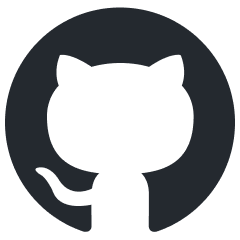
Awesome-LLM-Survey
An Awesome Collection for LLM Survey
Stars: 223
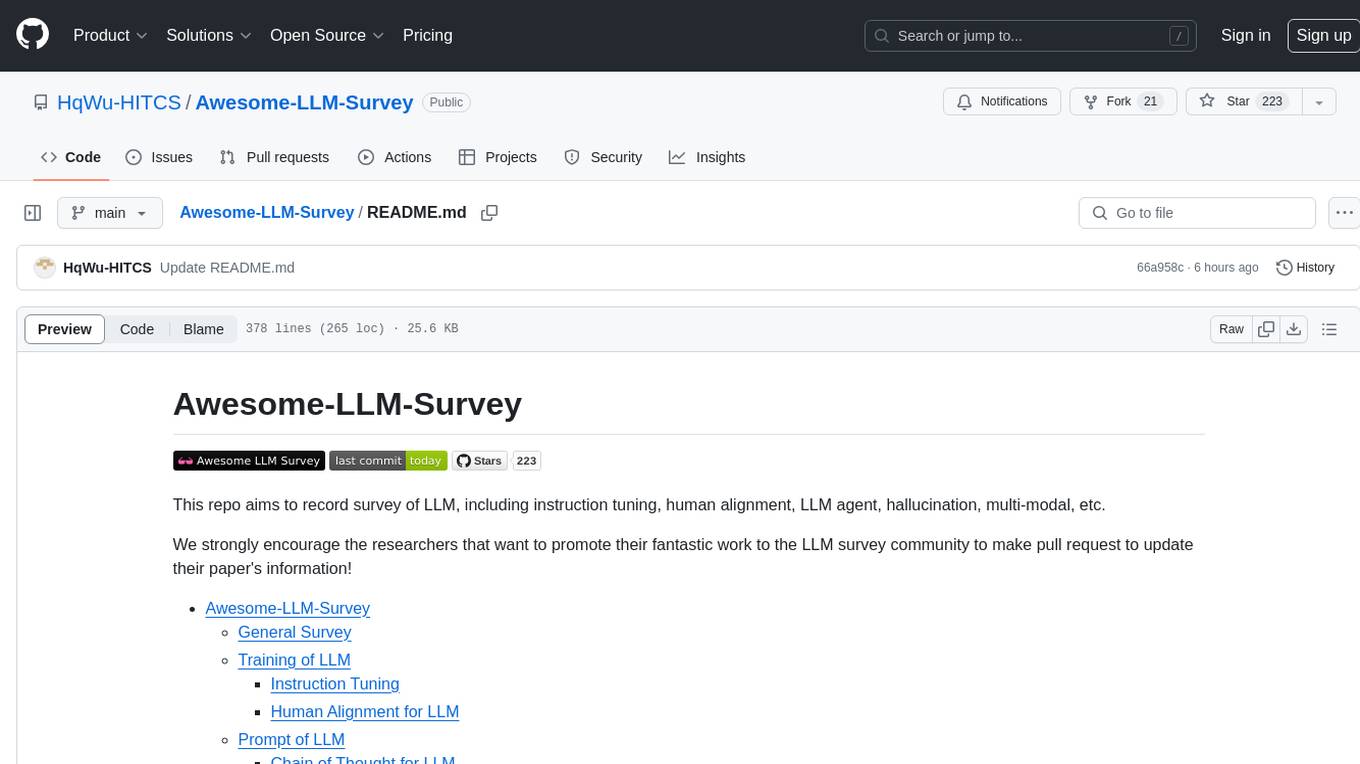
This repository, Awesome-LLM-Survey, serves as a comprehensive collection of surveys related to Large Language Models (LLM). It covers various aspects of LLM, including instruction tuning, human alignment, LLM agents, hallucination, multi-modal capabilities, and more. Researchers are encouraged to contribute by updating information on their papers to benefit the LLM survey community.
README:
This repo aims to record survey of LLM, including instruction tuning, human alignment, LLM agent, hallucination, multi-modal, etc.
We strongly encourage the researchers that want to promote their fantastic work to the LLM survey community to make pull request to update their paper's information!
-
Awesome-LLM-Survey
- General Survey
- Training of LLM
- Prompt of LLM
-
Challenge of LLM
- Hallucination in LLM
- Compression for LLM
- Evaluation of LLM
- Reasoning with LLM
- Explainability for LLM
- Fairness in LLM
- Graph for LLM
- Long-Context for LLM
- Factuality in LLM
- Knowledge for LLM
- Self-Correction for LLM
- Attributions for LLM
- Tool Using of LLM
- Calibration of LLM
- Agent of LLM
- Vulnerabilities of LLM
- Efficiency of LLM
- Data of LLM
- Security and Privacy of LLM
- Continual Learning of LLM
- Mulitmodal of LLM
- LLM for Domain Application
-
LLM for Downstream Tasks
- LLM for Recommendation
- LLM for Information Retrieval
- LLM for Software Engineering
- LLM for Autonomous Driving
- LLM for Time Series
- Detection of LLMs-Generated Content
- LLM for Society
- LLM for Citation
- LLM for Text Watermarking
- LLM for Math
- LLM for Environmental Disciplines
- LLM for Information Extraction
- LLM for Data Annotation
- LLM for Game
- Star History
-
A Survey of GPT-3 Family Large Language Models Including ChatGPT and GPT-4, 2023.10 [paper]
-
Challenges and Applications of Large Language Models, 2023.07 [paper]
-
Harnessing the Power of LLMs in Practice: A Survey on ChatGPT and Beyond, 2023.04 [paper][project]
-
A Comprehensive Survey on Pretrained Foundation Models: A History from BERT to ChatGPT, 2023.02 [paper]
-
Large language models: a comprehensive survey of its applications, challenges, limitations, and future prospects, 2023.12 [paper] [project]
-
The future of gpt: A taxonomy of existing chatgpt research, current challenges, and possible future directions, 2023.04 [paper]
-
A Review on Large Language Models: Architectures, Applications, Taxonomies, Open Issues and Challenges, 2023.10 [paper]
-
Understanding LLMs: A Comprehensive Overview from Training to Inference, 2024.01 [paper]
-
Are Prompts All the Story? No. A Comprehensive and Broader View of Instruction Learning, 2023.03 [paper] [project]
-
Vision-Language Instruction Tuning: A Review and Analysis, 2023,11 [paper][project]
-
Instruction Tuning for Large Language Models: A Survey, 2023.08 [paper]
-
A Survey on Data Selection for LLM Instruction Tuning, 2024.02 [paper]
-
AI Alignment: A Comprehensive Survey, 2023.10 [paper][project]
-
Large Language Model Alignment: A Survey, 2023.09 [paper]
-
From Instructions to Intrinsic Human Values -- A Survey of Alignment Goals for Big Model, 2023.08 [paper][project]
-
Aligning Large Language Models with Human: A Survey, 2023.07 [paper][project]
-
Towards Better Chain-of-Thought Prompting Strategies: A Survey, 2023.10 [paper]
-
A Survey of Chain of Thought Reasoning: Advances, Frontiers and Future, 2023.09 [paper][project]
-
Igniting Language Intelligence: The Hitchhiker's Guide From Chain-of-Thought Reasoning to Language Agents, 2023.11 [paper] [project]
-
Prompting Frameworks for Large Language Models: A Survey, 2023.11 [paper][project]
-
Unleashing the potential of prompt engineering in Large Language Models: a comprehensive review, 2023.10 [paper]
-
Towards Better Chain-of-Thought Prompting Strategies: A Survey, 2023.10 [paper]
- A Survey on Retrieval-Augmented Text Generation, 2022.02 [paper]
- Retrieval-Augmented Generation for Large Language Models: A Survey, 2023.12 [paper] [project]
- RAG and RAU: A Survey on Retrieval-Augmented Language Model in Natural Language Processing, 2024.04 [paper]
-
Can Knowledge Graphs Reduce Hallucinations in LLMs? : A Survey, 2023.11 [paper]
-
A Survey on Hallucination in Large Language Models: Principles, Taxonomy, Challenges, and Open Questions, 2023.11 [paper][project]
-
A Survey of Hallucination in “Large” Foundation Models, 2023.09 [paper][project]
-
Siren's Song in the AI Ocean: A Survey on Hallucination in Large Language Models, 2023.09 [paper][project]
-
Cognitive Mirage: A Review of Hallucinations in Large Language Models, 2023.09 [paper][project]
-
Augmenting LLMs with Knowledge: A survey on hallucination prevention, 2023.09 [paper]
-
A Comprehensive Survey of Hallucination Mitigation Techniques in Large Language Models, 2024.01 [paper]
-
Trustworthy LLMs: a Survey and Guideline for Evaluating Large Language Models' Alignment, 2023.08 [paper]
-
Hallucination of Multimodal Large Language Models: A Survey, 2024.04 [paper]
- A Survey on Model Compression for Large Language Models, 2023.08 [paper]
- A Comprehensive Survey of Compression Algorithms for Language Models, 2024.01 [paper]
-
Evaluating Large Language Models: A Comprehensive Survey, 2023.10 [paper][project]
-
A Survey on Evaluation of Large Language Models, 2023.07 [paper][project]
-
Reasoning with Language Model Prompting: A Survey, 2022.12 [paper][project]
-
A Survey of Reasoning with Foundation Models, 2023.12 [papaer][project]
- Explainability for Large Language Models: A Survey, 2023.09 [paper]
- The Mystery and Fascination of LLMs: A Comprehensive Survey on the Interpretation and Analysis of Emergent Abilitie, 2023.11 [paper]
- If LLM Is the Wizard, Then Code Is the Wand: A Survey on How Code Empowers Large Language Models to Serve as Intelligent Agents, 2024.01 [paper]
- From Understanding to Utilization: A Survey on Explainability for Large Language Models, 2024.01 [paper]
- A Survey on Fairness in Large Language Models, 2023.08 [paper]
- A Survey of Graph Meets Large Language Model: Progress and Future Directions, 2023.11 [paper]
- Large Language Models on Graphs: A Comprehensive Survey, 2023.12 [paper] [project]
- Advancing Transformer Architecture in Long-Context Large Language Models: A Comprehensive Survey, 2023.11 [paper]
- Length Extrapolation of Transformers: A Survey from the Perspective of Position Encoding, 2023.12 [paper]
-
A Survey on Factuality in Large Language Models: Knowledge, Retrieval and Domain-Specificity, 2023.10 [paper][project]
-
Give Me the Facts! A Survey on Factual Knowledge Probing in Pre-trained Language Models, 2023.10 [paper]
-
A Survey on Knowledge Distillation of Large Language Models, 2024.02 [paper]
-
Knowledge Unlearning for LLMs: Tasks, Methods, and Challenges, 2023.11 [paper]
-
Trends in Integration of Knowledge and Large Language Models: A Survey and Taxonomy of Methods, Benchmarks, and Applications, 2023.11 [paper]
-
Knowledge Editing for Large Language Models: A Survey, 2023.10 [paper]
-
Editing Large Language Models: Problems, Methods, and Opportunities, 2023.05 [paper][project]
-
Building trust in conversational ai: A comprehensive review and solution architecture for explainable, privacy-aware systems using llms and knowledge graph, 2023.08 [paper]
- Automatically Correcting Large Language Models: Surveying the landscape of diverse self-correction strategies, 2023.08 [paper][project]
-
Foundation Models for Decision Making: Problems, Methods, and Opportunities, 2023.03 [paper]
-
Augmented Language Models: a Survey, 2023.02 [paper]
- A Survey of Language Model Confidence Estimation and Calibration, 2023.11 [paper]
- A Survey on Large Language Model based Autonomous Agents, 2023.08 [paper][project]
- The Rise and Potential of Large Language Model Based Agents: A Survey, 2023.09 [paper][project]
- Large Language Models Empowered Agent-based Modeling and Simulation: A Survey and Perspectives, 2023.12 [paper]
- Large Multimodal Agents: A Survey, 2024.02 [paper][project]
- Survey of Vulnerabilities in Large Language Models Revealed by Adversarial Attacks, 2023.10 [paper]
-
The Efficiency Spectrum of Large Language Models: An Algorithmic Survey, 2023.12 [paper][project]
-
Efficient Large Language Models: A Survey, 2023.12 [paper][project]
-
Parameter-Efficient Fine-Tuning Methods for Pretrained Language Models: A Critical Review and Assessment, 2023.12 [paper]
-
A Survey on Hardware Accelerators for Large Language Models, 2024.01 [paper]
-
Model Compression and Efficient Inference for Large Language Models: A Survey, 2024.02 [paper]
- Data Management For Large Language Models: A Survey, 2023.12 [paper][project]
- A Survey on Data Selection for Language Models, 2024.02 [paper]
- Datasets for Large Language Models: A Comprehensive Survey, 2024.02 [paper][project]
- A Survey on Large Language Model (LLM) Security and Privacy: The Good, the Bad, and the Ugly, 2023.12 [paper]
- Continual Learning with Pre-Trained Models: A Survey, 2024.01 [paper] [project]
- Continual Learning of Large Language Models: A Comprehensive Survey, 2024.04 [paper]
-
The (R)Evolution of Multimodal Large Language Models: A Survey, 2024,02 [paper]
-
Vision-Language Instruction Tuning: A Review and Analysis, 2023,11 [paper][project]
-
How to Bridge the Gap between Modalities: A Comprehensive Survey on Multimodal Large Language Model, 2023.11 [paper]
-
A Survey on Multimodal Large Language Models, 2023.06 [paper][project]
-
Multimodal Large Language Models: A Survey, 2023.11 [paper]
-
Large Language Models Meet Computer Vision: A Brief Survey, 2023.11 [paper]
-
Foundational Models Defining a New Era in Vision: A Survey and Outlook, 2023.07 [paper][project]
-
Video Understanding with Large Language Models: A Survey, 2023.12 [paper] [project]
- A Survey of Neural Code Intelligence: Paradigms, Advances and Beyond, 2024.03 [paper][project]
- A Survey on Language Models for Code, 2023.11 [paper][project]
- Pitfalls in Language Models for Code Intelligence: A Taxonomy and Survey, 2023.10 [paper][project]
- Large Language Models Meet NL2Code: A Survey, 2022.12 [paper]
- A Prompt Learning Framework for Source Code Summarization, 2023.12 [paper]
- If LLM Is the Wizard, Then Code Is the Wand: A Survey on How Code Empowers Large Language Models to Serve as Intelligent Agents, 2024.01 [paper]
-
A Survey of Large Language Models in Medicine: Progress, Application, and Challenge, 2023.11 [paper][project]
-
Large Language Models Illuminate a Progressive Pathway to Artificial Healthcare Assistant: A Review, 2023.10 [paper][project]
-
Large AI Models in Health Informatics: Applications, Challenges, and the Future, 2023.03 [paper][project]
-
A SWOT (Strengths, Weaknesses, Opportunities, and Threats) Analysis of ChatGPT in the Medical Literature: Concise Review, 2023.11 [paper]
-
ChatGPT in Healthcare: A Taxonomy and Systematic Review, 2023.03 [paper]
- Large Language Models in Finance: A Survey, 2023.09 [paper]
- ChatGPT and Beyond: The Generative AI Revolution in Education, 2023.11 [paper]
- Large Language Models in Law: A Survey, 2023.12 [paper]
- A review of the explainability and safety of conversational agents for mental health to identify avenues for improvement, 2023.10 [paper]
- Towards a Psychological Generalist AI: A Survey of Current Applications of Large Language Models and Future Prospects, 2023.12 [paper]
- Large Language Models in Mental Health Care: a Scoping Review, 2024.01 [paper]
- Large Language Models for Robotics: A Survey, 2023.11 [paper]
- Foundation Models for Recommender Systems: A Survey and New Perspectives, 2024.02 [paper]
- User Modeling in the Era of Large Language Models: Current Research and Future Directions, 2023.12 [paper][project]
- A Survey on Large Language Models for Personalized and Explainable Recommendations, 2023.11 [paper]
- Large Language Models for Generative Recommendation: A Survey and Visionary Discussions, 2023.09 [paper]
- A Survey on Large Language Models for Recommendation, 2023.08 [paper][project]
- How Can Recommender Systems Benefit from Large Language Models: A Survey, 2023.06 [paper][project]
- Large Language Models for Software Engineering: Survey and Open Problems, 2023.10 [paper]
- Large Language Models for Software Engineering: A Systematic Literature Review, 2023.08 [paper]
- A Survey on Multimodal Large Language Models for Autonomous Driving, 2023.11 [paper]
- A Survey of Large Language Models for Autonomous Driving, 2023.11 [paper][project]
- Large Models for Time Series and Spatio-Temporal Data: A Survey and Outlook, 2023.10 [paper][project]
- A Survey on Detection of LLMs-Generated Content, 2023.10 [paper][project]
- A Survey on LLM-generated Text Detection: Necessity, Methods, and Future Directions, 2023.10 [paper] [project]
- Detecting ChatGPT: A Survey of the State of Detecting ChatGPT-Generated Text, 2023.09 [paper]
- Large Language Models as Subpopulation Representative Models: A Review, 2023.10 [paper]
- When Large Language Models Meet Citation: A Survey, 2023.09 [paper]
- A Survey of Text Watermarking in the Era of Large Language Models, 2023.12 [paper]
- Mathematical Language Models: A Survey, 2023.12 [paper]
- Recent applications of AI to environmental disciplines: A review, 2023.10 [paper]
- Opportunities and Challenges of Applying Large Language Models in Building Energy Efficiency and Decarbonization Studies: An Exploratory Overview, 2023.12 [paper]
- Large Language Models and Games: A Survey and Roadmap, 2024.02 [paper]
- A Survey on Game Playing Agents and Large Models: Methods, Applications, and Challenges, 2024.03 [paper] [project]
For Tasks:
Click tags to check more tools for each tasksFor Jobs:
Alternative AI tools for Awesome-LLM-Survey
Similar Open Source Tools
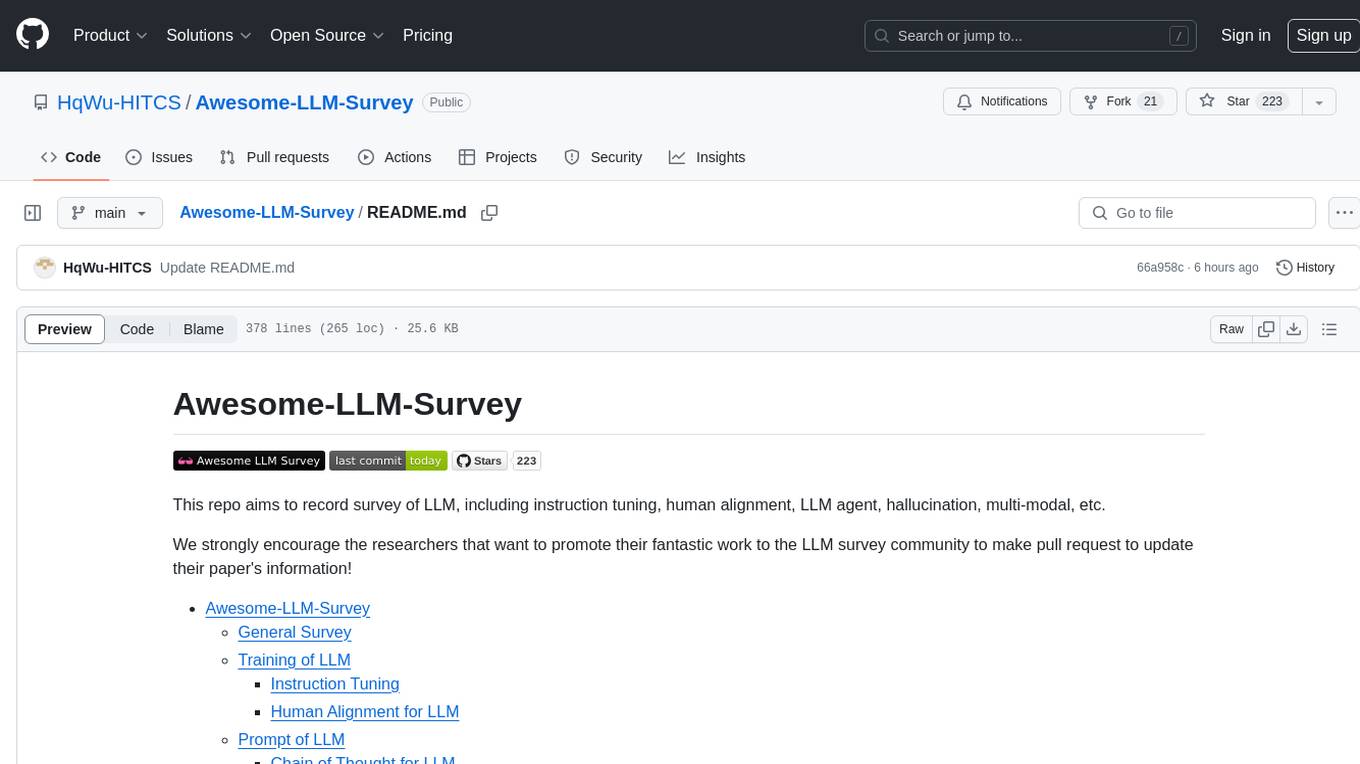
Awesome-LLM-Survey
This repository, Awesome-LLM-Survey, serves as a comprehensive collection of surveys related to Large Language Models (LLM). It covers various aspects of LLM, including instruction tuning, human alignment, LLM agents, hallucination, multi-modal capabilities, and more. Researchers are encouraged to contribute by updating information on their papers to benefit the LLM survey community.
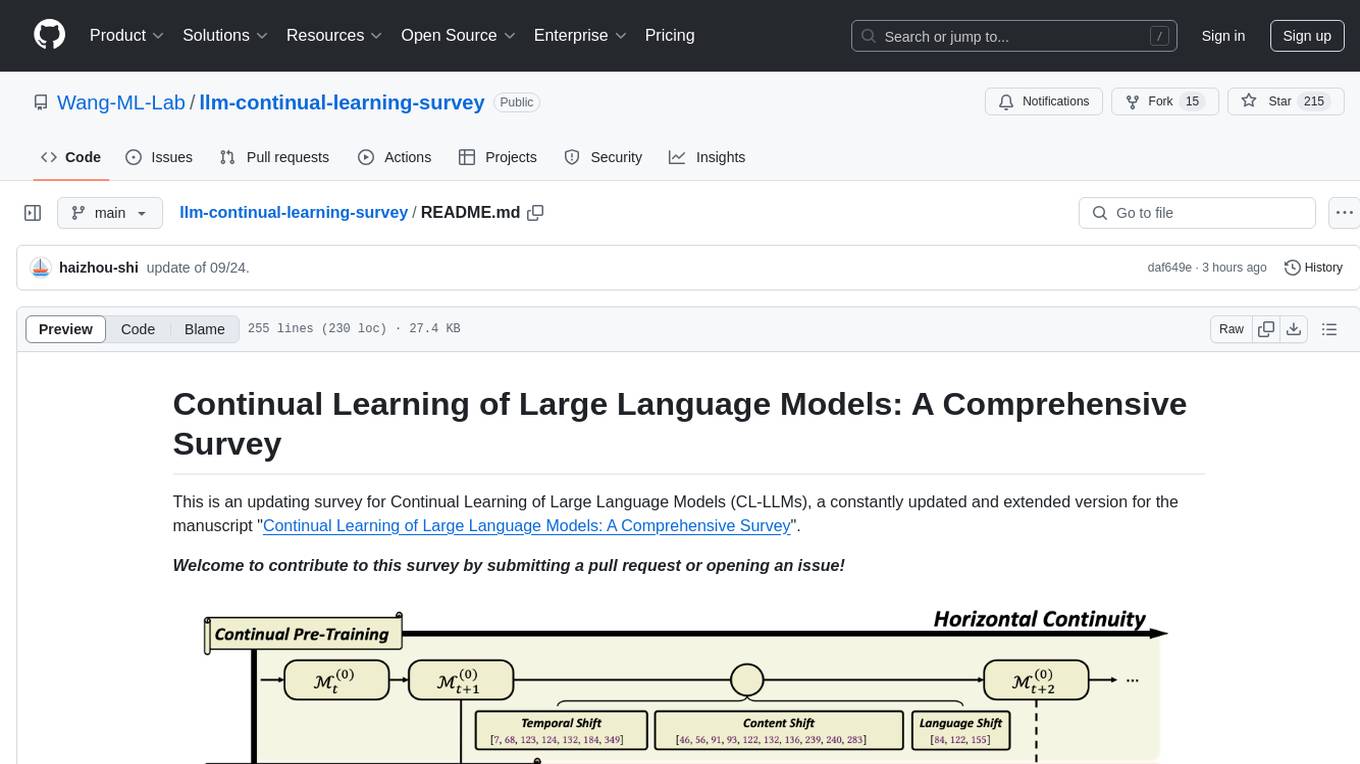
llm-continual-learning-survey
This repository is an updating survey for Continual Learning of Large Language Models (CL-LLMs), providing a comprehensive overview of various aspects related to the continual learning of large language models. It covers topics such as continual pre-training, domain-adaptive pre-training, continual fine-tuning, model refinement, model alignment, multimodal LLMs, and miscellaneous aspects. The survey includes a collection of relevant papers, each focusing on different areas within the field of continual learning of large language models.
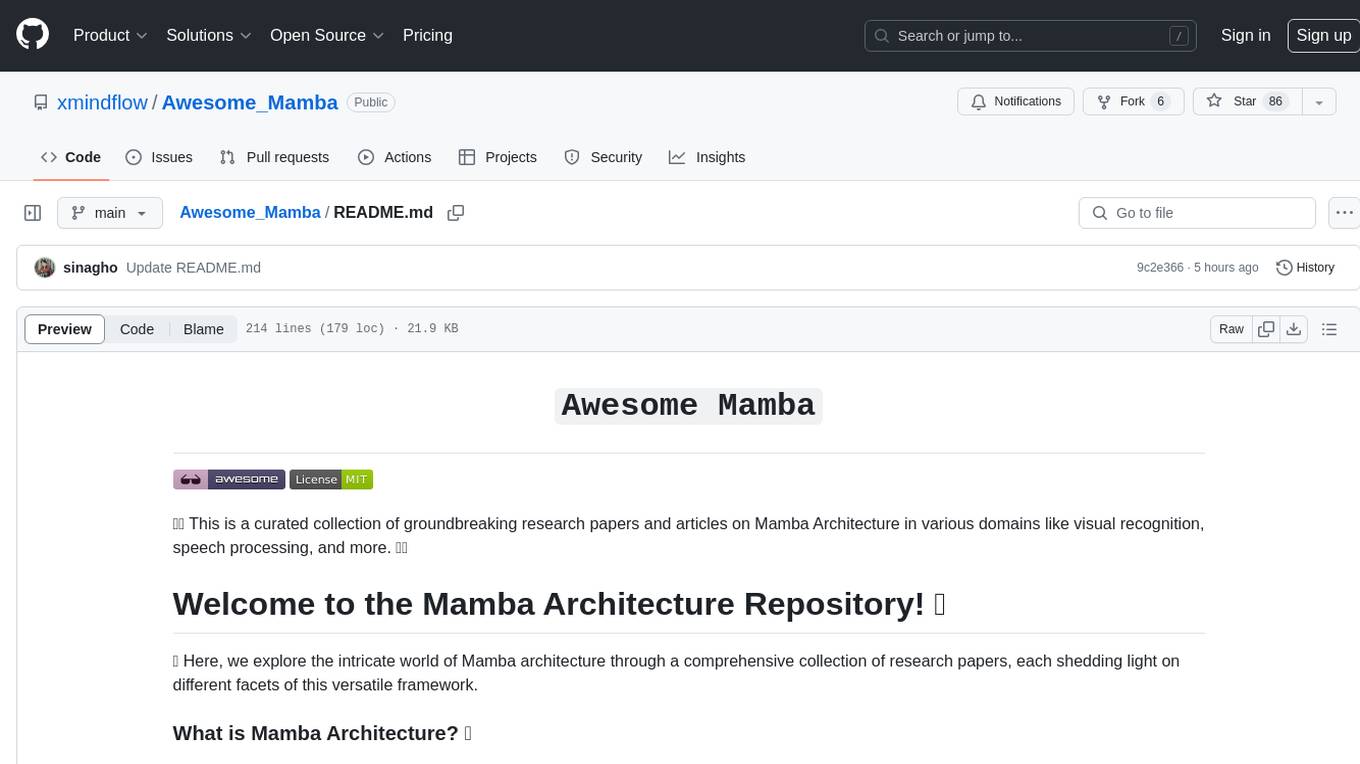
Awesome_Mamba
Awesome Mamba is a curated collection of groundbreaking research papers and articles on Mamba Architecture, a pioneering framework in deep learning known for its selective state spaces and efficiency in processing complex data structures. The repository offers a comprehensive exploration of Mamba architecture through categorized research papers covering various domains like visual recognition, speech processing, remote sensing, video processing, activity recognition, image enhancement, medical imaging, reinforcement learning, natural language processing, 3D recognition, multi-modal understanding, time series analysis, graph neural networks, point cloud analysis, and tabular data handling.
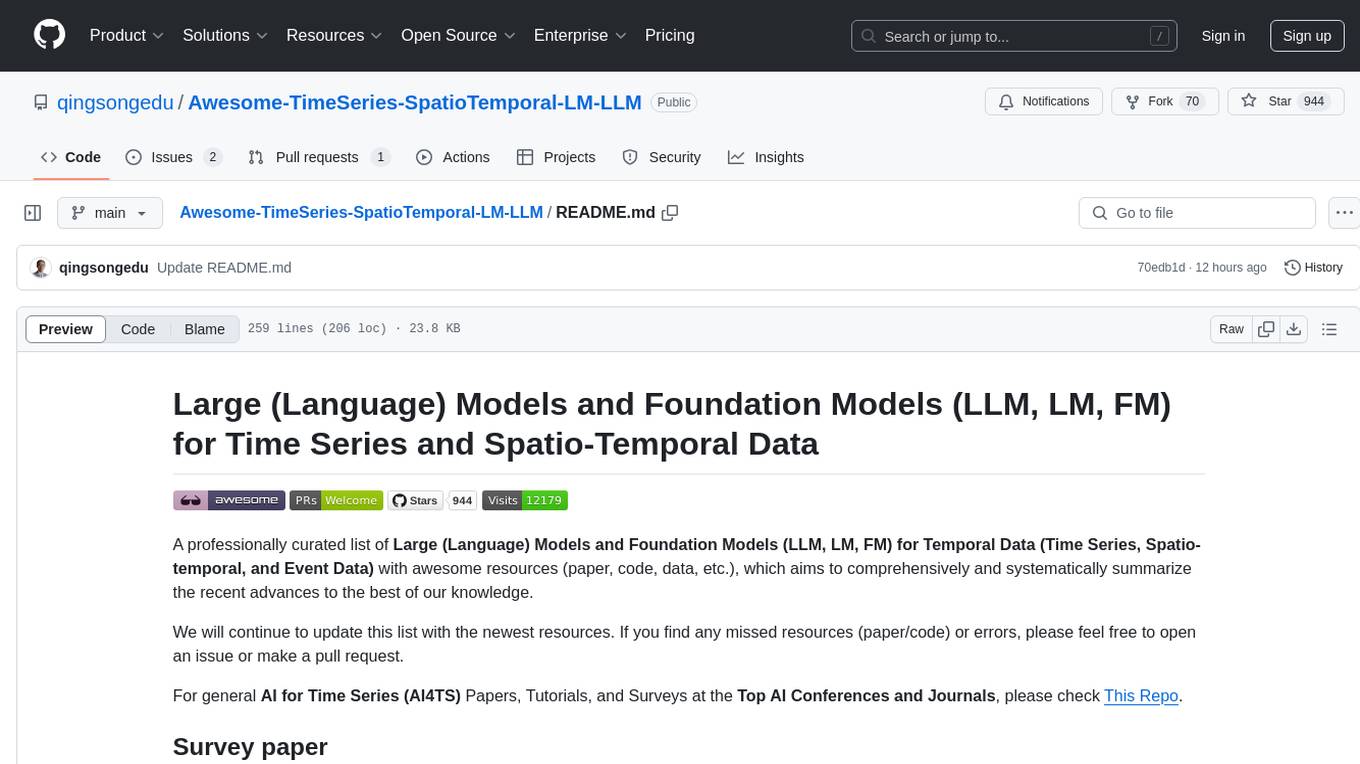
Awesome-TimeSeries-SpatioTemporal-LM-LLM
Awesome-TimeSeries-SpatioTemporal-LM-LLM is a curated list of Large (Language) Models and Foundation Models for Temporal Data, including Time Series, Spatio-temporal, and Event Data. The repository aims to summarize recent advances in Large Models and Foundation Models for Time Series and Spatio-Temporal Data with resources such as papers, code, and data. It covers various applications like General Time Series Analysis, Transportation, Finance, Healthcare, Event Analysis, Climate, Video Data, and more. The repository also includes related resources, surveys, and papers on Large Language Models, Foundation Models, and their applications in AIOps.
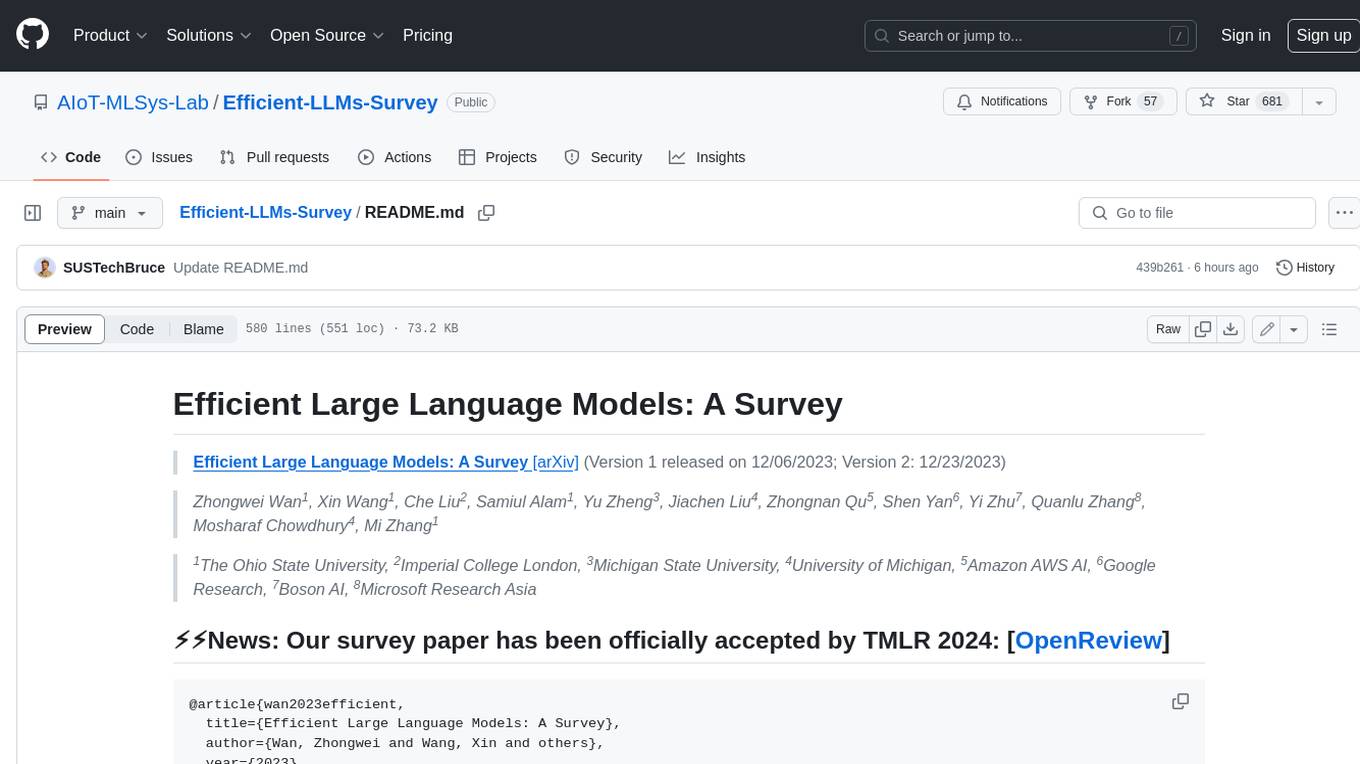
Efficient-LLMs-Survey
This repository provides a systematic and comprehensive review of efficient LLMs research. We organize the literature in a taxonomy consisting of three main categories, covering distinct yet interconnected efficient LLMs topics from **model-centric** , **data-centric** , and **framework-centric** perspective, respectively. We hope our survey and this GitHub repository can serve as valuable resources to help researchers and practitioners gain a systematic understanding of the research developments in efficient LLMs and inspire them to contribute to this important and exciting field.
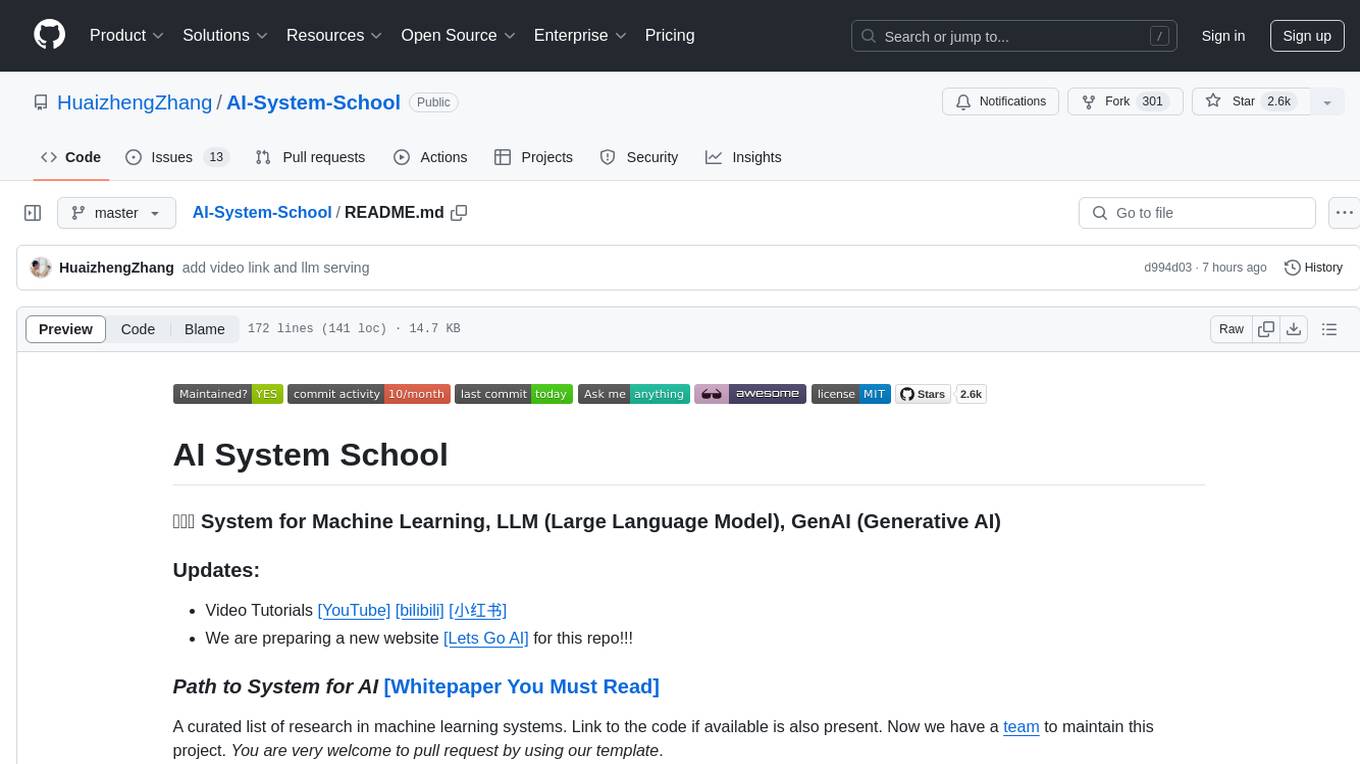
AI-System-School
AI System School is a curated list of research in machine learning systems, focusing on ML/DL infra, LLM infra, domain-specific infra, ML/LLM conferences, and general resources. It provides resources such as data processing, training systems, video systems, autoML systems, and more. The repository aims to help users navigate the landscape of AI systems and machine learning infrastructure, offering insights into conferences, surveys, books, videos, courses, and blogs related to the field.
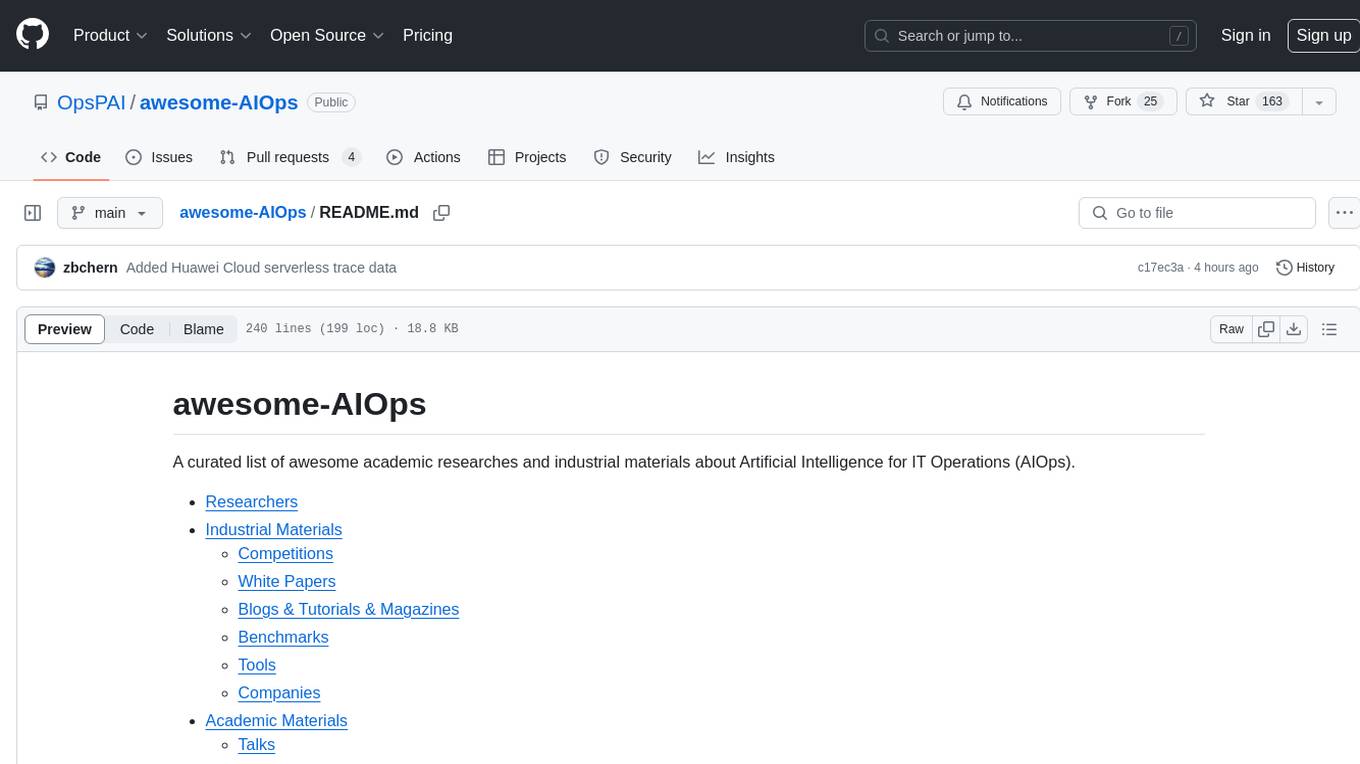
awesome-AIOps
awesome-AIOps is a curated list of academic researches and industrial materials related to Artificial Intelligence for IT Operations (AIOps). It includes resources such as competitions, white papers, blogs, tutorials, benchmarks, tools, companies, academic materials, talks, workshops, papers, and courses covering various aspects of AIOps like anomaly detection, root cause analysis, incident management, microservices, dependency tracing, and more.
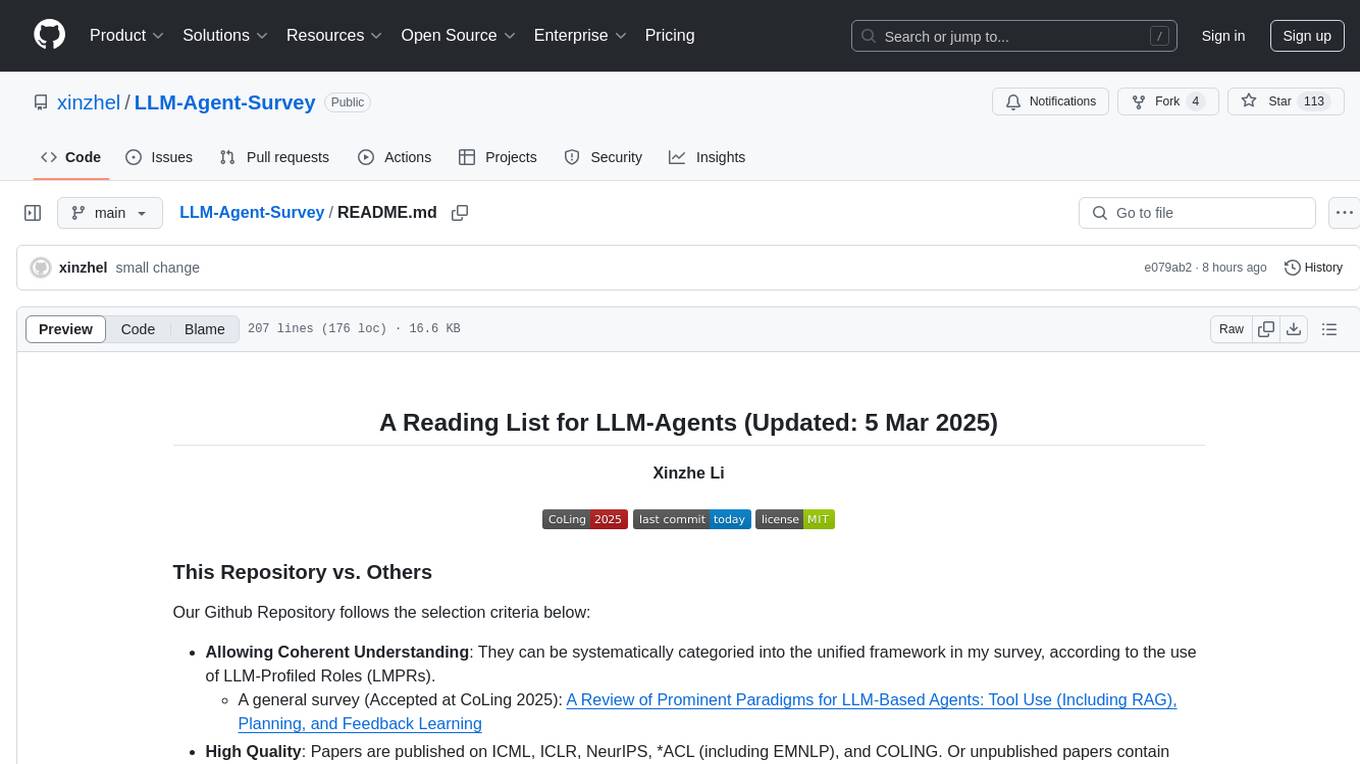
LLM-Agent-Survey
LLM-Agent-Survey is a comprehensive repository that provides a curated list of papers related to Large Language Model (LLM) agents. The repository categorizes papers based on LLM-Profiled Roles and includes high-quality publications from prestigious conferences and journals. It aims to offer a systematic understanding of LLM-based agents, covering topics such as tool use, planning, and feedback learning. The repository also includes unpublished papers with insightful analysis and novelty, marked for future updates. Users can explore a wide range of surveys, tool use cases, planning workflows, and benchmarks related to LLM agents.
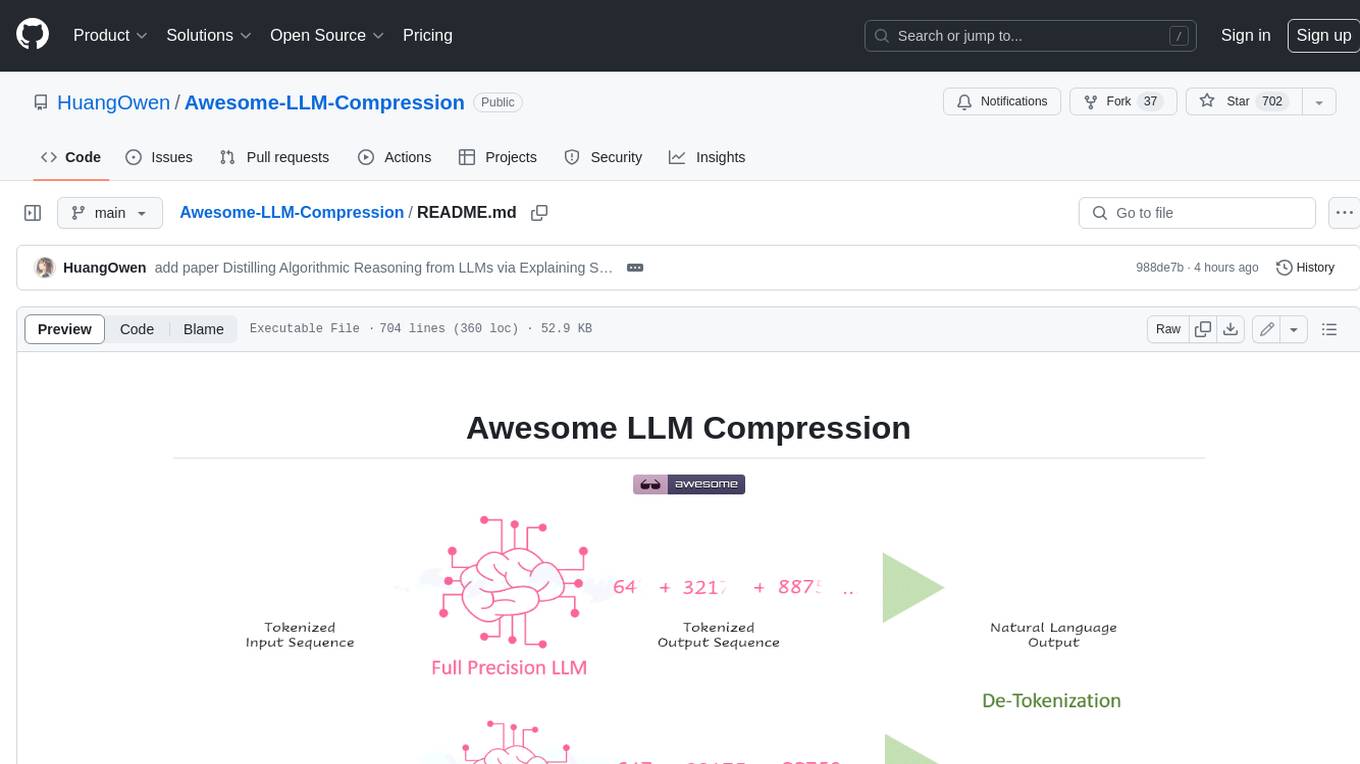
Awesome-LLM-Compression
Awesome LLM compression research papers and tools to accelerate LLM training and inference.
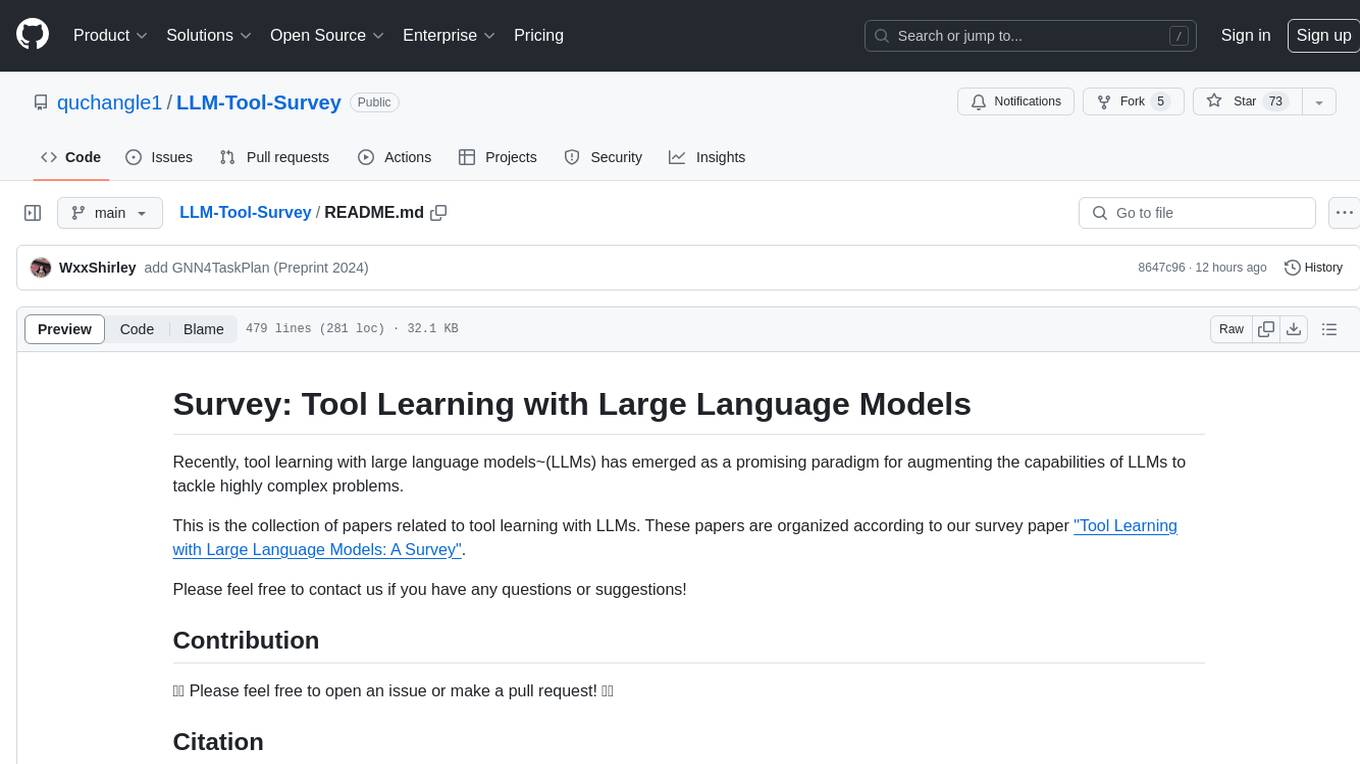
LLM-Tool-Survey
This repository contains a collection of papers related to tool learning with large language models (LLMs). The papers are organized according to the survey paper 'Tool Learning with Large Language Models: A Survey'. The survey focuses on the benefits and implementation of tool learning with LLMs, covering aspects such as task planning, tool selection, tool calling, response generation, benchmarks, evaluation, challenges, and future directions in the field. It aims to provide a comprehensive understanding of tool learning with LLMs and inspire further exploration in this emerging area.
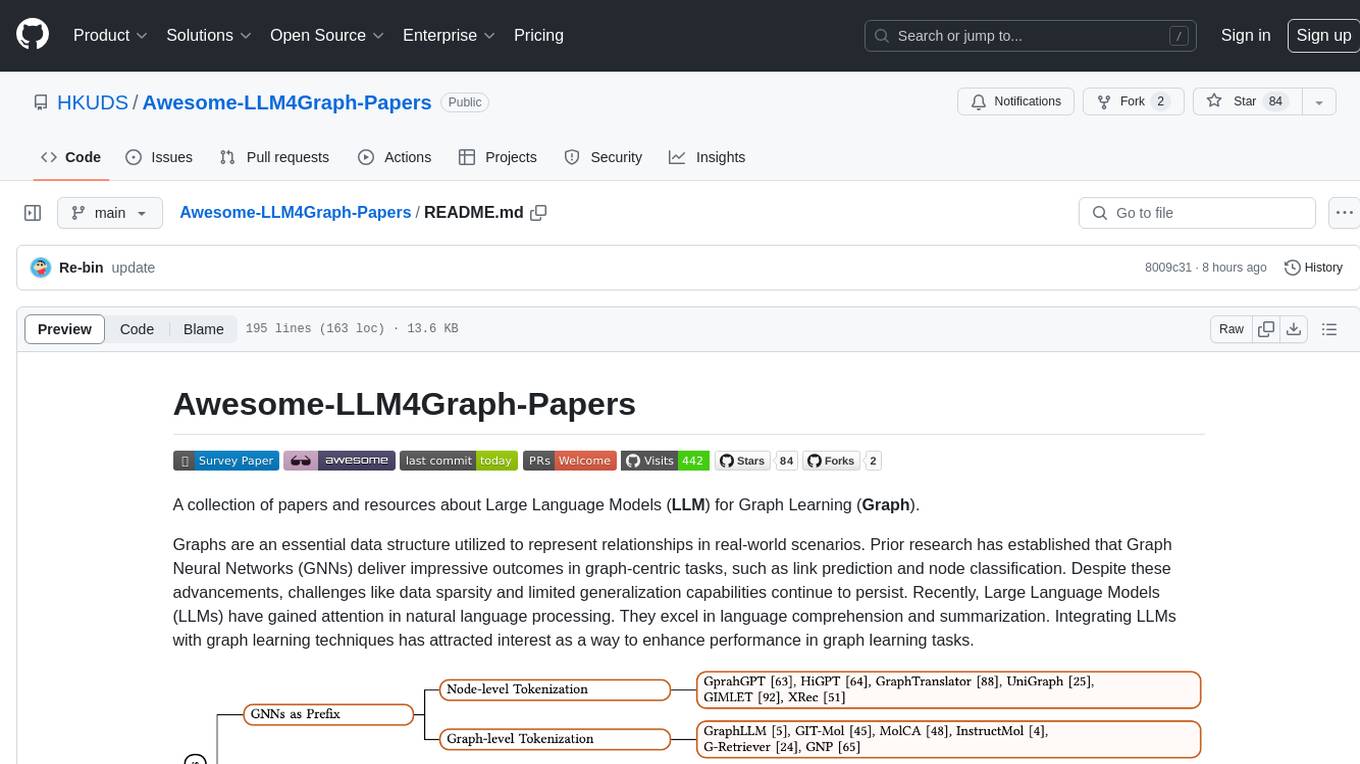
Awesome-LLM4Graph-Papers
A collection of papers and resources about Large Language Models (LLM) for Graph Learning (Graph). Integrating LLMs with graph learning techniques to enhance performance in graph learning tasks. Categorizes approaches based on four primary paradigms and nine secondary-level categories. Valuable for research or practice in self-supervised learning for recommendation systems.
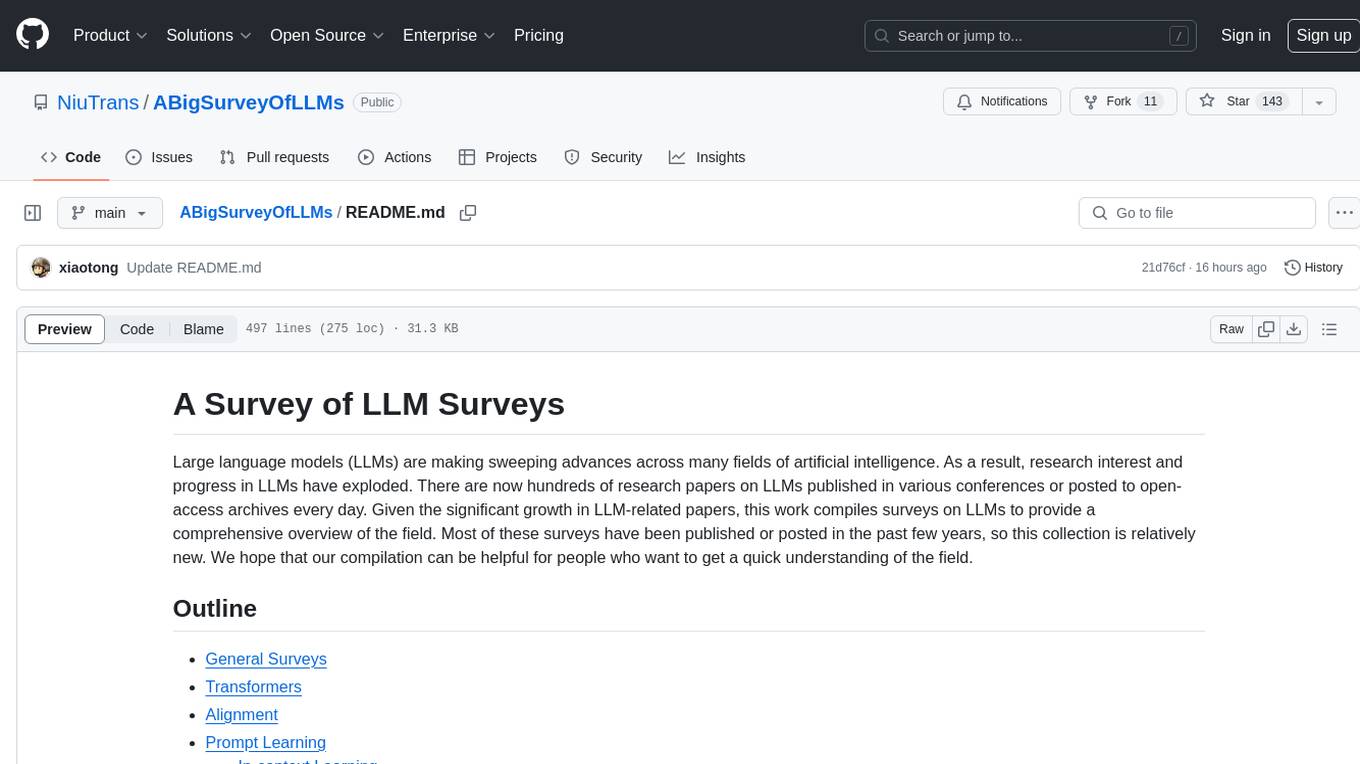
ABigSurveyOfLLMs
ABigSurveyOfLLMs is a repository that compiles surveys on Large Language Models (LLMs) to provide a comprehensive overview of the field. It includes surveys on various aspects of LLMs such as transformers, alignment, prompt learning, data management, evaluation, societal issues, safety, misinformation, attributes of LLMs, efficient LLMs, learning methods for LLMs, multimodal LLMs, knowledge-based LLMs, extension of LLMs, LLMs applications, and more. The repository aims to help individuals quickly understand the advancements and challenges in the field of LLMs through a collection of recent surveys and research papers.
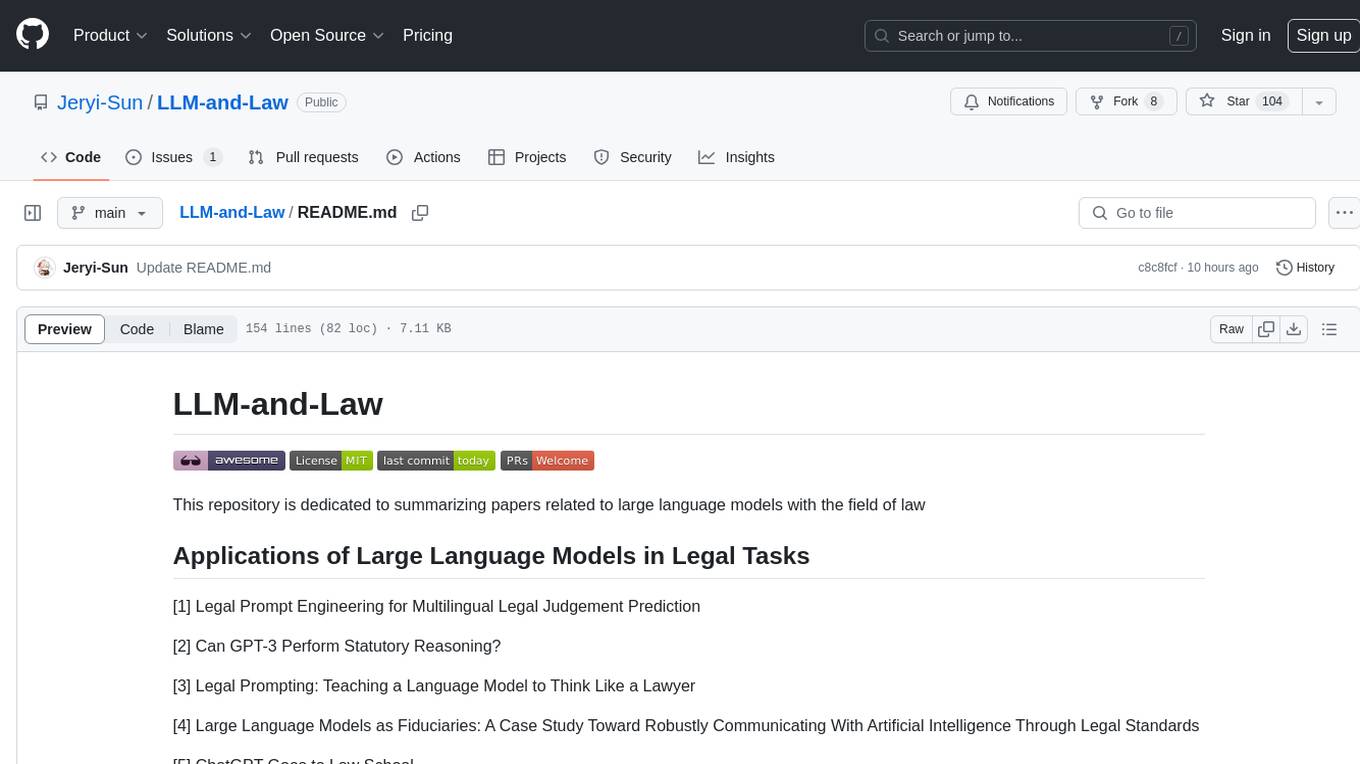
LLM-and-Law
This repository is dedicated to summarizing papers related to large language models with the field of law. It includes applications of large language models in legal tasks, legal agents, legal problems of large language models, data resources for large language models in law, law LLMs, and evaluation of large language models in the legal domain.
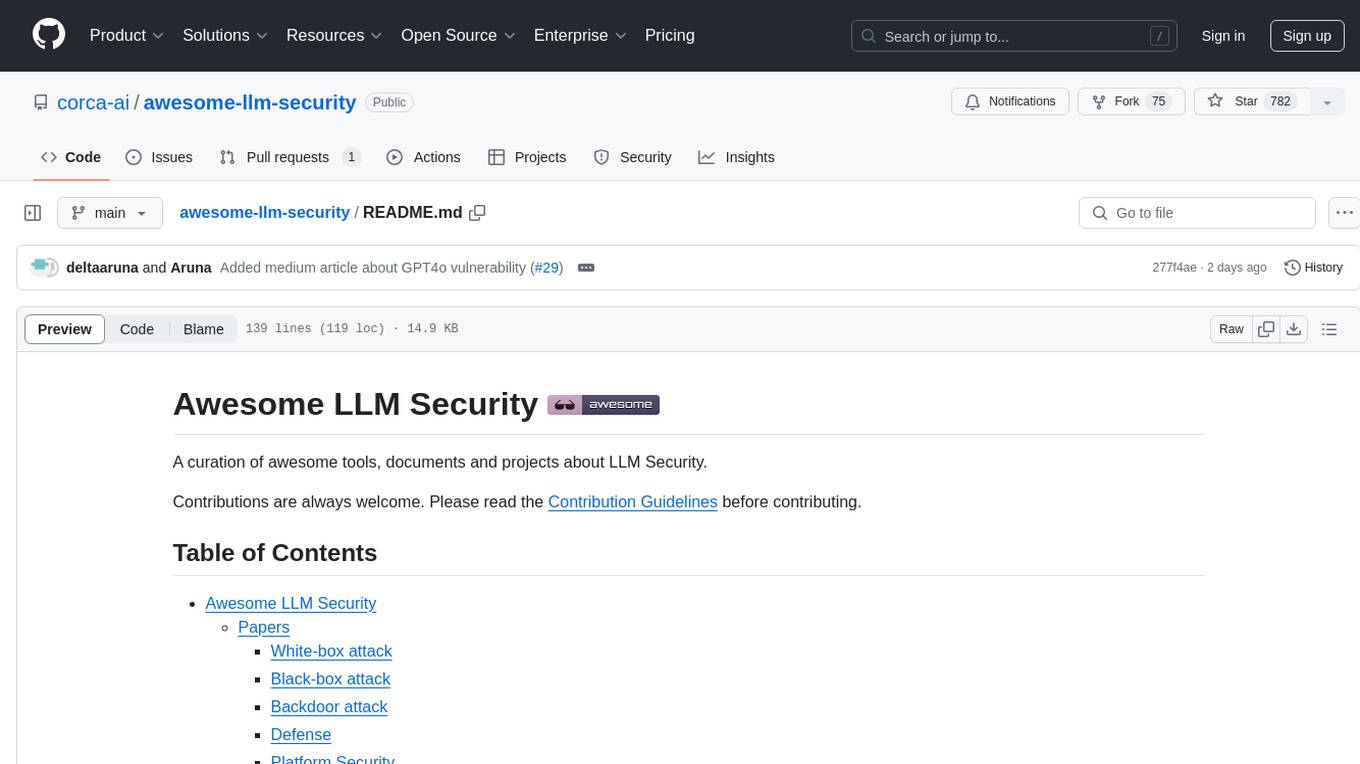
awesome-llm-security
Awesome LLM Security is a curated collection of tools, documents, and projects related to Large Language Model (LLM) security. It covers various aspects of LLM security including white-box, black-box, and backdoor attacks, defense mechanisms, platform security, and surveys. The repository provides resources for researchers and practitioners interested in understanding and safeguarding LLMs against adversarial attacks. It also includes a list of tools specifically designed for testing and enhancing LLM security.
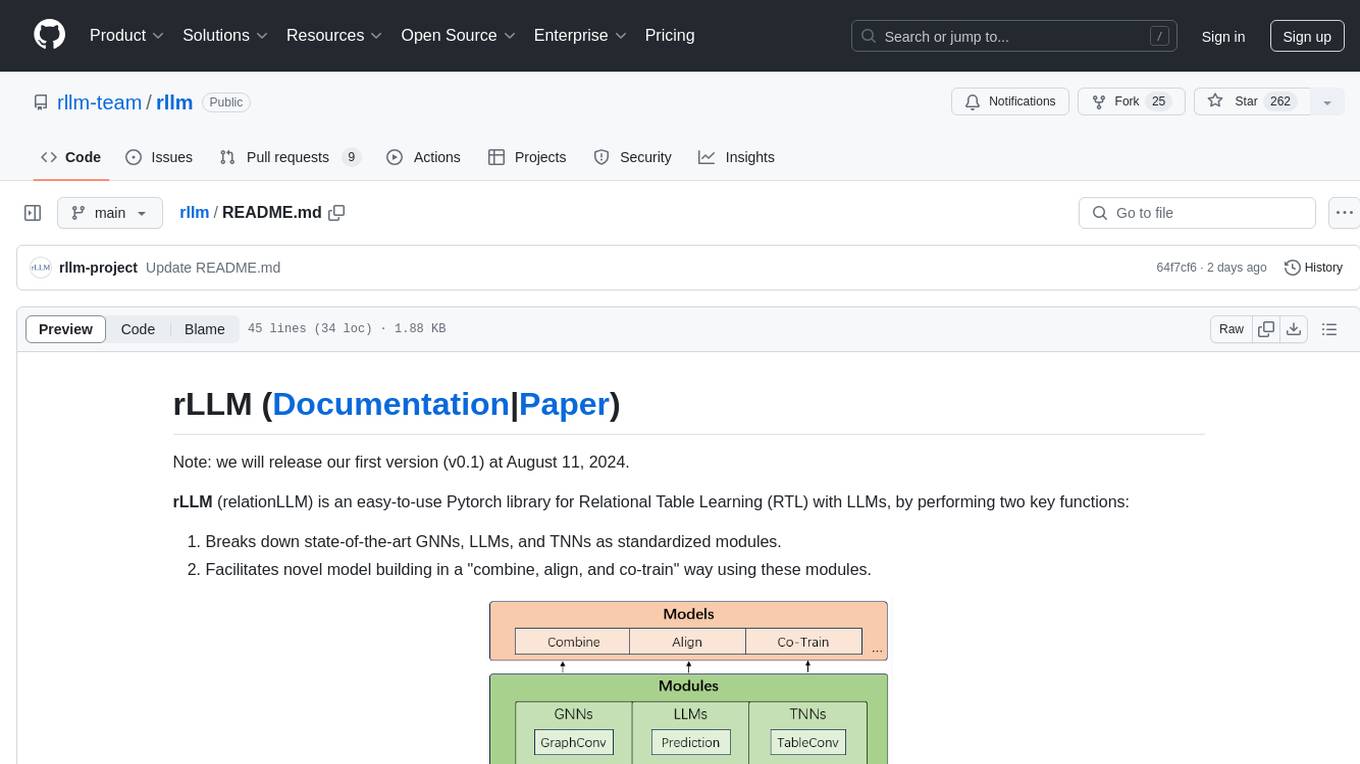
rllm
rLLM (relationLLM) is a Pytorch library for Relational Table Learning (RTL) with LLMs. It breaks down state-of-the-art GNNs, LLMs, and TNNs as standardized modules and facilitates novel model building in a 'combine, align, and co-train' way using these modules. The library is LLM-friendly, processes various graphs as multiple tables linked by foreign keys, introduces new relational table datasets, and is supported by students and teachers from Shanghai Jiao Tong University and Tsinghua University.
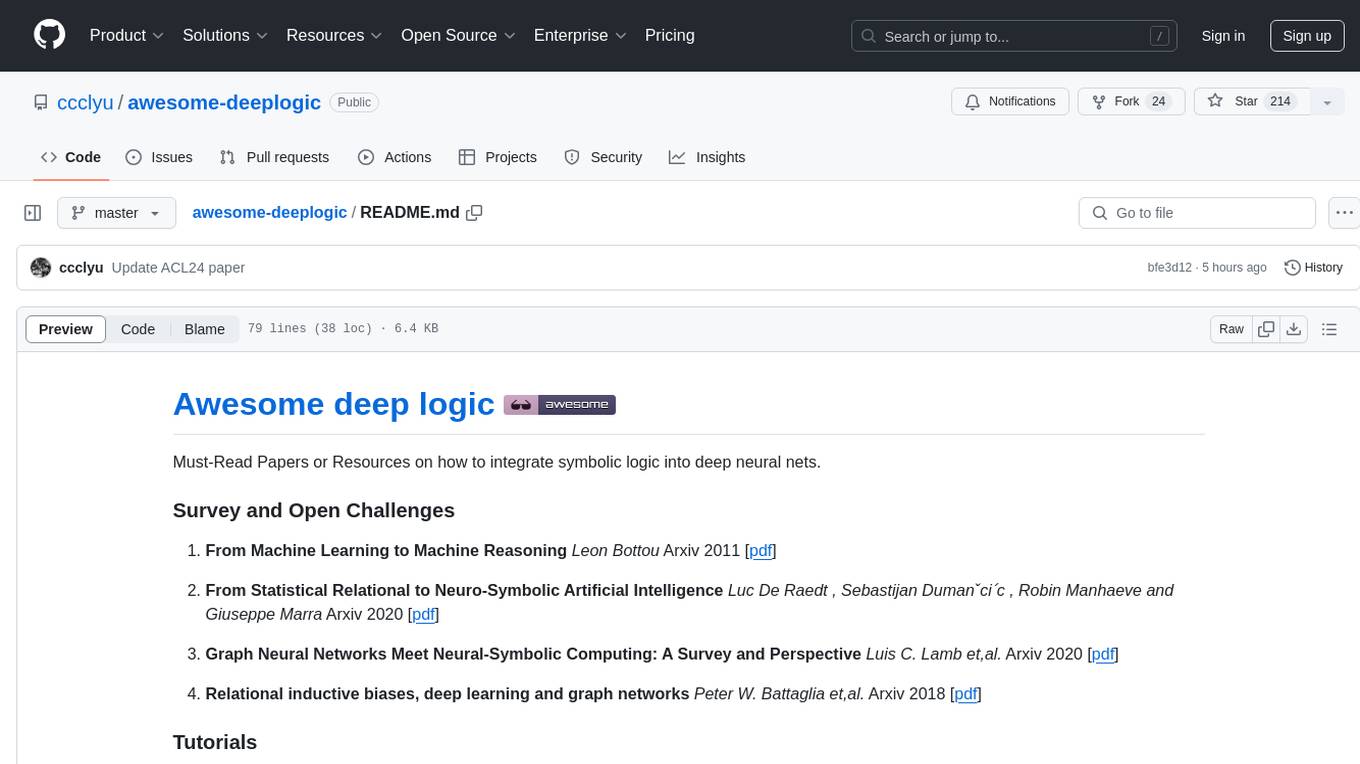
awesome-deeplogic
Awesome deep logic is a curated list of papers and resources focusing on integrating symbolic logic into deep neural networks. It includes surveys, tutorials, and research papers that explore the intersection of logic and deep learning. The repository aims to provide valuable insights and knowledge on how logic can be used to enhance reasoning, knowledge regularization, weak supervision, and explainability in neural networks.
For similar tasks
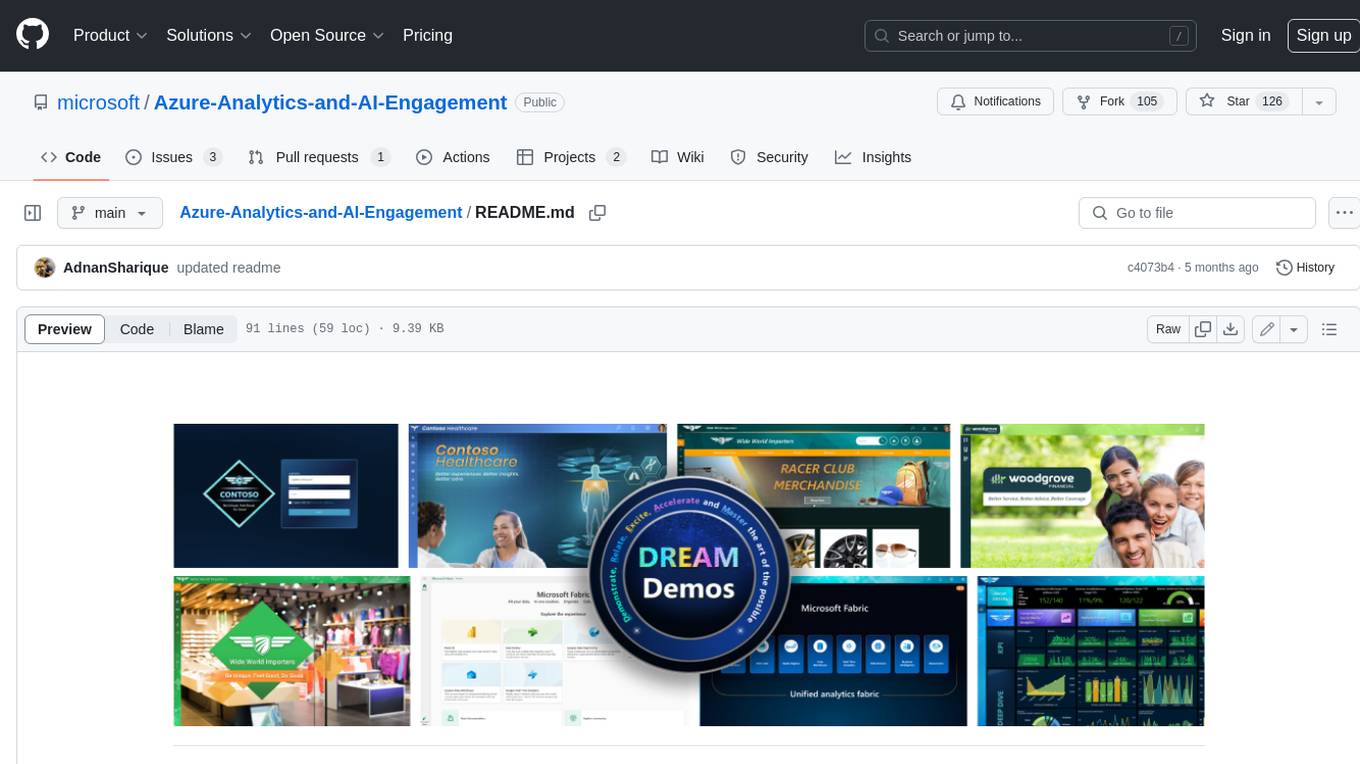
Azure-Analytics-and-AI-Engagement
The Azure-Analytics-and-AI-Engagement repository provides packaged Industry Scenario DREAM Demos with ARM templates (Containing a demo web application, Power BI reports, Synapse resources, AML Notebooks etc.) that can be deployed in a customer’s subscription using the CAPE tool within a matter of few hours. Partners can also deploy DREAM Demos in their own subscriptions using DPoC.
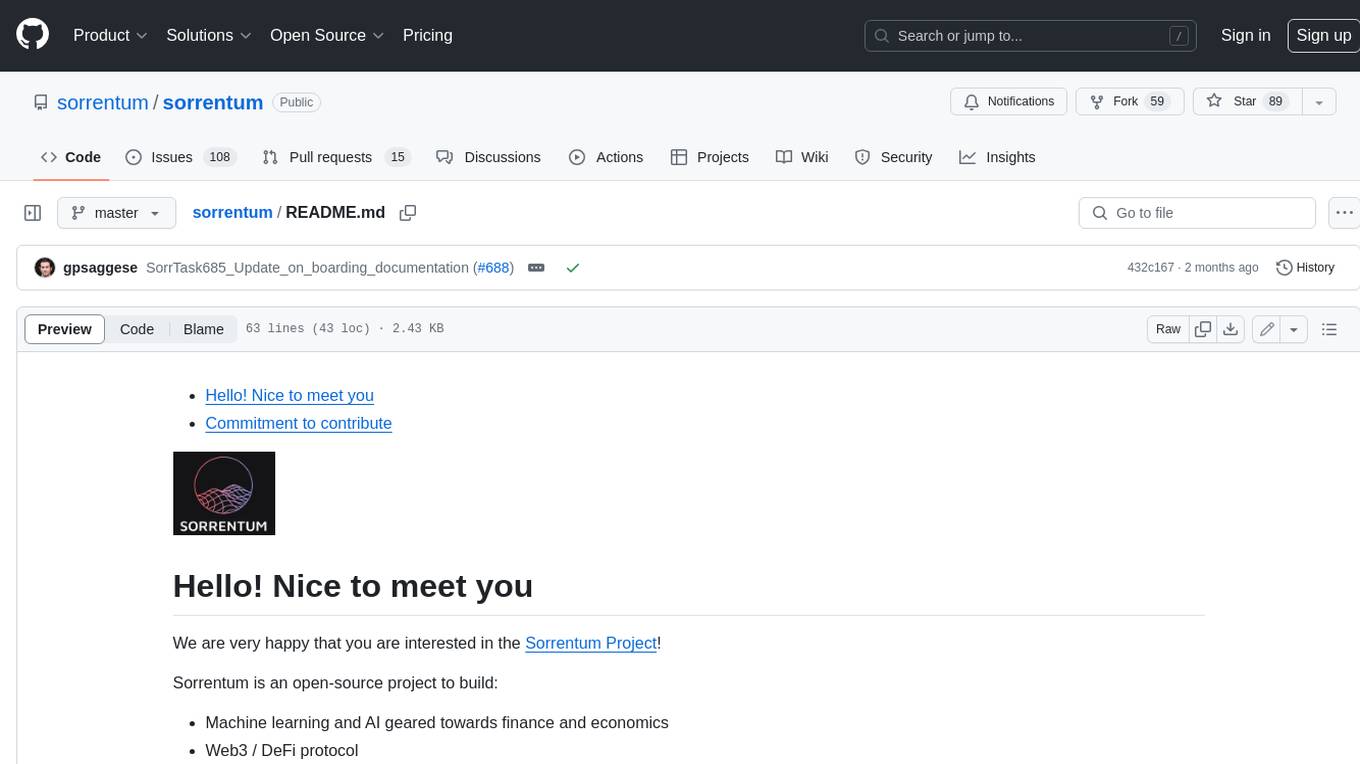
sorrentum
Sorrentum is an open-source project that aims to combine open-source development, startups, and brilliant students to build machine learning, AI, and Web3 / DeFi protocols geared towards finance and economics. The project provides opportunities for internships, research assistantships, and development grants, as well as the chance to work on cutting-edge problems, learn about startups, write academic papers, and get internships and full-time positions at companies working on Sorrentum applications.
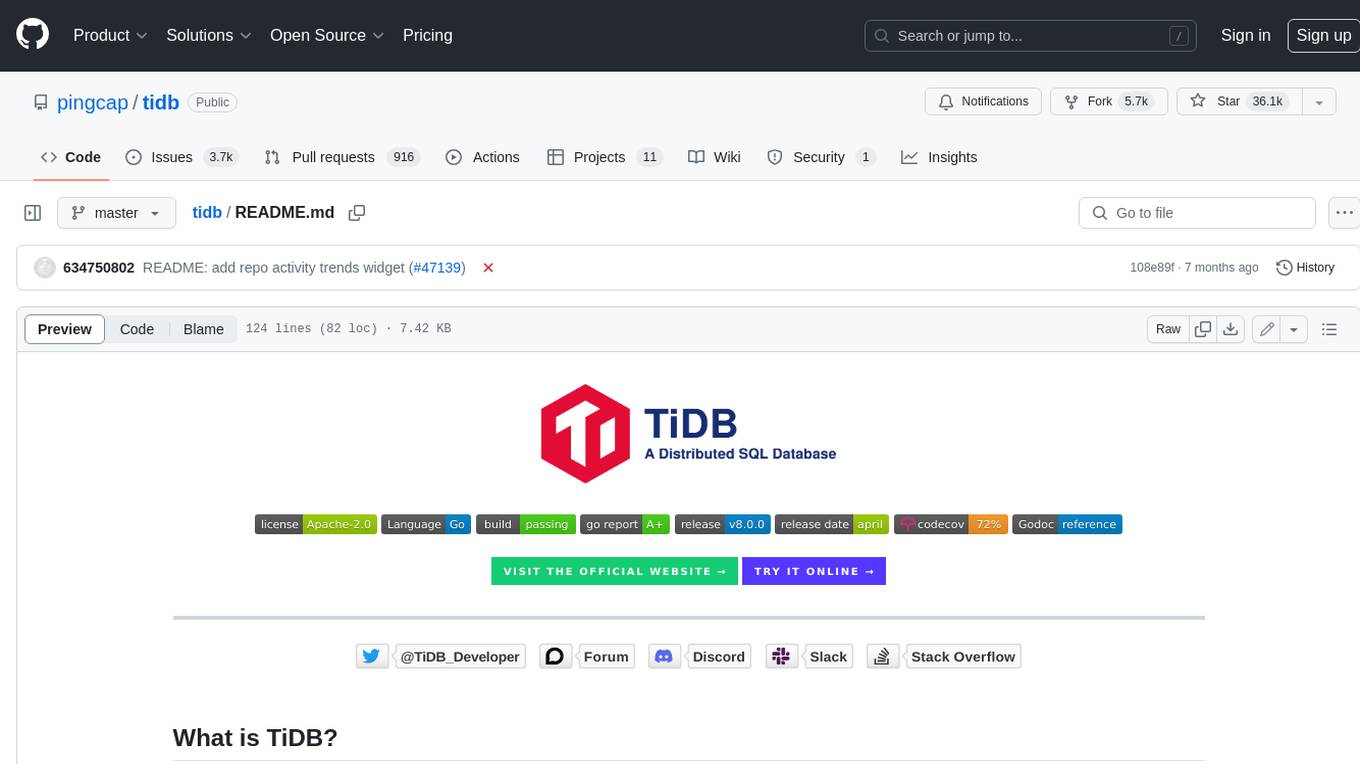
tidb
TiDB is an open-source distributed SQL database that supports Hybrid Transactional and Analytical Processing (HTAP) workloads. It is MySQL compatible and features horizontal scalability, strong consistency, and high availability.
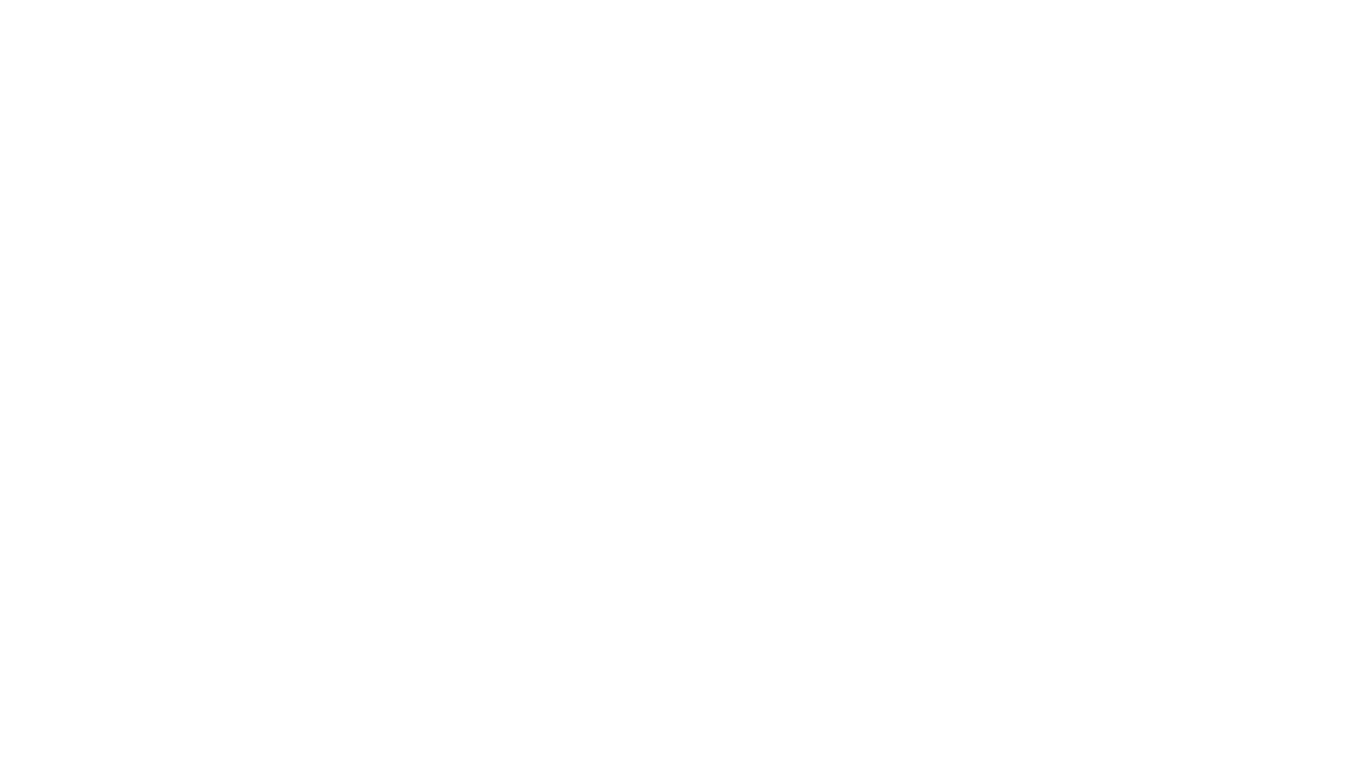
zep-python
Zep is an open-source platform for building and deploying large language model (LLM) applications. It provides a suite of tools and services that make it easy to integrate LLMs into your applications, including chat history memory, embedding, vector search, and data enrichment. Zep is designed to be scalable, reliable, and easy to use, making it a great choice for developers who want to build LLM-powered applications quickly and easily.
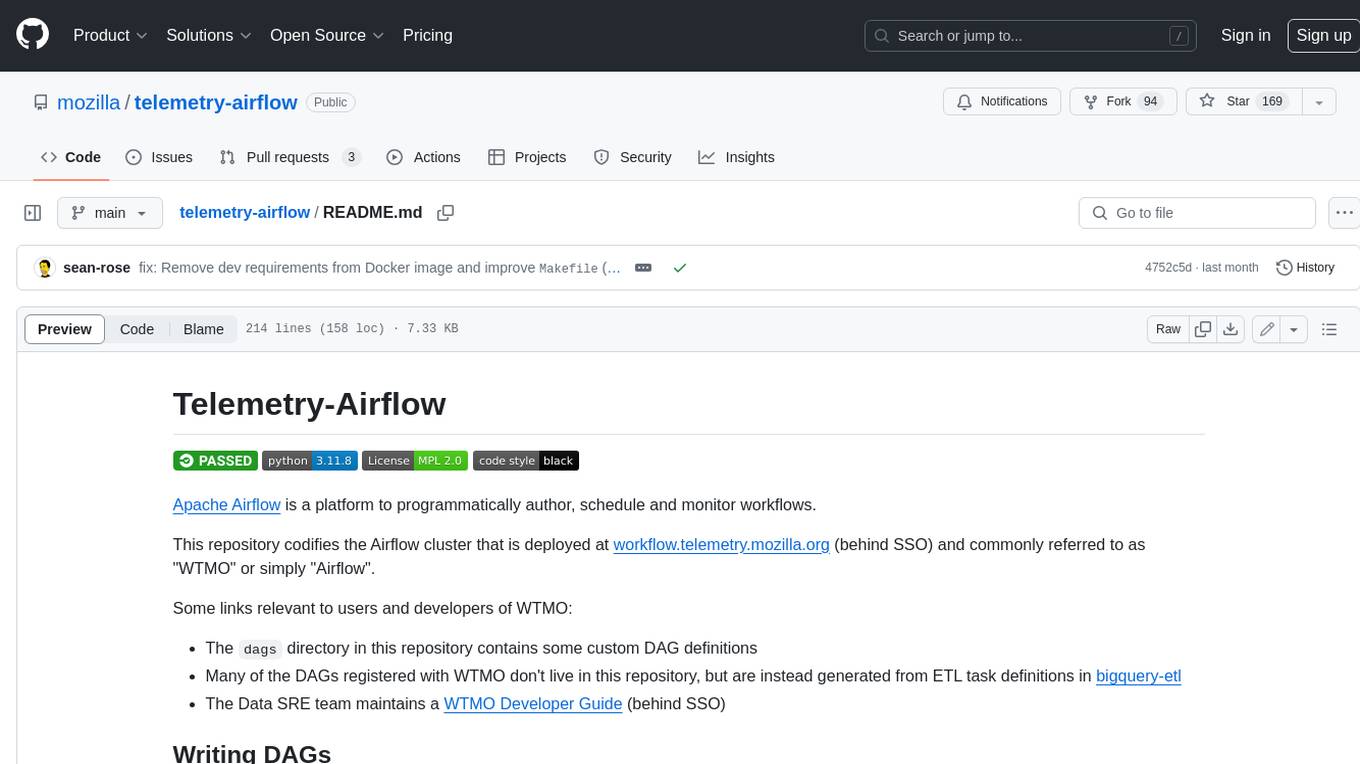
telemetry-airflow
This repository codifies the Airflow cluster that is deployed at workflow.telemetry.mozilla.org (behind SSO) and commonly referred to as "WTMO" or simply "Airflow". Some links relevant to users and developers of WTMO: * The `dags` directory in this repository contains some custom DAG definitions * Many of the DAGs registered with WTMO don't live in this repository, but are instead generated from ETL task definitions in bigquery-etl * The Data SRE team maintains a WTMO Developer Guide (behind SSO)
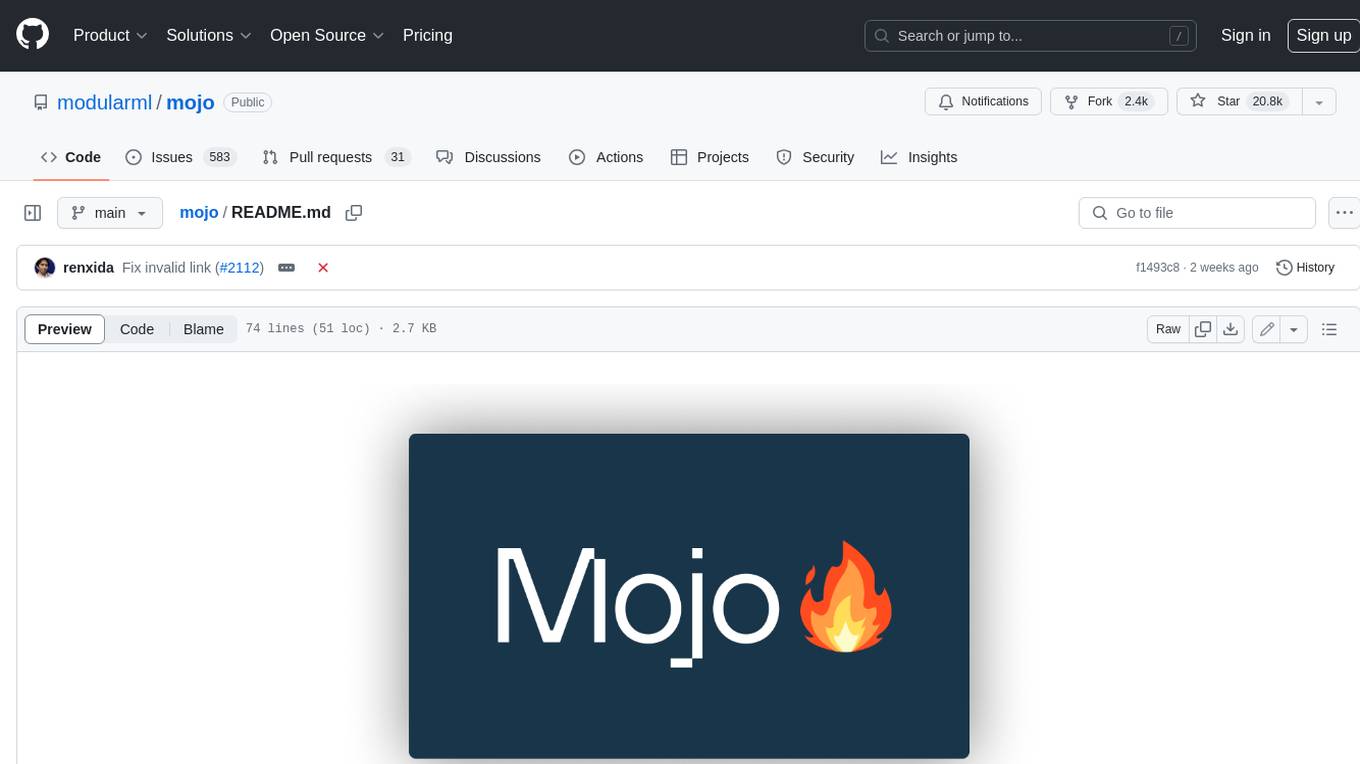
mojo
Mojo is a new programming language that bridges the gap between research and production by combining Python syntax and ecosystem with systems programming and metaprogramming features. Mojo is still young, but it is designed to become a superset of Python over time.
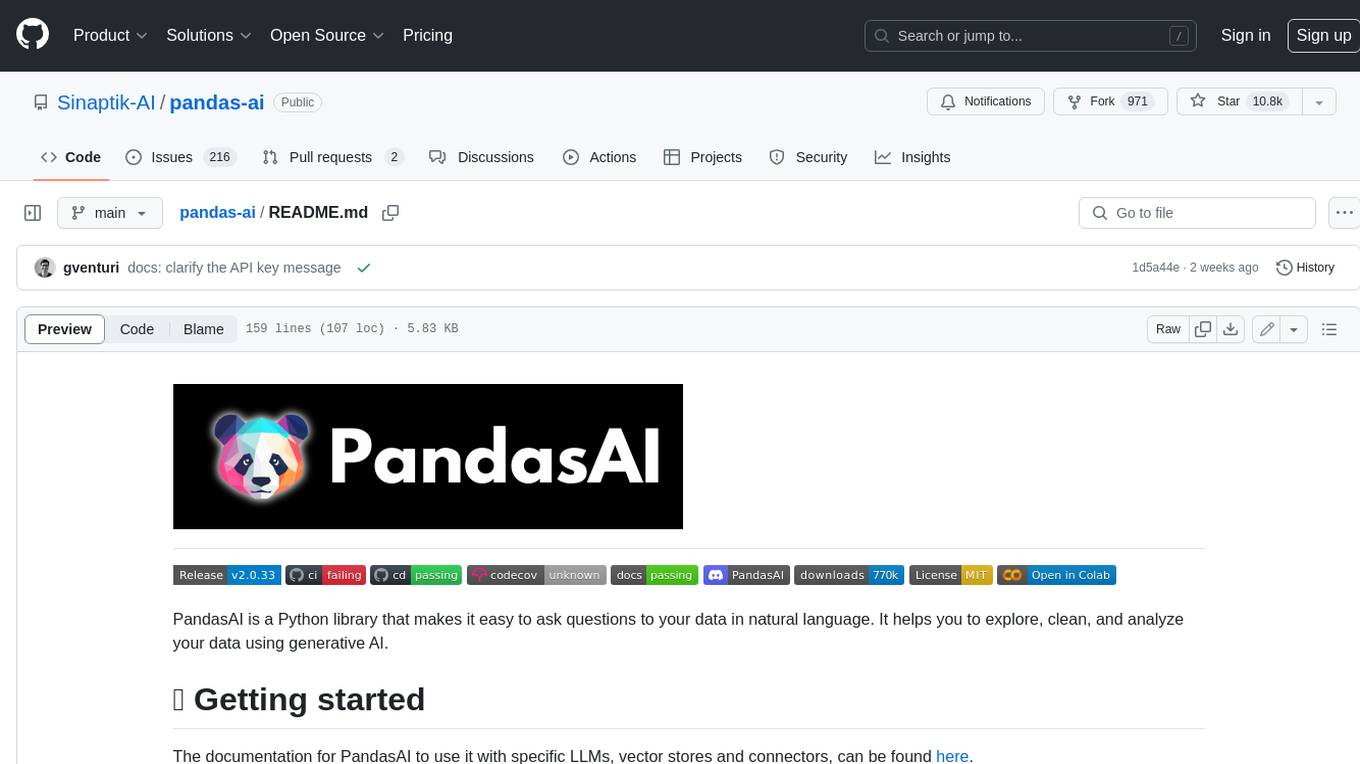
pandas-ai
PandasAI is a Python library that makes it easy to ask questions to your data in natural language. It helps you to explore, clean, and analyze your data using generative AI.
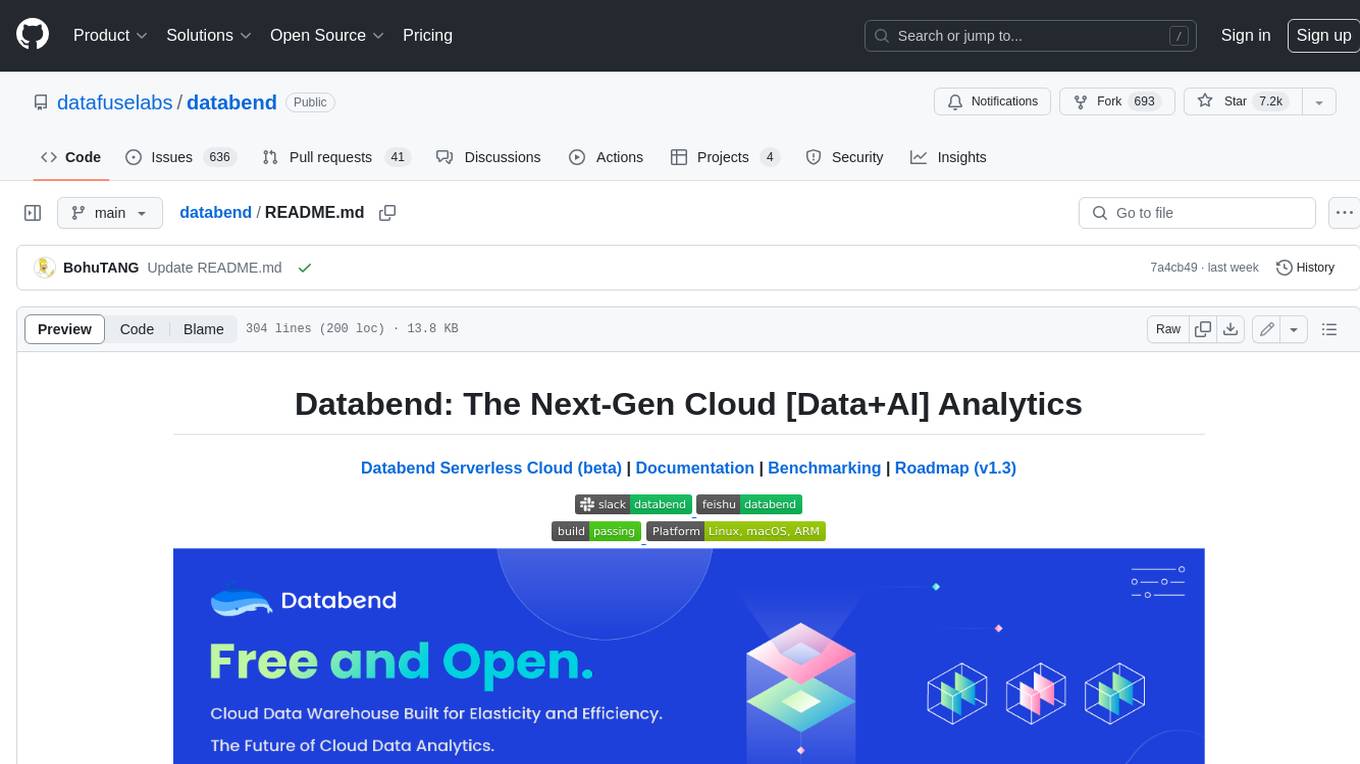
databend
Databend is an open-source cloud data warehouse that serves as a cost-effective alternative to Snowflake. With its focus on fast query execution and data ingestion, it's designed for complex analysis of the world's largest datasets.
For similar jobs
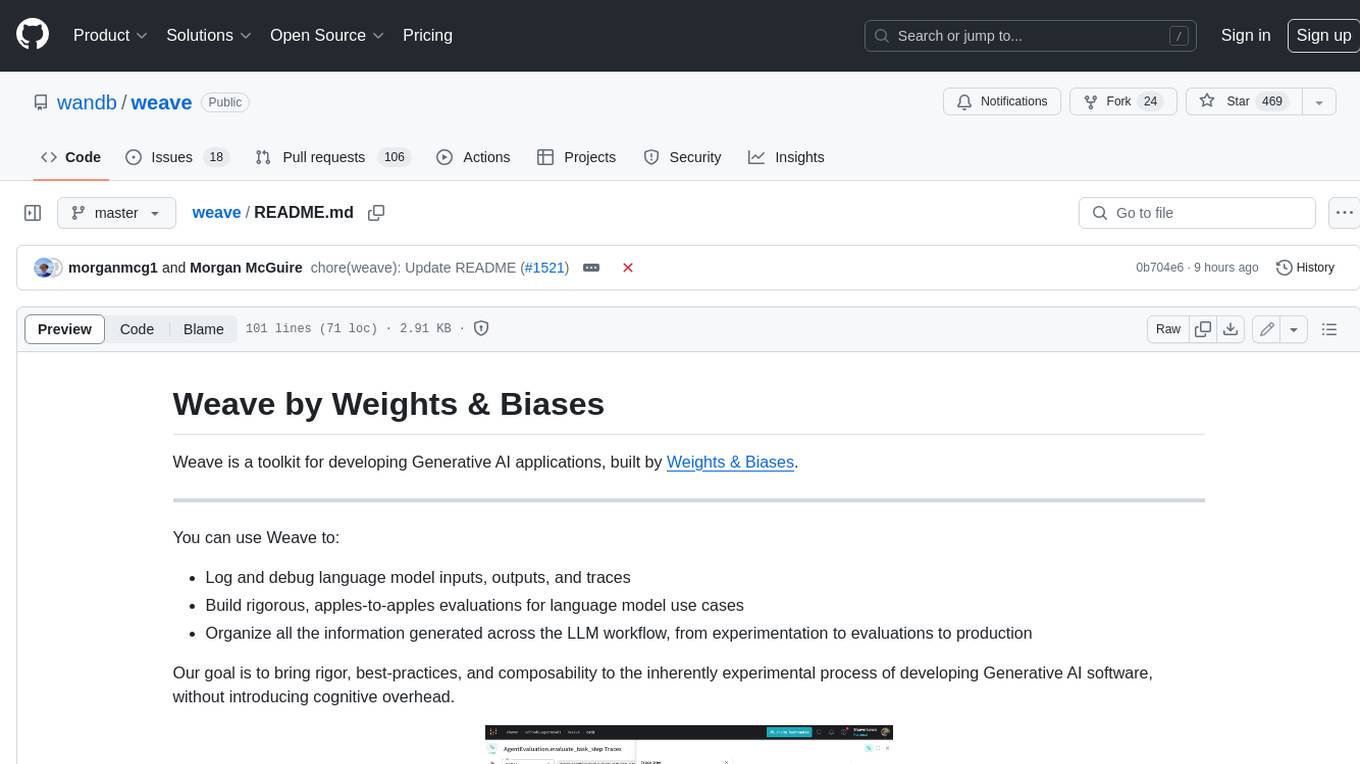
weave
Weave is a toolkit for developing Generative AI applications, built by Weights & Biases. With Weave, you can log and debug language model inputs, outputs, and traces; build rigorous, apples-to-apples evaluations for language model use cases; and organize all the information generated across the LLM workflow, from experimentation to evaluations to production. Weave aims to bring rigor, best-practices, and composability to the inherently experimental process of developing Generative AI software, without introducing cognitive overhead.
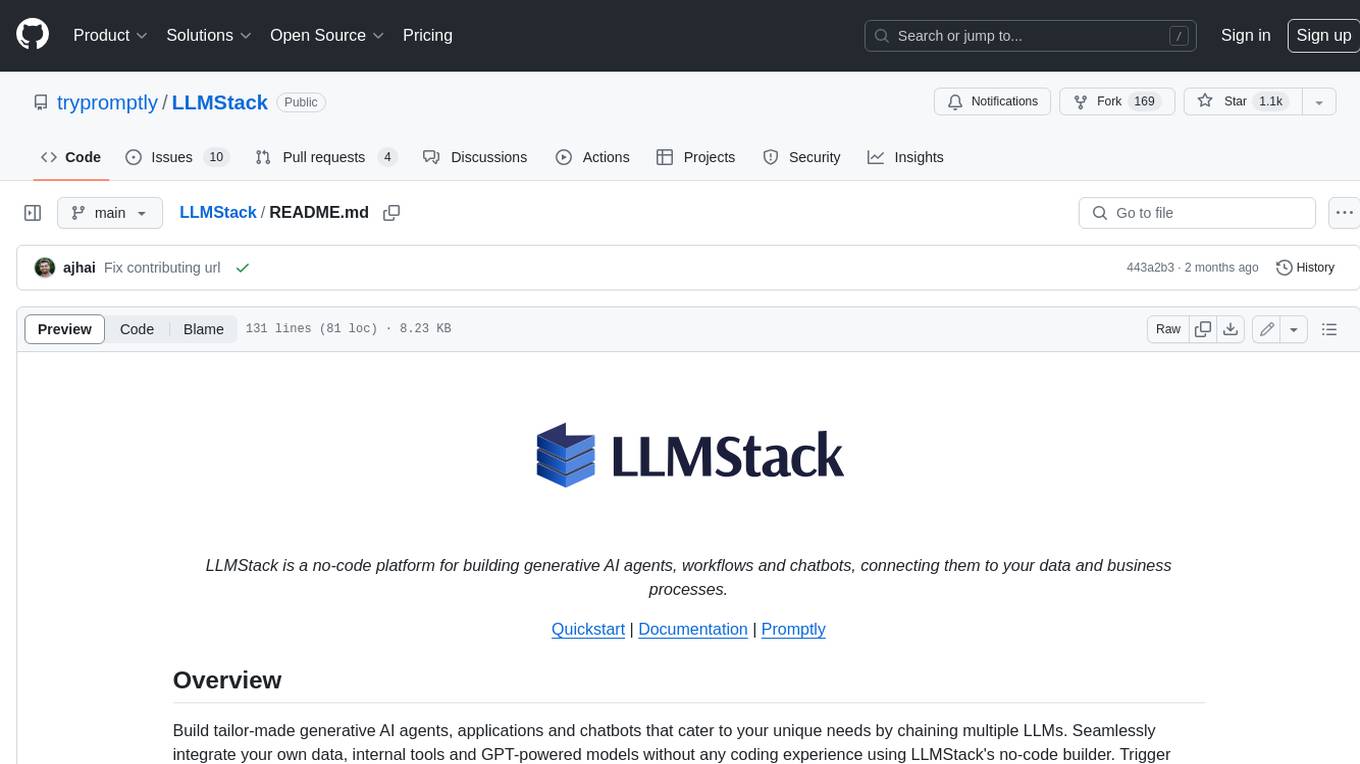
LLMStack
LLMStack is a no-code platform for building generative AI agents, workflows, and chatbots. It allows users to connect their own data, internal tools, and GPT-powered models without any coding experience. LLMStack can be deployed to the cloud or on-premise and can be accessed via HTTP API or triggered from Slack or Discord.
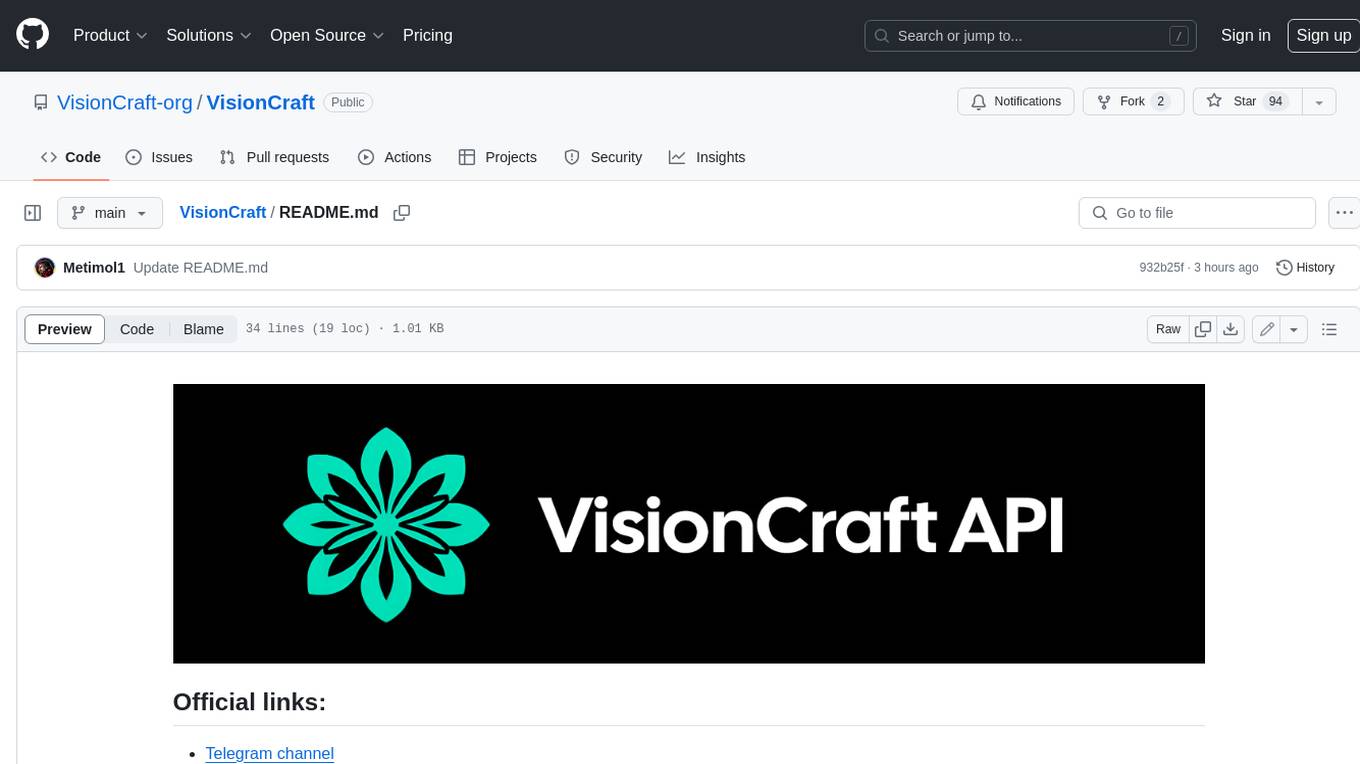
VisionCraft
The VisionCraft API is a free API for using over 100 different AI models. From images to sound.
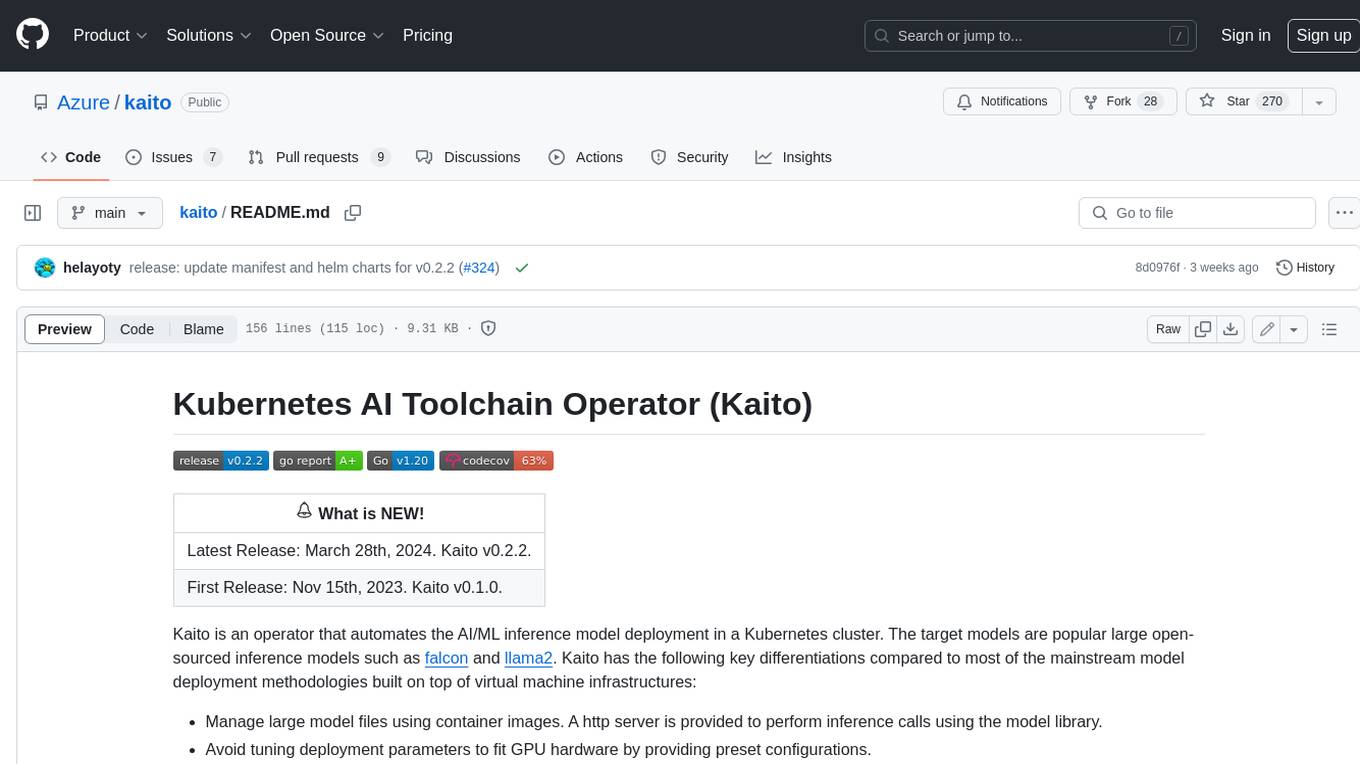
kaito
Kaito is an operator that automates the AI/ML inference model deployment in a Kubernetes cluster. It manages large model files using container images, avoids tuning deployment parameters to fit GPU hardware by providing preset configurations, auto-provisions GPU nodes based on model requirements, and hosts large model images in the public Microsoft Container Registry (MCR) if the license allows. Using Kaito, the workflow of onboarding large AI inference models in Kubernetes is largely simplified.
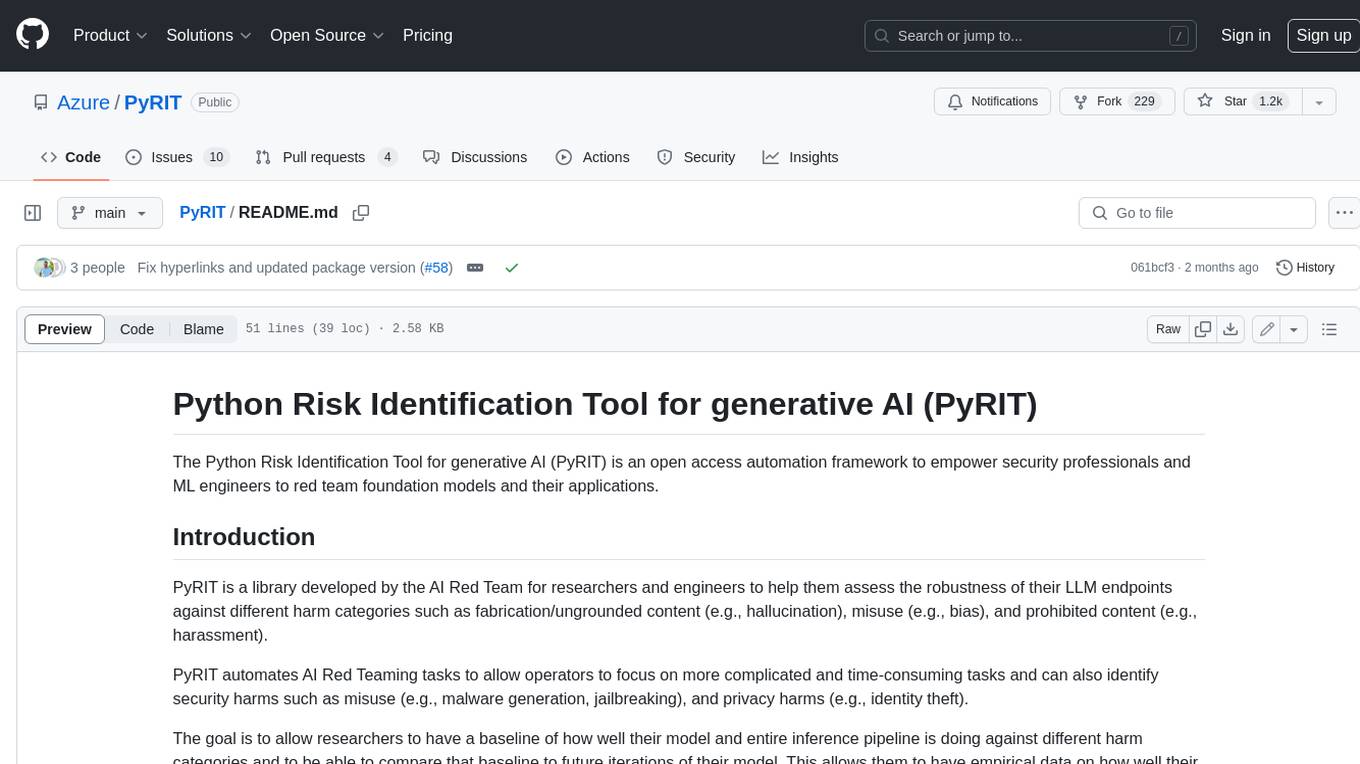
PyRIT
PyRIT is an open access automation framework designed to empower security professionals and ML engineers to red team foundation models and their applications. It automates AI Red Teaming tasks to allow operators to focus on more complicated and time-consuming tasks and can also identify security harms such as misuse (e.g., malware generation, jailbreaking), and privacy harms (e.g., identity theft). The goal is to allow researchers to have a baseline of how well their model and entire inference pipeline is doing against different harm categories and to be able to compare that baseline to future iterations of their model. This allows them to have empirical data on how well their model is doing today, and detect any degradation of performance based on future improvements.
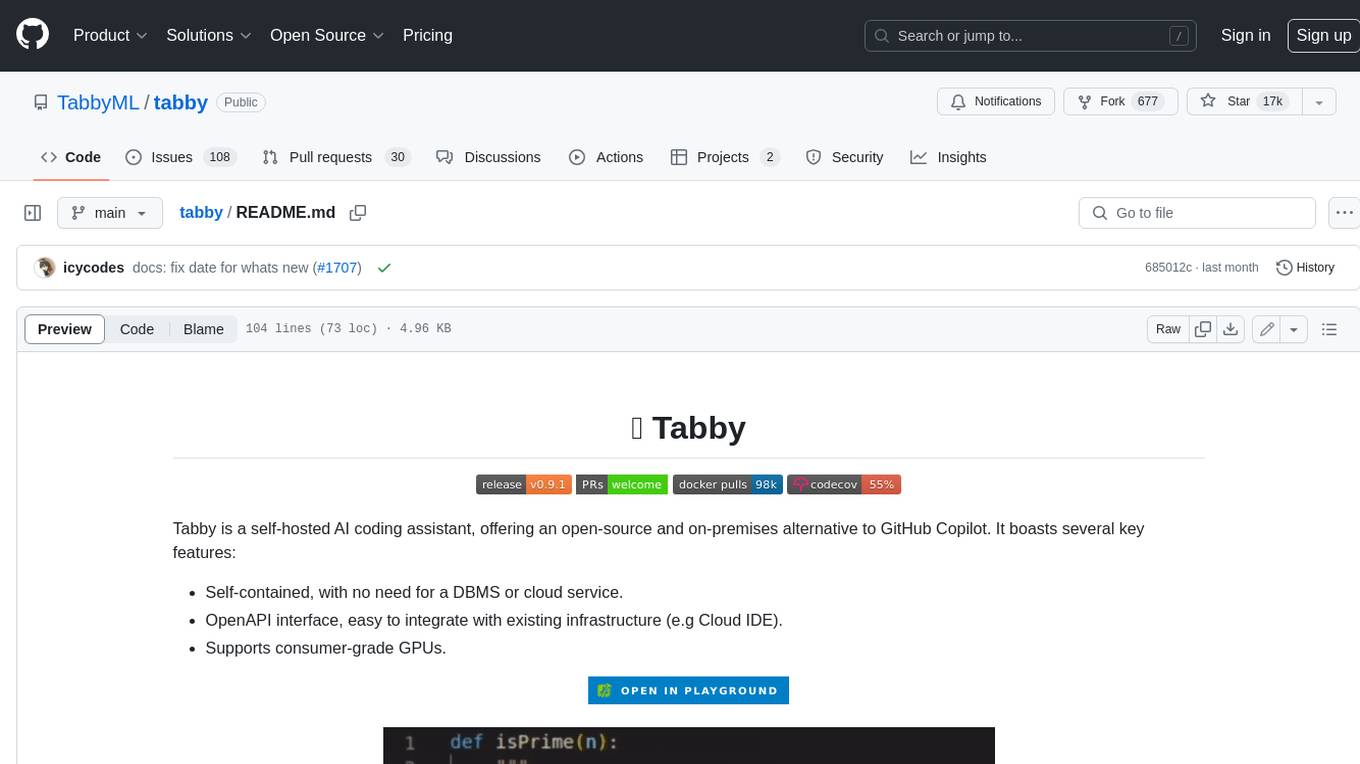
tabby
Tabby is a self-hosted AI coding assistant, offering an open-source and on-premises alternative to GitHub Copilot. It boasts several key features: * Self-contained, with no need for a DBMS or cloud service. * OpenAPI interface, easy to integrate with existing infrastructure (e.g Cloud IDE). * Supports consumer-grade GPUs.
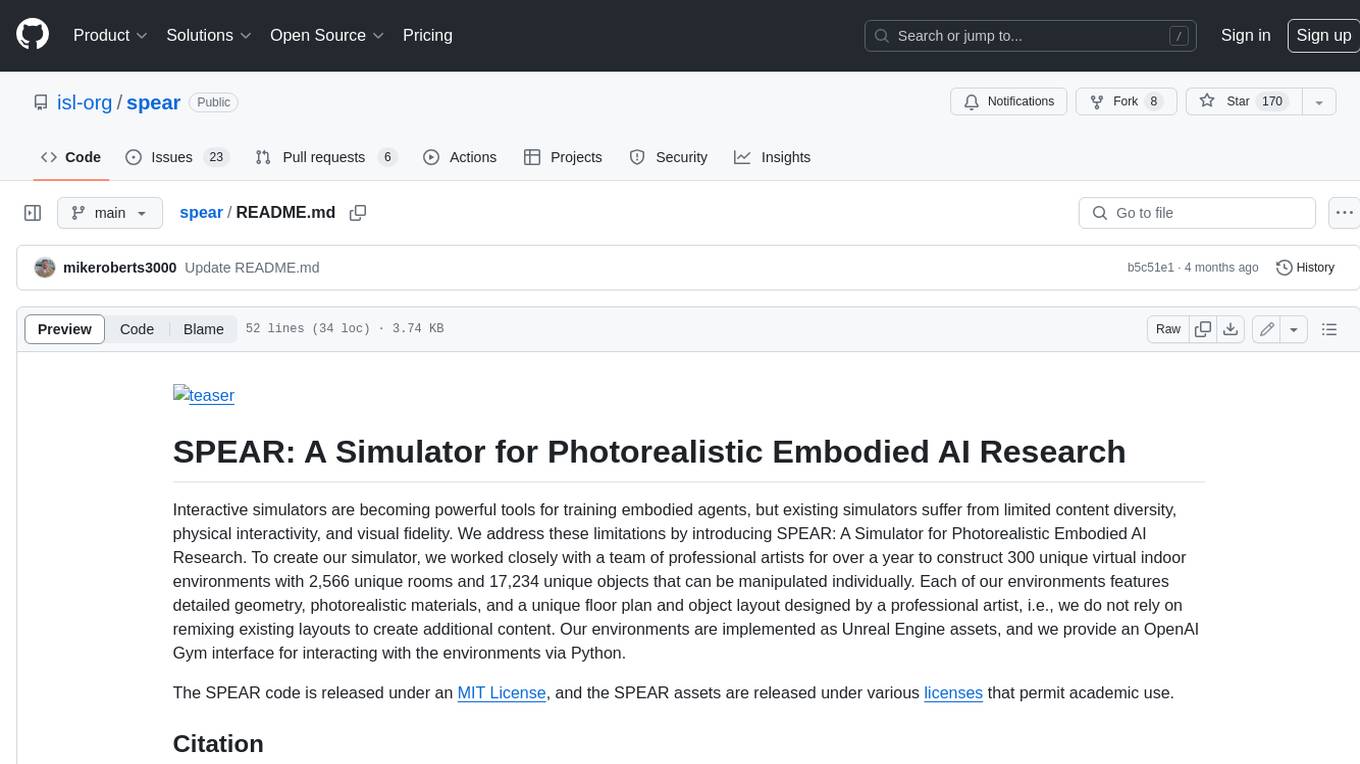
spear
SPEAR (Simulator for Photorealistic Embodied AI Research) is a powerful tool for training embodied agents. It features 300 unique virtual indoor environments with 2,566 unique rooms and 17,234 unique objects that can be manipulated individually. Each environment is designed by a professional artist and features detailed geometry, photorealistic materials, and a unique floor plan and object layout. SPEAR is implemented as Unreal Engine assets and provides an OpenAI Gym interface for interacting with the environments via Python.
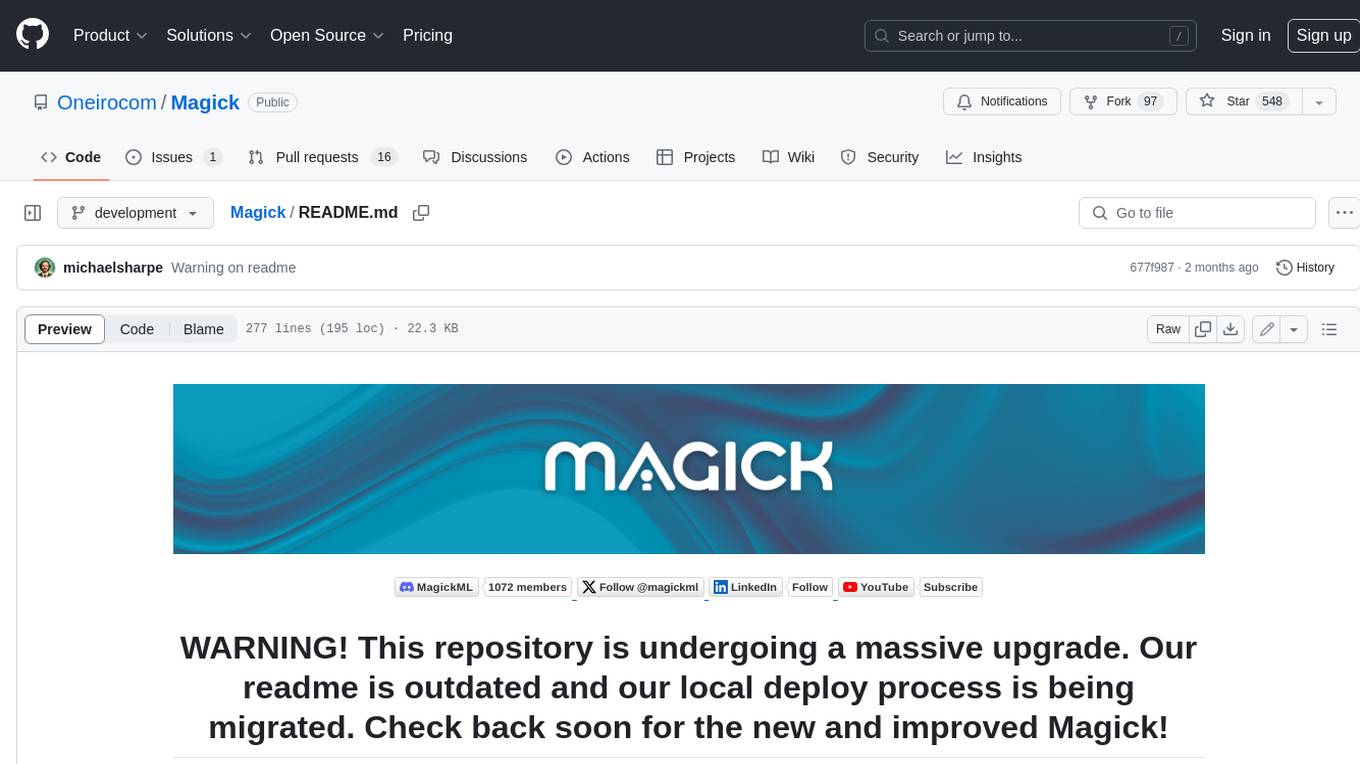
Magick
Magick is a groundbreaking visual AIDE (Artificial Intelligence Development Environment) for no-code data pipelines and multimodal agents. Magick can connect to other services and comes with nodes and templates well-suited for intelligent agents, chatbots, complex reasoning systems and realistic characters.