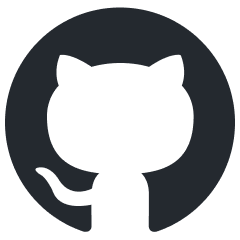
cb-tumblebug
Cloud-Barista Multi-Cloud Infra Management Framework
Stars: 52
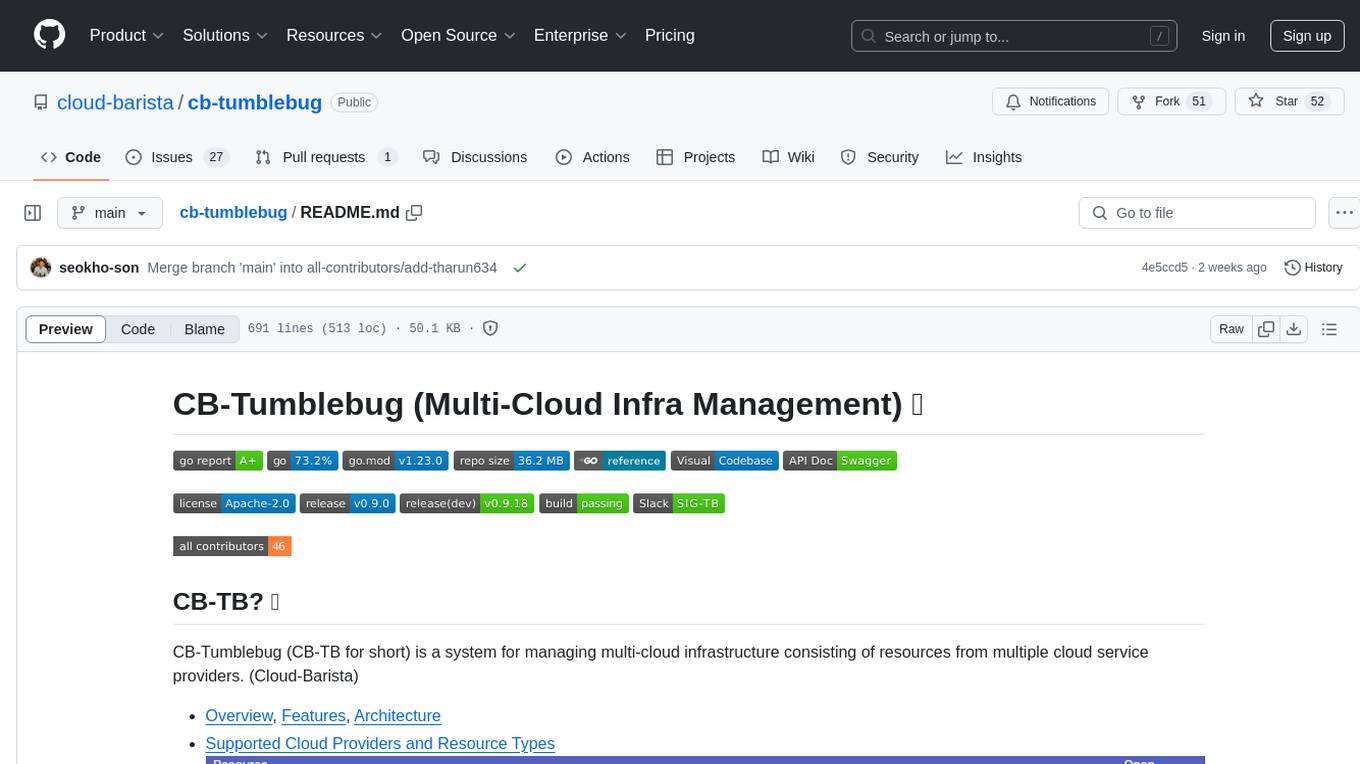
CB-Tumblebug (CB-TB) is a system for managing multi-cloud infrastructure consisting of resources from multiple cloud service providers. It provides an overview, features, and architecture. The tool supports various cloud providers and resource types, with ongoing development and localization efforts. Users can deploy a multi-cloud infra with GPUs, enjoy multiple LLMs in parallel, and utilize LLM-related scripts. The tool requires Linux, Docker, Docker Compose, and Golang for building the source. Users can run CB-TB with Docker Compose or from the Makefile, set up prerequisites, contribute to the project, and view a list of contributors. The tool is licensed under an open-source license.
README:
CB-Tumblebug (CB-TB for short) is a system for managing multi-cloud infrastructure consisting of resources from multiple cloud service providers. (Cloud-Barista)
- Overview, Features, Architecture
-
Supported Cloud Providers and Resource Types
- This is for reference only, and we do not guarantee its functionality. Regular updates are made.
- The support for Kubernetes is currently mostly a WIP, and even when available, it offers only a limited set of features.
Note: Ongoing Development of CB-Tumblebug
CB-TB has not reached version 1.0 yet. We welcome any new suggestions, issues, opinions, and contributors!
Please note that the functionalities of Cloud-Barista are not yet stable or secure.
Be cautious if you plan to use the current release in a production environment.
If you encounter any difficulties using Cloud-Barista,
please let us know by opening an issue or joining the Cloud-Barista Slack.
Note: Localization and Globalization of CB-Tumblebug
As an open-source project initiated by Korean members,
we aim to encourage participation from Korean contributors during the initial stages of this project.
Therefore, the CB-TB repository will accept the use of the Korean language in its early stages.
However, we hope this project will thrive regardless of contributors' countries in the long run.
To facilitate this, the maintainers recommend using English at least for
the titles of Issues, Pull Requests, and Commits, while accommodating local languages in the contents.
-
Deploy a Multi-Cloud Infra with GPUs and Enjoy muiltple LLMs in parallel (YouTube)
- LLM-related scripts
- Linux (recommend:
Ubuntu 22.04
) - Docker and Docker Compose
- Golang (recommend:
v1.23.0
) to build the source
Open source packages used in this project
-
Clone the CB-Tumblebug repository:
git clone https://github.com/cloud-barista/cb-tumblebug.git $HOME/go/src/github.com/cloud-barista/cb-tumblebug cd ~/go/src/github.com/cloud-barista/cb-tumblebug
Optionally, you can register aliases for the CB-Tumblebug directory to simplify navigation:
echo "alias cdtb='cd $HOME/go/src/github.com/cloud-barista/cb-tumblebug'" >> ~/.bashrc echo "alias cdtbsrc='cd $HOME/go/src/github.com/cloud-barista/cb-tumblebug/src'" >> ~/.bashrc echo "alias cdtbtest='cd $HOME/go/src/github.com/cloud-barista/cb-tumblebug/src/testclient/scripts'" >> ~/.bashrc source ~/.bashrc
-
Check Docker Compose Installation:
Ensure that Docker Engine and Docker Compose are installed on your system. If not, you can use the following script to install them (note: this script is not intended for production environments):
cd ~/go/src/github.com/cloud-barista/cb-tumblebug ./scripts/installDocker.sh
-
Start All Components Using Docker Compose:
To run all components, use the following command:
cd ~/go/src/github.com/cloud-barista/cb-tumblebug sudo docker compose up
This command will start all components as defined in the preconfigured docker-compose.yaml file. For configuration customization, please refer to the guide.
The following components will be started:
- ETCD: CB-Tumblebug KeyValue DB
- CB-Spider: a Cloud API controller
- CB-MapUI: a simple Map-based GUI web server
- CB-Tumblebug: the system with API server
After running the command, you should see output similar to the following:
Now, the CB-Tumblebug API server is accessible at: http://localhost:1323/tumblebug/api Additionally, CB-MapUI is accessible at: http://localhost:1324
Note: Before using CB-Tumblebug, you need to initialize it.
To provisioning multi-cloud infrastructures with CB-TB, it is necessary to register the connection information (credentials) for clouds, as well as commonly used images and specifications.
-
Create
credentials.yaml
file and input your cloud credentials-
Overview
-
credentials.yaml
is a file that includes multiple credentials to use API of Clouds supported by CB-TB (AWS, GCP, AZURE, ALIBABA, etc.) - It should be located in the
~/.cloud-barista/
directory and securely managed. - Refer to the
template.credentials.yaml
for the template
-
-
Create
credentials.yaml
the fileAutomatically generate the
credentials.yaml
file in the~/.cloud-barista/
directory using the CB-TB scriptcd ~/go/src/github.com/cloud-barista/cb-tumblebug ./init/genCredential.sh
-
Input credential data
Put credential data to
~/.cloud-barista/credentials.yaml
(Reference: How to obtain a credential for each CSP)### Cloud credentials for credential holders (default: admin) credentialholder: admin: alibaba: # ClientId(ClientId): client ID of the EIAM application # Example: app_mkv7rgt4d7i4u7zqtzev2mxxxx ClientId: # ClientSecret(ClientSecret): client secret of the EIAM application # Example: CSEHDcHcrUKHw1CuxkJEHPveWRXBGqVqRsxxxx ClientSecret: aws: # ClientId(aws_access_key_id) # ex: AKIASSSSSSSSSSS56DJH ClientId: # ClientSecret(aws_secret_access_key) # ex: jrcy9y0Psejjfeosifj3/yxYcgadklwihjdljMIQ0 ClientSecret: ...
-
-
Encrypt
credentials.yaml
intocredentials.yaml.enc
To protect sensitive information,
credentials.yaml
is not used directly. Instead, it must be encrypted usingencCredential.sh
. The encrypted filecredentials.yaml.enc
is then used byinit.py
. This approach ensures that sensitive credentials are not stored in plain text.If you need to update your credentials, decrypt the encrypted file using
decCredential.sh
, make the necessary changes tocredentials.yaml
, and then re-encrypt it. -
(INIT) Register all multi-cloud connection information and common resources
-
How to register
Refer to README.md for init.py, and execute the
init.py
script. (enter 'y' for confirmation prompts)cd ~/go/src/github.com/cloud-barista/cb-tumblebug ./init/init.sh
-
The credentials in
~/.cloud-barista/credentials.yaml.enc
(encrypted file from thecredentials.yaml
) will be automatically registered (all CSP and region information recorded incloudinfo.yaml
will be automatically registered in the system)- Note: You can check the latest regions and zones of CSP using
update-cloudinfo.py
and review the file for updates. (contributions to updates are welcome)
- Note: You can check the latest regions and zones of CSP using
-
Common images and specifications recorded in the
cloudimage.csv
andcloudspec.csv
files in theassets
directory will be automatically registered. -
init.py
will apply the hybrid encryption for secure transmission of credentials- Retrieve RSA Public Key: Use the
/credential/publicKey
API to get the public key. - Encrypt Credentials: Encrypt credentials with a randomly generated
AES
key, then encrypt theAES
key with theRSA public key
. - Transmit Encrypted Data: Send
the encrypted credentials
andAES key
to the server. The server decrypts the AES key and uses it to decrypt the credentials.
This method ensures your credentials are securely transmitted and protected during registration. See init.py for a Python implementation. In detail, check out Secure Credential Registration Guide (How to use the credential APIs)
- Retrieve RSA Public Key: Use the
-
-
-
Shutting down CB-TB and related components
-
Stop all containers by
ctrl
+c
or type the commandsudo docker compose stop
/sudo docker compose down
(When a shutdown event occurs to CB-TB, the system will be shutting down gracefully: API requests that can be processed within 10 seconds will be completed) -
In case of cleanup is needed due to internal system errors
- Check and delete resources created through CB-TB
- Delete CB-TB & CB-Spider metadata using the provided script
cd ~/go/src/github.com/cloud-barista/cb-tumblebug ./init/cleanDB.sh
-
-
Upgrading the CB-TB & CB-Spider versions
The following cleanup steps are unnecessary if you clearly understand the impact of the upgrade
- Check and delete resources created through CB-TB
- Delete CB-TB & CB-Spider metadata
cd ~/go/src/github.com/cloud-barista/cb-tumblebug ./init/cleanDB.sh
- Restart with the upgraded version
- Using CB-TB MapUI (recommended)
- Using CB-TB REST API (recommended)
- Using CB-TB Test Scripts
- With CB-MapUI, you can create, view, and control Mutli-Cloud infra.
- CB-MapUI is a project to visualize the deployment of MCI in a map GUI.
- CB-MapUI also run with CB-Tumblebug by default (edit
dockercompose.yaml
to disable)- If you run the CB-MapUI container using the CB-TB script, excute
cd ~/go/src/github.com/cloud-barista/cb-tumblebug ./scripts/runMapUI.sh
- If you run the CB-MapUI container using the CB-TB script, excute
- Access via web browser at http://{HostIP}:1324
-
Access to REST API dashboard
-
Using individual APIs
- Create resources required for VM provisioning by using Resource(multi-cloud infrastructure resources) management APIs
- Create, view, control, execute remote commands, shut down, and delete MCI using the MCI(multi-cloud infrastructure service) management APIs
- CB-TB optimal and dynamic provisioning
src/testclient/scripts/
provides Bash shell-based scripts that simplify and automate the MCI (MC-Infra) provisioning procedures, which require complex steps.
[Note] Details
- Step 1: Setup Test Environment
- Step 2: Integrated Tests
- Go to
src/testclient/scripts/
- Configure
conf.env
- Provide basic test information such as CB-Spider and CB-TB server endpoints, cloud regions, test image names, test spec names, etc.
- Much information for various cloud types has already been investigated and input, so it can be used without modification. (However, check for charges based on the specified spec)
- How to modify test VM image:
IMAGE_NAME[$IX,$IY]=ami-061eb2b23f9f8839c
- How to modify test VM spec:
SPEC_NAME[$IX,$IY]=m4.4xlarge
- How to modify test VM image:
- Configure
testSet.env
- Set the cloud and region configurations to be used for MCI provisioning in a file (you can change the existing
testSet.env
or copy and use it) - Specify the types of CSPs to combine
- Change the number in NumCSP= to specify the total number of CSPs to combine
- Specify the types of CSPs to combine by rearranging the lines in L15-L24 (use up to the number specified in NumCSP)
- Example: To combine aws and alibaba, change NumCSP=2 and rearrange
IndexAWS=$((++IX))
,IndexAlibaba=$((++IX))
- Specify the regions of the CSPs to combine
- Go to each CSP setting item
# AWS (Total: 21 Regions)
- Specify the number of regions to configure in
NumRegion[$IndexAWS]=2
(in the example, it is set to 2) - Set the desired regions by rearranging the lines of the region list (if
NumRegion[$IndexAWS]=2
, the top 2 listed regions will be selected)
- Go to each CSP setting item
-
Be aware!
- Be aware that creating VMs on public CSPs such as AWS, GCP, Azure, etc. may incur charges.
- With the default setting of
testSet.env
, TestClouds (TestCloud01
,TestCloud02
,TestCloud03
) will be used to create mock VMs. -
TestCloud01
,TestCloud02
,TestCloud03
are not real CSPs. They are used for testing purposes (do not support SSH into VM). - Anyway, please be aware of cloud usage costs when using public CSPs.
- Set the cloud and region configurations to be used for MCI provisioning in a file (you can change the existing
-
You can test the entire process at once by executing
create-all.sh
andclean-all.sh
included insrc/testclient/scripts/sequentialFullTest/
└── sequentialFullTest # Automatic testing from cloud information registration to NS creation, Resource creation, and MCI creation ├── check-test-config.sh # Check the multi-cloud infrastructure configuration specified in the current testSet ├── create-all.sh # Automatic testing from cloud information registration to NS creation, Resource creation, and MCI creation ├── gen-sshKey.sh # Generate SSH key files to access MCI ├── command-mci.sh # Execute remote commands on the created MCI (multiple VMs) ├── deploy-nginx-mci.sh # Automatically deploy Nginx on the created MCI (multiple VMs) ├── create-mci-for-df.sh # Create MCI for hosting CB-Dragonfly ├── deploy-dragonfly-docker.sh # Automatically deploy CB-Dragonfly on MCI and set up the environment ├── clean-all.sh # Delete all objects in reverse order of creation ├── create-k8scluster-only.sh # Create a K8s cluster for the multi-cloud infrastructure specified in the testSet ├── get-k8scluster.sh # Get K8s cluster information for the multi-cloud infrastructure specified in the testSet ├── clean-k8scluster-only.sh # Delete the K8s cluster for the multi-cloud infrastructure specified in the testSet ├── force-clean-k8scluster-only.sh # Force delete the K8s cluster for the multi-cloud infrastructure specified in the testSet if deletion fails ├── add-k8snodegroup.sh # Add a new K8s node group to the created K8s cluster ├── remove-k8snodegroup.sh # Delete the newly created K8s node group in the K8s cluster ├── set-k8snodegroup-autoscaling.sh # Change the autoscaling setting of the created K8s node group to off ├── change-k8snodegroup-autoscalesize.sh # Change the autoscale size of the created K8s node group ├── deploy-weavescope-to-k8scluster.sh # Deploy weavescope to the created K8s cluster └── executionStatus # Logs of the tests performed (information is added when testAll is executed and removed when cleanAll is executed. You can check the ongoing tasks)
-
MCI Creation Test
-
./create-all.sh -n shson -f ../testSetCustom.env
# Create MCI with the cloud combination configured in ../testSetCustom.env -
Automatically proceed with the process to check the MCI creation configuration specified in
../testSetCustom.env
-
Example of execution result
Table: All VMs in the MCI : cb-shson ID Status PublicIP PrivateIP CloudType CloudRegion CreatedTime -- ------ -------- --------- --------- ----------- ----------- aws-ap-southeast-1-0 Running xx.250.xx.73 192.168.2.180 aws ap-southeast-1 2021-09-17 14:59:30 aws-ca-central-1-0 Running x.97.xx.230 192.168.4.98 aws ca-central-1 2021-09-17 14:59:58 gcp-asia-east1-0 Running xx.229.xxx.26 192.168.3.2 gcp asia-east1 2021-09-17 14:59:42 [DATE: 17/09/2021 15:00:00] [ElapsedTime: 49s (0m:49s)] [Command: ./create-mci-only.sh all 1 shson ../testSetCustom.env 1] [Executed Command List] [Resource:aws-ap-southeast-1(28s)] create-resource-ns-cloud.sh (Resource) aws 1 shson ../testSetCustom.env [Resource:aws-ca-central-1(34s)] create-resource-ns-cloud.sh (Resource) aws 2 shson ../testSetCustom.env [Resource:gcp-asia-east1(93s)] create-resource-ns-cloud.sh (Resource) gcp 1 shson ../testSetCustom.env [MCI:cb-shsonvm4(19s+More)] create-mci-only.sh (MCI) all 1 shson ../testSetCustom.env [DATE: 17/09/2021 15:00:00] [ElapsedTime: 149s (2m:29s)] [Command: ./create-all.sh -n shson -f ../testSetCustom.env -x 1]
-
-
MCI Removal Test (Use the input parameters used in creation for deletion)
-
./clean-all.sh -n shson -f ../testSetCustom.env
# Perform removal of created resources according to../testSetCustom.env
-
Be aware!
- If you created MCI (VMs) for testing in public clouds, the VMs may incur charges.
- You need to terminate MCI by using
clean-all
to avoid unexpected billing. - Anyway, please be aware of cloud usage costs when using public CSPs.
-
-
Generate MCI SSH access keys and access each VM
-
./gen-sshKey.sh -n shson -f ../testSetCustom.env
# Return access keys for all VMs configured in MCI -
Example of execution result
... [GENERATED PRIVATE KEY (PEM, PPK)] [MCI INFO: mc-shson] [VMIP]: 13.212.254.59 [MCIID]: mc-shson [VMID]: aws-ap-southeast-1-0 ./sshkey-tmp/aws-ap-southeast-1-shson.pem ./sshkey-tmp/aws-ap-southeast-1-shson.ppk ... [SSH COMMAND EXAMPLE] [VMIP]: 13.212.254.59 [MCIID]: mc-shson [VMID]: aws-ap-southeast-1-0 ssh -i ./sshkey-tmp/aws-ap-southeast-1-shson.pem [email protected] -o StrictHostKeyChecking=no ... [VMIP]: 35.182.30.37 [MCIID]: mc-shson [VMID]: aws-ca-central-1-0 ssh -i ./sshkey-tmp/aws-ca-central-1-shson.pem [email protected] -o StrictHostKeyChecking=no
-
-
Verify MCI via SSH remote command execution
-
./command-mci.sh -n shson -f ../testSetCustom.env
# Execute IP and hostname retrieval for all VMs in MCI
-
-
K8s Cluster Test (WIP: Stability work in progress for each CSP)
./create-resource-ns-cloud.sh -n tb -f ../testSet.env` # Create Resource required for K8s cluster creation ./create-k8scluster-only.sh -n tb -f ../testSet.env -x 1 -z 1` # Create K8s cluster (-x maximum number of nodes, -z additional name for K8s node group and K8s cluster) ./get-k8scluster.sh -n tb -f ../testSet.env -z 1` # Get K8s cluster information ./add-k8snodegroup.sh -n tb -f ../testSet.env -x 1 -z 1` # Add a new K8s node group to the K8s cluster ./change-k8snodegroup-autoscalesize.sh -n tb -f ../testSet.env -x 1 -z 1` # Change the autoscale size of the specified K8s node group ./deploy-weavescope-to-k8scluster.sh -n tb -f ../testSet.env -y n` # Deploy weavescope to the created K8s cluster ./set-k8snodegroup-autoscaling.sh -n tb -f ../testSet.env -z 1` # Change the autoscaling setting of the new K8s node group to off ./remove-k8snodegroup.sh -n tb -f ../testSet.env -z 1` # Delete the newly created K8s node group ./clean-k8scluster-only.sh -n tb -f ../testSet.env -z 1` # Delete the created K8s cluster ./force-clean-k8scluster-only.sh -n tb -f ../testSet.env -z 1` # Force delete the created K8s cluster if deletion fails ./clean-resource-ns-cloud.h -n tb -f ../testSet.env` # Delete the created Resource
-
Setup required tools
-
Install: git, gcc, make
sudo apt update sudo apt install make gcc git
-
Install: Golang
-
Check https://golang.org/dl/ and setup Go
-
Download
wget https://go.dev/dl/go1.23.0.linux-amd64.tar.gz; sudo rm -rf /usr/local/go && sudo tar -C /usr/local -xzf go1.23.0.linux-amd64.tar.gz
-
Setup environment
echo 'export PATH=$PATH:/usr/local/go/bin:$HOME/go/bin' >> ~/.bashrc echo 'export GOPATH=$HOME/go' >> ~/.bashrc
source ~/.bashrc echo $GOPATH go env go version
-
-
-
-
Run Docker Compose with the build option
To build the current CB-Tumblebug source code into a container image and run it along with the other containers, use the following command:
cd ~/go/src/github.com/cloud-barista/cb-tumblebug sudo DOCKER_BUILDKIT=1 docker compose up --build
This command will automatically build the CB-Tumblebug from the local source code and start it within a Docker container, along with any other necessary services as defined in the
docker-compose.yml
file.DOCKER_BUILDKIT=1
setting is used to speed up the build by using the go build cache technique.
-
Build the Golang source code using the Makefile
cd ~/go/src/github.com/cloud-barista/cb-tumblebug/src make
All dependencies will be downloaded automatically by Go.
The initial build will take some time, but subsequent builds will be faster by the Go build cache.
Note To update the Swagger API documentation, run
make swag
- API documentation file will be generated at
cb-tumblebug/src/api/rest/docs/swagger.yaml
- API documentation can be viewed in a web browser at http://localhost:1323/tumblebug/api (provided when CB-TB is running)
- Detailed information on how to update the API
- API documentation file will be generated at
-
Set environment variables required to run CB-TB (in another tab)
- Check and configure the contents of
cb-tumblebug/conf/setup.env
(CB-TB environment variables, modify as needed)- Apply the environment variables to the system
cd ~/go/src/github.com/cloud-barista/cb-tumblebug source conf/setup.env
- (Optional) Automatically set the TB_SELF_ENDPOINT environment variable (an externally accessible address) using a script if needed
- This is necessary if you want to access and control the Swagger API Dashboard from outside when CB-TB is running
cd ~/go/src/github.com/cloud-barista/cb-tumblebug source ./scripts/setPublicIP.sh
- Apply the environment variables to the system
- Check and configure the contents of
-
Execute the built cb-tumblebug binary by using
make run
cd ~/go/src/github.com/cloud-barista/cb-tumblebug/src make run
CB-TB welcomes improvements from both new and experienced contributors!
Check out CONTRIBUTING.
Thanks goes to these wonderful people (emoji key):
For Tasks:
Click tags to check more tools for each tasksFor Jobs:
Alternative AI tools for cb-tumblebug
Similar Open Source Tools
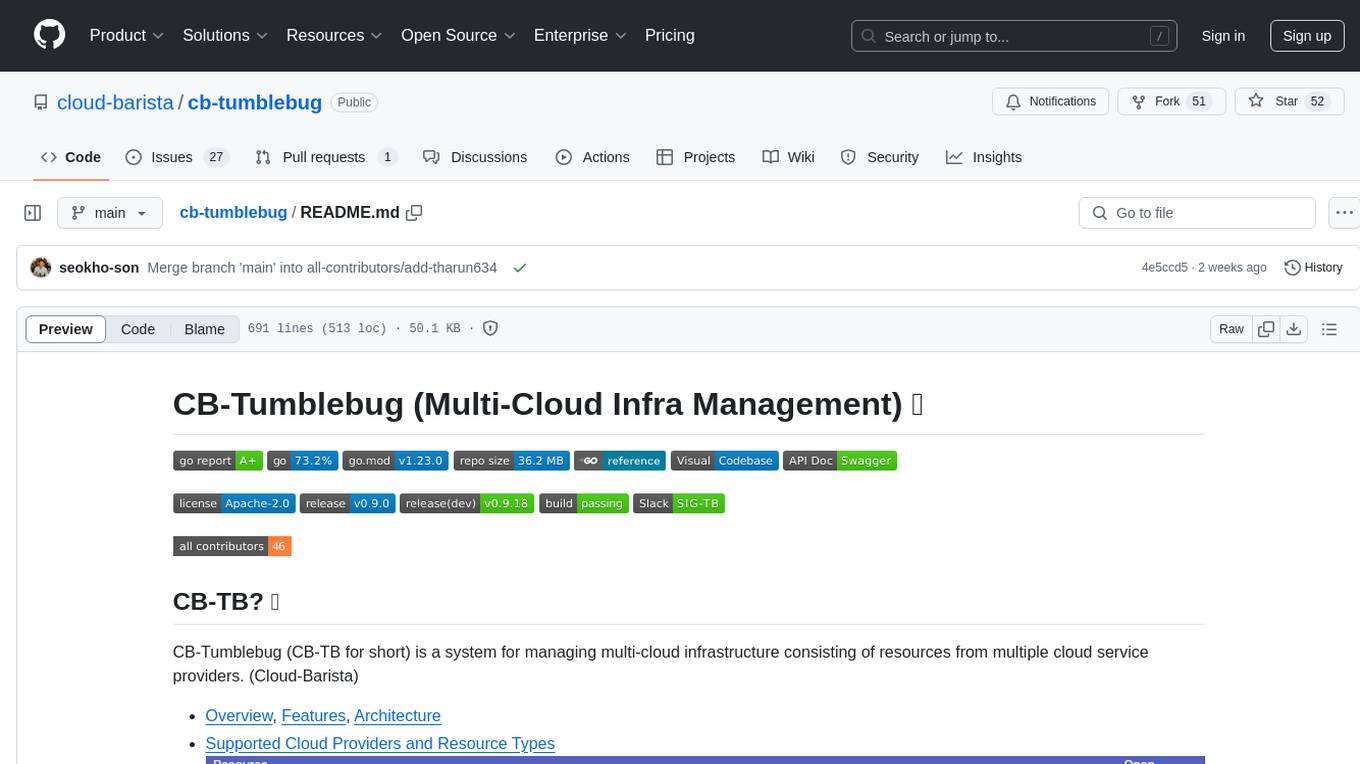
cb-tumblebug
CB-Tumblebug (CB-TB) is a system for managing multi-cloud infrastructure consisting of resources from multiple cloud service providers. It provides an overview, features, and architecture. The tool supports various cloud providers and resource types, with ongoing development and localization efforts. Users can deploy a multi-cloud infra with GPUs, enjoy multiple LLMs in parallel, and utilize LLM-related scripts. The tool requires Linux, Docker, Docker Compose, and Golang for building the source. Users can run CB-TB with Docker Compose or from the Makefile, set up prerequisites, contribute to the project, and view a list of contributors. The tool is licensed under an open-source license.
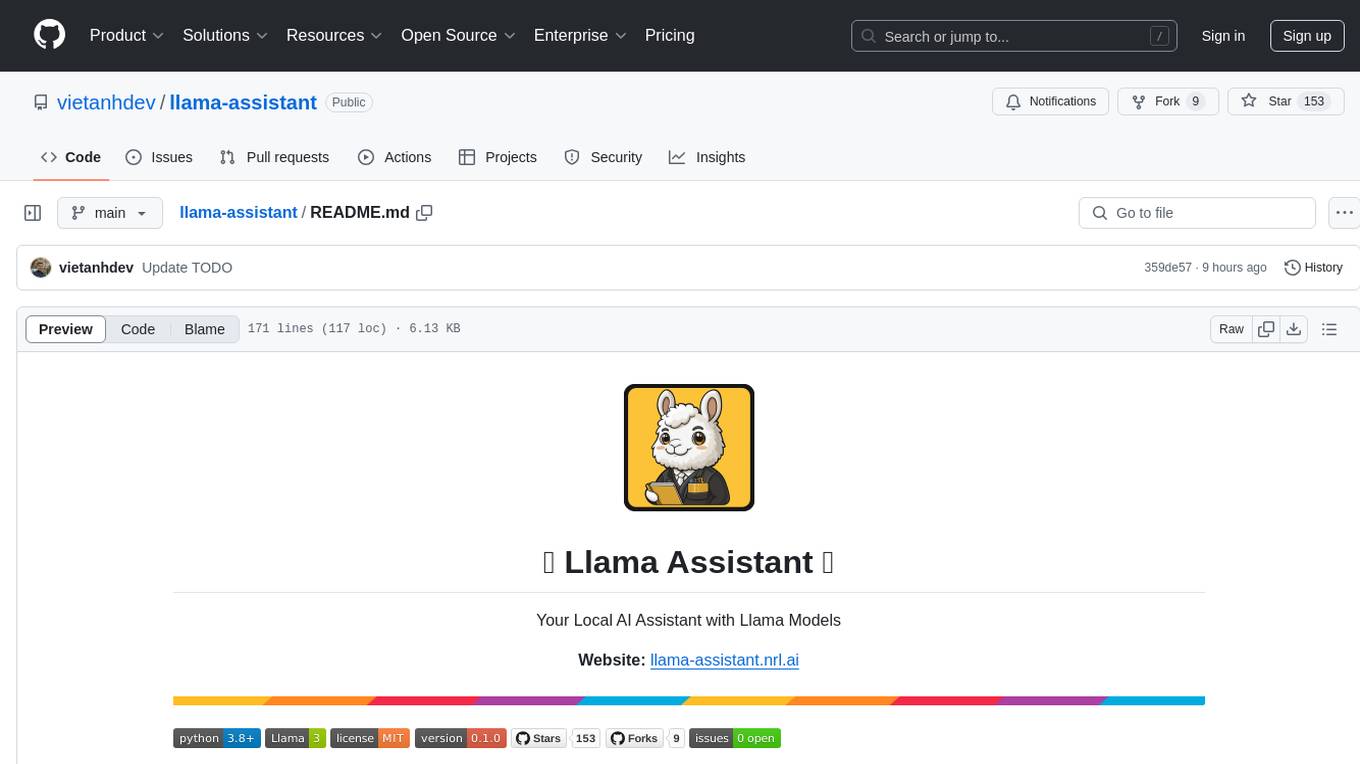
llama-assistant
Llama Assistant is an AI-powered assistant that helps with daily tasks, such as voice recognition, natural language processing, summarizing text, rephrasing sentences, answering questions, and more. It runs offline on your local machine, ensuring privacy by not sending data to external servers. The project is a work in progress with regular feature additions.
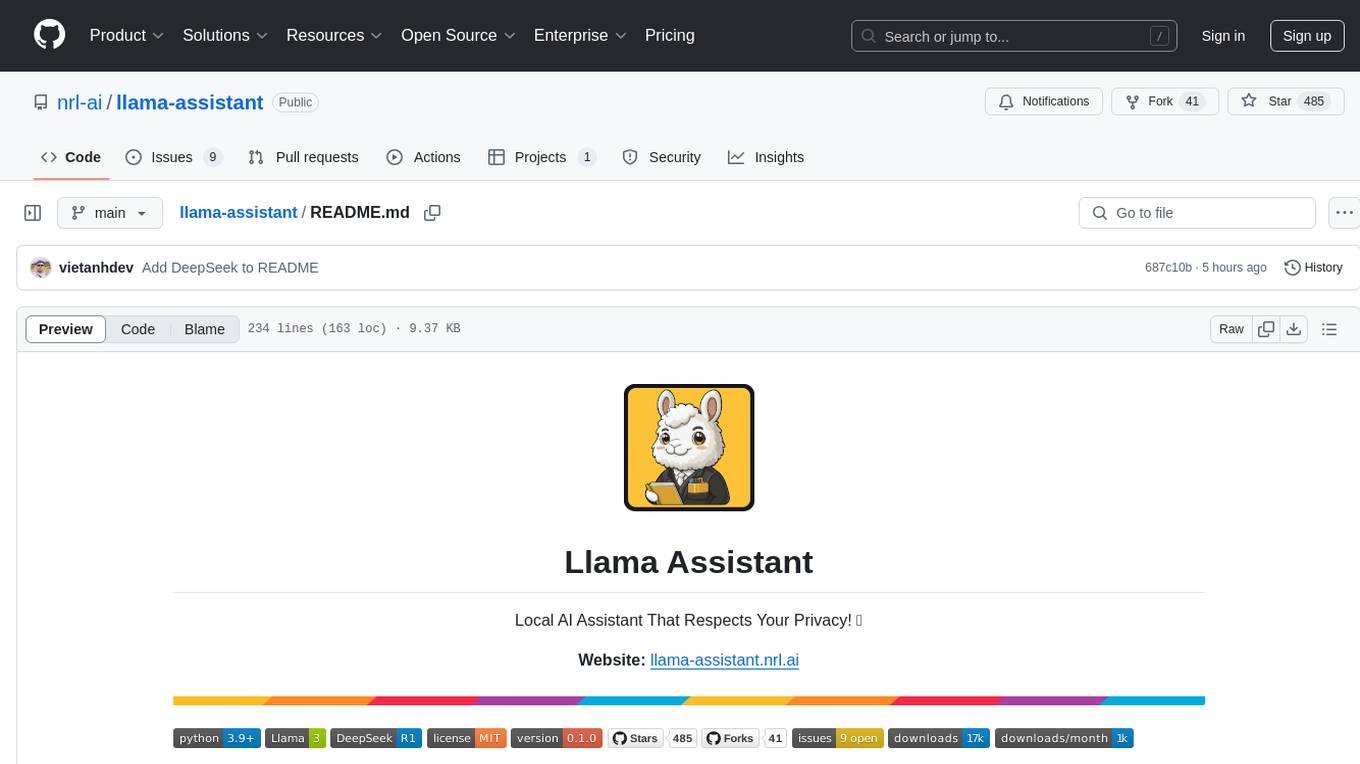
llama-assistant
Llama Assistant is a local AI assistant that respects your privacy. It is an AI-powered assistant that can recognize your voice, process natural language, and perform various actions based on your commands. It can help with tasks like summarizing text, rephrasing sentences, answering questions, writing emails, and more. The assistant runs offline on your local machine, ensuring privacy by not sending data to external servers. It supports voice recognition, natural language processing, and customizable UI with adjustable transparency. The project is a work in progress with new features being added regularly.
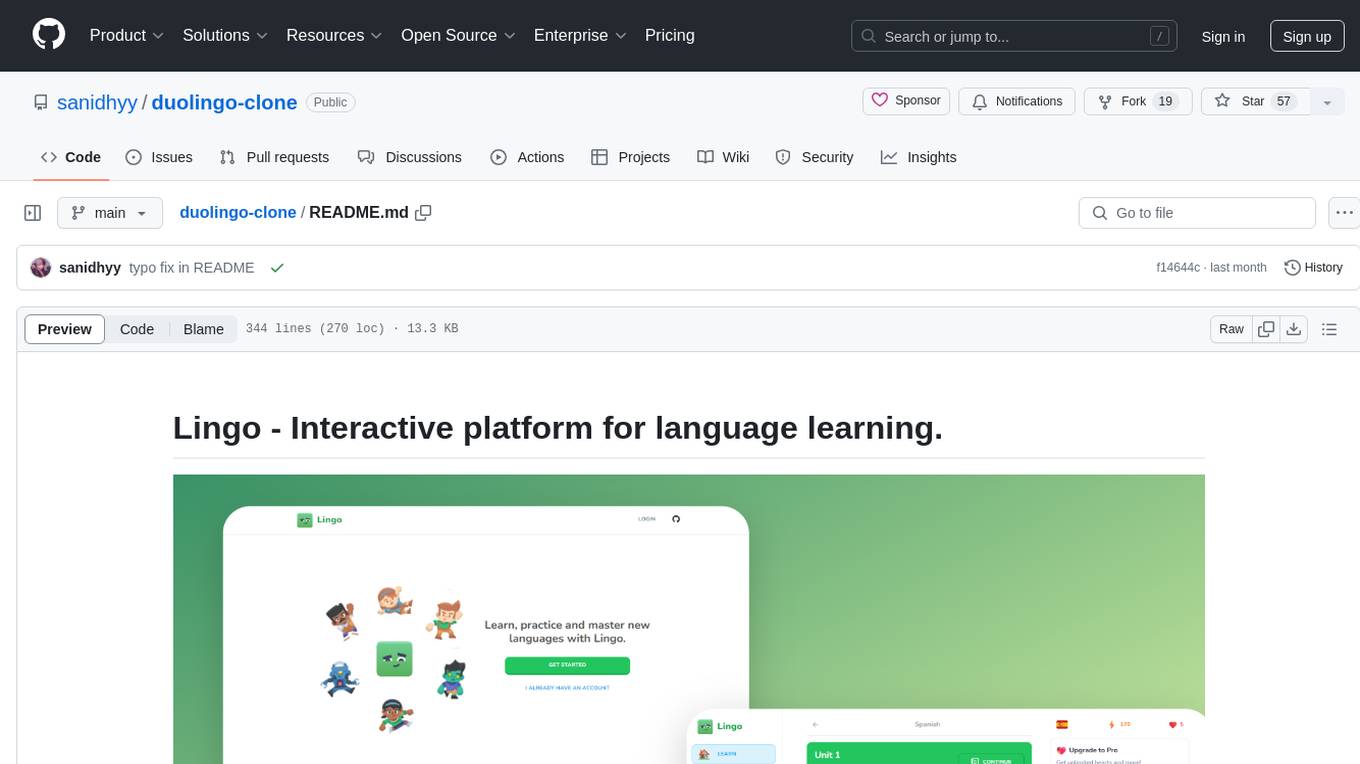
duolingo-clone
Lingo is an interactive platform for language learning that provides a modern UI/UX experience. It offers features like courses, quests, and a shop for users to engage with. The tech stack includes React JS, Next JS, Typescript, Tailwind CSS, Vercel, and Postgresql. Users can contribute to the project by submitting changes via pull requests. The platform utilizes resources from CodeWithAntonio, Kenney Assets, Freesound, Elevenlabs AI, and Flagpack. Key dependencies include @clerk/nextjs, @neondatabase/serverless, @radix-ui/react-avatar, and more. Users can follow the project creator on GitHub and Twitter, as well as subscribe to their YouTube channel for updates. To learn more about Next.js, users can refer to the Next.js documentation and interactive tutorial.
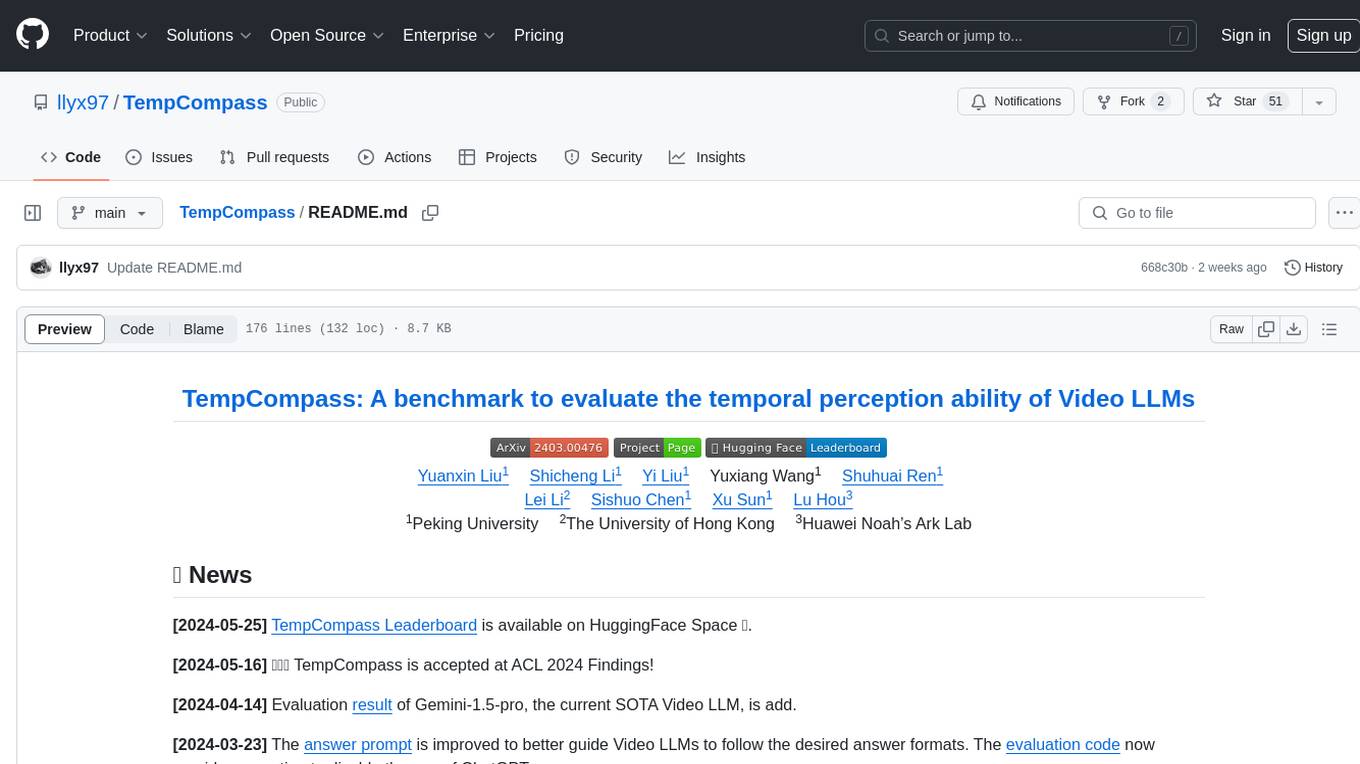
TempCompass
TempCompass is a benchmark designed to evaluate the temporal perception ability of Video LLMs. It encompasses a diverse set of temporal aspects and task formats to comprehensively assess the capability of Video LLMs in understanding videos. The benchmark includes conflicting videos to prevent models from relying on single-frame bias and language priors. Users can clone the repository, install required packages, prepare data, run inference using examples like Video-LLaVA and Gemini, and evaluate the performance of their models across different tasks such as Multi-Choice QA, Yes/No QA, Caption Matching, and Caption Generation.
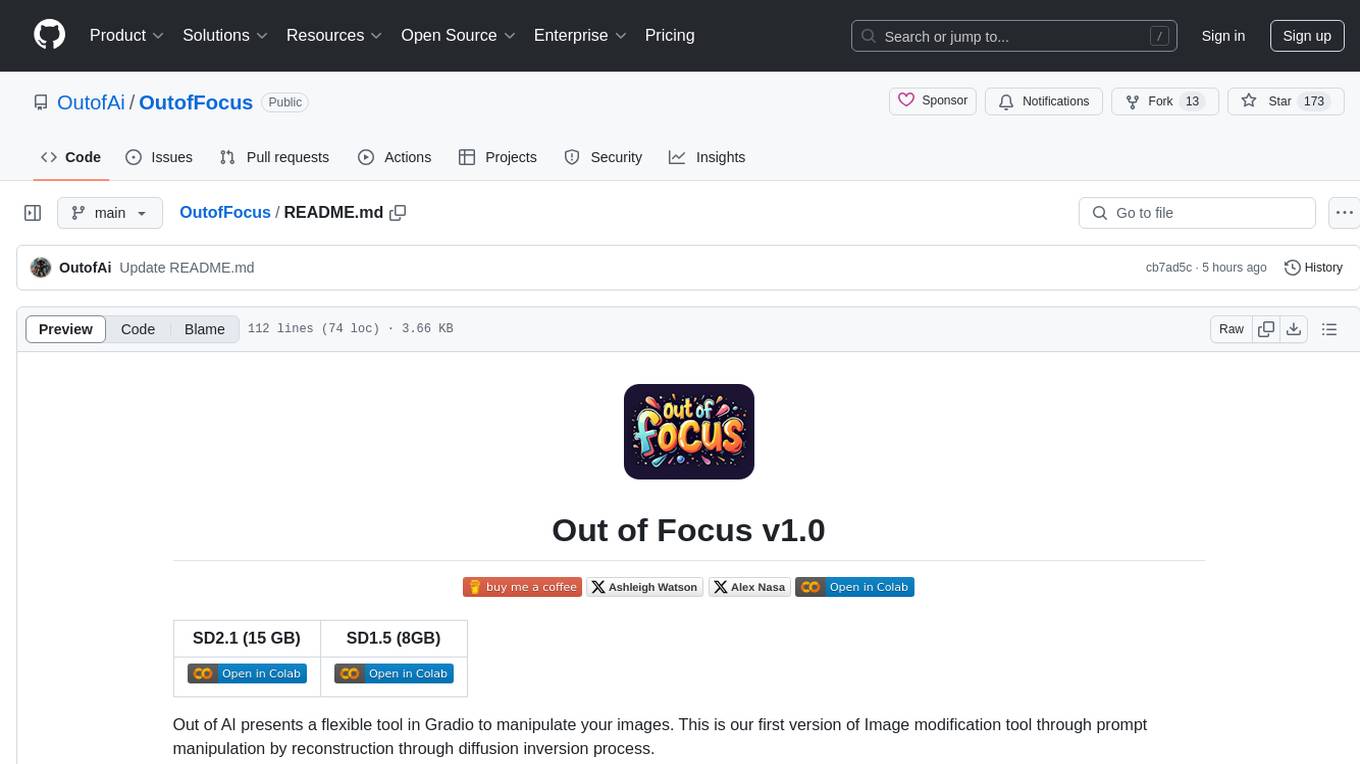
OutofFocus
Out of Focus v1.0 is a flexible tool in Gradio for image manipulation through prompt manipulation by reconstruction via diffusion inversion process. Users can modify images using this tool, which is the first version of the Image modification tool by Out of AI.
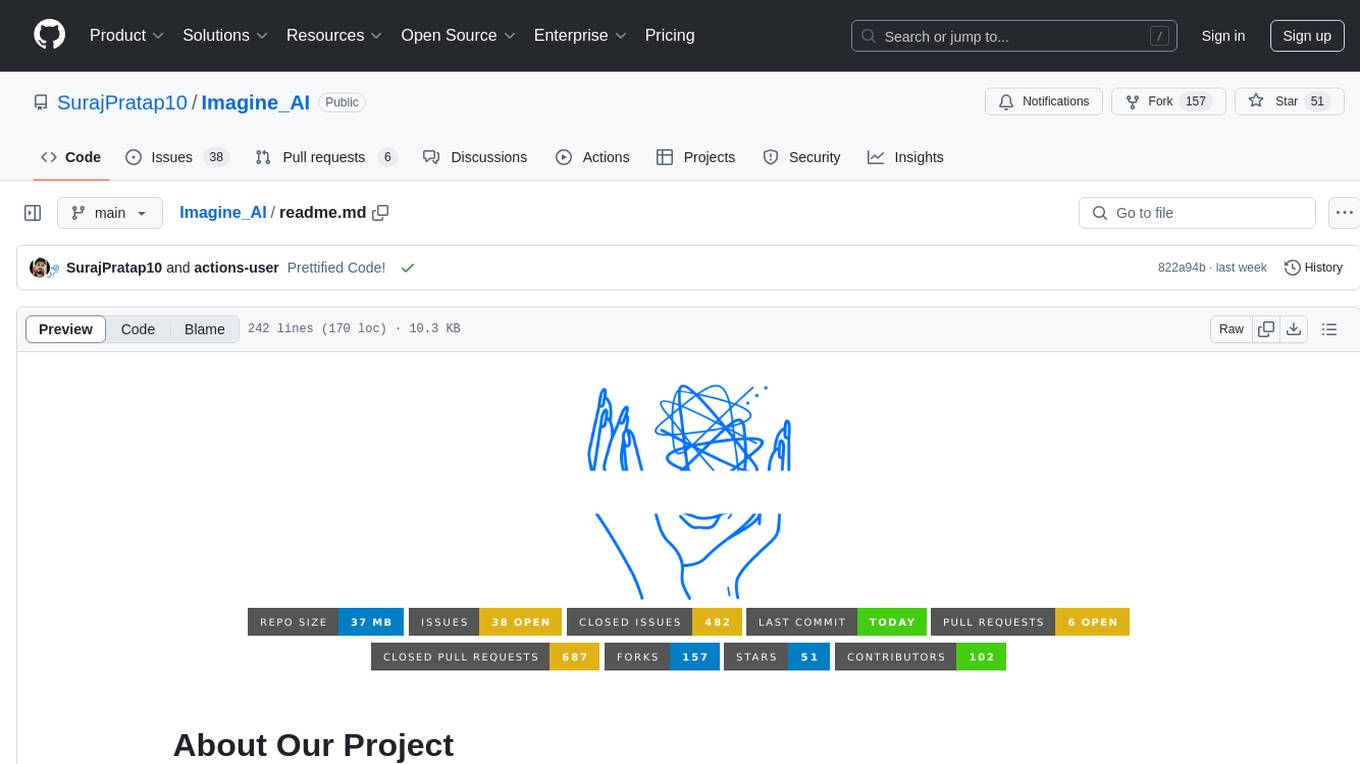
Imagine_AI
IMAGINE - AI is a groundbreaking image generator tool that leverages the power of OpenAI's DALL-E 2 API library to create extraordinary visuals. Developed using Node.js and Express, this tool offers a transformative way to unleash artistic creativity and imagination by generating unique and captivating images through simple prompts or keywords.
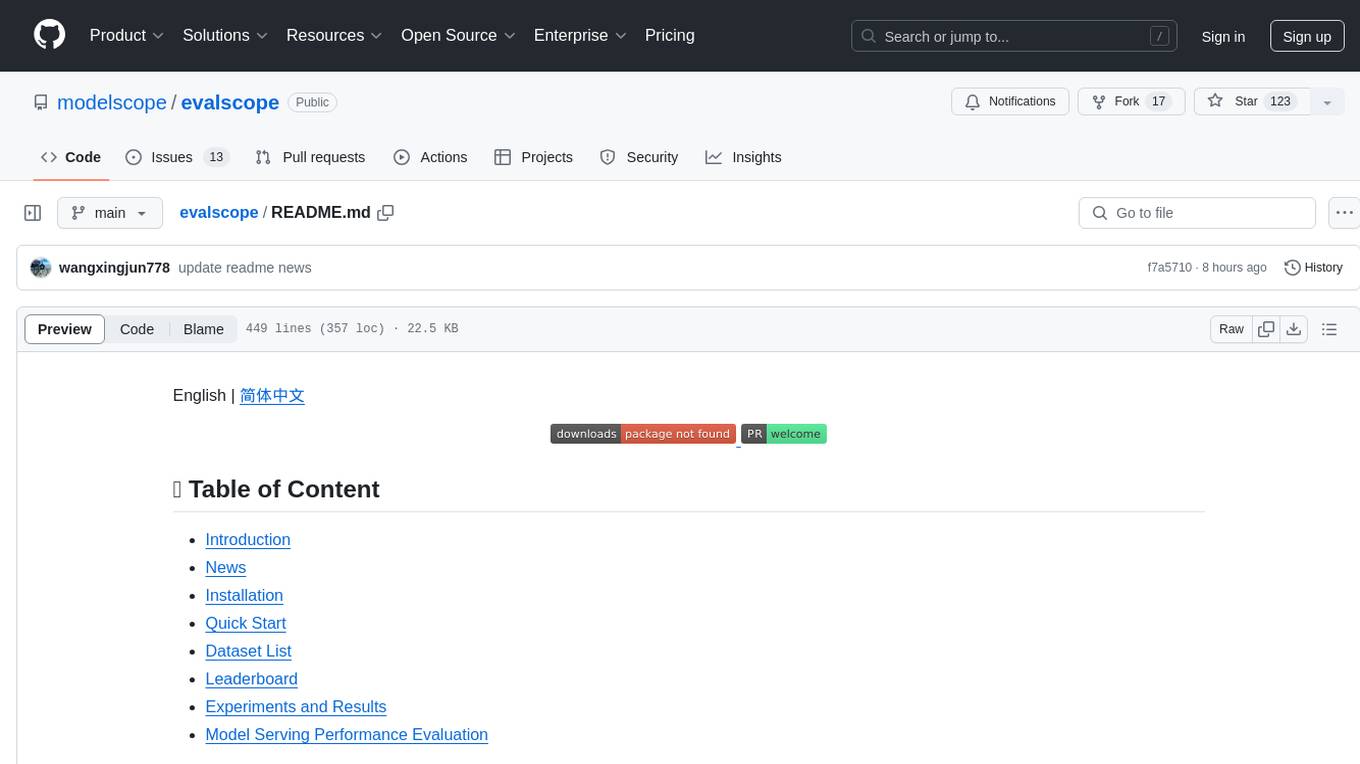
evalscope
Eval-Scope is a framework designed to support the evaluation of large language models (LLMs) by providing pre-configured benchmark datasets, common evaluation metrics, model integration, automatic evaluation for objective questions, complex task evaluation using expert models, reports generation, visualization tools, and model inference performance evaluation. It is lightweight, easy to customize, supports new dataset integration, model hosting on ModelScope, deployment of locally hosted models, and rich evaluation metrics. Eval-Scope also supports various evaluation modes like single mode, pairwise-baseline mode, and pairwise (all) mode, making it suitable for assessing and improving LLMs.
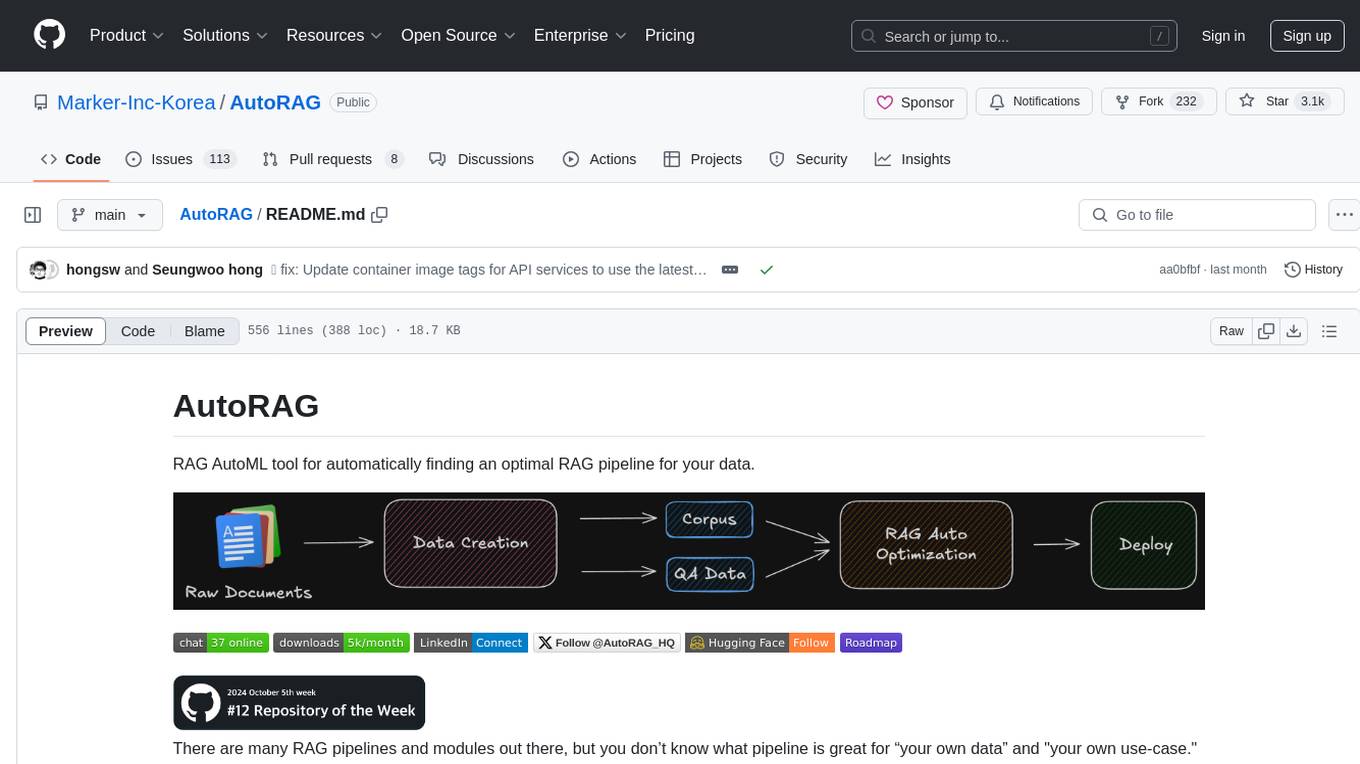
AutoRAG
AutoRAG is an AutoML tool designed to automatically find the optimal RAG pipeline for your data. It simplifies the process of evaluating various RAG modules to identify the best pipeline for your specific use-case. The tool supports easy evaluation of different module combinations, making it efficient to find the most suitable RAG pipeline for your needs. AutoRAG also offers a cloud beta version to assist users in running and optimizing the tool, along with building RAG evaluation datasets for a starting price of $9.99 per optimization.
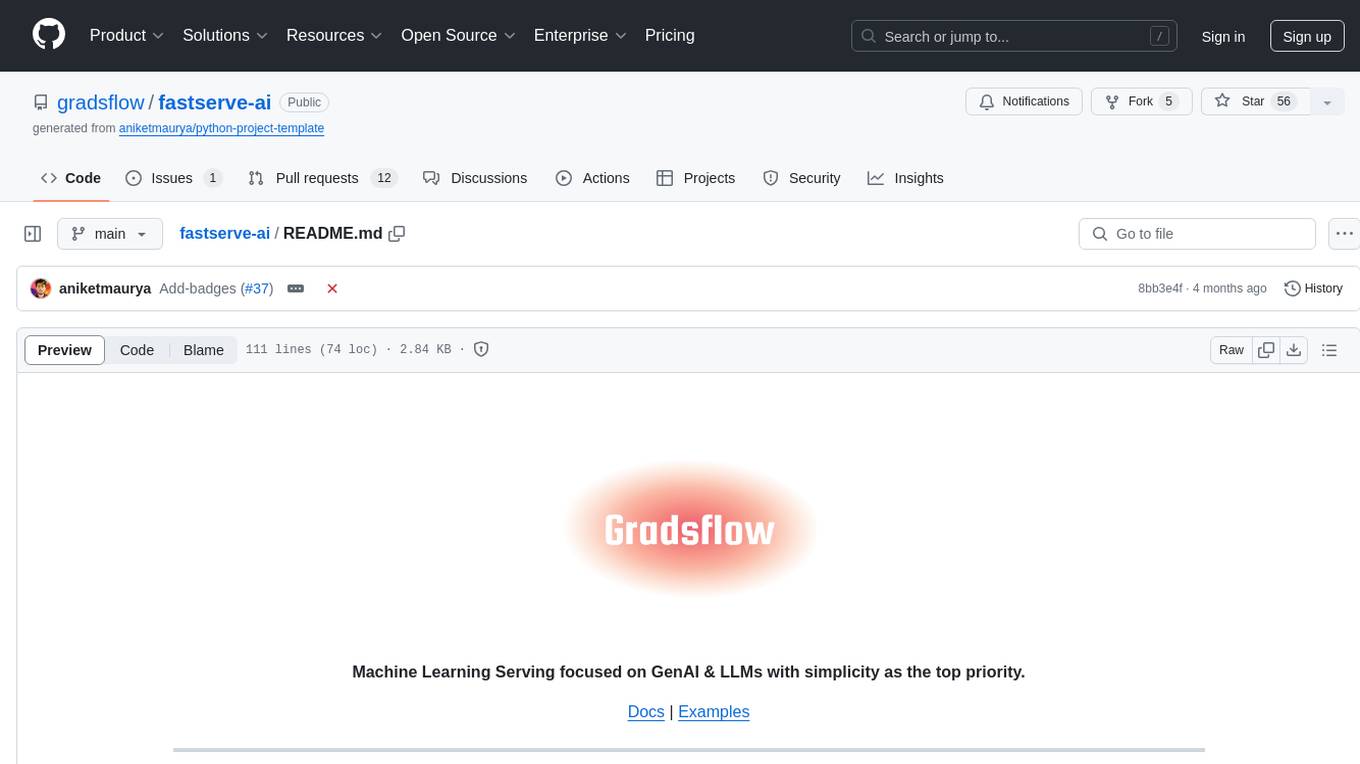
fastserve-ai
FastServe-AI is a machine learning serving tool focused on GenAI & LLMs with simplicity as the top priority. It allows users to easily serve custom models by implementing the 'handle' method for 'FastServe'. The tool provides a FastAPI server for custom models and can be deployed using Lightning AI Studio. Users can install FastServe-AI via pip and run it to serve their own GPT-like LLM models in minutes.
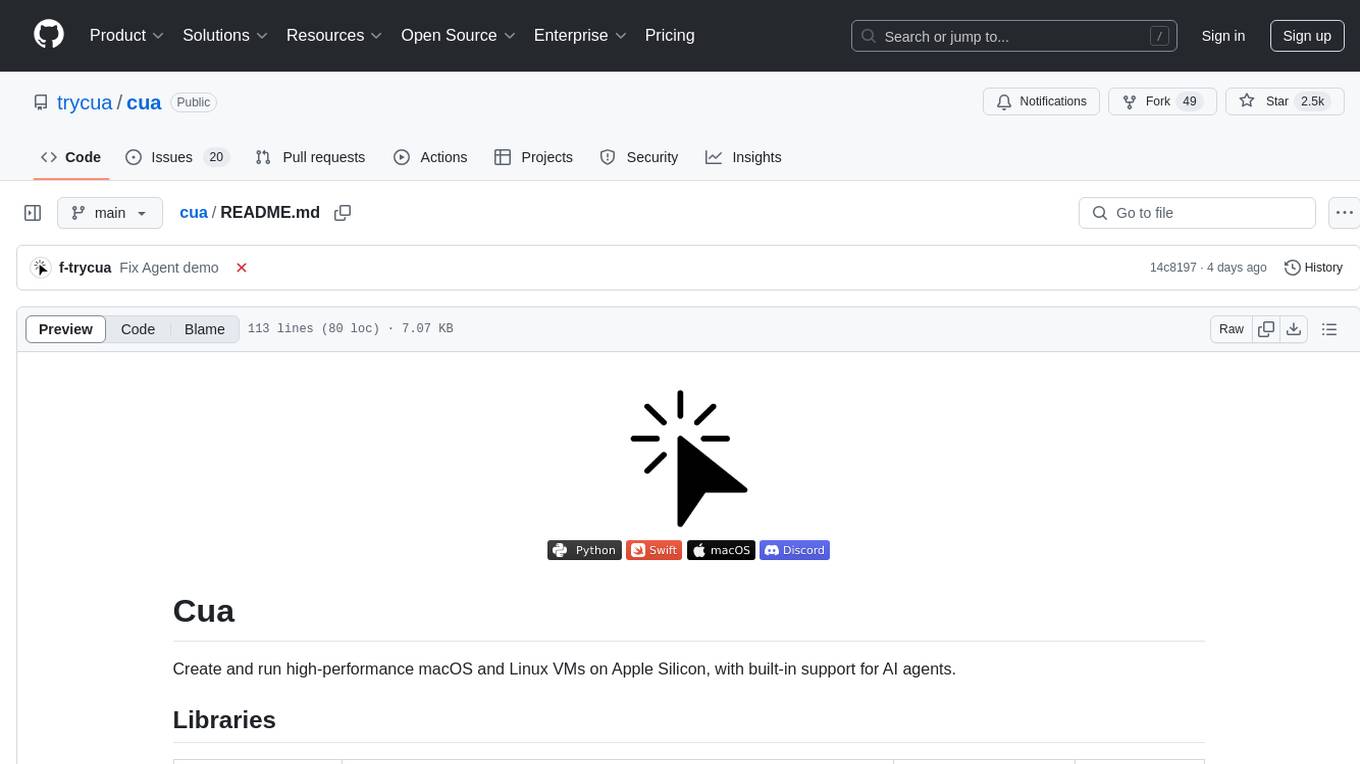
cua
Cua is a tool for creating and running high-performance macOS and Linux virtual machines on Apple Silicon, with built-in support for AI agents. It provides libraries like Lume for running VMs with near-native performance, Computer for interacting with sandboxes, and Agent for running agentic workflows. Users can refer to the documentation for onboarding, explore demos showcasing AI-Gradio and GitHub issue fixing, and utilize accessory libraries like Core, PyLume, Computer Server, and SOM. Contributions are welcome, and the tool is open-sourced under the MIT License.
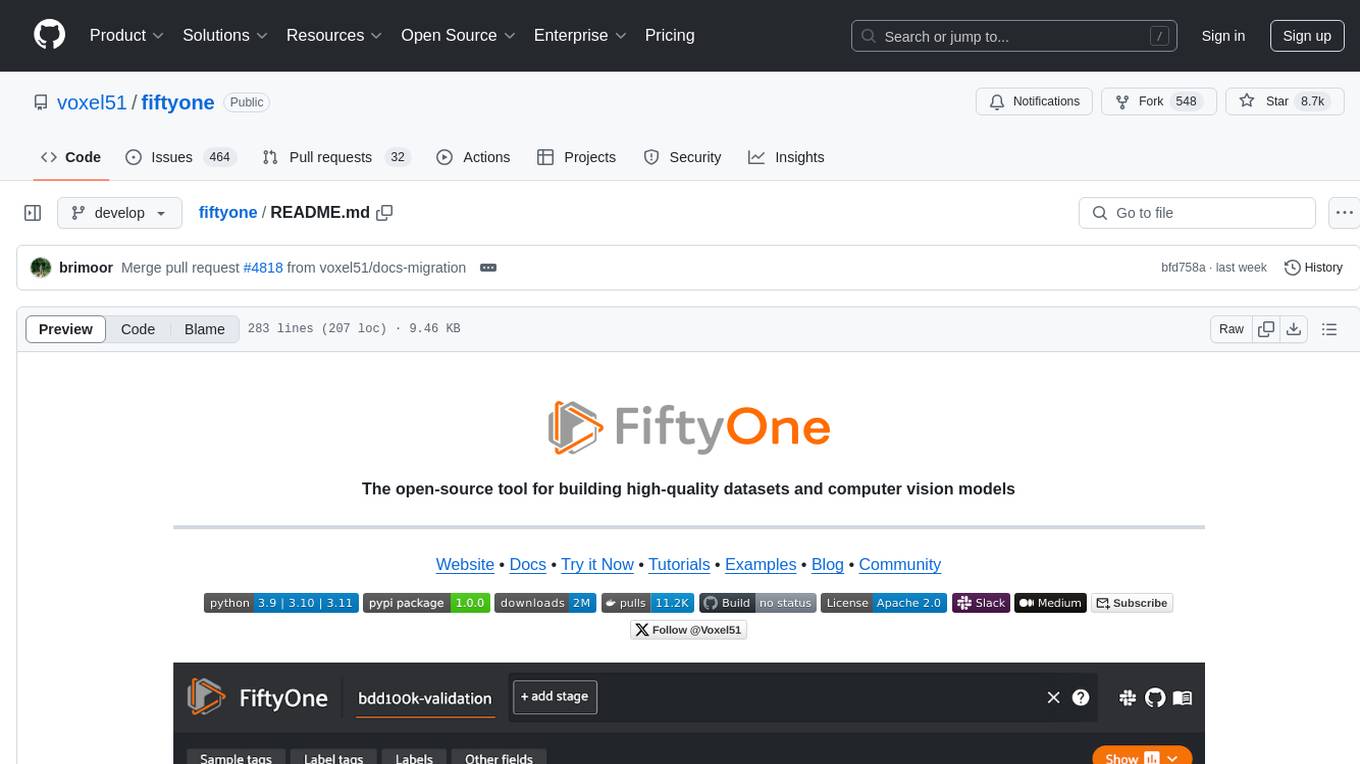
fiftyone
FiftyOne is an open-source tool designed for building high-quality datasets and computer vision models. It supercharges machine learning workflows by enabling users to visualize datasets, interpret models faster, and improve efficiency. With FiftyOne, users can explore scenarios, identify failure modes, visualize complex labels, evaluate models, find annotation mistakes, and much more. The tool aims to streamline the process of improving machine learning models by providing a comprehensive set of features for data analysis and model interpretation.
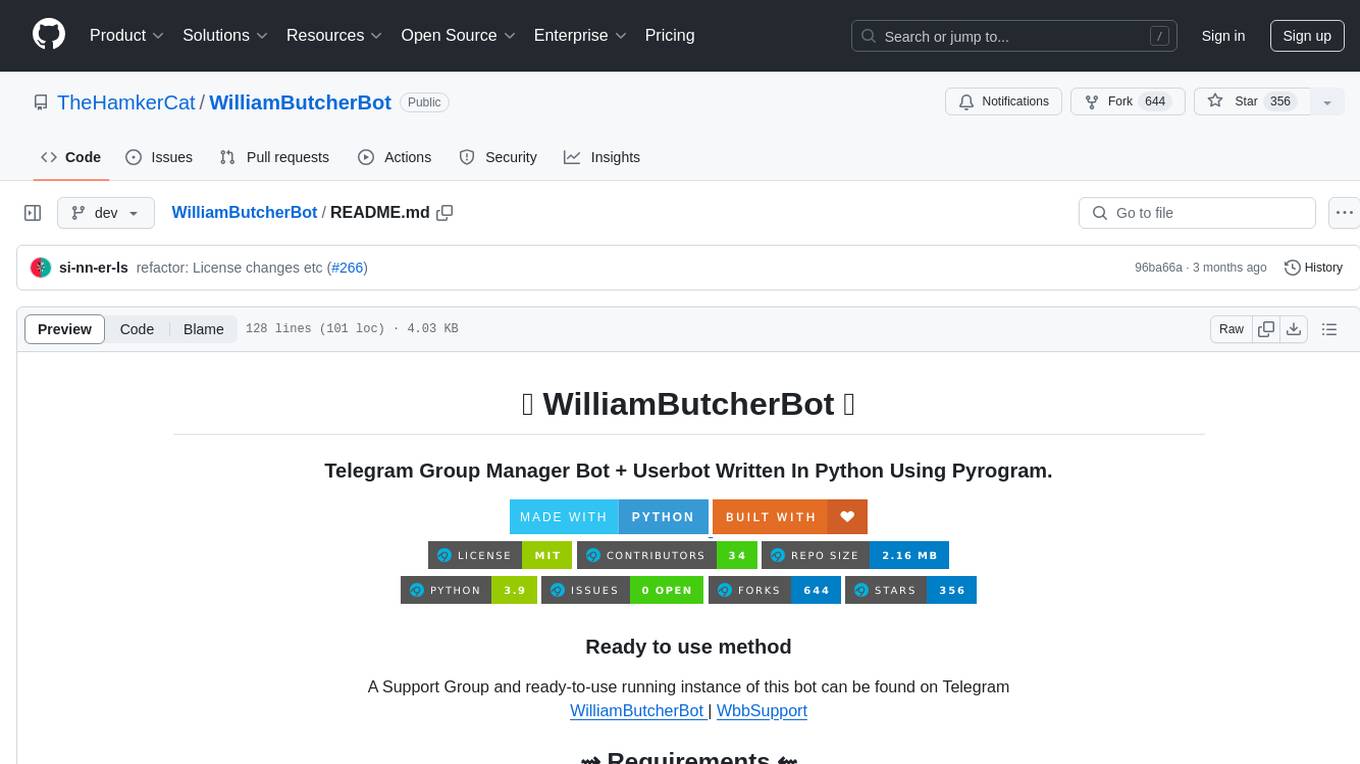
WilliamButcherBot
WilliamButcherBot is a Telegram Group Manager Bot and Userbot written in Python using Pyrogram. It provides features for managing Telegram groups and users, with ready-to-use methods available. The bot requires Python 3.9, Telegram API Key, Telegram Bot Token, and MongoDB URI. Users can install it locally or on a VPS, run it directly, generate Pyrogram session for Heroku, or use Docker for deployment. Additionally, users can write new modules to extend the bot's functionality by adding them to the wbb/modules/ directory.
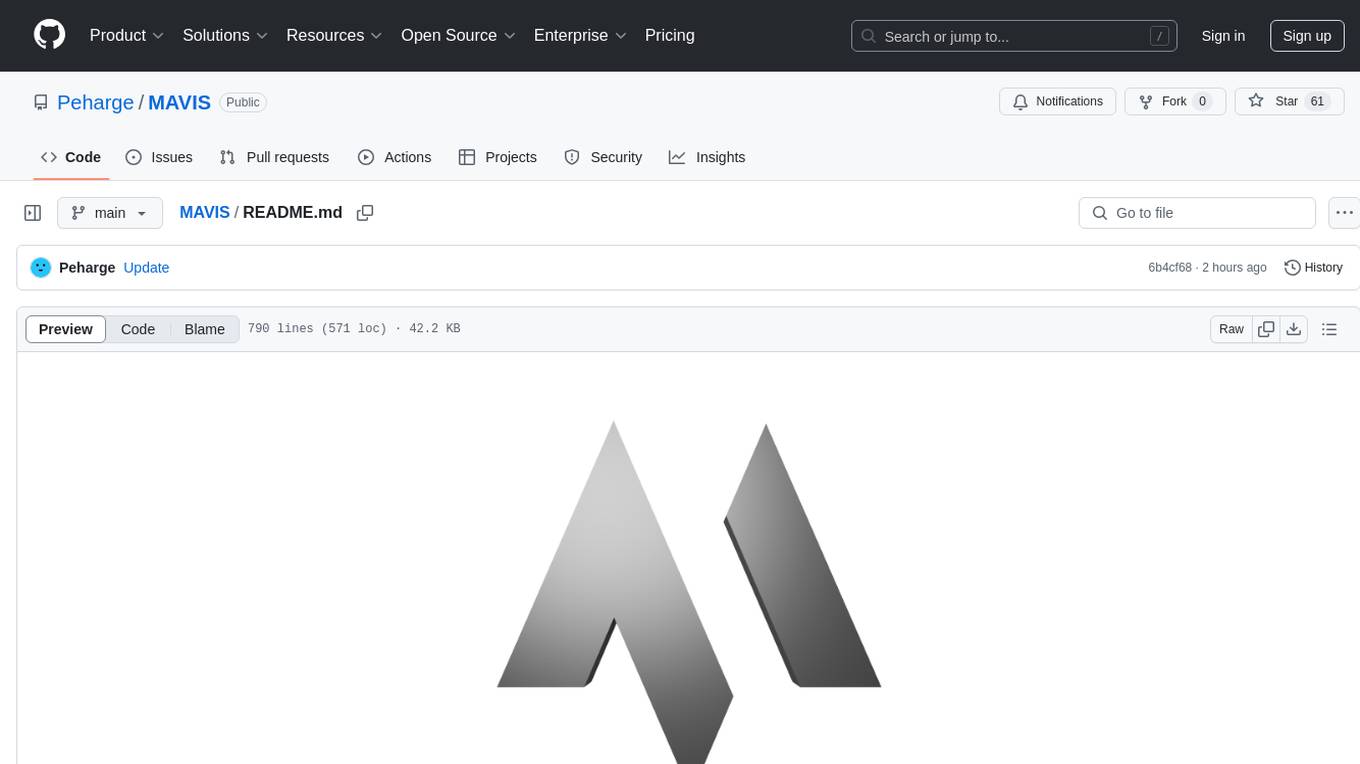
MAVIS
MAVIS (Math Visual Intelligent System) is an AI-driven application that allows users to analyze visual data such as images and generate interactive answers based on them. It can perform complex mathematical calculations, solve programming tasks, and create professional graphics. MAVIS supports Python for coding and frameworks like Matplotlib, Plotly, Seaborn, Altair, NumPy, Math, SymPy, and Pandas. It is designed to make projects more efficient and professional.
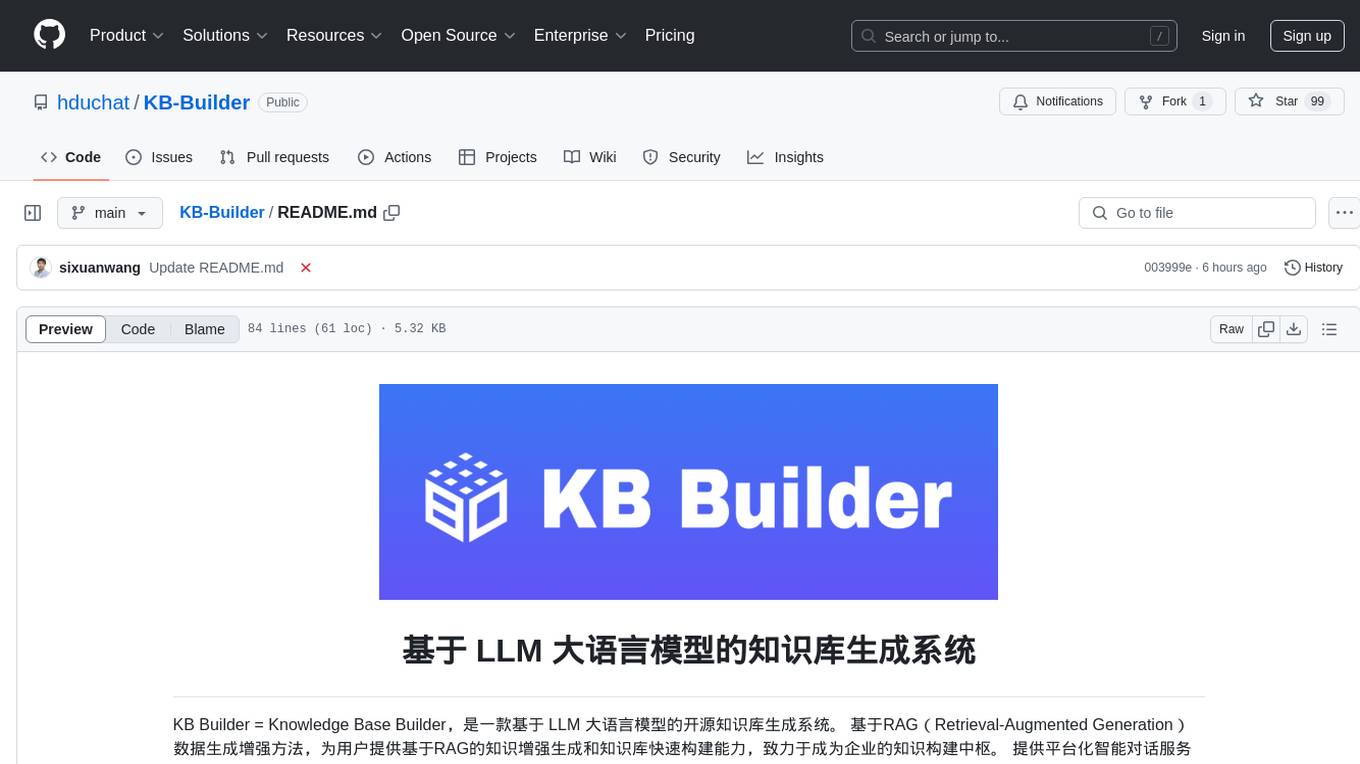
KB-Builder
KB Builder is an open-source knowledge base generation system based on the LLM large language model. It utilizes the RAG (Retrieval-Augmented Generation) data generation enhancement method to provide users with the ability to enhance knowledge generation and quickly build knowledge bases based on RAG. It aims to be the central hub for knowledge construction in enterprises, offering platform-based intelligent dialogue services and document knowledge base management functionality. Users can upload docx, pdf, txt, and md format documents and generate high-quality knowledge base question-answer pairs by invoking large models through the 'Parse Document' feature.
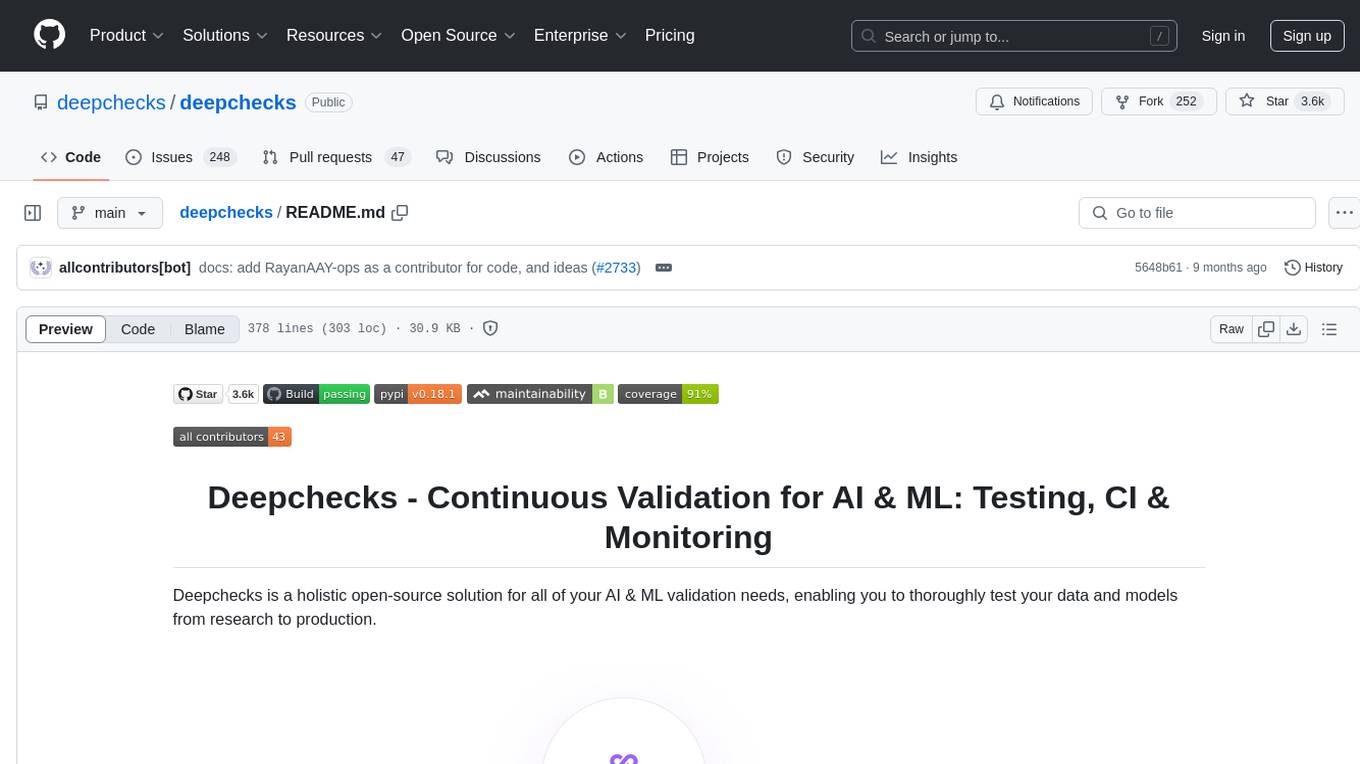
deepchecks
Deepchecks is a holistic open-source solution for AI & ML validation needs, enabling thorough testing of data and models from research to production. It includes components for testing, CI & testing management, and monitoring. Users can install and use Deepchecks for testing and monitoring their AI models, with customizable checks and suites for tabular, NLP, and computer vision data. The tool provides visual reports, pythonic/json output for processing, and a dynamic UI for collaboration and monitoring. Deepchecks is open source, with premium features available under a commercial license for monitoring components.
For similar tasks
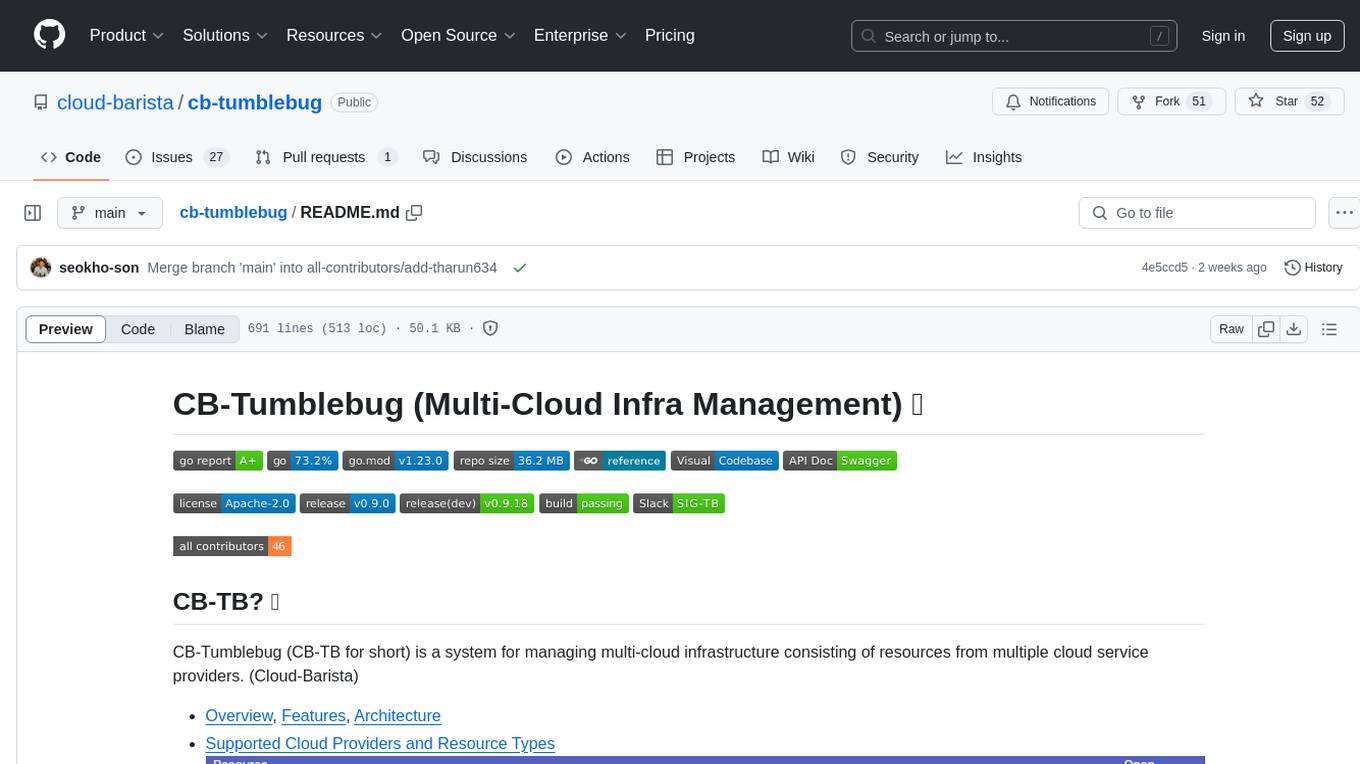
cb-tumblebug
CB-Tumblebug (CB-TB) is a system for managing multi-cloud infrastructure consisting of resources from multiple cloud service providers. It provides an overview, features, and architecture. The tool supports various cloud providers and resource types, with ongoing development and localization efforts. Users can deploy a multi-cloud infra with GPUs, enjoy multiple LLMs in parallel, and utilize LLM-related scripts. The tool requires Linux, Docker, Docker Compose, and Golang for building the source. Users can run CB-TB with Docker Compose or from the Makefile, set up prerequisites, contribute to the project, and view a list of contributors. The tool is licensed under an open-source license.
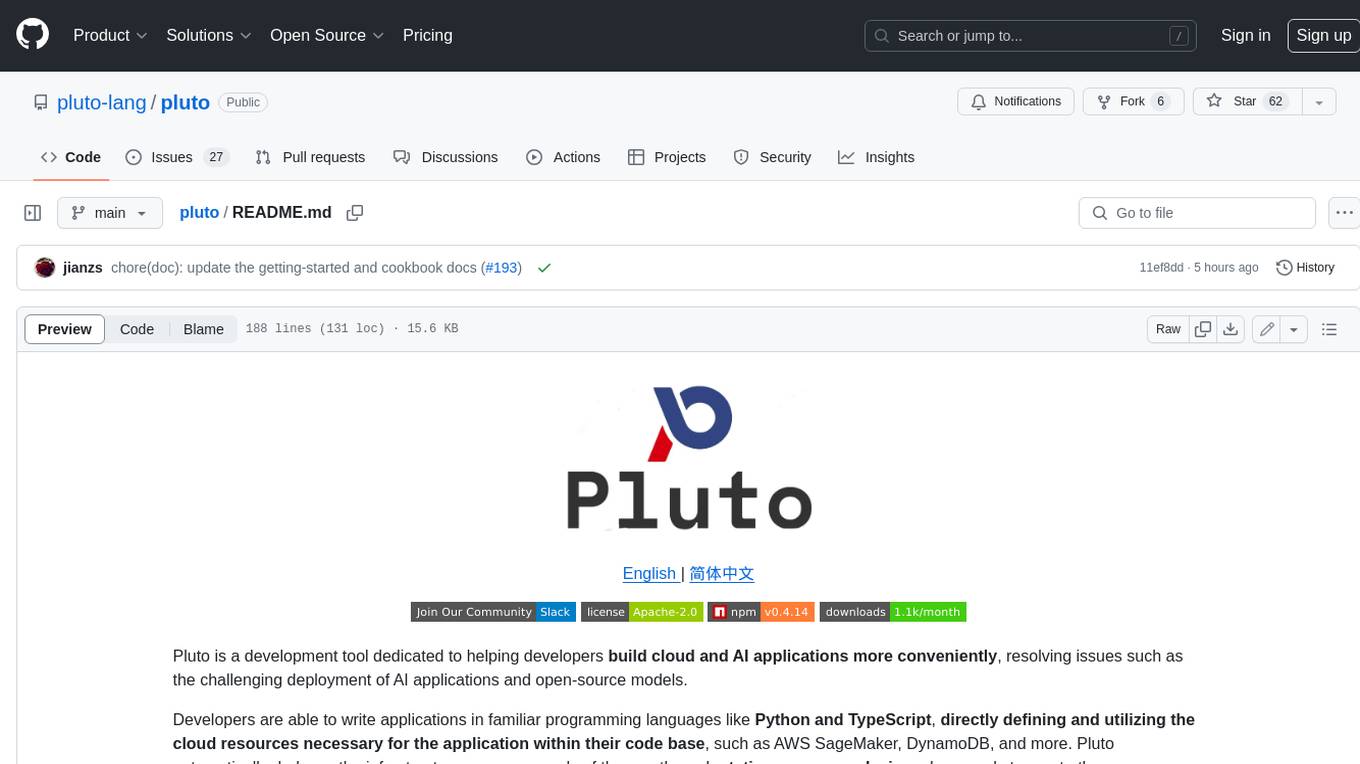
pluto
Pluto is a development tool dedicated to helping developers **build cloud and AI applications more conveniently** , resolving issues such as the challenging deployment of AI applications and open-source models. Developers are able to write applications in familiar programming languages like **Python and TypeScript** , **directly defining and utilizing the cloud resources necessary for the application within their code base** , such as AWS SageMaker, DynamoDB, and more. Pluto automatically deduces the infrastructure resource needs of the app through **static program analysis** and proceeds to create these resources on the specified cloud platform, **simplifying the resources creation and application deployment process**.
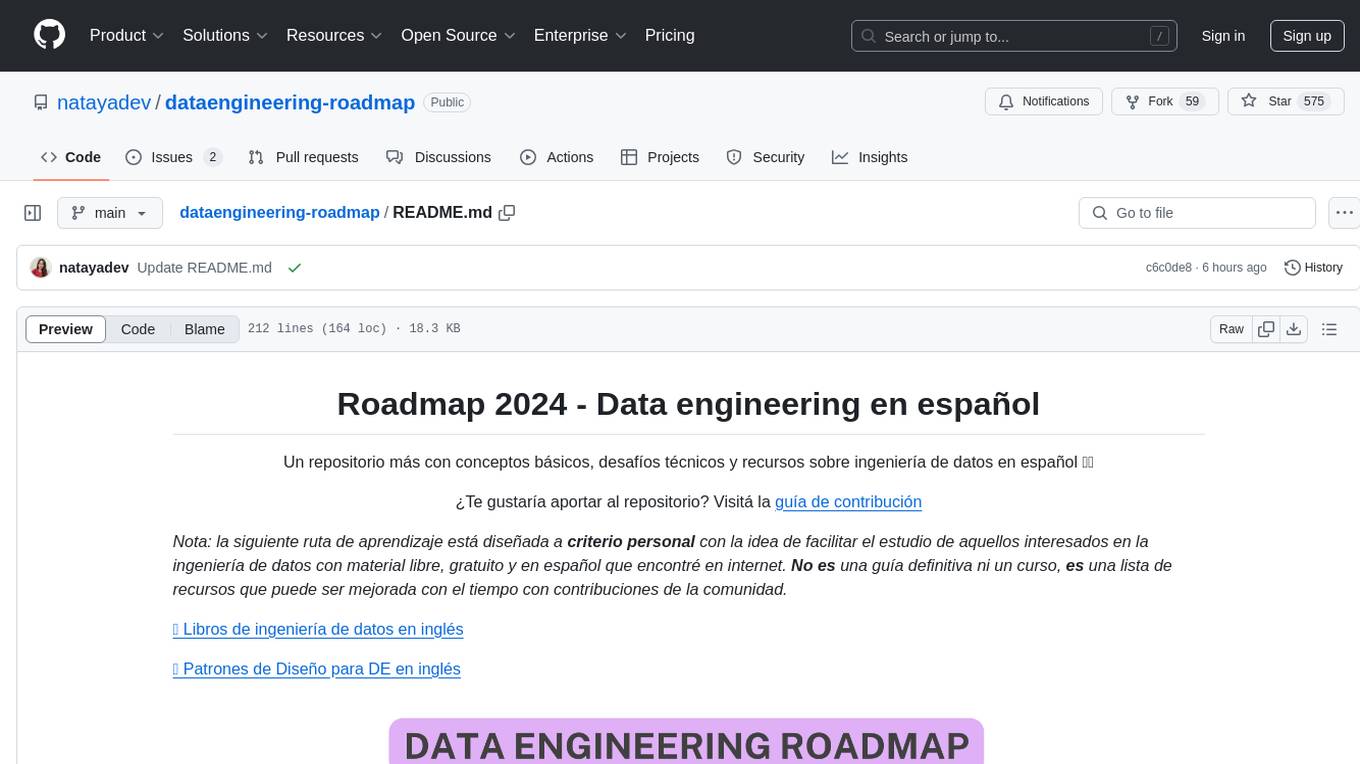
dataengineering-roadmap
A repository providing basic concepts, technical challenges, and resources on data engineering in Spanish. It is a curated list of free, Spanish-language materials found on the internet to facilitate the study of data engineering enthusiasts. The repository covers programming fundamentals, programming languages like Python, version control with Git, database fundamentals, SQL, design concepts, Big Data, analytics, cloud computing, data processing, and job search tips in the IT field.
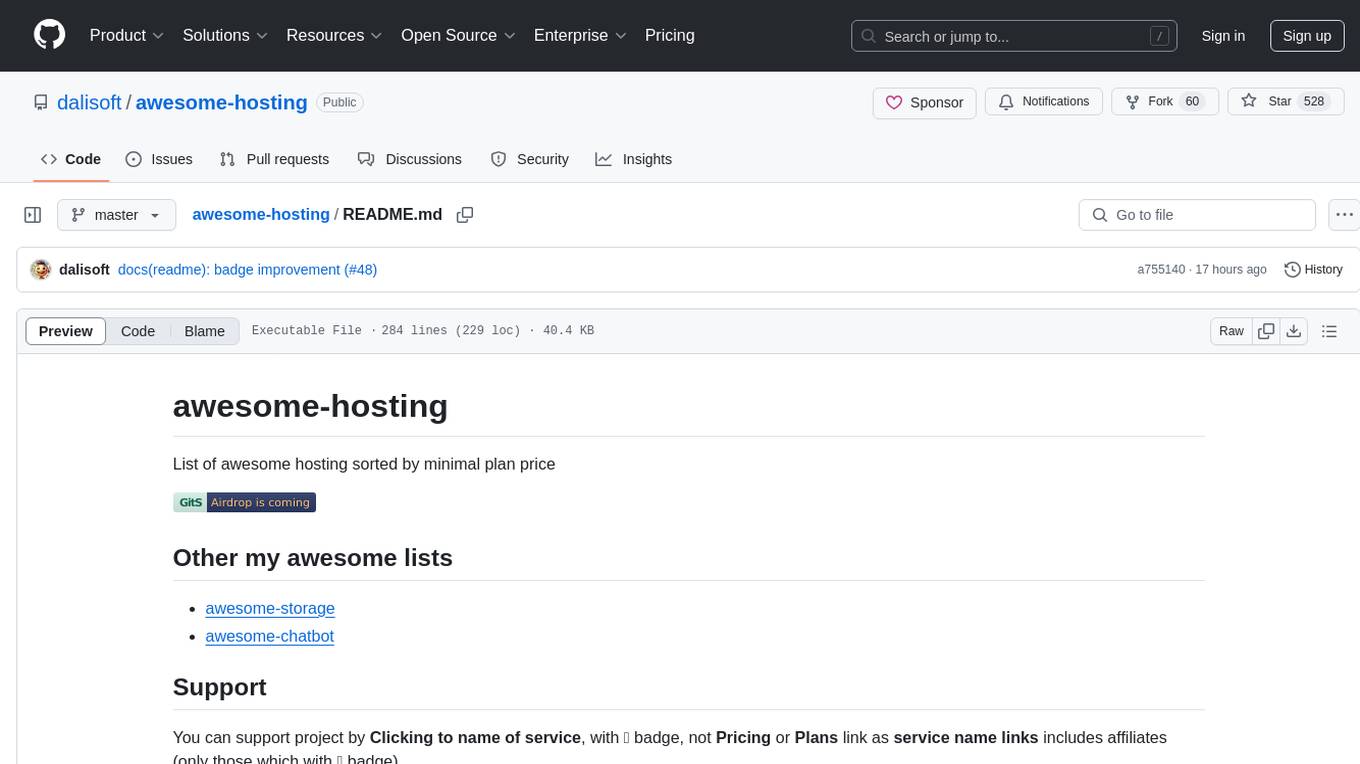
awesome-hosting
awesome-hosting is a curated list of hosting services sorted by minimal plan price. It includes various categories such as Web Services Platform, Backend-as-a-Service, Lambda, Node.js, Static site hosting, WordPress hosting, VPS providers, managed databases, GPU cloud services, and LLM/Inference API providers. Each category lists multiple service providers along with details on their minimal plan, trial options, free tier availability, open-source support, and specific features. The repository aims to help users find suitable hosting solutions based on their budget and requirements.
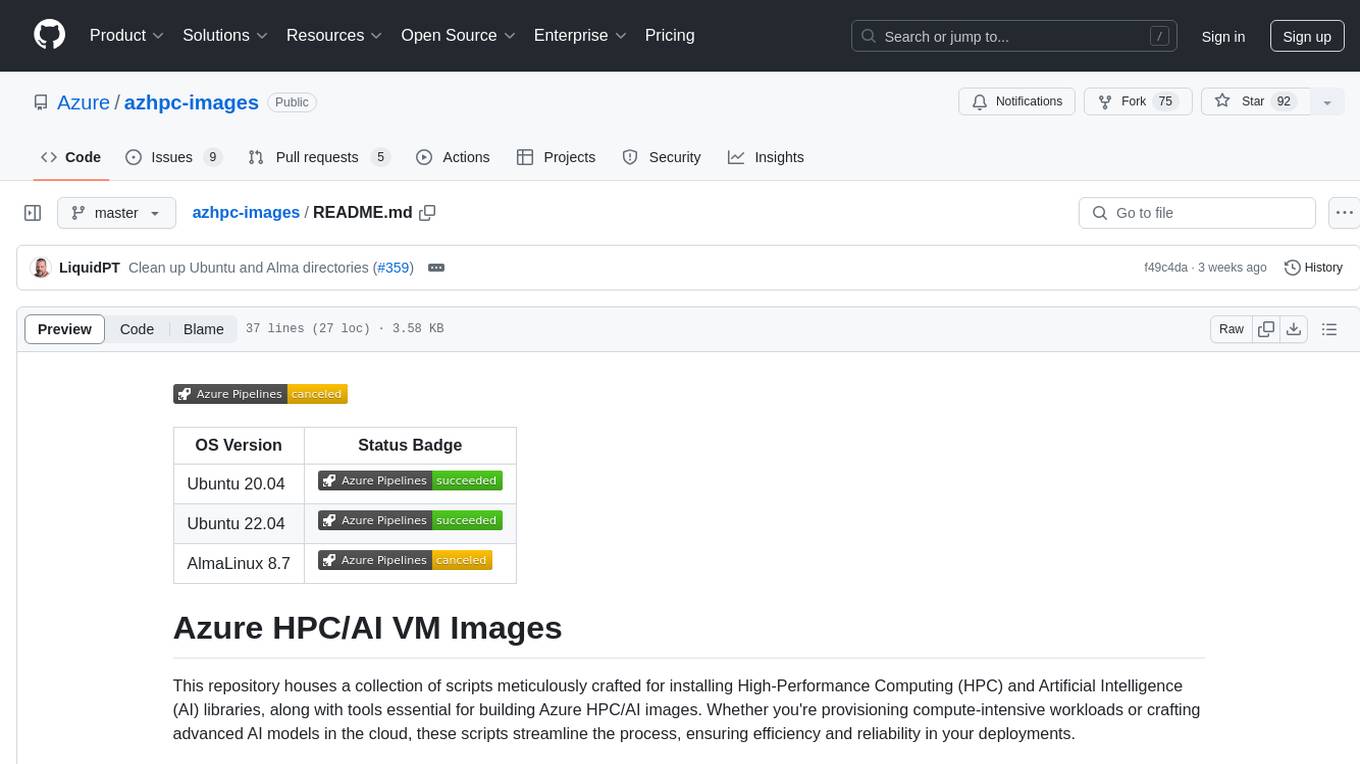
azhpc-images
This repository contains scripts for installing HPC and AI libraries and tools to build Azure HPC/AI images. It streamlines the process of provisioning compute-intensive workloads and crafting advanced AI models in the cloud, ensuring efficiency and reliability in deployments.
For similar jobs
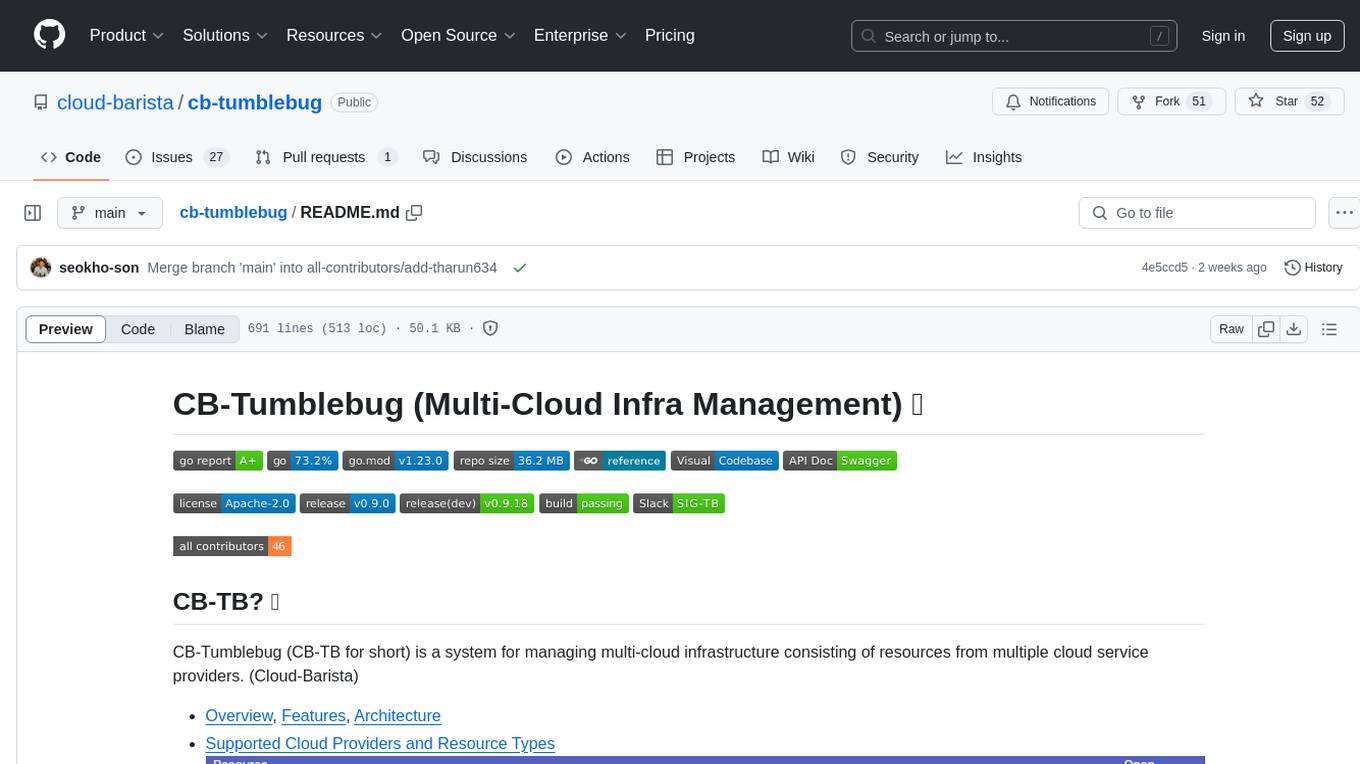
cb-tumblebug
CB-Tumblebug (CB-TB) is a system for managing multi-cloud infrastructure consisting of resources from multiple cloud service providers. It provides an overview, features, and architecture. The tool supports various cloud providers and resource types, with ongoing development and localization efforts. Users can deploy a multi-cloud infra with GPUs, enjoy multiple LLMs in parallel, and utilize LLM-related scripts. The tool requires Linux, Docker, Docker Compose, and Golang for building the source. Users can run CB-TB with Docker Compose or from the Makefile, set up prerequisites, contribute to the project, and view a list of contributors. The tool is licensed under an open-source license.
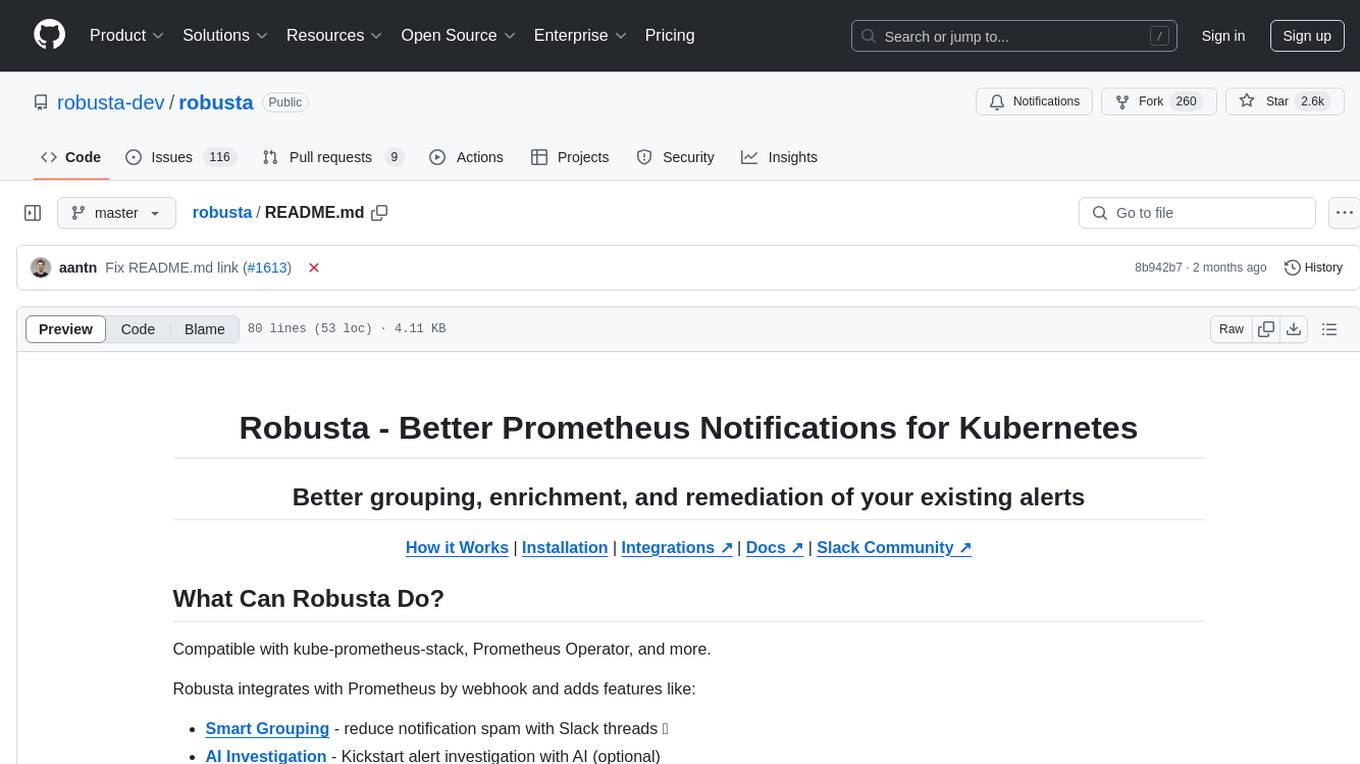
robusta
Robusta is a tool designed to enhance Prometheus notifications for Kubernetes environments. It offers features such as smart grouping to reduce notification spam, AI investigation for alert analysis, alert enrichment with additional data like pod logs, self-healing capabilities for defining auto-remediation rules, advanced routing options, problem detection without PromQL, change-tracking for Kubernetes resources, auto-resolve functionality, and integration with various external systems like Slack, Teams, and Jira. Users can utilize Robusta with or without Prometheus, and it can be installed alongside existing Prometheus setups or as part of an all-in-one Kubernetes observability stack.
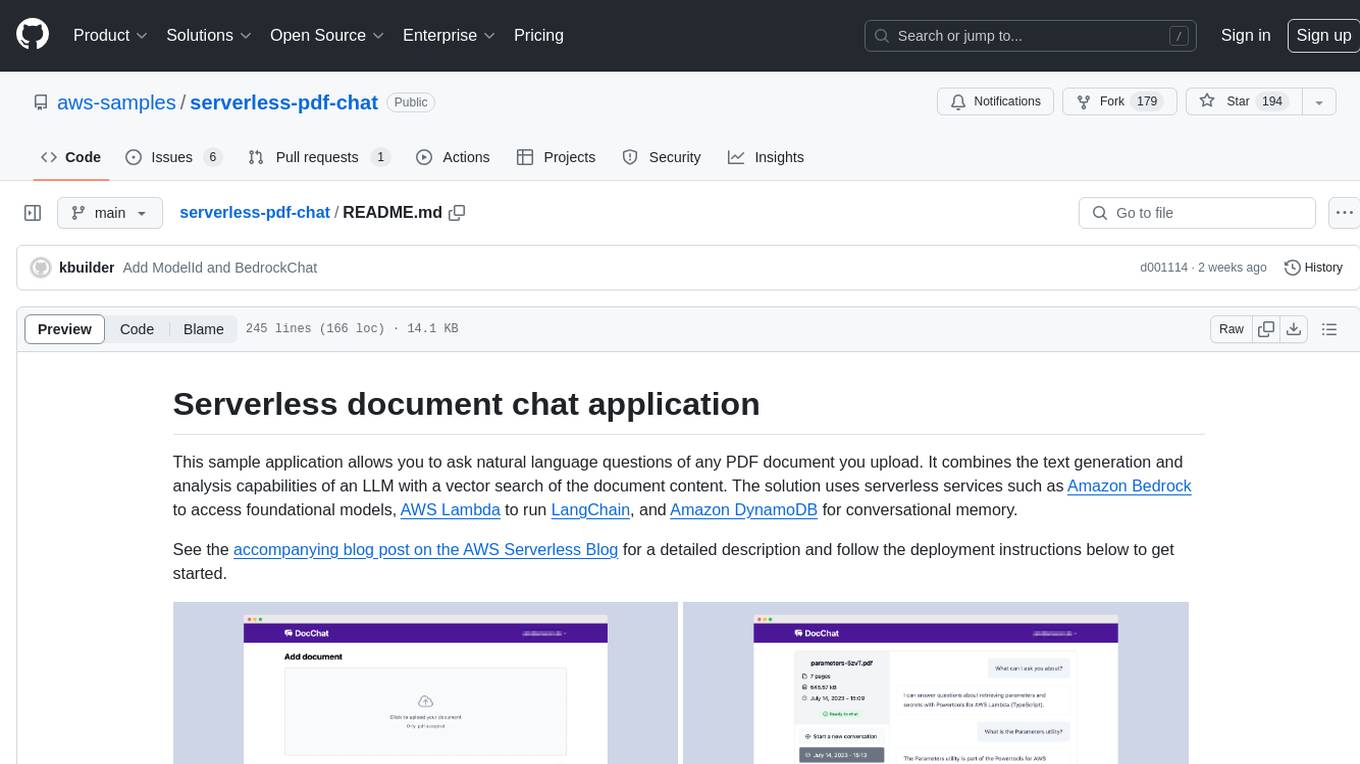
serverless-pdf-chat
The serverless-pdf-chat repository contains a sample application that allows users to ask natural language questions of any PDF document they upload. It leverages serverless services like Amazon Bedrock, AWS Lambda, and Amazon DynamoDB to provide text generation and analysis capabilities. The application architecture involves uploading a PDF document to an S3 bucket, extracting metadata, converting text to vectors, and using a LangChain to search for information related to user prompts. The application is not intended for production use and serves as a demonstration and educational tool.
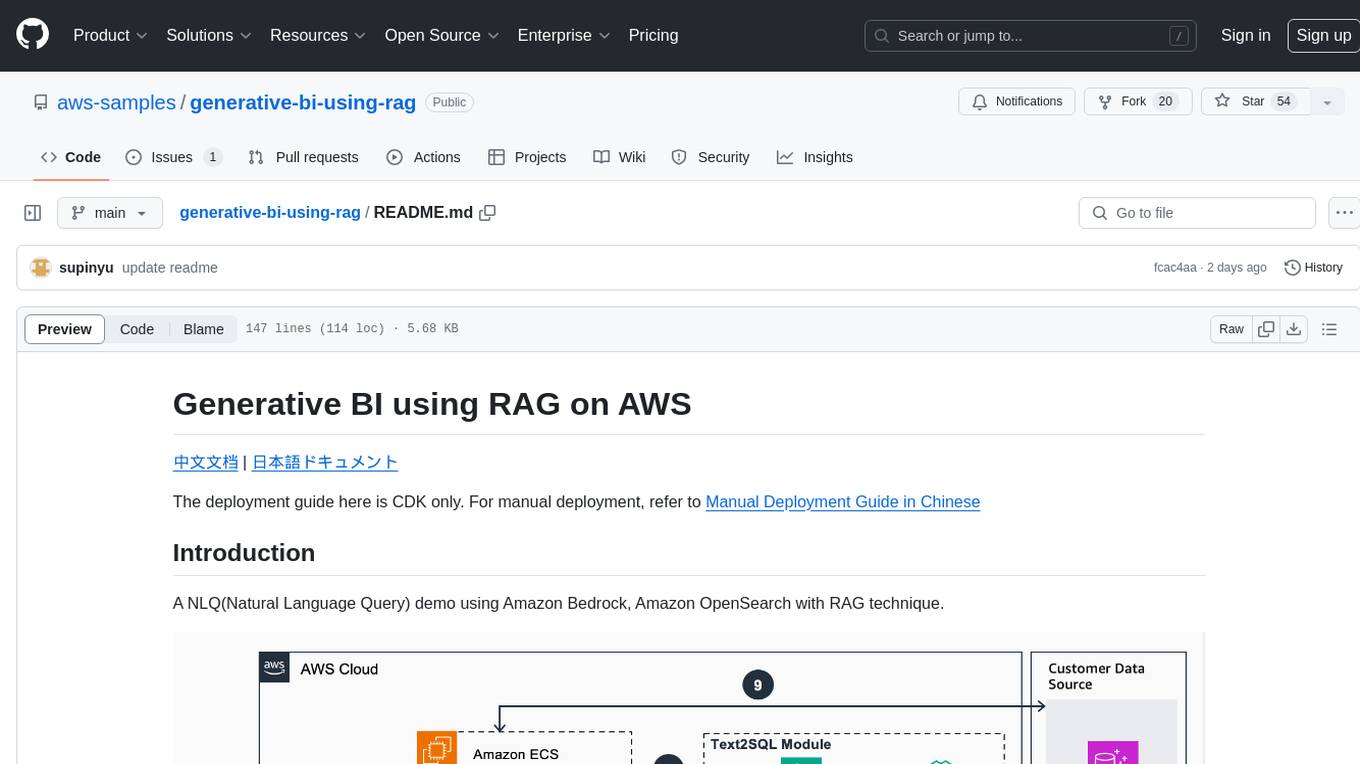
generative-bi-using-rag
Generative BI using RAG on AWS is a comprehensive framework designed to enable Generative BI capabilities on customized data sources hosted on AWS. It offers features such as Text-to-SQL functionality for querying data sources using natural language, user-friendly interface for managing data sources, performance enhancement through historical question-answer ranking, and entity recognition. It also allows customization of business information, handling complex attribution analysis problems, and provides an intuitive question-answering UI with a conversational approach for complex queries.
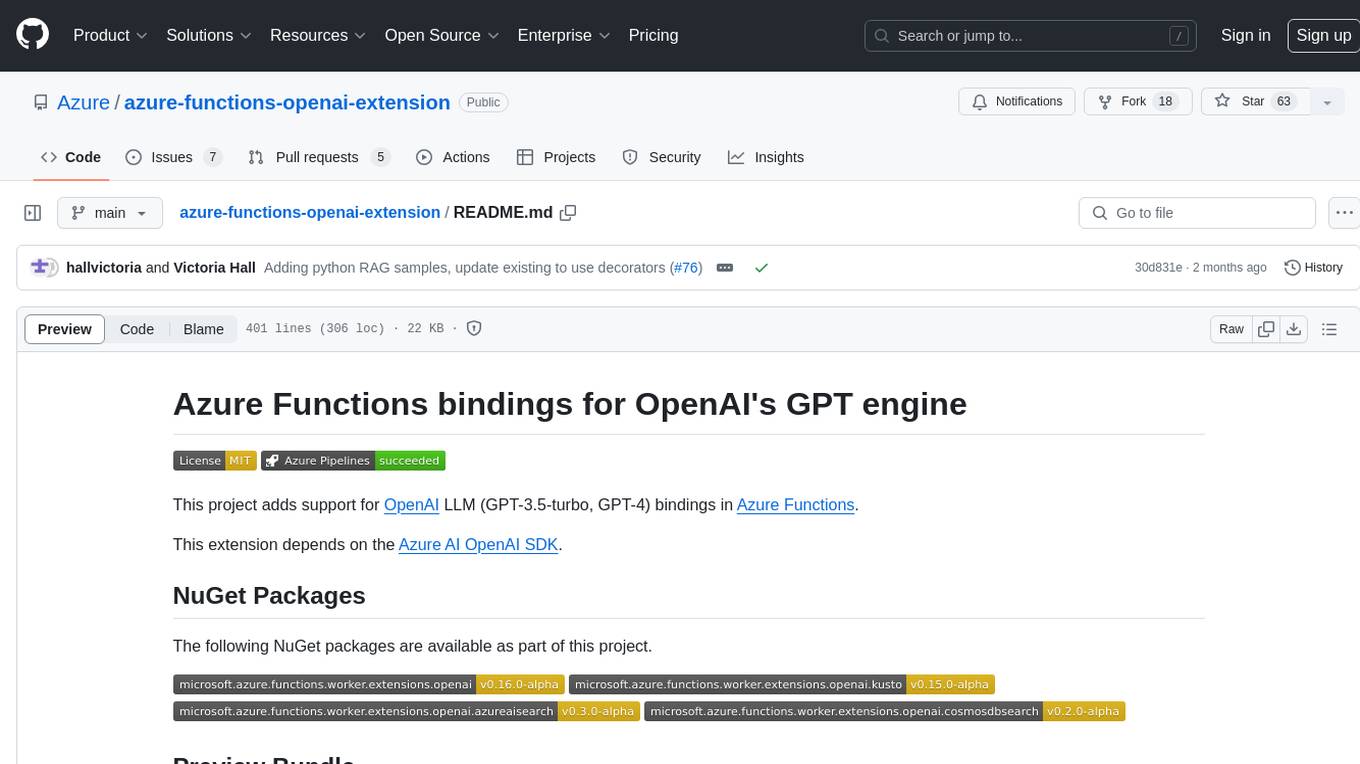
azure-functions-openai-extension
Azure Functions OpenAI Extension is a project that adds support for OpenAI LLM (GPT-3.5-turbo, GPT-4) bindings in Azure Functions. It provides NuGet packages for various functionalities like text completions, chat completions, assistants, embeddings generators, and semantic search. The project requires .NET 6 SDK or greater, Azure Functions Core Tools v4.x, and specific settings in Azure Function or local settings for development. It offers features like text completions, chat completion, assistants with custom skills, embeddings generators for text relatedness, and semantic search using vector databases. The project also includes examples in C# and Python for different functionalities.
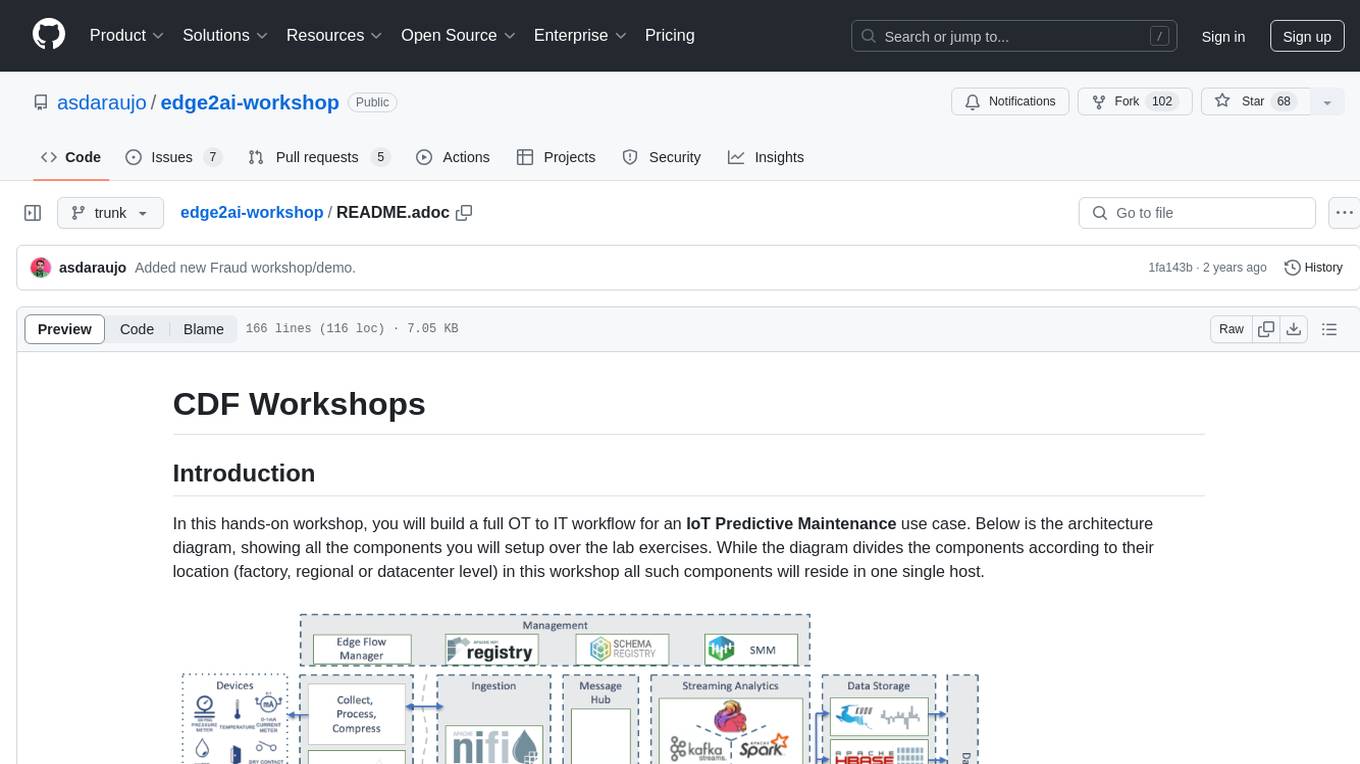
edge2ai-workshop
The edge2ai-workshop repository provides a hands-on workshop for building an IoT Predictive Maintenance workflow. It includes lab exercises for setting up components like NiFi, Streams Processing, Data Visualization, and more on a single host. The repository also covers use cases such as credit card fraud detection. Users can follow detailed instructions, prerequisites, and connectivity guidelines to connect to their cluster and explore various services. Additionally, troubleshooting tips are provided for common issues like MiNiFi not sending messages or CEM not picking up new NARs.
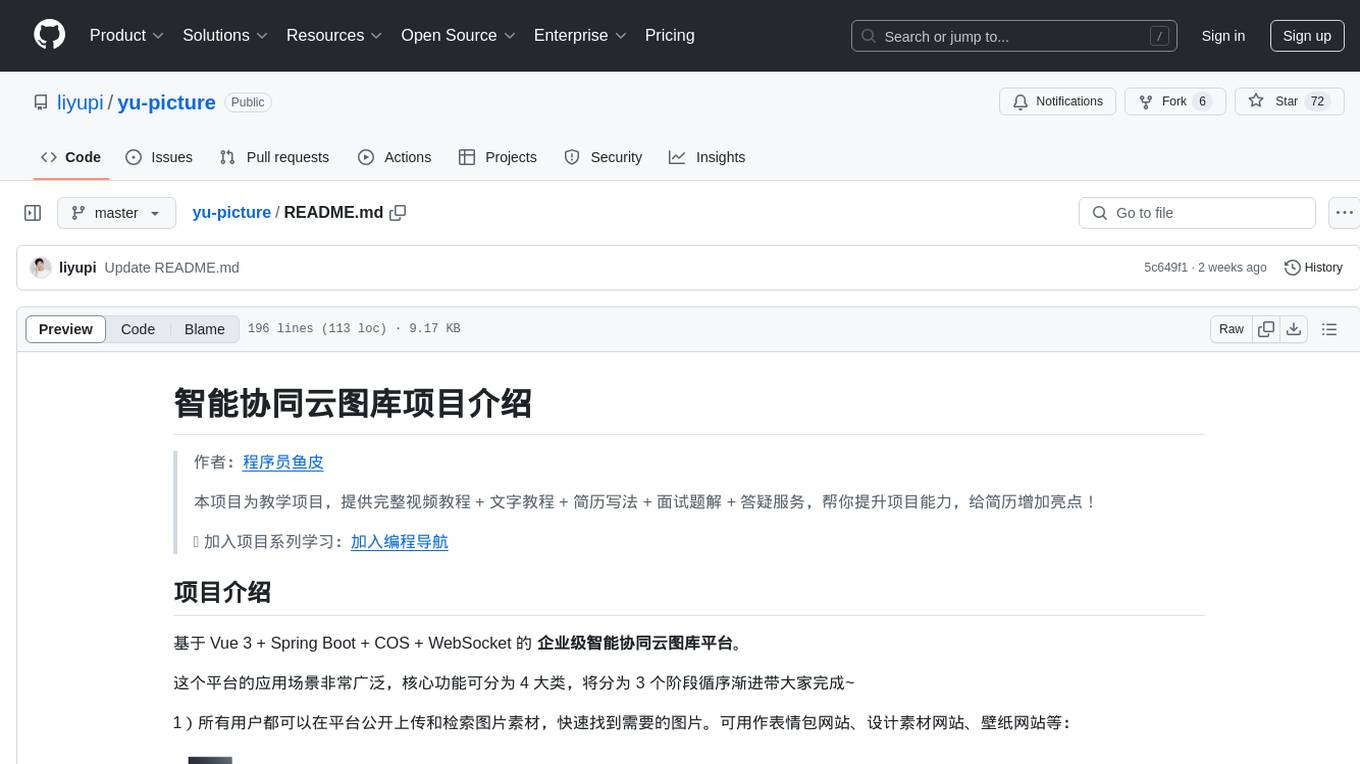
yu-picture
The 'yu-picture' project is an educational project that provides complete video tutorials, text tutorials, resume writing, interview question solutions, and Q&A services to help you improve your project skills and enhance your resume. It is an enterprise-level intelligent collaborative cloud image library platform based on Vue 3 + Spring Boot + COS + WebSocket. The platform has a wide range of applications, including public image uploading and retrieval, image analysis for administrators, private image management for individual users, and real-time collaborative image editing for enterprises. The project covers file management, content retrieval, permission control, and real-time collaboration, using various programming concepts, architectural design methods, and optimization strategies to ensure high-speed iteration and stable operation.
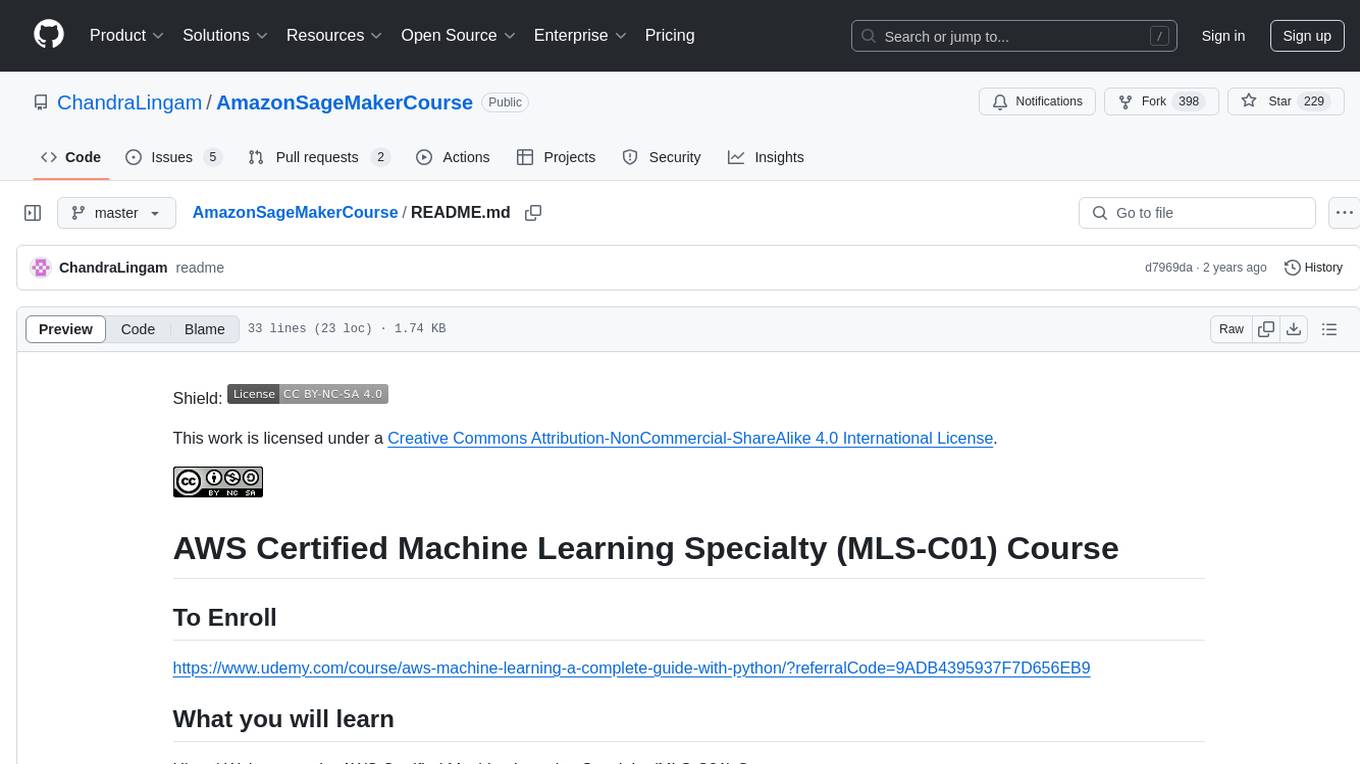
AmazonSageMakerCourse
Amazon SageMaker Course is a comprehensive guide for AWS Certified Machine Learning Specialty (MLS-C01) that covers training, optimizing, deploying, and integrating machine learning models in the AWS cloud. The course includes hands-on experience with AWS built-in algorithms, Bring Your Own models, and ready-to-use AI capabilities. It also provides a complete guide to AWS Certified Machine Learning – Specialty certification, along with a high-quality timed practice test. Participants will learn how to integrate trained models into their applications and receive prompt support through the course Q&A forum and private messaging.