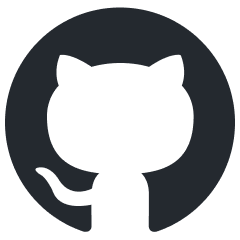
MathEval
MathEval is a benchmark dedicated to the holistic evaluation on mathematical capacities of LLMs.
Stars: 56
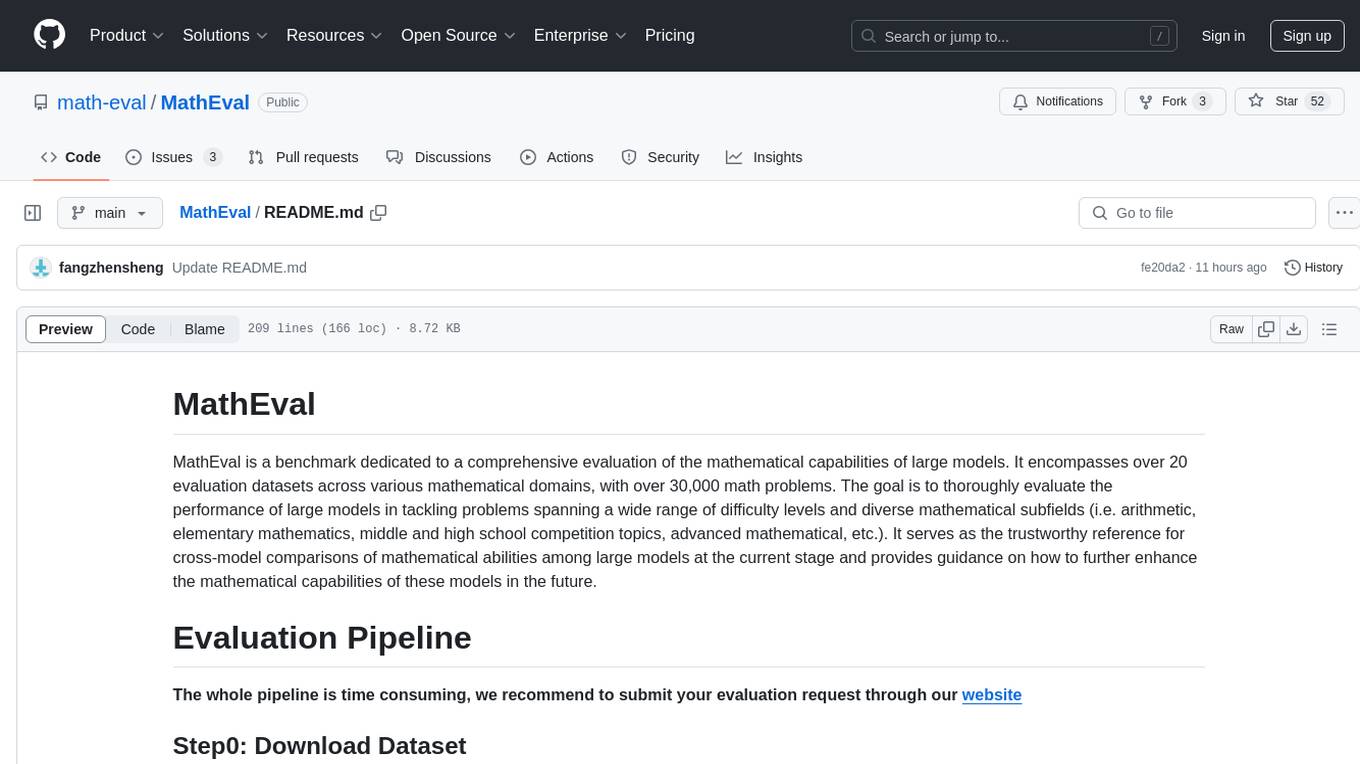
MathEval is a benchmark designed for evaluating the mathematical capabilities of large models. It includes over 20 evaluation datasets covering various mathematical domains with more than 30,000 math problems. The goal is to assess the performance of large models across different difficulty levels and mathematical subfields. MathEval serves as a reliable reference for comparing mathematical abilities among large models and offers guidance on enhancing their mathematical capabilities in the future.
README:
MathEval is a benchmark dedicated to a comprehensive evaluation of the mathematical capabilities of large models. It encompasses over 20 evaluation datasets across various mathematical domains, with over 30,000 math problems. The goal is to thoroughly evaluate the performance of large models in tackling problems spanning a wide range of difficulty levels and diverse mathematical subfields (i.e. arithmetic, elementary mathematics, middle and high school competition topics, advanced mathematical, etc.). It serves as the trustworthy reference for cross-model comparisons of mathematical abilities among large models at the current stage and provides guidance on how to further enhance the mathematical capabilities of these models in the future.
The whole pipeline is time consuming, we recommend to submit your evaluation request through our website
Download the evaluation datasets from Google Drive
We unify the input format of different mathematical dataset as following:
For zero shot settings:
{"conversations": [{"from":"human", "value":"xxx"}]}
For few shot settings:
{"conversations": [{"from":"human", "value":"example0-question"}, {"from":"gpt", "value":"example0-answer"}, {},{}, ...]}
Running Script:
python preprocess_dataset/build_dataset.py --input_dir ./datasets --output_dir ./output
where ./datasets is the path where you save your downloaded datasets
We format the input prompt for different base model and their chat edition based on the fastchat.
- If you have elaborate templates for your models, implement your templates inside the conversation.py file, and change the settings in generate_shell_config.py file with your own template_name;
- You can also use default template_name for your model. Please also change the data_dir variable in generate_shell_config.py file. The generate_shell_config.py contains all the preprocessing config for each dataset and each base model.
- We assume you have at least 2 80G-GPUs in your device to run over 70B size models. You need to ensure that the model can be successfully loaded with the given num_gpus. Please run the following script to generate the inference scripts, you need to specify the path to the model's output directory, the path to the log directory, and the path to save the running shell scripts:
We now only support using VLLM for inference acceleration. To enable VLLM acceleration, use the following command:
python run.py --configs ./generate_shell_config.py --accelerator vllm
To use VLLM for inference acceleration, you need to install it first. Please refer to the VLLM GitHub repository for installation instructions.
We now support two modes: "infer" and "eval". In "infer" mode, the system only generates model outputs. In "eval" mode, the system not only generates model outputs but also compares them with the real answers using the compare-model.
The default mode is "infer". To start in "eval" mode, you can use the following command:
python run.py --configs ./generate_shell_config.py --accelerator vllm --mode eval
We have separated the modes for greater flexibility. You can compare the answers using either GPT-4 or the compare-model.
- --configs ./generate_shell_config.py: Specifies the path to the configuration file.
- --accelerator vllm: Enables VLLM for inference acceleration.
If you have access to GPT4, please jump to Step 5.
If you do not have access to GPT4, we have released a compare-answer model in HuggingFace, please refer to: DeepSeek-Math-Compare-Answer
Now we implement compare compare_with_local_model.py
python compare_with_local_model.py --model_path /path/to/model --input_dir /path/to/input --output_dir /path/to/output --device_num 4
- --model_path: Path to the pre-trained model directory.
- --input_dir: Directory containing the input JSON files.
- --output_dir: Directory to save the output JSON files.
- --device_num: Number of GPUs to use for parallel processing.
We have provide our prompt in ./prompts/extraction_prompts folder.
We recommend to realize the send_chat_request function in run_gpt4_extraction.py by yourself, since we do not know the detailed GPT4 invoking function of yours.
We have provide our prompt in ./prompts/verification_prompts folder.
We recommend to realize the send_chat_request function in run_gpt4_extraction.py by yourself, since we do not know the detailed GPT4 invoking function of yours.
Why we want to use GPT4 for answer extraction and answer verification.
The precision comparison of using GPT4 and REGEX from OpenCompass for answer extractor
Dataset_name | Dataset_size |
---|---|
agieval-aqua-rat | 254 |
agieval-gaokao-mathcloze | 118 |
agieval-gaokao-mathqa | 351 |
agieval-math | 1000 |
agieval-ppl-aqua-rat | 254 |
agieval-ppl-gaokao-mathcloze | 118 |
agieval-ppl-gaokao-mathqa | 351 |
agieval-ppl-math | 1000 |
agieval-ppl-sat-math | 220 |
agieval-sat-math | 220 |
ape210k | 5000 |
ape210k-few3 | 5000 |
asdiv-a | 122 |
asdiv-a-few | 122 |
bbh-fewmultistep_arithmetic_two | 250 |
bbh-multistep_arithmetic_two | 250 |
ceval-few-test-advanced_mathematics | 173 |
ceval-few-test-discrete_mathematics | 153 |
ceval-few-test-high_school_mathematics | 166 |
ceval-few-test-middle_school_mathematics | 177 |
ceval-few-test-probability_and_statistics | 166 |
ceval-ppl-test-advanced_mathematics | 173 |
ceval-ppl-test-discrete_mathematics | 153 |
ceval-ppl-test-high_school_mathematics | 166 |
ceval-ppl-test-middle_school_mathematics | 177 |
ceval-ppl-test-probability_and_statistics | 166 |
ceval-test-advanced_mathematics | 173 |
ceval-test-discrete_mathematics | 153 |
ceval-test-high_school_mathematics | 166 |
ceval-test-middle_school_mathematics | 177 |
ceval-test-probability_and_statistics | 166 |
cmmlu-college_mathematics | 105 |
cmmlu-elementary_mathematics | 230 |
cmmlu-few-college_mathematics | 105 |
cmmlu-few-elementary_mathematics | 230 |
cmmlu-few-high_school_mathematics | 164 |
cmmlu-high_school_mathematics | 164 |
dolphin1878 | 187 |
dolphin1878-few | 187 |
draw | 200 |
draw-few | 200 |
GaokaoBench_2010-2022_Math_I_MCQs | 214 |
GaokaoBench_2010-2022_Math_II_MCQs | 218 |
GaokaoBench_few2010-2022_Math_I_MCQs | 214 |
GaokaoBench_few2010-2022_Math_II_MCQs | 218 |
gsm8k | 1319 |
gsm8k-few | 1319 |
hmwp | 550 |
hmwp-few | 550 |
lukaemon_mmlu_abstract_algebra | 100 |
lukaemon_mmlu_college_mathematics | 100 |
lukaemon_mmlu_elementary_mathematics | 378 |
lukaemon_mmlu_fewabstract_algebra | 100 |
lukaemon_mmlu_fewcollege_mathematics | 100 |
lukaemon_mmlu_fewelementary_mathematics | 378 |
lukaemon_mmlu_fewhigh_school_mathematics | 270 |
lukaemon_mmlu_high_school_mathematics | 270 |
math | 5000 |
math23k | 2317 |
math23k-few5 | 2317 |
math401 | 401 |
math401-few | 401 |
math-few | 5000 |
MathQA | 2985 |
MathQA-few | 2985 |
MathQA-ppl | 2985 |
mawps | 238 |
mawps-few | 238 |
mmlu_ppl_fewabstract_algebra | 100 |
mmlu_ppl_fewcollege_mathematics | 100 |
mmlu_ppl_fewelementary_mathematics | 378 |
mmlu_ppl_fewhigh_school_mathematics | 270 |
mmlu_pplabstract_algebra | 100 |
mmlu_pplcollege_mathematics | 100 |
mmlu_pplelementary_mathematics | 378 |
mmlu_pplhigh_school_mathematics | 270 |
scq_ch | 2000 |
scq_ch_few | 2000 |
scq_en | 2000 |
scq_en_few | 2000 |
svamp | 1000 |
svamp-few | 1000 |
For Tasks:
Click tags to check more tools for each tasksFor Jobs:
Alternative AI tools for MathEval
Similar Open Source Tools
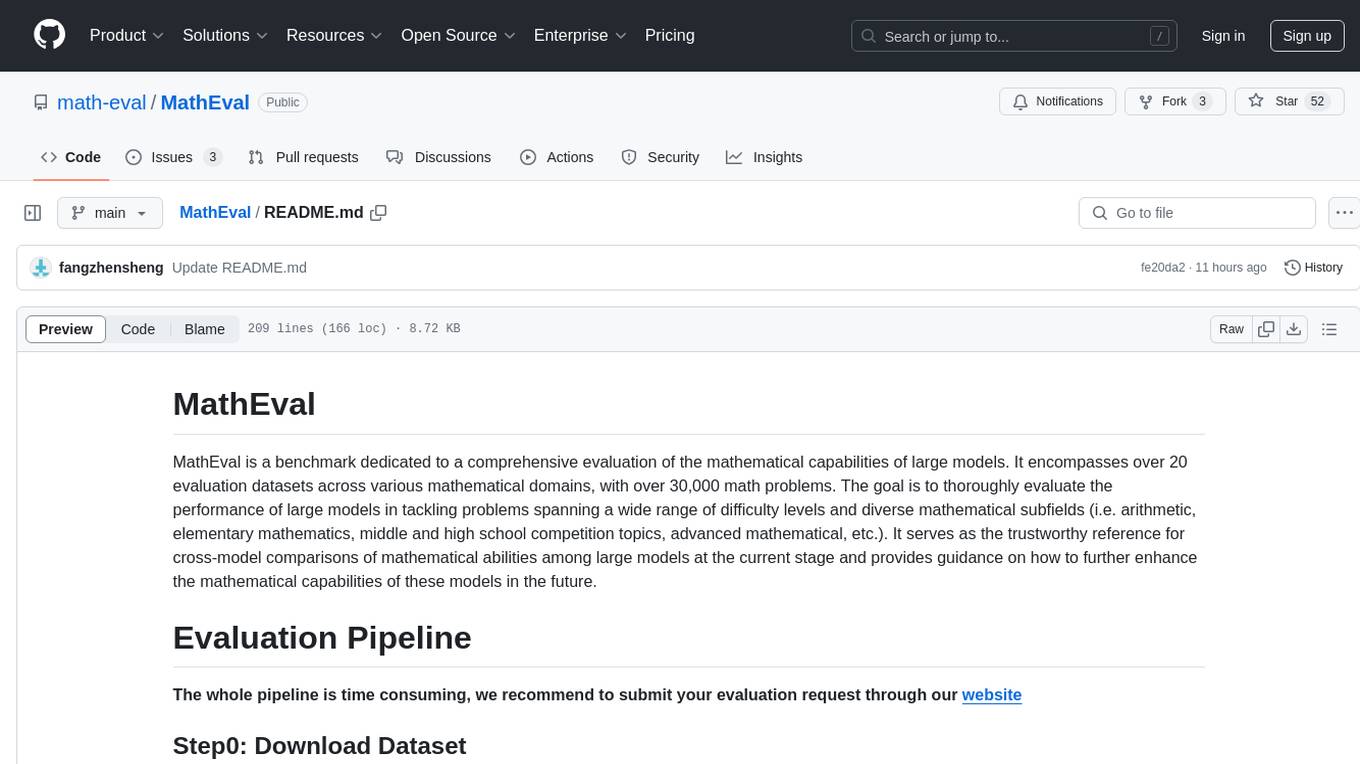
MathEval
MathEval is a benchmark designed for evaluating the mathematical capabilities of large models. It includes over 20 evaluation datasets covering various mathematical domains with more than 30,000 math problems. The goal is to assess the performance of large models across different difficulty levels and mathematical subfields. MathEval serves as a reliable reference for comparing mathematical abilities among large models and offers guidance on enhancing their mathematical capabilities in the future.
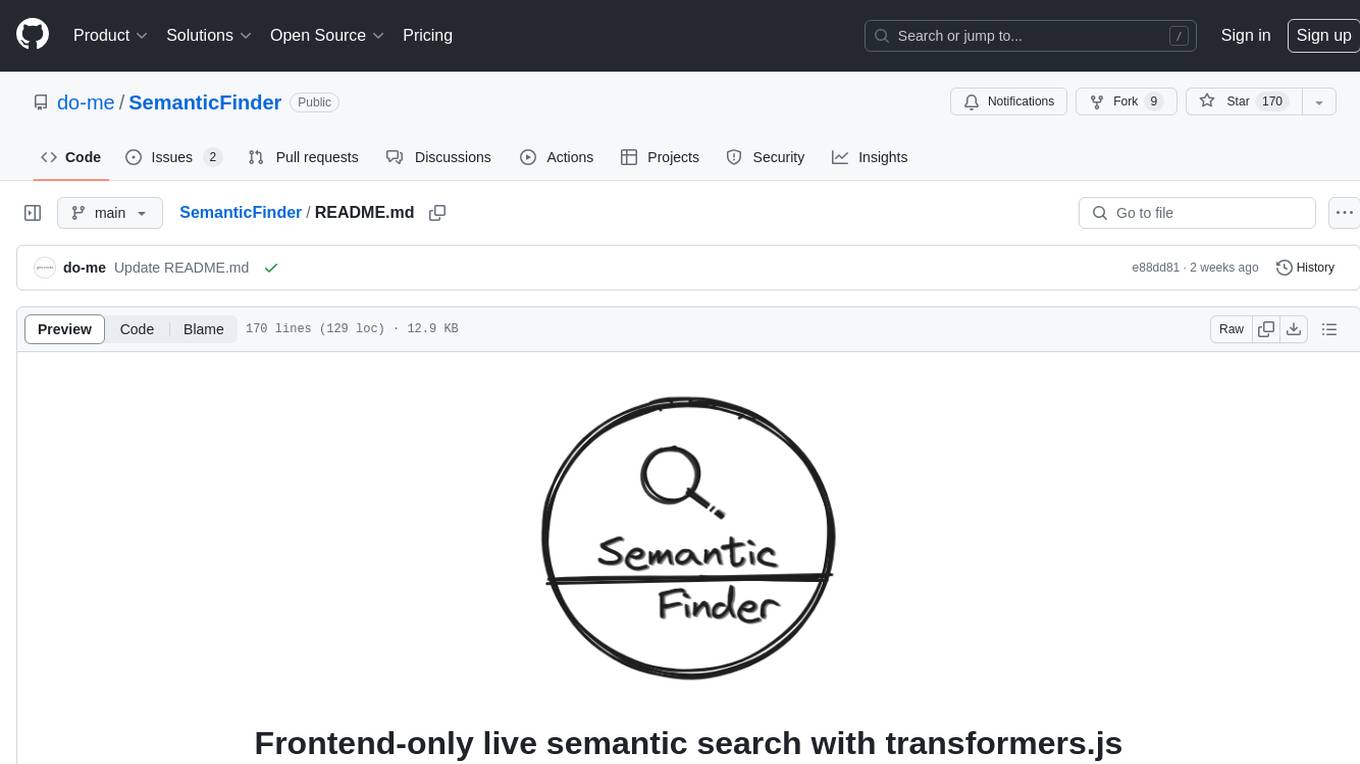
SemanticFinder
SemanticFinder is a frontend-only live semantic search tool that calculates embeddings and cosine similarity client-side using transformers.js and SOTA embedding models from Huggingface. It allows users to search through large texts like books with pre-indexed examples, customize search parameters, and offers data privacy by keeping input text in the browser. The tool can be used for basic search tasks, analyzing texts for recurring themes, and has potential integrations with various applications like wikis, chat apps, and personal history search. It also provides options for building browser extensions and future ideas for further enhancements and integrations.
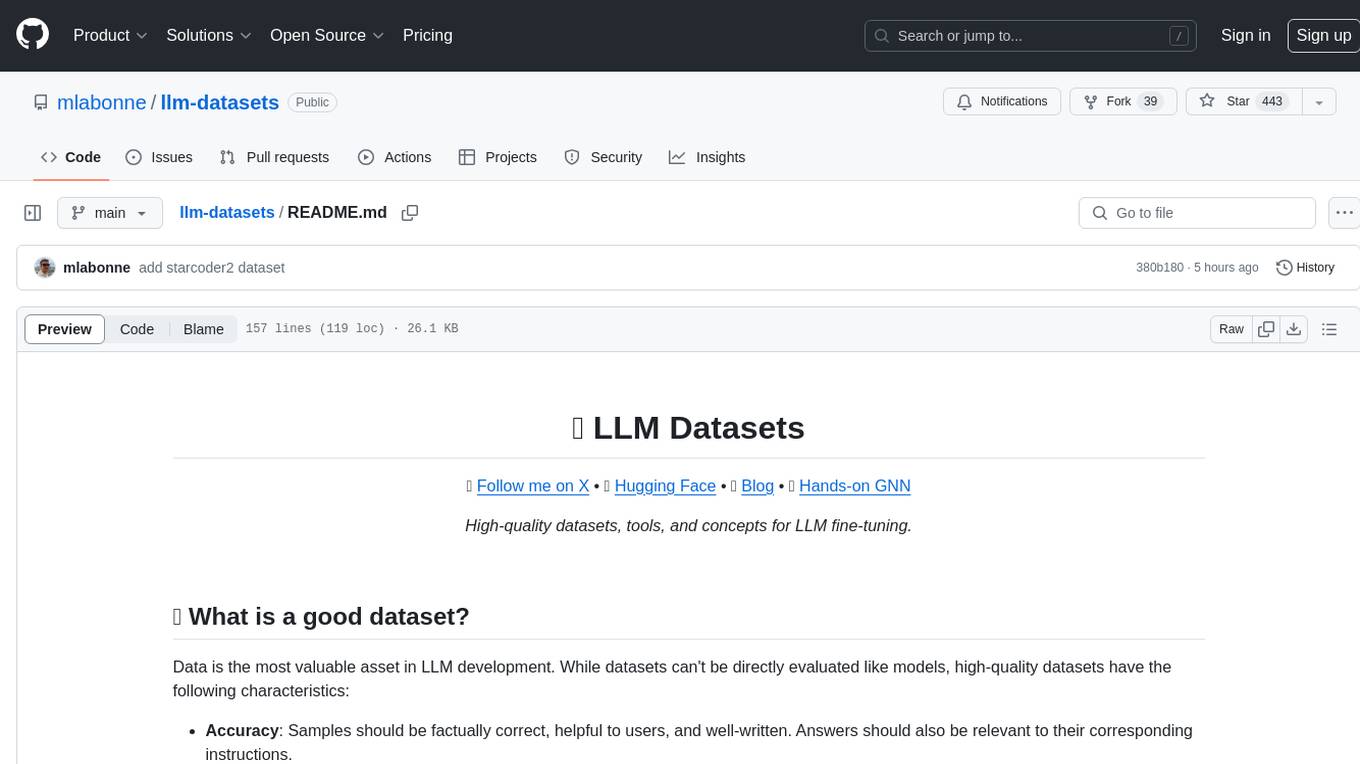
llm-datasets
LLM Datasets is a repository containing high-quality datasets, tools, and concepts for LLM fine-tuning. It provides datasets with characteristics like accuracy, diversity, and complexity to train large language models for various tasks. The repository includes datasets for general-purpose, math & logic, code, conversation & role-play, and agent & function calling domains. It also offers guidance on creating high-quality datasets through data deduplication, data quality assessment, data exploration, and data generation techniques.
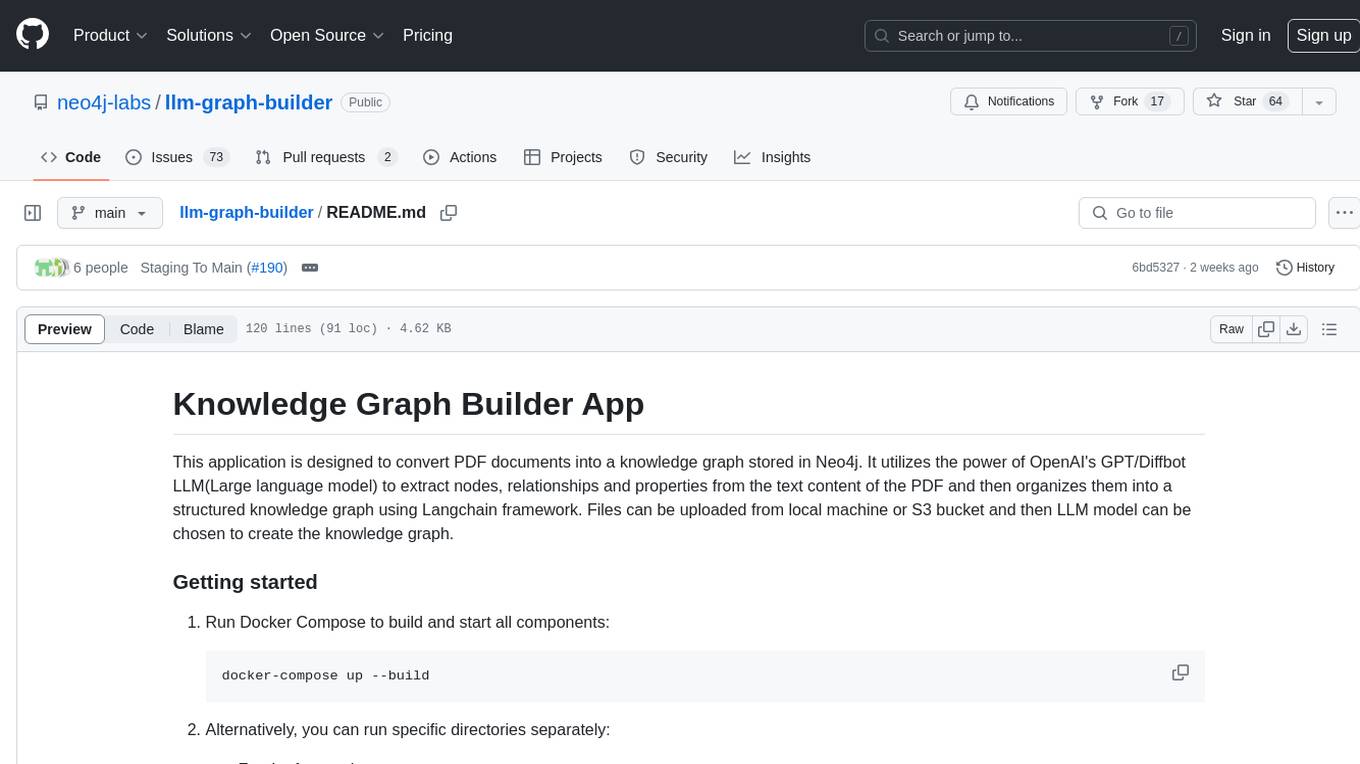
llm-graph-builder
Knowledge Graph Builder App is a tool designed to convert PDF documents into a structured knowledge graph stored in Neo4j. It utilizes OpenAI's GPT/Diffbot LLM to extract nodes, relationships, and properties from PDF text content. Users can upload files from local machine or S3 bucket, choose LLM model, and create a knowledge graph. The app integrates with Neo4j for easy visualization and querying of extracted information.
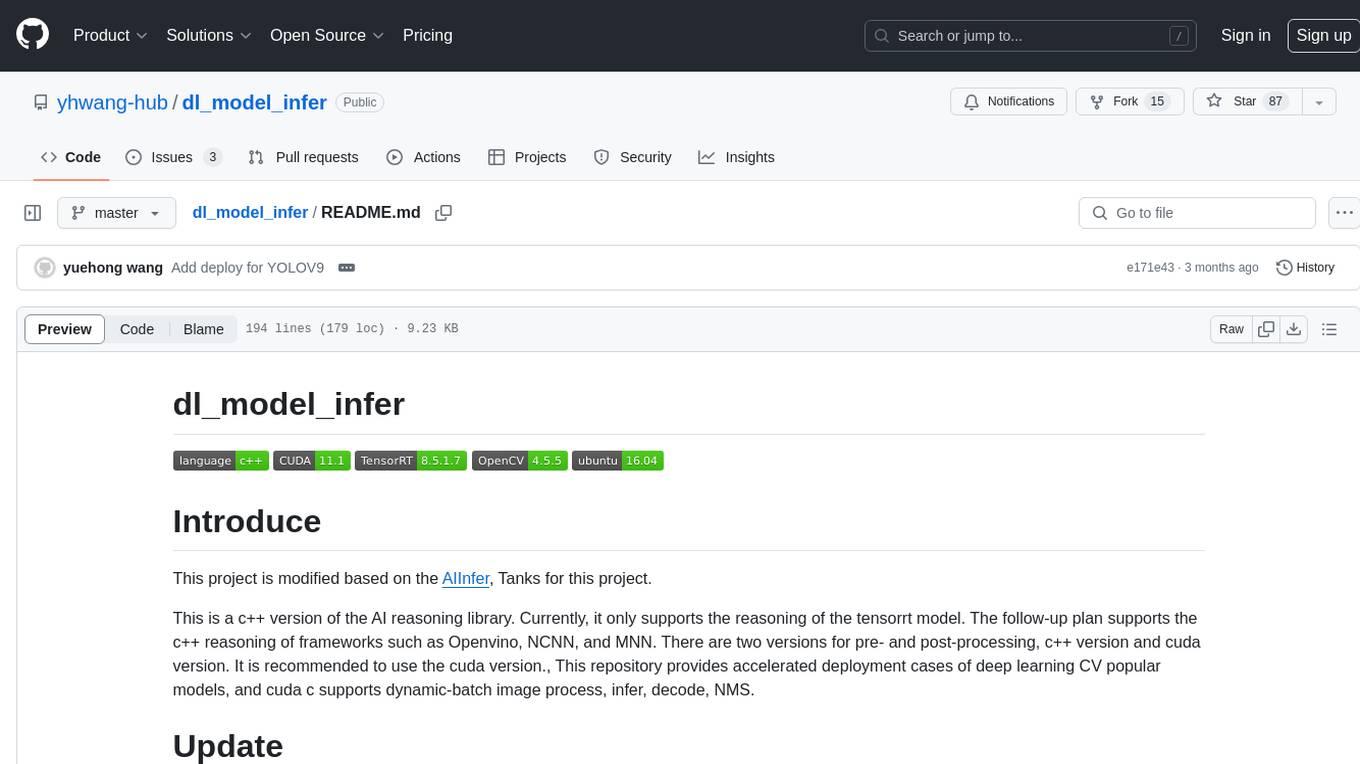
dl_model_infer
This project is a c++ version of the AI reasoning library that supports the reasoning of tensorrt models. It provides accelerated deployment cases of deep learning CV popular models and supports dynamic-batch image processing, inference, decode, and NMS. The project has been updated with various models and provides tutorials for model exports. It also includes a producer-consumer inference model for specific tasks. The project directory includes implementations for model inference applications, backend reasoning classes, post-processing, pre-processing, and target detection and tracking. Speed tests have been conducted on various models, and onnx downloads are available for different models.
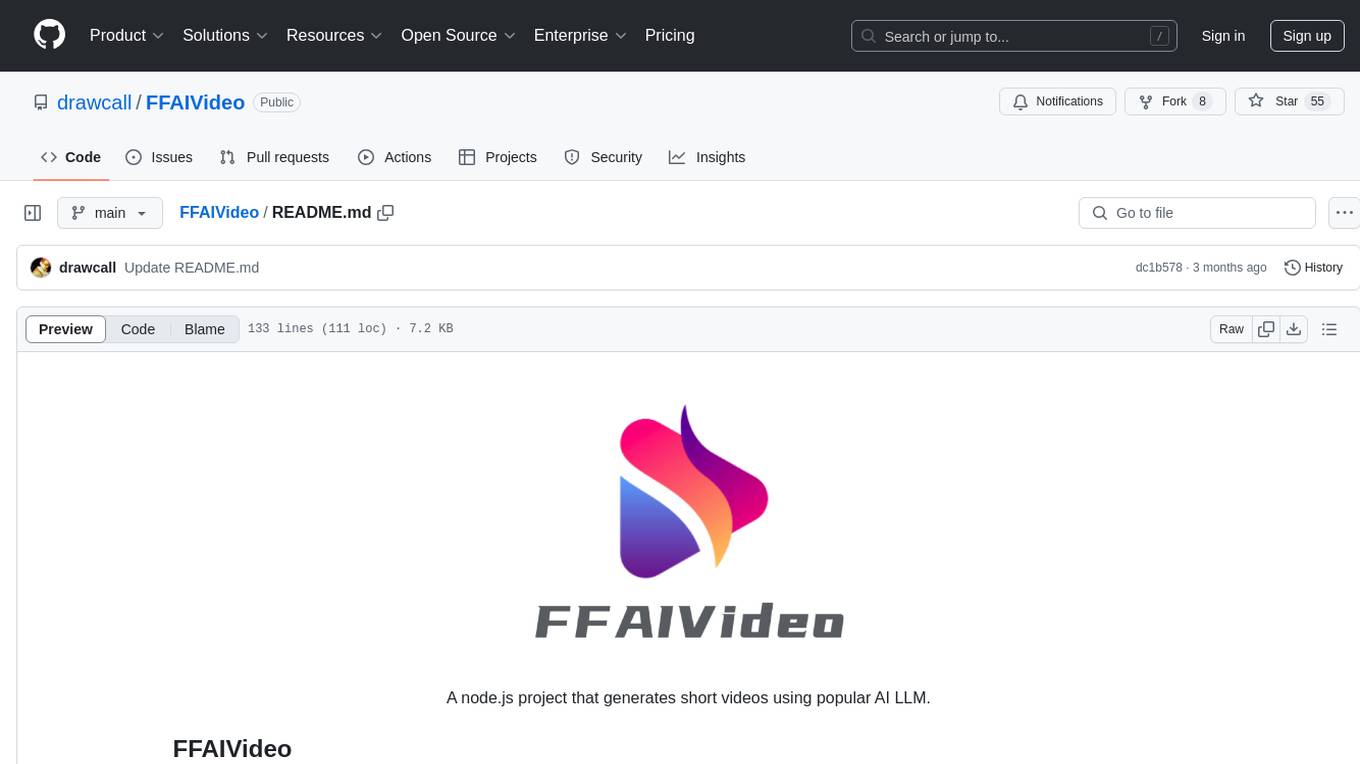
FFAIVideo
FFAIVideo is a lightweight node.js project that utilizes popular AI LLM to intelligently generate short videos. It supports multiple AI LLM models such as OpenAI, Moonshot, Azure, g4f, Google Gemini, etc. Users can input text to automatically synthesize exciting video content with subtitles, background music, and customizable settings. The project integrates Microsoft Edge's online text-to-speech service for voice options and uses Pexels website for video resources. Installation of FFmpeg is essential for smooth operation. Inspired by MoneyPrinterTurbo, MoneyPrinter, and MsEdgeTTS, FFAIVideo is designed for front-end developers with minimal dependencies and simple usage.
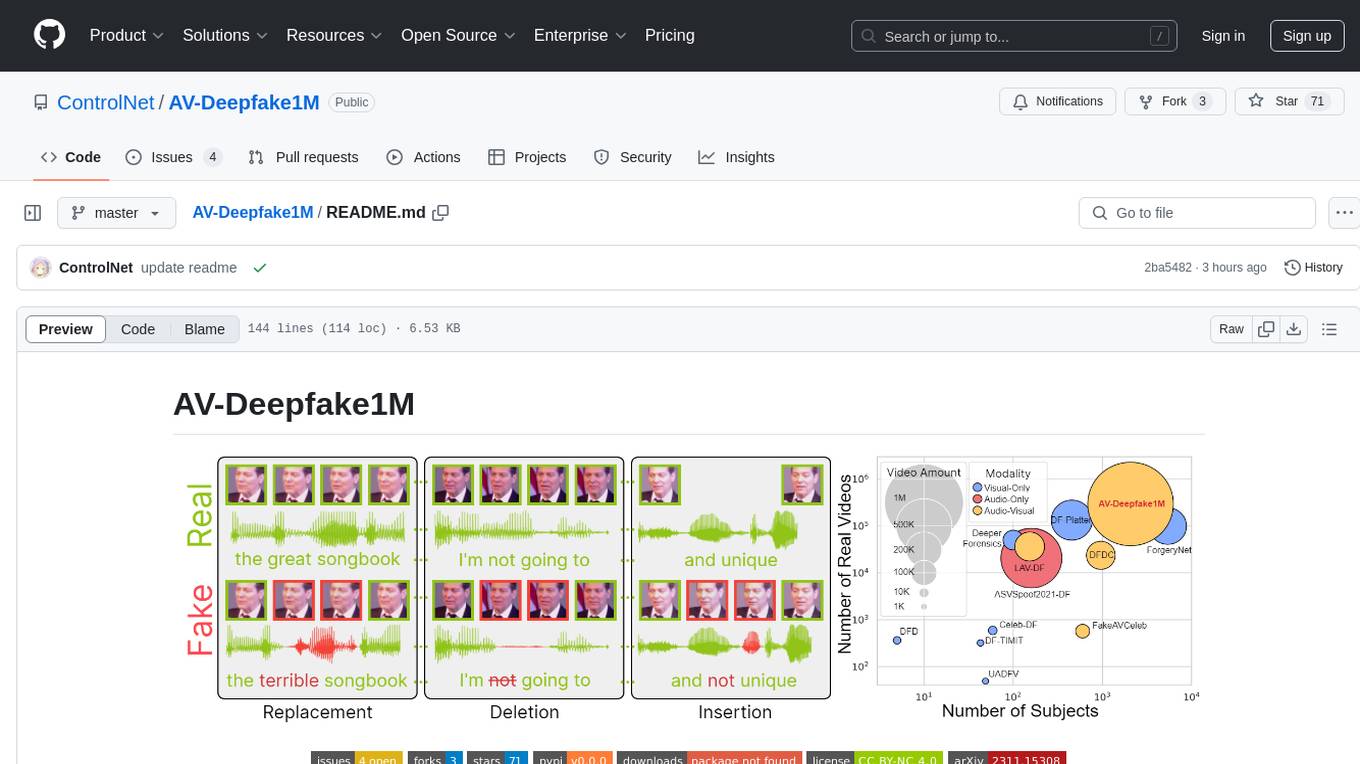
AV-Deepfake1M
The AV-Deepfake1M repository is the official repository for the paper AV-Deepfake1M: A Large-Scale LLM-Driven Audio-Visual Deepfake Dataset. It addresses the challenge of detecting and localizing deepfake audio-visual content by proposing a dataset containing video manipulations, audio manipulations, and audio-visual manipulations for over 2K subjects resulting in more than 1M videos. The dataset is crucial for developing next-generation deepfake localization methods.
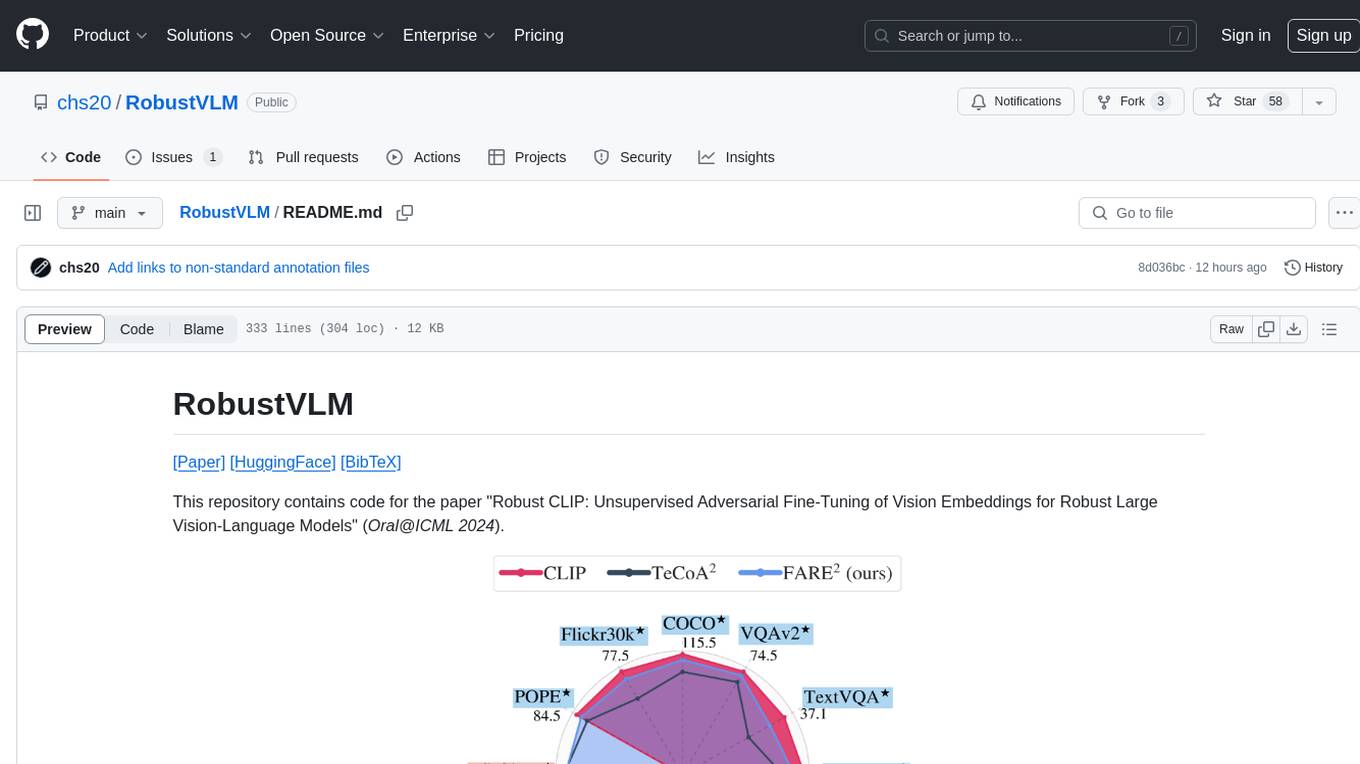
RobustVLM
This repository contains code for the paper 'Robust CLIP: Unsupervised Adversarial Fine-Tuning of Vision Embeddings for Robust Large Vision-Language Models'. It focuses on fine-tuning CLIP in an unsupervised manner to enhance its robustness against visual adversarial attacks. By replacing the vision encoder of large vision-language models with the fine-tuned CLIP models, it achieves state-of-the-art adversarial robustness on various vision-language tasks. The repository provides adversarially fine-tuned ViT-L/14 CLIP models and offers insights into zero-shot classification settings and clean accuracy improvements.
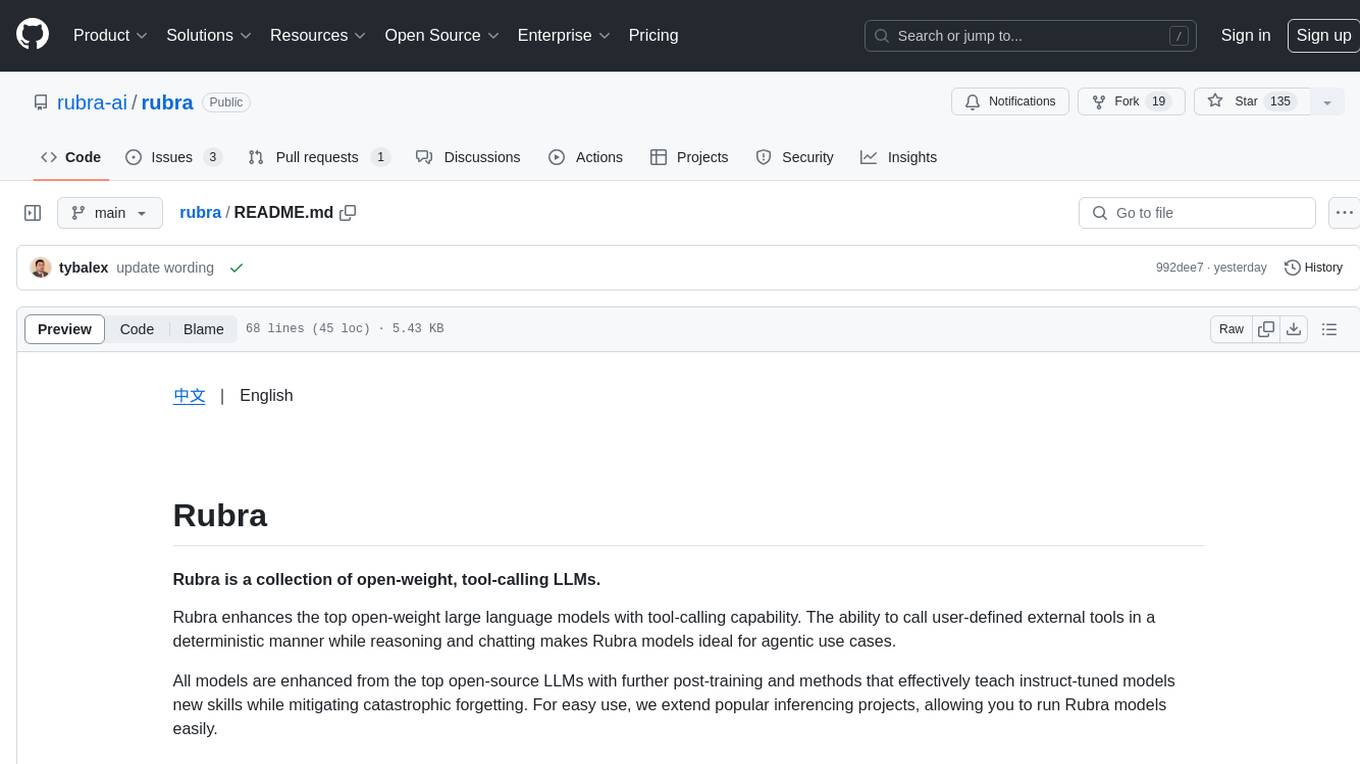
rubra
Rubra is a collection of open-weight large language models enhanced with tool-calling capability. It allows users to call user-defined external tools in a deterministic manner while reasoning and chatting, making it ideal for agentic use cases. The models are further post-trained to teach instruct-tuned models new skills and mitigate catastrophic forgetting. Rubra extends popular inferencing projects for easy use, enabling users to run the models easily.
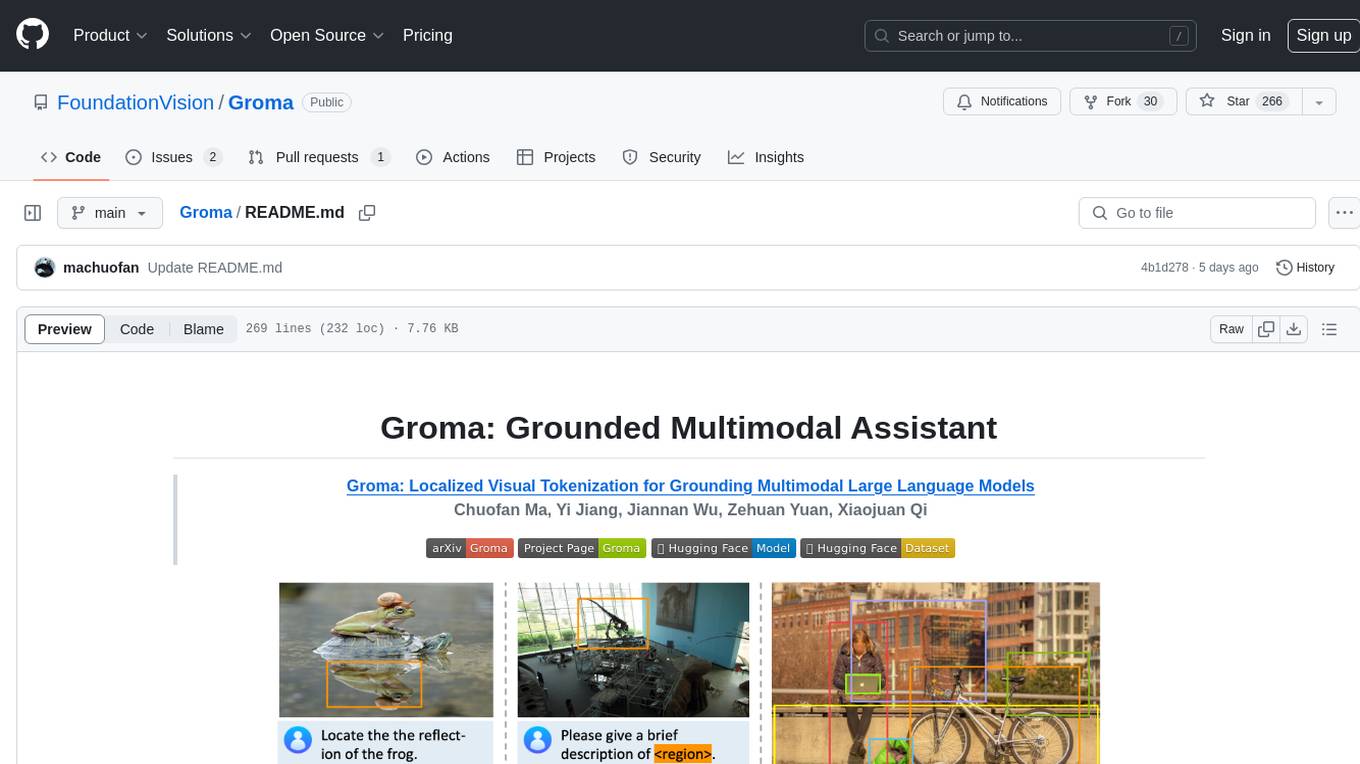
Groma
Groma is a grounded multimodal assistant that excels in region understanding and visual grounding. It can process user-defined region inputs and generate contextually grounded long-form responses. The tool presents a unique paradigm for multimodal large language models, focusing on visual tokenization for localization. Groma achieves state-of-the-art performance in referring expression comprehension benchmarks. The tool provides pretrained model weights and instructions for data preparation, training, inference, and evaluation. Users can customize training by starting from intermediate checkpoints. Groma is designed to handle tasks related to detection pretraining, alignment pretraining, instruction finetuning, instruction following, and more.
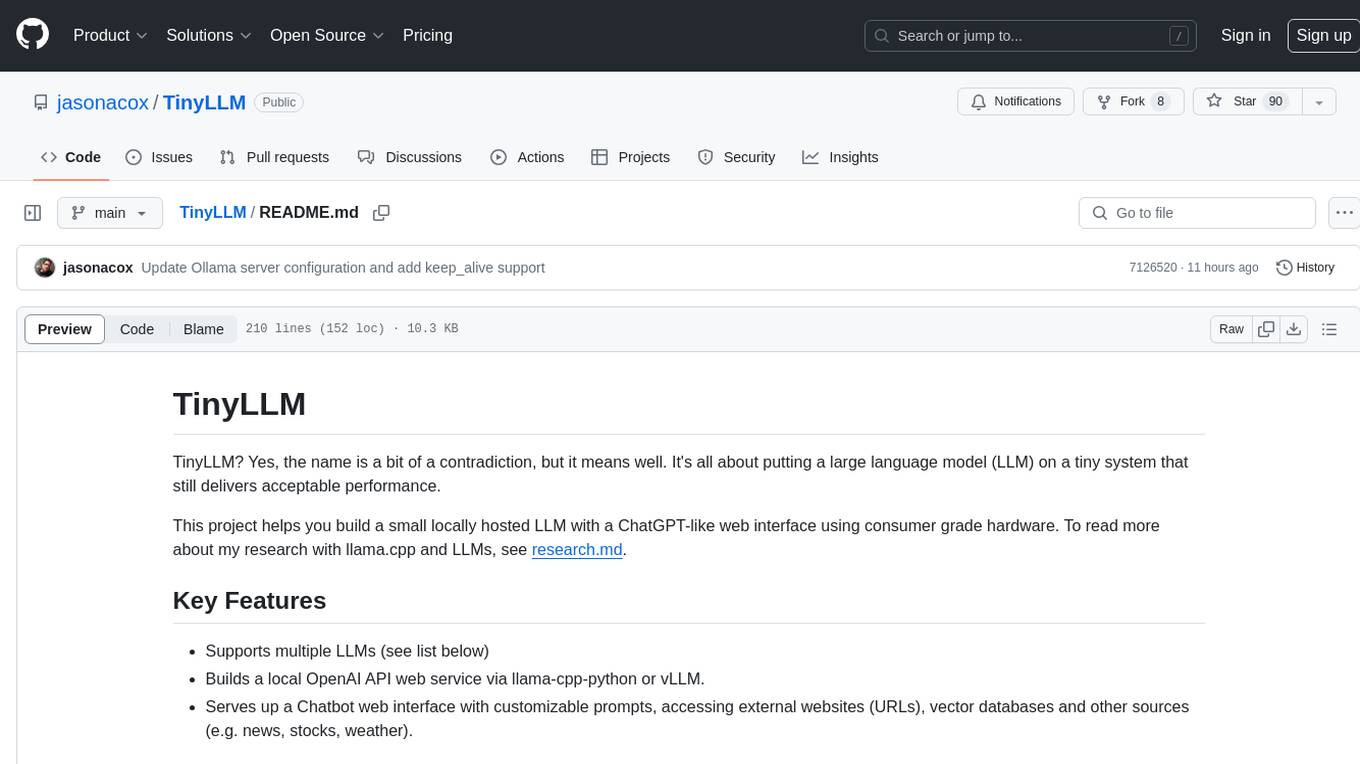
TinyLLM
TinyLLM is a project that helps build a small locally hosted language model with a web interface using consumer-grade hardware. It supports multiple language models, builds a local OpenAI API web service, and serves a Chatbot web interface with customizable prompts. The project requires specific hardware and software configurations for optimal performance. Users can run a local language model using inference servers like vLLM, llama-cpp-python, and Ollama. The Chatbot feature allows users to interact with the language model through a web-based interface, supporting features like summarizing websites, displaying news headlines, stock prices, weather conditions, and using vector databases for queries.
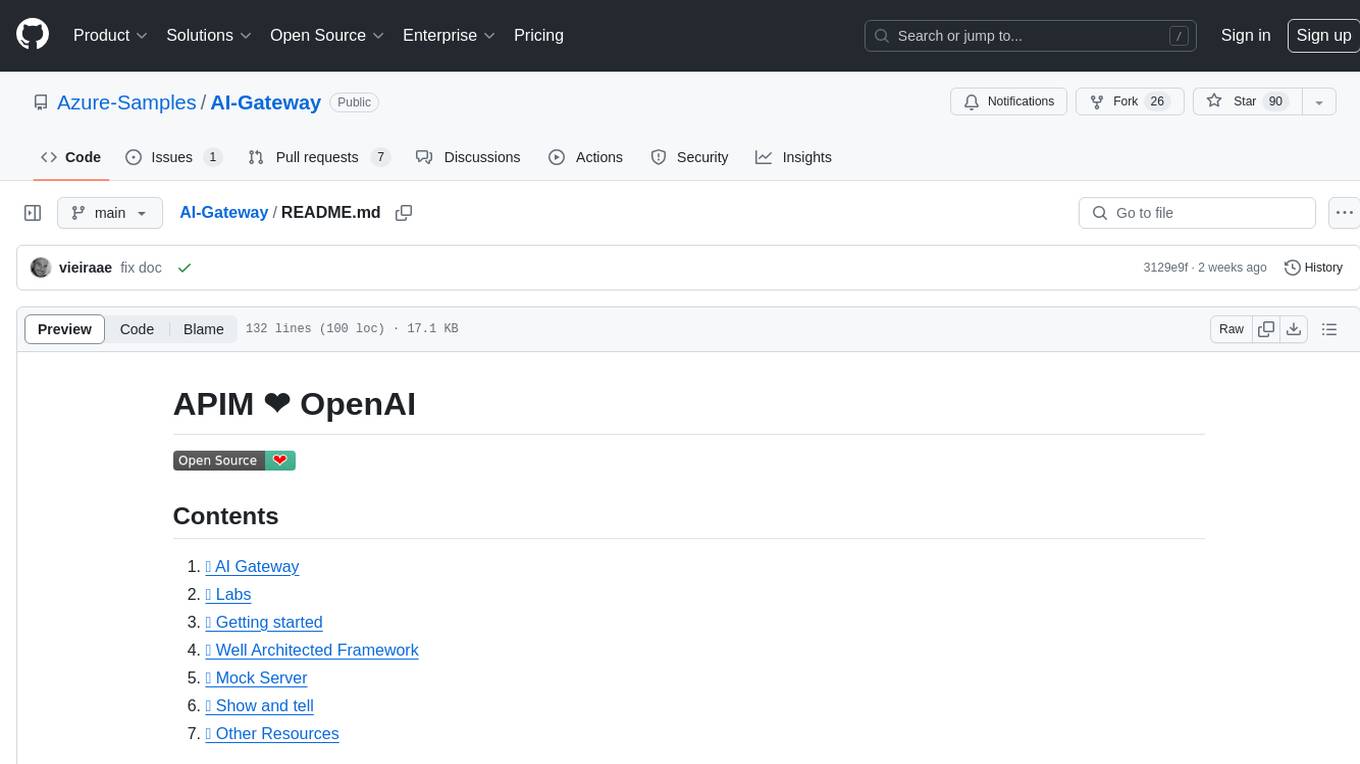
AI-Gateway
The AI-Gateway repository explores the AI Gateway pattern through a series of experimental labs, focusing on Azure API Management for handling AI services APIs. The labs provide step-by-step instructions using Jupyter notebooks with Python scripts, Bicep files, and APIM policies. The goal is to accelerate experimentation of advanced use cases and pave the way for further innovation in the rapidly evolving field of AI. The repository also includes a Mock Server to mimic the behavior of the OpenAI API for testing and development purposes.
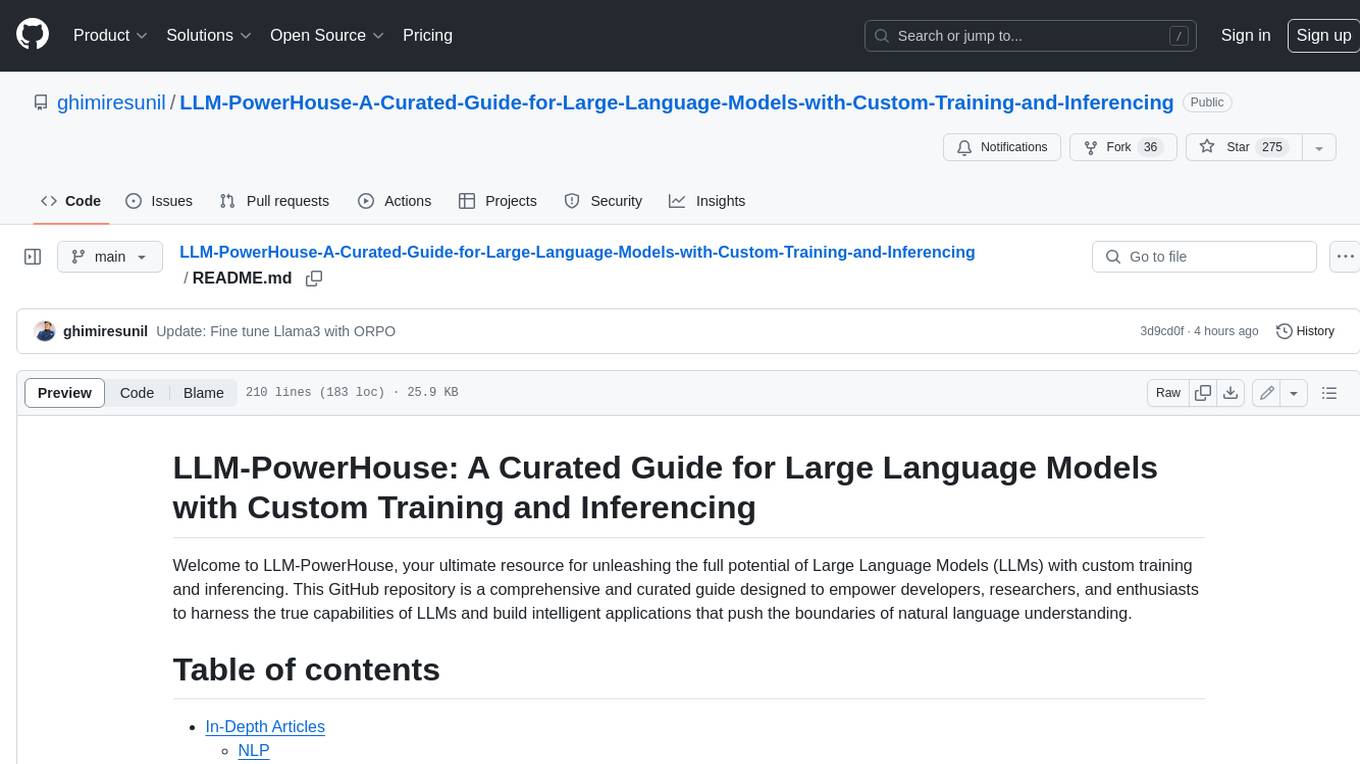
LLM-PowerHouse-A-Curated-Guide-for-Large-Language-Models-with-Custom-Training-and-Inferencing
LLM-PowerHouse is a comprehensive and curated guide designed to empower developers, researchers, and enthusiasts to harness the true capabilities of Large Language Models (LLMs) and build intelligent applications that push the boundaries of natural language understanding. This GitHub repository provides in-depth articles, codebase mastery, LLM PlayLab, and resources for cost analysis and network visualization. It covers various aspects of LLMs, including NLP, models, training, evaluation metrics, open LLMs, and more. The repository also includes a collection of code examples and tutorials to help users build and deploy LLM-based applications.
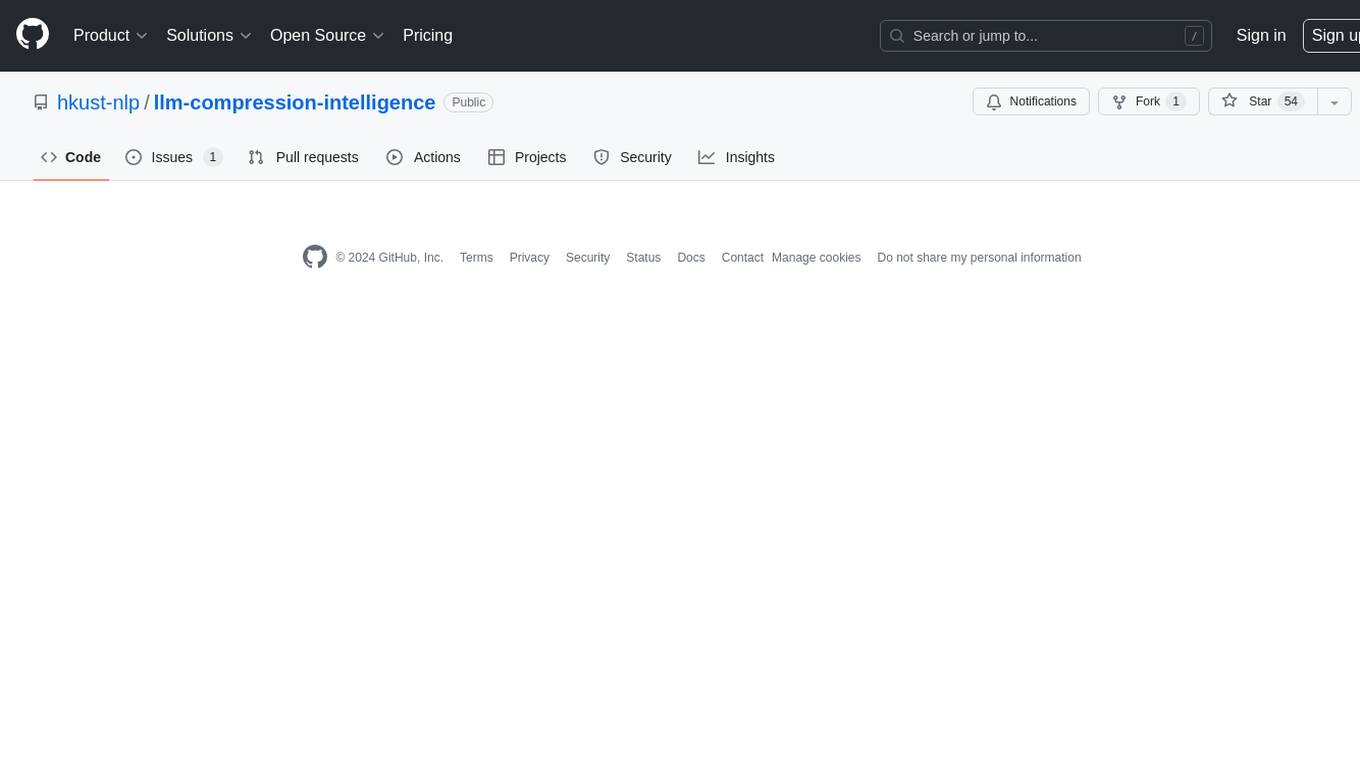
llm-compression-intelligence
This repository presents the findings of the paper "Compression Represents Intelligence Linearly". The study reveals a strong linear correlation between the intelligence of LLMs, as measured by benchmark scores, and their ability to compress external text corpora. Compression efficiency, derived from raw text corpora, serves as a reliable evaluation metric that is linearly associated with model capabilities. The repository includes the compression corpora used in the paper, code for computing compression efficiency, and data collection and processing pipelines.
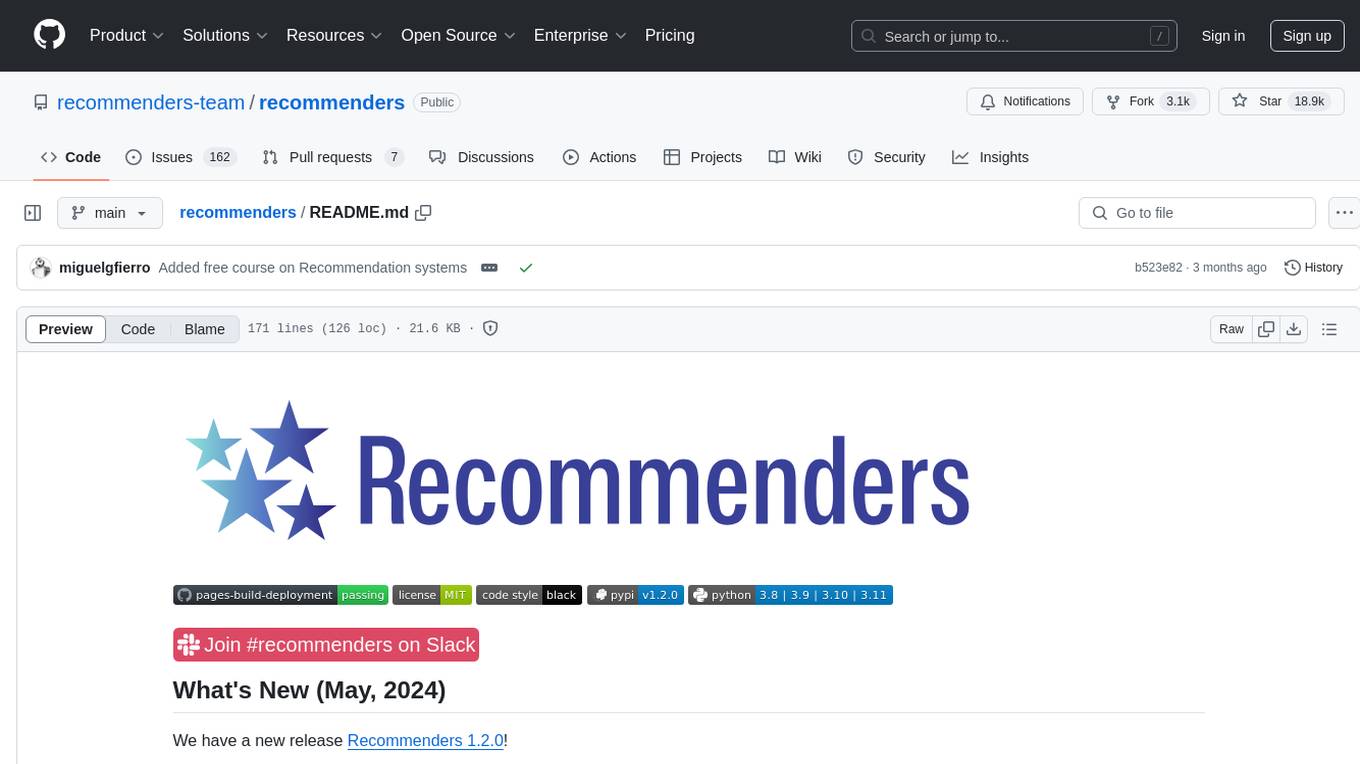
recommenders
Recommenders is a project under the Linux Foundation of AI and Data that assists researchers, developers, and enthusiasts in prototyping, experimenting with, and bringing to production a range of classic and state-of-the-art recommendation systems. The repository contains examples and best practices for building recommendation systems, provided as Jupyter notebooks. It covers tasks such as preparing data, building models using various recommendation algorithms, evaluating algorithms, tuning hyperparameters, and operationalizing models in a production environment on Azure. The project provides utilities to support common tasks like loading datasets, evaluating model outputs, and splitting training/test data. It includes implementations of state-of-the-art algorithms for self-study and customization in applications.
For similar tasks
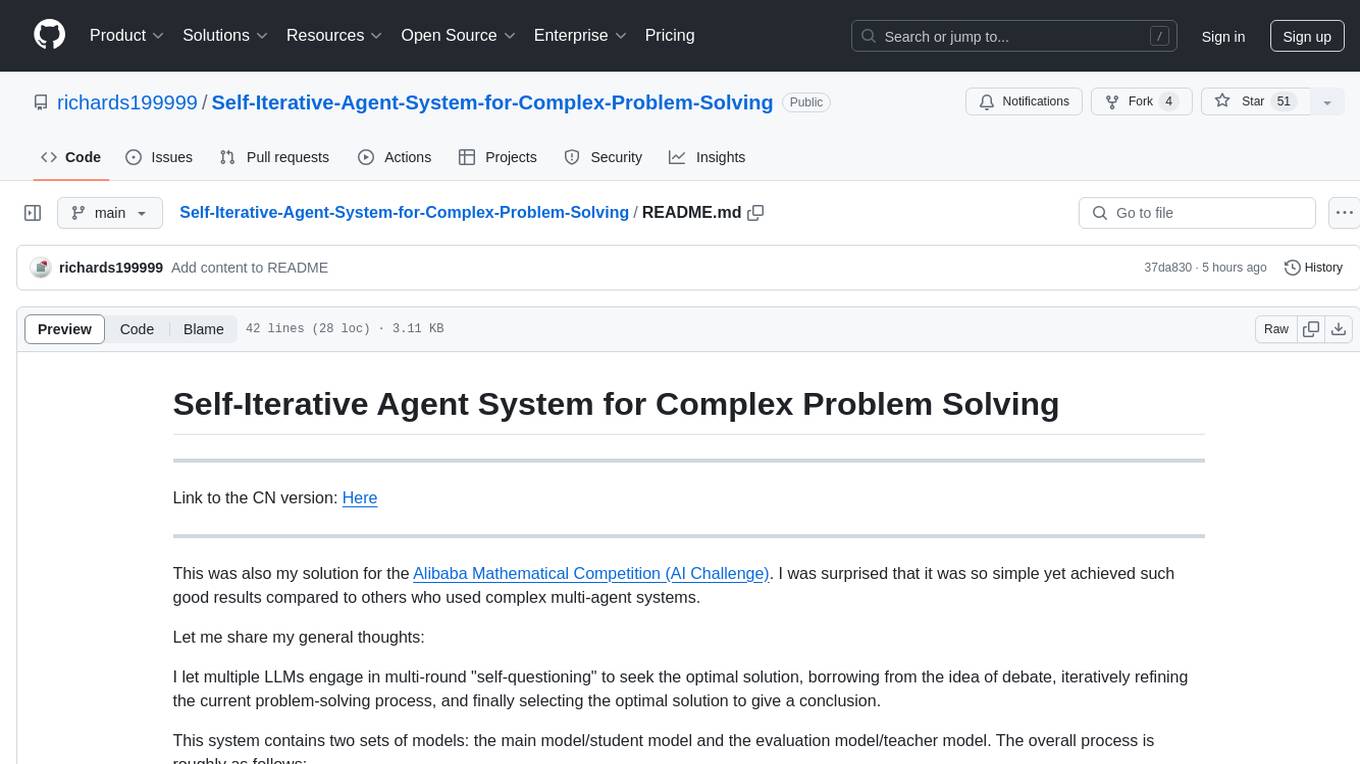
Self-Iterative-Agent-System-for-Complex-Problem-Solving
The Self-Iterative Agent System for Complex Problem Solving is a solution developed for the Alibaba Mathematical Competition (AI Challenge). It involves multiple LLMs engaging in multi-round 'self-questioning' to iteratively refine the problem-solving process and select optimal solutions. The system consists of main and evaluation models, with a process that includes detailed problem-solving steps, feedback loops, and iterative improvements. The approach emphasizes communication and reasoning between sub-agents, knowledge extraction, and the importance of Agent-like architectures in complex tasks. While effective, there is room for improvement in model capabilities and error prevention mechanisms.
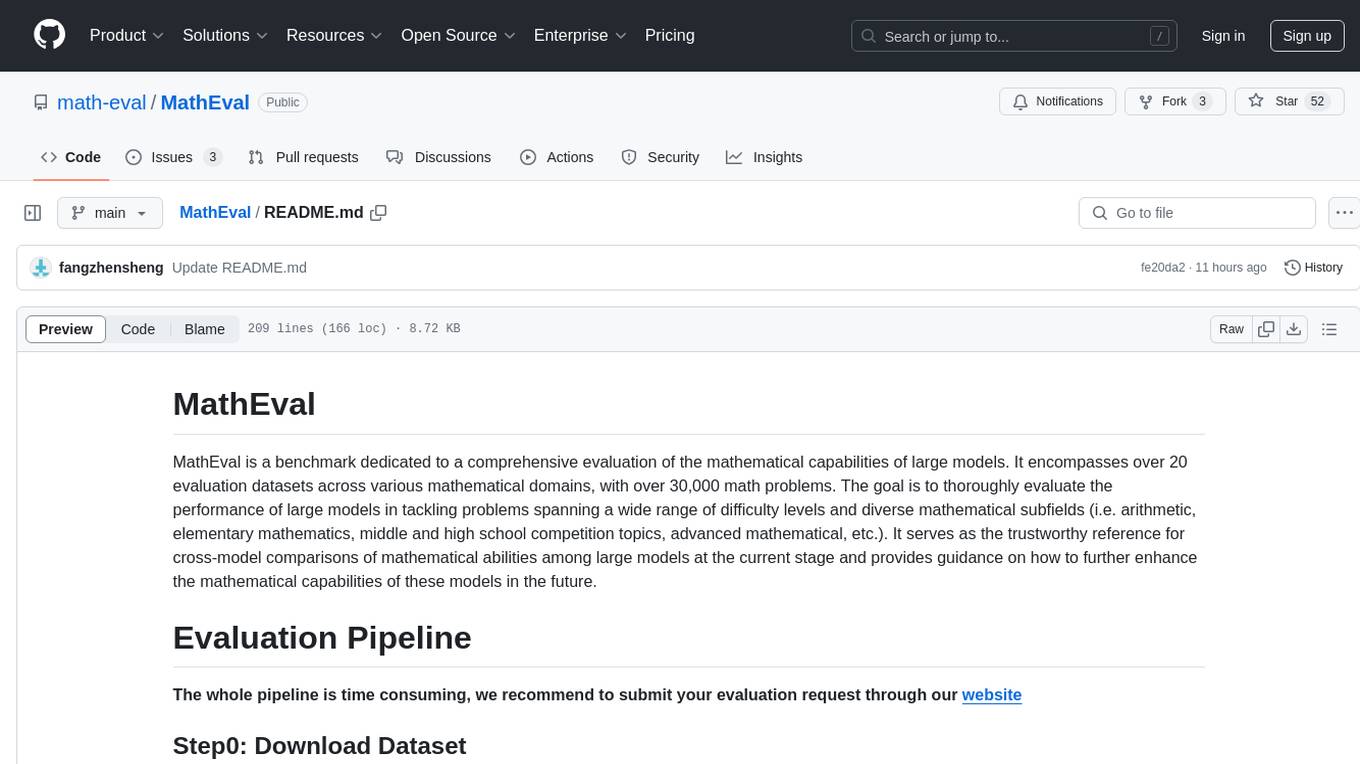
MathEval
MathEval is a benchmark designed for evaluating the mathematical capabilities of large models. It includes over 20 evaluation datasets covering various mathematical domains with more than 30,000 math problems. The goal is to assess the performance of large models across different difficulty levels and mathematical subfields. MathEval serves as a reliable reference for comparing mathematical abilities among large models and offers guidance on enhancing their mathematical capabilities in the future.
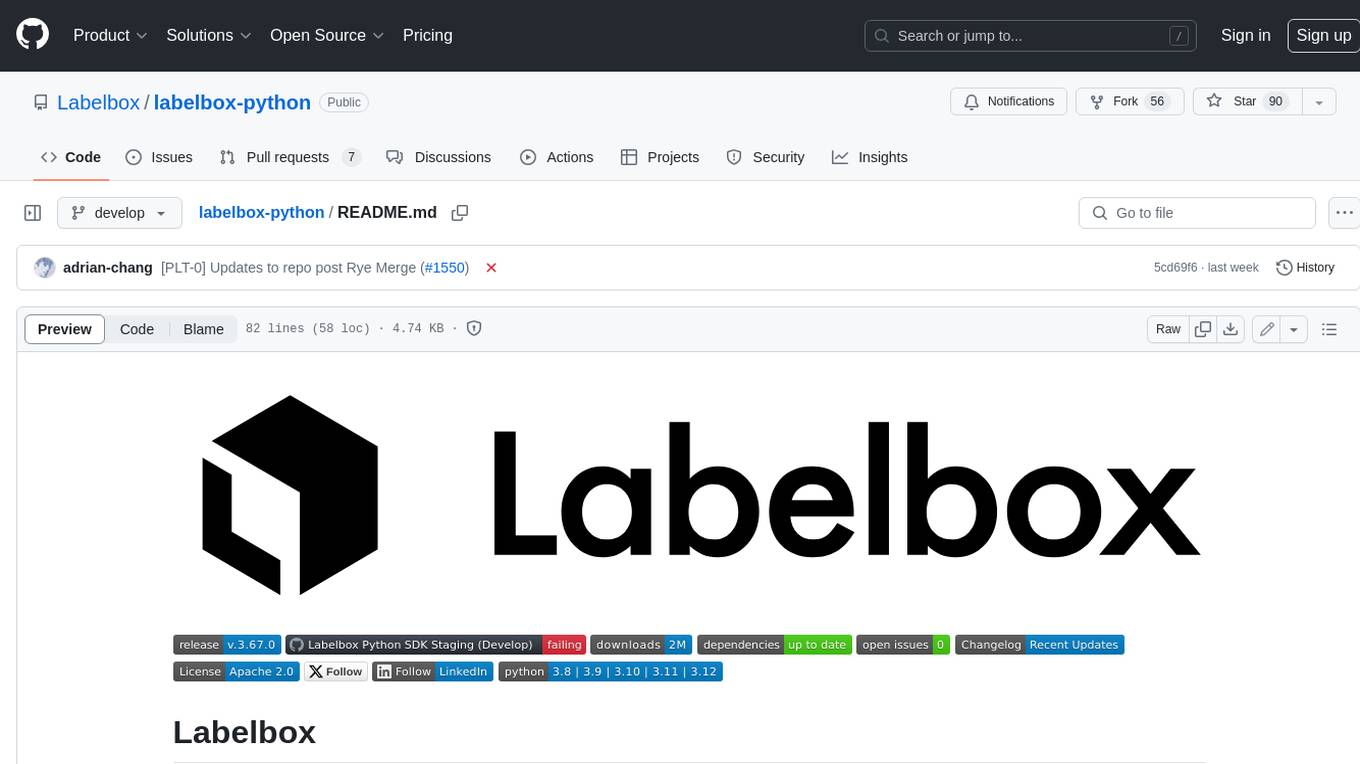
labelbox-python
Labelbox is a data-centric AI platform for enterprises to develop, optimize, and use AI to solve problems and power new products and services. Enterprises use Labelbox to curate data, generate high-quality human feedback data for computer vision and LLMs, evaluate model performance, and automate tasks by combining AI and human-centric workflows. The academic & research community uses Labelbox for cutting-edge AI research.
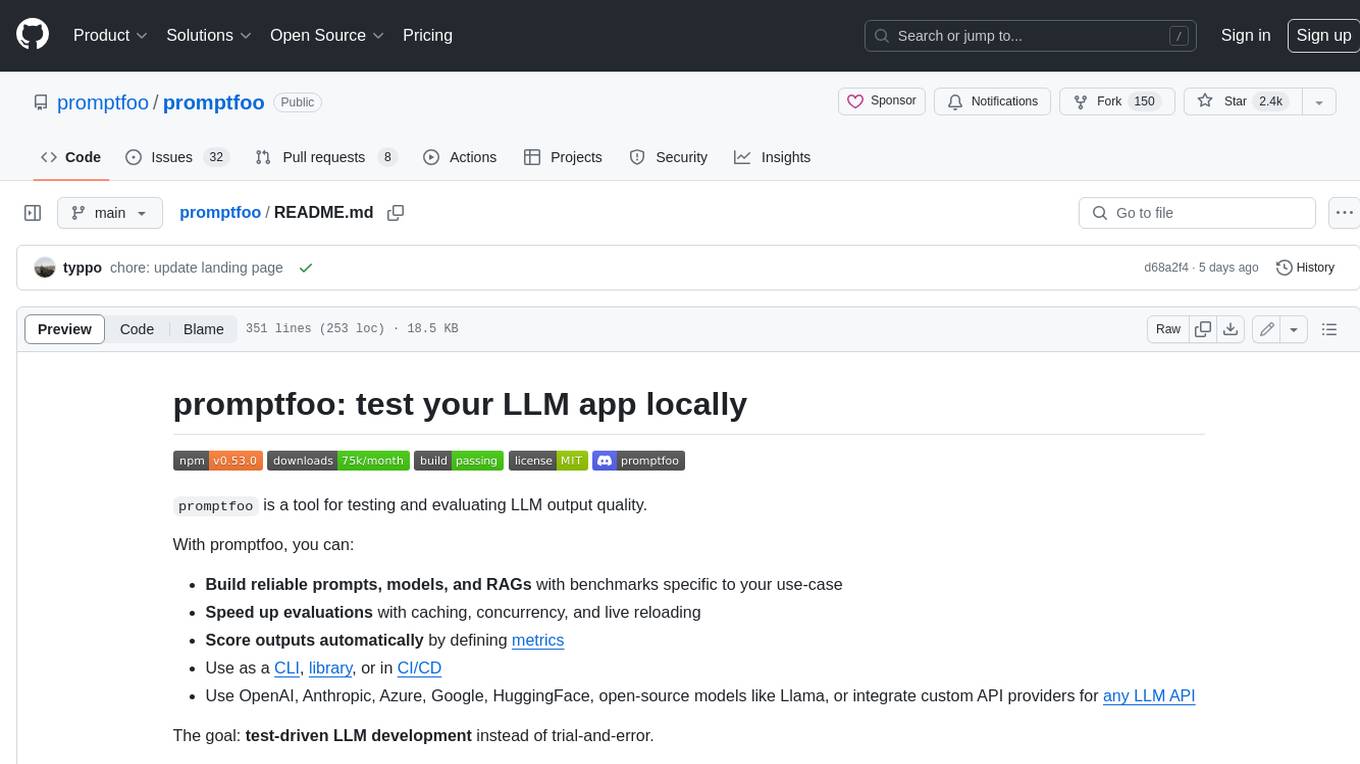
promptfoo
Promptfoo is a tool for testing and evaluating LLM output quality. With promptfoo, you can build reliable prompts, models, and RAGs with benchmarks specific to your use-case, speed up evaluations with caching, concurrency, and live reloading, score outputs automatically by defining metrics, use as a CLI, library, or in CI/CD, and use OpenAI, Anthropic, Azure, Google, HuggingFace, open-source models like Llama, or integrate custom API providers for any LLM API.
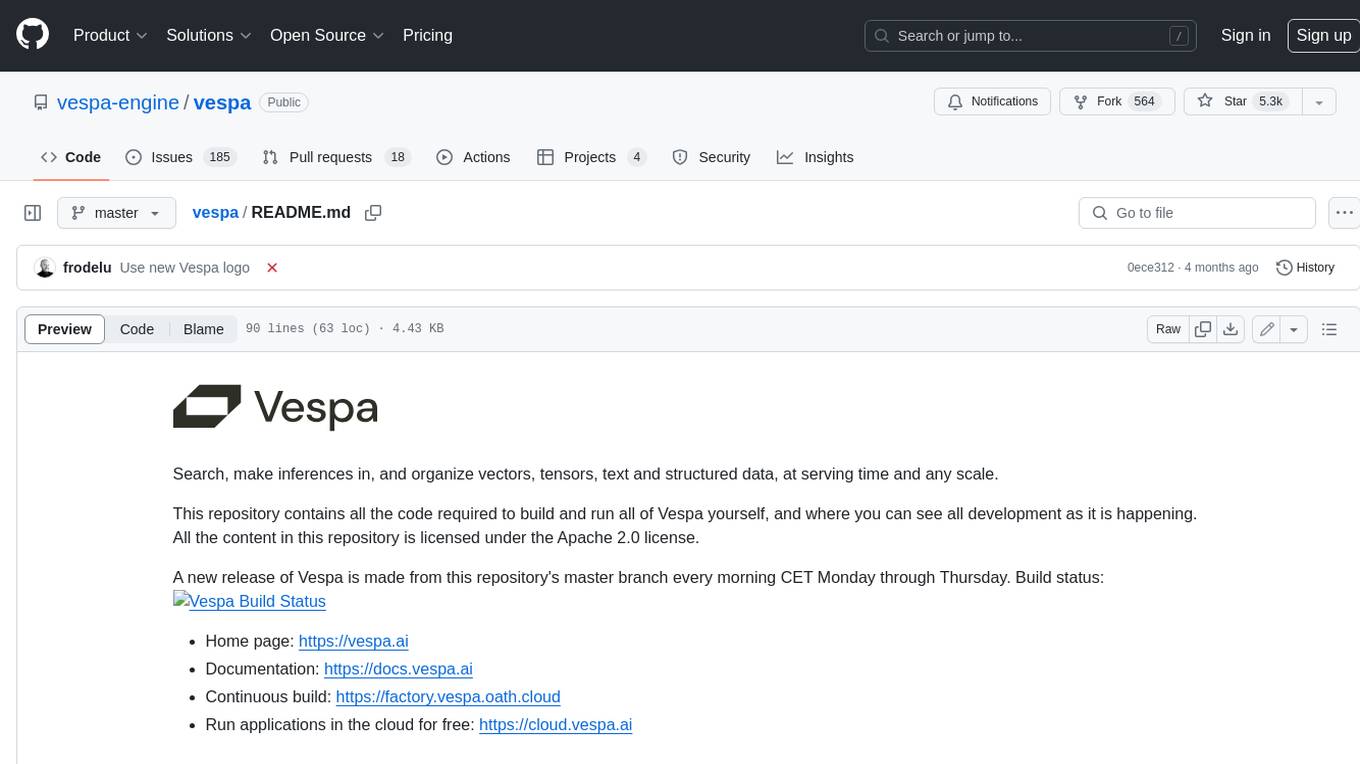
vespa
Vespa is a platform that performs operations such as selecting a subset of data in a large corpus, evaluating machine-learned models over the selected data, organizing and aggregating it, and returning it, typically in less than 100 milliseconds, all while the data corpus is continuously changing. It has been in development for many years and is used on a number of large internet services and apps which serve hundreds of thousands of queries from Vespa per second.
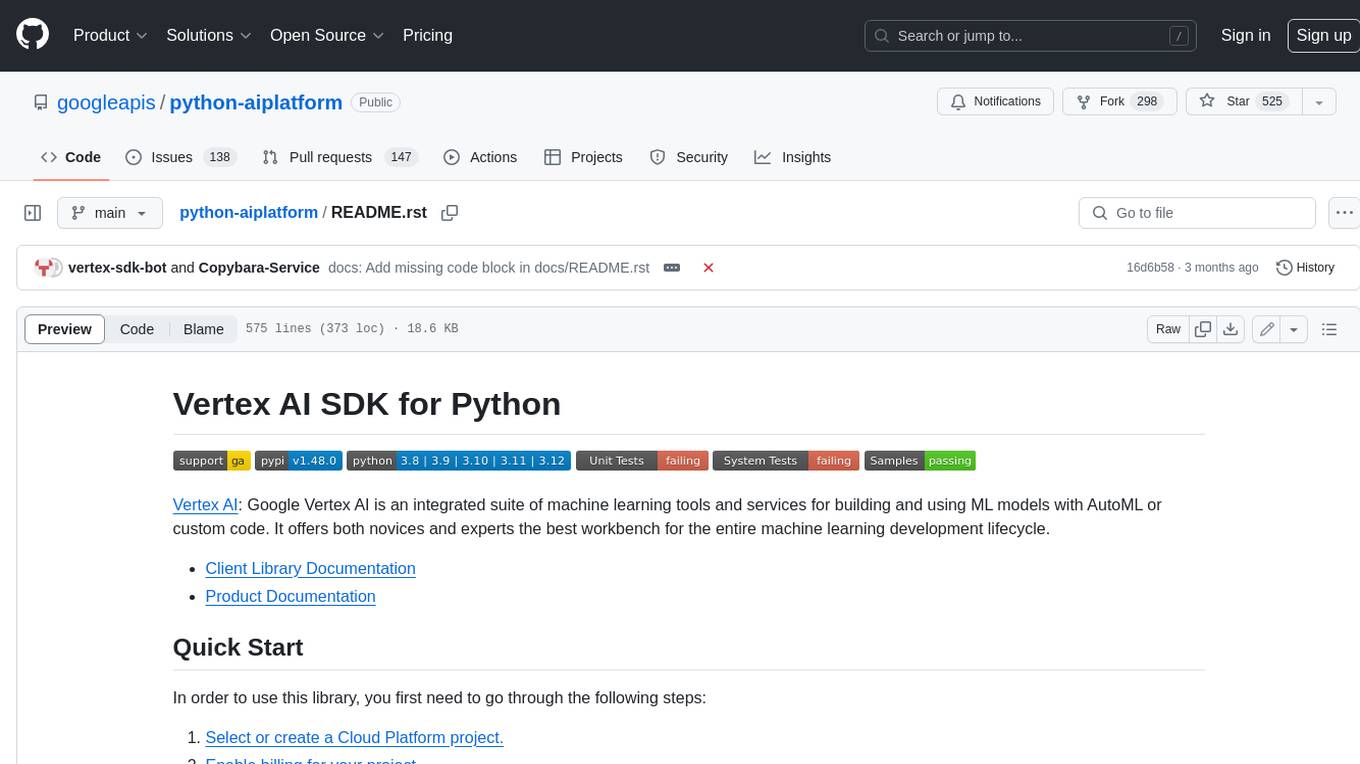
python-aiplatform
The Vertex AI SDK for Python is a library that provides a convenient way to use the Vertex AI API. It offers a high-level interface for creating and managing Vertex AI resources, such as datasets, models, and endpoints. The SDK also provides support for training and deploying custom models, as well as using AutoML models. With the Vertex AI SDK for Python, you can quickly and easily build and deploy machine learning models on Vertex AI.
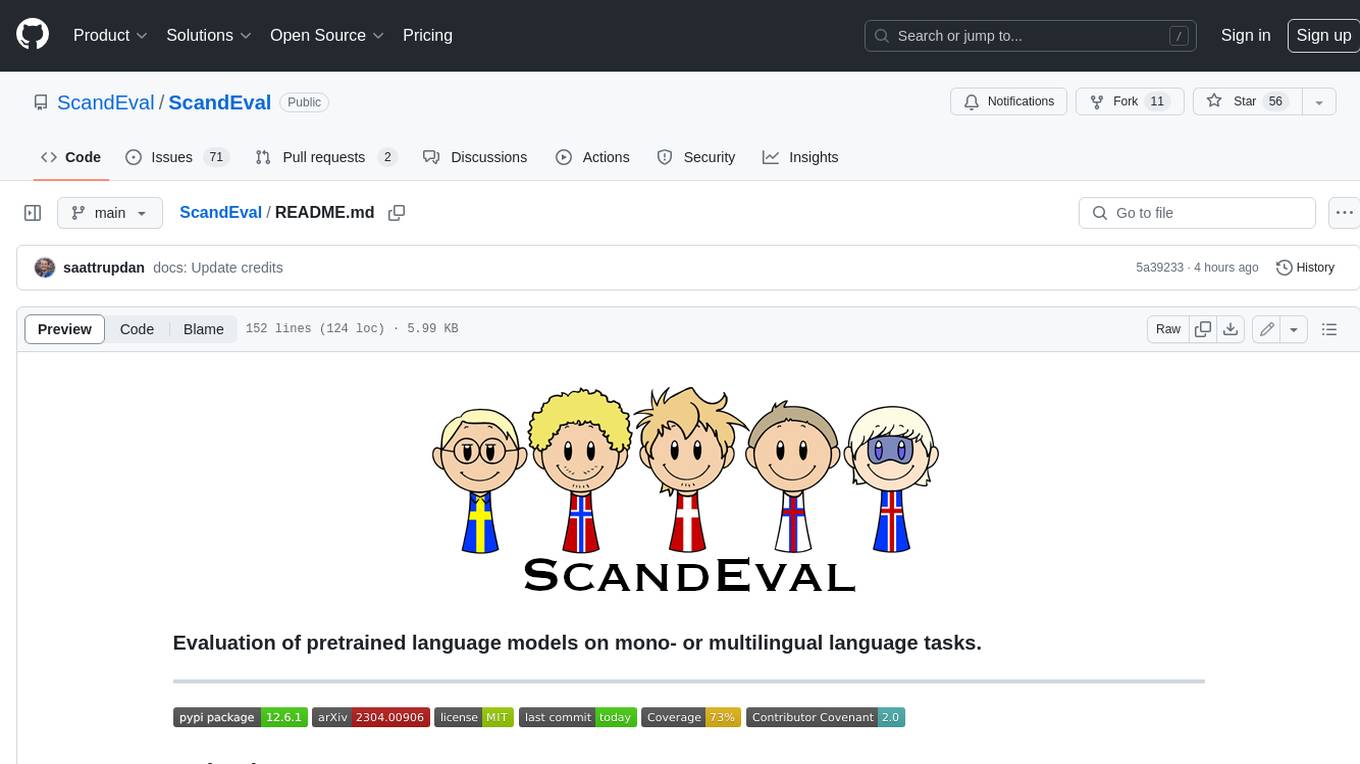
ScandEval
ScandEval is a framework for evaluating pretrained language models on mono- or multilingual language tasks. It provides a unified interface for benchmarking models on a variety of tasks, including sentiment analysis, question answering, and machine translation. ScandEval is designed to be easy to use and extensible, making it a valuable tool for researchers and practitioners alike.
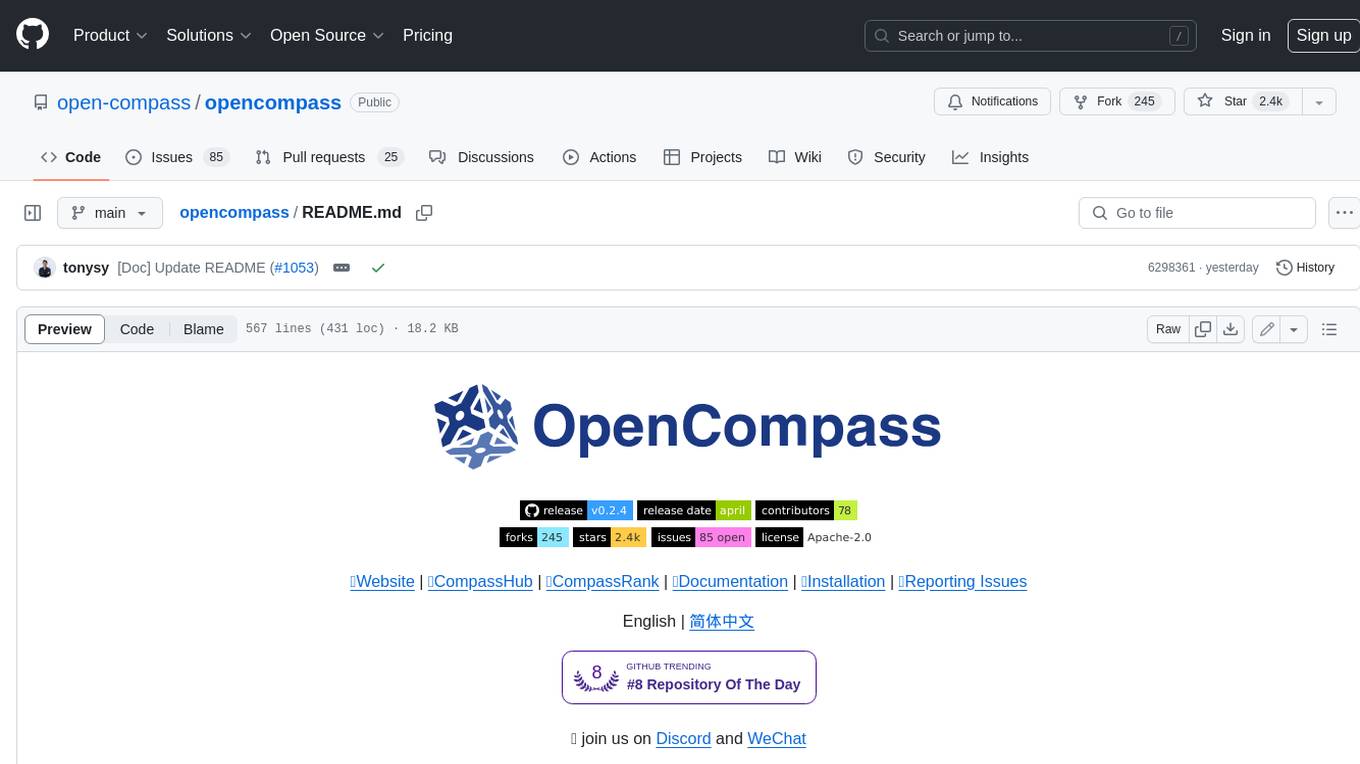
opencompass
OpenCompass is a one-stop platform for large model evaluation, aiming to provide a fair, open, and reproducible benchmark for large model evaluation. Its main features include: * Comprehensive support for models and datasets: Pre-support for 20+ HuggingFace and API models, a model evaluation scheme of 70+ datasets with about 400,000 questions, comprehensively evaluating the capabilities of the models in five dimensions. * Efficient distributed evaluation: One line command to implement task division and distributed evaluation, completing the full evaluation of billion-scale models in just a few hours. * Diversified evaluation paradigms: Support for zero-shot, few-shot, and chain-of-thought evaluations, combined with standard or dialogue-type prompt templates, to easily stimulate the maximum performance of various models. * Modular design with high extensibility: Want to add new models or datasets, customize an advanced task division strategy, or even support a new cluster management system? Everything about OpenCompass can be easily expanded! * Experiment management and reporting mechanism: Use config files to fully record each experiment, and support real-time reporting of results.
For similar jobs
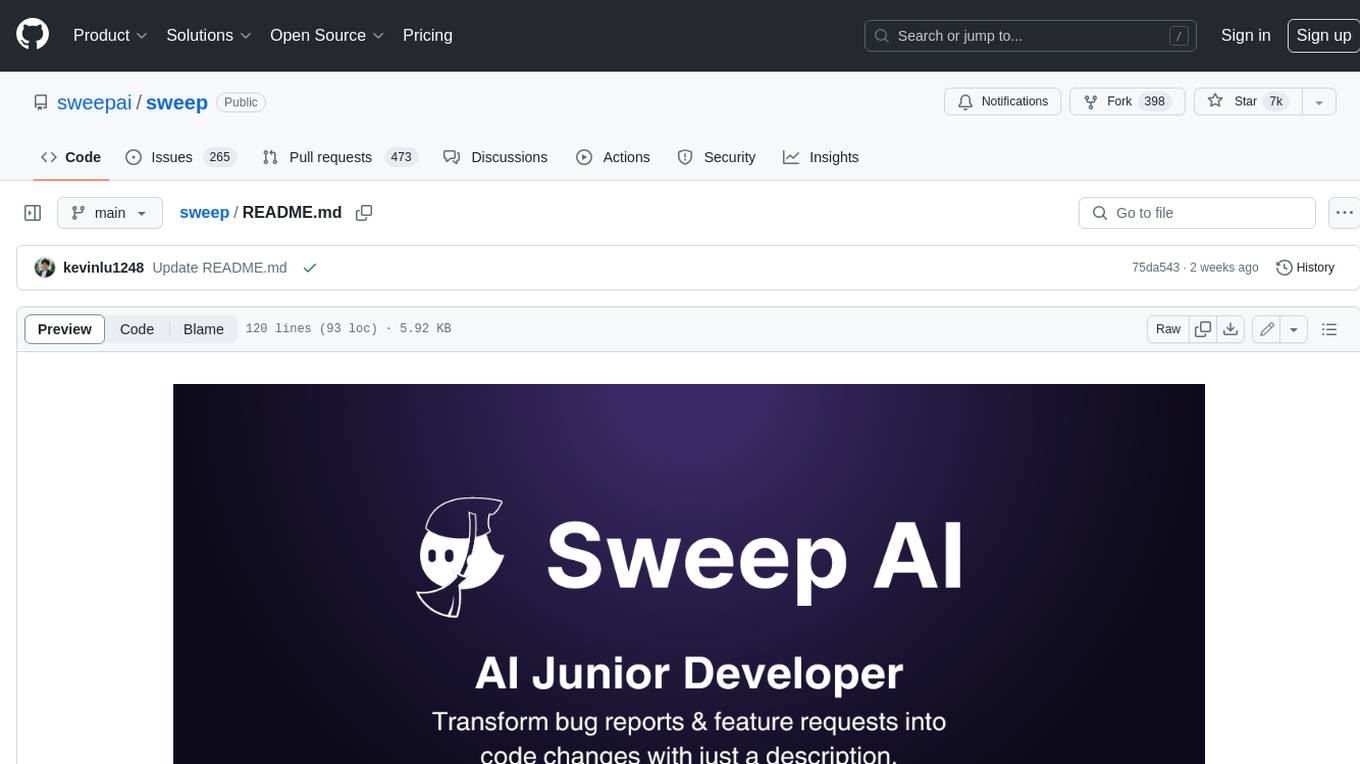
sweep
Sweep is an AI junior developer that turns bugs and feature requests into code changes. It automatically handles developer experience improvements like adding type hints and improving test coverage.
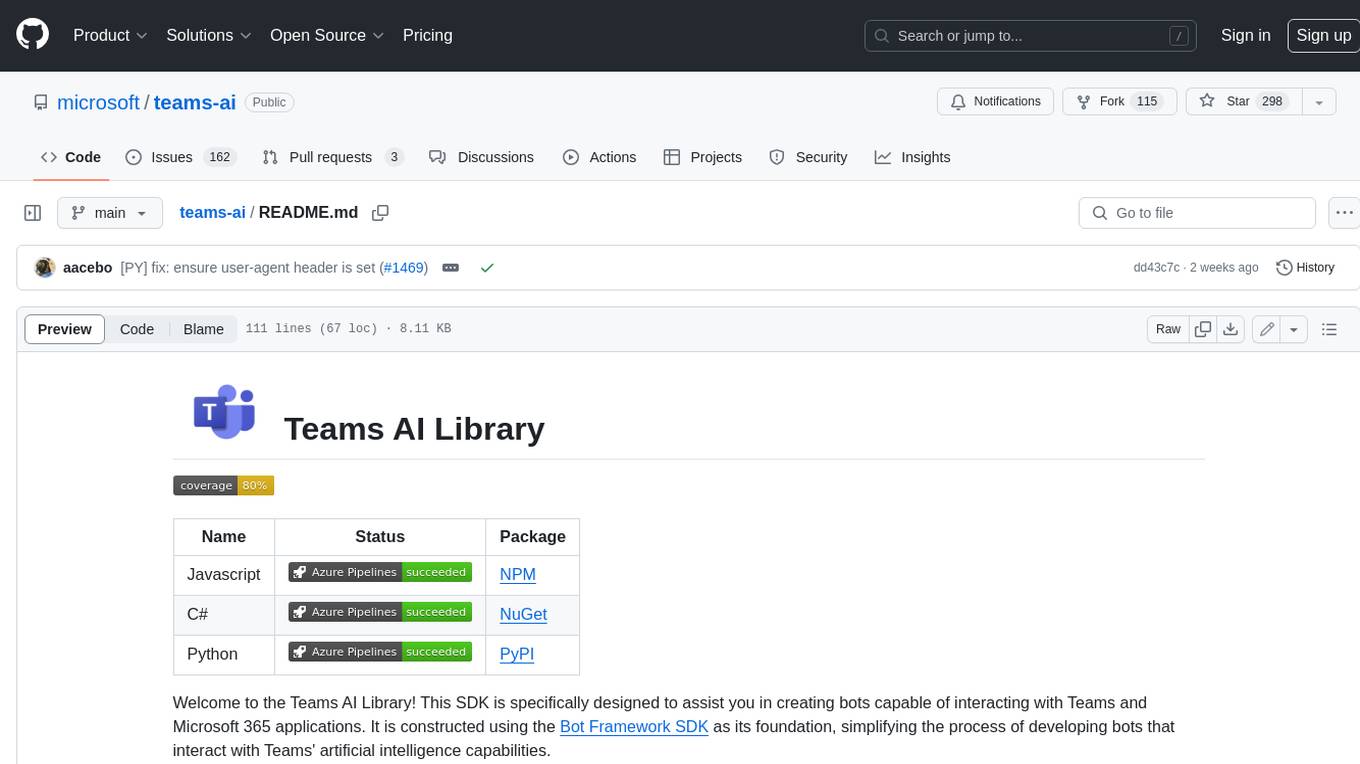
teams-ai
The Teams AI Library is a software development kit (SDK) that helps developers create bots that can interact with Teams and Microsoft 365 applications. It is built on top of the Bot Framework SDK and simplifies the process of developing bots that interact with Teams' artificial intelligence capabilities. The SDK is available for JavaScript/TypeScript, .NET, and Python.
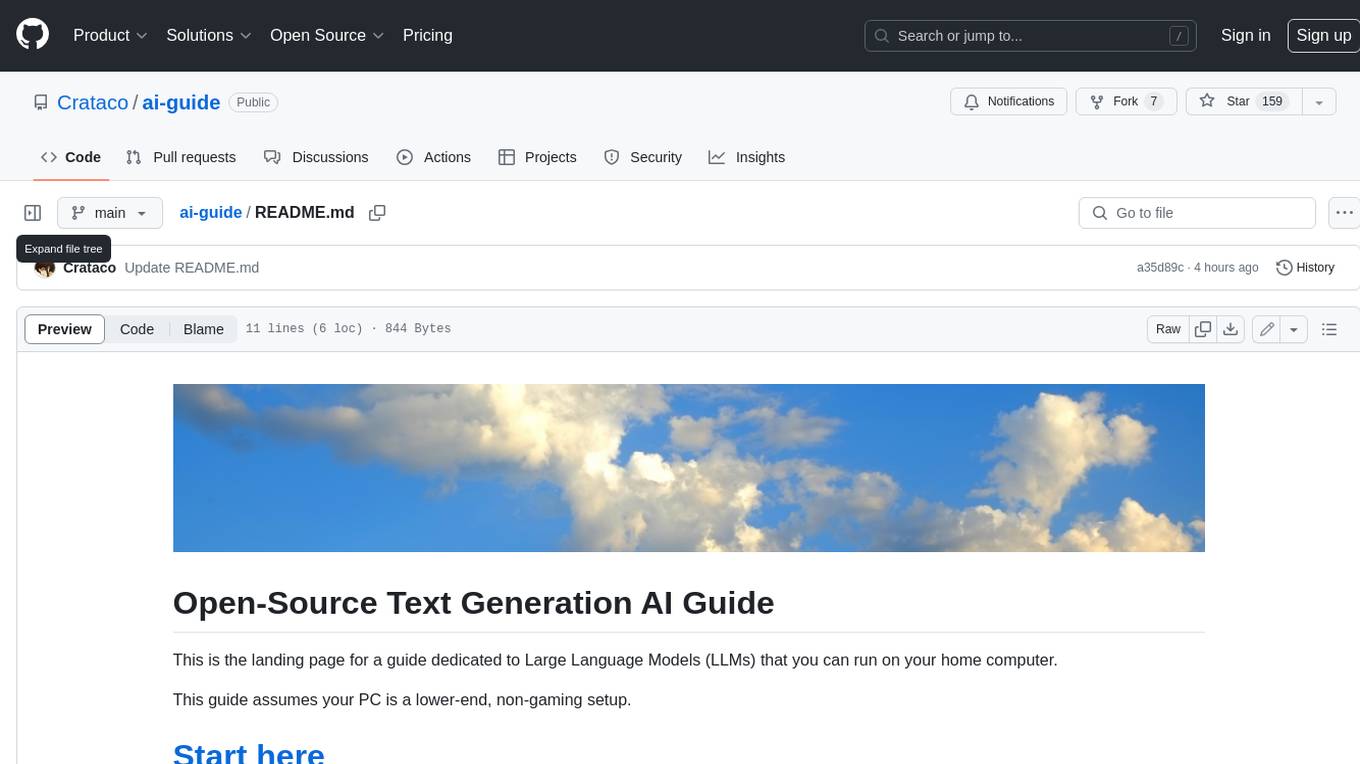
ai-guide
This guide is dedicated to Large Language Models (LLMs) that you can run on your home computer. It assumes your PC is a lower-end, non-gaming setup.
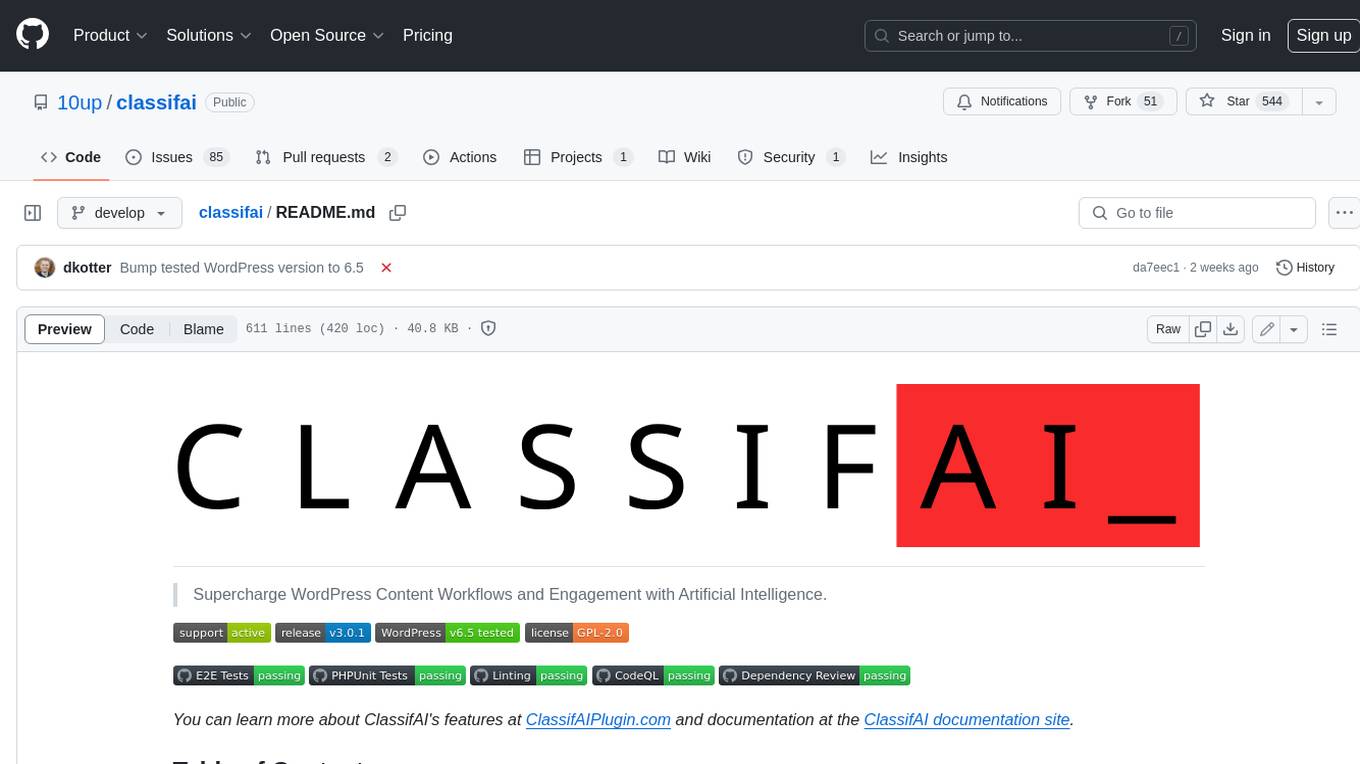
classifai
Supercharge WordPress Content Workflows and Engagement with Artificial Intelligence. Tap into leading cloud-based services like OpenAI, Microsoft Azure AI, Google Gemini and IBM Watson to augment your WordPress-powered websites. Publish content faster while improving SEO performance and increasing audience engagement. ClassifAI integrates Artificial Intelligence and Machine Learning technologies to lighten your workload and eliminate tedious tasks, giving you more time to create original content that matters.
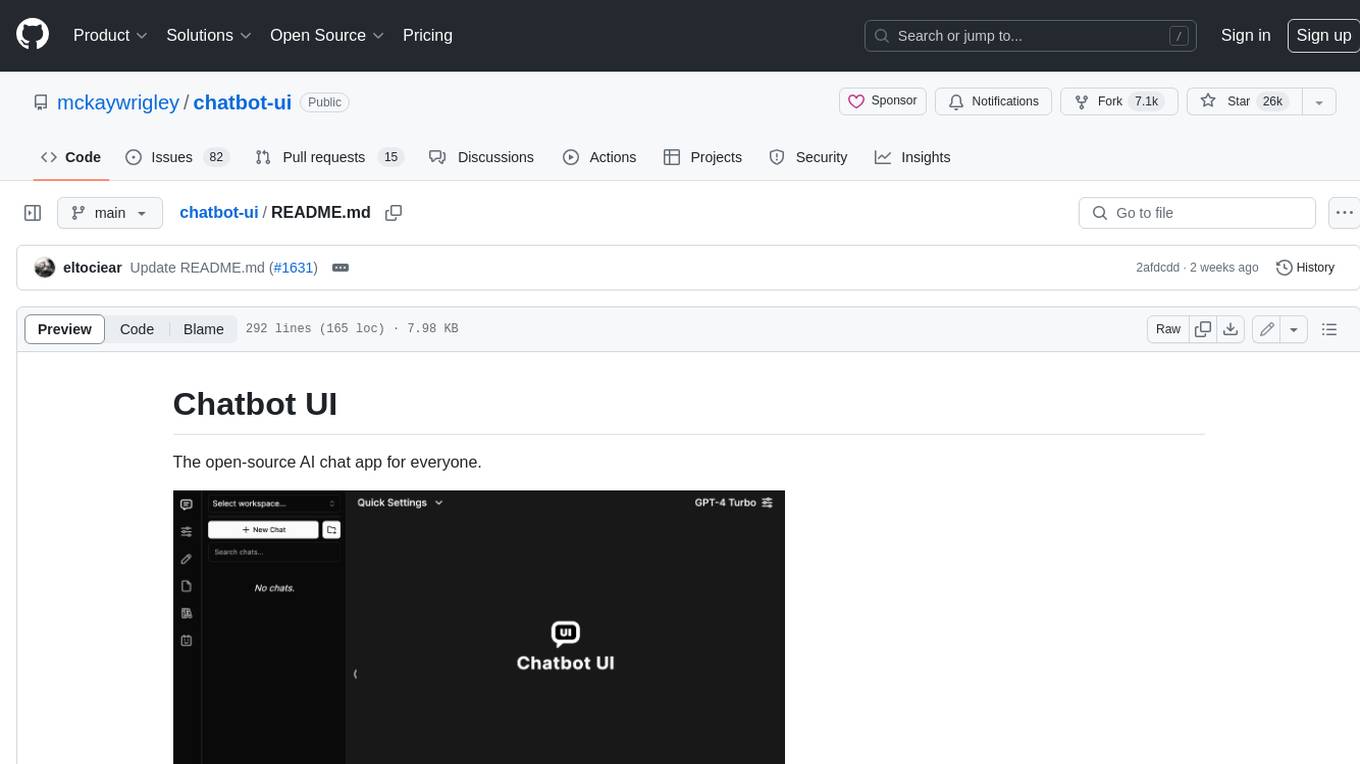
chatbot-ui
Chatbot UI is an open-source AI chat app that allows users to create and deploy their own AI chatbots. It is easy to use and can be customized to fit any need. Chatbot UI is perfect for businesses, developers, and anyone who wants to create a chatbot.
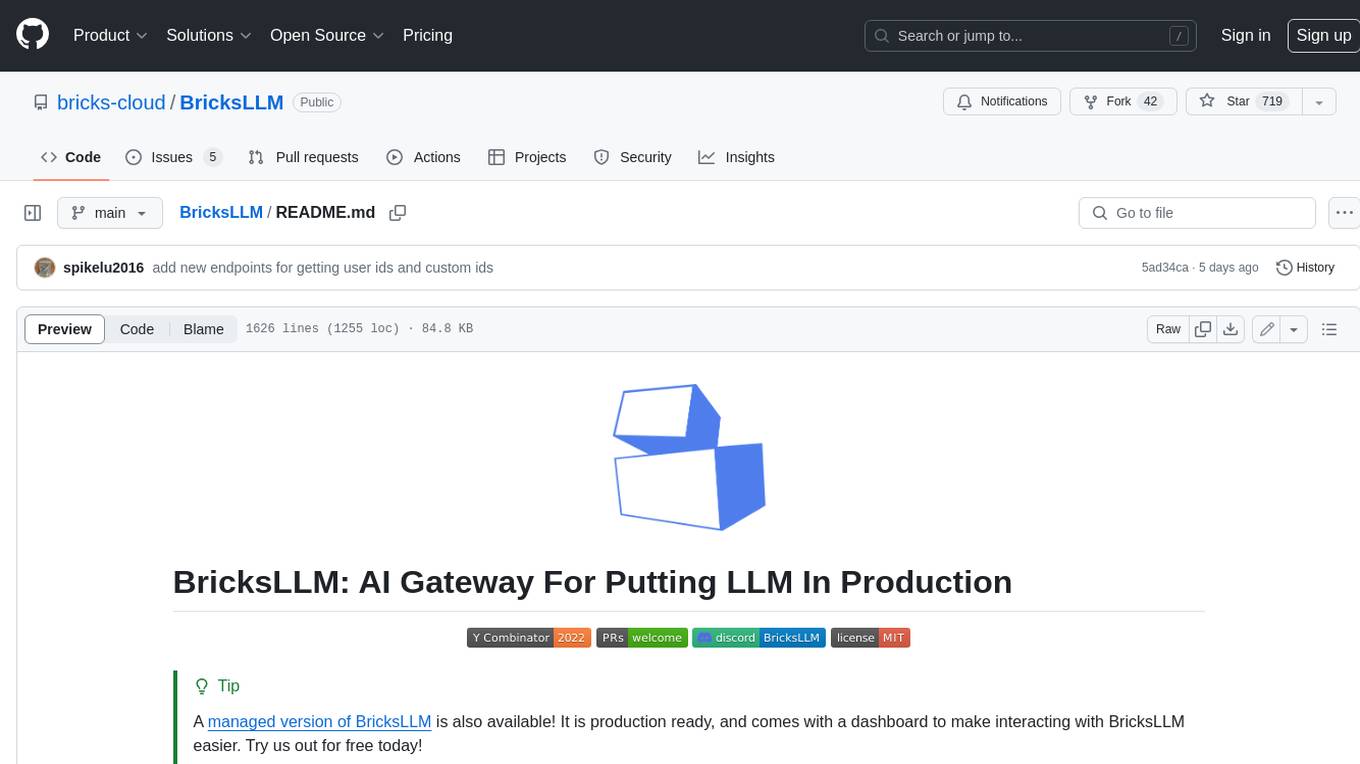
BricksLLM
BricksLLM is a cloud native AI gateway written in Go. Currently, it provides native support for OpenAI, Anthropic, Azure OpenAI and vLLM. BricksLLM aims to provide enterprise level infrastructure that can power any LLM production use cases. Here are some use cases for BricksLLM: * Set LLM usage limits for users on different pricing tiers * Track LLM usage on a per user and per organization basis * Block or redact requests containing PIIs * Improve LLM reliability with failovers, retries and caching * Distribute API keys with rate limits and cost limits for internal development/production use cases * Distribute API keys with rate limits and cost limits for students
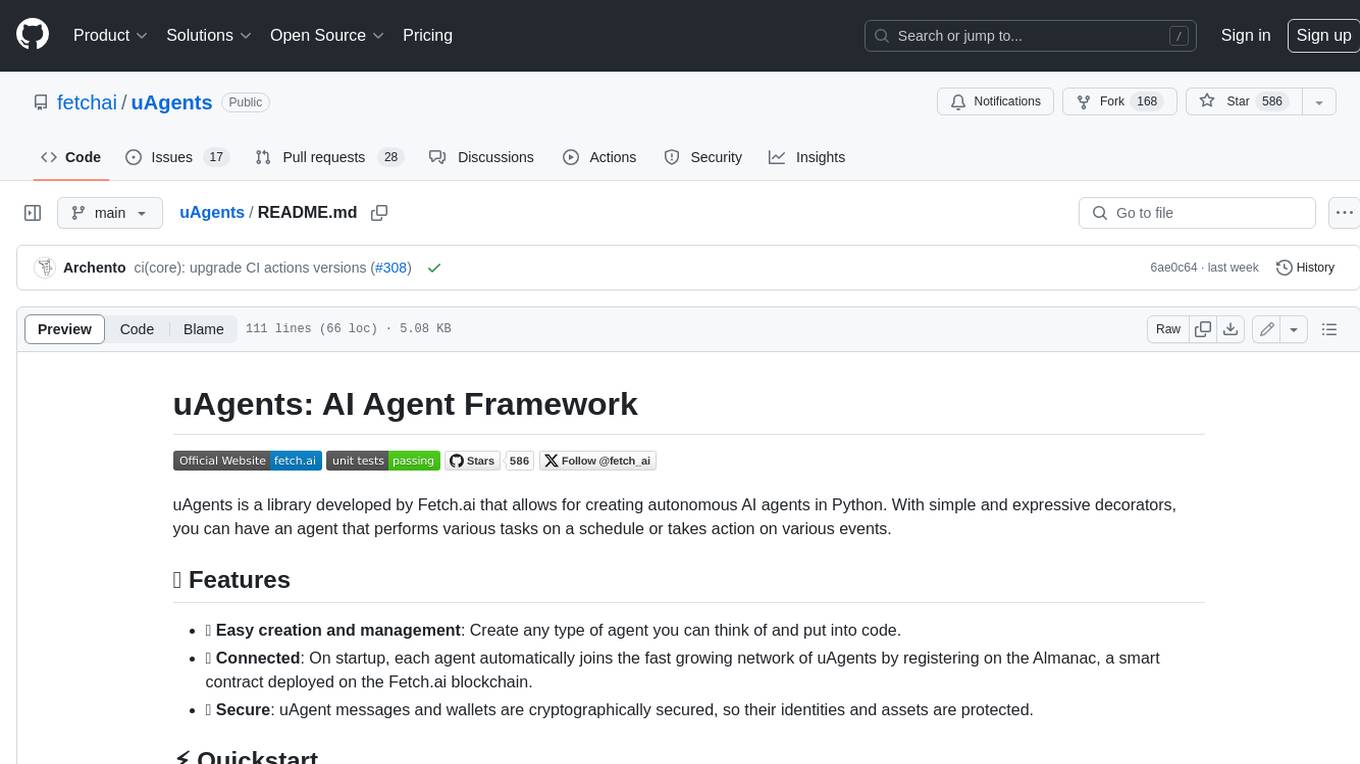
uAgents
uAgents is a Python library developed by Fetch.ai that allows for the creation of autonomous AI agents. These agents can perform various tasks on a schedule or take action on various events. uAgents are easy to create and manage, and they are connected to a fast-growing network of other uAgents. They are also secure, with cryptographically secured messages and wallets.
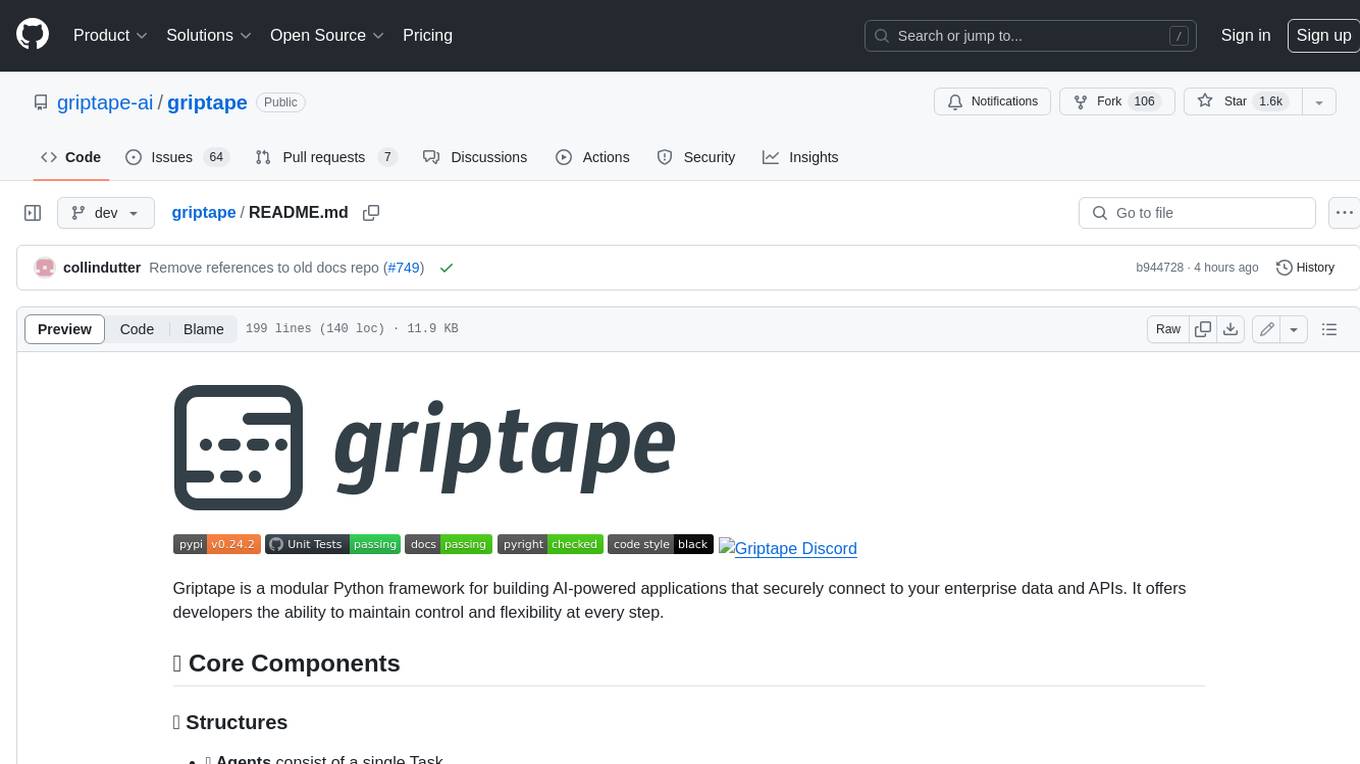
griptape
Griptape is a modular Python framework for building AI-powered applications that securely connect to your enterprise data and APIs. It offers developers the ability to maintain control and flexibility at every step. Griptape's core components include Structures (Agents, Pipelines, and Workflows), Tasks, Tools, Memory (Conversation Memory, Task Memory, and Meta Memory), Drivers (Prompt and Embedding Drivers, Vector Store Drivers, Image Generation Drivers, Image Query Drivers, SQL Drivers, Web Scraper Drivers, and Conversation Memory Drivers), Engines (Query Engines, Extraction Engines, Summary Engines, Image Generation Engines, and Image Query Engines), and additional components (Rulesets, Loaders, Artifacts, Chunkers, and Tokenizers). Griptape enables developers to create AI-powered applications with ease and efficiency.