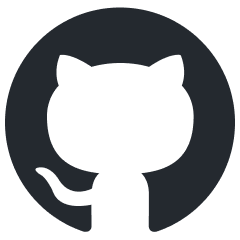
Awesome-TimeSeries-SpatioTemporal-LM-LLM
A professional list on Large (Language) Models and Foundation Models (LLM, LM, FM) for Time Series, Spatiotemporal, and Event Data.
Stars: 944
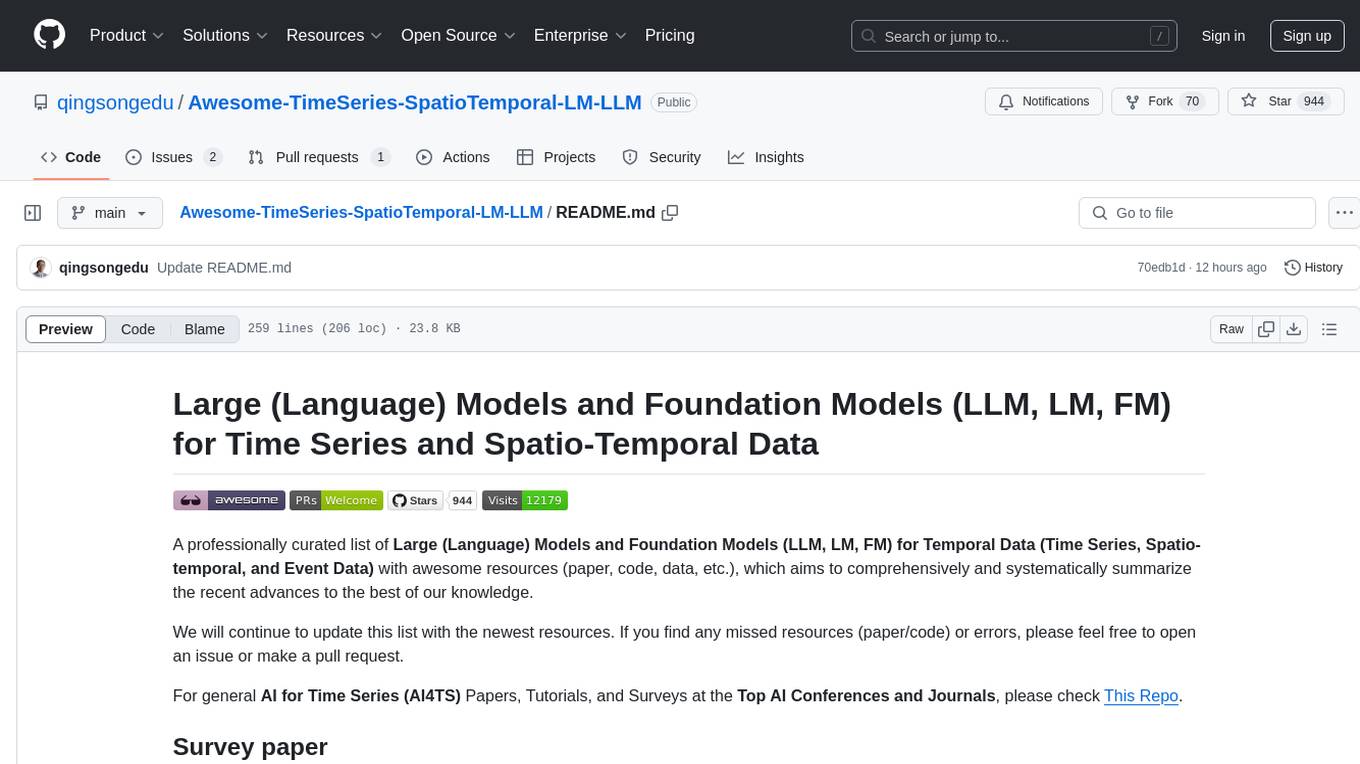
Awesome-TimeSeries-SpatioTemporal-LM-LLM is a curated list of Large (Language) Models and Foundation Models for Temporal Data, including Time Series, Spatio-temporal, and Event Data. The repository aims to summarize recent advances in Large Models and Foundation Models for Time Series and Spatio-Temporal Data with resources such as papers, code, and data. It covers various applications like General Time Series Analysis, Transportation, Finance, Healthcare, Event Analysis, Climate, Video Data, and more. The repository also includes related resources, surveys, and papers on Large Language Models, Foundation Models, and their applications in AIOps.
README:
Large (Language) Models and Foundation Models (LLM, LM, FM) for Time Series and Spatio-Temporal Data
A professionally curated list of Large (Language) Models and Foundation Models (LLM, LM, FM) for Temporal Data (Time Series, Spatio-temporal, and Event Data) with awesome resources (paper, code, data, etc.), which aims to comprehensively and systematically summarize the recent advances to the best of our knowledge.
We will continue to update this list with the newest resources. If you find any missed resources (paper/code) or errors, please feel free to open an issue or make a pull request.
For general AI for Time Series (AI4TS) Papers, Tutorials, and Surveys at the Top AI Conferences and Journals, please check This Repo.
Large Models for Time Series and Spatio-Temporal Data: A Survey and Outlook
Authors: Ming Jin, Qingsong Wen*, Yuxuan Liang, Chaoli Zhang, Siqiao Xue, Xue Wang, James Zhang, Yi Wang, Haifeng Chen, Xiaoli Li (IEEE Fellow), Shirui Pan*, Vincent S. Tseng (IEEE Fellow), Yu Zheng (IEEE Fellow), Lei Chen (IEEE Fellow), Hui Xiong (IEEE Fellow)
🌟 If you find this resource helpful, please consider to star this repository and cite our survey paper:
@article{jin2023lm4ts,
title={Large Models for Time Series and Spatio-Temporal Data: A Survey and Outlook},
author={Ming Jin and Qingsong Wen and Yuxuan Liang and Chaoli Zhang and Siqiao Xue and Xue Wang and James Zhang and Yi Wang and Haifeng Chen and Xiaoli Li and Shirui Pan and Vincent S. Tseng and Yu Zheng and Lei Chen and Hui Xiong},
journal={arXiv preprint arXiv:2310.10196},
year={2023}
}
- Position Paper: What Can Large Language Models Tell Us about Time Series Analysis, in ICML 2024, [paper]
- Time-FFM: Towards LM-Empowered Federated Foundation Model for Time Series Forecasting, NeurIPS 2024. [paper]
- Time-MMD: A New Multi-Domain Multimodal Dataset for Time Series Analysis, NeurIPS 2024. [paper] [official code]
- From News to Forecast: Integrating Event Analysis in LLM-Based Time Series Forecasting with Reflection, NeurIPS 2024. [paper] [official code]
- Autotimes: Autoregressive time series forecasters via large language models, NeurIPS 2024. [paper] [official code]
- S^2IP-LLM: Semantic Space Informed Prompt Learning with LLM for Time Series Forecasting, in ICML 2024, [paper]
- Multi-Patch Prediction: Adapting LLMs for Time Series Representation Learning, in ICML 2024, [paper] [official code]
- TimeCMA: Towards LLM-Empowered Multivariate Time Series Forecasting via Cross-Modality Alignment, in AAAI 2025, [paper]
- Time-LLM: Time Series Forecasting by Reprogramming Large Language Models, in ICLR 2024, [paper] [official code]
- TEMPO: Prompt-based Generative Pre-trained Transformer for Time Series Forecasting, in ICLR 2024, [paper]
- TEST: Text Prototype Aligned Embedding to Activate LLM's Ability for Time Series, in ICLR 2024, [paper]
- UniTime: A Language-Empowered Unified Model for Cross-Domain Time Series Forecasting, in WWW 2024, [paper]
- LLM4TS: Two-Stage Fine-Tuning for Time-Series Forecasting with Pre-Trained LLMs, in arXiv 2023, [paper]
- The first step is the hardest: Pitfalls of Representing and Tokenizing Temporal Data for Large Language Models, in arXiv 2023, [paper]
- PromptCast: A New Prompt-based Learning Paradigm for Time Series Forecasting, in TKDE 2023. [paper]
- One Fits All: Power General Time Series Analysis by Pretrained LM, in NeurIPS 2023, [paper] [official code]
- Large Language Models Are Zero-Shot Time Series Forecasters, in NeurIPS 2023, [paper] [official code]
- NuwaTS: a Foundation Model Mending Every Incomplete Time Series, in arXiv 2024, [paper]
- Where Would I Go Next? Large Language Models as Human Mobility Predictors, in arXiv 2023, [paper]
- Leveraging Language Foundation Models for Human Mobility Forecasting, in SIGSPATIAL 2022, [paper]
- Temporal Data Meets LLM -- Explainable Financial Time Series Forecasting, in arXiv 2023, [paper]
- BloombergGPT: A Large Language Model for Finance, in arXiv 2023, [paper]
- WeaverBird: Empowering Financial Decision-Making with Large Language Model, Knowledge Base, and Search Engine, in arXiv 2023, [paper][official-code]
- Can ChatGPT Forecast Stock Price Movements? Return Predictability and Large Language Models, in arXiv 2023, [paper]
- Instruct-FinGPT: Financial Sentiment Analysis by Instruction Tuning of General-Purpose Large Language Models, in arXiv 2023, [paper]
- The Wall Street Neophyte: A Zero-Shot Analysis of ChatGPT Over MultiModal Stock Movement Prediction Challenges, in arXiv 2023, [paper]
- Large Language Models are Few-Shot Health Learners, in arXiv 2023. [paper]
- Health system-scale language models are all-purpose prediction engines, in Nature 2023, [paper]
- A large language model for electronic health records, in NPJ Digit. Med. 2022, [paper]
- Drafting Event Schemas using Language Models, in arXiv 2023, [paper]
- Language Models Can Improve Event Prediction by Few-Shot Abductive Reasoning, in NeurIPS 2023, [paper], [official-code]
- Time-MoE: Billion-Scale Time Series Foundation Models with Mixture of Experts, arXiv 2024, [paper] [official code]
- Unified training of universal time series forecasting transformers, in ICML 2024, [paper] [official code]
- Moment: A family of open time-series foundation models, in ICML 2024, [paper] [official code]
- A decoder-only foundation model for time-series forecasting, in ICML 2024, [paper] [official code]
- Chronos: Learning the language of time series, in TMLR 2024, [paper] [official code]
- SimMTM: A Simple Pre-Training Framework for Masked Time-Series Modeling, in NeurIPS 2023, [paper]
- A Time Series is Worth 64 Words: Long-term Forecasting with Transformers, in ICLR 2023, [paper] [official code]
- Contrastive Learning for Unsupervised Domain Adaptation of Time Series, in ICLR 2023, [paper]
- TSMixer: Lightweight MLP-Mixer Model for Multivariate Time Series Forecasting, in KDD 2023, [paper]
- Self-Supervised Contrastive Pre-Training For Time Series via Time-Frequency Consistency, in NeurIPS 2022, [paper] [official code]
- Pre-training Enhanced Spatial-temporal Graph Neural Network for Multivariate Time Series Forecasting, in KDD 2022, [paper]
- TS2Vec: Towards Universal Representation of Time Series, in AAAI 2022, [paper] [official code]
- Voice2Series: Reprogramming Acoustic Models for Time Series Classification, in ICML 2021. [paper] [official code]
- Prompt-augmented Temporal Point Process for Streaming Event Sequence, in NeurIPS 2023, [paper] [official code]
- Language knowledge-Assisted Representation Learning for Skeleton-based Action Recognition, in arXiv 2023. [paper]
- Chatgpt-Informed Graph Neural Network for Stock Movement Prediction, in arXiv 2023. [paper]
- When do Contrastive Learning Signals help Spatio-Temporal Graph Forecasting? in SIGSPATIAL 2023. [paper]
- Mining Spatio-Temporal Relations via Self-Paced Graph Contrastive Learning, in KDD 2022. [paper]
- Accurate Medium-Range Global Weather Forecasting with 3D Neural Networks, in Nature 2023. [paper]
- ClimaX: A Foundation Model for Weather and Climate, in ICML 2023. [paper] [official code]
- GraphCast: Learning Skillful Medium-Range Global Weather Forecasting, in arXiv 2022. [paper]
- FourCastNet: A Global Data-driven High-resolution Weather Model using Adaptive Fourier Neural Operator, in arXiv 2022. [paper]
- Accurate Medium-Range Global Weather Forecasting with 3d Neural Networks, in Nature 2023. [paper]
- W-MAE: Pre-Trained Weather Model with Masked Autoencoder for Multi-Variable Weather Forecasting, in arXiv 2023. [paper]
- FengWu: Pushing the Skillful Global Medium-range Weather Forecast beyond 10 Days Lead, in arXiv 2023. [paper]
- Pre-trained Bidirectional Temporal Representation for Crowd Flows Prediction in Regular Region, in IEEE Access 2019. [paper]
- Trafficbert: Pretrained Model with Large-Scale Data for Long-Range Traffic Flow Forecasting, in Expert Systems with Applications 2021. [paper]
- Building Transportation Foundation Model via Generative Graph Transformer, in arXiv 2023. [paper]
- Zero-shot Video Question Answering via Frozen Bidirectional Language Models, in NeurIPS 2022. [paper]
- Language Models with Image Descriptors are Strong Few-Shot Video-Language Learners, in NeurIPS 2022. [paper]
- VideoLLM: Modeling Video Sequence with Large Language Models, in arXiv 2023. [paper]
- VALLEY: Viode Assitant with Large Language Model Enhanced Ability, in arXiv 2023. [paper]
- Vid2Seq: Large-Scale Pretraining of a Visual Language Model for Dense Video Captioning, in CVPR 2023. [paper]
- Retrieving-to-Answer: Zero-Shot Video Question Answering with Frozen Large Language Models, in NeurIPS 2022. [paper]
- VideoChat: Chat-Centric Video Understanding, in arXiv 2023. [paper]
- MovieChat: From Dense Token to Sparse Memory for Long Video Understanding, in arXiv 2023. [paper]
- Language Models are Causal Knowledge Extractors for Zero-shot Video Question Answering, in CVPR 2023. [paper]
- Video-LLaMA: An Instruction-tuned Audio-Visual Language Model for Video Understanding, in arXiv 2023. [paper]
- Learning Video Representations from Large Language Models, in CVPR 2023. [paper]
- Video-ChatGPT: Towards Detailed Video Understanding via Large Vision and Language Models, in arXiv 2023. [paper]
- Traffic-Domain Video Question Answering with Automatic Captioning, in arXiv 2023. [paper]
- LAVENDER: Unifying Video-Language Understanding as Masked Language Modeling, in CVPR 2023. [paper]
- OmniVL: One Foundation Model for Image-Language and Video-Language Tasks, in NeurIPS 2022. [paper]
- Youku-mPLUG: A 10 Million Large-scale Chinese Video-Language Dataset for Pre-training and Benchmarks, in arXiv 2023. [paper]
- PAXION: Patching Action Knowledge in Video-Language Foundation Models, in arXiv 2023. [paper]
- mPLUG-2: A Modularized Multi-modal Foundation Model Across Text, Image and Video, in ICML 2023. [paper]
- METR-LA [link]
- PEMS-BAY [link]
- PEMS04 [link]
- SUTD-TrafficQA [link]
- TaxiBJ \link]
- BikeNYC [link]
- TaxiNYC [link]
- Mobility [link]
- LargeST [link]
- PTB-XL [link]
- NYUTron [link]
- UF Health clinical corpus [link]
- i2b2-2012 [link]
- MIMIC-III [link]
- CirCor DigiScope [link]
- SEVIR [link]
- Shifts-Weather Prediction [link]
- AvePRE [link]
- SurTEMP [link]
- SurUPS [link]
- ERA5 reanalysis data [link]
- CMIP6 [link]
- ETT [link]
- M4 [link]
- Electricity [link]
- Alibaba Cluster Trace [link]
- TSSB [link]
- UCR TS Classification Archive [link]
- A Survey of Large Language Models, in arXiv 2023. [paper] [link]
- Harnessing the Power of LLMs in Practice: A Survey on ChatGPT and Beyond, in arXiv 2023. [paper] [link]
- LLM-Adapters: An Adapter Family for Parameter-Efficient Fine-Tuning of Large Language Models, in arXiv 2023. [paper] [link]
- Beyond One-Model-Fits-All: A Survey of Domain Specialization for Large Language Models, in arXiv 2023. [paper]
- Large AI Models in Health Informatics: Applications, Challenges, and the Future, in arXiv 2023. [paper] [link]
- FinGPT: Open-Source Financial Large Language Models, in arXiv 2023. [paper] [link]
- On the Opportunities and Challenges of Foundation Models for Geospatial Artificial Intelligence, in arXiv 2023. [paper]
- Awesome LLM. [link]
- Open LLMs. [link]
- Awesome LLMOps. [link]
- Awesome Foundation Models. [link]
- Awesome Graph LLM. [link]
- Awesome Multimodal Large Language Models. [link]
- Foundation Models for Time Series Analysis: A Tutorial and Survey, in KDD 2024. [paper]
- What Can Large Language Models Tell Us about Time Series Analysis, in ICML 2024. [paper]
- Deep Learning for Multivariate Time Series Imputation: A Survey, in arXiv 2024. [paper] [Website]
- Self-Supervised Learning for Time Series Analysis: Taxonomy, Progress, and Prospects, in TPAMI 2024. [paper] [Website]
- A Survey on Graph Neural Networks for Time Series: Forecasting, Classification, Imputation, and Anomaly Detection, in arXiv 2023. [paper] [Website]
- Transformers in Time Series: A Survey, in IJCAI 2023. [paper] [GitHub Repo]
- Time series data augmentation for deep learning: a survey, in IJCAI 2021. [paper]
- Time-series forecasting with deep learning: a survey, in Philosophical Transactions of the Royal Society A 2021. [paper]
- A review on outlier/anomaly detection in time series data, in CSUR 2021. [paper]
- Deep learning for time series classification: a review, in Data Mining and Knowledge Discovery 2019. [paper]
- A Survey on Time-Series Pre-Trained Models, in arXiv 2023. [paper] [link]
- Self-Supervised Learning for Time Series Analysis: Taxonomy, Progress, and Prospects, in arXiv 2023. [paper] [Website]
- A Survey on Graph Neural Networks for Time Series: Forecasting, Classification, Imputation, and Anomaly Detection, in arXiv 2023. [paper] [Website]
- AIOps: real-world challenges and research innovations, in ICSE 2019. [paper]
- A Survey of AIOps Methods for Failure Management, in TIST 2021. [paper]
- AI for IT Operations (AIOps) on Cloud Platforms: Reviews, Opportunities and Challenges, in arXiv 2023. [paper]
- Empowering Practical Root Cause Analysis by Large Language Models for Cloud Incidents, in arXiv 2023. [paper]
- Recommending Root-Cause and Mitigation Steps for Cloud Incidents using Large Language Models, in arXiv 2023. [paper]
- OpsEval: A Comprehensive Task-Oriented AIOps Benchmark for Large Language Models, in arXiv 2023. [paper]
For Tasks:
Click tags to check more tools for each tasksFor Jobs:
Alternative AI tools for Awesome-TimeSeries-SpatioTemporal-LM-LLM
Similar Open Source Tools
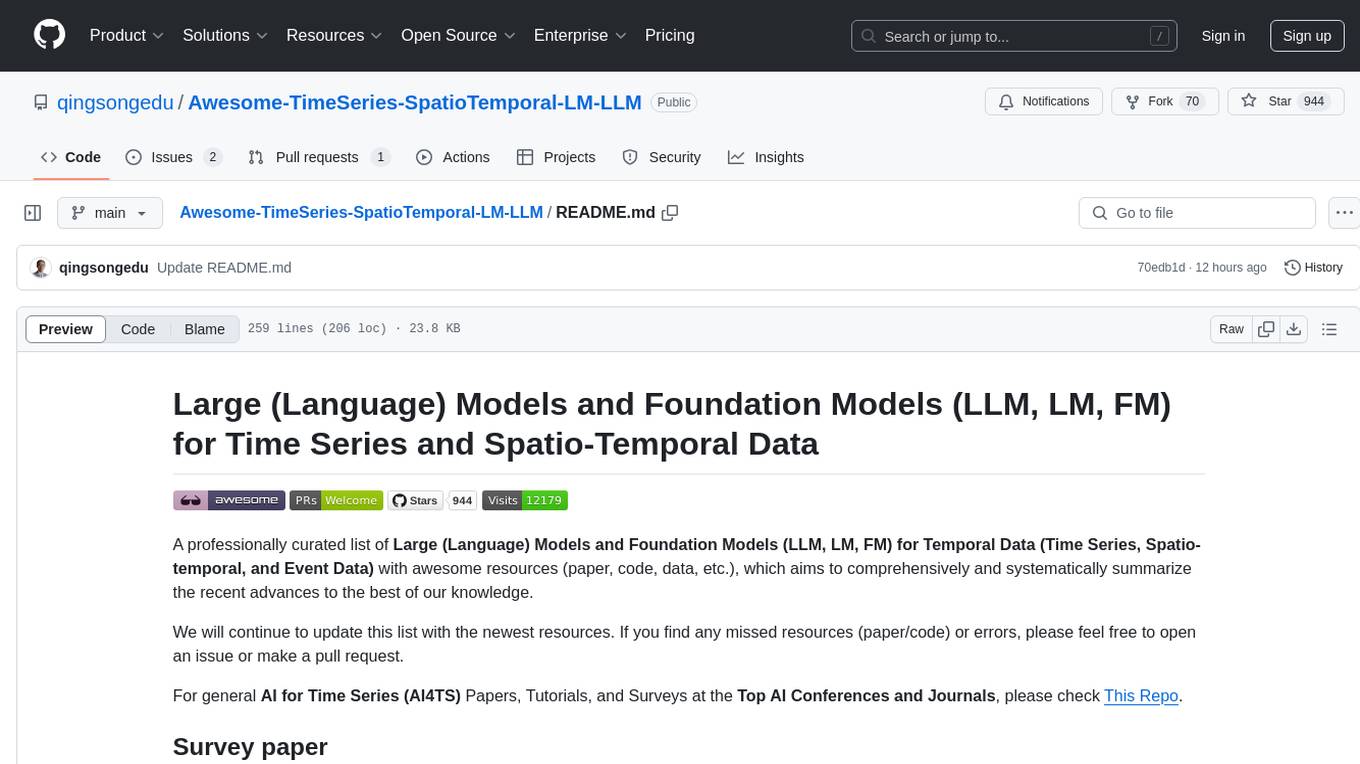
Awesome-TimeSeries-SpatioTemporal-LM-LLM
Awesome-TimeSeries-SpatioTemporal-LM-LLM is a curated list of Large (Language) Models and Foundation Models for Temporal Data, including Time Series, Spatio-temporal, and Event Data. The repository aims to summarize recent advances in Large Models and Foundation Models for Time Series and Spatio-Temporal Data with resources such as papers, code, and data. It covers various applications like General Time Series Analysis, Transportation, Finance, Healthcare, Event Analysis, Climate, Video Data, and more. The repository also includes related resources, surveys, and papers on Large Language Models, Foundation Models, and their applications in AIOps.
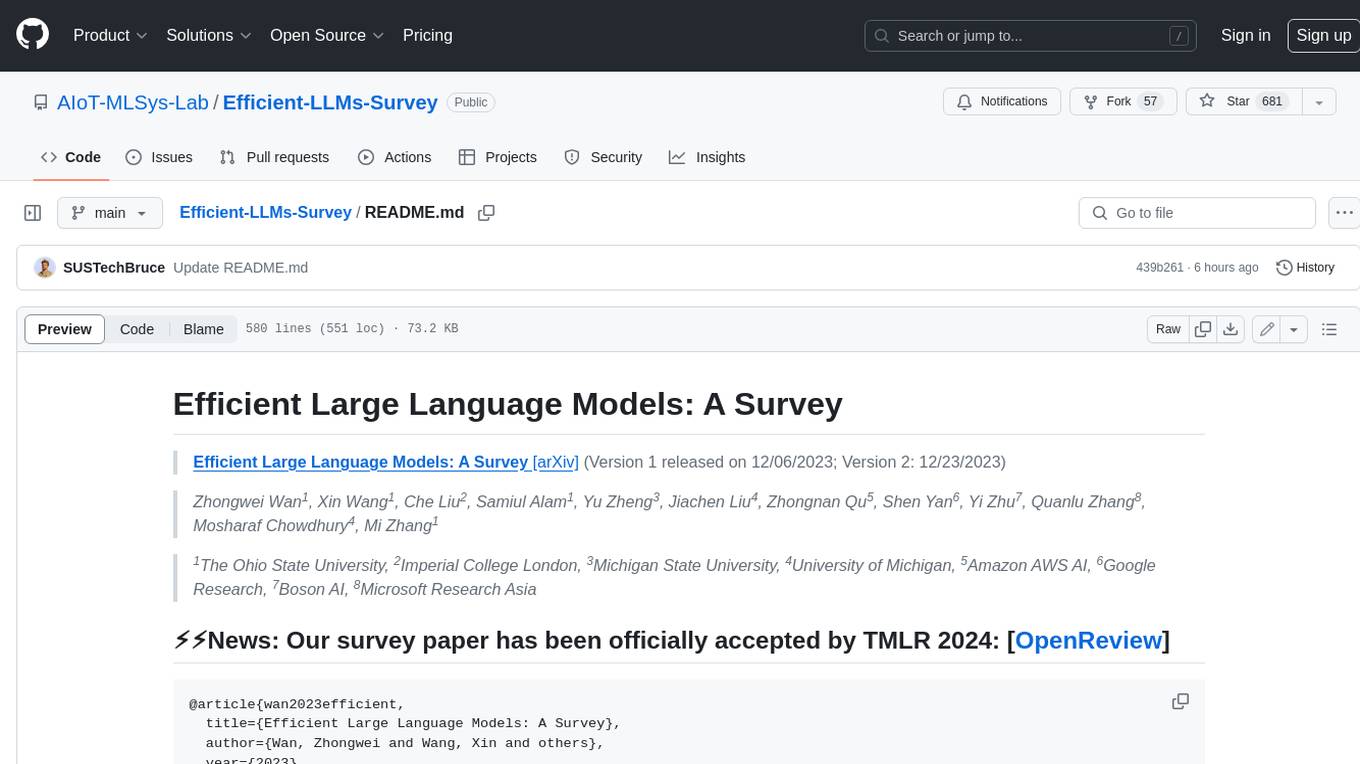
Efficient-LLMs-Survey
This repository provides a systematic and comprehensive review of efficient LLMs research. We organize the literature in a taxonomy consisting of three main categories, covering distinct yet interconnected efficient LLMs topics from **model-centric** , **data-centric** , and **framework-centric** perspective, respectively. We hope our survey and this GitHub repository can serve as valuable resources to help researchers and practitioners gain a systematic understanding of the research developments in efficient LLMs and inspire them to contribute to this important and exciting field.
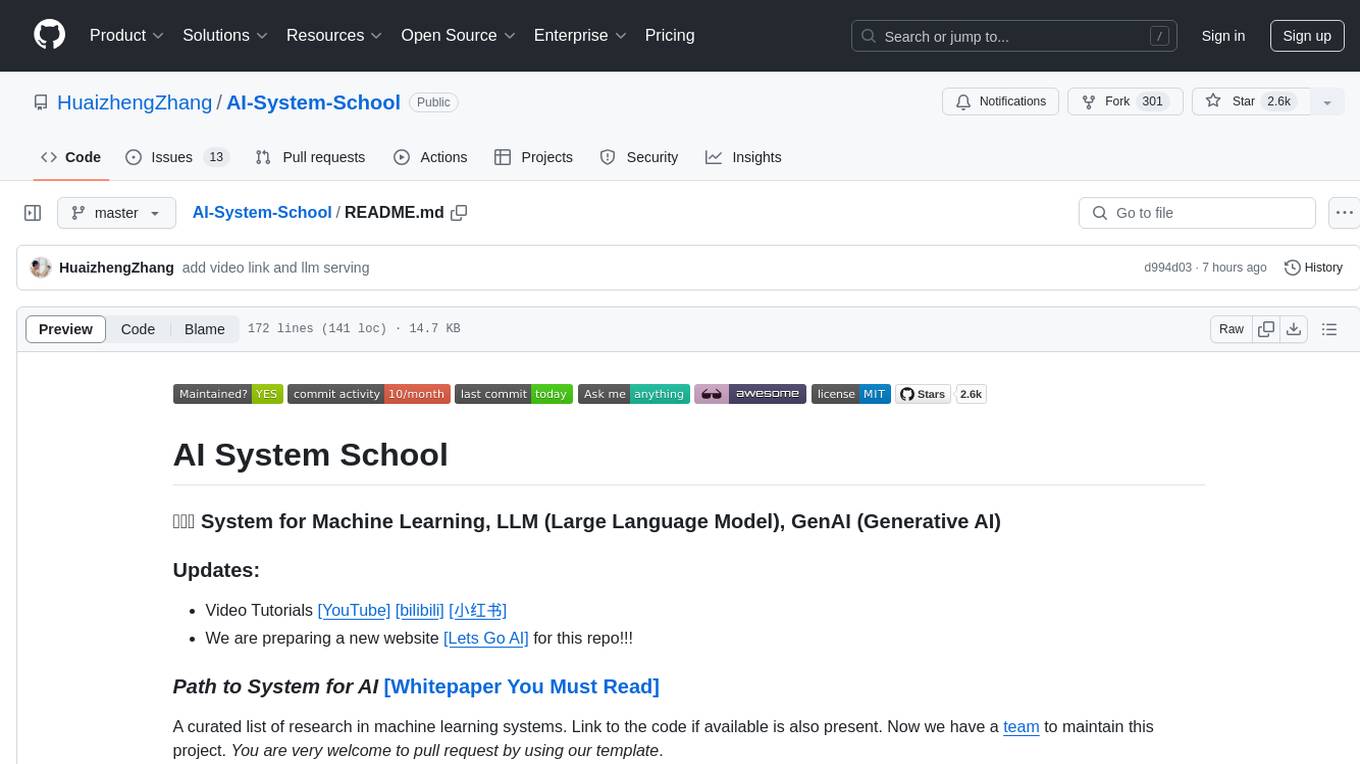
AI-System-School
AI System School is a curated list of research in machine learning systems, focusing on ML/DL infra, LLM infra, domain-specific infra, ML/LLM conferences, and general resources. It provides resources such as data processing, training systems, video systems, autoML systems, and more. The repository aims to help users navigate the landscape of AI systems and machine learning infrastructure, offering insights into conferences, surveys, books, videos, courses, and blogs related to the field.
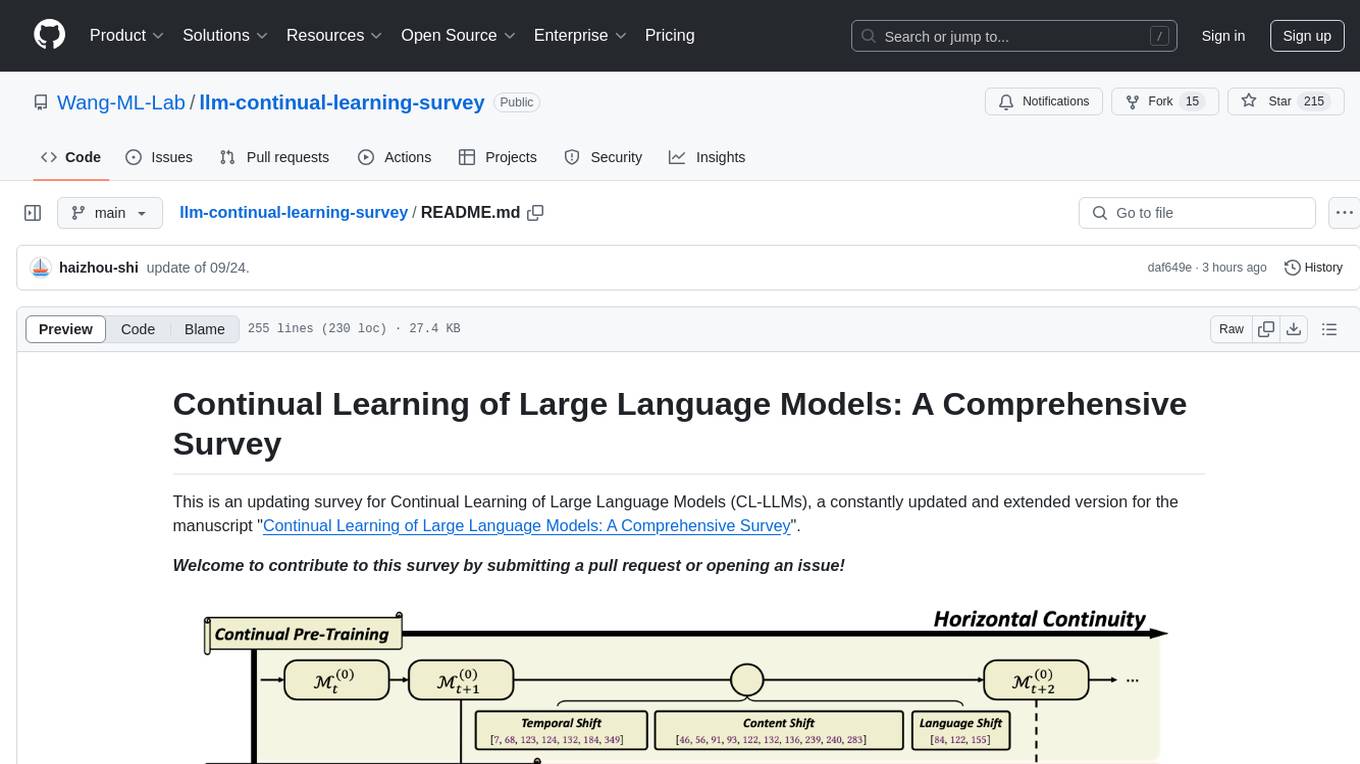
llm-continual-learning-survey
This repository is an updating survey for Continual Learning of Large Language Models (CL-LLMs), providing a comprehensive overview of various aspects related to the continual learning of large language models. It covers topics such as continual pre-training, domain-adaptive pre-training, continual fine-tuning, model refinement, model alignment, multimodal LLMs, and miscellaneous aspects. The survey includes a collection of relevant papers, each focusing on different areas within the field of continual learning of large language models.
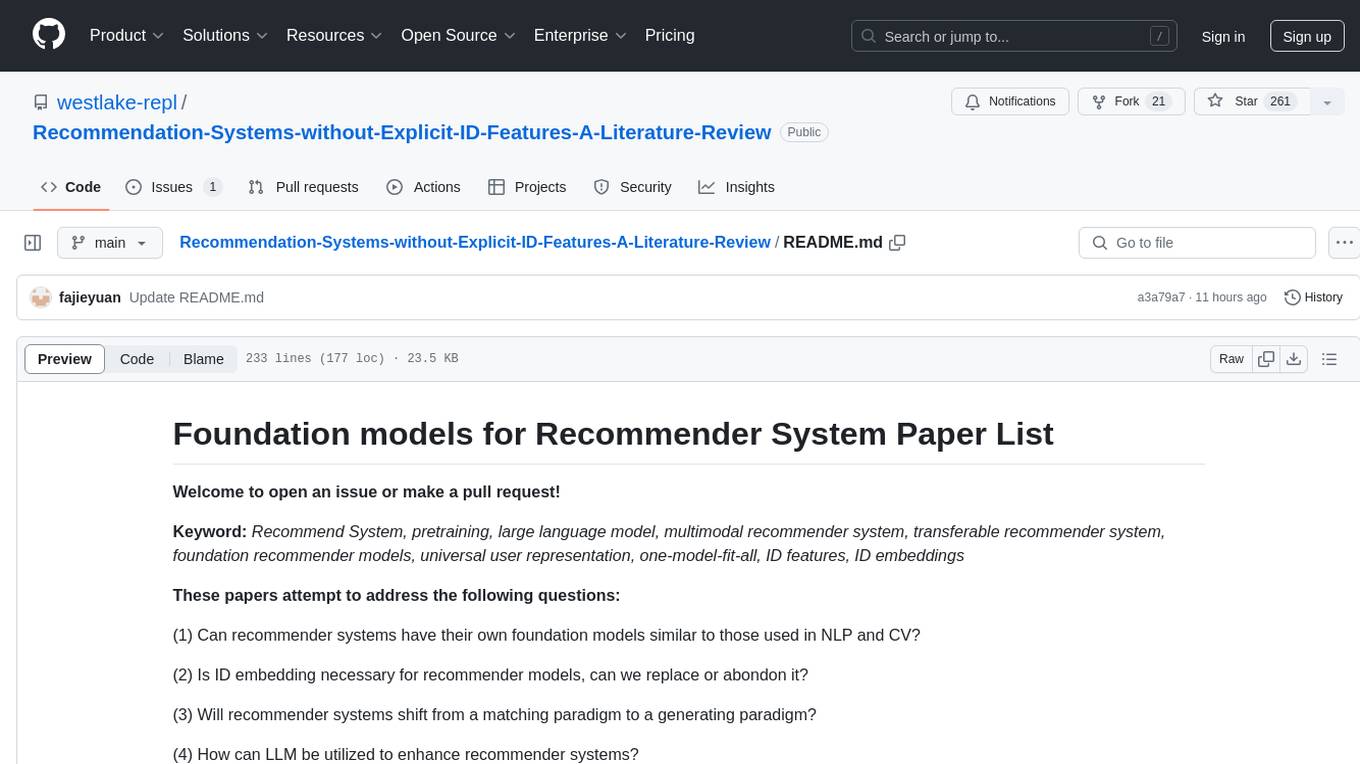
Recommendation-Systems-without-Explicit-ID-Features-A-Literature-Review
This repository is a collection of papers and resources related to recommendation systems, focusing on foundation models, transferable recommender systems, large language models, and multimodal recommender systems. It explores questions such as the necessity of ID embeddings, the shift from matching to generating paradigms, and the future of multimodal recommender systems. The papers cover various aspects of recommendation systems, including pretraining, user representation, dataset benchmarks, and evaluation methods. The repository aims to provide insights and advancements in the field of recommendation systems through literature reviews, surveys, and empirical studies.
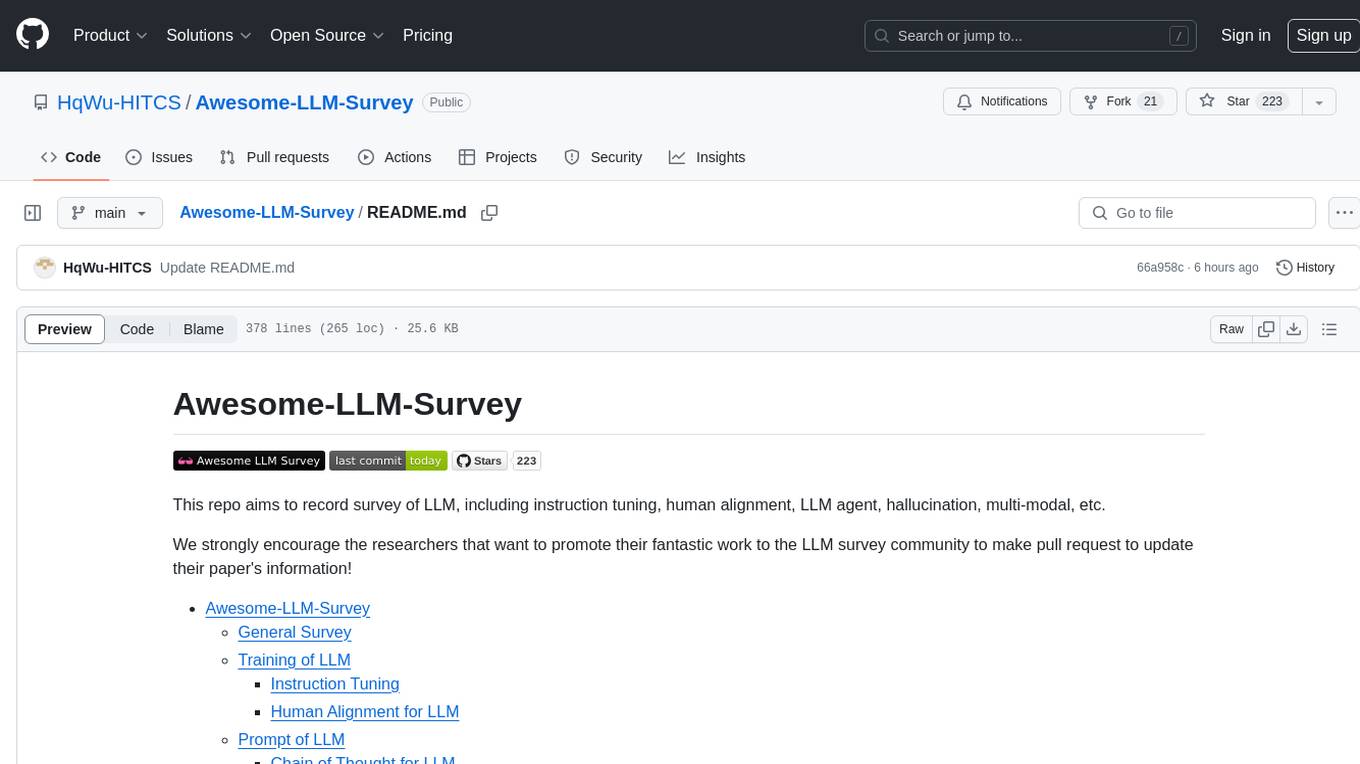
Awesome-LLM-Survey
This repository, Awesome-LLM-Survey, serves as a comprehensive collection of surveys related to Large Language Models (LLM). It covers various aspects of LLM, including instruction tuning, human alignment, LLM agents, hallucination, multi-modal capabilities, and more. Researchers are encouraged to contribute by updating information on their papers to benefit the LLM survey community.
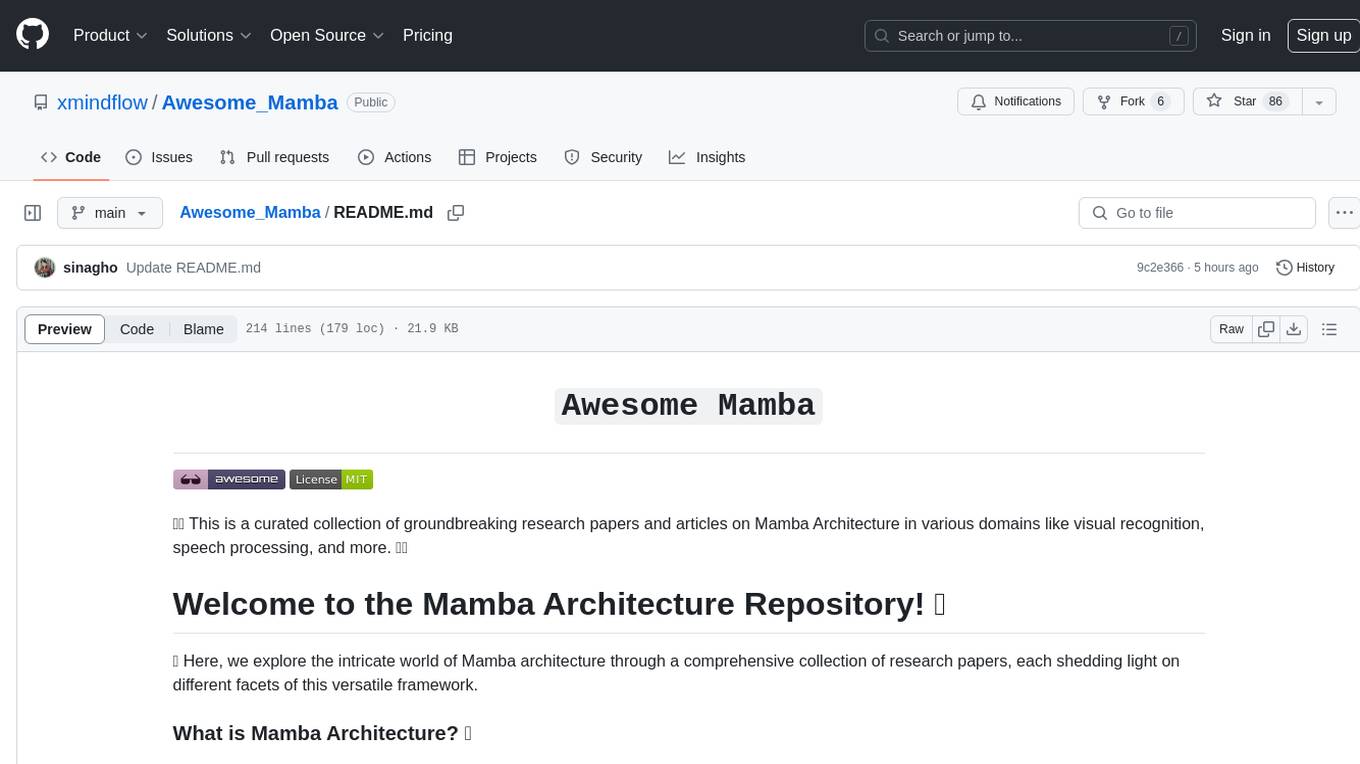
Awesome_Mamba
Awesome Mamba is a curated collection of groundbreaking research papers and articles on Mamba Architecture, a pioneering framework in deep learning known for its selective state spaces and efficiency in processing complex data structures. The repository offers a comprehensive exploration of Mamba architecture through categorized research papers covering various domains like visual recognition, speech processing, remote sensing, video processing, activity recognition, image enhancement, medical imaging, reinforcement learning, natural language processing, 3D recognition, multi-modal understanding, time series analysis, graph neural networks, point cloud analysis, and tabular data handling.
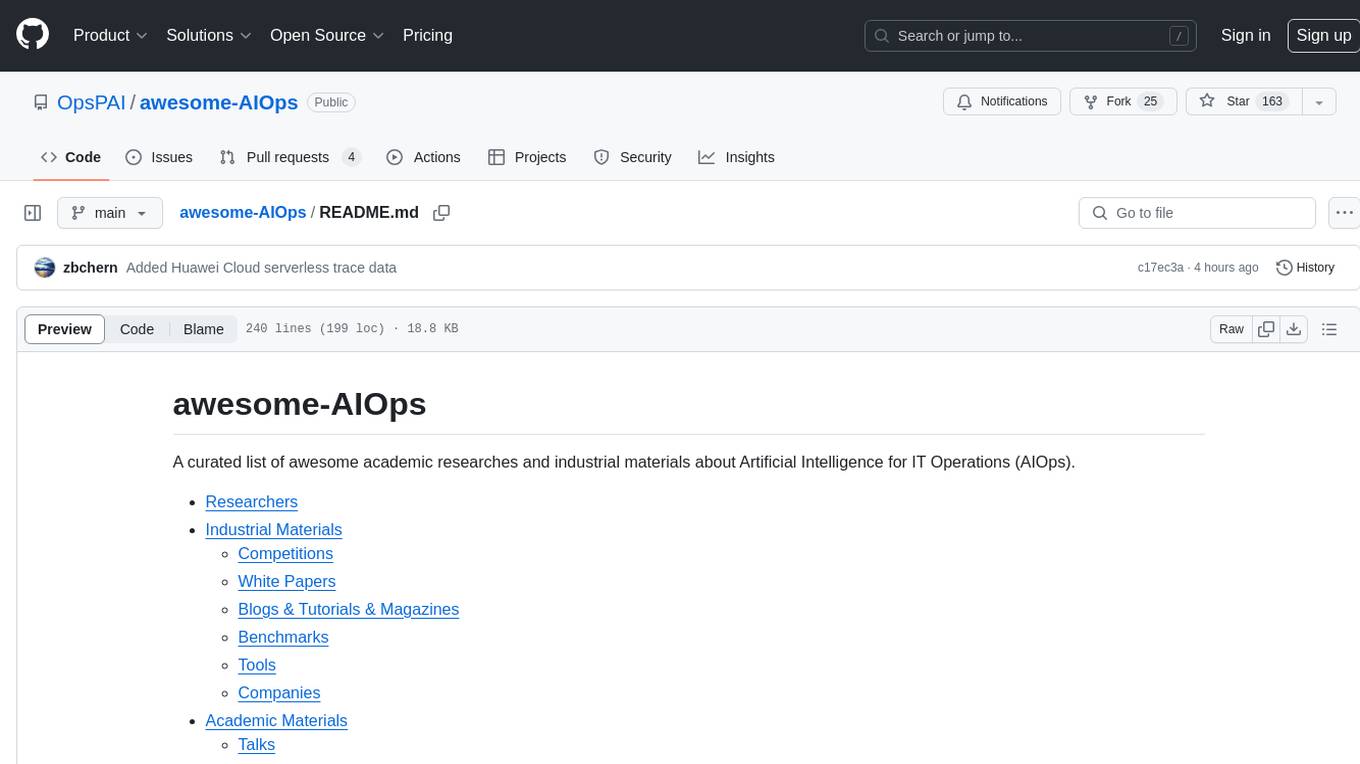
awesome-AIOps
awesome-AIOps is a curated list of academic researches and industrial materials related to Artificial Intelligence for IT Operations (AIOps). It includes resources such as competitions, white papers, blogs, tutorials, benchmarks, tools, companies, academic materials, talks, workshops, papers, and courses covering various aspects of AIOps like anomaly detection, root cause analysis, incident management, microservices, dependency tracing, and more.
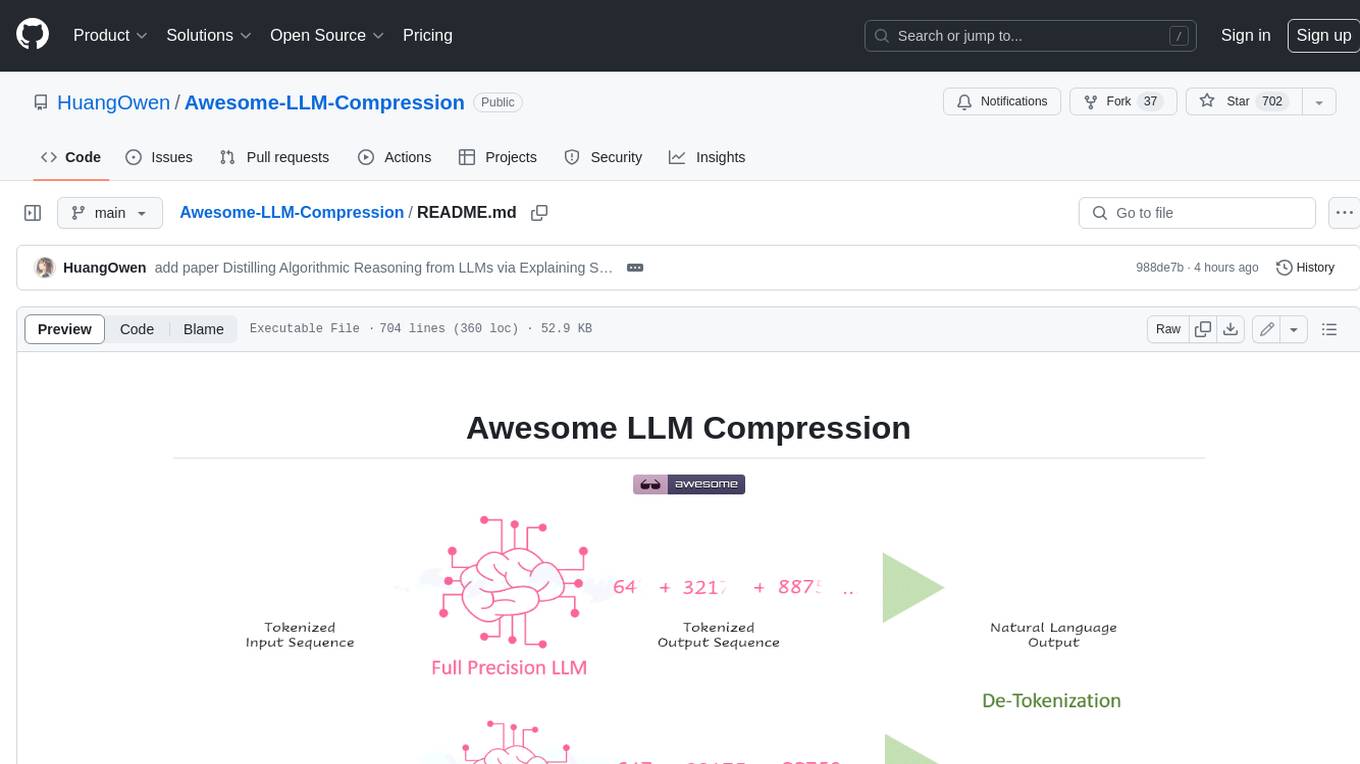
Awesome-LLM-Compression
Awesome LLM compression research papers and tools to accelerate LLM training and inference.
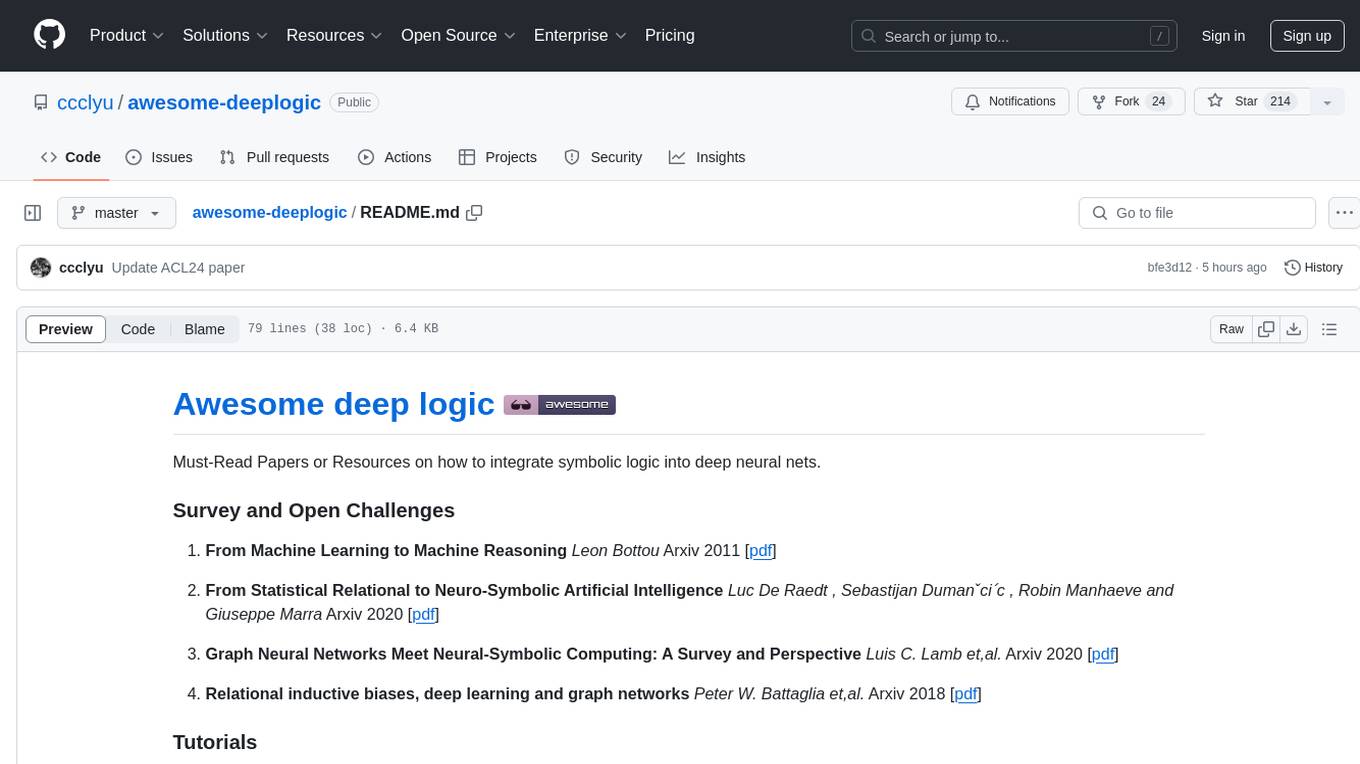
awesome-deeplogic
Awesome deep logic is a curated list of papers and resources focusing on integrating symbolic logic into deep neural networks. It includes surveys, tutorials, and research papers that explore the intersection of logic and deep learning. The repository aims to provide valuable insights and knowledge on how logic can be used to enhance reasoning, knowledge regularization, weak supervision, and explainability in neural networks.
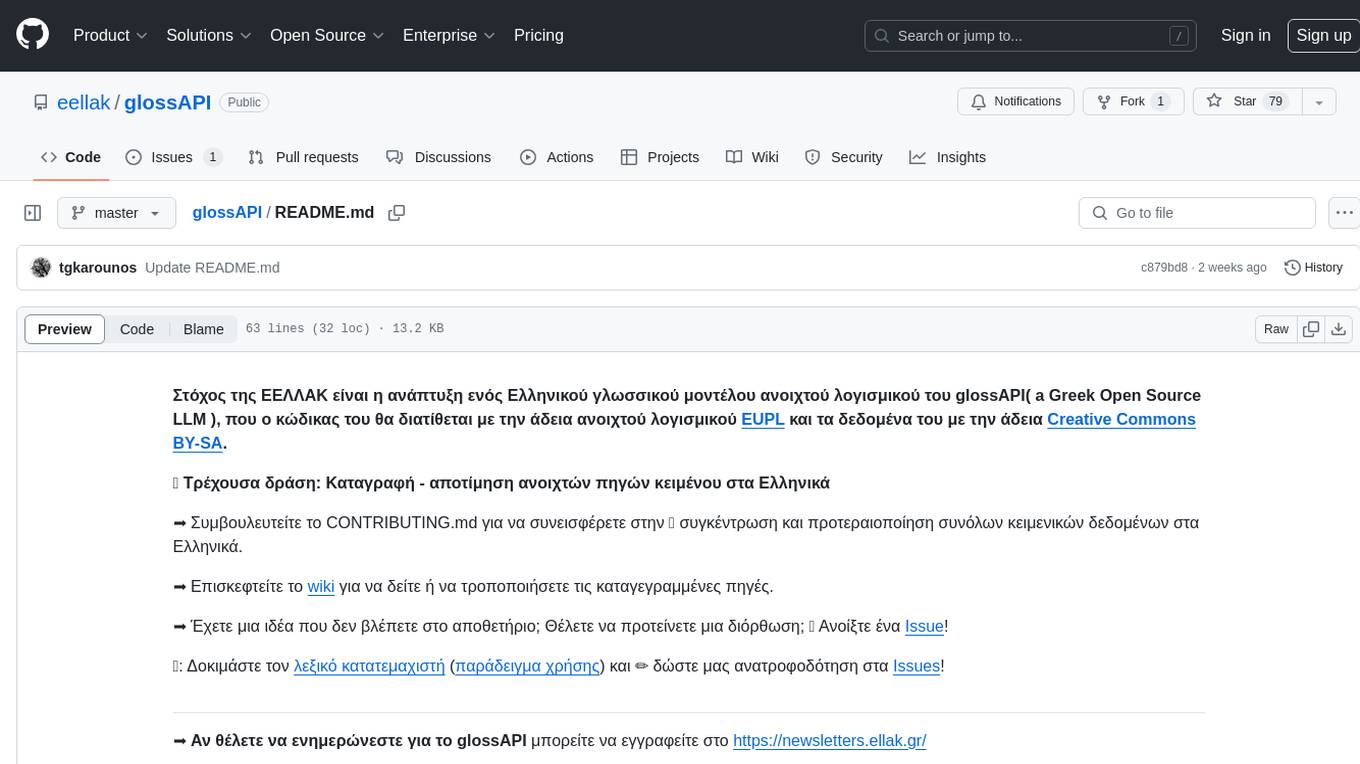
glossAPI
The glossAPI project aims to develop a Greek language model as open-source software, with code licensed under EUPL and data under Creative Commons BY-SA. The project focuses on collecting and evaluating open text sources in Greek, with efforts to prioritize and gather textual data sets. The project encourages contributions through the CONTRIBUTING.md file and provides resources in the wiki for viewing and modifying recorded sources. It also welcomes ideas and corrections through issue submissions. The project emphasizes the importance of open standards, ethically secured data, privacy protection, and addressing digital divides in the context of artificial intelligence and advanced language technologies.
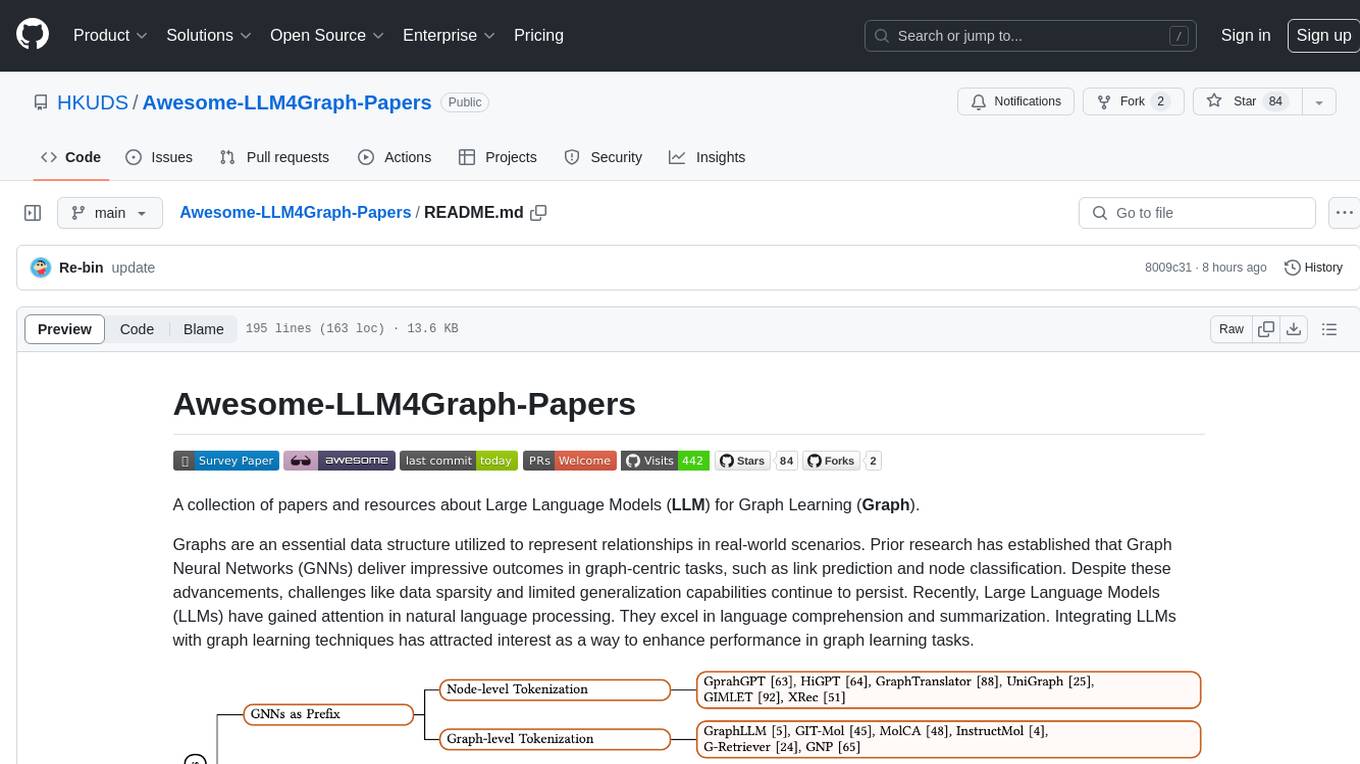
Awesome-LLM4Graph-Papers
A collection of papers and resources about Large Language Models (LLM) for Graph Learning (Graph). Integrating LLMs with graph learning techniques to enhance performance in graph learning tasks. Categorizes approaches based on four primary paradigms and nine secondary-level categories. Valuable for research or practice in self-supervised learning for recommendation systems.
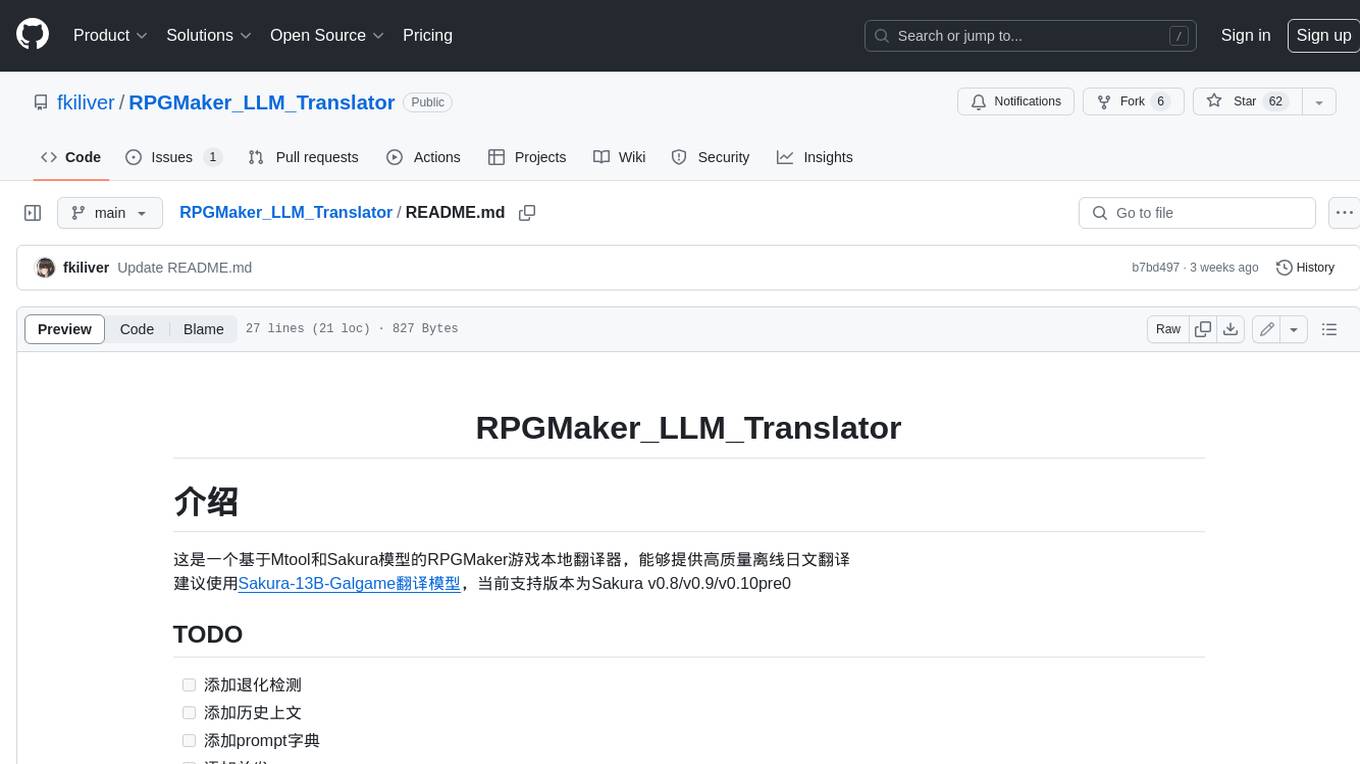
RPGMaker_LLM_Translator
This is an offline Japanese translator for RPGMaker games based on Mtool and the Sakura model, capable of providing high-quality offline Japanese translations. It is recommended to use the Sakura-13B-Galgame translation model, and the currently supported versions are Sakura v0.8/v0.9/v0.10pre0.
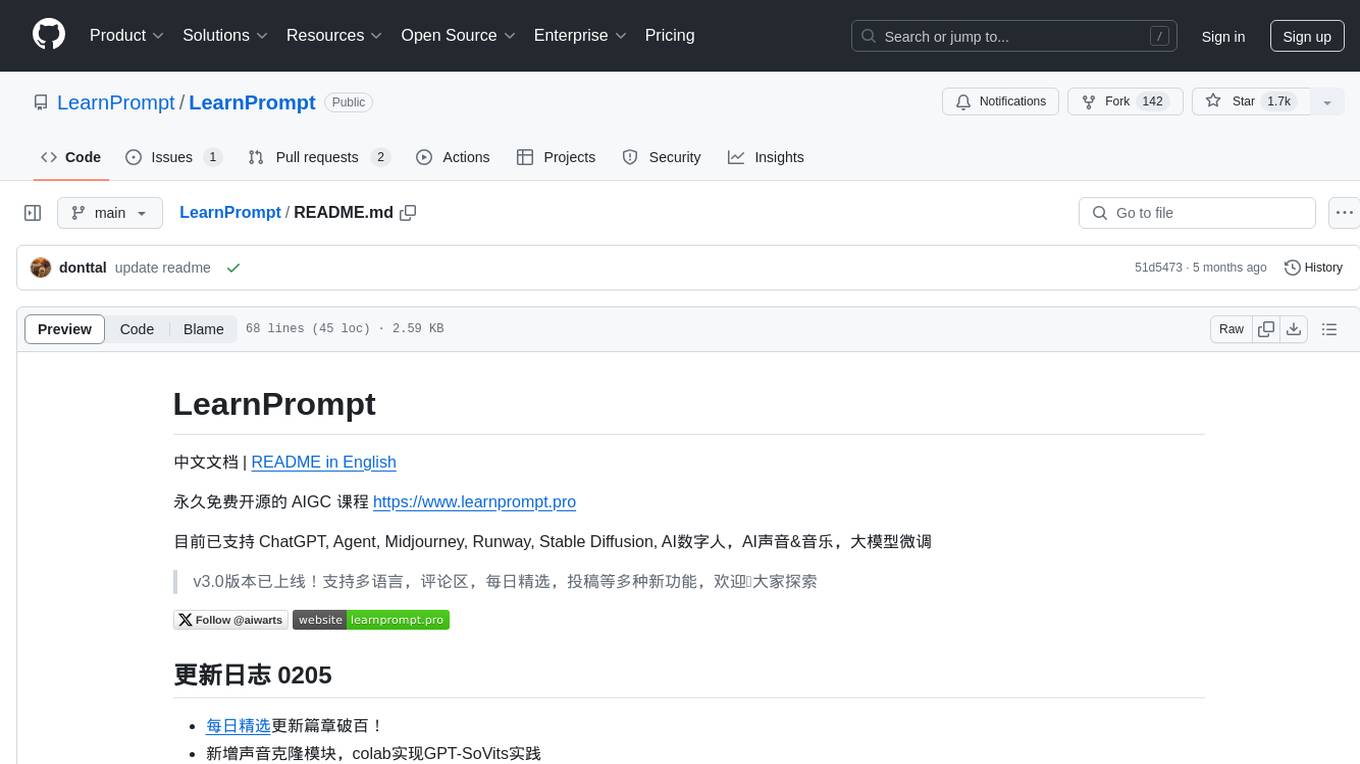
LearnPrompt
LearnPrompt is a permanent, free, open-source AIGC course platform that currently supports various tools like ChatGPT, Agent, Midjourney, Runway, Stable Diffusion, AI digital humans, AI voice & music, and large model fine-tuning. The platform offers features such as multilingual support, comment sections, daily selections, and submissions. Users can explore different modules, including sound cloning, RAG, GPT-SoVits, and OpenAI Sora world model. The platform aims to continuously update and provide tutorials, examples, and knowledge systems related to AI technologies.
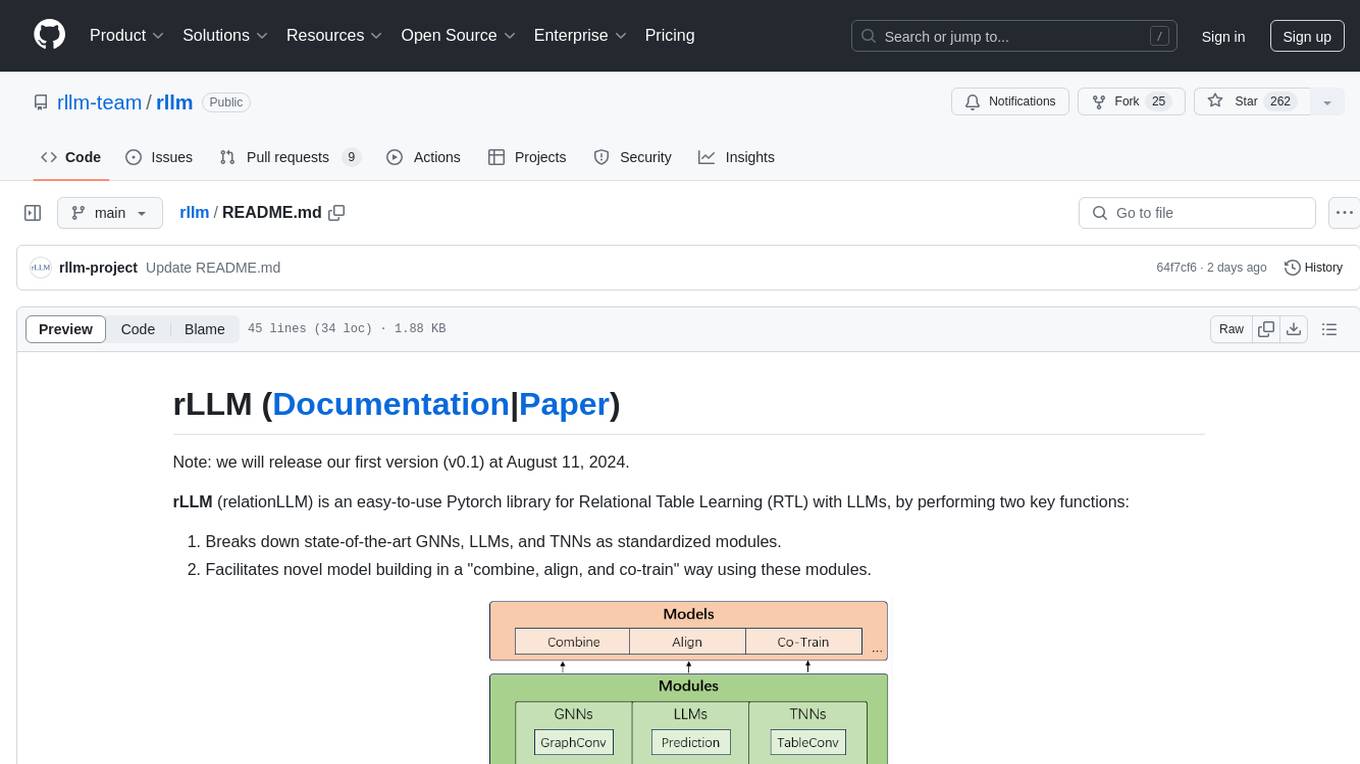
rllm
rLLM (relationLLM) is a Pytorch library for Relational Table Learning (RTL) with LLMs. It breaks down state-of-the-art GNNs, LLMs, and TNNs as standardized modules and facilitates novel model building in a 'combine, align, and co-train' way using these modules. The library is LLM-friendly, processes various graphs as multiple tables linked by foreign keys, introduces new relational table datasets, and is supported by students and teachers from Shanghai Jiao Tong University and Tsinghua University.
For similar tasks
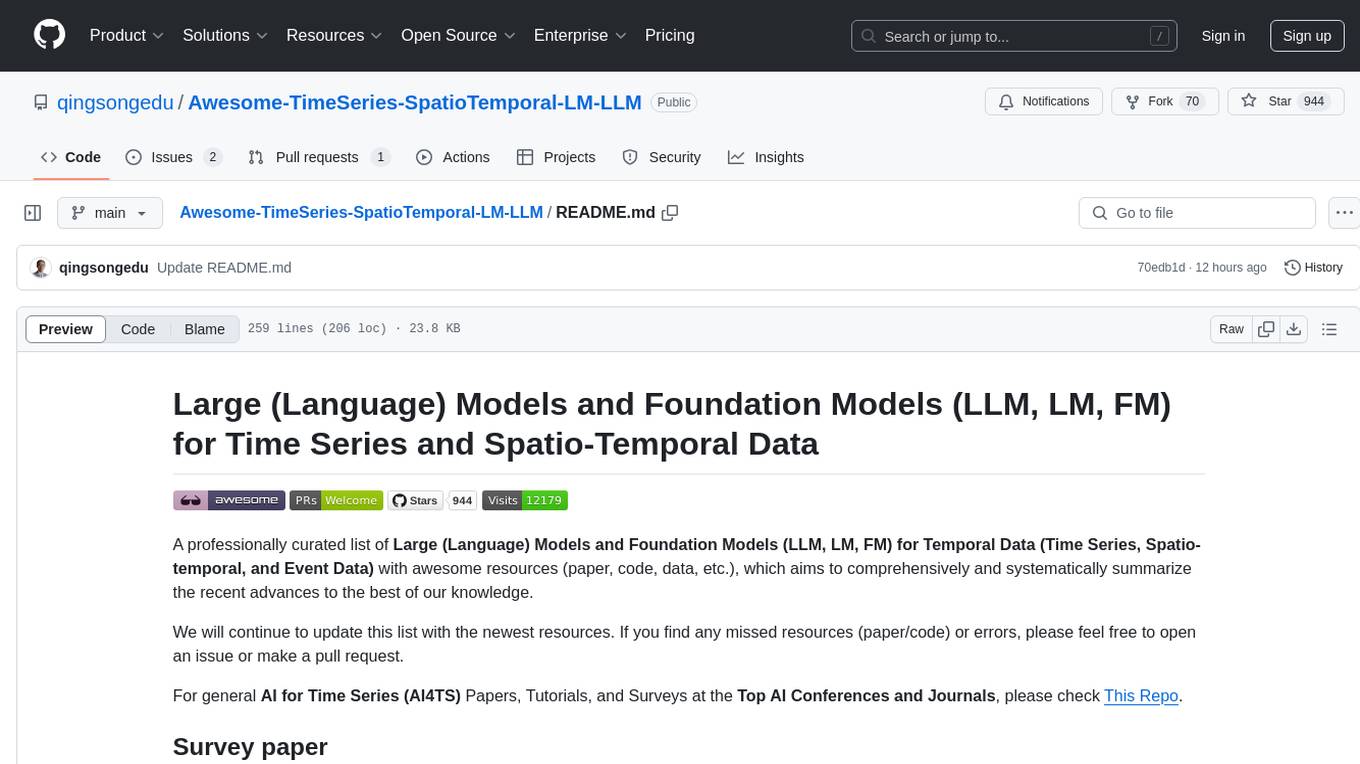
Awesome-TimeSeries-SpatioTemporal-LM-LLM
Awesome-TimeSeries-SpatioTemporal-LM-LLM is a curated list of Large (Language) Models and Foundation Models for Temporal Data, including Time Series, Spatio-temporal, and Event Data. The repository aims to summarize recent advances in Large Models and Foundation Models for Time Series and Spatio-Temporal Data with resources such as papers, code, and data. It covers various applications like General Time Series Analysis, Transportation, Finance, Healthcare, Event Analysis, Climate, Video Data, and more. The repository also includes related resources, surveys, and papers on Large Language Models, Foundation Models, and their applications in AIOps.
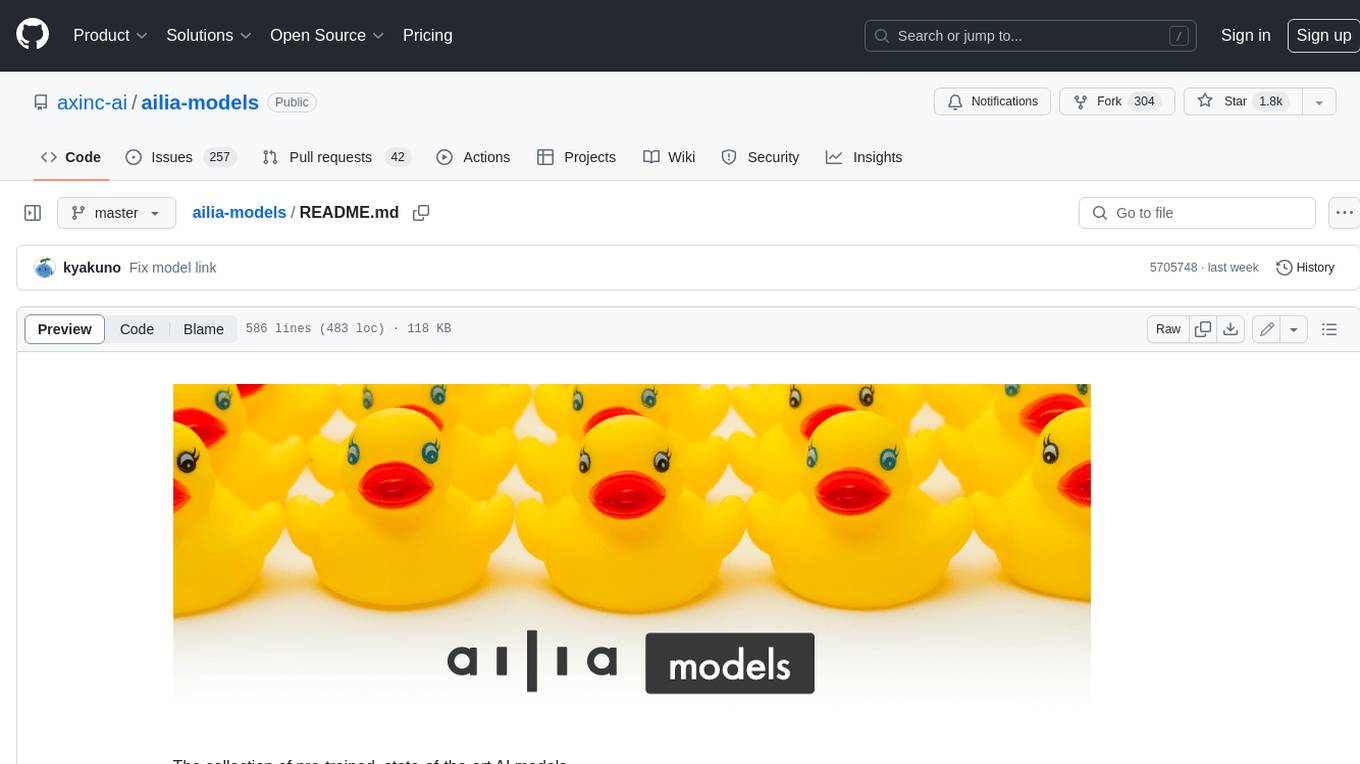
ailia-models
The collection of pre-trained, state-of-the-art AI models. ailia SDK is a self-contained, cross-platform, high-speed inference SDK for AI. The ailia SDK provides a consistent C++ API across Windows, Mac, Linux, iOS, Android, Jetson, and Raspberry Pi platforms. It also supports Unity (C#), Python, Rust, Flutter(Dart) and JNI for efficient AI implementation. The ailia SDK makes extensive use of the GPU through Vulkan and Metal to enable accelerated computing. # Supported models 323 models as of April 8th, 2024
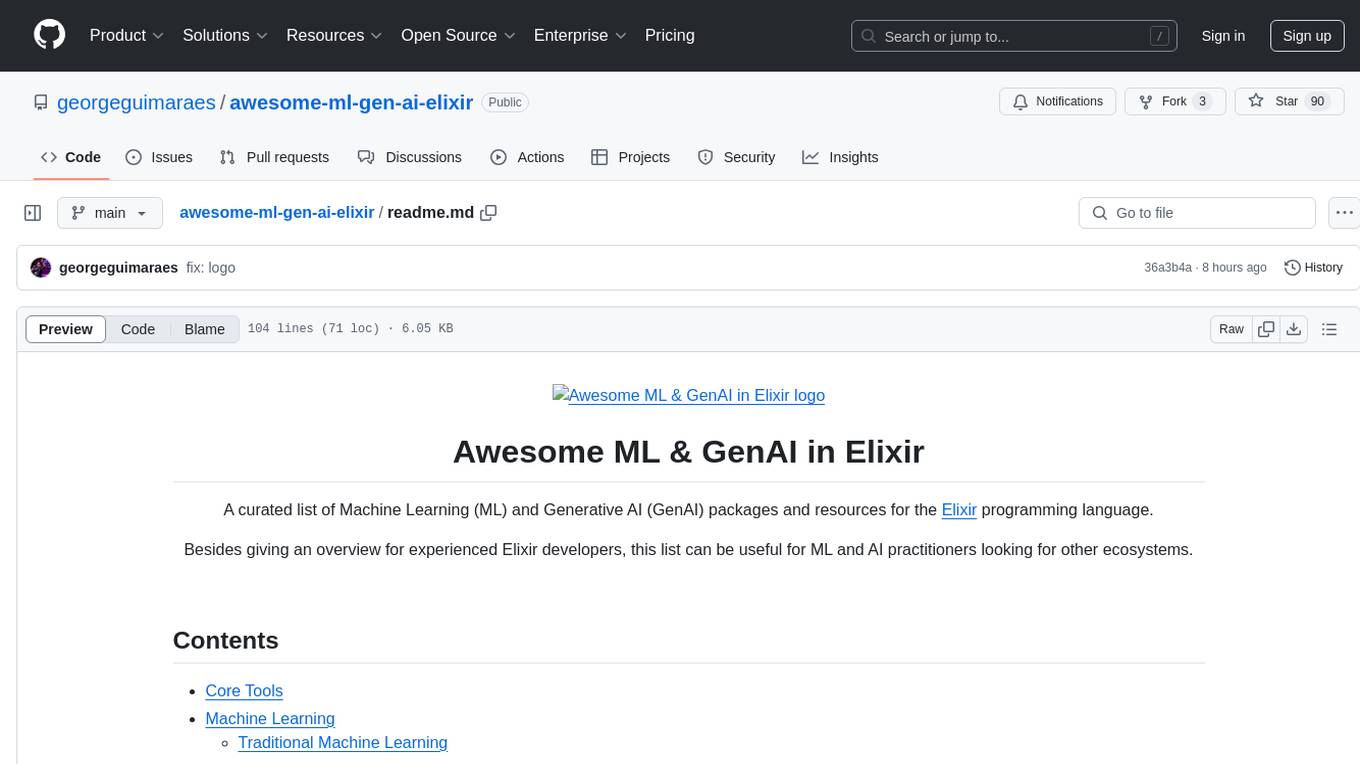
awesome-ml-gen-ai-elixir
A curated list of Machine Learning (ML) and Generative AI (GenAI) packages and resources for the Elixir programming language. It includes core tools for data exploration, traditional machine learning algorithms, deep learning models, computer vision libraries, generative AI tools, livebooks for interactive notebooks, and various resources such as books, videos, and articles. The repository aims to provide a comprehensive overview for experienced Elixir developers and ML/AI practitioners exploring different ecosystems.
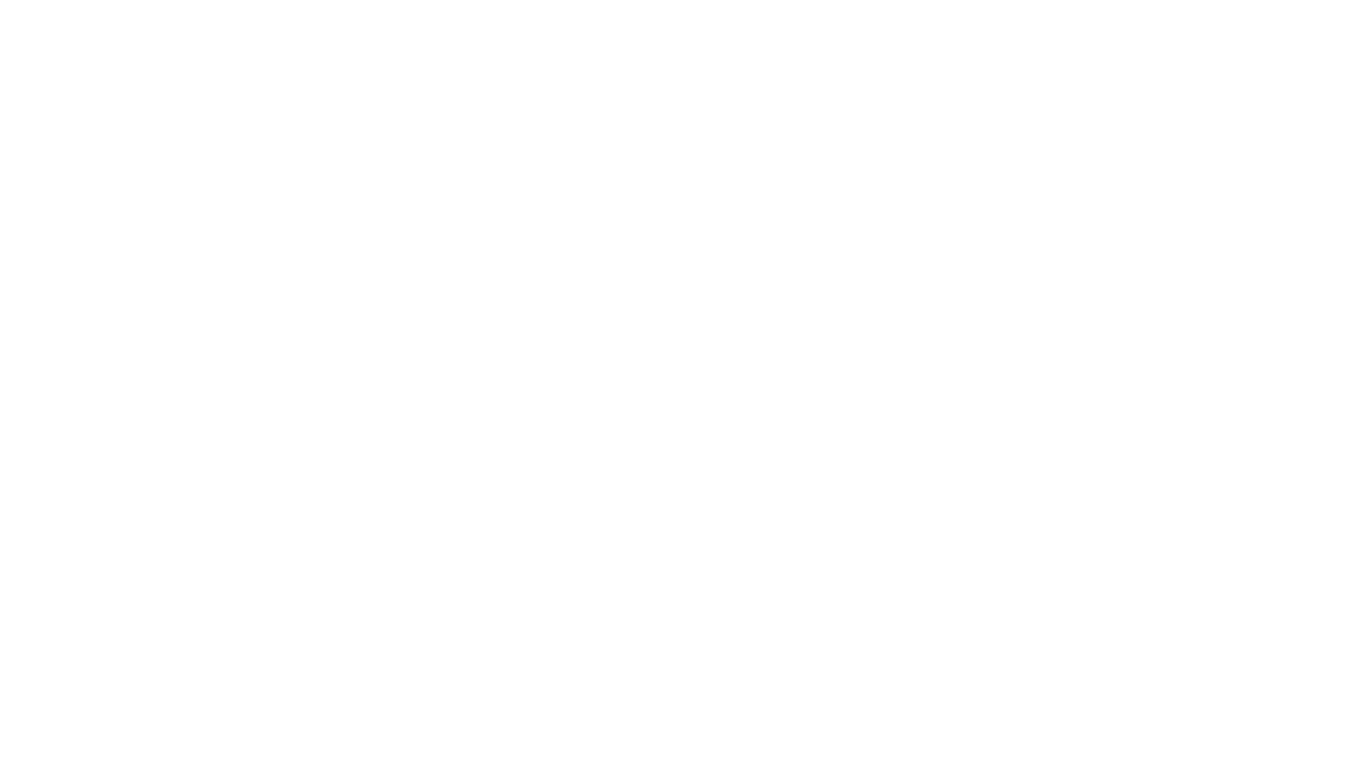
PIXIU
PIXIU is a project designed to support the development, fine-tuning, and evaluation of Large Language Models (LLMs) in the financial domain. It includes components like FinBen, a Financial Language Understanding and Prediction Evaluation Benchmark, FIT, a Financial Instruction Dataset, and FinMA, a Financial Large Language Model. The project provides open resources, multi-task and multi-modal financial data, and diverse financial tasks for training and evaluation. It aims to encourage open research and transparency in the financial NLP field.
For similar jobs
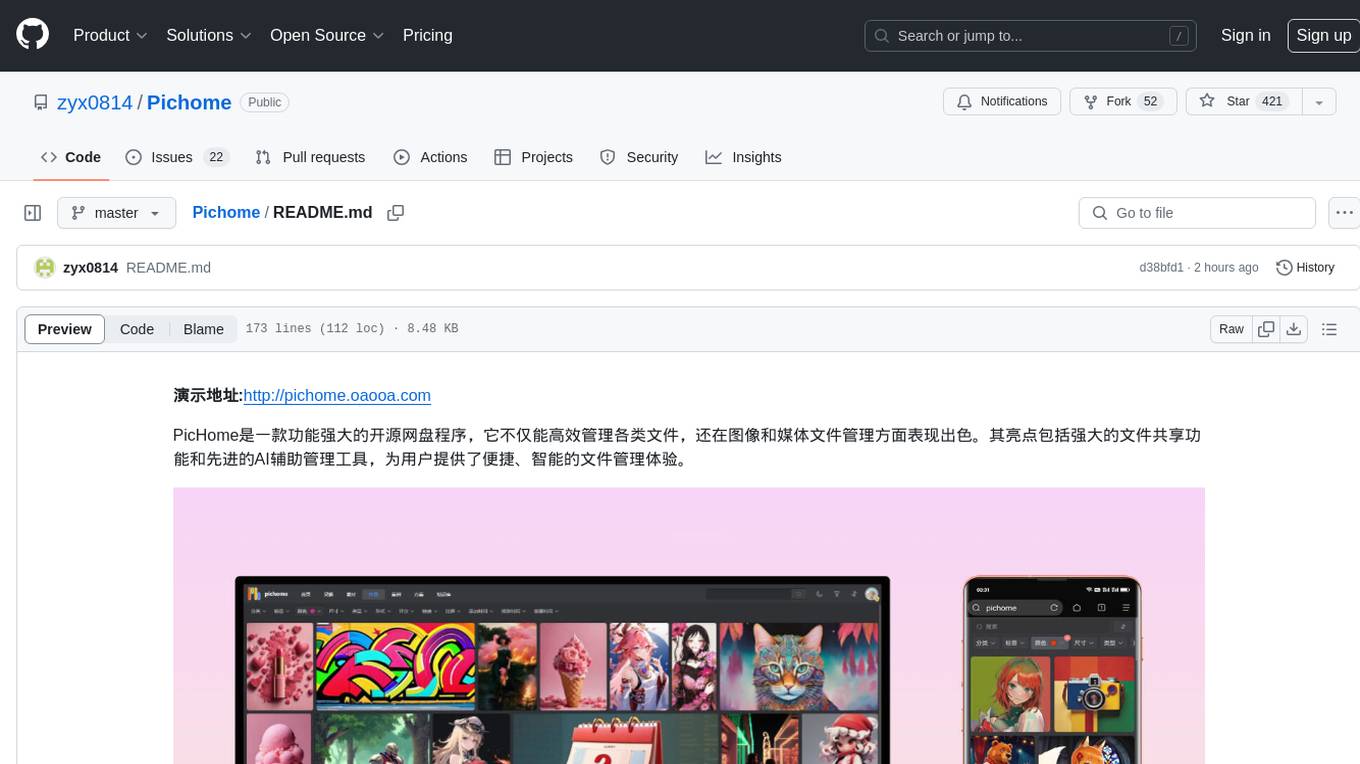
Pichome
PicHome is a powerful open-source cloud storage program that efficiently manages various types of files and excels in image and media file management. Its highlights include robust file sharing features and advanced AI-assisted management tools, providing users with a convenient and intelligent file management experience. The program offers diverse list modes, customizable file information display, enhanced quick file preview, advanced tagging, custom cover and preview images, multiple preview images, and multi-library management. Additionally, PicHome features strong file sharing capabilities, allowing users to share entire libraries, create personalized showcase web pages, and build complete data sharing websites. The AI-assisted management aspect includes AI file renaming, tagging, description writing, batch annotation, and file Q&A services, all aimed at improving file management efficiency. PicHome supports a wide range of file formats and can be applied in various scenarios such as e-commerce, gaming, design, development, enterprises, schools, labs, media, and entertainment institutions.
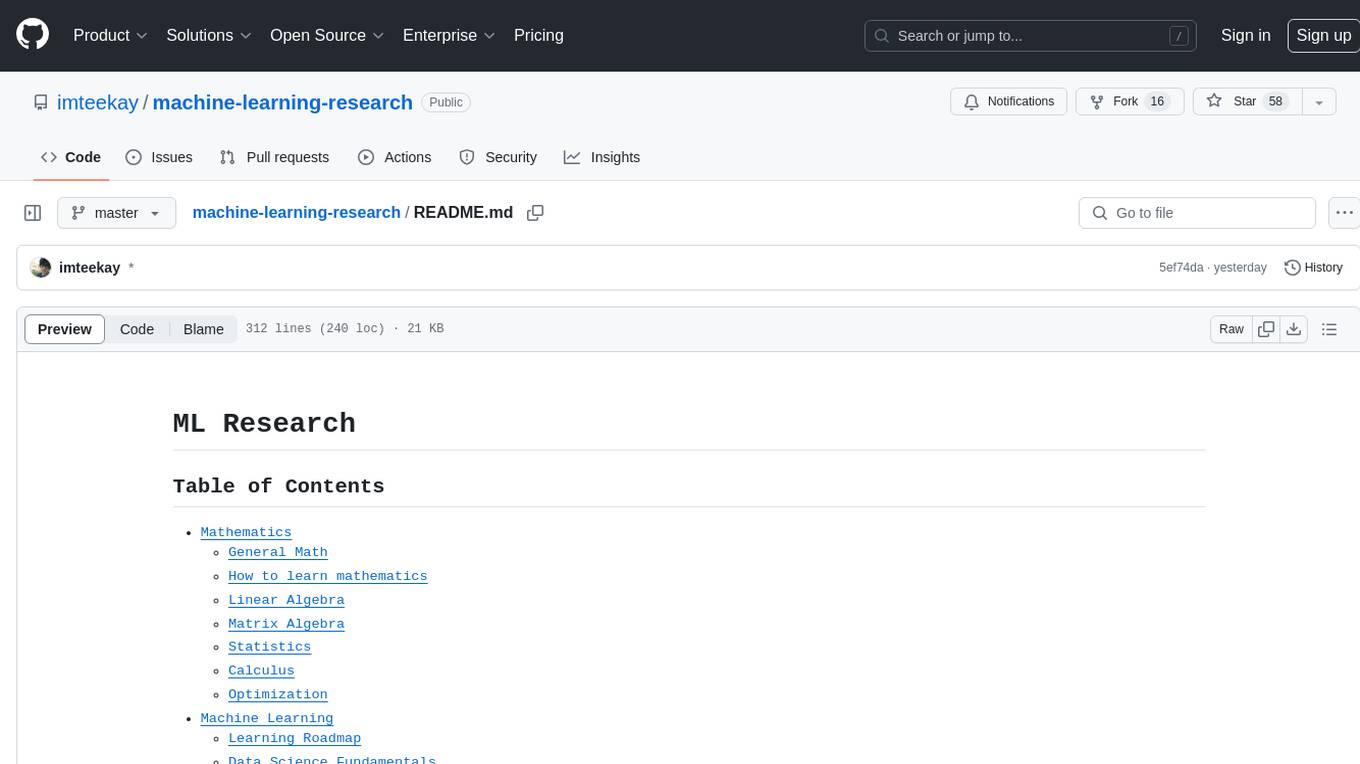
machine-learning-research
The 'machine-learning-research' repository is a comprehensive collection of resources related to mathematics, machine learning, deep learning, artificial intelligence, data science, and various scientific fields. It includes materials such as courses, tutorials, books, podcasts, communities, online courses, papers, and dissertations. The repository covers topics ranging from fundamental math skills to advanced machine learning concepts, with a focus on applications in healthcare, genetics, computational biology, precision health, and AI in science. It serves as a valuable resource for individuals interested in learning and researching in the fields of machine learning and related disciplines.
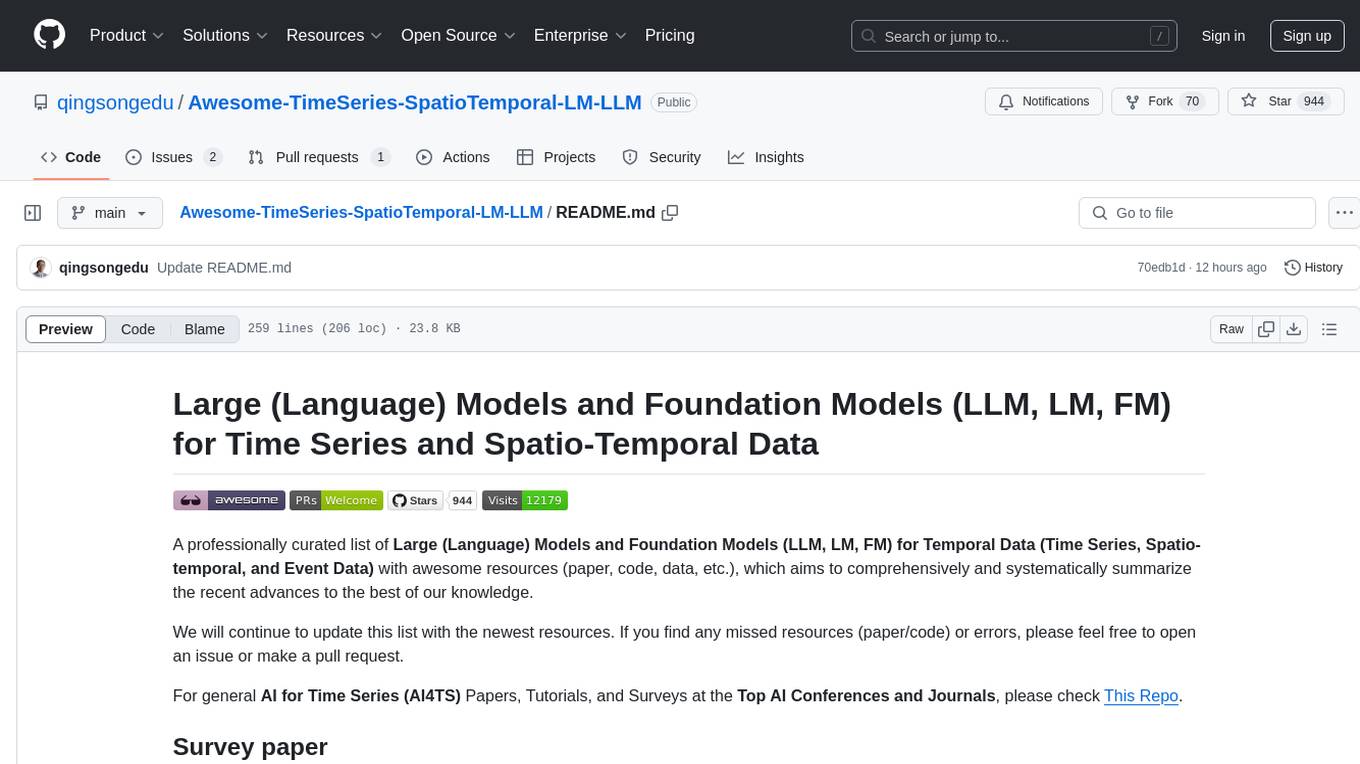
Awesome-TimeSeries-SpatioTemporal-LM-LLM
Awesome-TimeSeries-SpatioTemporal-LM-LLM is a curated list of Large (Language) Models and Foundation Models for Temporal Data, including Time Series, Spatio-temporal, and Event Data. The repository aims to summarize recent advances in Large Models and Foundation Models for Time Series and Spatio-Temporal Data with resources such as papers, code, and data. It covers various applications like General Time Series Analysis, Transportation, Finance, Healthcare, Event Analysis, Climate, Video Data, and more. The repository also includes related resources, surveys, and papers on Large Language Models, Foundation Models, and their applications in AIOps.
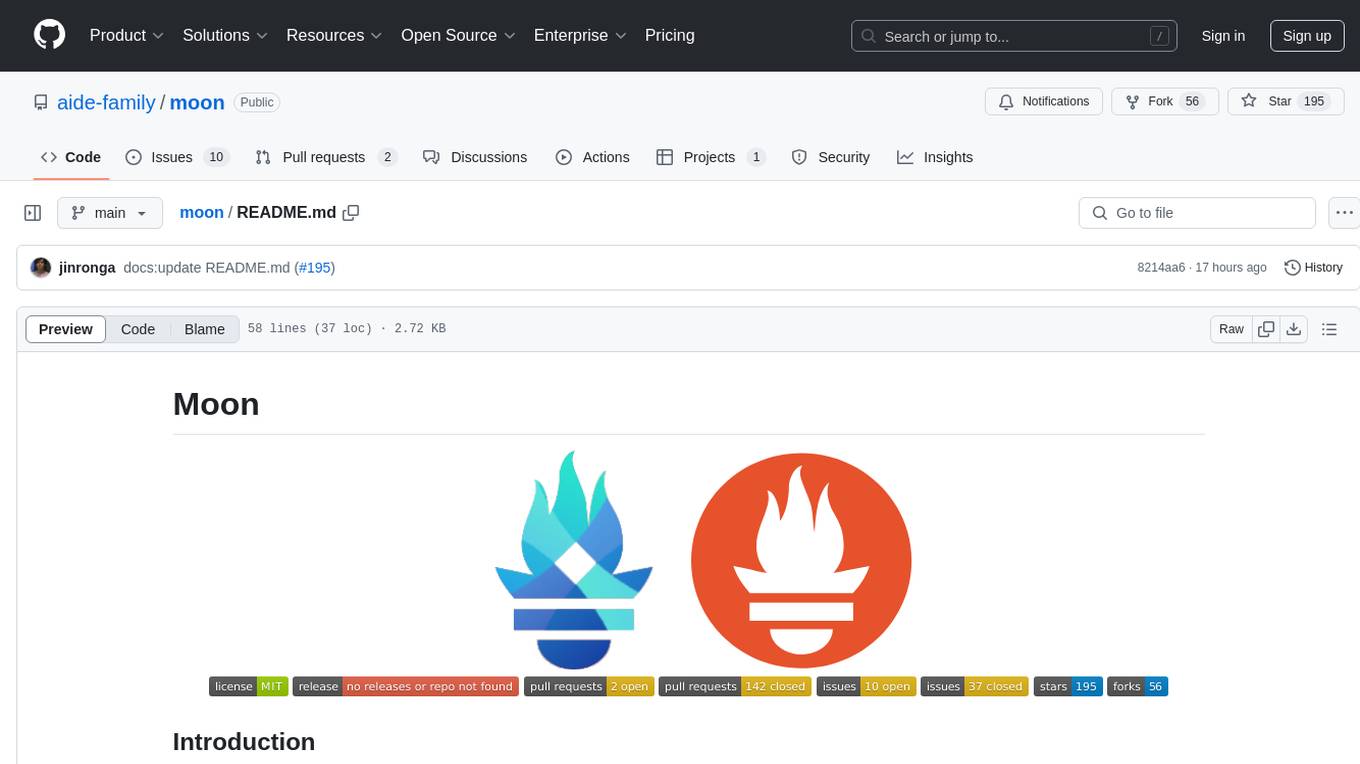
moon
Moon is a monitoring and alerting platform suitable for multiple domains, supporting various application scenarios such as cloud-native, Internet of Things (IoT), and Artificial Intelligence (AI). It simplifies operational work of cloud-native monitoring, boasts strong IoT and AI support capabilities, and meets diverse monitoring needs across industries. Capable of real-time data monitoring, intelligent alerts, and fault response for various fields.
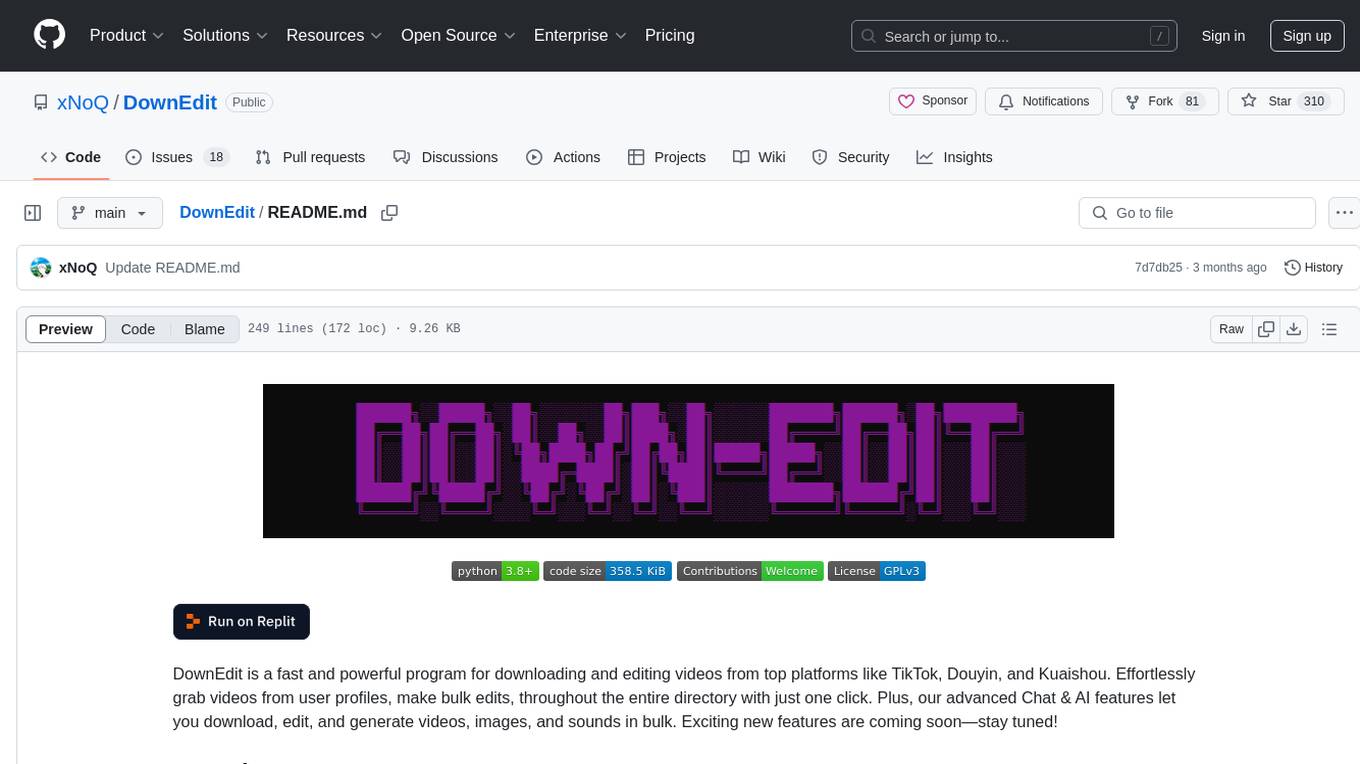
DownEdit
DownEdit is a fast and powerful program for downloading and editing videos from platforms like TikTok, Douyin, and Kuaishou. It allows users to effortlessly grab videos, make bulk edits, and utilize advanced AI features for generating videos, images, and sounds in bulk. The tool offers features like video, photo, and sound editing, downloading videos without watermarks, bulk AI generation, and AI editing for content enhancement.
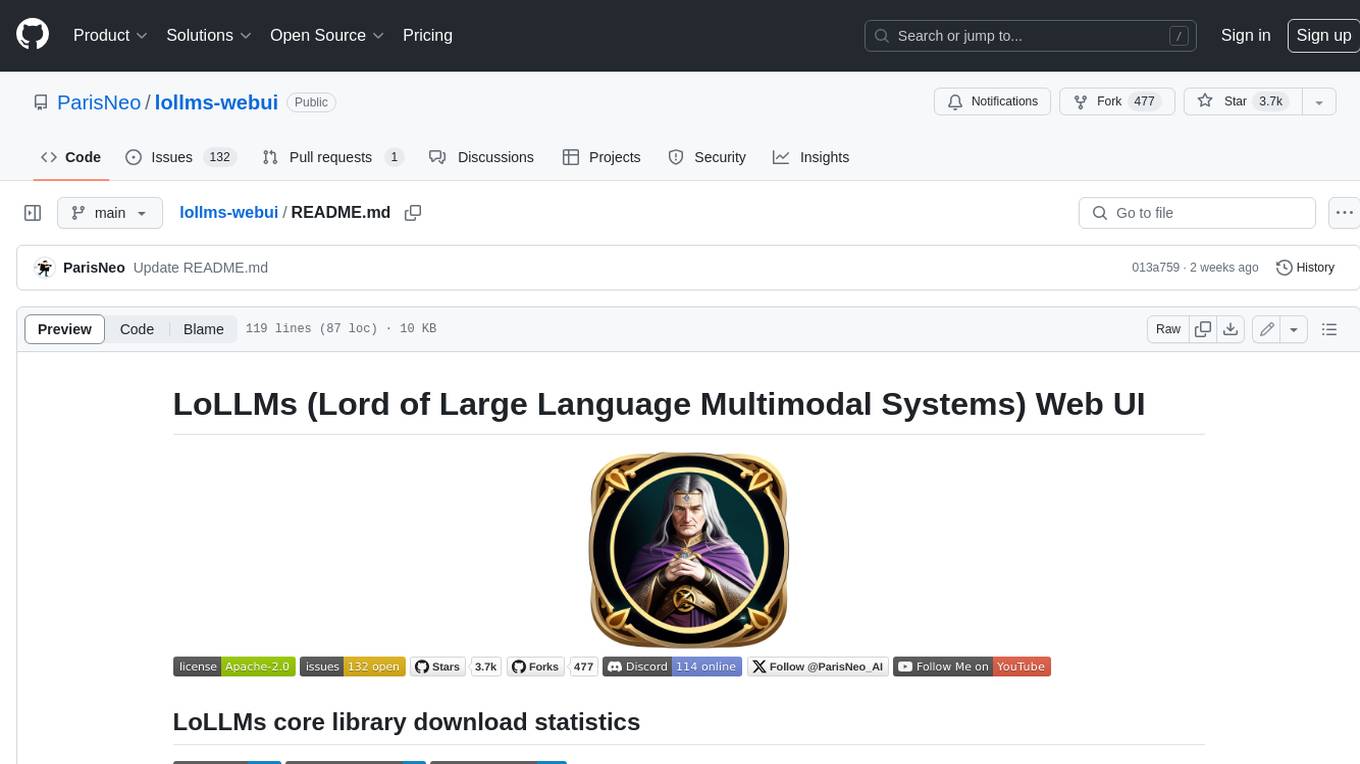
lollms-webui
LoLLMs WebUI (Lord of Large Language Multimodal Systems: One tool to rule them all) is a user-friendly interface to access and utilize various LLM (Large Language Models) and other AI models for a wide range of tasks. With over 500 AI expert conditionings across diverse domains and more than 2500 fine tuned models over multiple domains, LoLLMs WebUI provides an immediate resource for any problem, from car repair to coding assistance, legal matters, medical diagnosis, entertainment, and more. The easy-to-use UI with light and dark mode options, integration with GitHub repository, support for different personalities, and features like thumb up/down rating, copy, edit, and remove messages, local database storage, search, export, and delete multiple discussions, make LoLLMs WebUI a powerful and versatile tool.
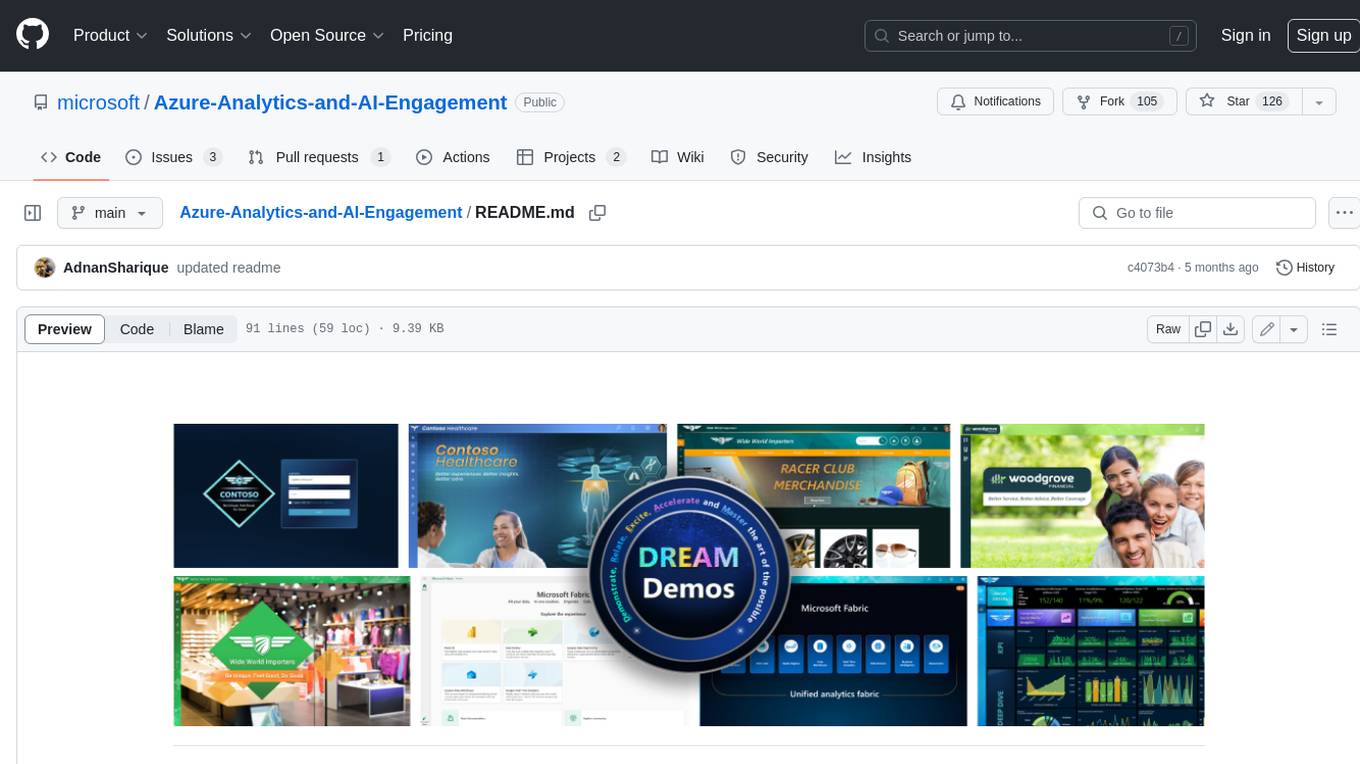
Azure-Analytics-and-AI-Engagement
The Azure-Analytics-and-AI-Engagement repository provides packaged Industry Scenario DREAM Demos with ARM templates (Containing a demo web application, Power BI reports, Synapse resources, AML Notebooks etc.) that can be deployed in a customer’s subscription using the CAPE tool within a matter of few hours. Partners can also deploy DREAM Demos in their own subscriptions using DPoC.
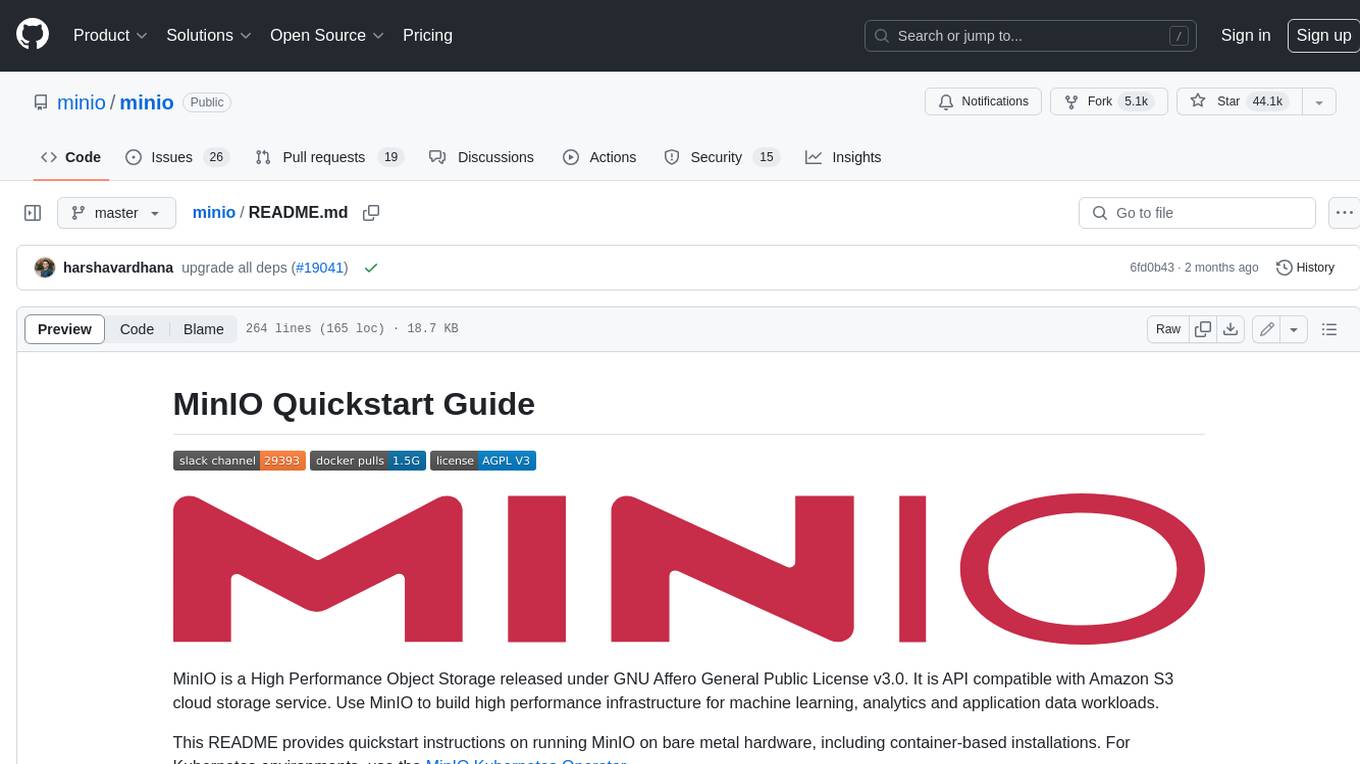
minio
MinIO is a High Performance Object Storage released under GNU Affero General Public License v3.0. It is API compatible with Amazon S3 cloud storage service. Use MinIO to build high performance infrastructure for machine learning, analytics and application data workloads.