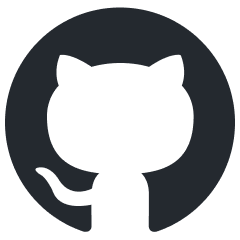
fiftyone
Refine high-quality datasets and visual AI models
Stars: 9109
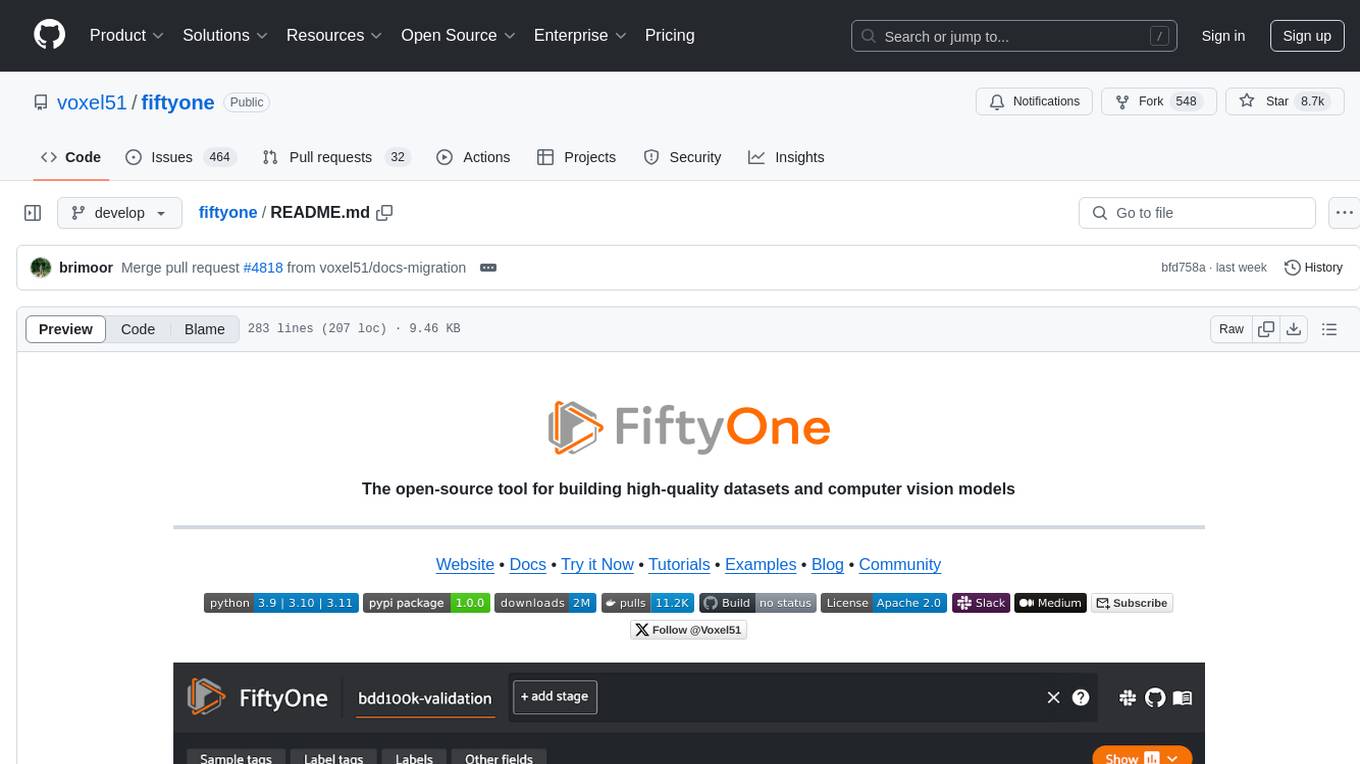
FiftyOne is an open-source tool designed for building high-quality datasets and computer vision models. It supercharges machine learning workflows by enabling users to visualize datasets, interpret models faster, and improve efficiency. With FiftyOne, users can explore scenarios, identify failure modes, visualize complex labels, evaluate models, find annotation mistakes, and much more. The tool aims to streamline the process of improving machine learning models by providing a comprehensive set of features for data analysis and model interpretation.
README:
The open-source tool for building high-quality datasets and computer vision models
Website • Docs • Try it Now • Tutorials • Examples • Blog • Community
We created an open-source tool that supercharges your computer vision and machine learning workflows by enabling you to visualize datasets, analyze models, and improve data quality more efficiently than ever before. Embark with us in this adventure 🤝. FiftyOne.
As simple as:
pip install fiftyone
More details here
FiftyOne supports Python 3.9 - 3.11. See the prerequisites section for system specific information. We provide two ways for being installed. The first one is through PyPI, and the second is through a local installation. PyPI is the straight forward installation method if you are not looking for any changes in the source code, if you want to make changes to the source code, then a local installation is recommended.
We strongly recommend that you install FiftyOne in a virtual environment to maintain a clean workspace. The prerequisites section also contains instructions for creating system specific virtual environments.
Installing the library from PyPI with pip
is the easiest way to get started
with fiftyone. You can install the latest stable version of fiftyone
via
pip
:
Consult the installation guide for troubleshooting and other information about getting up-and-running with FiftyOne.
Install from source
To install from source, you need to clone the repository and install the
library using pip
with editable mode enabled. The instructions below are for
macOS and Linux systems. Windows users may need to make adjustments. If you are
working in Google Colab, skip to here.
First, clone the repository:
git clone https://github.com/voxel51/fiftyone
cd fiftyone
Then run the install script:
# Mac or Linux
bash install.bash
# Windows
.\install.bat
NOTE: If you run into issues importing FiftyOne, you may need to add the
path to the cloned repository to your PYTHONPATH
:
export PYTHONPATH=$PYTHONPATH:/path/to/fiftyone
NOTE: The install script adds to your nvm
settings in your ~/.bashrc
or
~/.bash_profile
, which is needed for installing and building the App
NOTE: When you pull in new changes to the App, you will need to rebuild it,
which you can do either by rerunning the install script or just running
yarn build
in the ./app
directory.
To upgrade an existing source installation to the bleeding edge, simply pull
the latest develop
branch and rerun the install script:
git checkout develop
git pull
bash install.bash
If you would like to
contribute to FiftyOne,
you should perform a developer installation using the -d
flag of the install
script:
# Mac or Linux
bash install.bash -d
# Windows
.\install.bat -d
Although not required, developers typically prefer to configure their FiftyOne installation to connect to a self-installed and managed instance of MongoDB, which you can do by following these simple steps.
You can install from source in Google Colab by running the following in a cell and then restarting the runtime:
%%shell
git clone --depth 1 https://github.com/voxel51/fiftyone.git
cd fiftyone
# Mac or Linux
bash install.bash
# Windows
.\install.bat
See the docs guide for information on building and contributing to the documentation.
You can uninstall FiftyOne as follows:
pip uninstall fiftyone fiftyone-brain fiftyone-db
Prerequisites for beginners
FiftyOne supports Python 3.9 - 3.11. To get started, select the guide for your operating system or environment, if you are an experienced developer you can skip this section. If you are looking for scaling solution to be installed in Cloud Enterprise Systems, please take a look of FiftyOne Teams here
Windows
Note:
Download a Python installer from python.org. Choose Python 3.9, 3.10, or 3.11 and make sure to pick a 64-bit version. For example, this Python 3.10.11 installer. Double-click on the installer to run it, and follow the steps in the installer.
- Check the box to add Python to your PATH, and to install py.
- At the end of the installer, there is an option to disable the PATH length limit. It is recommended to click this.
Download Git from this link. Double-click on the installer to run it, and follow the steps in the installer.
Download Microsoft Visual C++ Redistributable. Double-click on the installer to run it, and follow the steps in the installer.
Download FFmpeg binary from here. Set FFmpeg's path (e.g., C:\ffmpeg\bin) to the PATH environmental variable on Windows.
- Press
Win + R
. typecmd
, and pressEnter
. Alternatively, search Command Prompt in the Start Menu. - Navigate to your project.
cd C:\path\to\your\project
- Create the environment
python -m venv fiftyone_env
- Activate the environment typing this in the command line window
fiftyone_env\Scripts\activate
- After activation, your command prompt should change and show the name of
the virtual environment
(fiftyon_env) C:\path\to\your\project
- Now you are ready to install FiftyOne. Full instructions can be found here.
- Once you want to deactivate your environment, just type
deactivate
Linux
You may need to install some additional libraries on Ubuntu Linux. These steps work on a clean install of Ubuntu Desktop 24.04, and should also work on Ubuntu 24.04 and 22.04, and on Ubuntu Server.
sudo apt-get update
sudo apt-get upgrade
sudo apt-get install python3-venv build-essential python3-dev git-all libgl1-mesa-dev ffmpeg
-
On Linux, you will need at least the
openssl
andlibcurl
packages. -
On Debian-based distributions, you will need to install
libcurl4
orlibcurl3
instead oflibcurl
, depending on the age of your distribution.For example:
# Ubuntu
sudo apt install libcurl4 openssl
# Fedora
sudo dnf install libcurl openssl
python3 -m venv fiftyone_env
source fiftyone_env/bin/activate
Now you are ready to install FiftyOne. Full instructions can be found here
MacOS
xcode-select --install
/bin/bash -c "$(curl -fsSL https://raw.githubusercontent.com/Homebrew/install/HEAD/install.sh)"
After you install it, follow the instructions from the Homebrew installation to set it up.
brew install [email protected]
brew install protobuf
# optional but recommendeded for full video dataset support
brew install ffmpeg
python3 -m venv fiftyone_env
source fiftyone_env/bin/activate
Now you are ready to install FiftyOne. Full instructions can be found here.
Docker
Refer to these instructions to see how to build and run Docker images containing source or release builds of FiftyOne.
Important Notes: Remember, you will need...
- Python (3.9 - 3.11)
- Node.js - on Linux, we recommend using nvm to install an up-to-date version.
-
Yarn - once Node.js is installed, you can
enable Yarn via
corepack enable
Dive right into FiftyOne by opening a Python shell and running the snippet below, which downloads a small dataset and launches the FiftyOne App so you can explore it:
import fiftyone as fo
import fiftyone.zoo as foz
dataset = foz.load_zoo_dataset("quickstart")
session = fo.launch_app(dataset)
Then check out this Colab notebook to see some common workflows on the quickstart dataset.
Note that if you are running the above code in a script, you must include
session.wait()
to block execution until you close the App. See
this page
for more information.
- Visualize Complex Datasets: Easily explore images, videos, and associated labels in a powerful visual interface.
https://github.com/user-attachments/assets/af8cd626-57b7-4f2a-96bf-1c8a513c2e2b
- Explore Embeddings: Select points of interest and view the corresponding samples/labels.
https://github.com/user-attachments/assets/d119de24-fc44-40bc-83ff-ddfdd4329977
- Analyze and Improve Models: Evaluate model performance, identify failure modes, and fine-tune your models.
https://github.com/user-attachments/assets/fc06d33d-8d17-4f67-af26-8c1a5abb5d9d
- Advanced Data Curation: Quickly find and fix data issues, annotation errors, and edge cases.
https://github.com/user-attachments/assets/8c4ff038-8926-4a42-b829-4f43bc2d8d6a
https://github.com/user-attachments/assets/da97d84d-1213-40cf-a501-7a0d7efbe426
- Rich Integration: Works with popular deep learning libraries like TensorFlow, PyTorch, Keras, and more.
https://github.com/user-attachments/assets/670a684a-0f6c-49cc-8f51-fbe15530c5e3
- Open and Extensible Customize and extend FiftyOne to fit your specific needs.
https://github.com/user-attachments/assets/dd91272d-2808-4373-90c5-5e906a0b80f1
Full documentation for FiftyOne is available at fiftyone.ai. In particular, see these resources:
Do you need to securely collaborate on datasets with millions of samples in the cloud and leverage built-in workflow automations? Check out FiftyOne Teams.
This page lists common issues encountered when installing FiftyOne and possible solutions. If you encounter an issue that this page doesn’t help you resolve, feel free to open an issue on GitHub or contact us on Slack or Discord.
FAQ: Maybe you are facing a situation already solved, take a look of the frequently asked questions.
FiftyOne and FiftyOne Brain are open source and community contributions are welcome!
Check out the contribution guide to learn how to get involved.
Connect with us through your preferred channels:
Share your workflow improvements on social media and tag us @Voxel51 and #FiftyOne!.
🎊 You will be in our rewarded list. 🎊
Special thanks to these amazing people for contributing to FiftyOne! 🙌
If you use FiftyOne in your research, feel free to cite the project (but only if you love it 😊):
@article{moore2020fiftyone,
title={FiftyOne},
author={Moore, B. E. and Corso, J. J.},
journal={GitHub. Note: https://github.com/voxel51/fiftyone},
year={2020}
}
For Tasks:
Click tags to check more tools for each tasksFor Jobs:
Alternative AI tools for fiftyone
Similar Open Source Tools
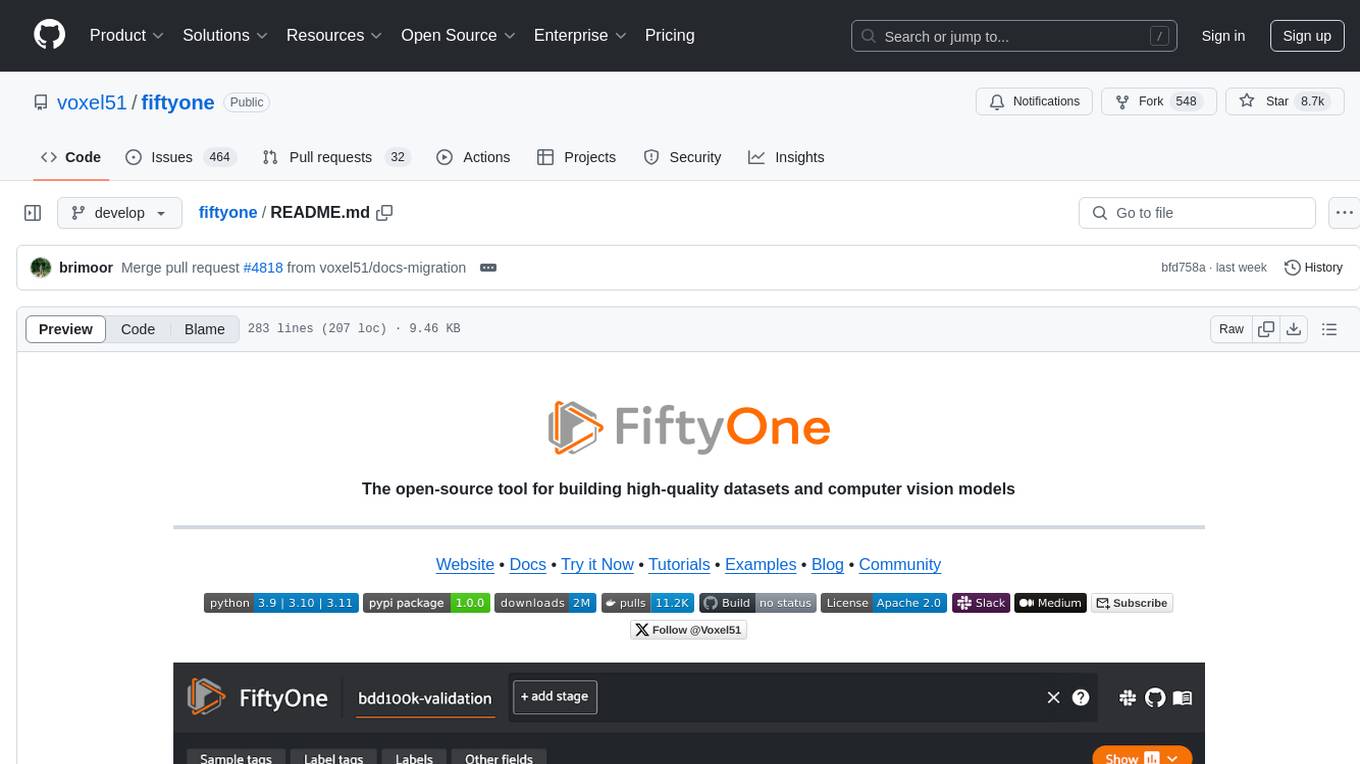
fiftyone
FiftyOne is an open-source tool designed for building high-quality datasets and computer vision models. It supercharges machine learning workflows by enabling users to visualize datasets, interpret models faster, and improve efficiency. With FiftyOne, users can explore scenarios, identify failure modes, visualize complex labels, evaluate models, find annotation mistakes, and much more. The tool aims to streamline the process of improving machine learning models by providing a comprehensive set of features for data analysis and model interpretation.
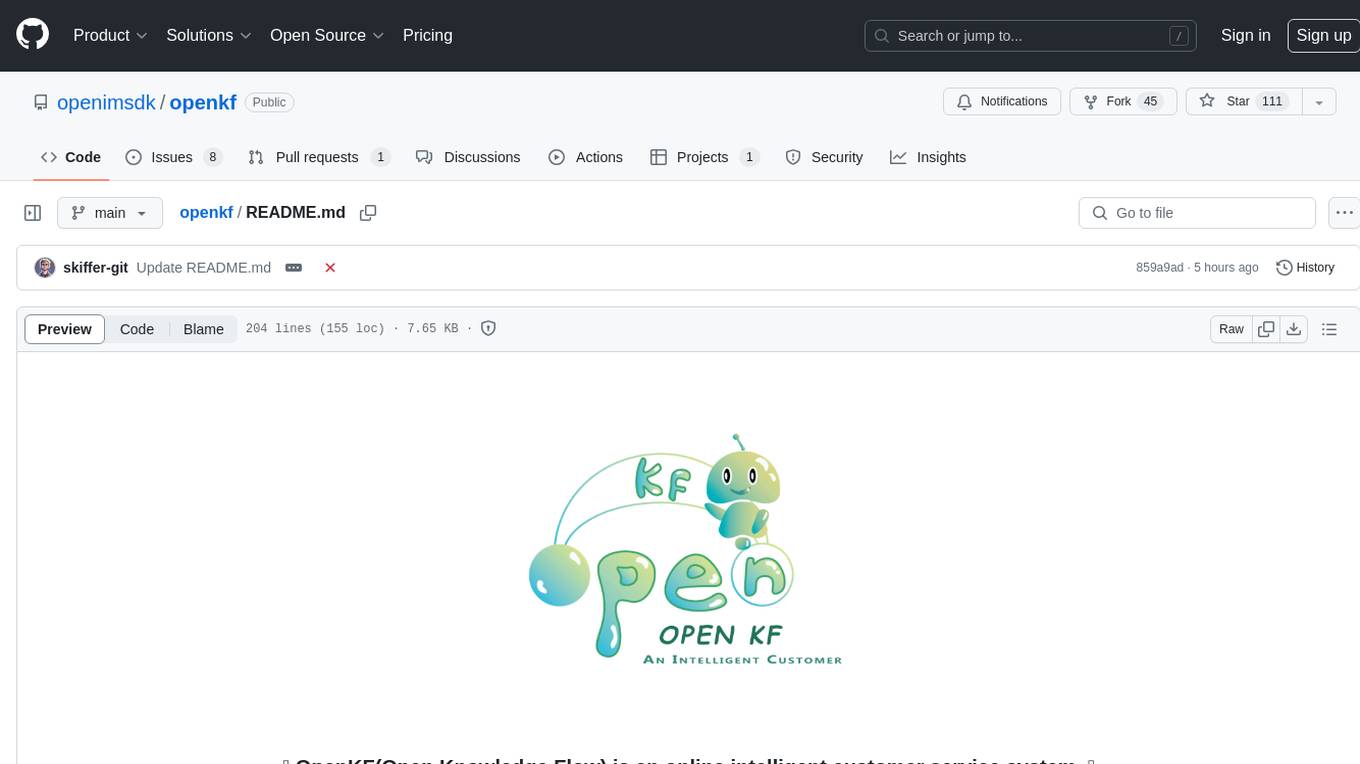
openkf
OpenKF (Open Knowledge Flow) is an online intelligent customer service system. It is an open-source customer service system based on OpenIM, supporting LLM (Local Knowledgebase) customer service and multi-channel customer service. It is easy to integrate with third-party systems, deploy, and perform secondary development. The system provides features like login page, config page, dashboard page, platform page, and session page. Users can quickly get started with OpenKF by following the installation and run instructions. The architecture follows MVC design with a standardized directory structure. The community encourages involvement through community meetings, contributions, and development. OpenKF is licensed under the Apache 2.0 license.
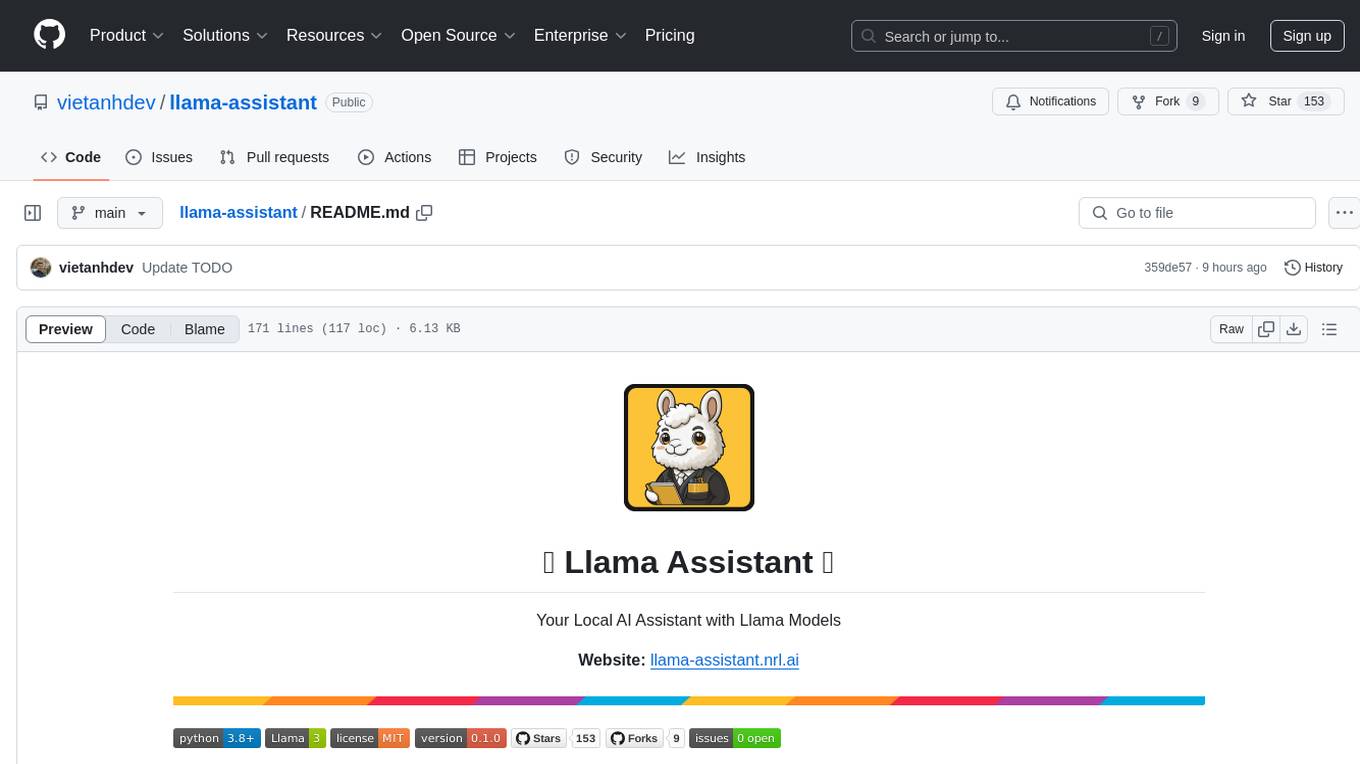
llama-assistant
Llama Assistant is an AI-powered assistant that helps with daily tasks, such as voice recognition, natural language processing, summarizing text, rephrasing sentences, answering questions, and more. It runs offline on your local machine, ensuring privacy by not sending data to external servers. The project is a work in progress with regular feature additions.
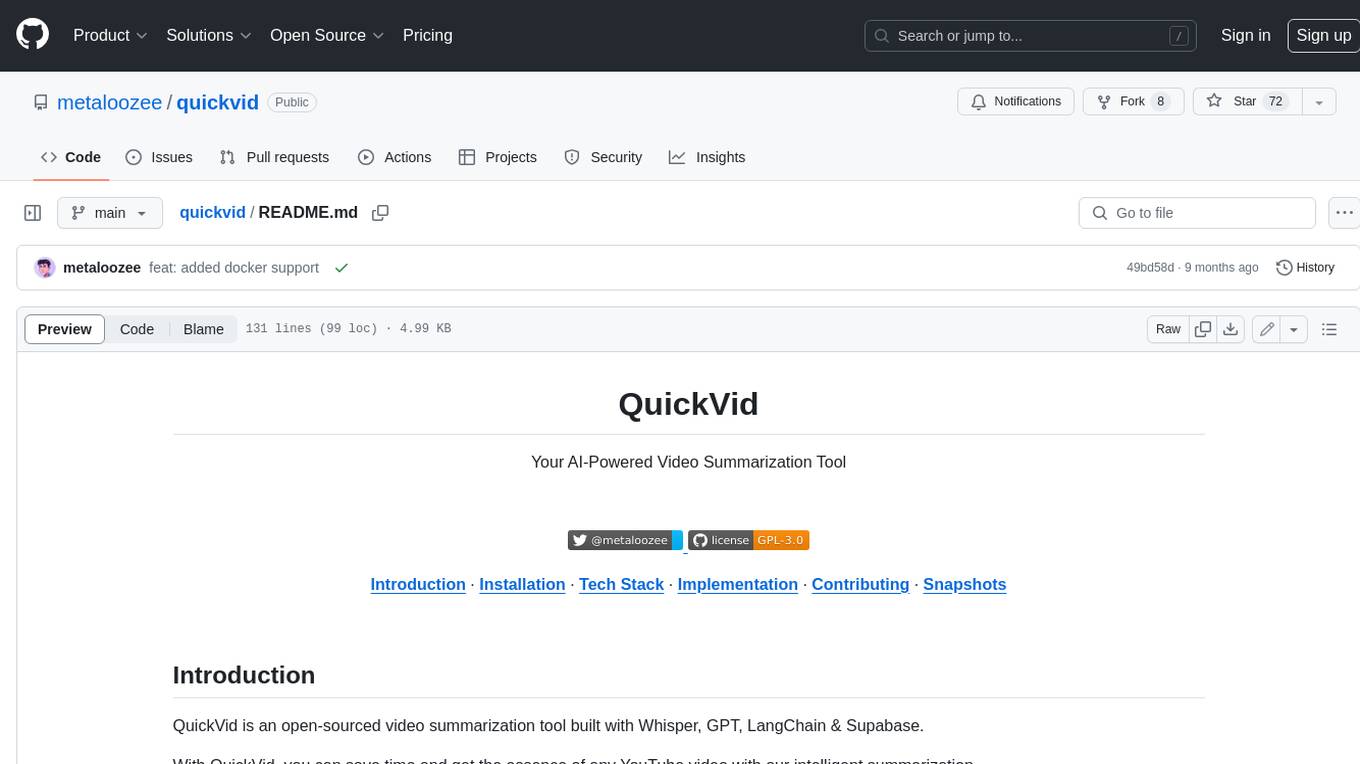
quickvid
QuickVid is an open-source video summarization tool that uses AI to generate summaries of YouTube videos. It is built with Whisper, GPT, LangChain, and Supabase. QuickVid can be used to save time and get the essence of any YouTube video with intelligent summarization.
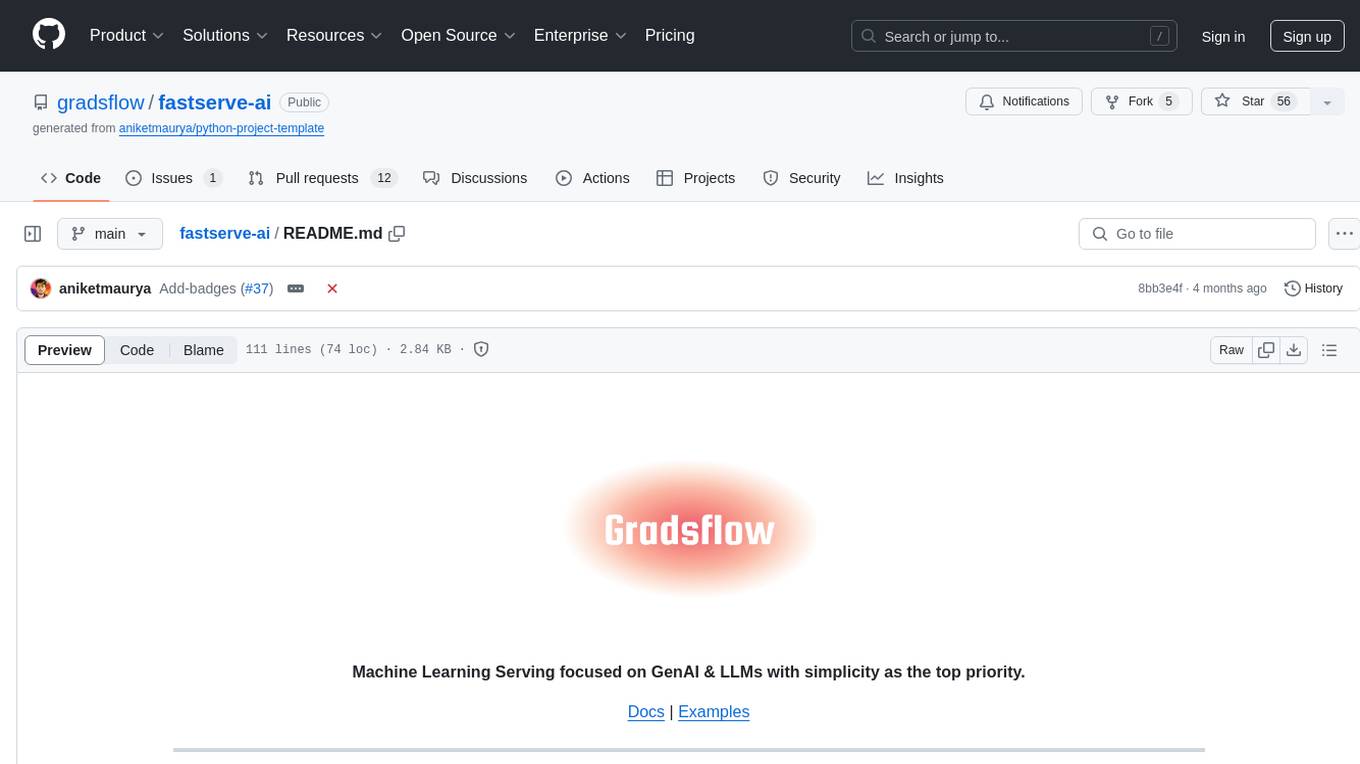
fastserve-ai
FastServe-AI is a machine learning serving tool focused on GenAI & LLMs with simplicity as the top priority. It allows users to easily serve custom models by implementing the 'handle' method for 'FastServe'. The tool provides a FastAPI server for custom models and can be deployed using Lightning AI Studio. Users can install FastServe-AI via pip and run it to serve their own GPT-like LLM models in minutes.
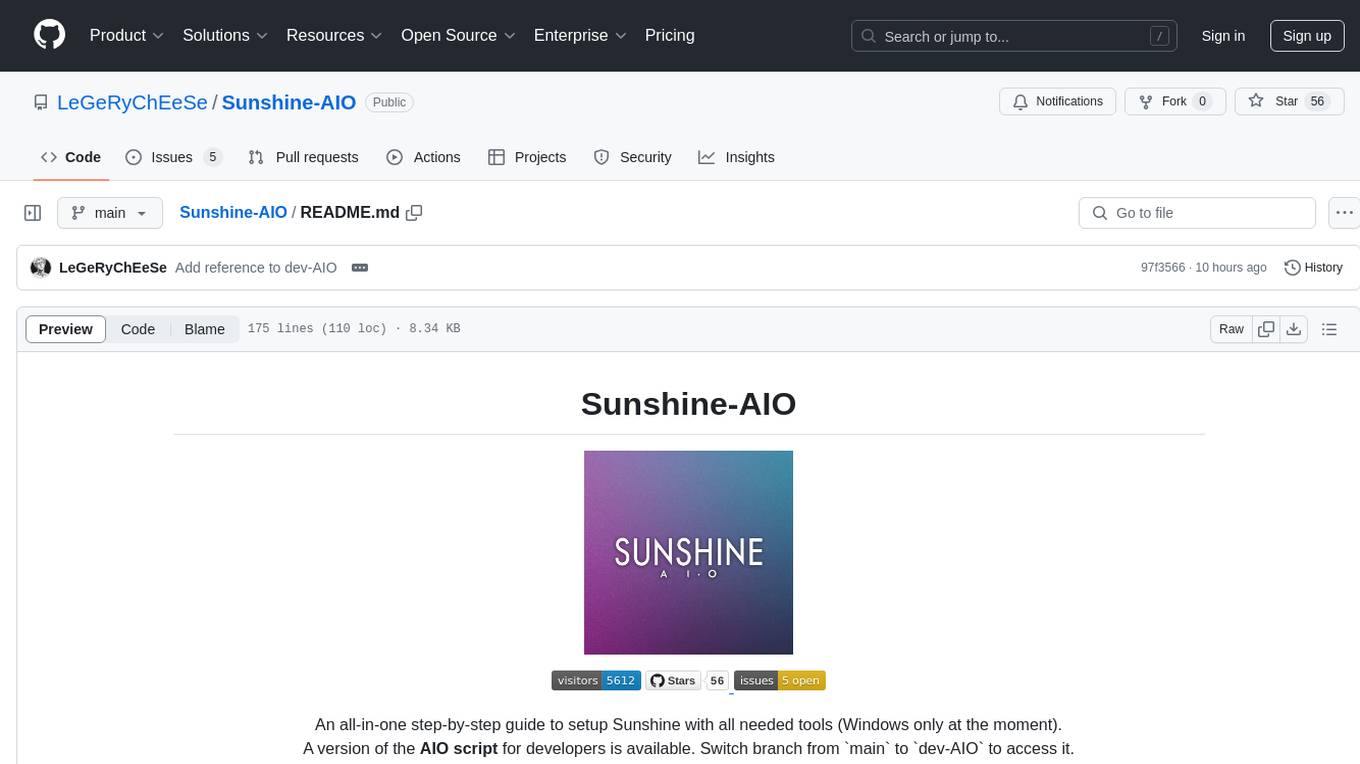
Sunshine-AIO
Sunshine-AIO is an all-in-one step-by-step guide to set up Sunshine with all necessary tools for Windows users. It provides a dedicated display for game streaming, virtual monitor switching, automatic resolution adjustment, resource-saving features, game launcher integration, and stream management. The project aims to evolve into an AIO tool as it progresses, welcoming contributions from users.
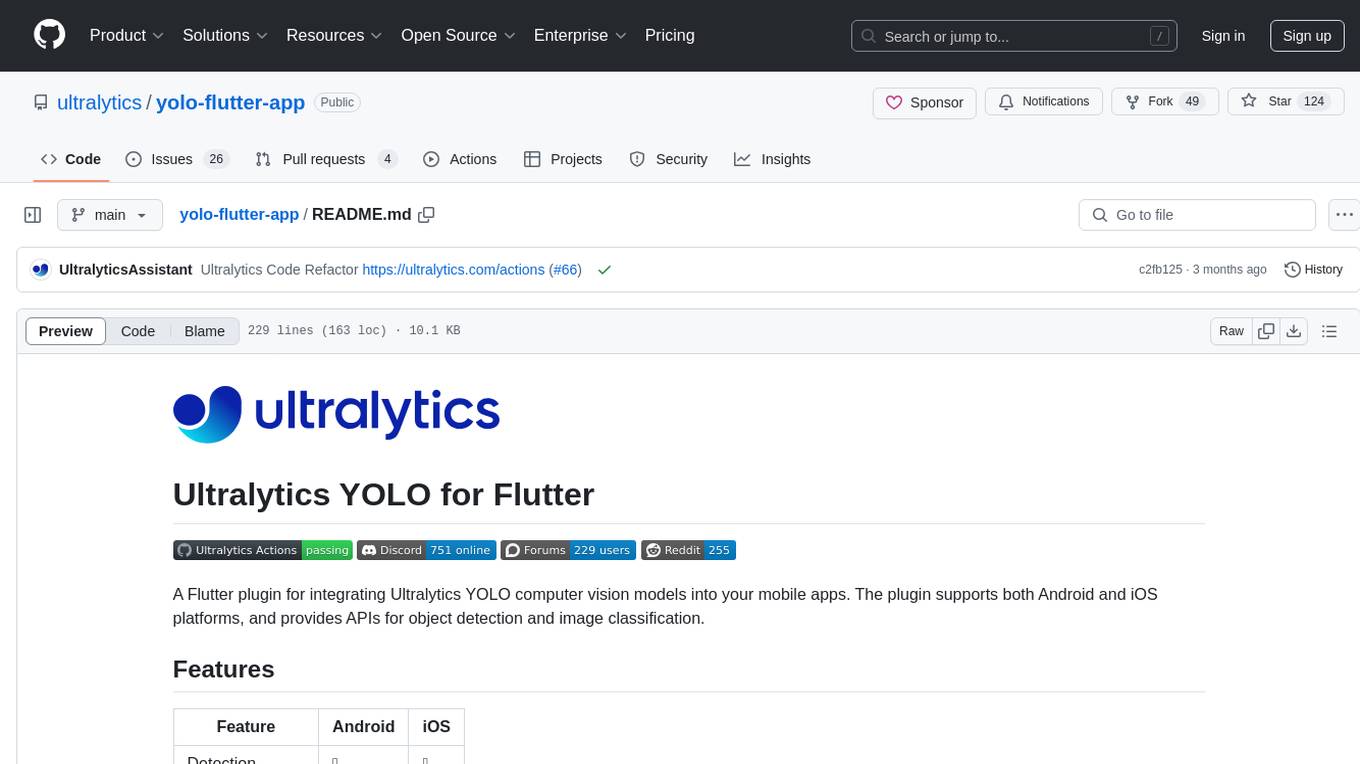
yolo-flutter-app
Ultralytics YOLO for Flutter is a Flutter plugin that allows you to integrate Ultralytics YOLO computer vision models into your mobile apps. It supports both Android and iOS platforms, providing APIs for object detection and image classification. The plugin leverages Flutter Platform Channels for seamless communication between the client and host, handling all processing natively. Before using the plugin, you need to export the required models in `.tflite` and `.mlmodel` formats. The plugin provides support for tasks like detection and classification, with specific instructions for Android and iOS platforms. It also includes features like camera preview and methods for object detection and image classification on images. Ultralytics YOLO thrives on community collaboration and offers different licensing paths for open-source and commercial use cases.
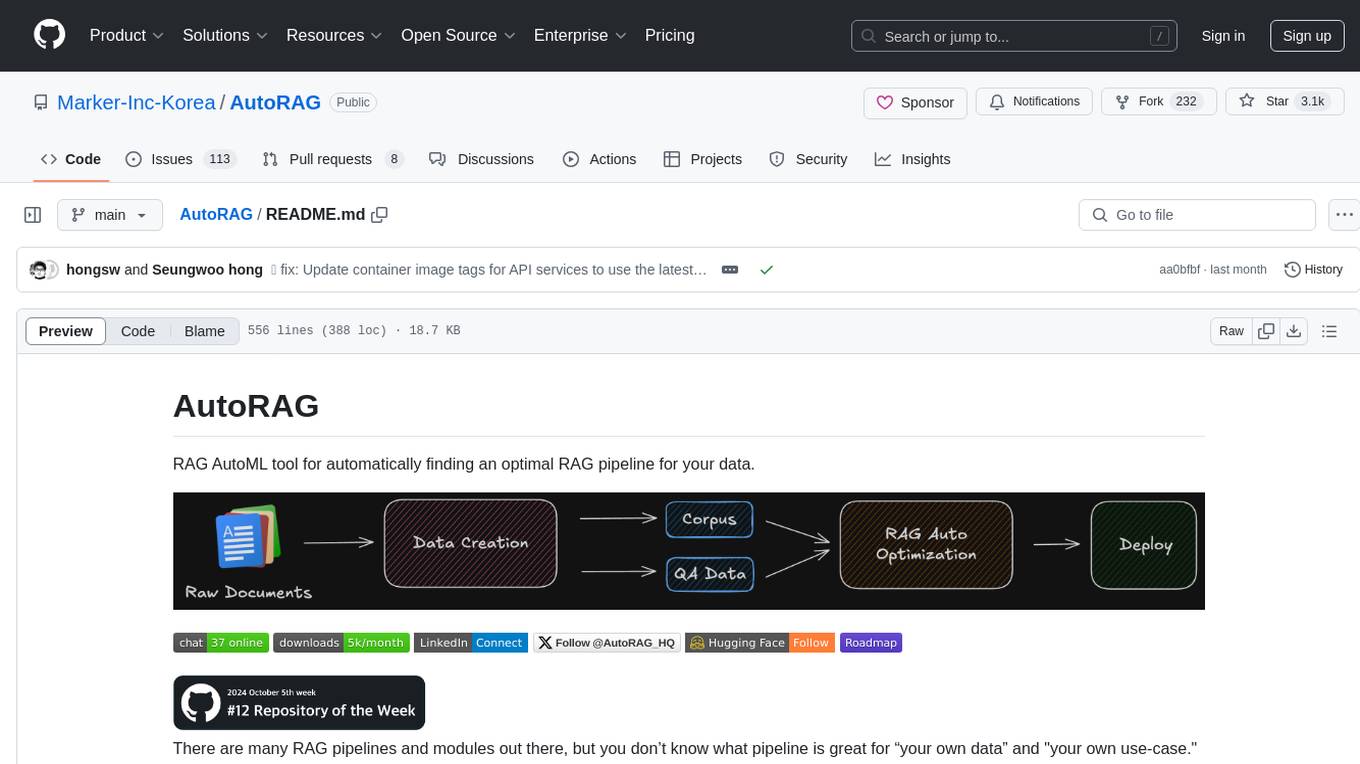
AutoRAG
AutoRAG is an AutoML tool designed to automatically find the optimal RAG pipeline for your data. It simplifies the process of evaluating various RAG modules to identify the best pipeline for your specific use-case. The tool supports easy evaluation of different module combinations, making it efficient to find the most suitable RAG pipeline for your needs. AutoRAG also offers a cloud beta version to assist users in running and optimizing the tool, along with building RAG evaluation datasets for a starting price of $9.99 per optimization.
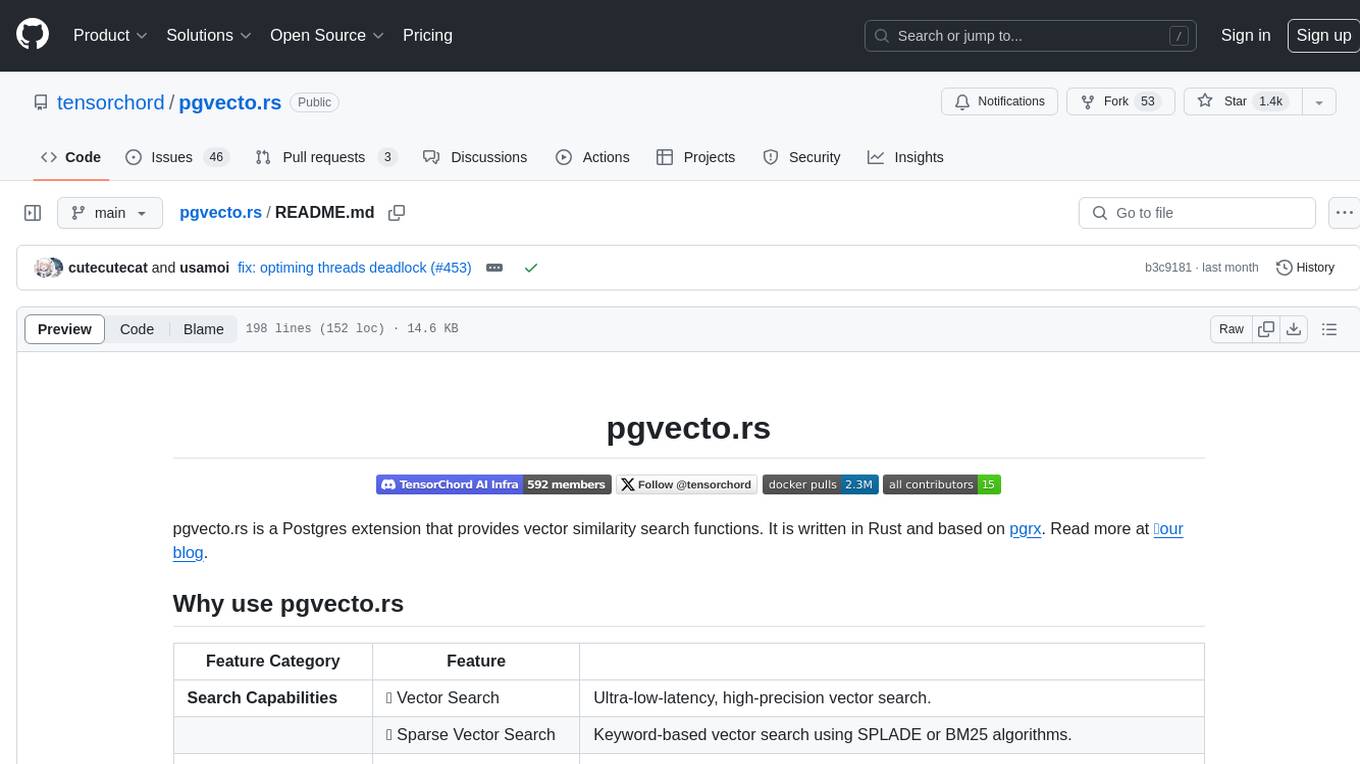
pgvecto.rs
pgvecto.rs is a Postgres extension written in Rust that provides vector similarity search functions. It offers ultra-low-latency, high-precision vector search capabilities, including sparse vector search and full-text search. With complete SQL support, async indexing, and easy data management, it simplifies data handling. The extension supports various data types like FP16/INT8, binary vectors, and Matryoshka embeddings. It ensures system performance with production-ready features, high availability, and resource efficiency. Security and permissions are managed through easy access control. The tool allows users to create tables with vector columns, insert vector data, and calculate distances between vectors using different operators. It also supports half-precision floating-point numbers for better performance and memory usage optimization.
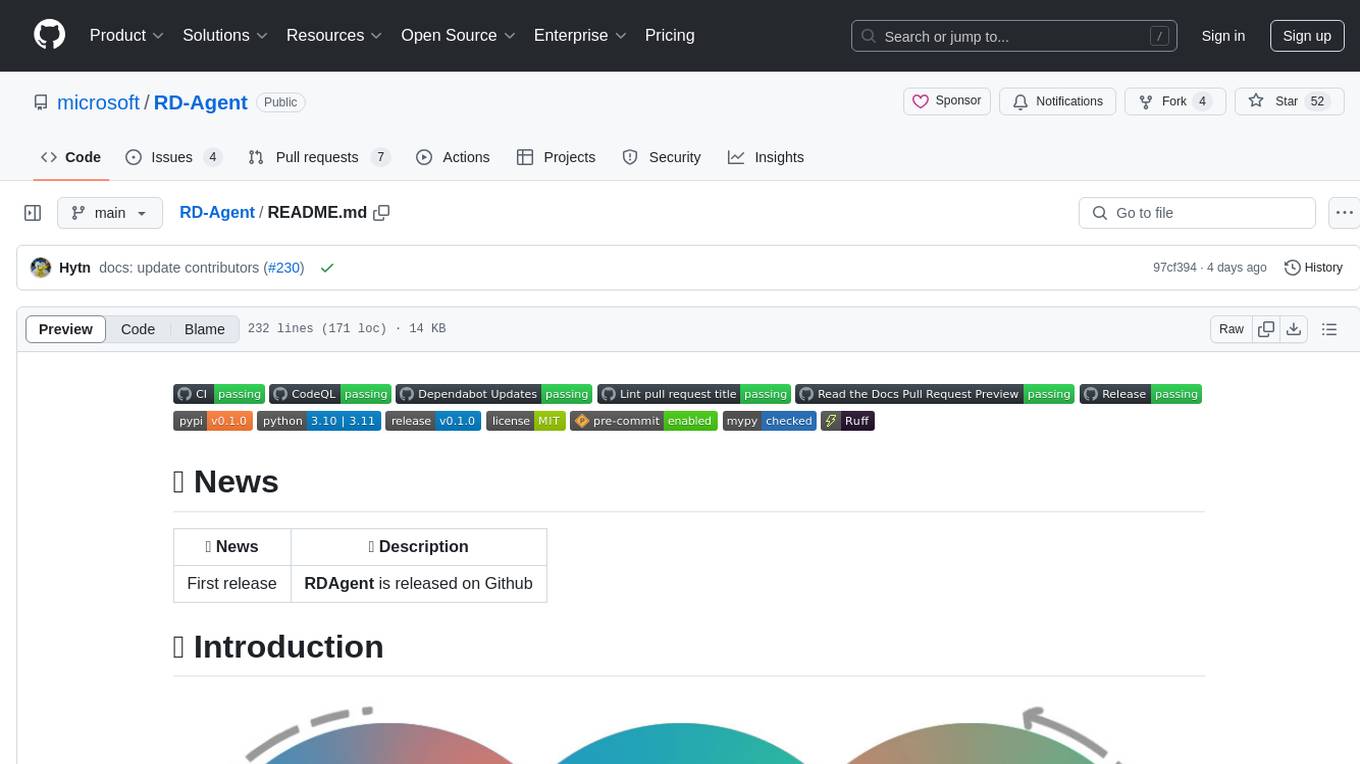
RD-Agent
RD-Agent is a tool designed to automate critical aspects of industrial R&D processes, focusing on data-driven scenarios to streamline model and data development. It aims to propose new ideas ('R') and implement them ('D') automatically, leading to solutions of significant industrial value. The tool supports scenarios like Automated Quantitative Trading, Data Mining Agent, Research Copilot, and more, with a framework to push the boundaries of research in data science. Users can create a Conda environment, install the RDAgent package from PyPI, configure GPT model, and run various applications for tasks like quantitative trading, model evolution, medical prediction, and more. The tool is intended to enhance R&D processes and boost productivity in industrial settings.
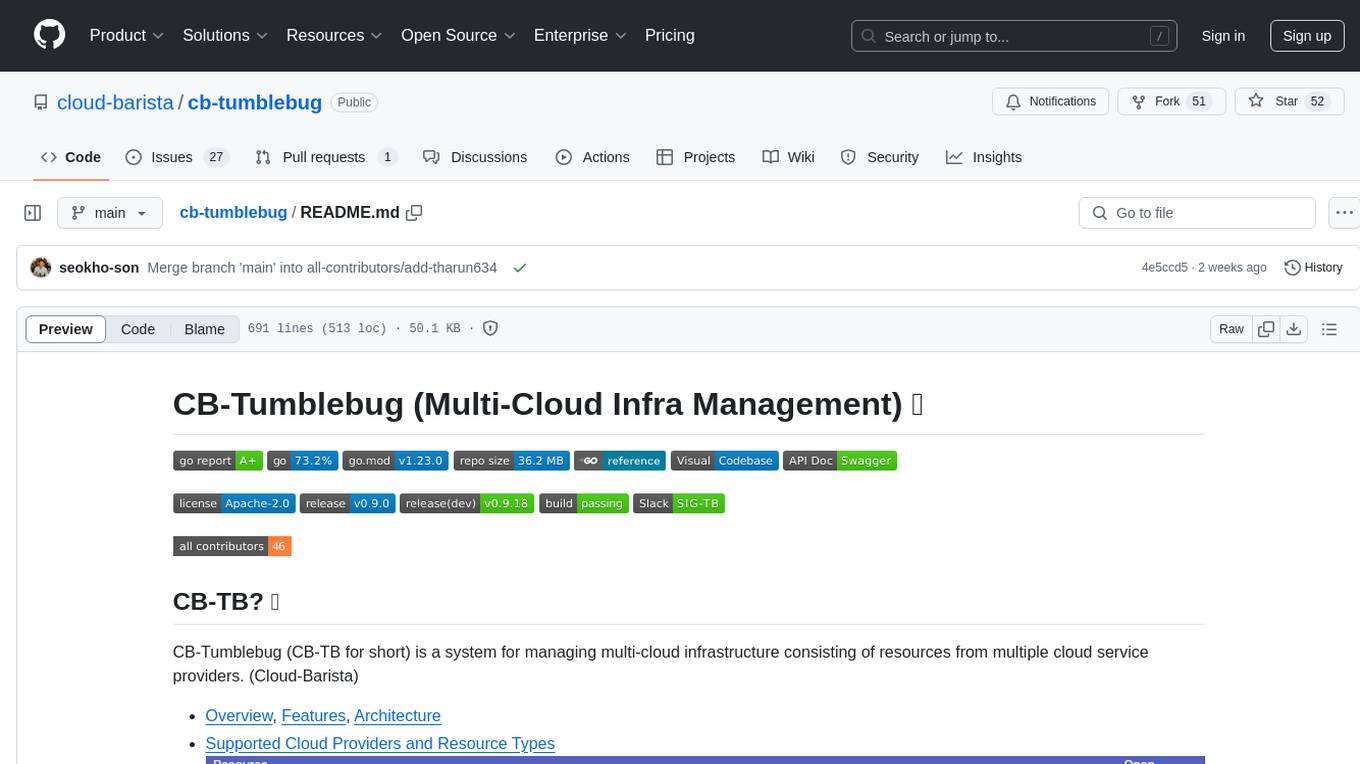
cb-tumblebug
CB-Tumblebug (CB-TB) is a system for managing multi-cloud infrastructure consisting of resources from multiple cloud service providers. It provides an overview, features, and architecture. The tool supports various cloud providers and resource types, with ongoing development and localization efforts. Users can deploy a multi-cloud infra with GPUs, enjoy multiple LLMs in parallel, and utilize LLM-related scripts. The tool requires Linux, Docker, Docker Compose, and Golang for building the source. Users can run CB-TB with Docker Compose or from the Makefile, set up prerequisites, contribute to the project, and view a list of contributors. The tool is licensed under an open-source license.
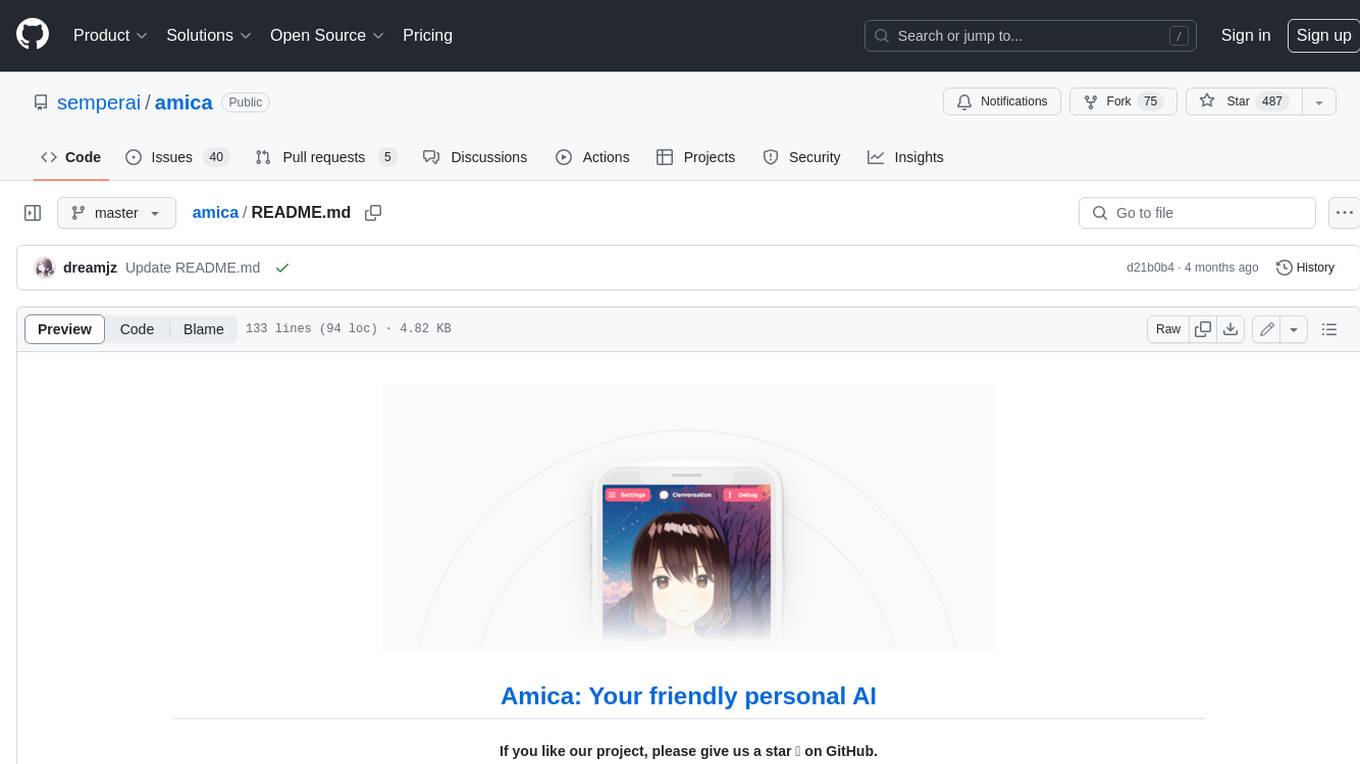
amica
Amica is an application that allows you to easily converse with 3D characters in your browser. You can import VRM files, adjust the voice to fit the character, and generate response text that includes emotional expressions.
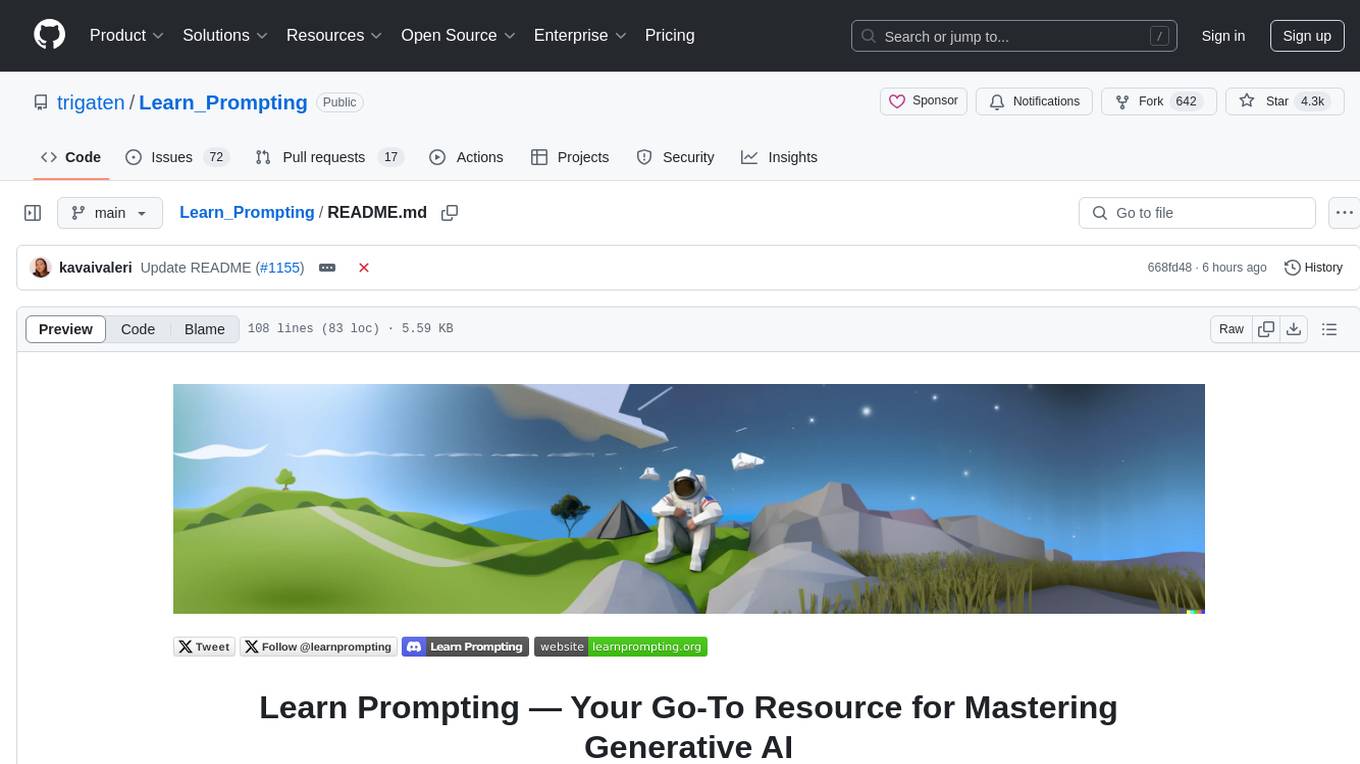
Learn_Prompting
Learn Prompting is a platform offering free resources, courses, and webinars to master prompt engineering and generative AI. It provides a Prompt Engineering Guide, courses on Generative AI, workshops, and the HackAPrompt competition. The platform also offers AI Red Teaming and AI Safety courses, research reports on prompting techniques, and welcomes contributions in various forms such as content suggestions, translations, artwork, and typo fixes. Users can locally develop the website using Visual Studio Code, Git, and Node.js, and run it in development mode to preview changes.
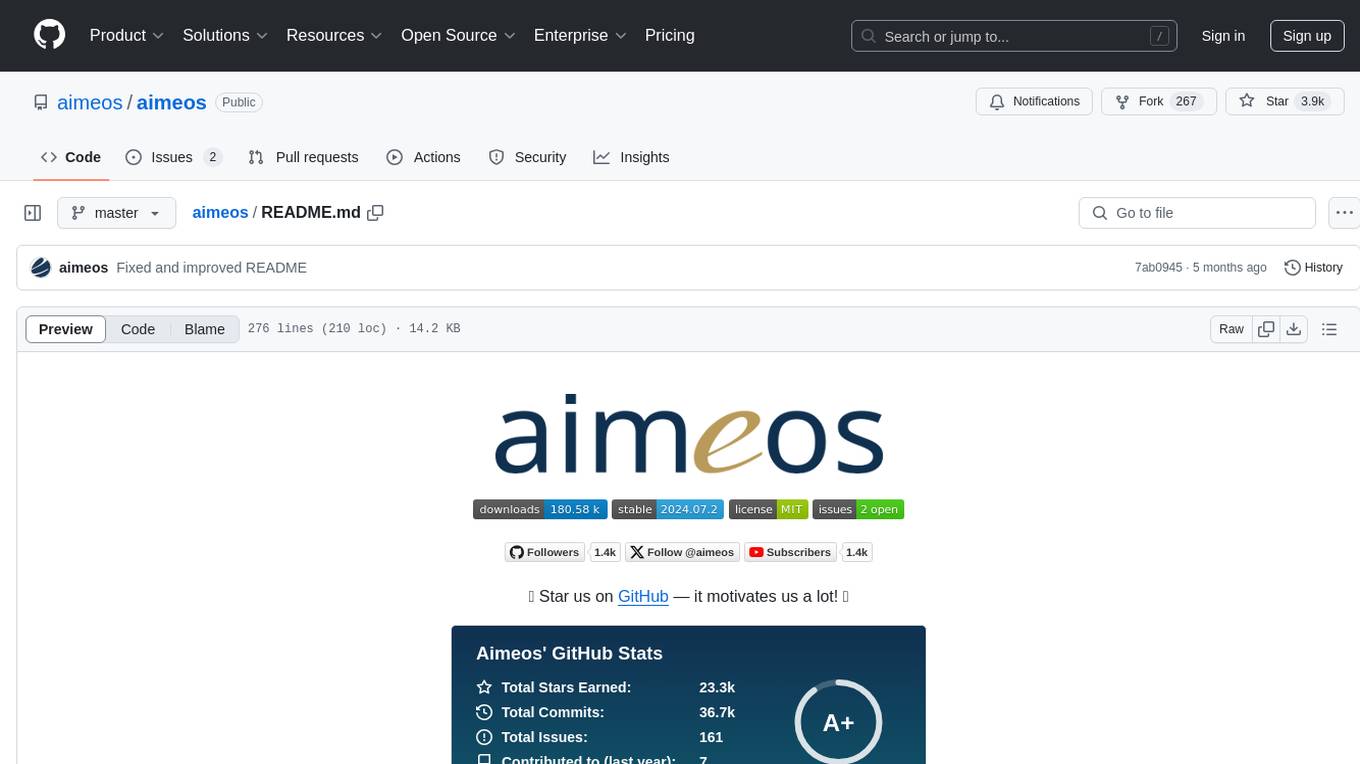
aimeos
Aimeos is a full-featured e-commerce platform that is ultra-fast, cloud-native, and API-first. It offers a wide range of features including JSON REST API, GraphQL API, multi-vendor support, various product types, subscriptions, multiple payment gateways, admin backend, modular structure, SEO optimization, multi-language support, AI-based text translation, mobile optimization, and high-quality source code. It is highly configurable and extensible, making it suitable for e-commerce SaaS solutions, marketplaces, and various cloud environments. Aimeos is designed for scalability, security, and performance, catering to a diverse range of e-commerce needs.
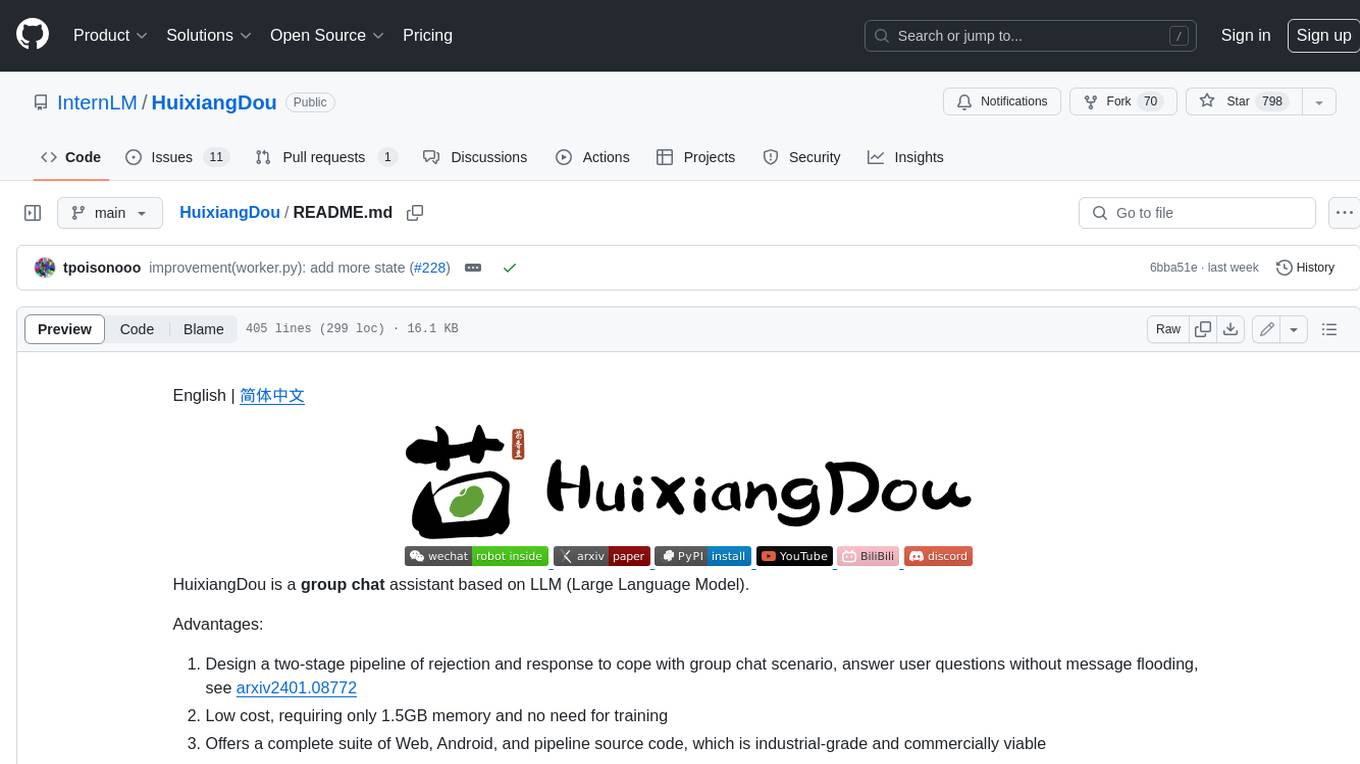
HuixiangDou
HuixiangDou is a **group chat** assistant based on LLM (Large Language Model). Advantages: 1. Design a two-stage pipeline of rejection and response to cope with group chat scenario, answer user questions without message flooding, see arxiv2401.08772 2. Low cost, requiring only 1.5GB memory and no need for training 3. Offers a complete suite of Web, Android, and pipeline source code, which is industrial-grade and commercially viable Check out the scenes in which HuixiangDou are running and join WeChat Group to try AI assistant inside. If this helps you, please give it a star ⭐
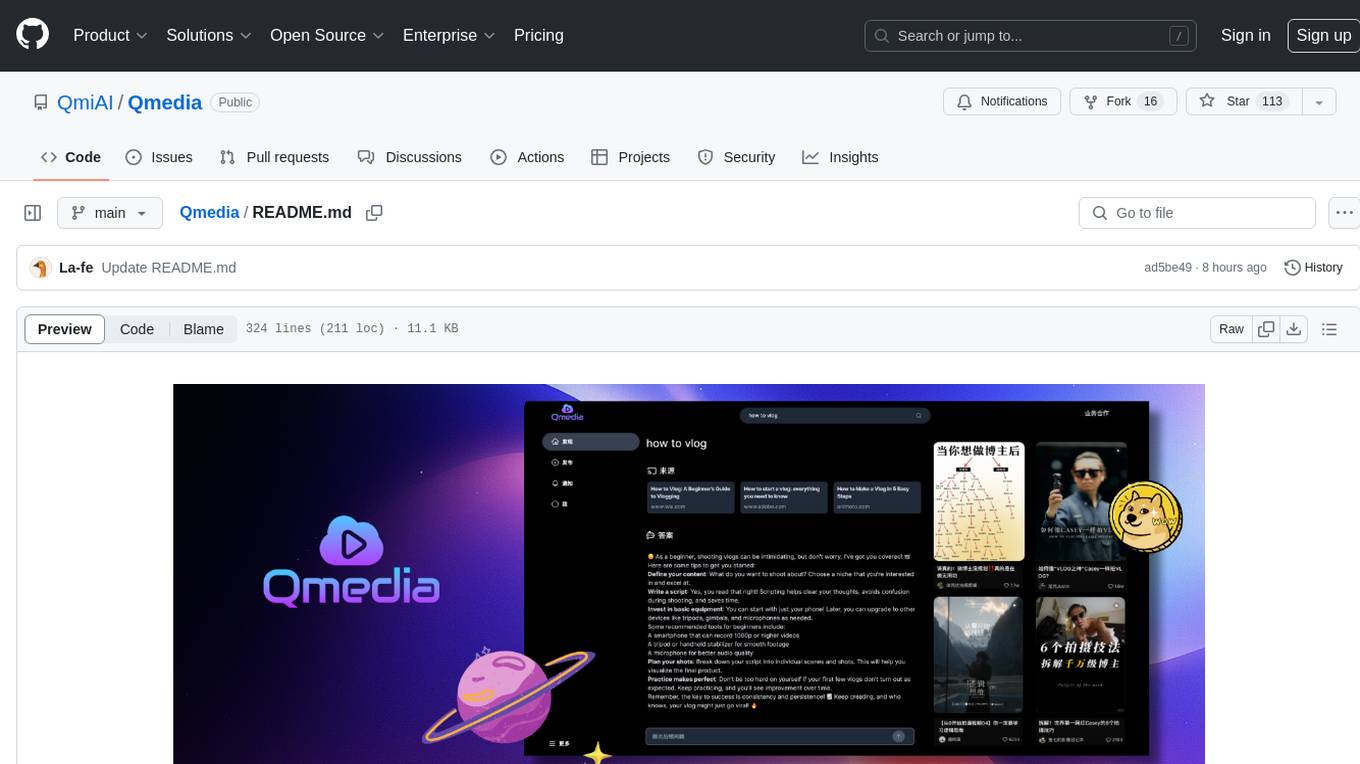
Qmedia
QMedia is an open-source multimedia AI content search engine designed specifically for content creators. It provides rich information extraction methods for text, image, and short video content. The tool integrates unstructured text, image, and short video information to build a multimodal RAG content Q&A system. Users can efficiently search for image/text and short video materials, analyze content, provide content sources, and generate customized search results based on user interests and needs. QMedia supports local deployment for offline content search and Q&A for private data. The tool offers features like content cards display, multimodal content RAG search, and pure local multimodal models deployment. Users can deploy different types of models locally, manage language models, feature embedding models, image models, and video models. QMedia aims to spark new ideas for content creation and share AI content creation concepts in an open-source manner.
For similar tasks
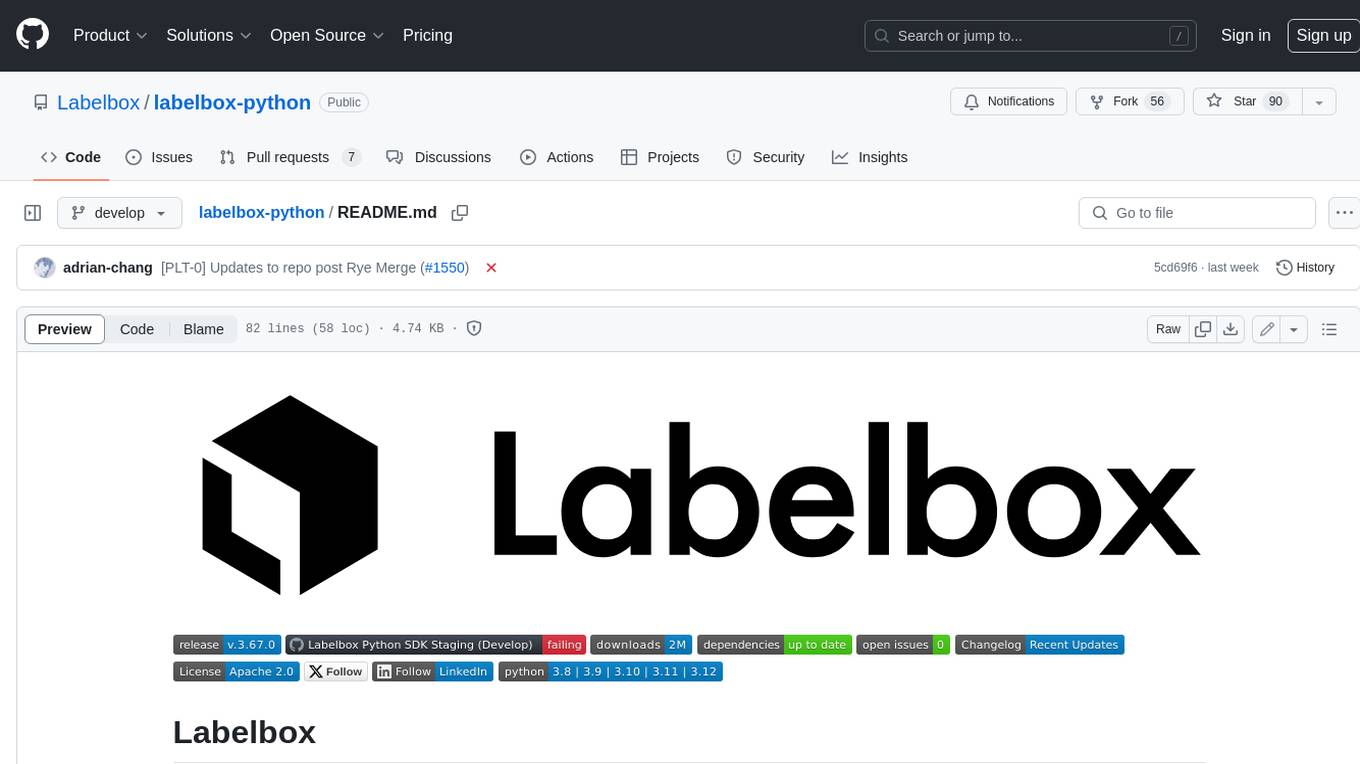
labelbox-python
Labelbox is a data-centric AI platform for enterprises to develop, optimize, and use AI to solve problems and power new products and services. Enterprises use Labelbox to curate data, generate high-quality human feedback data for computer vision and LLMs, evaluate model performance, and automate tasks by combining AI and human-centric workflows. The academic & research community uses Labelbox for cutting-edge AI research.
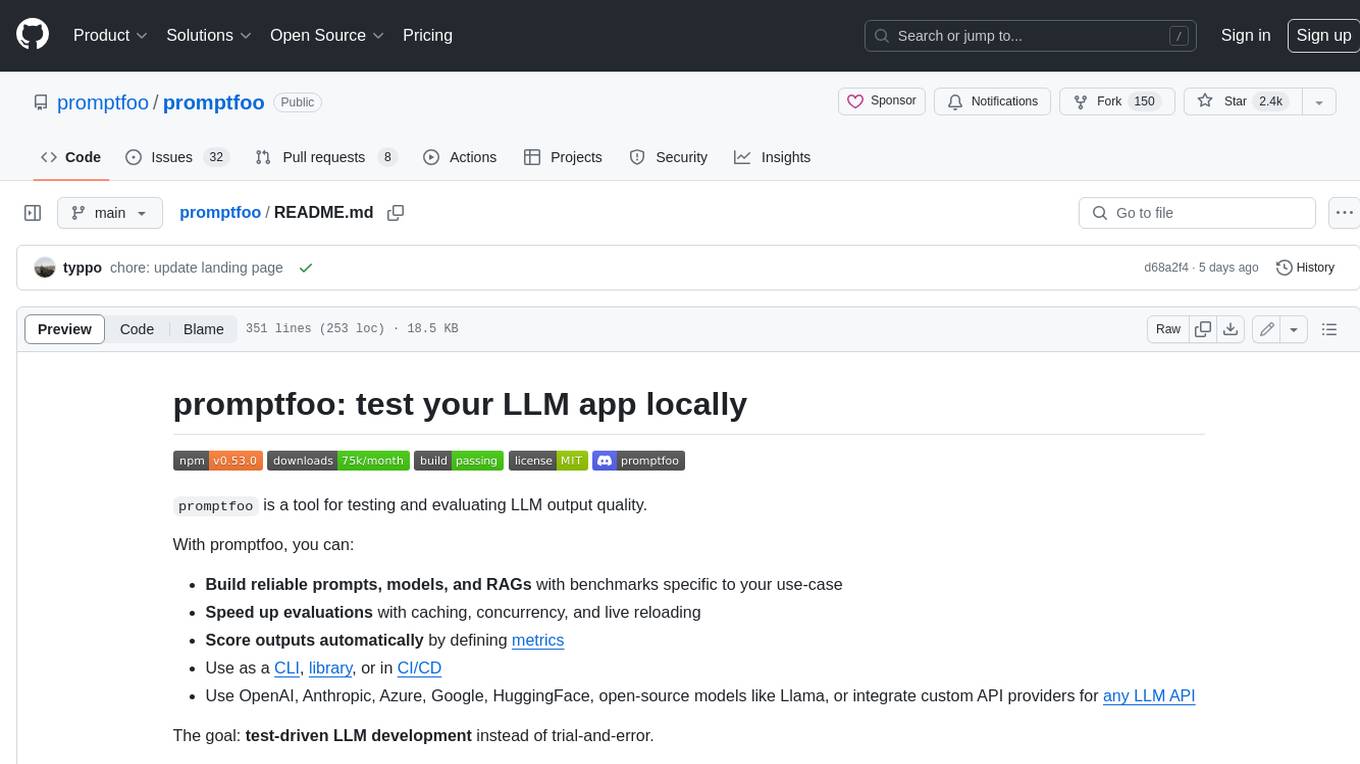
promptfoo
Promptfoo is a tool for testing and evaluating LLM output quality. With promptfoo, you can build reliable prompts, models, and RAGs with benchmarks specific to your use-case, speed up evaluations with caching, concurrency, and live reloading, score outputs automatically by defining metrics, use as a CLI, library, or in CI/CD, and use OpenAI, Anthropic, Azure, Google, HuggingFace, open-source models like Llama, or integrate custom API providers for any LLM API.
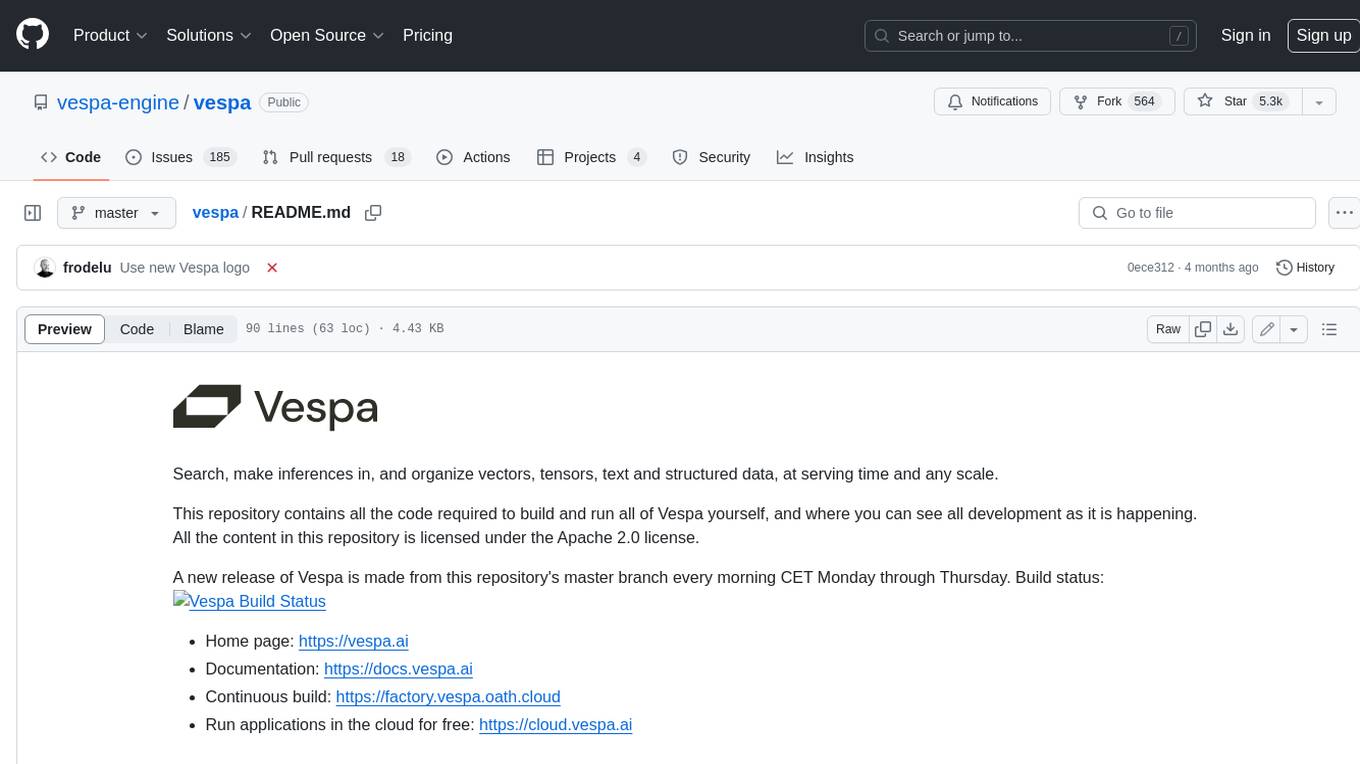
vespa
Vespa is a platform that performs operations such as selecting a subset of data in a large corpus, evaluating machine-learned models over the selected data, organizing and aggregating it, and returning it, typically in less than 100 milliseconds, all while the data corpus is continuously changing. It has been in development for many years and is used on a number of large internet services and apps which serve hundreds of thousands of queries from Vespa per second.
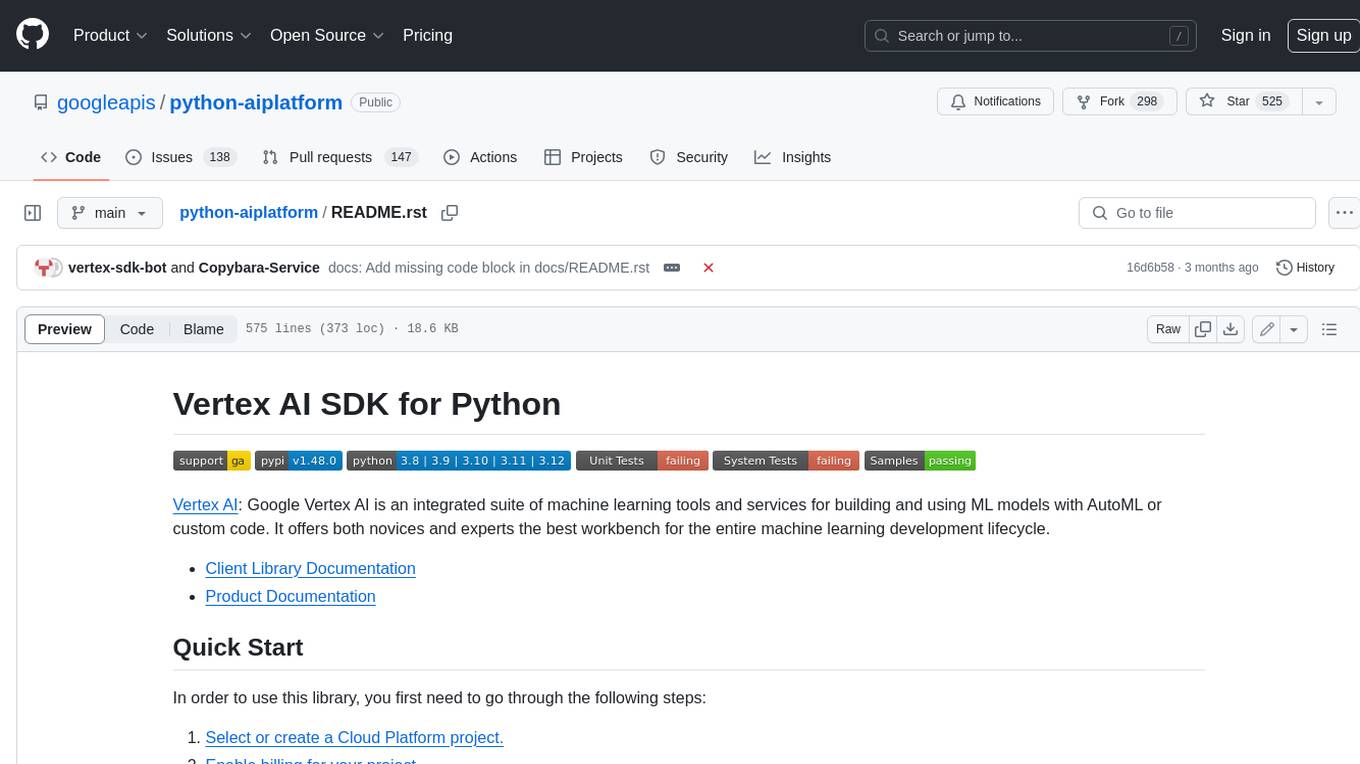
python-aiplatform
The Vertex AI SDK for Python is a library that provides a convenient way to use the Vertex AI API. It offers a high-level interface for creating and managing Vertex AI resources, such as datasets, models, and endpoints. The SDK also provides support for training and deploying custom models, as well as using AutoML models. With the Vertex AI SDK for Python, you can quickly and easily build and deploy machine learning models on Vertex AI.
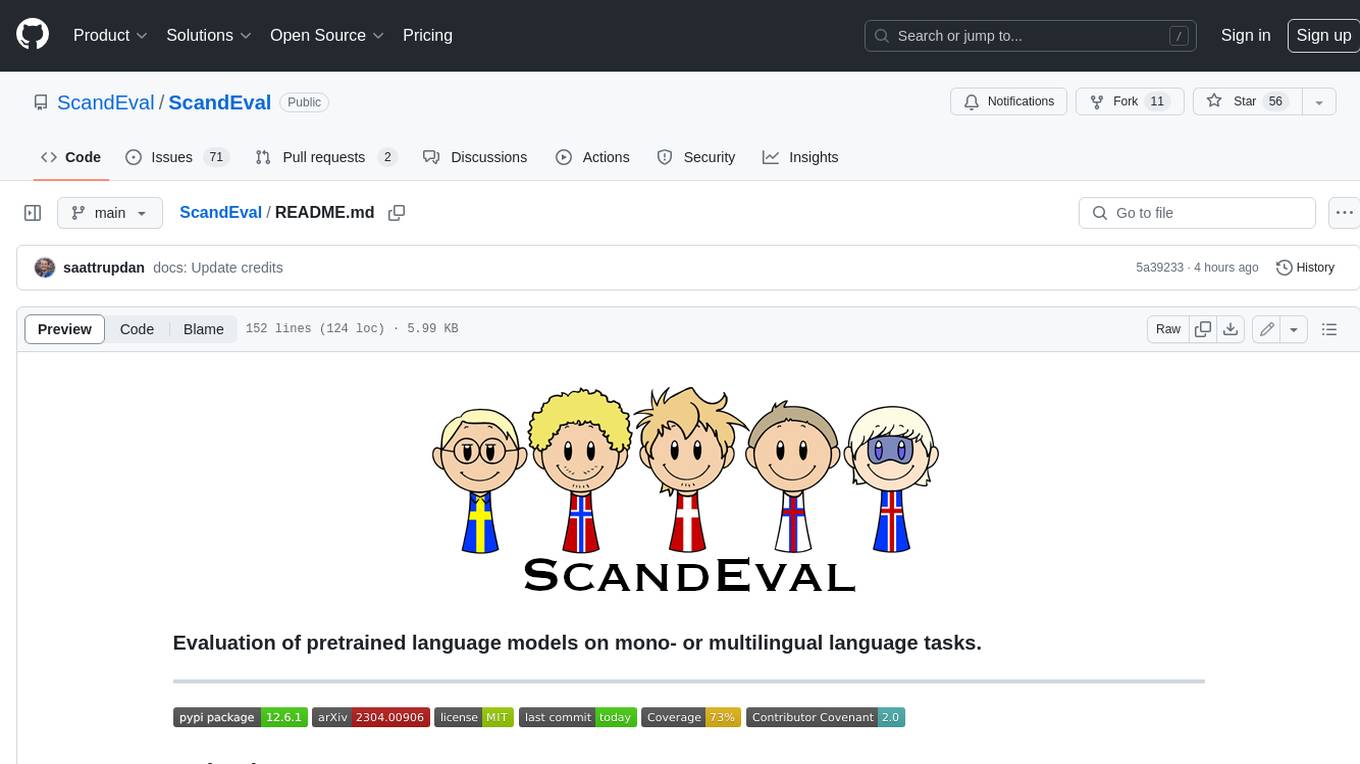
ScandEval
ScandEval is a framework for evaluating pretrained language models on mono- or multilingual language tasks. It provides a unified interface for benchmarking models on a variety of tasks, including sentiment analysis, question answering, and machine translation. ScandEval is designed to be easy to use and extensible, making it a valuable tool for researchers and practitioners alike.
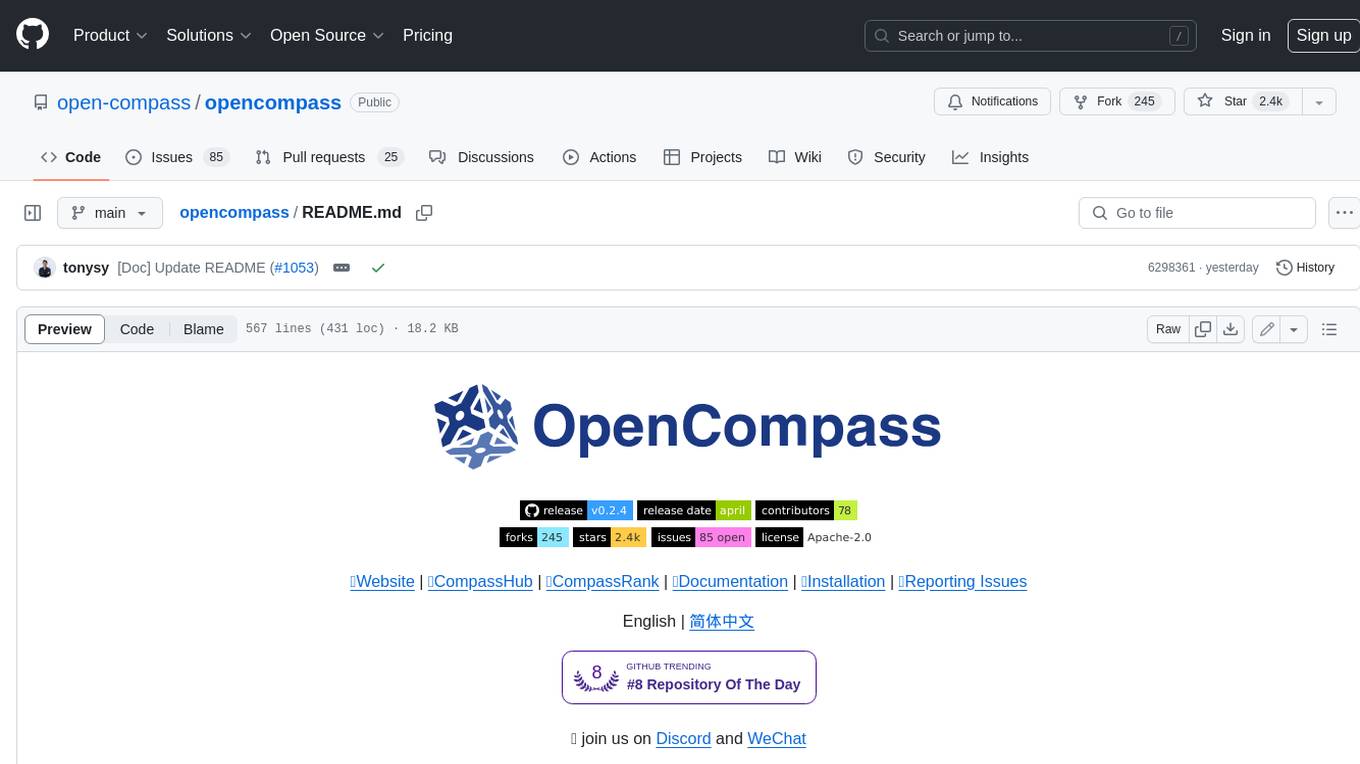
opencompass
OpenCompass is a one-stop platform for large model evaluation, aiming to provide a fair, open, and reproducible benchmark for large model evaluation. Its main features include: * Comprehensive support for models and datasets: Pre-support for 20+ HuggingFace and API models, a model evaluation scheme of 70+ datasets with about 400,000 questions, comprehensively evaluating the capabilities of the models in five dimensions. * Efficient distributed evaluation: One line command to implement task division and distributed evaluation, completing the full evaluation of billion-scale models in just a few hours. * Diversified evaluation paradigms: Support for zero-shot, few-shot, and chain-of-thought evaluations, combined with standard or dialogue-type prompt templates, to easily stimulate the maximum performance of various models. * Modular design with high extensibility: Want to add new models or datasets, customize an advanced task division strategy, or even support a new cluster management system? Everything about OpenCompass can be easily expanded! * Experiment management and reporting mechanism: Use config files to fully record each experiment, and support real-time reporting of results.
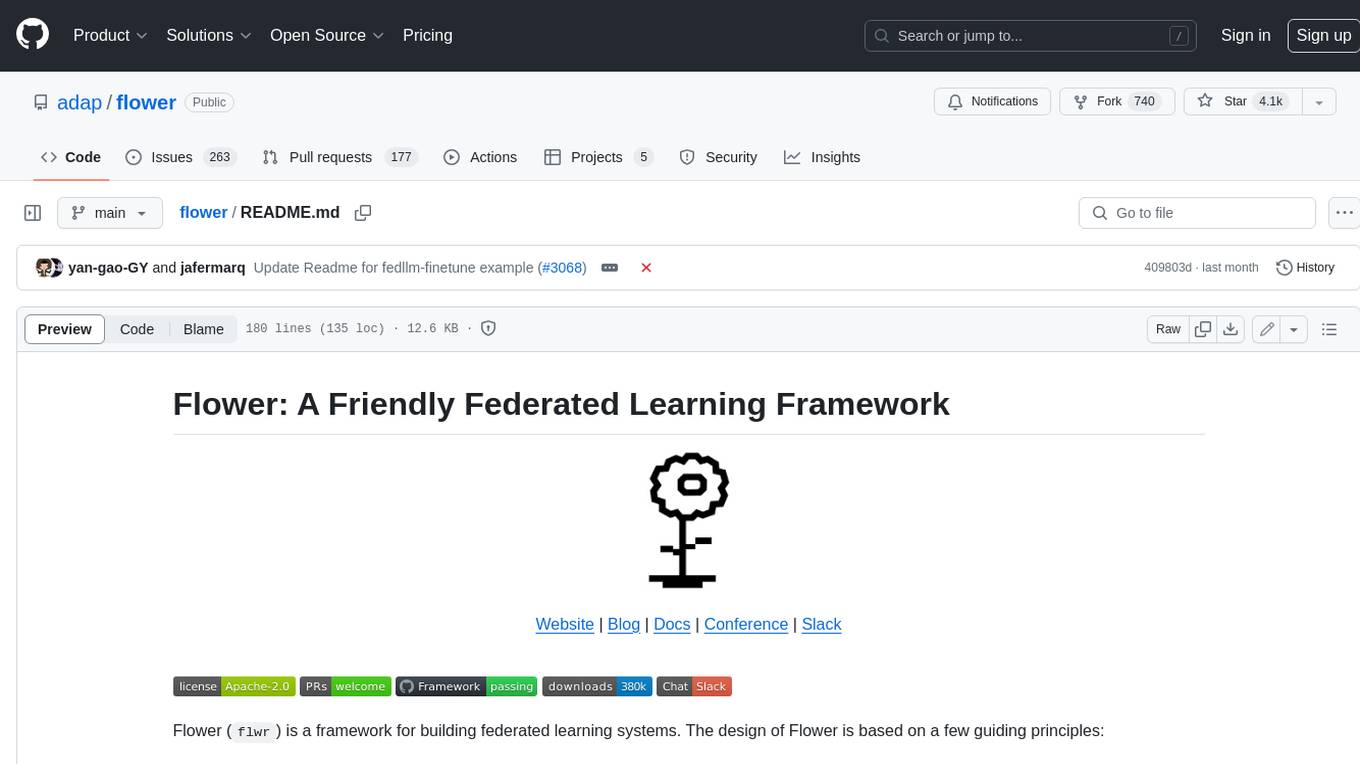
flower
Flower is a framework for building federated learning systems. It is designed to be customizable, extensible, framework-agnostic, and understandable. Flower can be used with any machine learning framework, for example, PyTorch, TensorFlow, Hugging Face Transformers, PyTorch Lightning, scikit-learn, JAX, TFLite, MONAI, fastai, MLX, XGBoost, Pandas for federated analytics, or even raw NumPy for users who enjoy computing gradients by hand.
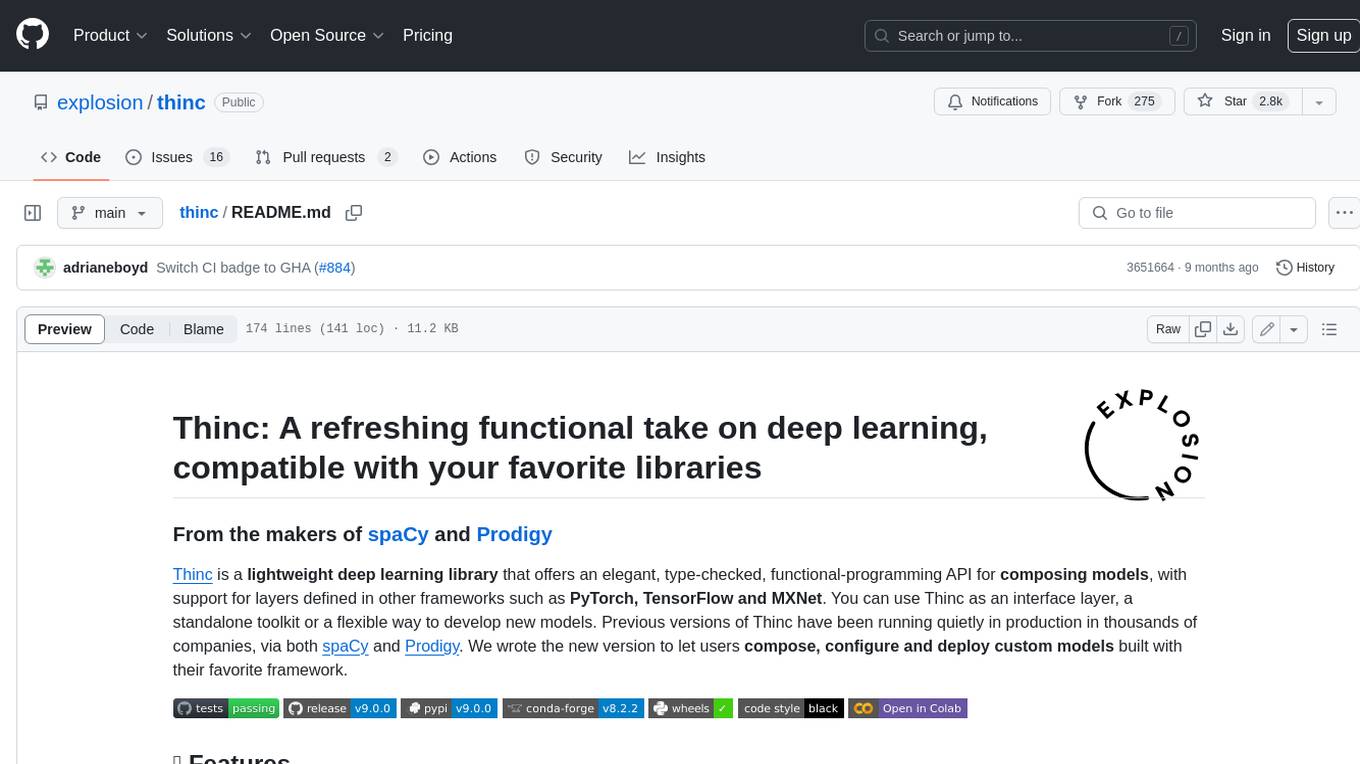
thinc
Thinc is a lightweight deep learning library that offers an elegant, type-checked, functional-programming API for composing models, with support for layers defined in other frameworks such as PyTorch, TensorFlow and MXNet. You can use Thinc as an interface layer, a standalone toolkit or a flexible way to develop new models.
For similar jobs
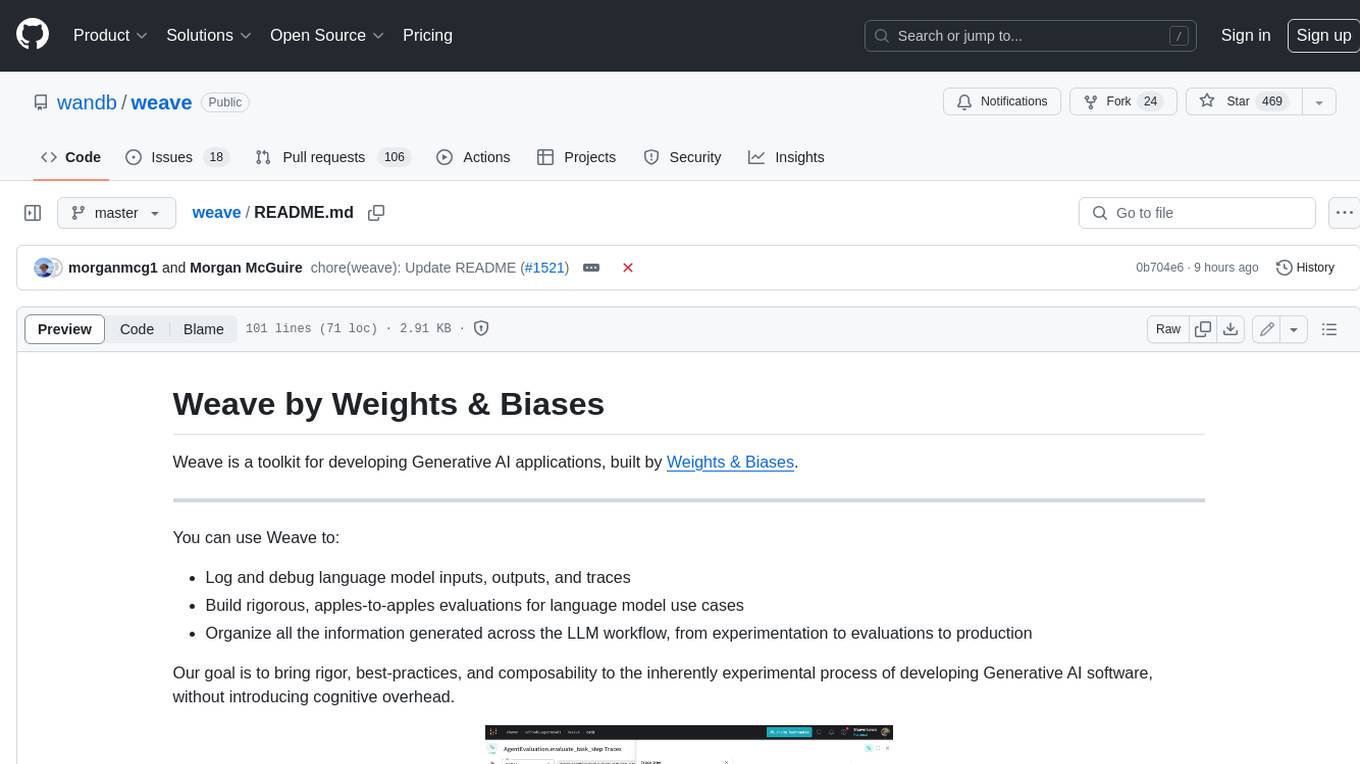
weave
Weave is a toolkit for developing Generative AI applications, built by Weights & Biases. With Weave, you can log and debug language model inputs, outputs, and traces; build rigorous, apples-to-apples evaluations for language model use cases; and organize all the information generated across the LLM workflow, from experimentation to evaluations to production. Weave aims to bring rigor, best-practices, and composability to the inherently experimental process of developing Generative AI software, without introducing cognitive overhead.
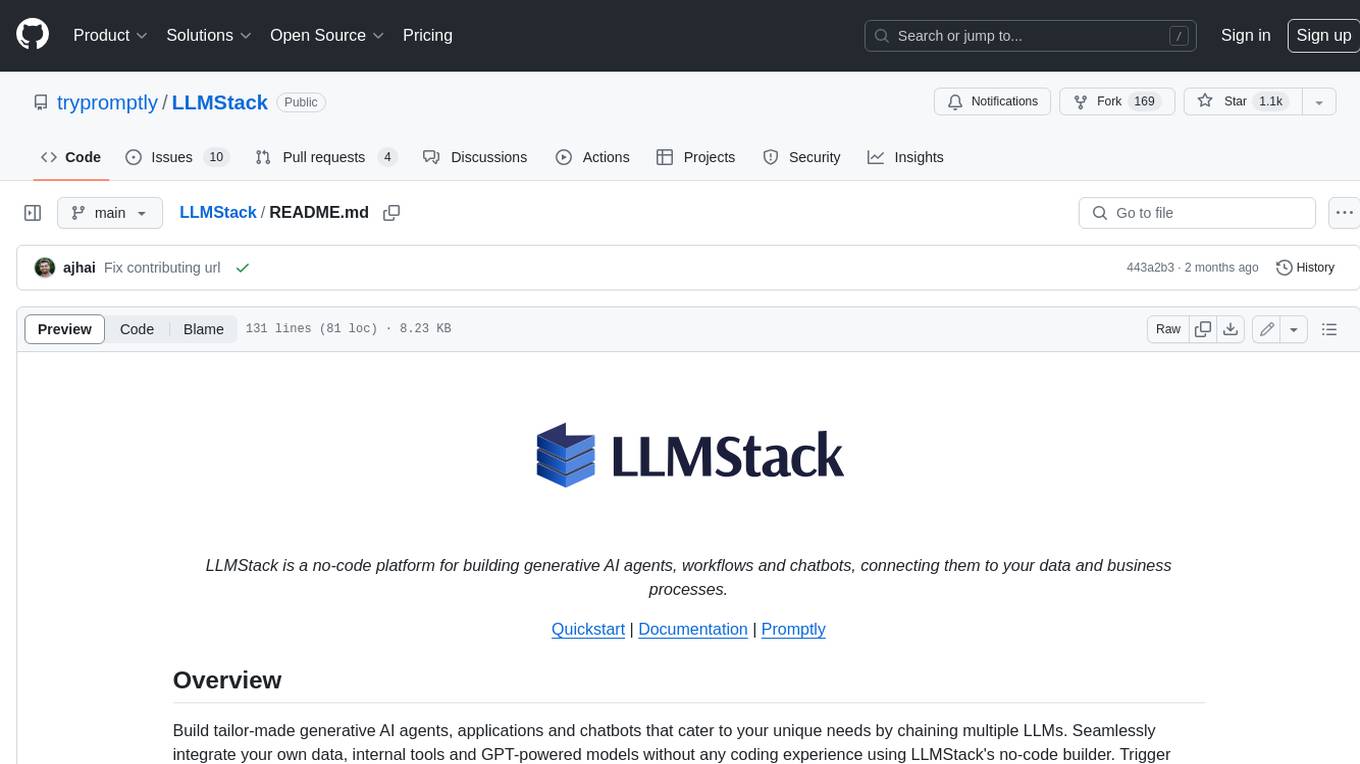
LLMStack
LLMStack is a no-code platform for building generative AI agents, workflows, and chatbots. It allows users to connect their own data, internal tools, and GPT-powered models without any coding experience. LLMStack can be deployed to the cloud or on-premise and can be accessed via HTTP API or triggered from Slack or Discord.
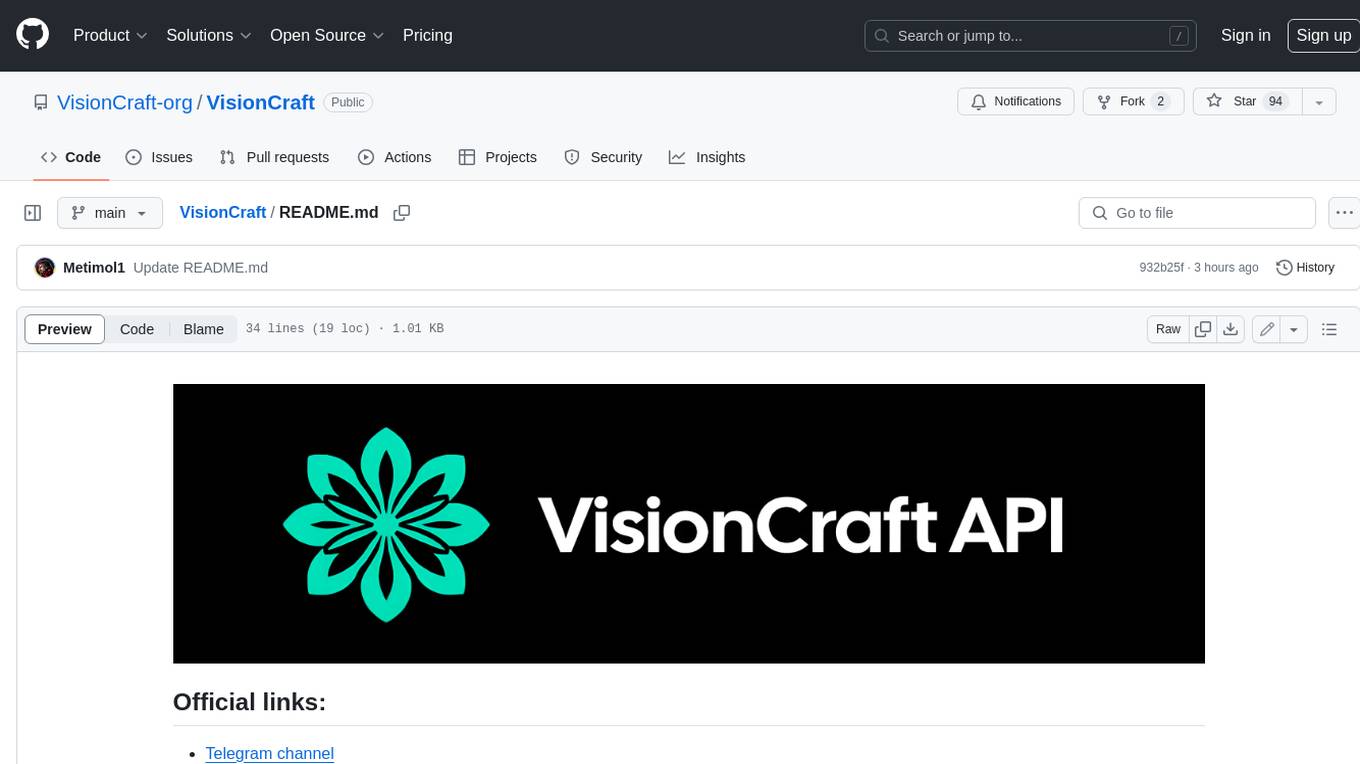
VisionCraft
The VisionCraft API is a free API for using over 100 different AI models. From images to sound.
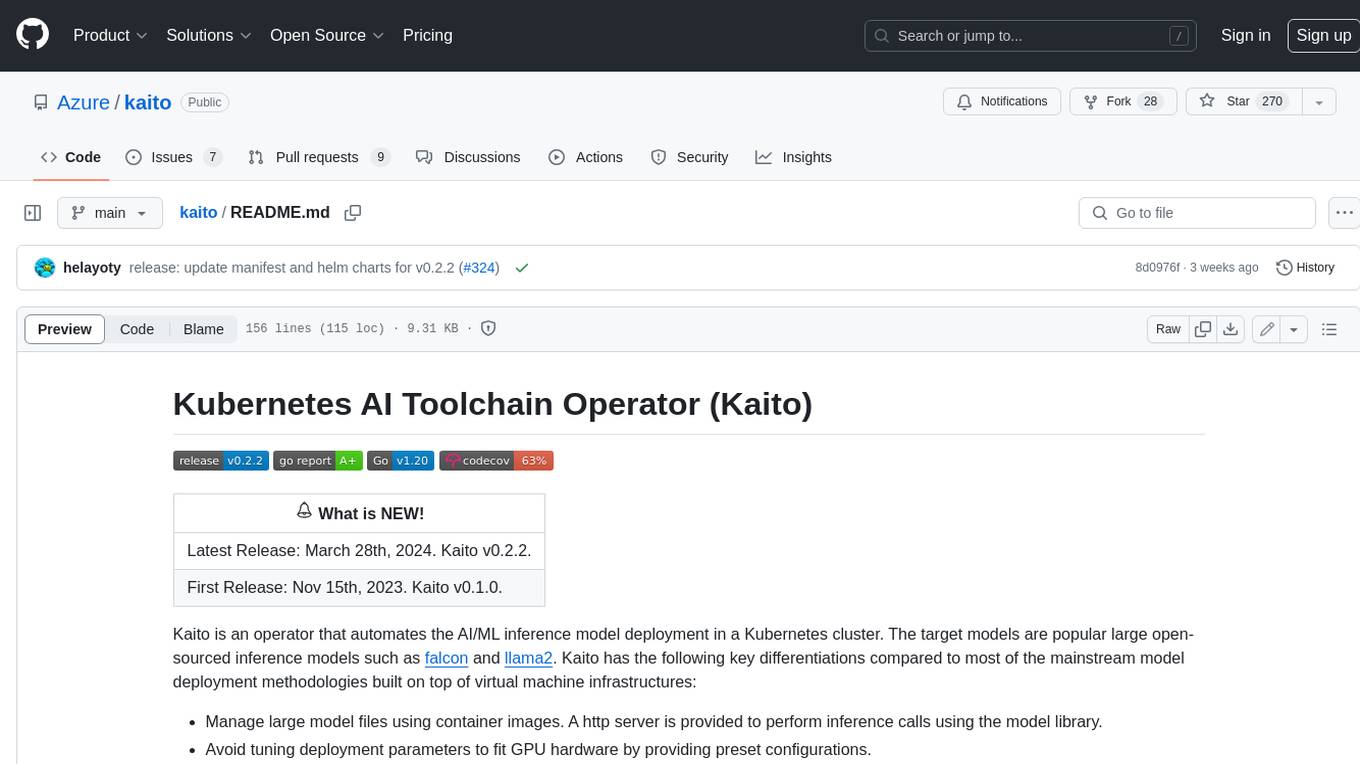
kaito
Kaito is an operator that automates the AI/ML inference model deployment in a Kubernetes cluster. It manages large model files using container images, avoids tuning deployment parameters to fit GPU hardware by providing preset configurations, auto-provisions GPU nodes based on model requirements, and hosts large model images in the public Microsoft Container Registry (MCR) if the license allows. Using Kaito, the workflow of onboarding large AI inference models in Kubernetes is largely simplified.
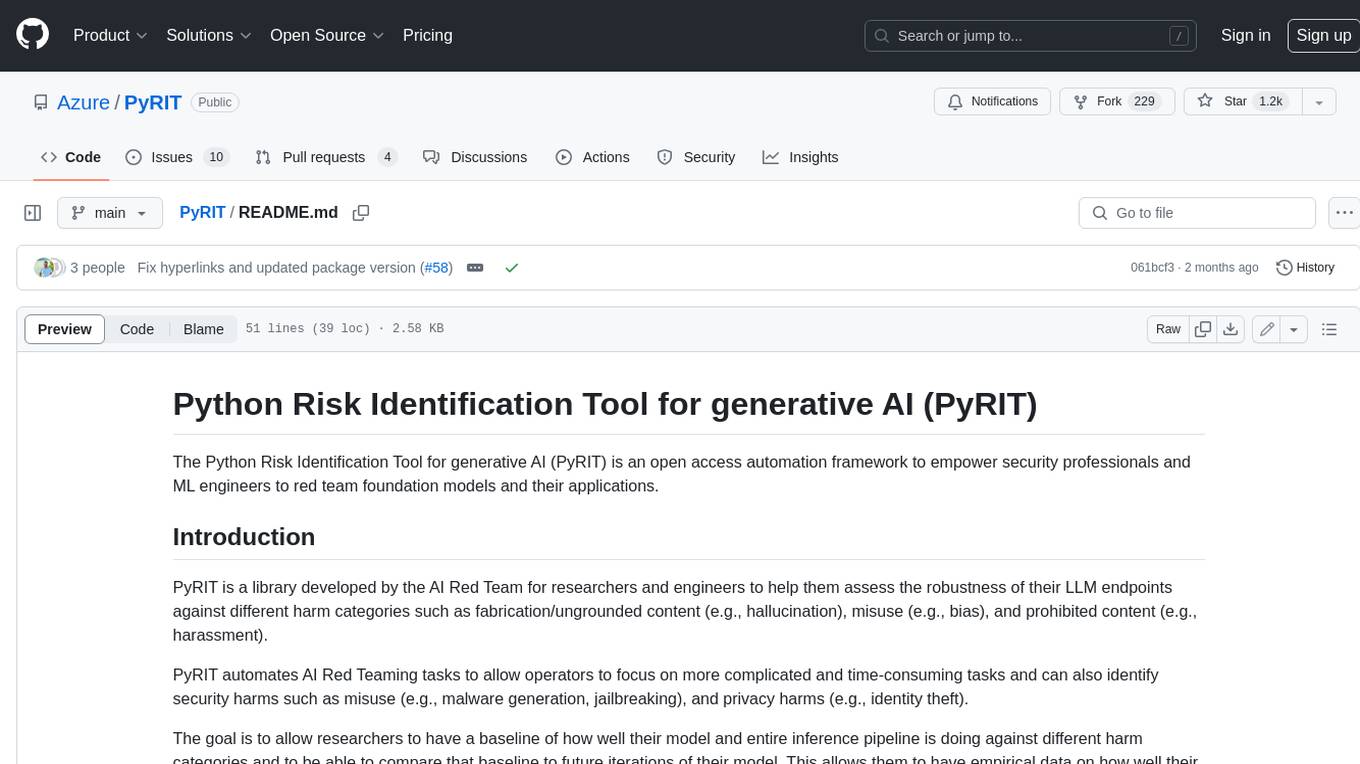
PyRIT
PyRIT is an open access automation framework designed to empower security professionals and ML engineers to red team foundation models and their applications. It automates AI Red Teaming tasks to allow operators to focus on more complicated and time-consuming tasks and can also identify security harms such as misuse (e.g., malware generation, jailbreaking), and privacy harms (e.g., identity theft). The goal is to allow researchers to have a baseline of how well their model and entire inference pipeline is doing against different harm categories and to be able to compare that baseline to future iterations of their model. This allows them to have empirical data on how well their model is doing today, and detect any degradation of performance based on future improvements.
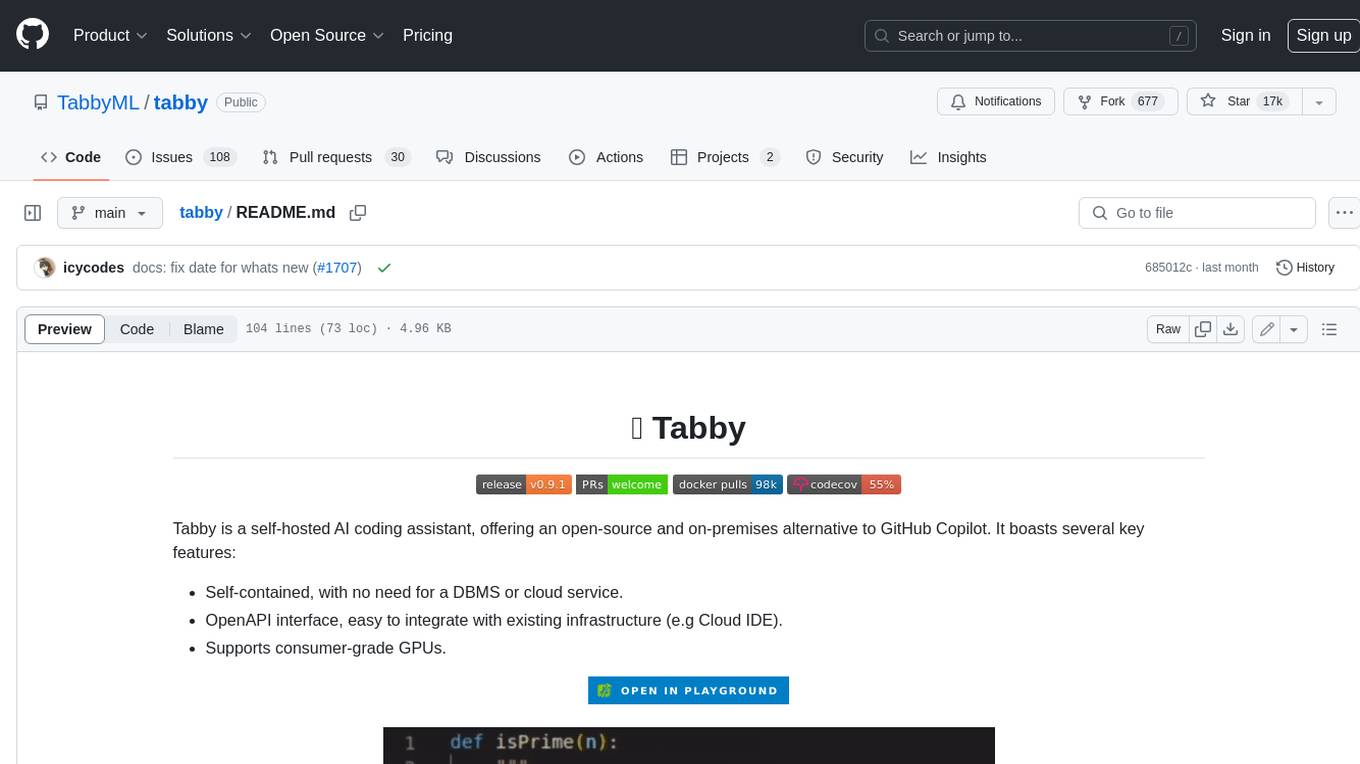
tabby
Tabby is a self-hosted AI coding assistant, offering an open-source and on-premises alternative to GitHub Copilot. It boasts several key features: * Self-contained, with no need for a DBMS or cloud service. * OpenAPI interface, easy to integrate with existing infrastructure (e.g Cloud IDE). * Supports consumer-grade GPUs.
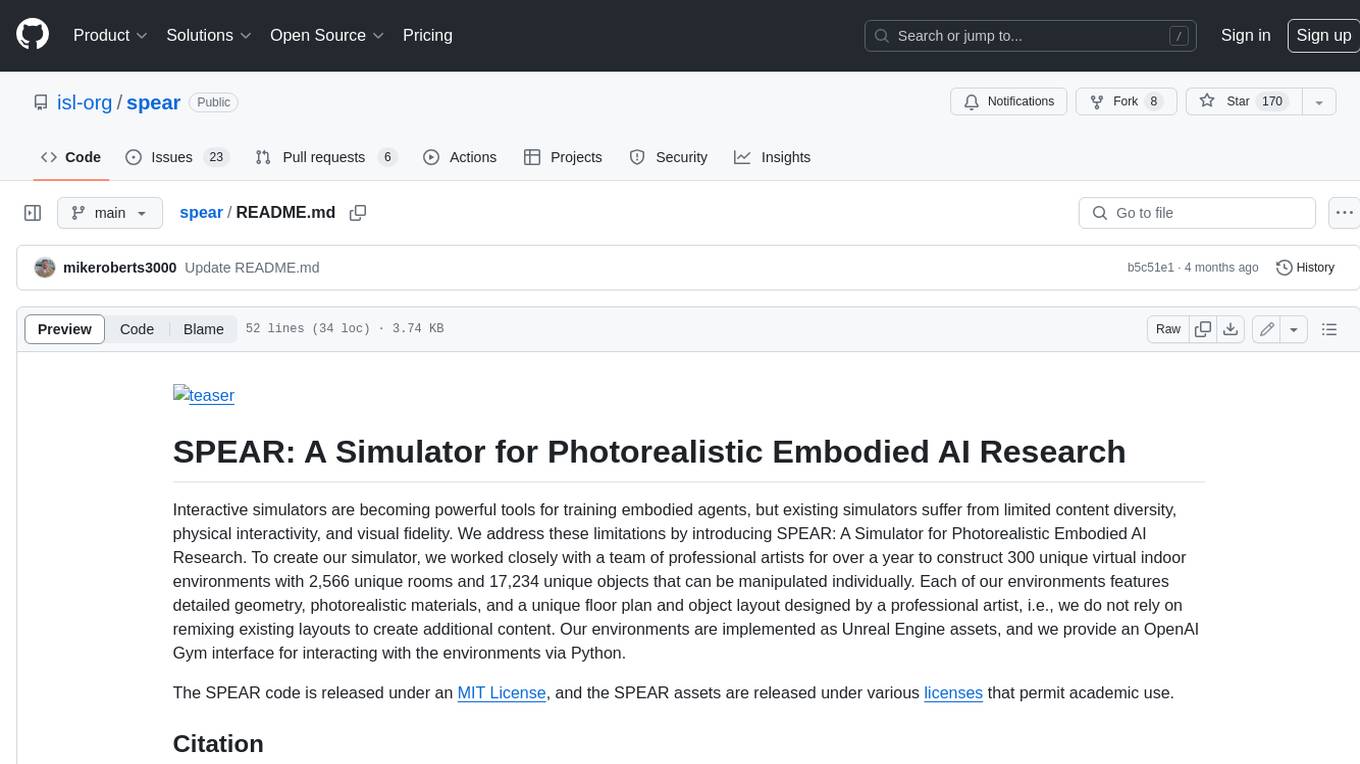
spear
SPEAR (Simulator for Photorealistic Embodied AI Research) is a powerful tool for training embodied agents. It features 300 unique virtual indoor environments with 2,566 unique rooms and 17,234 unique objects that can be manipulated individually. Each environment is designed by a professional artist and features detailed geometry, photorealistic materials, and a unique floor plan and object layout. SPEAR is implemented as Unreal Engine assets and provides an OpenAI Gym interface for interacting with the environments via Python.
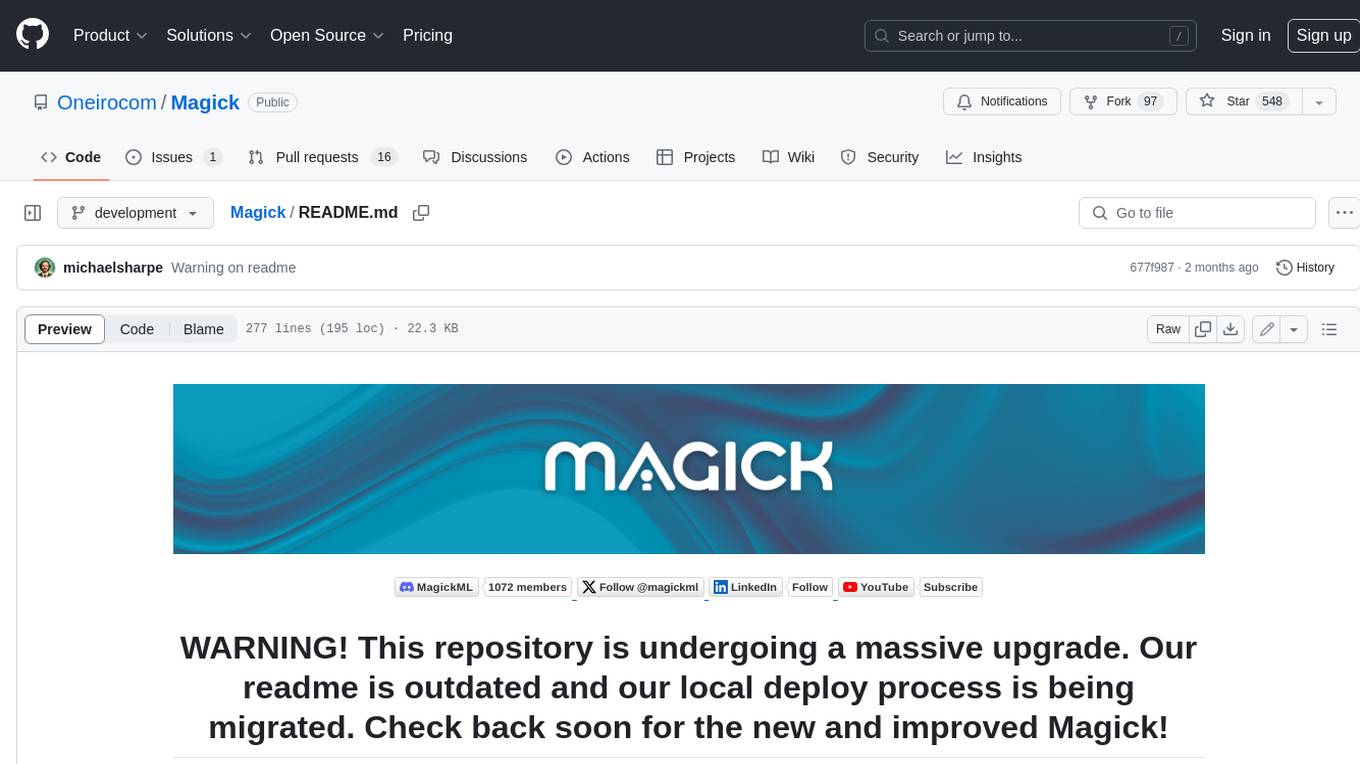
Magick
Magick is a groundbreaking visual AIDE (Artificial Intelligence Development Environment) for no-code data pipelines and multimodal agents. Magick can connect to other services and comes with nodes and templates well-suited for intelligent agents, chatbots, complex reasoning systems and realistic characters.