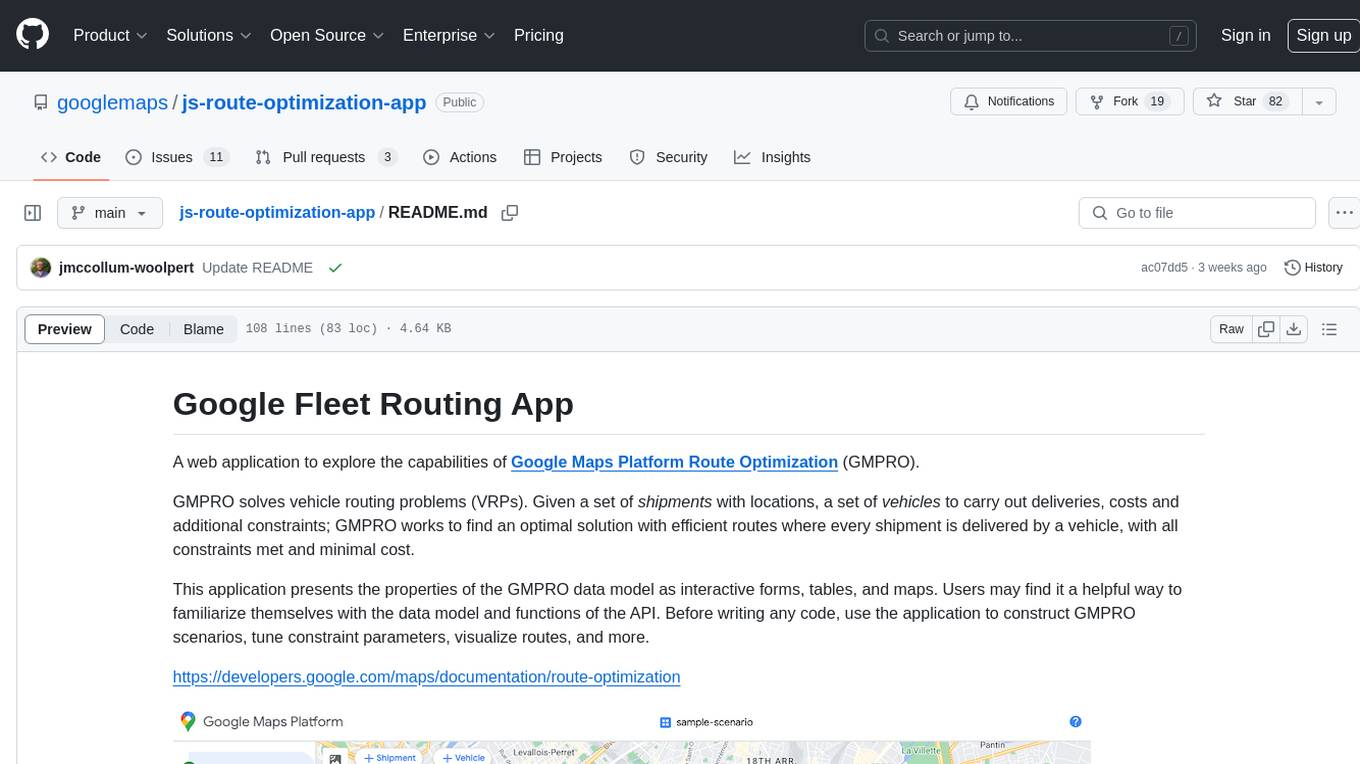
js-route-optimization-app
A web application to explore the capabilities of Google Maps Platform Route Optimization (GMPRO). It helps users understand the data model and functions of the API by presenting interactive forms, tables, and maps. The tool is intended for exploratory use only and should not be deployed in production. Users can construct scenarios, tune constraint parameters, and visualize routes before implementing their own solutions for integrating Route Optimization into their business processes. The application incurs charges related to cloud resources and API usage, and users should be cautious about generating high usage volumes, especially for large scenarios.
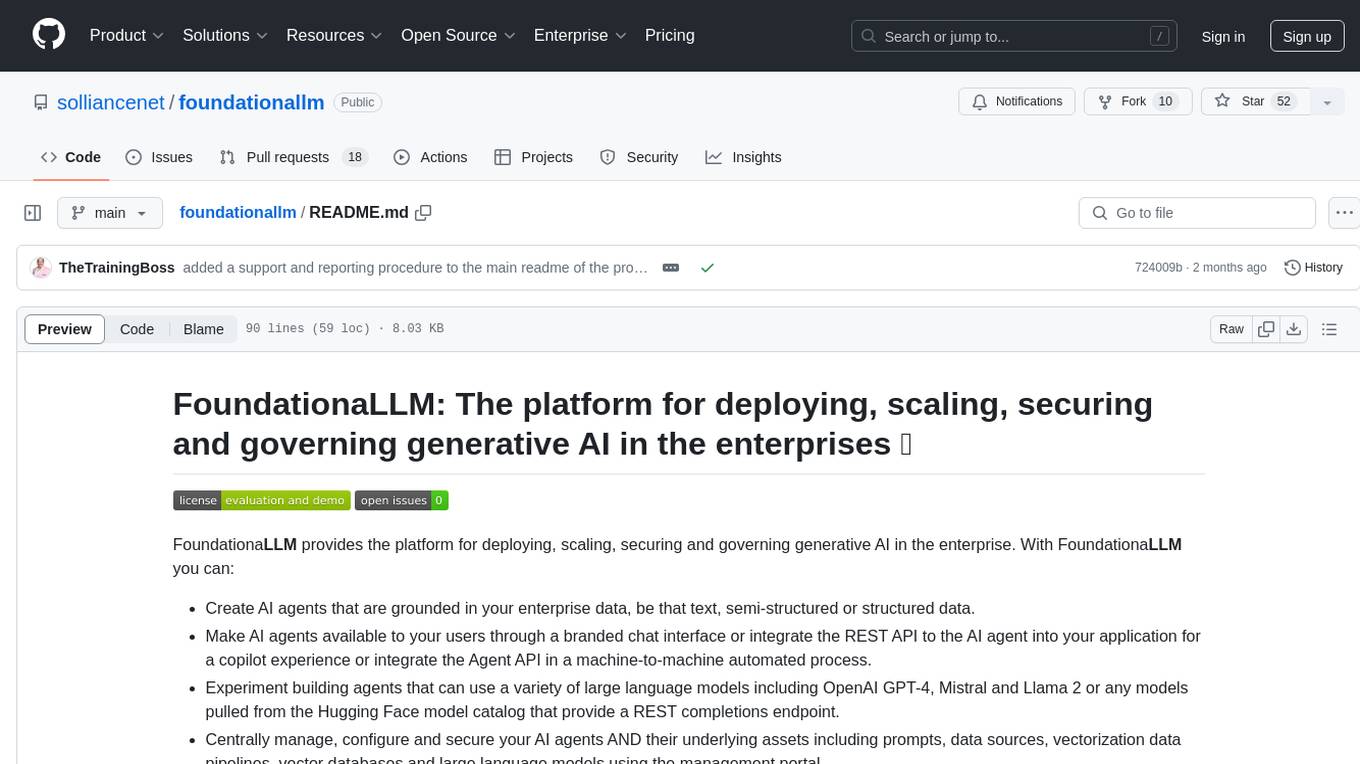
foundationallm
FoundationaLLM is a platform designed for deploying, scaling, securing, and governing generative AI in enterprises. It allows users to create AI agents grounded in enterprise data, integrate REST APIs, experiment with large language models, centrally manage AI agents and assets, deploy scalable vectorization data pipelines, enable non-developer users to create their own AI agents, control access with role-based access controls, and harness capabilities from Azure AI and Azure OpenAI. The platform simplifies integration with enterprise data sources, provides fine-grain security controls, load balances across multiple endpoints, and is extensible to new data sources and orchestrators. FoundationaLLM addresses the need for customized copilots or AI agents that are secure, licensed, flexible, and suitable for enterprise-scale production.
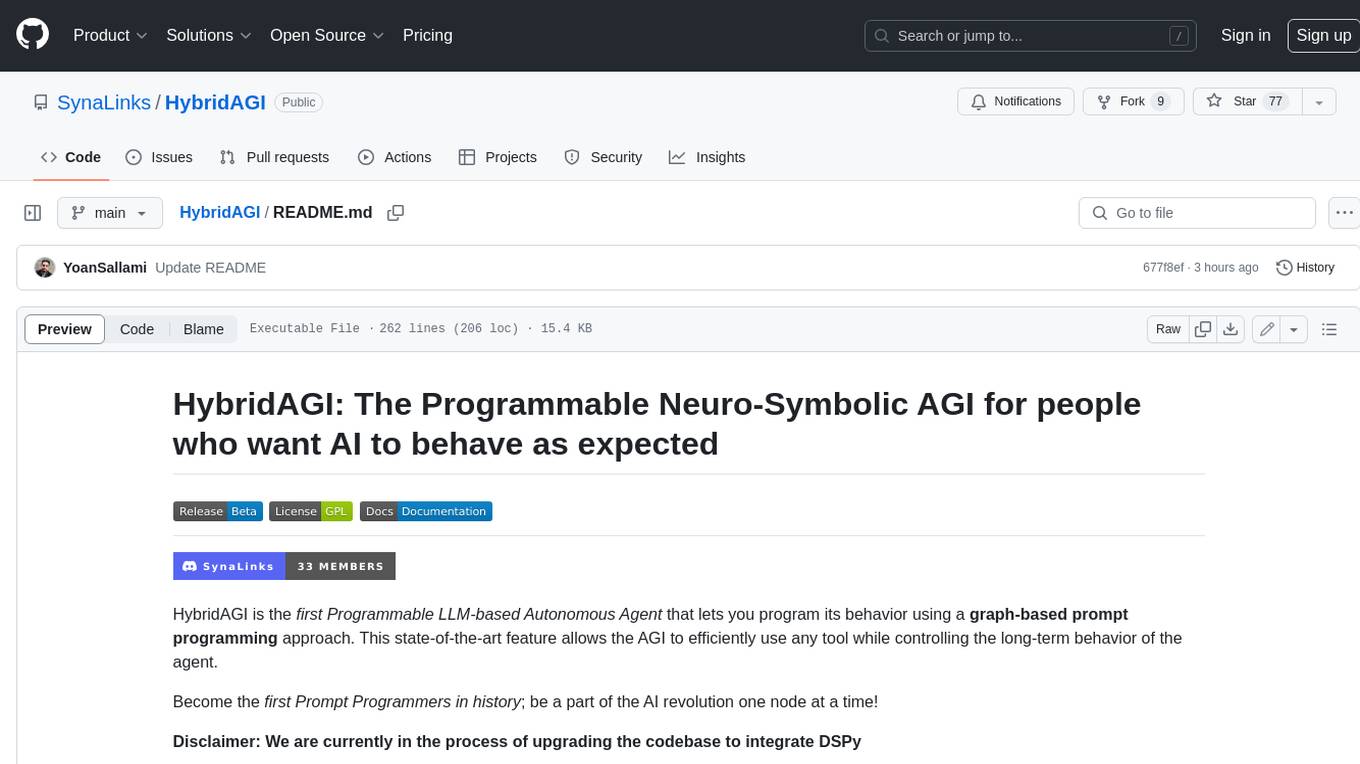
HybridAGI
HybridAGI is the first Programmable LLM-based Autonomous Agent that lets you program its behavior using a **graph-based prompt programming** approach. This state-of-the-art feature allows the AGI to efficiently use any tool while controlling the long-term behavior of the agent. Become the _first Prompt Programmers in history_ ; be a part of the AI revolution one node at a time! **Disclaimer: We are currently in the process of upgrading the codebase to integrate DSPy**
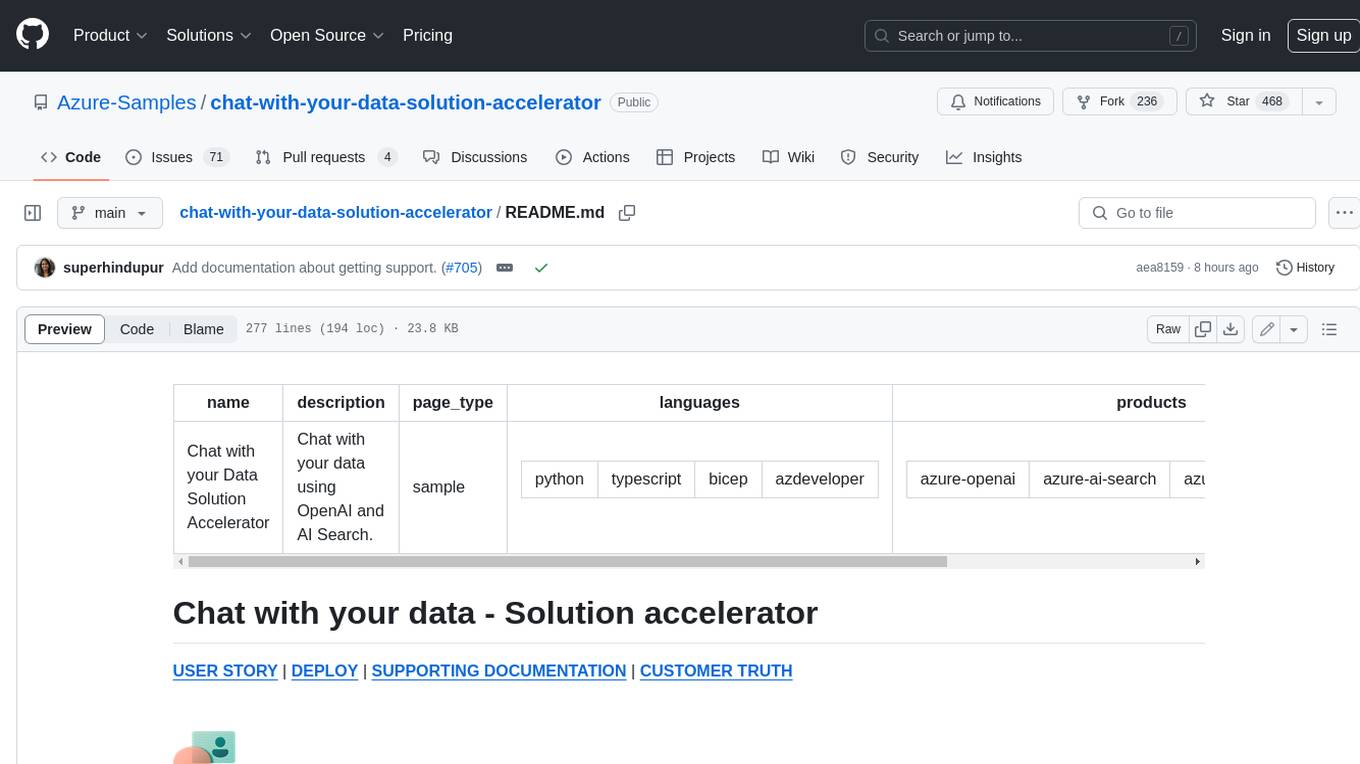
chat-with-your-data-solution-accelerator
Chat with your data using OpenAI and AI Search. This solution accelerator uses an Azure OpenAI GPT model and an Azure AI Search index generated from your data, which is integrated into a web application to provide a natural language interface, including speech-to-text functionality, for search queries. Users can drag and drop files, point to storage, and take care of technical setup to transform documents. There is a web app that users can create in their own subscription with security and authentication.
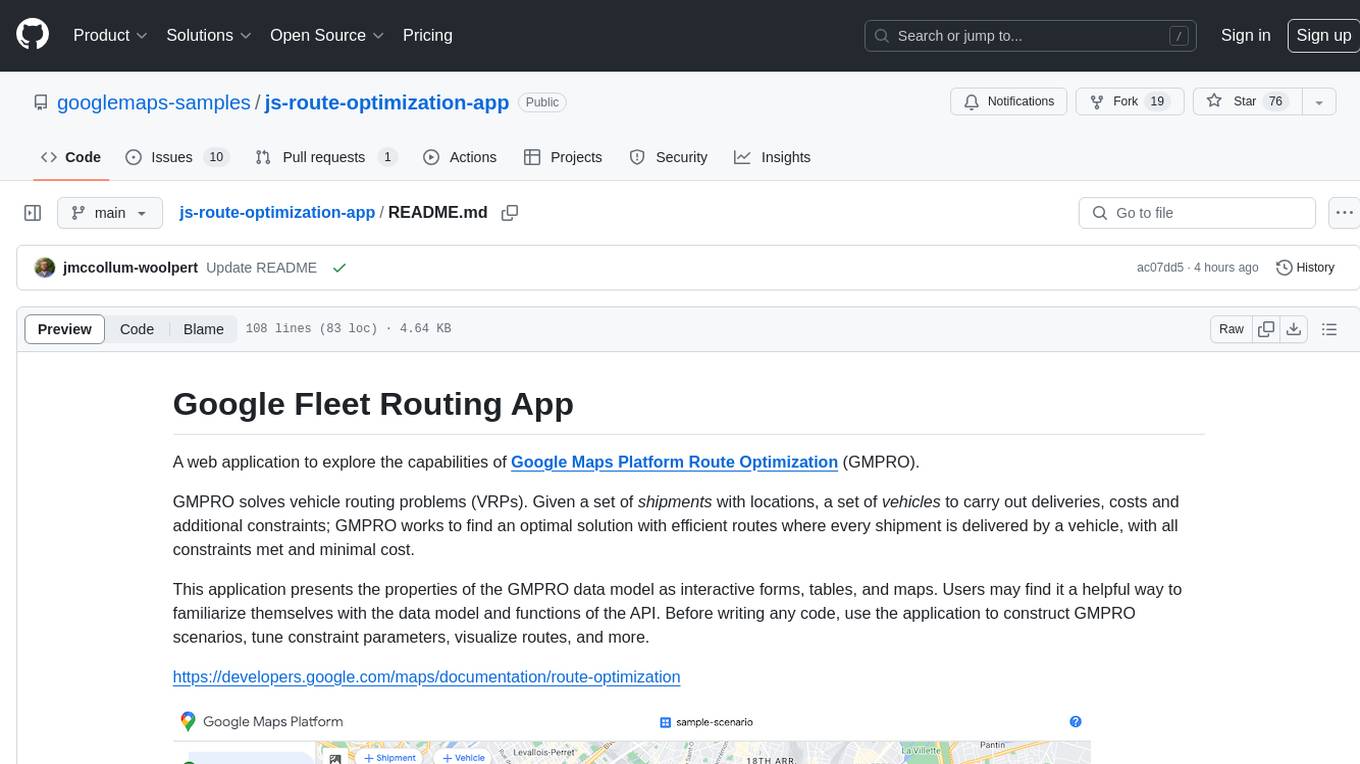
js-route-optimization-app
A web application to explore the capabilities of Google Maps Platform Route Optimization (GMPRO) for solving vehicle routing problems. Users can interact with the GMPRO data model through forms, tables, and maps to construct scenarios, tune constraints, and visualize routes. The application is intended for exploration purposes only and should not be deployed in production. Users are responsible for billing related to cloud resources and API usage. It is important to understand the pricing models for Maps Platform and Route Optimization before using the application.
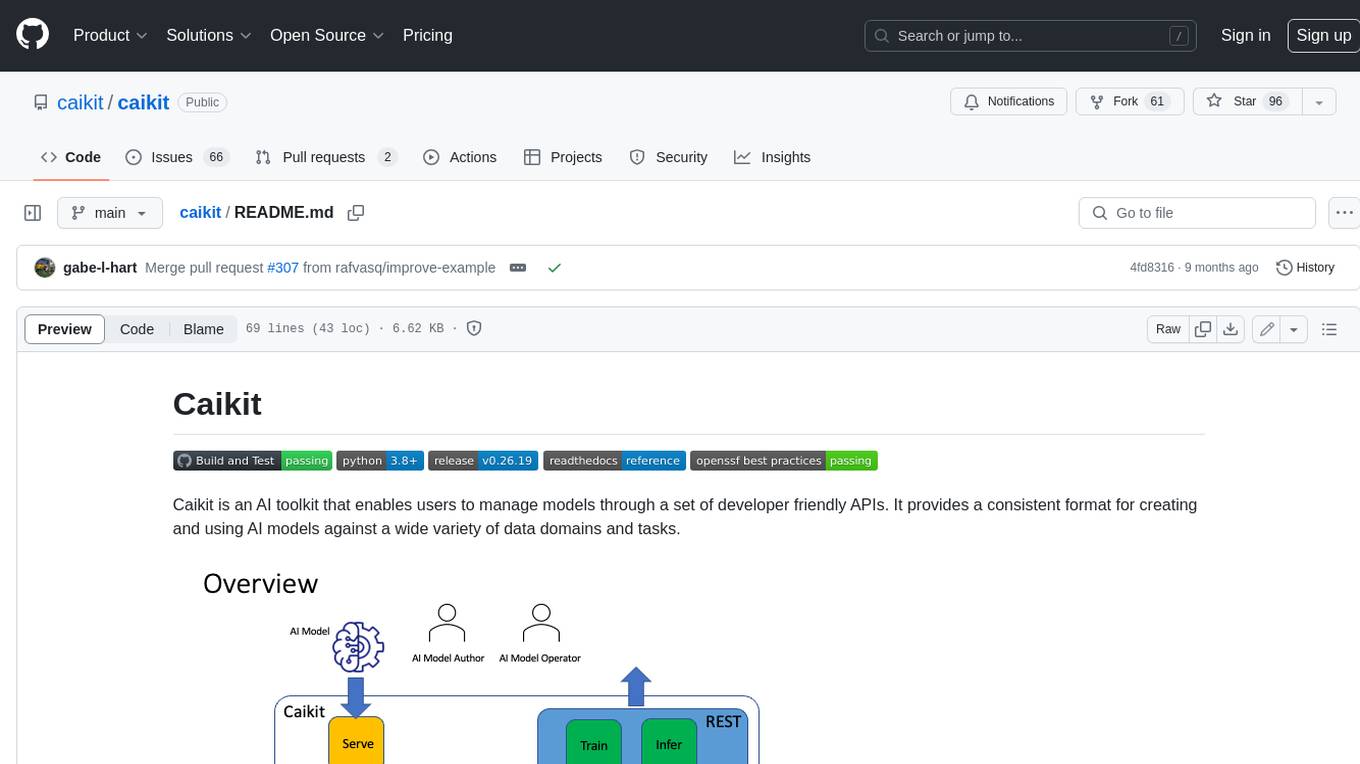
caikit
Caikit is an AI toolkit that enables users to manage models through a set of developer friendly APIs. It provides a consistent format for creating and using AI models against a wide variety of data domains and tasks.
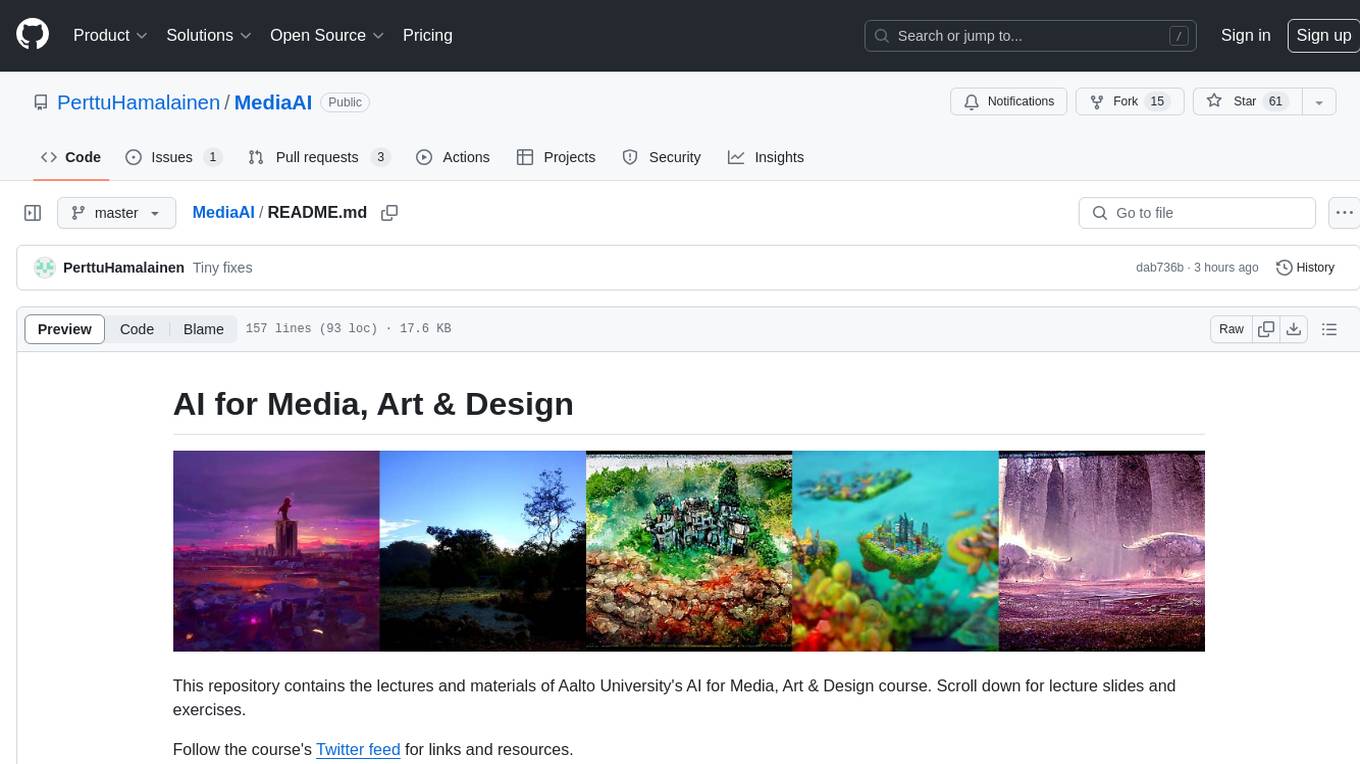
MediaAI
MediaAI is a repository containing lectures and materials for Aalto University's AI for Media, Art & Design course. The course is a hands-on, project-based crash course focusing on deep learning and AI techniques for artists and designers. It covers common AI algorithms & tools, their applications in art, media, and design, and provides hands-on practice in designing, implementing, and using these tools. The course includes lectures, exercises, and a final project based on students' interests. Students can complete the course without programming by creatively utilizing existing tools like ChatGPT and DALL-E. The course emphasizes collaboration, peer-to-peer tutoring, and project-based learning. It covers topics such as text generation, image generation, optimization, and game AI.
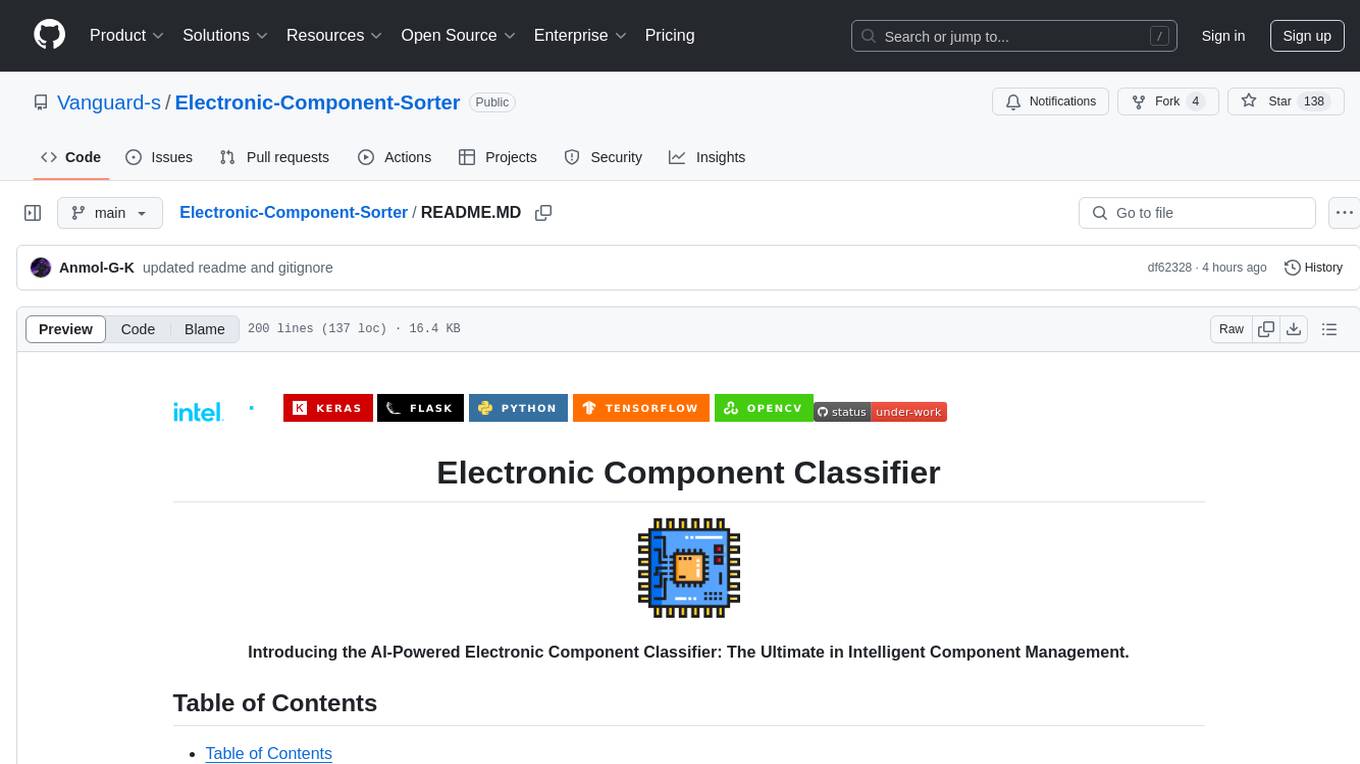
Electronic-Component-Sorter
The Electronic Component Classifier is a project that uses machine learning and artificial intelligence to automate the identification and classification of electrical and electronic components. It features component classification into seven classes, user-friendly design, and integration with Flask for a user-friendly interface. The project aims to reduce human error in component identification, make the process safer and more reliable, and potentially help visually impaired individuals in identifying electronic components.
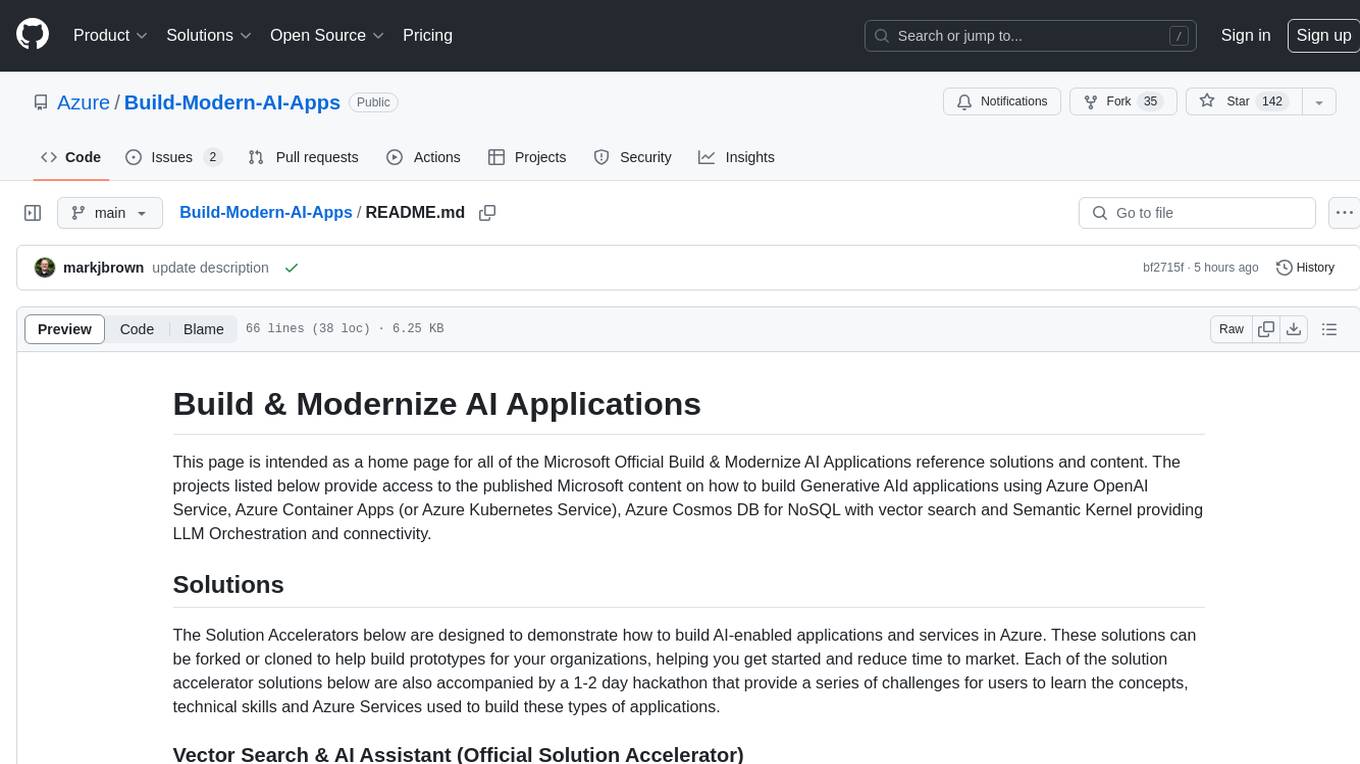
Build-Modern-AI-Apps
This repository serves as a hub for Microsoft Official Build & Modernize AI Applications reference solutions and content. It provides access to projects demonstrating how to build Generative AI applications using Azure services like Azure OpenAI, Azure Container Apps, Azure Kubernetes, and Azure Cosmos DB. The solutions include Vector Search & AI Assistant, Real-Time Payment and Transaction Processing, and Medical Claims Processing. Additionally, there are workshops like the Intelligent App Workshop for Microsoft Copilot Stack, focusing on infusing intelligence into traditional software systems using foundation models and design thinking.
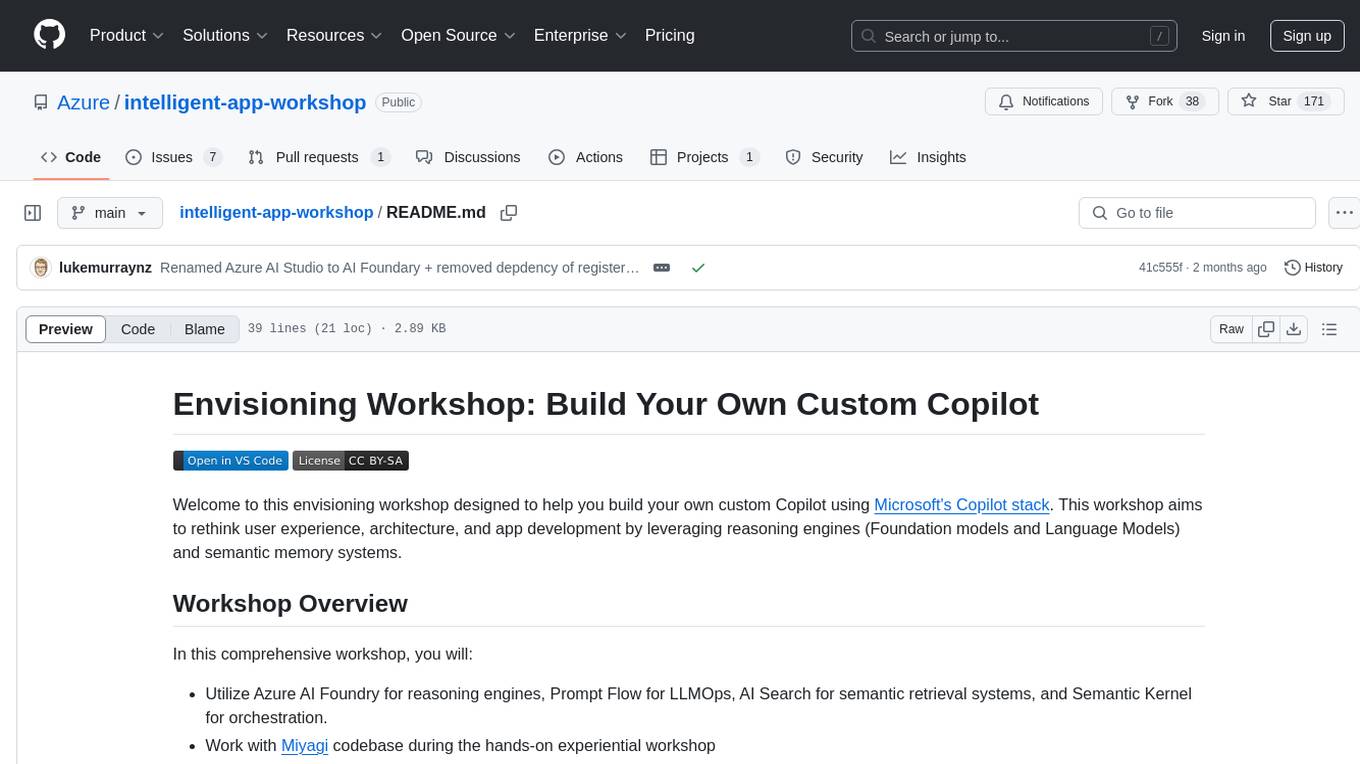
intelligent-app-workshop
Welcome to the envisioning workshop designed to help you build your own custom Copilot using Microsoft's Copilot stack. This workshop aims to rethink user experience, architecture, and app development by leveraging reasoning engines and semantic memory systems. You will utilize Azure AI Foundry, Prompt Flow, AI Search, and Semantic Kernel. Work with Miyagi codebase, explore advanced capabilities like AutoGen and GraphRag. This workshop guides you through the entire lifecycle of app development, including identifying user needs, developing a production-grade app, and deploying on Azure with advanced capabilities. By the end, you will have a deeper understanding of leveraging Microsoft's tools to create intelligent applications.
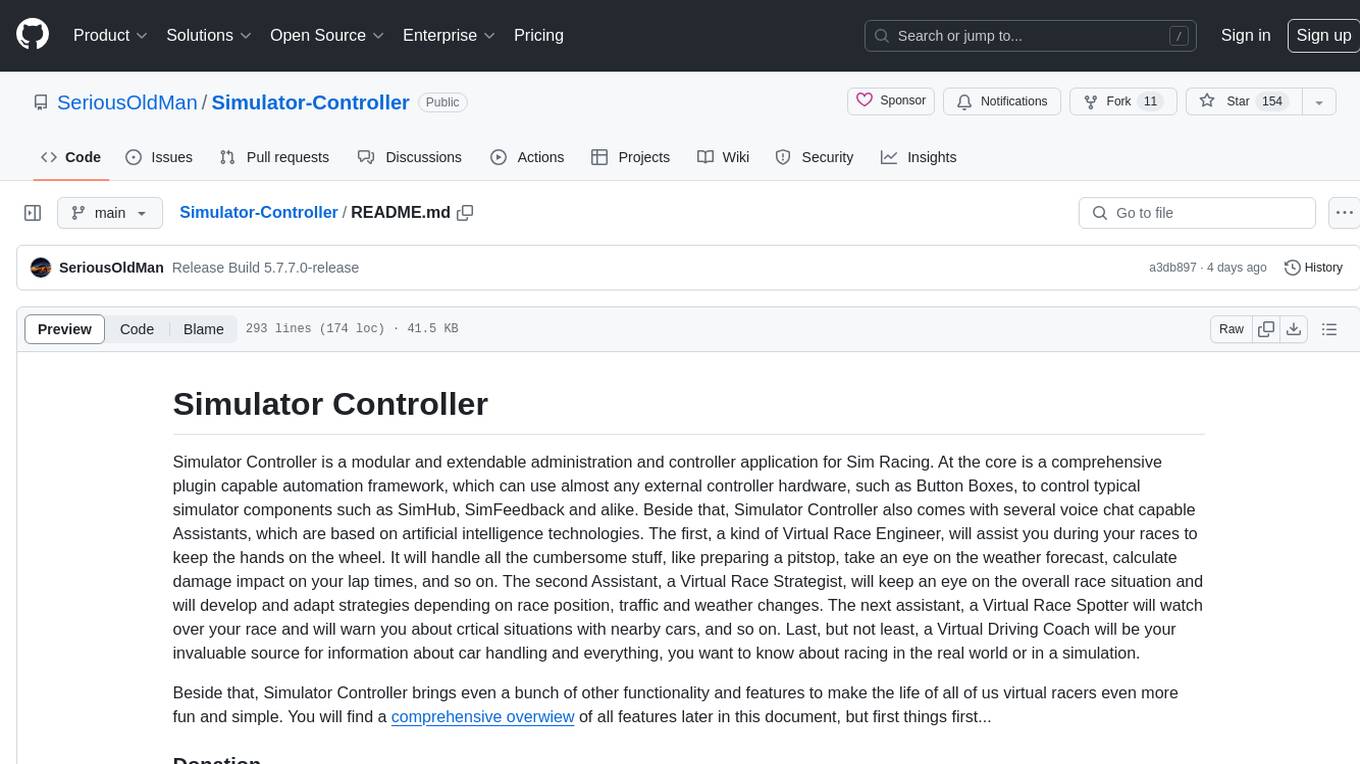
Simulator-Controller
Simulator Controller is a modular administration and controller application for Sim Racing, featuring a comprehensive plugin automation framework for external controller hardware. It includes voice chat capable Assistants like Virtual Race Engineer, Race Strategist, Race Spotter, and Driving Coach. The tool offers features for setup, strategy development, monitoring races, and more. Developed in AutoHotkey, it supports various simulation games and integrates with third-party applications for enhanced functionality.
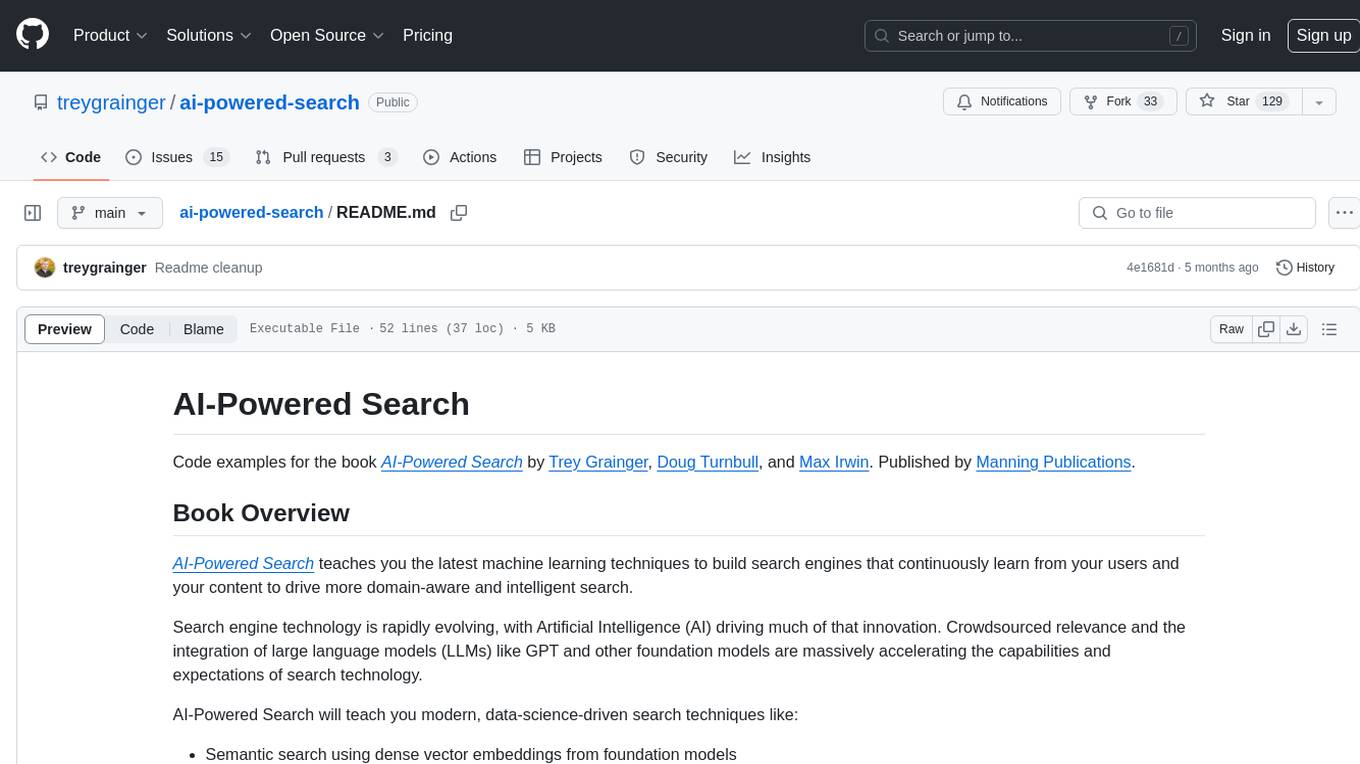
ai-powered-search
AI-Powered Search provides code examples for the book 'AI-Powered Search' by Trey Grainger, Doug Turnbull, and Max Irwin. The book teaches modern machine learning techniques for building search engines that continuously learn from users and content to deliver more intelligent and domain-aware search experiences. It covers semantic search, retrieval augmented generation, question answering, summarization, fine-tuning transformer-based models, personalized search, machine-learned ranking, click models, and more. The code examples are in Python, leveraging PySpark for data processing and Apache Solr as the default search engine. The repository is open source under the Apache License, Version 2.0.
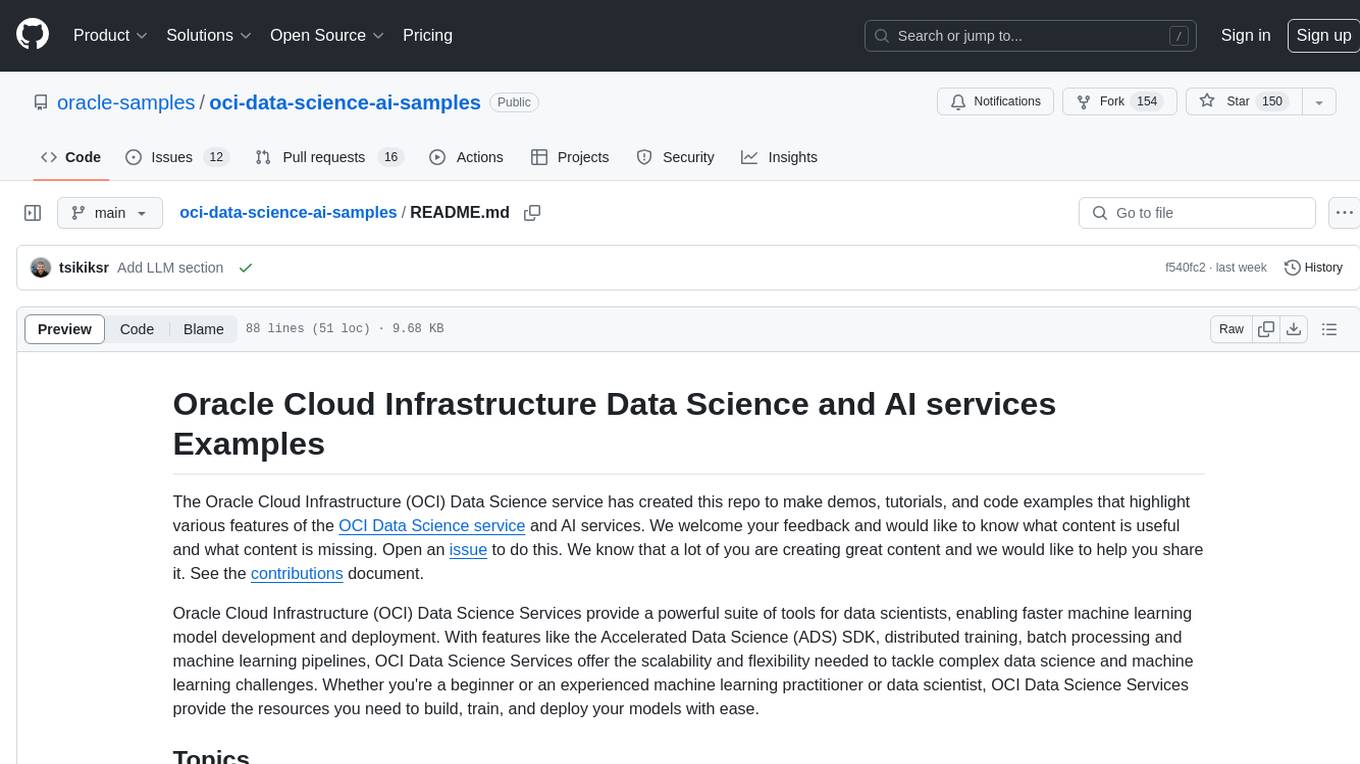
oci-data-science-ai-samples
The Oracle Cloud Infrastructure Data Science and AI services Examples repository provides demos, tutorials, and code examples showcasing various features of the OCI Data Science service and AI services. It offers tools for data scientists to develop and deploy machine learning models efficiently, with features like Accelerated Data Science SDK, distributed training, batch processing, and machine learning pipelines. Whether you're a beginner or an experienced practitioner, OCI Data Science Services provide the resources needed to build, train, and deploy models easily.
For similar tasks
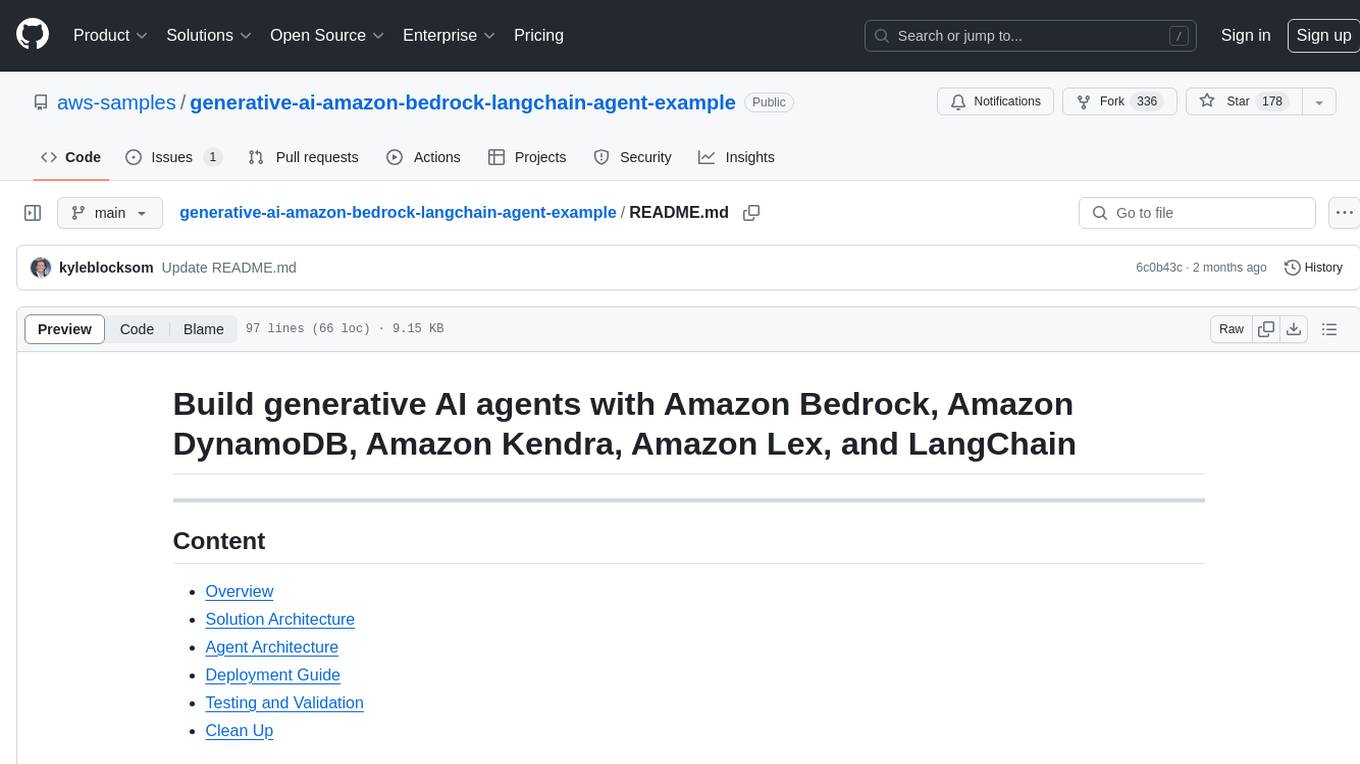
generative-ai-amazon-bedrock-langchain-agent-example
This repository provides a sample solution for building generative AI agents using Amazon Bedrock, Amazon DynamoDB, Amazon Kendra, Amazon Lex, and LangChain. The solution creates a generative AI financial services agent capable of assisting users with account information, loan applications, and answering natural language questions. It serves as a launchpad for developers to create personalized conversational agents for applications like chatbots and virtual assistants.
For similar jobs
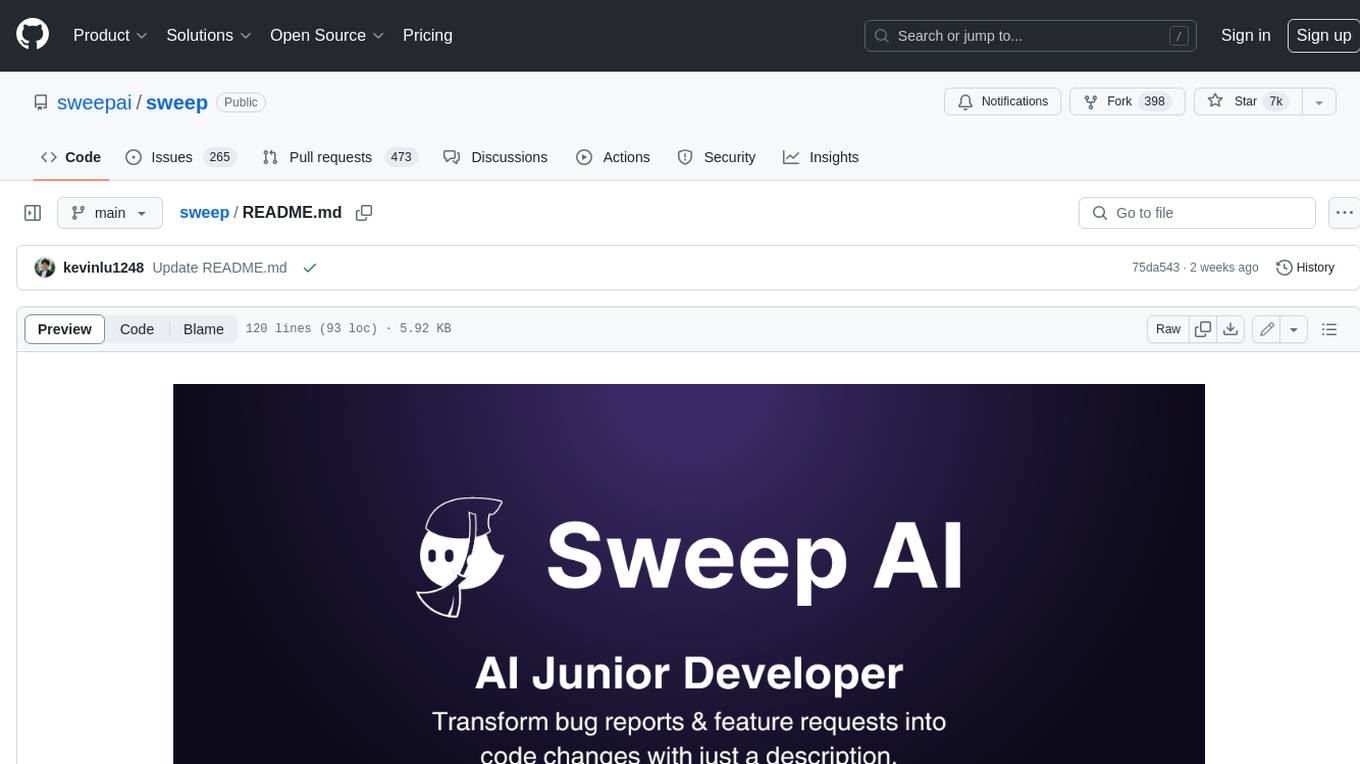
sweep
Sweep is an AI junior developer that turns bugs and feature requests into code changes. It automatically handles developer experience improvements like adding type hints and improving test coverage.
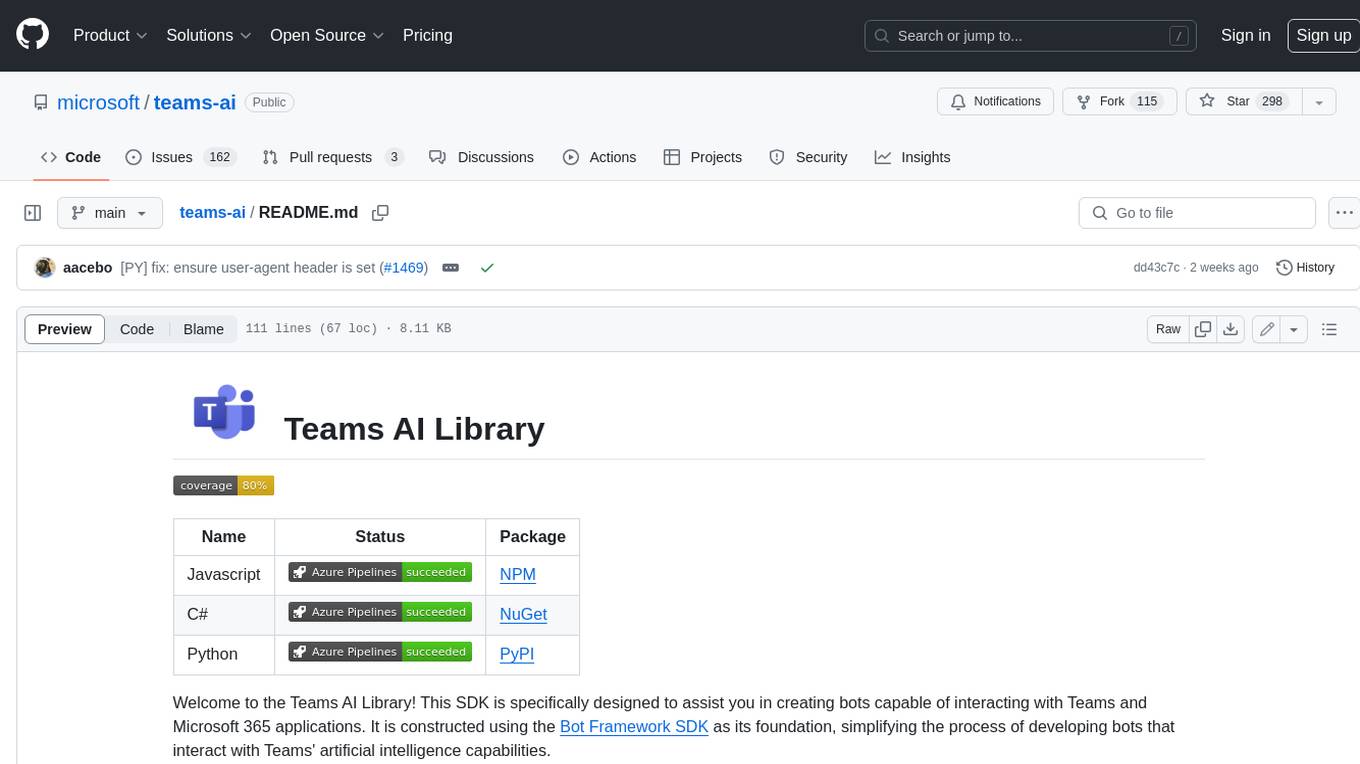
teams-ai
The Teams AI Library is a software development kit (SDK) that helps developers create bots that can interact with Teams and Microsoft 365 applications. It is built on top of the Bot Framework SDK and simplifies the process of developing bots that interact with Teams' artificial intelligence capabilities. The SDK is available for JavaScript/TypeScript, .NET, and Python.
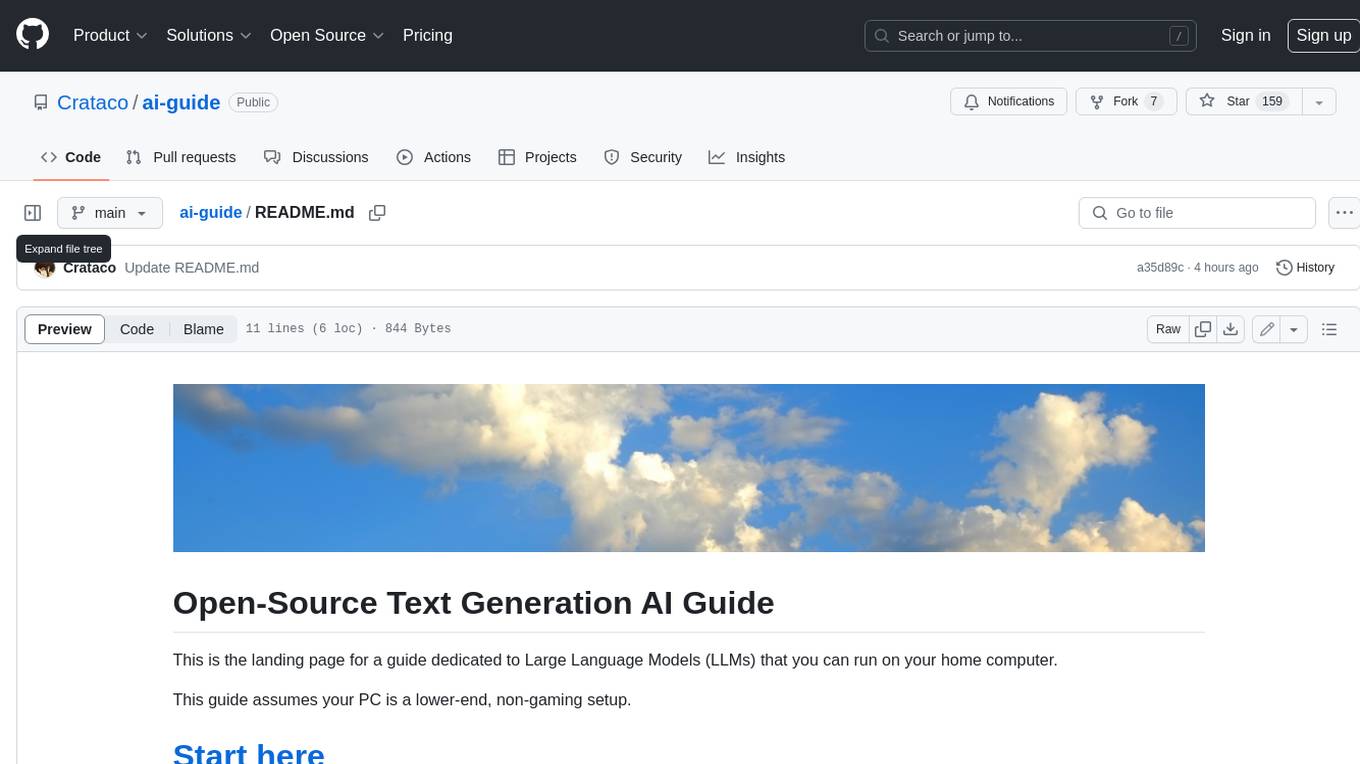
ai-guide
This guide is dedicated to Large Language Models (LLMs) that you can run on your home computer. It assumes your PC is a lower-end, non-gaming setup.
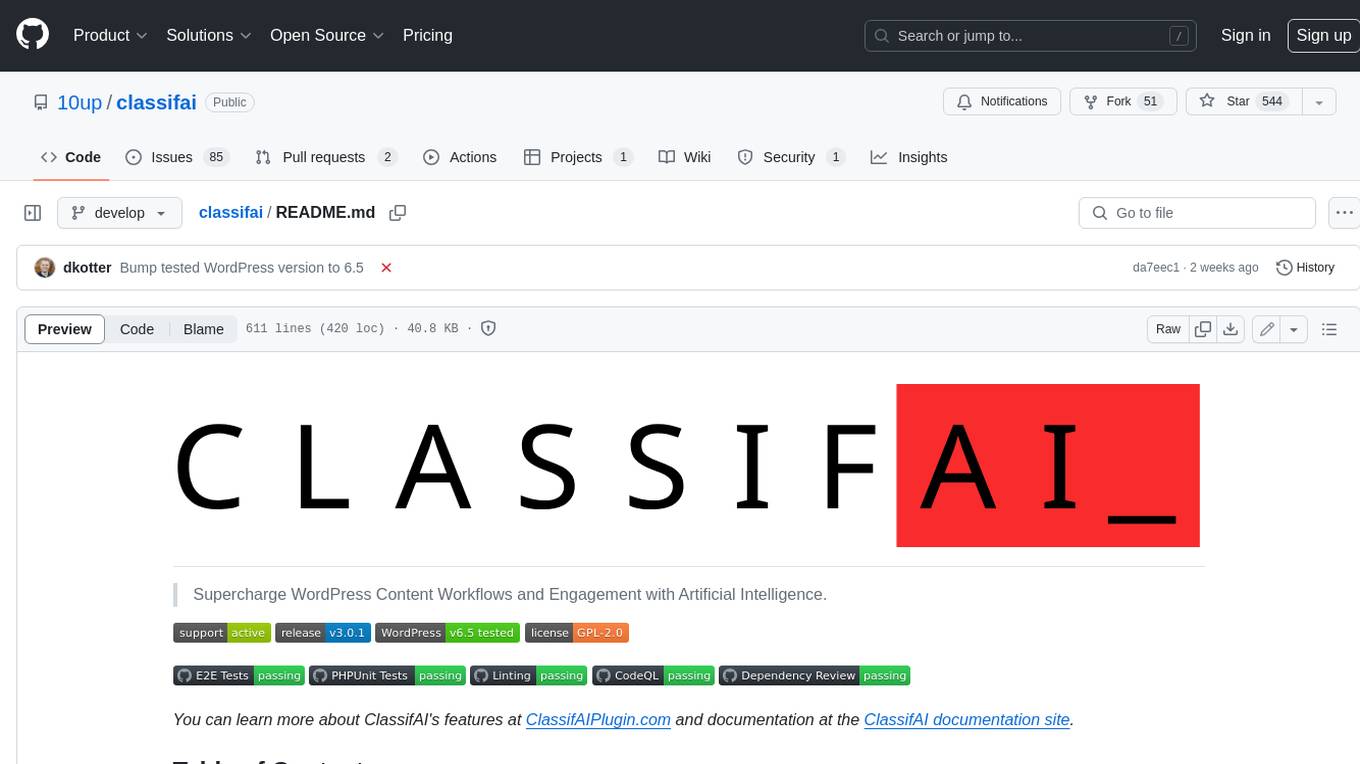
classifai
Supercharge WordPress Content Workflows and Engagement with Artificial Intelligence. Tap into leading cloud-based services like OpenAI, Microsoft Azure AI, Google Gemini and IBM Watson to augment your WordPress-powered websites. Publish content faster while improving SEO performance and increasing audience engagement. ClassifAI integrates Artificial Intelligence and Machine Learning technologies to lighten your workload and eliminate tedious tasks, giving you more time to create original content that matters.
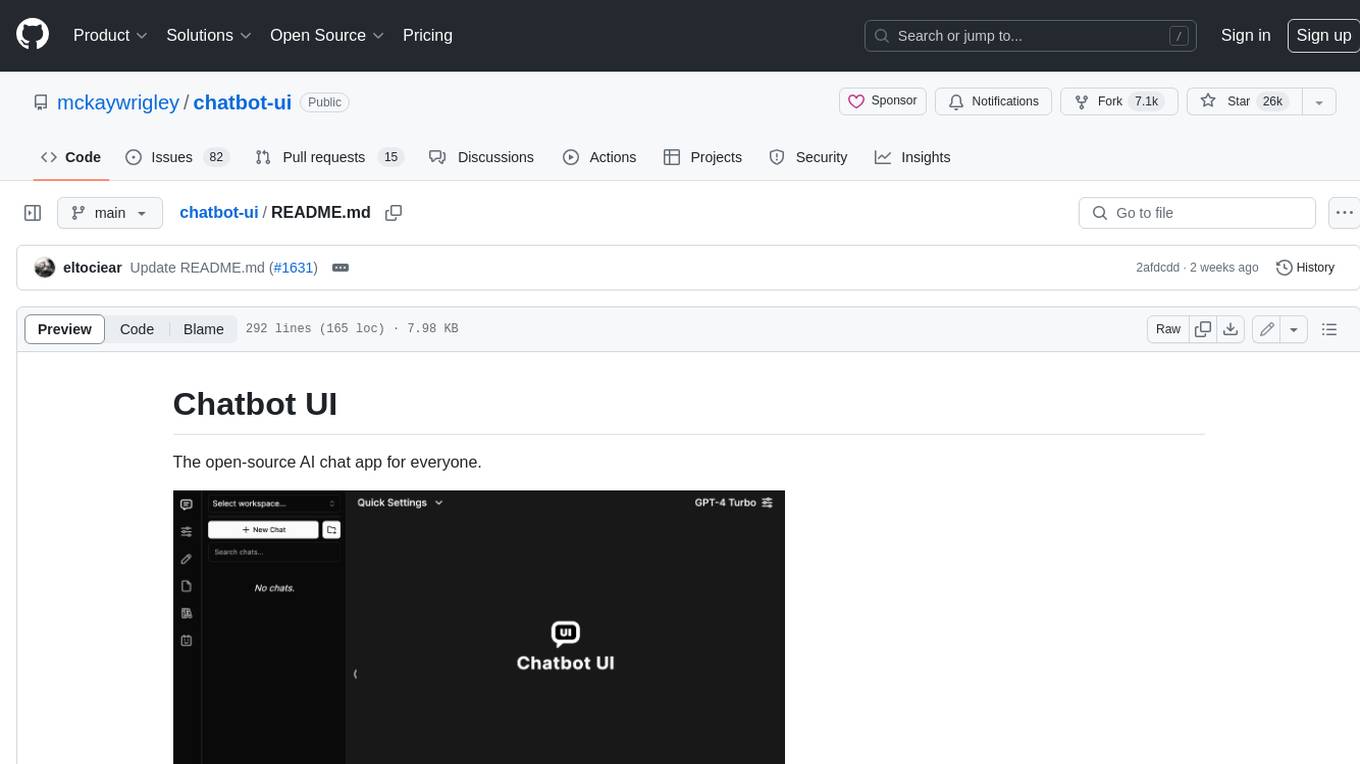
chatbot-ui
Chatbot UI is an open-source AI chat app that allows users to create and deploy their own AI chatbots. It is easy to use and can be customized to fit any need. Chatbot UI is perfect for businesses, developers, and anyone who wants to create a chatbot.
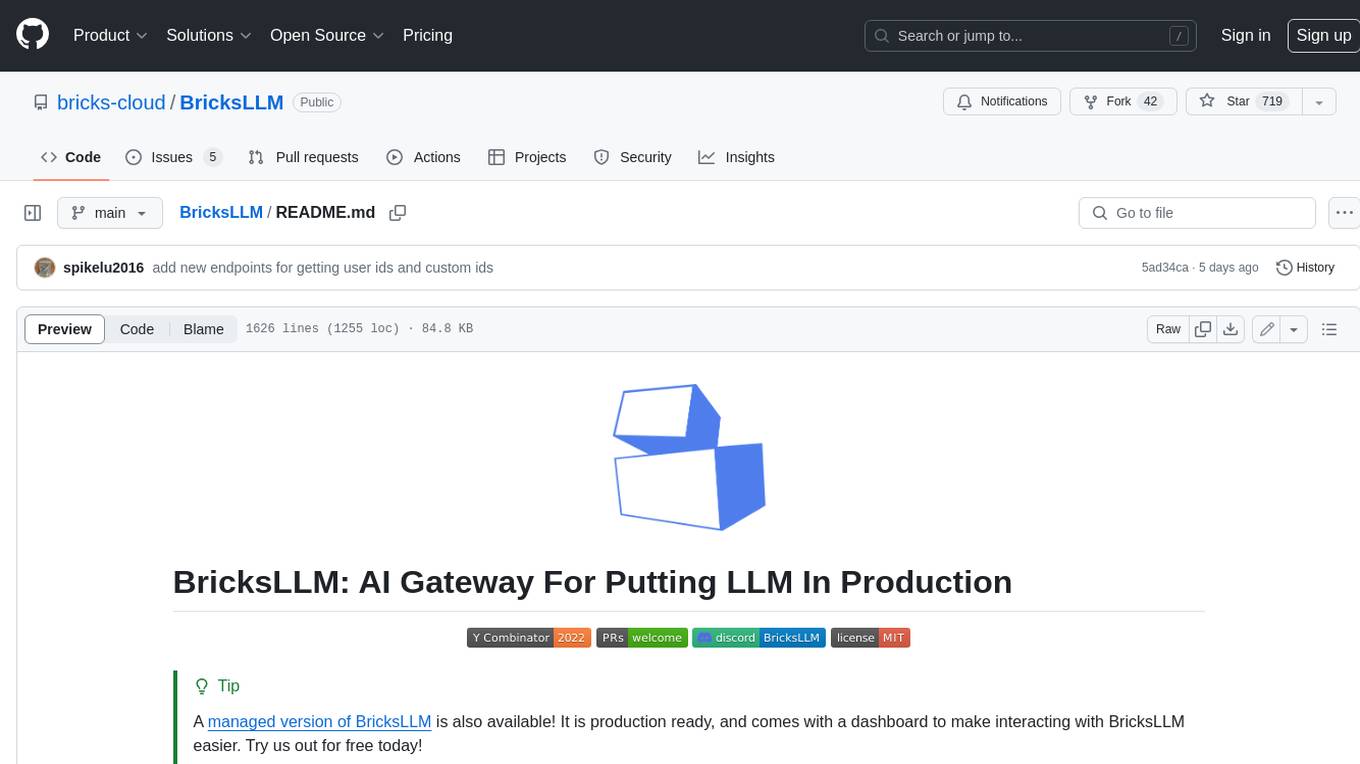
BricksLLM
BricksLLM is a cloud native AI gateway written in Go. Currently, it provides native support for OpenAI, Anthropic, Azure OpenAI and vLLM. BricksLLM aims to provide enterprise level infrastructure that can power any LLM production use cases. Here are some use cases for BricksLLM: * Set LLM usage limits for users on different pricing tiers * Track LLM usage on a per user and per organization basis * Block or redact requests containing PIIs * Improve LLM reliability with failovers, retries and caching * Distribute API keys with rate limits and cost limits for internal development/production use cases * Distribute API keys with rate limits and cost limits for students
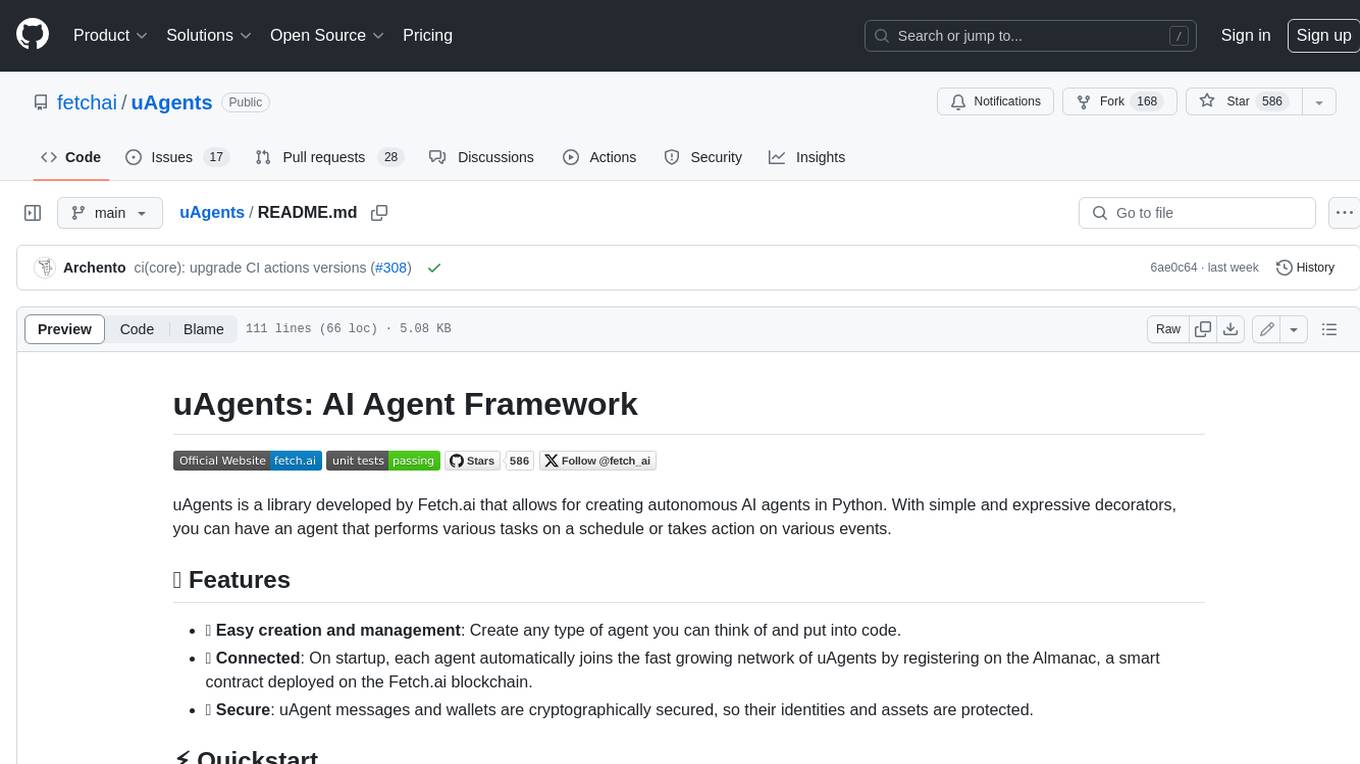
uAgents
uAgents is a Python library developed by Fetch.ai that allows for the creation of autonomous AI agents. These agents can perform various tasks on a schedule or take action on various events. uAgents are easy to create and manage, and they are connected to a fast-growing network of other uAgents. They are also secure, with cryptographically secured messages and wallets.
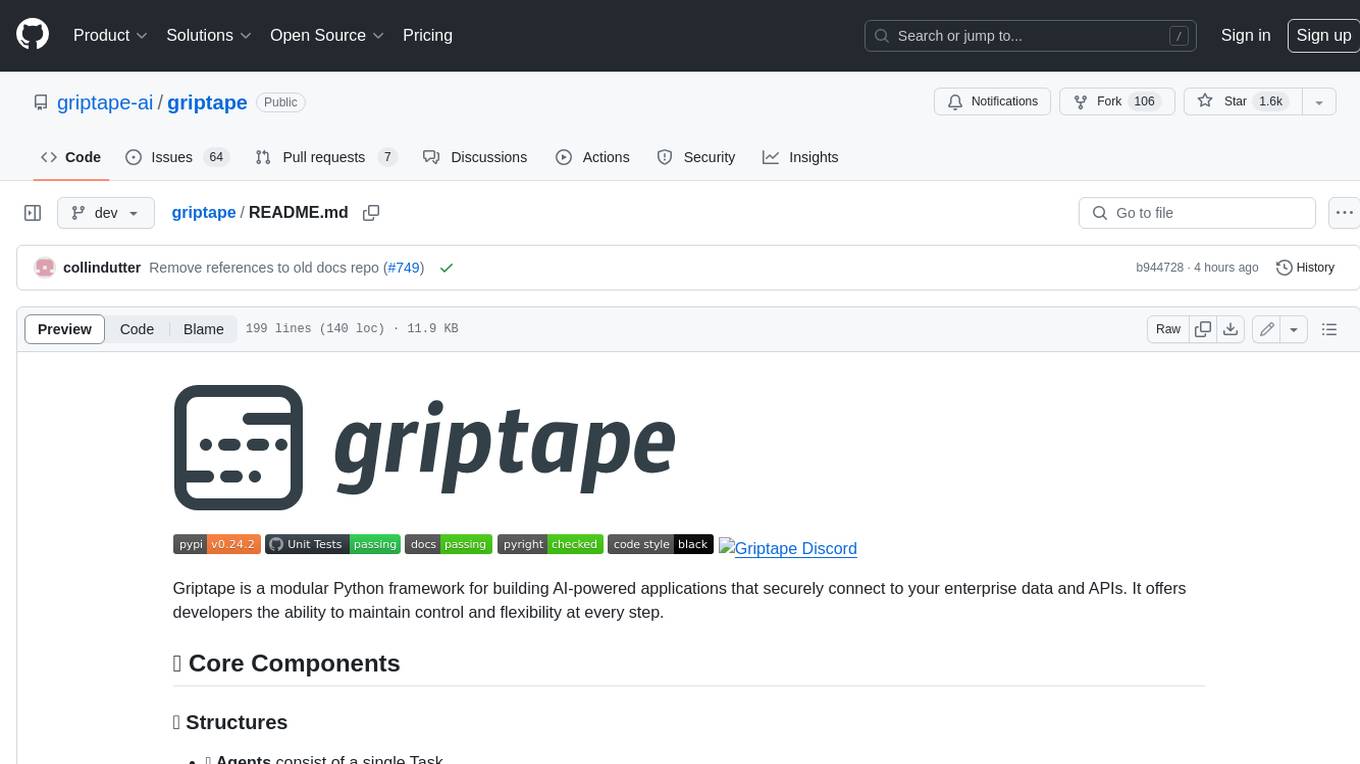
griptape
Griptape is a modular Python framework for building AI-powered applications that securely connect to your enterprise data and APIs. It offers developers the ability to maintain control and flexibility at every step. Griptape's core components include Structures (Agents, Pipelines, and Workflows), Tasks, Tools, Memory (Conversation Memory, Task Memory, and Meta Memory), Drivers (Prompt and Embedding Drivers, Vector Store Drivers, Image Generation Drivers, Image Query Drivers, SQL Drivers, Web Scraper Drivers, and Conversation Memory Drivers), Engines (Query Engines, Extraction Engines, Summary Engines, Image Generation Engines, and Image Query Engines), and additional components (Rulesets, Loaders, Artifacts, Chunkers, and Tokenizers). Griptape enables developers to create AI-powered applications with ease and efficiency.