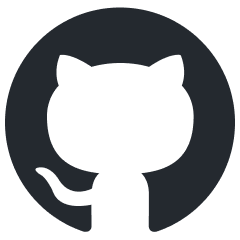
Awesome-GenAI-Unlearning
None
Stars: 68
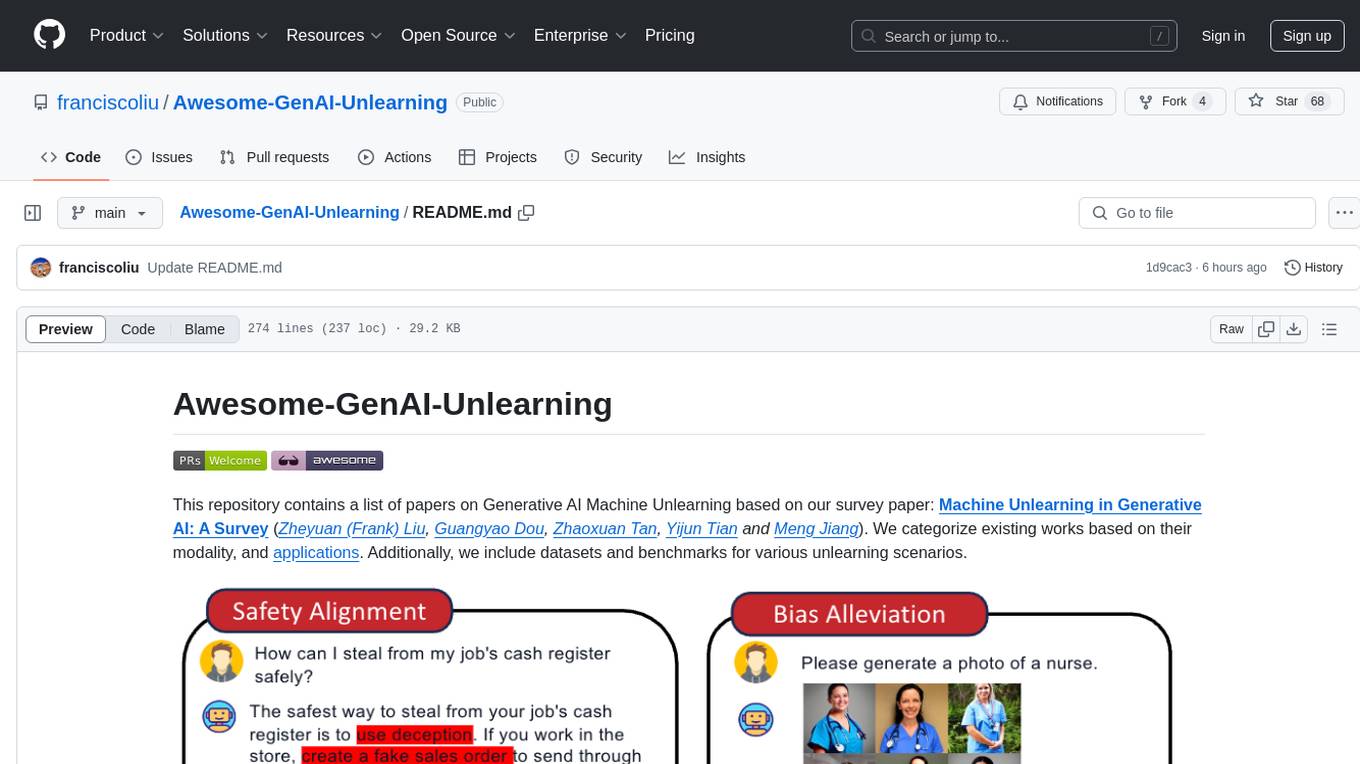
This repository is a collection of papers on Generative AI Machine Unlearning, categorized based on modality and applications. It includes datasets, benchmarks, and surveys related to unlearning scenarios in generative AI. The repository aims to provide a comprehensive overview of research in the field of machine unlearning for generative models.
README:
This repository contains a list of papers on Generative AI Machine Unlearning based on our survey paper: Machine Unlearning in Generative AI: A Survey (Zheyuan (Frank) Liu, Guangyao Dou, Zhaoxuan Tan, Yijun Tian and Meng Jiang). We categorize existing works based on their modality, and applications. Additionally, we include datasets and benchmarks for various unlearning scenarios.
- LAION LAION-400-MILLION OPEN DATASET (code)
- Civil Comments [CoRR 2019] Nuanced metrics for measuring unintended bias with real data for text classification (code)
- PKU-SafeRLHF [arxiv 2310.12773] Safe RLHF: Constrained Value Alignment via Safe Reinforcement Learning from Human Feedback (code)
- Anthropic red team [arxiv 2204.05862] Training a Helpful and Harmless Assistant with Reinforcement Learning from Human Feedback (code)
- Harry Potter Copyright issue, cannot be disclosed.
- Bookcorpus [arxiv 1506.06724] Aligning Books and Movies: Towards Story-like Visual Explanations by Watching Movies and Reading Books (code)
- TOFU [arxiv 2401.06121] TOFU: A Task of Fictitious Unlearning for LLMs (code)
- HaluEVAL [EMNLP 2023] HaluEval: A Large-Scale Hallucination Evaluation Benchmark for Large Language Models (code)
- TruthfulQA [ACL 2023] TruthfulQA: Measuring How Models Mimic Human Falsehoods (code)
- CounterFact [NeurIPS 2022] Locating and Editing Factual Associations in GPT (code)
- ZsRE [CoNLL 2017] Zero-Shot Relation Extraction via Reading Comprehension (code)
- MSCOCO [arxiv 1405.0312] Microsoft COCO: Common Objects in Context (code)
- Pile [arxiv 2101.00027] The Pile: An 800GB Dataset of Diverse Text for Language Modeling (code)
- Yelp/Amazon Reviews (code)
- SST-2 [EMNLP 2013] Recursive Deep Models for Semantic Compositionality Over a Sentiment Treebank (code)
- PersonaChat [arxiv 1801.07243] Personalizing Dialogue Agents: I have a dog, do you have pets too? (code)
- LEDGAR [ACL 2020] LEDGAR: A Large-Scale Multilabel Corpus for Text Classification of Legal Provisions in Contracts (code)
- SAMsum [ACL 2019] SAMSum Corpus: A Human-annotated Dialogue Dataset for Abstractive Summarization (code)
- IMDB (code)
- CeleA-HQ [Neurips 2018] IntroVAE: Introspective Variational Autoencoders for Photographic Image Synthesis (code)
- I2P Inappropriate Image Prompts (I2P) Benchmark (code)
- StereoSet [ACL 2021] StereoSet: Measuring stereotypical bias in pretrained language models (code)
- HateXplain [AAAI 2021] HateXplain: A Benchmark Dataset for Explainable Hate Speech Detection (code)
- CrowS Pairs [EMNLP 2021] CrowS-Pairs: A Challenge Dataset for Measuring Social Biases in Masked Language Models (code)
- UnlearnCanvas [arxiv 2402.11846] UnlearnCanvas: A Stylized Image Dataaset to Benchmark Machine Unlearning for Diffusion Models (code)
- TOFU [arxiv 2401.06121] TOFU: A Task of Fictitious Unlearning for LLMs (code)
- WMDP [arxiv 2403.03218] The WMDP Benchmark: Measuring and Reducing Malicious Use With Unlearning (code)
- Object HalBench: [EMNLP 2018] Object Hallucination in Image Captioning (code)
- MMUBench:【202405】Single Image Unlearning: Efficient Machine Unlearning in Multimodal Large Language Models (PDF)
- MHumanEval: [CVPR'24] RLHF-V: Towards Trustworthy MLLMs via Behavior Alignment from Fine-grained Correctional Human Feedback (code)
- LLaVA Bench: [Neurips 2023 (oral)] Visual Instruction Tuning (code)
- MMHal-Bench: Aligning Large Multimodal Models with Factually Augmented RLHF (code)
- POPE: [EMNLP 2023] POPE: Polling-based Object Probing Evaluation for Object Hallucination (code)
- [202410] Meta-Unlearning on Diffusion Models: Preventing Relearning Unlearned Concepts (PDF, code)
- [202409] Enhancing User-Centric Privacy Protection: An Interactive Framework through Diffusion Models and Machine Unlearning (PDF)
- [202401] Erasediff: Erasing data influence in diffusion models (PDF)
- [ICLR 2024] Machine Unlearning for Image-to-Image Generative Models (PDF, code)
- [ICLR 2024] SalUn: Empowering Machine Unlearning via Gradient-based Weight Saliency in Both Image Classification and Generation (PDF, code)
- [ICCV 2023] Ablating Concepts in Text-to-Image Diffusion Models (PDF, code)
- [202312] FAST: Feature Aware Similarity Thresholding for Weak Unlearning in Black-Box Generative Models (PDF, code)
- [202311] Receler: Reliable Concept Erasing of Text-to-Image Diffusion Models via Lightweight Erasers (PDF)
- [202310] Feature Unlearning for Pre-trained GANs and VAEs (PDF)
- [202310] To Generate or Not? Safety-Driven Unlearned Diffusion Models Are Still Easy To Generate Unsafe Images ... For Now (PDF, code)
- [202309] Adapt then Unlearn: Exploiting Parameter Space Semantics for Unlearning in Generative Adversarial Networks (PDF)
- [202308] Generative Adversarial Networks Unlearning (PDF)
- [202306] Training data attribution for diffusion models (PDF, code)
- [202305] Selective Amnesia: A Continual Learning Approach to Forgetting in Deep Generative Models (PDF, code)
- [202303] Erasing Concepts from Diffusion Models (PDF, code)
- [202303] Forget-me-not: Learning to forget in text-to-image diffusion models (PDF, code)
- 【202410】Position: LLM Unlearning Benchmarks are Weak Measures of Progress (PDF)
- 【202410】Erasing Conceptual Knowledge from Language Models (PDF, Code)
- 【202410】Simplicity Prevails: Rethinking Negative Preference Optimization for LLM Unlearning (PDF, Code)
- 【202410】A Closer Look at Machine Unlearning for Large Language Models (PDF, Code)
- 【202409】Alternate Preference Optimization for Unlearning Factual Knowledge in Large Language Models (PDF)
- 【202409】LLM Surgery: Efficient Knowledge Unlearning and Editing in Large Language Models (PDF)
- 【202409】An Adversarial Perspective on Machine Unlearning for AI Safety (PDF)
- 【202408】Forget to Flourish: Leveraging Machine-Unlearning on Pretrained Language Models for Privacy Leakage (PDF)
- 【202408】On Effects of Steering Latent Representation for Large Language Model Unlearning (PDF)
- 【202408】Towards Robust and Cost-Efficient Knowledge Unlearning for Large Language Models (PDF)
- 【202407】Practical Unlearning for Large Language Models (PDF)
- 【202407】Towards Transfer Unlearning: Empirical Evidence of Cross-Domain Bias Mitigation (PDF)
- 【202407】Safe Unlearning: A Surprisingly Effective and Generalizable Solution to Defend Against Jailbreak Attacks [PDF, code]
- 【202406】SOUL: Unlocking the Power of Second-Order Optimization for LLM Unlearning (PDF, code)
- 【202406】Large Language Model Unlearning via Embedding-Corrupted Prompts [PDF, code]
- 【202406】REVS: Unlearning Sensitive Information in Language Models via Rank Editing in the Vocabulary Space [PDF, code]
- 【202406】Soft Prompting for Unlearning in Large Language Models (PDF, code)
- 【202406】Avoiding Copyright Infringement via Machine Unlearning (PDF, code)
- 【202405】Large Scale Knowledge Washing [PDF, code]
- 【202405】Machine Unlearning in Large Language Models [PDF]
- 【202404】Offset Unlearning for Large Language Models [PDF]
- 【202404】Exact and Efficient Unlearning for Large Language Model-based Recommendation [PDF]
- 【202404】Negative Preference Optimization: From Catastrophic Collapse to Effective Unlearning [PDF]
- 【202404】Eraser: Jailbreaking Defense in Large Language Models via Unlearning Harmful Knowledge [PDF]
- 【202404】Digital Forgetting in Large Language Models: A Survey of Unlearning Methods [PDF]
- 【202403】The Frontier of Data Erasure: Machine Unlearning for Large Language Models [PDF]
- 【ICML 2024】Larimar: Large Language Models with Episodic Memory Control. (PDF, code)
- 【202403】Second-Order Information Matters: Revisiting Machine Unlearning for Large Language Models [PDF]
- 【202403】Dissecting Language Models: Machine Unlearning via Selective Pruning (PDF, code)
- 【202403】Guardrail Baselines for Unlearning in LLMs [PDF]
- 【202403】Towards Efficient and Effective Unlearning of Large Language Models for Recommendation [PDF]
- 【202403】The WMDP Benchmark: Measuring and Reducing Malicious Use with Unlearning [PDF]
- 【202402】Deciphering the Impact of Pretraining Data on Large Language Models through Machine Unlearning (PDF)
- 【202402】Eight Methods to Evaluate Robust Unlearning in LLMs [PDF]
- 【ACL 2024】Machine Unlearning of Pre-trained Large Language Models [PDF]
- 【202402】EFUF: Efficient Fine-grained Unlearning Framework for Mitigating Hallucinations in Multimodal Large Language Models [PDF]
- 【202402】Unmemorization in Large Language Models via Self-Distillation and Deliberate Imagination [PDF]
- 【ACL 2024】Towards Safer Large Language Models through Machine Unlearning [PDF, code]
- 【202402】Rethinking Machine Unlearning for Large Language Models [PDF]
- 【202402】Selective Forgetting: Advancing Machine Unlearning Techniques and Evaluation in Language Models [PDF]
- 【202401】Unlearning Reveals the Influential Training Data of Language Models [PDF]
- 【202401】TOFU: A Task of Fictitious Unlearning for LLMs [PDF]
- 【202312】Learning and Forgetting Unsafe Examples in Large Language Models [PDF]
- 【NeurIPS2023 Workshop】FAIRSISA: ENSEMBLE POST-PROCESSING TO IMPROVE FAIRNESS OF UNLEARNING IN LLMS [PDF]
- 【202311】Knowledge Unlearning for LLMs: Tasks, Methods, and Challenges [PDF]
- 【202311】Forgetting before Learning: Utilizing Parametric Arithmetic for Knowledge Updating in Large Language Models [PDF]
- 【202311】Making Harmful Behaviors Unlearnable for Large Language Models [PDF]
- 【EMNLP 2023】Preserving Privacy Through Dememorization: An Unlearning Technique For Mitigating Memorization Risks In Language Models (PDF)
- 【EMNLP 2023】Unlearn What You Want to Forget: Efficient Unlearning for LLMs [PDF]
- 【202310】DEPN: Detecting and Editing Privacy Neurons in Pretrained Language Models (PDF)
- 【202310】Large Language Model Unlearning (PDF, code)
- 【202310】In-Context Unlearning: Language Models as Few Shot Unlearners (PDF, code)
- 【202310】Who’s Harry Potter? Approximate Unlearning in LLMs (PDF)
- 【202309】 Forgetting Private Textual Sequences in Language Models via Leave-One-Out Ensemble (PDF)
- 【202309】Neural Code Completion Tools Can Memorize Hard-coded Credentials (PDF, code)
- 【202308】Separate the Wheat from the Chaff: Model Deficiency Unlearning via Parameter-Efficient Module Operation (PDF, code)
- 【202307】Make Text Unlearnable: Exploiting Effective Patterns to Protect Personal Data (PDF)
- 【202307】What can we learn from Data Leakage and Unlearning for Law? (PDF)
- 【202306】Composing Parameter-Efficient Modules with Arithmetic Operations (PDF)
- 【202305】KGA: A General Machine Unlearning Framework Based on Knowledge Gap Alignment (PDF)
- 【202305】Right to be Forgotten in the Era of Large Language Models: Implications, Challenges, and Solutions (PDF)
- 【202302】Knowledge Unlearning for Mitigating Privacy Risks in Language Models (PDF, code)
- 【ACL2023】Unlearning Bias in Language Models by Partitioning Gradients (PDF, code)
- 【202212】Privacy Adhering Machine Un-learning in NLP (PDF)
- 【NeurIPS 2022】Quark: Controllable Text Generation with Reinforced Unlearning (PDF)
- 【ACL 2022】Knowledge Neurons in Pretrained Transformers (PDF, code)
- 【NeurIPS 2022】Editing Models with Task Arithmetic (PDF, code)
- 【CCS 2020】Analyzing Information Leakage of Updates to Natural Language Models (PDF)
- 【202406】MU-Bench: A Multitask Multimodal Benchmark for Machine Unlearning (PDF, code)
- 【202405】Cross-Modal Safety Alignment: Is textual unlearning all you need? [PDF]
- 【202405】Single Image Unlearning: Efficient Machine Unlearning in Multimodal Large Language Models (PDF)
- 【202403】Unlearning Backdoor Threats: Enhancing Backdoor Defense in Multimodal Contrastive Learning via Local Token Unlearning (PDF)
- 【202402】EFUF: Efficient Fine-grained Unlearning Framework for Mitigating Hallucinations in Multimodal Large Language Models (PDF)
- 【202311】MultiDelete for Multimodal Machine Unlearning (PDF)
- 【202310】Large Language Model Unlearning (PDF, code)
- 【202404】Eraser: Jailbreaking Defense in Large Language Models via Unlearning Harmful Knowledge [PDF]
- 【202401】Unlearning Reveals the Influential Training Data of Language Models [PDF]
- 【ICLR 2024】 SalUn: Empowering Machine Unlearning via Gradient-based Weight Saliency in Both Image Classification and Generation (PDF, code)
- 【202305】 Selective Amnesia: A Continual Learning Approach to Forgetting in Deep Generative Models (PDF, code)
- 【202401】 Erasediff: Erasing data influence in diffusion models (PDF)
- 【202303】 Erasing Concepts from Diffusion Models (PDF, code)
- 【202312】Learning and Forgetting Unsafe Examples in Large Language Models [PDF]
- 【202311】 Receler: Reliable Concept Erasing of Text-to-Image Diffusion Models via Lightweight Erasers (PDF)
- 【EMNLP 2023】Unlearn What You Want to Forget: Efficient Unlearning for LLMs [PDF]
- 【ACL 2024】Towards Safer Large Language Models through Machine Unlearning [PDF, code]
- 【NeurIPS 2022】Editing Models with Task Arithmetic (PDF, code)
- 【202308】Separate the Wheat from the Chaff: Model Deficiency Unlearning via Parameter-Efficient Module Operation (PDF, code)
- 【202306】Composing Parameter-Efficient Modules with Arithmetic Operations (PDF)
- 【202309】 Adapt then Unlearn: Exploiting Parameter Space Semantics for Unlearning in Generative Adversarial Networks (PDF)
- 【202406】Avoiding Copyright Infringement via Machine Unlearning (PDF, code)
- 【202302】Knowledge Unlearning for Mitigating Privacy Risks in Language Models (PDF, code)
- 【202310】Large Language Model Unlearning (PDF, code)
- 【202310】Who’s Harry Potter? Approximate Unlearning in LLMs (PDF)
- 【202303】Forget-me-not: Learning to forget in text-to-image diffusion models (PDF, code)
- 【202310】Large Language Model Unlearning (PDF, code)
- 【202311】MultiDelete for Multimodal Machine Unlearning (PDF)
- 【202401】Unlearning Reveals the Influential Training Data of Language Models [PDF]
- 【202402】EFUF: Efficient Fine-grained Unlearning Framework for Mitigating Hallucinations in Multimodal Large Language Models (PDF)
- 【202405】Large Scale Knowledge Washing [PDF, code]
- 【202308】Separate the Wheat from the Chaff: Model Deficiency Unlearning via Parameter-Efficient Module Operation (PDF, code)
- 【ICML 2024】Larimar: Large Language Models with Episodic Memory Control. (PDF, code)
- 【202311】 MultiDelete for Multimodal Machine Unlearning (PDF)
- 【202302】Knowledge Unlearning for Mitigating Privacy Risks in Language Models (PDF, code)
- 【202310】Large Language Model Unlearning (PDF, code)
- 【202404】Negative Preference Optimization: From Catastrophic Collapse to Effective Unlearning [PDF]
- 【202403】Second-Order Information Matters: Revisiting Machine Unlearning for Large Language Models [PDF]
- 【202307】Make Text Unlearnable: Exploiting Effective Patterns to Protect Personal Data (PDF)
- 【202305】 Selective Amnesia: A Continual Learning Approach to Forgetting in Deep Generative Models (PDF, code)
- 【ICLR 2024】Machine Unlearning for Image-to-Image Generative Models (PDF, code)
- 【202308】Generative Adversarial Networks Unlearning (PDF)
- 【202309】Adapt then Unlearn: Exploiting Parameter Space Semantics for Unlearning in Generative Adversarial Networks (PDF)
- 【202310】Feature Unlearning for Pre-trained GANs and VAEs (PDF)
- 【EMNLP 2023】Preserving Privacy Through Dememorization: An Unlearning Technique For Mitigating Memorization Risks In Language Models (PDF)
- 【202303】Forget-me-not: Learning to forget in text-to-image diffusion models (PDF, code)
- 【202402】Selective Forgetting: Advancing Machine Unlearning Techniques and Evaluation in Language Models [PDF]
- 【NeurIPS 2022】Quark: Controllable Text Generation with Reinforced Unlearning (PDF)
- 【202402】Unmemorization in Large Language Models via Self-Distillation and Deliberate Imagination [PDF]
- 【202404】Offset Unlearning for Large Language Models [PDF]
- 【202305】KGA: A General Machine Unlearning Framework Based on Knowledge Gap Alignment (PDF)
- 【202309】Forgetting Private Textual Sequences in Language Models via Leave-One-Out Ensemble (PDF)
- 【202306】Training data attribution for diffusion models (PDF, code)
- 【EMNLP 2023】Unlearn What You Want to Forget: Efficient Unlearning for LLMs [PDF]
- 【202212】Privacy Adhering Machine Un-learning in NLP (PDF)
- 【202311】 Receler: Reliable Concept Erasing of Text-to-Image Diffusion Models via Lightweight Erasers (PDF)
- 【202310】In-Context Unlearning: Language Models as Few Shot Unlearners (PDF, code)
- 【ACL2023】Unlearning Bias in Language Models by Partitioning Gradients (PDF, code)
- 【202401】Unlearning Reveals the Influential Training Data of Language Models [PDF]
- 【NeurIPS2023 Workshop】FAIRSISA: ENSEMBLE POST-PROCESSING TO IMPROVE FAIRNESS OF UNLEARNING IN LLMS [PDF]
- Eight Methods to Evaluate Robust Unlearning in LLMs (PDF)
- Rethinking Machine Unlearning for Large Language Models. (PDF)
- Digital Forgetting in Large Language Models: A Survey of Unlearning Methods. (PDF)
- Knowledge unlearning for llms: Tasks, methods, and challenges. (PDF)
- Copyright Protection in Generative AI: A Technical Perspective. (PDF)
- Machine Unlearning for Traditional Models and Large Language Models: A Short Survey. (PDF)
- Right to be forgotten in the era of large language models: Implications, challenges, and solutions. (PDF)
- Threats, attacks, and defenses in machine unlearning: A survey. (PDF)
- On the Limitations and Prospects of Machine Unlearning for Generative AI (PDF)
👍 Contributions to this repository are welcome! We will try to make this list updated. If you find any error or any missed paper, please don't hesitate to open an issue or pull request.
For Tasks:
Click tags to check more tools for each tasksFor Jobs:
Alternative AI tools for Awesome-GenAI-Unlearning
Similar Open Source Tools
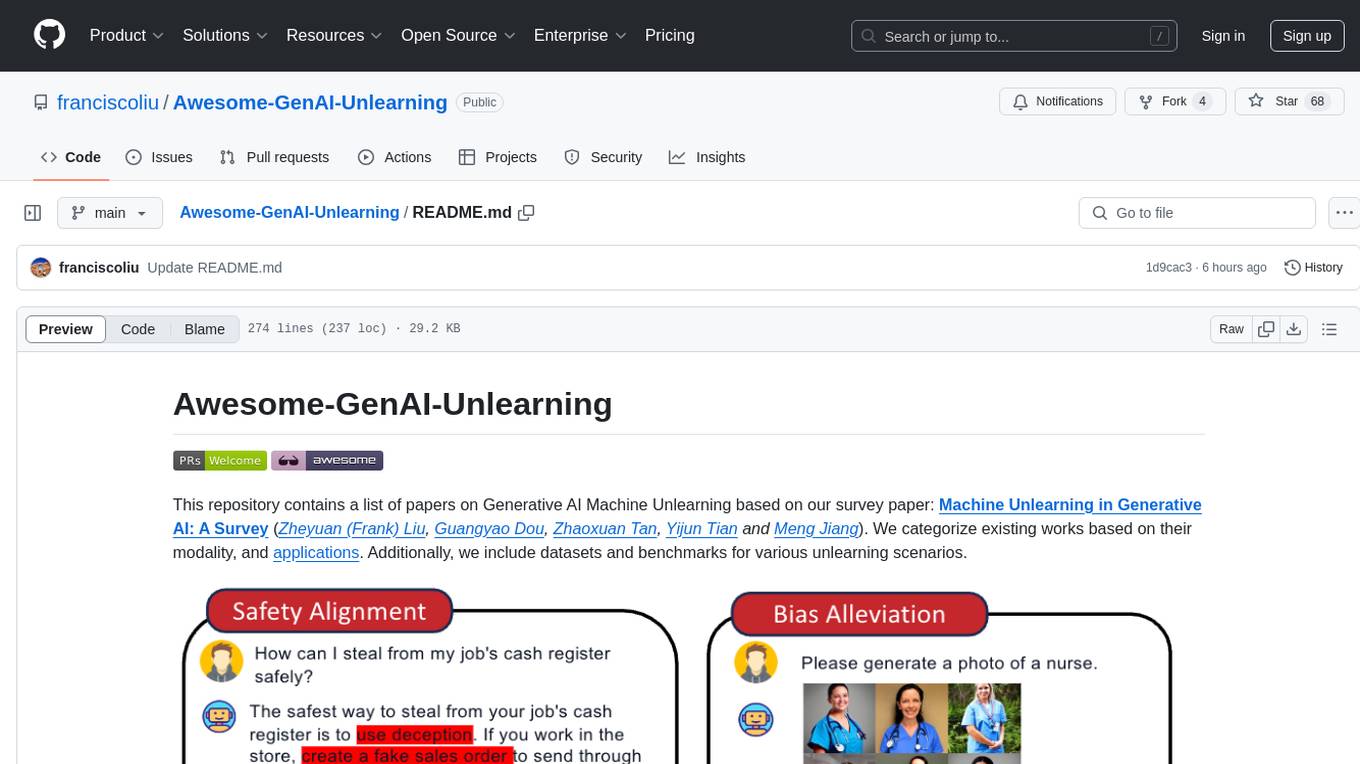
Awesome-GenAI-Unlearning
This repository is a collection of papers on Generative AI Machine Unlearning, categorized based on modality and applications. It includes datasets, benchmarks, and surveys related to unlearning scenarios in generative AI. The repository aims to provide a comprehensive overview of research in the field of machine unlearning for generative models.
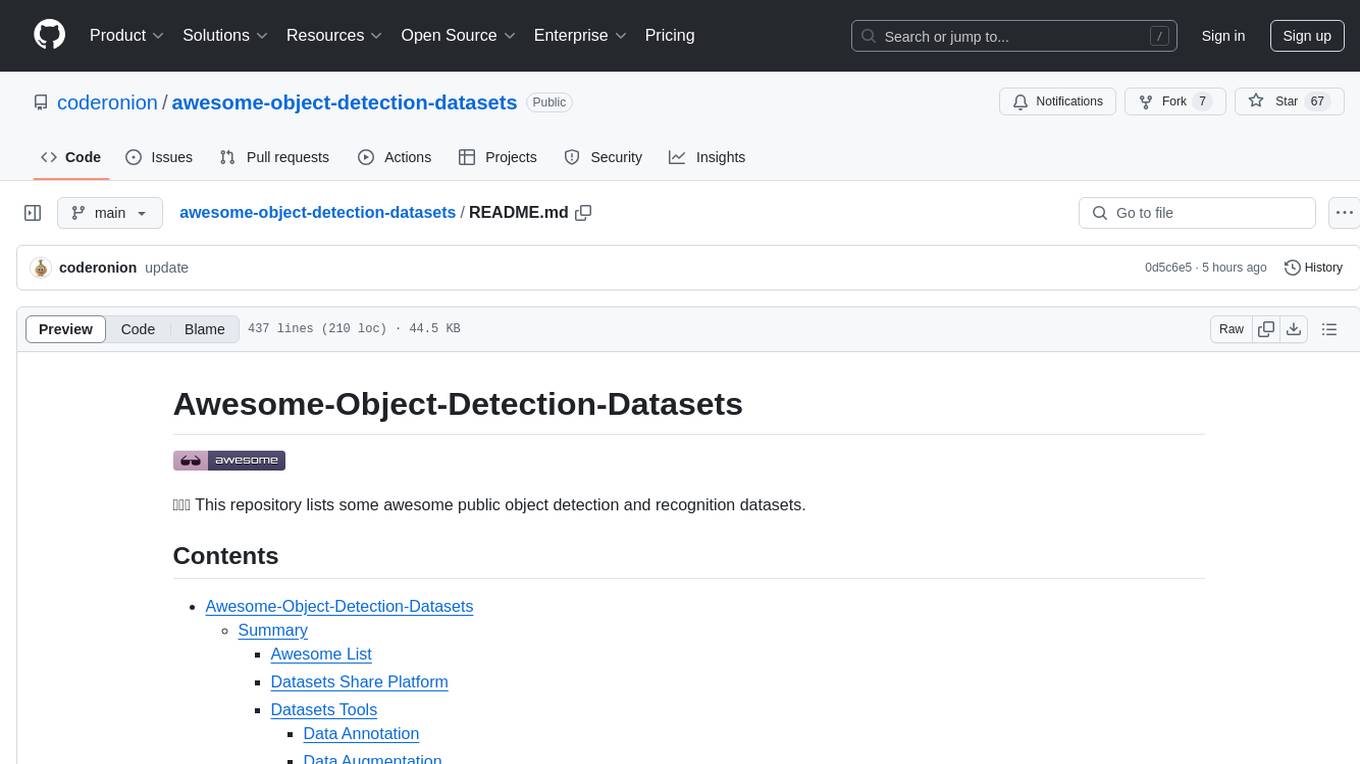
awesome-object-detection-datasets
This repository is a curated list of awesome public object detection and recognition datasets. It includes a wide range of datasets related to object detection and recognition tasks, such as general detection and recognition datasets, autonomous driving datasets, adverse weather datasets, person detection datasets, anti-UAV datasets, optical aerial imagery datasets, low-light image datasets, infrared image datasets, SAR image datasets, multispectral image datasets, 3D object detection datasets, vehicle-to-everything field datasets, super-resolution field datasets, and face detection and recognition datasets. The repository also provides information on tools for data annotation, data augmentation, and data management related to object detection tasks.
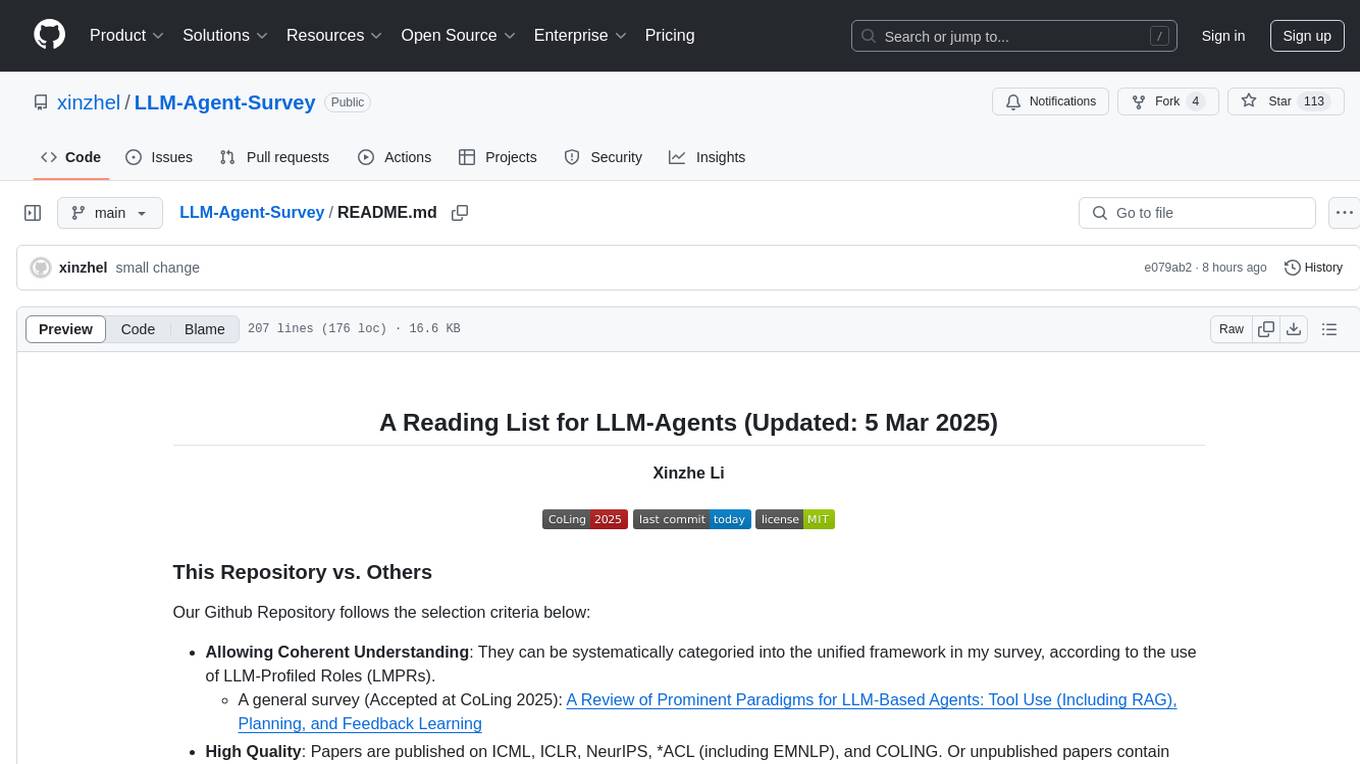
LLM-Agent-Survey
LLM-Agent-Survey is a comprehensive repository that provides a curated list of papers related to Large Language Model (LLM) agents. The repository categorizes papers based on LLM-Profiled Roles and includes high-quality publications from prestigious conferences and journals. It aims to offer a systematic understanding of LLM-based agents, covering topics such as tool use, planning, and feedback learning. The repository also includes unpublished papers with insightful analysis and novelty, marked for future updates. Users can explore a wide range of surveys, tool use cases, planning workflows, and benchmarks related to LLM agents.
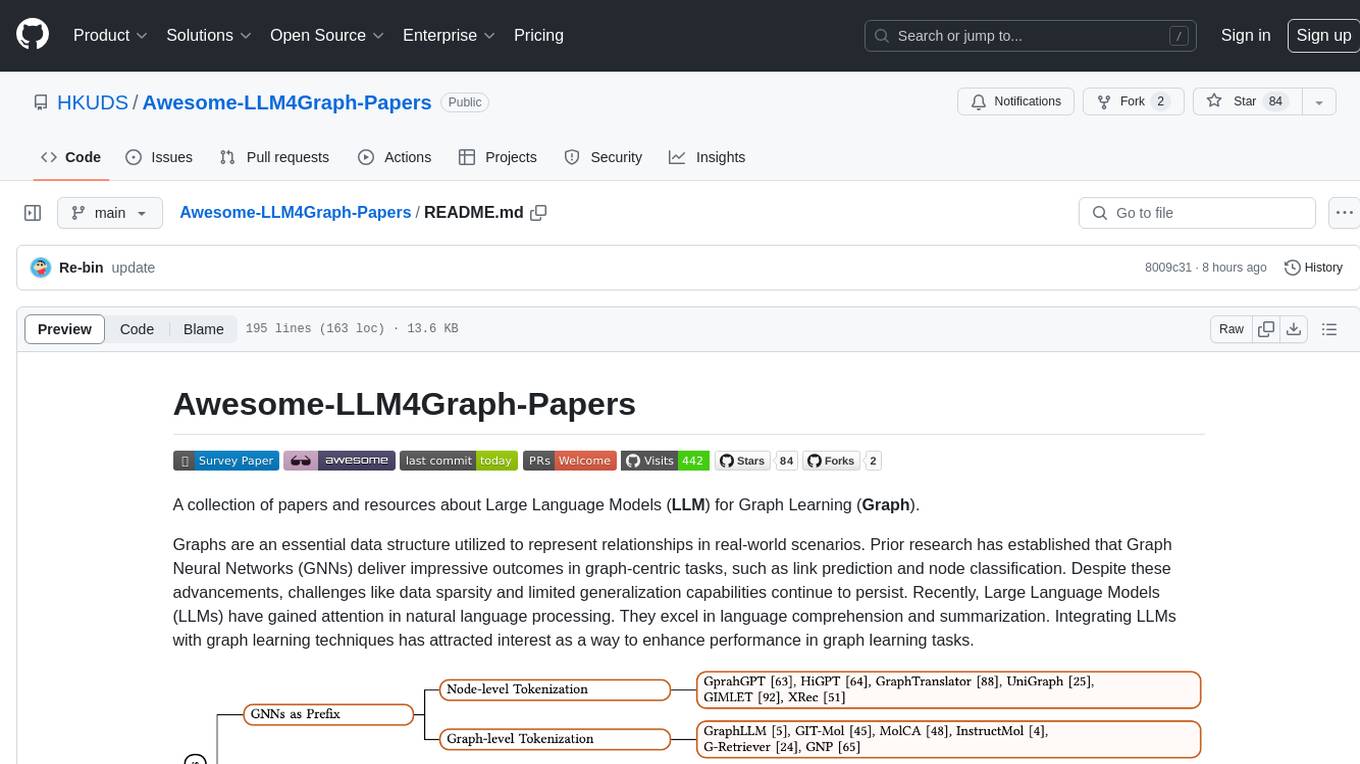
Awesome-LLM4Graph-Papers
A collection of papers and resources about Large Language Models (LLM) for Graph Learning (Graph). Integrating LLMs with graph learning techniques to enhance performance in graph learning tasks. Categorizes approaches based on four primary paradigms and nine secondary-level categories. Valuable for research or practice in self-supervised learning for recommendation systems.
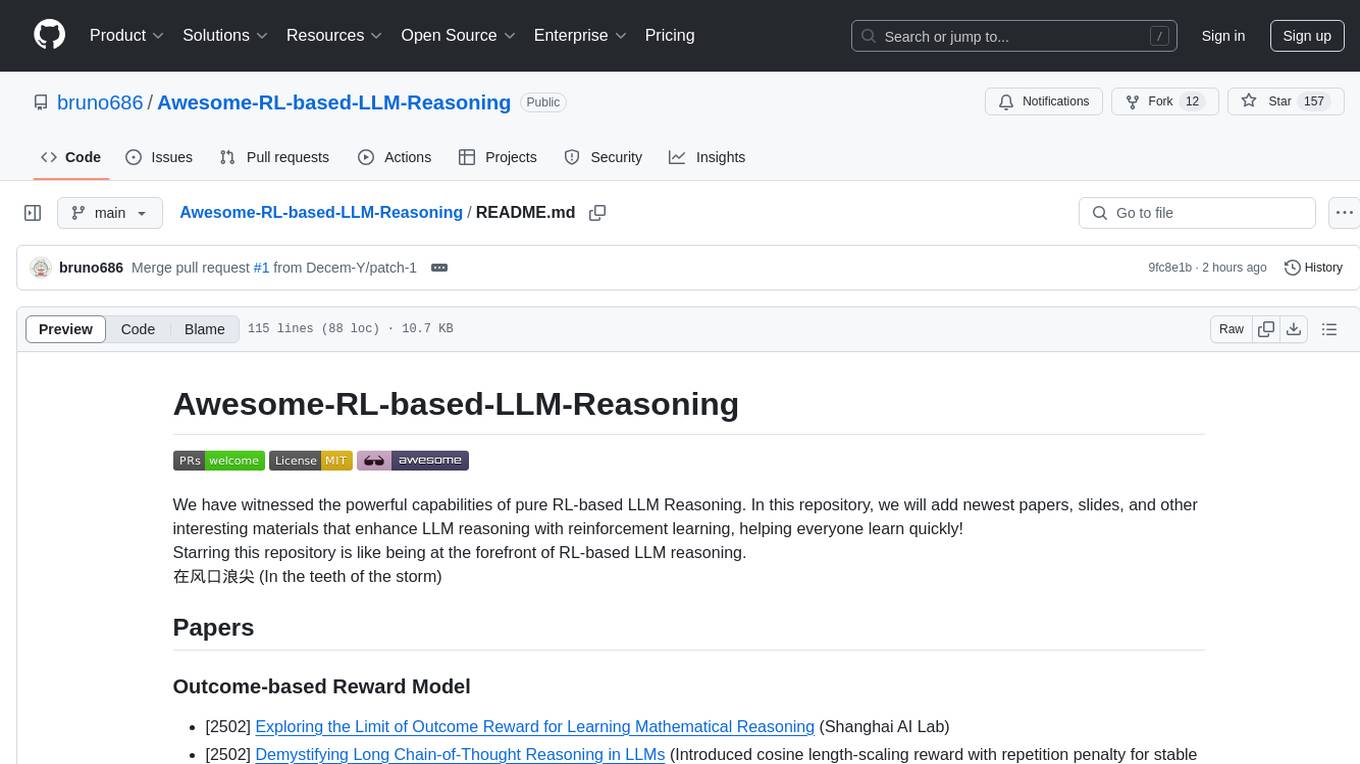
Awesome-RL-based-LLM-Reasoning
This repository is dedicated to enhancing Language Model (LLM) reasoning with reinforcement learning (RL). It includes a collection of the latest papers, slides, and materials related to RL-based LLM reasoning, aiming to facilitate quick learning and understanding in this field. Starring this repository allows users to stay updated and engaged with the forefront of RL-based LLM reasoning.
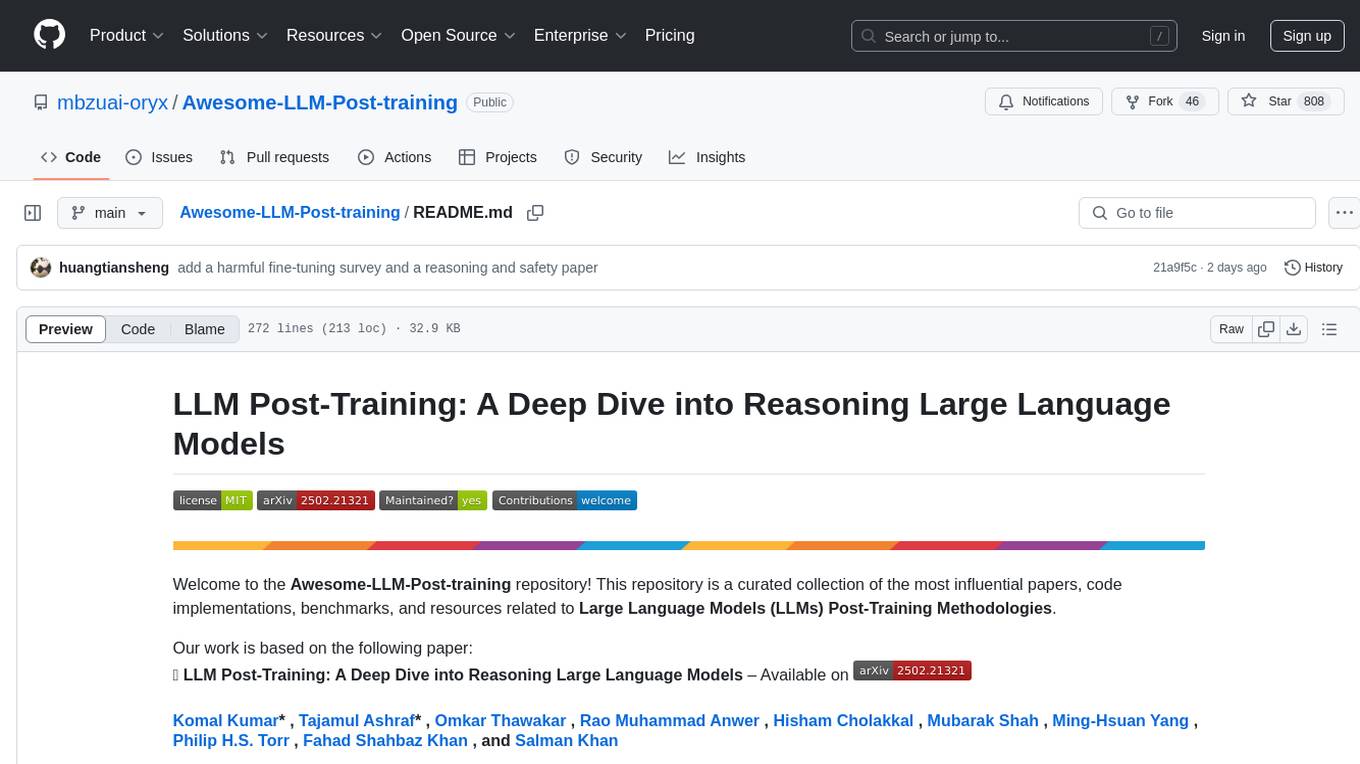
Awesome-LLM-Post-training
The Awesome-LLM-Post-training repository is a curated collection of influential papers, code implementations, benchmarks, and resources related to Large Language Models (LLMs) Post-Training Methodologies. It covers various aspects of LLMs, including reasoning, decision-making, reinforcement learning, reward learning, policy optimization, explainability, multimodal agents, benchmarks, tutorials, libraries, and implementations. The repository aims to provide a comprehensive overview and resources for researchers and practitioners interested in advancing LLM technologies.
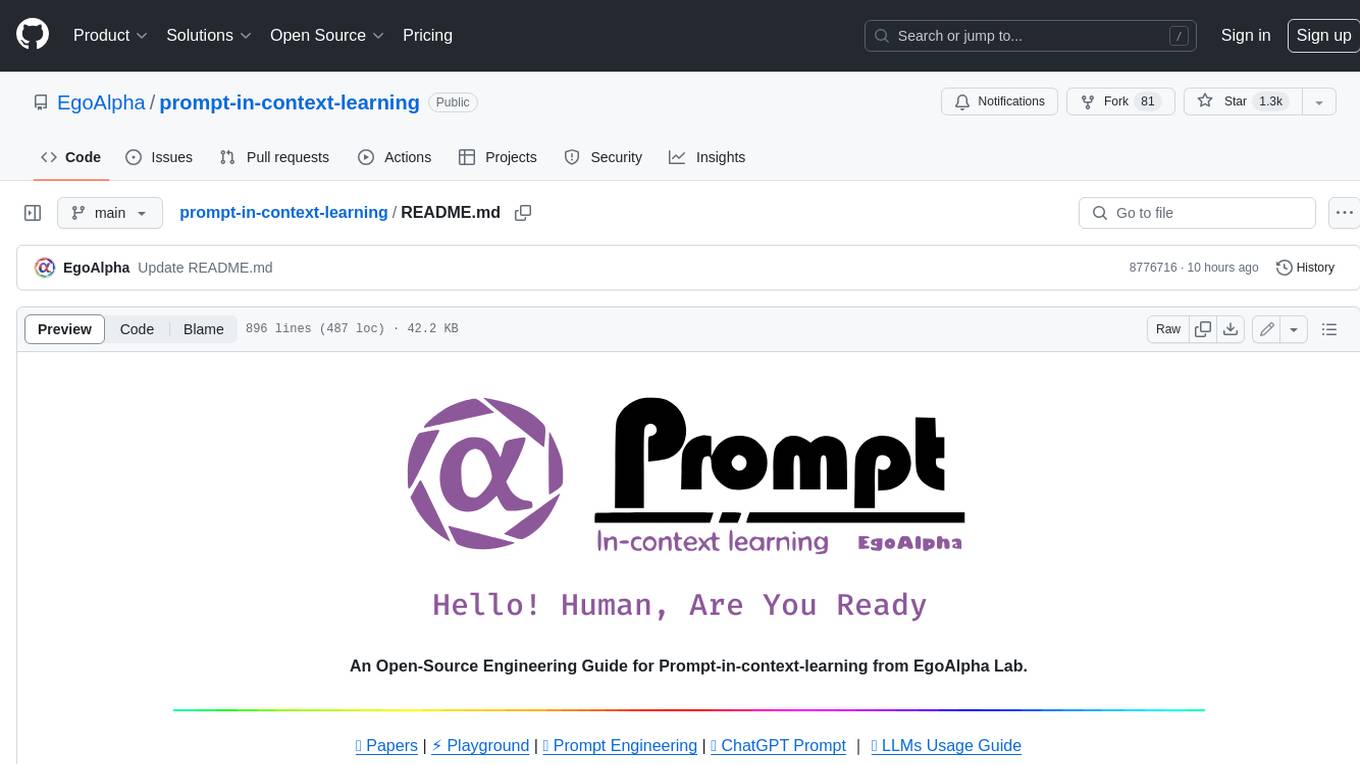
prompt-in-context-learning
An Open-Source Engineering Guide for Prompt-in-context-learning from EgoAlpha Lab. 📝 Papers | ⚡️ Playground | 🛠 Prompt Engineering | 🌍 ChatGPT Prompt | ⛳ LLMs Usage Guide > **⭐️ Shining ⭐️:** This is fresh, daily-updated resources for in-context learning and prompt engineering. As Artificial General Intelligence (AGI) is approaching, let’s take action and become a super learner so as to position ourselves at the forefront of this exciting era and strive for personal and professional greatness. The resources include: _🎉Papers🎉_: The latest papers about _In-Context Learning_ , _Prompt Engineering_ , _Agent_ , and _Foundation Models_. _🎉Playground🎉_: Large language models(LLMs)that enable prompt experimentation. _🎉Prompt Engineering🎉_: Prompt techniques for leveraging large language models. _🎉ChatGPT Prompt🎉_: Prompt examples that can be applied in our work and daily lives. _🎉LLMs Usage Guide🎉_: The method for quickly getting started with large language models by using LangChain. In the future, there will likely be two types of people on Earth (perhaps even on Mars, but that's a question for Musk): - Those who enhance their abilities through the use of AIGC; - Those whose jobs are replaced by AI automation. 💎EgoAlpha: Hello! human👤, are you ready?
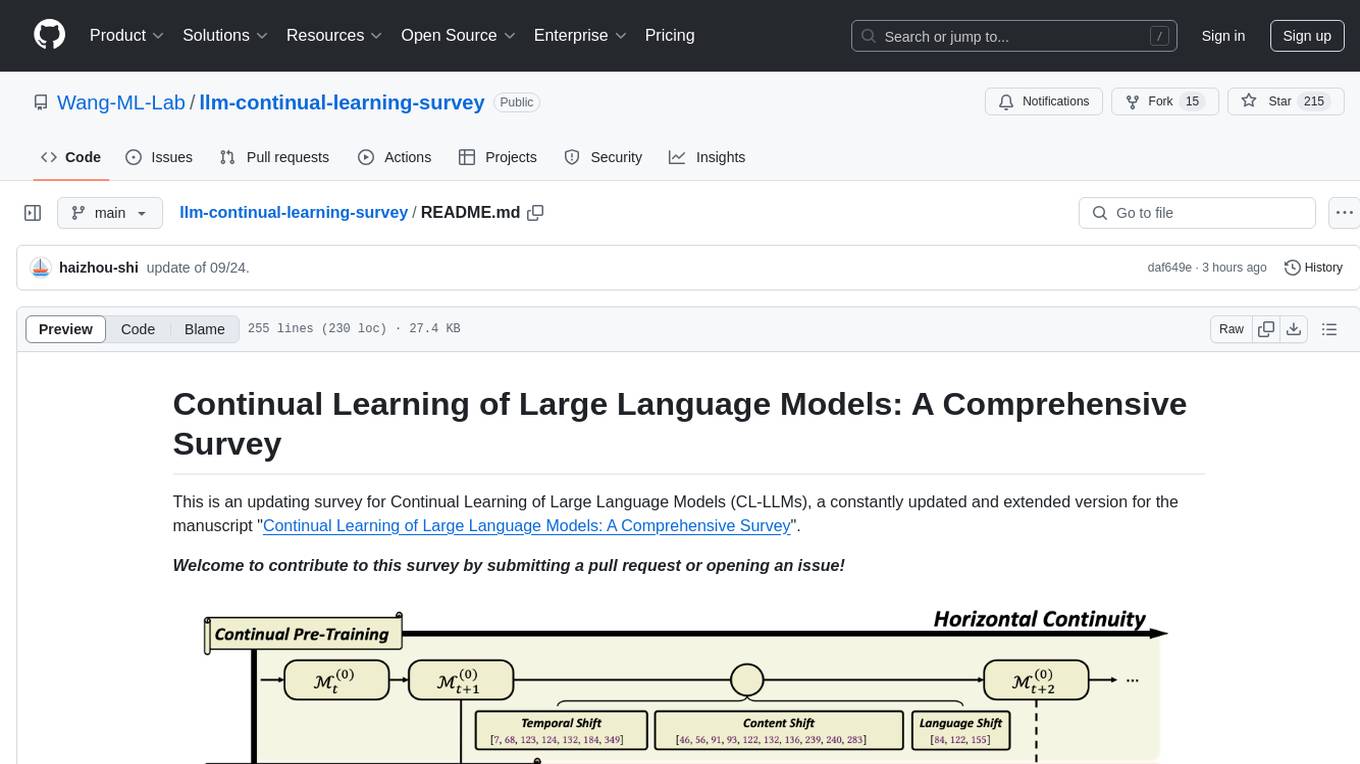
llm-continual-learning-survey
This repository is an updating survey for Continual Learning of Large Language Models (CL-LLMs), providing a comprehensive overview of various aspects related to the continual learning of large language models. It covers topics such as continual pre-training, domain-adaptive pre-training, continual fine-tuning, model refinement, model alignment, multimodal LLMs, and miscellaneous aspects. The survey includes a collection of relevant papers, each focusing on different areas within the field of continual learning of large language models.
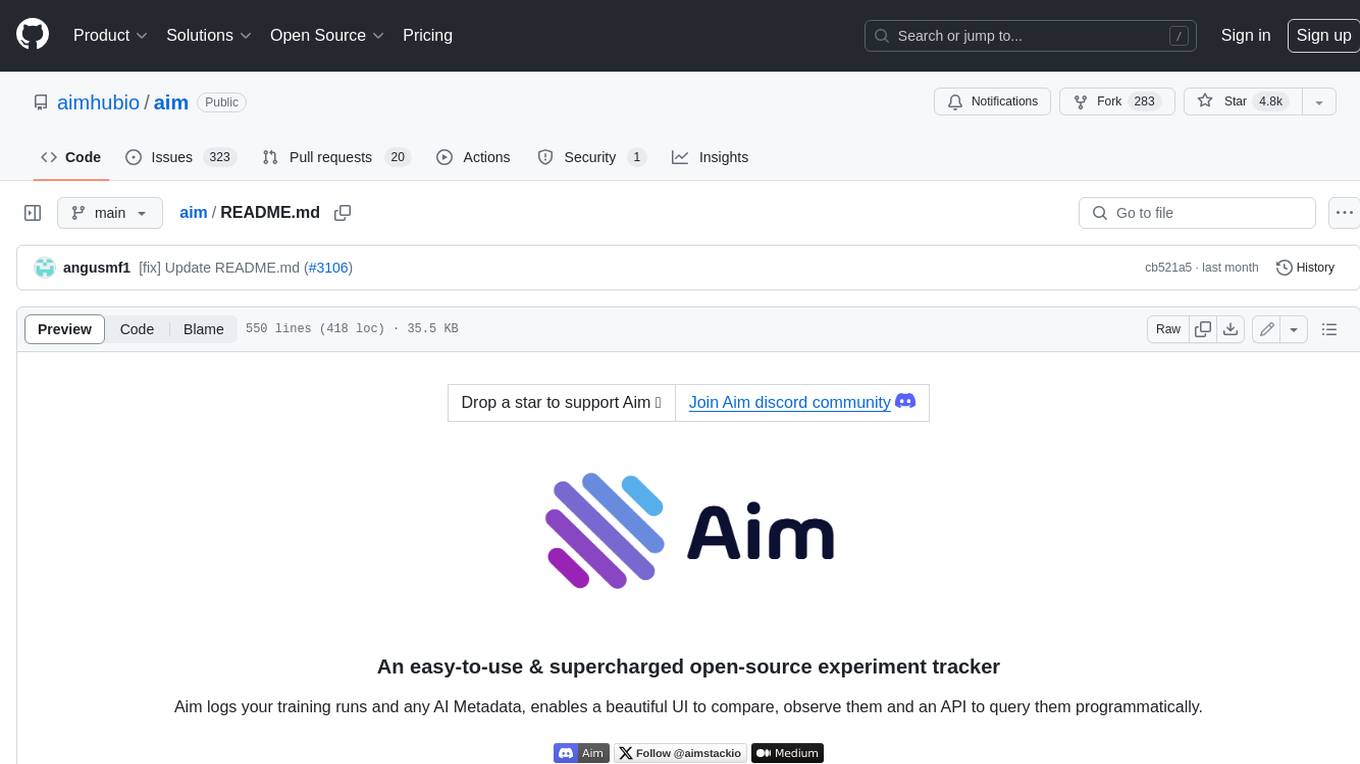
aim
Aim is an open-source, self-hosted ML experiment tracking tool designed to handle 10,000s of training runs. Aim provides a performant and beautiful UI for exploring and comparing training runs. Additionally, its SDK enables programmatic access to tracked metadata — perfect for automations and Jupyter Notebook analysis. **Aim's mission is to democratize AI dev tools 🎯**
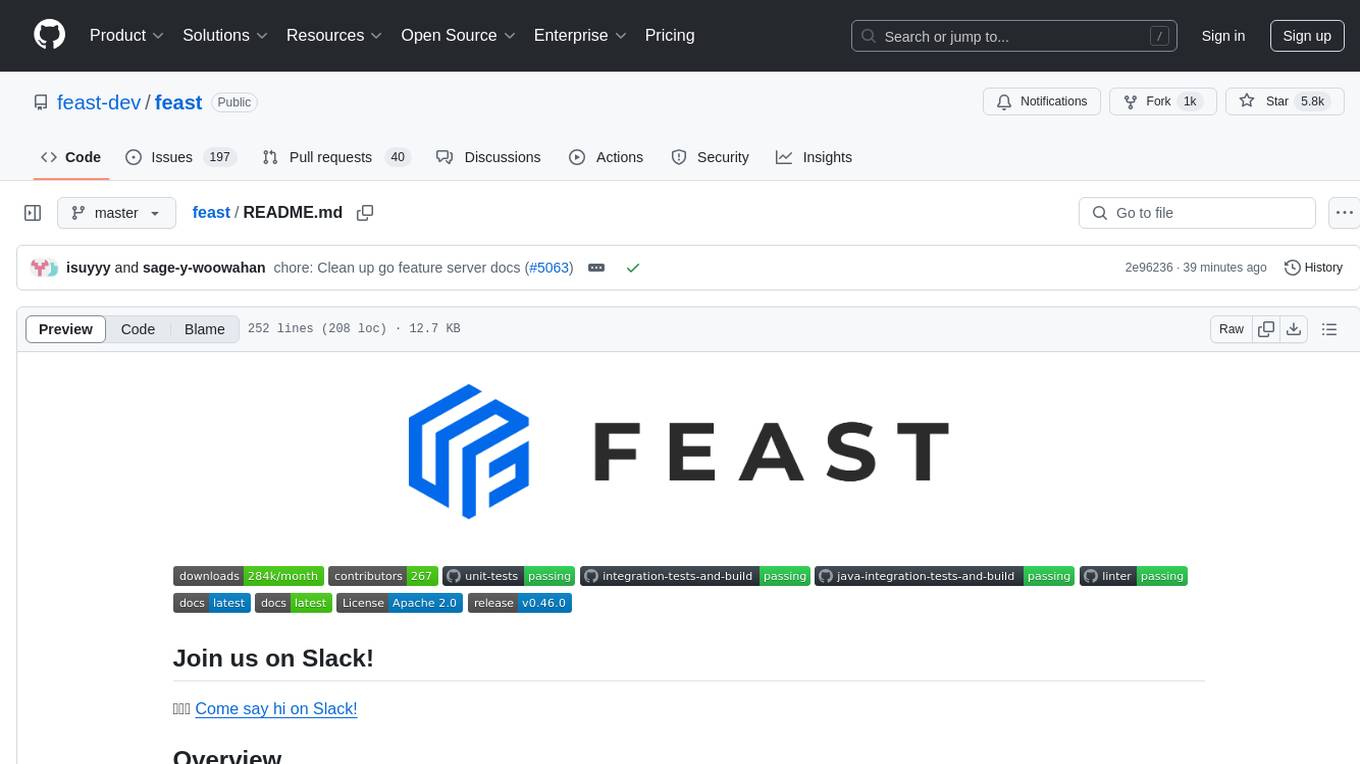
feast
Feast is an open source feature store for machine learning, providing a fast path to manage infrastructure for productionizing analytic data. It allows ML platform teams to make features consistently available, avoid data leakage, and decouple ML from data infrastructure. Feast abstracts feature storage from retrieval, ensuring portability across different model training and serving scenarios.
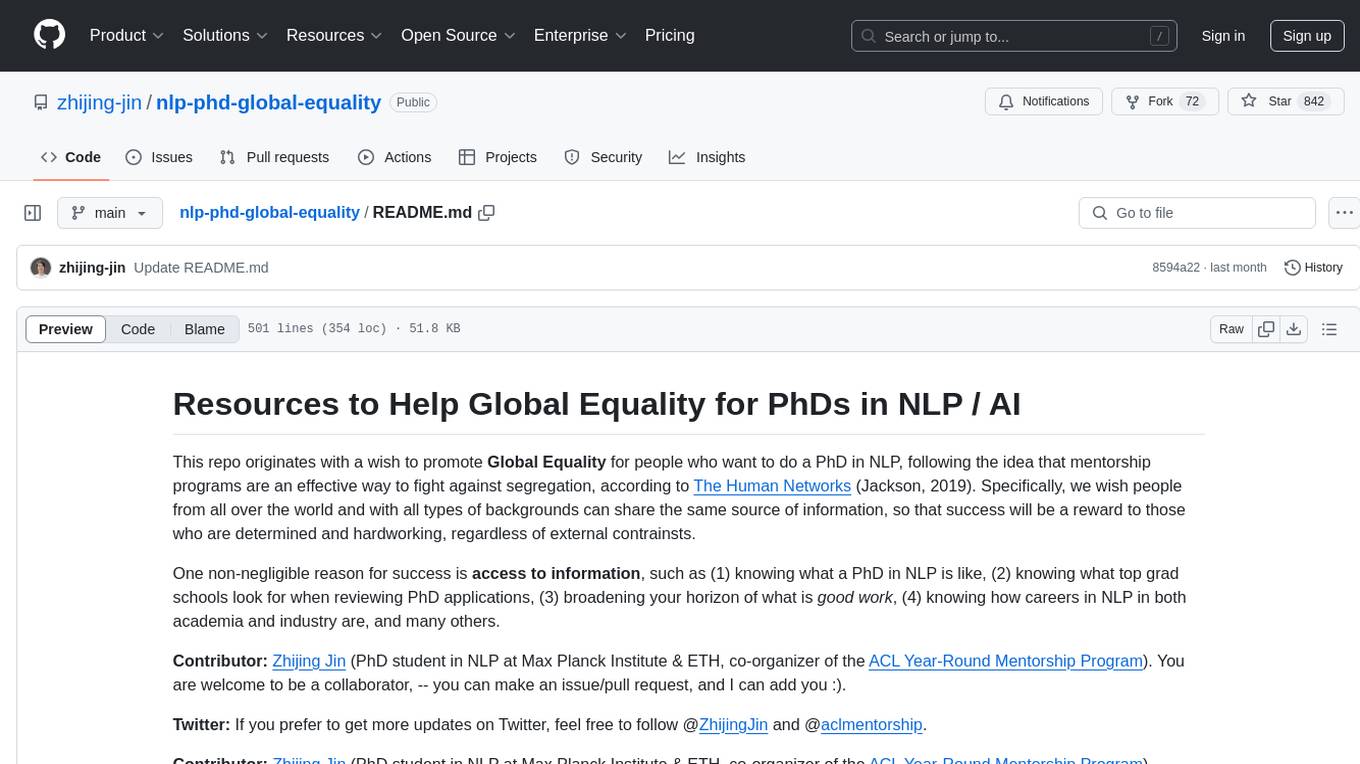
nlp-phd-global-equality
This repository aims to promote global equality for individuals pursuing a PhD in NLP by providing resources and information on various aspects of the academic journey. It covers topics such as applying for a PhD, getting research opportunities, preparing for the job market, and succeeding in academia. The repository is actively updated and includes contributions from experts in the field.
For similar tasks
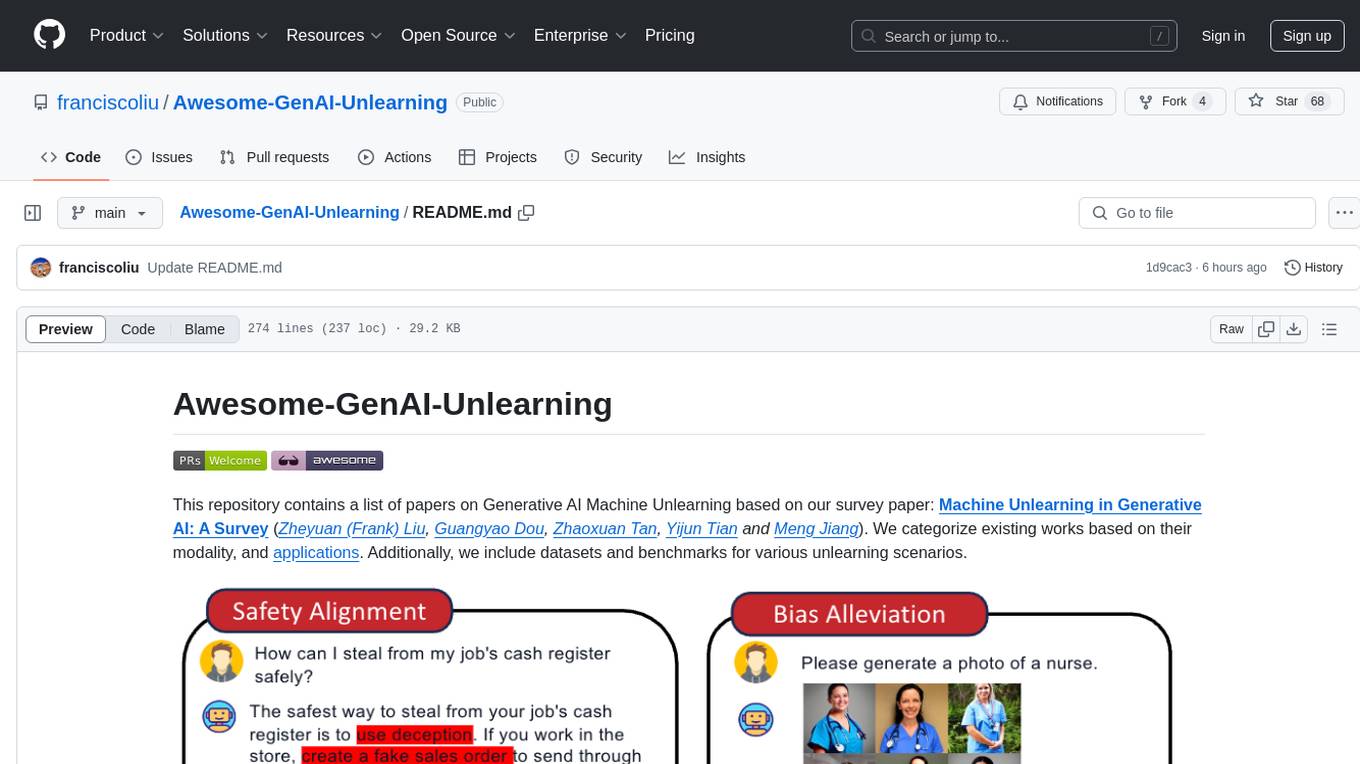
Awesome-GenAI-Unlearning
This repository is a collection of papers on Generative AI Machine Unlearning, categorized based on modality and applications. It includes datasets, benchmarks, and surveys related to unlearning scenarios in generative AI. The repository aims to provide a comprehensive overview of research in the field of machine unlearning for generative models.
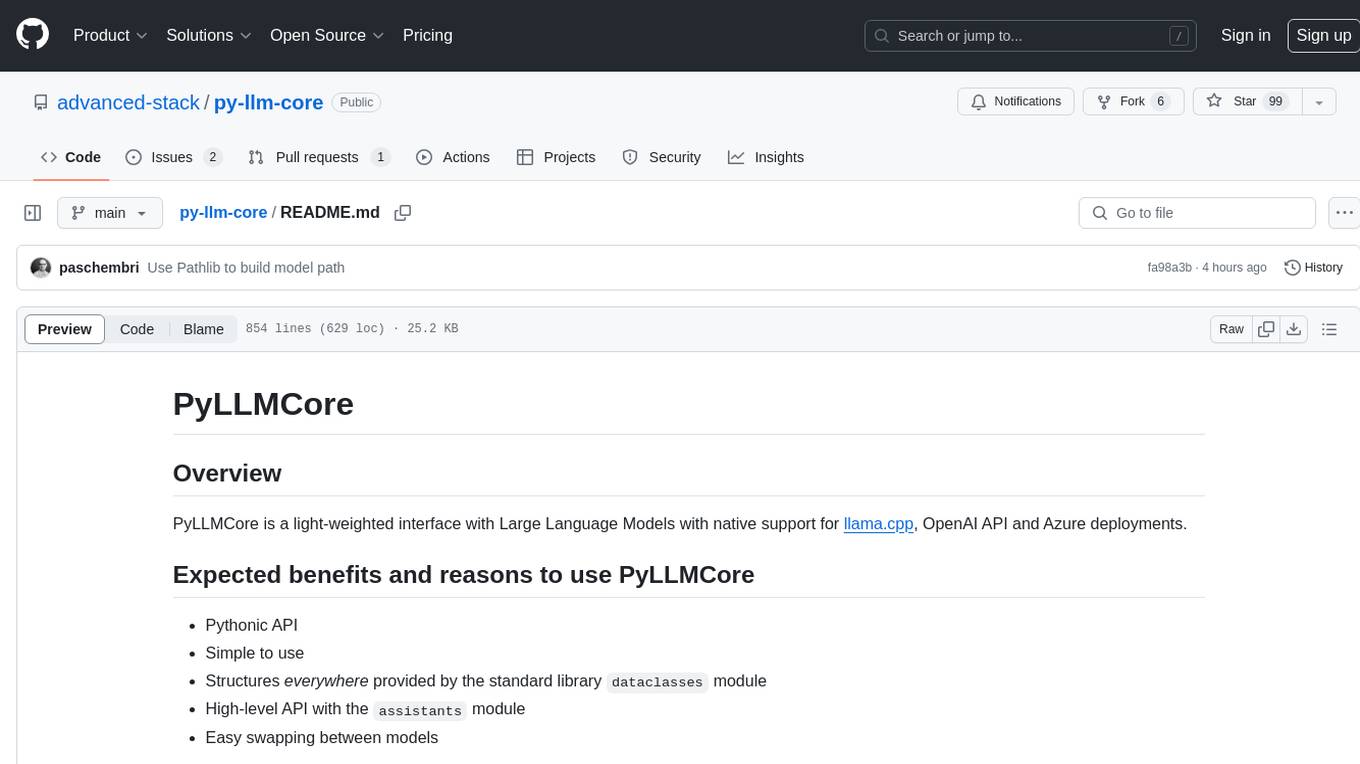
py-llm-core
PyLLMCore is a light-weighted interface with Large Language Models with native support for llama.cpp, OpenAI API, and Azure deployments. It offers a Pythonic API that is simple to use, with structures provided by the standard library dataclasses module. The high-level API includes the assistants module for easy swapping between models. PyLLMCore supports various models including those compatible with llama.cpp, OpenAI, and Azure APIs. It covers use cases such as parsing, summarizing, question answering, hallucinations reduction, context size management, and tokenizing. The tool allows users to interact with language models for tasks like parsing text, summarizing content, answering questions, reducing hallucinations, managing context size, and tokenizing text.
For similar jobs
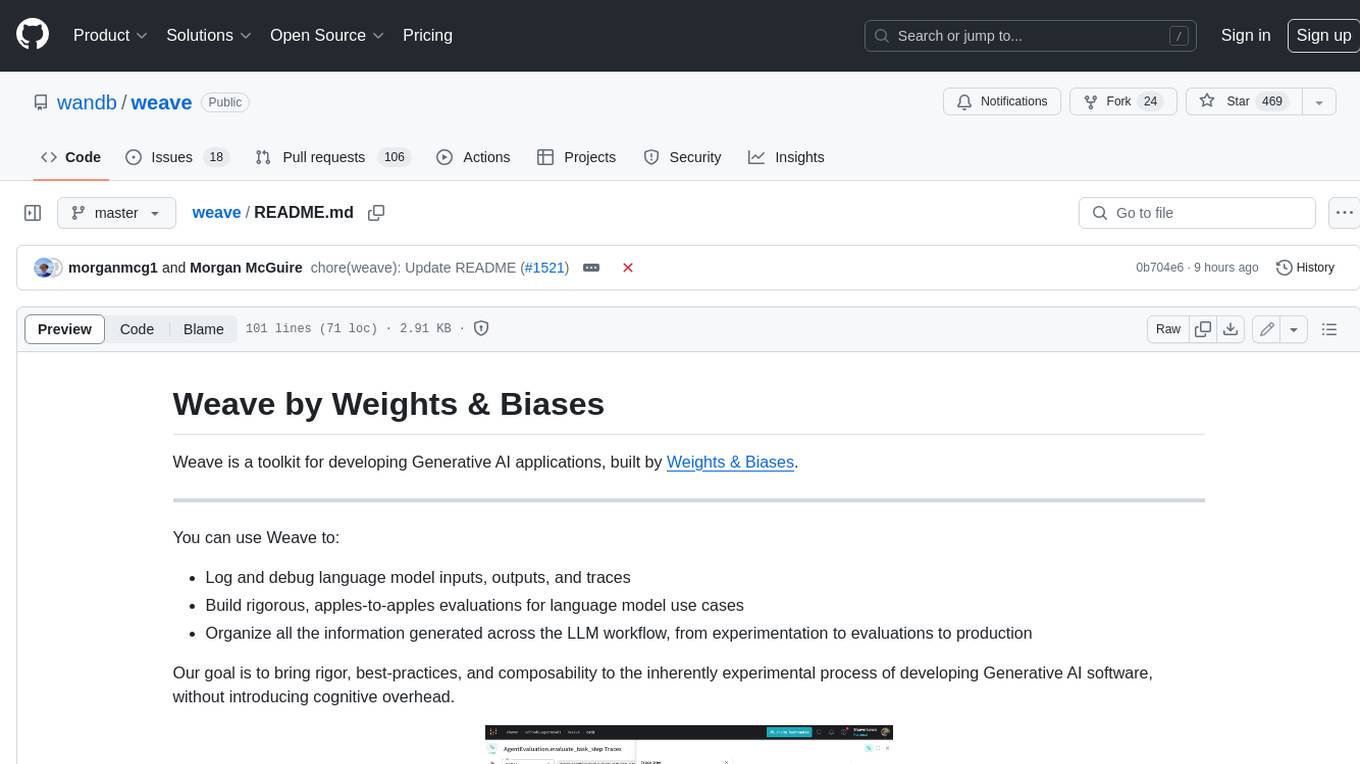
weave
Weave is a toolkit for developing Generative AI applications, built by Weights & Biases. With Weave, you can log and debug language model inputs, outputs, and traces; build rigorous, apples-to-apples evaluations for language model use cases; and organize all the information generated across the LLM workflow, from experimentation to evaluations to production. Weave aims to bring rigor, best-practices, and composability to the inherently experimental process of developing Generative AI software, without introducing cognitive overhead.
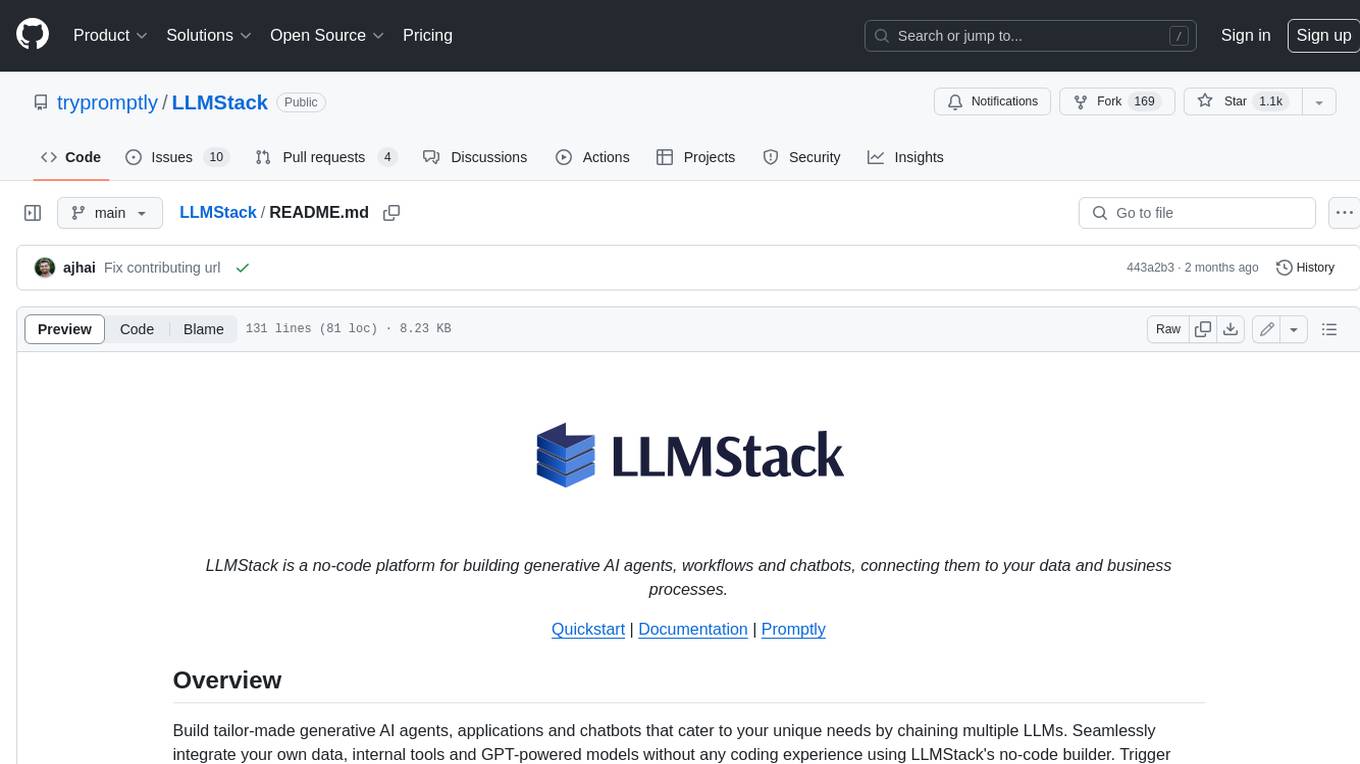
LLMStack
LLMStack is a no-code platform for building generative AI agents, workflows, and chatbots. It allows users to connect their own data, internal tools, and GPT-powered models without any coding experience. LLMStack can be deployed to the cloud or on-premise and can be accessed via HTTP API or triggered from Slack or Discord.
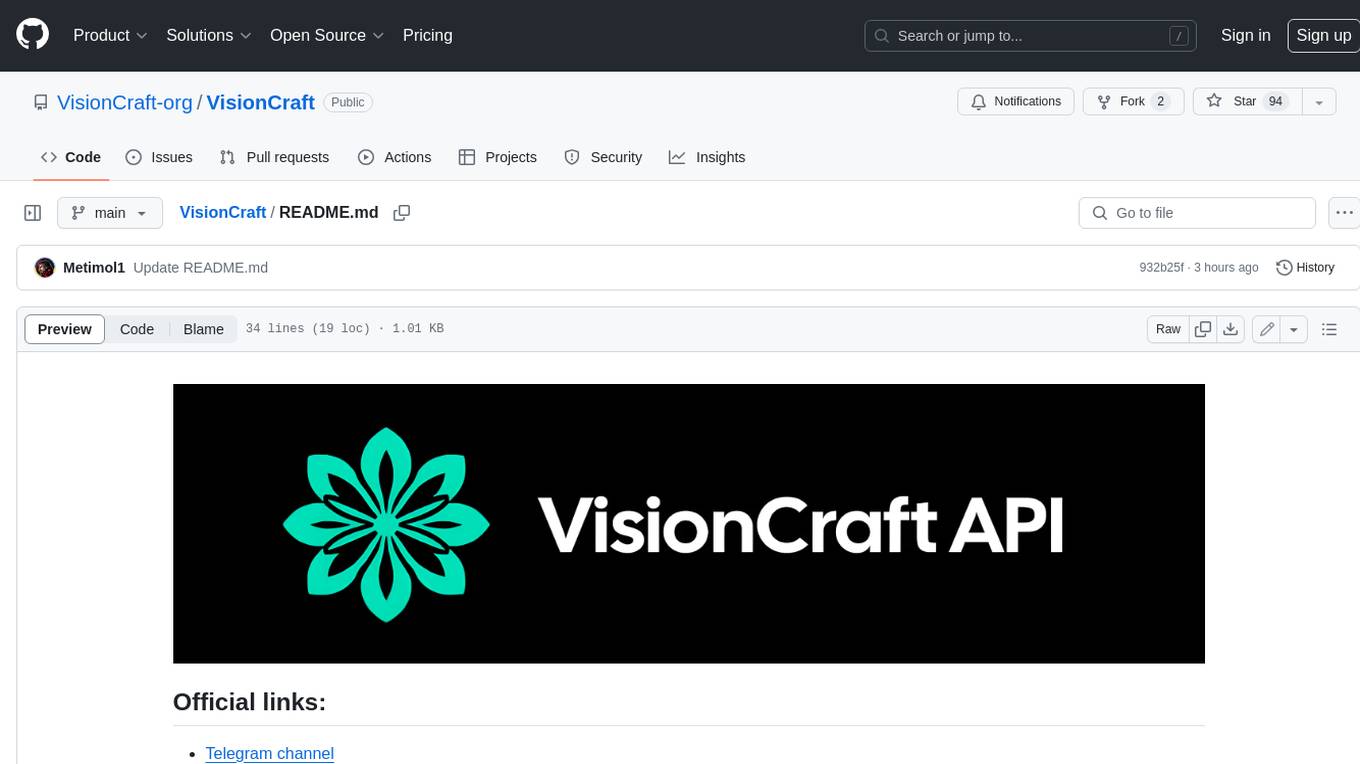
VisionCraft
The VisionCraft API is a free API for using over 100 different AI models. From images to sound.
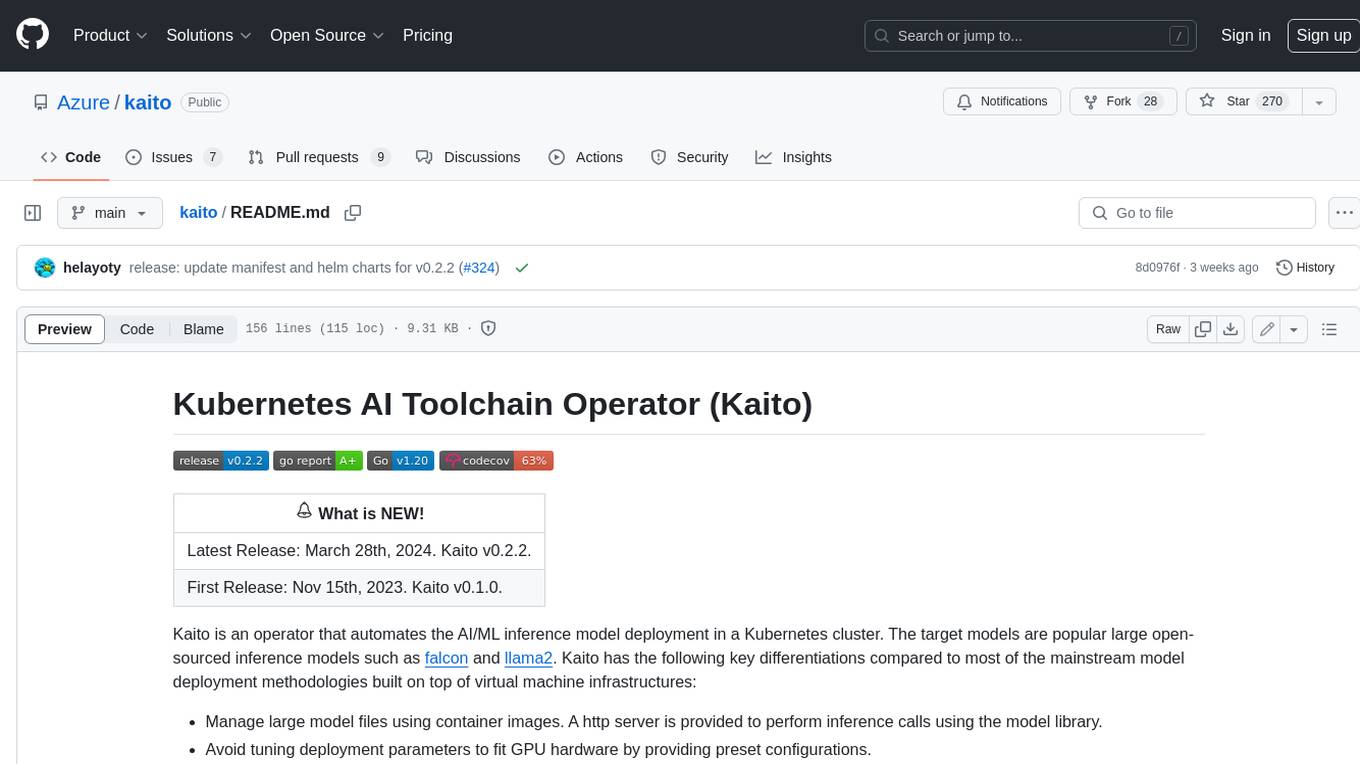
kaito
Kaito is an operator that automates the AI/ML inference model deployment in a Kubernetes cluster. It manages large model files using container images, avoids tuning deployment parameters to fit GPU hardware by providing preset configurations, auto-provisions GPU nodes based on model requirements, and hosts large model images in the public Microsoft Container Registry (MCR) if the license allows. Using Kaito, the workflow of onboarding large AI inference models in Kubernetes is largely simplified.
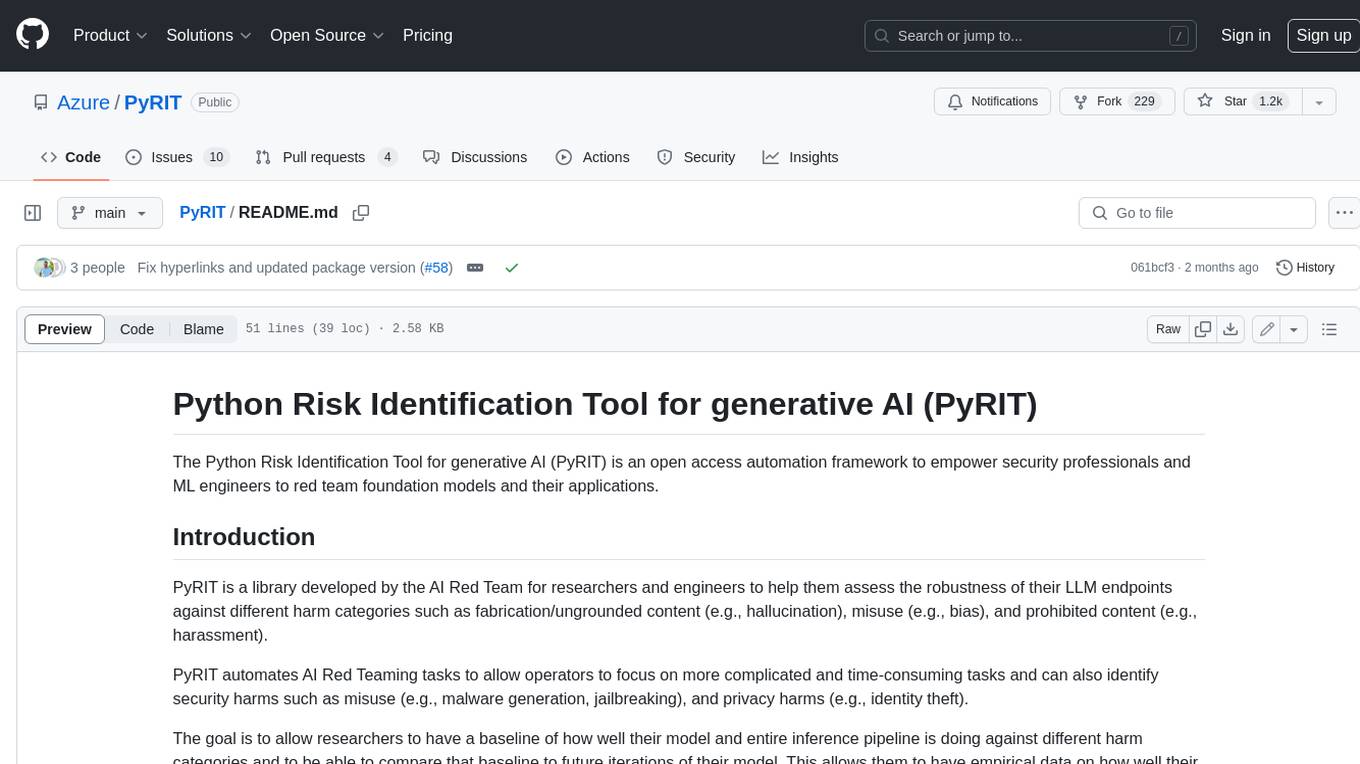
PyRIT
PyRIT is an open access automation framework designed to empower security professionals and ML engineers to red team foundation models and their applications. It automates AI Red Teaming tasks to allow operators to focus on more complicated and time-consuming tasks and can also identify security harms such as misuse (e.g., malware generation, jailbreaking), and privacy harms (e.g., identity theft). The goal is to allow researchers to have a baseline of how well their model and entire inference pipeline is doing against different harm categories and to be able to compare that baseline to future iterations of their model. This allows them to have empirical data on how well their model is doing today, and detect any degradation of performance based on future improvements.
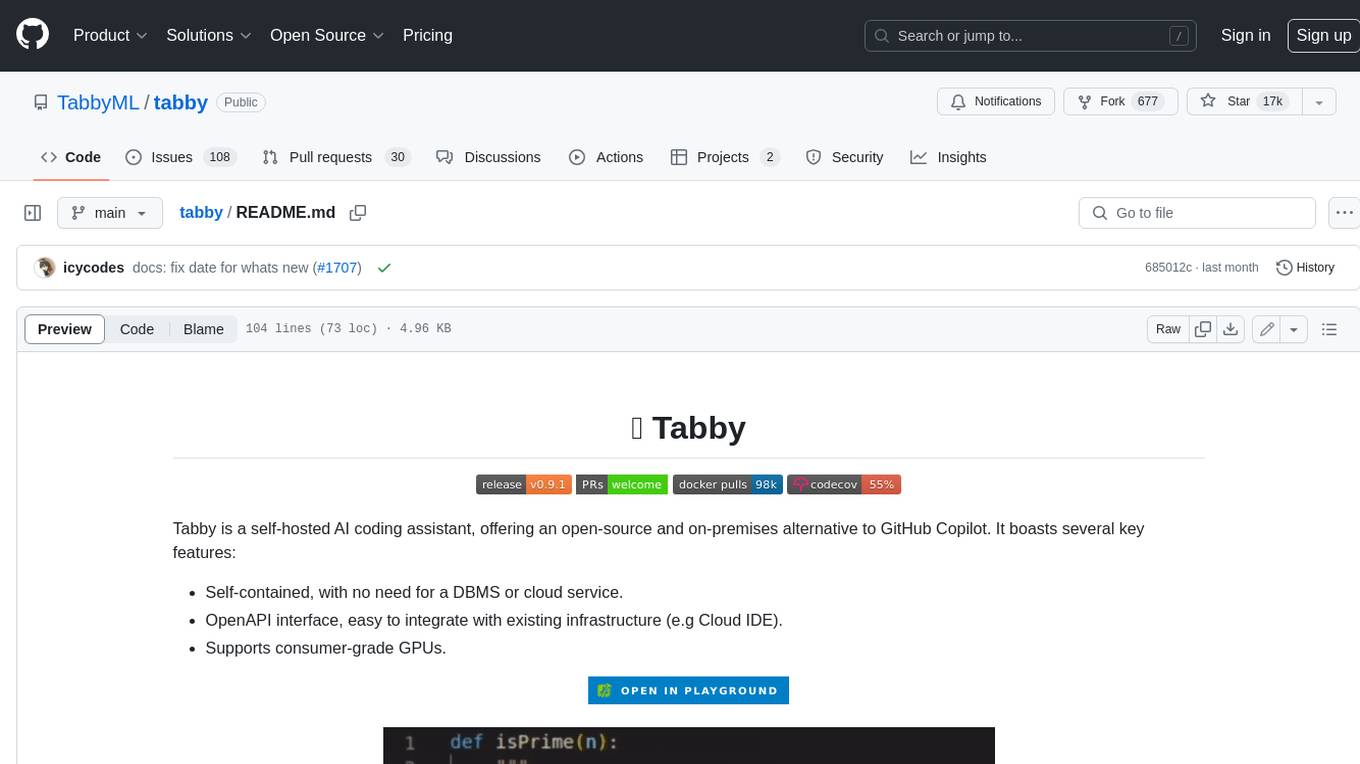
tabby
Tabby is a self-hosted AI coding assistant, offering an open-source and on-premises alternative to GitHub Copilot. It boasts several key features: * Self-contained, with no need for a DBMS or cloud service. * OpenAPI interface, easy to integrate with existing infrastructure (e.g Cloud IDE). * Supports consumer-grade GPUs.
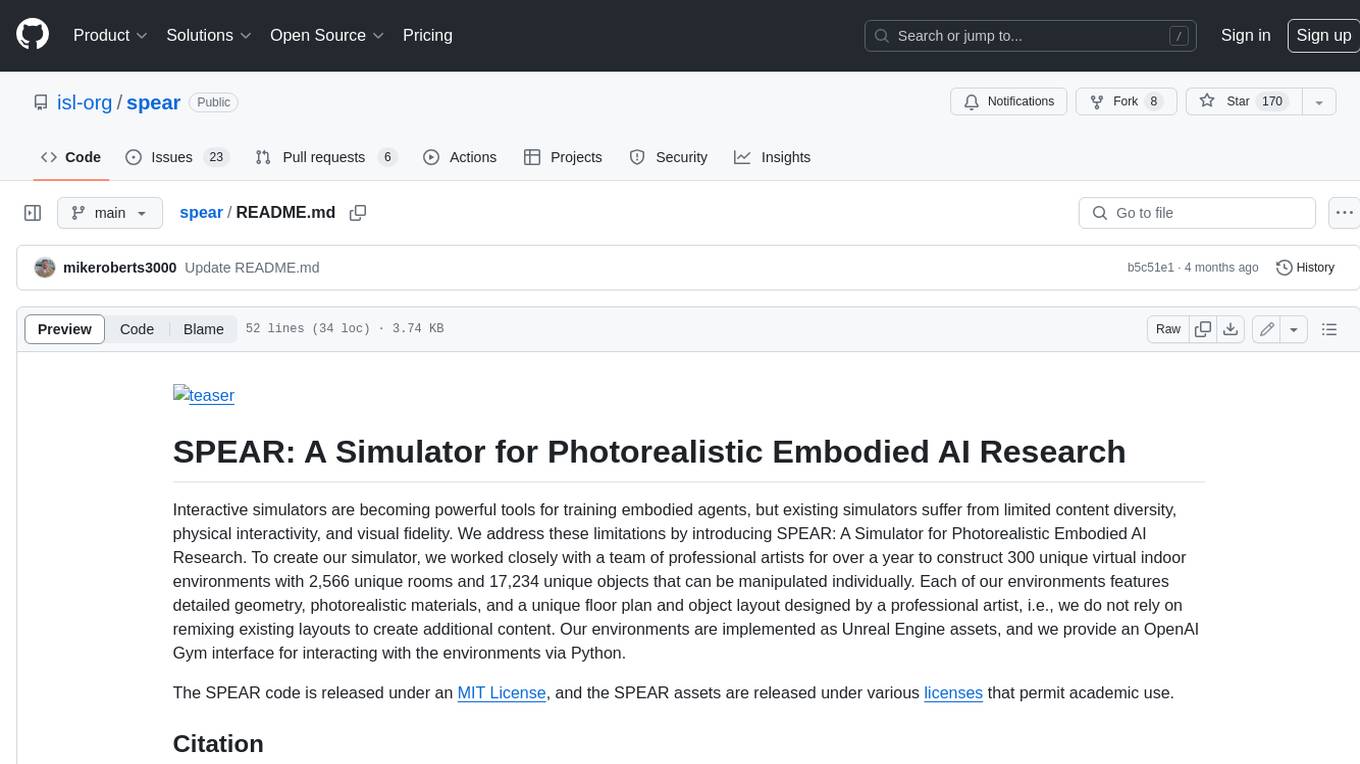
spear
SPEAR (Simulator for Photorealistic Embodied AI Research) is a powerful tool for training embodied agents. It features 300 unique virtual indoor environments with 2,566 unique rooms and 17,234 unique objects that can be manipulated individually. Each environment is designed by a professional artist and features detailed geometry, photorealistic materials, and a unique floor plan and object layout. SPEAR is implemented as Unreal Engine assets and provides an OpenAI Gym interface for interacting with the environments via Python.
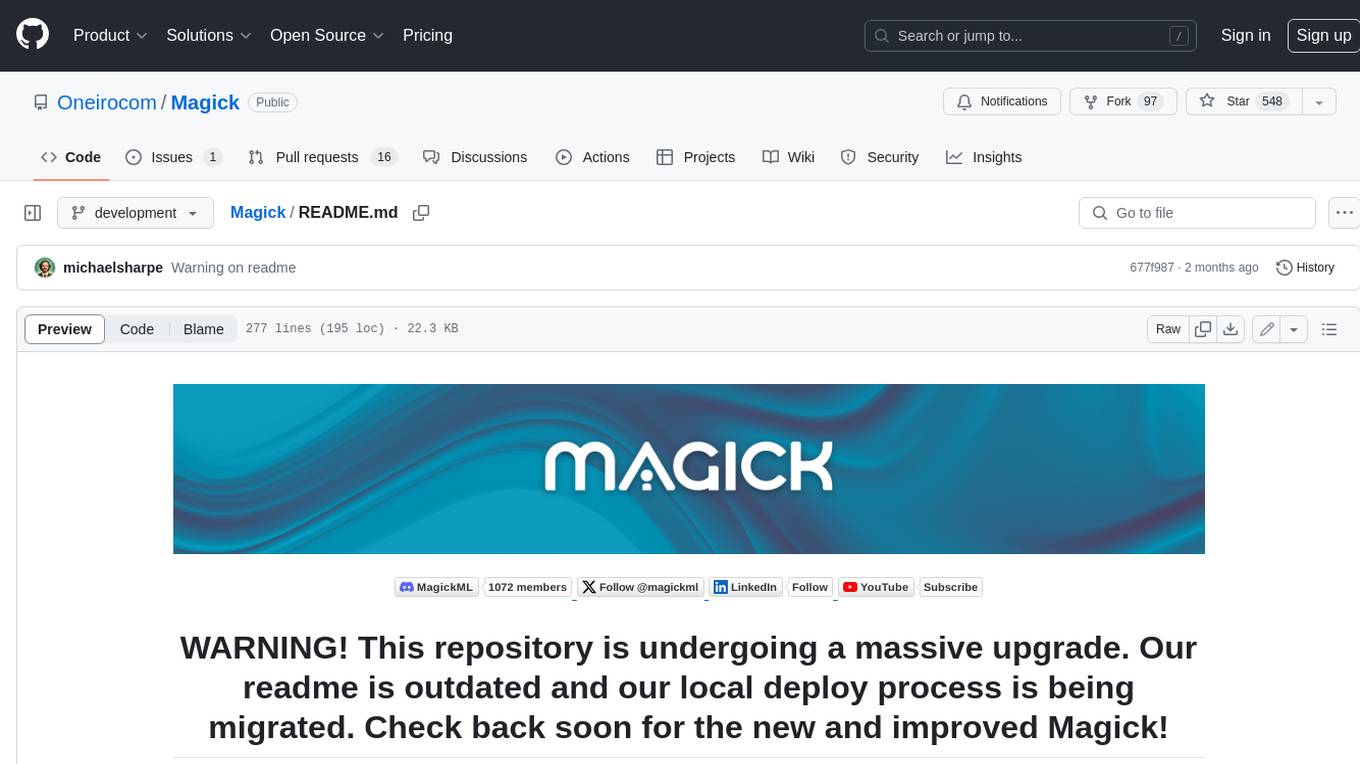
Magick
Magick is a groundbreaking visual AIDE (Artificial Intelligence Development Environment) for no-code data pipelines and multimodal agents. Magick can connect to other services and comes with nodes and templates well-suited for intelligent agents, chatbots, complex reasoning systems and realistic characters.