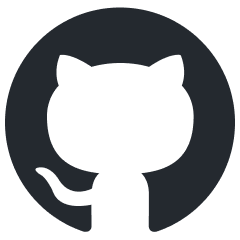
aiomisc
aiomisc - miscellaneous utils for asyncio
Stars: 388
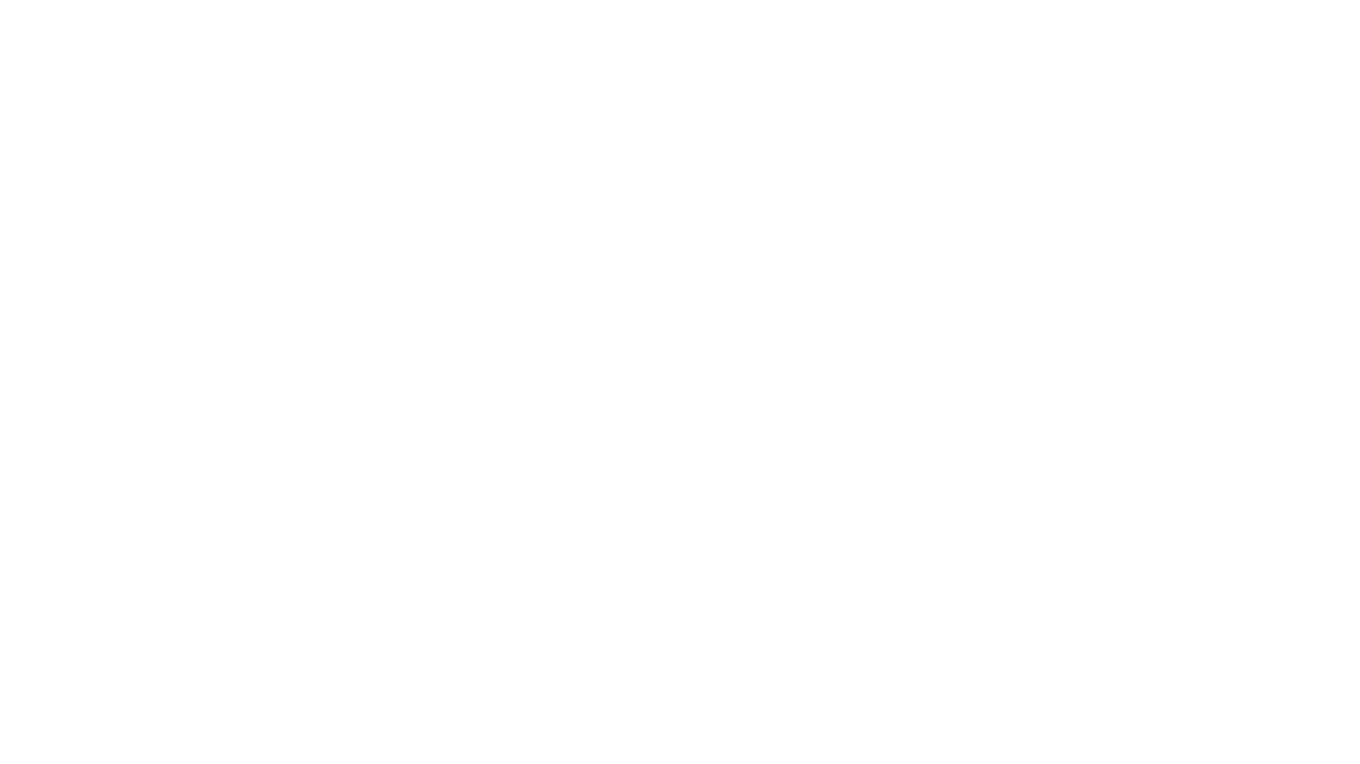
aiomisc is a Python library that provides a collection of utility functions and classes for working with asynchronous I/O in a more intuitive and efficient way. It offers features like worker pools, connection pools, circuit breaker pattern, and retry mechanisms to make asyncio code more robust and easier to maintain. The library simplifies the architecture of software using asynchronous I/O, making it easier for developers to write reliable and scalable asynchronous code.
README:
.. image:: https://coveralls.io/repos/github/aiokitchen/aiomisc/badge.svg?branch=master :target: https://coveralls.io/github/aiokitchen/aiomisc :alt: Coveralls
.. image:: https://github.com/aiokitchen/aiomisc/workflows/tox/badge.svg :target: https://github.com/aiokitchen/aiomisc/actions?query=workflow%3Atox :alt: Actions
.. image:: https://img.shields.io/pypi/v/aiomisc.svg :target: https://pypi.python.org/pypi/aiomisc/ :alt: Latest Version
.. image:: https://img.shields.io/pypi/wheel/aiomisc.svg :target: https://pypi.python.org/pypi/aiomisc/
.. image:: https://img.shields.io/pypi/pyversions/aiomisc.svg :target: https://pypi.python.org/pypi/aiomisc/
.. image:: https://img.shields.io/pypi/l/aiomisc.svg :target: https://pypi.python.org/pypi/aiomisc/
Miscellaneous utils for asyncio.
As a programmer, you are no stranger to the challenges that come with building and maintaining software applications. One area that can be particularly difficult is making architecture of the software that using asynchronous I/O.
This is where aiomisc comes in. aiomisc is a Python library that provides a
collection of utility functions and classes for working with asynchronous I/O
in a more intuitive and efficient way. It is built on top of the asyncio
library and is designed to make it easier for developers to write
asynchronous code that is both reliable and scalable.
With aiomisc, you can take advantage of powerful features like
worker pools
, connection pools
, circuit breaker pattern
,
and retry mechanisms such as asyncbackoff
and asyncretry
to make your
asyncio code more robust and easier to maintain. In this documentation,
we'll take a closer look at what aiomisc
has to offer and how it can
help you streamline your asyncio service development.
Installation is possible in standard ways, such as PyPI or installation from a git repository directly.
Installing from PyPI_:
.. code-block:: bash
pip3 install aiomisc
Installing from github.com:
.. code-block:: bash
# Using git tool
pip3 install git+https://github.com/aiokitchen/aiomisc.git
# Alternative way using http
pip3 install \
https://github.com/aiokitchen/aiomisc/archive/refs/heads/master.zip
The package contains several extras and you can install additional dependencies if you specify them in this way.
With uvloop_:
.. code-block:: bash
pip3 install "aiomisc[uvloop]"
With aiohttp_:
.. code-block:: bash
pip3 install "aiomisc[aiohttp]"
Complete table of extras bellow:
+-----------------------------------+------------------------------------------------+
| example | description |
+===================================+================================================+
| pip install aiomisc[aiohttp]
| For running aiohttp_ applications. |
+-----------------------------------+------------------------------------------------+
| pip install aiomisc[asgi]
| For running ASGI_ applications |
+-----------------------------------+------------------------------------------------+
| pip install aiomisc[carbon]
| Sending metrics to carbon_ (part of graphite_) |
+-----------------------------------+------------------------------------------------+
| pip install aiomisc[cron]
| use croniter_ for scheduling tasks |
+-----------------------------------+------------------------------------------------+
| pip install aiomisc[raven]
| Sending exceptions to sentry_ using raven_ |
+-----------------------------------+------------------------------------------------+
| pip install aiomisc[rich]
| You might using rich_ for logging |
+-----------------------------------+------------------------------------------------+
| pip install aiomisc[uvicorn]
| For running ASGI_ application using uvicorn_ |
+-----------------------------------+------------------------------------------------+
| pip install aiomisc[uvloop]
| use uvloop_ as a default event loop |
+-----------------------------------+------------------------------------------------+
.. _ASGI: https://asgi.readthedocs.io/en/latest/ .. _PyPI: https://pypi.org/ .. _aiohttp: https://pypi.org/project/aiohttp .. _carbon: https://pypi.org/project/carbon .. _croniter: https://pypi.org/project/croniter .. _graphite: http://graphiteapp.org .. _raven: https://pypi.org/project/raven .. _rich: https://pypi.org/project/rich .. _sentry: https://sentry.io/ .. _uvloop: https://pypi.org/project/uvloop .. _uvicorn: https://pypi.org/project/uvicorn
You can combine extras values by separating them with commas, for example:
.. code-block:: bash
pip3 install "aiomisc[aiohttp,cron,rich,uvloop]"
This section will cover how this library creates and uses the event loop and
creates services. Of course, you can't write about everything here, but you
can read about a lot in the Tutorial_ section, and you can
always refer to the Modules_ and API reference
_ sections for help.
Event-loop and entrypoint +++++++++++++++++++++++++
Let's look at this simple example first:
.. code-block:: python
import asyncio
import logging
import aiomisc
log = logging.getLogger(__name__)
async def main():
log.info('Starting')
await asyncio.sleep(3)
log.info('Exiting')
if __name__ == '__main__':
with aiomisc.entrypoint(log_level="info", log_format="color") as loop:
loop.run_until_complete(main())
This code declares an asynchronous main()
function that exits after
3 seconds. It would seem nothing interesting, but the whole point is in
the entrypoint
.
What does the entrypoint
do, it would seem not so much, it creates an
event-loop and transfers control to the user. However, under the hood, the
logger is configured in a separate thread, a pool of threads is created,
services are started, but more on that later and there are no services
in this example.
Alternatively, you can choose not to use an entrypoint, just create an event-loop and set this as a default event loop for current thread:
.. code-block:: python :name: test_index_get_loop
import asyncio
import aiomisc
# * Installs uvloop event loop is it's has been installed.
# * Creates and set `aiomisc.thread_pool.ThreadPoolExecutor`
# as a default executor
# * Sets just created event-loop as a current event-loop for this thread.
aiomisc.new_event_loop()
async def main():
await asyncio.sleep(1)
if __name__ == '__main__':
loop = asyncio.get_event_loop()
loop.run_until_complete(main())
The example above is useful if your code is already using an implicitly created
event loop, you will have to modify less code, just add
aiomisc.new_event_loop()
and all calls to asyncio.get_event_loop()
will return the created instance.
However, you can do with one call. Following example closes implicitly created asyncio event loop and install a new one:
.. code-block:: python :name: test_index_new_loop
import asyncio
import aiomisc
async def main():
await asyncio.sleep(3)
if __name__ == '__main__':
loop = aiomisc.new_event_loop()
loop.run_until_complete(main())
Services ++++++++
The main thing that an entrypoint
does is start and gracefully
stop services.
The service concept within this library means a class derived from
the aiosmic.Service
class and implementing the
async def start(self) -> None:
method and optionally the
async def stop(self, exc: Optional[ Exception]) -> None
method.
The concept of stopping a service is not necessarily is pressing Ctrl+C
keys by user, it's actually just exiting the entrypoint
context manager.
The example below shows what your service might look like:
.. code-block:: python
from aiomisc import entrypoint, Service
class MyService(Service):
async def start(self):
do_something_when_start()
async def stop(self, exc):
do_graceful_shutdown()
with entrypoint(MyService()) as loop:
loop.run_forever()
The entry point can start as many instances of the service as it likes, and all of them will start concurrently.
There is also a way if the start
method is a payload for a service,
and then there is no need to implement the stop method, since the running
task with the start
function will be canceled at the stop stage.
But in this case, you will have to notify the entrypoint
that the
initialization of the service instance is complete and it can continue.
Like this:
.. code-block:: python
import asyncio
from threading import Event
from aiomisc import entrypoint, Service
event = Event()
class MyService(Service):
async def start(self):
# Send signal to entrypoint for continue running
self.start_event.set()
await asyncio.sleep(3600)
with entrypoint(MyService()) as loop:
assert event.is_set()
.. note::
The ``entrypoint`` passes control to the body of the context manager only
after all service instances have started. As mentioned above, a start is
considered to be the completion of the ``start`` method or the setting of
an start event with ``self.start_event.set()``.
The whole power of this library is in the set of already implemented or
abstract services.
Such as: AIOHTTPService
, ASGIService
, TCPServer
,
UDPServer
, TCPClient
, PeriodicService
, CronService
and so on.
Unfortunately in this section it is not possible to pay more attention to this,
please pay attention to the Tutorial_ section section, there are more
examples and explanations, and of cource you always can find out an answer on
the /api/index
or in the source code. The authors have tried to make
the source code as clear and simple as possible, so feel free to explore it.
This software follows Semantic Versioning
_
Summary: it's given a version number MAJOR.MINOR.PATCH, increment the:
- MAJOR version when you make incompatible API changes
- MINOR version when you add functionality in a backwards compatible manner
- PATCH version when you make backwards compatible bug fixes
- Additional labels for pre-release and build metadata are available as extensions to the MAJOR.MINOR.PATCH format.
In this case, the package version is assigned automatically with poem-plugins_, it using on the tag in the repository as a major and minor and the counter, which takes the number of commits between tag to the head of branch.
.. _poem-plugins: https://pypi.org/project/poem-plugins
Summary: it's given a version number MAJOR.MINOR.PATCH, increment the:
- MAJOR version when you make incompatible API changes
- MINOR version when you add functionality in a backwards compatible manner
- PATCH version when you make backwards compatible bug fixes
- Additional labels for pre-release and build metadata are available as extensions to the MAJOR.MINOR.PATCH format.
In this case, the package version is assigned automatically with poem-plugins_, it using on the tag in the repository as a major and minor and the counter, which takes the number of commits between tag to the head of branch.
.. _poem-plugins: https://pypi.org/project/poem-plugins
This project, like most open source projects, is developed by enthusiasts, you can join the development, submit issues, or send your merge requests.
In order to start developing in this repository, you need to do the following things.
Should be installed:
- Python 3.7+ as
python3
- Installed Poetry_ as
poetry
.. _Poetry: https://python-poetry.org/docs/
For setting up developer environment just execute:
.. code-block::
# installing all dependencies
poetry install
# setting up pre-commit hooks
poetry run pre-commit install
# adding poem-plugins to the poetry
poetry self add poem-plugins
.. _Semantic Versioning: http://semver.org/
.. _API reference: https://docs.aiomisc.com/api/index.html .. _Modules: https://docs.aiomisc.com/modules.html .. _Tutorial: https://docs.aiomisc.com/tutorial.html
For Tasks:
Click tags to check more tools for each tasksFor Jobs:
Alternative AI tools for aiomisc
Similar Open Source Tools
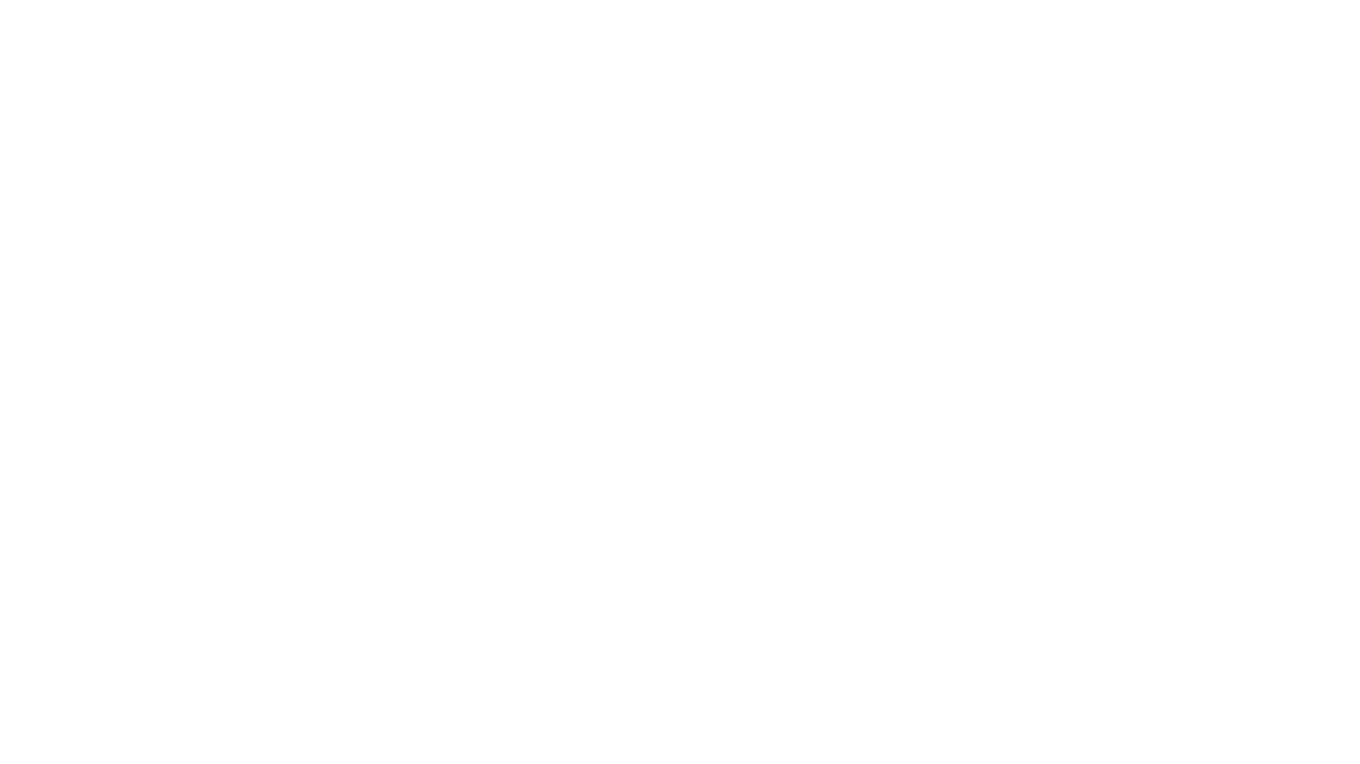
aiomisc
aiomisc is a Python library that provides a collection of utility functions and classes for working with asynchronous I/O in a more intuitive and efficient way. It offers features like worker pools, connection pools, circuit breaker pattern, and retry mechanisms to make asyncio code more robust and easier to maintain. The library simplifies the architecture of software using asynchronous I/O, making it easier for developers to write reliable and scalable asynchronous code.
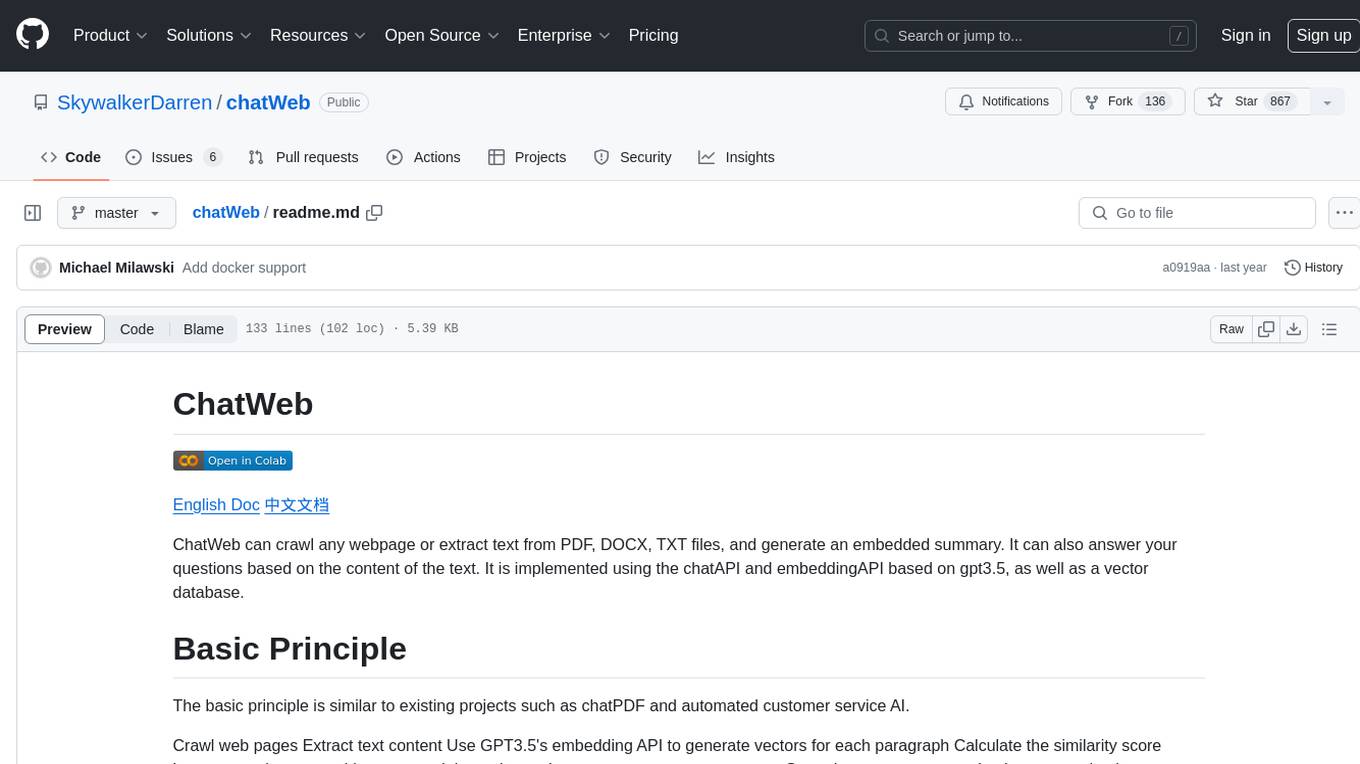
chatWeb
ChatWeb is a tool that can crawl web pages, extract text from PDF, DOCX, TXT files, and generate an embedded summary. It can answer questions based on text content using chatAPI and embeddingAPI based on GPT3.5. The tool calculates similarity scores between text vectors to generate summaries, performs nearest neighbor searches, and designs prompts to answer user questions. It aims to extract relevant content from text and provide accurate search results based on keywords. ChatWeb supports various modes, languages, and settings, including temperature control and PostgreSQL integration.
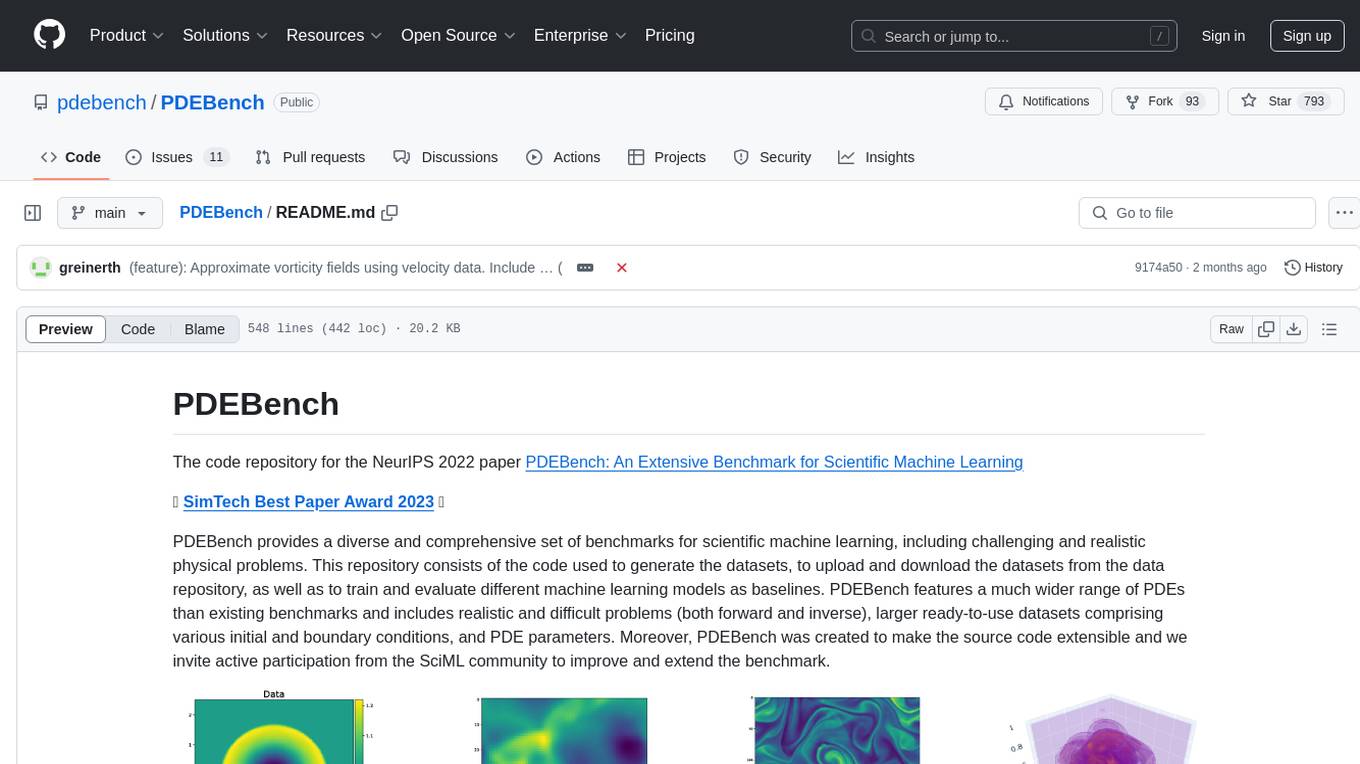
PDEBench
PDEBench provides a diverse and comprehensive set of benchmarks for scientific machine learning, including challenging and realistic physical problems. The repository consists of code for generating datasets, uploading and downloading datasets, training and evaluating machine learning models as baselines. It features a wide range of PDEs, realistic and difficult problems, ready-to-use datasets with various conditions and parameters. PDEBench aims for extensibility and invites participation from the SciML community to improve and extend the benchmark.
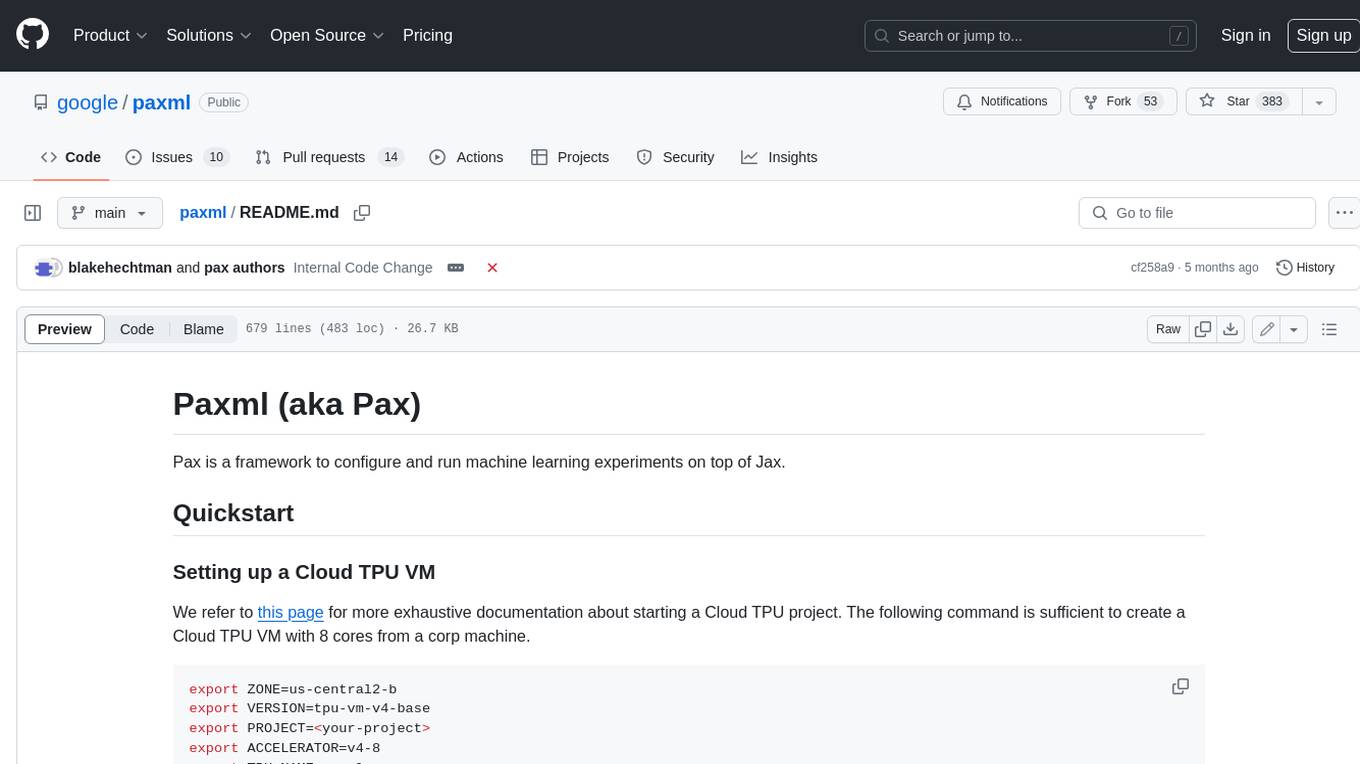
paxml
Pax is a framework to configure and run machine learning experiments on top of Jax.
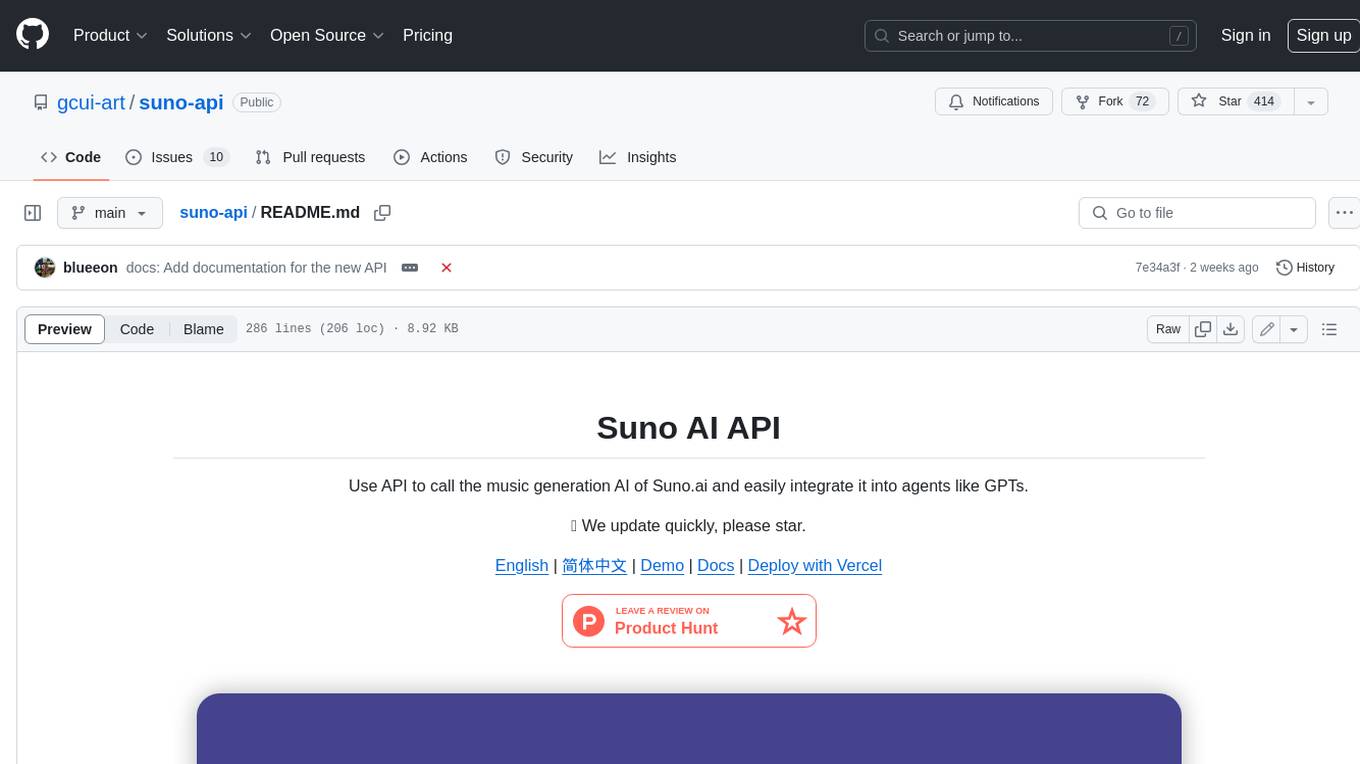
suno-api
Suno AI API is an open-source project that allows developers to integrate the music generation capabilities of Suno.ai into their own applications. The API provides a simple and convenient way to generate music, lyrics, and other audio content using Suno.ai's powerful AI models. With Suno AI API, developers can easily add music generation functionality to their apps, websites, and other projects.
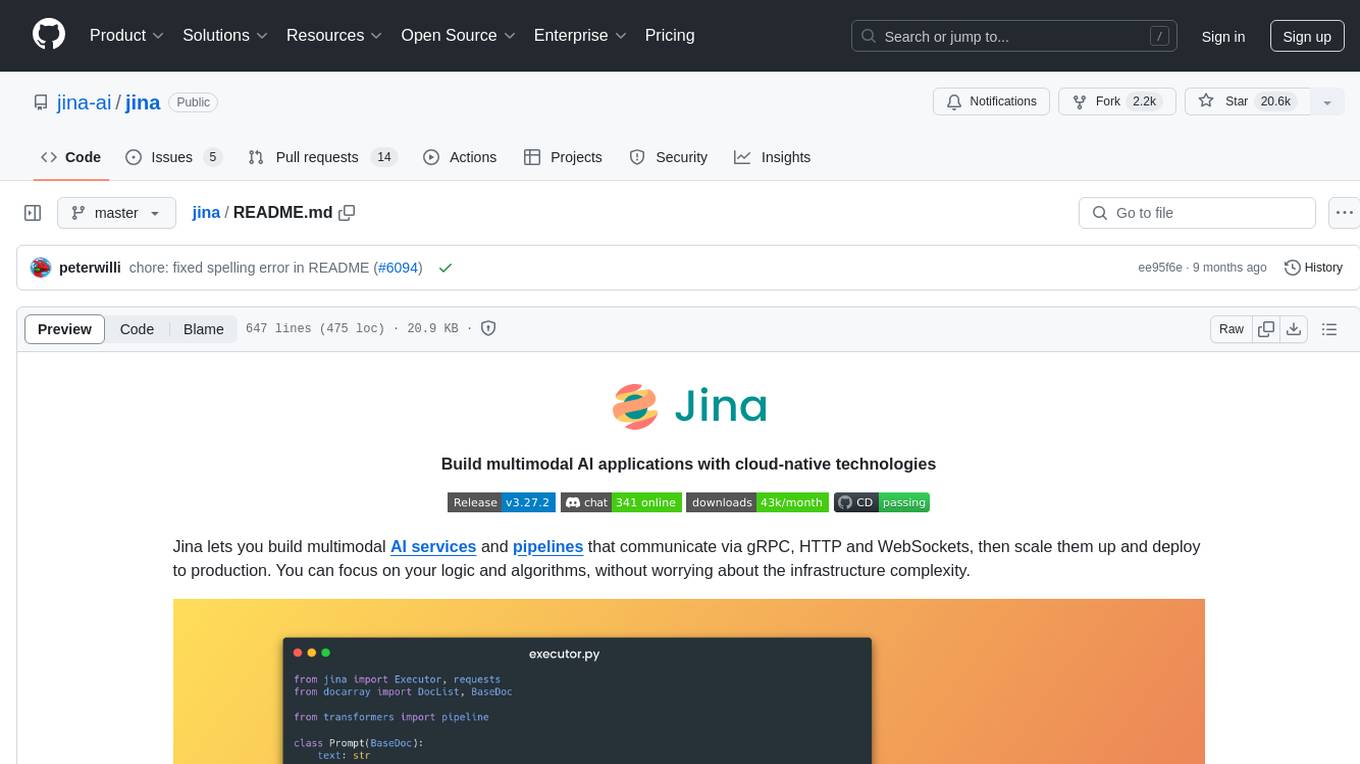
jina
Jina is a tool that allows users to build multimodal AI services and pipelines using cloud-native technologies. It provides a Pythonic experience for serving ML models and transitioning from local deployment to advanced orchestration frameworks like Docker-Compose, Kubernetes, or Jina AI Cloud. Users can build and serve models for any data type and deep learning framework, design high-performance services with easy scaling, serve LLM models while streaming their output, integrate with Docker containers via Executor Hub, and host on CPU/GPU using Jina AI Cloud. Jina also offers advanced orchestration and scaling capabilities, a smooth transition to the cloud, and easy scalability and concurrency features for applications. Users can deploy to their own cloud or system with Kubernetes and Docker Compose integration, and even deploy to JCloud for autoscaling and monitoring.
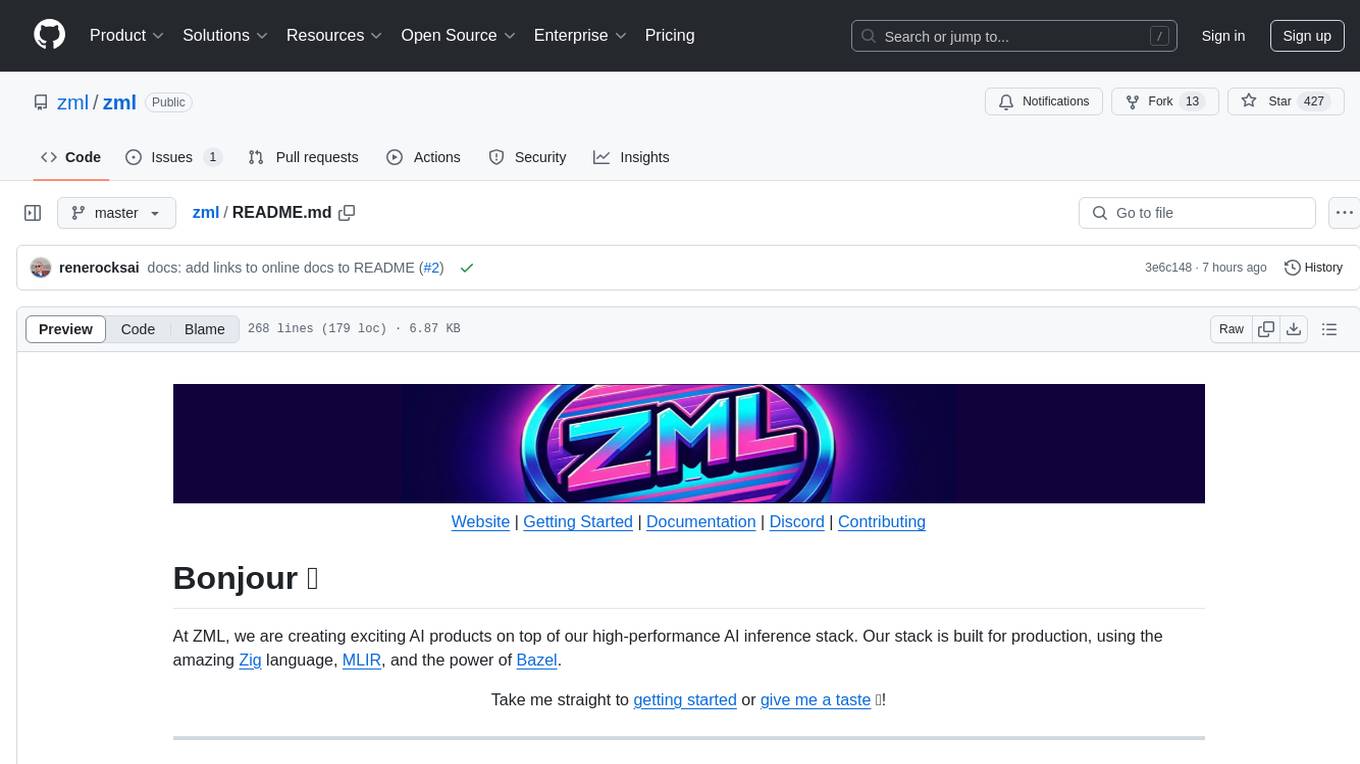
zml
ZML is a high-performance AI inference stack built for production, using Zig language, MLIR, and Bazel. It allows users to create exciting AI projects, run pre-packaged models like MNIST, TinyLlama, OpenLLama, and Meta Llama, and compile models for accelerator runtimes. Users can also run tests, explore examples, and contribute to the project. ZML is licensed under the Apache 2.0 license.
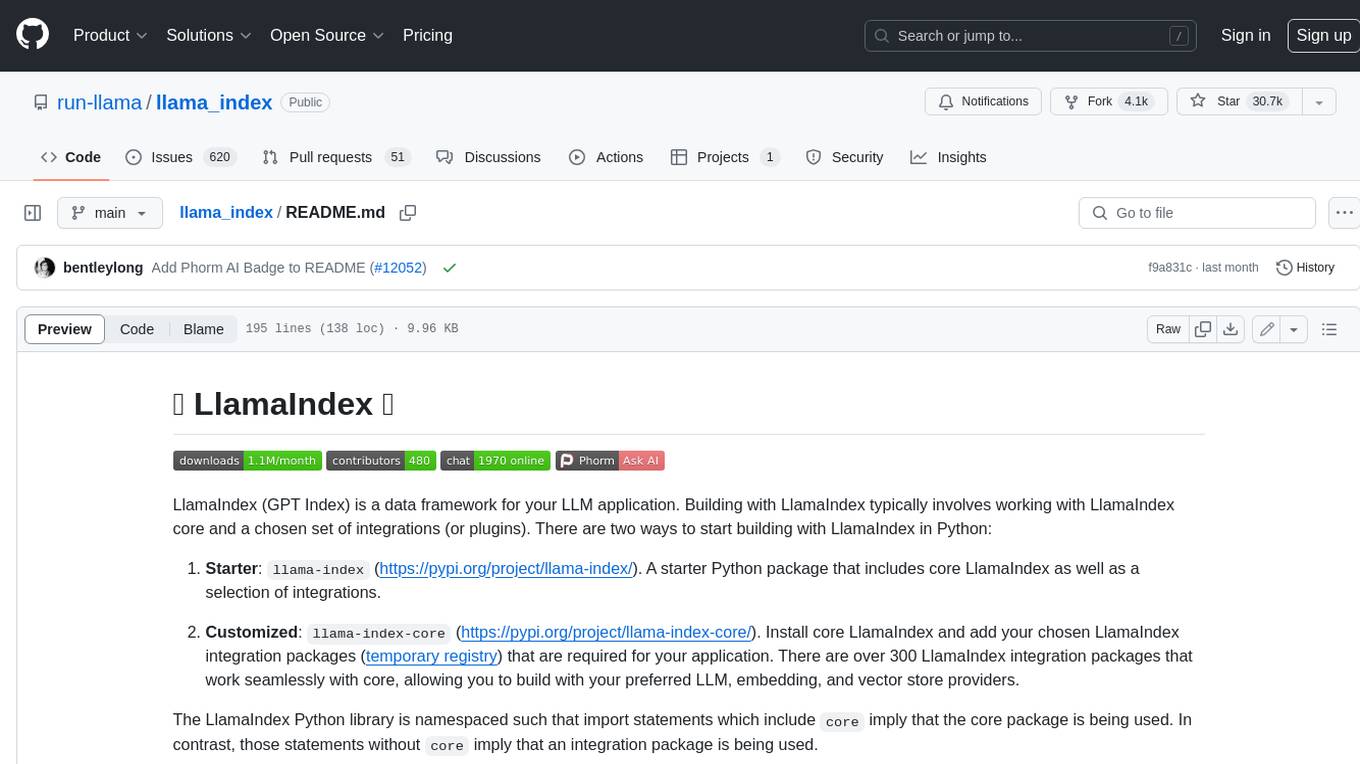
llama_index
LlamaIndex is a data framework for building LLM applications. It provides tools for ingesting, structuring, and querying data, as well as integrating with LLMs and other tools. LlamaIndex is designed to be easy to use for both beginner and advanced users, and it provides a comprehensive set of features for building LLM applications.
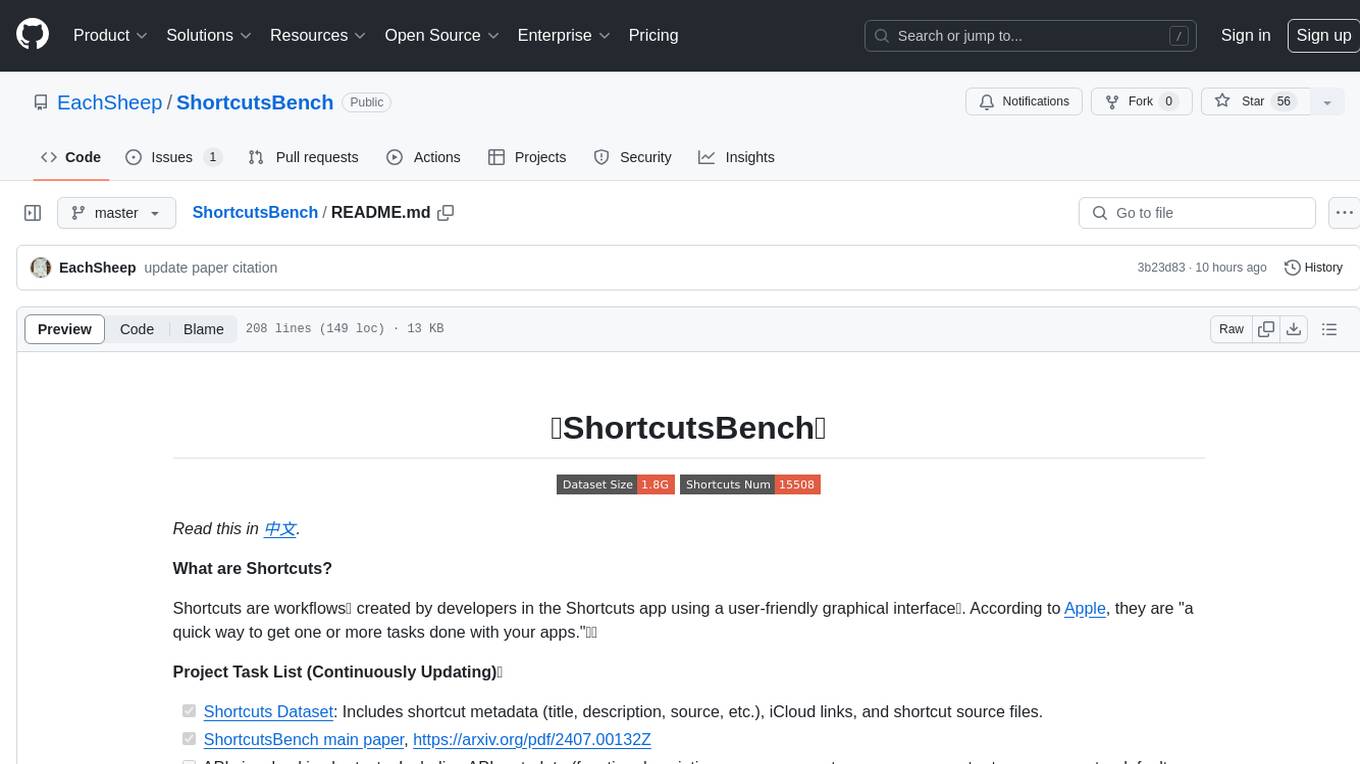
ShortcutsBench
ShortcutsBench is a project focused on collecting and analyzing workflows created in the Shortcuts app, providing a dataset of shortcut metadata, source files, and API information. It aims to study the integration of large language models with Apple devices, particularly focusing on the role of shortcuts in enhancing user experience. The project offers insights for Shortcuts users, enthusiasts, and researchers to explore, customize workflows, and study automated workflows, low-code programming, and API-based agents.
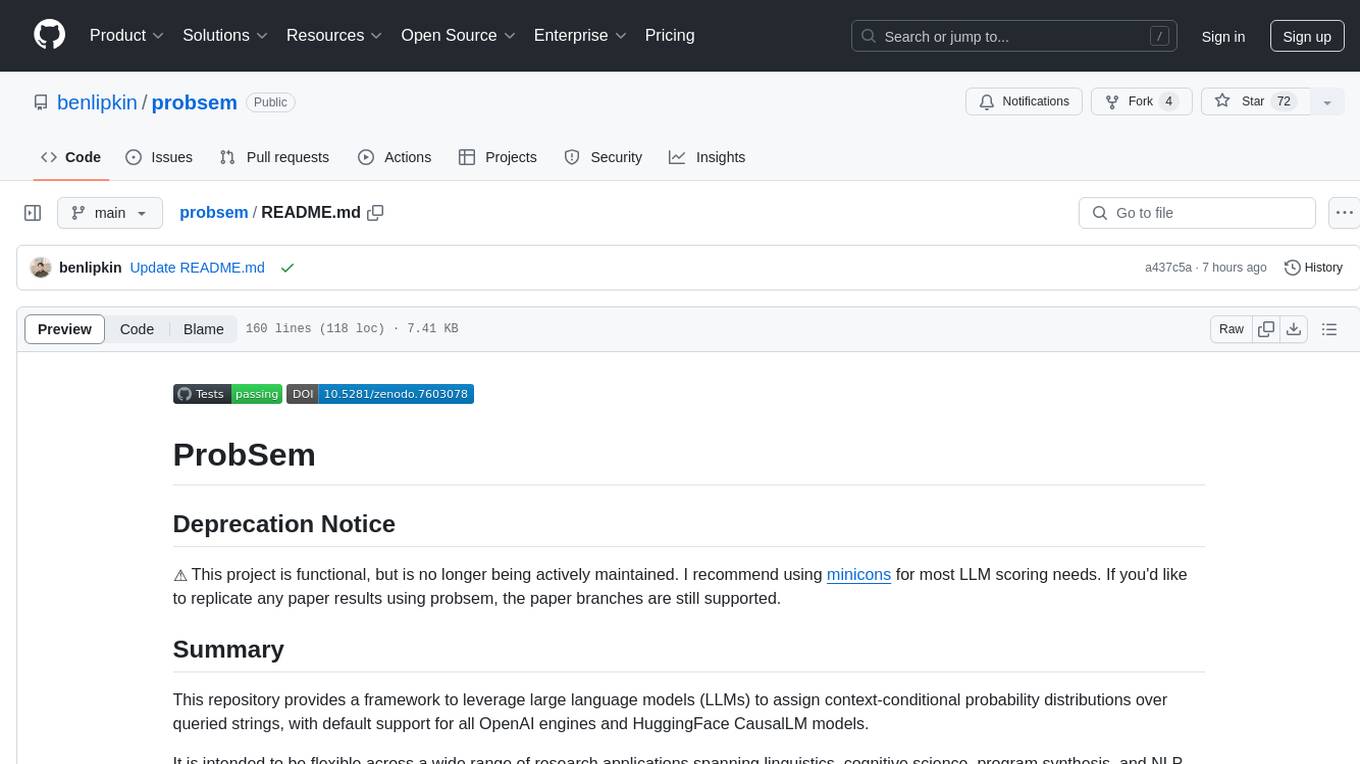
probsem
ProbSem is a repository that provides a framework to leverage large language models (LLMs) for assigning context-conditional probability distributions over queried strings. It supports OpenAI engines and HuggingFace CausalLM models, and is flexible for research applications in linguistics, cognitive science, program synthesis, and NLP. Users can define prompts, contexts, and queries to derive probability distributions over possible completions, enabling tasks like cloze completion, multiple-choice QA, semantic parsing, and code completion. The repository offers CLI and API interfaces for evaluation, with options to customize models, normalize scores, and adjust temperature for probability distributions.
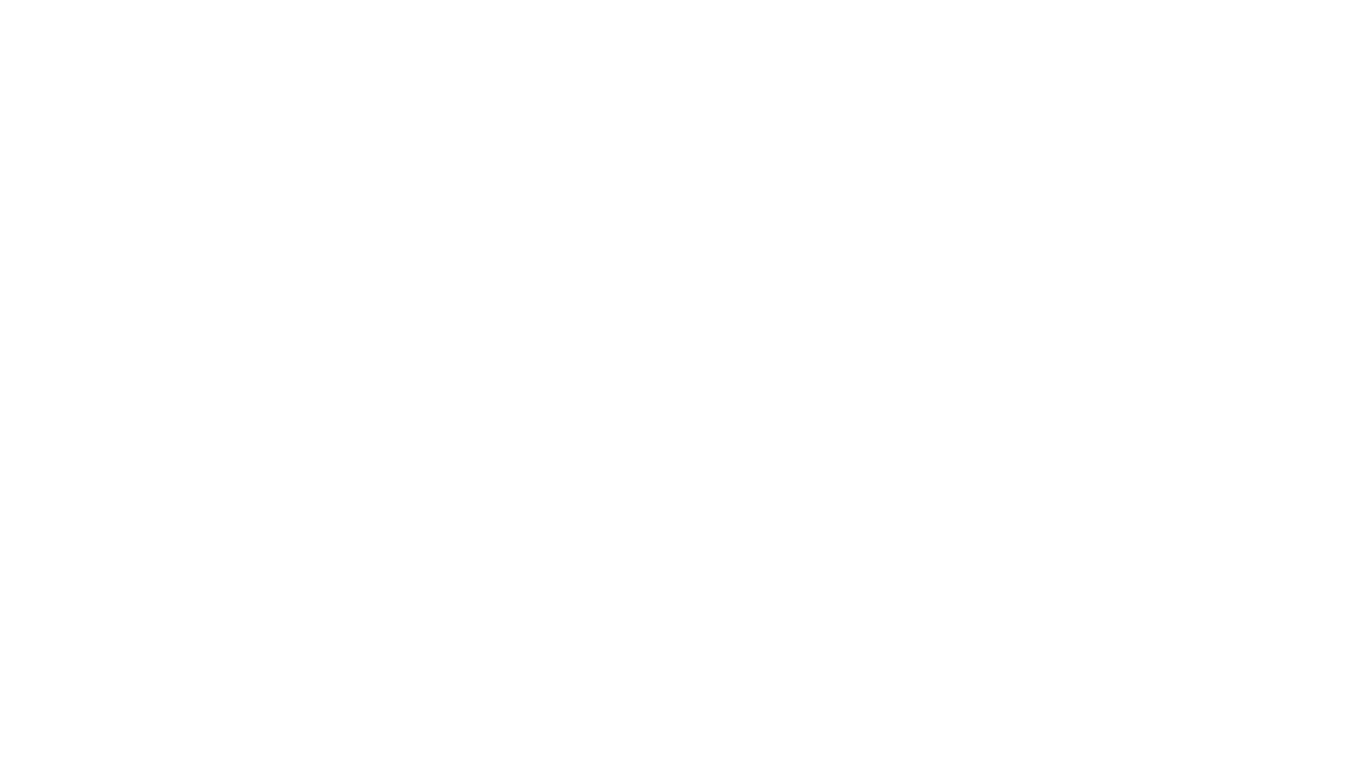
upgini
Upgini is an intelligent data search engine with a Python library that helps users find and add relevant features to their ML pipeline from various public, community, and premium external data sources. It automates the optimization of connected data sources by generating an optimal set of machine learning features using large language models, GraphNNs, and recurrent neural networks. The tool aims to simplify feature search and enrichment for external data to make it a standard approach in machine learning pipelines. It democratizes access to data sources for the data science community.
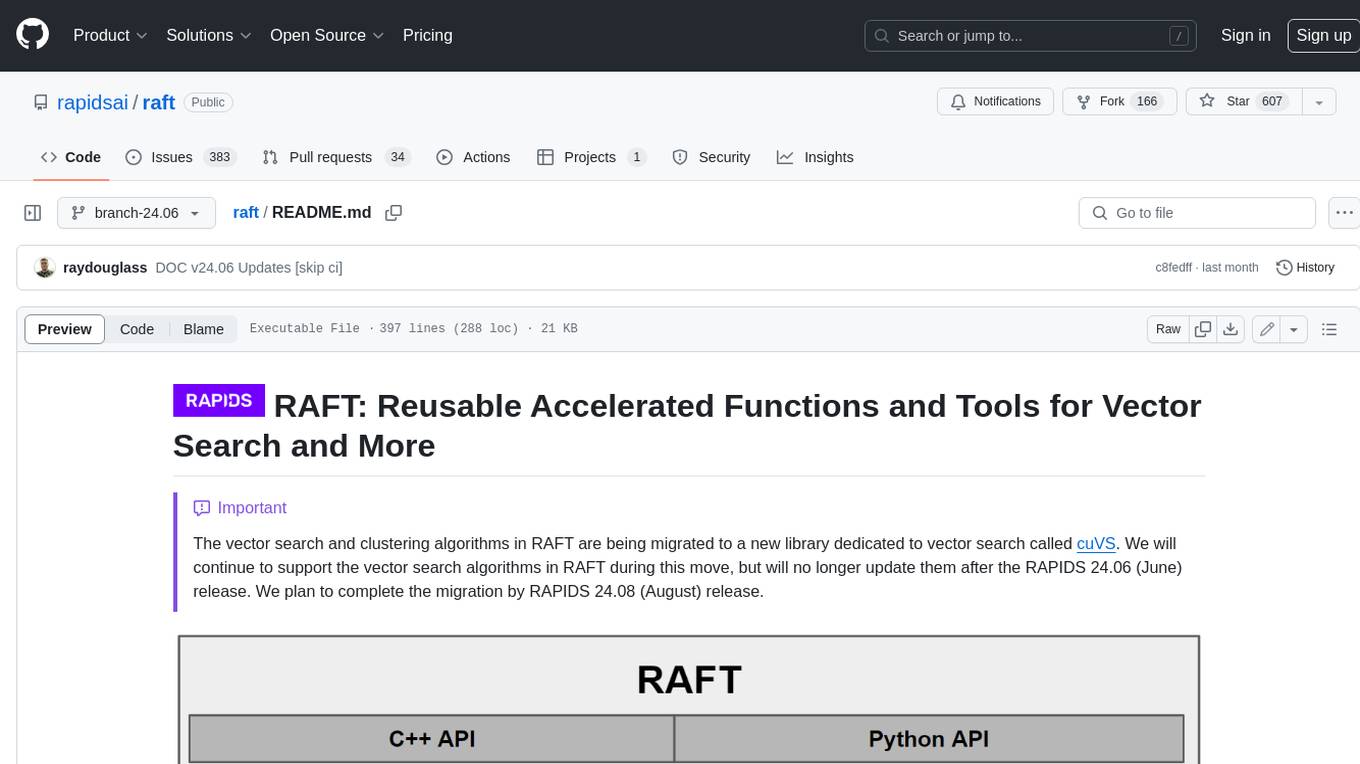
raft
RAFT (Reusable Accelerated Functions and Tools) is a C++ header-only template library with an optional shared library that contains fundamental widely-used algorithms and primitives for machine learning and information retrieval. The algorithms are CUDA-accelerated and form building blocks for more easily writing high performance applications.
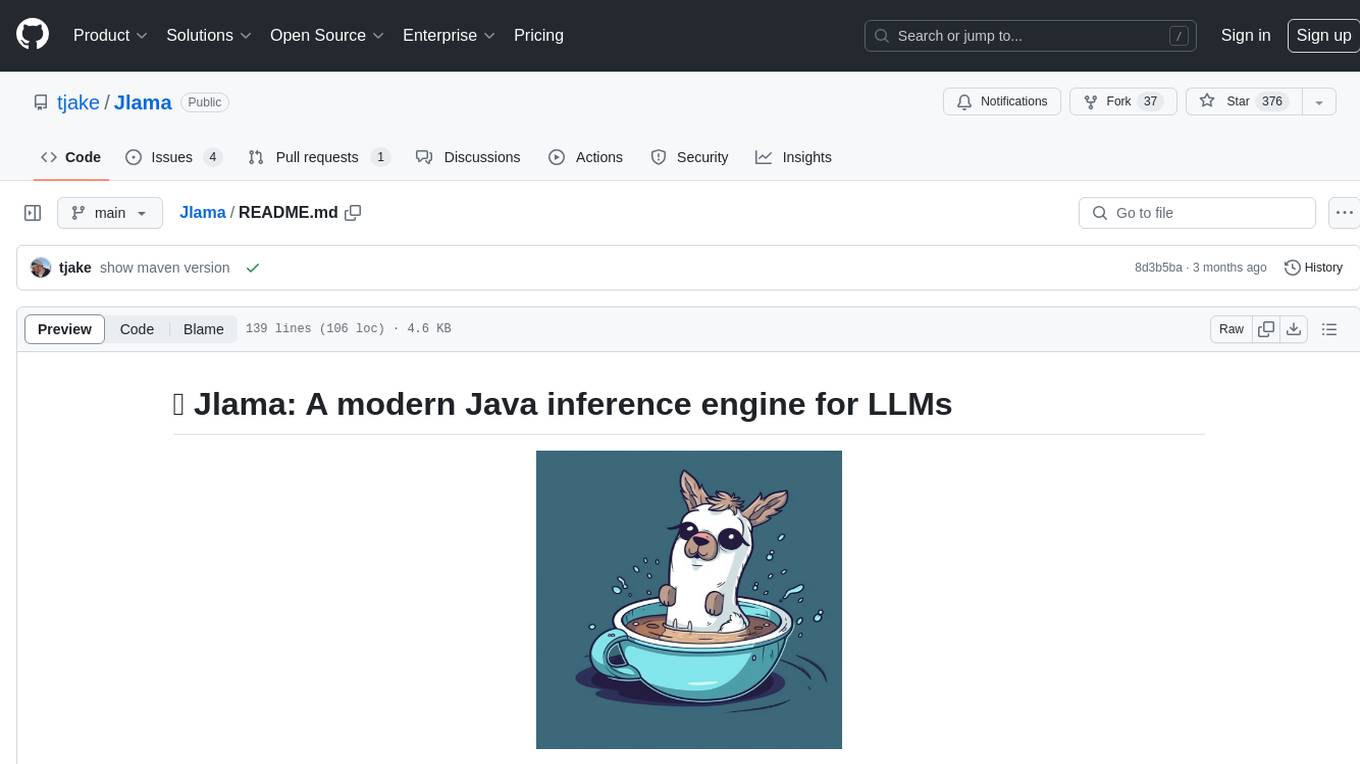
Jlama
Jlama is a modern Java inference engine designed for large language models. It supports various model types such as Gemma, Llama, Mistral, GPT-2, BERT, and more. The tool implements features like Flash Attention, Mixture of Experts, and supports different model quantization formats. Built with Java 21 and utilizing the new Vector API for faster inference, Jlama allows users to add LLM inference directly to their Java applications. The tool includes a CLI for running models, a simple UI for chatting with LLMs, and examples for different model types.
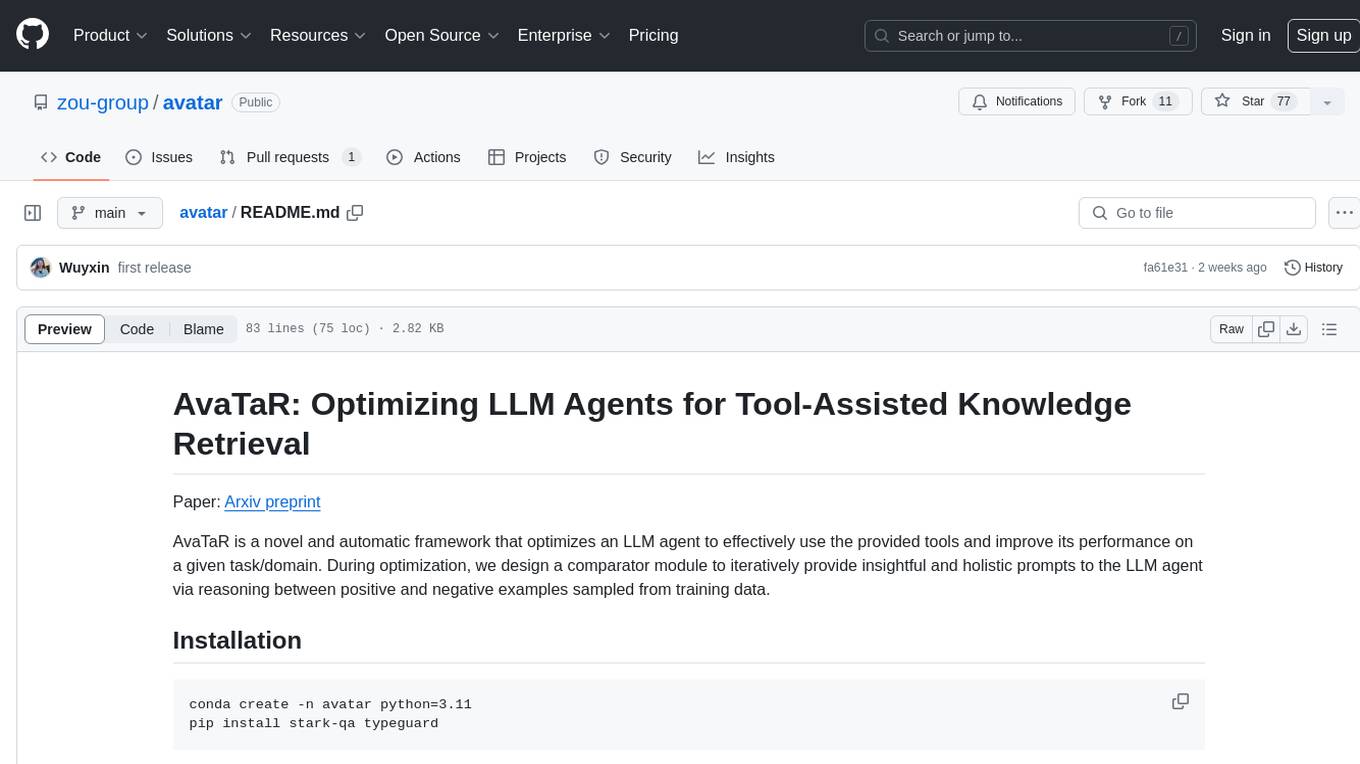
avatar
AvaTaR is a novel and automatic framework that optimizes an LLM agent to effectively use provided tools and improve performance on a given task/domain. It designs a comparator module to provide insightful prompts to the LLM agent via reasoning between positive and negative examples from training data.
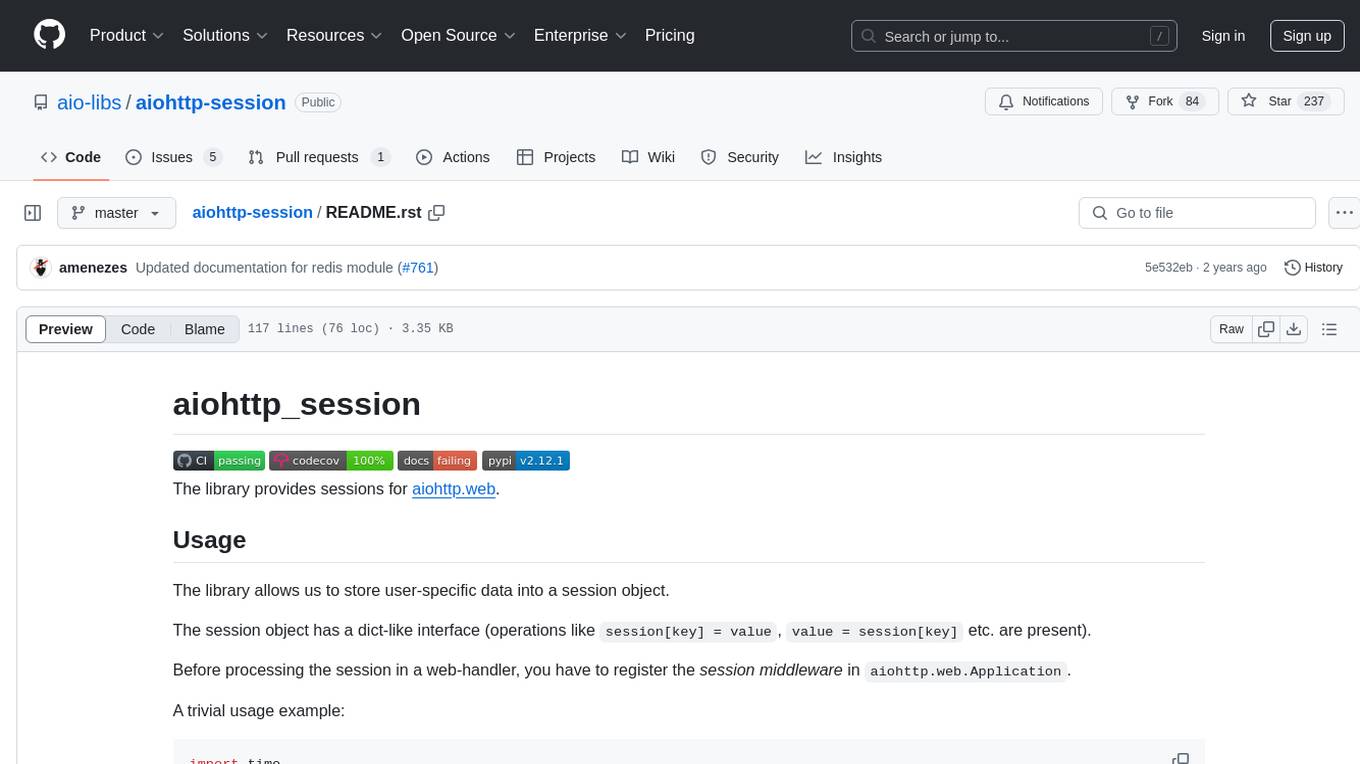
aiohttp-session
aiohttp_session is a Python library that provides session management for aiohttp.web applications. It allows storing user-specific data in session objects with a dict-like interface. The library offers different session storage options, including SimpleCookieStorage for testing, EncryptedCookieStorage for secure data storage, and RedisStorage for storing data in Redis. Users can easily integrate session management into their aiohttp.web applications by registering the session middleware. The library is designed to simplify session handling and enhance the security of web applications.
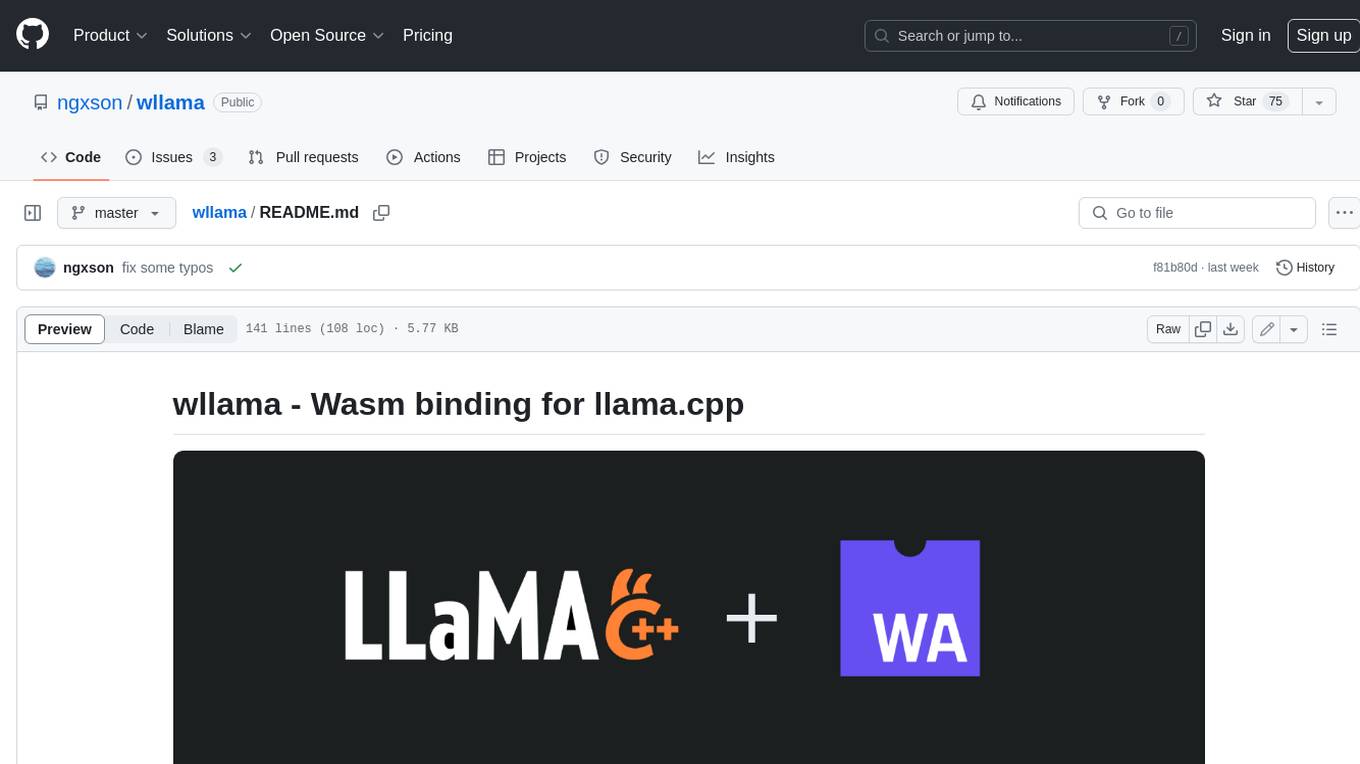
wllama
Wllama is a WebAssembly binding for llama.cpp, a high-performance and lightweight language model library. It enables you to run inference directly on the browser without the need for a backend or GPU. Wllama provides both high-level and low-level APIs, allowing you to perform various tasks such as completions, embeddings, tokenization, and more. It also supports model splitting, enabling you to load large models in parallel for faster download. With its Typescript support and pre-built npm package, Wllama is easy to integrate into your React Typescript projects.
For similar tasks
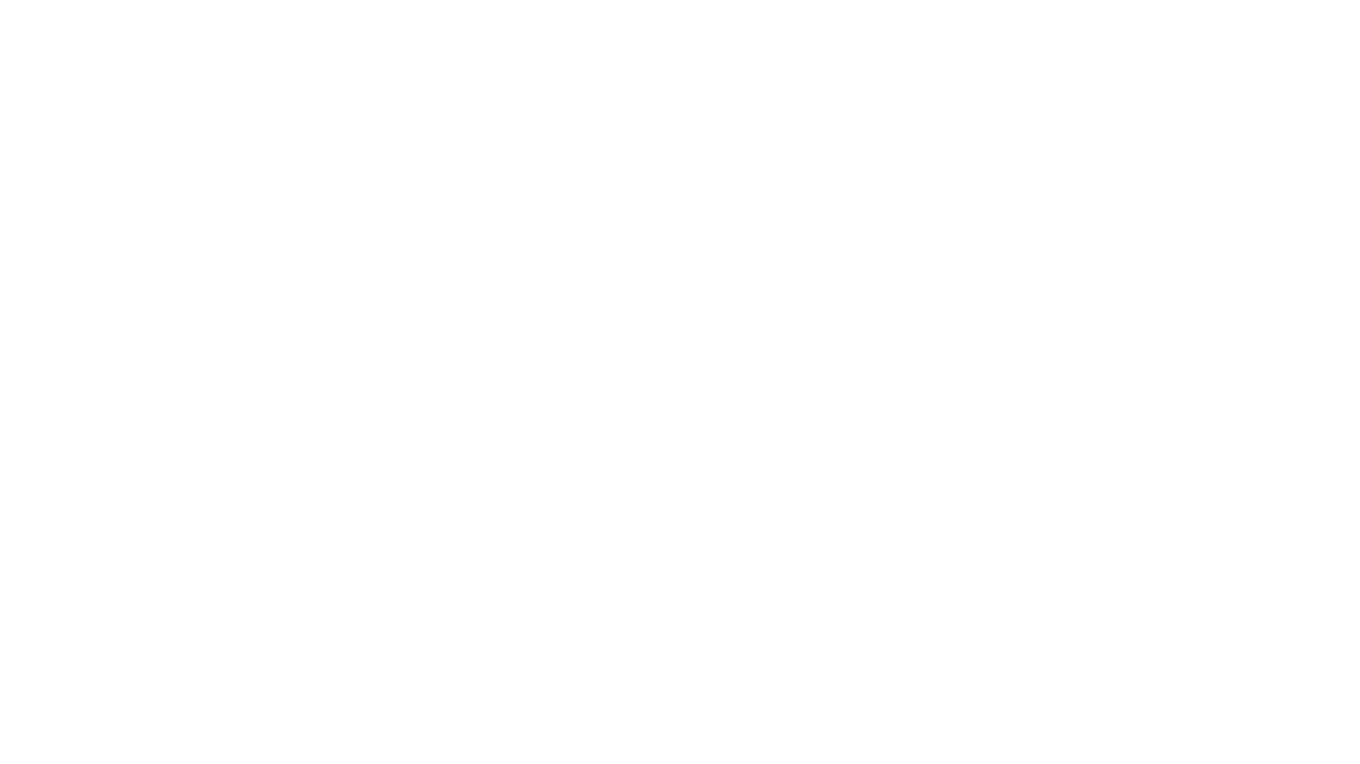
aiomisc
aiomisc is a Python library that provides a collection of utility functions and classes for working with asynchronous I/O in a more intuitive and efficient way. It offers features like worker pools, connection pools, circuit breaker pattern, and retry mechanisms to make asyncio code more robust and easier to maintain. The library simplifies the architecture of software using asynchronous I/O, making it easier for developers to write reliable and scalable asynchronous code.
For similar jobs
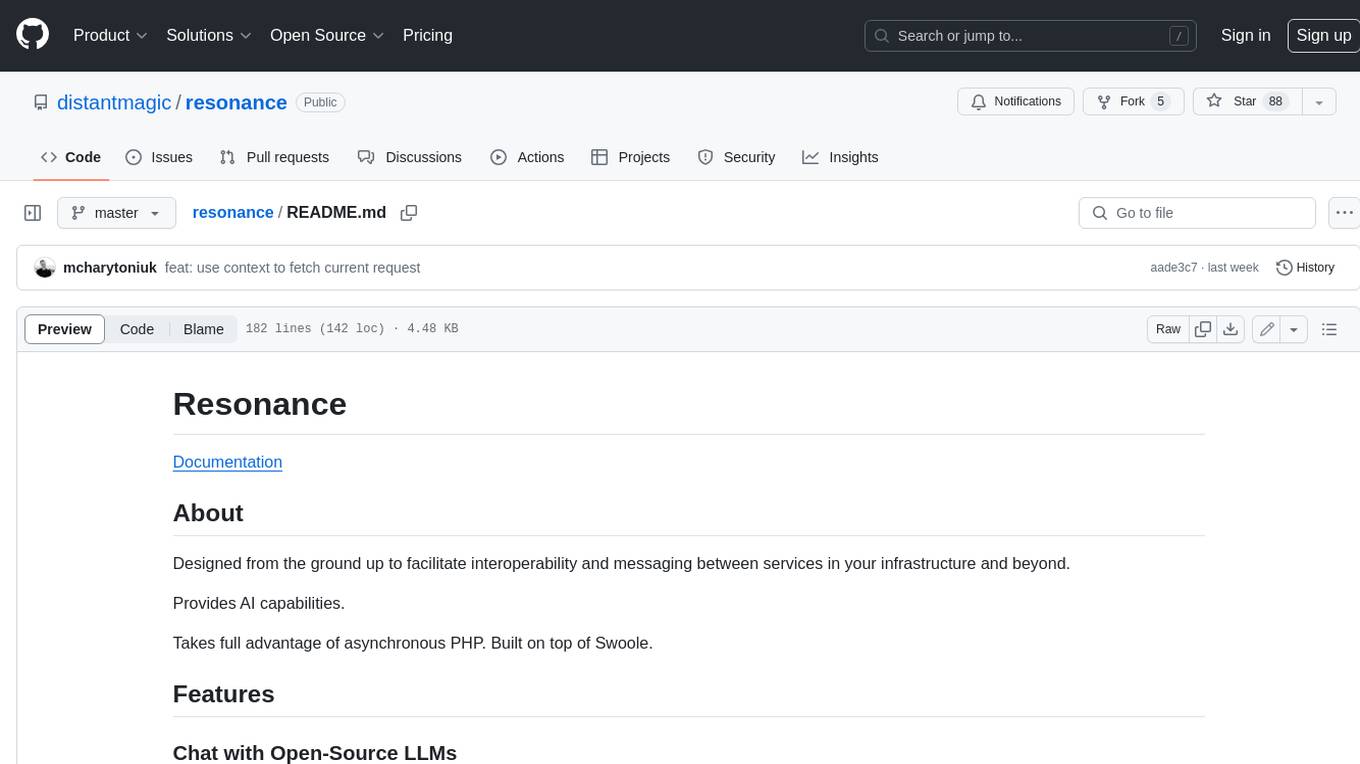
resonance
Resonance is a framework designed to facilitate interoperability and messaging between services in your infrastructure and beyond. It provides AI capabilities and takes full advantage of asynchronous PHP, built on top of Swoole. With Resonance, you can: * Chat with Open-Source LLMs: Create prompt controllers to directly answer user's prompts. LLM takes care of determining user's intention, so you can focus on taking appropriate action. * Asynchronous Where it Matters: Respond asynchronously to incoming RPC or WebSocket messages (or both combined) with little overhead. You can set up all the asynchronous features using attributes. No elaborate configuration is needed. * Simple Things Remain Simple: Writing HTTP controllers is similar to how it's done in the synchronous code. Controllers have new exciting features that take advantage of the asynchronous environment. * Consistency is Key: You can keep the same approach to writing software no matter the size of your project. There are no growing central configuration files or service dependencies registries. Every relation between code modules is local to those modules. * Promises in PHP: Resonance provides a partial implementation of Promise/A+ spec to handle various asynchronous tasks. * GraphQL Out of the Box: You can build elaborate GraphQL schemas by using just the PHP attributes. Resonance takes care of reusing SQL queries and optimizing the resources' usage. All fields can be resolved asynchronously.
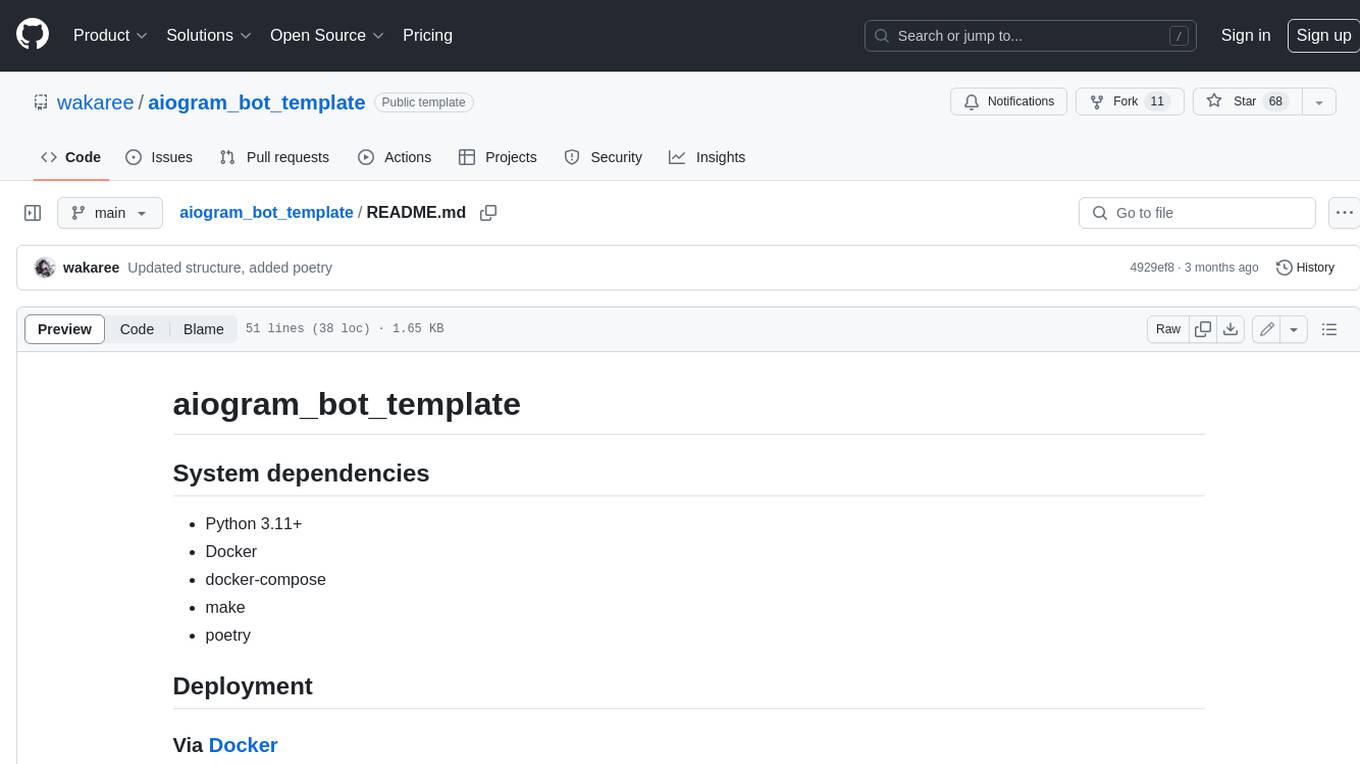
aiogram_bot_template
Aiogram bot template is a boilerplate for creating Telegram bots using Aiogram framework. It provides a solid foundation for building robust and scalable bots with a focus on code organization, database integration, and localization.
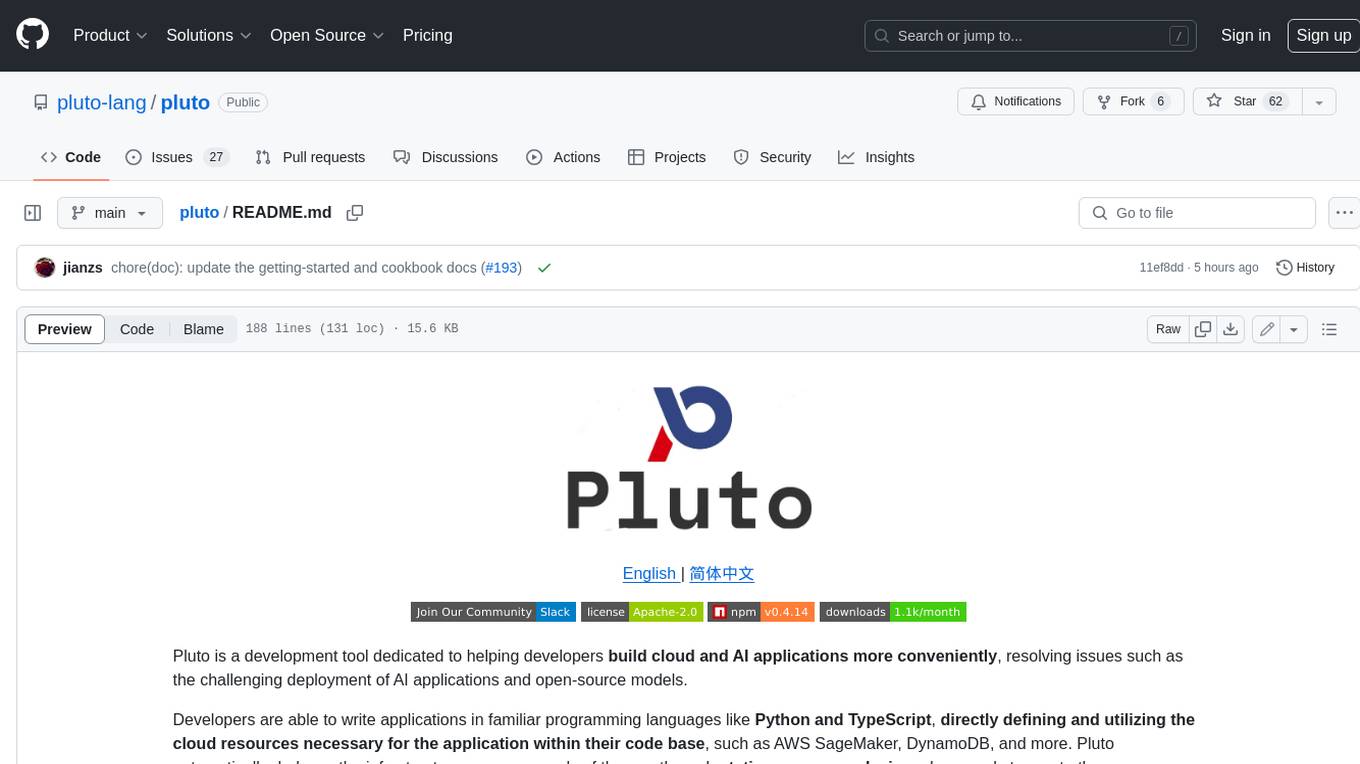
pluto
Pluto is a development tool dedicated to helping developers **build cloud and AI applications more conveniently** , resolving issues such as the challenging deployment of AI applications and open-source models. Developers are able to write applications in familiar programming languages like **Python and TypeScript** , **directly defining and utilizing the cloud resources necessary for the application within their code base** , such as AWS SageMaker, DynamoDB, and more. Pluto automatically deduces the infrastructure resource needs of the app through **static program analysis** and proceeds to create these resources on the specified cloud platform, **simplifying the resources creation and application deployment process**.
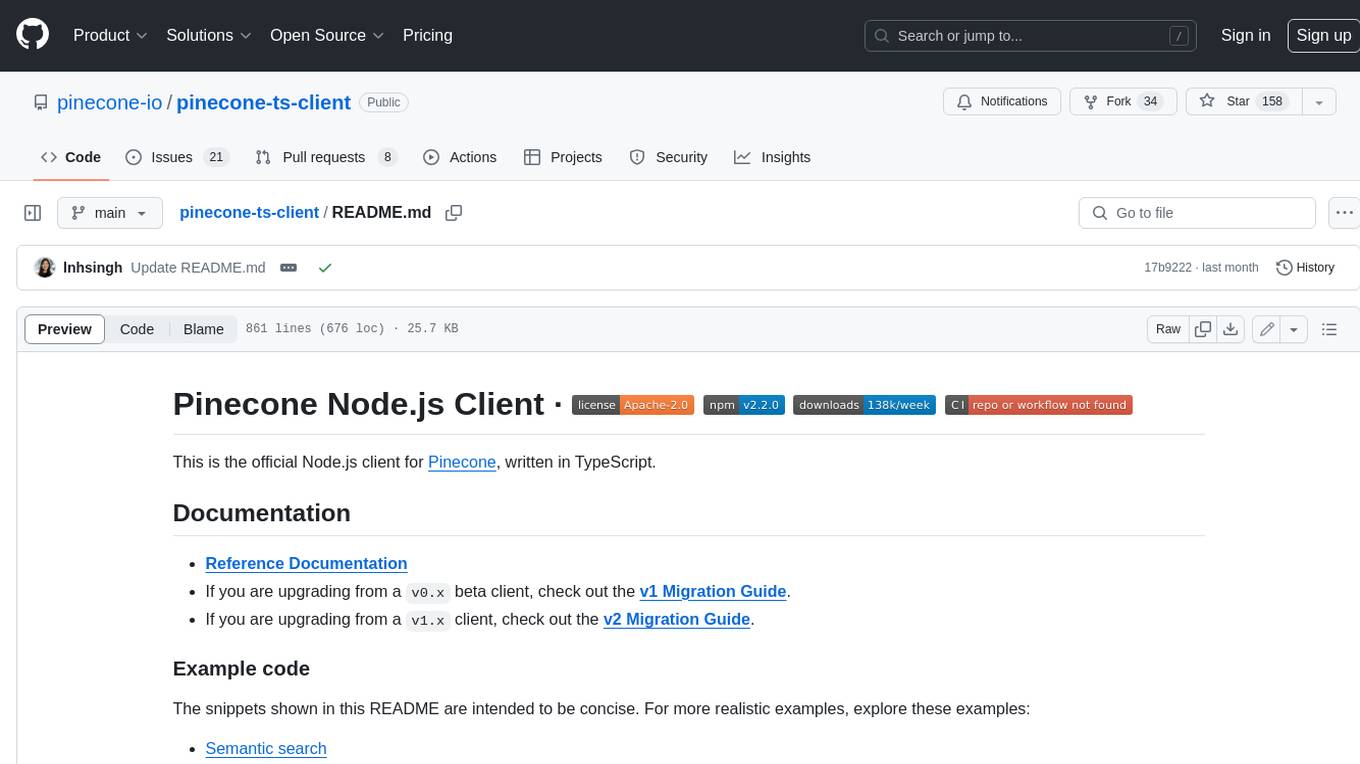
pinecone-ts-client
The official Node.js client for Pinecone, written in TypeScript. This client library provides a high-level interface for interacting with the Pinecone vector database service. With this client, you can create and manage indexes, upsert and query vector data, and perform other operations related to vector search and retrieval. The client is designed to be easy to use and provides a consistent and idiomatic experience for Node.js developers. It supports all the features and functionality of the Pinecone API, making it a comprehensive solution for building vector-powered applications in Node.js.
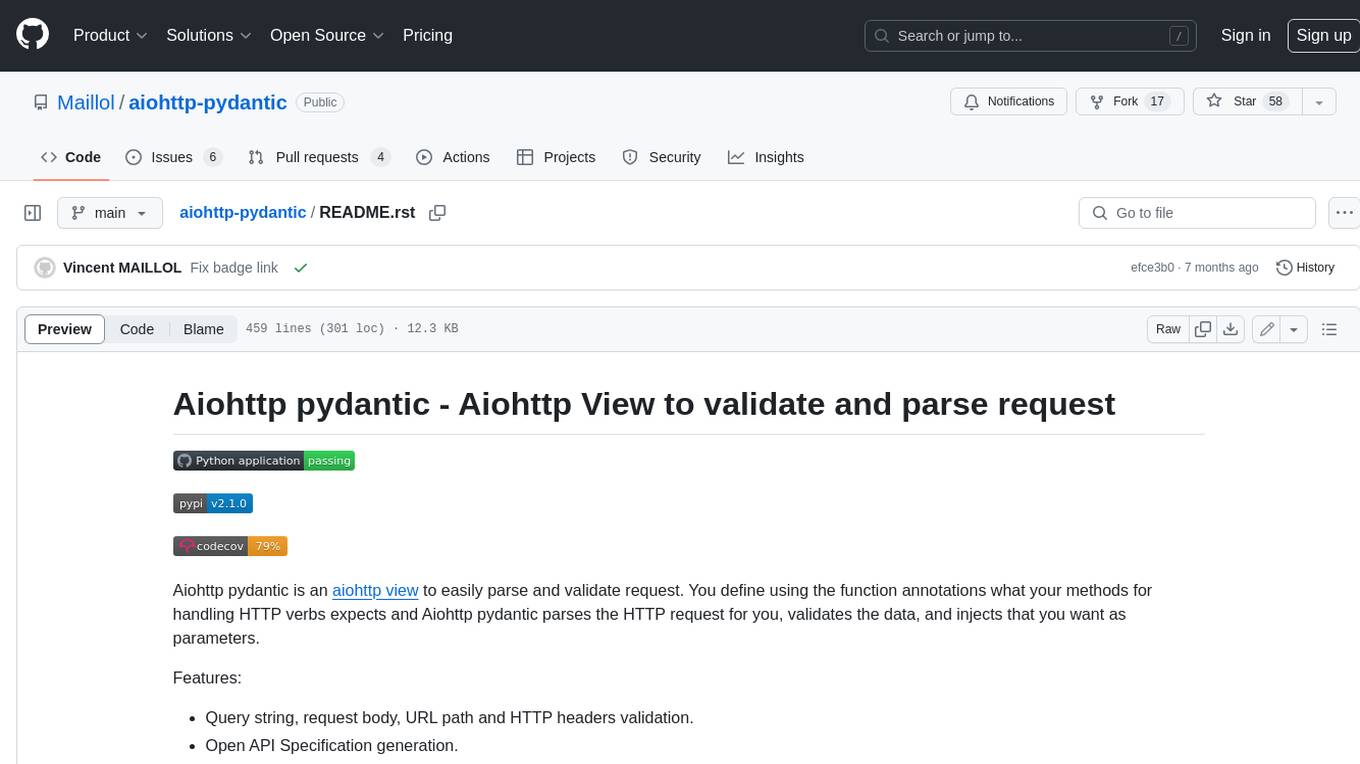
aiohttp-pydantic
Aiohttp pydantic is an aiohttp view to easily parse and validate requests. You define using function annotations what your methods for handling HTTP verbs expect, and Aiohttp pydantic parses the HTTP request for you, validates the data, and injects the parameters you want. It provides features like query string, request body, URL path, and HTTP headers validation, as well as Open API Specification generation.
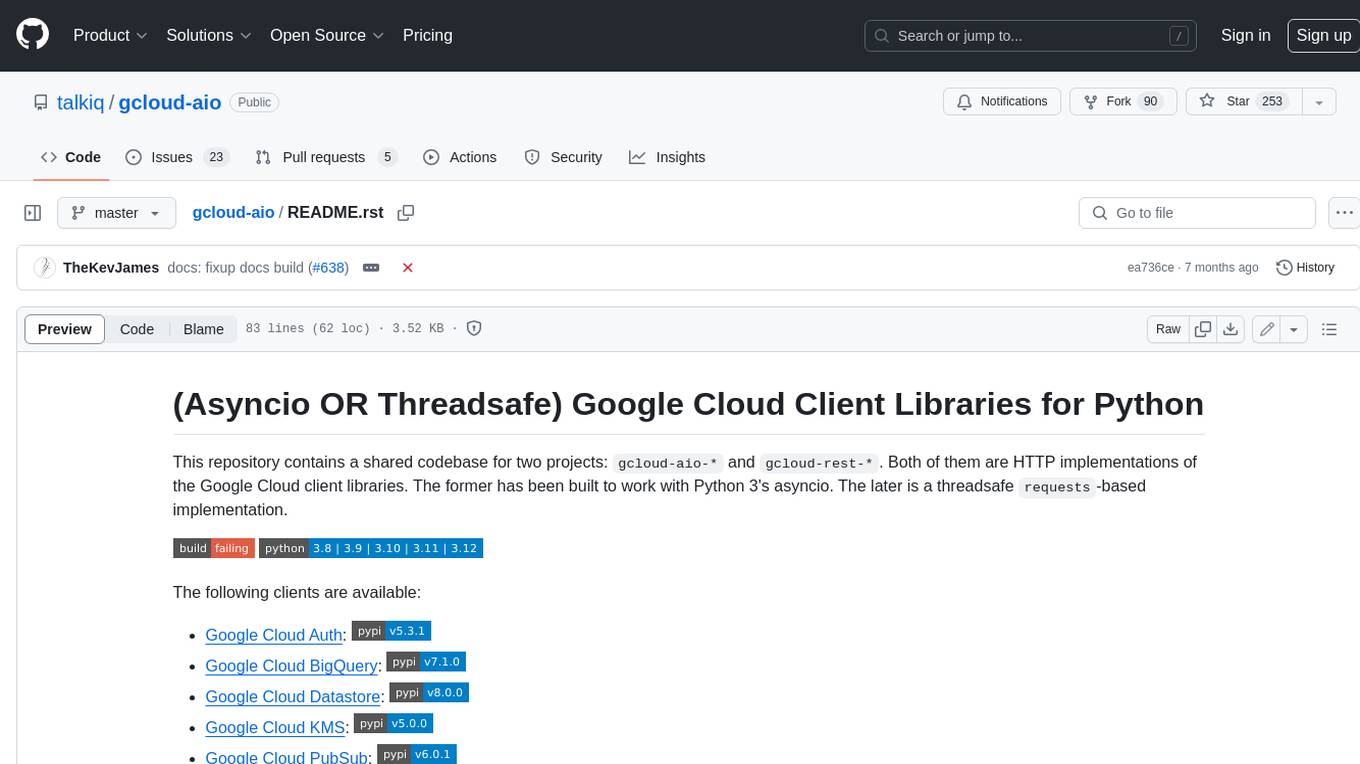
gcloud-aio
This repository contains shared codebase for two projects: gcloud-aio and gcloud-rest. gcloud-aio is built for Python 3's asyncio, while gcloud-rest is a threadsafe requests-based implementation. It provides clients for Google Cloud services like Auth, BigQuery, Datastore, KMS, PubSub, Storage, and Task Queue. Users can install the library using pip and refer to the documentation for usage details. Developers can contribute to the project by following the contribution guide.
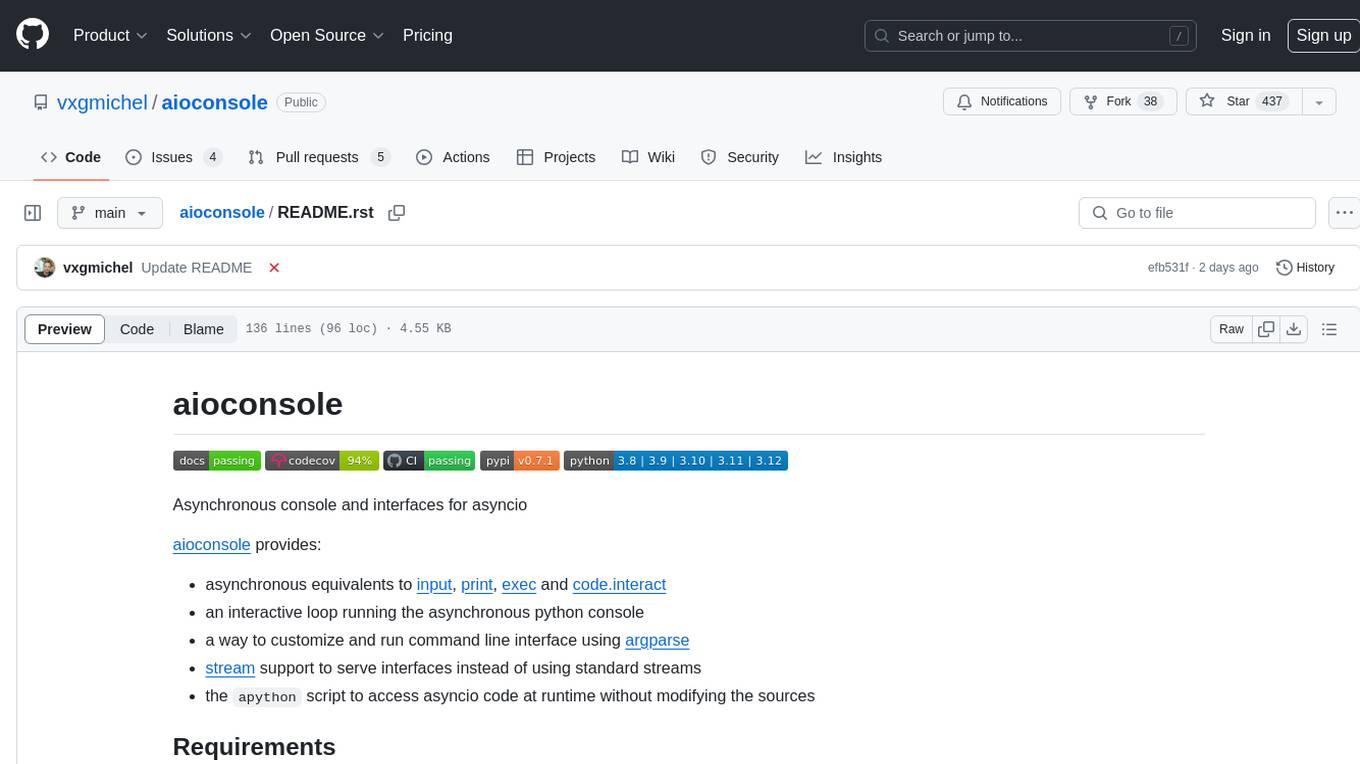
aioconsole
aioconsole is a Python package that provides asynchronous console and interfaces for asyncio. It offers asynchronous equivalents to input, print, exec, and code.interact, an interactive loop running the asynchronous Python console, customization and running of command line interfaces using argparse, stream support to serve interfaces instead of using standard streams, and the apython script to access asyncio code at runtime without modifying the sources. The package requires Python version 3.8 or higher and can be installed from PyPI or GitHub. It allows users to run Python files or modules with a modified asyncio policy, replacing the default event loop with an interactive loop. aioconsole is useful for scenarios where users need to interact with asyncio code in a console environment.
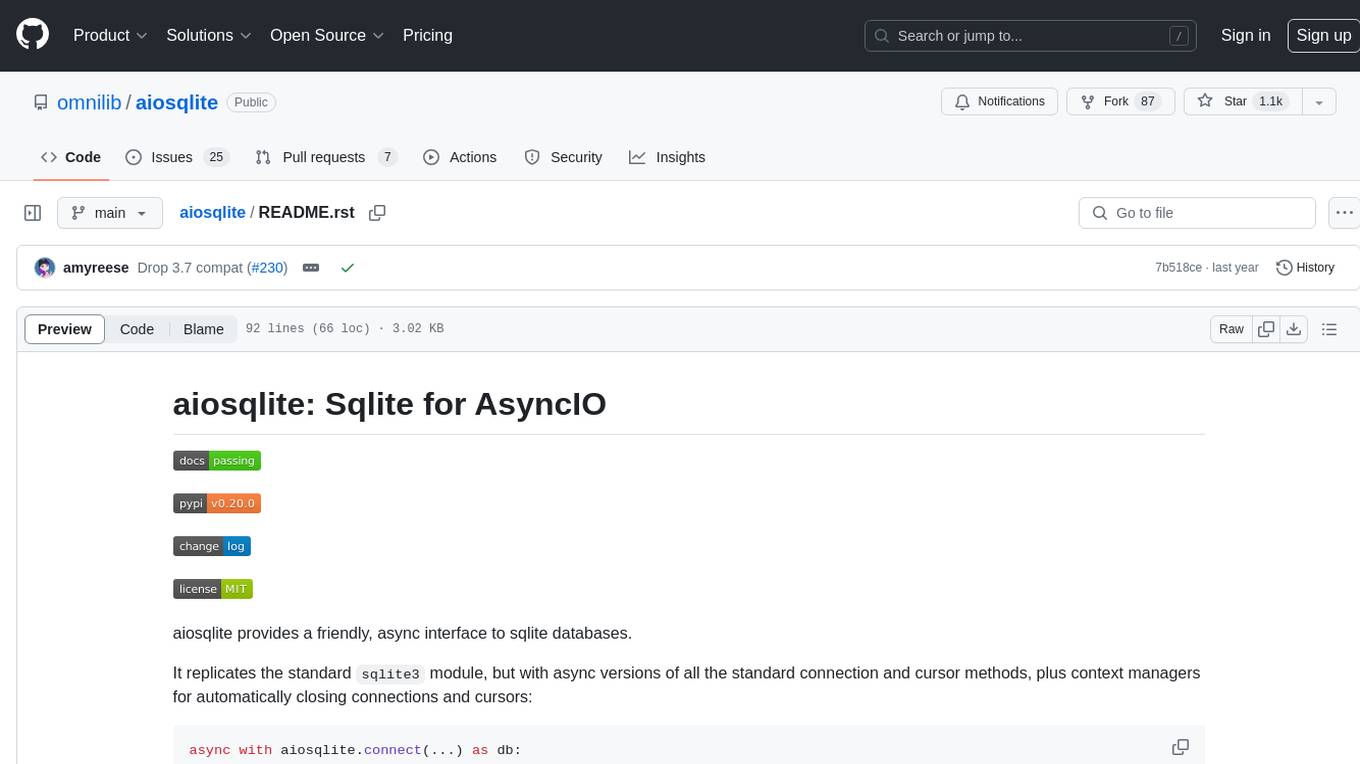
aiosqlite
aiosqlite is a Python library that provides a friendly, async interface to SQLite databases. It replicates the standard sqlite3 module but with async versions of all the standard connection and cursor methods, along with context managers for automatically closing connections and cursors. It allows interaction with SQLite databases on the main AsyncIO event loop without blocking execution of other coroutines while waiting for queries or data fetches. The library also replicates most of the advanced features of sqlite3, such as row factories and total changes tracking.