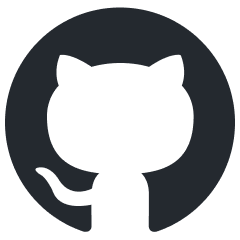
awesome-ai4db-paper
Paper related to AI4DB techniques
Stars: 53
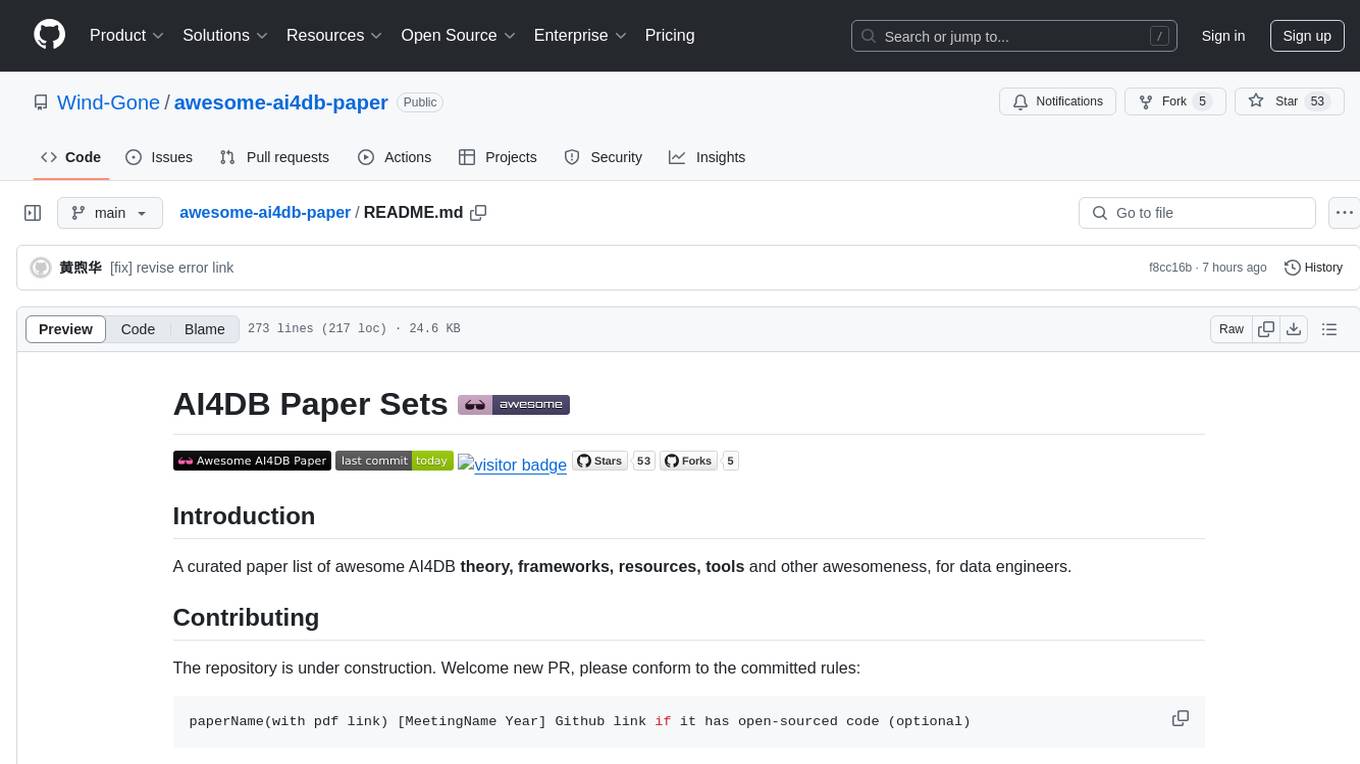
The 'awesome-ai4db-paper' repository is a curated paper list focusing on AI for database (AI4DB) theory, frameworks, resources, and tools for data engineers. It includes a collection of research papers related to learning-based query optimization, training data set preparation, cardinality estimation, query-driven approaches, data-driven techniques, hybrid methods, pretraining models, plan hints, cost models, SQL embedding, join order optimization, query rewriting, end-to-end systems, text-to-SQL conversion, traditional database technologies, storage solutions, learning-based index design, and a learning-based configuration advisor. The repository aims to provide a comprehensive resource for individuals interested in AI applications in the field of database management.
README:
A curated paper list of awesome AI4DB theory, frameworks, resources, tools and other awesomeness, for data engineers.
The repository is under construction. Welcome new PR, please conform to the committed rules:
paperName(with pdf link) [MeetingName Year] Github link if it has open-sourced code (optional)
Thanks to all authors of the paper/repository I cite :D
- AI4DB Paper Sets
- LEON: A New Framework for ML-Aided Query Optimization [VLDB 23]
- LOGER: A Learned Optimizer towards Generating Efficient and Robust Query Execution Plans [VLDB 23]
- Eraser: Eliminating Performance Regression on Learned Query Optimizer [VLDB 24]
- AutoSteer: Learned Query Optimization for Any SQL Database [VLDB 24]
- Modeling Shifting Workloads for Learned Database Systems [SIGMOD 24]
- Stage: Query Execution Time Prediction in Amazon Redshift [SIGMOD 24]
- Roq: Robust Query Optimization Based on a Risk-aware Learned Cost Model [arXiv 24]
- RobOpt: A Tool for Robust Workload Optimization Based on Uncertainty-Aware Machine Learning [SIGMOD Demo 24]
- Towards Exploratory Query Optimization for Template-based SQL Workloads [ICDE 24]
- DSB: a decision support benchmark for workload-driven and traditional database systems [VLDB 21]
- Expand your training limits! generating training data for ml-based data management [VLDB 21]
- LearnedSQLGen: Constraint-aware SQL Generation using Reinforcement Learning [SIGMOD 22]
-
Hit the Gym: Accelerating Query Execution to Efficiently Bootstrap Behavior Models for Self-Driving Database Management Systems [VLDB 24]
- Cardinality Estimation: An Experimental Survey [VLDB 17]
- Are We Ready For Learned Cardinality Estimation? [VLDB 21]
- Cardinality Estimation in DBMS: A Comprehensive Benchmark Evaluation [VLDB 21]
- Learned cardinality estimation: A design space exploration and a comparative evaluation [VLDB 22]
-
Learned Cardinality Estimation: An In-depth Study [SIGMOD 22]
- A Comparative Study and Component Analysis of Query Plan Representation Techniques in ML4DB Studies [VLDB 24]
- Selectivity estimation for range predicates using lightweight models [VLDB 19]
- Deep learning models for selectivity estimation of multiattribute queries [SIGMOD 20]
- Learned Cardinalities: Estimating Correlated Joins with Deep Learning [CIDR 2019]
-
An End-to-End Learning-based Cost Estimator [VLDB 19]
- Flow-Loss: Learning Cardinality Estimates That Matter [VLDB 21]
-
Speeding Up End-to-end Query Execution via Learning-based Progressive Cardinality Estimation [SIGMOD 23]
- Robust Query Driven Cardinality Estimation under Changing Workloads[VLDB 23]
- AutoCE: An Accurate and Efficient Model Advisor for Learned Cardinality Estimation [ICDE 23]
- Asm: Harmonizing autoregressive model, sampling, and multi-dimensional statistics merging for cardinality estimation [SIGMOD 24]
- Adding Domain Knowledge to Query-Driven Learned Databases [SIGMOD 24]
- Self-tuning, gpu-accelerated kernel density models for multidimensional selectivity estimation [SIGMOD 15]
-
Deep Unsupervised Cardinality Estimation [VLDB 19]
-
Quicksel: Quick selectivity learning with mixture models [SIGMOD 20]
-
Pre-training Summarization Models of Structured Datasets for Cardinality Estimation [VLDB 22]
-
DeepDB: Learn from Data, not from Queries! [VLDB 20]
-
NeuroCard: One Cardinality Estimator for All Tables [VLDB 21]
- FLAT: Fast, Lightweight and Accurate Method for Cardinality Estimation [VLDB 21]
-
BayesCard: Revitilizing Bayesian Frameworks for Cardinality Estimation [aiXiv 21]
- Glue: Adaptively Merging Single Table Cardinality to Estimate Join Query Size [aiXiv 21]
- Fauce: fast and accurate deep ensembles with uncertainty for cardinality estimation [VLDB 21]
-
FACE: a normalizing flow based cardinality estimator [VLDB 22]
- FactorJoin: A New Cardinality Estimation Framework for Join Queries [SIGMOD 22] (Bounded)
- Cardinality Estimation of LIKE Predicate Queries using Deep Learning [SIGMOD 25]
- Bao: Making Learned Query Optimization Practical [SIMOD 21]
- FASTgres: Making Learned Query Optimizer Hinting Effective [VLDB 23]
-
COOOL: A Learning-To-Rank Approach for SQL Hint Recommendations [VLDB 23]
- Zero-Shot Cost Models for Out-of-the-box Learned Cost Prediction [VLDB 22]
-
Cost-based or Learning-based? A Hybrid Query Optimizer for Query Plan Selection [VLDB 22]
- Lero: A Learning-to-Rank Qery Optimizer [VLDB 23]
- Lero: applying learning-to-rank in query optimizer [VLDBJ 24]
- Learning to Optimize Join queries With Deep Reinforcement Learning [SIGMOD 16]
- Deep Reinforcement Learning for Join Order Enumeration[arXiv 18]
- Reinforcement Learning with Tree-LSTM for Join Order Selection [ICDE 20]
- Modeling Shifting Workloads for Learned Database Systems [SIGMOD 24]
- Db2une: Tuning Under Pressure via Deep Learning [VLDB 24]
- PilotScope: Steering Databases with Machine Learning Drivers [VLDB 24]
- Cosine: A Cloud-Cost Optimized Self-Designing Key-Value Storage Engine [VLDB 22]
-
TreeLine: An Update-In-Place Key-Value Store for Modern Storage [VLDB 22]
- Learning to Optimize LSM-trees: Towards A Reinforcement Learning based Key-Value Store for Dynamic Workloads [SIGMOD 23]
- Limousine: Blending Learned and Classical Indexes to Self-Design Larger-than-Memory Cloud Storage Engines [SIGMOD 24]
- The Case for Learned Index Structures [SIGMOD 18]
- FITing-Tree: A Data-aware Index Structure [SIGMOD 19]
- ALEX: An Updatable Adaptive Learned Index [aiXiv 20]
- The PGM-index: a fully-dynamic compressed learned index with provable worst-case bounds [VLDB 20]
- RadixSpline: a single-pass learned index [aiDM 20]
- Why Are Learned Indexes So Effective? [ICML 20]
- A Pluggable Learned Index Method via Sampling and Gap Insertion [aiXiv 21]
- Updatable Learned Index with Precise Positions [VLDB 21]
- The next 50 years in database indexing or: the case for automatically generated index structures [VLDB 21]
- Tuning Hierarchical Learned Indexes on Disk and Beyond [SIGMOD 22]
- APEX: A High-Performance Learned Index on Persistent Memory [VLDB 22]
- FINEdex: A Fine-grained Learned Index Scheme for Scalable and Concurrent Memory Systems [VLDB 22]
- Are Updatable Learned Indexes Ready? [VLDB 22]
- CARMI: A Cache-Aware Learned Index with a Cost-based Construction Algorithm [VLDB 22]
- NFL: Robust Learned Index via Distribution Transformation [VLDB 22]
- Cutting Learned Index into Pieces: An In-depth Inquiry into Updatable Learned Indexes [ICDE 23]
- Learning Multi-dimensional Indexes [SIGMOD 20]
- LISA: A Learned Index Structure for Spatial Data [SIGMOD 20]
- Effectively Learning Spatial Indices [VLDB 20]
- The ML-Index: A Multidimensional, Learned Index for Point, Range, and Nearest-Neighbor Queries [EDBT 20]
- Tsunami: A Learned Multi-dimensional Index for Correlated Data and Skewed Workloads [VLDB 21]
- NEIST: a Neural-Enhanced Index for Spatio-Temporal Queries [TKDE 21]
- RW-Tree: A Learned Workload-aware Framework for R-tree Construction [ICDE 22]
- The Case for Automatic Database Administration using Deep Reinforcement Learning [arXiv 18]
- AI Meets AI: Leveraging Query Executions to Improve Index Recommendations [SIGMOD 19]
- Online Index Selection Using Deep Reinforcement Learning for a Cluster Database [ICDEW 20]
- SMARTIX: A database indexing agent based on reinforcement learning [Applied Intelligence 20]
-
Magic mirror in my hand, which is the best in the land? An Experimental Evaluation of Index Selection Algorithms [VLDB 20]
- An Index Advisor Using Deep Reinforcement Learning [CIKM 20]
- Automated Database Indexing Using Model-Free Reinforcement Learning [ICAPS 20]
- DBA bandits: Self-driving index tuning under ad-hoc, analytical workloads with safety guarantees [ICDE 21]
- Index selection for NoSQL database with deep reinforcement learning [Information Sciences 21]
- MANTIS: Multiple Type and Attribute Index Selection using Deep Reinforcement Learning [IDEAS 21]
- AutoIndex: An Incremental Index Management System for Dynamic Workloads [ICDE 22]
- SWIRL: Selection of Workload-aware Indexes using Reinforcement Learning [EDBT 22]
- Indexer++: Workload-Aware Online Index Tuning with Transformers and Reinforcement Learning [SAC 22]
- Budget-aware Index Tuning with Reinforcement Learning [SIGMOD 22]
- ISUM: Efficiently Compressing Large and Complex Workloads for Scalable Index Tuning [SIGMOD 22]
- DISTILL: low-overhead data-driven techniques for filtering and costing indexes for scalable index tuning [VLDB 22]
-
HMAB: Self-Driving Hierarchy of Bandits for Integrated Physical Database Design Tuning [VLDB 22]
- SmartIndex: An Index Advisor with Learned Cost Estimator [CIKM 22]
-
Learned Index Benefits: Machine Learning Based Index Performance Estimation [VLDB 23]
- No DBA? No Regret! Multi-Armed Bandits for Index Tuning of Analytical and HTAP Workloads With Provable Guarantees [TKDE 23]
- IA2: Leveraging Instance-Aware Index Advisor with Reinforcement Learning for Diverse Workloads [EuroMLSys 24]
- Leveraging Dynamic and Heterogeneous Workload Knowledge to Boost the Performance of Index Advisors [PVLDB 24]
- Refactoring Index Tuning Process with Benefit Estimation [PVLDB 24]
- Breaking It Down: An In-Depth Study of Index Advisors [PVLDB 24]
- TRAP: Tailored Robustness Assessment for Index Advisors via Adversarial Perturbation [ICDE 24]
- Automatic Database Index Tuning: A Survey [TKDE 24]
- Robustness of Updatable Learning-based Index Advisors against Poisoning Attack [SIGMOD 24]
- Wii: Dynamic Budget Reallocation In Index Tuning [SIGMOD 24]
- Wred: Workload Reduction for Scalable Index Tuning [SIGMOD 24]
- ML-Powered Index Tuning: An Overview of Recent Progress and Open Challenges [SIGMOD 24]
- Automatic Database Management System Tuning Through Large-scale Machine Learning [SIGMOD 17]
- Deploying a Steered Query Optimizer in Production at Microsof [SIGMOD 22]
- Detect, Distill and Update: Learned DB Systems Facing Out of Distribution Data [SIGMOD 23]
- AutoSteer: Learned Query Optimization for Any SQL Database [SIGMOD 23]
- Auto-WLM: Machine Learning Enhanced Workload Management in Amazon Redshif [SIGMOD 23]
- GPTuner: A Manual-Reading Database Tuning System via GPT-Guided Bayesian Optimization [VLDB 24]
- D-Bot: Database Diagnosis System using Large Language Models [VLDB 24]
- LLMTune: Accelerate Database Knob Tuning with Large Language Models [VLDB 24]
- ArcheType: A Novel Framework for Open-Source Column Type Annotation using Large Language Models [VLDB 24]
- A Survey on Large Language Models for Code Generation [arXiv 24]
- Fuzz4All: Universal Fuzzing with Large Language Models [ICSE 24]
- LLM-PBE: Assessing Data Privacy in Large Language Models [VLDB 24]
- Are Large Language Models a Good Replacement of Taxonomies? [VLDB 24]
- A survey on augmenting knowledge graphs (KGs) with large language models (LLMs): models, evaluation metrics, benchmarks, and challenges [Discover Artificial Intelligence 24]
- 𝜆-Tune: Harnessing Large Language Models for Automated Database System Tuning [SIGMOD 25]
- LLM-R2 : A Large Language Model Enhanced Rule-based Rewrite System for Boosting Query Efficiency [VLDB 25]
- Chameleon: a Heterogeneous and Disaggregated Accelerator System for Retrieval-Augmented Language Models [VLDB 25]
- Large Language Model-Based Agents for Software Engineering: A Survey [arXiv 25]
- Evaluating Instruction-Tuned Large Language Models on Code Comprehension and Generation [arXiv 25]
For Tasks:
Click tags to check more tools for each tasksFor Jobs:
Alternative AI tools for awesome-ai4db-paper
Similar Open Source Tools
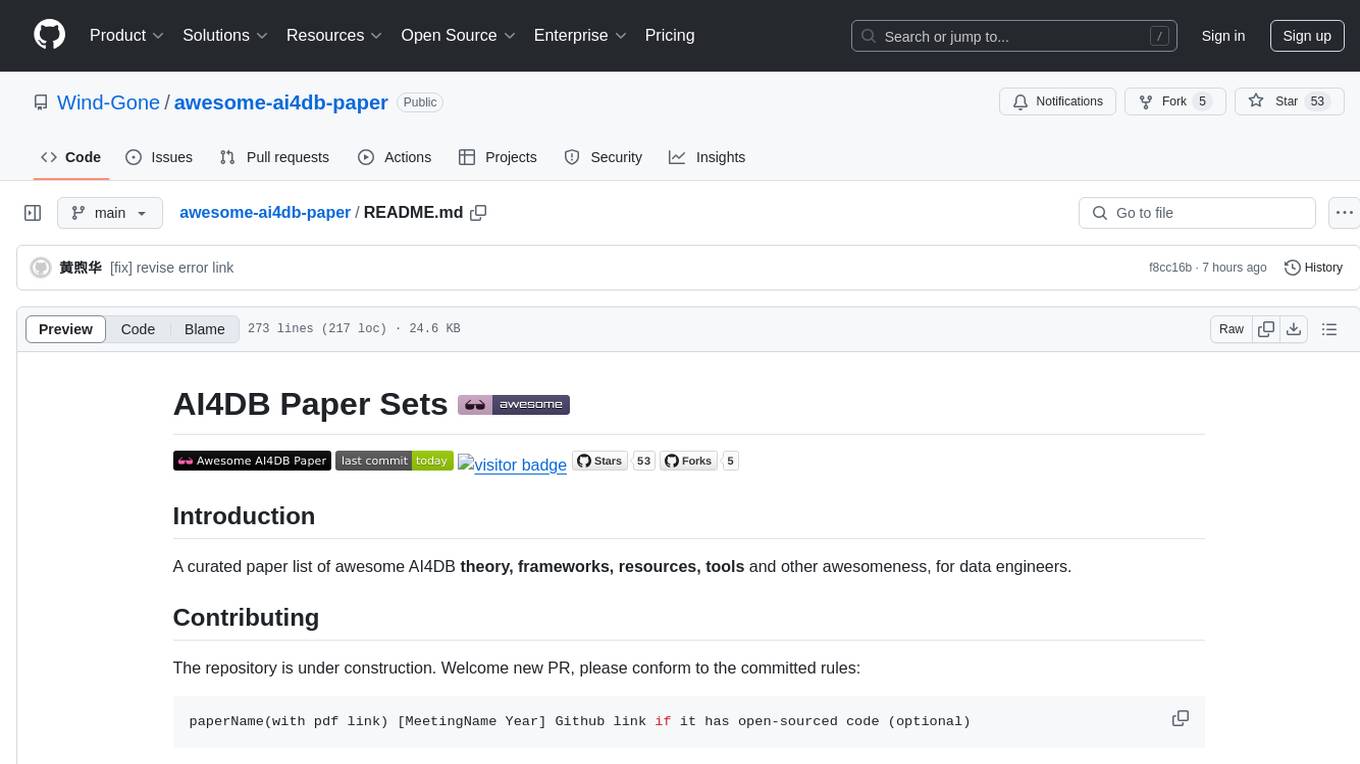
awesome-ai4db-paper
The 'awesome-ai4db-paper' repository is a curated paper list focusing on AI for database (AI4DB) theory, frameworks, resources, and tools for data engineers. It includes a collection of research papers related to learning-based query optimization, training data set preparation, cardinality estimation, query-driven approaches, data-driven techniques, hybrid methods, pretraining models, plan hints, cost models, SQL embedding, join order optimization, query rewriting, end-to-end systems, text-to-SQL conversion, traditional database technologies, storage solutions, learning-based index design, and a learning-based configuration advisor. The repository aims to provide a comprehensive resource for individuals interested in AI applications in the field of database management.
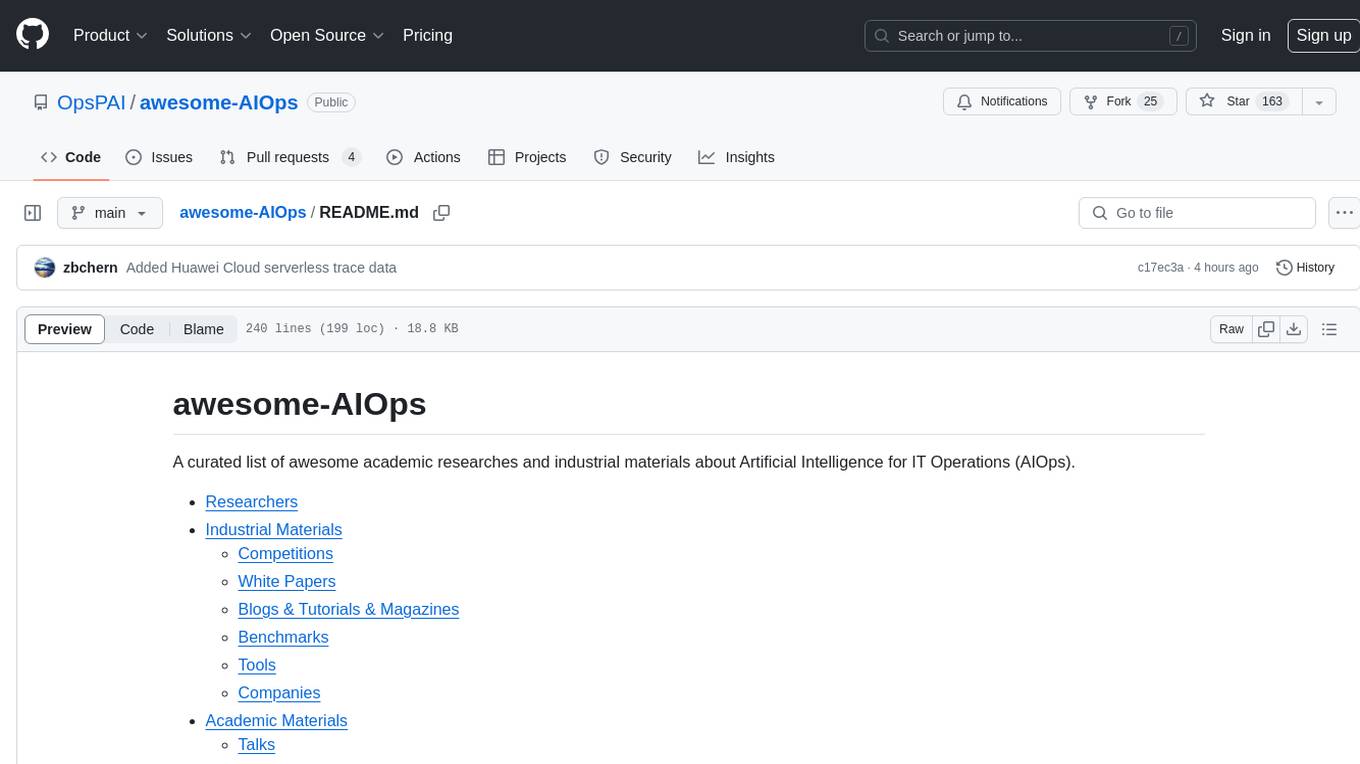
awesome-AIOps
awesome-AIOps is a curated list of academic researches and industrial materials related to Artificial Intelligence for IT Operations (AIOps). It includes resources such as competitions, white papers, blogs, tutorials, benchmarks, tools, companies, academic materials, talks, workshops, papers, and courses covering various aspects of AIOps like anomaly detection, root cause analysis, incident management, microservices, dependency tracing, and more.
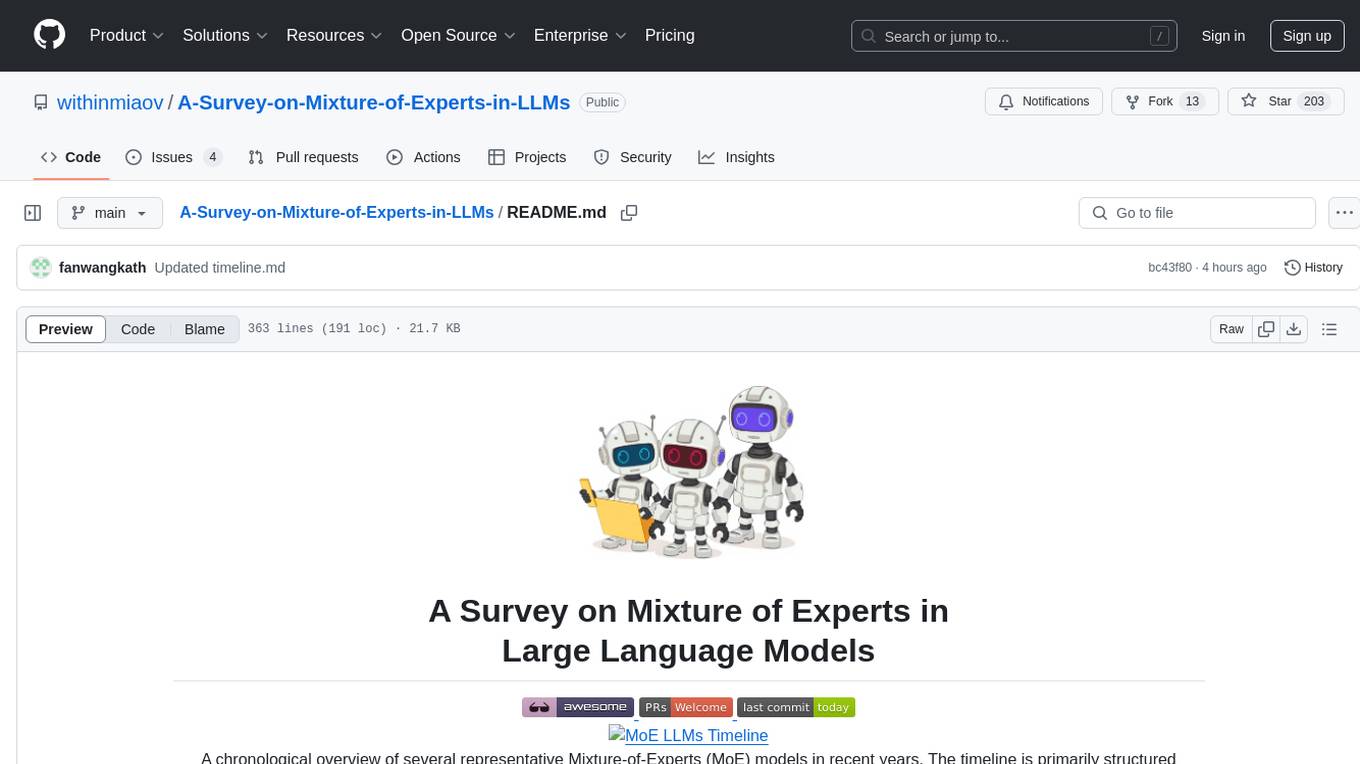
A-Survey-on-Mixture-of-Experts-in-LLMs
A curated collection of papers and resources on Mixture of Experts in Large Language Models. The repository provides a chronological overview of several representative Mixture-of-Experts (MoE) models in recent years, structured according to release dates. It covers MoE models from various domains like Natural Language Processing (NLP), Computer Vision, Multimodal, and Recommender Systems. The repository aims to offer insights into Inference Optimization Techniques, Sparsity exploration, Attention mechanisms, and safety enhancements in MoE models.
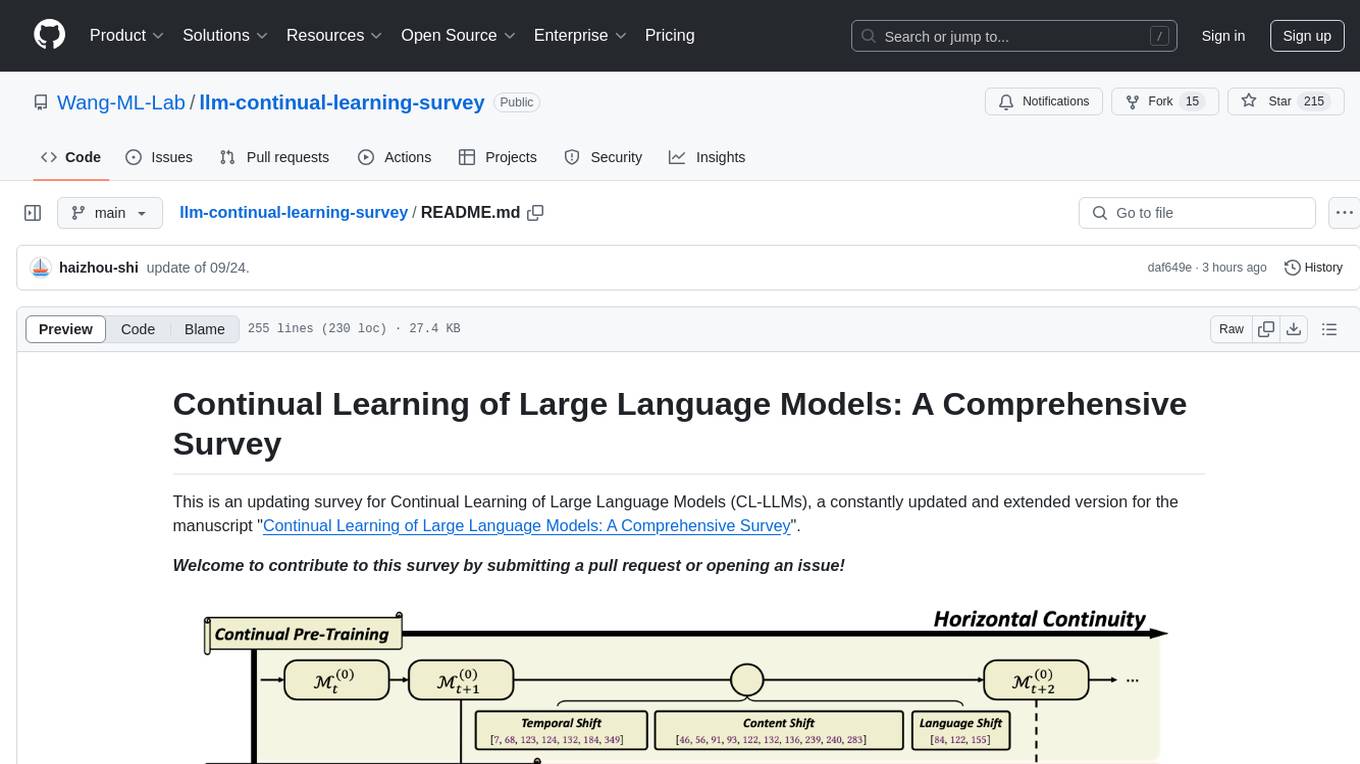
llm-continual-learning-survey
This repository is an updating survey for Continual Learning of Large Language Models (CL-LLMs), providing a comprehensive overview of various aspects related to the continual learning of large language models. It covers topics such as continual pre-training, domain-adaptive pre-training, continual fine-tuning, model refinement, model alignment, multimodal LLMs, and miscellaneous aspects. The survey includes a collection of relevant papers, each focusing on different areas within the field of continual learning of large language models.
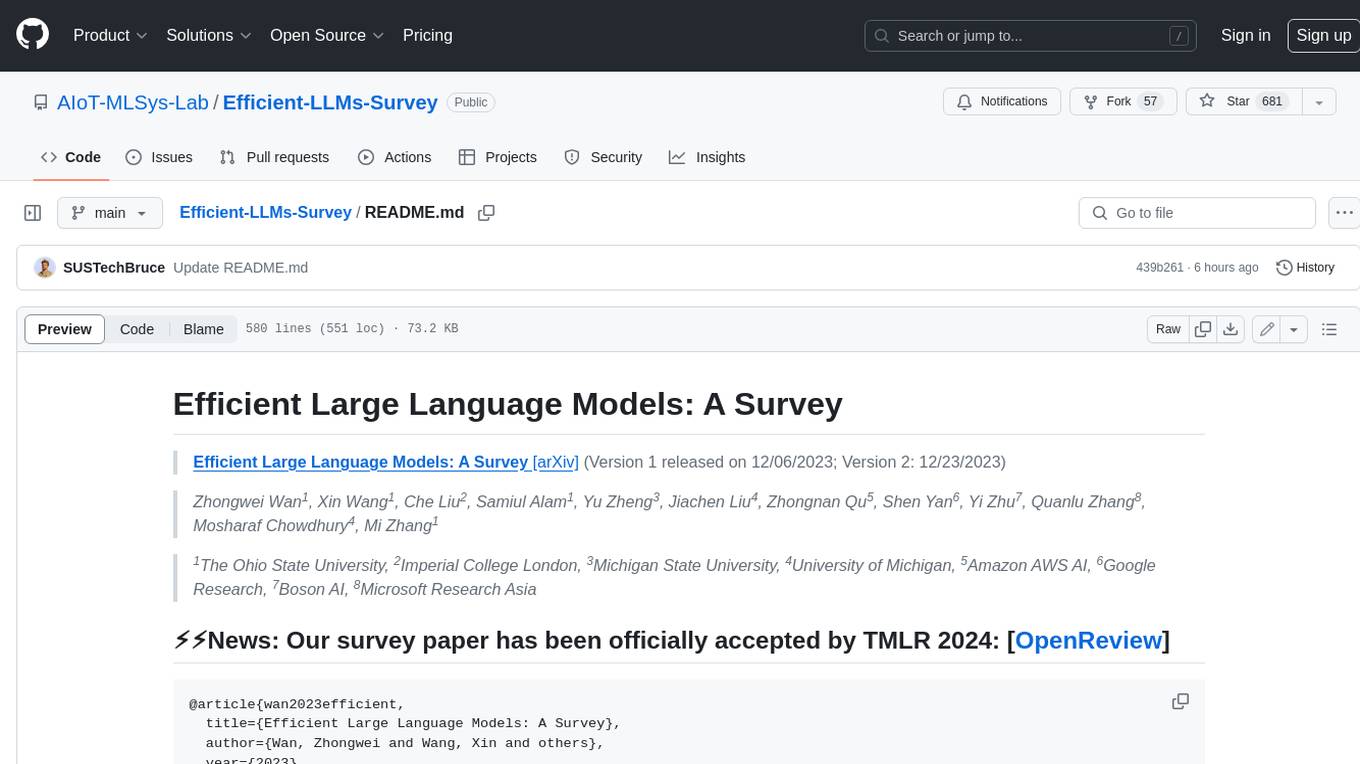
Efficient-LLMs-Survey
This repository provides a systematic and comprehensive review of efficient LLMs research. We organize the literature in a taxonomy consisting of three main categories, covering distinct yet interconnected efficient LLMs topics from **model-centric** , **data-centric** , and **framework-centric** perspective, respectively. We hope our survey and this GitHub repository can serve as valuable resources to help researchers and practitioners gain a systematic understanding of the research developments in efficient LLMs and inspire them to contribute to this important and exciting field.
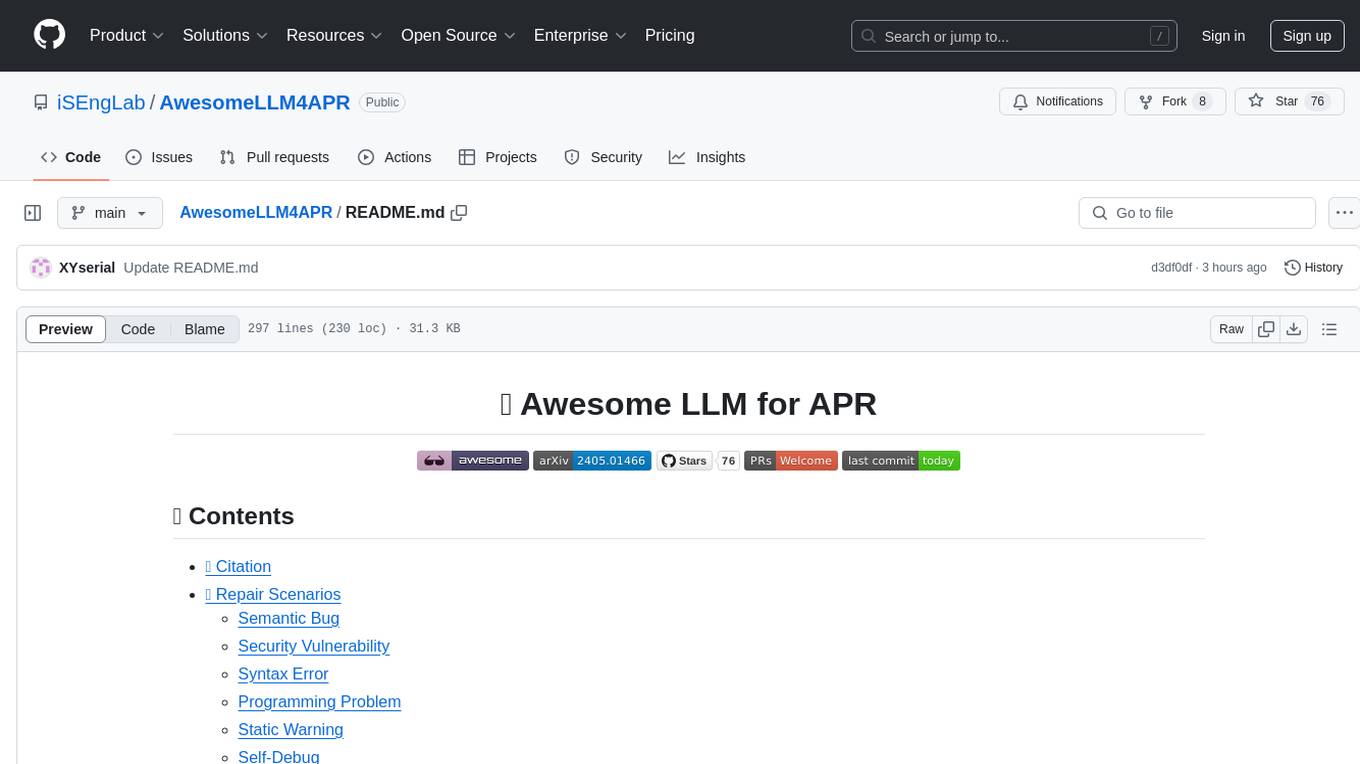
AwesomeLLM4APR
Awesome LLM for APR is a repository dedicated to exploring the capabilities of Large Language Models (LLMs) in Automated Program Repair (APR). It provides a comprehensive collection of research papers, tools, and resources related to using LLMs for various scenarios such as repairing semantic bugs, security vulnerabilities, syntax errors, programming problems, static warnings, self-debugging, type errors, web UI tests, smart contracts, hardware bugs, performance bugs, API misuses, crash bugs, test case repairs, formal proofs, GitHub issues, code reviews, motion planners, human studies, and patch correctness assessments. The repository serves as a valuable reference for researchers and practitioners interested in leveraging LLMs for automated program repair.
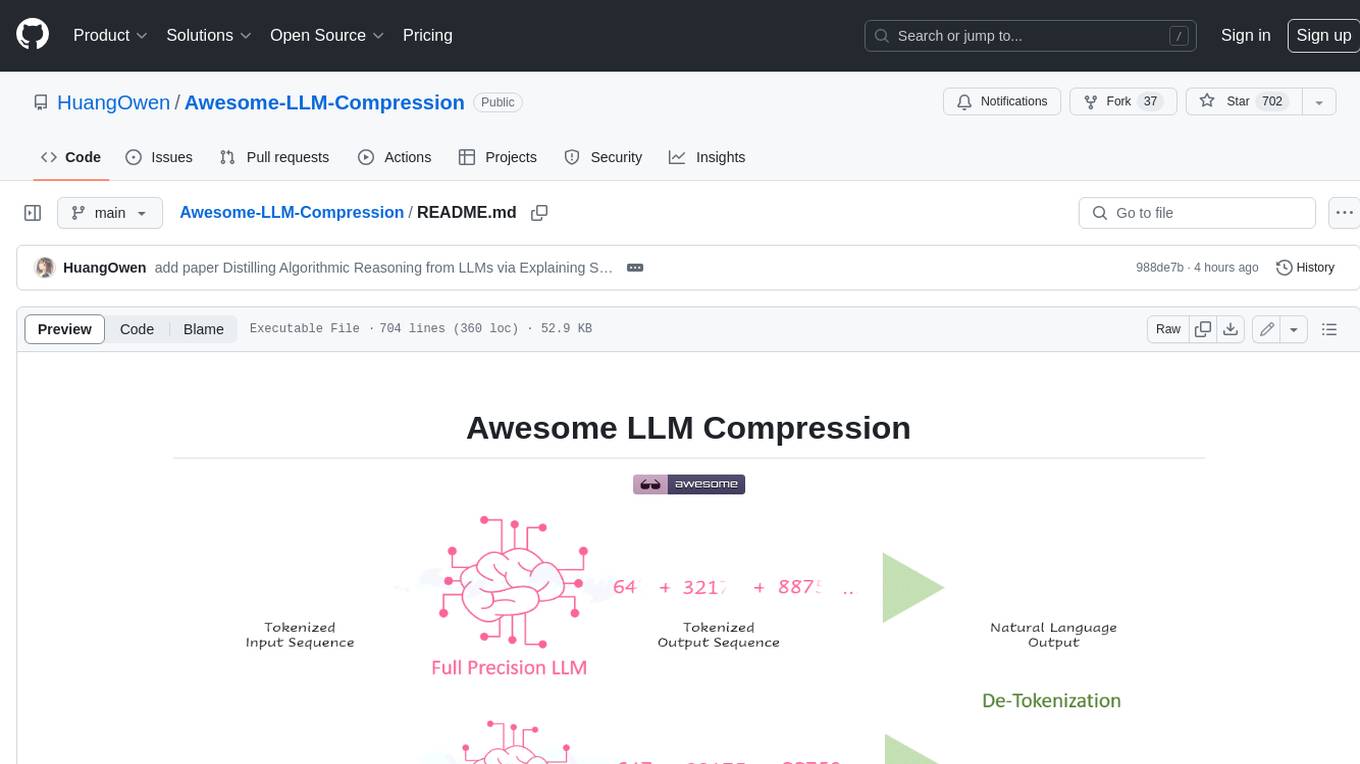
Awesome-LLM-Compression
Awesome LLM compression research papers and tools to accelerate LLM training and inference.
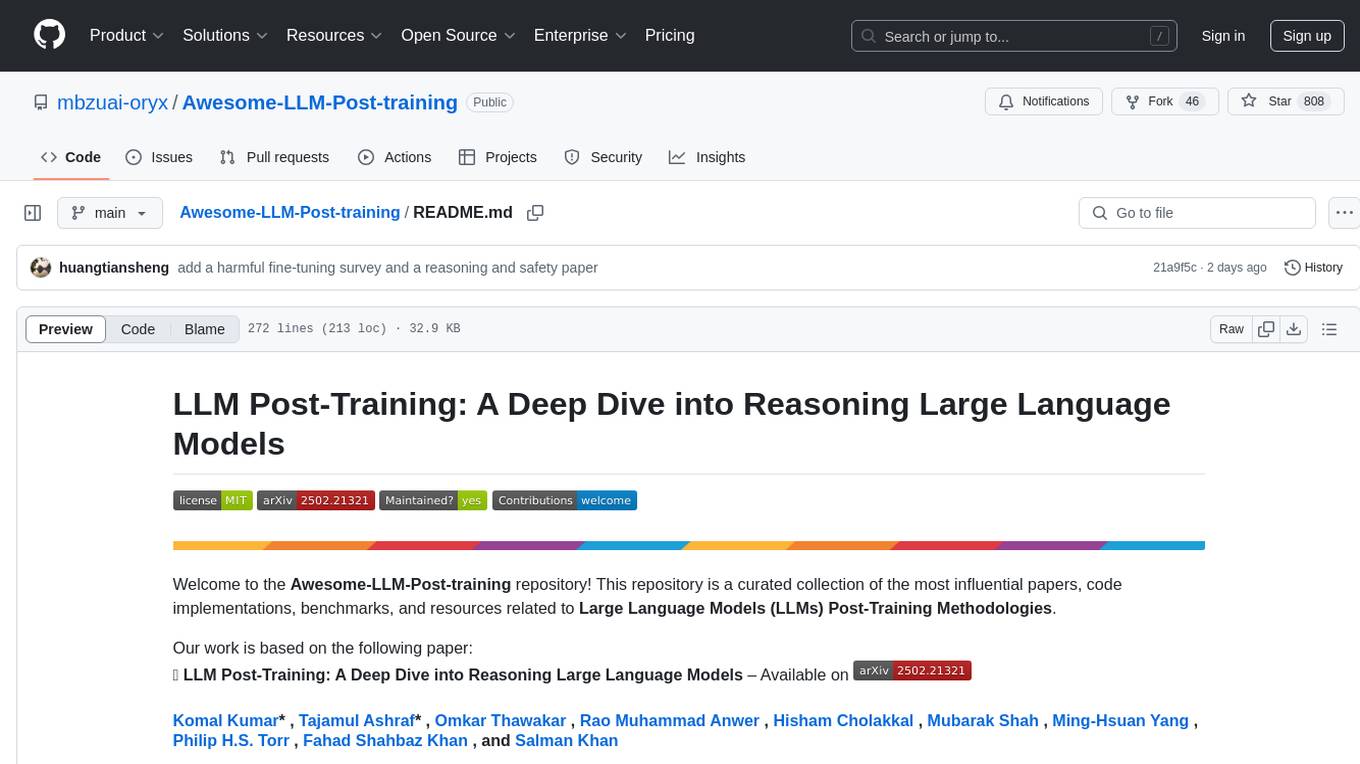
Awesome-LLM-Post-training
The Awesome-LLM-Post-training repository is a curated collection of influential papers, code implementations, benchmarks, and resources related to Large Language Models (LLMs) Post-Training Methodologies. It covers various aspects of LLMs, including reasoning, decision-making, reinforcement learning, reward learning, policy optimization, explainability, multimodal agents, benchmarks, tutorials, libraries, and implementations. The repository aims to provide a comprehensive overview and resources for researchers and practitioners interested in advancing LLM technologies.
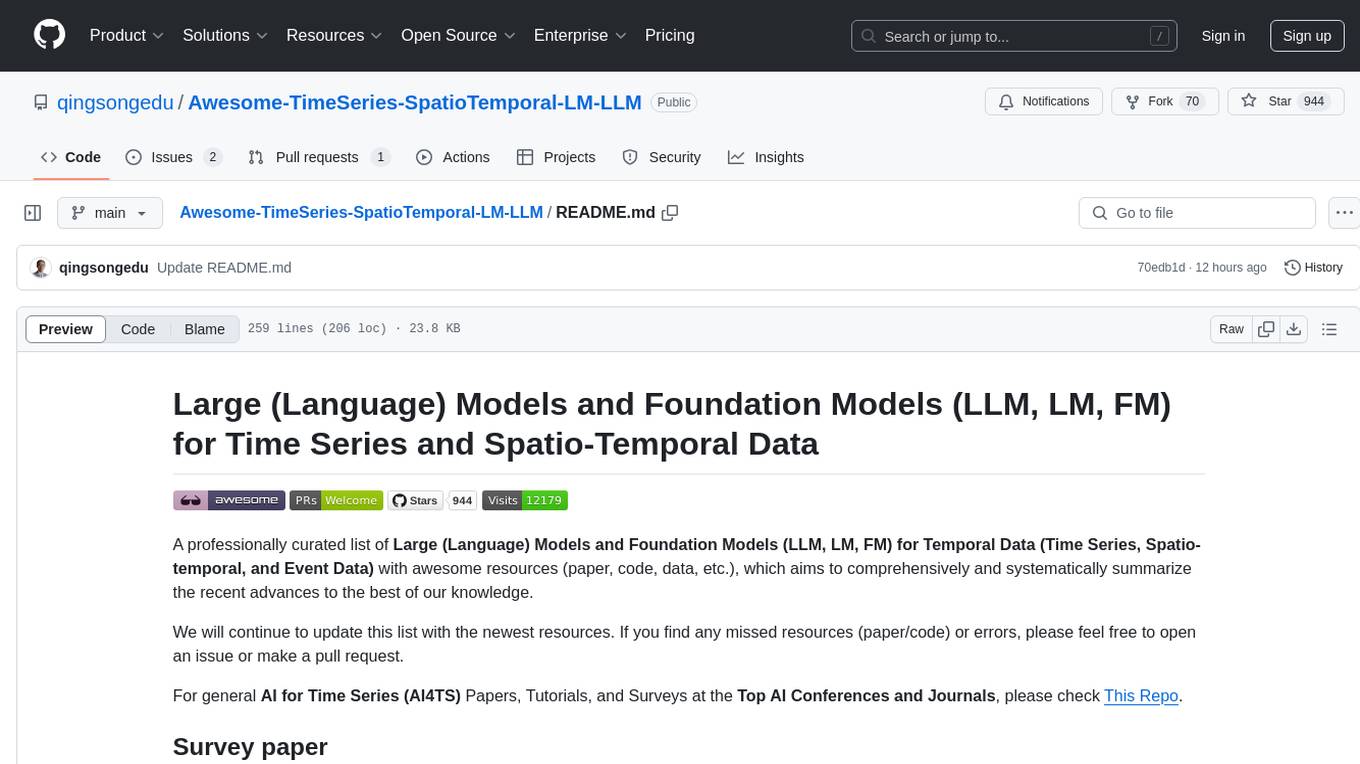
Awesome-TimeSeries-SpatioTemporal-LM-LLM
Awesome-TimeSeries-SpatioTemporal-LM-LLM is a curated list of Large (Language) Models and Foundation Models for Temporal Data, including Time Series, Spatio-temporal, and Event Data. The repository aims to summarize recent advances in Large Models and Foundation Models for Time Series and Spatio-Temporal Data with resources such as papers, code, and data. It covers various applications like General Time Series Analysis, Transportation, Finance, Healthcare, Event Analysis, Climate, Video Data, and more. The repository also includes related resources, surveys, and papers on Large Language Models, Foundation Models, and their applications in AIOps.
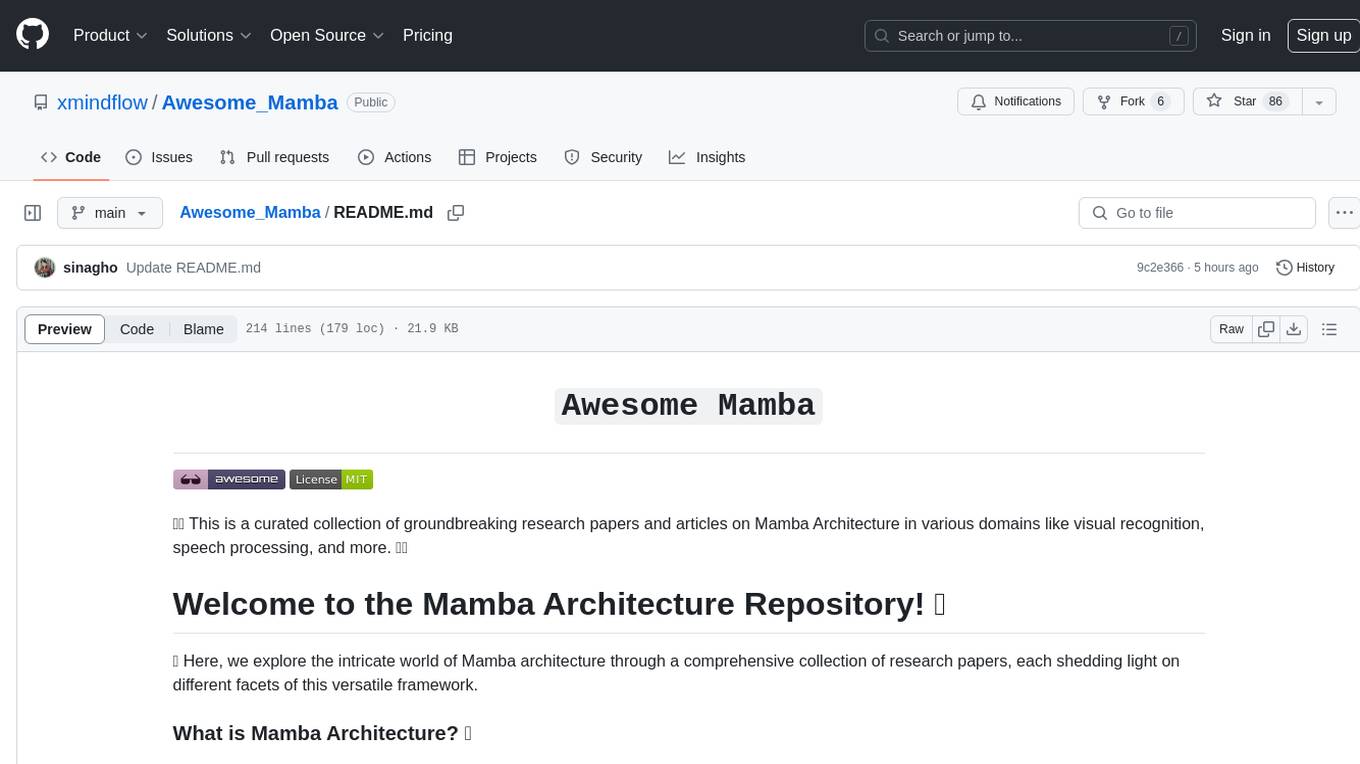
Awesome_Mamba
Awesome Mamba is a curated collection of groundbreaking research papers and articles on Mamba Architecture, a pioneering framework in deep learning known for its selective state spaces and efficiency in processing complex data structures. The repository offers a comprehensive exploration of Mamba architecture through categorized research papers covering various domains like visual recognition, speech processing, remote sensing, video processing, activity recognition, image enhancement, medical imaging, reinforcement learning, natural language processing, 3D recognition, multi-modal understanding, time series analysis, graph neural networks, point cloud analysis, and tabular data handling.
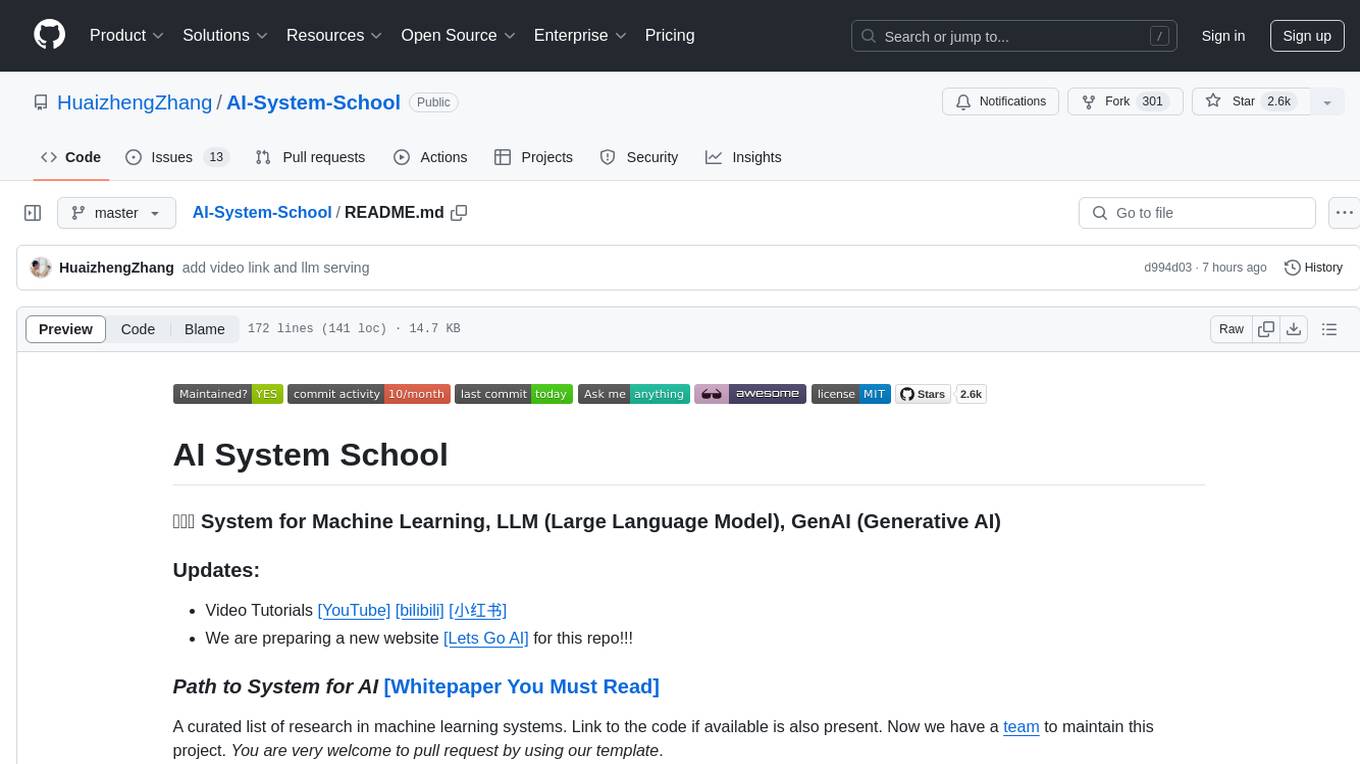
AI-System-School
AI System School is a curated list of research in machine learning systems, focusing on ML/DL infra, LLM infra, domain-specific infra, ML/LLM conferences, and general resources. It provides resources such as data processing, training systems, video systems, autoML systems, and more. The repository aims to help users navigate the landscape of AI systems and machine learning infrastructure, offering insights into conferences, surveys, books, videos, courses, and blogs related to the field.
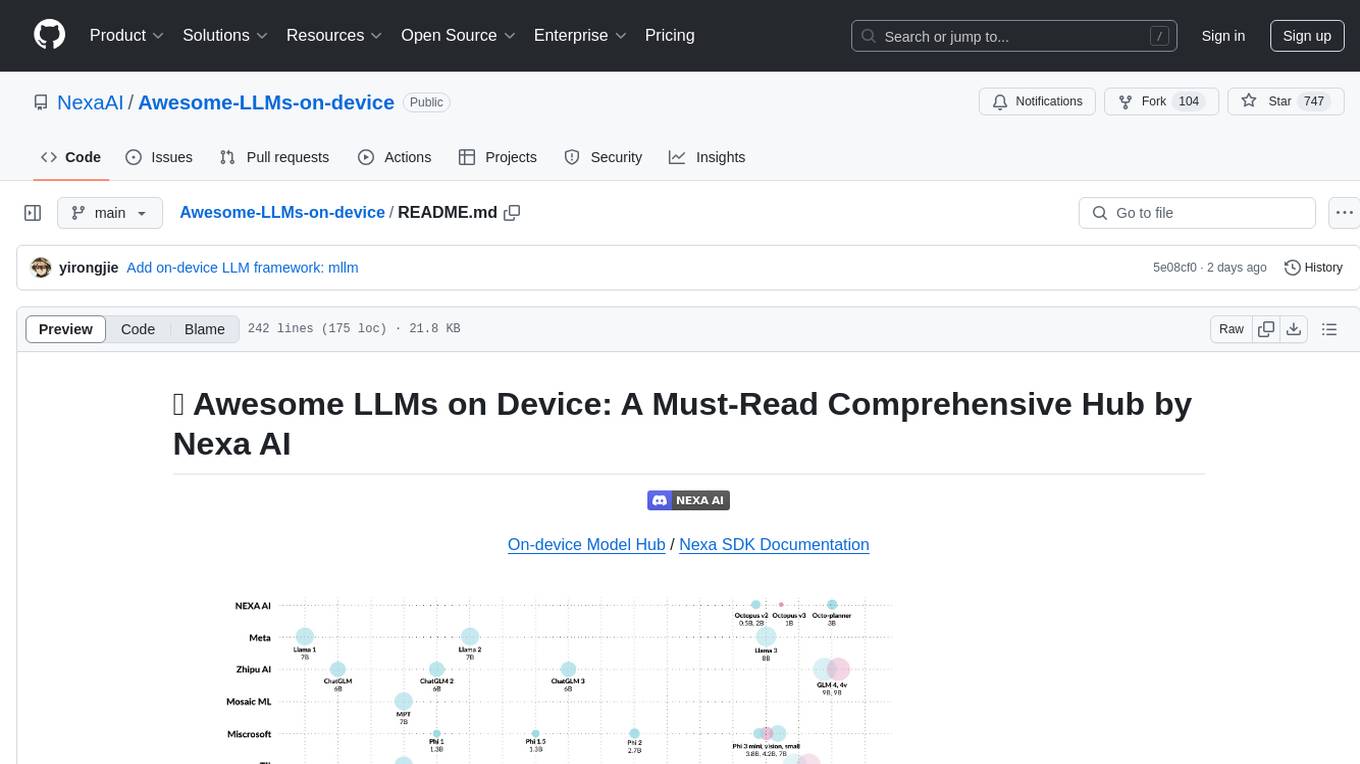
Awesome-LLMs-on-device
Welcome to the ultimate hub for on-device Large Language Models (LLMs)! This repository is your go-to resource for all things related to LLMs designed for on-device deployment. Whether you're a seasoned researcher, an innovative developer, or an enthusiastic learner, this comprehensive collection of cutting-edge knowledge is your gateway to understanding, leveraging, and contributing to the exciting world of on-device LLMs.
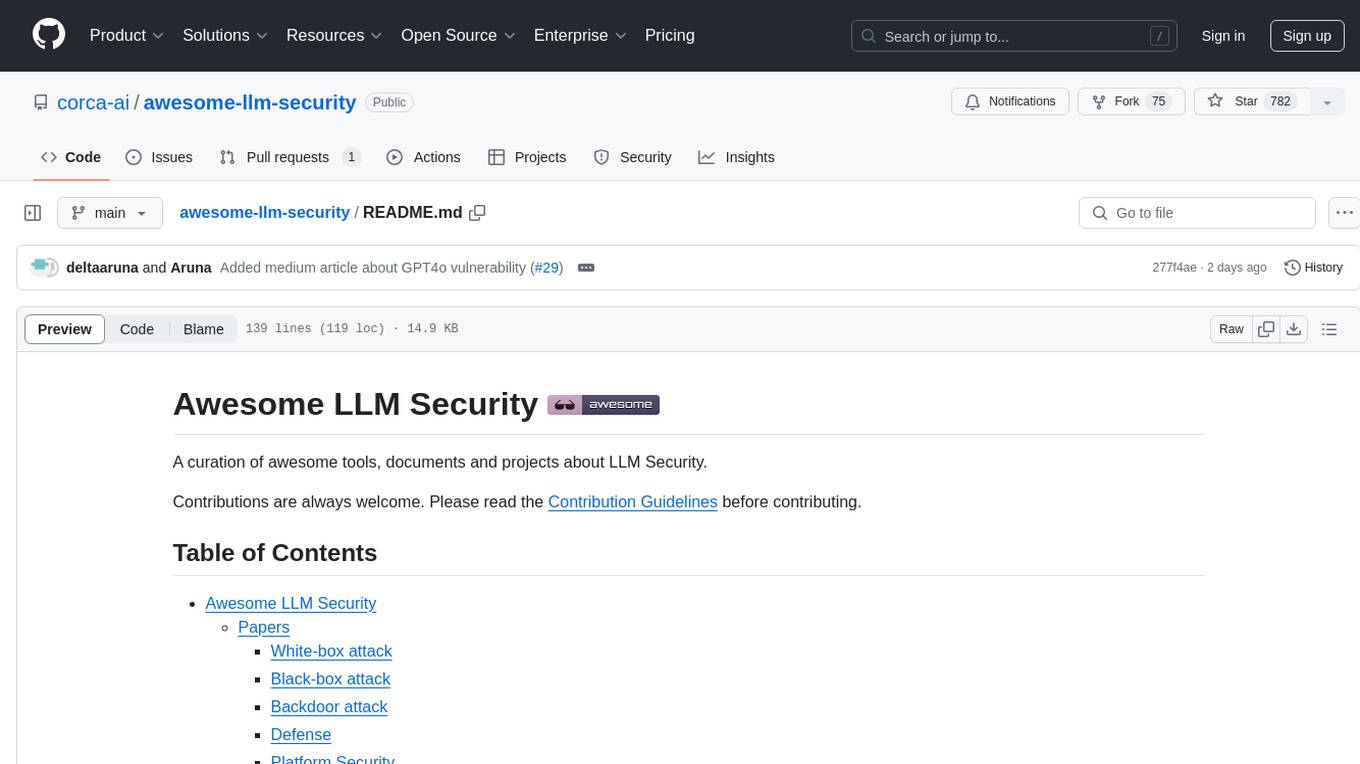
awesome-llm-security
Awesome LLM Security is a curated collection of tools, documents, and projects related to Large Language Model (LLM) security. It covers various aspects of LLM security including white-box, black-box, and backdoor attacks, defense mechanisms, platform security, and surveys. The repository provides resources for researchers and practitioners interested in understanding and safeguarding LLMs against adversarial attacks. It also includes a list of tools specifically designed for testing and enhancing LLM security.
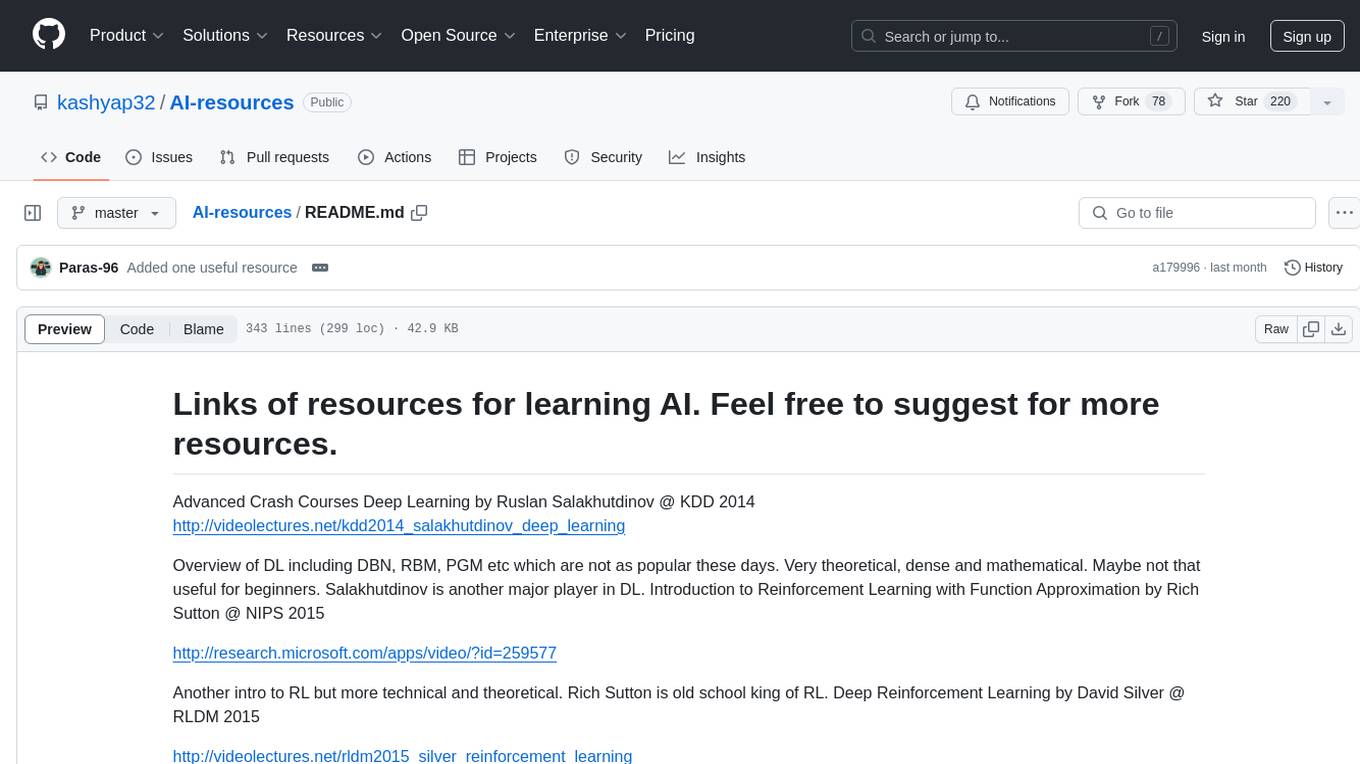
AI-resources
AI-resources is a repository containing links to various resources for learning Artificial Intelligence. It includes video lectures, courses, tutorials, and open-source libraries related to deep learning, reinforcement learning, machine learning, and more. The repository categorizes resources for beginners, average users, and advanced users/researchers, providing a comprehensive collection of materials to enhance knowledge and skills in AI.
For similar tasks
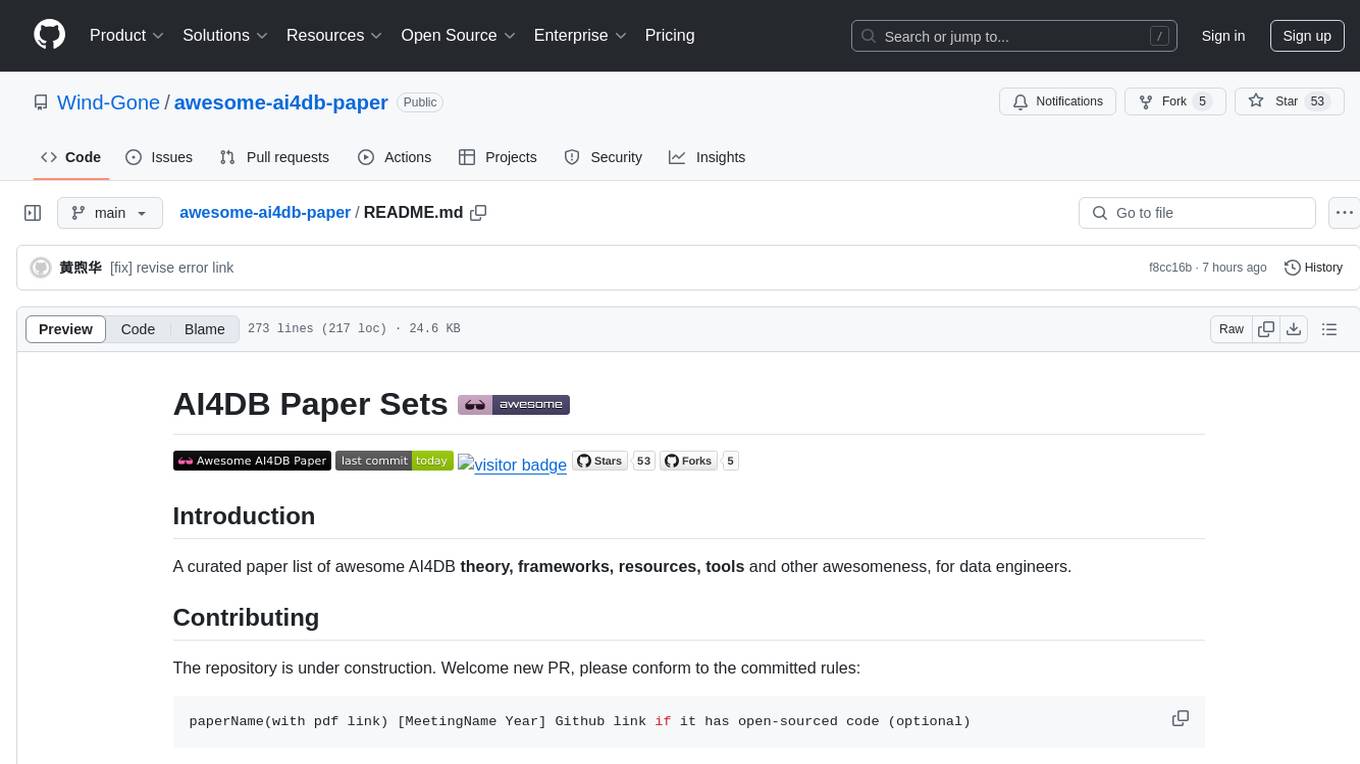
awesome-ai4db-paper
The 'awesome-ai4db-paper' repository is a curated paper list focusing on AI for database (AI4DB) theory, frameworks, resources, and tools for data engineers. It includes a collection of research papers related to learning-based query optimization, training data set preparation, cardinality estimation, query-driven approaches, data-driven techniques, hybrid methods, pretraining models, plan hints, cost models, SQL embedding, join order optimization, query rewriting, end-to-end systems, text-to-SQL conversion, traditional database technologies, storage solutions, learning-based index design, and a learning-based configuration advisor. The repository aims to provide a comprehensive resource for individuals interested in AI applications in the field of database management.
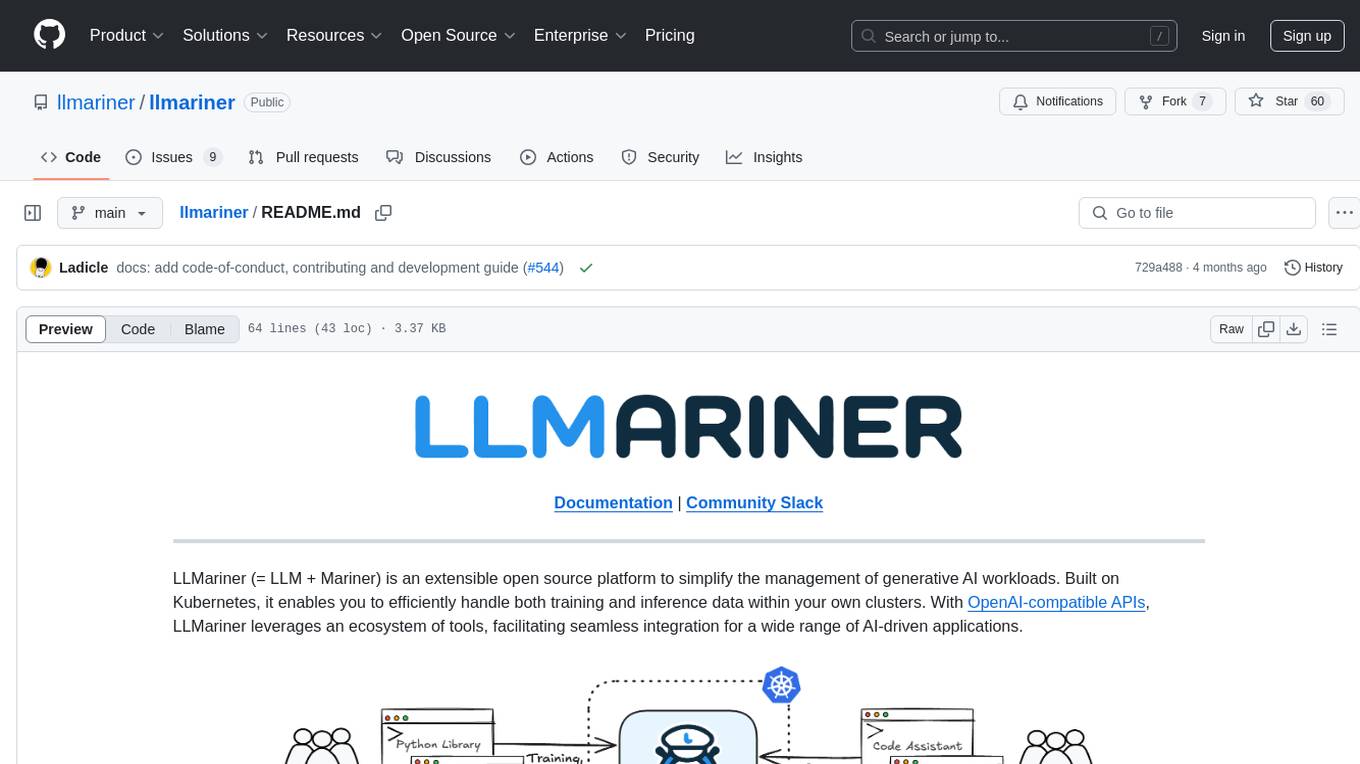
llmariner
LLMariner is an extensible open source platform built on Kubernetes to simplify the management of generative AI workloads. It enables efficient handling of training and inference data within clusters, with OpenAI-compatible APIs for seamless integration with a wide range of AI-driven applications.
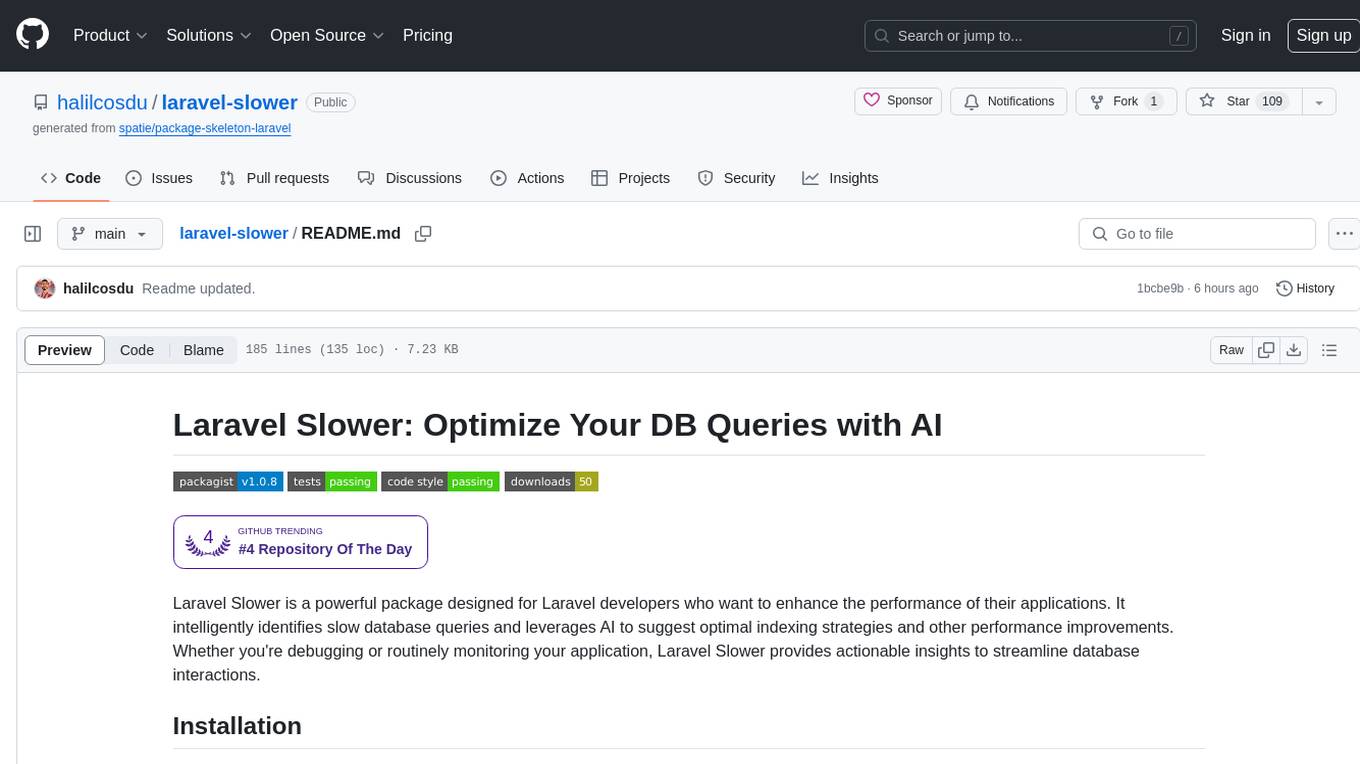
laravel-slower
Laravel Slower is a powerful package designed for Laravel developers to optimize the performance of their applications by identifying slow database queries and providing AI-driven suggestions for optimal indexing strategies and performance improvements. It offers actionable insights for debugging and monitoring database interactions, enhancing efficiency and scalability.
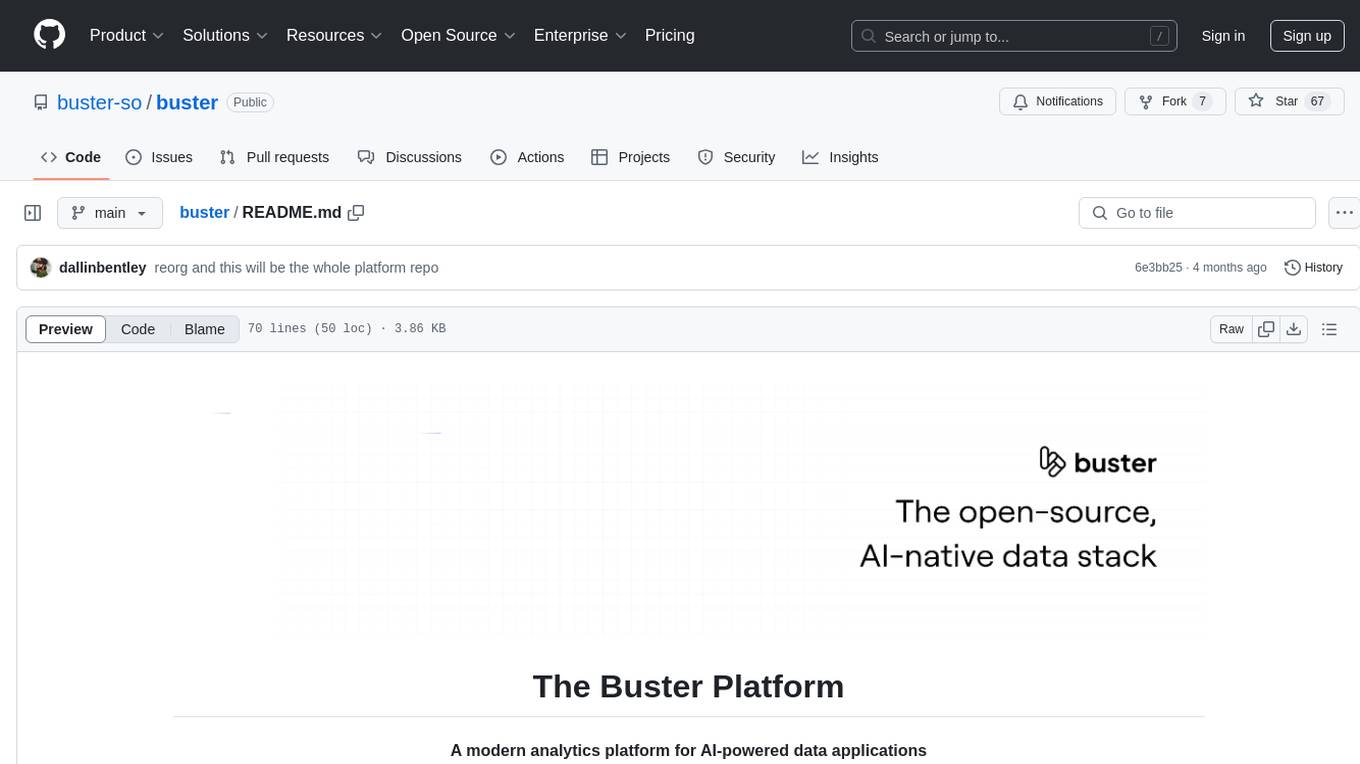
buster
Buster is a modern analytics platform designed with AI in mind, focusing on self-serve experiences powered by Large Language Models. It addresses pain points in existing tools by advocating for AI-centric app development, cost-effective data warehousing, improved CI/CD processes, and empowering data teams to create powerful, user-friendly data experiences. The platform aims to revolutionize AI analytics by enabling data teams to build deep integrations and own their entire analytics stack.
For similar jobs
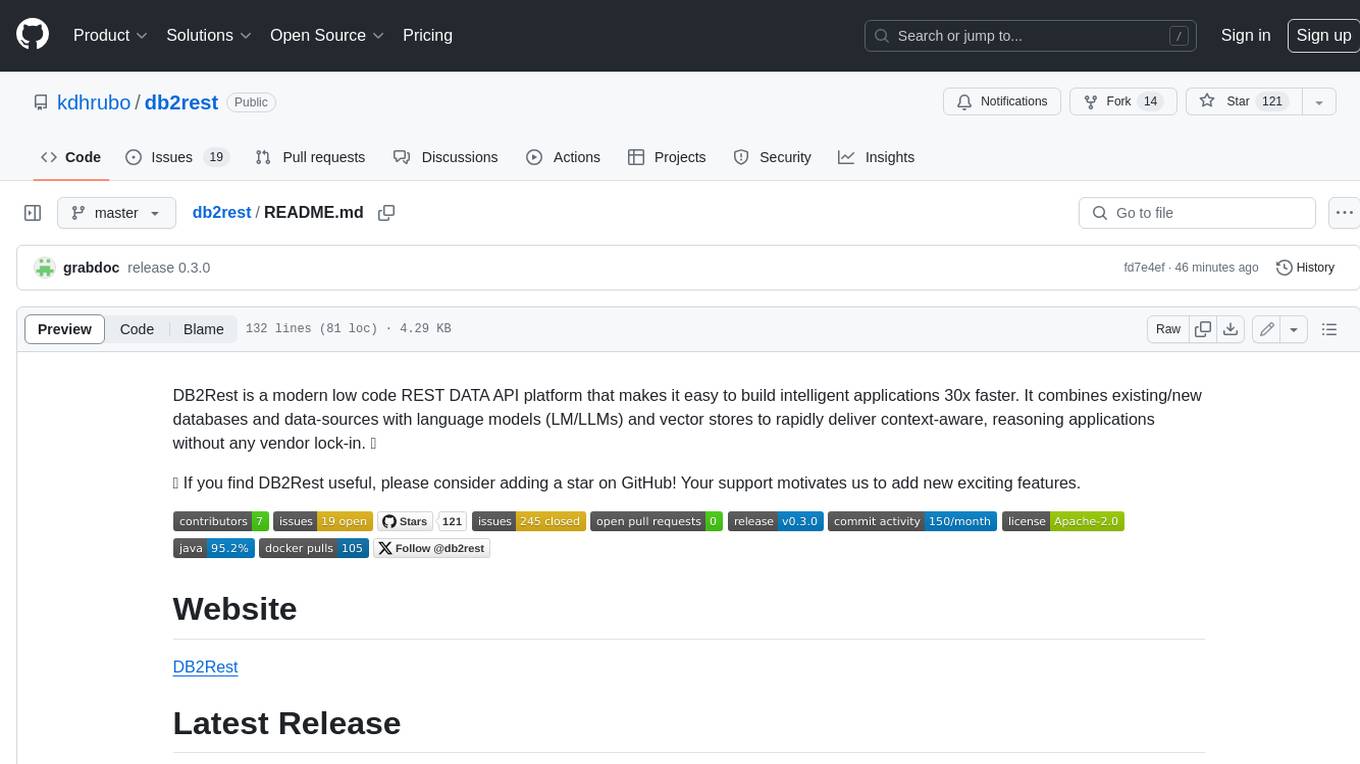
db2rest
DB2Rest is a modern low-code REST DATA API platform that simplifies the development of intelligent applications. It seamlessly integrates existing and new databases with language models (LMs/LLMs) and vector stores, enabling the rapid delivery of context-aware, reasoning applications without vendor lock-in.
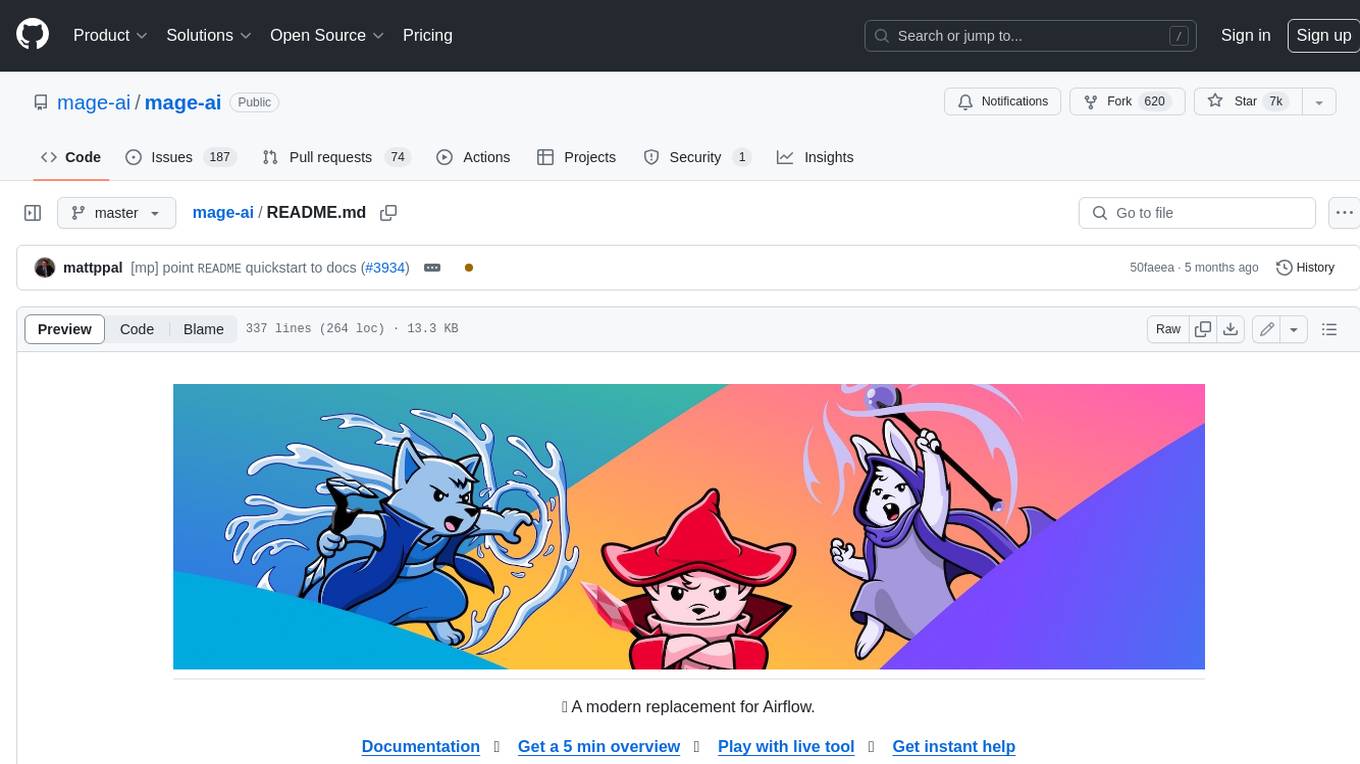
mage-ai
Mage is an open-source data pipeline tool for transforming and integrating data. It offers an easy developer experience, engineering best practices built-in, and data as a first-class citizen. Mage makes it easy to build, preview, and launch data pipelines, and provides observability and scaling capabilities. It supports data integrations, streaming pipelines, and dbt integration.
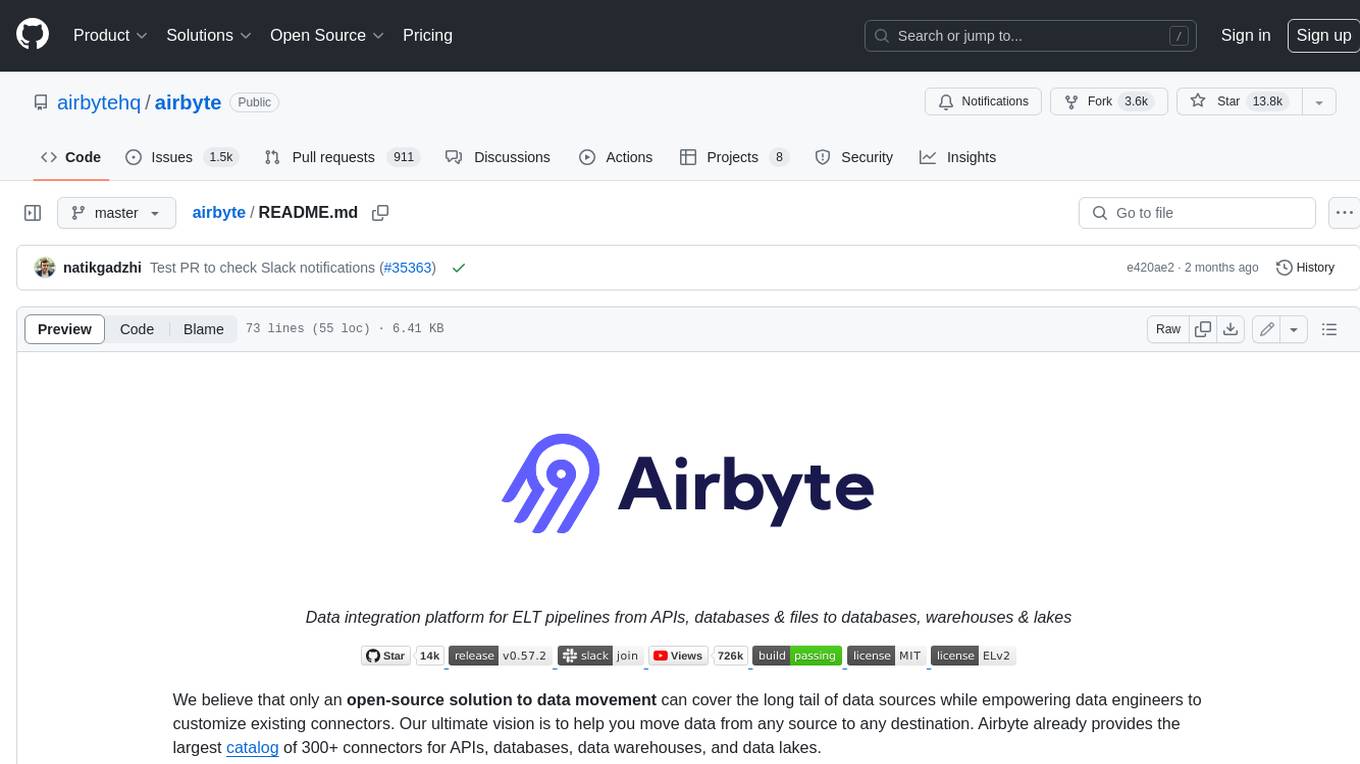
airbyte
Airbyte is an open-source data integration platform that makes it easy to move data from any source to any destination. With Airbyte, you can build and manage data pipelines without writing any code. Airbyte provides a library of pre-built connectors that make it easy to connect to popular data sources and destinations. You can also create your own connectors using Airbyte's no-code Connector Builder or low-code CDK. Airbyte is used by data engineers and analysts at companies of all sizes to build and manage their data pipelines.
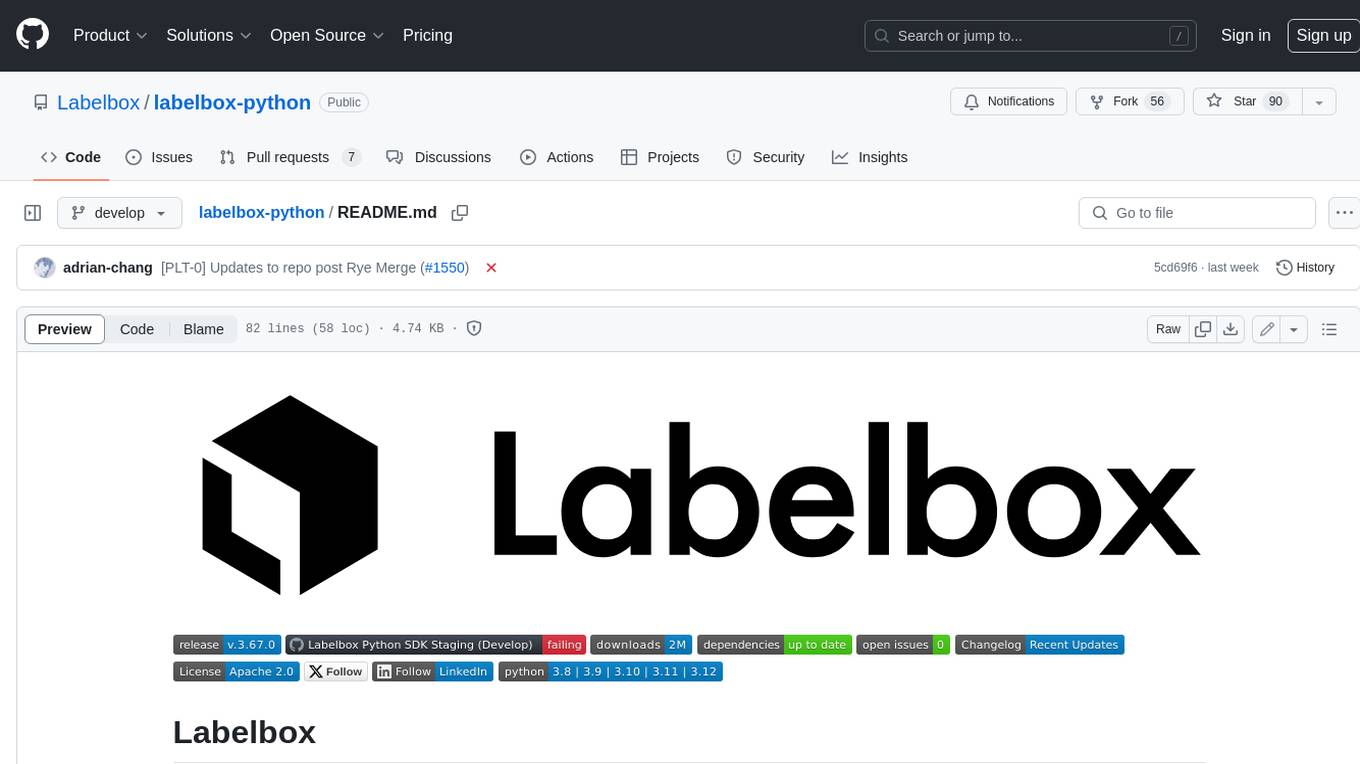
labelbox-python
Labelbox is a data-centric AI platform for enterprises to develop, optimize, and use AI to solve problems and power new products and services. Enterprises use Labelbox to curate data, generate high-quality human feedback data for computer vision and LLMs, evaluate model performance, and automate tasks by combining AI and human-centric workflows. The academic & research community uses Labelbox for cutting-edge AI research.
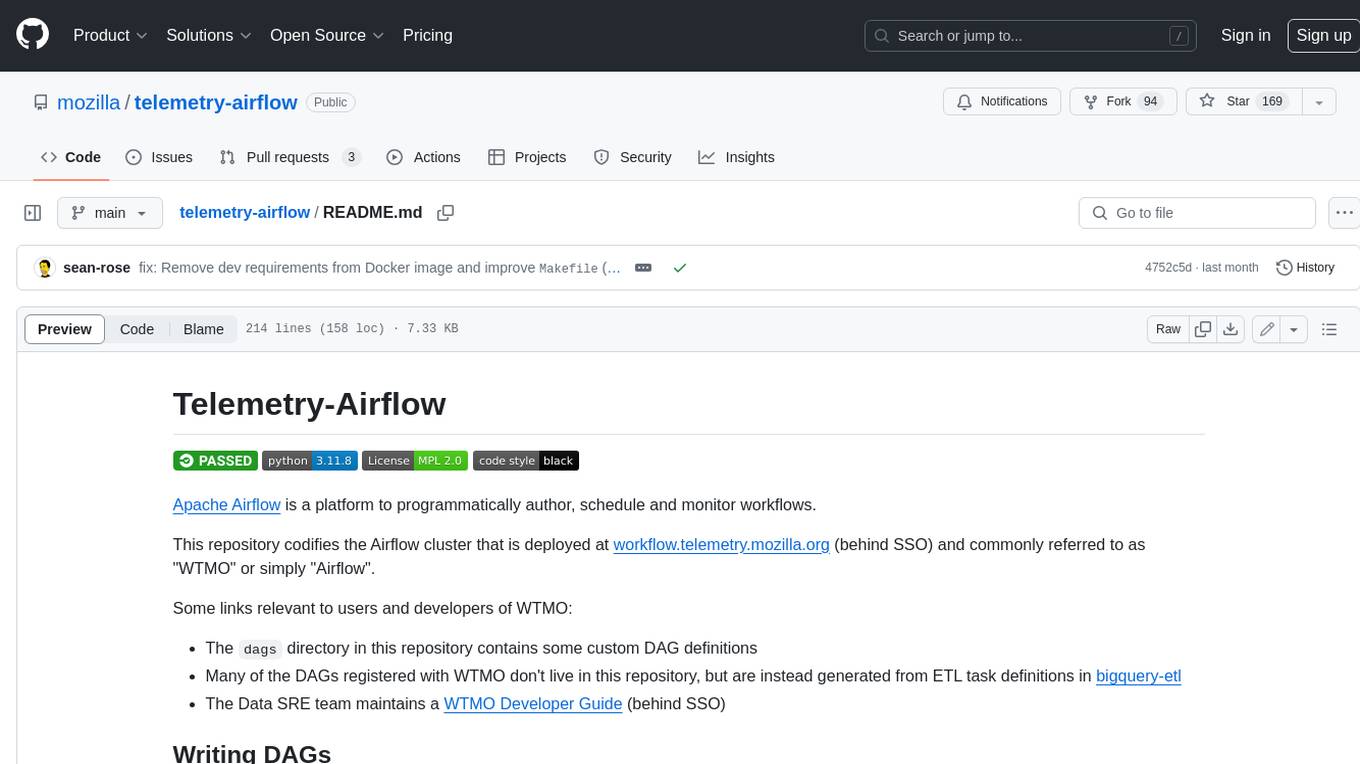
telemetry-airflow
This repository codifies the Airflow cluster that is deployed at workflow.telemetry.mozilla.org (behind SSO) and commonly referred to as "WTMO" or simply "Airflow". Some links relevant to users and developers of WTMO: * The `dags` directory in this repository contains some custom DAG definitions * Many of the DAGs registered with WTMO don't live in this repository, but are instead generated from ETL task definitions in bigquery-etl * The Data SRE team maintains a WTMO Developer Guide (behind SSO)
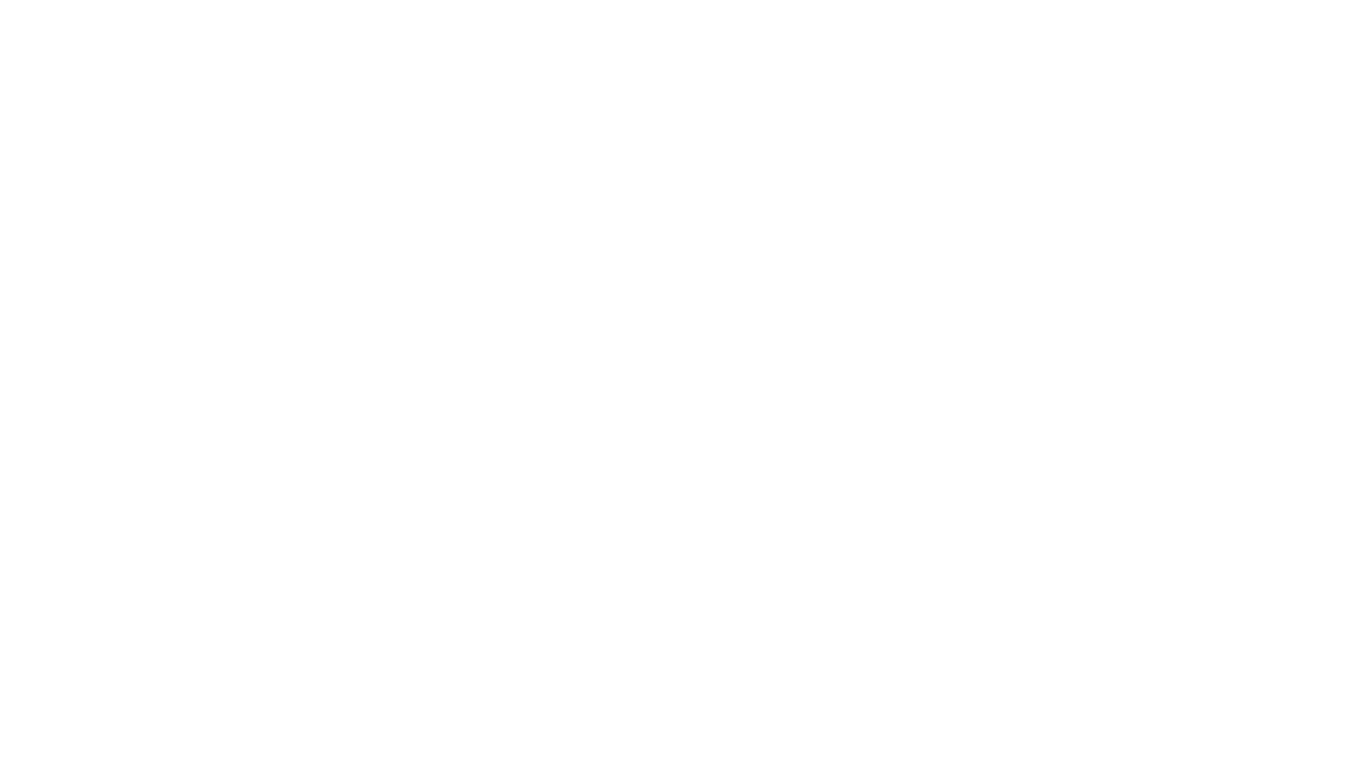
airflow
Apache Airflow (or simply Airflow) is a platform to programmatically author, schedule, and monitor workflows. When workflows are defined as code, they become more maintainable, versionable, testable, and collaborative. Use Airflow to author workflows as directed acyclic graphs (DAGs) of tasks. The Airflow scheduler executes your tasks on an array of workers while following the specified dependencies. Rich command line utilities make performing complex surgeries on DAGs a snap. The rich user interface makes it easy to visualize pipelines running in production, monitor progress, and troubleshoot issues when needed.
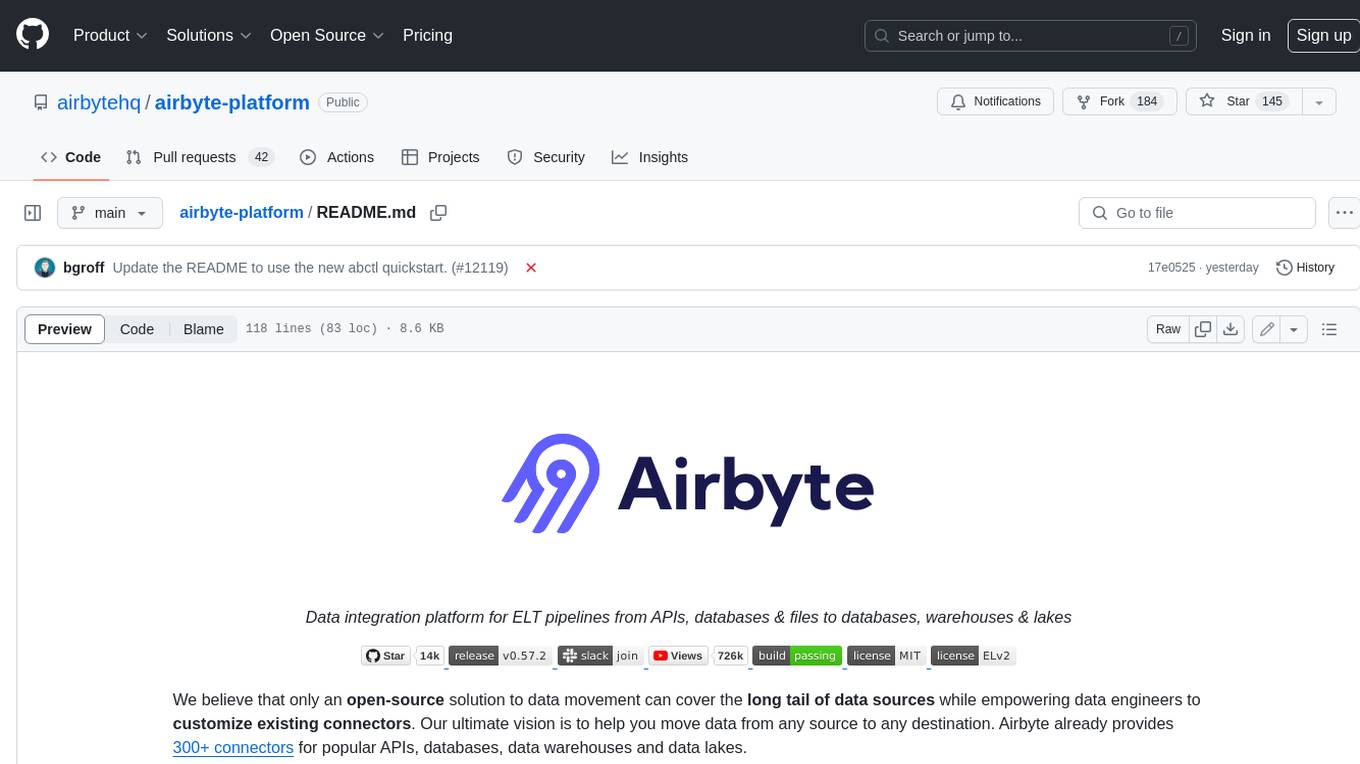
airbyte-platform
Airbyte is an open-source data integration platform that makes it easy to move data from any source to any destination. With Airbyte, you can build and manage data pipelines without writing any code. Airbyte provides a library of pre-built connectors that make it easy to connect to popular data sources and destinations. You can also create your own connectors using Airbyte's low-code Connector Development Kit (CDK). Airbyte is used by data engineers and analysts at companies of all sizes to move data for a variety of purposes, including data warehousing, data analysis, and machine learning.
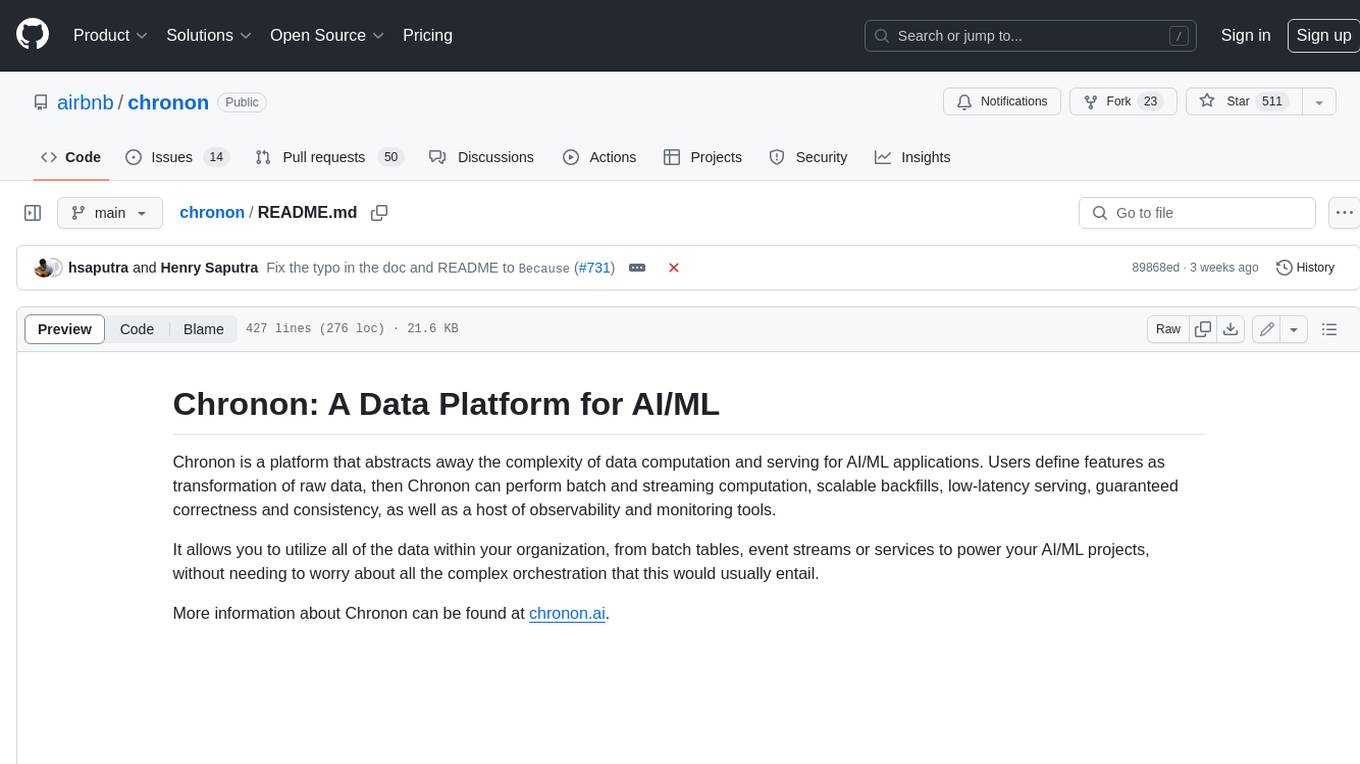
chronon
Chronon is a platform that simplifies and improves ML workflows by providing a central place to define features, ensuring point-in-time correctness for backfills, simplifying orchestration for batch and streaming pipelines, offering easy endpoints for feature fetching, and guaranteeing and measuring consistency. It offers benefits over other approaches by enabling the use of a broad set of data for training, handling large aggregations and other computationally intensive transformations, and abstracting away the infrastructure complexity of data plumbing.