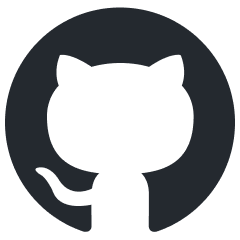
ABigSurveyOfLLMs
A collection of 150+ surveys on LLMs
Stars: 177
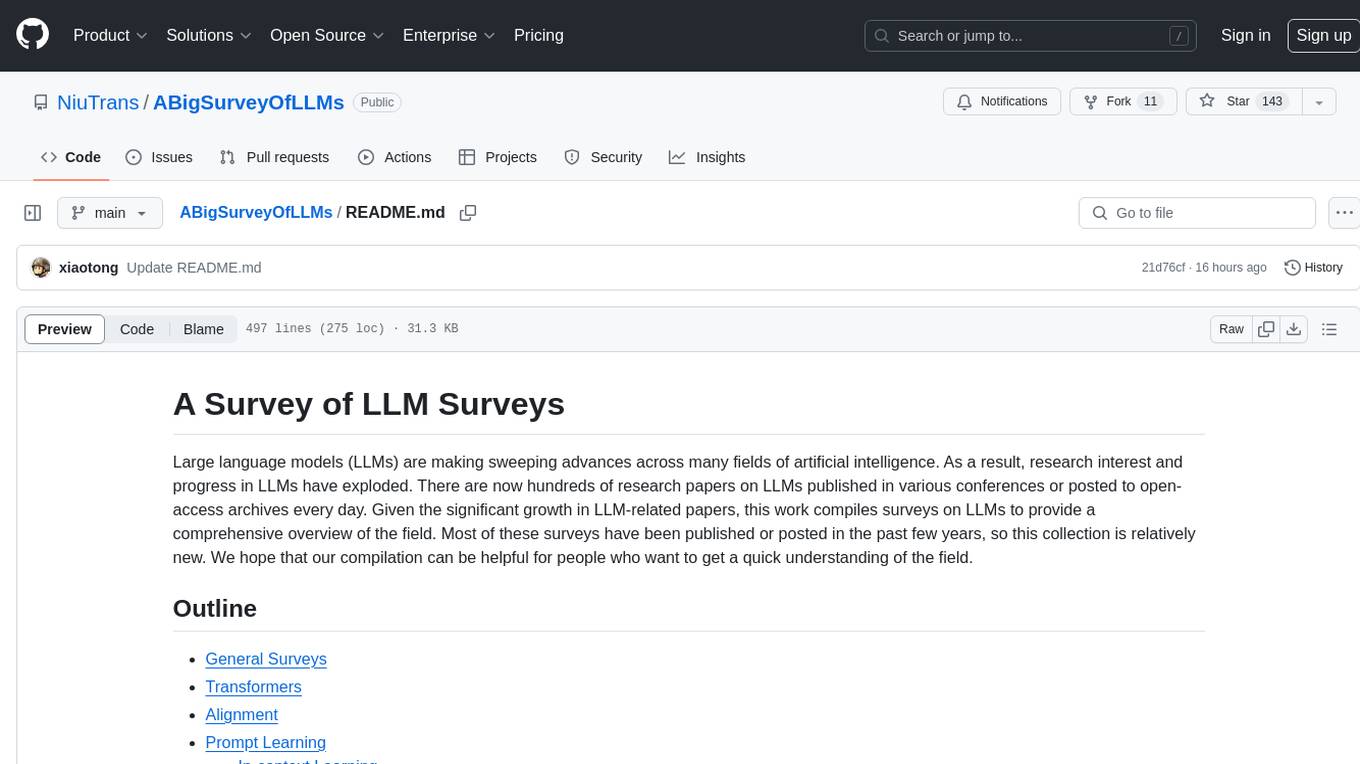
ABigSurveyOfLLMs is a repository that compiles surveys on Large Language Models (LLMs) to provide a comprehensive overview of the field. It includes surveys on various aspects of LLMs such as transformers, alignment, prompt learning, data management, evaluation, societal issues, safety, misinformation, attributes of LLMs, efficient LLMs, learning methods for LLMs, multimodal LLMs, knowledge-based LLMs, extension of LLMs, LLMs applications, and more. The repository aims to help individuals quickly understand the advancements and challenges in the field of LLMs through a collection of recent surveys and research papers.
README:
Large language models (LLMs) are making sweeping advances across many fields of artificial intelligence. As a result, research interest and progress in LLMs have exploded. There are now hundreds of research papers on LLMs published in various conferences or posted to open-access archives every day. Given the significant growth in LLM-related papers, this work compiles surveys on LLMs to provide a comprehensive overview of the field. Most of these surveys have been published or posted in the past few years, so this collection is relatively new. We hope that our compilation can be helpful for people who want to get a quick understanding of the field.
- General Surveys
- Transformers
- Alignment
- Prompt Learning
- Data
- Evaluation
- Societal Issues
- Safety
- Misinformation
- Attributes of LLMs
- Efficient LLMs
- Learning Methods for LLMs
- Multimodal LLMs
- Knowledge Based LLMs
- Extension of LLMs
- Long Sequence LLMs
- LLMs Applications
-
Large Language Models: A Survey, arXiv 2024.02 [Paper]
-
A Comprehensive Survey of AI-Generated Content (AIGC): A History of Generative AI from GAN to ChatGPT, arXiv 2023.03 [Paper]
-
A Survey of Large Language Models, arXiv 2023.11 [Paper] [GitHub]
-
Challenges and Applications of Large Language Models, arXiv 2023.07 [Paper]
-
Harnessing the Power of LLMs in Practice: A Survey on ChatGPT and Beyond, arXiv 2023.04 [Paper] [GitHub]
-
A Survey on Large Language Models: Applications, Challenges, Limitations, and Practical Usage, TechRxiv 2023.07 [Paper] [GitHub]
-
A Comprehensive Survey on Pretrained Foundation Models: A History from BERT to ChatGPT, arXiv 2023.05 [Paper]
-
A Comprehensive Overview of Large Language Models, arXiv 2023.07 [Paper] [GitHub]
-
Pre-train, Prompt, and Predict: A Systematic Survey of Prompting Methods in Natural Language Processing, ACM Computing Surveys 2023.01 [Paper]
-
A survey of transformers, arXiv 2022.10 [Paper]
-
Introduction to Transformers: an NLP Perspective, arXiv 2023.11 [Paper] [GitHub]
-
Efficient Transformers: A Survey, arXiv 2022.12 [Paper]
-
A Practical Survey on Faster and Lighter Transformers, arXiv 2023.07 [Paper]
-
Attention Mechanism, Transformers, BERT, and GPT: Tutorial and Survey, arXiv 2020.12 [Paper]
-
Bridging the Gap: A Survey on Integrating (Human) Feedback for Natural Language Generation, arXiv 2023.06 [Paper]
-
AI Alignment: A Comprehensive Survey, arXiv 2024.02 [Paper]
-
Large Language Model Alignment: A Survey, arXiv 2023.09 [Paper]
-
From Instructions to Intrinsic Human Values -- A Survey of Alignment Goals for Big Models, arXiv 2023.09 [Paper] [GitHub]
-
Aligning Large Language Models with Human: A Survey, arXiv 2023.07 [Paper] [GitHub]
-
Instruction Tuning for Large Language Models: A Survey, arXiv 2023.08 [Paper]
-
A Comprehensive Survey on Instruction Following, arXiv 2024.01 [Paper] [GitHub]
-
A Practical Survey on Zero-shot Prompt Design for In-context Learning, ranlp 2023.09 [Paper]
-
A Survey on In-context Learning, arXiv 2023.06 [Paper]
-
A Survey of Chain of Thought Reasoning: Advances, Frontiers and Future, arXiv 2023.10 [Paper] [GitHub]
-
Towards Better Chain-of-Thought Prompting Strategies: A Survey, arXiv 2023.10 [Paper]
-
Igniting Language Intelligence: The Hitchhiker's Guide From Chain-of-Thought Reasoning to Language Agents, arXiv 2023.11 [Paper] [GitHub]
-
Prompting Frameworks for Large Language Models: A Survey, arXiv 2023.11 [Paper] [GitHub]
-
Unleashing the potential of prompt engineering in Large Language Models: a comprehensive review, arXiv 2023.10 [Paper]
-
Towards Reasoning in Large Language Models: A Survey, arXiv 2022.12 [Paper] [GitHub]
-
A Survey of Reasoning with Foundation Models, arXiv 2023.12 [Paper] [GitHub]
-
Data Management For Large Language Models: A Survey, arXiv 2023.12 [Paper] [GitHub]
-
A Survey on Data Selection for Language Models, arXiv 2024.02 [Paper]
-
Datasets for Large Language Models: A Comprehensive Survey, arXiv 2024.02 [Paper] [GitHub]
-
Large Language Models for Data Annotation: A Survey, arXiv 2024.02 [Paper] [GitHub]
-
A Survey on Data Selection for LLM Instruction Tuning, arXiv 2024.02 [Paper]
-
A Survey on Knowledge Distillation of Large Language Models, arXiv 2024.02 [Paper]
-
Evaluating Large Language Models: A Comprehensive Survey, arXiv 2023.10 [Paper] [GitHub]
-
A Survey on Evaluation of Large Language Models, arXiv 2023.07 [Paper] [GitHub]
-
Baby steps in evaluating the capacities of large language models, arXiv 2023.06 [Paper]
-
A Survey on Fairness in Large Language Models, arXiv 2023.08 [Paper]
-
Large Language Models as Subpopulation Representative Models: A Review, arXiv 2023.10 [Paper]
-
Perception, performance, and detectability of conversational artificial intelligence across 32 university courses, SCI REP-UK 2023.08 [Paper]
-
Should chatgpt be biased? challenges and risks of bias in large language models, arXiv 2023.04 [Paper]
-
Bias and Fairness in Large Language Models: A Survey, arXiv 2023.09 [Paper] [GitHub]
-
A Survey on Detection of LLMs-Generated Content, arXiv 2023.10 [Paper] [GitHub]
-
A Survey on LLM-generated Text Detection: Necessity, Methods, and Future Directions, arXiv 2023.10 [Paper] [GitHub]
-
Detecting ChatGPT: A Survey of the State of Detecting ChatGPT-Generated Text, arXiv 2023.09 [Paper]
-
The Science of Detecting LLM-Generated Texts, arXiv 2023.02 [Paper]
-
Security and Privacy Challenges of Large Language Models: A Survey, arXiv 2024.01 [Paper]
-
Survey of Vulnerabilities in Large Language Models Revealed by Adversarial Attacks, arXiv 2023.10 [Paper]
-
A Survey on Large Language Model (LLM) Security and Privacy: The Good, the Bad, and the Ugly, arXiv 2023.12 [Paper]
-
Tricking LLMs into Disobedience: Formalizing, Analyzing, and Detecting Jailbreaks, arXiv 2023.05 [Paper]
-
A Survey of Safety and Trustworthiness of Large Language Models through the Lens of Verification and Validation, arXiv 2023.05 [Paper]
-
Can Knowledge Graphs Reduce Hallucinations in LLMs? : A Survey, arXiv 2023.11 [Paper]
-
A Survey on Hallucination in Large Language Models: Principles, Taxonomy, Challenges, and Open Questions, arXiv 2023.11 [Paper] [GitHub]
-
A Survey of Hallucination in “Large” Foundation Models, arXiv 2023.09 [Paper] [GitHub]
-
Siren's Song in the AI Ocean: A Survey on Hallucination in Large Language Models, arXiv 2023.09 [Paper] [GitHub]
-
Cognitive Mirage: A Review of Hallucinations in Large Language Models, arXiv 2023.09 [Paper] [GitHub]
-
Augmenting LLMs with Knowledge: A survey on hallucination prevention, arXiv 2023.09 [Paper]
-
A Comprehensive Survey of Hallucination Mitigation Techniques in Large Language Models, arXiv 2024.01 [Paper]
-
Trustworthy LLMs: a Survey and Guideline for Evaluating Large Language Models' Alignment, arXiv 2023.08 [Paper]
-
Survey on Factuality in Large Language Models: Knowledge, Retrieval and Domain-Specificity, arXiv 2023.10 [Paper] [GitHub]
-
Give Me the Facts! A Survey on Factual Knowledge Probing in Pre-trained Language Models, arXiv 2023.10 [Paper]
-
Explainability for Large Language Models: A Survey, arXiv 2023.09 [Paper]
-
The Mystery and Fascination of LLMs: A Comprehensive Survey on the Interpretation and Analysis of Emergent Abilitie, arXiv 2023.11 [Paper]
-
From Understanding to Utilization: A Survey on Explainability for Large Language Models, arXiv 2024.01 [Paper]
-
A Survey of Large Language Models Attribution, arXiv 2023.11 [Paper] [GitHub]
-
A Survey of Language Model Confidence Estimation and Calibration, arXiv 2023.11 [Paper]
-
Shortcut Learning of Large Language Models in Natural Language Understanding, COMMUN ACM 2023.12 [Paper]
-
Automatically Correcting Large Language Models: Surveying the landscape of diverse self-correction strategies, arXiv 2023.08 [Paper] [GitHub]
-
Efficient Large Language Models: A Survey, arXiv 2023.12 [Paper] [GitHub]
-
LLM Inference Unveiled: Survey and Roofline Model Insights, arXiv 2024.03 [Paper]
-
Towards Efficient Generative Large Language Model Serving: A Survey from Algorithms to Systems, arXiv 2023.12 [Paper]
-
A Survey on Model Compression for Large Language Models, arXiv 2023.08 [Paper]
-
A Comprehensive Survey of Compression Algorithms for Language Models, arXiv 2024.01 [Paper]
-
The Efficiency Spectrum of Large Language Models: An Algorithmic Survey, arXiv 2023.10 [Paper] [GitHub]
-
Parameter-Efficient Fine-Tuning Methods for Pretrained Language Models: A Critical Review and Assessment, arXiv 2023.12 [Paper]
-
Model Compression and Efficient Inference for Large Language Models: A Survey, arXiv 2024.02 [Paper]
-
Unlocking Efficiency in Large Language Model Inference: A Comprehensive Survey of Speculative Decoding, arXiv 2024.01 [Paper] [GitHub]
-
A Survey on Hardware Accelerators for Large Language Models, arXiv 2024.01 [Paper]
-
Knowledge Unlearning for LLMs: Tasks, Methods, and Challenges, arXiv 2023.11 [Paper]
-
Continual Learning with Pre-Trained Models: A Survey, arXiv 2024.01 [Paper] [GitHub]
-
Continual Learning for Large Language Models: A Survey, arXiv 2024.02 [Paper]
-
Scaling Down to Scale Up: A Guide to Parameter-Efficient Fine-Tuning, arXiv 2023.03 [Paper]
-
Vision-Language Instruction Tuning: A Review and Analysis, arXiv 2023,11 [Paper] [GitHub]
-
Large Language Models Meet Computer Vision: A Brief Survey, arXiv 2023.11 [Paper]
-
Foundational Models Defining a New Era in Vision: A Survey and Outlook, arXiv 2023.07 [Paper] [GitHub]
-
Video Understanding with Large Language Models: A Survey, arXiv 2023.12 [Paper] [GitHub]
-
Large Models for Time Series and Spatio-Temporal Data: A Survey and Outlook, arXiv 2023.10 [Paper] [GitHub]
-
Sparks of large audio models: A survey and outlook, arXiv 2023.08 [Paper] [GitHub]
-
How to Bridge the Gap between Modalities: A Comprehensive Survey on Multimodal Large Language Model, arXiv 2023.11 [Paper]
-
A Survey on Multimodal Large Language Models, arXiv 2023.06 [Paper]
-
Multimodal Large Language Models: A Survey, arXiv 2023.11 [Paper]
-
Building trust in conversational ai: A comprehensive review and solution architecture for explainable, privacy-aware systems using llms and knowledge graph, arXiv 2023.08 [Paper]
-
A Survey on Retrieval-Augmented Text Generation, arXiv 2022.02 [Paper]
-
Retrieval-Augmented Generation for Large Language Models: A Survey, arXiv 2023.12 [Paper] [GitHub]
-
Trends in Integration of Knowledge and Large Language Models: A Survey and Taxonomy of Methods, Benchmarks, and Applications, arXiv 2023.11 [Paper]
-
Knowledge Editing for Large Language Models: A Survey, arXiv 2023.10 [Paper]
-
Editing Large Language Models: Problems, Methods, and Opportunities, arXiv 2023.05 [Paper]
-
A Survey of Neural Code Intelligence: Paradigms, Advances and Beyond, arXiv 2024.03 [Paper] [GitHub]
-
Foundation Models for Decision Making: Problems, Methods, and Opportunities, arXiv 2023.03 [Paper]
-
Augmented Language Models: a Survey, arXiv 2023.02 [Paper]
-
Pitfalls in Language Models for Code Intelligence: A Taxonomy and Survey, arXiv 2023.10 [Paper] [GitHub]
-
Large Language Models Meet NL2Code: A Survey, arXiv 2022.12 [Paper]
-
Large Language Models for Robotics: A Survey, arXiv 2023.11 [Paper]
-
A Survey on Multimodal Large Language Models for Autonomous Driving, WACV workshop 2023.11 [Paper]
-
LLM4Drive: A Survey of Large Language Models for Autonomous Driving, arXiv 2023.11 [Paper] [GitHub]
-
A Survey on Large Language Model based Autonomous Agents, arXiv 2023.08 [Paper] [GitHub]
-
The Rise and Potential of Large Language Model Based Agents: A Survey, arXiv 2023.09 [Paper] [GitHub]
-
Large Language Models Empowered Agent-based Modeling and Simulation: A Survey and Perspectives, arXiv 2023.12 [Paper]
-
Large Multimodal Agents: A Survey, arXiv 2024.02 [Paper] [GitHub]
-
Role play with large language models, arXiv 2023.11 [Paper]
-
Advancing Transformer Architecture in Long-Context Large Language Models: A Comprehensive Survey, arXiv 2023.11 [Paper]
-
Length Extrapolation of Transformers: A Survey from the Perspective of Position Encoding, arXiv 2023.12 [Paper]
-
ChatGPT and Beyond: The Generative AI Revolution in Education, arXiv 2023.11 [Paper]
-
ChatGPT and large language models in academia: opportunities and challenges, arXiv 2023.07 [Paper]
-
ChatGPT for good? On opportunities and challenges of large language models for education, arXiv 2023.04 [Paper]
-
Large Language Models in Law: A Survey, arXiv 2023.11 [Paper]
-
A short survey of viewing large language models in legal aspect, arXiv 2023.03 [Paper]
-
A Survey of Large Language Models in Medicine: Progress, Application, and Challenge, arXiv 2023.11 [Paper] [GitHub]
-
Large Language Models Illuminate a Progressive Pathway to Artificial Healthcare Assistant: A Review, arXiv 2023.11 [Paper] [GitHub]
-
Large AI Models in Health Informatics: Applications, Challenges, and the Future, arXiv 2023.03 [Paper] [GitHub]
-
A SWOT (Strengths, Weaknesses, Opportunities, and Threats) Analysis of ChatGPT in the Medical Literature: Concise Review, JMIR 2023.11 [Paper]
-
ChatGPT in Healthcare: A Taxonomy and Systematic Review, Computer Methods and Programs in Biomedicine 2024.01 [Paper]
-
A review of the explainability and safety of conversational agents for mental health to identify avenues for improvement, NCBI 2023.10 [Paper]
-
Towards a Psychological Generalist AI: A Survey of Current Applications of Large Language Models and Future Prospects, arXiv 2023.12 [Paper]
-
Large Language Models in Mental Health Care: a Scoping Review, arXiv 2024.01 [Paper]
-
The utility of ChatGPT as an example of large language models in healthcare education, research and practice: Systematic review on the future perspectives and, arXiv 2023.12 [Paper]
-
The imperative for regulatory oversight of large language models (or generative AI) in healthcare, arXiv 2023.07 [Paper]
-
A Survey of Large Language Models for Healthcare: from Data, Technology, and Applications to Accountability and Ethics, arXiv 2023.10 [Paper] [GitHub]
-
The Shaky Foundations of Clinical Foundation Models: A Survey of Large Language Models and Foundation Models for EMRs, arXiv 2023.03 [Paper]
-
Large Language Models and Games: A Survey and Roadmap, arXiv 2024.02 [Paper]
-
Large Language Models and Video Games: A Preliminary Scoping Review, arXiv 2024.03 [Paper]
-
Large Language Models for Information Retrieval: A Survey, arXiv 2023.08 [Paper] [GitHub]
-
Large Language Models for Generative Information Extraction: A Survey, arXiv 2023.12 [Paper] [GitHub]
-
Recent Advances in Natural Language Processing via Large Pre-Trained Language Models: A Survey, arXiv 2021.11 [Paper]
-
If LLM Is the Wizard, Then Code Is the Wand: A Survey on How Code Empowers Large Language Models to Serve as Intelligent Agents, arXiv 2024.01 [Paper]
-
Large Language Models for Software Engineering: Survey and Open Problems, arXiv 2023.10 [Paper]
-
Large Language Models for Software Engineering: A Systematic Literature Review, arXiv 2023.08 [Paper]
-
Software Testing with Large Language Models: Survey, Landscape, and Vision, arXiv 2023.07 [Paper]
-
Unifying the Perspectives of NLP and Software Engineering: A Survey on Language Models for Code, arXiv 2024.01 [Paper] [GitHub]
-
Foundation Models for Recommender Systems: A Survey and New Perspectives, arXiv 2024.02 [Paper]
-
User Modeling in the Era of Large Language Models: Current Research and Future Directions, arXiv 2023.11 [Paper] [GitHub]
-
A Survey on Large Language Models for Personalized and Explainable Recommendations, arXiv 2023.11 [Paper]
-
Large Language Models for Generative Recommendation: A Survey and Visionary Discussions, arXiv 2023.09 [Paper]
-
A Survey on Large Language Models for Recommendation, arXiv 2023.05 [Paper] [GitHub]
-
How Can Recommender Systems Benefit from Large Language Models: A Survey, arXiv 2023.06 [Paper] [GitHub]
-
A Survey of Graph Meets Large Language Model: Progress and Future Directions, arXiv 2023.11 [Paper]
-
Large Language Models on Graphs: A Comprehensive Survey, arXiv 2023.12 [Paper] [GitHub]
-
The Contribution of Knowledge in Visiolinguistic Learning: A Survey on Tasks and Challenges, arXiv 2023.03 [Paper]
-
Large Language Models in Finance: A Survey, ICAIF 2023.11 [Paper]
-
Mathematical Language Models: A Survey, arXiv 2023.12 [Paper]
-
Recent applications of AI to environmental disciplines: A review, SCI TOTAL ENVIRON 2023.10 [Paper]
-
Opportunities and Challenges of Applying Large Language Models in Building Energy Efficiency and Decarbonization Studies: An Exploratory Overview, arXiv 2023.12 [Paper]
-
When Large Language Models Meet Citation: A Survey, arXiv 2023.09 [Paper]
-
A Survey of Text Watermarking in the Era of Large Language Models, arXiv 2023.12 [Paper]
-
The future of gpt: A taxonomy of existing chatgpt research, current challenges, and possible future directions, SSRN 2023.04 [Paper]
-
Summary of ChatGPT-Related Research and Perspective Towards the Future of Large Language Models, Meta-Radiology 2023.09 [Paper]
We would like to thank the people who have contributed to this project. The core contributors are
Junhao Ruan, Long Meng, Weiqiao Shan, Tong Xiao, Jingbo Zhu
For Tasks:
Click tags to check more tools for each tasksFor Jobs:
Alternative AI tools for ABigSurveyOfLLMs
Similar Open Source Tools
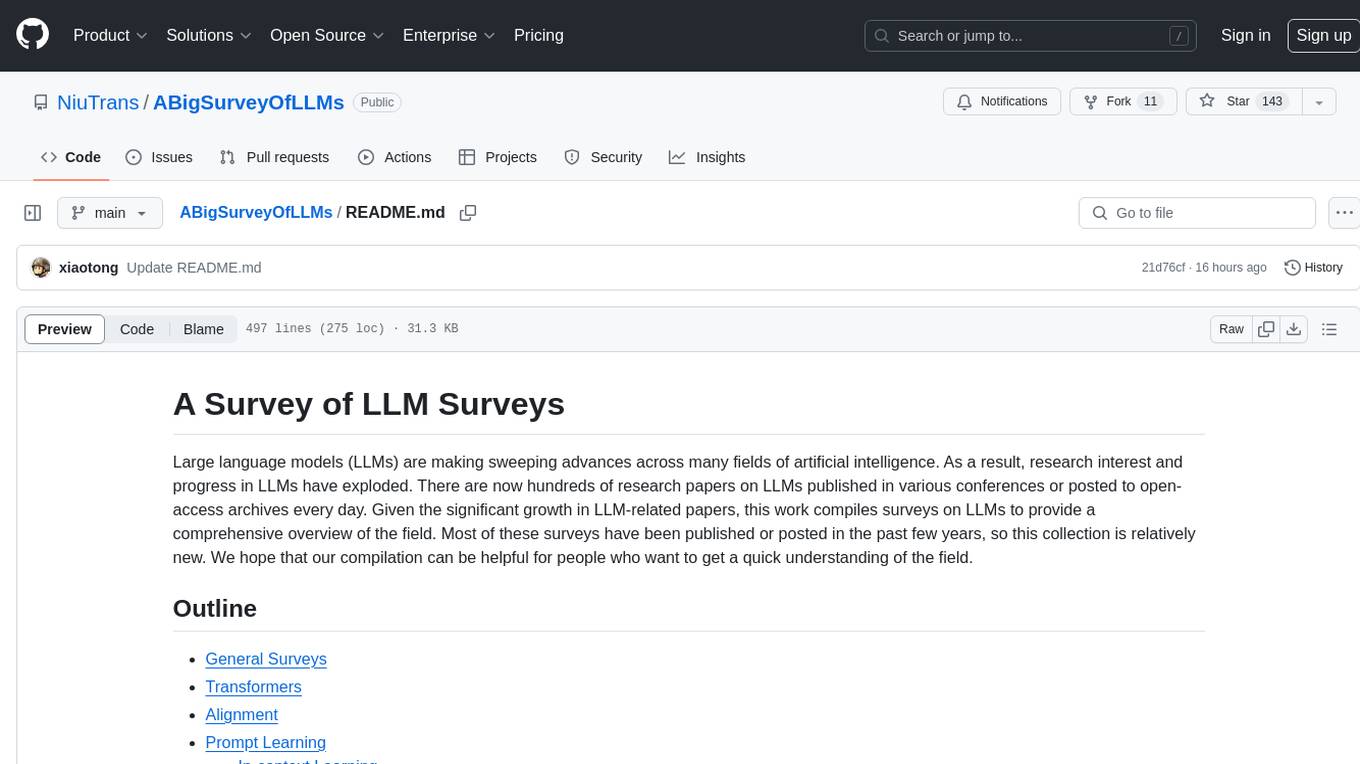
ABigSurveyOfLLMs
ABigSurveyOfLLMs is a repository that compiles surveys on Large Language Models (LLMs) to provide a comprehensive overview of the field. It includes surveys on various aspects of LLMs such as transformers, alignment, prompt learning, data management, evaluation, societal issues, safety, misinformation, attributes of LLMs, efficient LLMs, learning methods for LLMs, multimodal LLMs, knowledge-based LLMs, extension of LLMs, LLMs applications, and more. The repository aims to help individuals quickly understand the advancements and challenges in the field of LLMs through a collection of recent surveys and research papers.
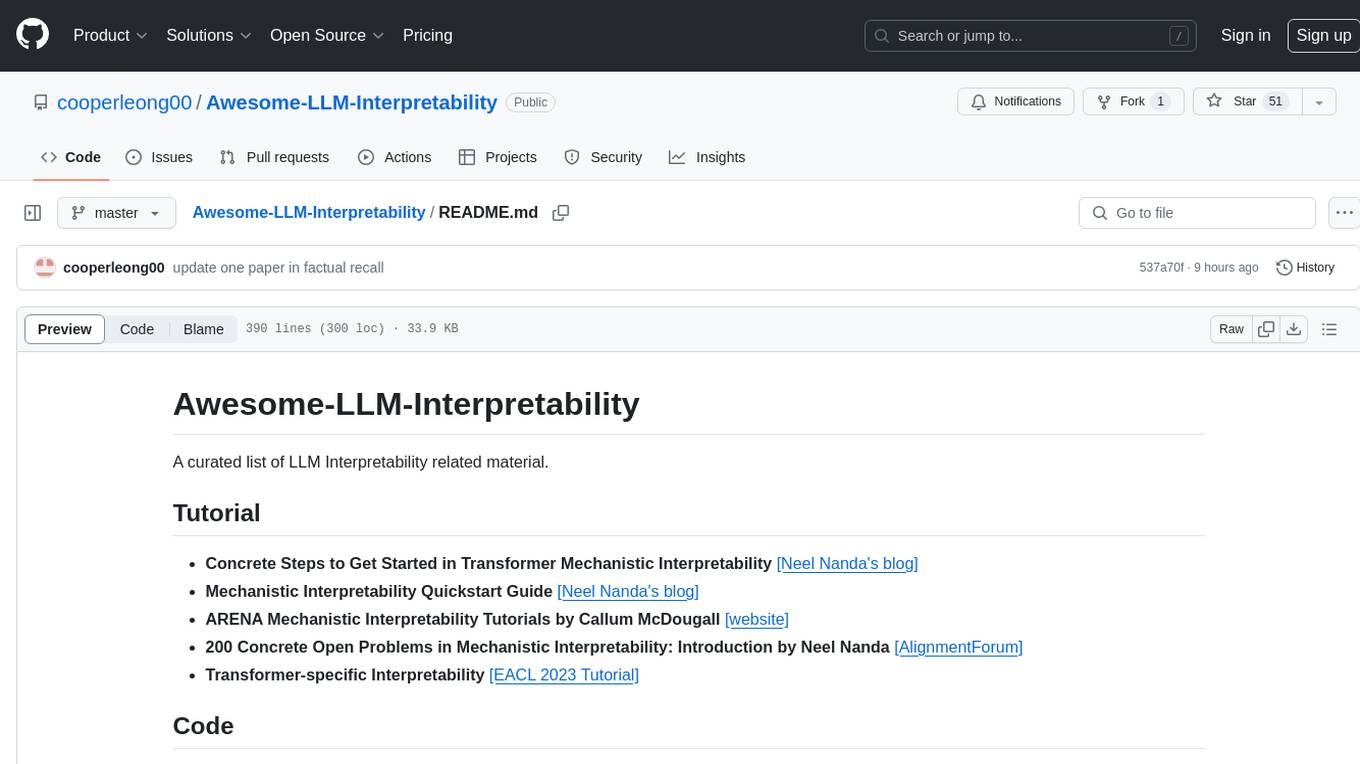
Awesome-LLM-Interpretability
Awesome-LLM-Interpretability is a curated list of materials related to LLM (Large Language Models) interpretability, covering tutorials, code libraries, surveys, videos, papers, and blogs. It includes resources on transformer mechanistic interpretability, visualization, interventions, probing, fine-tuning, feature representation, learning dynamics, knowledge editing, hallucination detection, and redundancy analysis. The repository aims to provide a comprehensive overview of tools, techniques, and methods for understanding and interpreting the inner workings of large language models.
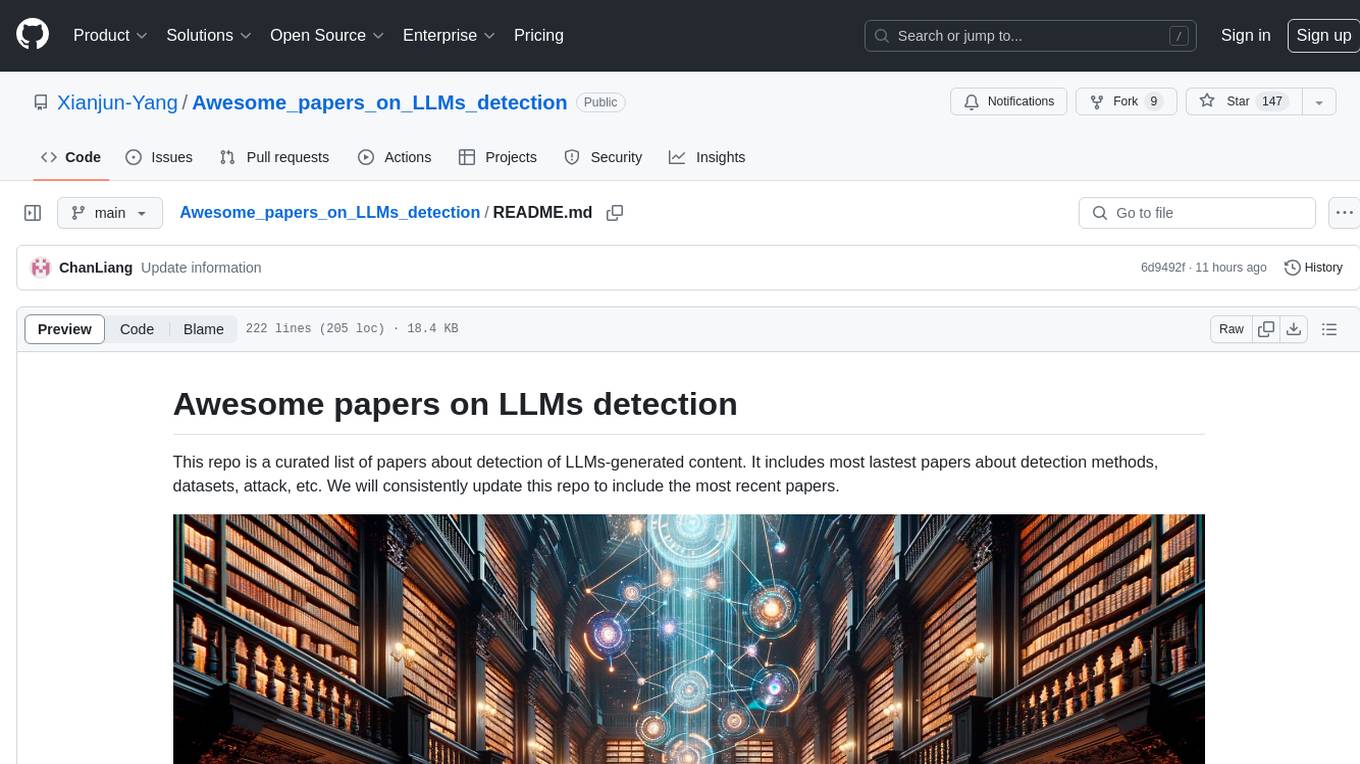
Awesome_papers_on_LLMs_detection
This repository is a curated list of papers focused on the detection of Large Language Models (LLMs)-generated content. It includes the latest research papers covering detection methods, datasets, attacks, and more. The repository is regularly updated to include the most recent papers in the field.
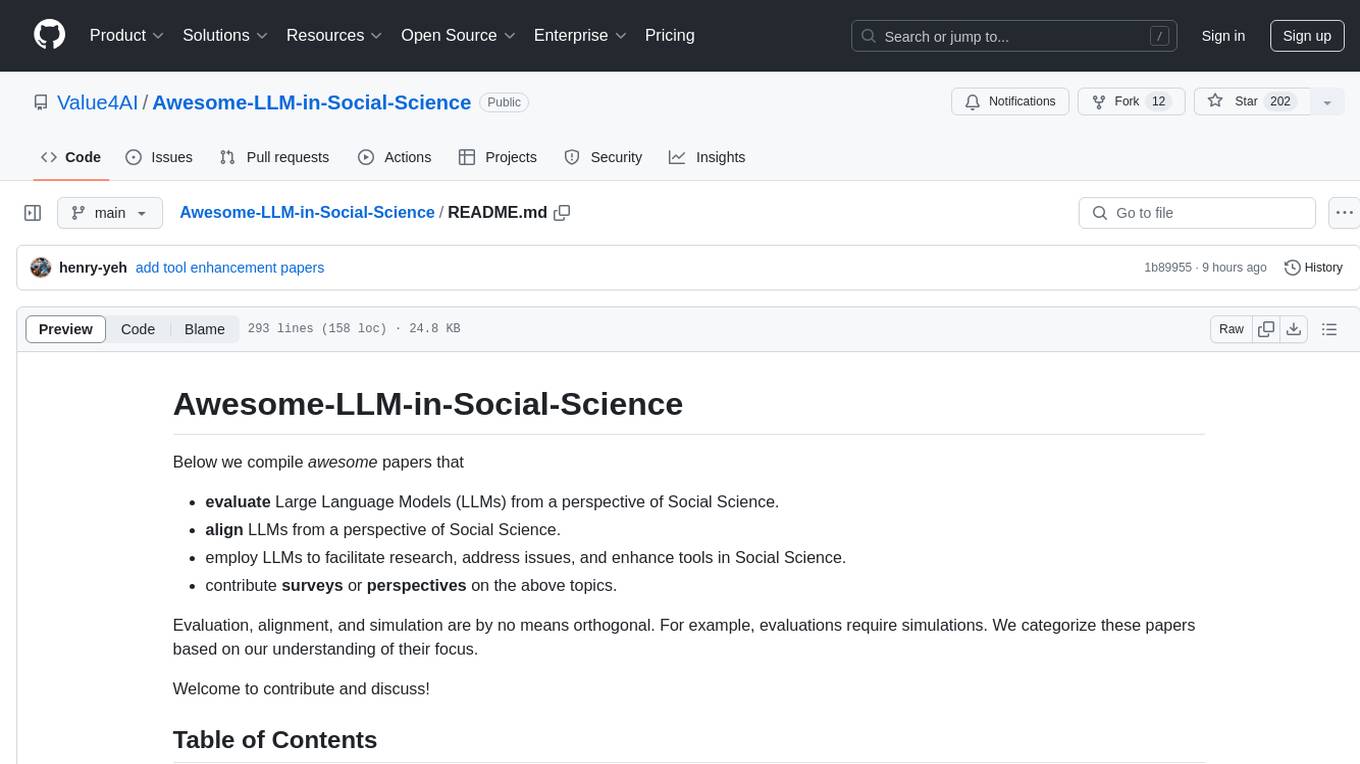
Awesome-LLM-in-Social-Science
Awesome-LLM-in-Social-Science is a repository that compiles papers evaluating Large Language Models (LLMs) from a social science perspective. It includes papers on evaluating, aligning, and simulating LLMs, as well as enhancing tools in social science research. The repository categorizes papers based on their focus on attitudes, opinions, values, personality, morality, and more. It aims to contribute to discussions on the potential and challenges of using LLMs in social science research.
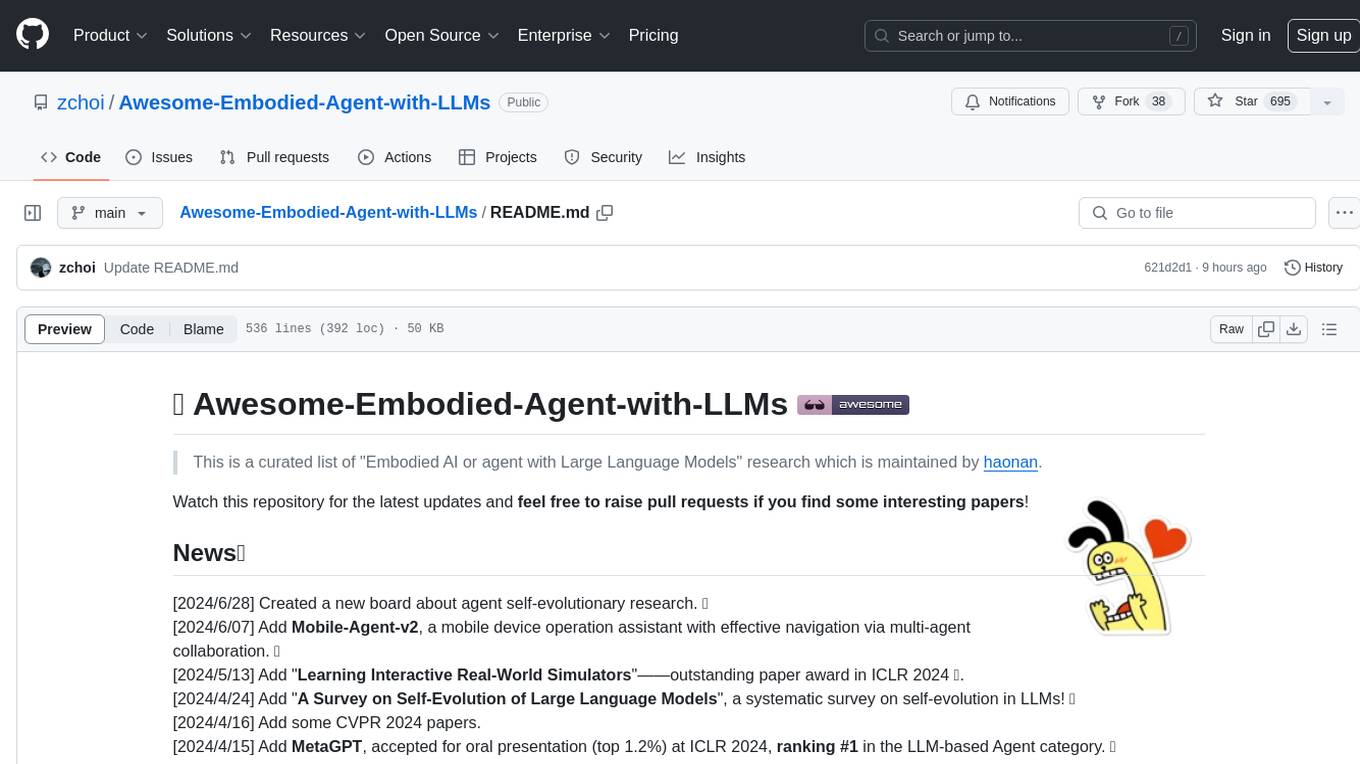
Awesome-Embodied-Agent-with-LLMs
This repository, named Awesome-Embodied-Agent-with-LLMs, is a curated list of research related to Embodied AI or agents with Large Language Models. It includes various papers, surveys, and projects focusing on topics such as self-evolving agents, advanced agent applications, LLMs with RL or world models, planning and manipulation, multi-agent learning and coordination, vision and language navigation, detection, 3D grounding, interactive embodied learning, rearrangement, benchmarks, simulators, and more. The repository provides a comprehensive collection of resources for individuals interested in exploring the intersection of embodied agents and large language models.
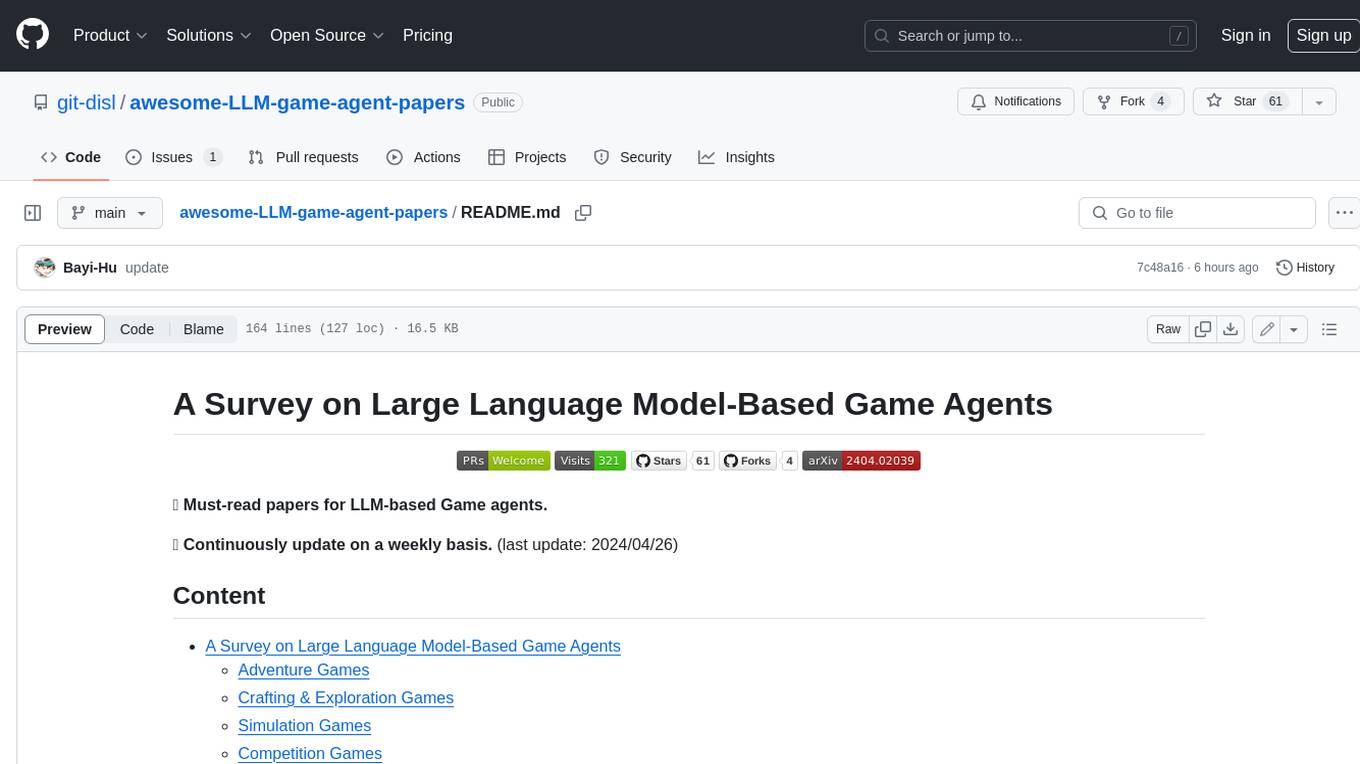
awesome-LLM-game-agent-papers
This repository provides a comprehensive survey of research papers on large language model (LLM)-based game agents. LLMs are powerful AI models that can understand and generate human language, and they have shown great promise for developing intelligent game agents. This survey covers a wide range of topics, including adventure games, crafting and exploration games, simulation games, competition games, cooperation games, communication games, and action games. For each topic, the survey provides an overview of the state-of-the-art research, as well as a discussion of the challenges and opportunities for future work.
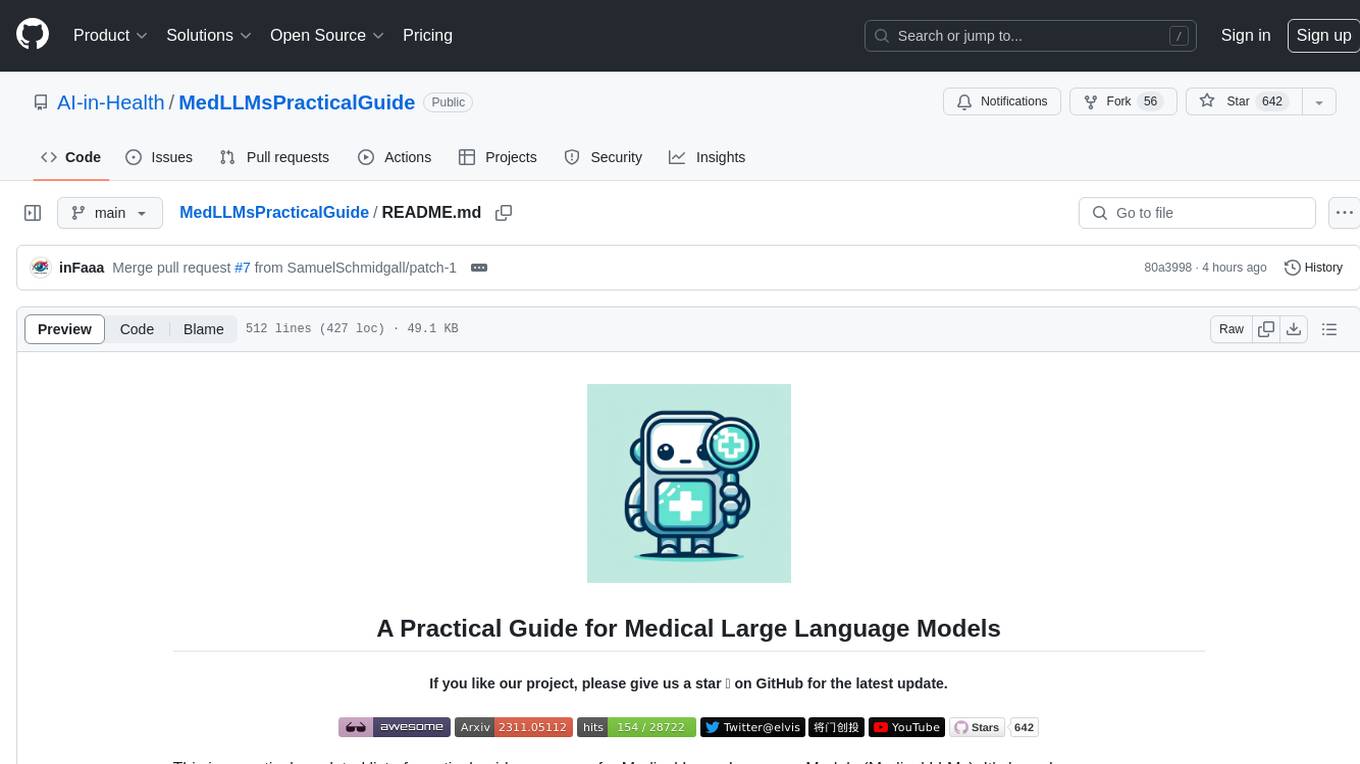
MedLLMsPracticalGuide
This repository serves as a practical guide for Medical Large Language Models (Medical LLMs) and provides resources, surveys, and tools for building, fine-tuning, and utilizing LLMs in the medical domain. It covers a wide range of topics including pre-training, fine-tuning, downstream biomedical tasks, clinical applications, challenges, future directions, and more. The repository aims to provide insights into the opportunities and challenges of LLMs in medicine and serve as a practical resource for constructing effective medical LLMs.
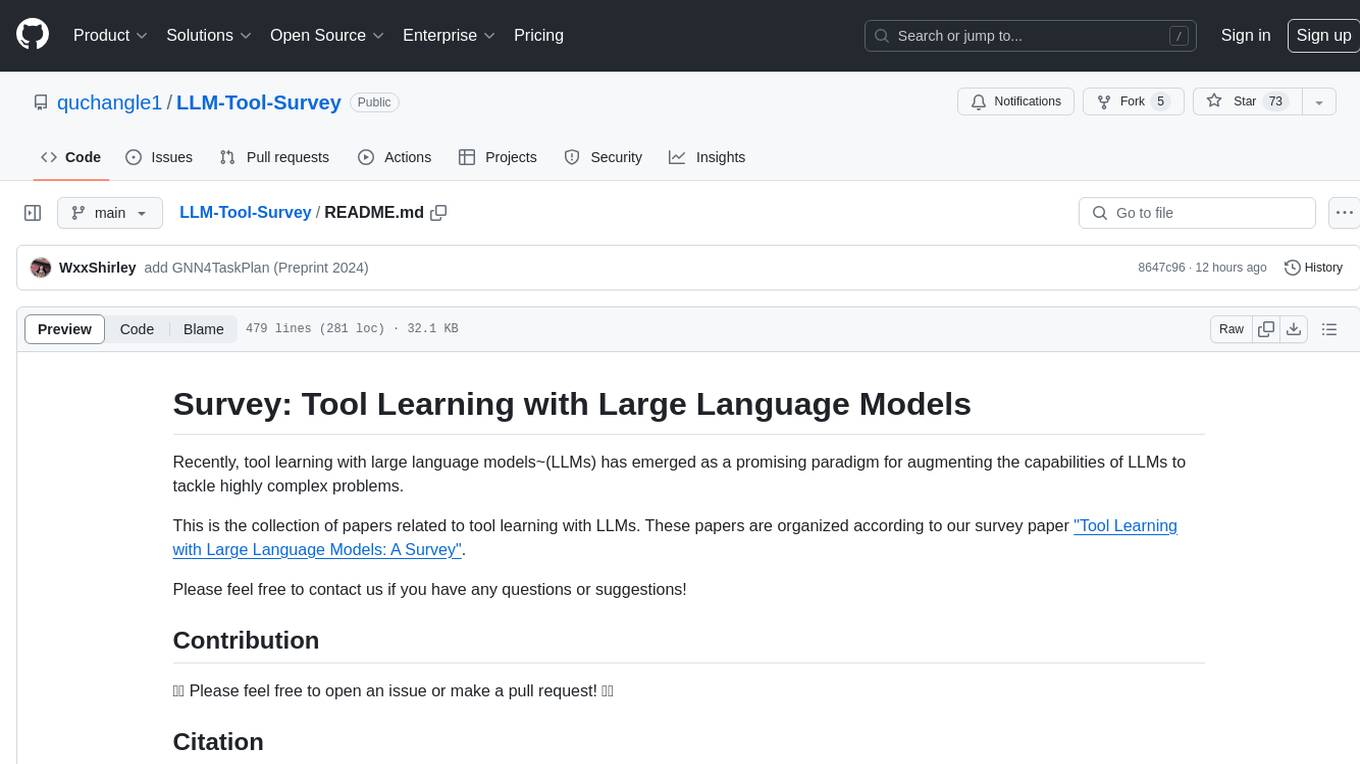
LLM-Tool-Survey
This repository contains a collection of papers related to tool learning with large language models (LLMs). The papers are organized according to the survey paper 'Tool Learning with Large Language Models: A Survey'. The survey focuses on the benefits and implementation of tool learning with LLMs, covering aspects such as task planning, tool selection, tool calling, response generation, benchmarks, evaluation, challenges, and future directions in the field. It aims to provide a comprehensive understanding of tool learning with LLMs and inspire further exploration in this emerging area.
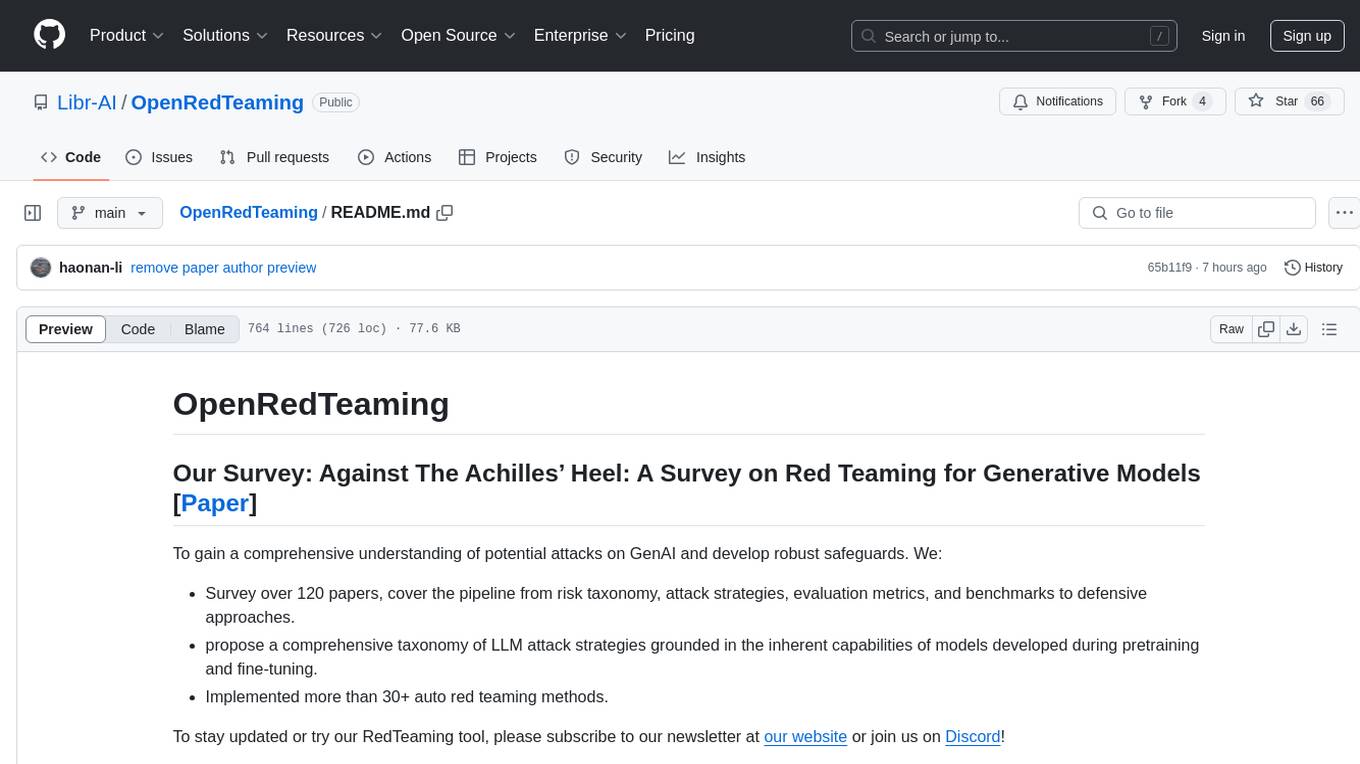
OpenRedTeaming
OpenRedTeaming is a repository focused on red teaming for generative models, specifically large language models (LLMs). The repository provides a comprehensive survey on potential attacks on GenAI and robust safeguards. It covers attack strategies, evaluation metrics, benchmarks, and defensive approaches. The repository also implements over 30 auto red teaming methods. It includes surveys, taxonomies, attack strategies, and risks related to LLMs. The goal is to understand vulnerabilities and develop defenses against adversarial attacks on large language models.
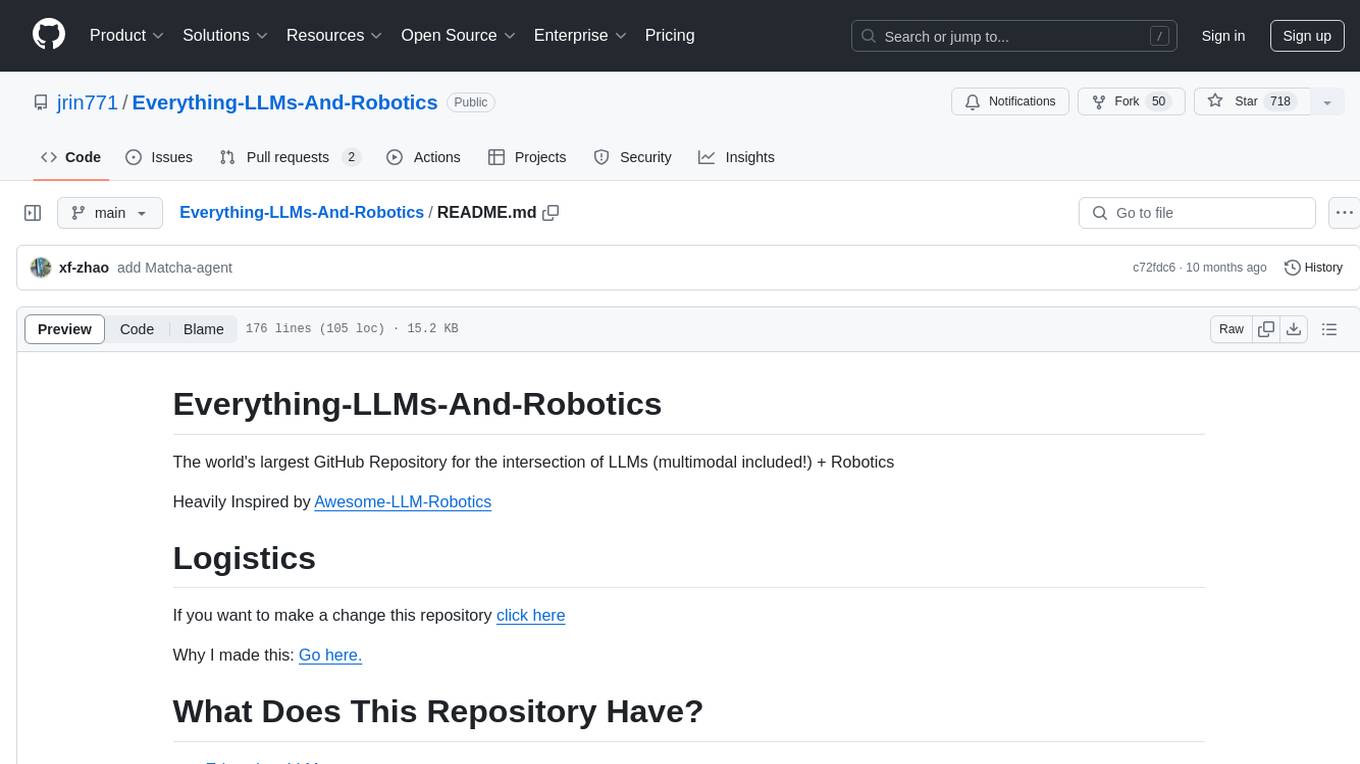
Everything-LLMs-And-Robotics
The Everything-LLMs-And-Robotics repository is the world's largest GitHub repository focusing on the intersection of Large Language Models (LLMs) and Robotics. It provides educational resources, research papers, project demos, and Twitter threads related to LLMs, Robotics, and their combination. The repository covers topics such as reasoning, planning, manipulation, instructions and navigation, simulation frameworks, perception, and more, showcasing the latest advancements in the field.
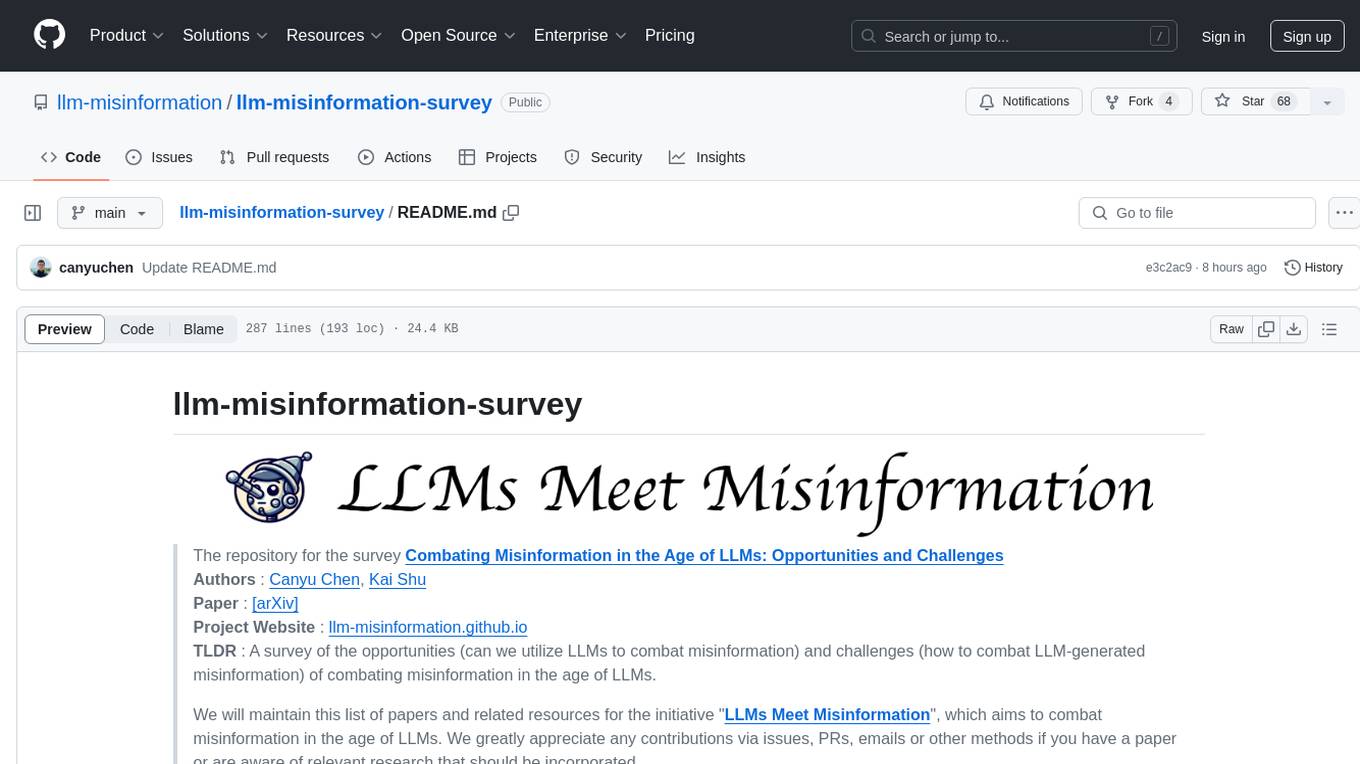
llm-misinformation-survey
The 'llm-misinformation-survey' repository is dedicated to the survey on combating misinformation in the age of Large Language Models (LLMs). It explores the opportunities and challenges of utilizing LLMs to combat misinformation, providing insights into the history of combating misinformation, current efforts, and future outlook. The repository serves as a resource hub for the initiative 'LLMs Meet Misinformation' and welcomes contributions of relevant research papers and resources. The goal is to facilitate interdisciplinary efforts in combating LLM-generated misinformation and promoting the responsible use of LLMs in fighting misinformation.
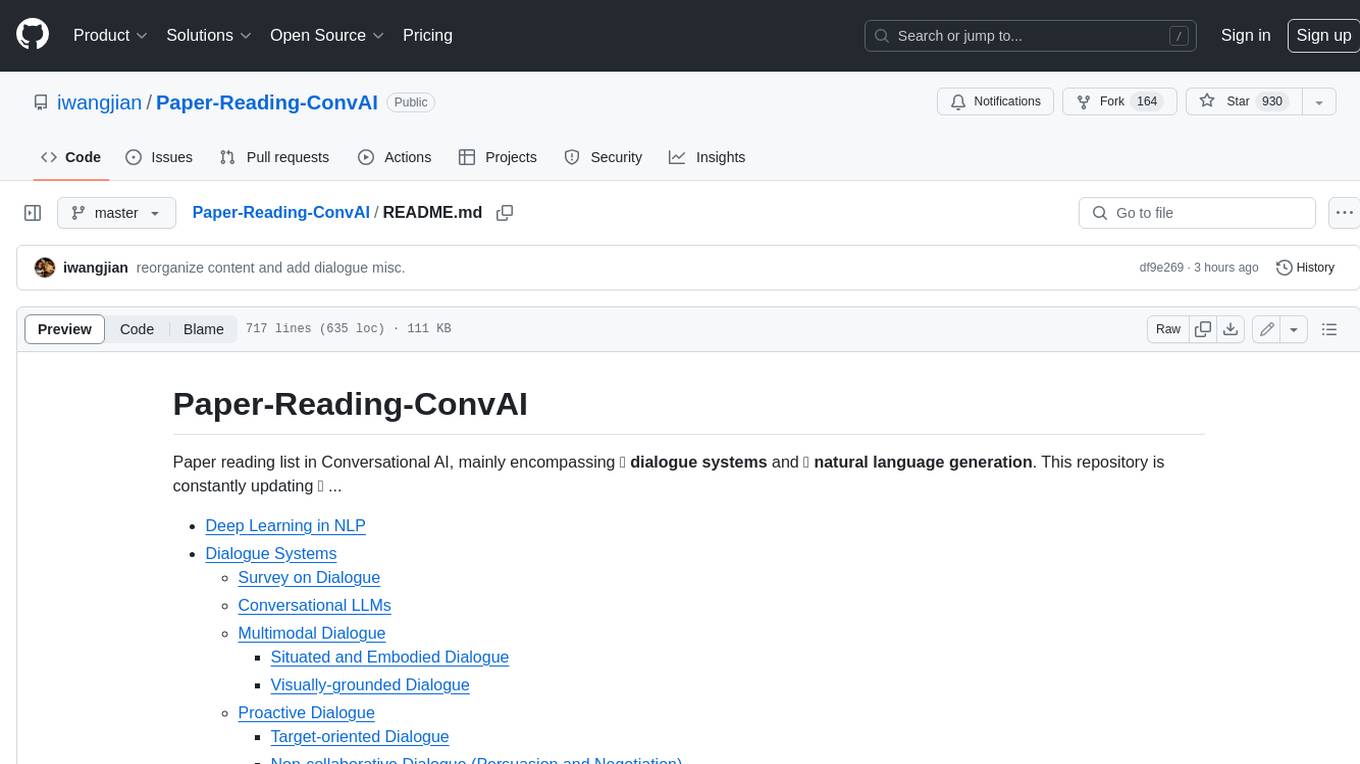
Paper-Reading-ConvAI
Paper-Reading-ConvAI is a repository that contains a list of papers, datasets, and resources related to Conversational AI, mainly encompassing dialogue systems and natural language generation. This repository is constantly updating.
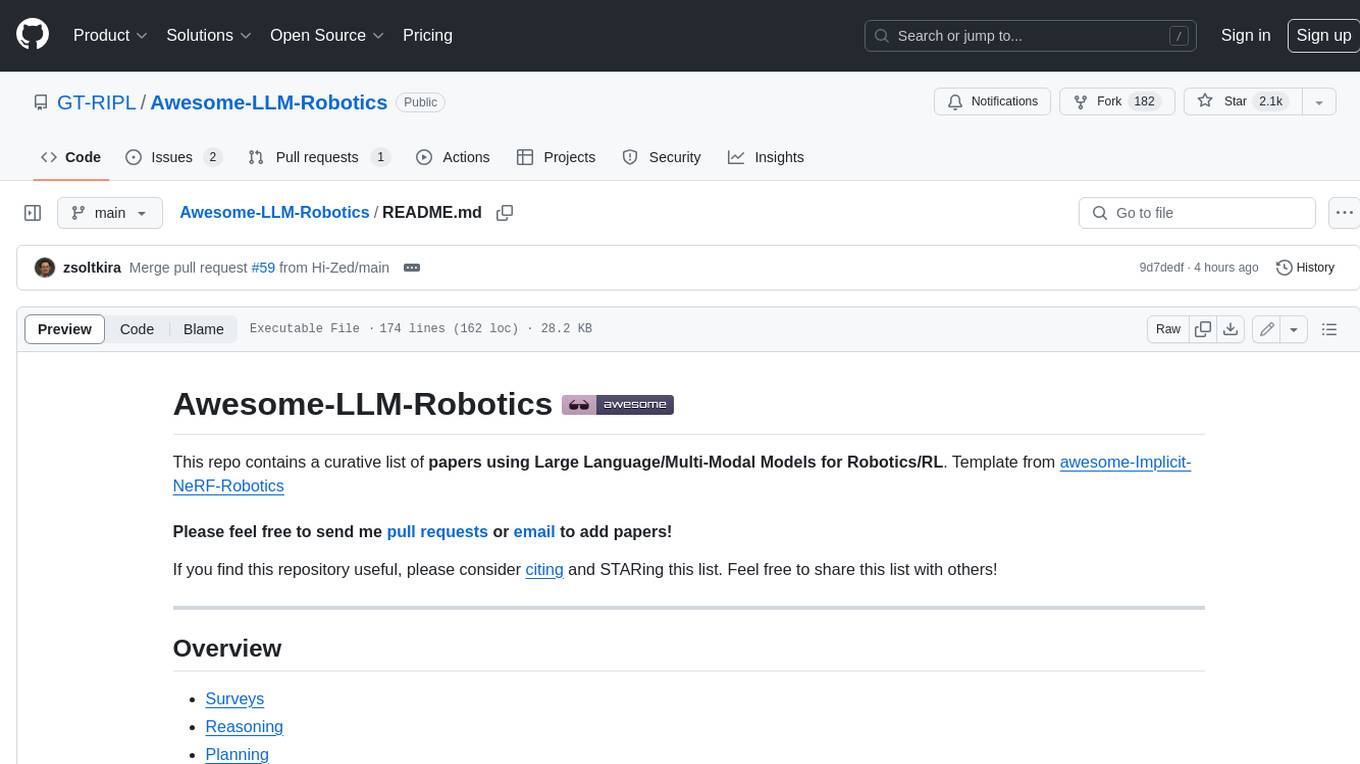
Awesome-LLM-Robotics
This repository contains a curated list of **papers using Large Language/Multi-Modal Models for Robotics/RL**. Template from awesome-Implicit-NeRF-Robotics Please feel free to send me pull requests or email to add papers! If you find this repository useful, please consider citing and STARing this list. Feel free to share this list with others! ## Overview * Surveys * Reasoning * Planning * Manipulation * Instructions and Navigation * Simulation Frameworks * Citation
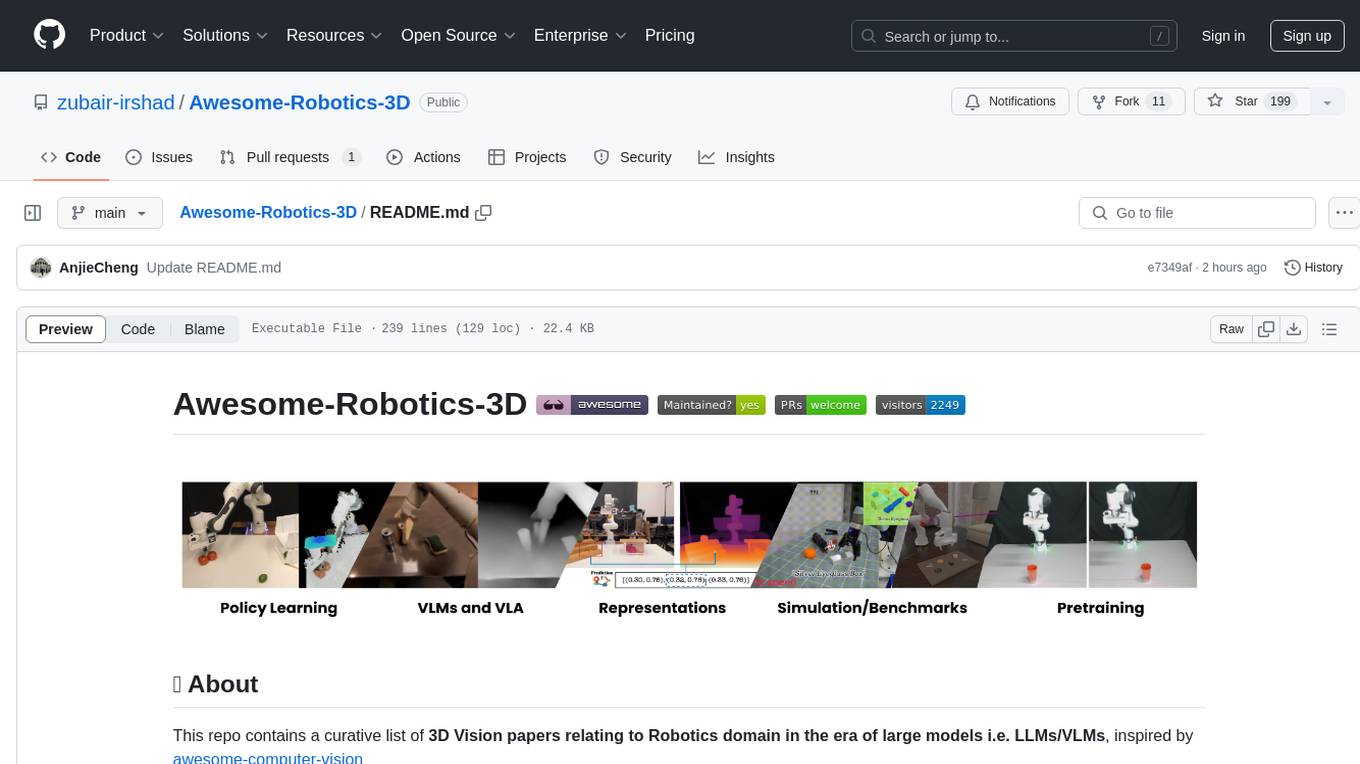
Awesome-Robotics-3D
Awesome-Robotics-3D is a curated list of 3D Vision papers related to Robotics domain, focusing on large models like LLMs/VLMs. It includes papers on Policy Learning, Pretraining, VLM and LLM, Representations, and Simulations, Datasets, and Benchmarks. The repository is maintained by Zubair Irshad and welcomes contributions and suggestions for adding papers. It serves as a valuable resource for researchers and practitioners in the field of Robotics and Computer Vision.
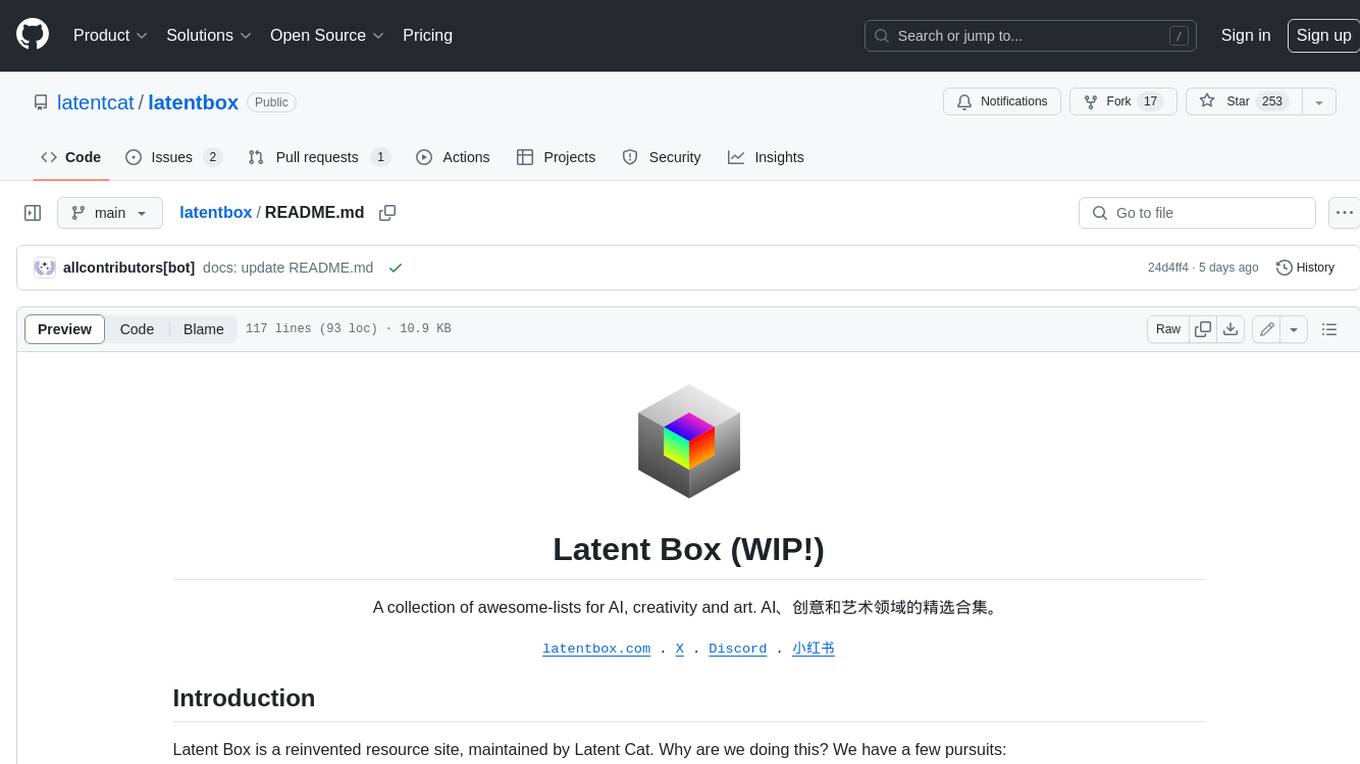
latentbox
Latent Box is a curated collection of resources for AI, creativity, and art. It aims to bridge the information gap with high-quality content, promote diversity and interdisciplinary collaboration, and maintain updates through community co-creation. The website features a wide range of resources, including articles, tutorials, tools, and datasets, covering various topics such as machine learning, computer vision, natural language processing, generative art, and creative coding.
For similar tasks
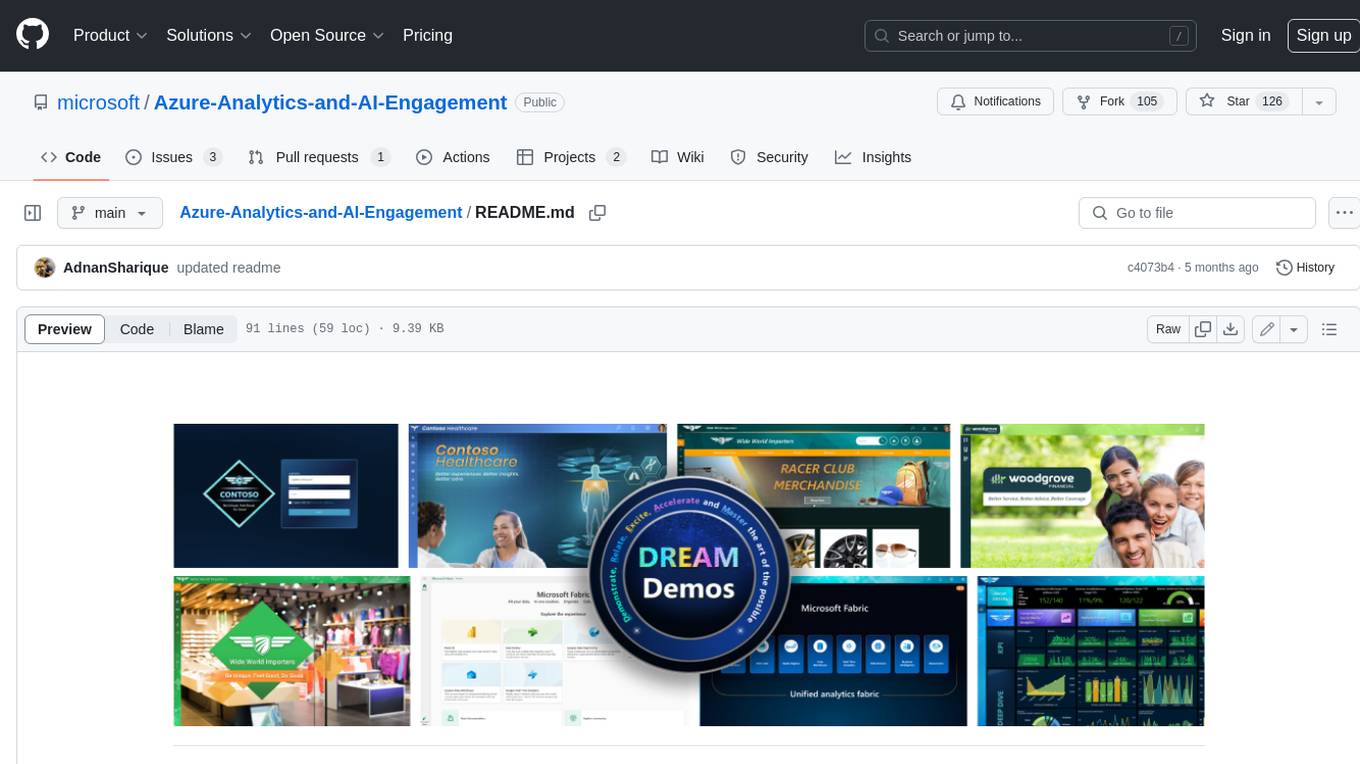
Azure-Analytics-and-AI-Engagement
The Azure-Analytics-and-AI-Engagement repository provides packaged Industry Scenario DREAM Demos with ARM templates (Containing a demo web application, Power BI reports, Synapse resources, AML Notebooks etc.) that can be deployed in a customer’s subscription using the CAPE tool within a matter of few hours. Partners can also deploy DREAM Demos in their own subscriptions using DPoC.
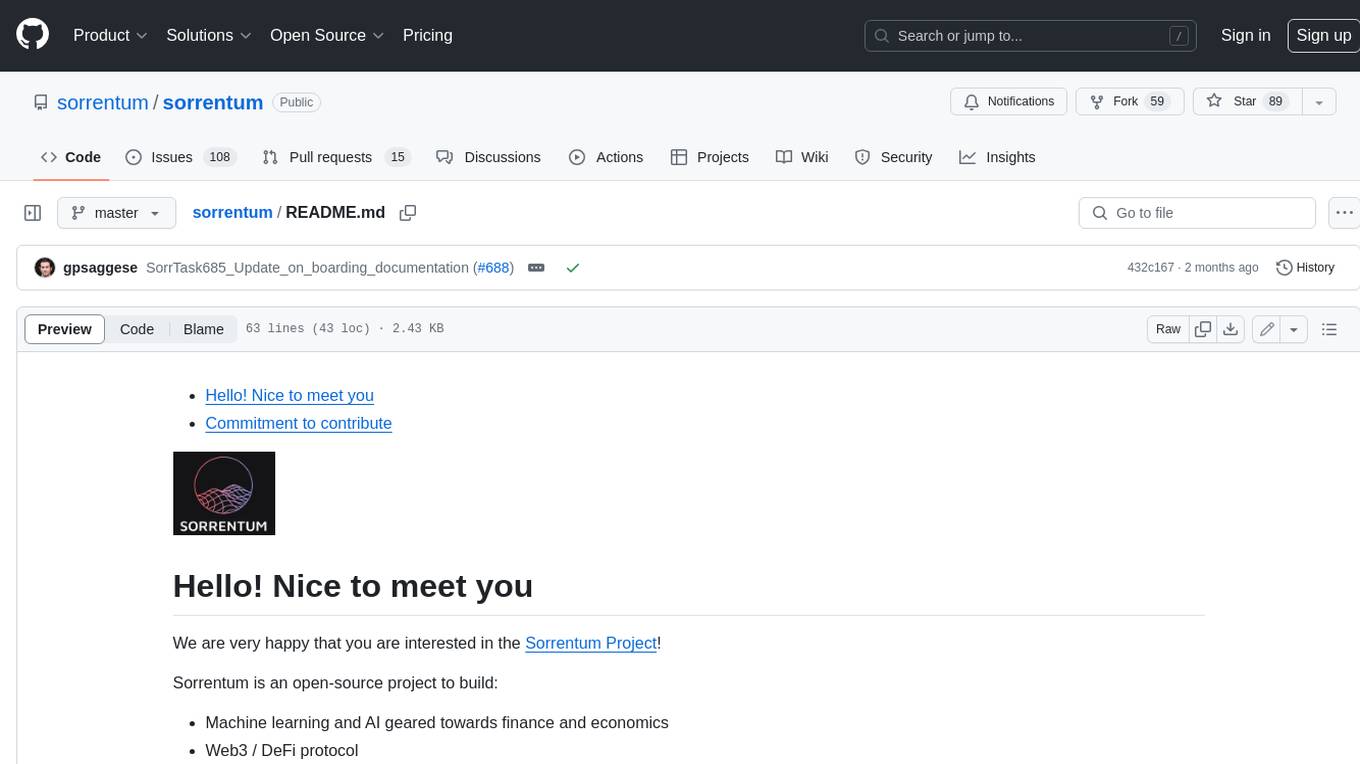
sorrentum
Sorrentum is an open-source project that aims to combine open-source development, startups, and brilliant students to build machine learning, AI, and Web3 / DeFi protocols geared towards finance and economics. The project provides opportunities for internships, research assistantships, and development grants, as well as the chance to work on cutting-edge problems, learn about startups, write academic papers, and get internships and full-time positions at companies working on Sorrentum applications.
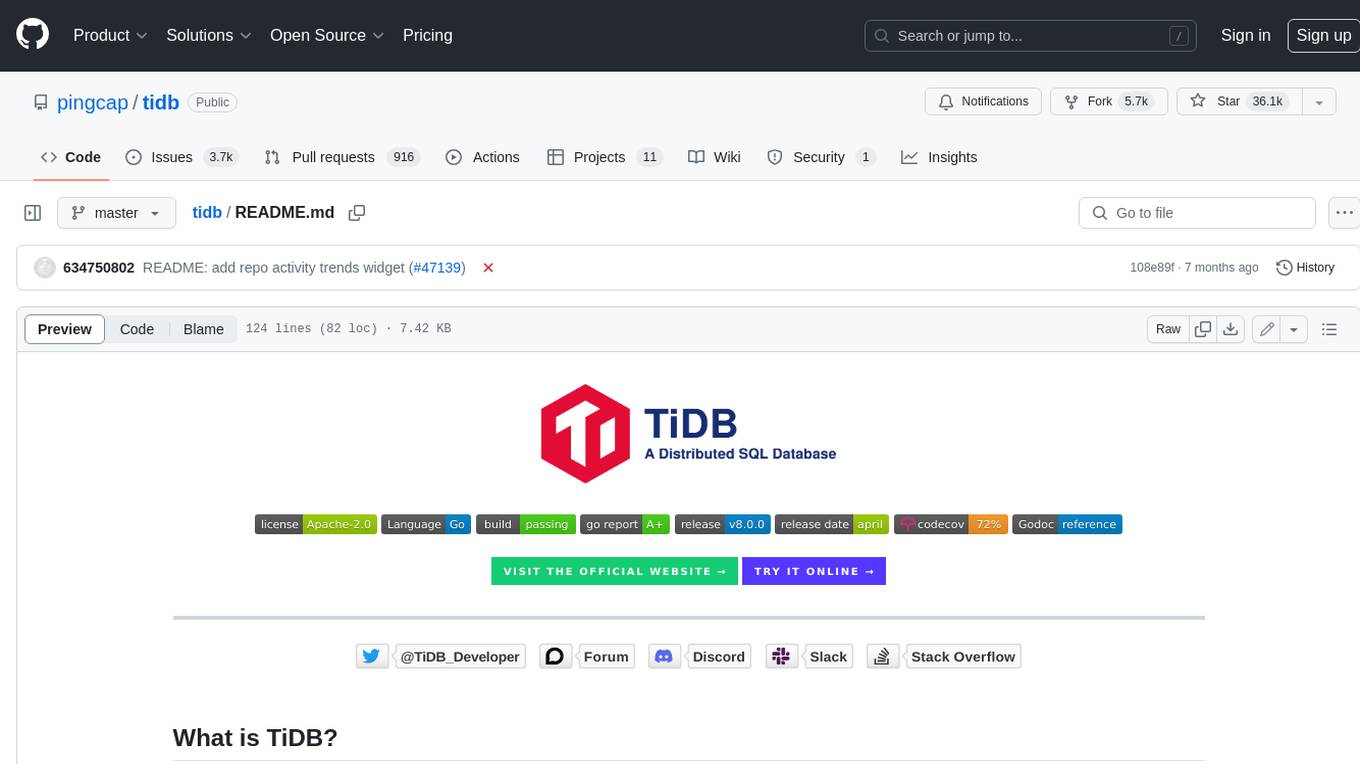
tidb
TiDB is an open-source distributed SQL database that supports Hybrid Transactional and Analytical Processing (HTAP) workloads. It is MySQL compatible and features horizontal scalability, strong consistency, and high availability.
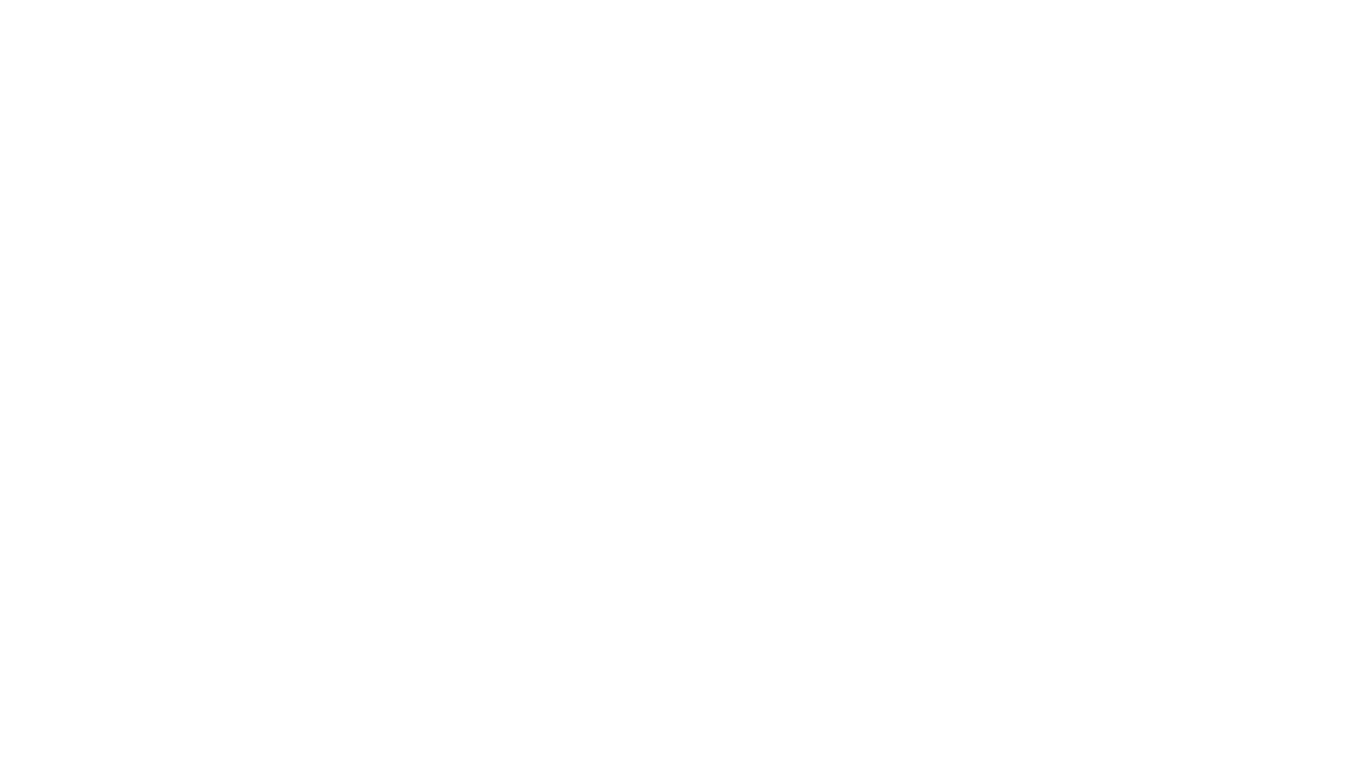
zep-python
Zep is an open-source platform for building and deploying large language model (LLM) applications. It provides a suite of tools and services that make it easy to integrate LLMs into your applications, including chat history memory, embedding, vector search, and data enrichment. Zep is designed to be scalable, reliable, and easy to use, making it a great choice for developers who want to build LLM-powered applications quickly and easily.
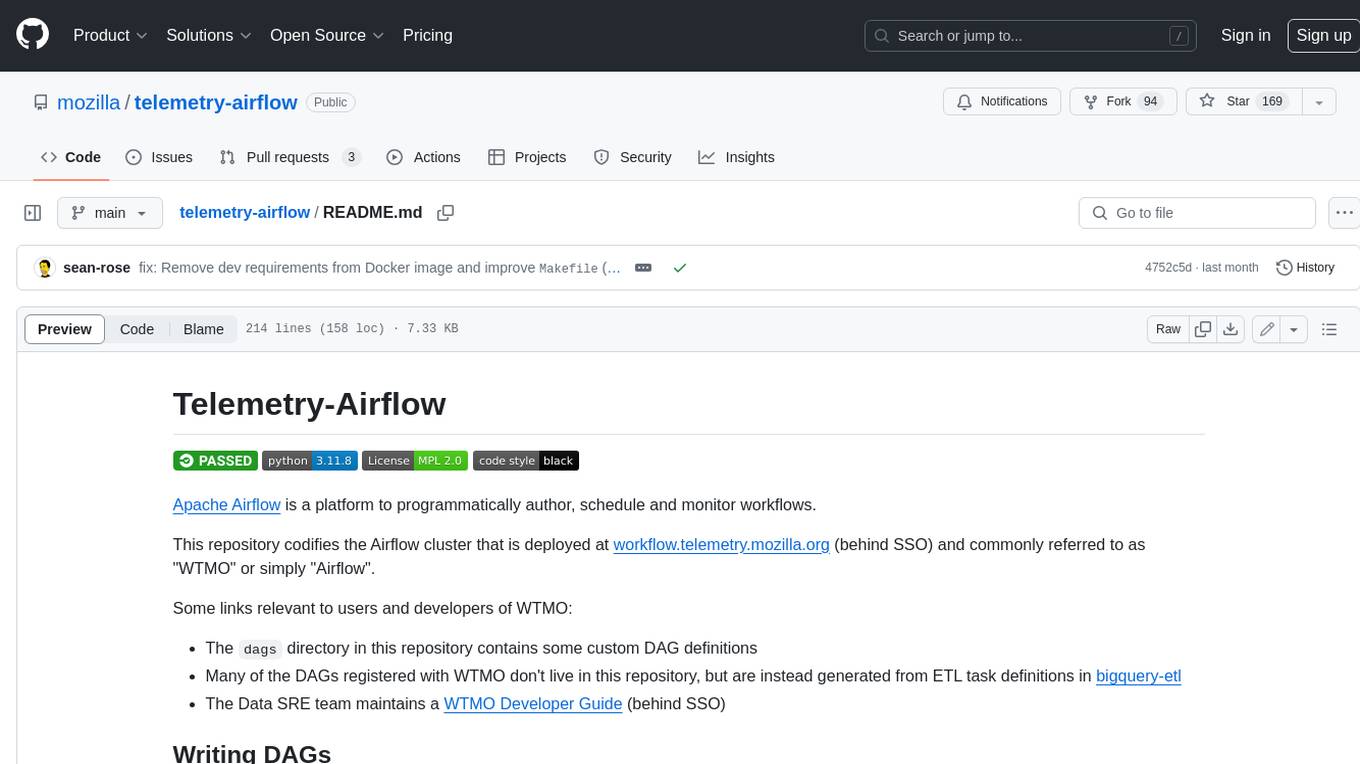
telemetry-airflow
This repository codifies the Airflow cluster that is deployed at workflow.telemetry.mozilla.org (behind SSO) and commonly referred to as "WTMO" or simply "Airflow". Some links relevant to users and developers of WTMO: * The `dags` directory in this repository contains some custom DAG definitions * Many of the DAGs registered with WTMO don't live in this repository, but are instead generated from ETL task definitions in bigquery-etl * The Data SRE team maintains a WTMO Developer Guide (behind SSO)
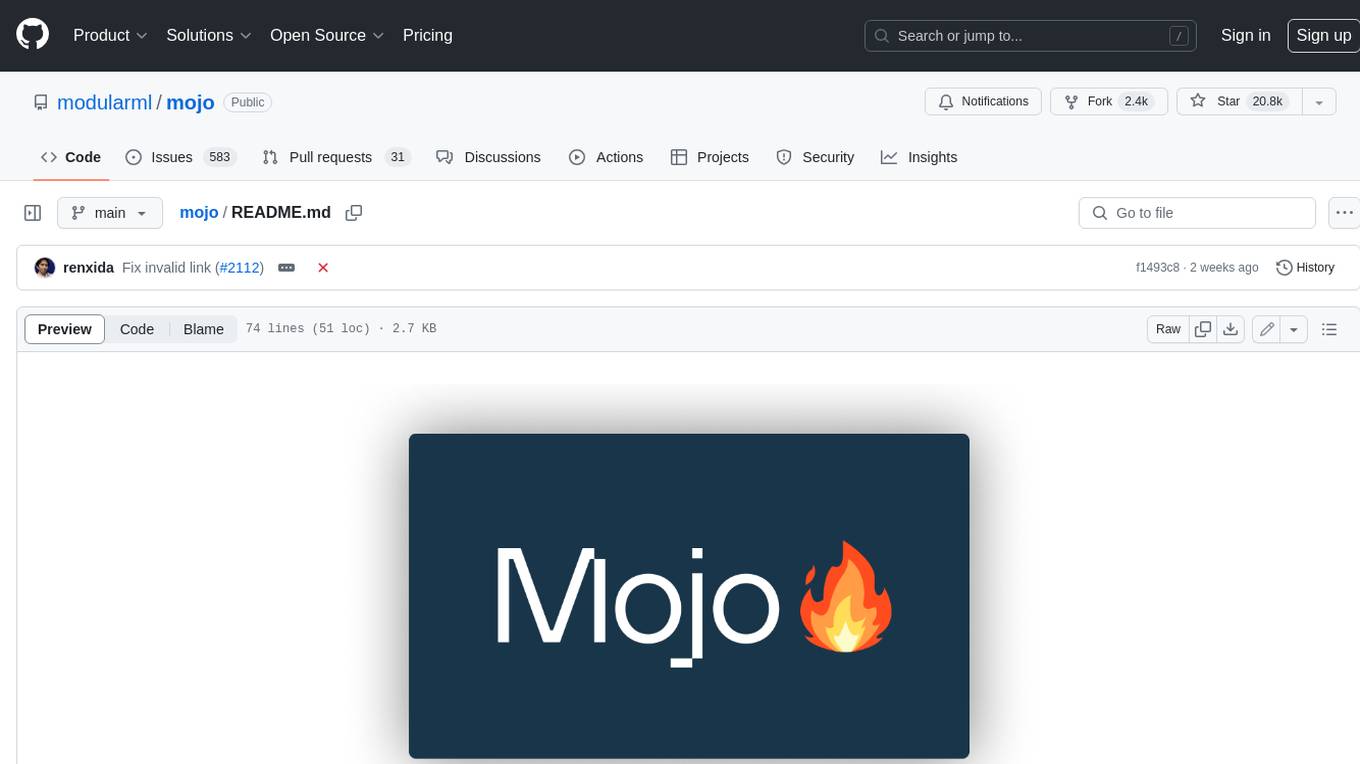
mojo
Mojo is a new programming language that bridges the gap between research and production by combining Python syntax and ecosystem with systems programming and metaprogramming features. Mojo is still young, but it is designed to become a superset of Python over time.
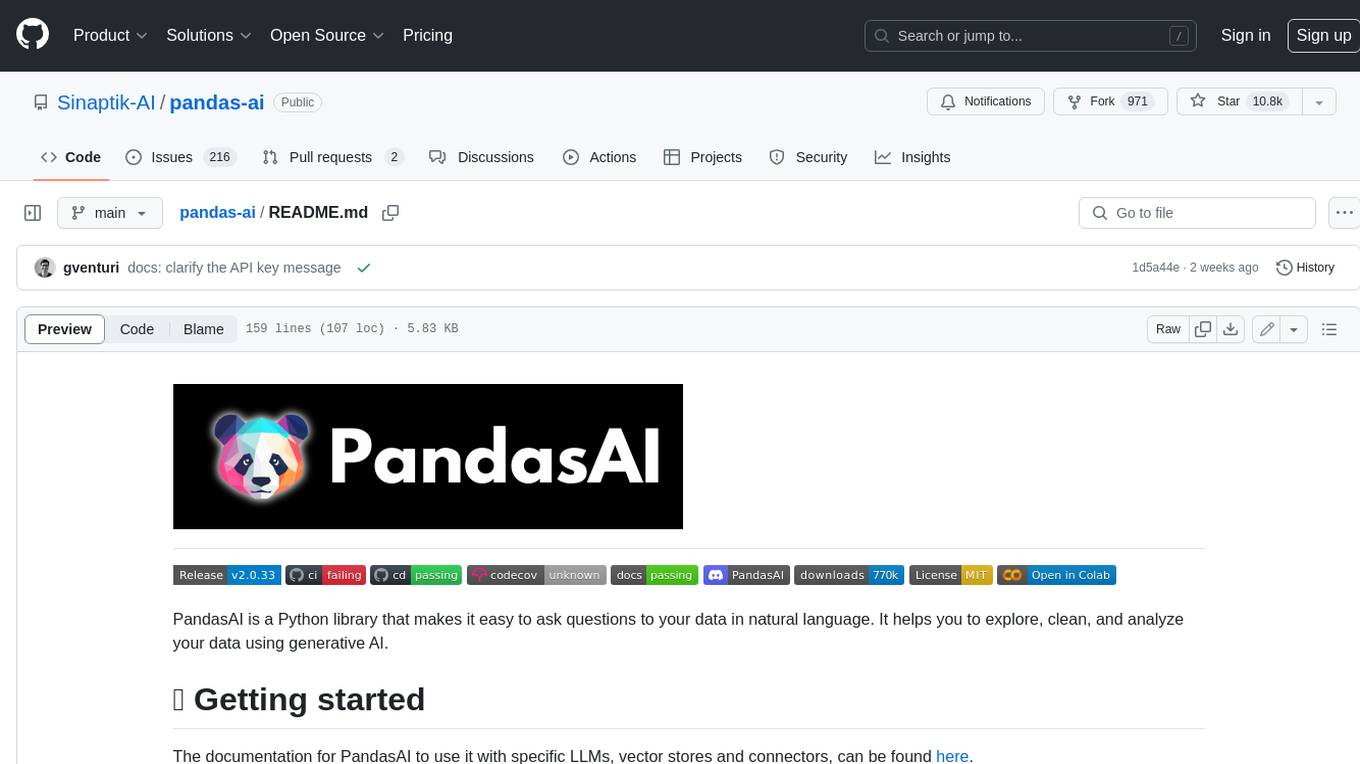
pandas-ai
PandasAI is a Python library that makes it easy to ask questions to your data in natural language. It helps you to explore, clean, and analyze your data using generative AI.
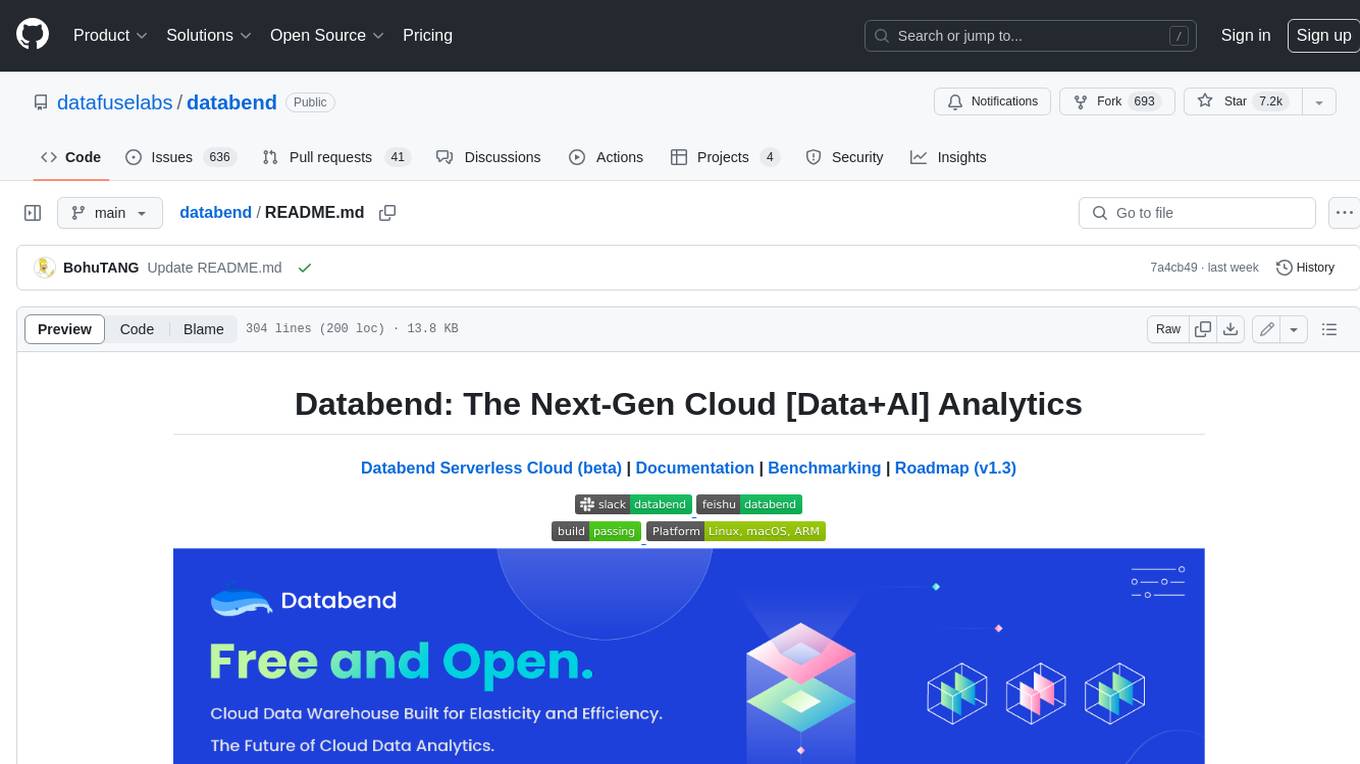
databend
Databend is an open-source cloud data warehouse that serves as a cost-effective alternative to Snowflake. With its focus on fast query execution and data ingestion, it's designed for complex analysis of the world's largest datasets.
For similar jobs
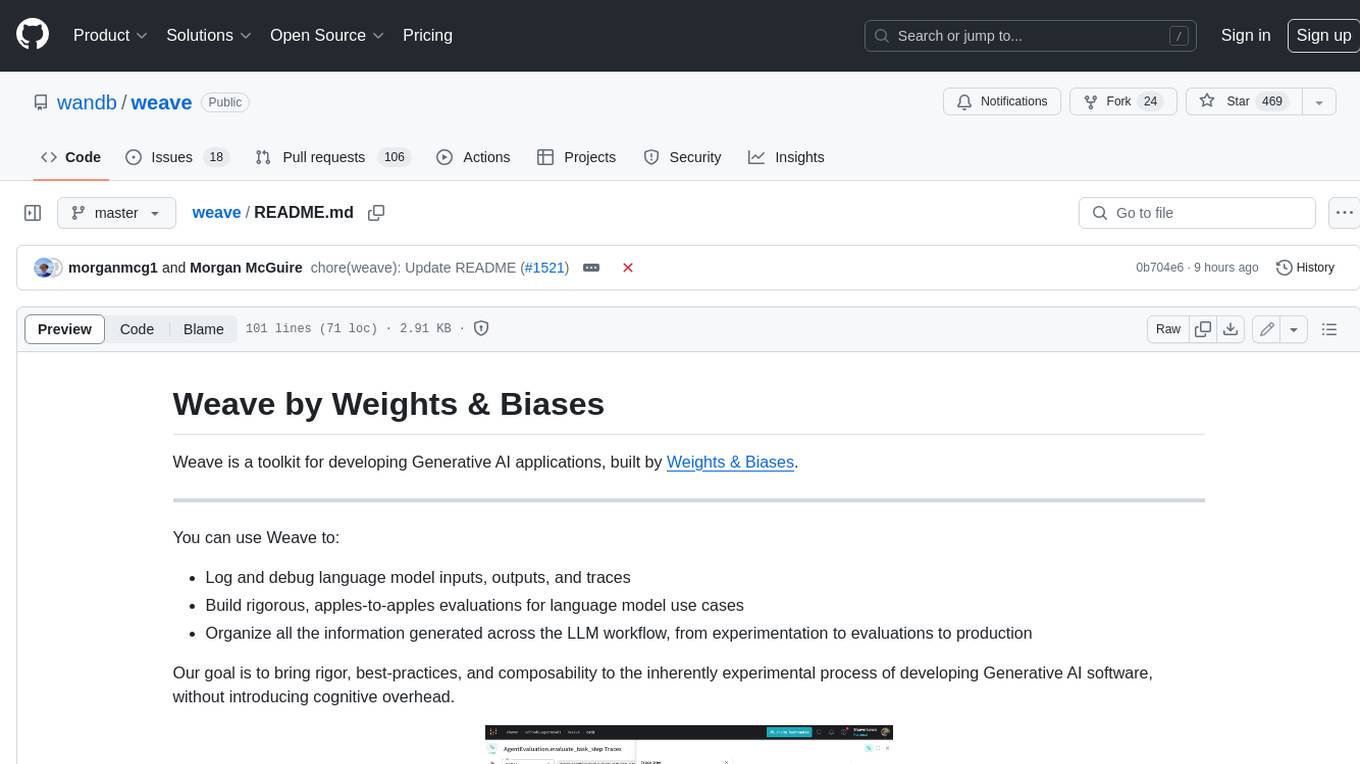
weave
Weave is a toolkit for developing Generative AI applications, built by Weights & Biases. With Weave, you can log and debug language model inputs, outputs, and traces; build rigorous, apples-to-apples evaluations for language model use cases; and organize all the information generated across the LLM workflow, from experimentation to evaluations to production. Weave aims to bring rigor, best-practices, and composability to the inherently experimental process of developing Generative AI software, without introducing cognitive overhead.
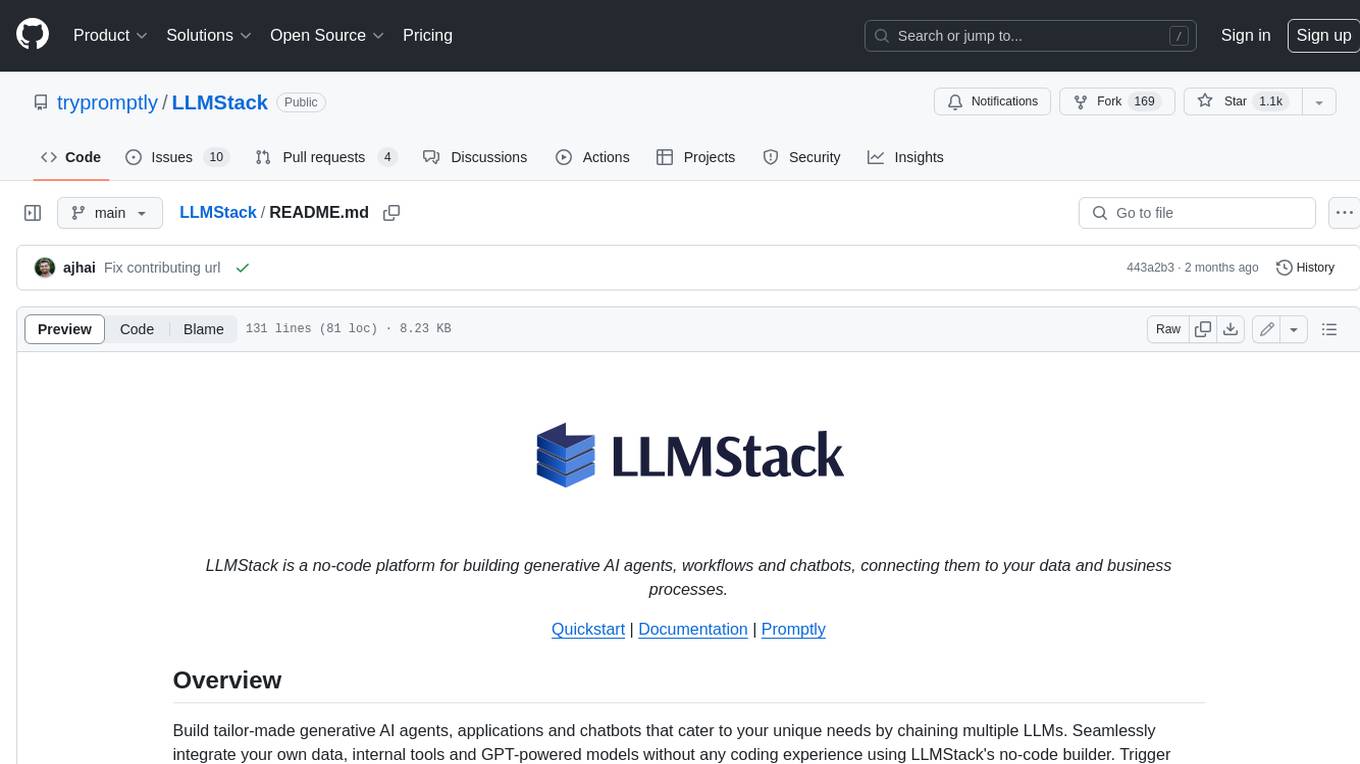
LLMStack
LLMStack is a no-code platform for building generative AI agents, workflows, and chatbots. It allows users to connect their own data, internal tools, and GPT-powered models without any coding experience. LLMStack can be deployed to the cloud or on-premise and can be accessed via HTTP API or triggered from Slack or Discord.
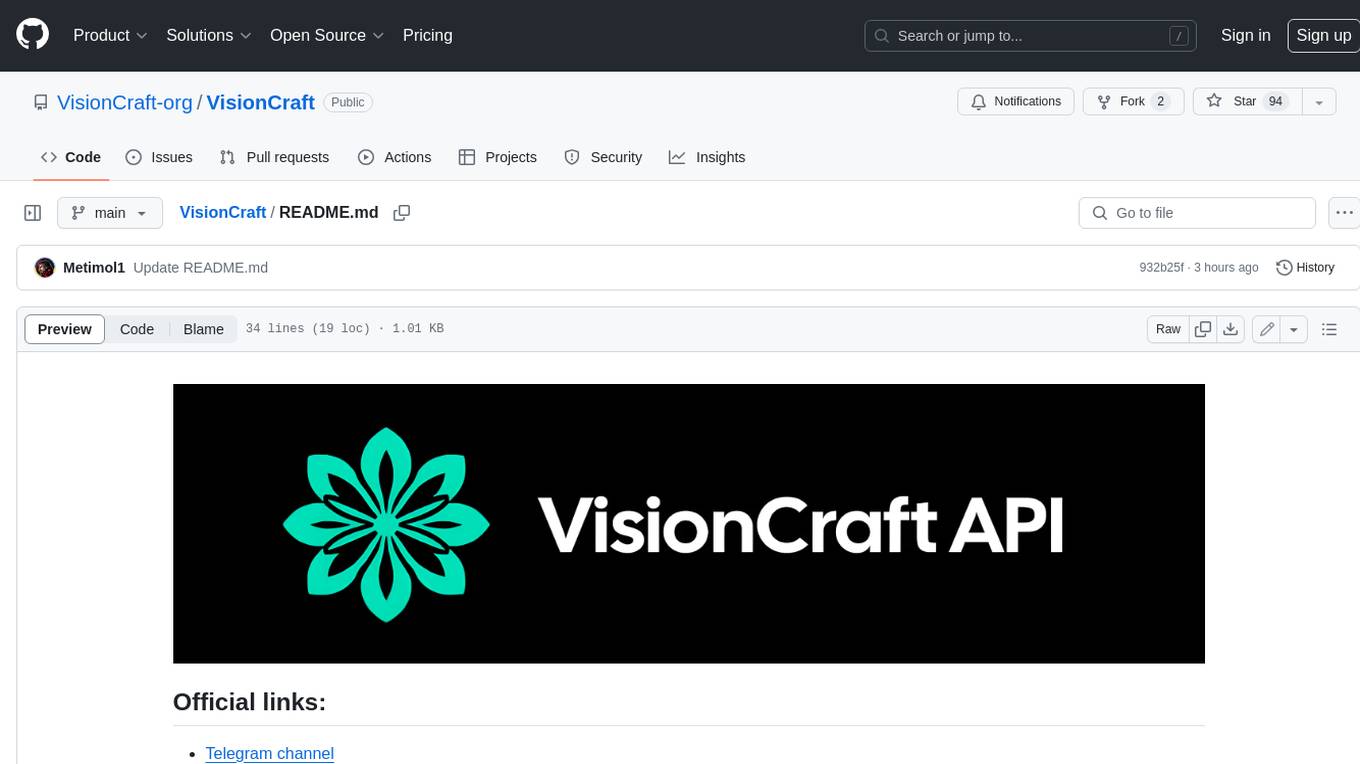
VisionCraft
The VisionCraft API is a free API for using over 100 different AI models. From images to sound.
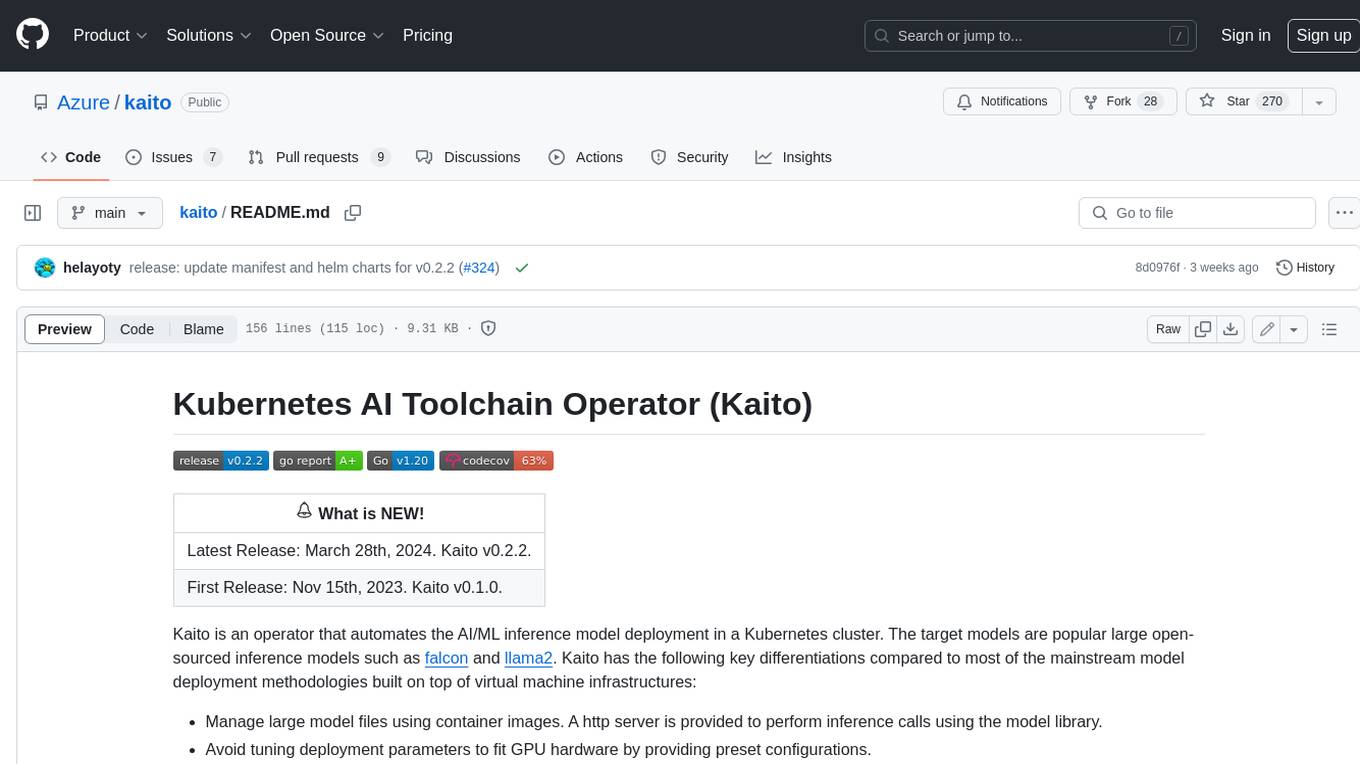
kaito
Kaito is an operator that automates the AI/ML inference model deployment in a Kubernetes cluster. It manages large model files using container images, avoids tuning deployment parameters to fit GPU hardware by providing preset configurations, auto-provisions GPU nodes based on model requirements, and hosts large model images in the public Microsoft Container Registry (MCR) if the license allows. Using Kaito, the workflow of onboarding large AI inference models in Kubernetes is largely simplified.
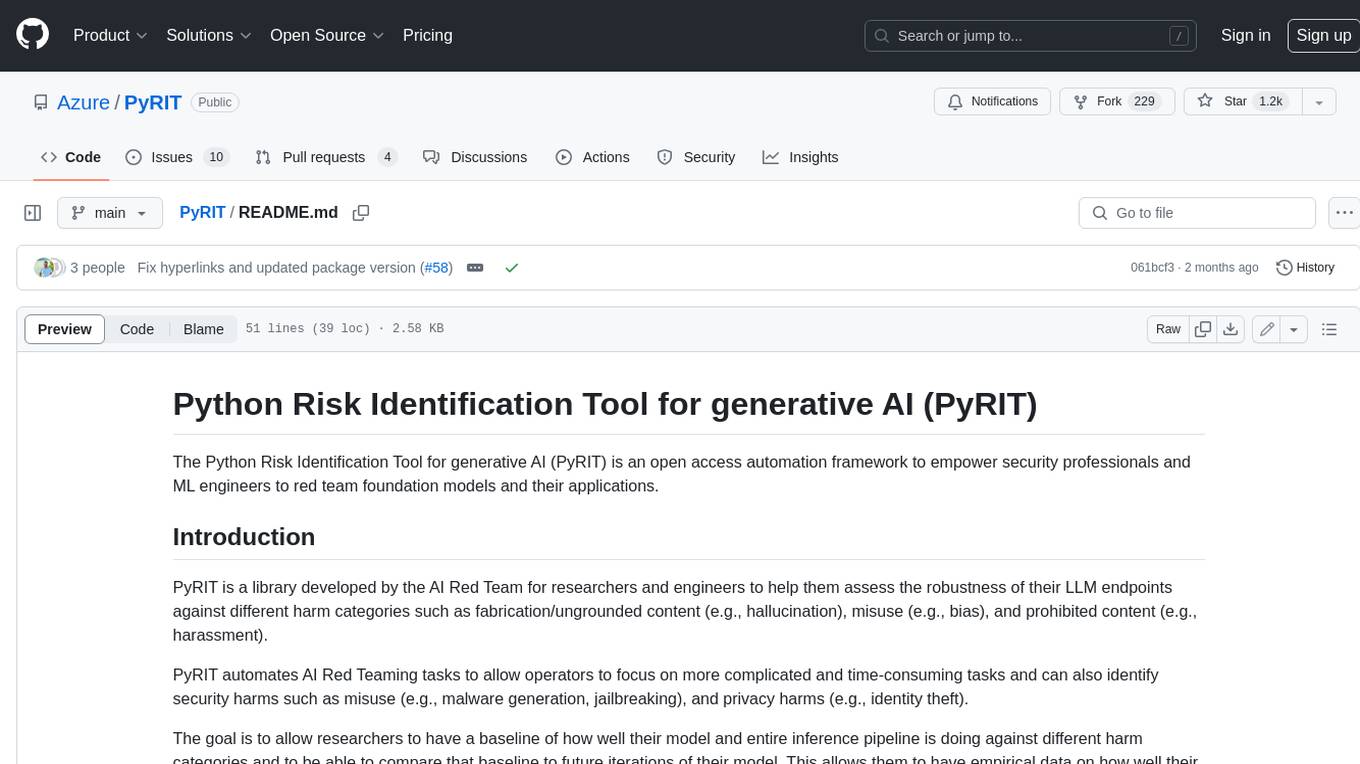
PyRIT
PyRIT is an open access automation framework designed to empower security professionals and ML engineers to red team foundation models and their applications. It automates AI Red Teaming tasks to allow operators to focus on more complicated and time-consuming tasks and can also identify security harms such as misuse (e.g., malware generation, jailbreaking), and privacy harms (e.g., identity theft). The goal is to allow researchers to have a baseline of how well their model and entire inference pipeline is doing against different harm categories and to be able to compare that baseline to future iterations of their model. This allows them to have empirical data on how well their model is doing today, and detect any degradation of performance based on future improvements.
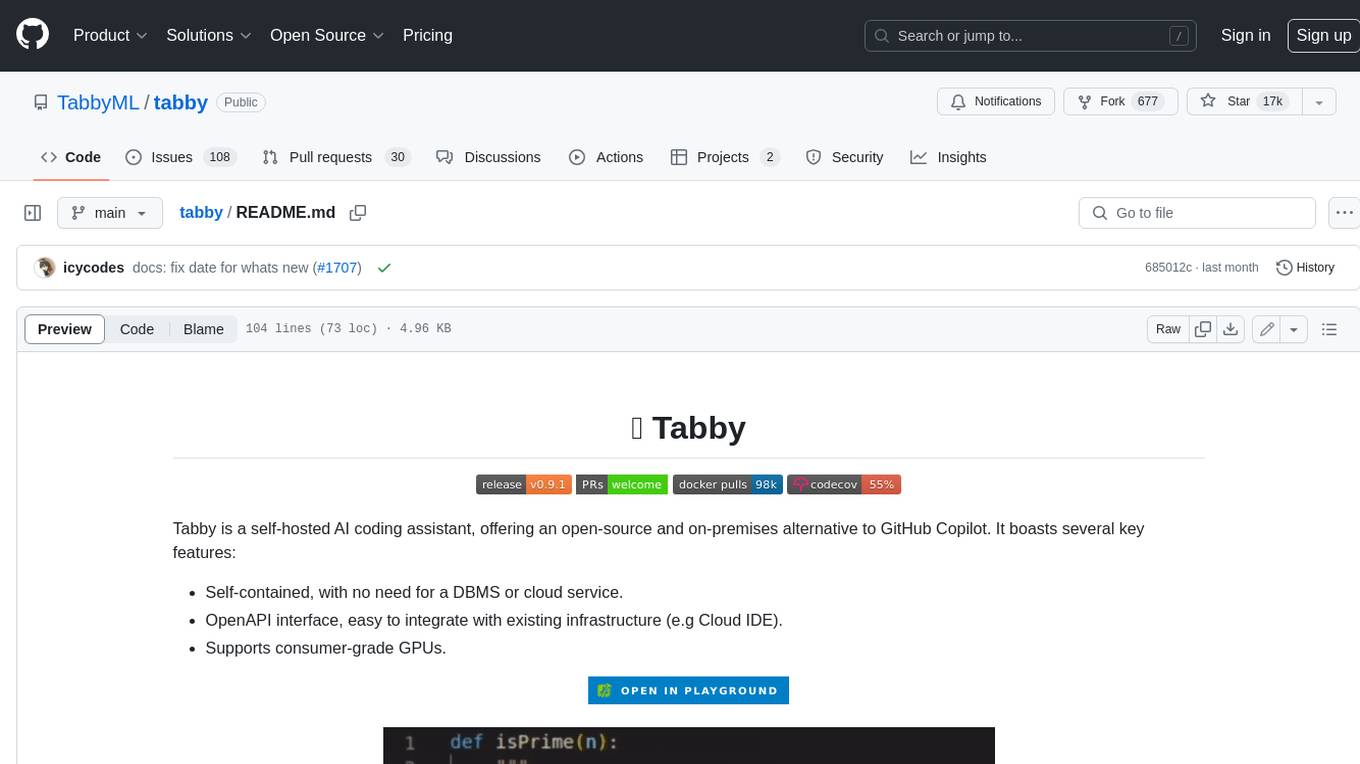
tabby
Tabby is a self-hosted AI coding assistant, offering an open-source and on-premises alternative to GitHub Copilot. It boasts several key features: * Self-contained, with no need for a DBMS or cloud service. * OpenAPI interface, easy to integrate with existing infrastructure (e.g Cloud IDE). * Supports consumer-grade GPUs.
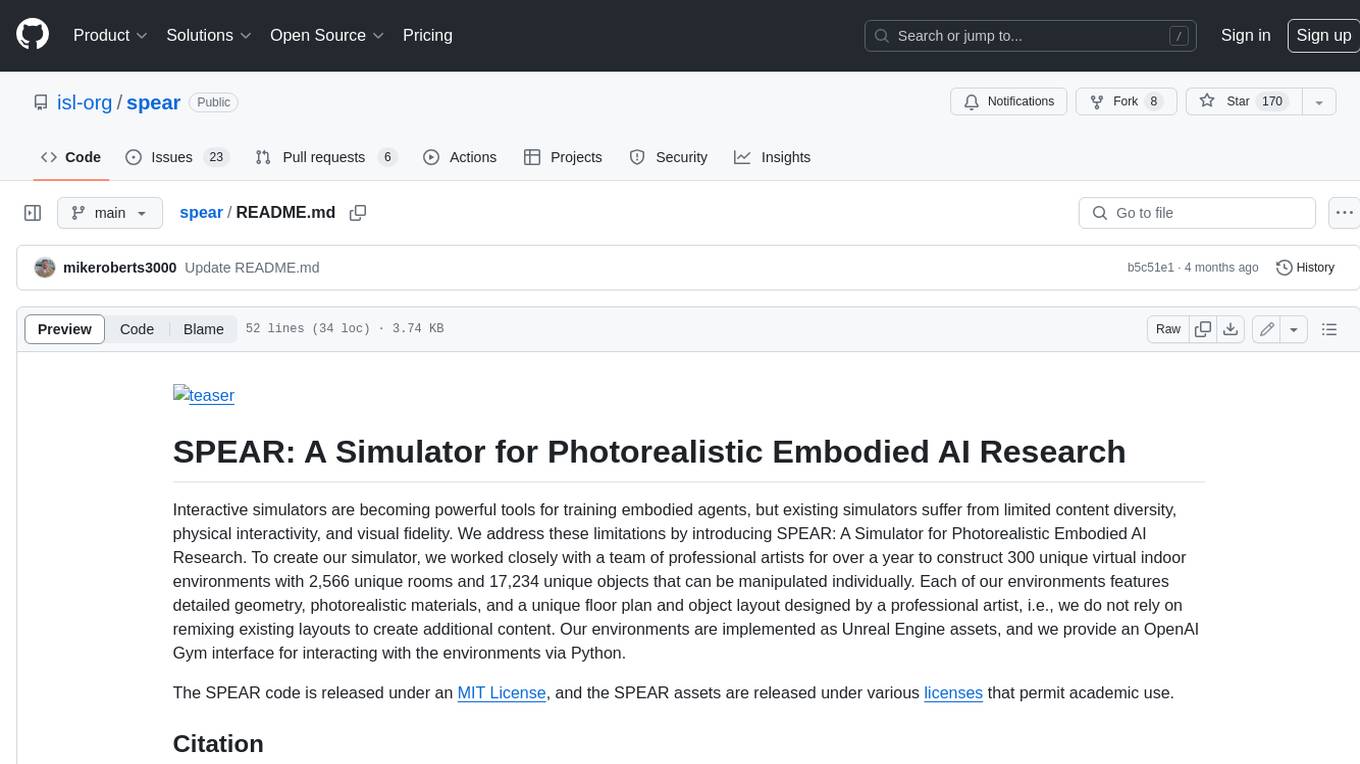
spear
SPEAR (Simulator for Photorealistic Embodied AI Research) is a powerful tool for training embodied agents. It features 300 unique virtual indoor environments with 2,566 unique rooms and 17,234 unique objects that can be manipulated individually. Each environment is designed by a professional artist and features detailed geometry, photorealistic materials, and a unique floor plan and object layout. SPEAR is implemented as Unreal Engine assets and provides an OpenAI Gym interface for interacting with the environments via Python.
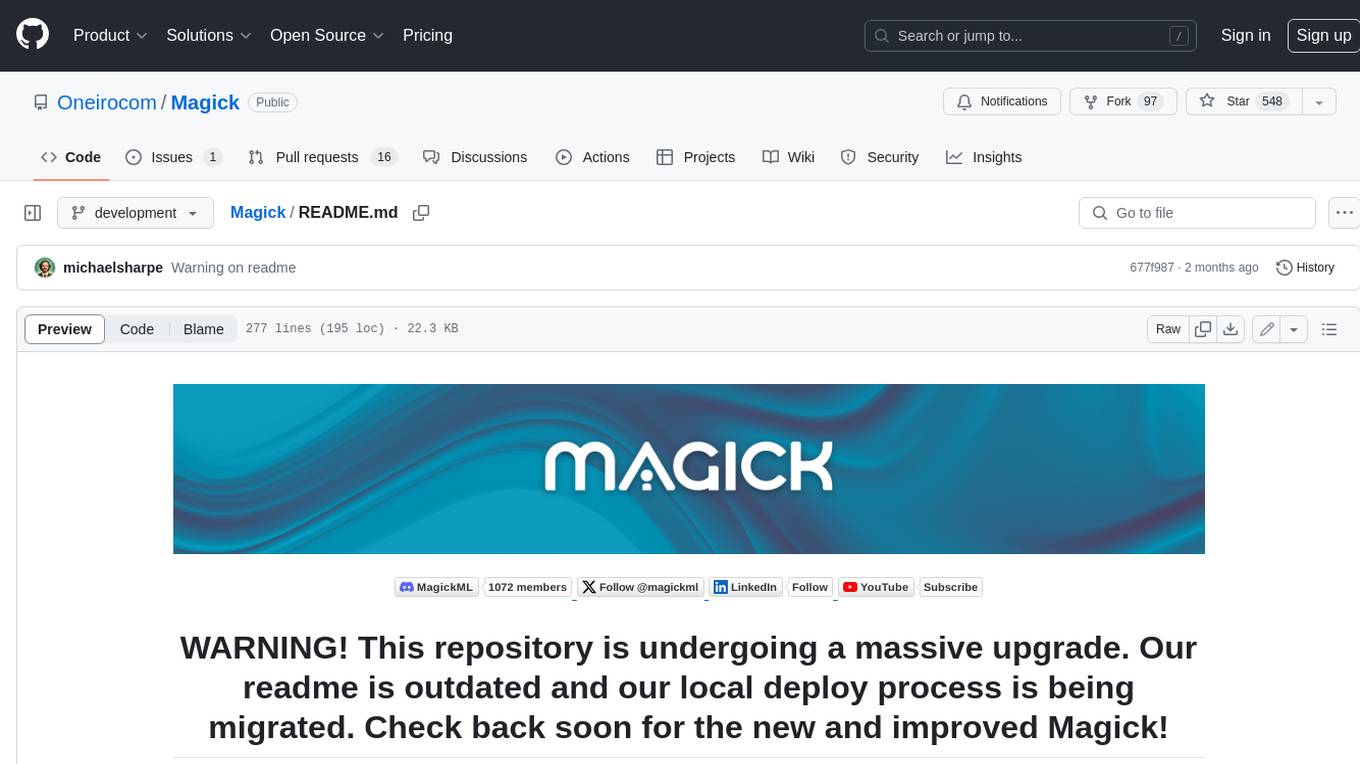
Magick
Magick is a groundbreaking visual AIDE (Artificial Intelligence Development Environment) for no-code data pipelines and multimodal agents. Magick can connect to other services and comes with nodes and templates well-suited for intelligent agents, chatbots, complex reasoning systems and realistic characters.