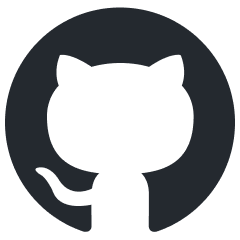
llm-continual-learning-survey
Continual Learning of Large Language Models: A Comprehensive Survey
Stars: 215
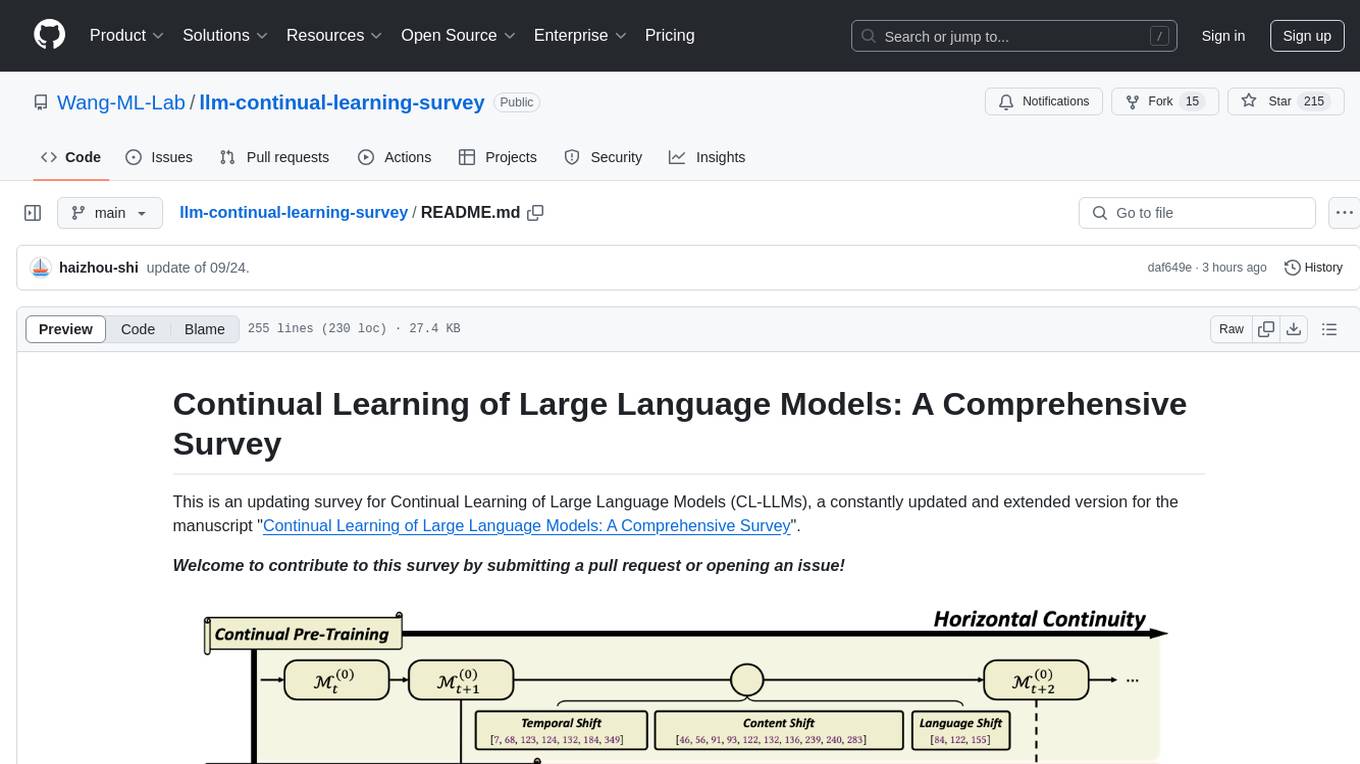
This repository is an updating survey for Continual Learning of Large Language Models (CL-LLMs), providing a comprehensive overview of various aspects related to the continual learning of large language models. It covers topics such as continual pre-training, domain-adaptive pre-training, continual fine-tuning, model refinement, model alignment, multimodal LLMs, and miscellaneous aspects. The survey includes a collection of relevant papers, each focusing on different areas within the field of continual learning of large language models.
README:
This is an updating survey for Continual Learning of Large Language Models (CL-LLMs), a constantly updated and extended version for the manuscript "Continual Learning of Large Language Models: A Comprehensive Survey".
Welcome to contribute to this survey by submitting a pull request or opening an issue!
- [09/2024] (🔥) new papers: 07/2024 - 09/2024.
- [07/2024] new papers: 06/2024 - 07/2024.
- [07/2024] the updated version of the paper has been released on arXiv.
- [06/2024] new papers: 05/2024 - 06/2024.
- [05/2024] new papers: 02/2024 - 05/2024.
- [04/2024] initial release.
- Relevant Survey Papers
- Continual Pre-Training of LLMs (CPT)
- Domain-Adaptive Pre-Training of LLMs (DAP)
- Continual Fine-Tuning of LLMs (CFT)
- Continual LLMs Miscs
- Towards Lifelong Learning of Large Language Models: A Survey [paper][code]
- Recent Advances of Foundation Language Models-based Continual Learning: A Survey [paper]
- A Comprehensive Survey of Continual Learning: Theory, Method and Application (TPAMI 2024) [paper]
- Continual Learning for Large Language Models: A Survey [paper]
- Continual Lifelong Learning in Natural Language Processing: A Survey (COLING 2020) [paper]
- Continual Learning of Natural Language Processing Tasks: A Survey [paper]
- A Survey on Knowledge Distillation of Large Language Models [paper]
- 🔥 A Practice of Post-Training on Llama-3 70B with Optimal Selection of Additional Language Mixture Ratio [paper]
- 🔥 Towards Effective and Efficient Continual Pre-training of Large Language Models [paper][code]
- Bilingual Adaptation of Monolingual Foundation Models [paper]
- Mix-CPT: A Domain Adaptation Framework via Decoupling Knowledge Learning and Format Alignment [paper]
- Breaking Language Barriers: Cross-Lingual Continual Pre-Training at Scale [paper]
- LLaMA-MoE: Building Mixture-of-Experts from LLaMA with Continual Pre-training [paper][code]
- Efficient Continual Pre-training by Mitigating the Stability Gap [paper][huggingface]
- How Do Large Language Models Acquire Factual Knowledge During Pretraining? [paper]
- DHA: Learning Decoupled-Head Attention from Transformer Checkpoints via Adaptive Heads Fusion [paper]
- MoRA: High-Rank Updating for Parameter-Efficient Fine-Tuning [paper][code]
- Large Language Model Can Continue Evolving From Mistakes [paper]
- Rho-1: Not All Tokens Are What You Need [paper][code]
- Simple and Scalable Strategies to Continually Pre-train Large Language Models [paper]
- Investigating Continual Pretraining in Large Language Models: Insights and Implications [paper]
- Take the Bull by the Horns: Hard Sample-Reweighted Continual Training Improves LLM Generalization [paper][code]
- TimeLMs: Diachronic Language Models from Twitter (ACL 2022, Demo Track) [paper][code]
- Continual Pre-Training of Large Language Models: How to (re)warm your model? [paper]
- Continual Learning Under Language Shift [paper]
- Examining Forgetting in Continual Pre-training of Aligned Large Language Models [paper]
- Towards Continual Knowledge Learning of Language Models (ICLR 2022) [paper][code]
- Lifelong Pretraining: Continually Adapting Language Models to Emerging Corpora (NAACL 2022) [paper]
- TemporalWiki: A Lifelong Benchmark for Training and Evaluating Ever-Evolving Language Models (EMNLP 2022) [paper][code]
- Continual Training of Language Models for Few-Shot Learning (EMNLP 2022) [paper][code]
- ERNIE 2.0: A Continual Pre-Training Framework for Language Understanding (AAAI 2020) [paper][code]
- Dynamic Language Models for Continuously Evolving Content (KDD 2021) [paper]
- Continual Pre-Training Mitigates Forgetting in Language and Vision [paper][code]
- DEMix Layers: Disentangling Domains for Modular Language Modeling (NAACL 2022) [paper][code]
- Time-Aware Language Models as Temporal Knowledge Bases (TACL 2022) [paper]
- Recyclable Tuning for Continual Pre-training (ACL 2023 Findings) [paper][code]
- Lifelong Language Pretraining with Distribution-Specialized Experts (ICML 2023) [paper]
- ELLE: Efficient Lifelong Pre-training for Emerging Data (ACL 2022 Findings) [paper][code]
- 🔥 Amuro & Char: Analyzing the Relationship between Pre-Training and Fine-Tuning of Large Language Models [paper]
- CMR Scaling Law: Predicting Critical Mixture Ratios for Continual Pre-training of Language Models [paper]
- Task Oriented In-Domain Data Augmentation [paper]
- Instruction Pre-Training: Language Models are Supervised Multitask Learners [paper][code][huggingface]
- D-CPT Law: Domain-specific Continual Pre-Training Scaling Law for Large Language Models [paper]
- BLADE: Enhancing Black-box Large Language Models with Small Domain-Specific Models [paper]
- Tag-LLM: Repurposing General-Purpose LLMs for Specialized Domains [paper]
- Adapting Large Language Models via Reading Comprehension (ICLR 2024) [paper][code]
- SaulLM-7B: A pioneering Large Language Model for Law [paper][huggingface]
- Lawyer LLaMA Technical Report [paper]
- PediatricsGPT: Large Language Models as Chinese Medical Assistants for Pediatric Applications [paper]
- Hippocrates: An Open-Source Framework for Advancing Large Language Models in Healthcare [paper][project][huggingface]
- Me LLaMA: Foundation Large Language Models for Medical Applications [paper][code]
- BioMedGPT: Open Multimodal Generative Pre-trained Transformer for BioMedicine [paper][code]
- Continuous Training and Fine-tuning for Domain-Specific Language Models in Medical Question Answering [paper]
- PMC-LLaMA: Towards Building Open-source Language Models for Medicine [paper][code]
- AF Adapter: Continual Pretraining for Building Chinese Biomedical Language Model [paper]
- Continual Domain-Tuning for Pretrained Language Models [paper]
- HuatuoGPT-II, One-stage Training for Medical Adaption of LLMs [paper][code]
- 🔥 Open-FinLLMs: Open Multimodal Large Language Models for Financial Applications [paper]
- Domain Adaptation of Llama3-70B-Instruct through Continual Pre-Training and Model Merging: A Comprehensive Evaluation [paper][huggingface]
- Construction of Domain-specified Japanese Large Language Model for Finance through Continual Pre-training [paper]
- Pretraining and Updating Language- and Domain-specific Large Language Model: A Case Study in Japanese Business Domain [paper][huggingface]
- BBT-Fin: Comprehensive Construction of Chinese Financial Domain Pre-trained Language Model, Corpus and Benchmark [paper][code]
- CFGPT: Chinese Financial Assistant with Large Language Model [paper][code]
- Efficient Continual Pre-training for Building Domain Specific Large Language Models [paper]
- WeaverBird: Empowering Financial Decision-Making with Large Language Model, Knowledge Base, and Search Engine [paper][code][huggingface][demo]
- XuanYuan 2.0: A Large Chinese Financial Chat Model with Hundreds of Billions Parameters [paper][huggingface]
- 🔥 SciLitLLM: How to Adapt LLMs for Scientific Literature Understanding [paper]
- PRESTO: Progressive Pretraining Enhances Synthetic Chemistry Outcomes [paper][code]
- ClimateGPT: Towards AI Synthesizing Interdisciplinary Research on Climate Change [paper][hugginface]
- AstroLLaMA: Towards Specialized Foundation Models in Astronomy [paper]
- OceanGPT: A Large Language Model for Ocean Science Tasks [paper][code]
- K2: A Foundation Language Model for Geoscience Knowledge Understanding and Utilization [paper][code][huggingface]
- MarineGPT: Unlocking Secrets of "Ocean" to the Public [paper][code]
- GeoGalactica: A Scientific Large Language Model in Geoscience [paper][code][huggingface]
- Llemma: An Open Language Model For Mathematics [paper][code][huggingface]
- PLLaMa: An Open-source Large Language Model for Plant Science [paper][code][huggingface]
- CodeGen: An Open Large Language Model for Code with Multi-Turn Program Synthesis [paper][code][huggingface]
- Code Needs Comments: Enhancing Code LLMs with Comment Augmentation [code]
- StarCoder: may the source be with you! [ppaer][code]
- DeepSeek-Coder: When the Large Language Model Meets Programming -- The Rise of Code Intelligence [paper][code][huggingface]
- IRCoder: Intermediate Representations Make Language Models Robust Multilingual Code Generators [paper][code]
- Code Llama: Open Foundation Models for Code [paper][code]
- 🔥 RedWhale: An Adapted Korean LLM Through Efficient Continual Pretraining [paper]
- Unlocking the Potential of Model Merging for Low-Resource Languages [paper]
- Mitigating Catastrophic Forgetting in Language Transfer via Model Merging [paper]
- Enhancing Translation Accuracy of Large Language Models through Continual Pre-Training on Parallel Data [paper]
- BAMBINO-LM: (Bilingual-)Human-Inspired Continual Pretraining of BabyLM [paper]
- InstructionCP: A fast approach to transfer Large Language Models into target language [paper]
- Continual Pre-Training for Cross-Lingual LLM Adaptation: Enhancing Japanese Language Capabilities [paper]
- Sailor: Open Language Models for South-East Asia [paper][code]
- Aurora-M: The First Open Source Multilingual Language Model Red-teamed according to the U.S. Executive Order [paper][huggingface]
- LLaMA Pro: Progressive LLaMA with Block Expansion [paper][code][huggingface]
- ECONET: Effective Continual Pretraining of Language Models for Event Temporal Reasoning [paper][code]
- Pre-training Text-to-Text Transformers for Concept-centric Common Sense [paper][code][project]
- Don't Stop Pretraining: Adapt Language Models to Domains and Tasks (ACL 2020) [paper][code]
- EcomGPT-CT: Continual Pre-training of E-commerce Large Language Models with Semi-structured Data [paper]
- 🔥 MoFO: Momentum-Filtered Optimizer for Mitigating Forgetting in LLM Fine-Tuning [paper]
- Learn it or Leave it: Module Composition and Pruning for Continual Learning [paper]
- Unlocking Continual Learning Abilities in Language Models [paper][code]
- Achieving Forgetting Prevention and Knowledge Transfer in Continual Learning (NeurIPS 2021) [paper][code]
- Can BERT Refrain from Forgetting on Sequential Tasks? A Probing Study (ICLR 2023) [paper][code]
- CIRCLE: Continual Repair across Programming Languages (ISSTA 2022) [paper]
- ConPET: Continual Parameter-Efficient Tuning for Large Language Models [paper][code]
- Enhancing Continual Learning with Global Prototypes: Counteracting Negative Representation Drift [paper]
- Investigating Forgetting in Pre-Trained Representations Through Continual Learning [paper]
- Learn or Recall? Revisiting Incremental Learning with Pre-trained Language Models [paper][code]
- LFPT5: A Unified Framework for Lifelong Few-shot Language Learning Based on Prompt Tuning of T5 (ICLR 2022) [paper][code]
- On the Usage of Continual Learning for Out-of-Distribution Generalization in Pre-trained Language Models of Code [paper]
- Overcoming Catastrophic Forgetting in Massively Multilingual Continual Learning (ACL 2023 Findings) [paper]
- Parameterizing Context: Unleashing the Power of Parameter-Efficient Fine-Tuning and In-Context Tuning for Continual Table Semantic Parsing (NeurIPS 2023) [paper][code]
- Fine-tuned Language Models are Continual Learners [paper][code]
- TRACE: A Comprehensive Benchmark for Continual Learning in Large Language Models [paper][code]
- Large-scale Lifelong Learning of In-context Instructions and How to Tackle It [paper]
- CITB: A Benchmark for Continual Instruction Tuning [paper][code]
- Mitigating Catastrophic Forgetting in Large Language Models with Self-Synthesized Rehearsal [paper]
- Don't Half-listen: Capturing Key-part Information in Continual Instruction Tuning [paper]
- ConTinTin: Continual Learning from Task Instructions [paper]
- Orthogonal Subspace Learning for Language Model Continual Learning [paper][code]
- SAPT: A Shared Attention Framework for Parameter-Efficient Continual Learning of Large Language Models [paper]
- InsCL: A Data-efficient Continual Learning Paradigm for Fine-tuning Large Language Models with Instructions [paper]
- LEMoE: Advanced Mixture of Experts Adaptor for Lifelong Model Editing of Large Language Models [paper]
- WISE: Rethinking the Knowledge Memory for Lifelong Model Editing of Large Language Models [paper][code]
- Aging with GRACE: Lifelong Model Editing with Discrete Key-Value Adaptors [paper][code]
- On Continual Model Refinement in Out-of-Distribution Data Streams [paper][code][project]
- Melo: Enhancing model editing with neuron-indexed dynamic lora [paper][code]
- Larimar: Large language models with episodic memory control [paper]
- Wilke: Wise-layer knowledge editor for lifelong knowledge editing [paper]
- Mind the Interference: Retaining Pre-trained Knowledge in Parameter Efficient Continual Learning of Vision-Language Models [paper]
- Online Merging Optimizers for Boosting Rewards and Mitigating Tax in Alignment [paper][code]
- Alpaca: A Strong, Replicable Instruction-Following Model [project] [code]
- Self-training Improves Pre-training for Few-shot Learning in Task-oriented Dialog Systems [paper] [code]
- Training language models to follow instructions with human feedback (NeurIPS 2022) [paper]
- Direct preference optimization: Your language model is secretly a reward model (NeurIPS 2023) [paper]
- Copf: Continual learning human preference through optimal policy fitting [paper]
- CPPO: Continual Learning for Reinforcement Learning with Human Feedback (ICLR 2024) [paper]
- A Moral Imperative: The Need for Continual Superalignment of Large Language Models [paper]
- Mitigating the Alignment Tax of RLHF [paper]
- CLIP with Generative Latent Replay: a Strong Baseline for Incremental Learning [paper]
- Continually Learn to Map Visual Concepts to Large Language Models in Resource-constrained Environments [paper]
- Mind the Interference: Retaining Pre-trained Knowledge in Parameter Efficient Continual Learning of Vision-Language Models [paper]
- CLIP model is an Efficient Online Lifelong Learner [paper]
- CLAP4CLIP: Continual Learning with Probabilistic Finetuning for Vision-Language Models [paper][code]
- Boosting Continual Learning of Vision-Language Models via Mixture-of-Experts Adapters (CVPR 2024) [paper][code]
- CoLeCLIP: Open-Domain Continual Learning via Joint Task Prompt and Vocabulary Learning [paper]
- Select and Distill: Selective Dual-Teacher Knowledge Transfer for Continual Learning on Vision-Language Models [paper]
- Investigating the Catastrophic Forgetting in Multimodal Large Language Models (PMLR 2024) [paper]
- MiniGPT-4: Enhancing Vision-Language Understanding with Advanced Large Language Models [paper] [code]
- Visual Instruction Tuning (NeurIPS 2023, Oral) [paper] [code]
- Continual Instruction Tuning for Large Multimodal Models [paper]
- CoIN: A Benchmark of Continual Instruction tuNing for Multimodel Large Language Model [paper] [code]
- Model Tailor: Mitigating Catastrophic Forgetting in Multi-modal Large Language Models [paper]
- Reconstruct before Query: Continual Missing Modality Learning with Decomposed Prompt Collaboration [paper] [code]
- How Do Large Language Models Acquire Factual Knowledge During Pretraining? [paper]
- Data Mixing Laws: Optimizing Data Mixtures by Predicting Language Modeling Performance [paper][code]
- Evaluating the External and Parametric Knowledge Fusion of Large Language Models [paper]
- Demystifying Forgetting in Language Model Fine-Tuning with Statistical Analysis of Example Associations [paper]
- AdapterSwap: Continuous Training of LLMs with Data Removal and Access-Control Guarantees [paper]
- COPAL: Continual Pruning in Large Language Generative Models [paper]
- HippoRAG: Neurobiologically Inspired Long-Term Memory for Large Language Models [paper][code]
- Reawakening knowledge: Anticipatory recovery from catastrophic interference via structured training [paper][code]
If you find our survey or this collection of papers useful, please consider citing our work by
@article{shi2024continual,
title={Continual Learning of Large Language Models: A Comprehensive Survey},
author={Shi, Haizhou and
Xu, Zihao and
Wang, Hengyi and
Qin, Weiyi and
Wang, Wenyuan and
Wang, Yibin and
Wang, Zifeng and
Ebrahimi, Sayna and
Wang, Hao},
journal={arXiv preprint arXiv:2404.16789},
year={2024}
}
For Tasks:
Click tags to check more tools for each tasksFor Jobs:
Alternative AI tools for llm-continual-learning-survey
Similar Open Source Tools
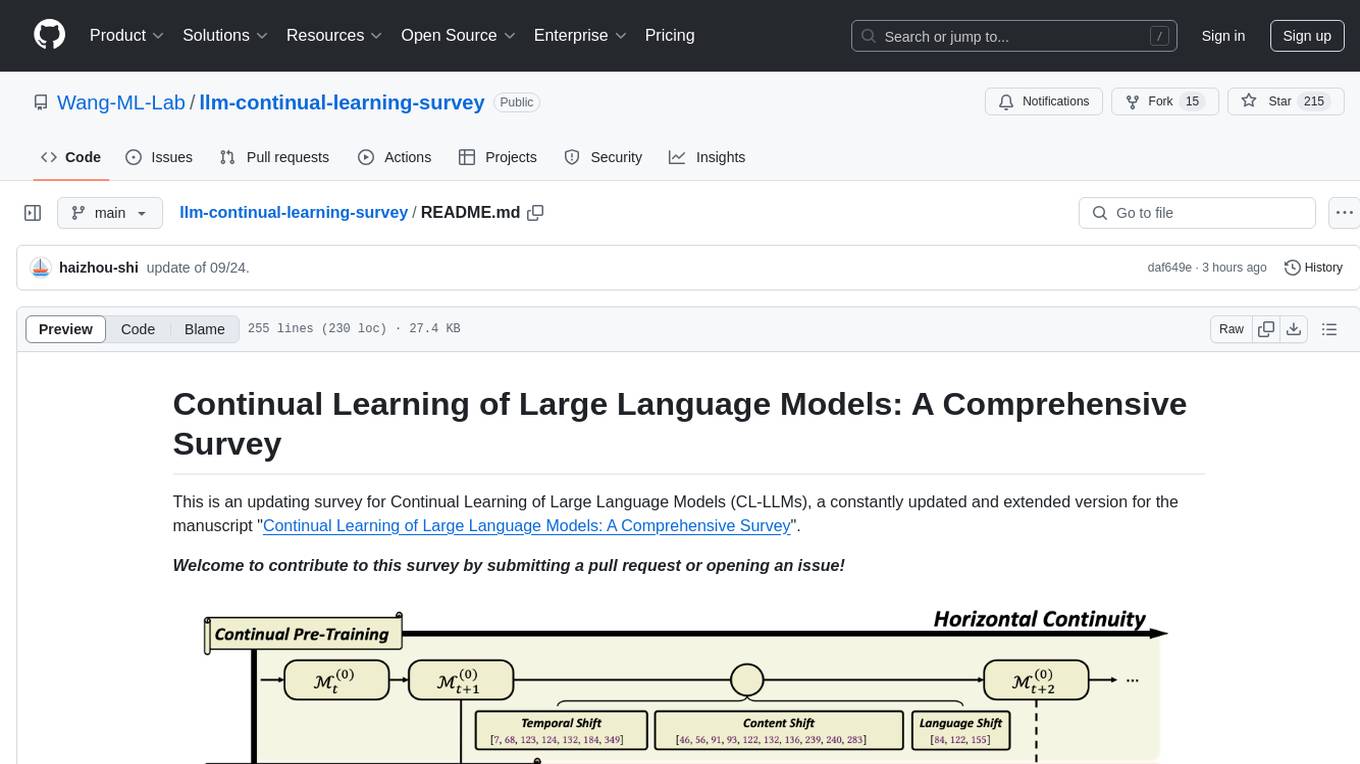
llm-continual-learning-survey
This repository is an updating survey for Continual Learning of Large Language Models (CL-LLMs), providing a comprehensive overview of various aspects related to the continual learning of large language models. It covers topics such as continual pre-training, domain-adaptive pre-training, continual fine-tuning, model refinement, model alignment, multimodal LLMs, and miscellaneous aspects. The survey includes a collection of relevant papers, each focusing on different areas within the field of continual learning of large language models.
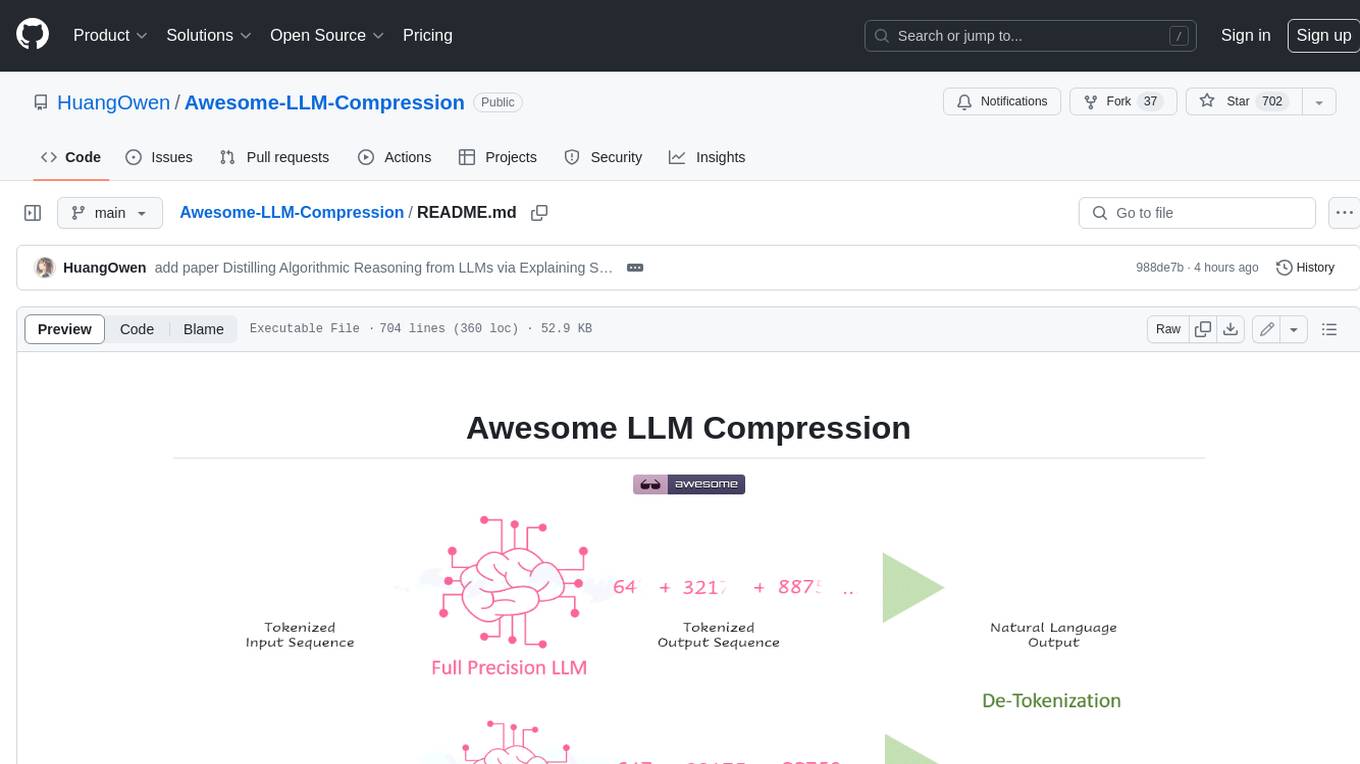
Awesome-LLM-Compression
Awesome LLM compression research papers and tools to accelerate LLM training and inference.
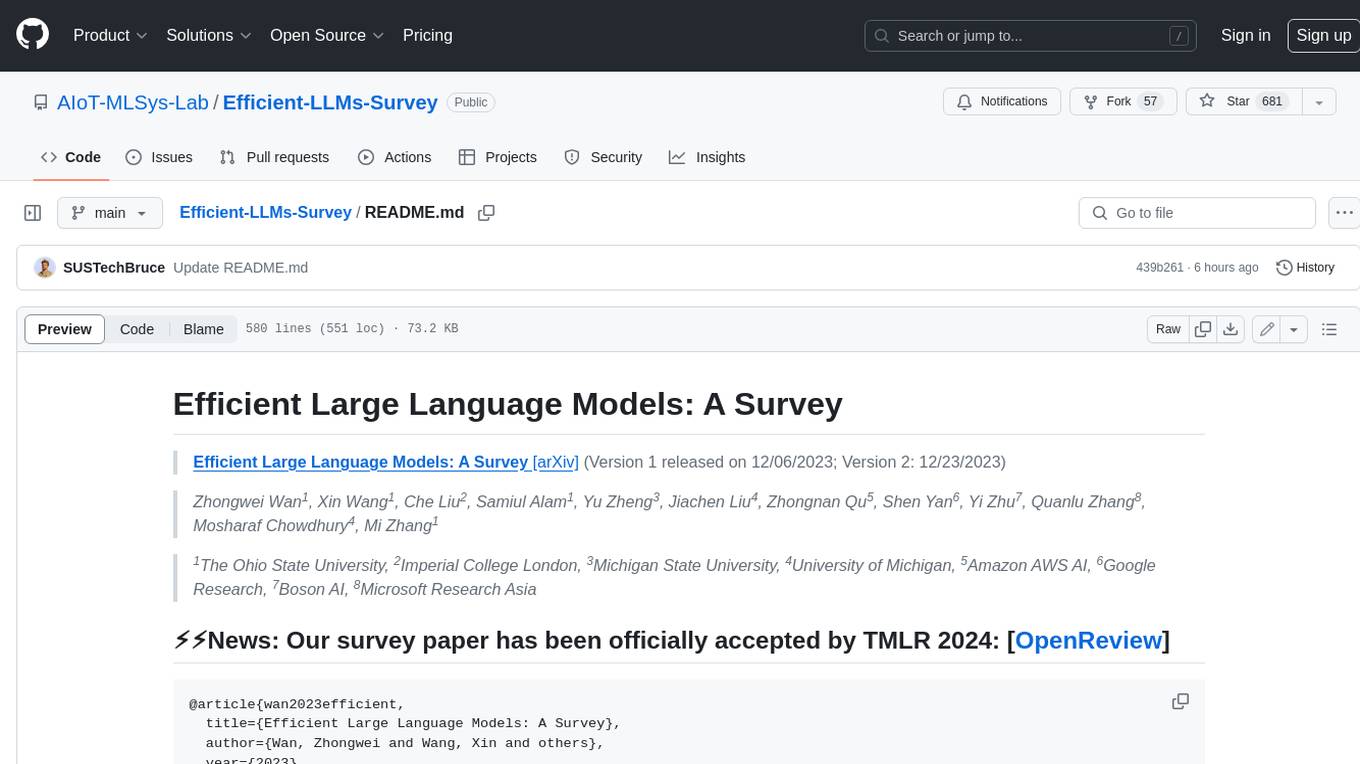
Efficient-LLMs-Survey
This repository provides a systematic and comprehensive review of efficient LLMs research. We organize the literature in a taxonomy consisting of three main categories, covering distinct yet interconnected efficient LLMs topics from **model-centric** , **data-centric** , and **framework-centric** perspective, respectively. We hope our survey and this GitHub repository can serve as valuable resources to help researchers and practitioners gain a systematic understanding of the research developments in efficient LLMs and inspire them to contribute to this important and exciting field.
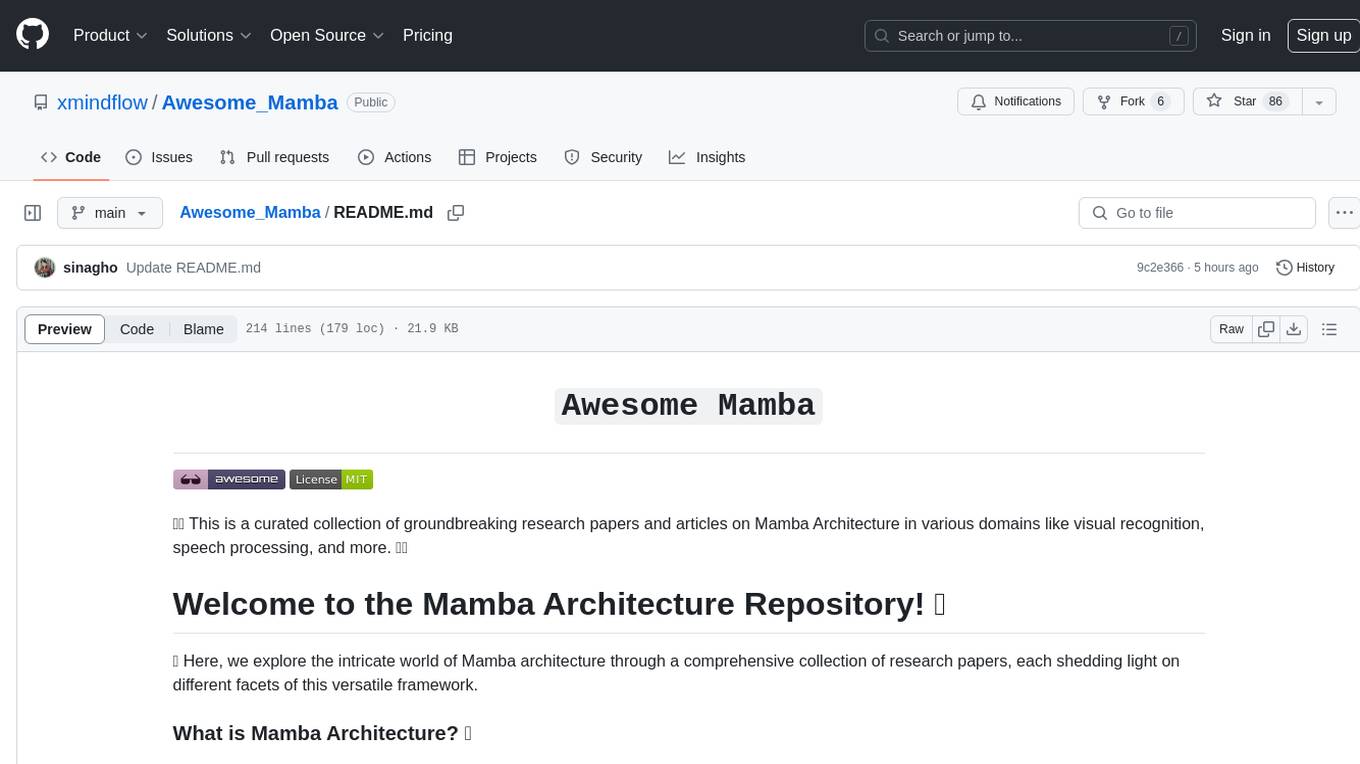
Awesome_Mamba
Awesome Mamba is a curated collection of groundbreaking research papers and articles on Mamba Architecture, a pioneering framework in deep learning known for its selective state spaces and efficiency in processing complex data structures. The repository offers a comprehensive exploration of Mamba architecture through categorized research papers covering various domains like visual recognition, speech processing, remote sensing, video processing, activity recognition, image enhancement, medical imaging, reinforcement learning, natural language processing, 3D recognition, multi-modal understanding, time series analysis, graph neural networks, point cloud analysis, and tabular data handling.
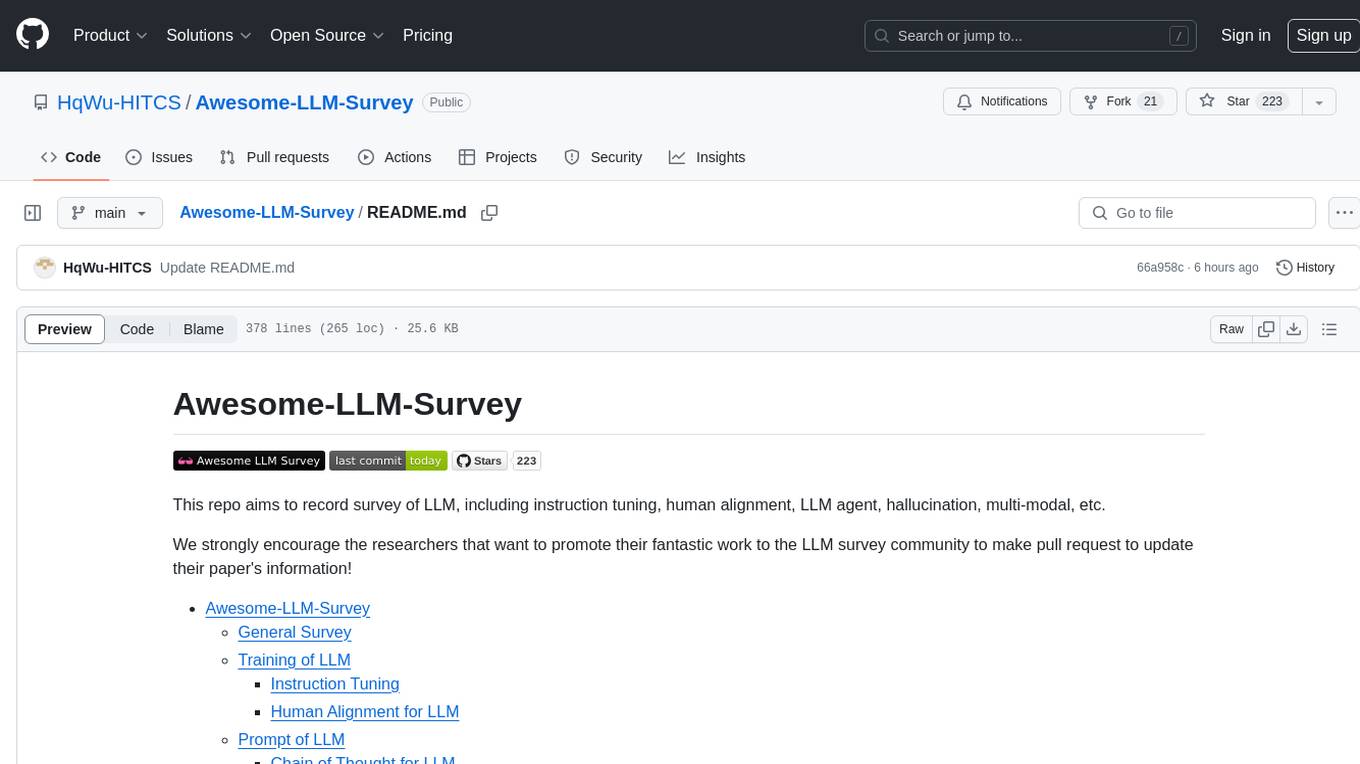
Awesome-LLM-Survey
This repository, Awesome-LLM-Survey, serves as a comprehensive collection of surveys related to Large Language Models (LLM). It covers various aspects of LLM, including instruction tuning, human alignment, LLM agents, hallucination, multi-modal capabilities, and more. Researchers are encouraged to contribute by updating information on their papers to benefit the LLM survey community.
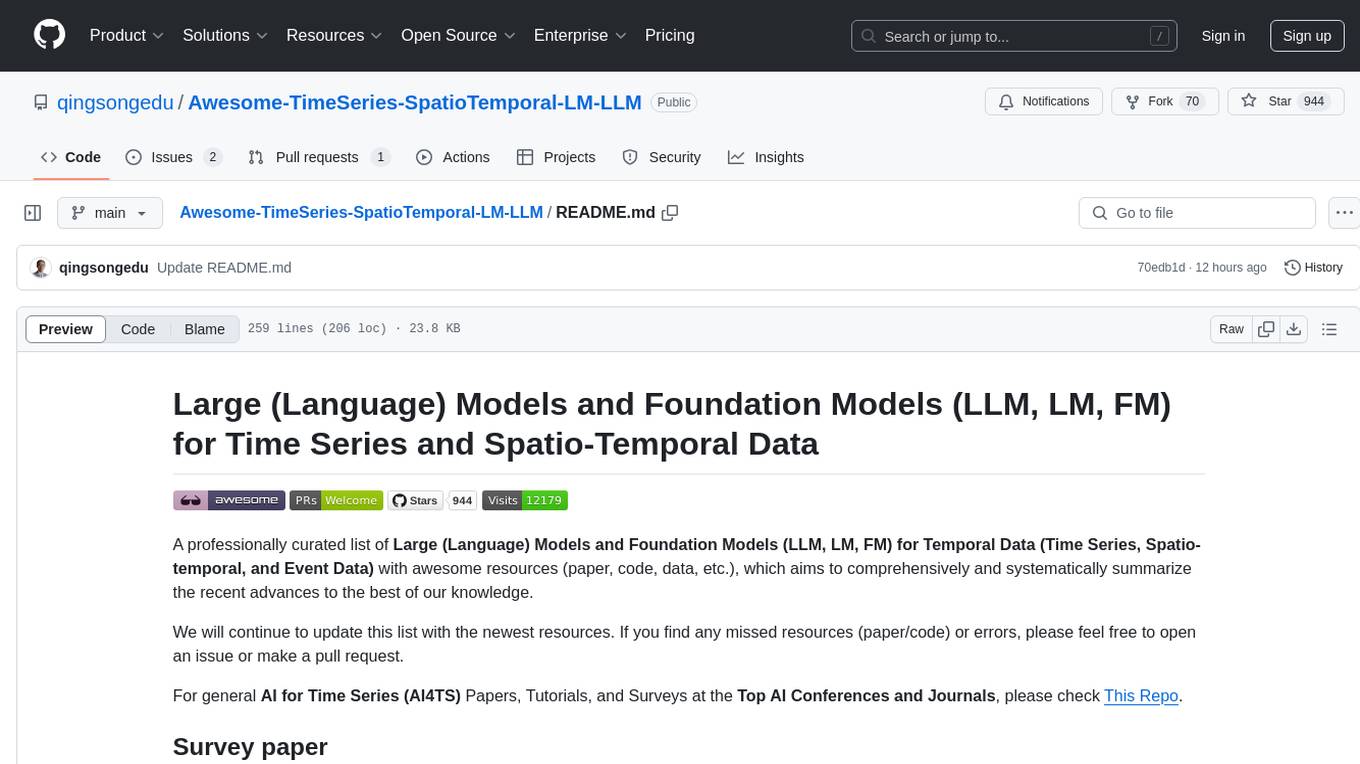
Awesome-TimeSeries-SpatioTemporal-LM-LLM
Awesome-TimeSeries-SpatioTemporal-LM-LLM is a curated list of Large (Language) Models and Foundation Models for Temporal Data, including Time Series, Spatio-temporal, and Event Data. The repository aims to summarize recent advances in Large Models and Foundation Models for Time Series and Spatio-Temporal Data with resources such as papers, code, and data. It covers various applications like General Time Series Analysis, Transportation, Finance, Healthcare, Event Analysis, Climate, Video Data, and more. The repository also includes related resources, surveys, and papers on Large Language Models, Foundation Models, and their applications in AIOps.
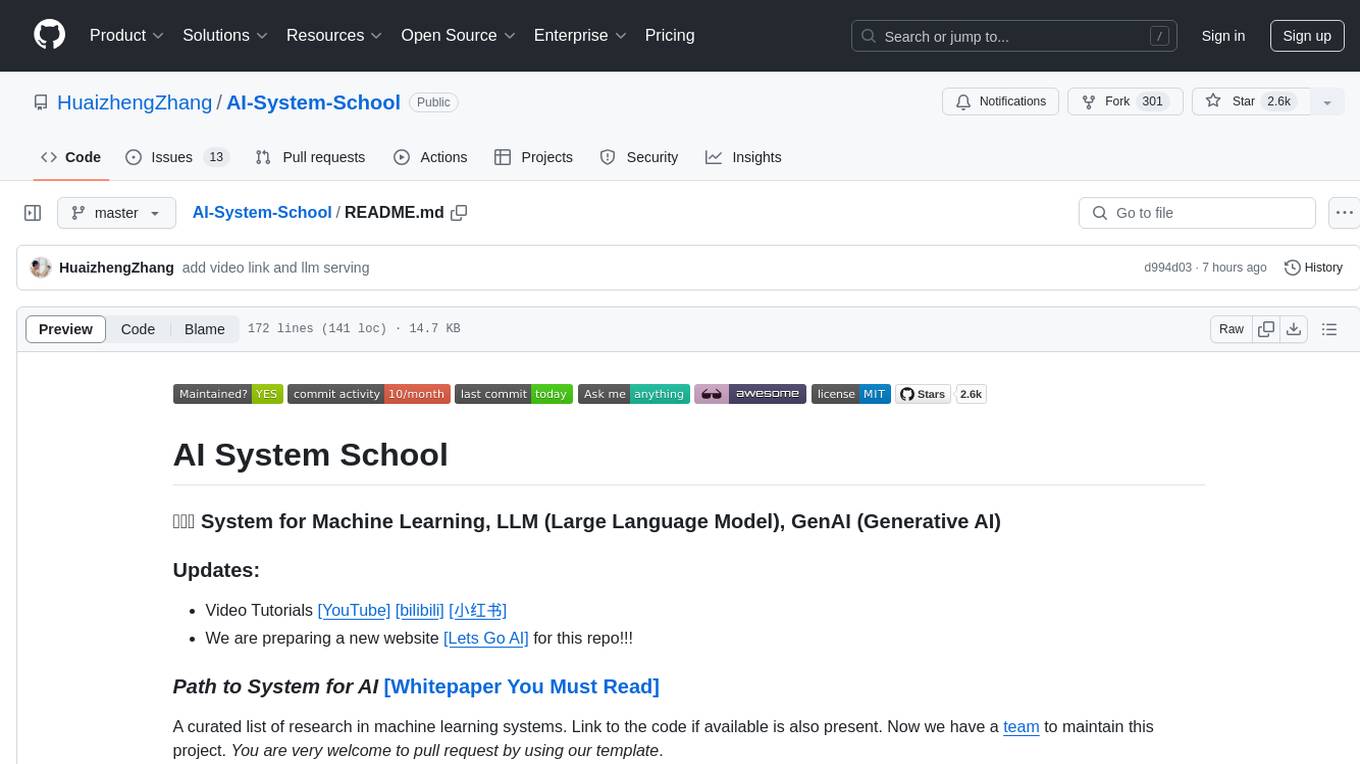
AI-System-School
AI System School is a curated list of research in machine learning systems, focusing on ML/DL infra, LLM infra, domain-specific infra, ML/LLM conferences, and general resources. It provides resources such as data processing, training systems, video systems, autoML systems, and more. The repository aims to help users navigate the landscape of AI systems and machine learning infrastructure, offering insights into conferences, surveys, books, videos, courses, and blogs related to the field.
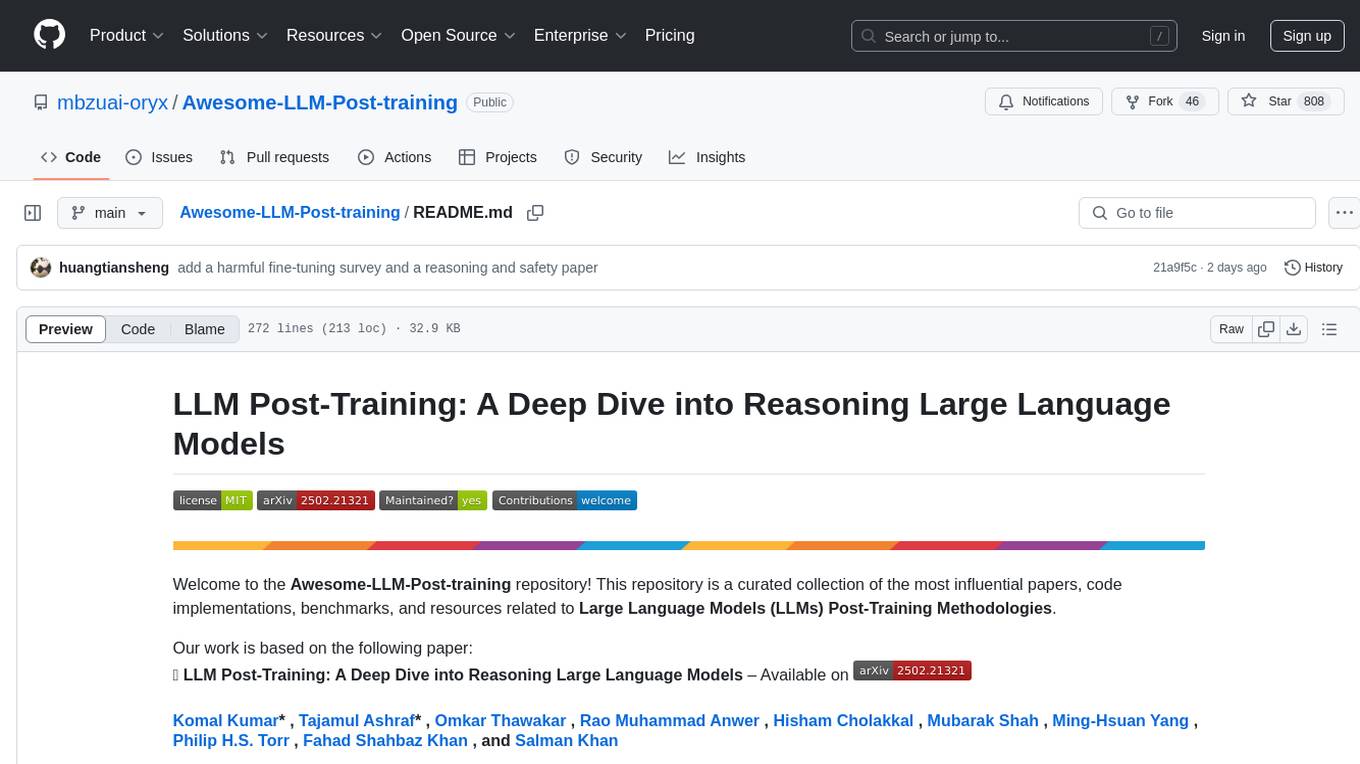
Awesome-LLM-Post-training
The Awesome-LLM-Post-training repository is a curated collection of influential papers, code implementations, benchmarks, and resources related to Large Language Models (LLMs) Post-Training Methodologies. It covers various aspects of LLMs, including reasoning, decision-making, reinforcement learning, reward learning, policy optimization, explainability, multimodal agents, benchmarks, tutorials, libraries, and implementations. The repository aims to provide a comprehensive overview and resources for researchers and practitioners interested in advancing LLM technologies.
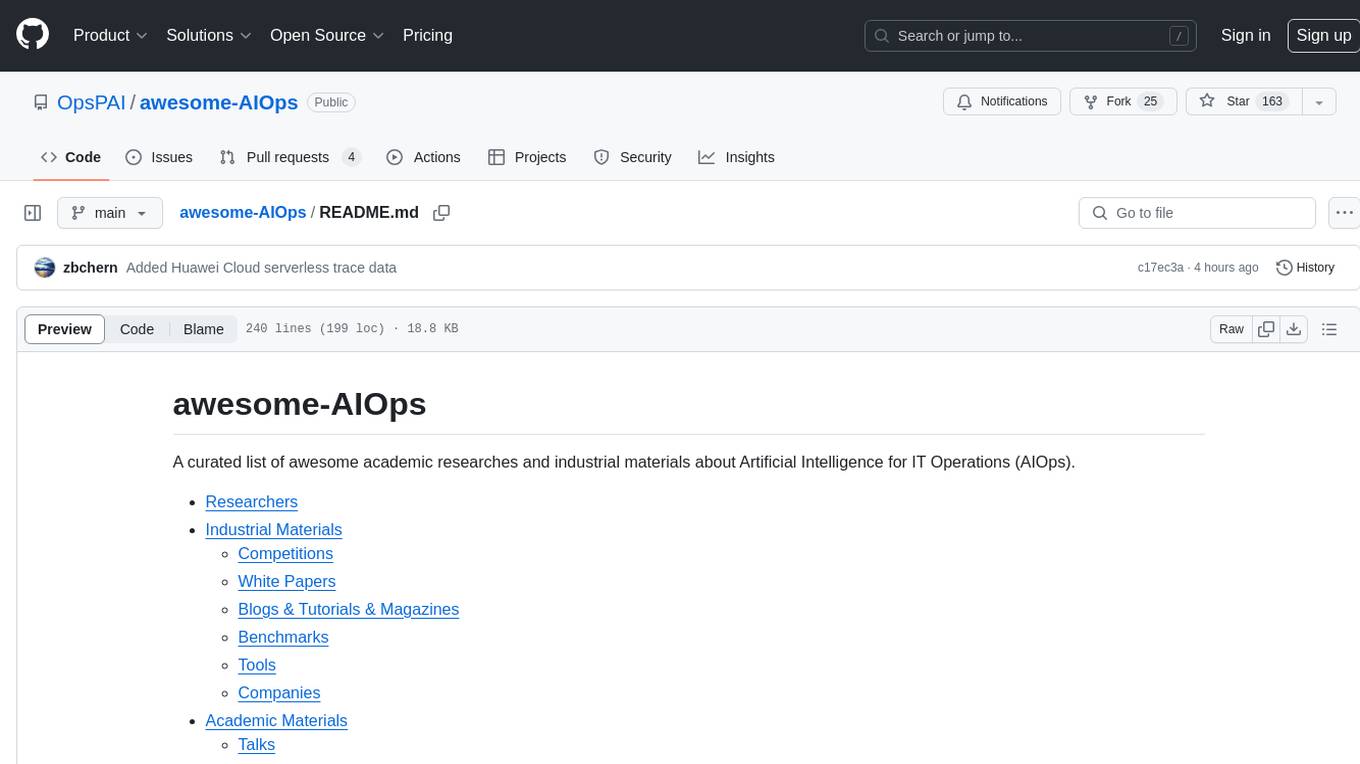
awesome-AIOps
awesome-AIOps is a curated list of academic researches and industrial materials related to Artificial Intelligence for IT Operations (AIOps). It includes resources such as competitions, white papers, blogs, tutorials, benchmarks, tools, companies, academic materials, talks, workshops, papers, and courses covering various aspects of AIOps like anomaly detection, root cause analysis, incident management, microservices, dependency tracing, and more.
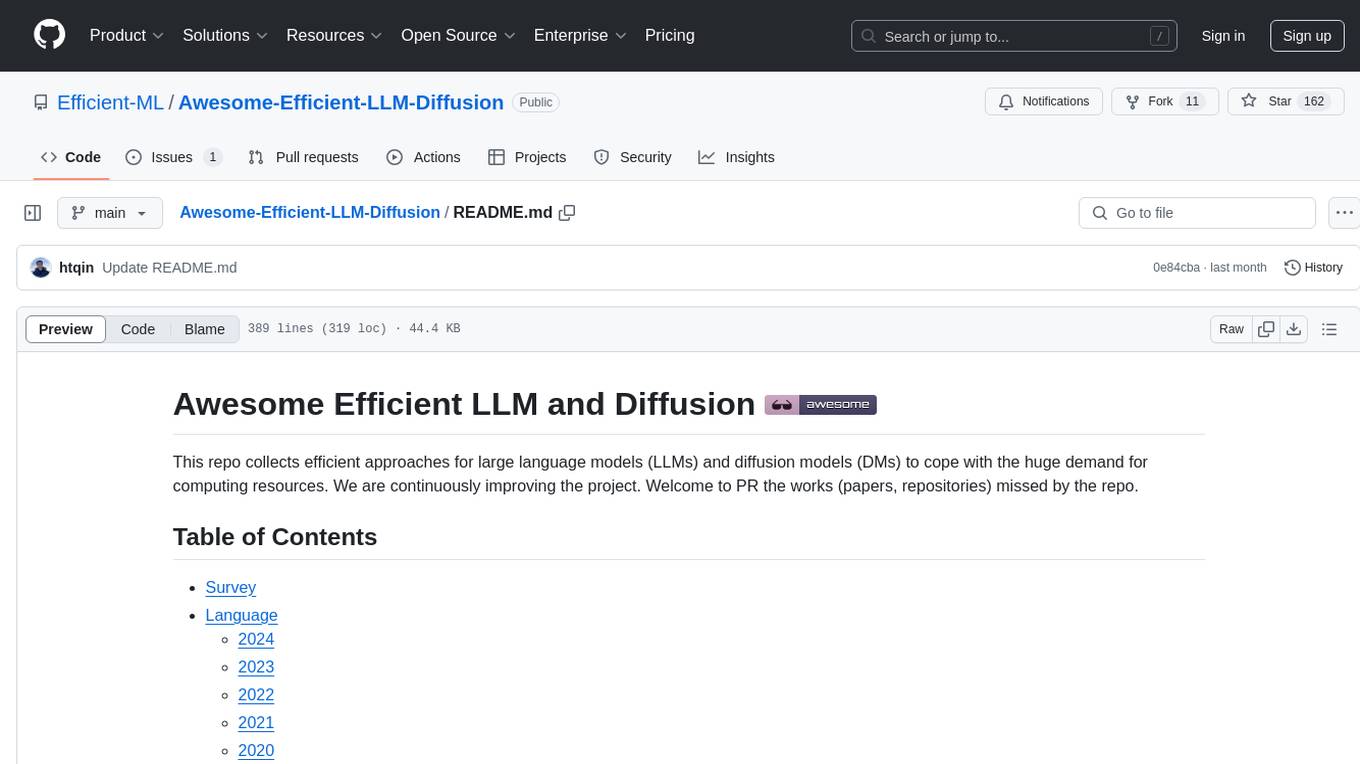
Awesome-Efficient-AIGC
This repository, Awesome Efficient AIGC, collects efficient approaches for AI-generated content (AIGC) to cope with its huge demand for computing resources. It includes efficient Large Language Models (LLMs), Diffusion Models (DMs), and more. The repository is continuously improving and welcomes contributions of works like papers and repositories that are missed by the collection.
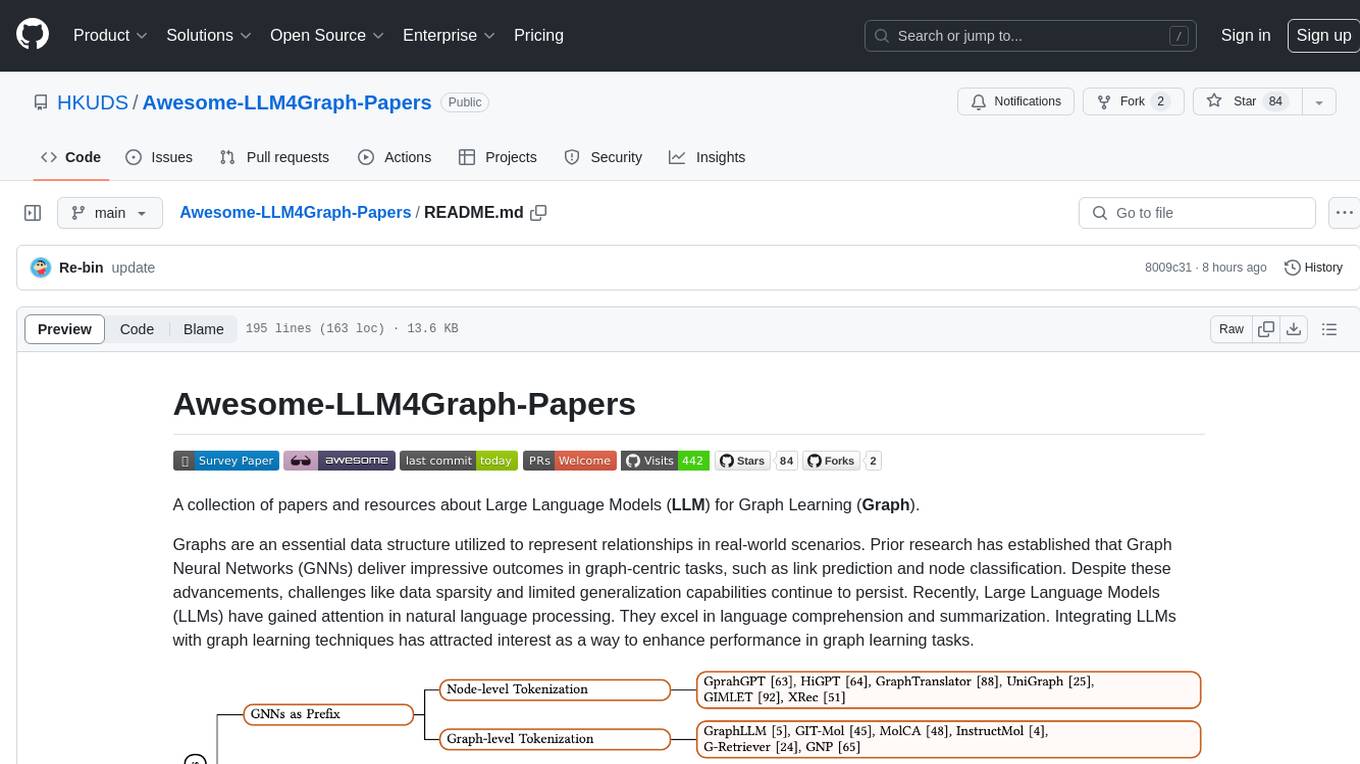
Awesome-LLM4Graph-Papers
A collection of papers and resources about Large Language Models (LLM) for Graph Learning (Graph). Integrating LLMs with graph learning techniques to enhance performance in graph learning tasks. Categorizes approaches based on four primary paradigms and nine secondary-level categories. Valuable for research or practice in self-supervised learning for recommendation systems.
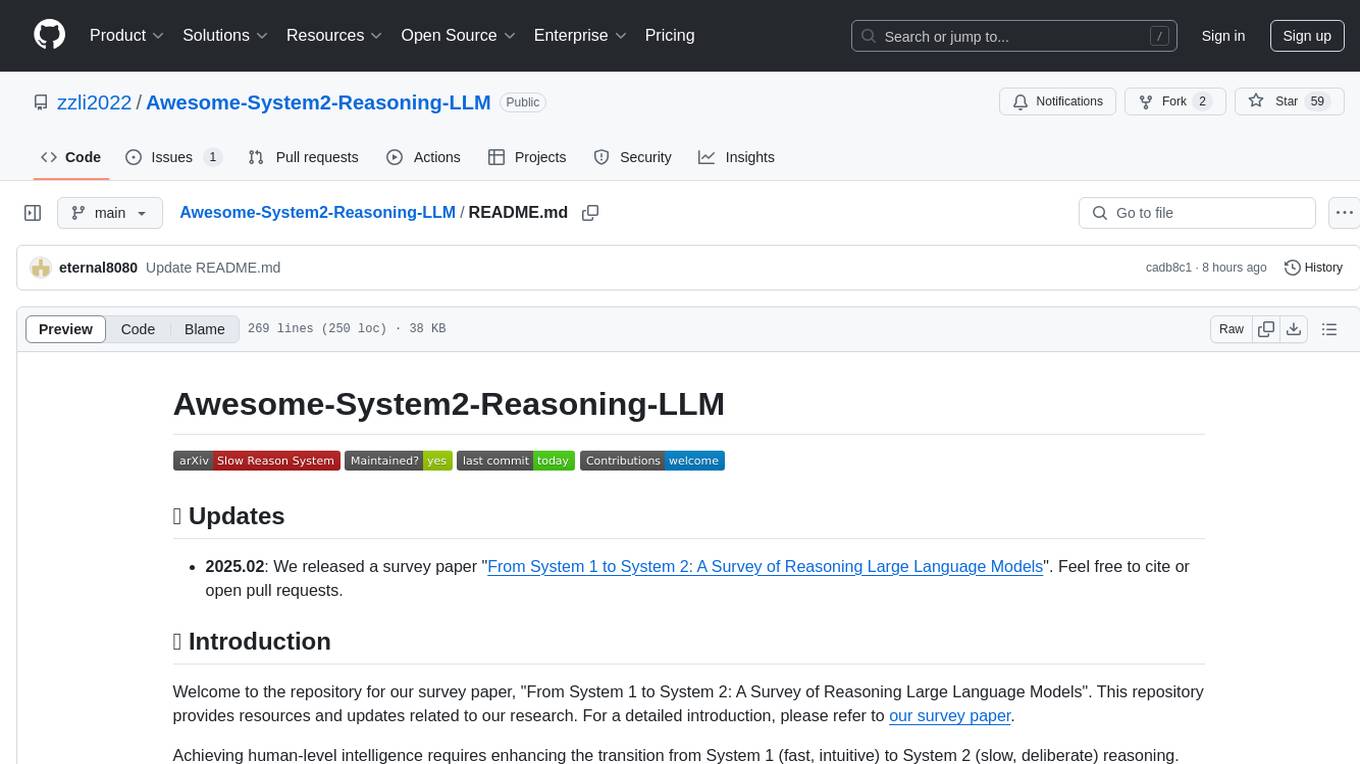
Awesome-System2-Reasoning-LLM
The Awesome-System2-Reasoning-LLM repository is dedicated to a survey paper titled 'From System 1 to System 2: A Survey of Reasoning Large Language Models'. It explores the development of reasoning Large Language Models (LLMs), their foundational technologies, benchmarks, and future directions. The repository provides resources and updates related to the research, tracking the latest developments in the field of reasoning LLMs.
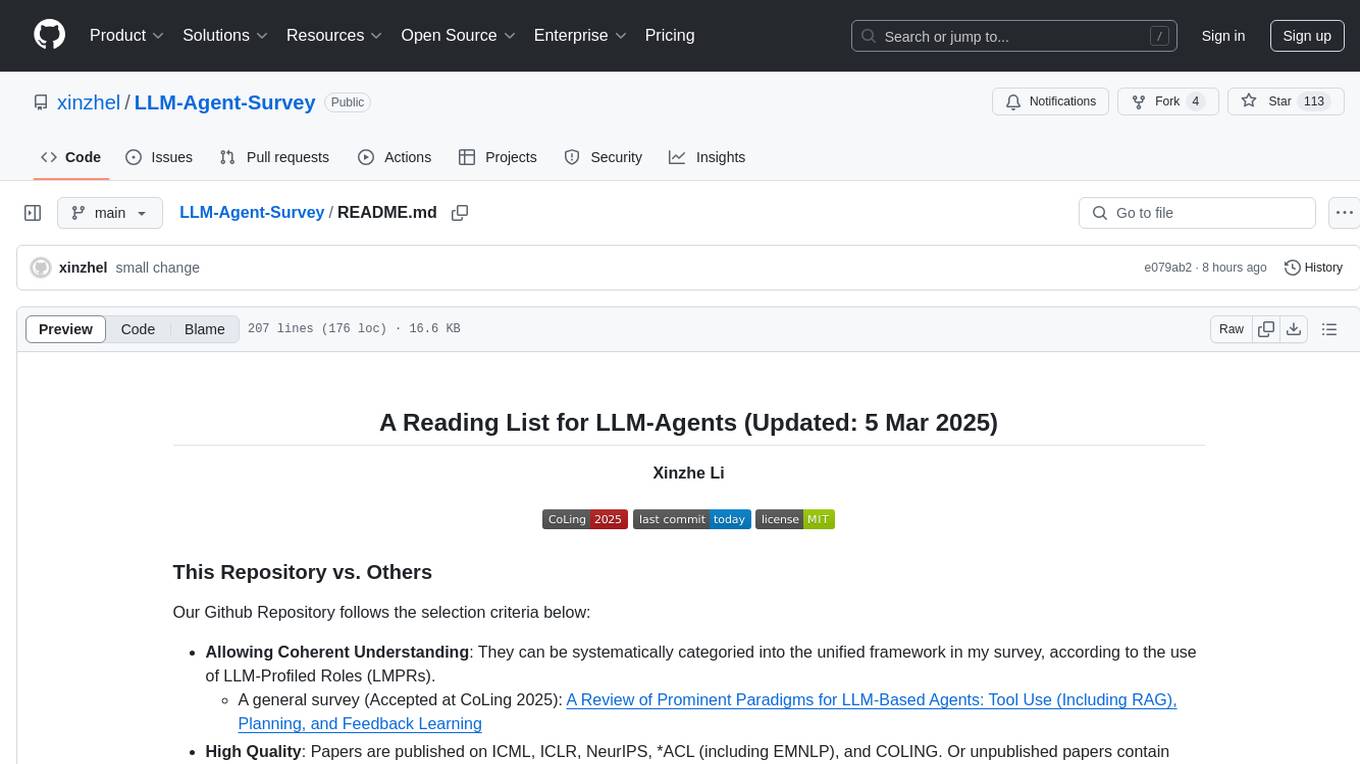
LLM-Agent-Survey
LLM-Agent-Survey is a comprehensive repository that provides a curated list of papers related to Large Language Model (LLM) agents. The repository categorizes papers based on LLM-Profiled Roles and includes high-quality publications from prestigious conferences and journals. It aims to offer a systematic understanding of LLM-based agents, covering topics such as tool use, planning, and feedback learning. The repository also includes unpublished papers with insightful analysis and novelty, marked for future updates. Users can explore a wide range of surveys, tool use cases, planning workflows, and benchmarks related to LLM agents.
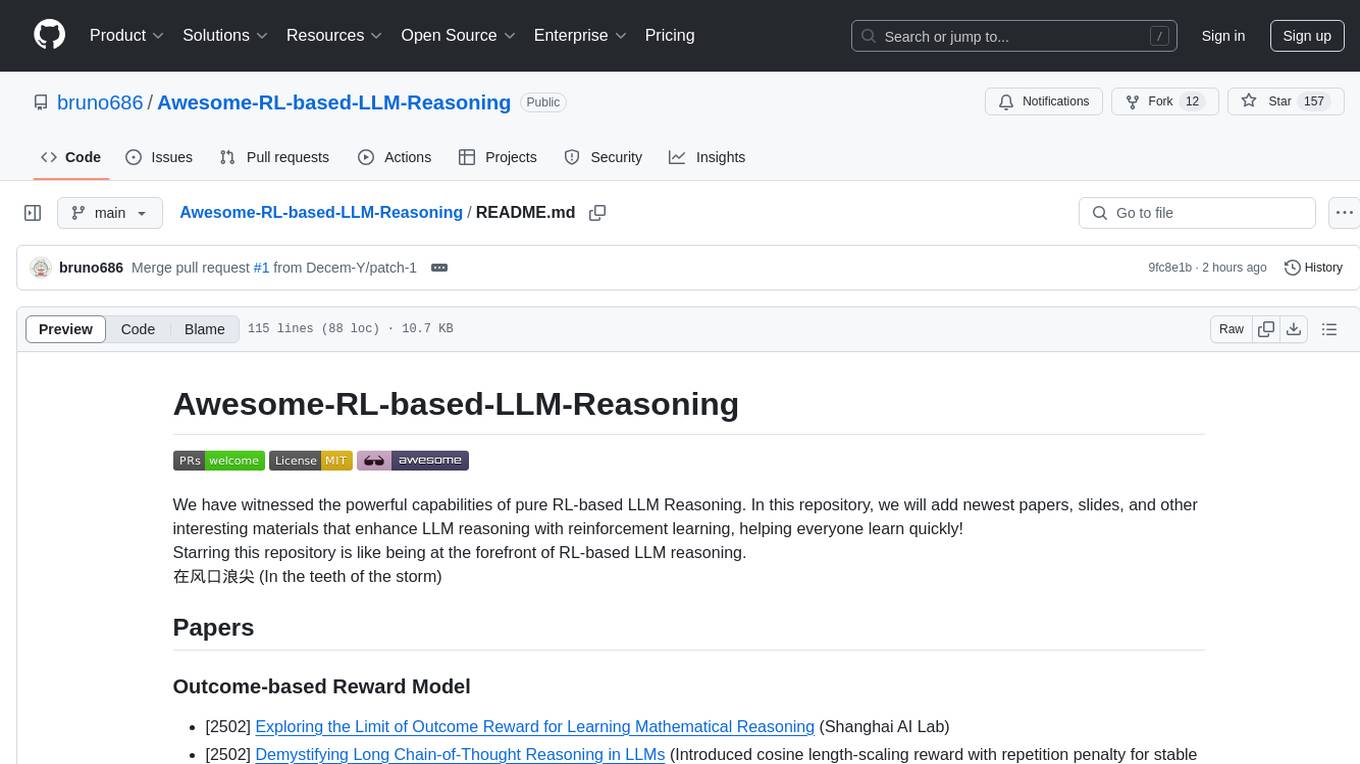
Awesome-RL-based-LLM-Reasoning
This repository is dedicated to enhancing Language Model (LLM) reasoning with reinforcement learning (RL). It includes a collection of the latest papers, slides, and materials related to RL-based LLM reasoning, aiming to facilitate quick learning and understanding in this field. Starring this repository allows users to stay updated and engaged with the forefront of RL-based LLM reasoning.
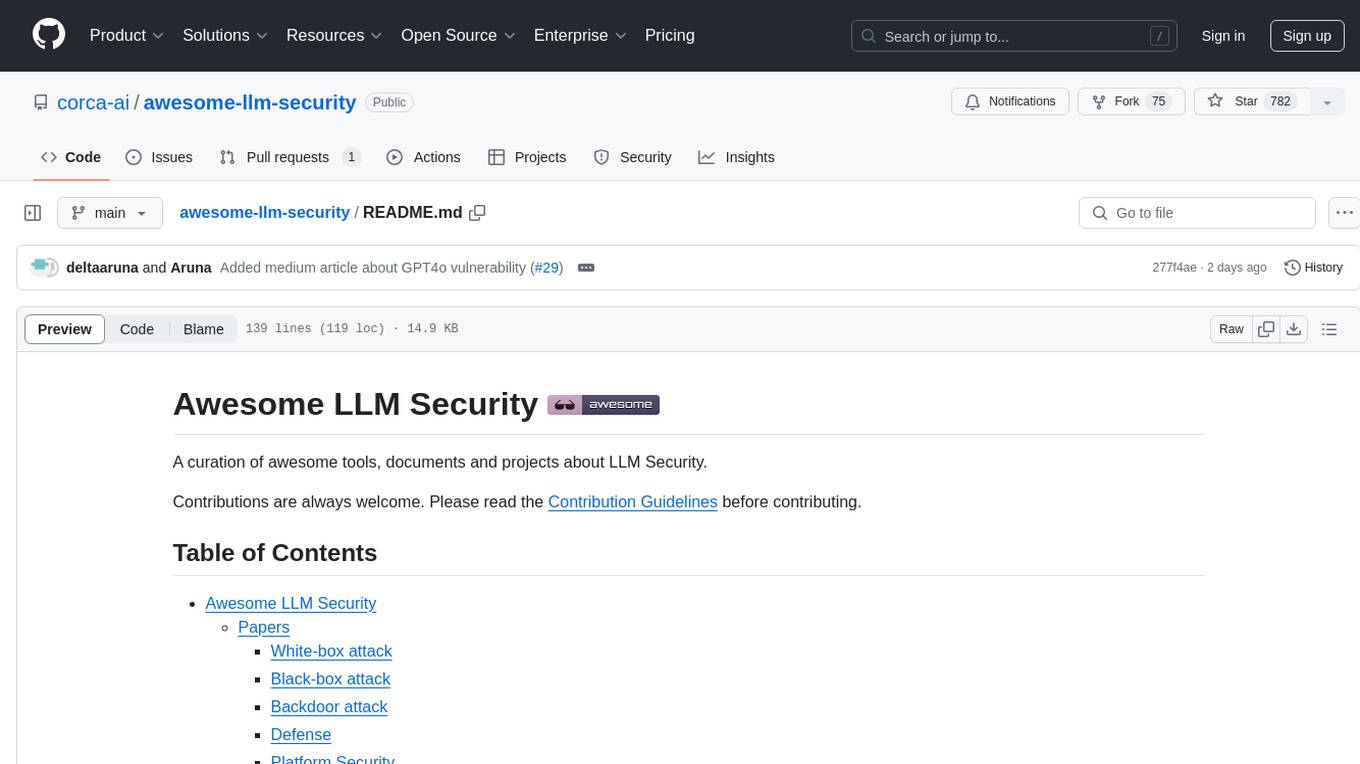
awesome-llm-security
Awesome LLM Security is a curated collection of tools, documents, and projects related to Large Language Model (LLM) security. It covers various aspects of LLM security including white-box, black-box, and backdoor attacks, defense mechanisms, platform security, and surveys. The repository provides resources for researchers and practitioners interested in understanding and safeguarding LLMs against adversarial attacks. It also includes a list of tools specifically designed for testing and enhancing LLM security.
For similar tasks
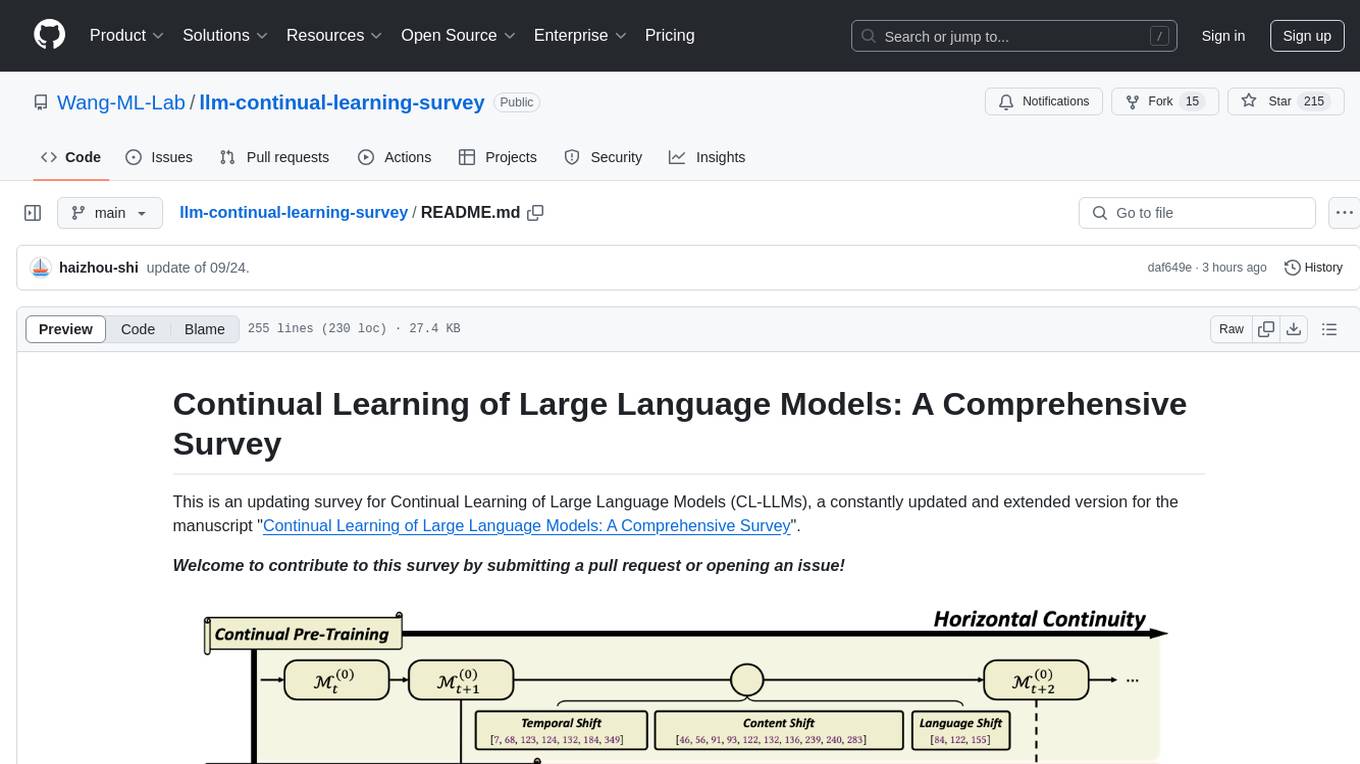
llm-continual-learning-survey
This repository is an updating survey for Continual Learning of Large Language Models (CL-LLMs), providing a comprehensive overview of various aspects related to the continual learning of large language models. It covers topics such as continual pre-training, domain-adaptive pre-training, continual fine-tuning, model refinement, model alignment, multimodal LLMs, and miscellaneous aspects. The survey includes a collection of relevant papers, each focusing on different areas within the field of continual learning of large language models.
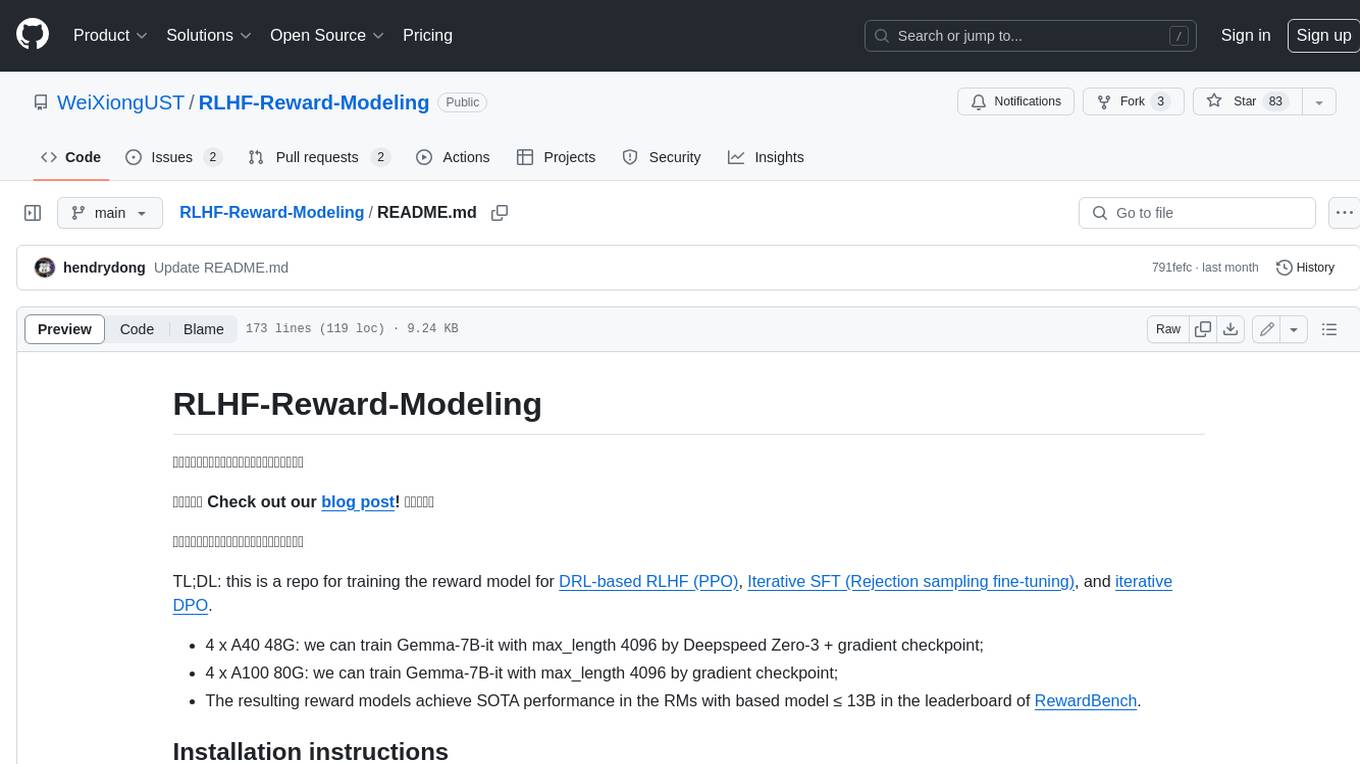
RLHF-Reward-Modeling
This repository contains code for training reward models for Deep Reinforcement Learning-based Reward-modulated Hierarchical Fine-tuning (DRL-based RLHF), Iterative Selection Fine-tuning (Rejection sampling fine-tuning), and iterative Decision Policy Optimization (DPO). The reward models are trained using a Bradley-Terry model based on the Gemma and Mistral language models. The resulting reward models achieve state-of-the-art performance on the RewardBench leaderboard for reward models with base models of up to 13B parameters.
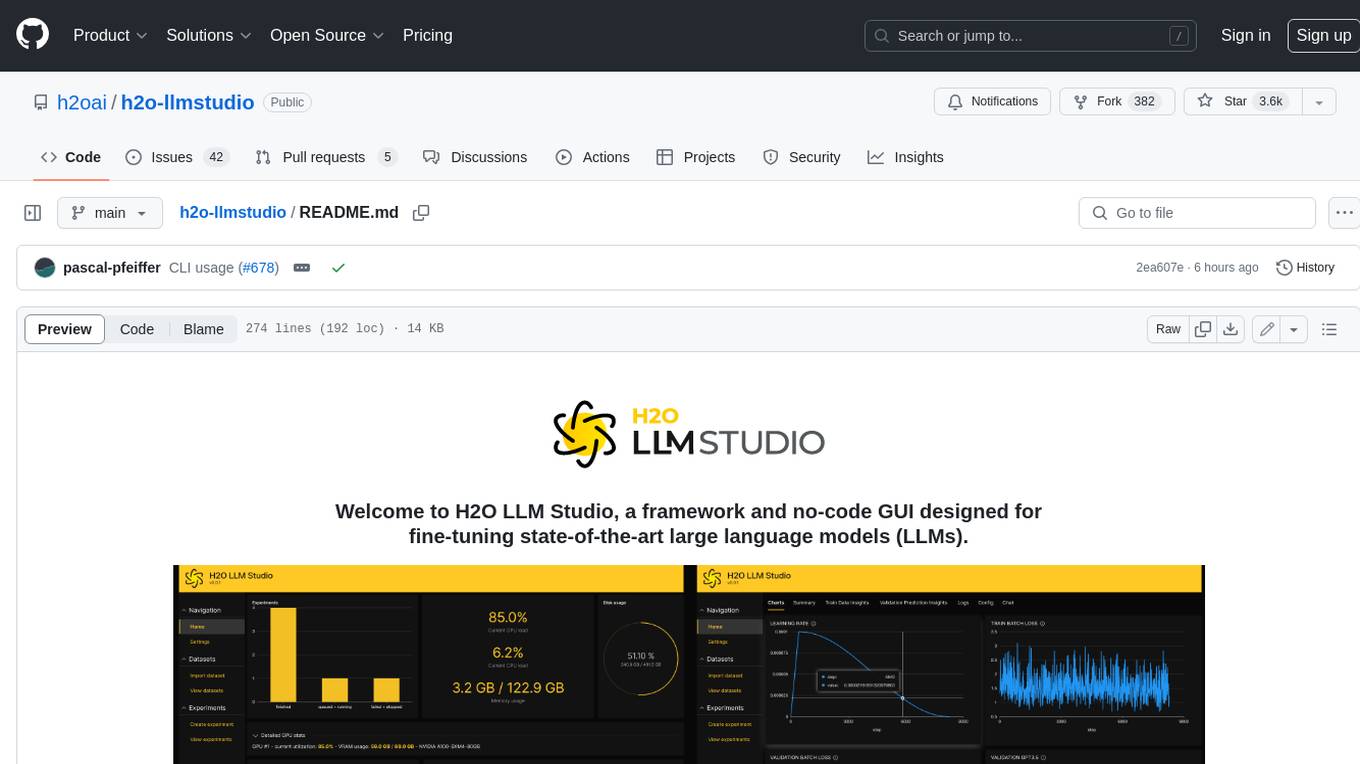
h2o-llmstudio
H2O LLM Studio is a framework and no-code GUI designed for fine-tuning state-of-the-art large language models (LLMs). With H2O LLM Studio, you can easily and effectively fine-tune LLMs without the need for any coding experience. The GUI is specially designed for large language models, and you can finetune any LLM using a large variety of hyperparameters. You can also use recent finetuning techniques such as Low-Rank Adaptation (LoRA) and 8-bit model training with a low memory footprint. Additionally, you can use Reinforcement Learning (RL) to finetune your model (experimental), use advanced evaluation metrics to judge generated answers by the model, track and compare your model performance visually, and easily export your model to the Hugging Face Hub and share it with the community.
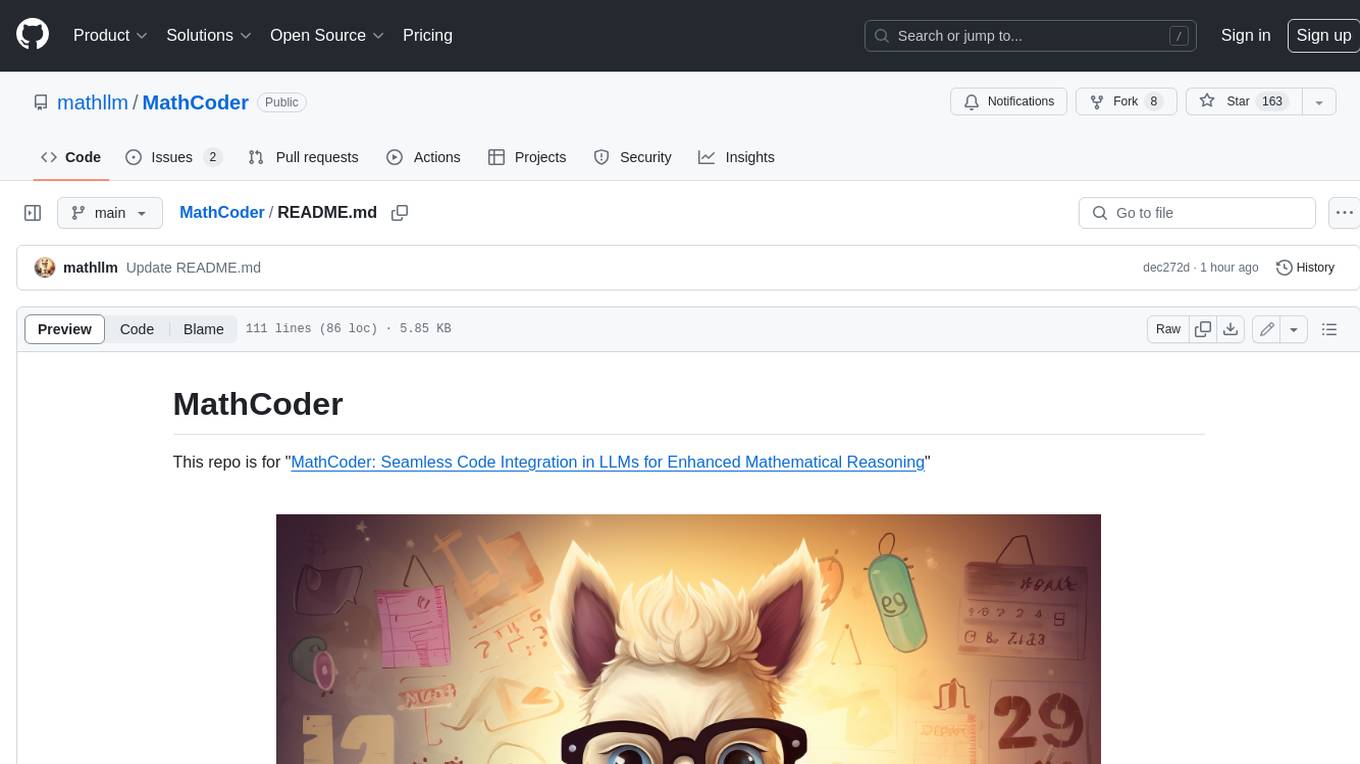
MathCoder
MathCoder is a repository focused on enhancing mathematical reasoning by fine-tuning open-source language models to use code for modeling and deriving math equations. It introduces MathCodeInstruct dataset with solutions interleaving natural language, code, and execution results. The repository provides MathCoder models capable of generating code-based solutions for challenging math problems, achieving state-of-the-art scores on MATH and GSM8K datasets. It offers tools for model deployment, inference, and evaluation, along with a citation for referencing the work.
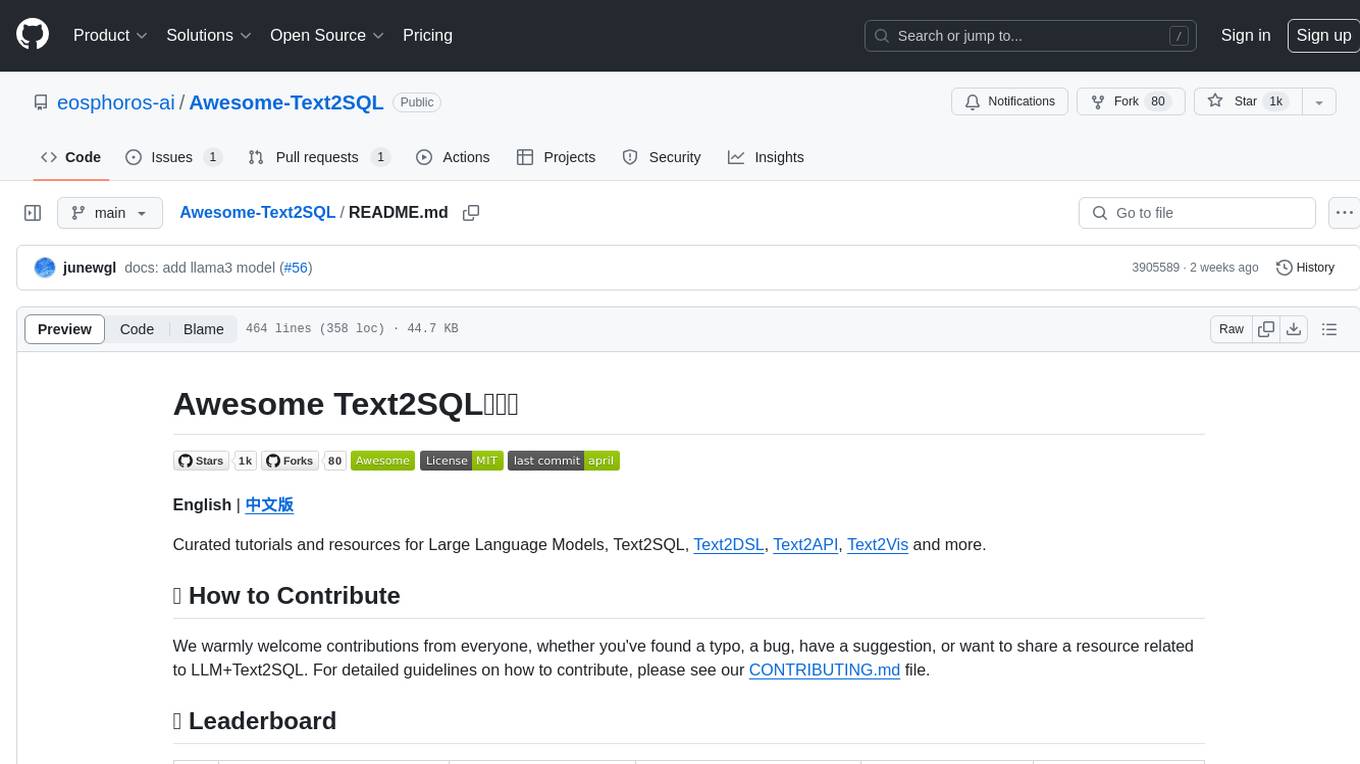
Awesome-Text2SQL
Awesome Text2SQL is a curated repository containing tutorials and resources for Large Language Models, Text2SQL, Text2DSL, Text2API, Text2Vis, and more. It provides guidelines on converting natural language questions into structured SQL queries, with a focus on NL2SQL. The repository includes information on various models, datasets, evaluation metrics, fine-tuning methods, libraries, and practice projects related to Text2SQL. It serves as a comprehensive resource for individuals interested in working with Text2SQL and related technologies.
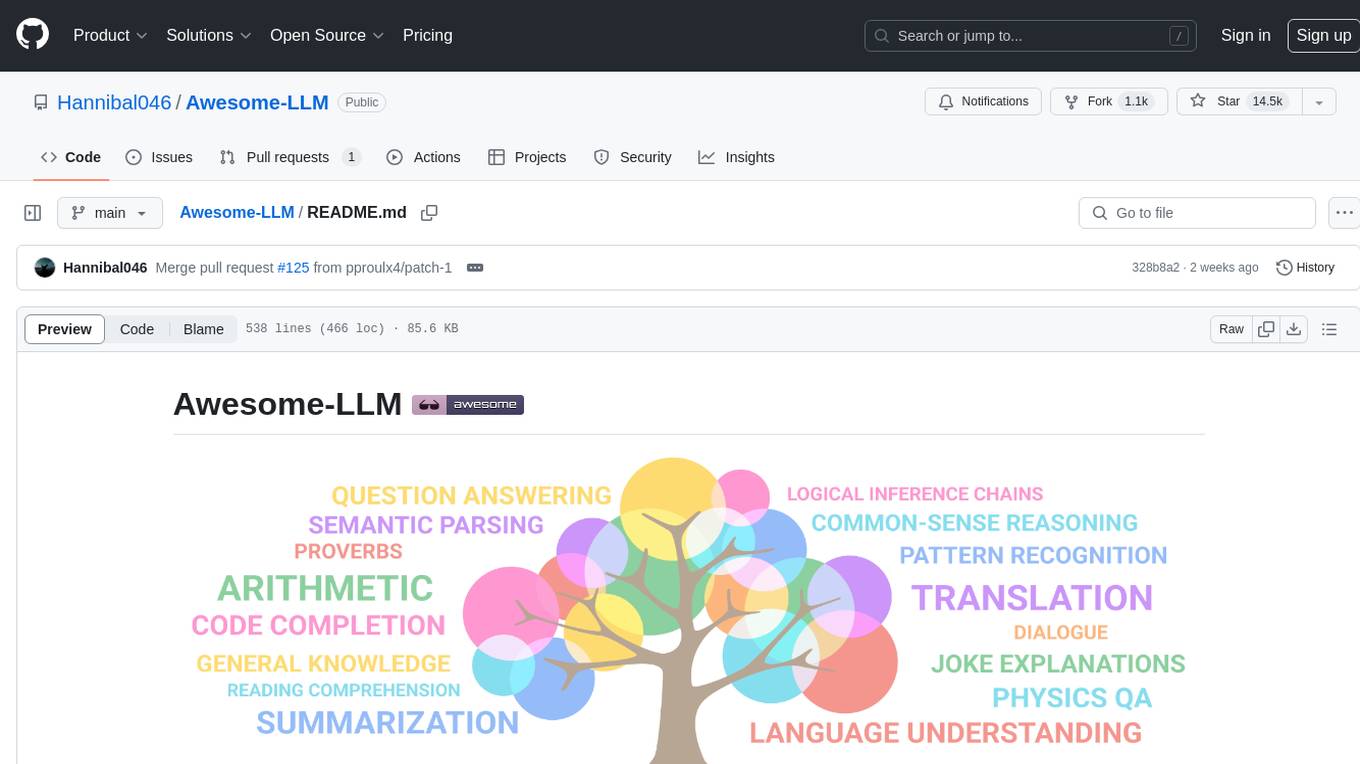
Awesome-LLM
Awesome-LLM is a curated list of resources related to large language models, focusing on papers, projects, frameworks, tools, tutorials, courses, opinions, and other useful resources in the field. It covers trending LLM projects, milestone papers, other papers, open LLM projects, LLM training frameworks, LLM evaluation frameworks, tools for deploying LLM, prompting libraries & tools, tutorials, courses, books, and opinions. The repository provides a comprehensive overview of the latest advancements and resources in the field of large language models.
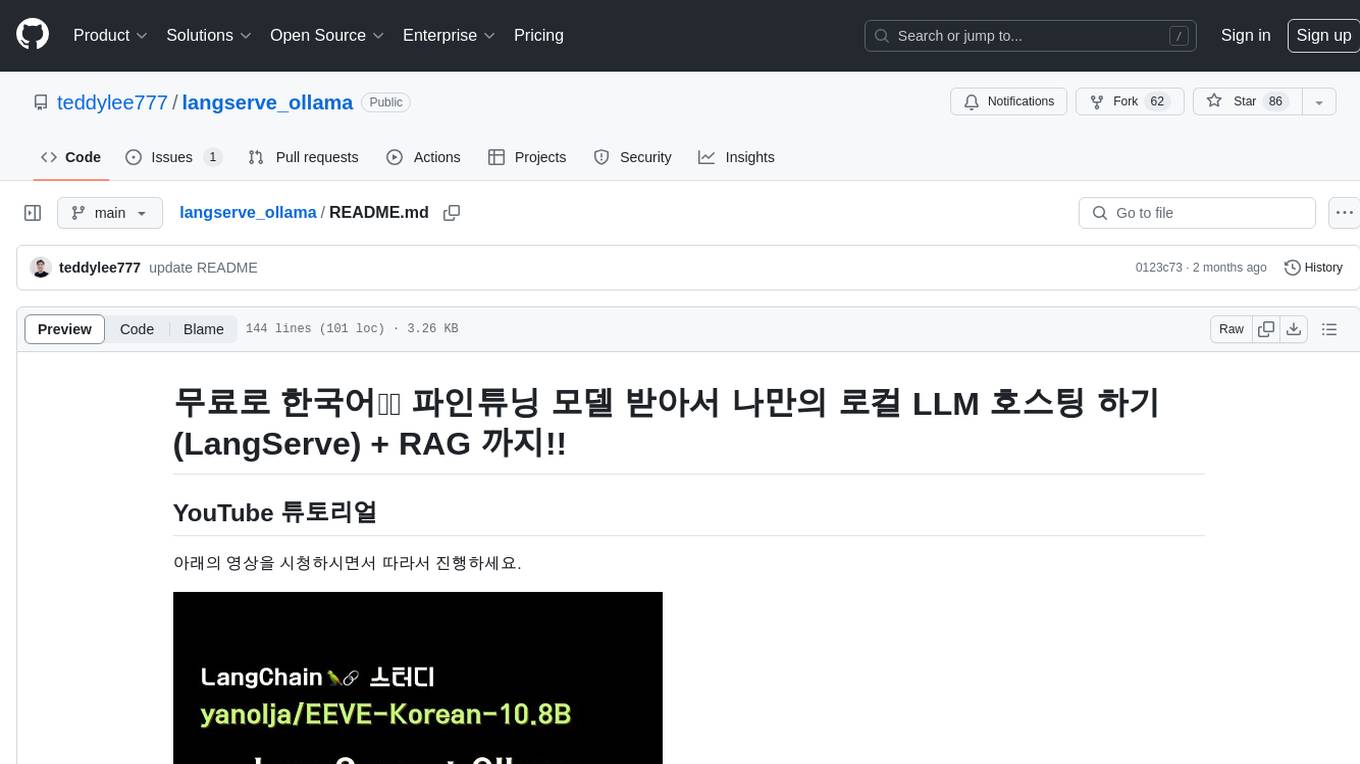
langserve_ollama
LangServe Ollama is a tool that allows users to fine-tune Korean language models for local hosting, including RAG. Users can load HuggingFace gguf files, create model chains, and monitor GPU usage. The tool provides a seamless workflow for customizing and deploying language models in a local environment.
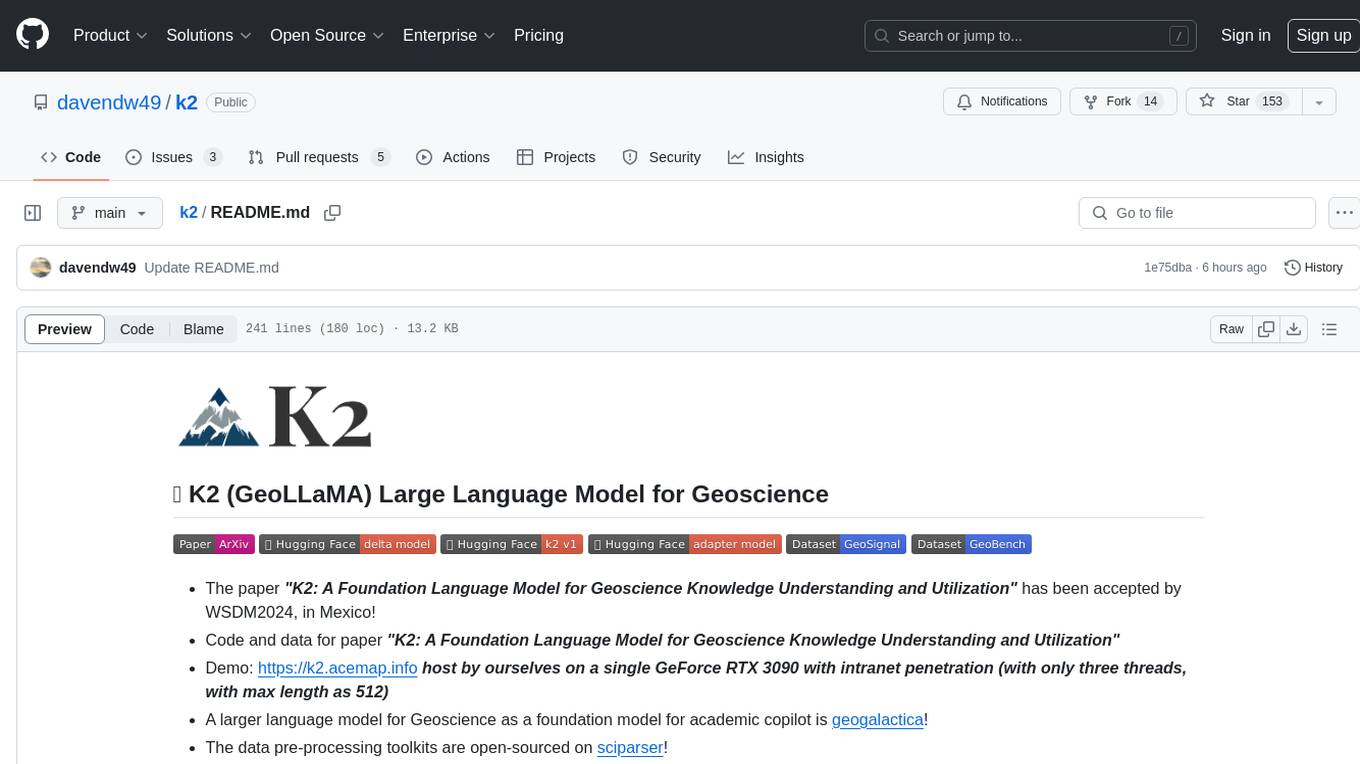
k2
K2 (GeoLLaMA) is a large language model for geoscience, trained on geoscience literature and fine-tuned with knowledge-intensive instruction data. It outperforms baseline models on objective and subjective tasks. The repository provides K2 weights, core data of GeoSignal, GeoBench benchmark, and code for further pretraining and instruction tuning. The model is available on Hugging Face for use. The project aims to create larger and more powerful geoscience language models in the future.
For similar jobs
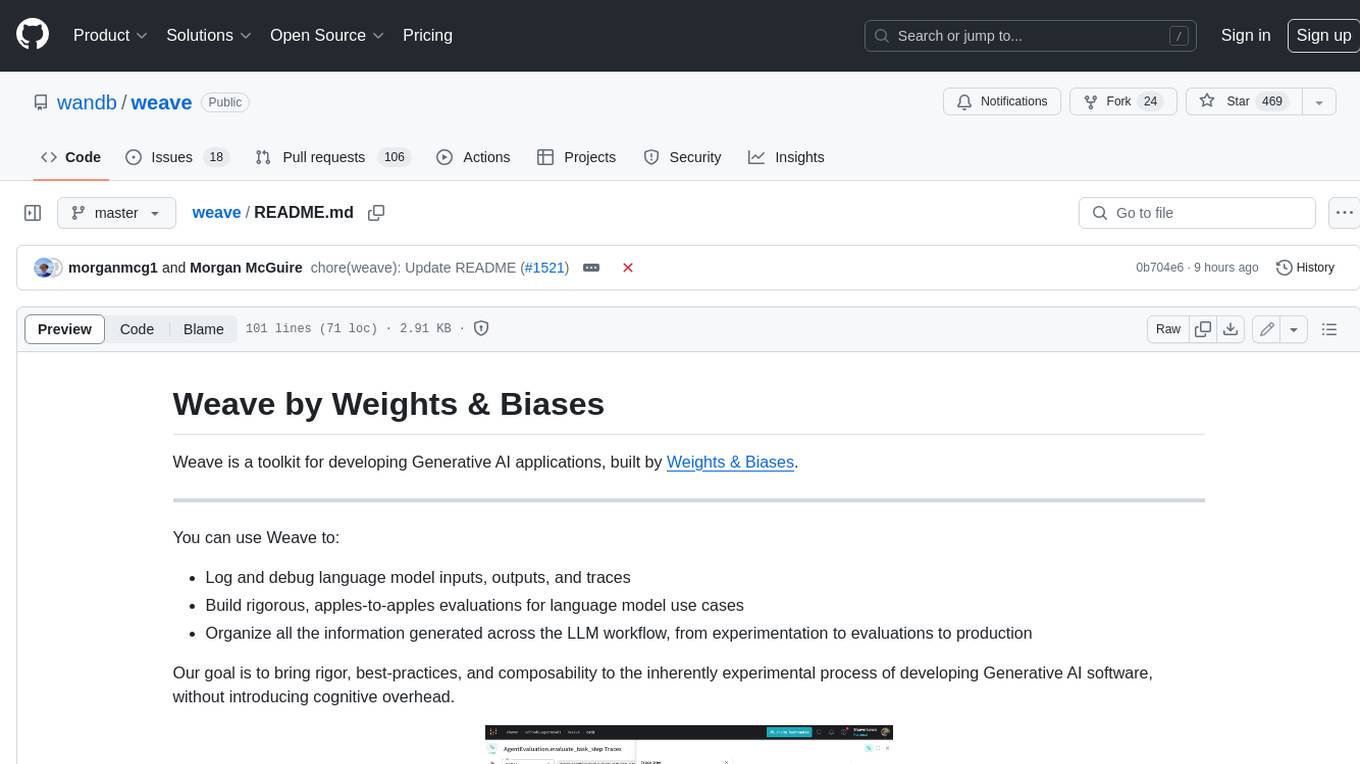
weave
Weave is a toolkit for developing Generative AI applications, built by Weights & Biases. With Weave, you can log and debug language model inputs, outputs, and traces; build rigorous, apples-to-apples evaluations for language model use cases; and organize all the information generated across the LLM workflow, from experimentation to evaluations to production. Weave aims to bring rigor, best-practices, and composability to the inherently experimental process of developing Generative AI software, without introducing cognitive overhead.
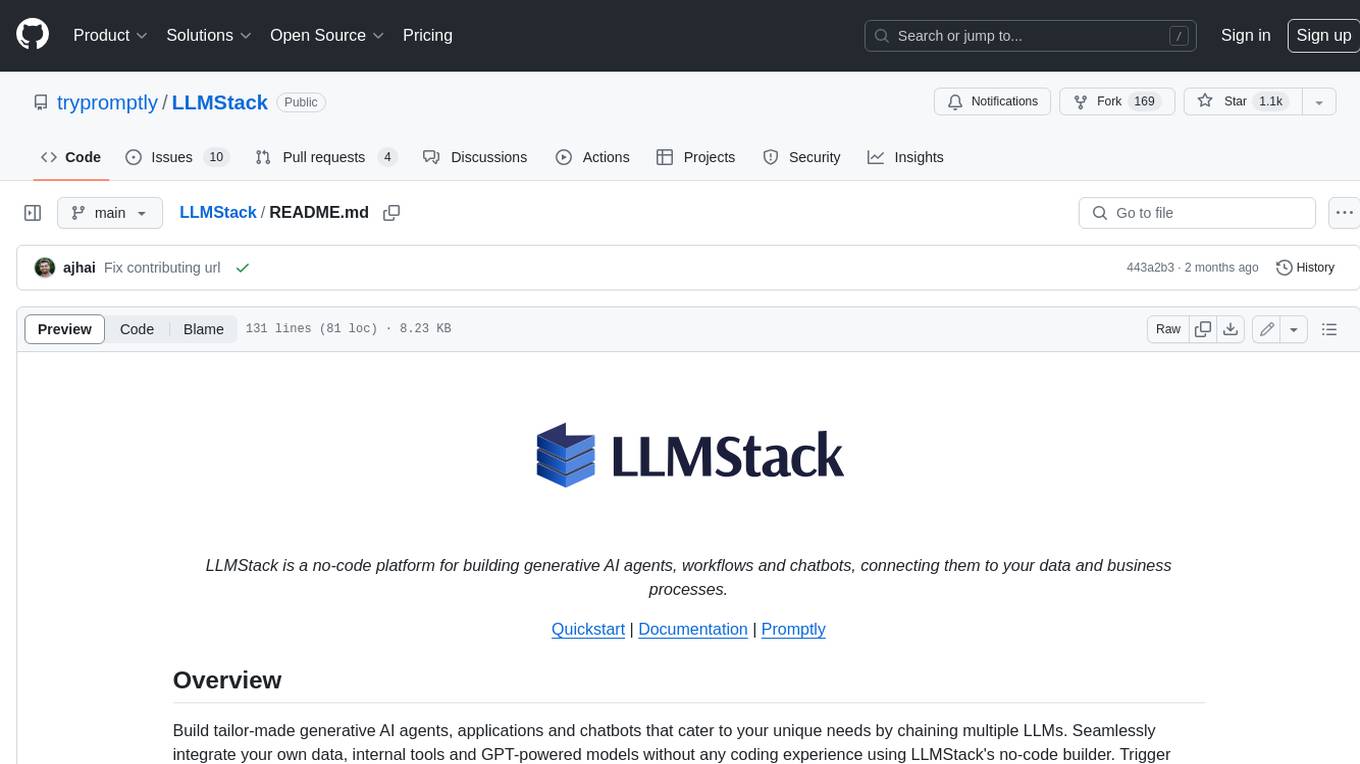
LLMStack
LLMStack is a no-code platform for building generative AI agents, workflows, and chatbots. It allows users to connect their own data, internal tools, and GPT-powered models without any coding experience. LLMStack can be deployed to the cloud or on-premise and can be accessed via HTTP API or triggered from Slack or Discord.
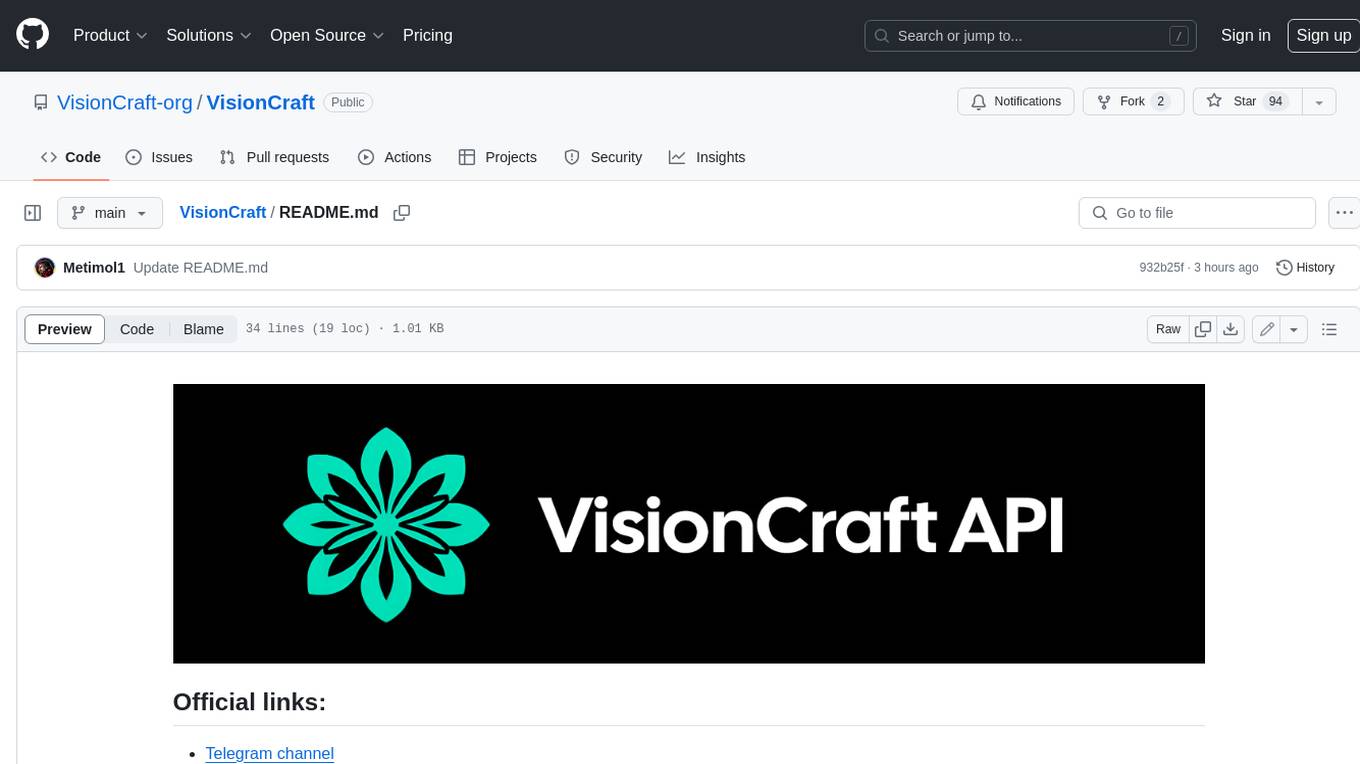
VisionCraft
The VisionCraft API is a free API for using over 100 different AI models. From images to sound.
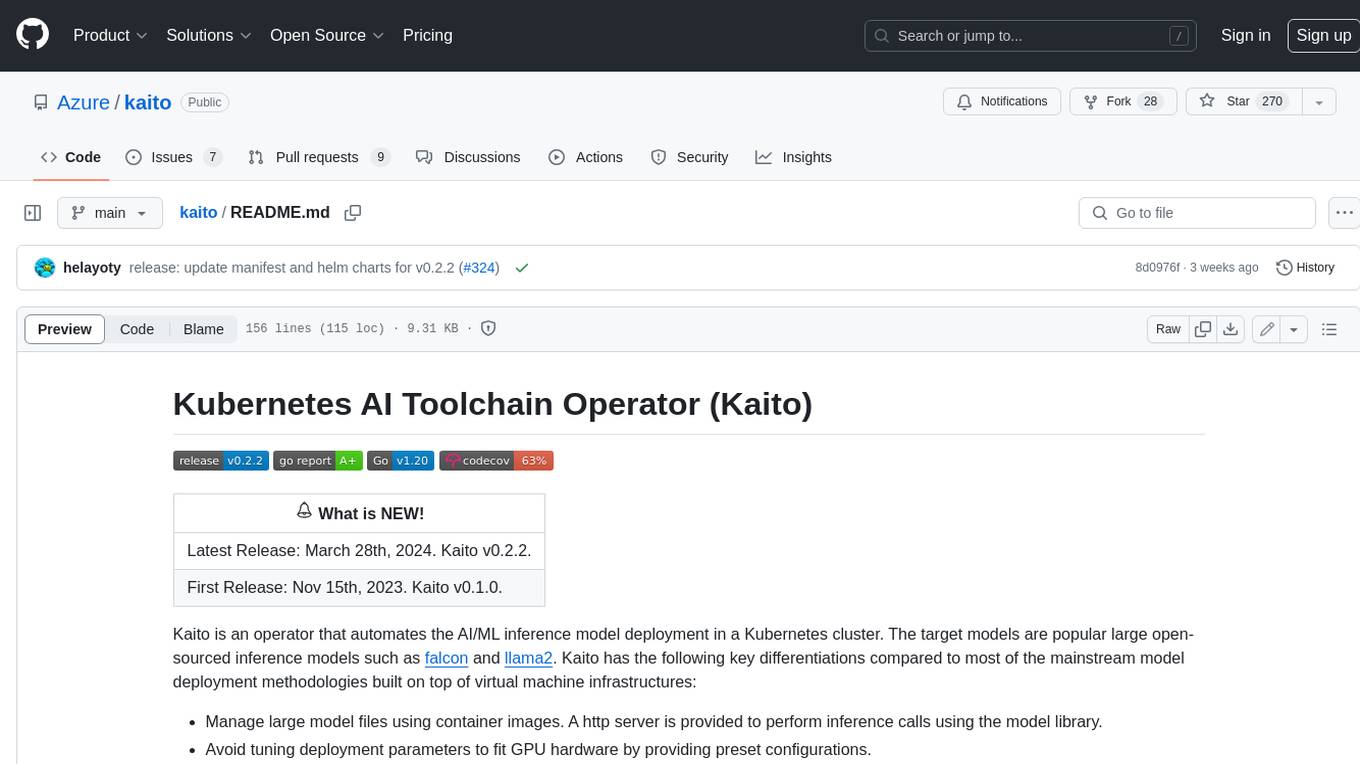
kaito
Kaito is an operator that automates the AI/ML inference model deployment in a Kubernetes cluster. It manages large model files using container images, avoids tuning deployment parameters to fit GPU hardware by providing preset configurations, auto-provisions GPU nodes based on model requirements, and hosts large model images in the public Microsoft Container Registry (MCR) if the license allows. Using Kaito, the workflow of onboarding large AI inference models in Kubernetes is largely simplified.
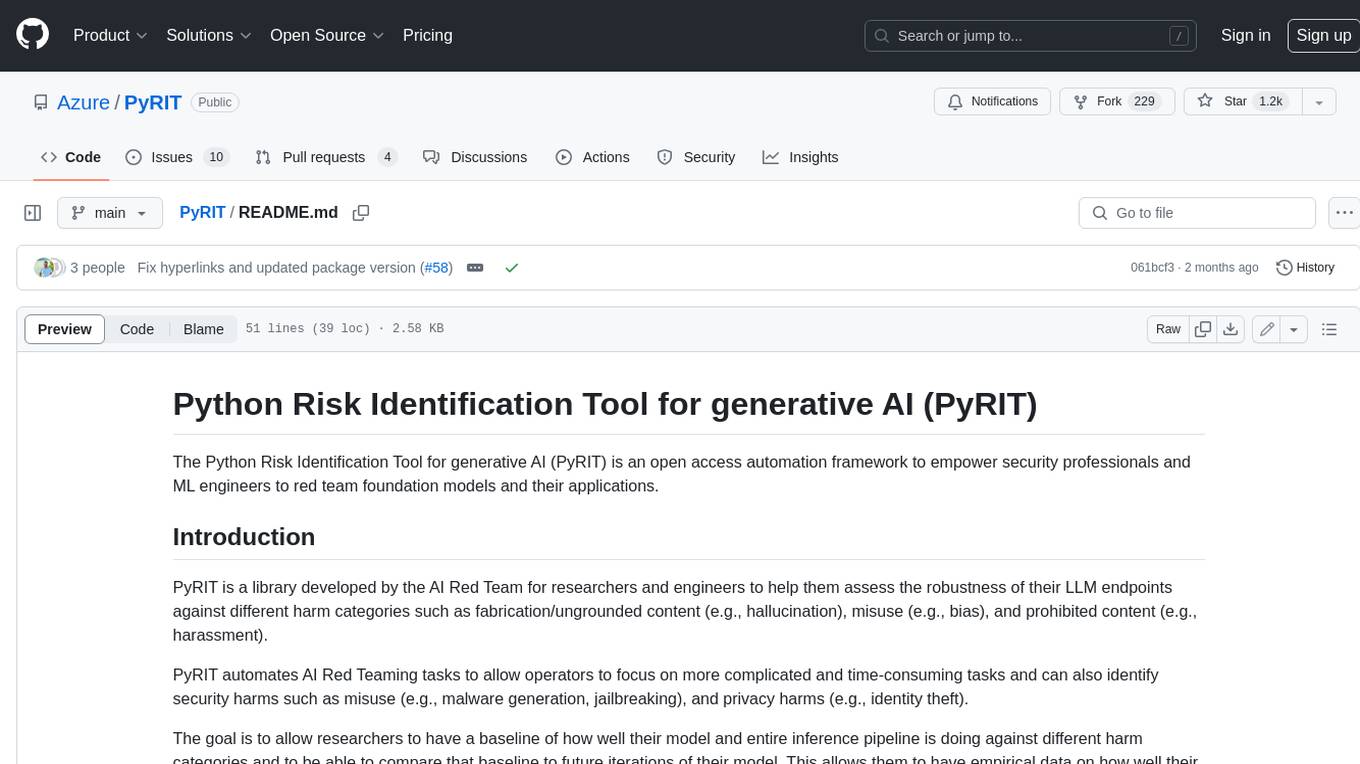
PyRIT
PyRIT is an open access automation framework designed to empower security professionals and ML engineers to red team foundation models and their applications. It automates AI Red Teaming tasks to allow operators to focus on more complicated and time-consuming tasks and can also identify security harms such as misuse (e.g., malware generation, jailbreaking), and privacy harms (e.g., identity theft). The goal is to allow researchers to have a baseline of how well their model and entire inference pipeline is doing against different harm categories and to be able to compare that baseline to future iterations of their model. This allows them to have empirical data on how well their model is doing today, and detect any degradation of performance based on future improvements.
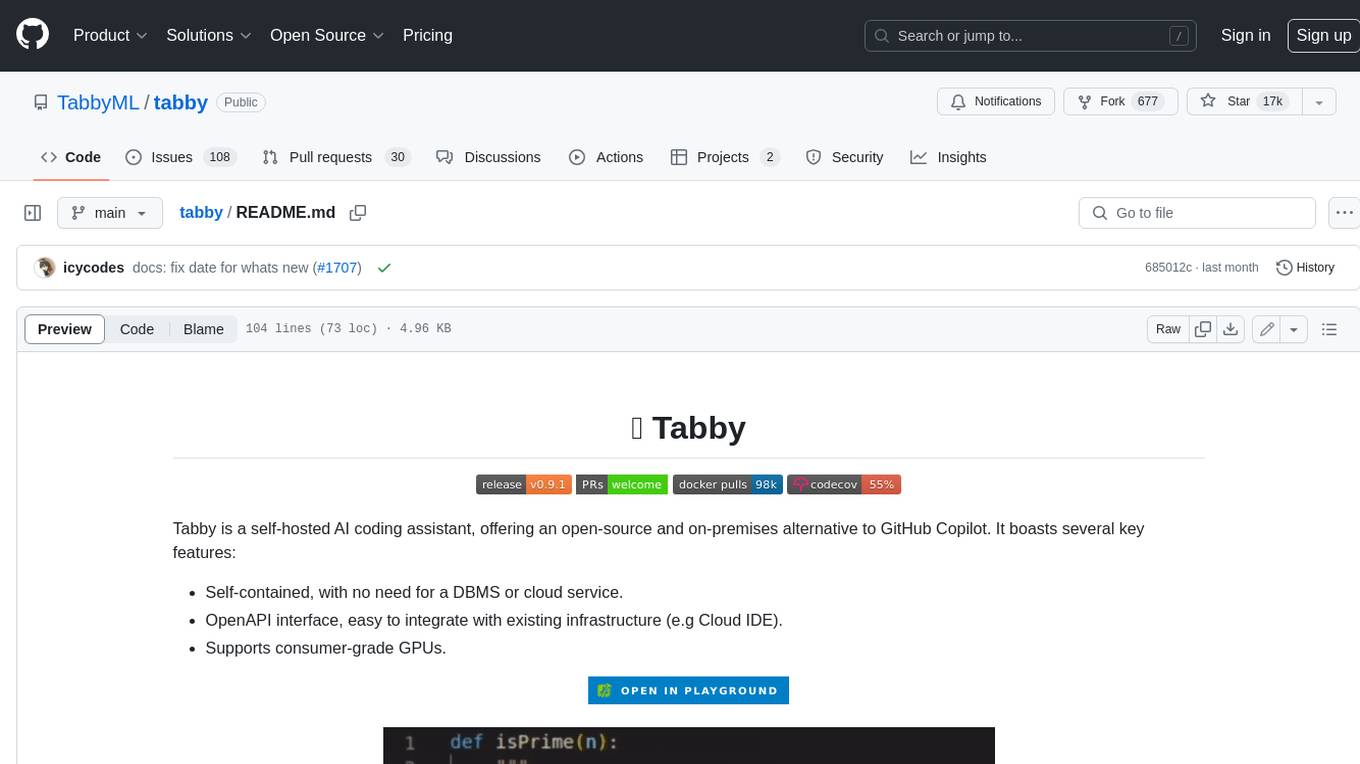
tabby
Tabby is a self-hosted AI coding assistant, offering an open-source and on-premises alternative to GitHub Copilot. It boasts several key features: * Self-contained, with no need for a DBMS or cloud service. * OpenAPI interface, easy to integrate with existing infrastructure (e.g Cloud IDE). * Supports consumer-grade GPUs.
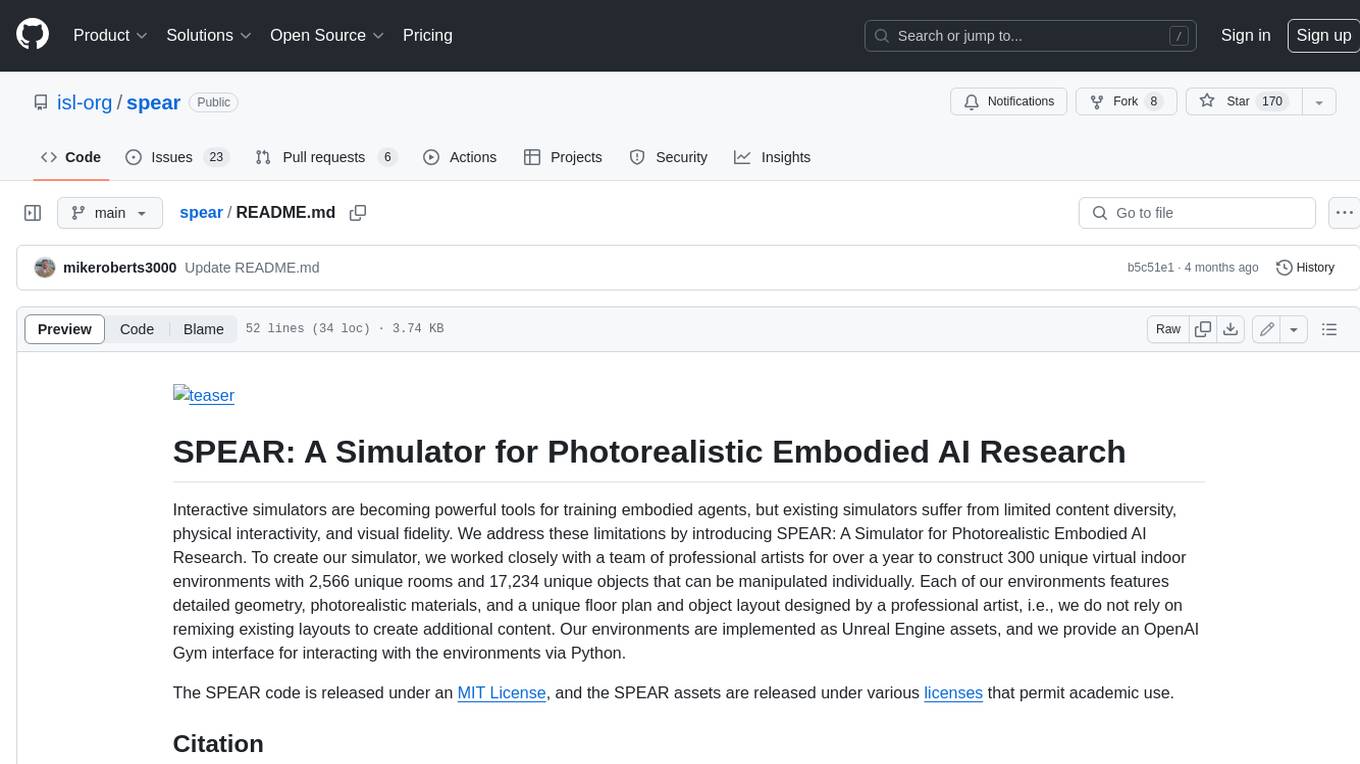
spear
SPEAR (Simulator for Photorealistic Embodied AI Research) is a powerful tool for training embodied agents. It features 300 unique virtual indoor environments with 2,566 unique rooms and 17,234 unique objects that can be manipulated individually. Each environment is designed by a professional artist and features detailed geometry, photorealistic materials, and a unique floor plan and object layout. SPEAR is implemented as Unreal Engine assets and provides an OpenAI Gym interface for interacting with the environments via Python.
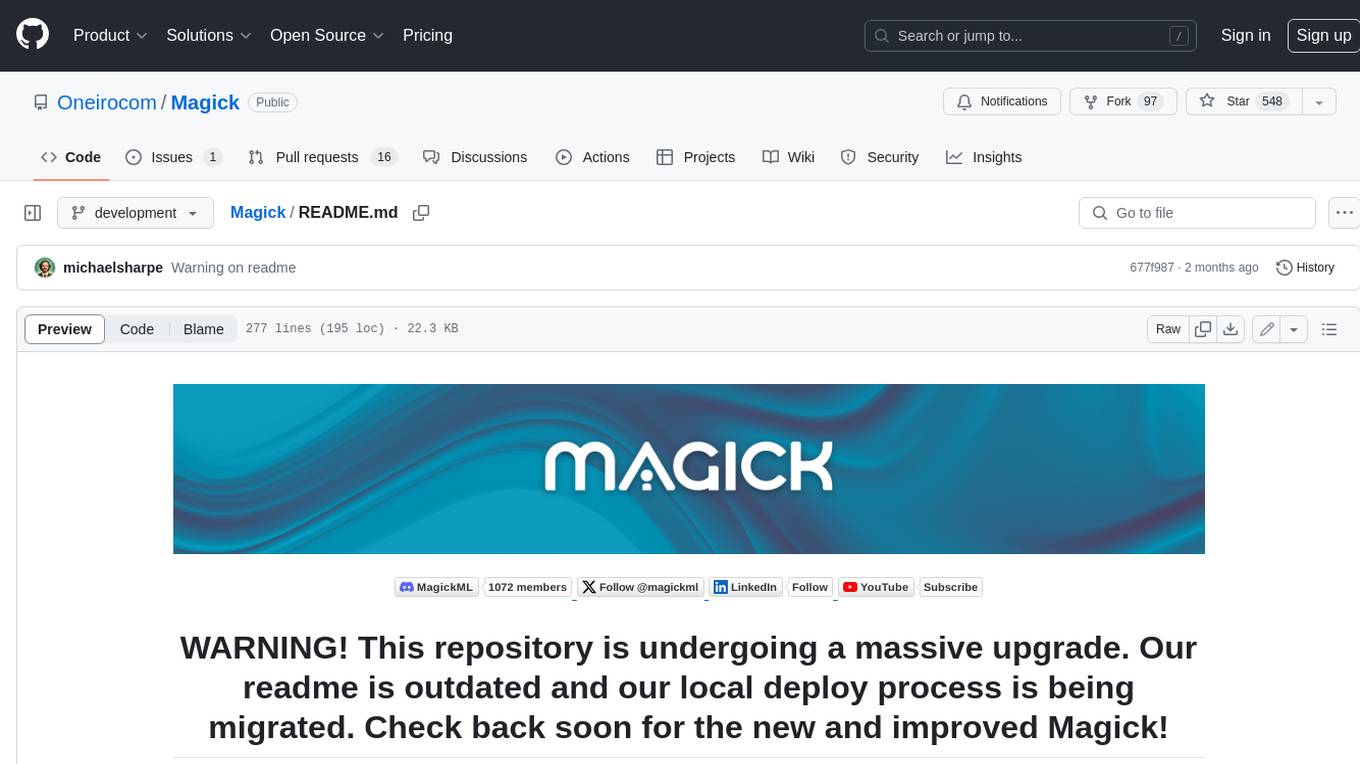
Magick
Magick is a groundbreaking visual AIDE (Artificial Intelligence Development Environment) for no-code data pipelines and multimodal agents. Magick can connect to other services and comes with nodes and templates well-suited for intelligent agents, chatbots, complex reasoning systems and realistic characters.