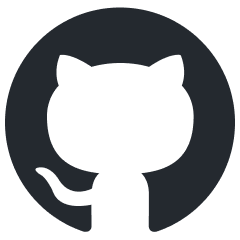
multipack_sampler
Multipack distributed sampler for fast padding-free training of LLMs
Stars: 162
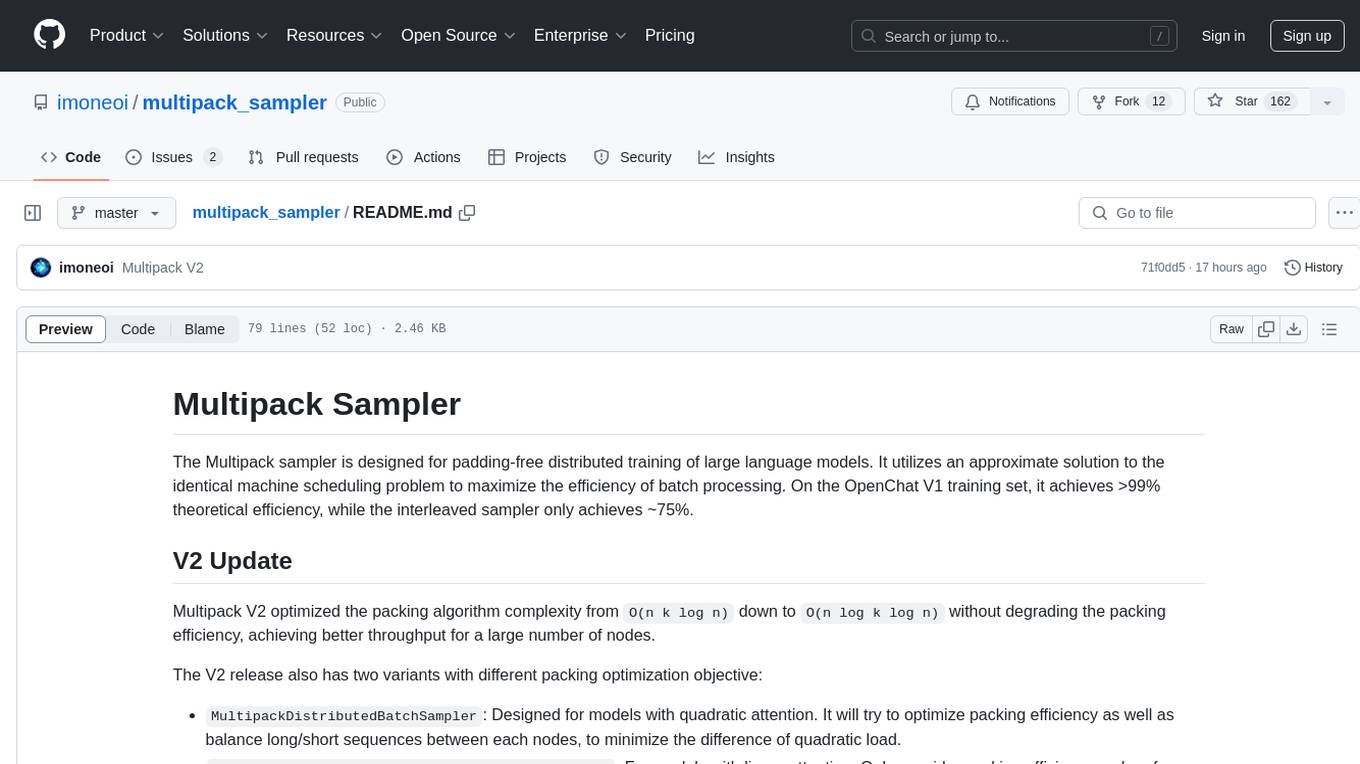
The Multipack sampler is a tool designed for padding-free distributed training of large language models. It optimizes batch processing efficiency using an approximate solution to the identical machine scheduling problem. The V2 update further enhances the packing algorithm complexity, achieving better throughput for a large number of nodes. It includes two variants for models with different attention types, aiming to balance sequence lengths and optimize packing efficiency. Users can refer to the provided benchmark for evaluating efficiency, utilization, and L^2 lag. The tool is compatible with PyTorch DataLoader and is released under the MIT license.
README:
The Multipack sampler is designed for padding-free distributed training of large language models. It utilizes an approximate solution to the identical machine scheduling problem to maximize the efficiency of batch processing. On the OpenChat V1 training set, it achieves >99% theoretical efficiency, while the interleaved sampler only achieves ~75%.
Multipack V2 optimized the packing algorithm complexity from O(n k log n)
down to O(n log k log n)
without degrading the packing efficiency, achieving better throughput for a large number of nodes.
The V2 release also has two variants with different packing optimization objective:
-
MultipackDistributedBatchSampler
: Designed for models with quadratic attention. It will try to optimize packing efficiency as well as balance long/short sequences between each nodes, to minimize the difference of quadratic load. -
MultipackDistributedBatchSampler_LinearAttention
: For models with linear attention. Only consider packing efficiency and performs better on it than Quadratic variant, however this algorithm tends to put all long sequences into one node.
Please refer to test_multipack.ipynb
-
Efficiency: Percentage of actual batch size to max batch size
=
number of tokens per batch / max capacity of tokens per batch
-
Utilization: all nodes waiting for the slowest node
=
number of tokens per batch / max number of tokens on a single node * node count
L^2 lag: sqrt(max over node(sum length^2) - min over node(sum length^2))
OpenChat V1 (testdata.json)
Sampler Multipack QuadraticAttention:
Batch count for ranks: [37, 37, 37, 37, 37, 37, 37, 37]
Packing Time: 20ms
L^2 lag avg: 438 max: 717
Efficiency: 98.16%
Utilization: 99.70%
==========
Sampler Multipack LinearAttention:
Batch count for ranks: [36, 36, 36, 36, 36, 36, 36, 36]
Packing Time: 18ms
L^2 lag avg: 6500 max: 6761
Efficiency: 99.64%
Utilization: 99.64%
==========
Sampler Interleaved:
Batch count for ranks: [48, 48, 48, 48, 48, 48, 48, 48]
Packing Time: 0ms
L^2 lag avg: 1914 max: 2000
Efficiency: 75.67%
Utilization: 96.79%
==========
Compatible with PyTorch DataLoader
batch_max_len = 16 * 2048 # batch size * max context length
lengths = np.array([len(tokens) for tokens in data])
sampler = MultipackDistributedBatchSampler(
batch_max_length=batch_max_len,
lengths=lengths,
seed=0
)
dataloader = DataLoader(data, batch_sampler=sampler)
MIT
For Tasks:
Click tags to check more tools for each tasksFor Jobs:
Alternative AI tools for multipack_sampler
Similar Open Source Tools
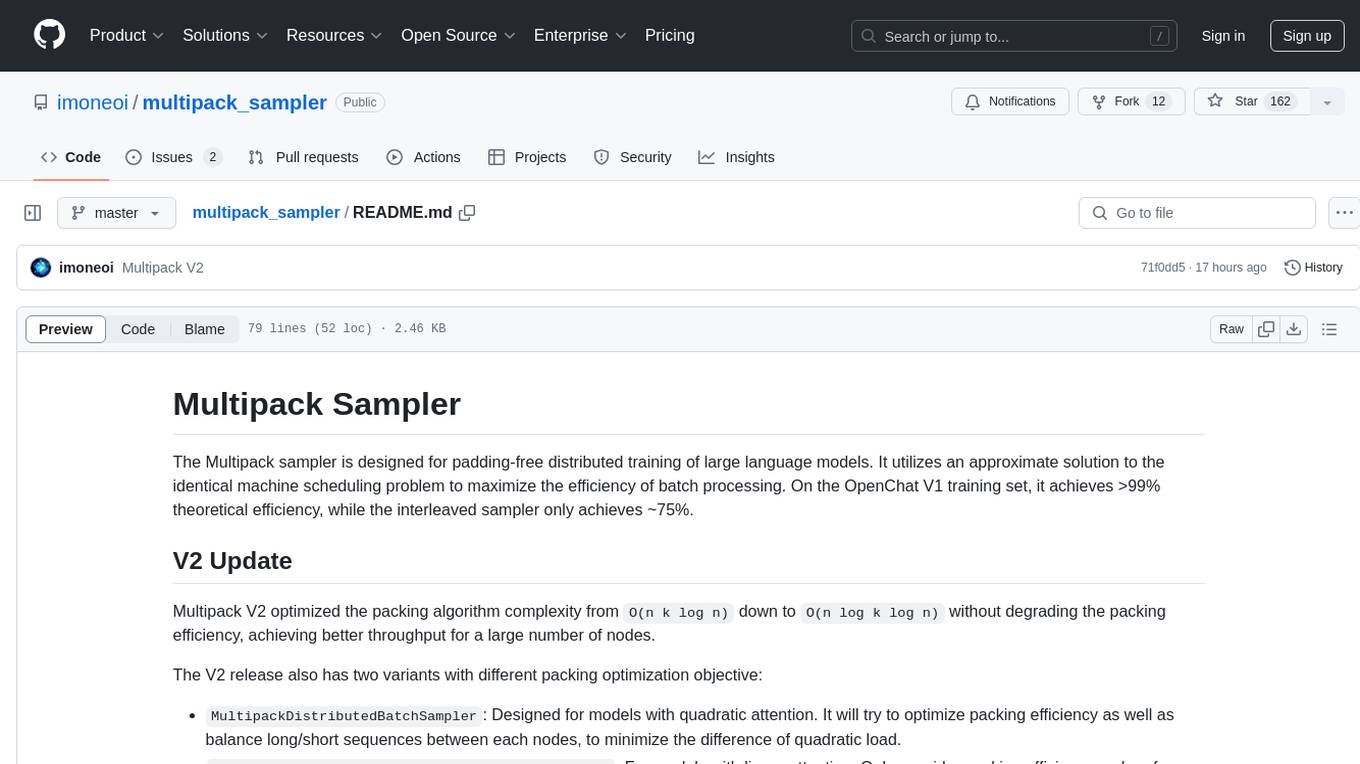
multipack_sampler
The Multipack sampler is a tool designed for padding-free distributed training of large language models. It optimizes batch processing efficiency using an approximate solution to the identical machine scheduling problem. The V2 update further enhances the packing algorithm complexity, achieving better throughput for a large number of nodes. It includes two variants for models with different attention types, aiming to balance sequence lengths and optimize packing efficiency. Users can refer to the provided benchmark for evaluating efficiency, utilization, and L^2 lag. The tool is compatible with PyTorch DataLoader and is released under the MIT license.
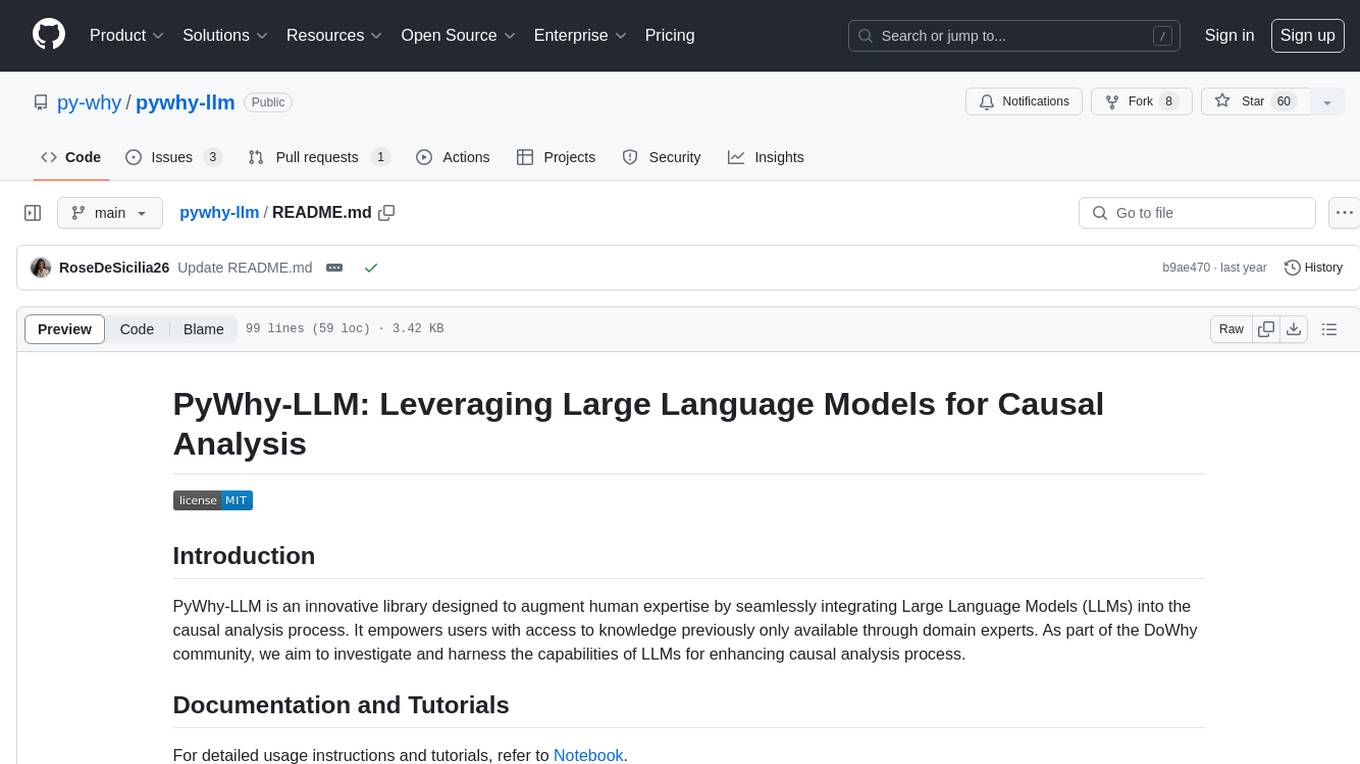
pywhy-llm
PyWhy-LLM is an innovative library that integrates Large Language Models (LLMs) into the causal analysis process, empowering users with knowledge previously only available through domain experts. It seamlessly augments existing causal inference processes by suggesting potential confounders, relationships between variables, backdoor sets, front door sets, IV sets, estimands, critiques of DAGs, latent confounders, and negative controls. By leveraging LLMs and formalizing human-LLM collaboration, PyWhy-LLM aims to enhance causal analysis accessibility and insight.
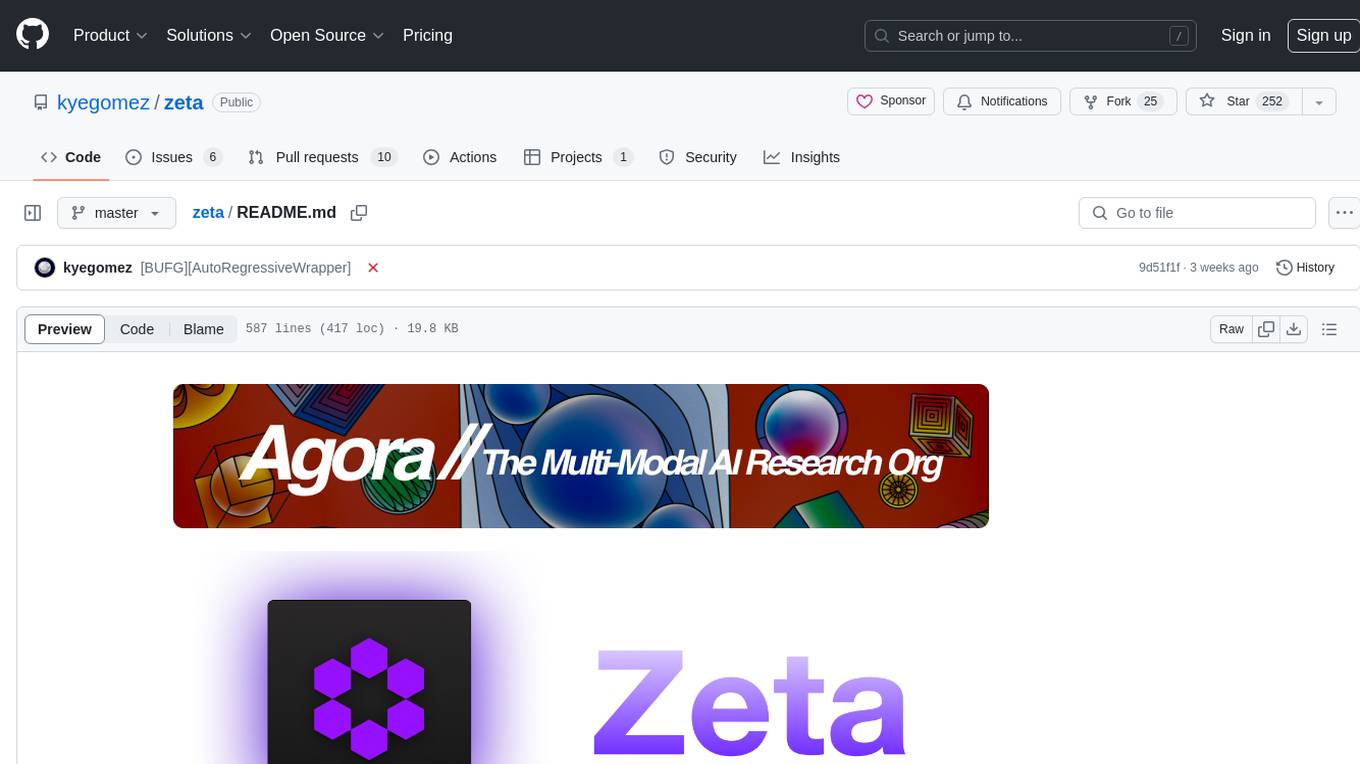
zeta
Zeta is a tool designed to build state-of-the-art AI models faster by providing modular, high-performance, and scalable building blocks. It addresses the common issues faced while working with neural nets, such as chaotic codebases, lack of modularity, and low performance modules. Zeta emphasizes usability, modularity, and performance, and is currently used in hundreds of models across various GitHub repositories. It enables users to prototype, train, optimize, and deploy the latest SOTA neural nets into production. The tool offers various modules like FlashAttention, SwiGLUStacked, RelativePositionBias, FeedForward, BitLinear, PalmE, Unet, VisionEmbeddings, niva, FusedDenseGELUDense, FusedDropoutLayerNorm, MambaBlock, Film, hyper_optimize, DPO, and ZetaCloud for different tasks in AI model development.
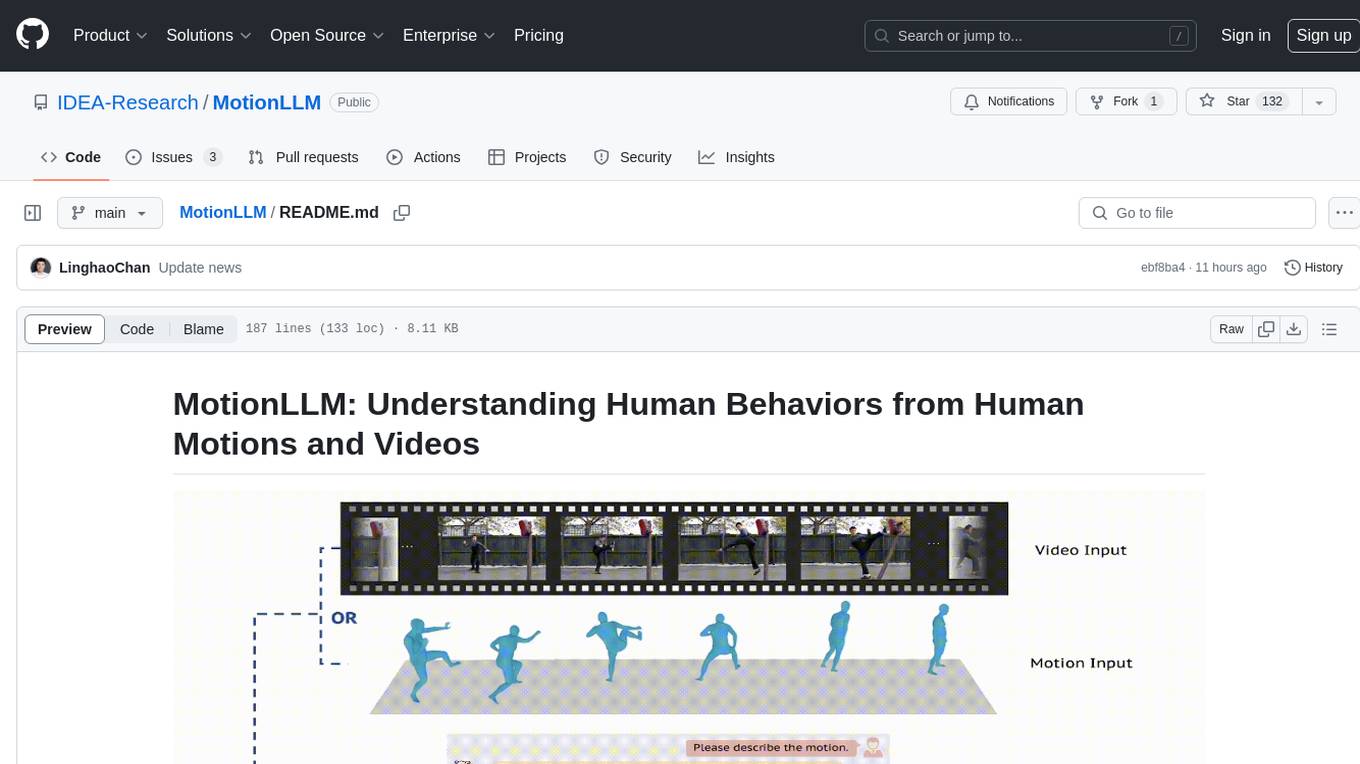
MotionLLM
MotionLLM is a framework for human behavior understanding that leverages Large Language Models (LLMs) to jointly model videos and motion sequences. It provides a unified training strategy, dataset MoVid, and MoVid-Bench for evaluating human behavior comprehension. The framework excels in captioning, spatial-temporal comprehension, and reasoning abilities.
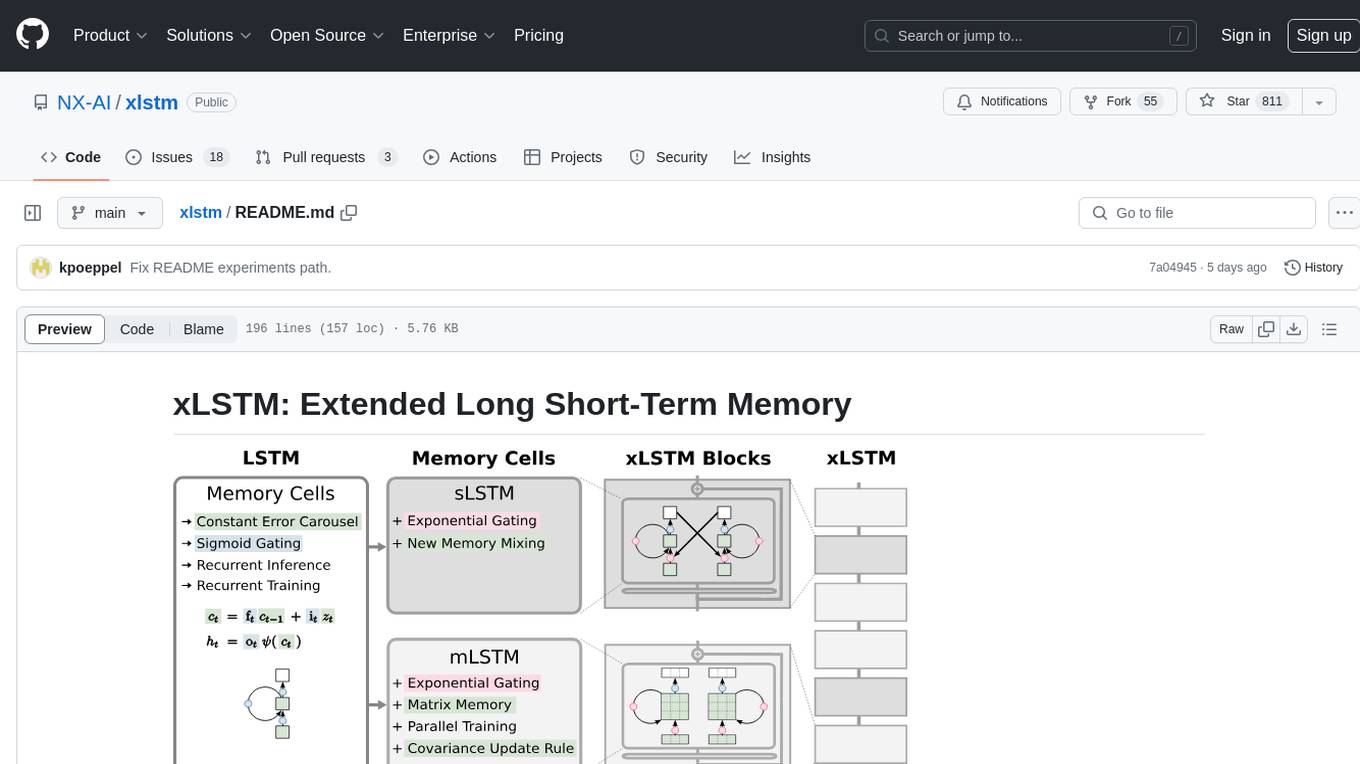
xlstm
xLSTM is a new Recurrent Neural Network architecture based on ideas of the original LSTM. Through Exponential Gating with appropriate normalization and stabilization techniques and a new Matrix Memory it overcomes the limitations of the original LSTM and shows promising performance on Language Modeling when compared to Transformers or State Space Models. The package is based on PyTorch and was tested for versions >=1.8. For the CUDA version of xLSTM, you need Compute Capability >= 8.0. The xLSTM tool provides two main components: xLSTMBlockStack for non-language applications or integrating in other architectures, and xLSTMLMModel for language modeling or other token-based applications.
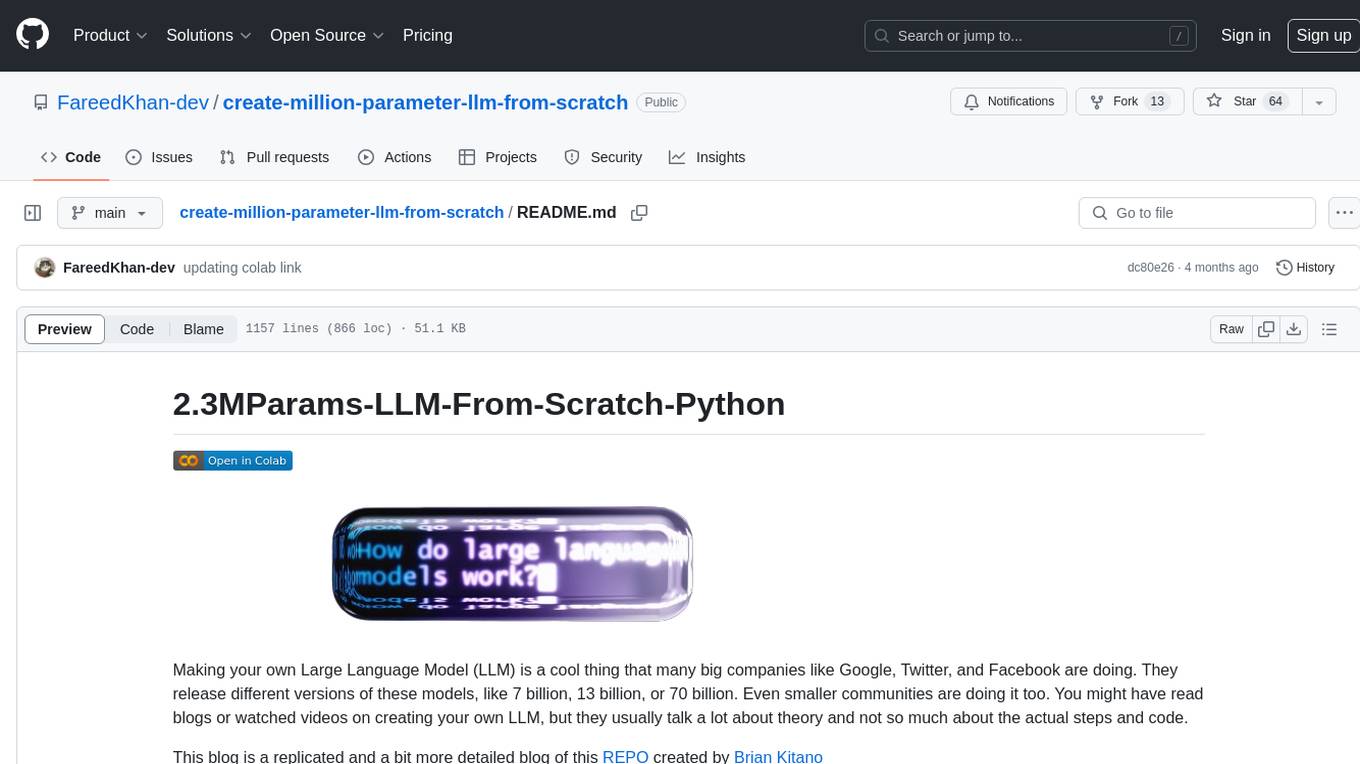
create-million-parameter-llm-from-scratch
The 'create-million-parameter-llm-from-scratch' repository provides a detailed guide on creating a Large Language Model (LLM) with 2.3 million parameters from scratch. The blog replicates the LLaMA approach, incorporating concepts like RMSNorm for pre-normalization, SwiGLU activation function, and Rotary Embeddings. The model is trained on a basic dataset to demonstrate the ease of creating a million-parameter LLM without the need for a high-end GPU.
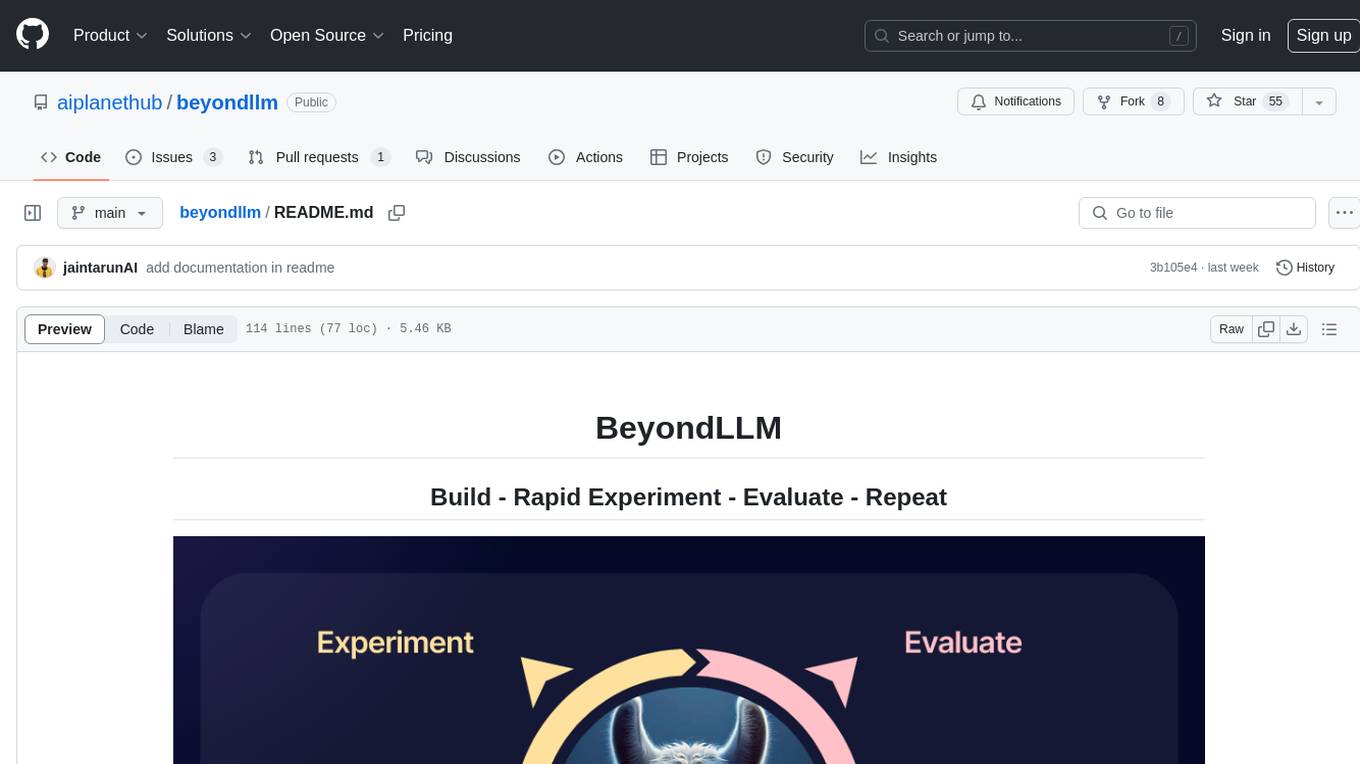
beyondllm
Beyond LLM offers an all-in-one toolkit for experimentation, evaluation, and deployment of Retrieval-Augmented Generation (RAG) systems. It simplifies the process with automated integration, customizable evaluation metrics, and support for various Large Language Models (LLMs) tailored to specific needs. The aim is to reduce LLM hallucination risks and enhance reliability.
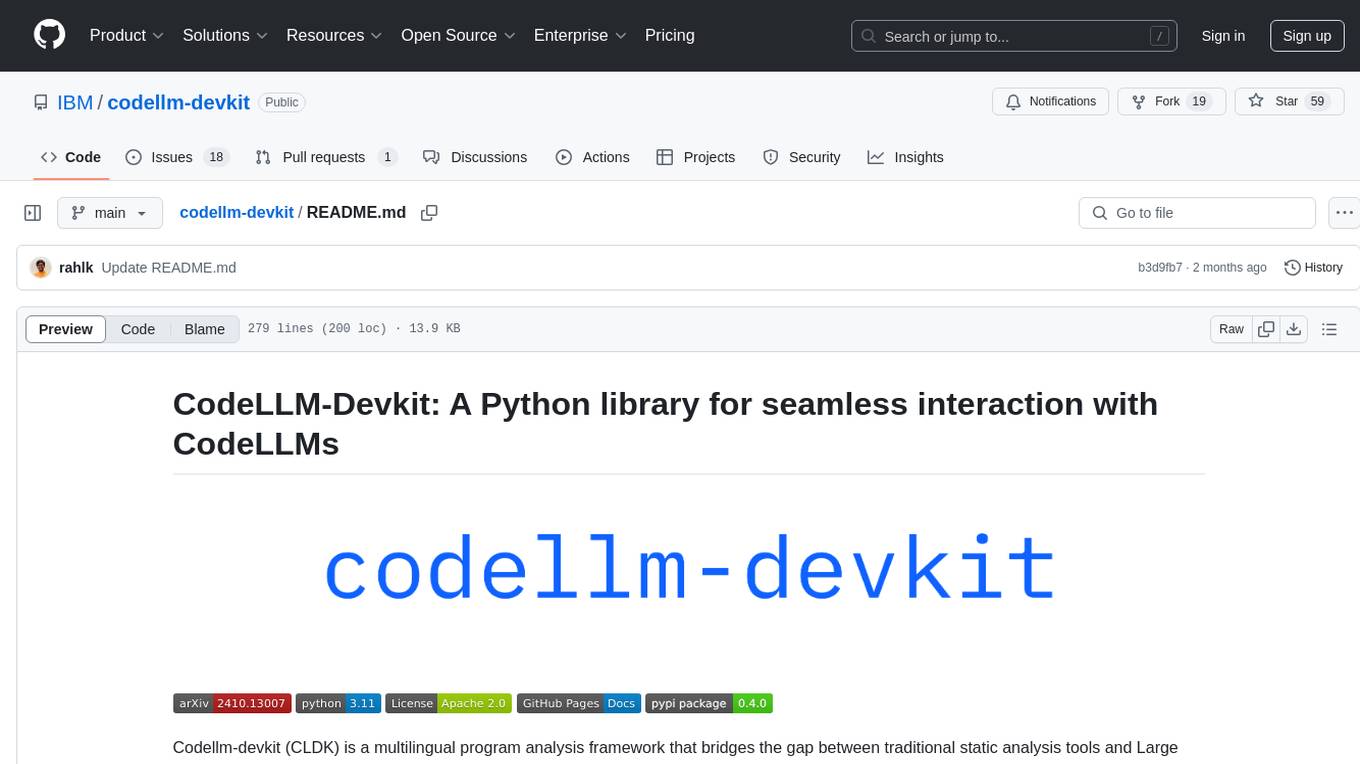
codellm-devkit
Codellm-devkit (CLDK) is a Python library that serves as a multilingual program analysis framework bridging traditional static analysis tools and Large Language Models (LLMs) specialized for code (CodeLLMs). It simplifies the process of analyzing codebases across multiple programming languages, enabling the extraction of meaningful insights and facilitating LLM-based code analysis. The library provides a unified interface for integrating outputs from various analysis tools and preparing them for effective use by CodeLLMs. Codellm-devkit aims to enable the development and experimentation of robust analysis pipelines that combine traditional program analysis tools and CodeLLMs, reducing friction in multi-language code analysis and ensuring compatibility across different tools and LLM platforms. It is designed to seamlessly integrate with popular analysis tools like WALA, Tree-sitter, LLVM, and CodeQL, acting as a crucial intermediary layer for efficient communication between these tools and CodeLLMs. The project is continuously evolving to include new tools and frameworks, maintaining its versatility for code analysis and LLM integration.
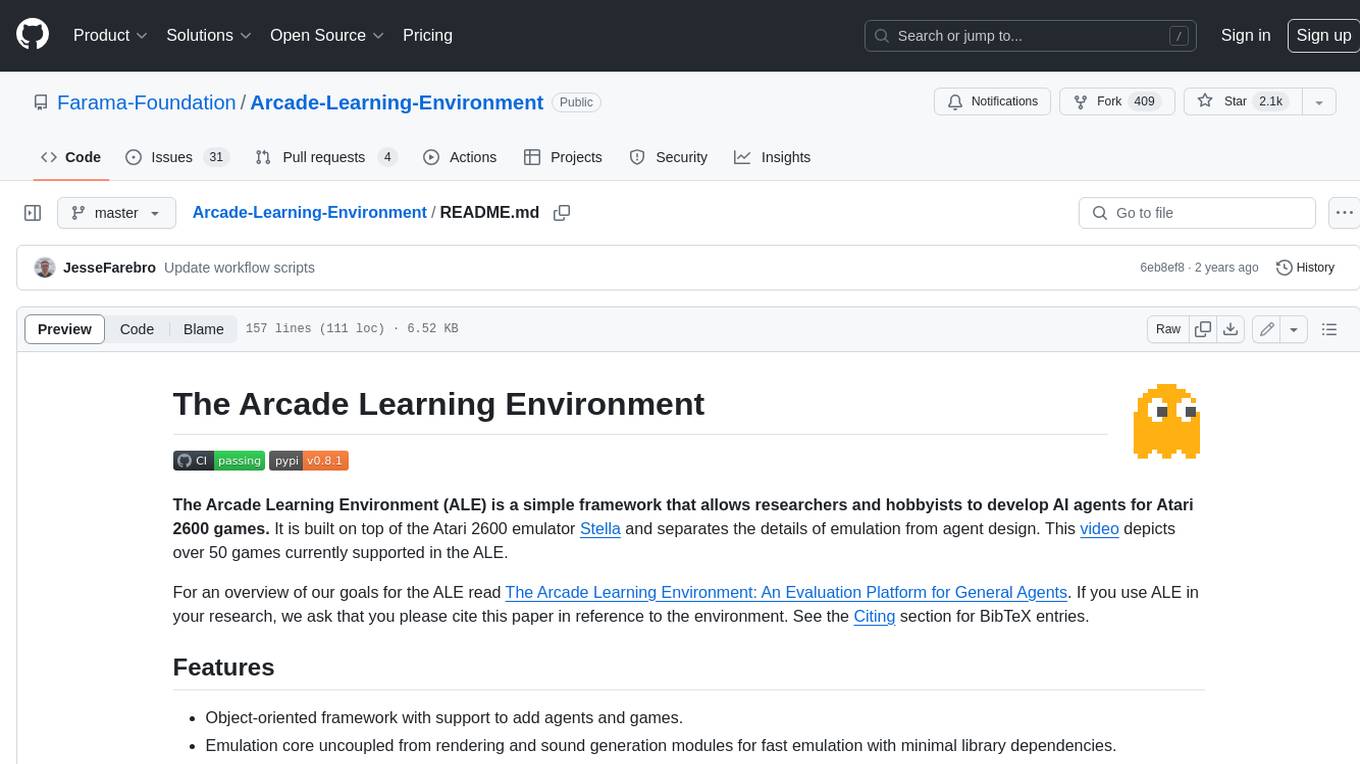
Arcade-Learning-Environment
The Arcade Learning Environment (ALE) is a simple framework that allows researchers and hobbyists to develop AI agents for Atari 2600 games. It is built on top of the Atari 2600 emulator Stella and separates the details of emulation from agent design. The ALE currently supports three different interfaces: C++, Python, and OpenAI Gym.
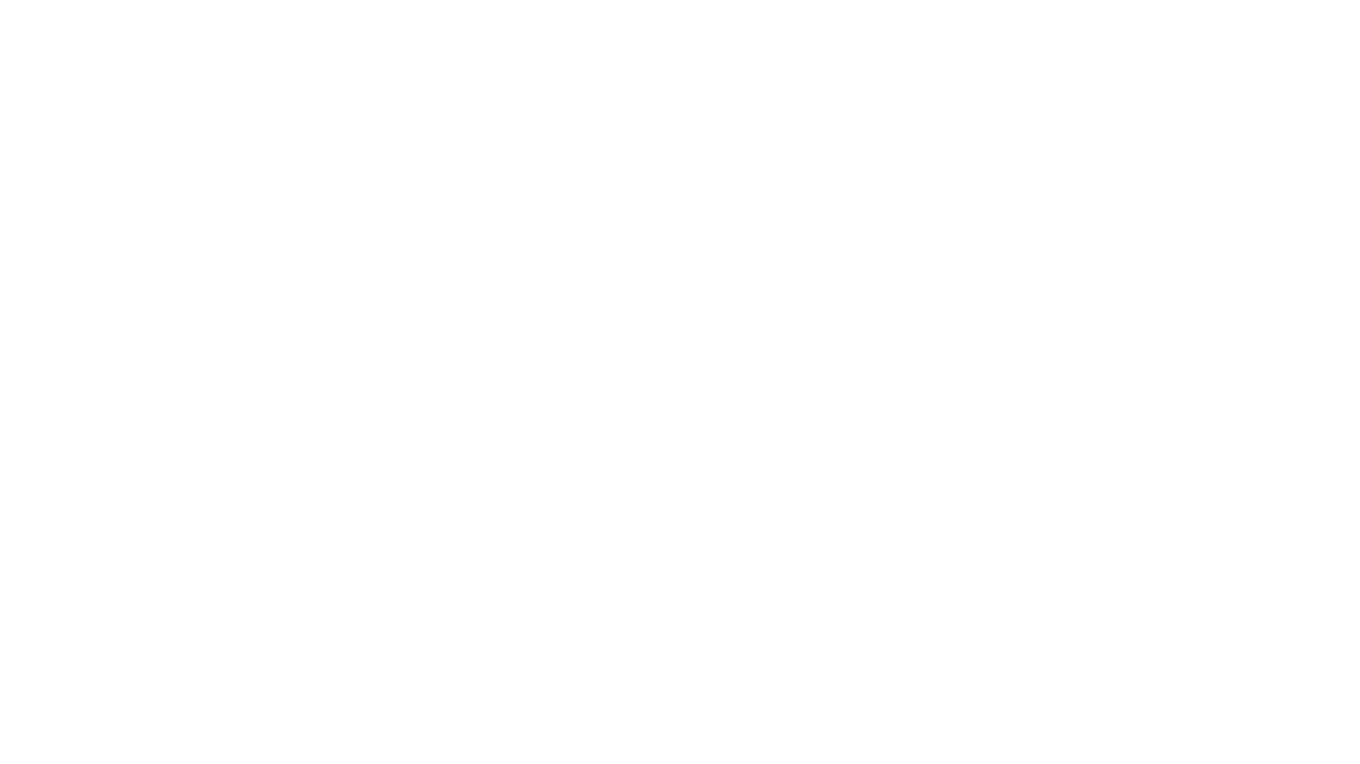
aiomisc
aiomisc is a Python library that provides a collection of utility functions and classes for working with asynchronous I/O in a more intuitive and efficient way. It offers features like worker pools, connection pools, circuit breaker pattern, and retry mechanisms to make asyncio code more robust and easier to maintain. The library simplifies the architecture of software using asynchronous I/O, making it easier for developers to write reliable and scalable asynchronous code.
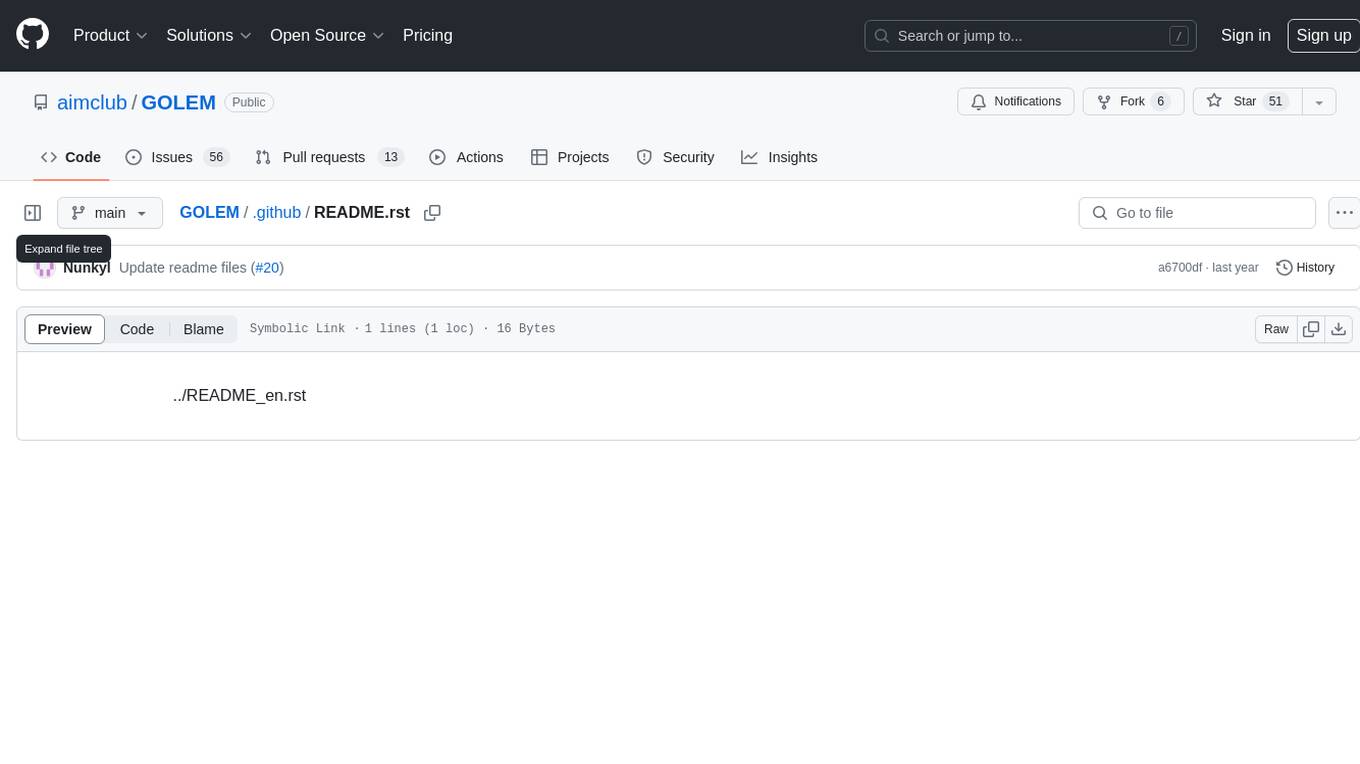
GOLEM
GOLEM is an open-source AI framework focused on optimization and learning of structured graph-based models using meta-heuristic methods. It emphasizes the potential of meta-heuristics in complex problem spaces where gradient-based methods are not suitable, and the importance of structured models in various problem domains. The framework offers features like structured model optimization, metaheuristic methods, multi-objective optimization, constrained optimization, extensibility, interpretability, and reproducibility. It can be applied to optimization problems represented as directed graphs with defined fitness functions. GOLEM has applications in areas like AutoML, Bayesian network structure search, differential equation discovery, geometric design, and neural architecture search. The project structure includes packages for core functionalities, adapters, graph representation, optimizers, genetic algorithms, utilities, serialization, visualization, examples, and testing. Contributions are welcome, and the project is supported by ITMO University's Research Center Strong Artificial Intelligence in Industry.
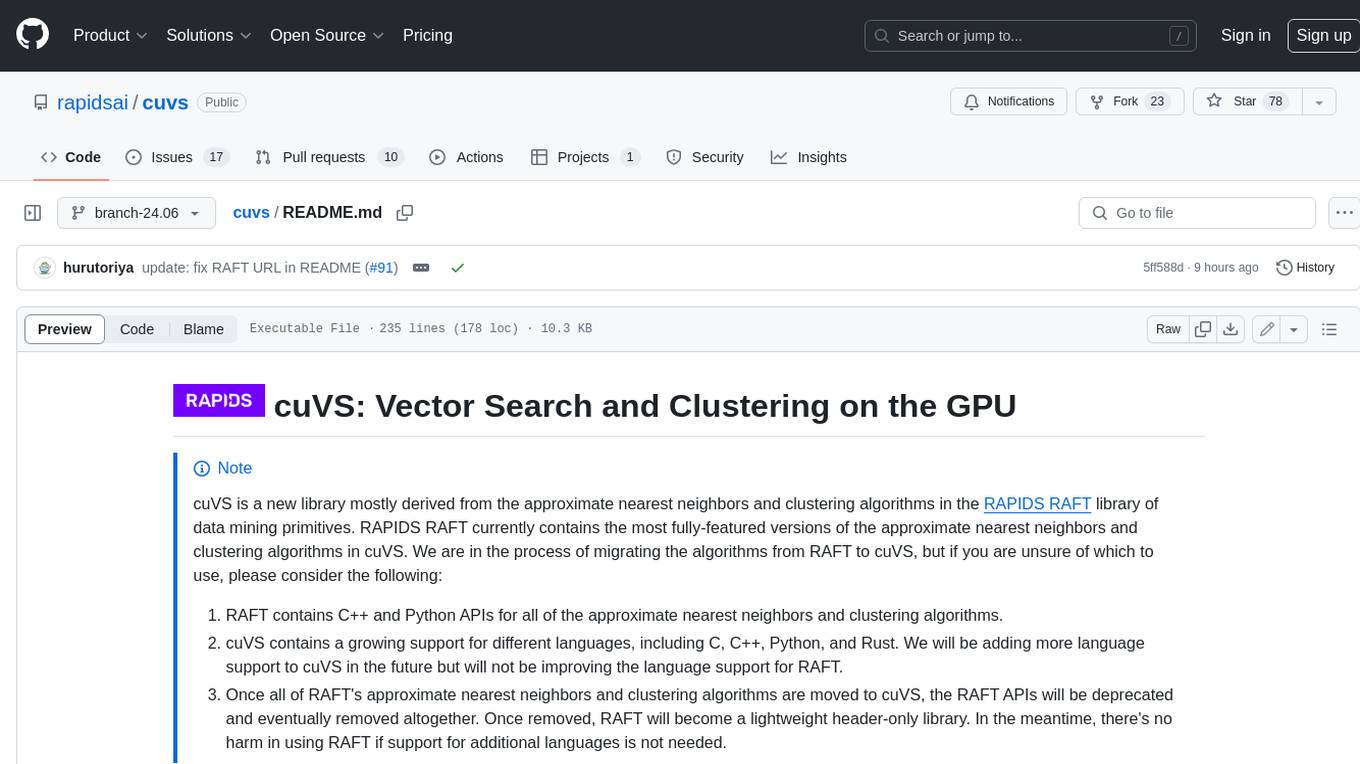
cuvs
cuVS is a library that contains state-of-the-art implementations of several algorithms for running approximate nearest neighbors and clustering on the GPU. It can be used directly or through the various databases and other libraries that have integrated it. The primary goal of cuVS is to simplify the use of GPUs for vector similarity search and clustering.
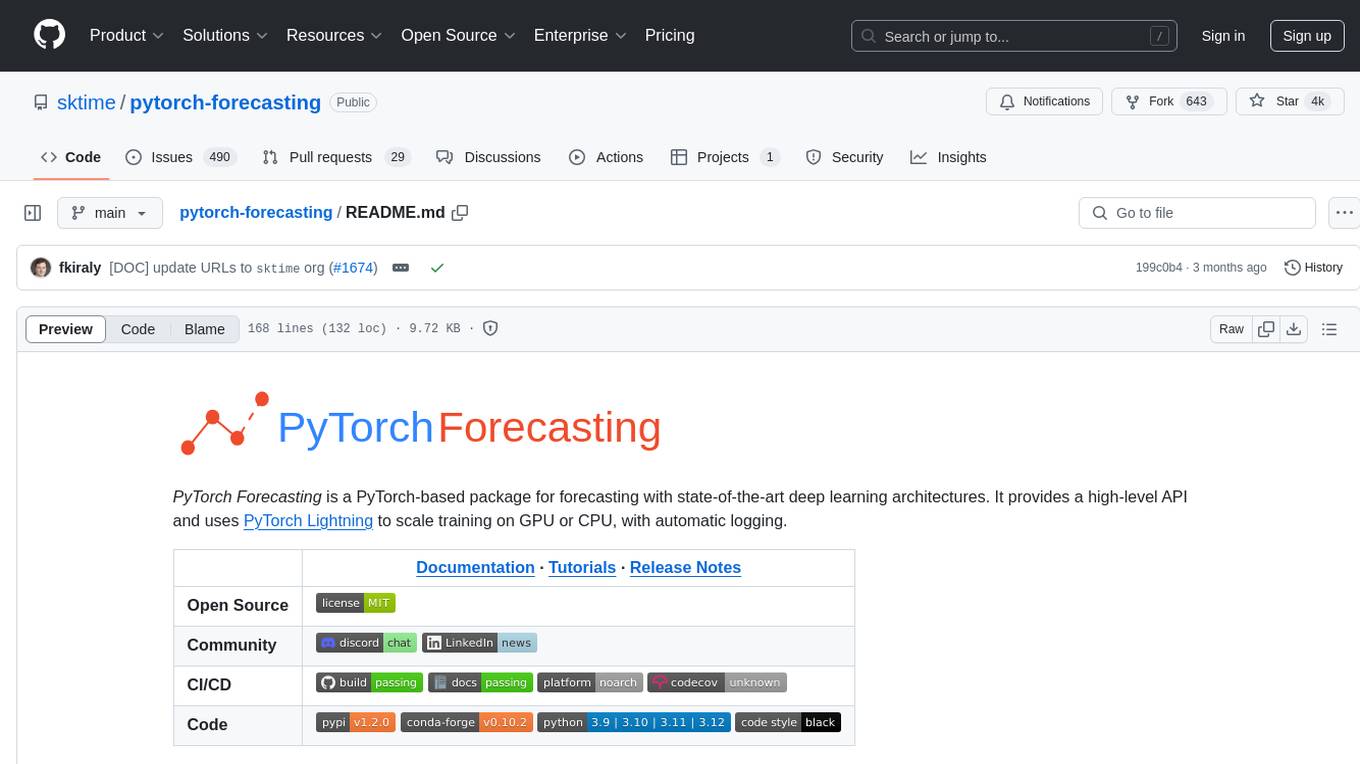
pytorch-forecasting
PyTorch Forecasting is a PyTorch-based package designed for state-of-the-art timeseries forecasting using deep learning architectures. It offers a high-level API and leverages PyTorch Lightning for efficient training on GPU or CPU with automatic logging. The package aims to simplify timeseries forecasting tasks by providing a flexible API for professionals and user-friendly defaults for beginners. It includes features such as a timeseries dataset class for handling data transformations, missing values, and subsampling, various neural network architectures optimized for real-world deployment, multi-horizon timeseries metrics, and hyperparameter tuning with optuna. Built on pytorch-lightning, it supports training on CPUs, single GPUs, and multiple GPUs out-of-the-box.
For similar tasks
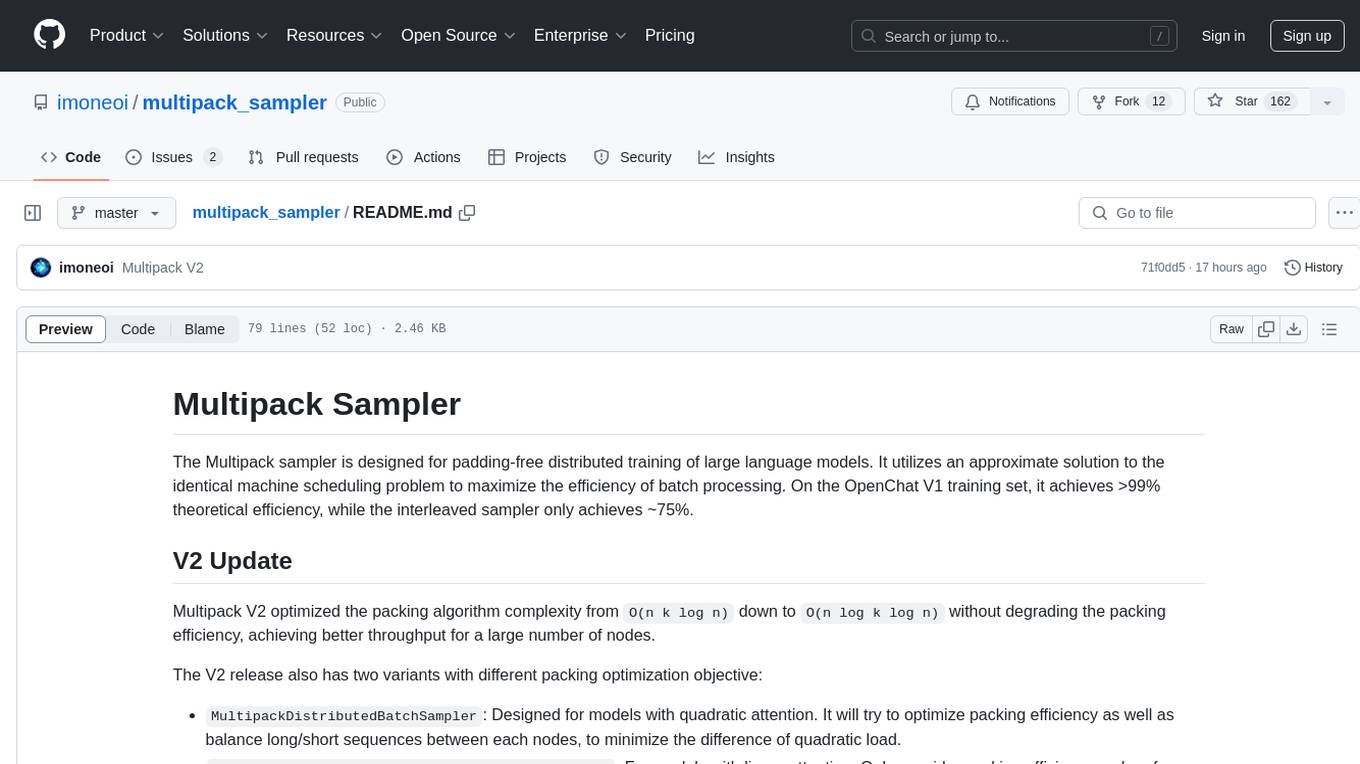
multipack_sampler
The Multipack sampler is a tool designed for padding-free distributed training of large language models. It optimizes batch processing efficiency using an approximate solution to the identical machine scheduling problem. The V2 update further enhances the packing algorithm complexity, achieving better throughput for a large number of nodes. It includes two variants for models with different attention types, aiming to balance sequence lengths and optimize packing efficiency. Users can refer to the provided benchmark for evaluating efficiency, utilization, and L^2 lag. The tool is compatible with PyTorch DataLoader and is released under the MIT license.
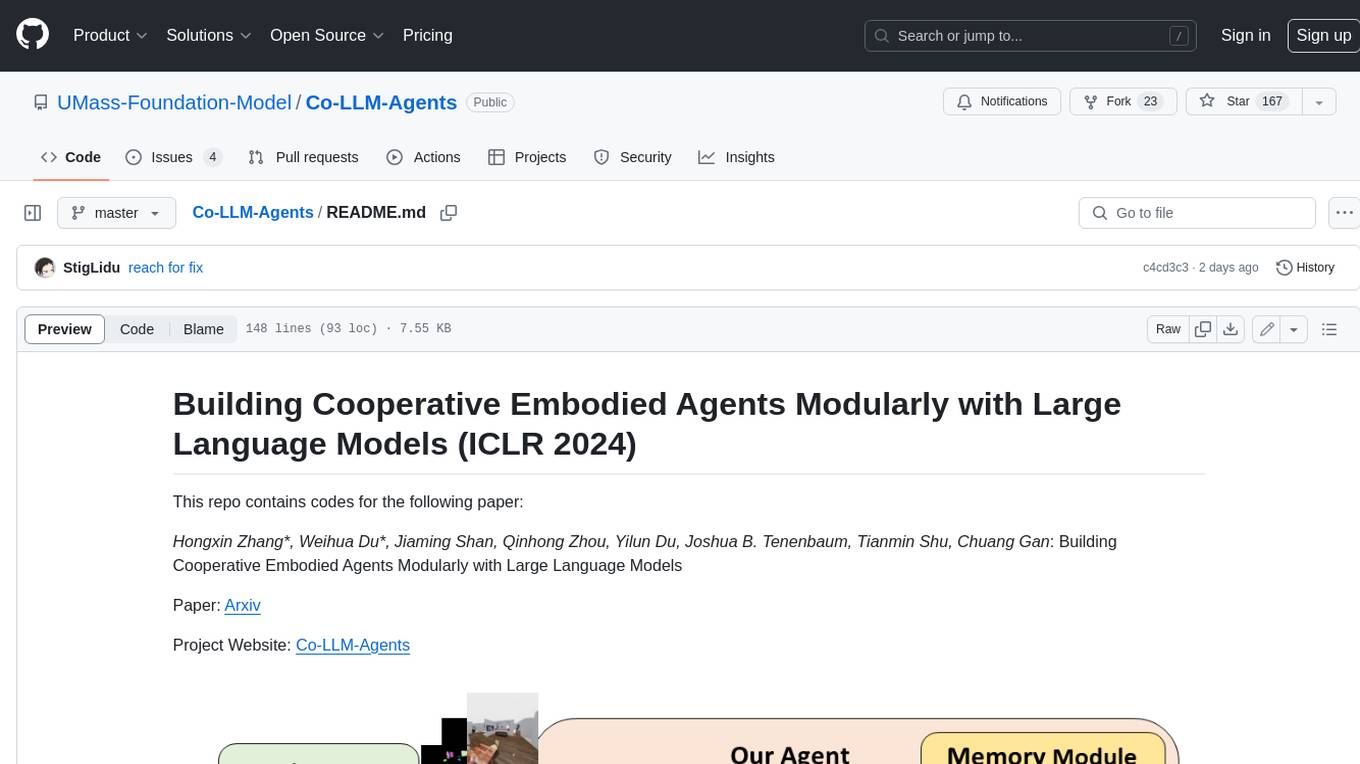
Co-LLM-Agents
This repository contains code for building cooperative embodied agents modularly with large language models. The agents are trained to perform tasks in two different environments: ThreeDWorld Multi-Agent Transport (TDW-MAT) and Communicative Watch-And-Help (C-WAH). TDW-MAT is a multi-agent environment where agents must transport objects to a goal position using containers. C-WAH is an extension of the Watch-And-Help challenge, which enables agents to send messages to each other. The code in this repository can be used to train agents to perform tasks in both of these environments.
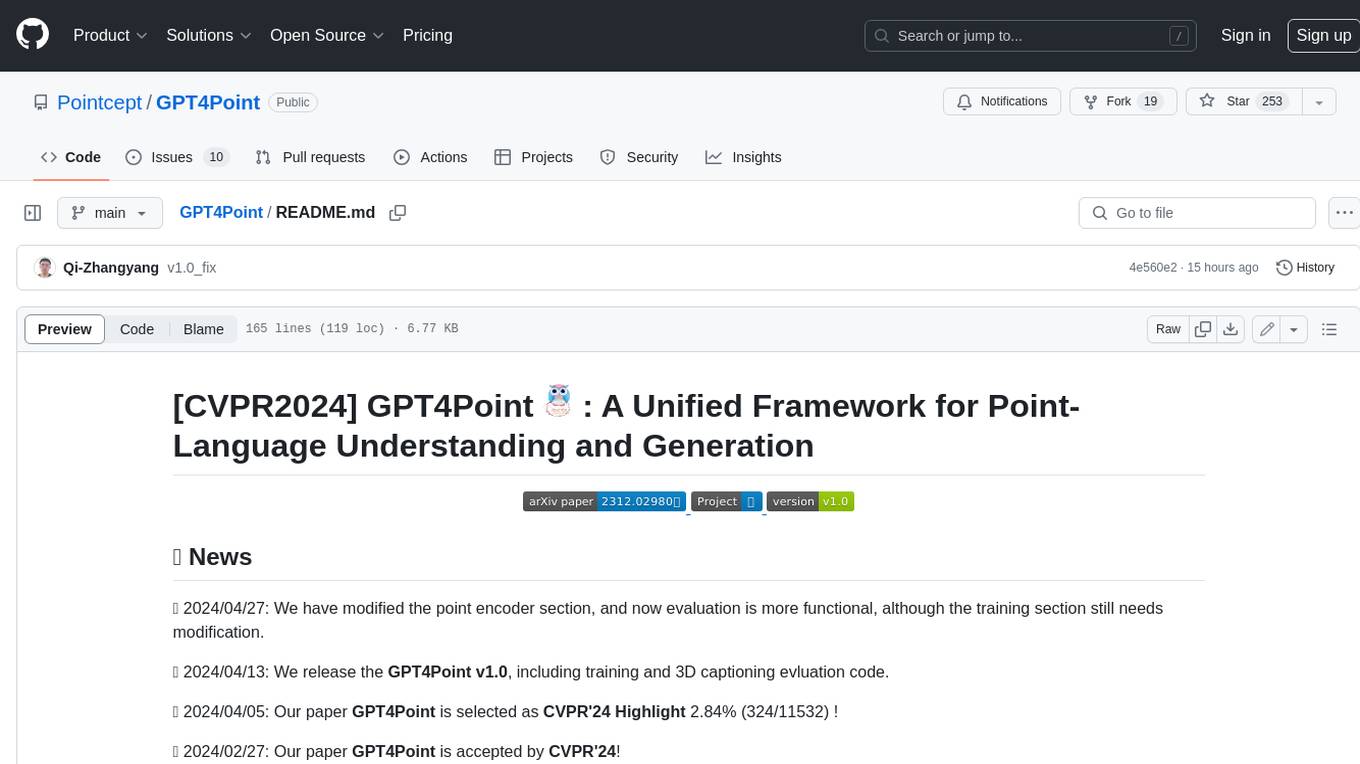
GPT4Point
GPT4Point is a unified framework for point-language understanding and generation. It aligns 3D point clouds with language, providing a comprehensive solution for tasks such as 3D captioning and controlled 3D generation. The project includes an automated point-language dataset annotation engine, a novel object-level point cloud benchmark, and a 3D multi-modality model. Users can train and evaluate models using the provided code and datasets, with a focus on improving models' understanding capabilities and facilitating the generation of 3D objects.
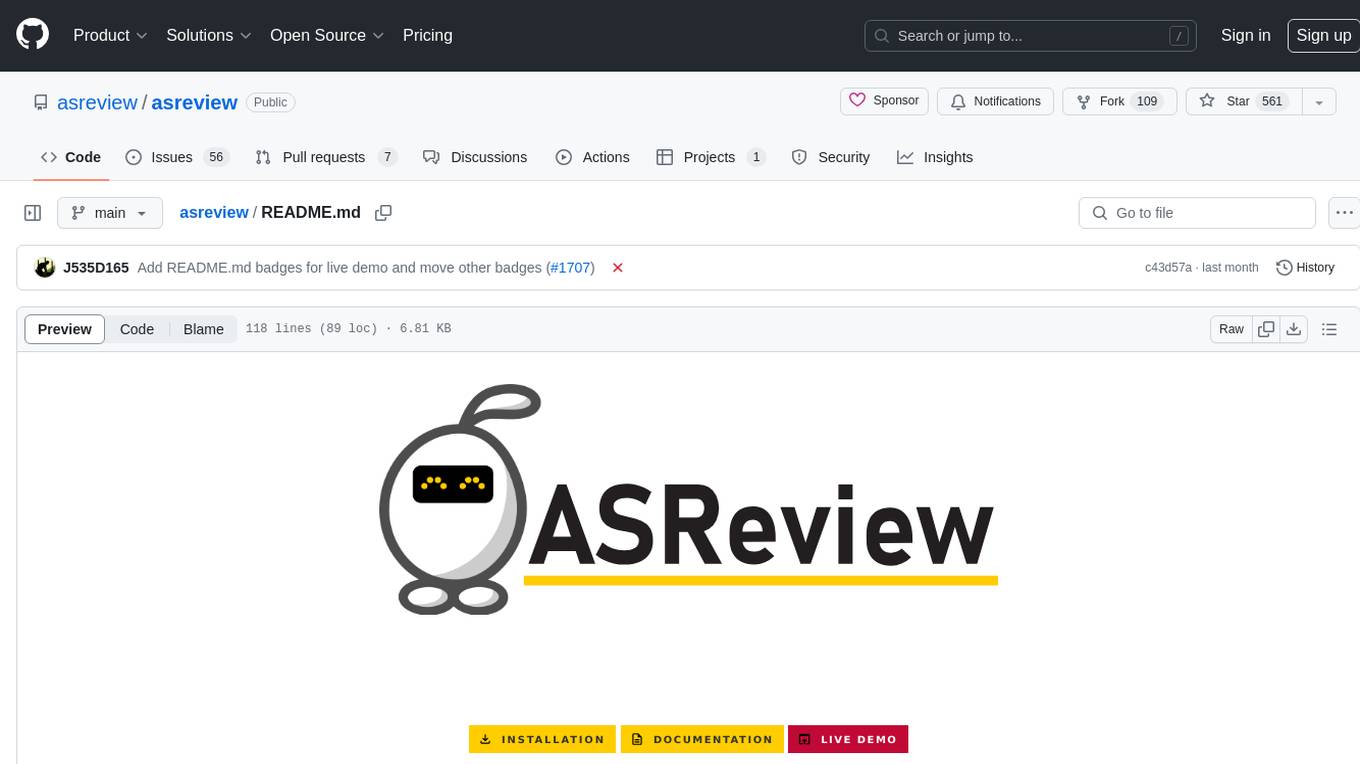
asreview
The ASReview project implements active learning for systematic reviews, utilizing AI-aided pipelines to assist in finding relevant texts for search tasks. It accelerates the screening of textual data with minimal human input, saving time and increasing output quality. The software offers three modes: Oracle for interactive screening, Exploration for teaching purposes, and Simulation for evaluating active learning models. ASReview LAB is designed to support decision-making in any discipline or industry by improving efficiency and transparency in screening large amounts of textual data.
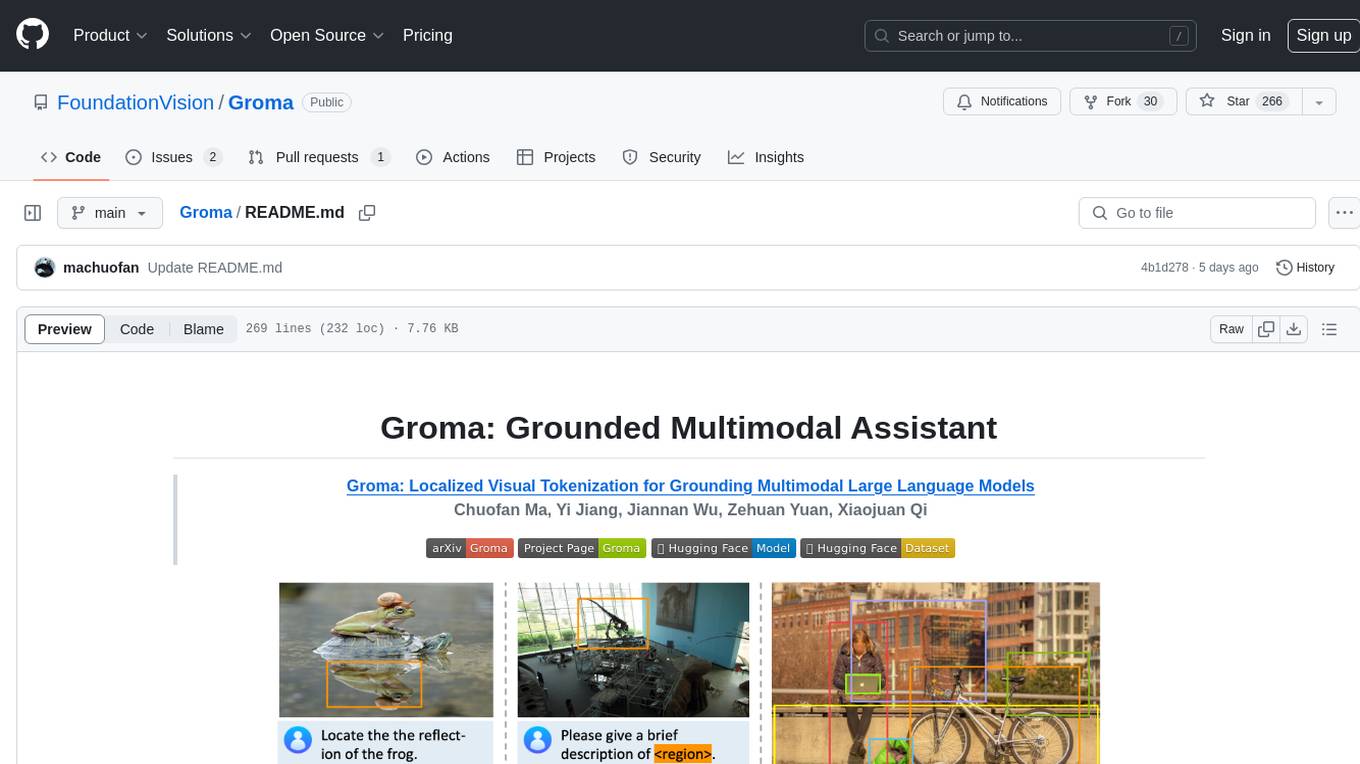
Groma
Groma is a grounded multimodal assistant that excels in region understanding and visual grounding. It can process user-defined region inputs and generate contextually grounded long-form responses. The tool presents a unique paradigm for multimodal large language models, focusing on visual tokenization for localization. Groma achieves state-of-the-art performance in referring expression comprehension benchmarks. The tool provides pretrained model weights and instructions for data preparation, training, inference, and evaluation. Users can customize training by starting from intermediate checkpoints. Groma is designed to handle tasks related to detection pretraining, alignment pretraining, instruction finetuning, instruction following, and more.
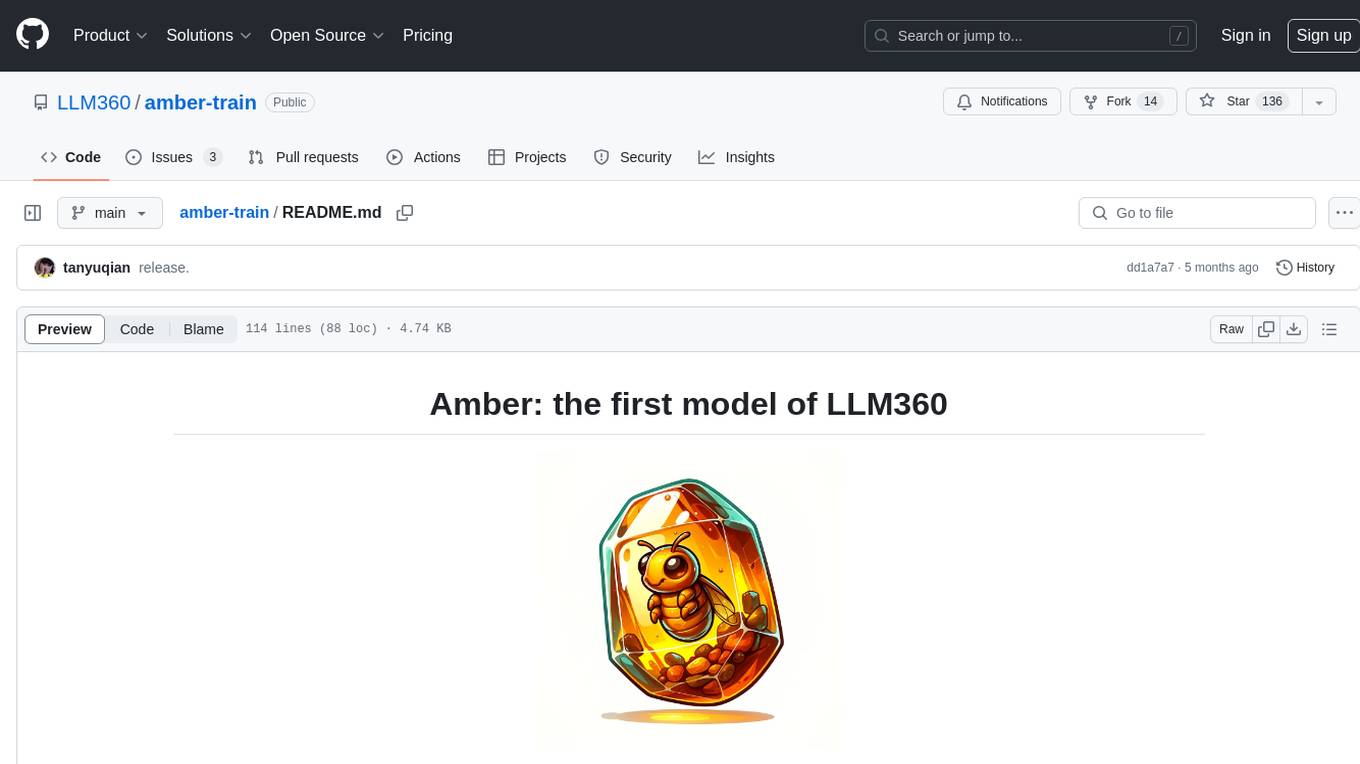
amber-train
Amber is the first model in the LLM360 family, an initiative for comprehensive and fully open-sourced LLMs. It is a 7B English language model with the LLaMA architecture. The model type is a language model with the same architecture as LLaMA-7B. It is licensed under Apache 2.0. The resources available include training code, data preparation, metrics, and fully processed Amber pretraining data. The model has been trained on various datasets like Arxiv, Book, C4, Refined-Web, StarCoder, StackExchange, and Wikipedia. The hyperparameters include a total of 6.7B parameters, hidden size of 4096, intermediate size of 11008, 32 attention heads, 32 hidden layers, RMSNorm ε of 1e^-6, max sequence length of 2048, and a vocabulary size of 32000.
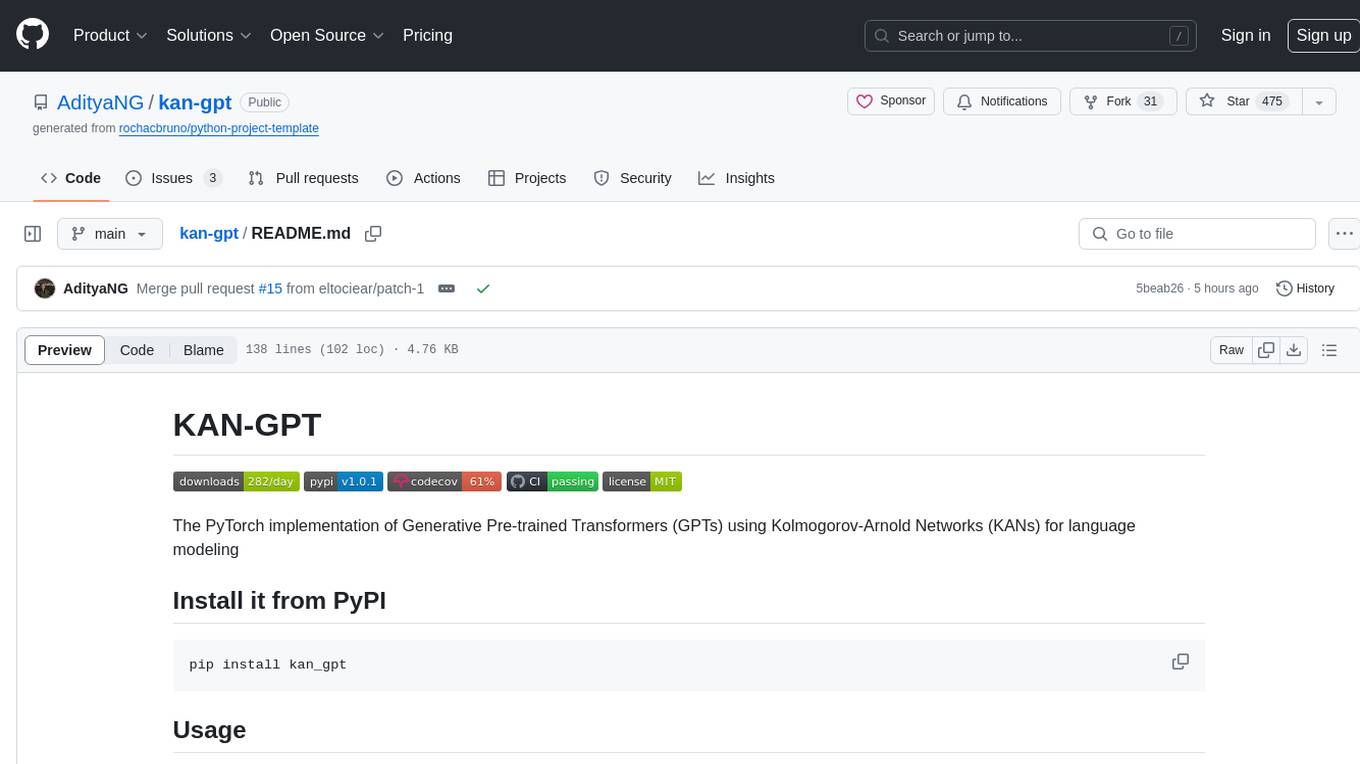
kan-gpt
The KAN-GPT repository is a PyTorch implementation of Generative Pre-trained Transformers (GPTs) using Kolmogorov-Arnold Networks (KANs) for language modeling. It provides a model for generating text based on prompts, with a focus on improving performance compared to traditional MLP-GPT models. The repository includes scripts for training the model, downloading datasets, and evaluating model performance. Development tasks include integrating with other libraries, testing, and documentation.
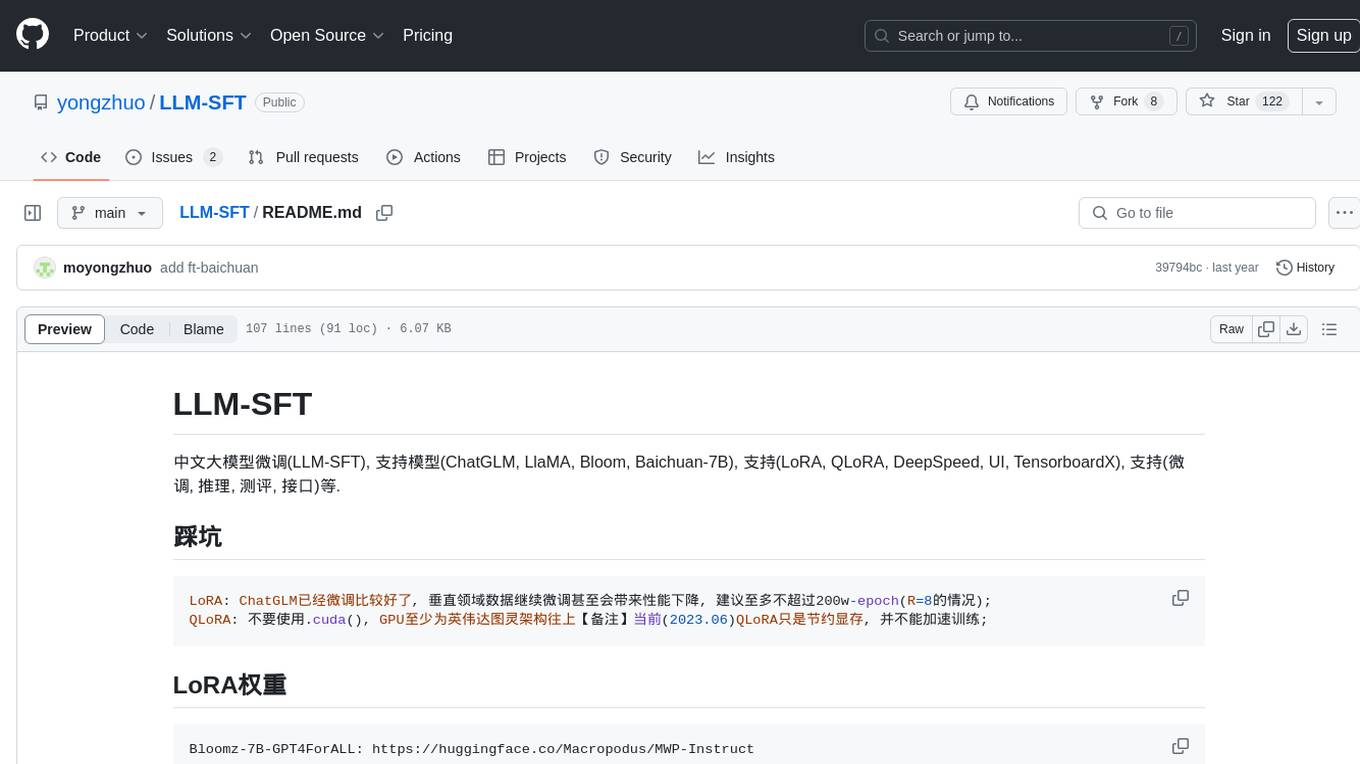
LLM-SFT
LLM-SFT is a Chinese large model fine-tuning tool that supports models such as ChatGLM, LlaMA, Bloom, Baichuan-7B, and frameworks like LoRA, QLoRA, DeepSpeed, UI, and TensorboardX. It facilitates tasks like fine-tuning, inference, evaluation, and API integration. The tool provides pre-trained weights for various models and datasets for Chinese language processing. It requires specific versions of libraries like transformers and torch for different functionalities.
For similar jobs
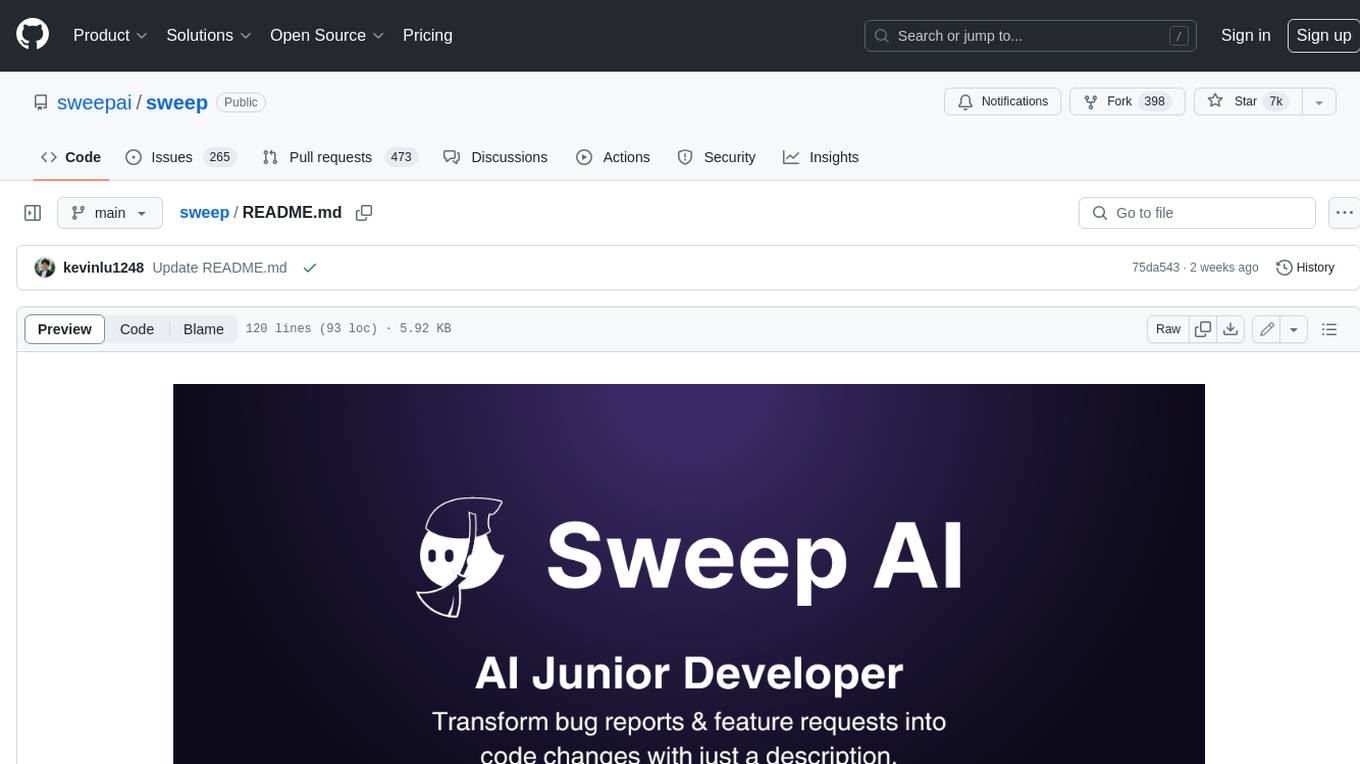
sweep
Sweep is an AI junior developer that turns bugs and feature requests into code changes. It automatically handles developer experience improvements like adding type hints and improving test coverage.
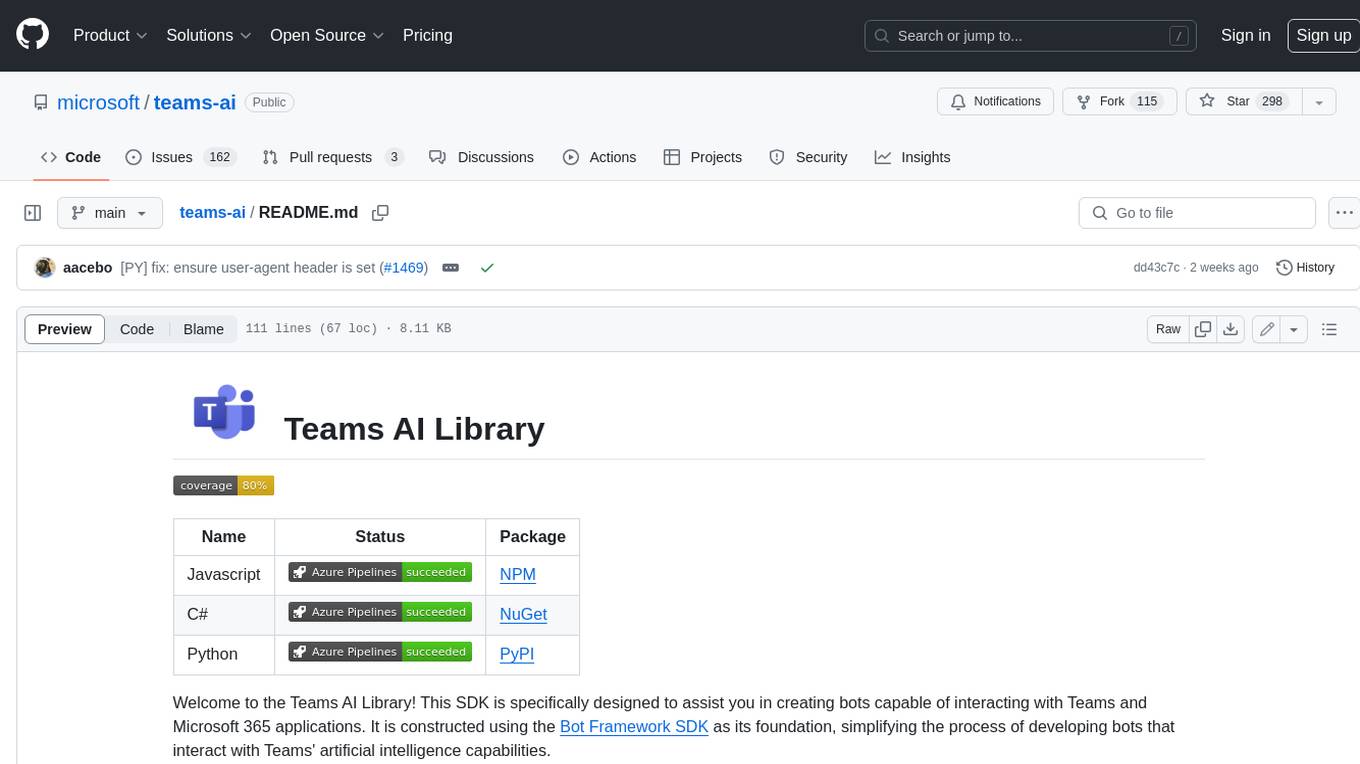
teams-ai
The Teams AI Library is a software development kit (SDK) that helps developers create bots that can interact with Teams and Microsoft 365 applications. It is built on top of the Bot Framework SDK and simplifies the process of developing bots that interact with Teams' artificial intelligence capabilities. The SDK is available for JavaScript/TypeScript, .NET, and Python.
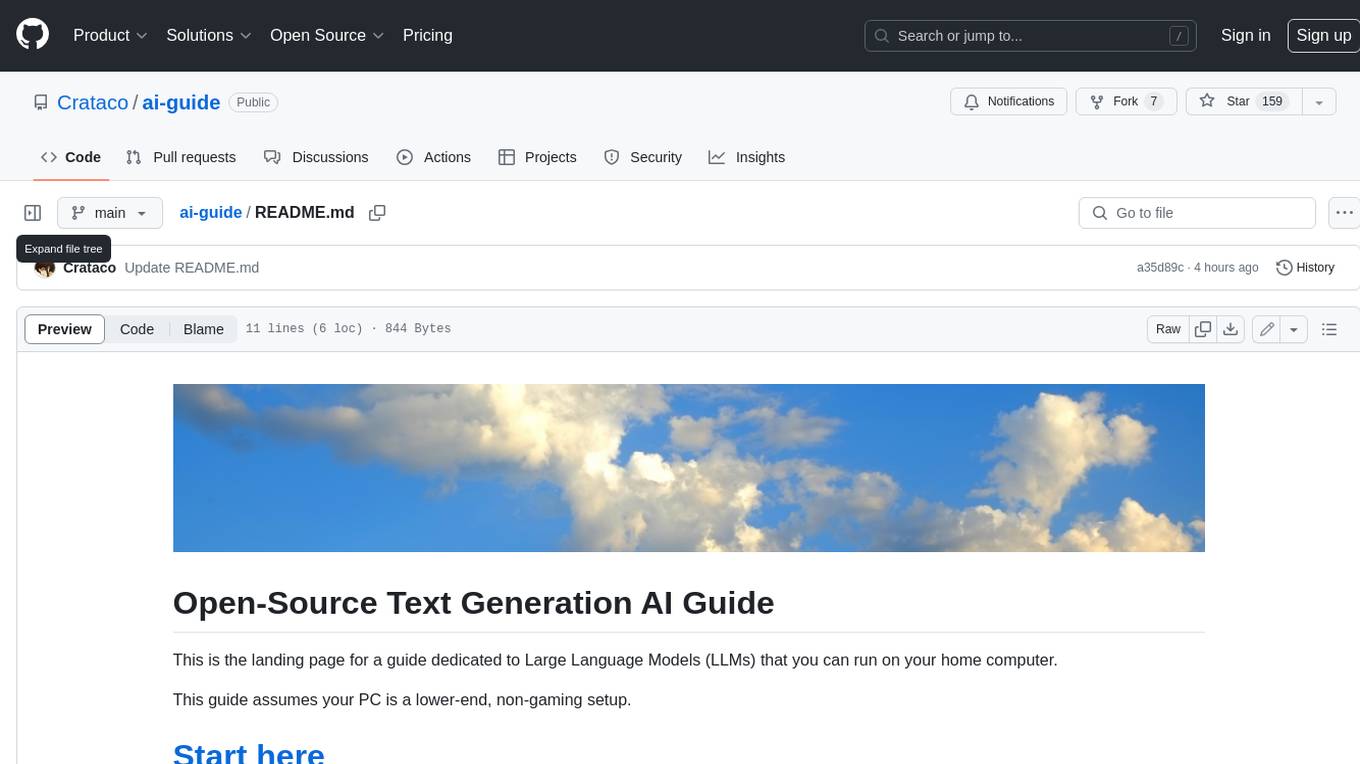
ai-guide
This guide is dedicated to Large Language Models (LLMs) that you can run on your home computer. It assumes your PC is a lower-end, non-gaming setup.
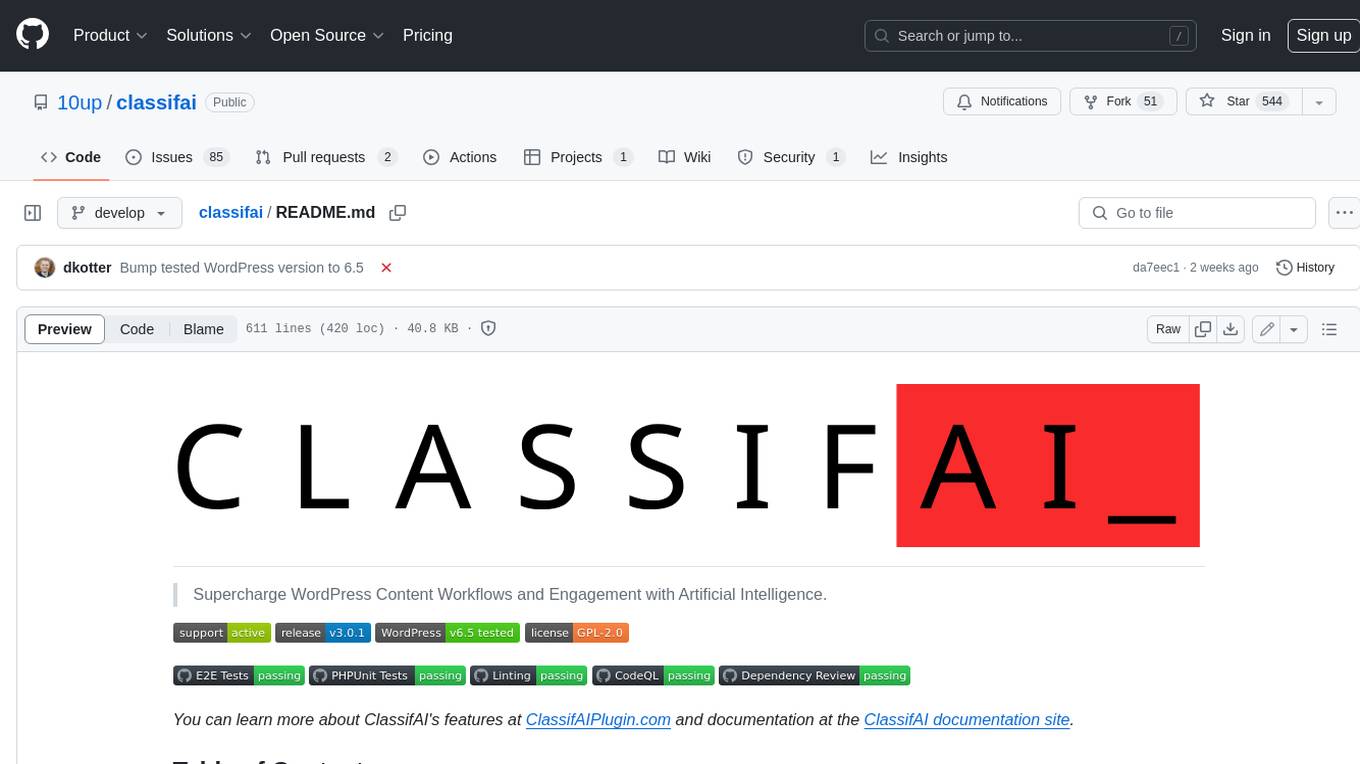
classifai
Supercharge WordPress Content Workflows and Engagement with Artificial Intelligence. Tap into leading cloud-based services like OpenAI, Microsoft Azure AI, Google Gemini and IBM Watson to augment your WordPress-powered websites. Publish content faster while improving SEO performance and increasing audience engagement. ClassifAI integrates Artificial Intelligence and Machine Learning technologies to lighten your workload and eliminate tedious tasks, giving you more time to create original content that matters.
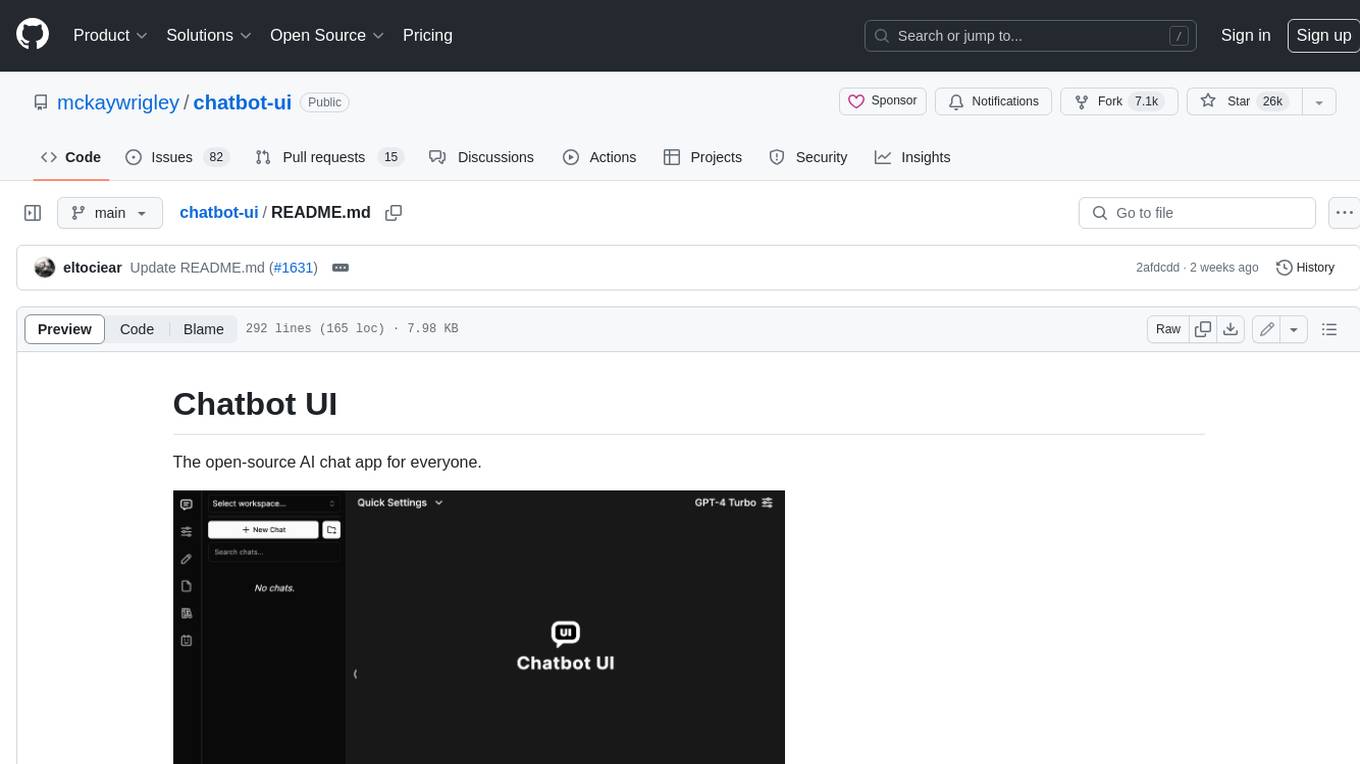
chatbot-ui
Chatbot UI is an open-source AI chat app that allows users to create and deploy their own AI chatbots. It is easy to use and can be customized to fit any need. Chatbot UI is perfect for businesses, developers, and anyone who wants to create a chatbot.
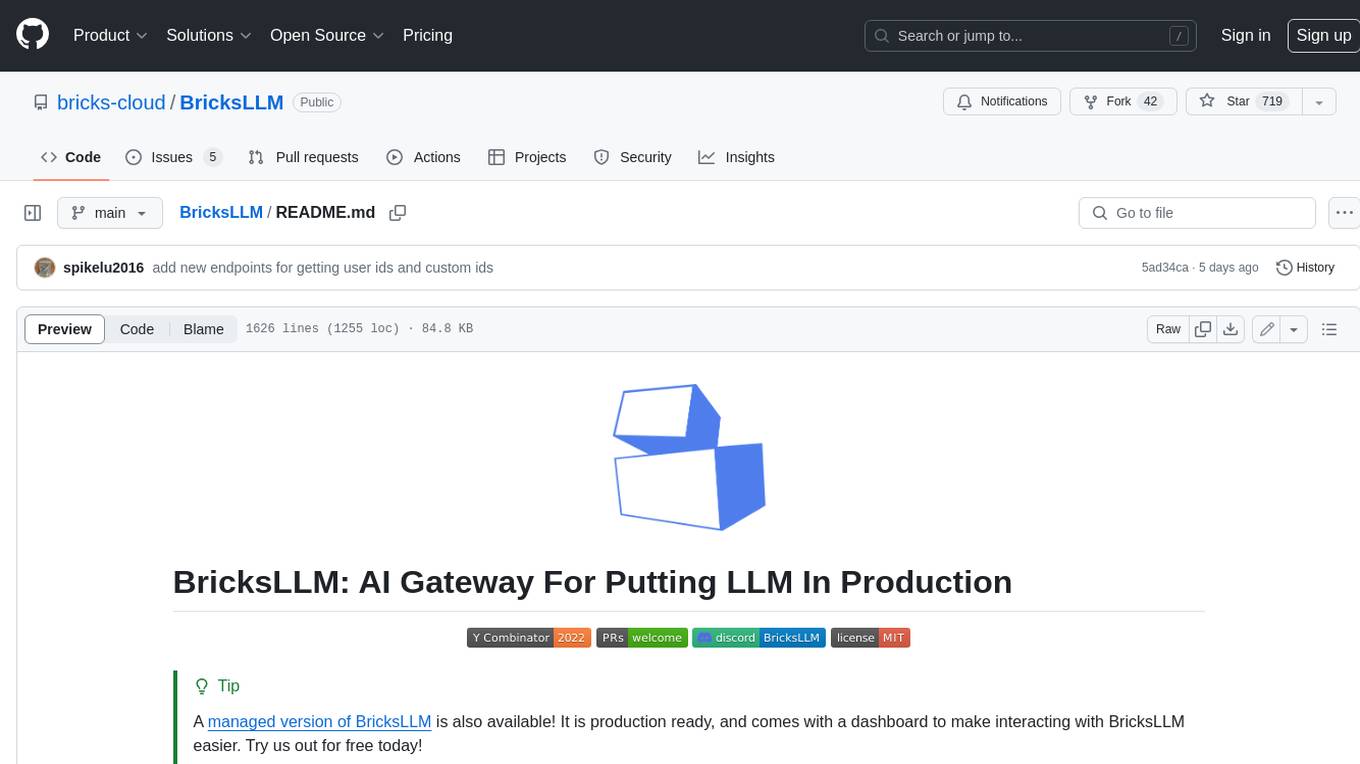
BricksLLM
BricksLLM is a cloud native AI gateway written in Go. Currently, it provides native support for OpenAI, Anthropic, Azure OpenAI and vLLM. BricksLLM aims to provide enterprise level infrastructure that can power any LLM production use cases. Here are some use cases for BricksLLM: * Set LLM usage limits for users on different pricing tiers * Track LLM usage on a per user and per organization basis * Block or redact requests containing PIIs * Improve LLM reliability with failovers, retries and caching * Distribute API keys with rate limits and cost limits for internal development/production use cases * Distribute API keys with rate limits and cost limits for students
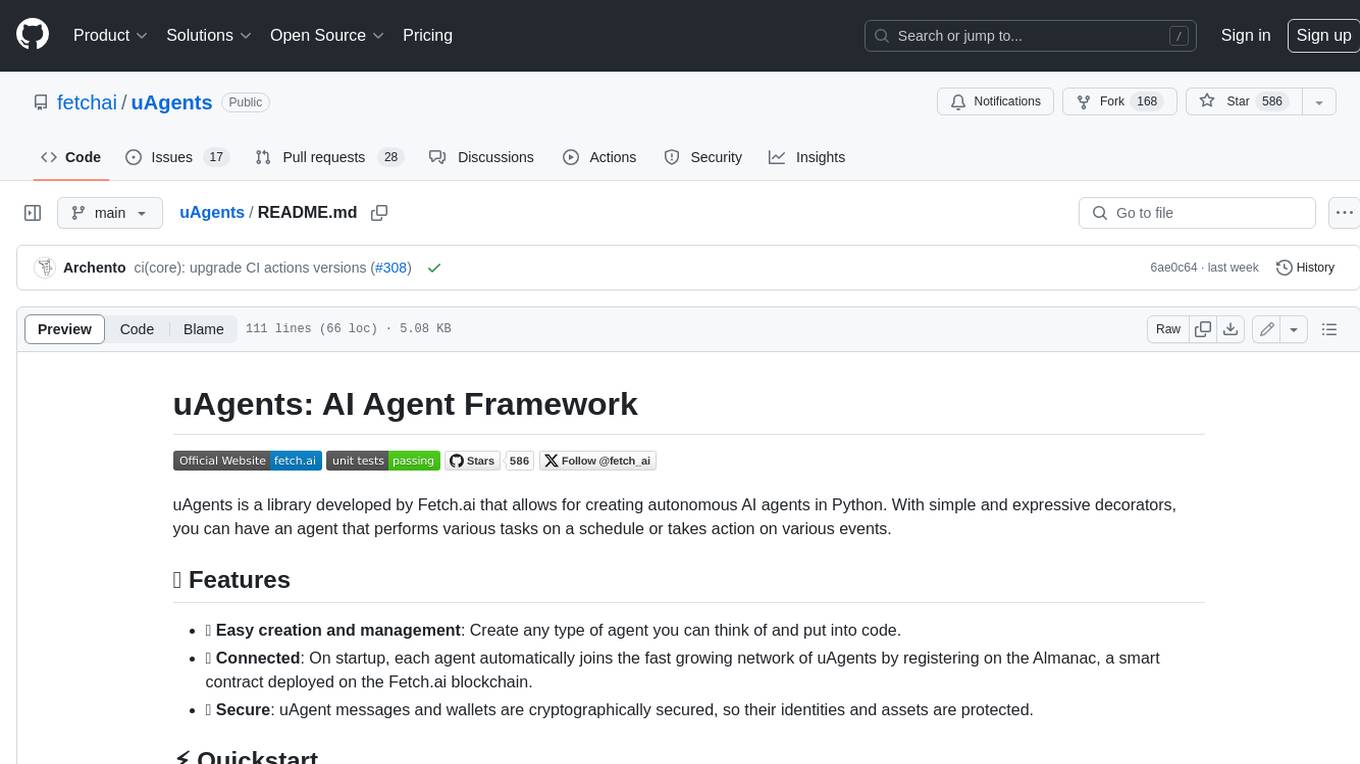
uAgents
uAgents is a Python library developed by Fetch.ai that allows for the creation of autonomous AI agents. These agents can perform various tasks on a schedule or take action on various events. uAgents are easy to create and manage, and they are connected to a fast-growing network of other uAgents. They are also secure, with cryptographically secured messages and wallets.
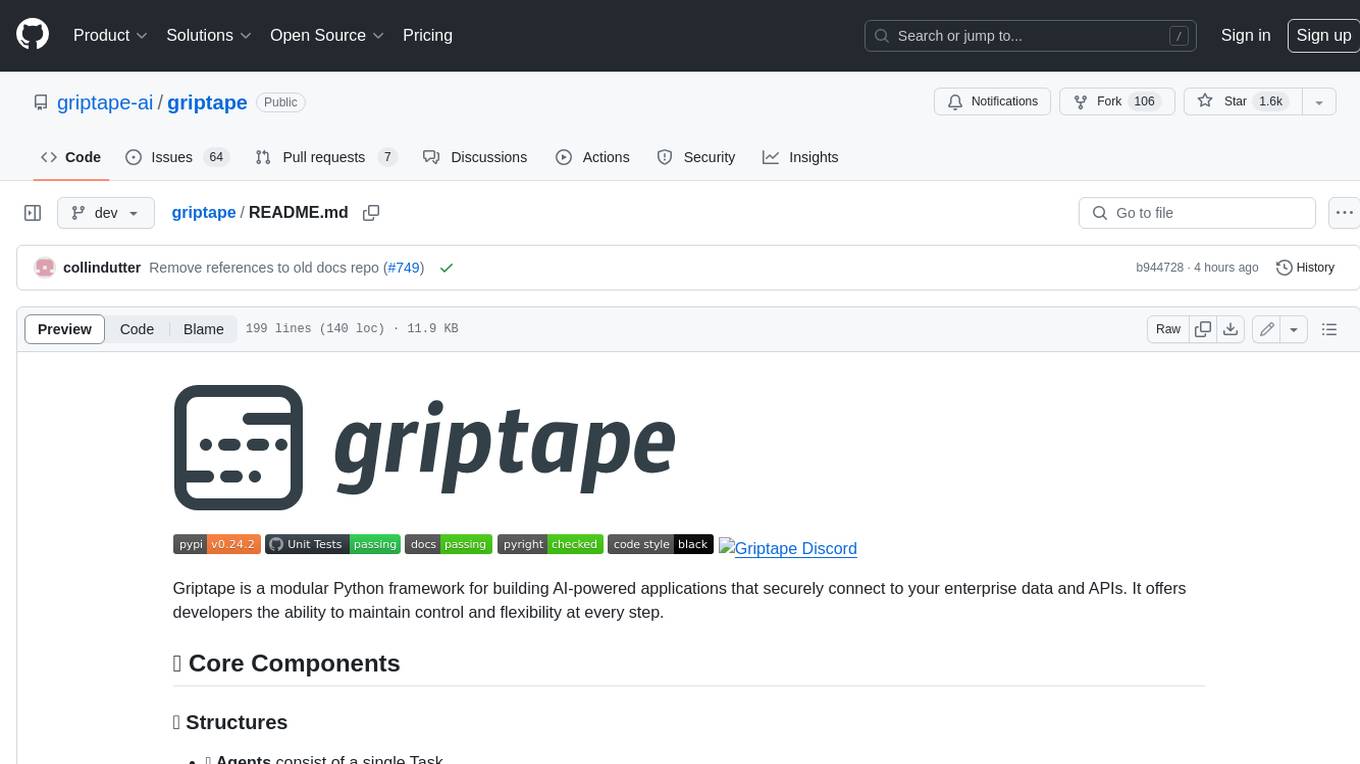
griptape
Griptape is a modular Python framework for building AI-powered applications that securely connect to your enterprise data and APIs. It offers developers the ability to maintain control and flexibility at every step. Griptape's core components include Structures (Agents, Pipelines, and Workflows), Tasks, Tools, Memory (Conversation Memory, Task Memory, and Meta Memory), Drivers (Prompt and Embedding Drivers, Vector Store Drivers, Image Generation Drivers, Image Query Drivers, SQL Drivers, Web Scraper Drivers, and Conversation Memory Drivers), Engines (Query Engines, Extraction Engines, Summary Engines, Image Generation Engines, and Image Query Engines), and additional components (Rulesets, Loaders, Artifacts, Chunkers, and Tokenizers). Griptape enables developers to create AI-powered applications with ease and efficiency.