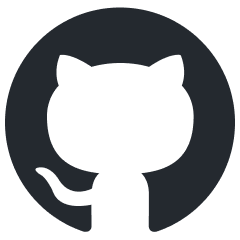
LLM-Tuning
Tuning LLMs with no tears💦; Sample Design Engineering (SDE) for more efficient downstream-tuning.
Stars: 897
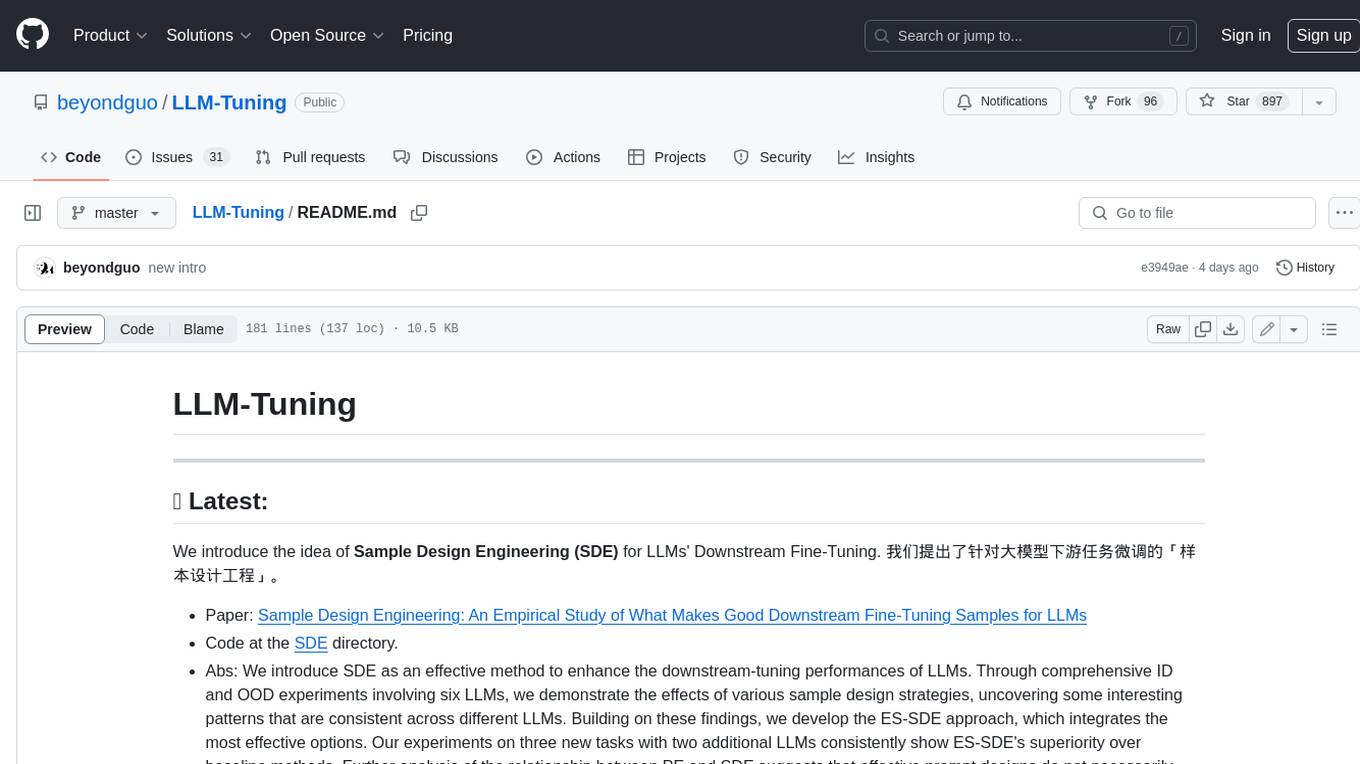
LLM-Tuning is a collection of tools and resources for fine-tuning large language models (LLMs). It includes a library of pre-trained LoRA models, a set of tutorials and examples, and a community forum for discussion and support. LLM-Tuning makes it easy to fine-tune LLMs for a variety of tasks, including text classification, question answering, and dialogue generation. With LLM-Tuning, you can quickly and easily improve the performance of your LLMs on downstream tasks.
README:
We introduce the idea of Sample Design Engineering (SDE) for LLMs' Downstream Fine-Tuning. 我们提出了针对大模型下游任务微调的「样本设计工程」。
- Paper: Sample Design Engineering: An Empirical Study of What Makes Good Downstream Fine-Tuning Samples for LLMs
- Code at the SDE directory.
- Abs: We introduce SDE as an effective method to enhance the downstream-tuning performances of LLMs. Through comprehensive ID and OOD experiments involving six LLMs, we demonstrate the effects of various sample design strategies, uncovering some interesting patterns that are consistent across different LLMs. Building on these findings, we develop the ES-SDE approach, which integrates the most effective options. Our experiments on three new tasks with two additional LLMs consistently show ES-SDE's superiority over baseline methods. Further analysis of the relationship between PE and SDE suggests that effective prompt designs do not necessarily translate to successful sample designs. This observation opens up avenues for more detailed investigations into the mechanisms of SDE in future research.
- 简介:提示工程(Prompt Engineering)已经成为提升大模型的零样本、少样本推理能力的基本操作。然而,在大模型实际落地解决下游业务问题的时候,我们往往还需要一些针对性的样本对模型进行微调训练。我们在大模型实际落地研发中发现:虽然大模型已经足够强大,但是微调样本的不同设计,依然会显著影响大模型微调后的效果。因此,如何设计更好的微调样本,成为了一个新的问题。对此,本文首次提出了样本设计工程(Sample Design Engineering, SDE)的概念,系统性地探究了影响大模型下游任务微调的多种设计选项,发现了诸多有趣且引人深思的结论,并提出了一种在多个复杂下游任务上均稳定优异设计方案。本研究表明,细致地考虑大模型微调样本的设计,可以使用更少的样本训练出在下游任务上表现更好的模型。
💻 可复现的小项目:
- baichuan-RLHF:基于 LoRA 的 RLHF 教程,让 baichaun 活泼如网友!(New!🔥)
- ChatBaichuan:基于 HC3 数据集让 百川大模型(baichuan-7B)有对话能力!
- 【娱乐向】RulaiGPT:如来~诶,它真来了吗?如~来~(拍桌!)
💬 相关讨论区:
🤖 目前支持:
- Meta LLaMA2 的 LoRA 微调
- 通义千问大模型 Qwen1.5 的 LoRA 微调
- 中文羊驼大模型 Chinese-LLaMA-Alpaca 的 LoRA 微调
- 上海 AILab 书生大模型 InternLM-7B 的 LoRA 微调
- 百川智能 Baichaun-7B, Baichuan2-7B 的 LoRA 微调和 RLHF 全流程
- 清华 ChatGLM2-6B 的 LoRA 微调
- 清华 ChatGLM-6B 的 LoRA 微调
🎯 两行代码开启 LoRA 训练:
- 数据集分词预处理:
sh tokenize.sh
,对比不同的 LLM,需在 tokenize.sh 文件里切换 model_checkpoint 参数 - 开启 LoRA 微调:
sh train.sh
,对于不同的 LLM,需切换不同的 python 文件来执行:- ChatGLM-6B 应使用
chatglm_lora_tuning.py
- ChatGLM2-6B 应使用
chatglm2_lora_tuning.py
- baichuan-7B 应使用
baichuan_lora_tuning.py
- baichuan2-7B 应使用
baichuan2_lora_tuning.py
- internlm-chat/base-7b 应使用
intermlm_lora_tuning.py
- chinese-llama2/alpaca2-7b 应使用
chinese_llama2_alpaca2_lora_tuning.py
- ChatGLM-6B 应使用
🎯 手把手的 RLHF 教程:见 LoRA-based-RLHF
环境准备:
pip install transformers datasets accelerate sentencepiece tensorboard peft
目前测试的环境为:
- Python 3.9.16
- torch, Version: 2.0.1
- transformers, Version: 4.29.1
- datasets, Version: 2.12.0
- accelerate, Version: 0.19.0
- peft, Version: 0.3.0
- sentencepiece, Version: 0.1.99
- tensorboard, Version: 2.13.0
下面的教程以及代码使用 ChatGLM-6B
作为例子,如果更换其他模型,可能需要略微修改具体文件代码。
原始文件的准备
指令微调数据一般有输入和输出两部分,输入是特定的content加上instruction,这里我们将二者直接拼在一起,不单独区分;输出则是希望模型的回答。
我们统一使用json
的格式在整理数据,可以自定义输出输出的字段名,例如下面的例子中我使用的是q
和a
代表模型的输入和输出:
{"q": "请计算:39 * 0 = 什么?", "a": "这是简单的乘法运算,39乘以0得到的是0"}
{"q": "题目:51/186的答案是什么?", "a": "这是简单的除法运算,51除以186大概为0.274"}
{"q": "鹿妈妈买了24个苹果,她想平均分给她的3只小鹿吃,每只小鹿可以分到几个苹果?", "a": "鹿妈妈买了24个苹果,平均分给3只小鹿吃,那么每只小鹿可以分到的苹果数就是总苹果数除以小鹿的只数。\n24÷3=8\n每只小鹿可以分到8个苹果。所以,答案是每只小鹿可以分到8个苹果。"}
...
整理好数据后,保存为.json
或者.jsonl
文件,然后放入目录中的data/
文件夹中。
对数据集进行分词
为了避免每次训练的时候都要重新对数据集分词,我们先分好词形成特征后保存成可直接用于训练的数据集。
例如,
- 我们的原始指令微调文件为:
data/
文件夹下的simple_math_4op.json
文件 - 输入字段为
q
,输出字段为a
- 希望经过 tokenize 之后保存到
data/tokenized_data/
下名为simple_math_4op
的文件夹中 - 设定文本最大程度为 2000
则我们可以直接使用下面这段命令(即tokenize.sh
文件)进行处理:
CUDA_VISIBLE_DEVICES=0,1 python tokenize_dataset_rows.py \
--model_checkpoint THUDM/chatglm-6b \
--input_file simple_math_4op.json \
--prompt_key q \
--target_key a \
--save_name simple_math_4op \
--max_seq_length 2000 \
--skip_overlength False
处理完毕之后,我们会在 data/tokenized_data/
下发现名为 simple_math_4op
的文件夹,这就是下一步中我们可以直接用于训练的数据。
得到 tokenize 之后的数据集,就可以直接运行 chatglm_lora_tuning.py
来训练 LoRA 模型了,具体可设置的主要参数包括:
-
tokenized_dataset
, 分词后的数据集,即在 data/tokenized_data/ 地址下的文件夹名称 -
lora_rank
, 设置 LoRA 的秩,推荐为4或8,显存够的话使用8 -
per_device_train_batch_size
, 每块 GPU 上的 batch size -
gradient_accumulation_steps
, 梯度累加,可以在不提升显存占用的情况下增大 batch size -
max_steps
, 训练步数 -
save_steps
, 多少步保存一次 -
save_total_limit
, 保存多少个checkpoint -
logging_steps
, 多少步打印一次训练情况(loss, lr, etc.) -
output_dir
, 模型文件保存地址
例如我们的数据集为 simple_math_4op,希望保存到 weights/simple_math_4op ,则执行下面命令(即train.sh
文件):
CUDA_VISIBLE_DEVICES=2,3 python chatglm_lora_tuning.py \
--tokenized_dataset simple_math_4op \
--lora_rank 8 \
--per_device_train_batch_size 10 \
--gradient_accumulation_steps 1 \
--max_steps 100000 \
--save_steps 200 \
--save_total_limit 2 \
--learning_rate 1e-4 \
--fp16 \
--remove_unused_columns false \
--logging_steps 50 \
--output_dir weights/simple_math_4op
训练完之后,可以在 output_dir 中找到 LoRA 的相关模型权重,主要是adapter_model.bin
和adapter_config.json
两个文件。
如何查看 tensorboard:
- 在 output_dir 中找到 runs 文件夹,复制其中日期最大的文件夹的地址,假设为
your_log_path
- 执行
tensorboard --logdir your_log_path
命令,就会在 http://localhost:6006/ 上开启tensorboard - 如果是在服务器上开启,则还需要做端口映射到本地。推荐使用 VSCode 在服务器上写代码,可以自动帮你进行端口映射。
- 如果要自己手动进行端口映射,具体方式是在使用 ssh 登录时,后面加上
-L 6006:127.0.0.1:6006
参数,将服务器端的6006端口映射到本地的6006端口。
我们可以把上面的 output_dir 打包带走,假设文件夹为 weights/simple_math_4op
, 其中(至少)包含 adapter_model.bin
和 adapter_config.json
两个文件,则我们可以用下面的方式直接加载,并推理
from peft import PeftModel
from transformers import AutoTokenizer, AutoModel
import torch
device = torch.device(1)
# 加载原始 LLM
model_path = "THUDM/chatglm-6b"
model = AutoModel.from_pretrained(model_path, trust_remote_code=True).half().to(device)
tokenizer = AutoTokenizer.from_pretrained(model_path, trust_remote_code=True)
model.chat(tokenizer, "你好", history=[])
# 给原始 LLM 安装上你的 LoRA tool
model = PeftModel.from_pretrained(model, "weights/simple_math_4op").half()
model.chat(tokenizer, "你好", history=[])
理论上,可以通过多次执行 model = PeftModel.from_pretrained(model, "weights/simple_math_4op").half()
的方式,加载多个 LoRA 模型,从而混合不同Tool的能力,但实际测试的时候,由于暂时还不支持设置不同 LoRA weights的权重,往往效果不太好,存在覆盖或者遗忘的情况。
- 首先最感谢的是 🤗Huggingface 团队开源的 peft 工具包,懂的都懂!
- ChatGLM 的 LoRA 微调代码主要基于 ChatGLM-Tuning 项目中的 LoRA 微调部分修改而来;
- baichuan-7B 微调部分,参考了 LLaMA-Efficient-Tuning 项目中的解决方案;
对这些优秀开源项目表示感谢!
For Tasks:
Click tags to check more tools for each tasksFor Jobs:
Alternative AI tools for LLM-Tuning
Similar Open Source Tools
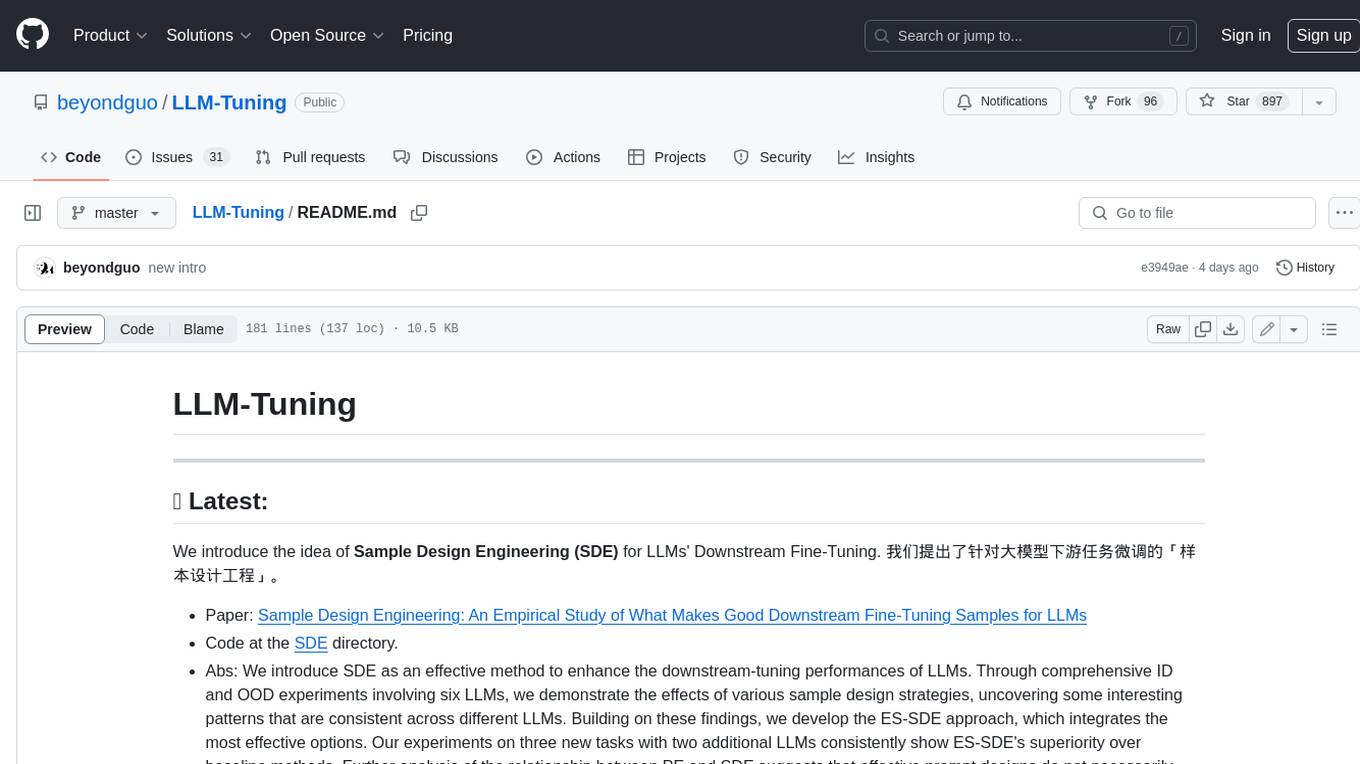
LLM-Tuning
LLM-Tuning is a collection of tools and resources for fine-tuning large language models (LLMs). It includes a library of pre-trained LoRA models, a set of tutorials and examples, and a community forum for discussion and support. LLM-Tuning makes it easy to fine-tune LLMs for a variety of tasks, including text classification, question answering, and dialogue generation. With LLM-Tuning, you can quickly and easily improve the performance of your LLMs on downstream tasks.
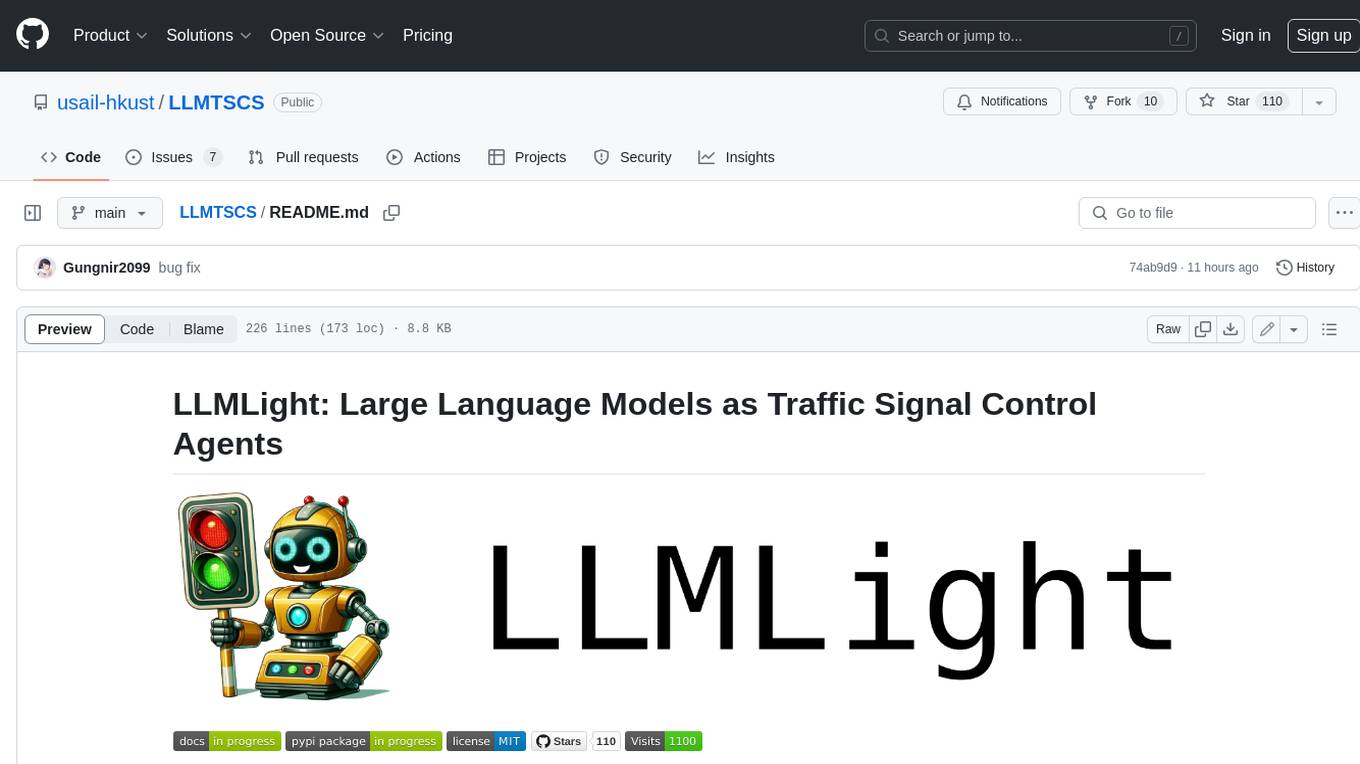
LLMTSCS
LLMLight is a novel framework that employs Large Language Models (LLMs) as decision-making agents for Traffic Signal Control (TSC). The framework leverages the advanced generalization capabilities of LLMs to engage in a reasoning and decision-making process akin to human intuition for effective traffic control. LLMLight has been demonstrated to be remarkably effective, generalizable, and interpretable against various transportation-based and RL-based baselines on nine real-world and synthetic datasets.
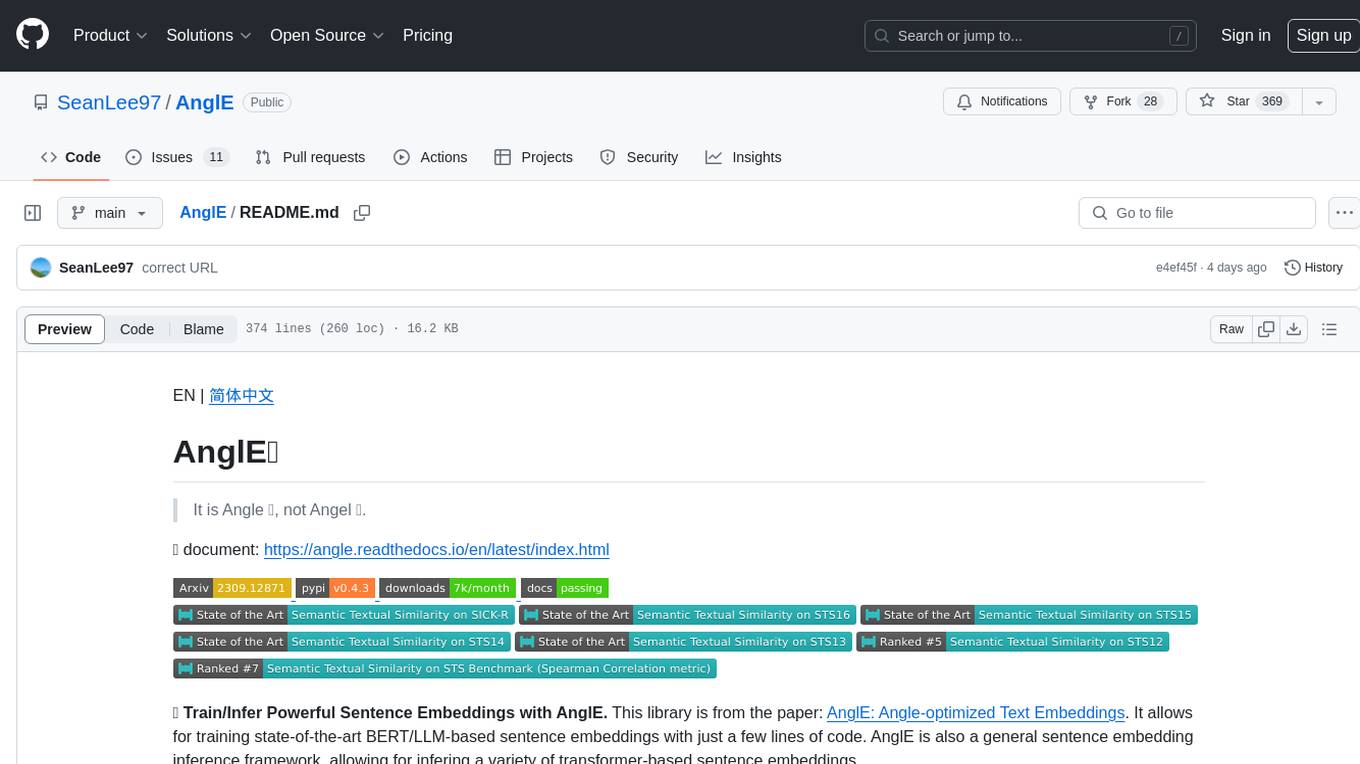
AnglE
AnglE is a library for training state-of-the-art BERT/LLM-based sentence embeddings with just a few lines of code. It also serves as a general sentence embedding inference framework, allowing for inferring a variety of transformer-based sentence embeddings. The library supports various loss functions such as AnglE loss, Contrastive loss, CoSENT loss, and Espresso loss. It provides backbones like BERT-based models, LLM-based models, and Bi-directional LLM-based models for training on single or multi-GPU setups. AnglE has achieved significant performance on various benchmarks and offers official pretrained models for both BERT-based and LLM-based models.
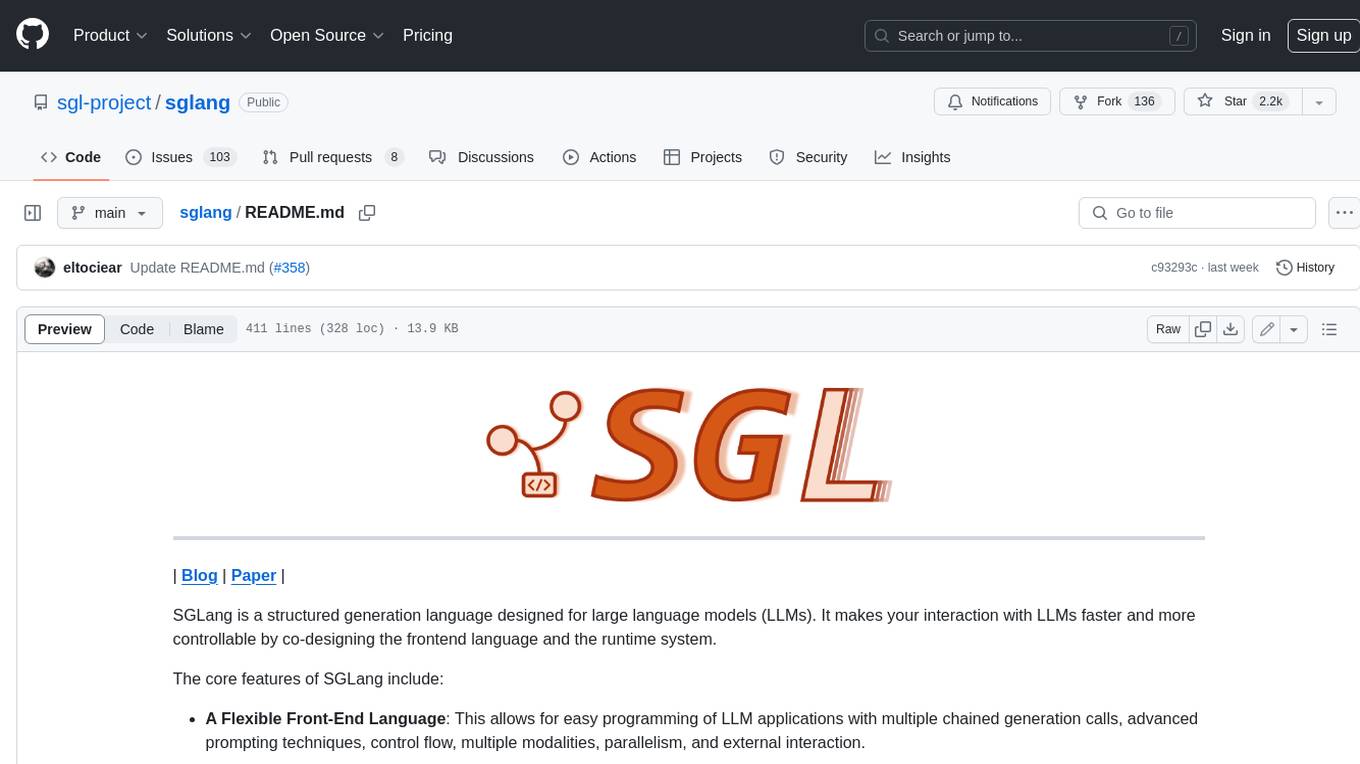
sglang
SGLang is a structured generation language designed for large language models (LLMs). It makes your interaction with LLMs faster and more controllable by co-designing the frontend language and the runtime system. The core features of SGLang include: - **A Flexible Front-End Language**: This allows for easy programming of LLM applications with multiple chained generation calls, advanced prompting techniques, control flow, multiple modalities, parallelism, and external interaction. - **A High-Performance Runtime with RadixAttention**: This feature significantly accelerates the execution of complex LLM programs by automatic KV cache reuse across multiple calls. It also supports other common techniques like continuous batching and tensor parallelism.
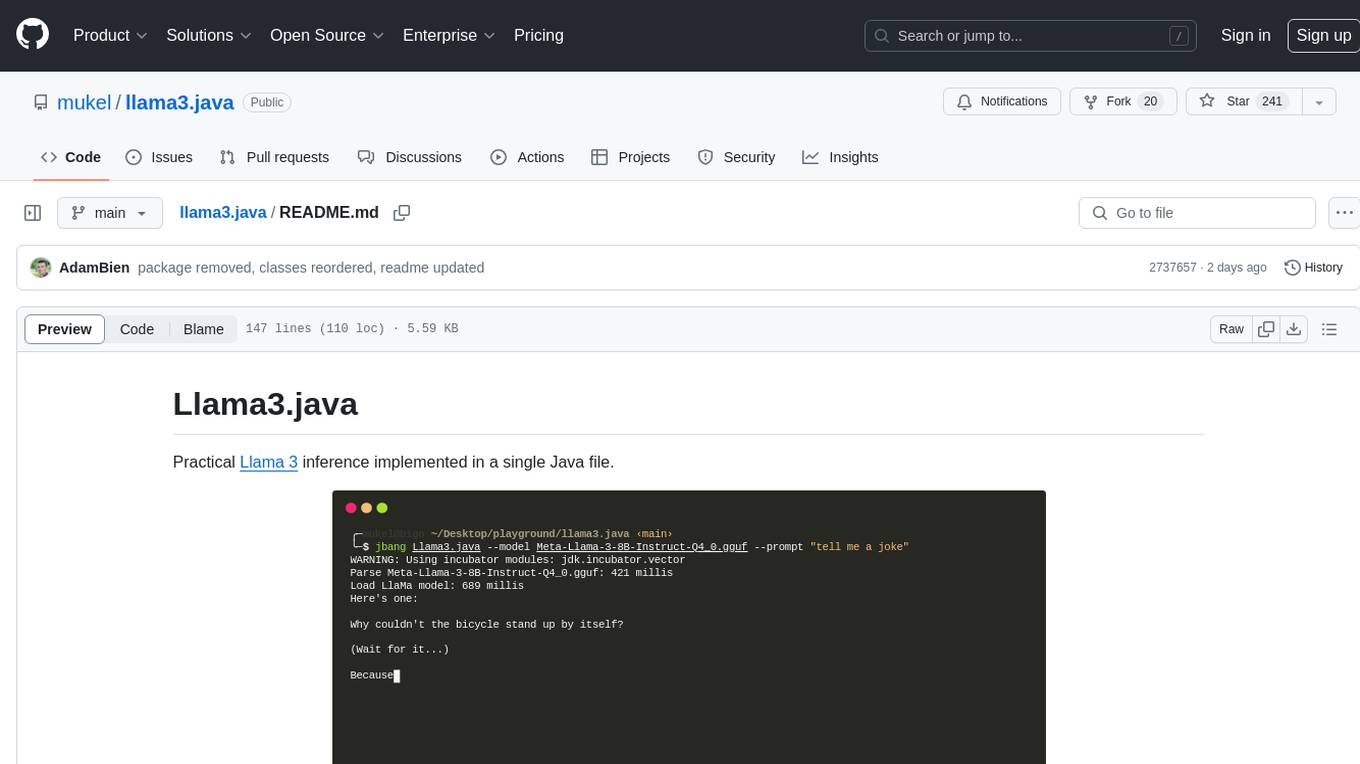
llama3.java
Llama3.java is a practical Llama 3 inference tool implemented in a single Java file. It serves as the successor of llama2.java and is designed for testing and tuning compiler optimizations and features on the JVM, especially for the Graal compiler. The tool features a GGUF format parser, Llama 3 tokenizer, Grouped-Query Attention inference, support for Q8_0 and Q4_0 quantizations, fast matrix-vector multiplication routines using Java's Vector API, and a simple CLI with 'chat' and 'instruct' modes. Users can download quantized .gguf files from huggingface.co for model usage and can also manually quantize to pure 'Q4_0'. The tool requires Java 21+ and supports running from source or building a JAR file for execution. Performance benchmarks show varying tokens/s rates for different models and implementations on different hardware setups.
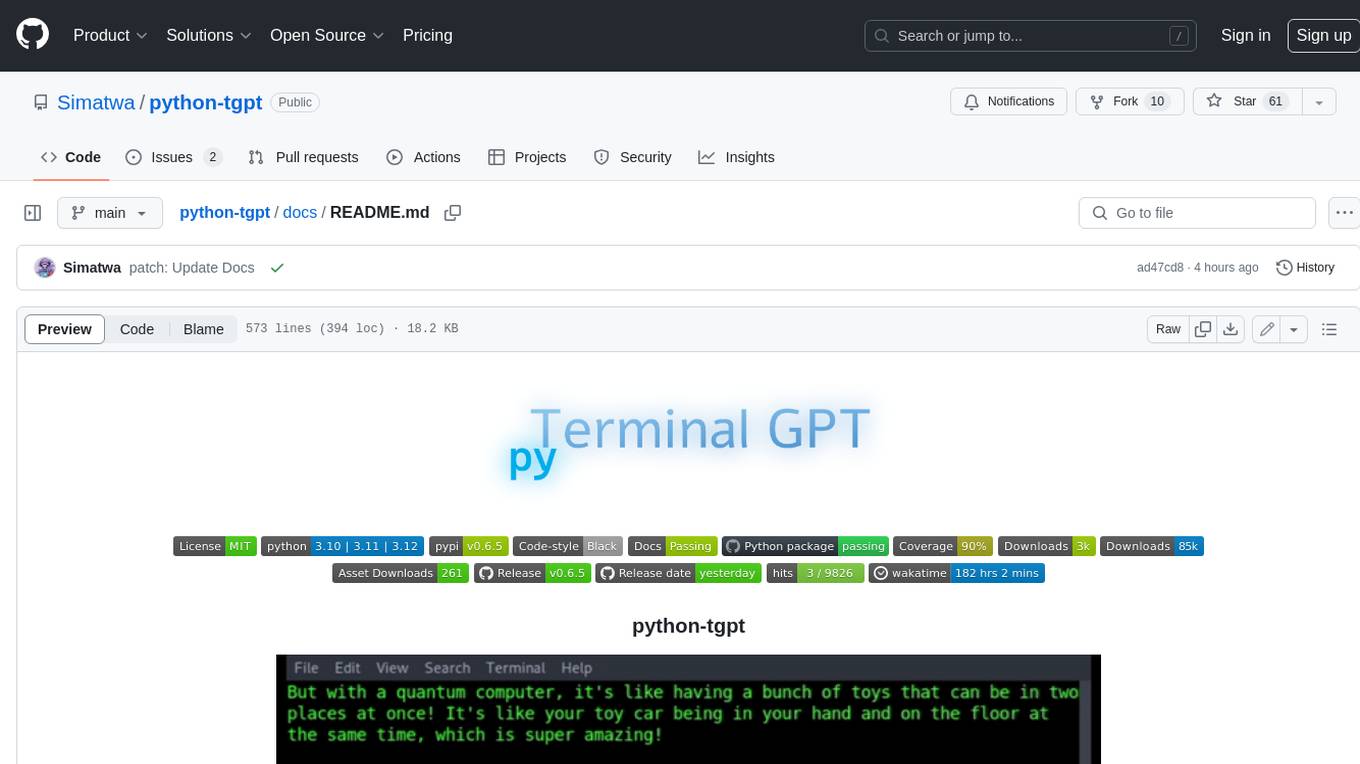
python-tgpt
Python-tgpt is a Python package that enables seamless interaction with over 45 free LLM providers without requiring an API key. It also provides image generation capabilities. The name _python-tgpt_ draws inspiration from its parent project tgpt, which operates on Golang. Through this Python adaptation, users can effortlessly engage with a number of free LLMs available, fostering a smoother AI interaction experience.
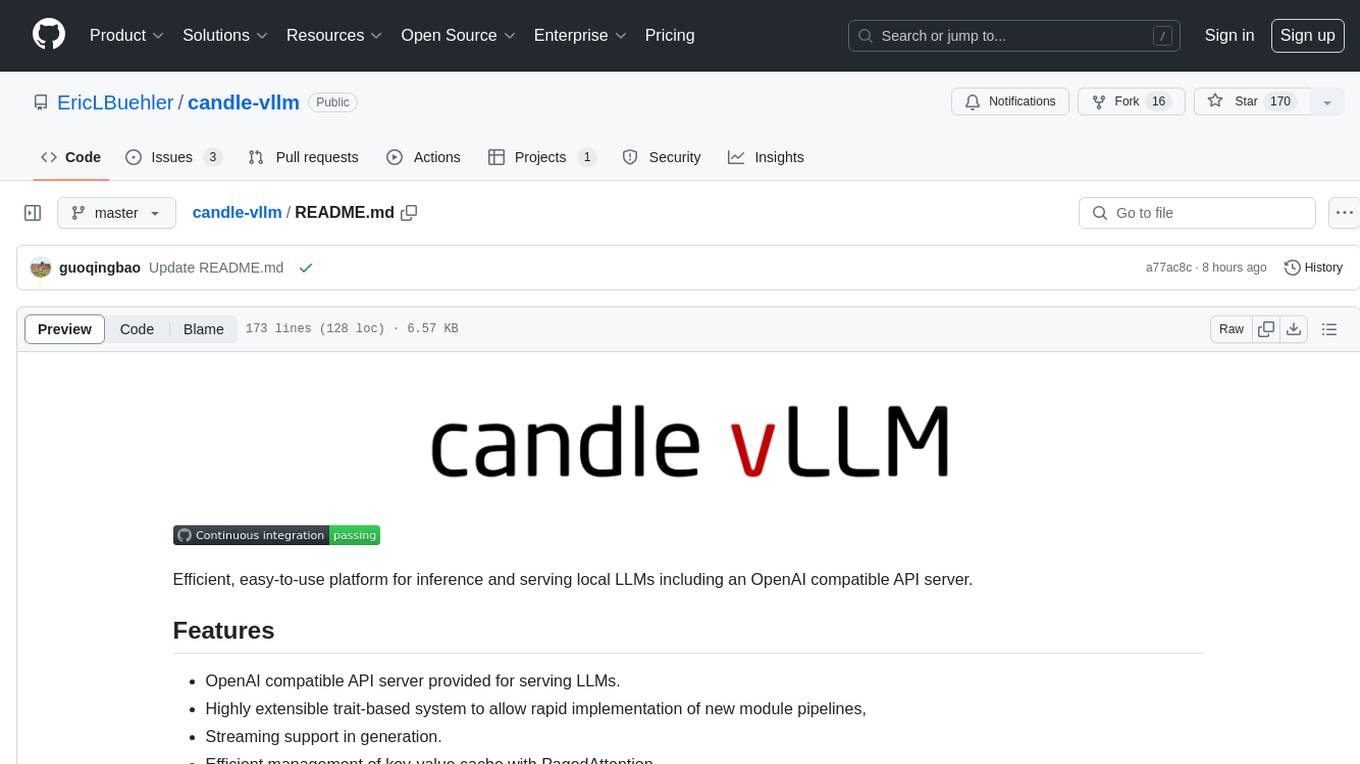
candle-vllm
Candle-vllm is an efficient and easy-to-use platform designed for inference and serving local LLMs, featuring an OpenAI compatible API server. It offers a highly extensible trait-based system for rapid implementation of new module pipelines, streaming support in generation, efficient management of key-value cache with PagedAttention, and continuous batching. The tool supports chat serving for various models and provides a seamless experience for users to interact with LLMs through different interfaces.
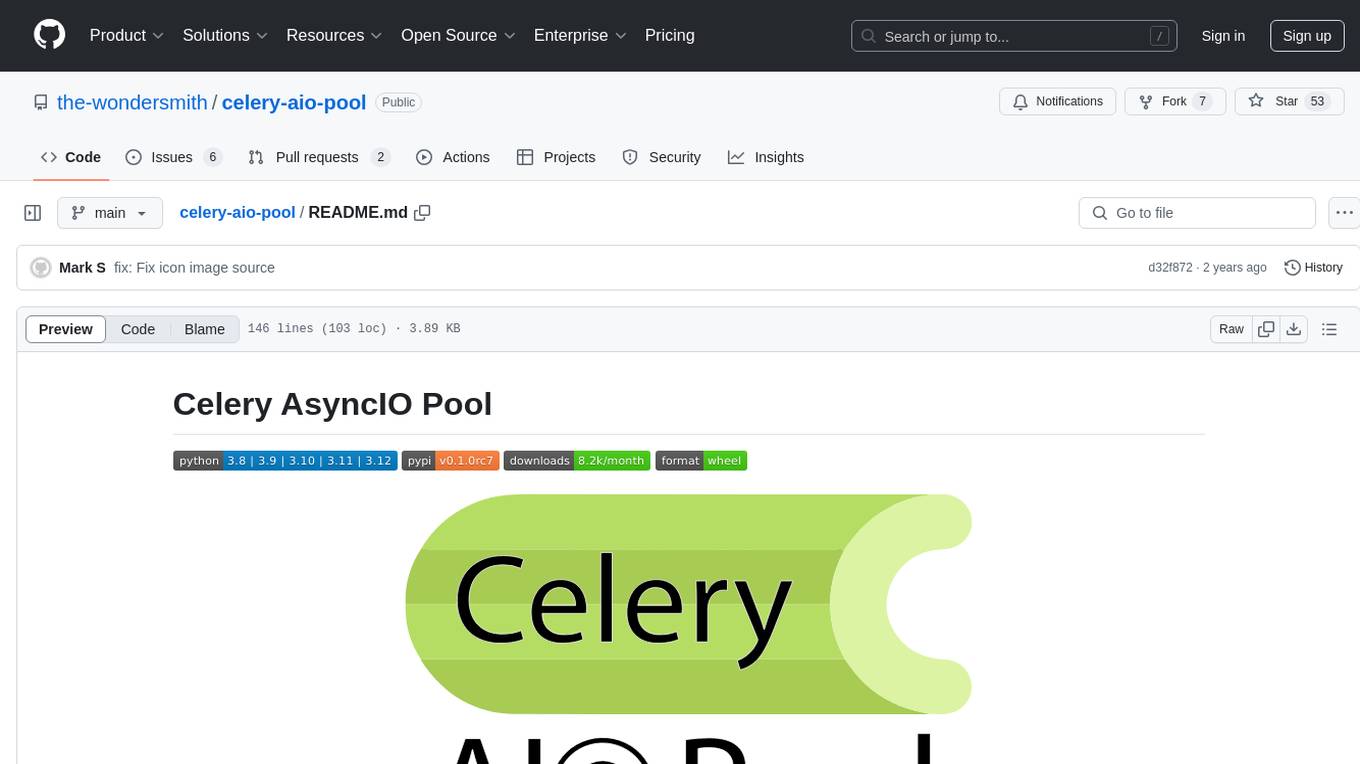
celery-aio-pool
Celery AsyncIO Pool is a free software tool licensed under GNU Affero General Public License v3+. It provides an AsyncIO worker pool for Celery, enabling users to leverage the power of AsyncIO in their Celery applications. The tool allows for easy installation using Poetry, pip, or directly from GitHub. Users can configure Celery to use the AsyncIO pool provided by celery-aio-pool, or they can wait for the upcoming support for out-of-tree worker pools in Celery 5.3. The tool is actively maintained and welcomes contributions from the community.
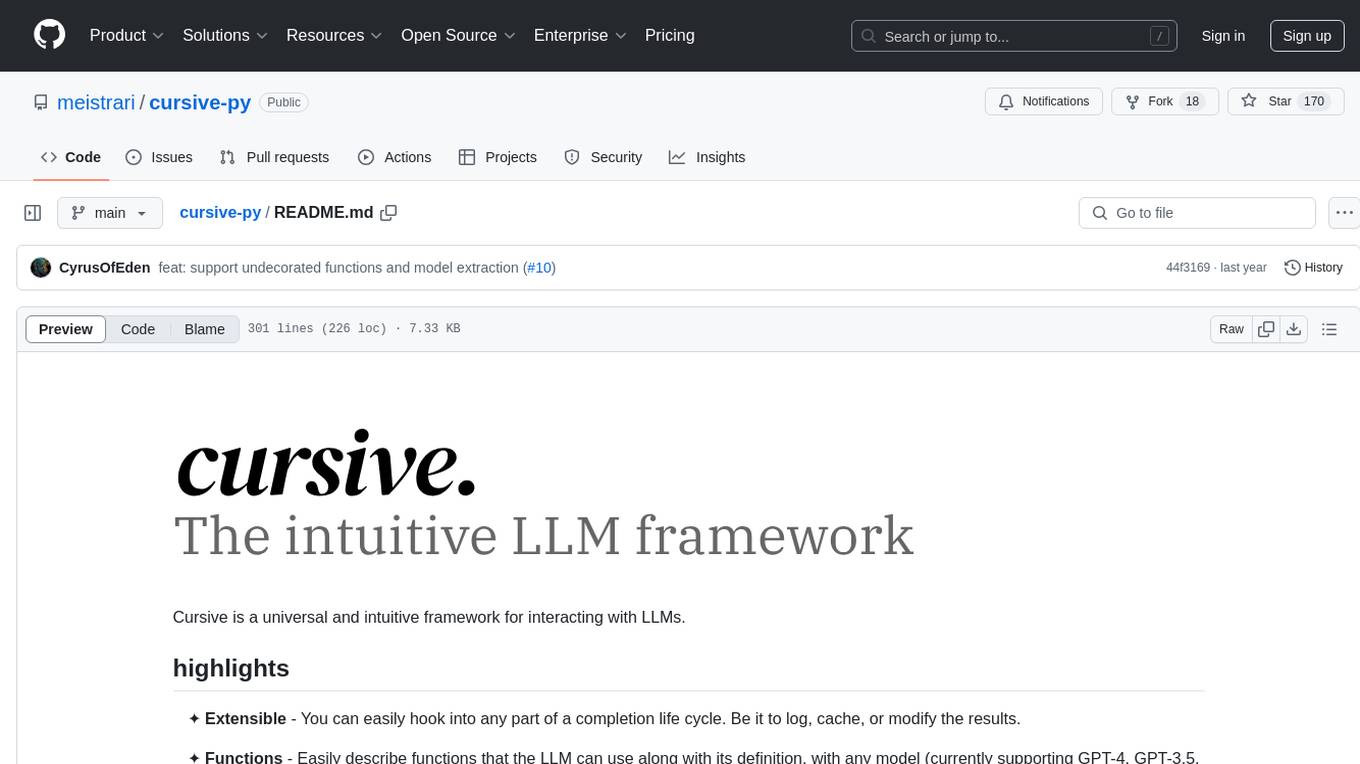
cursive-py
Cursive is a universal and intuitive framework for interacting with LLMs. It is extensible, allowing users to hook into any part of a completion life cycle. Users can easily describe functions that LLMs can use with any supported model. Cursive aims to bridge capabilities between different models, providing a single interface for users to choose any model. It comes with built-in token usage and costs calculations, automatic retry, and model expanding features. Users can define and describe functions, generate Pydantic BaseModels, hook into completion life cycle, create embeddings, and configure retry and model expanding behavior. Cursive supports various models from OpenAI, Anthropic, OpenRouter, Cohere, and Replicate, with options to pass API keys for authentication.
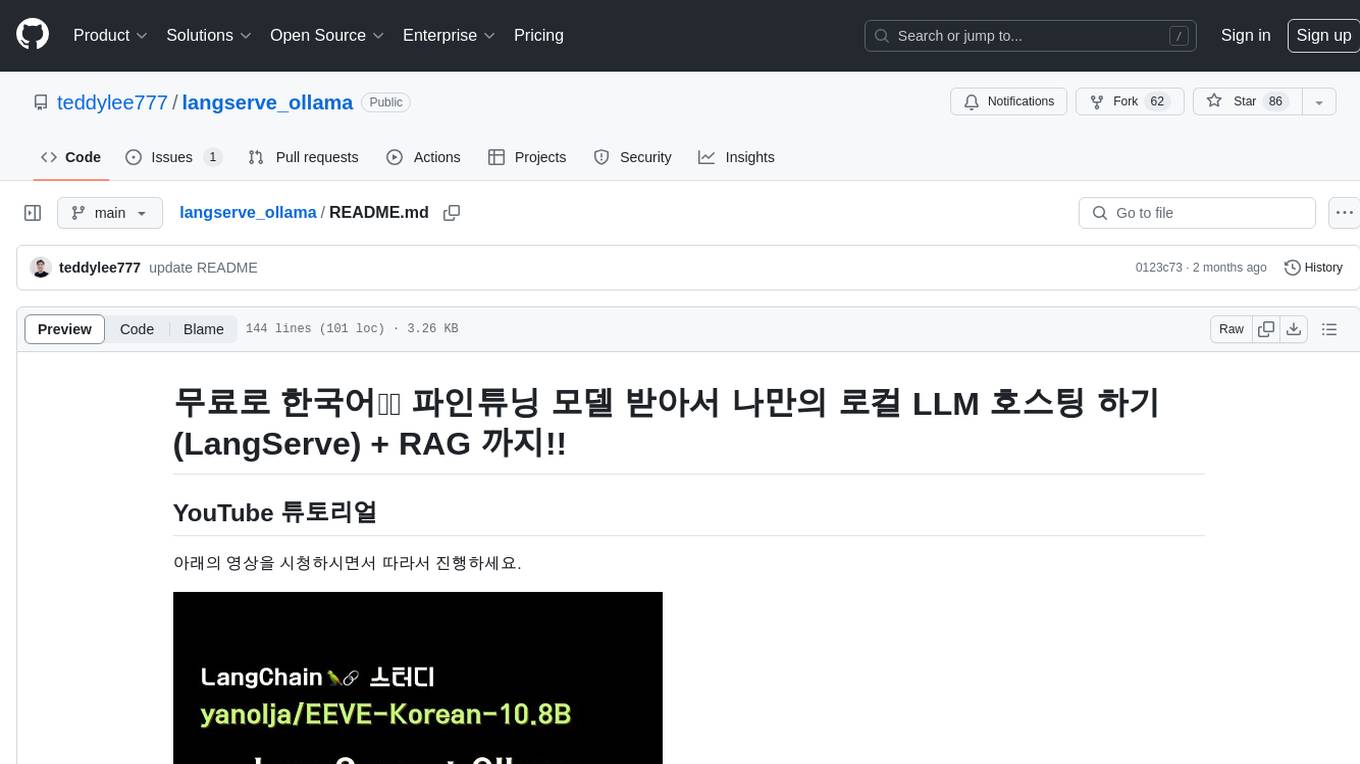
langserve_ollama
LangServe Ollama is a tool that allows users to fine-tune Korean language models for local hosting, including RAG. Users can load HuggingFace gguf files, create model chains, and monitor GPU usage. The tool provides a seamless workflow for customizing and deploying language models in a local environment.
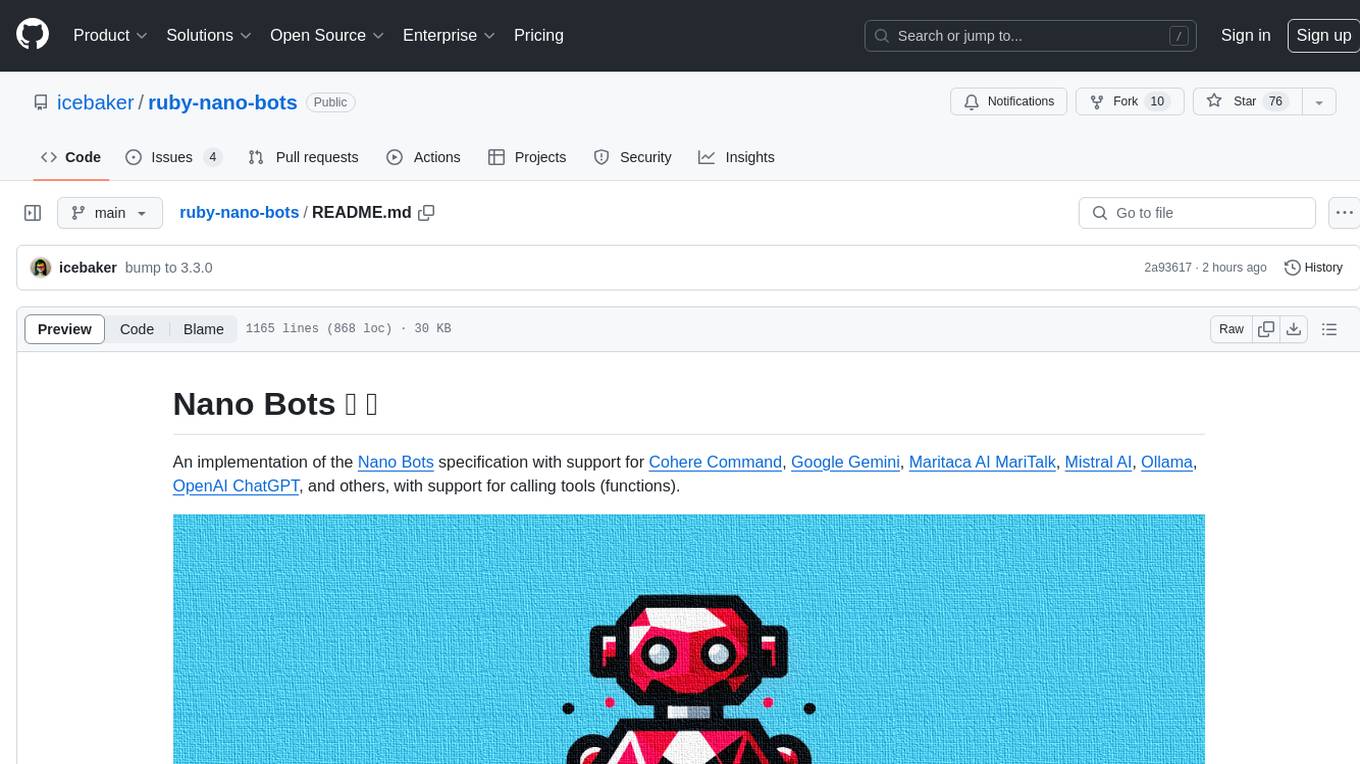
ruby-nano-bots
Ruby Nano Bots is an implementation of the Nano Bots specification supporting various AI providers like Cohere Command, Google Gemini, Maritaca AI MariTalk, Mistral AI, Ollama, OpenAI ChatGPT, and others. It allows calling tools (functions) and provides a helpful assistant for interacting with AI language models. The tool can be used both from the command line and as a library in Ruby projects, offering features like REPL, debugging, and encryption for data privacy.
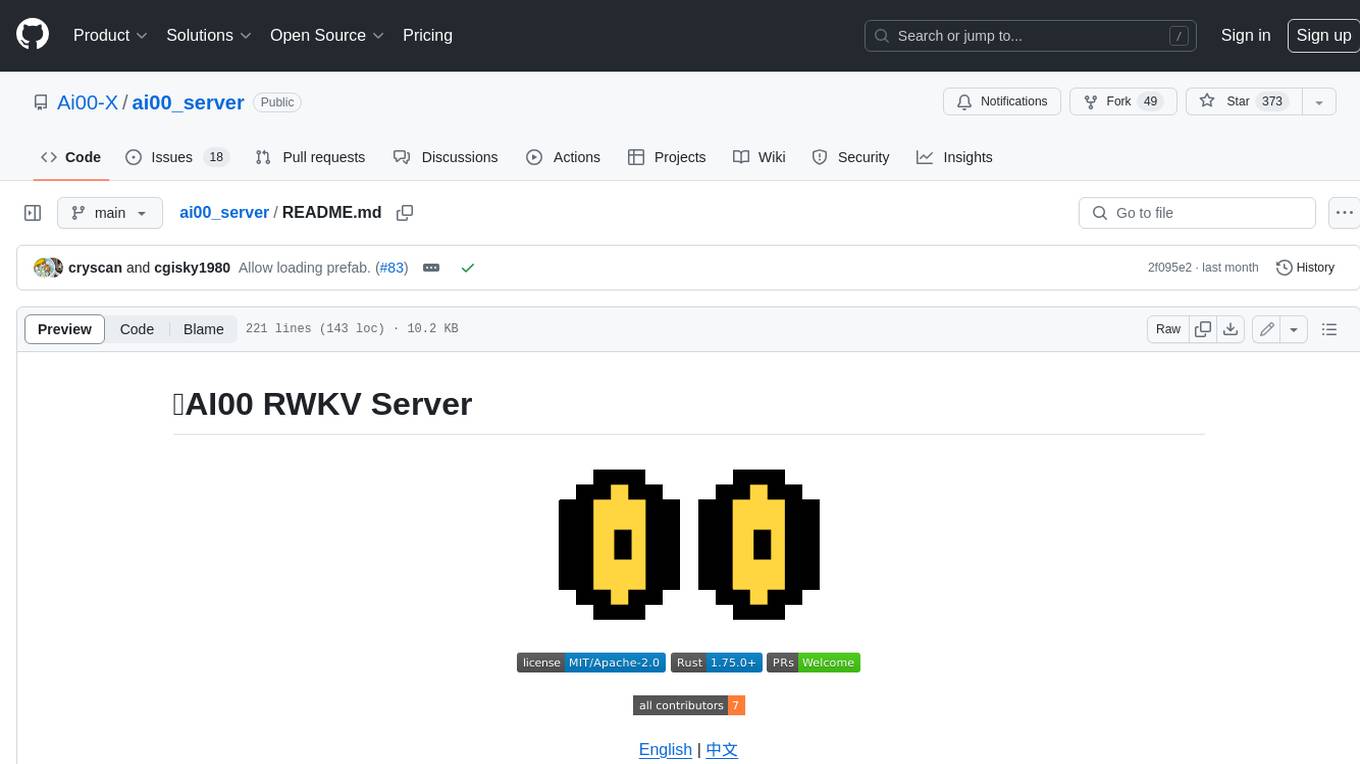
ai00_server
AI00 RWKV Server is an inference API server for the RWKV language model based upon the web-rwkv inference engine. It supports VULKAN parallel and concurrent batched inference and can run on all GPUs that support VULKAN. No need for Nvidia cards!!! AMD cards and even integrated graphics can be accelerated!!! No need for bulky pytorch, CUDA and other runtime environments, it's compact and ready to use out of the box! Compatible with OpenAI's ChatGPT API interface. 100% open source and commercially usable, under the MIT license. If you are looking for a fast, efficient, and easy-to-use LLM API server, then AI00 RWKV Server is your best choice. It can be used for various tasks, including chatbots, text generation, translation, and Q&A.
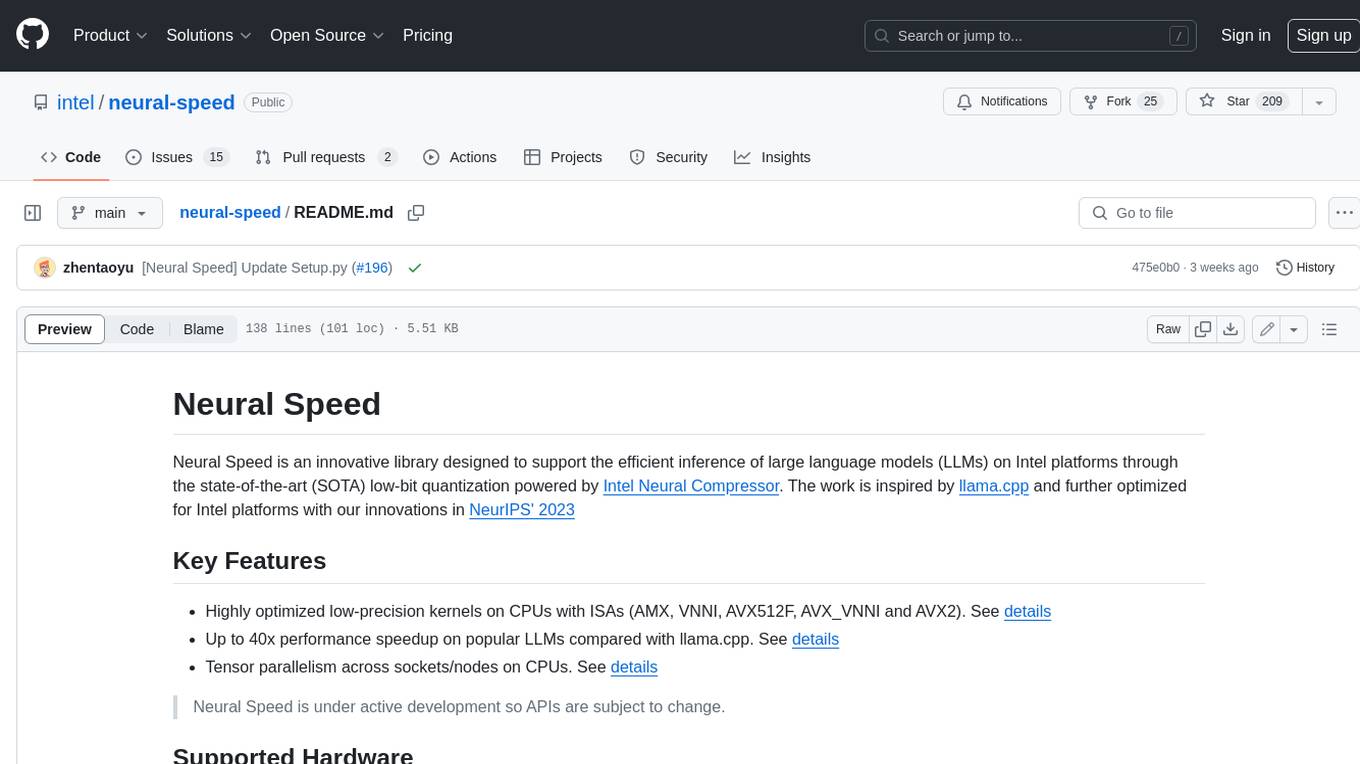
neural-speed
Neural Speed is an innovative library designed to support the efficient inference of large language models (LLMs) on Intel platforms through the state-of-the-art (SOTA) low-bit quantization powered by Intel Neural Compressor. The work is inspired by llama.cpp and further optimized for Intel platforms with our innovations in NeurIPS' 2023
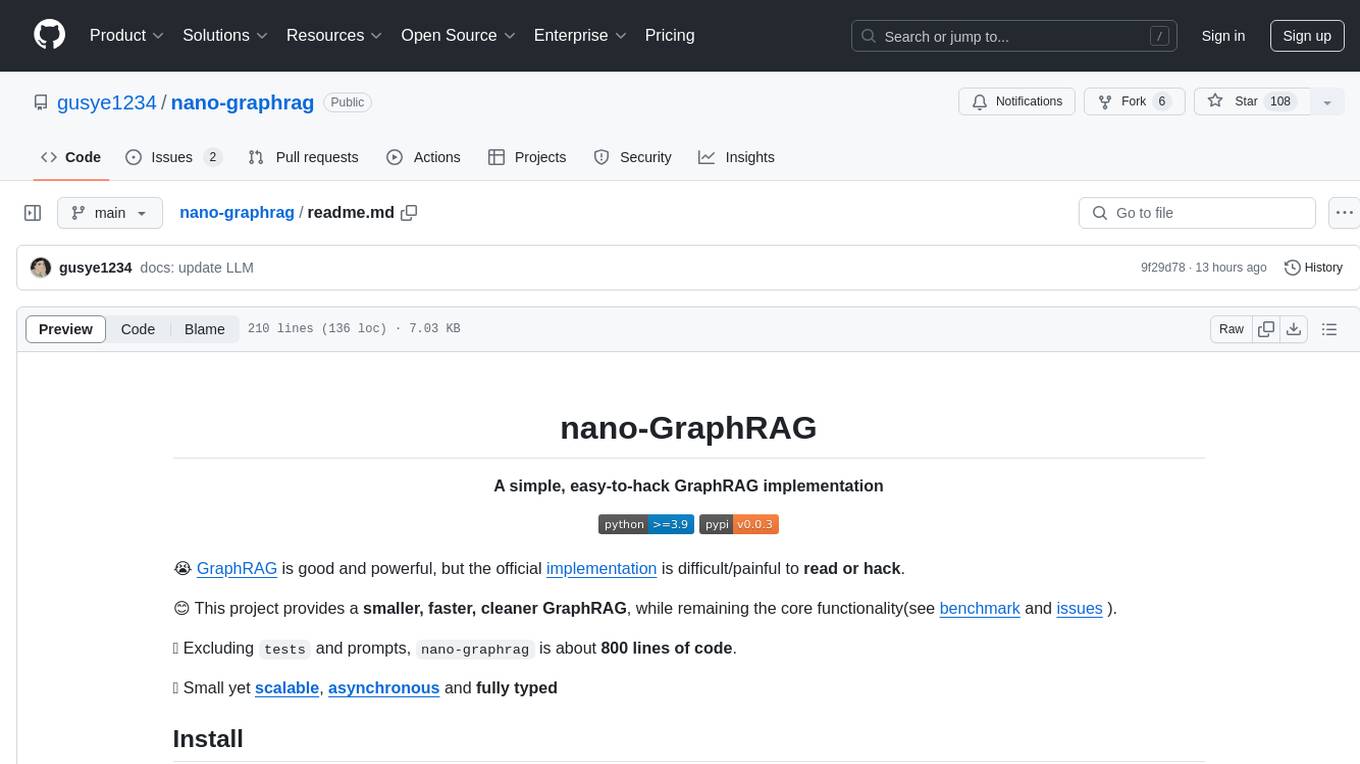
nano-graphrag
nano-GraphRAG is a simple, easy-to-hack implementation of GraphRAG that provides a smaller, faster, and cleaner version of the official implementation. It is about 800 lines of code, small yet scalable, asynchronous, and fully typed. The tool supports incremental insert, async methods, and various parameters for customization. Users can replace storage components and LLM functions as needed. It also allows for embedding function replacement and comes with pre-defined prompts for entity extraction and community reports. However, some features like covariates and global search implementation differ from the original GraphRAG. Future versions aim to address issues related to data source ID, community description truncation, and add new components.
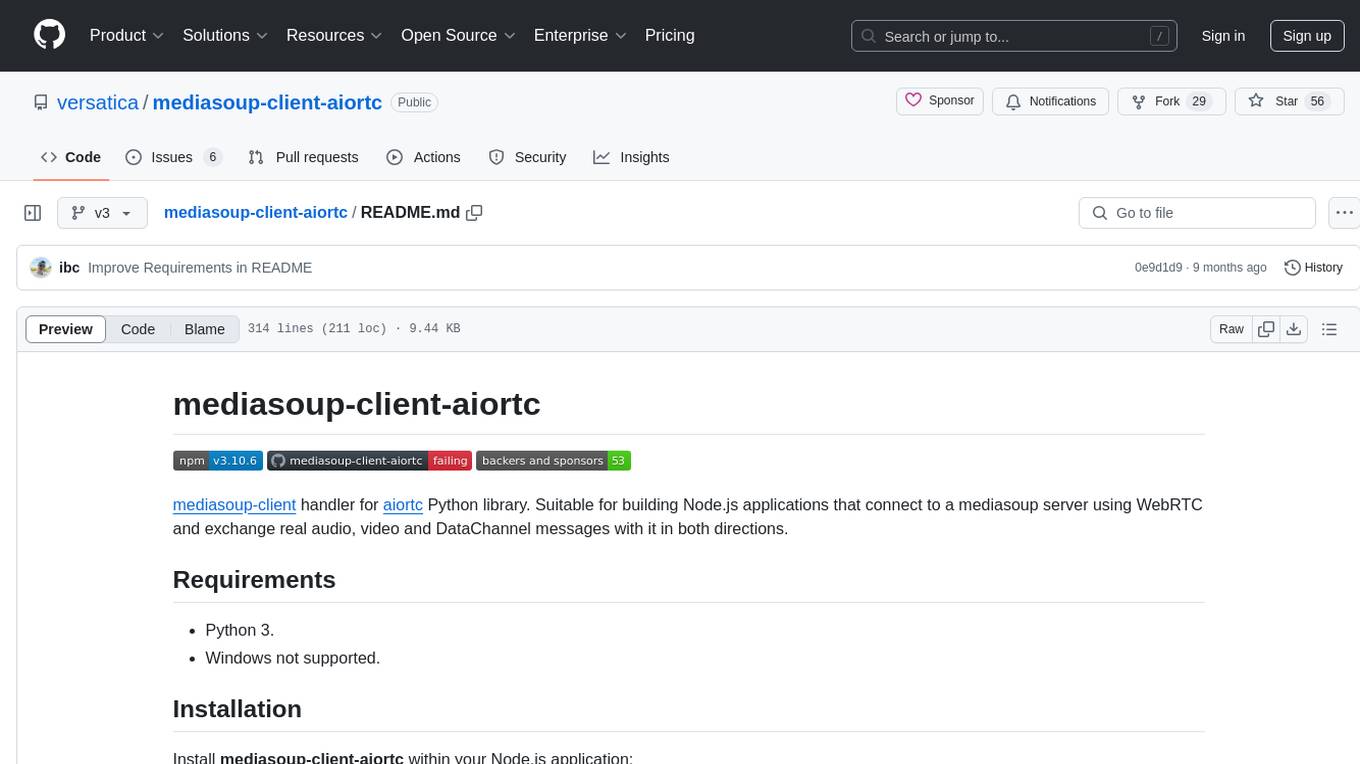
mediasoup-client-aiortc
mediasoup-client-aiortc is a handler for the aiortc Python library, allowing Node.js applications to connect to a mediasoup server using WebRTC for real-time audio, video, and DataChannel communication. It facilitates the creation of Worker instances to manage Python subprocesses, obtain audio/video tracks, and create mediasoup-client handlers. The tool supports features like getUserMedia, handlerFactory creation, and event handling for subprocess closure and unexpected termination. It provides custom classes for media stream and track constraints, enabling diverse audio/video sources like devices, files, or URLs. The tool enhances WebRTC capabilities in Node.js applications through seamless Python subprocess communication.
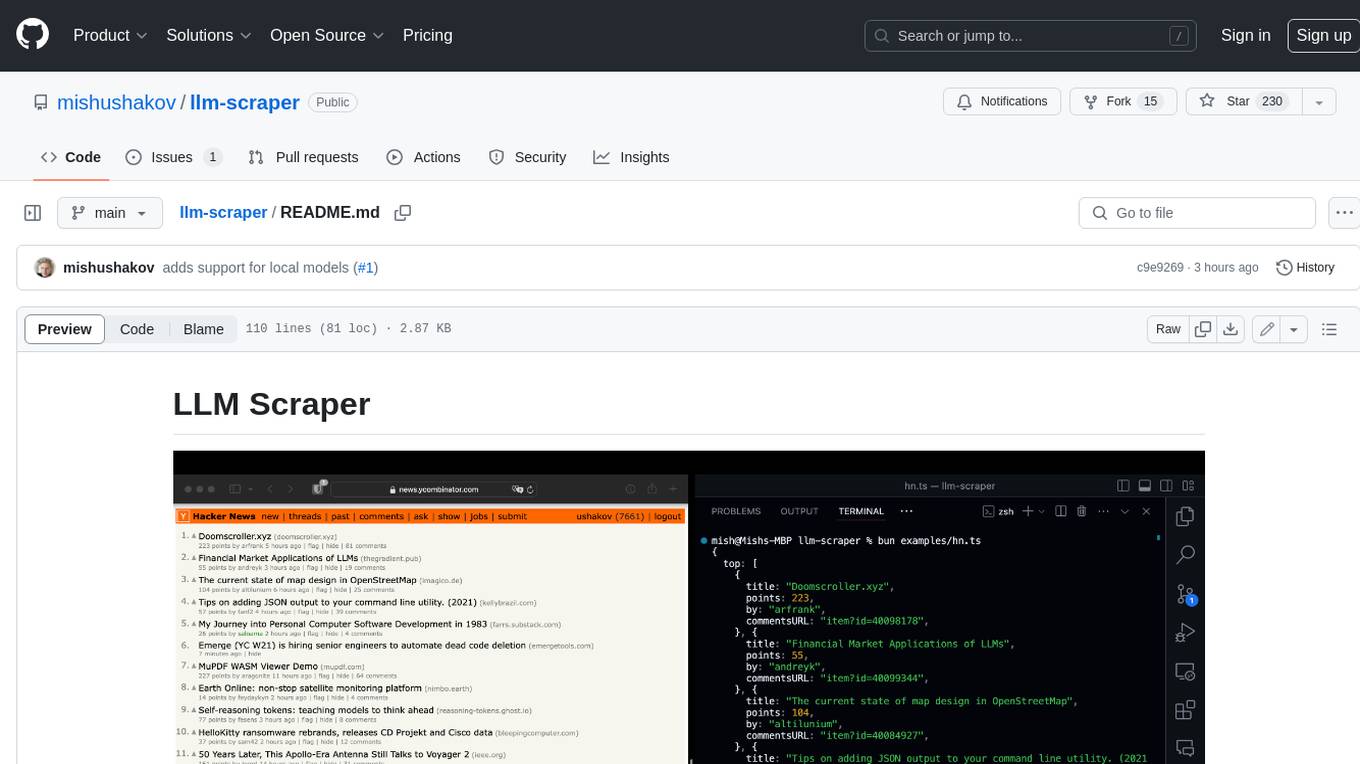
llm-scraper
LLM Scraper is a TypeScript library that allows you to convert any webpages into structured data using LLMs. It supports Local (GGUF), OpenAI, Groq chat models, and schemas defined with Zod. With full type-safety in TypeScript and based on the Playwright framework, it offers streaming when crawling multiple pages and supports four input modes: html, markdown, text, and image.
For similar tasks
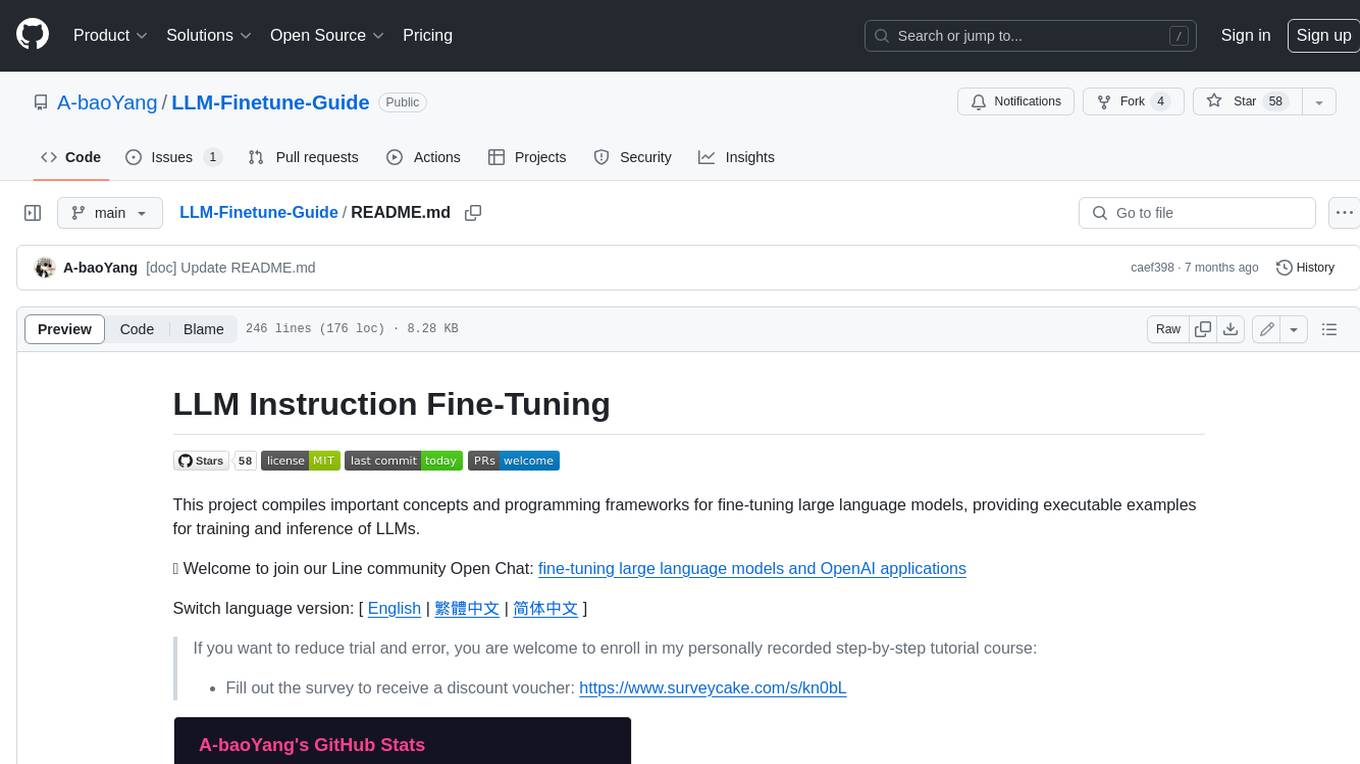
LLM-Finetune-Guide
This project provides a comprehensive guide to fine-tuning large language models (LLMs) with efficient methods like LoRA and P-tuning V2. It includes detailed instructions, code examples, and performance benchmarks for various LLMs and fine-tuning techniques. The guide also covers data preparation, evaluation, prediction, and running inference on CPU environments. By leveraging this guide, users can effectively fine-tune LLMs for specific tasks and applications.
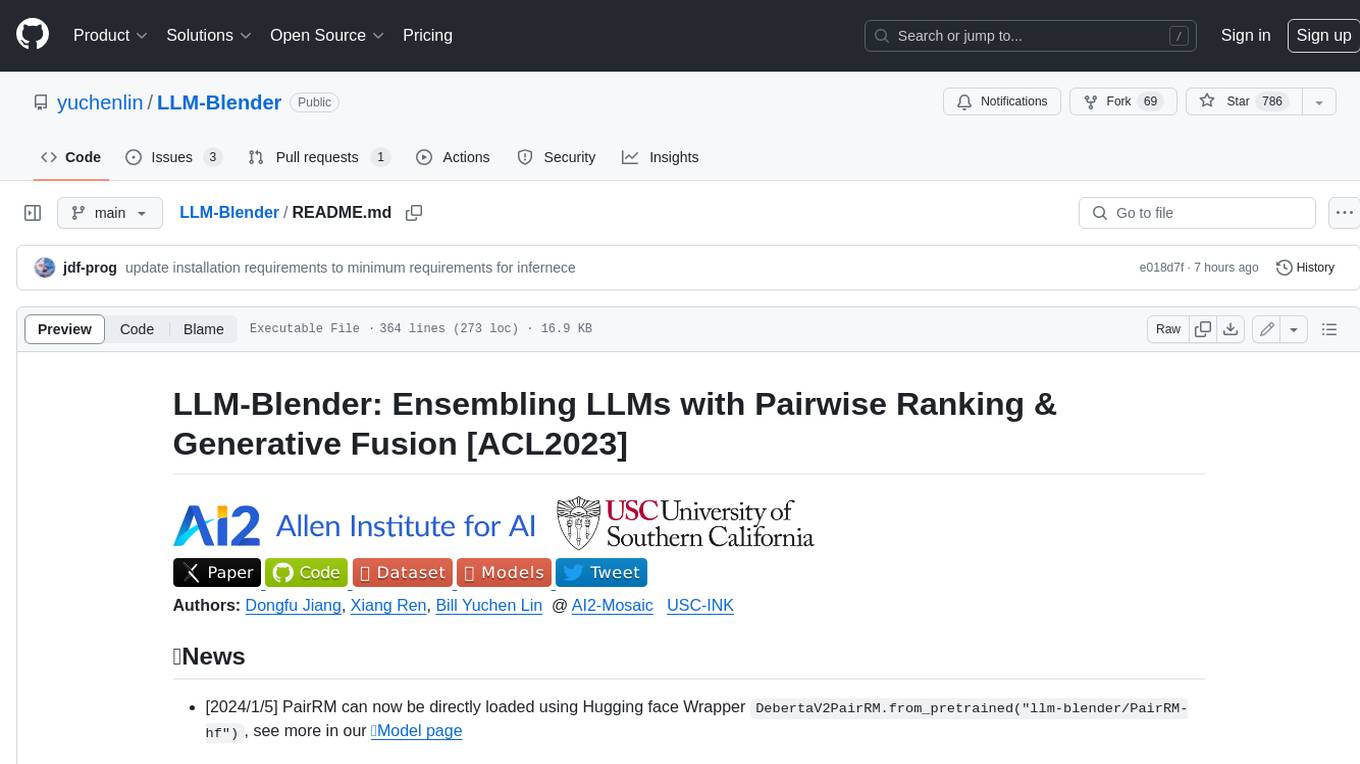
LLM-Blender
LLM-Blender is a framework for ensembling large language models (LLMs) to achieve superior performance. It consists of two modules: PairRanker and GenFuser. PairRanker uses pairwise comparisons to distinguish between candidate outputs, while GenFuser merges the top-ranked candidates to create an improved output. LLM-Blender has been shown to significantly surpass the best LLMs and baseline ensembling methods across various metrics on the MixInstruct benchmark dataset.
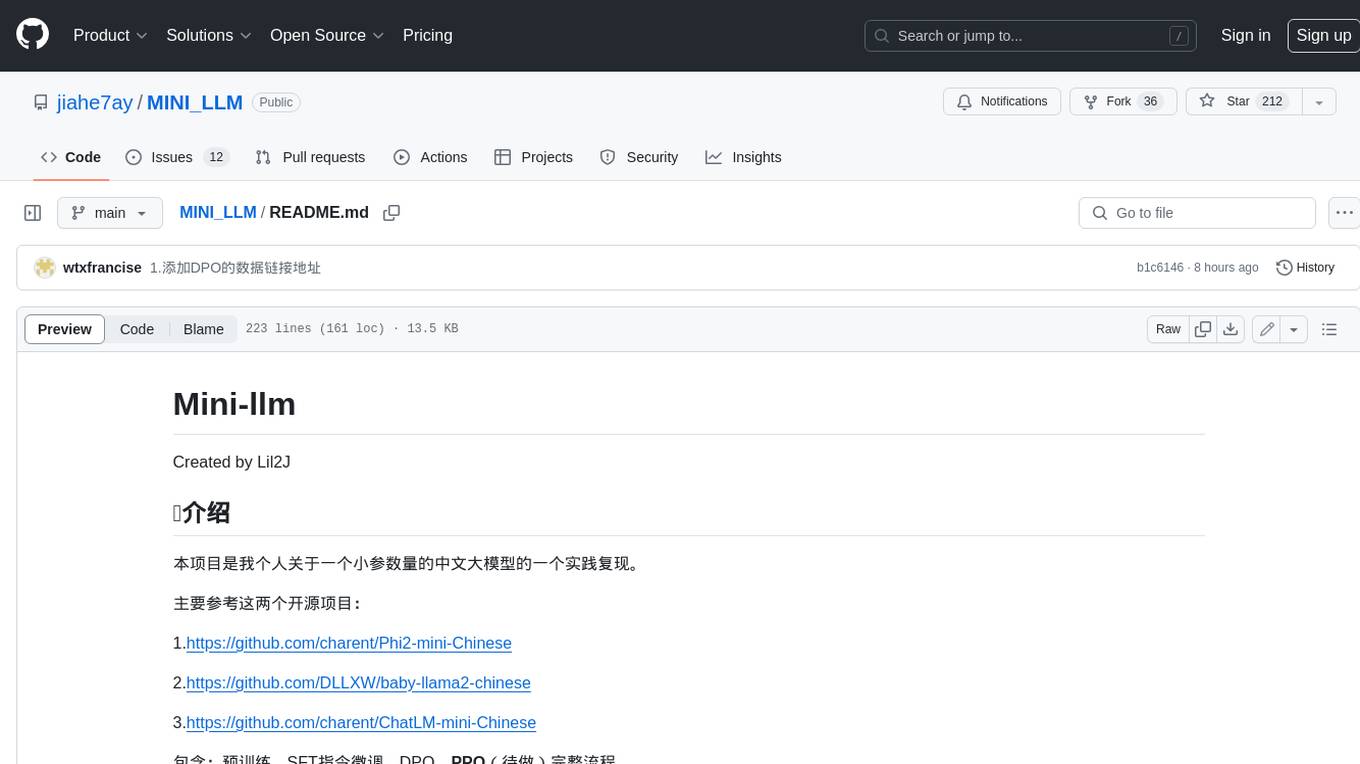
MINI_LLM
This project is a personal implementation and reproduction of a small-parameter Chinese LLM. It mainly refers to these two open source projects: https://github.com/charent/Phi2-mini-Chinese and https://github.com/DLLXW/baby-llama2-chinese. It includes the complete process of pre-training, SFT instruction fine-tuning, DPO, and PPO (to be done). I hope to share it with everyone and hope that everyone can work together to improve it!
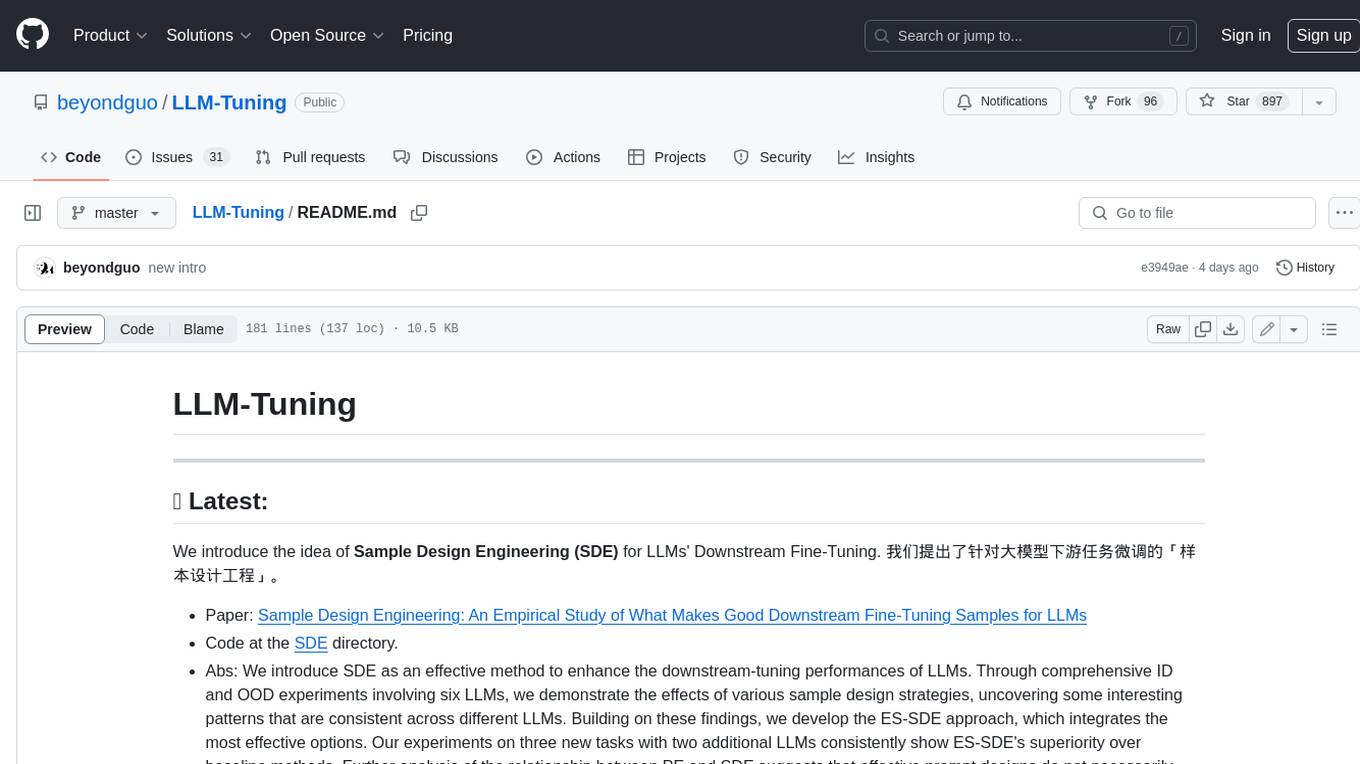
LLM-Tuning
LLM-Tuning is a collection of tools and resources for fine-tuning large language models (LLMs). It includes a library of pre-trained LoRA models, a set of tutorials and examples, and a community forum for discussion and support. LLM-Tuning makes it easy to fine-tune LLMs for a variety of tasks, including text classification, question answering, and dialogue generation. With LLM-Tuning, you can quickly and easily improve the performance of your LLMs on downstream tasks.
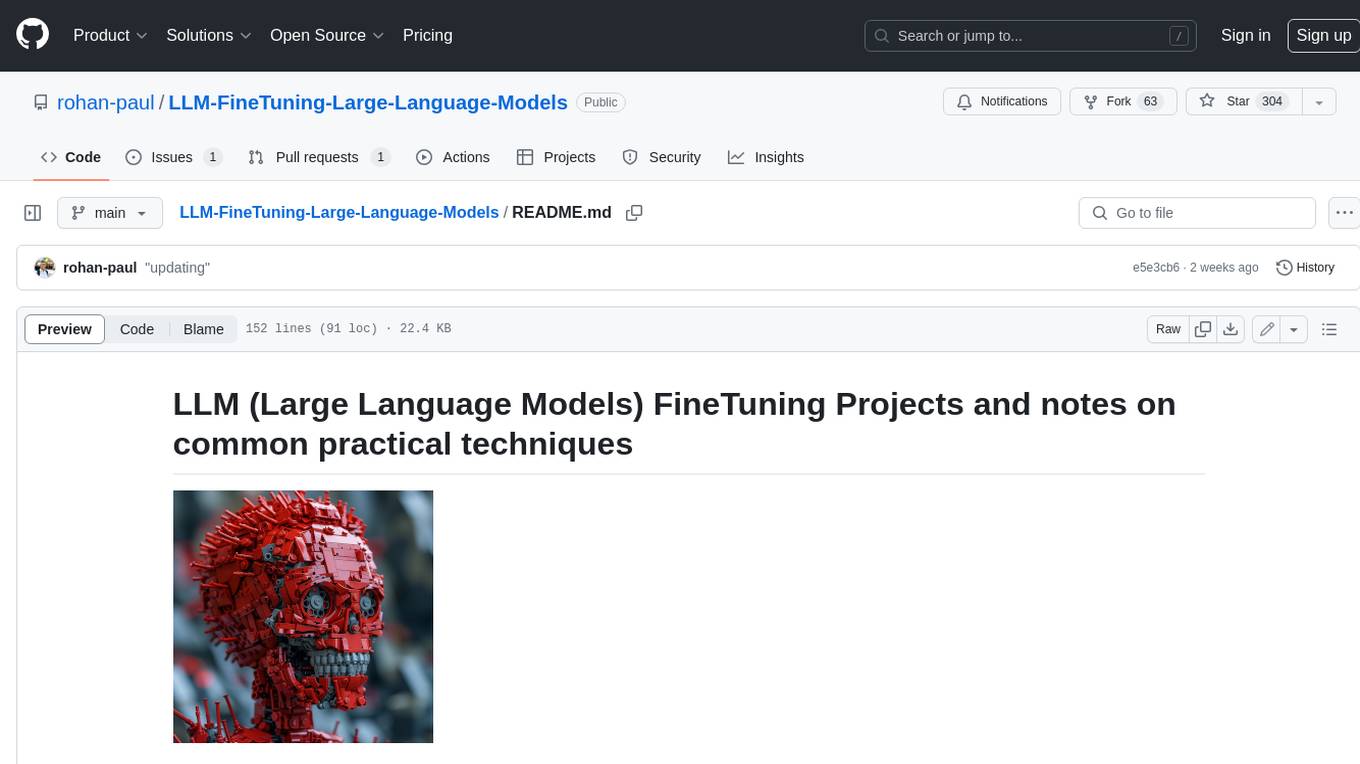
LLM-FineTuning-Large-Language-Models
This repository contains projects and notes on common practical techniques for fine-tuning Large Language Models (LLMs). It includes fine-tuning LLM notebooks, Colab links, LLM techniques and utils, and other smaller language models. The repository also provides links to YouTube videos explaining the concepts and techniques discussed in the notebooks.
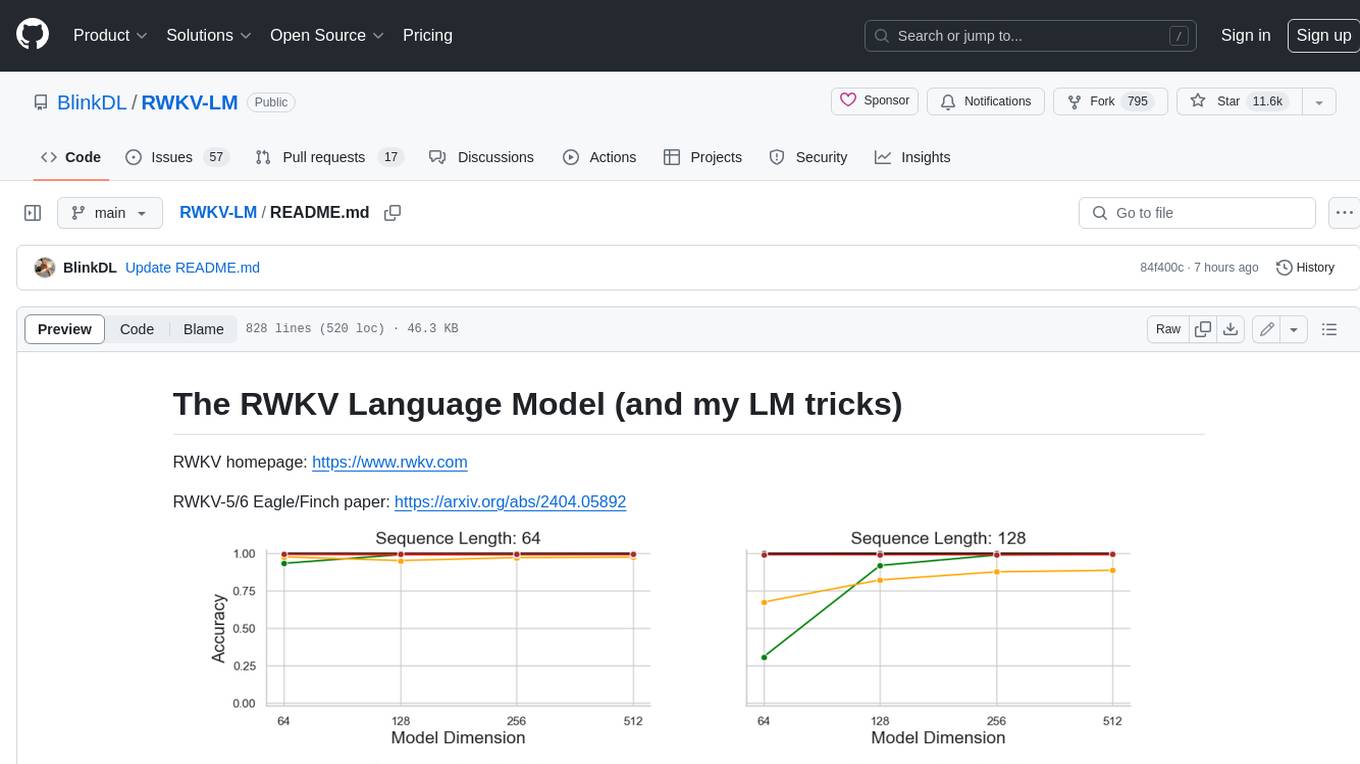
RWKV-LM
RWKV is an RNN with Transformer-level LLM performance, which can also be directly trained like a GPT transformer (parallelizable). And it's 100% attention-free. You only need the hidden state at position t to compute the state at position t+1. You can use the "GPT" mode to quickly compute the hidden state for the "RNN" mode. So it's combining the best of RNN and transformer - **great performance, fast inference, saves VRAM, fast training, "infinite" ctx_len, and free sentence embedding** (using the final hidden state).
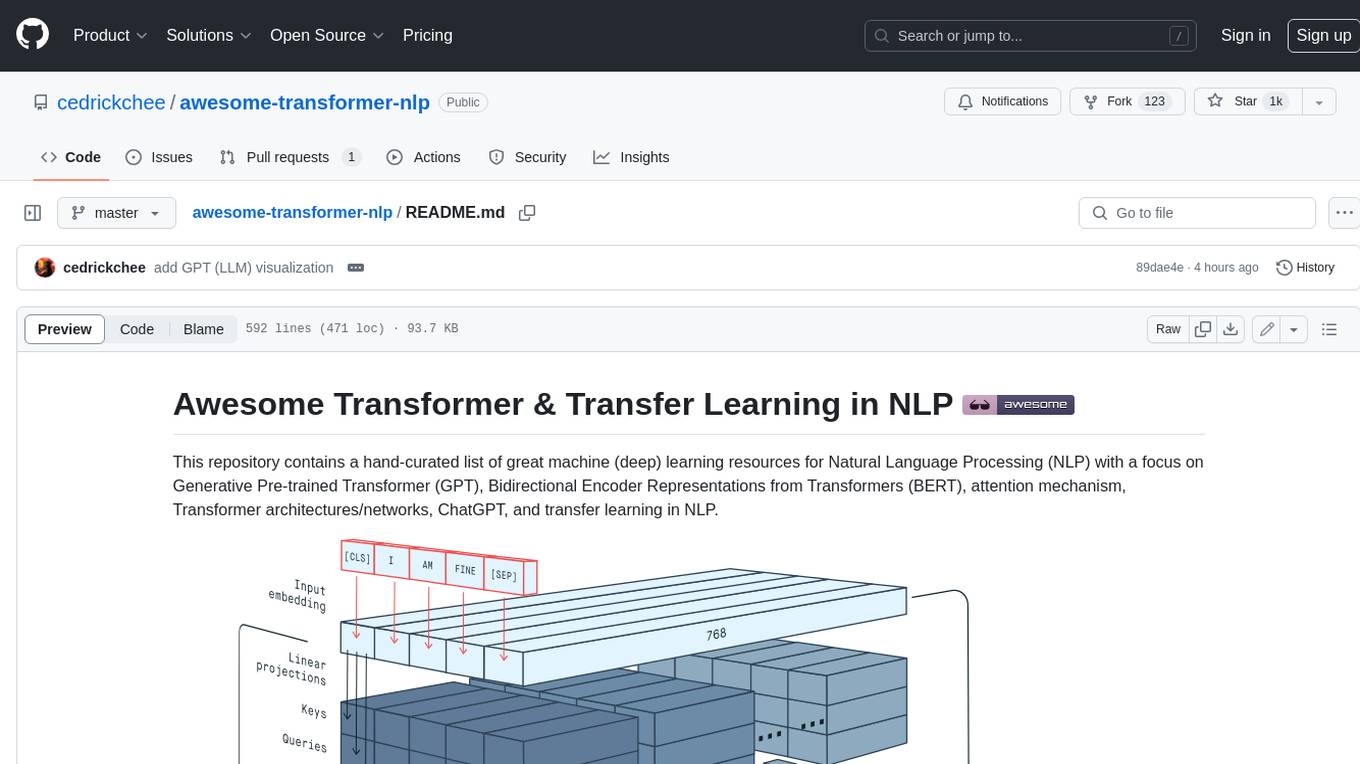
awesome-transformer-nlp
This repository contains a hand-curated list of great machine (deep) learning resources for Natural Language Processing (NLP) with a focus on Generative Pre-trained Transformer (GPT), Bidirectional Encoder Representations from Transformers (BERT), attention mechanism, Transformer architectures/networks, Chatbot, and transfer learning in NLP.
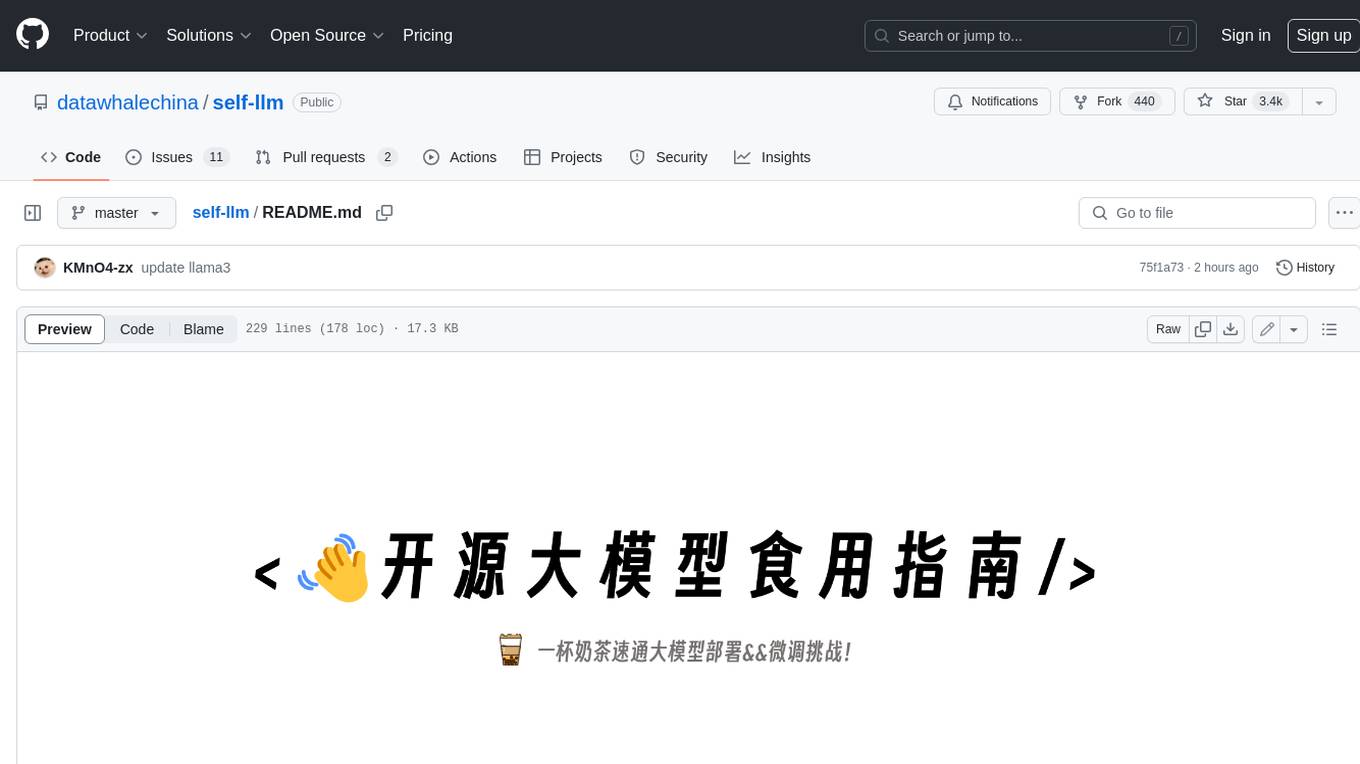
self-llm
This project is a Chinese tutorial for domestic beginners based on the AutoDL platform, providing full-process guidance for various open-source large models, including environment configuration, local deployment, and efficient fine-tuning. It simplifies the deployment, use, and application process of open-source large models, enabling more ordinary students and researchers to better use open-source large models and helping open and free large models integrate into the lives of ordinary learners faster.
For similar jobs
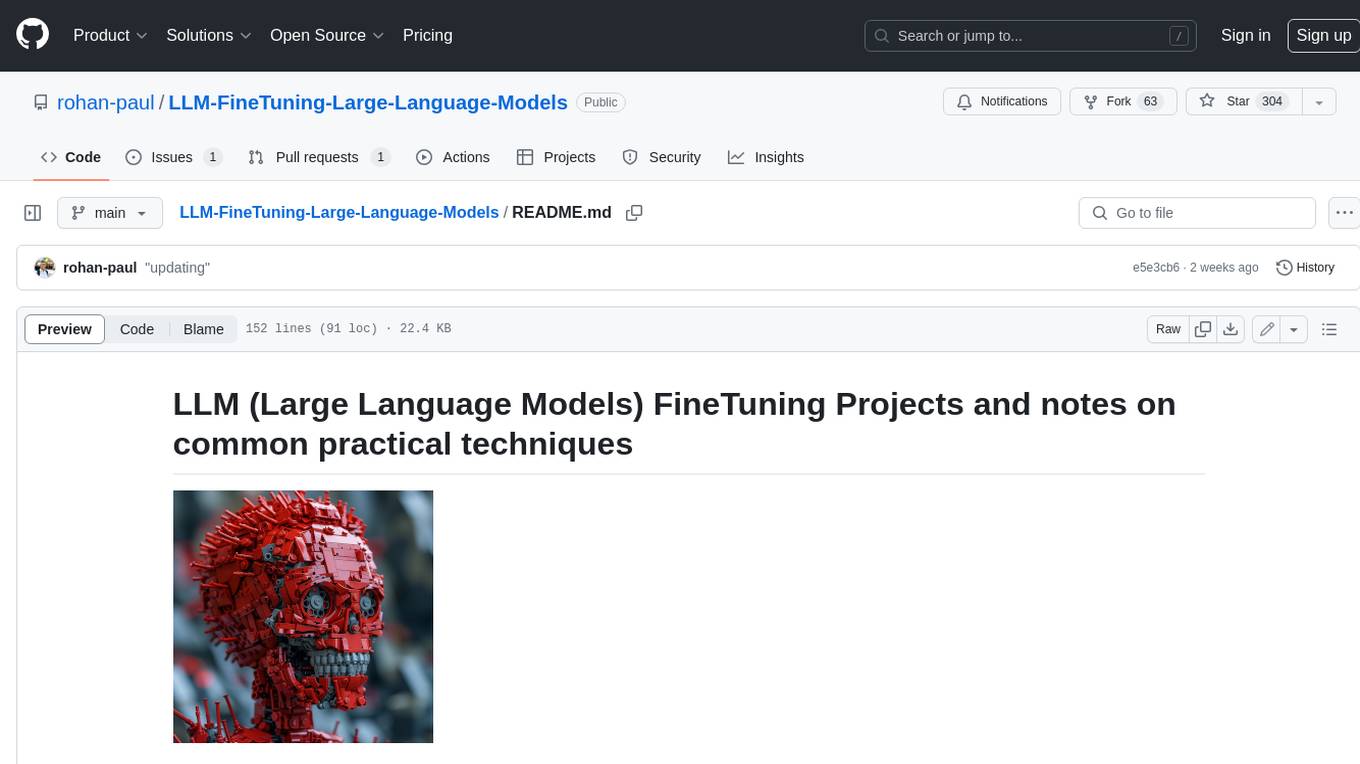
LLM-FineTuning-Large-Language-Models
This repository contains projects and notes on common practical techniques for fine-tuning Large Language Models (LLMs). It includes fine-tuning LLM notebooks, Colab links, LLM techniques and utils, and other smaller language models. The repository also provides links to YouTube videos explaining the concepts and techniques discussed in the notebooks.
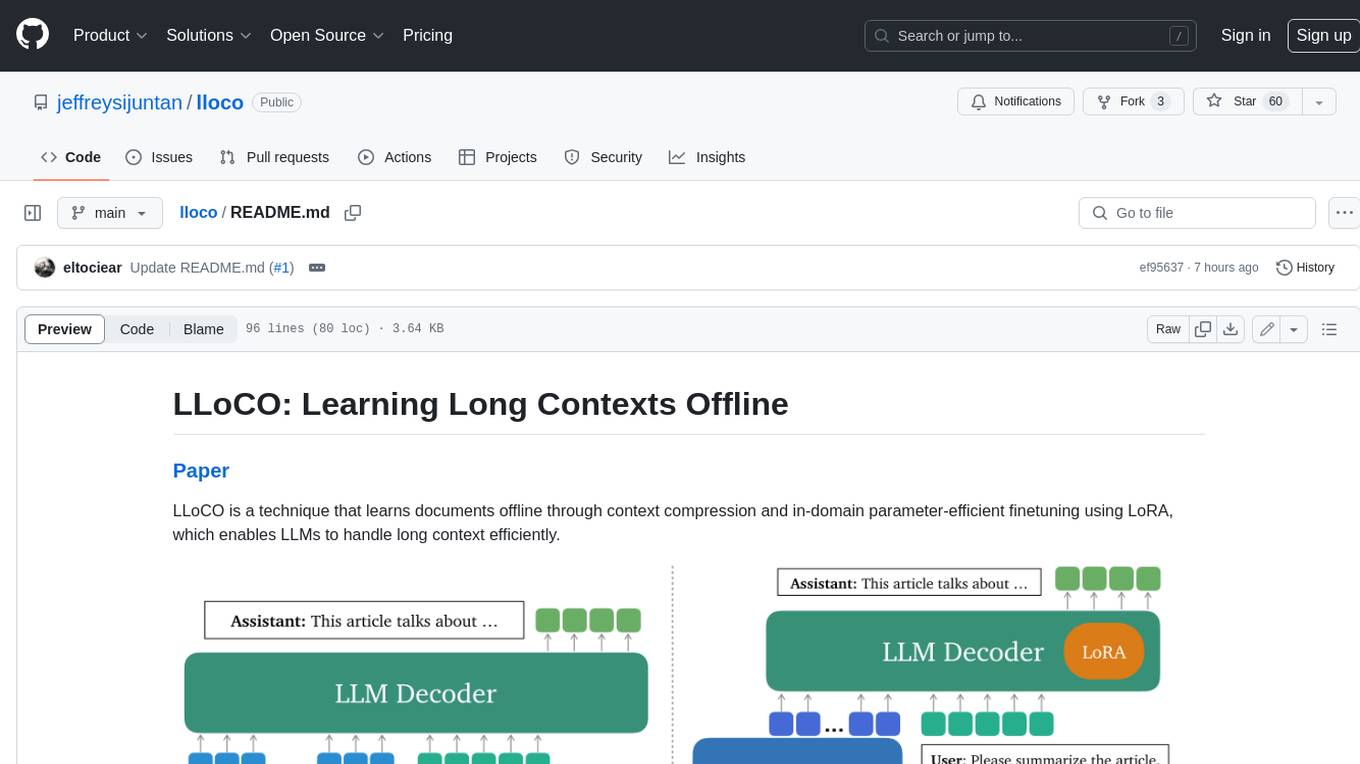
lloco
LLoCO is a technique that learns documents offline through context compression and in-domain parameter-efficient finetuning using LoRA, which enables LLMs to handle long context efficiently.
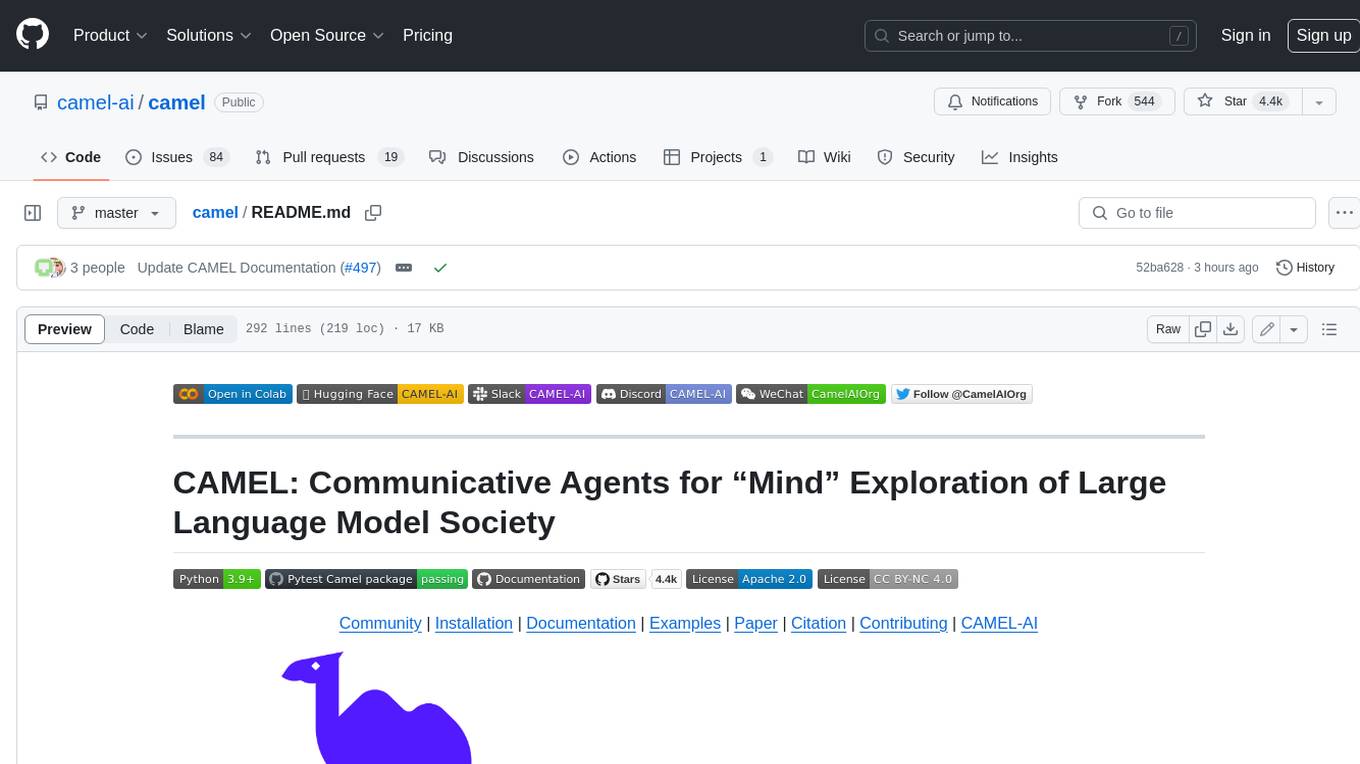
camel
CAMEL is an open-source library designed for the study of autonomous and communicative agents. We believe that studying these agents on a large scale offers valuable insights into their behaviors, capabilities, and potential risks. To facilitate research in this field, we implement and support various types of agents, tasks, prompts, models, and simulated environments.
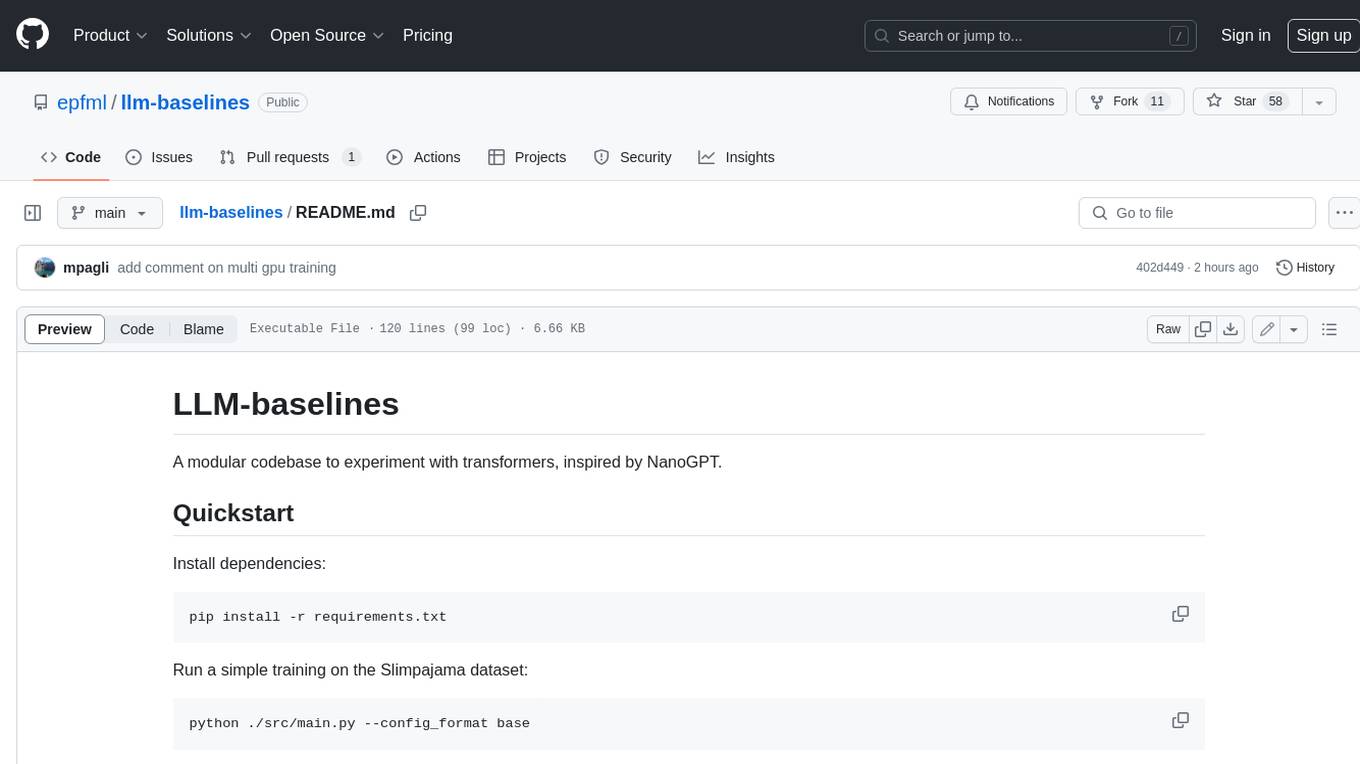
llm-baselines
LLM-baselines is a modular codebase to experiment with transformers, inspired from NanoGPT. It provides a quick and easy way to train and evaluate transformer models on a variety of datasets. The codebase is well-documented and easy to use, making it a great resource for researchers and practitioners alike.
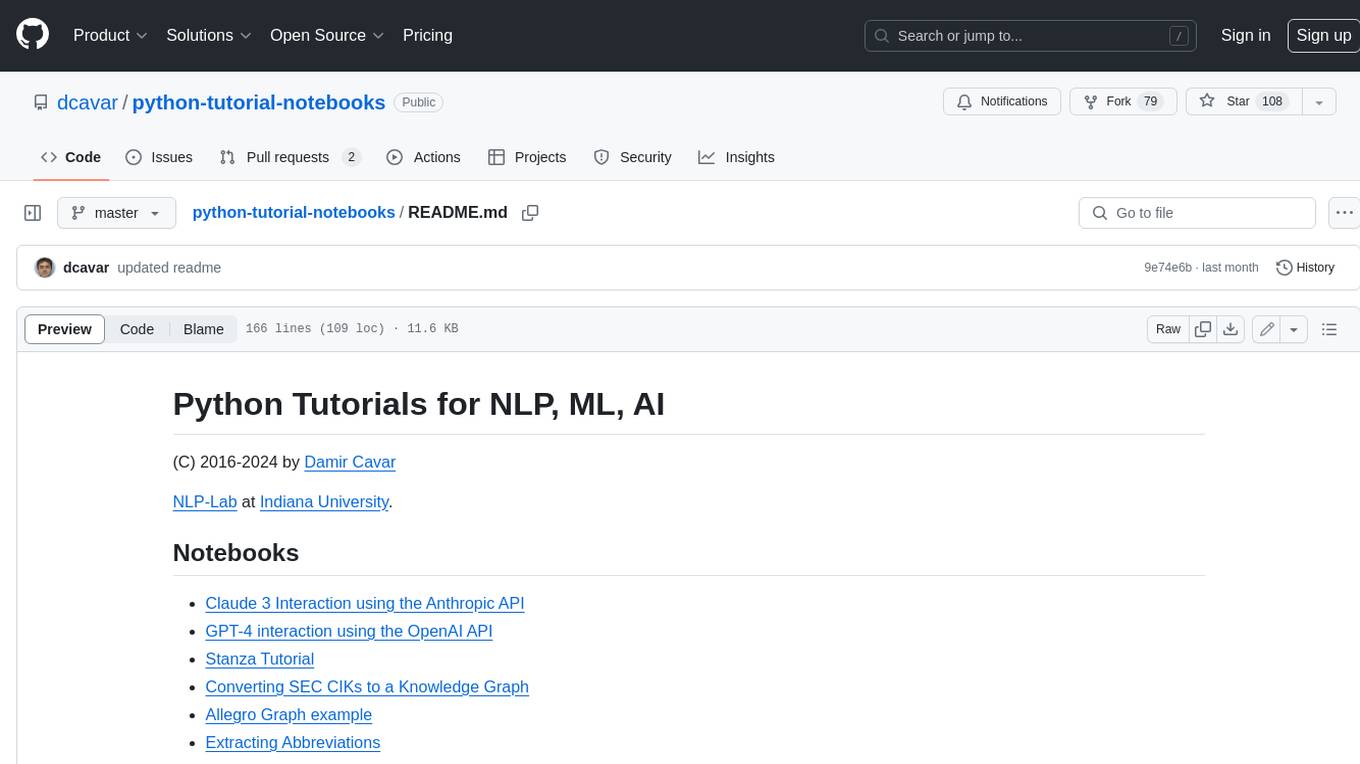
python-tutorial-notebooks
This repository contains Jupyter-based tutorials for NLP, ML, AI in Python for classes in Computational Linguistics, Natural Language Processing (NLP), Machine Learning (ML), and Artificial Intelligence (AI) at Indiana University.
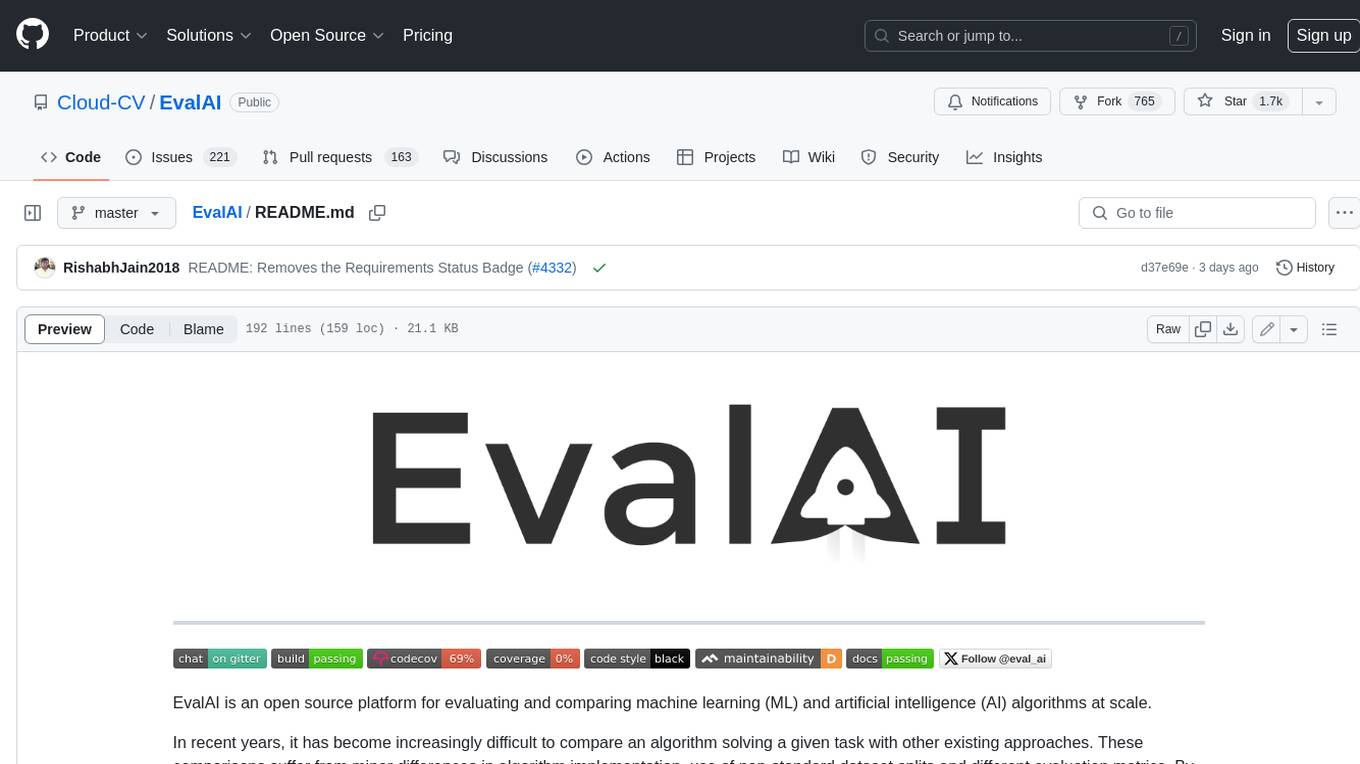
EvalAI
EvalAI is an open-source platform for evaluating and comparing machine learning (ML) and artificial intelligence (AI) algorithms at scale. It provides a central leaderboard and submission interface, making it easier for researchers to reproduce results mentioned in papers and perform reliable & accurate quantitative analysis. EvalAI also offers features such as custom evaluation protocols and phases, remote evaluation, evaluation inside environments, CLI support, portability, and faster evaluation.
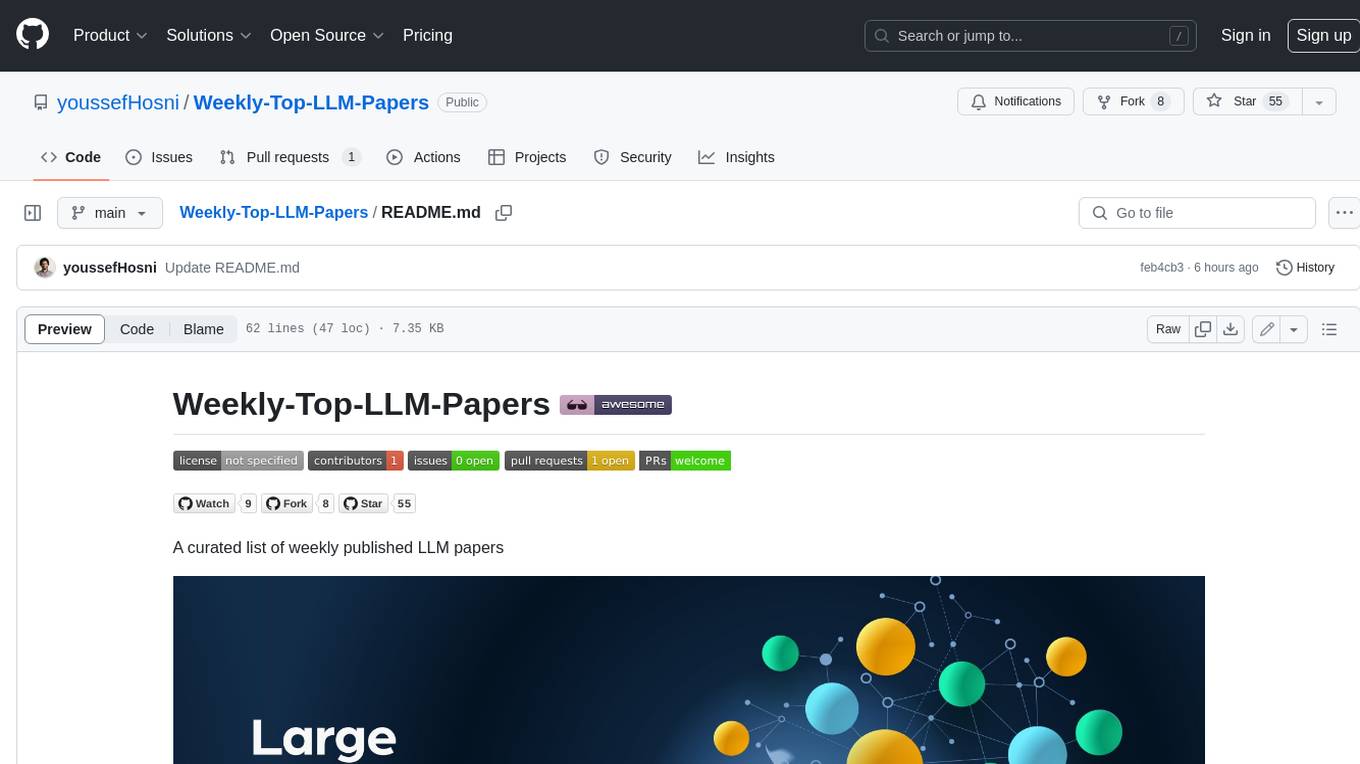
Weekly-Top-LLM-Papers
This repository provides a curated list of weekly published Large Language Model (LLM) papers. It includes top important LLM papers for each week, organized by month and year. The papers are categorized into different time periods, making it easy to find the most recent and relevant research in the field of LLM.
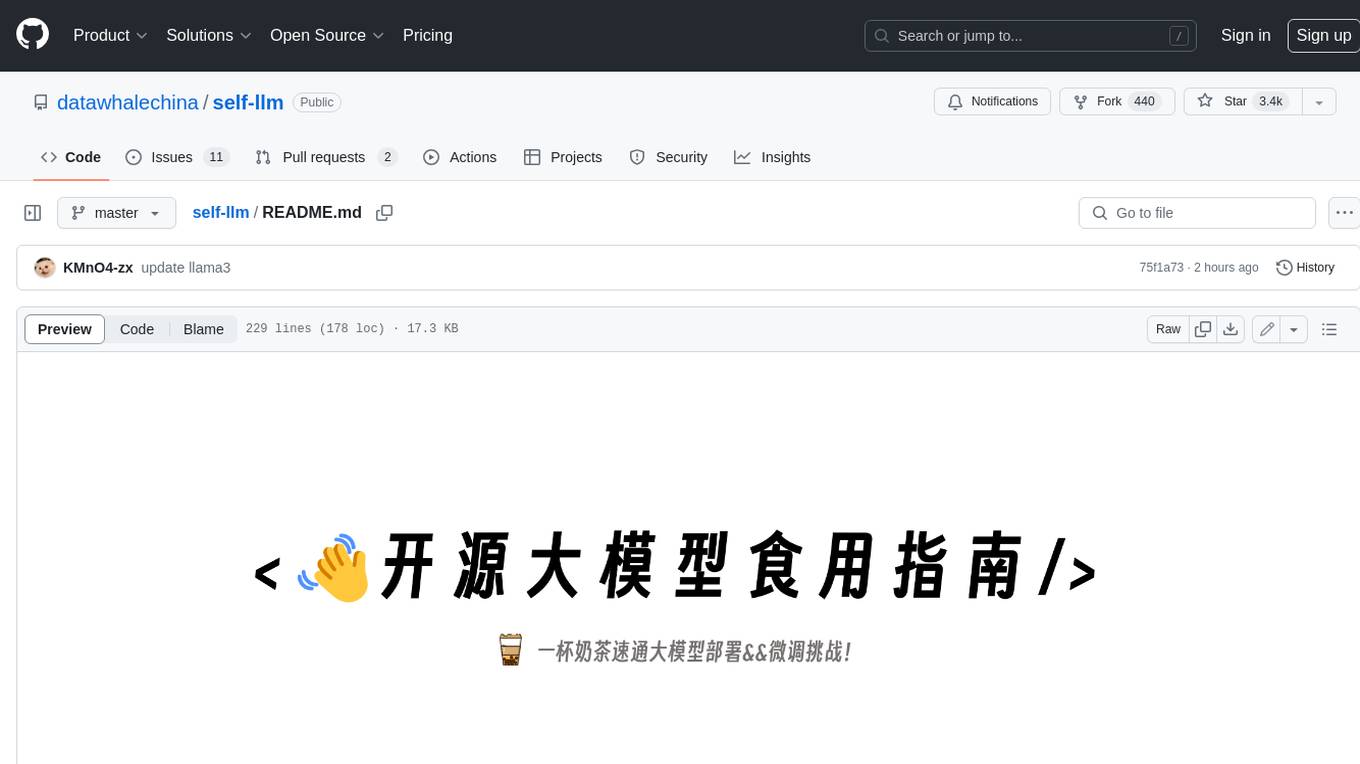
self-llm
This project is a Chinese tutorial for domestic beginners based on the AutoDL platform, providing full-process guidance for various open-source large models, including environment configuration, local deployment, and efficient fine-tuning. It simplifies the deployment, use, and application process of open-source large models, enabling more ordinary students and researchers to better use open-source large models and helping open and free large models integrate into the lives of ordinary learners faster.