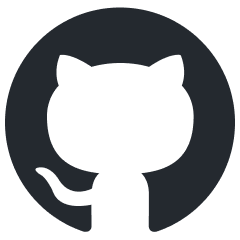
prompt-in-context-learning
Awesome resources for in-context learning and prompt engineering: Mastery of the LLMs such as ChatGPT, GPT-3, and FlanT5, with up-to-date and cutting-edge updates.
Stars: 1486
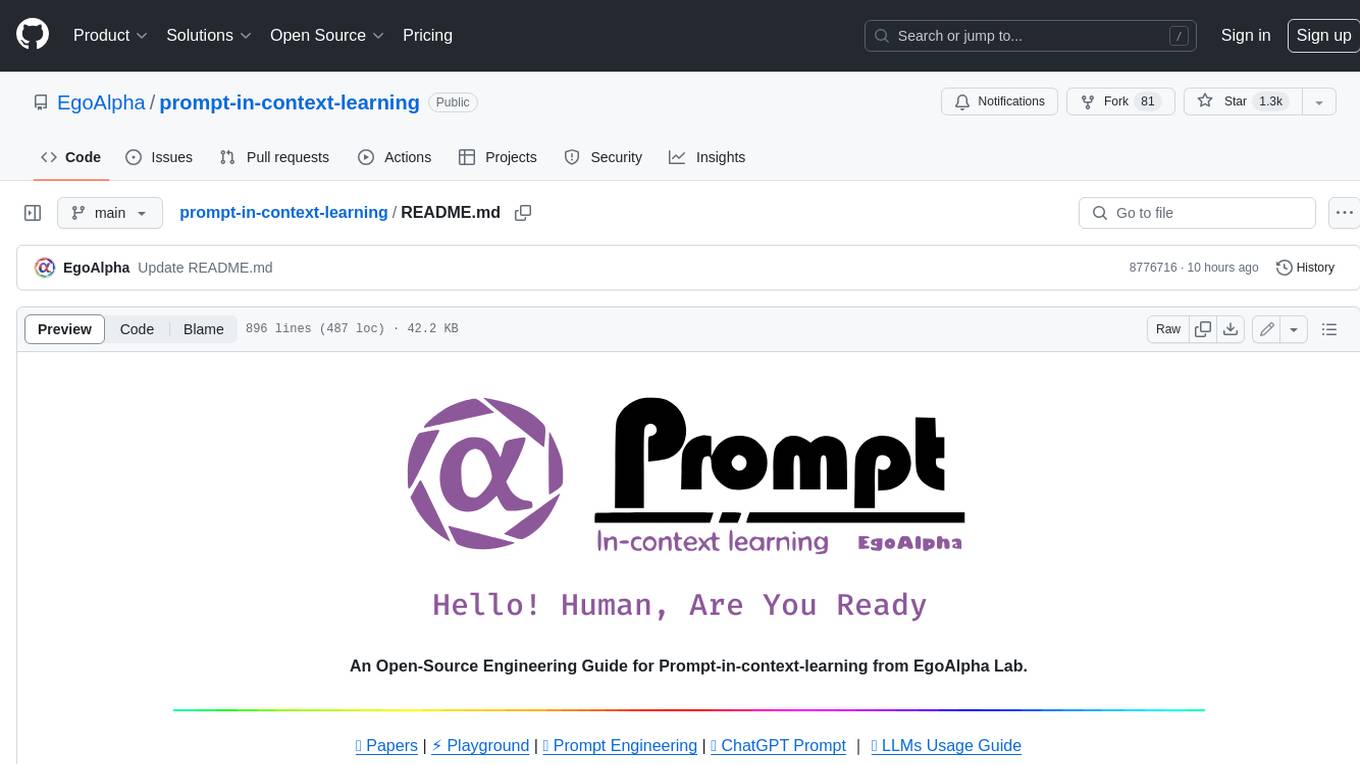
An Open-Source Engineering Guide for Prompt-in-context-learning from EgoAlpha Lab. 📝 Papers | ⚡️ Playground | 🛠 Prompt Engineering | 🌍 ChatGPT Prompt | ⛳ LLMs Usage Guide > **⭐️ Shining ⭐️:** This is fresh, daily-updated resources for in-context learning and prompt engineering. As Artificial General Intelligence (AGI) is approaching, let’s take action and become a super learner so as to position ourselves at the forefront of this exciting era and strive for personal and professional greatness. The resources include: _🎉Papers🎉_: The latest papers about _In-Context Learning_ , _Prompt Engineering_ , _Agent_ , and _Foundation Models_. _🎉Playground🎉_: Large language models(LLMs)that enable prompt experimentation. _🎉Prompt Engineering🎉_: Prompt techniques for leveraging large language models. _🎉ChatGPT Prompt🎉_: Prompt examples that can be applied in our work and daily lives. _🎉LLMs Usage Guide🎉_: The method for quickly getting started with large language models by using LangChain. In the future, there will likely be two types of people on Earth (perhaps even on Mars, but that's a question for Musk): - Those who enhance their abilities through the use of AIGC; - Those whose jobs are replaced by AI automation. 💎EgoAlpha: Hello! human👤, are you ready?
README:
An Open-Source Engineering Guide for Prompt-in-context-learning from EgoAlpha Lab.

📝 Papers | ⚡️ Playground | 🛠 Prompt Engineering | 🌍 ChatGPT Prompt | ⛳ LLMs Usage Guide
⭐️ Shining ⭐️: This is fresh, daily-updated resources for in-context learning and prompt engineering. As Artificial General Intelligence (AGI) is approaching, let’s take action and become a super learner so as to position ourselves at the forefront of this exciting era and strive for personal and professional greatness.
The resources include:
🎉Papers🎉: The latest papers about In-Context Learning, Prompt Engineering, Agent, and Foundation Models.
🎉Playground🎉: Large language models(LLMs)that enable prompt experimentation.
🎉Prompt Engineering🎉: Prompt techniques for leveraging large language models.
🎉ChatGPT Prompt🎉: Prompt examples that can be applied in our work and daily lives.
🎉LLMs Usage Guide🎉: The method for quickly getting started with large language models by using LangChain.
In the future, there will likely be two types of people on Earth (perhaps even on Mars, but that's a question for Musk):
- Those who enhance their abilities through the use of AIGC;
- Those whose jobs are replaced by AI automation.
💎EgoAlpha: Hello! human👤, are you ready?
☄️ EgoAlpha releases the TrustGPT focuses on reasoning. Trust the GPT with the strongest reasoning abilities for authentic and reliable answers. You can click here or visit the Playgrounds directly to experience it。
-
[2024.10.5]
-
[2024.10.4]
-
[2024.10.3]
-
[2024.10.2]
-
[2024.10.1]
-
[2024.9.30]
-
[2024.9.29]
-
[2024.9.28]
-
[2024.9.27]
-
[2024.9.26]
-
[2024.9.25]
-
[2024.9.24]
-
[2024.9.23]
-
[2024.9.22]
-
[2024.9.21]
-
[2024.9.20]
-
[2024.9.19]
-
[2024.9.18]
-
[2024.9.17]
-
[2024.9.16]
-
[2024.9.15]
-
[2024.9.14]
-
[2024.9.13]
-
[2024.9.12]
-
[2024.9.11]
-
[2024.9.10]
-
[2024.9.9]
-
[2024.9.8]
-
[2024.9.7]
-
[2024.9.6]
You can directly click on the title to jump to the corresponding PDF link location
Motion meets Attention: Video Motion Prompts (2024.07.03)
Towards a Personal Health Large Language Model (2024.06.10)
Husky: A Unified, Open-Source Language Agent for Multi-Step Reasoning (2024.06.10)
Towards Lifelong Learning of Large Language Models: A Survey (2024.06.10)
Towards Semantic Equivalence of Tokenization in Multimodal LLM (2024.06.07)
LLMs Meet Multimodal Generation and Editing: A Survey (2024.05.29)
Tool Learning with Large Language Models: A Survey (2024.05.28)
When LLMs step into the 3D World: A Survey and Meta-Analysis of 3D Tasks via Multi-modal Large Language Models (2024.05.16)
Uncertainty Estimation and Quantification for LLMs: A Simple Supervised Approach (2024.04.24)
A Survey on the Memory Mechanism of Large Language Model based Agents (2024.04.21)
👉Complete paper list 🔗 for "Survey"👈
LLaRA: Supercharging Robot Learning Data for Vision-Language Policy (2024.06.28)
Dataset Size Recovery from LoRA Weights (2024.06.27)
Dual-Phase Accelerated Prompt Optimization (2024.06.19)
VoCo-LLaMA: Towards Vision Compression with Large Language Models (2024.06.18)
LaMDA: Large Model Fine-Tuning via Spectrally Decomposed Low-Dimensional Adaptation (2024.06.18)
The Impact of Initialization on LoRA Finetuning Dynamics (2024.06.12)
An Empirical Study on Parameter-Efficient Fine-Tuning for MultiModal Large Language Models (2024.06.07)
Cross-Context Backdoor Attacks against Graph Prompt Learning (2024.05.28)
Yuan 2.0-M32: Mixture of Experts with Attention Router (2024.05.28)
👉Complete paper list 🔗 for "Prompt Design"👈
An Empirical Study on Parameter-Efficient Fine-Tuning for MultiModal Large Language Models (2024.06.07)
Cantor: Inspiring Multimodal Chain-of-Thought of MLLM (2024.04.24)
Visualization-of-Thought Elicits Spatial Reasoning in Large Language Models (2024.04.04)
Can Small Language Models Help Large Language Models Reason Better?: LM-Guided Chain-of-Thought (2024.04.04)
Visual CoT: Unleashing Chain-of-Thought Reasoning in Multi-Modal Language Models (2024.03.25)
A Chain-of-Thought Prompting Approach with LLMs for Evaluating Students' Formative Assessment Responses in Science (2024.03.21)
NavCoT: Boosting LLM-Based Vision-and-Language Navigation via Learning Disentangled Reasoning (2024.03.12)
ERA-CoT: Improving Chain-of-Thought through Entity Relationship Analysis (2024.03.11)
Bias-Augmented Consistency Training Reduces Biased Reasoning in Chain-of-Thought (2024.03.08)
👉Complete paper list 🔗 for "Chain of Thought"👈
LaMDA: Large Model Fine-Tuning via Spectrally Decomposed Low-Dimensional Adaptation (2024.06.18)
The Impact of Initialization on LoRA Finetuning Dynamics (2024.06.12)
An Empirical Study on Parameter-Efficient Fine-Tuning for MultiModal Large Language Models (2024.06.07)
Leveraging Visual Tokens for Extended Text Contexts in Multi-Modal Learning (2024.06.04)
Learning to grok: Emergence of in-context learning and skill composition in modular arithmetic tasks (2024.06.04)
Long Context is Not Long at All: A Prospector of Long-Dependency Data for Large Language Models (2024.05.28)
Efficient Prompt Tuning by Multi-Space Projection and Prompt Fusion (2024.05.19)
MAML-en-LLM: Model Agnostic Meta-Training of LLMs for Improved In-Context Learning (2024.05.19)
Improving Diversity of Commonsense Generation by Large Language Models via In-Context Learning (2024.04.25)
Stronger Random Baselines for In-Context Learning (2024.04.19)
👉Complete paper list 🔗 for "In-context Learning"👈
Retrieval-Augmented Mixture of LoRA Experts for Uploadable Machine Learning (2024.06.24)
Enhancing RAG Systems: A Survey of Optimization Strategies for Performance and Scalability (2024.06.04)
Enhancing Noise Robustness of Retrieval-Augmented Language Models with Adaptive Adversarial Training (2024.05.31)
Accelerating Inference of Retrieval-Augmented Generation via Sparse Context Selection (2024.05.25)
DocReLM: Mastering Document Retrieval with Language Model (2024.05.19)
UniRAG: Universal Retrieval Augmentation for Multi-Modal Large Language Models (2024.05.16)
ChatHuman: Language-driven 3D Human Understanding with Retrieval-Augmented Tool Reasoning (2024.05.07)
REASONS: A benchmark for REtrieval and Automated citationS Of scieNtific Sentences using Public and Proprietary LLMs (2024.05.03)
Superposition Prompting: Improving and Accelerating Retrieval-Augmented Generation (2024.04.10)
Untangle the KNOT: Interweaving Conflicting Knowledge and Reasoning Skills in Large Language Models (2024.04.04)
👉Complete paper list 🔗 for "Retrieval Augmented Generation"👈
CELLO: Causal Evaluation of Large Vision-Language Models (2024.06.27)
PrExMe! Large Scale Prompt Exploration of Open Source LLMs for Machine Translation and Summarization Evaluation (2024.06.26)
Revisiting Referring Expression Comprehension Evaluation in the Era of Large Multimodal Models (2024.06.24)
OR-Bench: An Over-Refusal Benchmark for Large Language Models (2024.05.31)
TimeChara: Evaluating Point-in-Time Character Hallucination of Role-Playing Large Language Models (2024.05.28)
HW-GPT-Bench: Hardware-Aware Architecture Benchmark for Language Models (2024.05.16)
Multimodal LLMs Struggle with Basic Visual Network Analysis: a VNA Benchmark (2024.05.10)
Vibe-Eval: A hard evaluation suite for measuring progress of multimodal language models (2024.05.03)
Causal Evaluation of Language Models (2024.05.01)
👉Complete paper list 🔗 for "Evaluation & Reliability"👈
Cooperative Multi-Agent Deep Reinforcement Learning Methods for UAV-aided Mobile Edge Computing Networks (2024.07.03)
Symbolic Learning Enables Self-Evolving Agents (2024.06.26)
Adversarial Attacks on Multimodal Agents (2024.06.18)
DigiRL: Training In-The-Wild Device-Control Agents with Autonomous Reinforcement Learning (2024.06.14)
Transforming Wearable Data into Health Insights using Large Language Model Agents (2024.06.10)
Neuromorphic dreaming: A pathway to efficient learning in artificial agents (2024.05.24)
Fine-Tuning Large Vision-Language Models as Decision-Making Agents via Reinforcement Learning (2024.05.16)
Learning Multi-Agent Communication from Graph Modeling Perspective (2024.05.14)
Smurfs: Leveraging Multiple Proficiency Agents with Context-Efficiency for Tool Planning (2024.05.09)
Unveiling Disparities in Web Task Handling Between Human and Web Agent (2024.05.07)
👉Complete paper list 🔗 for "Agent"👈
InternLM-XComposer-2.5: A Versatile Large Vision Language Model Supporting Long-Contextual Input and Output (2024.07.03)
LLaRA: Supercharging Robot Learning Data for Vision-Language Policy (2024.06.28)
Web2Code: A Large-scale Webpage-to-Code Dataset and Evaluation Framework for Multimodal LLMs (2024.06.28)
LLaVolta: Efficient Multi-modal Models via Stage-wise Visual Context Compression (2024.06.28)
Cambrian-1: A Fully Open, Vision-Centric Exploration of Multimodal LLMs (2024.06.24)
VoCo-LLaMA: Towards Vision Compression with Large Language Models (2024.06.18)
Beyond LLaVA-HD: Diving into High-Resolution Large Multimodal Models (2024.06.12)
An Empirical Study on Parameter-Efficient Fine-Tuning for MultiModal Large Language Models (2024.06.07)
Leveraging Visual Tokens for Extended Text Contexts in Multi-Modal Learning (2024.06.04)
DeCo: Decoupling Token Compression from Semantic Abstraction in Multimodal Large Language Models (2024.05.31)
👉Complete paper list 🔗 for "Multimodal Prompt"👈
IncogniText: Privacy-enhancing Conditional Text Anonymization via LLM-based Private Attribute Randomization (2024.07.03)
Web2Code: A Large-scale Webpage-to-Code Dataset and Evaluation Framework for Multimodal LLMs (2024.06.28)
OMG-LLaVA: Bridging Image-level, Object-level, Pixel-level Reasoning and Understanding (2024.06.27)
Adversarial Search Engine Optimization for Large Language Models (2024.06.26)
VideoLLM-online: Online Video Large Language Model for Streaming Video (2024.06.17)
Regularizing Hidden States Enables Learning Generalizable Reward Model for LLMs (2024.06.14)
Autoregressive Model Beats Diffusion: Llama for Scalable Image Generation (2024.06.10)
PaCE: Parsimonious Concept Engineering for Large Language Models (2024.06.06)
Yuan 2.0-M32: Mixture of Experts with Attention Router (2024.05.28)
👉Complete paper list 🔗 for "Prompt Application"👈
TheoremLlama: Transforming General-Purpose LLMs into Lean4 Experts (2024.07.03)
Pedestrian 3D Shape Understanding for Person Re-Identification via Multi-View Learning (2024.07.01)
Token Erasure as a Footprint of Implicit Vocabulary Items in LLMs (2024.06.28)
OMG-LLaVA: Bridging Image-level, Object-level, Pixel-level Reasoning and Understanding (2024.06.27)
Fundamental Problems With Model Editing: How Should Rational Belief Revision Work in LLMs? (2024.06.27)
Efficient World Models with Context-Aware Tokenization (2024.06.27)
The Remarkable Robustness of LLMs: Stages of Inference? (2024.06.27)
ResumeAtlas: Revisiting Resume Classification with Large-Scale Datasets and Large Language Models (2024.06.26)
AITTI: Learning Adaptive Inclusive Token for Text-to-Image Generation (2024.06.18)
Unveiling Encoder-Free Vision-Language Models (2024.06.17)
👉Complete paper list 🔗 for "Foundation Models"👈

Large language models (LLMs) are becoming a revolutionary technology that is shaping the development of our era. Developers can create applications that were previously only possible in our imaginations by building LLMs. However, using these LLMs often comes with certain technical barriers, and even at the introductory stage, people may be intimidated by cutting-edge technology: Do you have any questions like the following?
- ❓ How can LLM be built using programming?
- ❓ How can it be used and deployed in your own programs?
💡 If there was a tutorial that could be accessible to all audiences, not just computer science professionals, it would provide detailed and comprehensive guidance to quickly get started and operate in a short amount of time, ultimately achieving the goal of being able to use LLMs flexibly and creatively to build the programs they envision. And now, just for you: the most detailed and comprehensive Langchain beginner's guide, sourced from the official langchain website but with further adjustments to the content, accompanied by the most detailed and annotated code examples, teaching code lines by line and sentence by sentence to all audiences.
Click 👉here👈 to take a quick tour of getting started with LLM.

This repo is maintained by EgoAlpha Lab. Questions and discussions are welcome via [email protected]
.
We are willing to engage in discussions with friends from the academic and industrial communities, and explore the latest developments in prompt engineering and in-context learning together.

Thanks to the PhD students from EgoAlpha Lab and other workers who participated in this repo. We will improve the project in the follow-up period and maintain this community well. We also would like to express our sincere gratitude to the authors of the relevant resources. Your efforts have broadened our horizons and enabled us to perceive a more wonderful world.
For Tasks:
Click tags to check more tools for each tasksFor Jobs:
Alternative AI tools for prompt-in-context-learning
Similar Open Source Tools
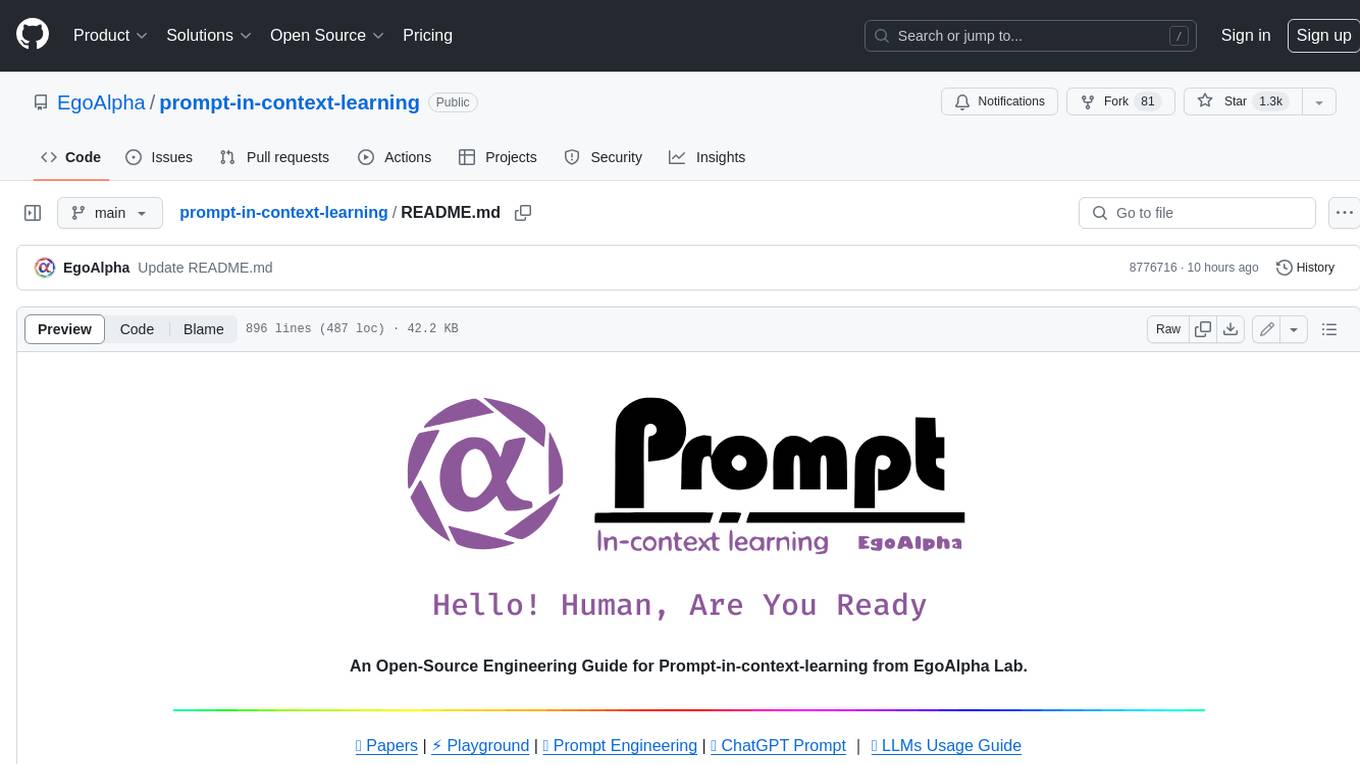
prompt-in-context-learning
An Open-Source Engineering Guide for Prompt-in-context-learning from EgoAlpha Lab. 📝 Papers | ⚡️ Playground | 🛠 Prompt Engineering | 🌍 ChatGPT Prompt | ⛳ LLMs Usage Guide > **⭐️ Shining ⭐️:** This is fresh, daily-updated resources for in-context learning and prompt engineering. As Artificial General Intelligence (AGI) is approaching, let’s take action and become a super learner so as to position ourselves at the forefront of this exciting era and strive for personal and professional greatness. The resources include: _🎉Papers🎉_: The latest papers about _In-Context Learning_ , _Prompt Engineering_ , _Agent_ , and _Foundation Models_. _🎉Playground🎉_: Large language models(LLMs)that enable prompt experimentation. _🎉Prompt Engineering🎉_: Prompt techniques for leveraging large language models. _🎉ChatGPT Prompt🎉_: Prompt examples that can be applied in our work and daily lives. _🎉LLMs Usage Guide🎉_: The method for quickly getting started with large language models by using LangChain. In the future, there will likely be two types of people on Earth (perhaps even on Mars, but that's a question for Musk): - Those who enhance their abilities through the use of AIGC; - Those whose jobs are replaced by AI automation. 💎EgoAlpha: Hello! human👤, are you ready?
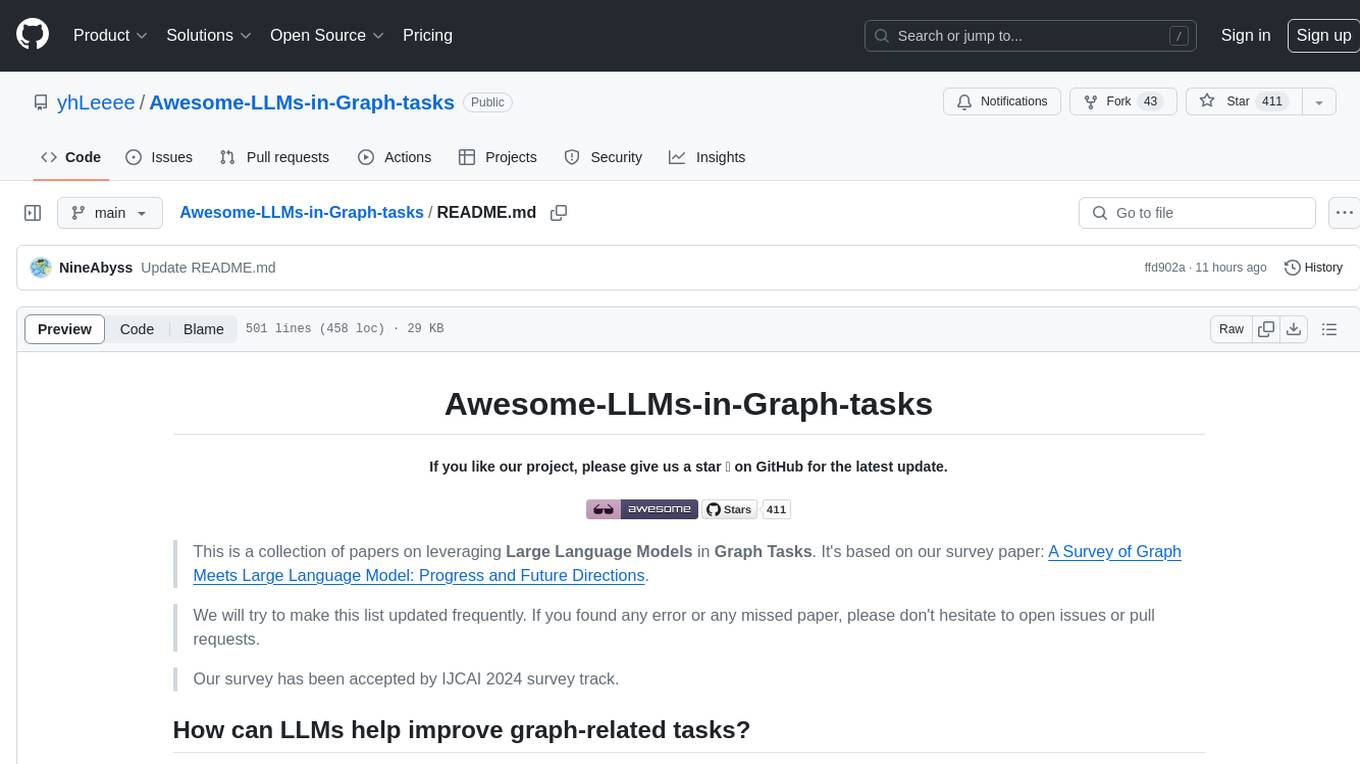
Awesome-LLMs-in-Graph-tasks
This repository is a collection of papers on leveraging Large Language Models (LLMs) in Graph Tasks. It provides a comprehensive overview of how LLMs can enhance graph-related tasks by combining them with traditional Graph Neural Networks (GNNs). The integration of LLMs with GNNs allows for capturing both structural and contextual aspects of nodes in graph data, leading to more powerful graph learning. The repository includes summaries of various models that leverage LLMs to assist in graph-related tasks, along with links to papers and code repositories for further exploration.
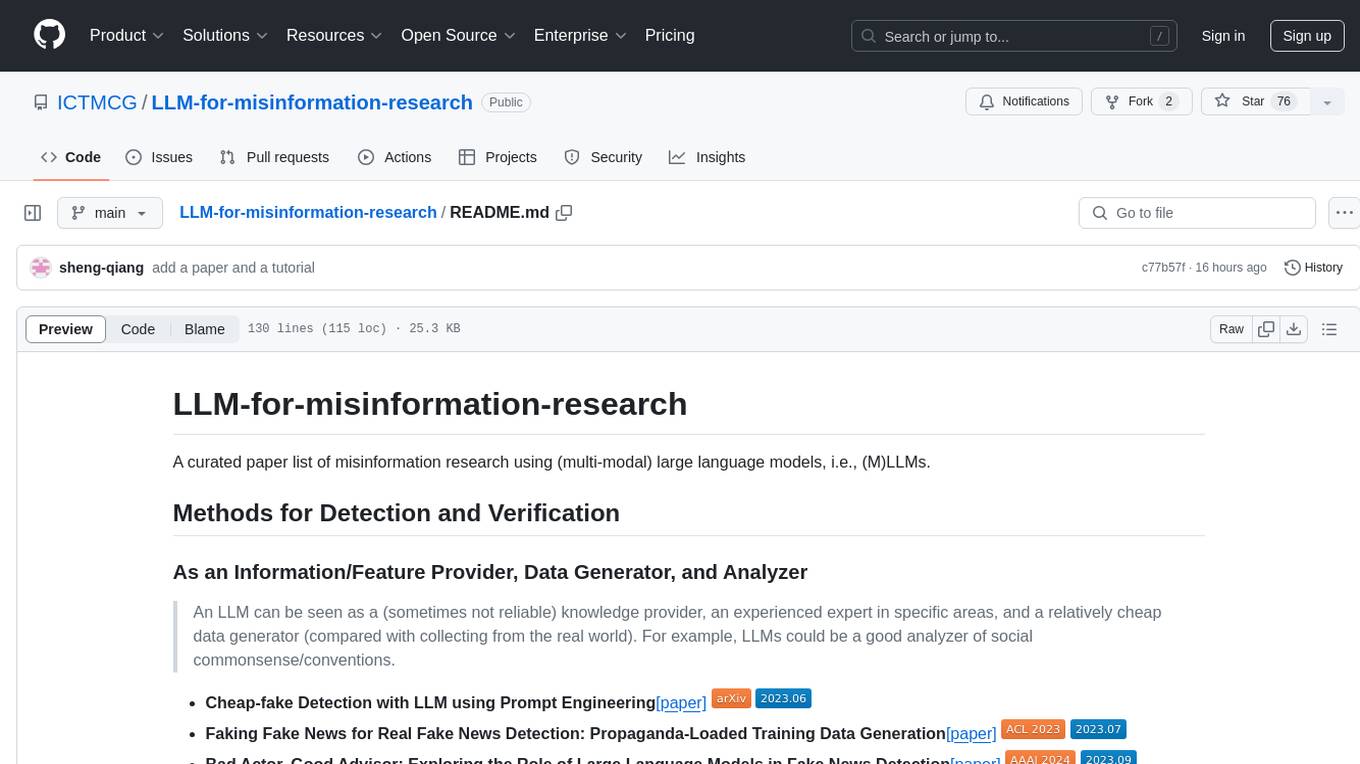
LLM-for-misinformation-research
LLM-for-misinformation-research is a curated paper list of misinformation research using large language models (LLMs). The repository covers methods for detection and verification, tools for fact-checking complex claims, decision-making and explanation, claim matching, post-hoc explanation generation, and other tasks related to combating misinformation. It includes papers on fake news detection, rumor detection, fact verification, and more, showcasing the application of LLMs in various aspects of misinformation research.
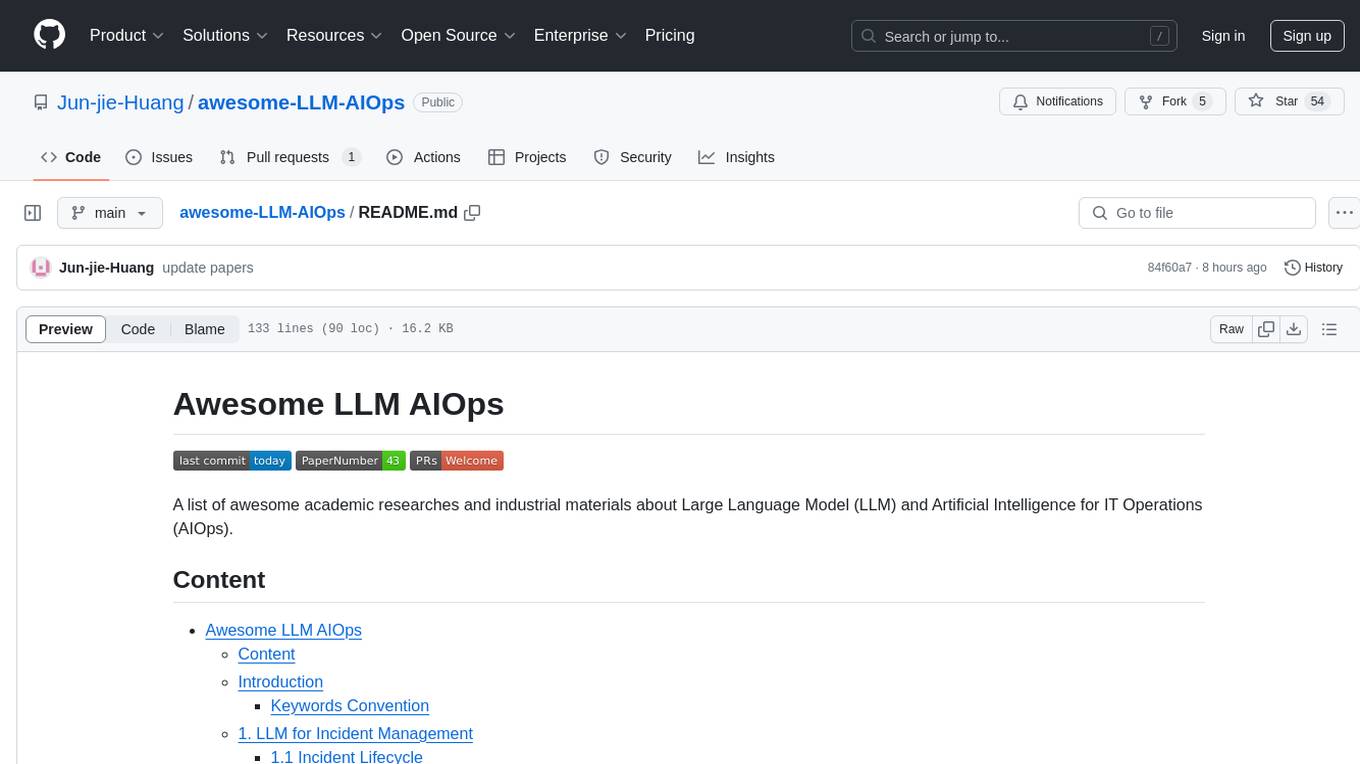
awesome-LLM-AIOps
The 'awesome-LLM-AIOps' repository is a curated list of academic research and industrial materials related to Large Language Models (LLM) and Artificial Intelligence for IT Operations (AIOps). It covers various topics such as incident management, log analysis, root cause analysis, incident mitigation, and incident postmortem analysis. The repository provides a comprehensive collection of papers, projects, and tools related to the application of LLM and AI in IT operations, offering valuable insights and resources for researchers and practitioners in the field.
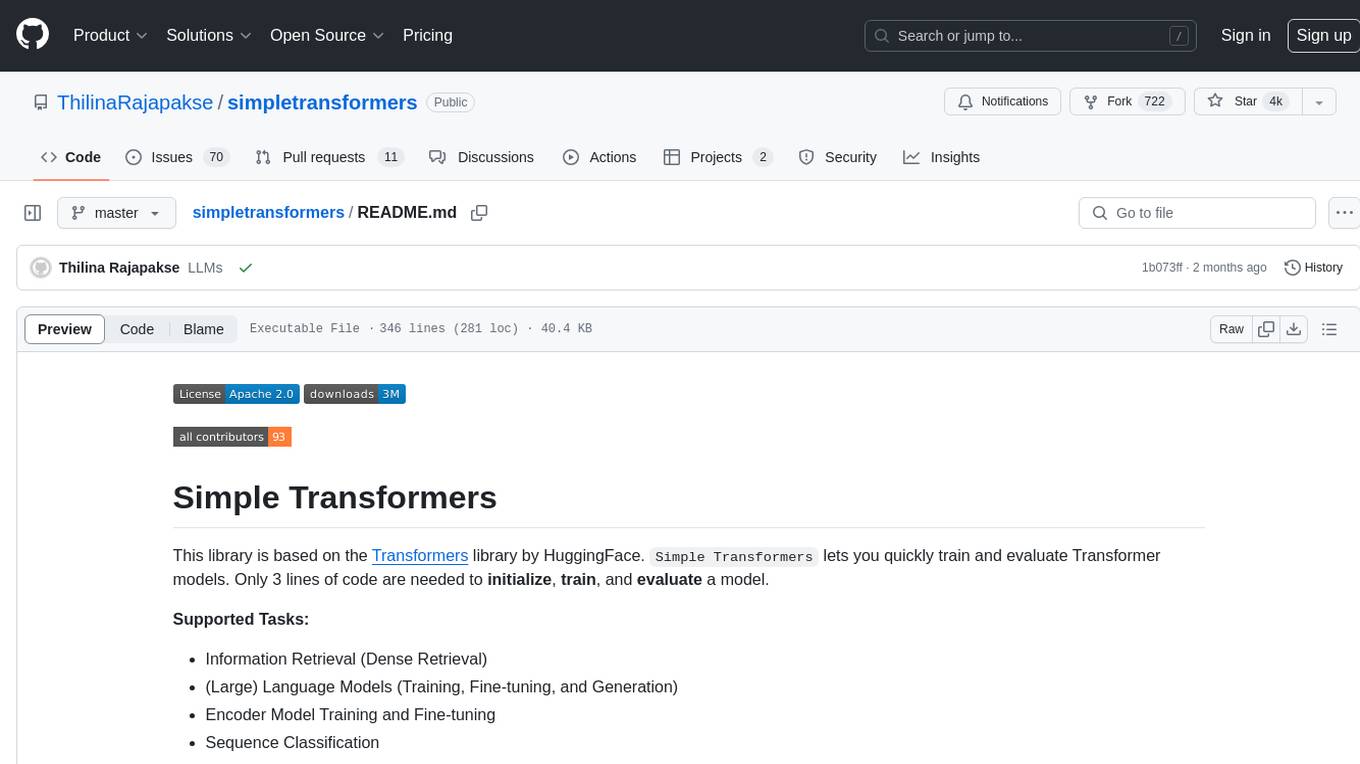
simpletransformers
Simple Transformers is a library based on the Transformers library by HuggingFace, allowing users to quickly train and evaluate Transformer models with only 3 lines of code. It supports various tasks such as Information Retrieval, Language Models, Encoder Model Training, Sequence Classification, Token Classification, Question Answering, Language Generation, T5 Model, Seq2Seq Tasks, Multi-Modal Classification, and Conversational AI.
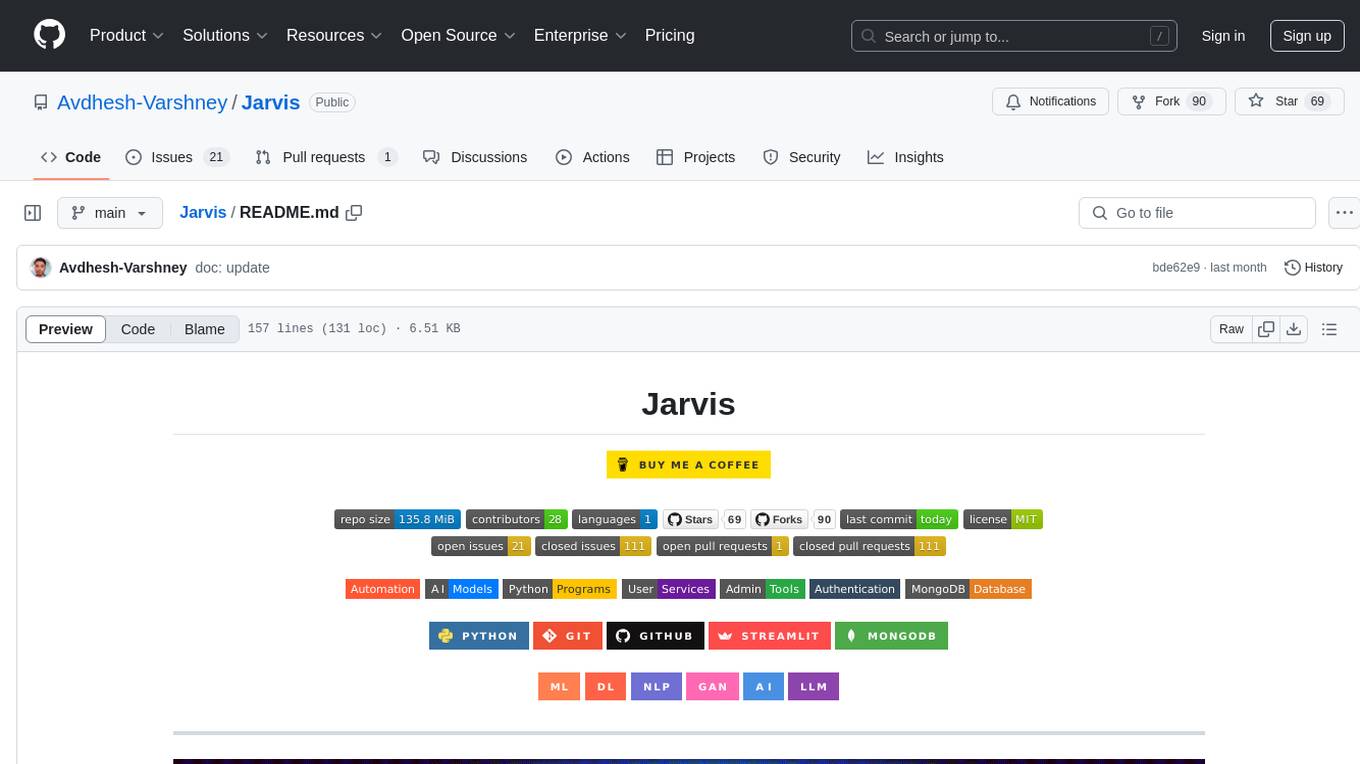
Jarvis
Jarvis is a powerful virtual AI assistant designed to simplify daily tasks through voice command integration. It features automation, device management, and personalized interactions, transforming technology engagement. Built using Python and AI models, it serves personal and administrative needs efficiently, making processes seamless and productive.
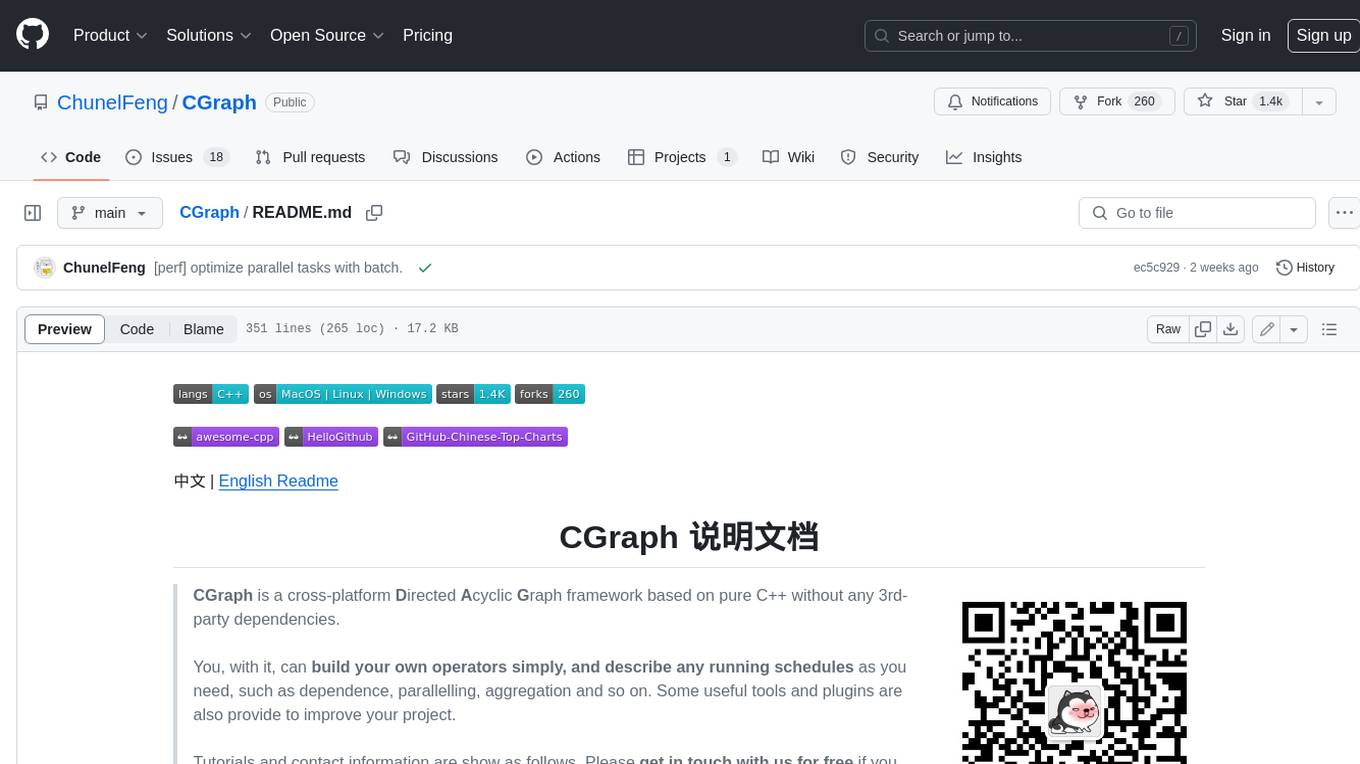
CGraph
CGraph is a cross-platform **D** irected **A** cyclic **G** raph framework based on pure C++ without any 3rd-party dependencies. You, with it, can **build your own operators simply, and describe any running schedules** as you need, such as dependence, parallelling, aggregation and so on. Some useful tools and plugins are also provide to improve your project. Tutorials and contact information are show as follows. Please **get in touch with us for free** if you need more about this repository.
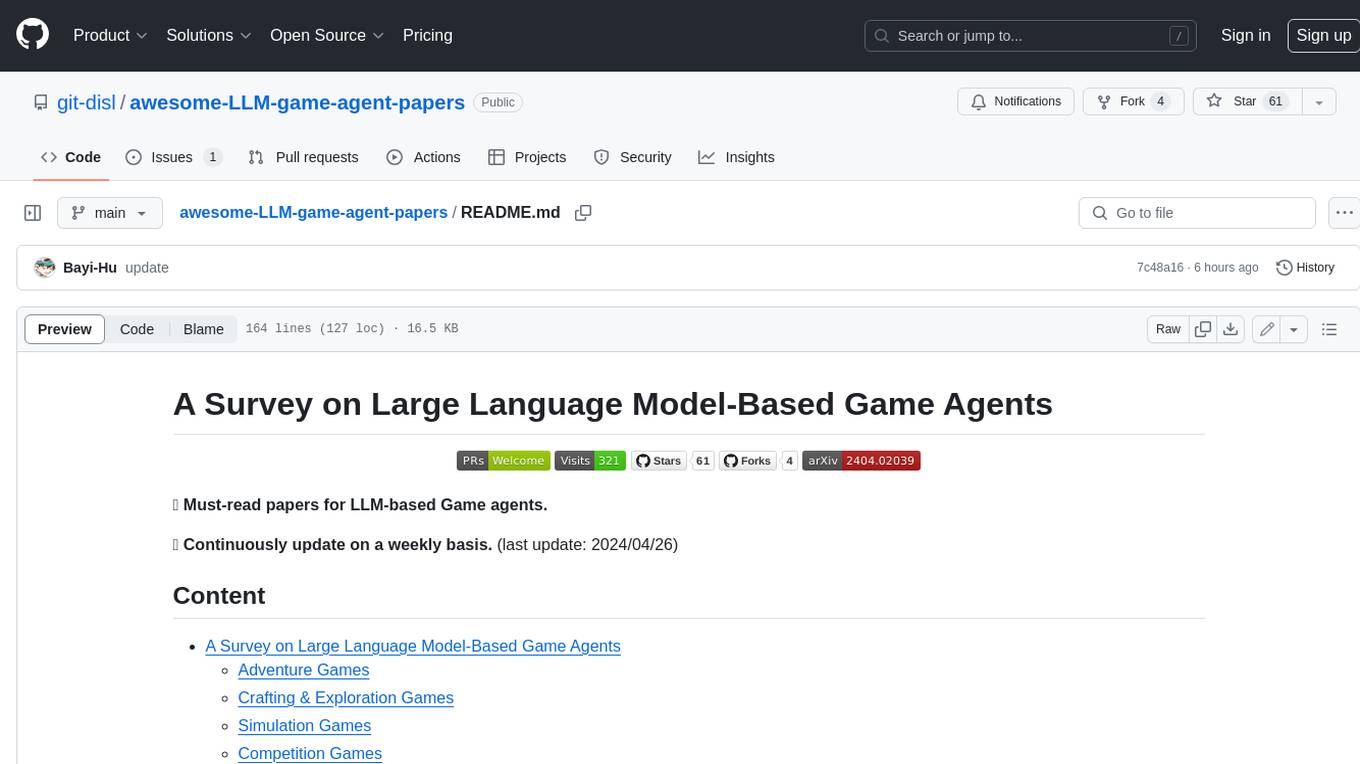
awesome-LLM-game-agent-papers
This repository provides a comprehensive survey of research papers on large language model (LLM)-based game agents. LLMs are powerful AI models that can understand and generate human language, and they have shown great promise for developing intelligent game agents. This survey covers a wide range of topics, including adventure games, crafting and exploration games, simulation games, competition games, cooperation games, communication games, and action games. For each topic, the survey provides an overview of the state-of-the-art research, as well as a discussion of the challenges and opportunities for future work.
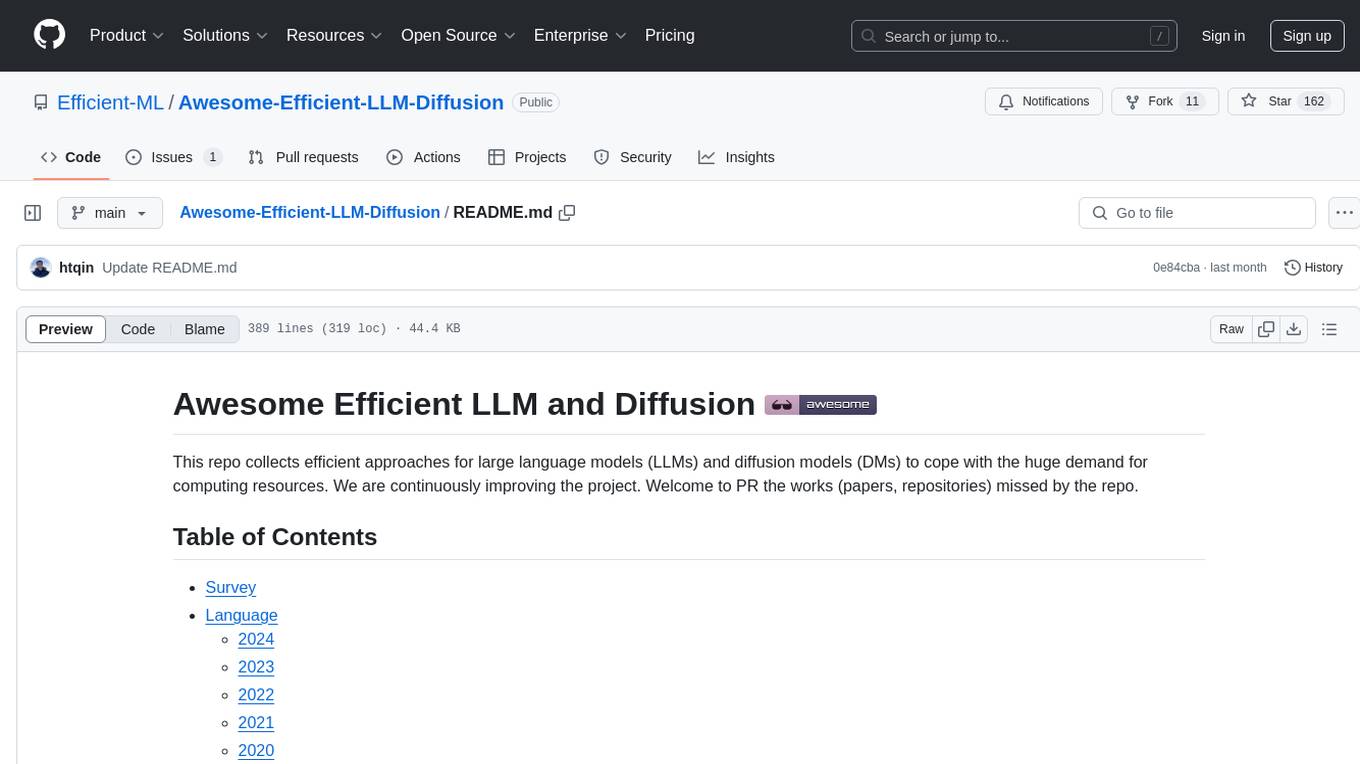
Awesome-Efficient-AIGC
This repository, Awesome Efficient AIGC, collects efficient approaches for AI-generated content (AIGC) to cope with its huge demand for computing resources. It includes efficient Large Language Models (LLMs), Diffusion Models (DMs), and more. The repository is continuously improving and welcomes contributions of works like papers and repositories that are missed by the collection.
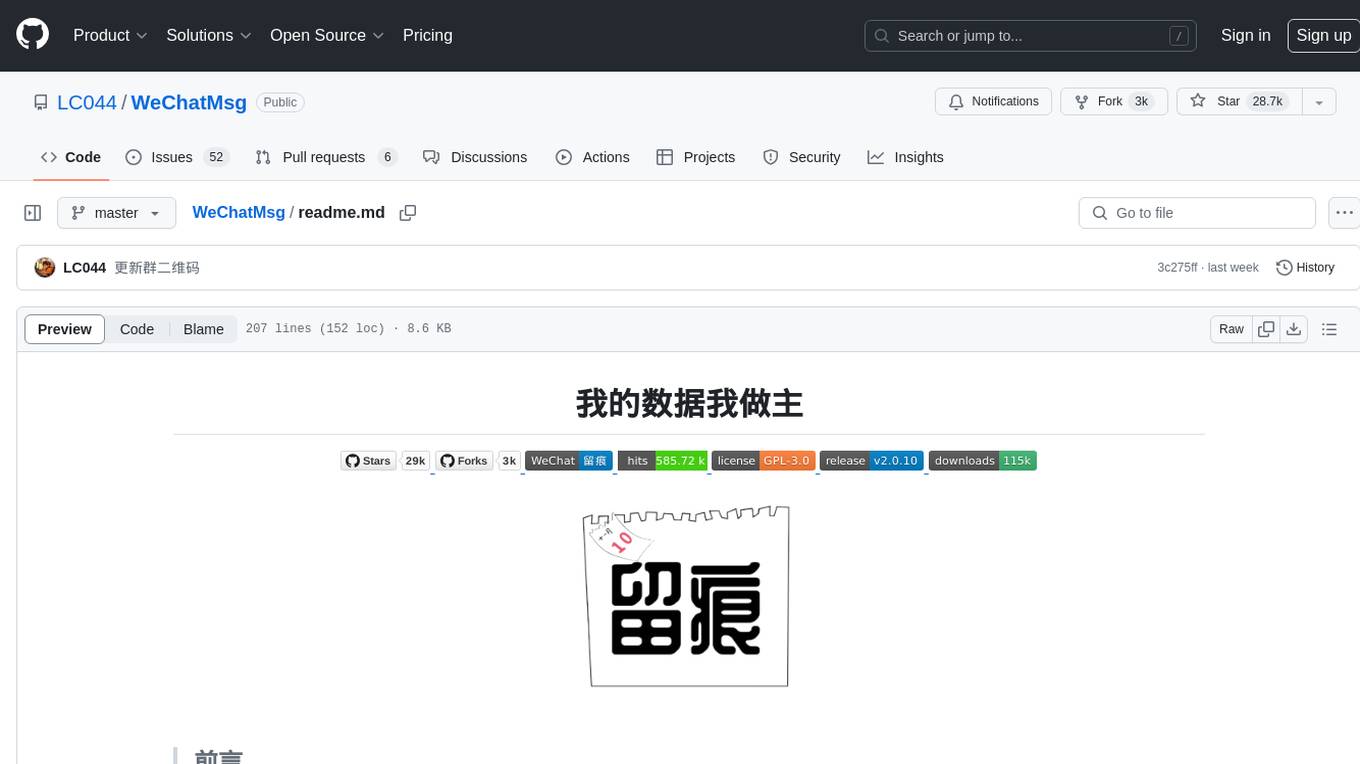
WeChatMsg
WeChatMsg is a tool designed to help users manage and analyze their WeChat data. It aims to provide users with the ability to preserve their precious memories and create a personalized AI companion. The tool allows users to extract and export various types of data from WeChat, such as text, images, contacts, and more. Additionally, it offers features like analyzing chat data and generating visual annual reports. WeChatMsg is built on the idea of empowering users to take control of their data and foster emotional connections through technology.
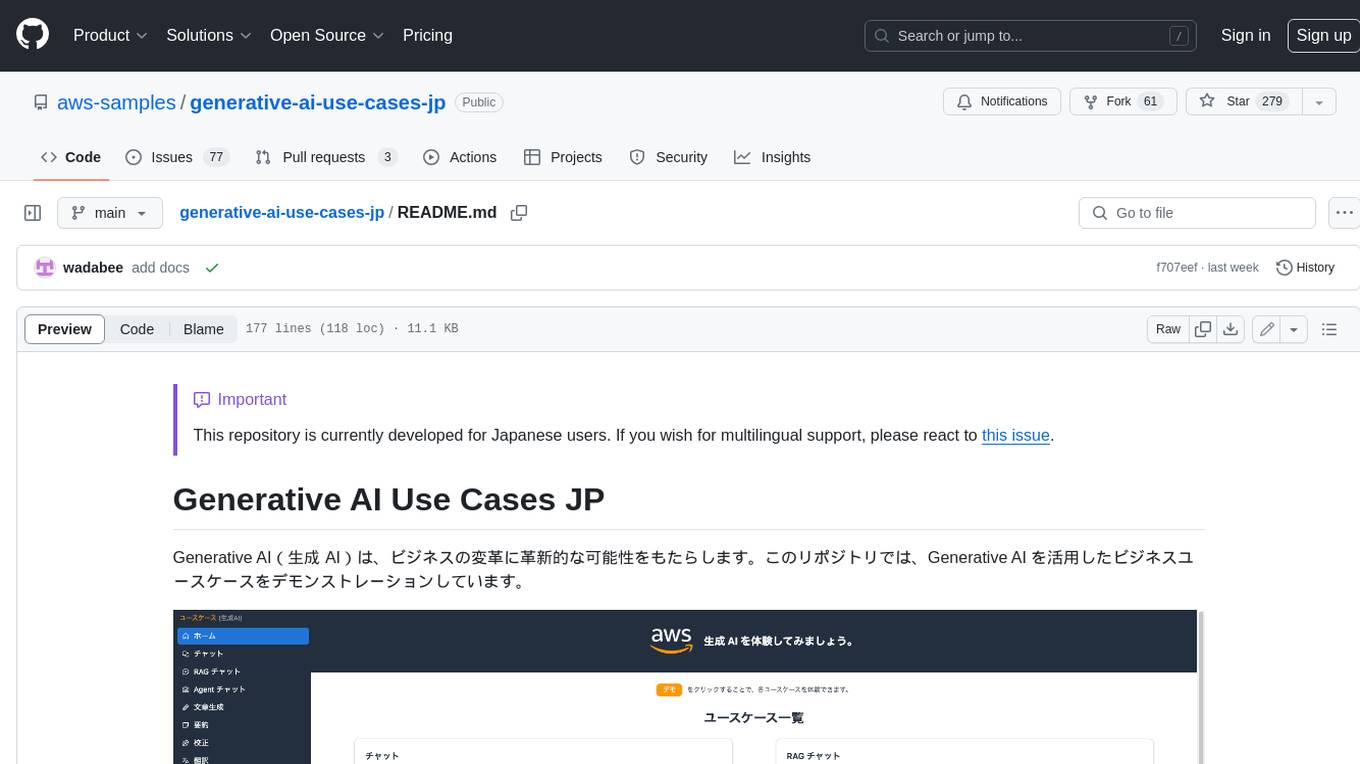
generative-ai-use-cases-jp
Generative AI (生成 AI) brings revolutionary potential to transform businesses. This repository demonstrates business use cases leveraging Generative AI.
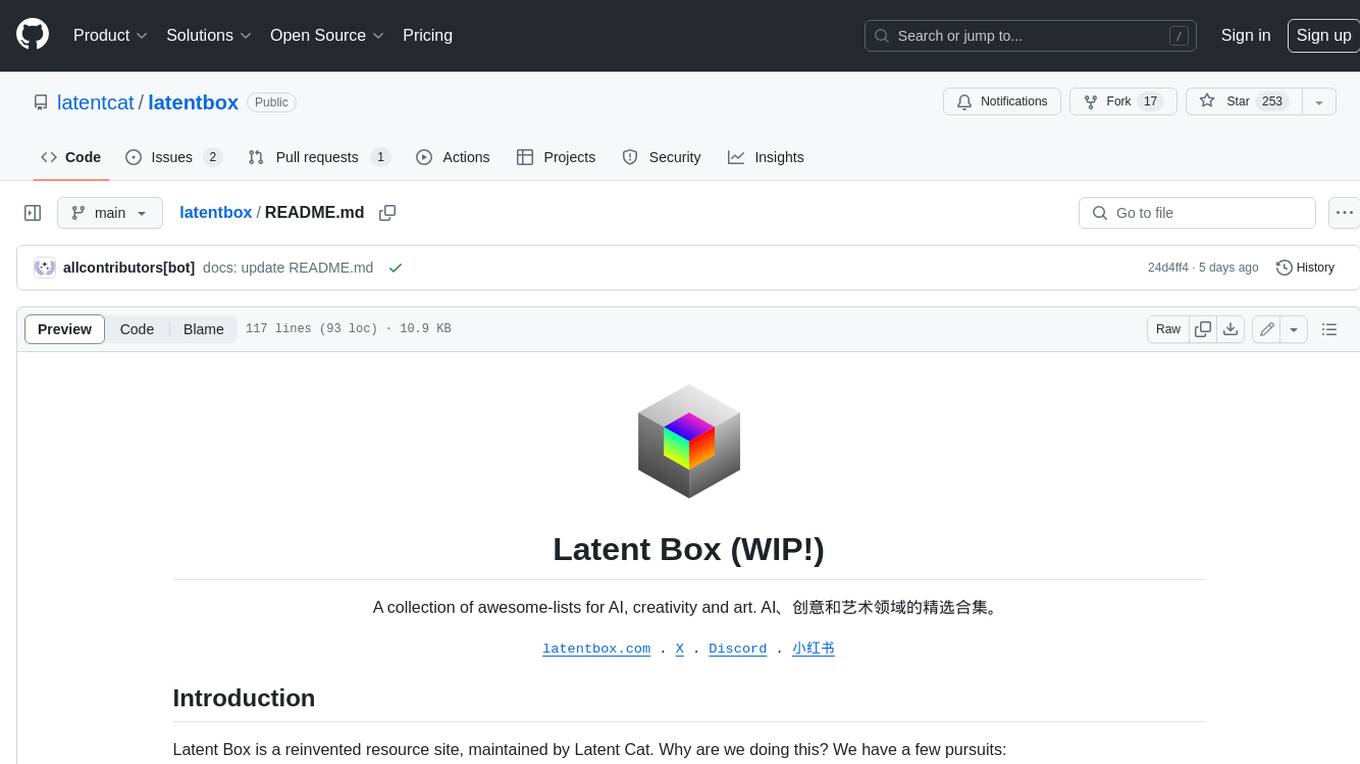
latentbox
Latent Box is a curated collection of resources for AI, creativity, and art. It aims to bridge the information gap with high-quality content, promote diversity and interdisciplinary collaboration, and maintain updates through community co-creation. The website features a wide range of resources, including articles, tutorials, tools, and datasets, covering various topics such as machine learning, computer vision, natural language processing, generative art, and creative coding.
For similar tasks
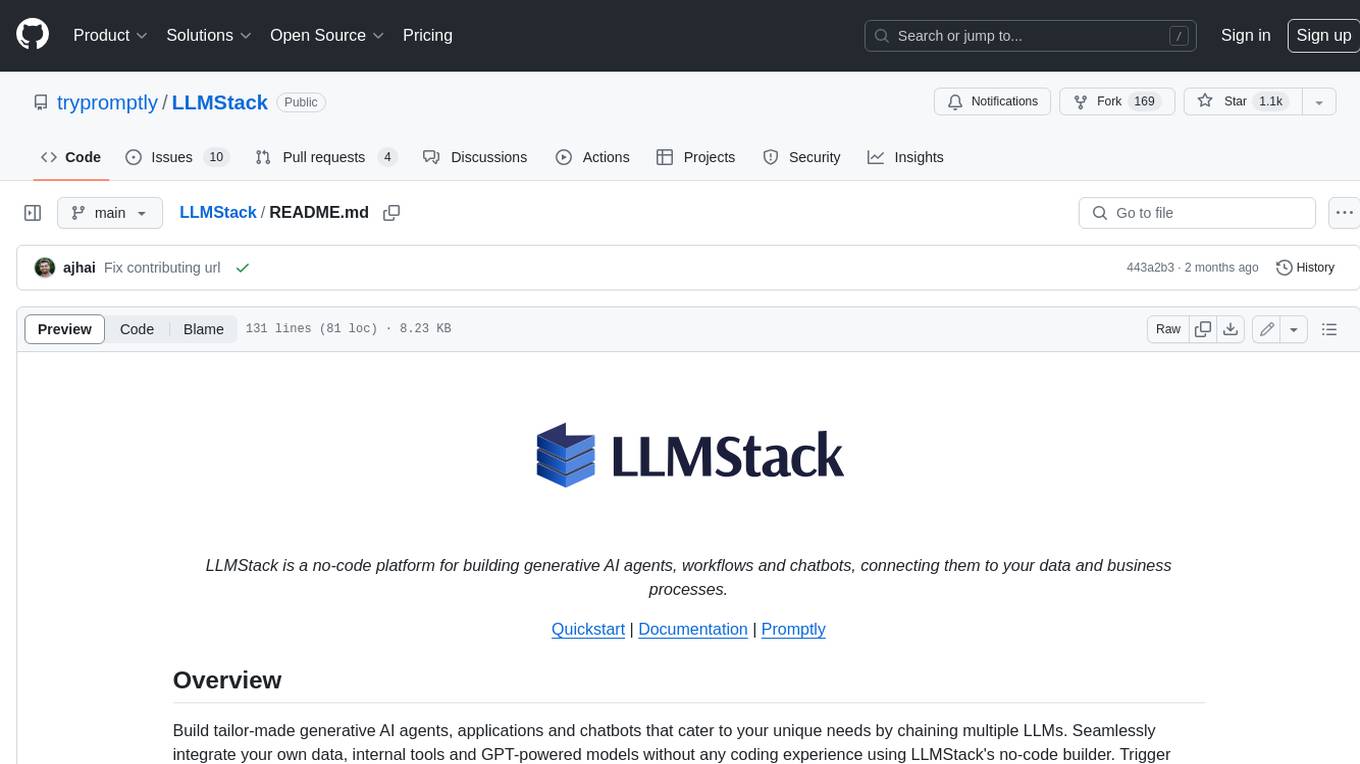
LLMStack
LLMStack is a no-code platform for building generative AI agents, workflows, and chatbots. It allows users to connect their own data, internal tools, and GPT-powered models without any coding experience. LLMStack can be deployed to the cloud or on-premise and can be accessed via HTTP API or triggered from Slack or Discord.
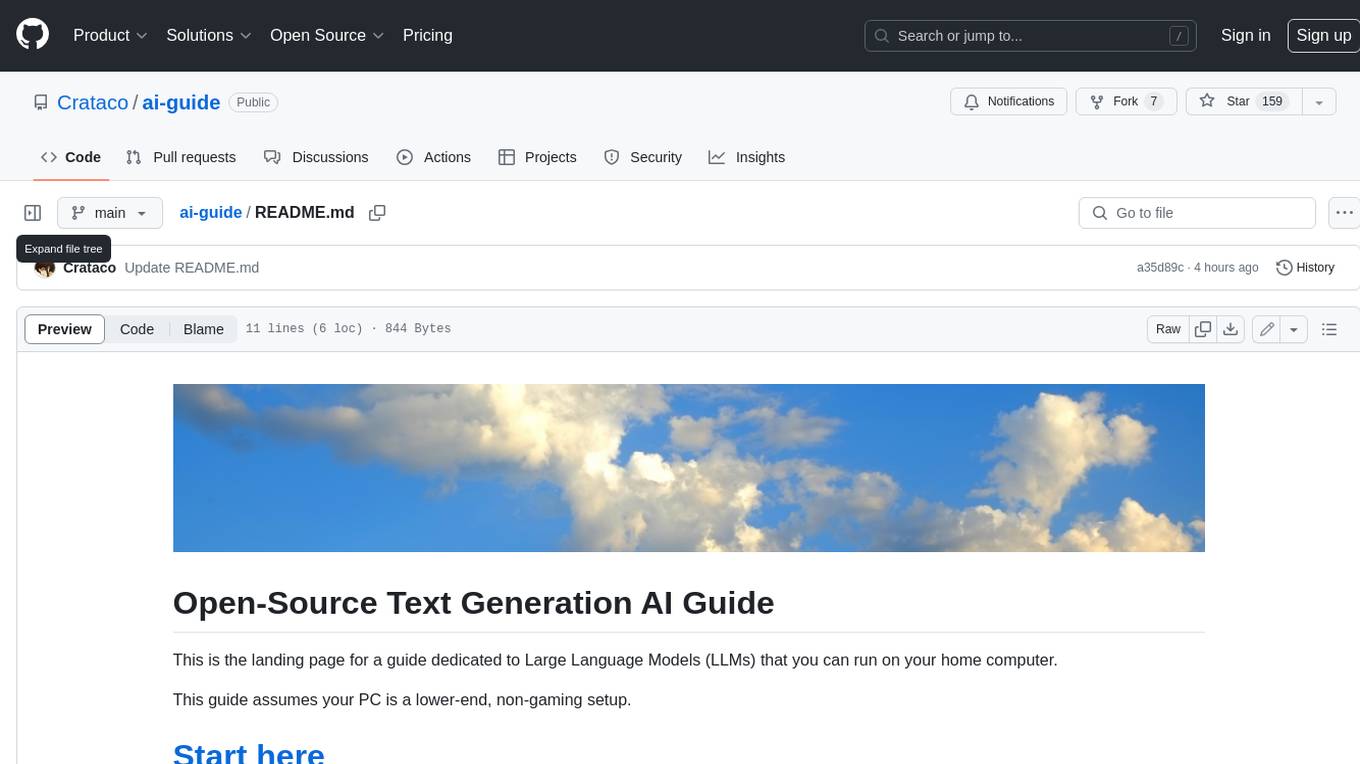
ai-guide
This guide is dedicated to Large Language Models (LLMs) that you can run on your home computer. It assumes your PC is a lower-end, non-gaming setup.
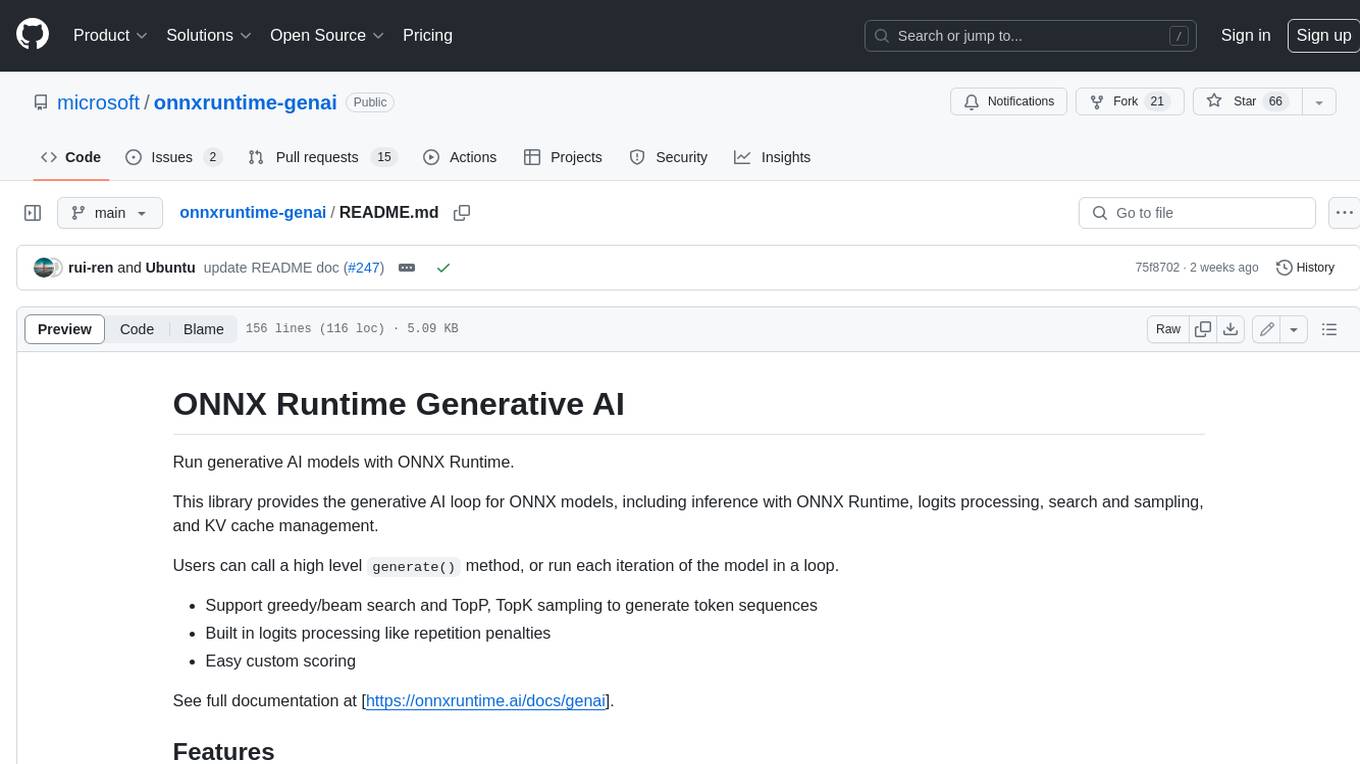
onnxruntime-genai
ONNX Runtime Generative AI is a library that provides the generative AI loop for ONNX models, including inference with ONNX Runtime, logits processing, search and sampling, and KV cache management. Users can call a high level `generate()` method, or run each iteration of the model in a loop. It supports greedy/beam search and TopP, TopK sampling to generate token sequences, has built in logits processing like repetition penalties, and allows for easy custom scoring.
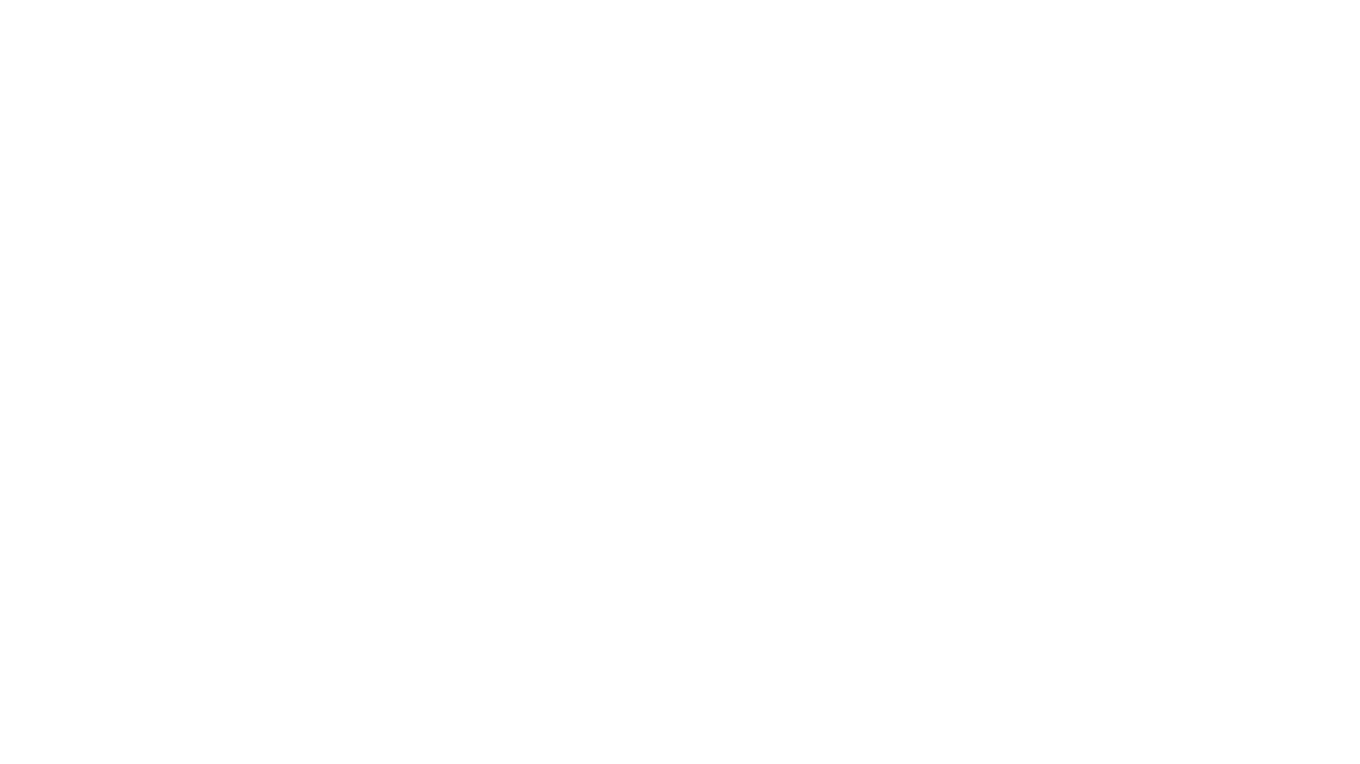
jupyter-ai
Jupyter AI connects generative AI with Jupyter notebooks. It provides a user-friendly and powerful way to explore generative AI models in notebooks and improve your productivity in JupyterLab and the Jupyter Notebook. Specifically, Jupyter AI offers: * An `%%ai` magic that turns the Jupyter notebook into a reproducible generative AI playground. This works anywhere the IPython kernel runs (JupyterLab, Jupyter Notebook, Google Colab, Kaggle, VSCode, etc.). * A native chat UI in JupyterLab that enables you to work with generative AI as a conversational assistant. * Support for a wide range of generative model providers, including AI21, Anthropic, AWS, Cohere, Gemini, Hugging Face, NVIDIA, and OpenAI. * Local model support through GPT4All, enabling use of generative AI models on consumer grade machines with ease and privacy.
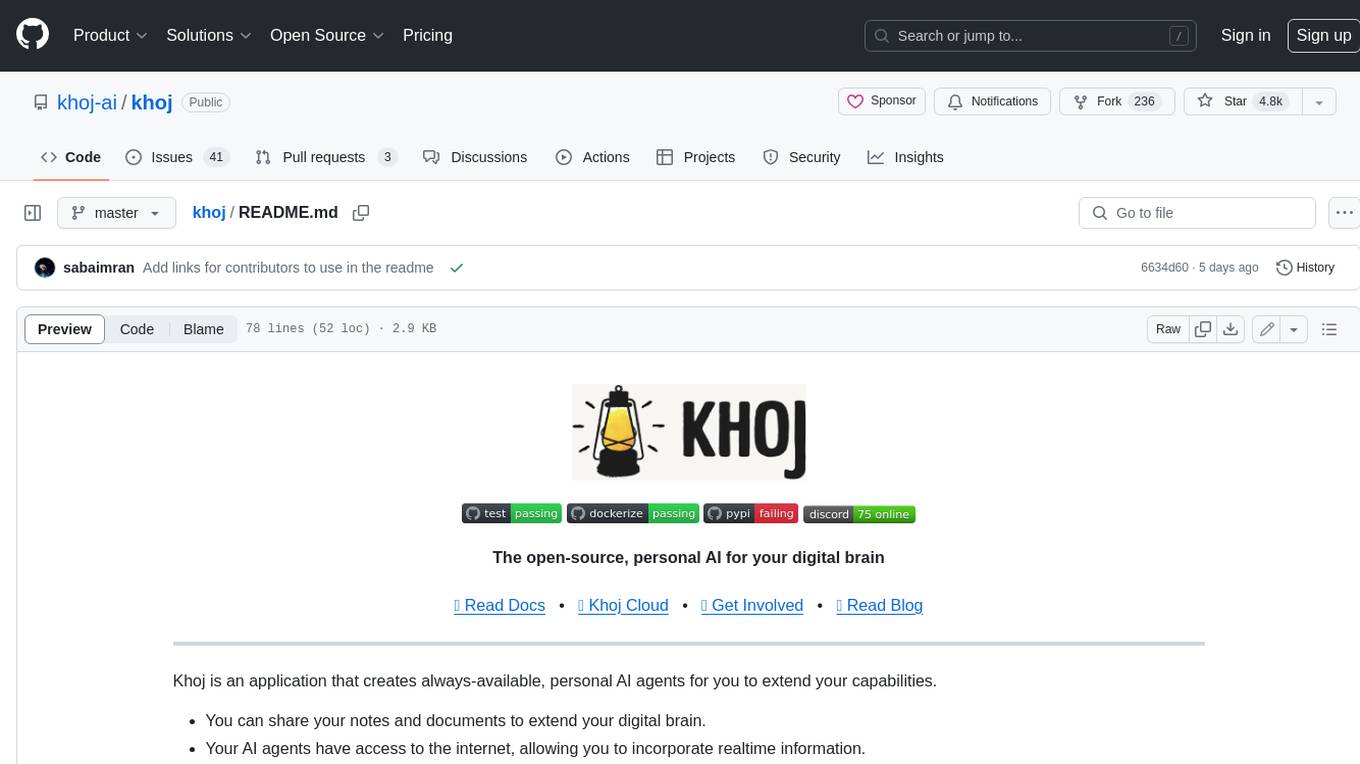
khoj
Khoj is an open-source, personal AI assistant that extends your capabilities by creating always-available AI agents. You can share your notes and documents to extend your digital brain, and your AI agents have access to the internet, allowing you to incorporate real-time information. Khoj is accessible on Desktop, Emacs, Obsidian, Web, and Whatsapp, and you can share PDF, markdown, org-mode, notion files, and GitHub repositories. You'll get fast, accurate semantic search on top of your docs, and your agents can create deeply personal images and understand your speech. Khoj is self-hostable and always will be.
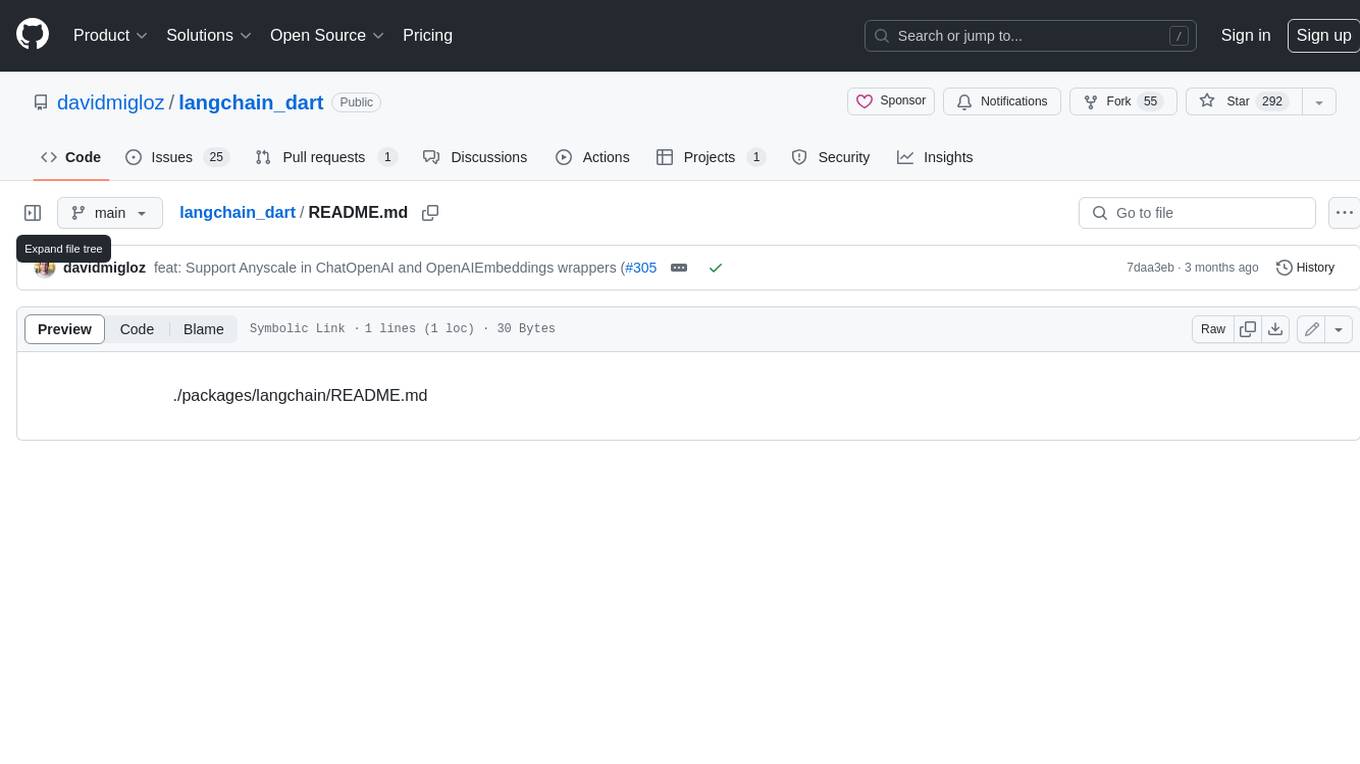
langchain_dart
LangChain.dart is a Dart port of the popular LangChain Python framework created by Harrison Chase. LangChain provides a set of ready-to-use components for working with language models and a standard interface for chaining them together to formulate more advanced use cases (e.g. chatbots, Q&A with RAG, agents, summarization, extraction, etc.). The components can be grouped into a few core modules: * **Model I/O:** LangChain offers a unified API for interacting with various LLM providers (e.g. OpenAI, Google, Mistral, Ollama, etc.), allowing developers to switch between them with ease. Additionally, it provides tools for managing model inputs (prompt templates and example selectors) and parsing the resulting model outputs (output parsers). * **Retrieval:** assists in loading user data (via document loaders), transforming it (with text splitters), extracting its meaning (using embedding models), storing (in vector stores) and retrieving it (through retrievers) so that it can be used to ground the model's responses (i.e. Retrieval-Augmented Generation or RAG). * **Agents:** "bots" that leverage LLMs to make informed decisions about which available tools (such as web search, calculators, database lookup, etc.) to use to accomplish the designated task. The different components can be composed together using the LangChain Expression Language (LCEL).
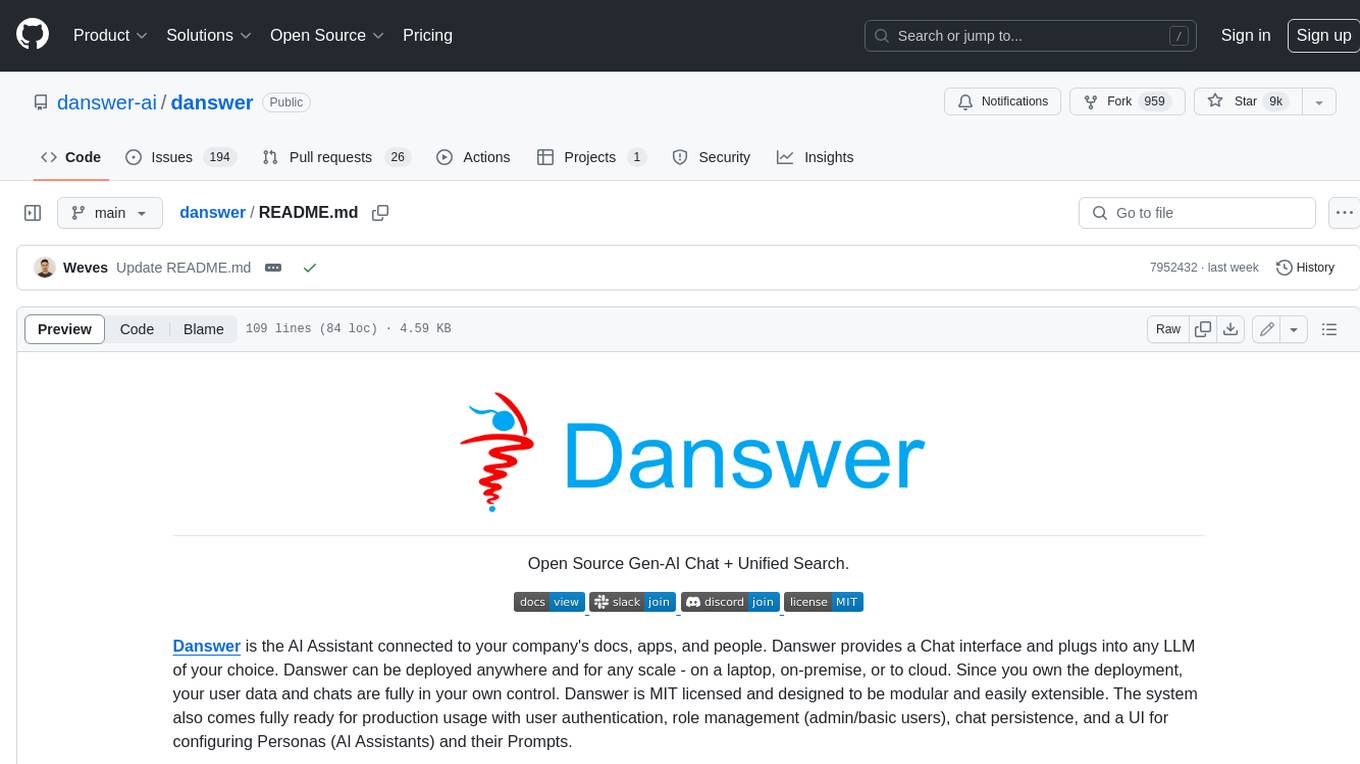
danswer
Danswer is an open-source Gen-AI Chat and Unified Search tool that connects to your company's docs, apps, and people. It provides a Chat interface and plugs into any LLM of your choice. Danswer can be deployed anywhere and for any scale - on a laptop, on-premise, or to cloud. Since you own the deployment, your user data and chats are fully in your own control. Danswer is MIT licensed and designed to be modular and easily extensible. The system also comes fully ready for production usage with user authentication, role management (admin/basic users), chat persistence, and a UI for configuring Personas (AI Assistants) and their Prompts. Danswer also serves as a Unified Search across all common workplace tools such as Slack, Google Drive, Confluence, etc. By combining LLMs and team specific knowledge, Danswer becomes a subject matter expert for the team. Imagine ChatGPT if it had access to your team's unique knowledge! It enables questions such as "A customer wants feature X, is this already supported?" or "Where's the pull request for feature Y?"
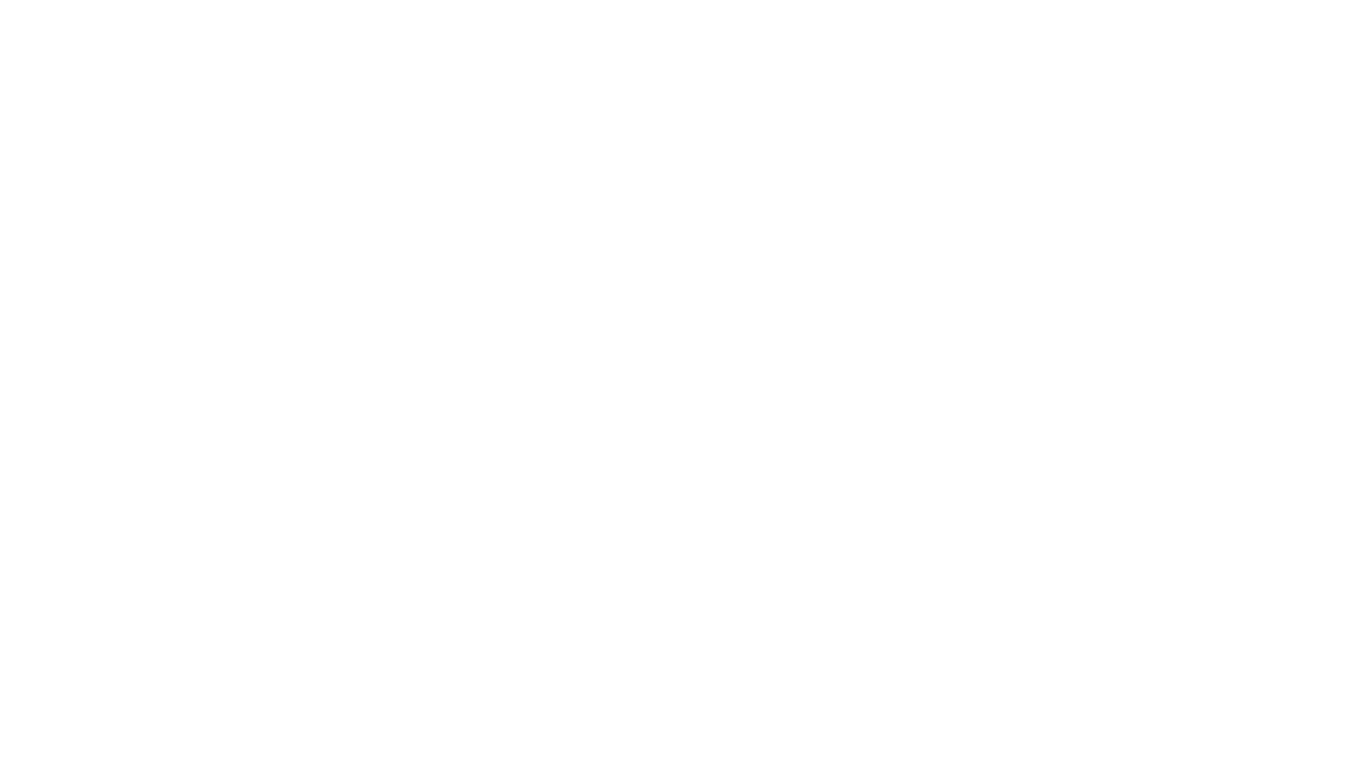
infinity
Infinity is an AI-native database designed for LLM applications, providing incredibly fast full-text and vector search capabilities. It supports a wide range of data types, including vectors, full-text, and structured data, and offers a fused search feature that combines multiple embeddings and full text. Infinity is easy to use, with an intuitive Python API and a single-binary architecture that simplifies deployment. It achieves high performance, with 0.1 milliseconds query latency on million-scale vector datasets and up to 15K QPS.
For similar jobs
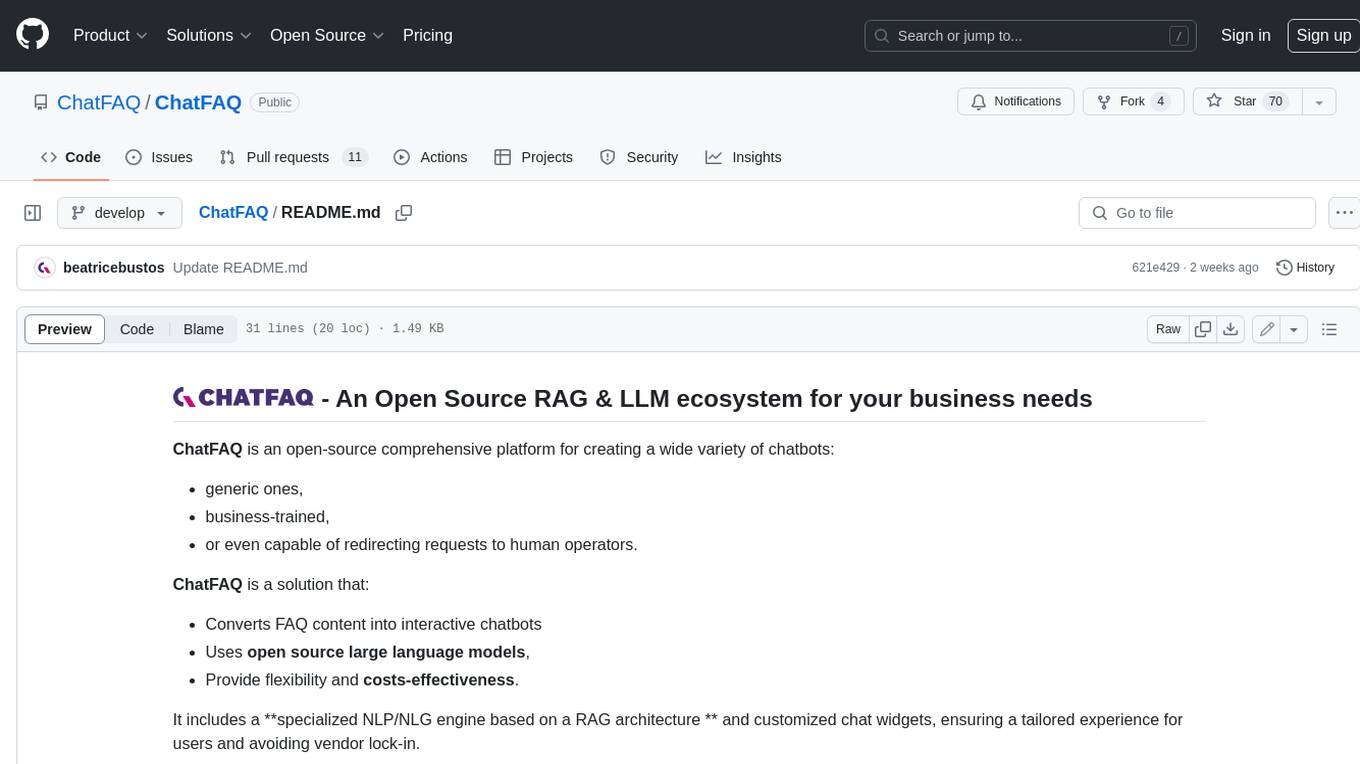
ChatFAQ
ChatFAQ is an open-source comprehensive platform for creating a wide variety of chatbots: generic ones, business-trained, or even capable of redirecting requests to human operators. It includes a specialized NLP/NLG engine based on a RAG architecture and customized chat widgets, ensuring a tailored experience for users and avoiding vendor lock-in.
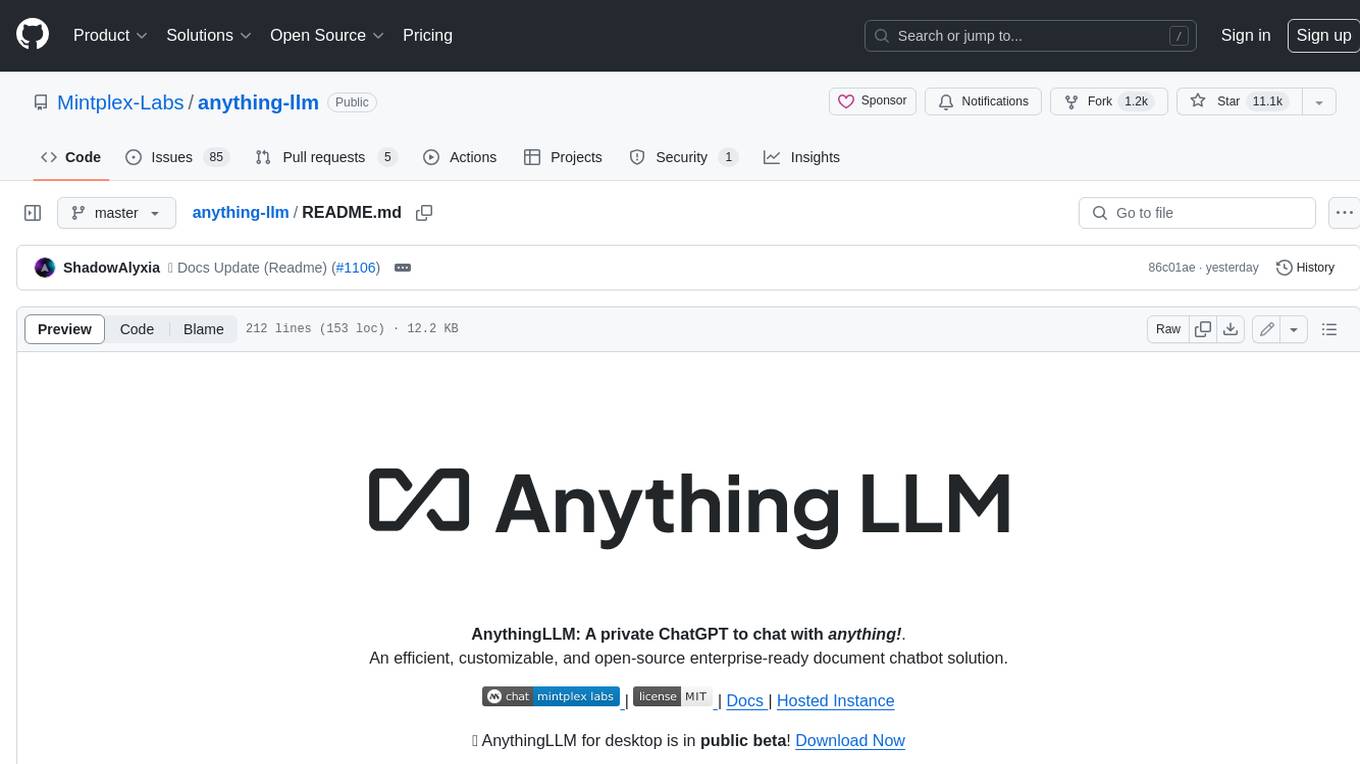
anything-llm
AnythingLLM is a full-stack application that enables you to turn any document, resource, or piece of content into context that any LLM can use as references during chatting. This application allows you to pick and choose which LLM or Vector Database you want to use as well as supporting multi-user management and permissions.
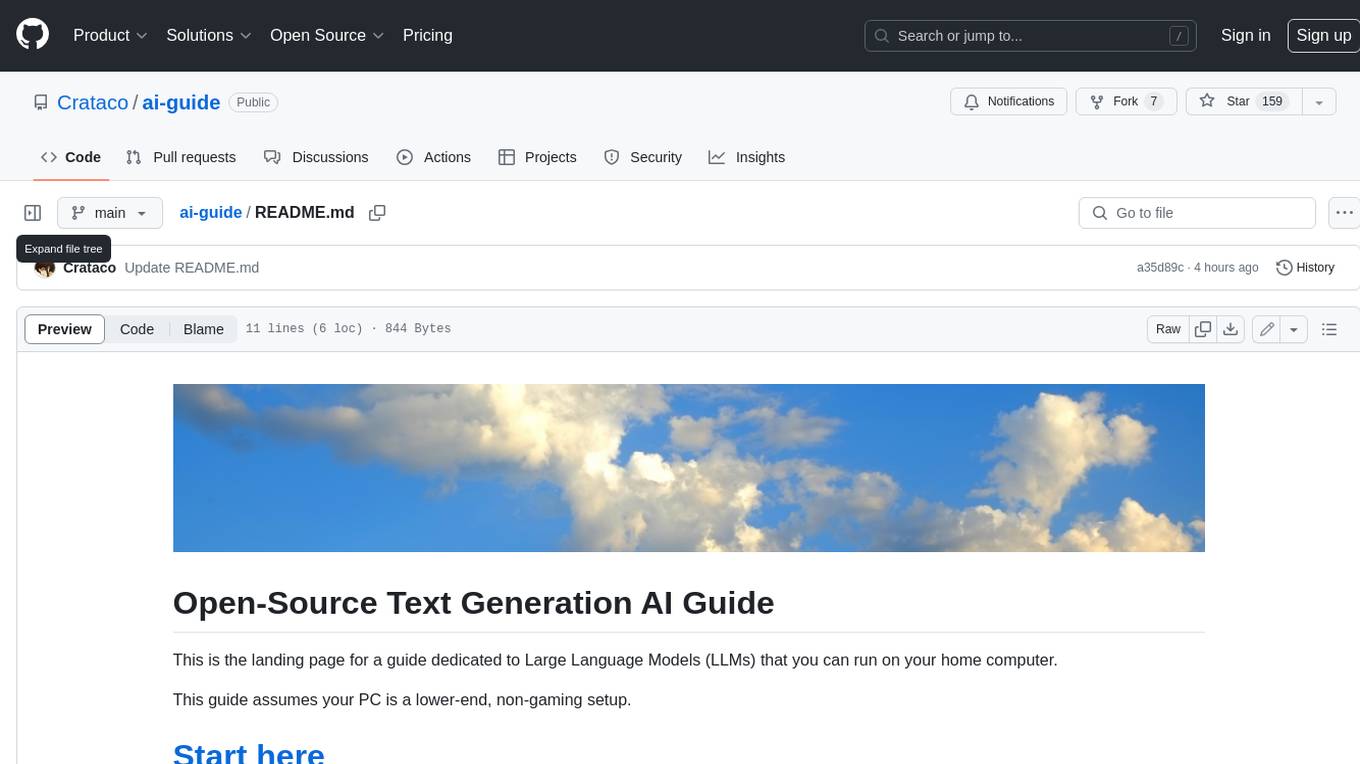
ai-guide
This guide is dedicated to Large Language Models (LLMs) that you can run on your home computer. It assumes your PC is a lower-end, non-gaming setup.
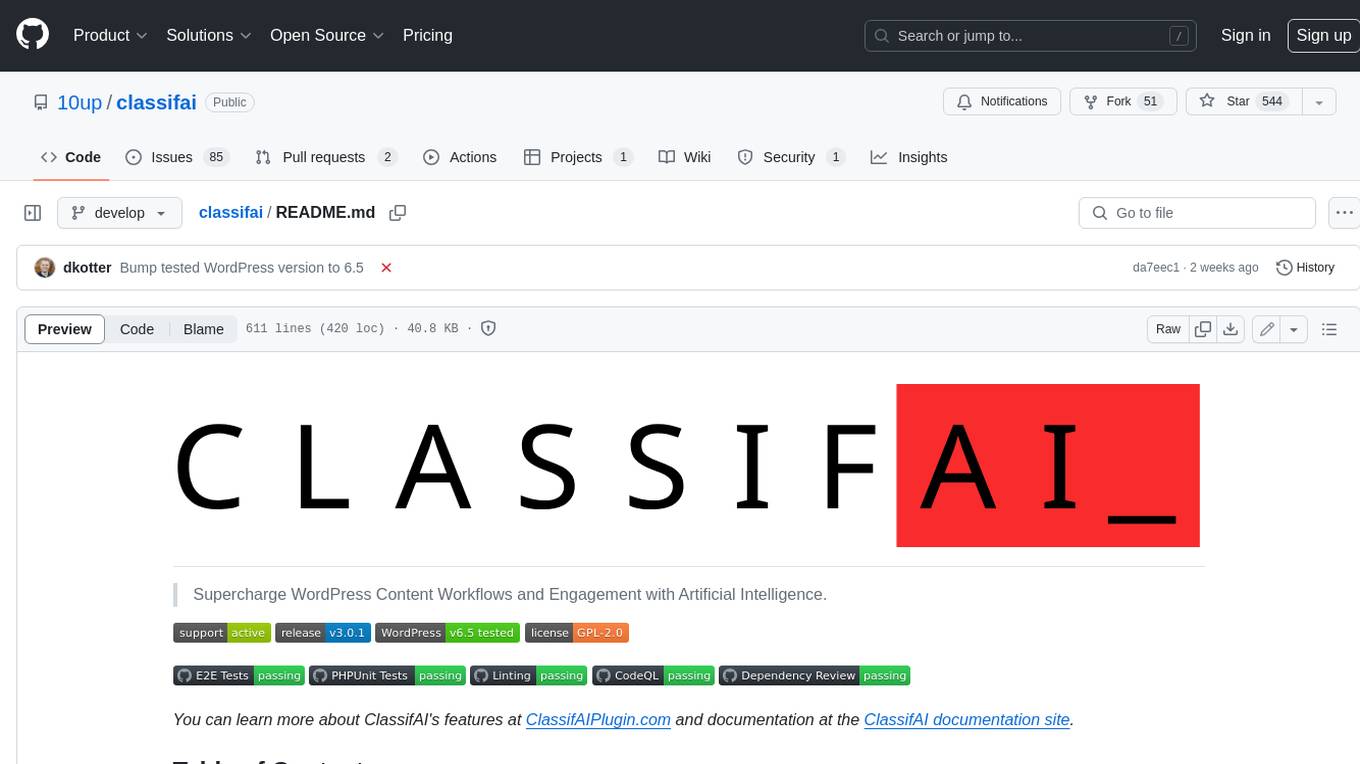
classifai
Supercharge WordPress Content Workflows and Engagement with Artificial Intelligence. Tap into leading cloud-based services like OpenAI, Microsoft Azure AI, Google Gemini and IBM Watson to augment your WordPress-powered websites. Publish content faster while improving SEO performance and increasing audience engagement. ClassifAI integrates Artificial Intelligence and Machine Learning technologies to lighten your workload and eliminate tedious tasks, giving you more time to create original content that matters.
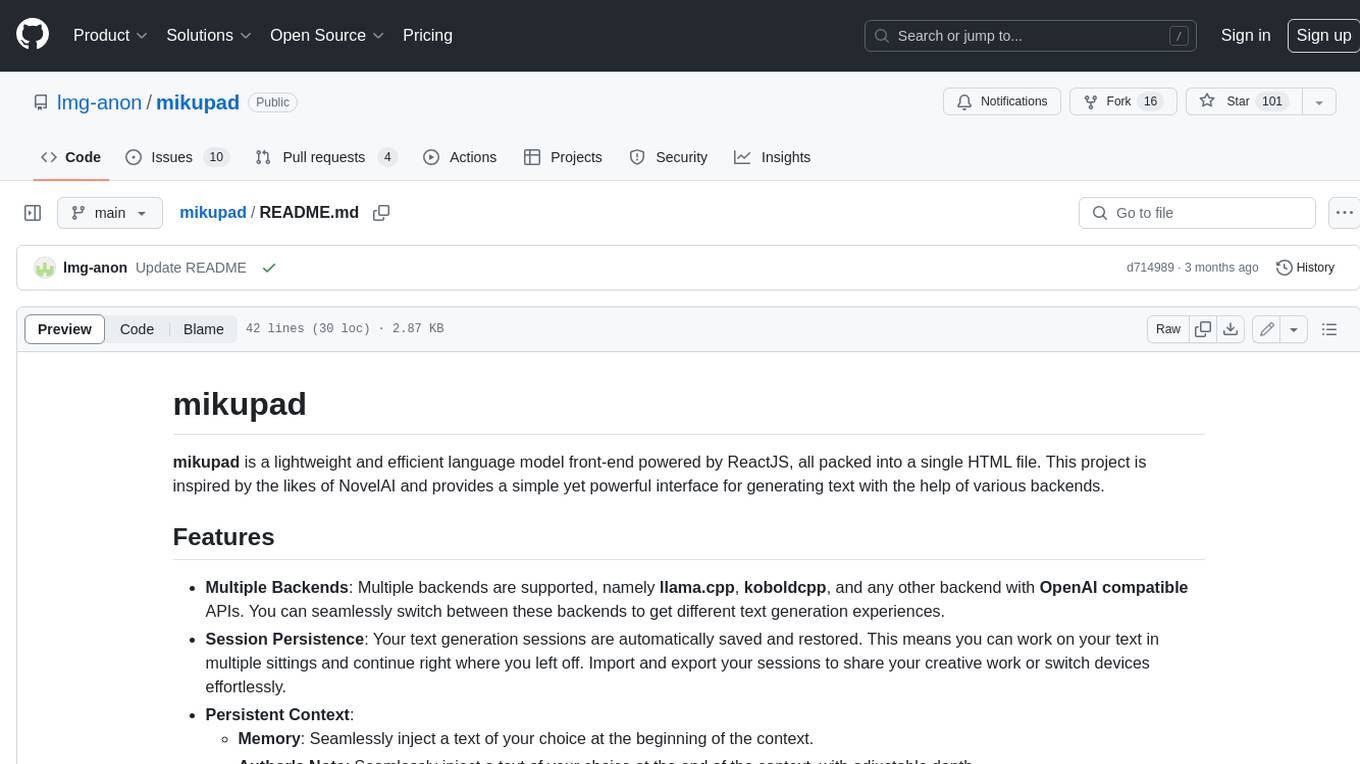
mikupad
mikupad is a lightweight and efficient language model front-end powered by ReactJS, all packed into a single HTML file. Inspired by the likes of NovelAI, it provides a simple yet powerful interface for generating text with the help of various backends.
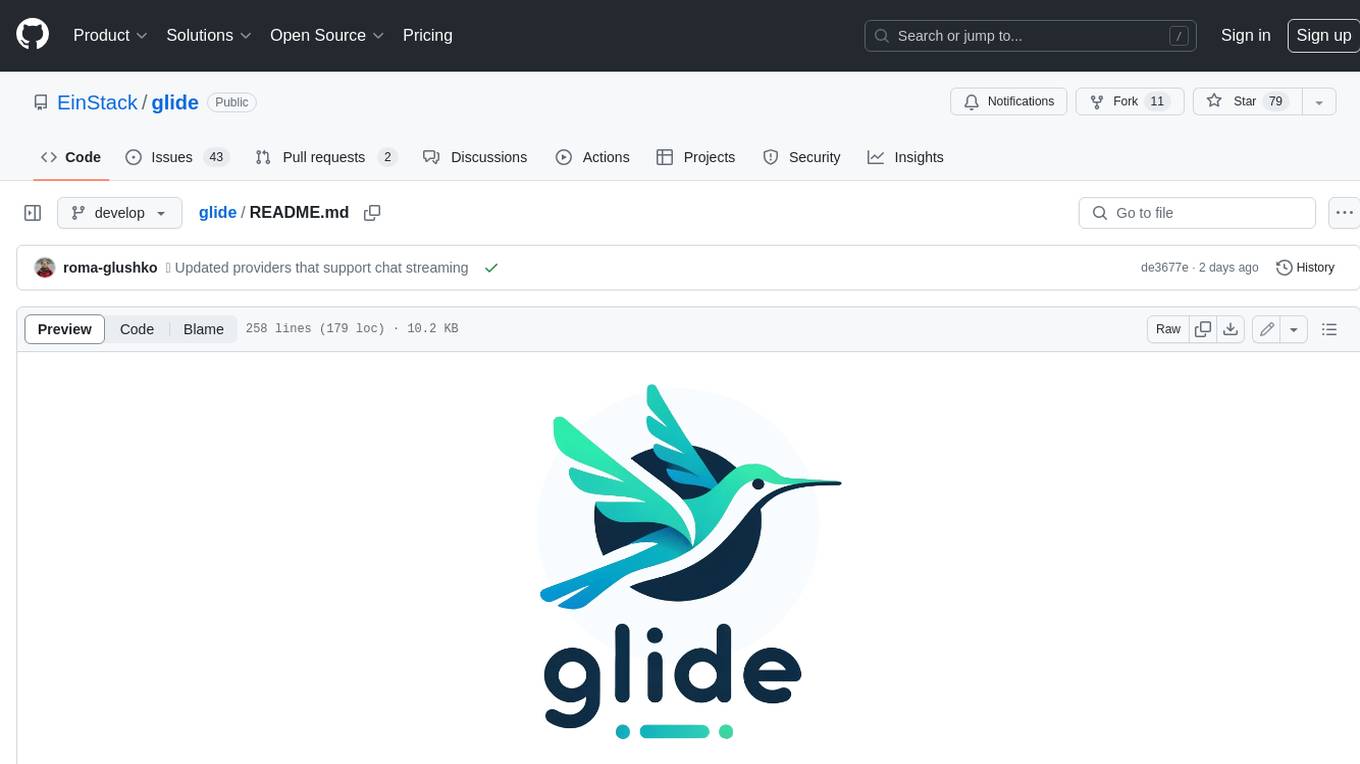
glide
Glide is a cloud-native LLM gateway that provides a unified REST API for accessing various large language models (LLMs) from different providers. It handles LLMOps tasks such as model failover, caching, key management, and more, making it easy to integrate LLMs into applications. Glide supports popular LLM providers like OpenAI, Anthropic, Azure OpenAI, AWS Bedrock (Titan), Cohere, Google Gemini, OctoML, and Ollama. It offers high availability, performance, and observability, and provides SDKs for Python and NodeJS to simplify integration.
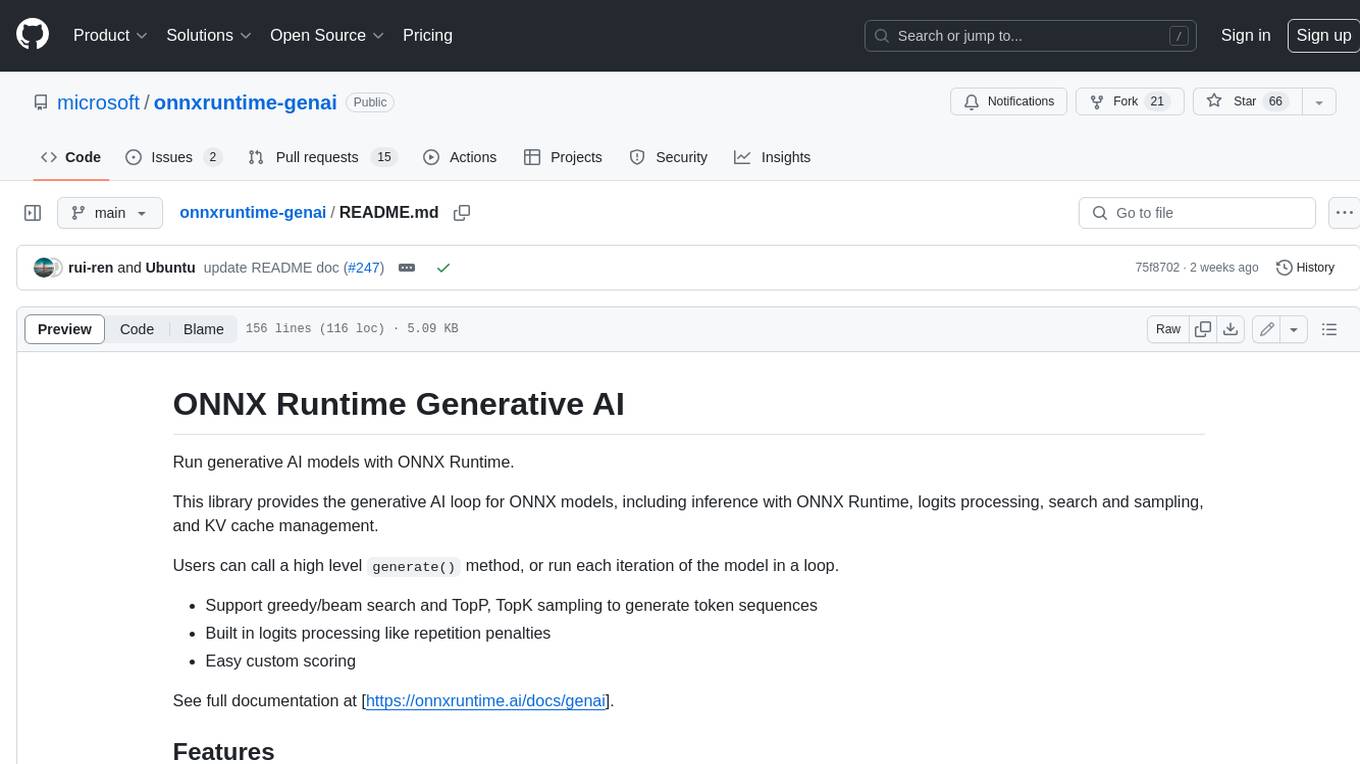
onnxruntime-genai
ONNX Runtime Generative AI is a library that provides the generative AI loop for ONNX models, including inference with ONNX Runtime, logits processing, search and sampling, and KV cache management. Users can call a high level `generate()` method, or run each iteration of the model in a loop. It supports greedy/beam search and TopP, TopK sampling to generate token sequences, has built in logits processing like repetition penalties, and allows for easy custom scoring.
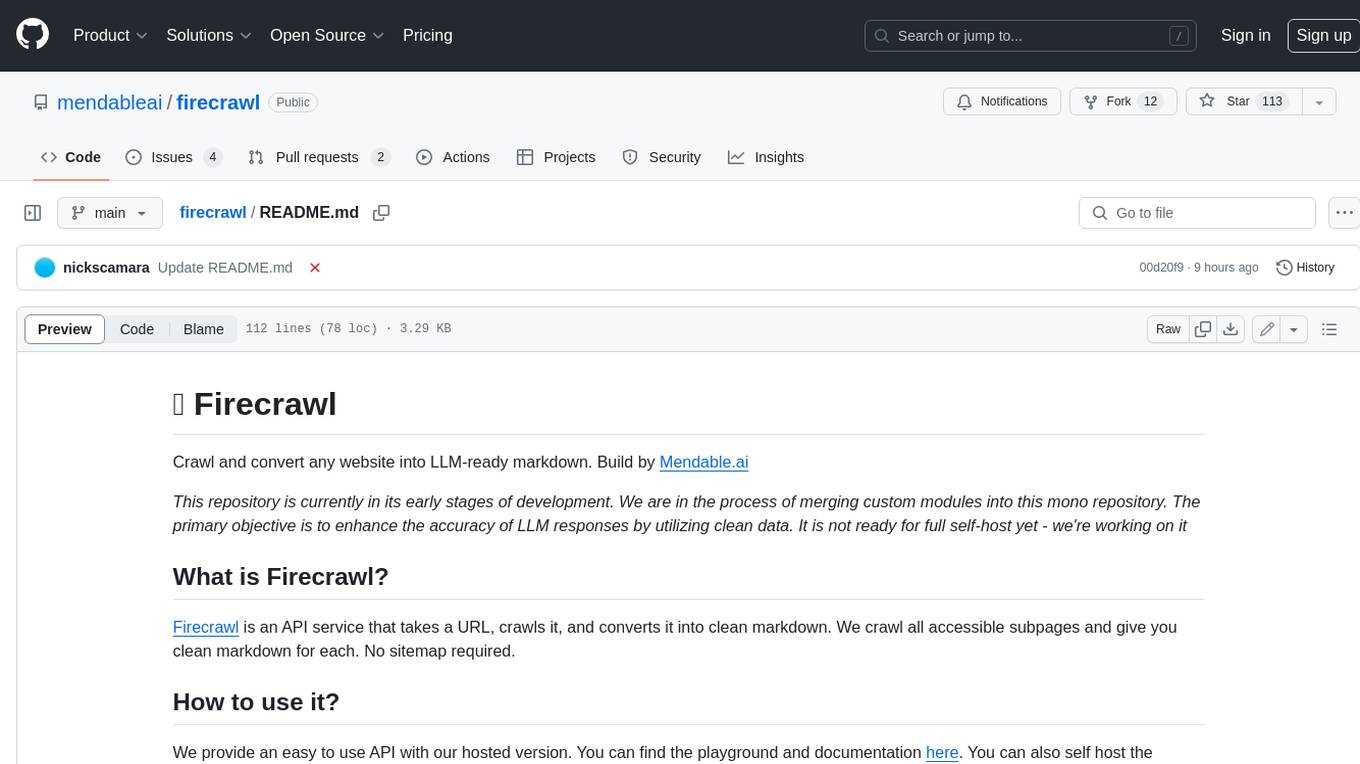
firecrawl
Firecrawl is an API service that takes a URL, crawls it, and converts it into clean markdown. It crawls all accessible subpages and provides clean markdown for each, without requiring a sitemap. The API is easy to use and can be self-hosted. It also integrates with Langchain and Llama Index. The Python SDK makes it easy to crawl and scrape websites in Python code.