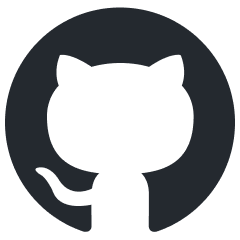
Customer-Service-Conversational-Insights-with-Azure-OpenAI-Services
A solution accelerator built on top of Microsoft Fabric, Azure OpenAI Service, and Azure AI Speech that enables customers with large amounts of conversational data to use generative AI to surface key phrases alongside operational metrics, unlocking valuable insights for targeted business impact.
Stars: 191
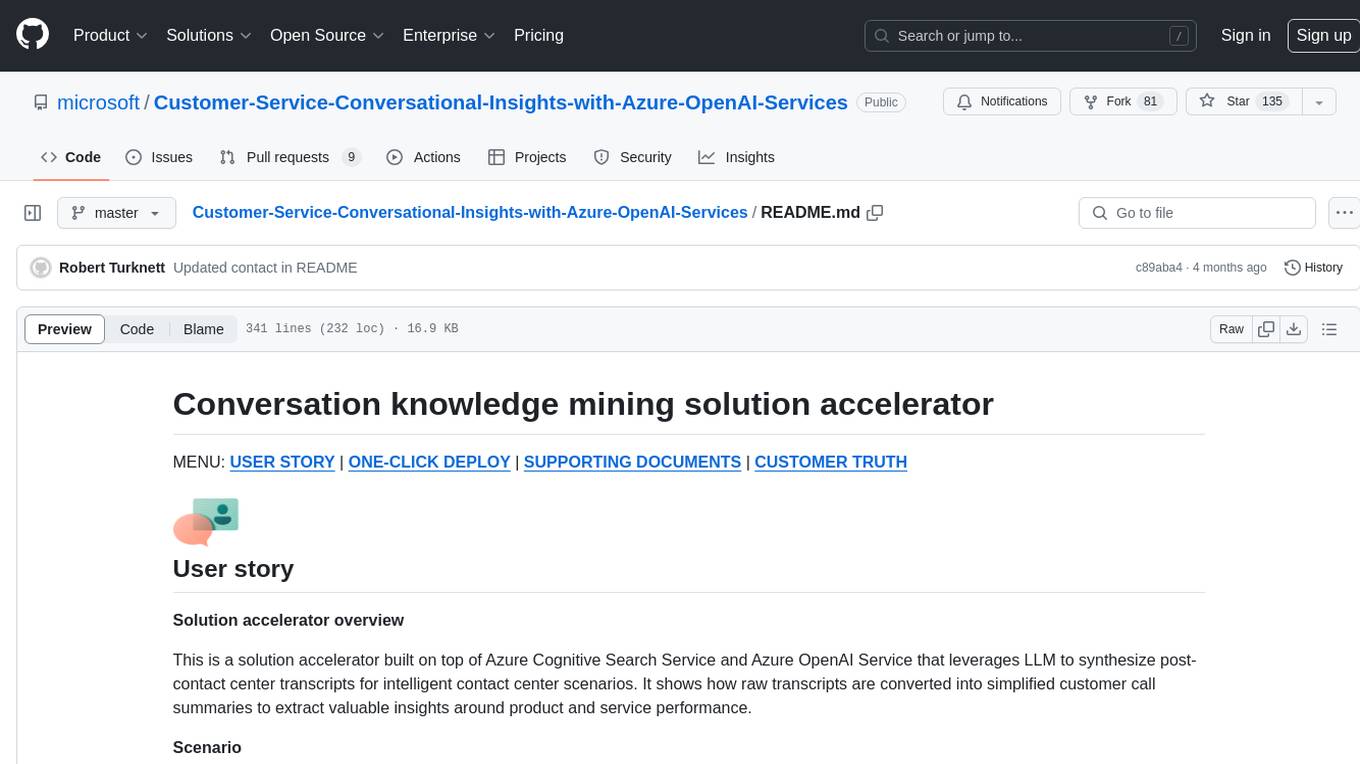
This solution accelerator is built on Azure Cognitive Search Service and Azure OpenAI Service to synthesize post-contact center transcripts for intelligent contact center scenarios. It converts raw transcripts into customer call summaries to extract insights around product and service performance. Key features include conversation summarization, key phrase extraction, speech-to-text transcription, sensitive information extraction, sentiment analysis, and opinion mining. The tool enables data professionals to quickly analyze call logs for improvement in contact center operations.
README:
MENU: USER STORY | SIMPLE DEPLOY | SUPPORTING DOCUMENTATION | CUSTOMER TRUTH
This solution accelerator enables customers with large amounts of conversational data to use generative AI to surface key phrases alongside operational metrics. This enables users to discover valuable insights for targeted business impact.
Version history: An updated version of the Conversation Knowledge Mining solution accelerator was published on 08/15/2024. If you deployed the accelerator prior to that date, please see “Version history” in the Supporting documentation section.
- Data processing: Microsoft Fabric processes both audio and conversation files at scale, leveraging its benefits for efficient and scalable data handling
- Summarization and key phrase extraction: Azure OpenAI is used to summarize long conversations into concise paragraphs and extract relevant key phrases
- Speech transcription and diarization: Azure Speech is used to transcribe audio files, including speaker diarization for post-call analytics. Diarization is the process of recognizing and separating individual speakers into mono-channel audio data
- Analytics dashboard: Power BI is used to visualize the correlation between operational metrics and AI-generated conversational data
A contact center manager reviews contact center performance to ensure resources are being used efficiently. To identify areas for improvement, they need to understand the correlation between conversational and operational data.
The contact center manager uses their dashboard to identify how LLM-generated conversational analytics and insights are impacting operations to make an informed decision about how to improve their center’s performance.
Company personnel (employees, executives) looking to gain conversational insights in correlation with operational Contact Center metrics would leverage this accelerator to find what they need quickly.
- Conversation analysis: Generative AI analyzes call transcripts, summarizes content, identifies and aggregates key phrases for data visualization
- Automated customer satisfaction: Generative AI determines the post-call satisfaction rating of a customer’s experience with their agent
- Operational clarity: Relevant metrics such as call volume, handling time, and call resolution are pulled from the same call logs for operational data visualization
- Unified data: Unstructured (call transcripts) and structured (operational metrics) data are both analyzed and visualized within the same application
- Targeted decision enablement: Enable agents and managers to achieve glanceable insight recognition, corollary information analysis, and accelerated decision making
-
Azure Speech Service
-
Azure OpenAI
-
Microsoft Fabric Capacity
-
The user deploying the template must have permission to create resources and resource groups.
-
Deploy Azure resources
Click the following deployment button to create the required resources for this accelerator directly in your Azure Subscription.-
Most fields will have a default name set already. You will need to update the following Azure OpenAI settings:
-
Region - the region where the resources will be created in
-
Solution Prefix - provide a 6 alphanumeric value that will be used to prefix resources
-
Location - location of resources, by default it will use the resource group's location
-
-
-
Create Fabric workspace
- Navigate to (Fabric Workspace)
- Click on Workspaces from left Navigation
- Click on + New Workspace
- Provide Name of Workspace
- Provide Description of Workspace (optional)
- Click Apply
- Open Workspace
- Retrieve Workspace ID from URL, refer to documentation additional assistance (here)
-
Deploy Fabric resources and artifacts
- Navigate to (Azure Portal)
- Click on Azure Cloud Shell in the top right of navigation Menu (add image)
- Run the run the following commands:
-
az login
***Follow instructions in Azure Cloud Shell for login instructions rm -rf ./Customer-Service-Conversational-Insights-with-Azure-OpenAI-Services
git clone https://github.com/microsoft/Customer-Service-Conversational-Insights-with-Azure-OpenAI-Services
cd ./Customer-Service-Conversational-Insights-with-Azure-OpenAI-Services/Deployment/scripts/fabric_scripts
-
sh ./run_fabric_items_scripts.sh keyvault_param workspaceid_param solutionprefix_param
- keyvault_param - the name of the keyvault that was created in Step 1
- workspaceid_param - the workspaceid created in Step 2
- solutionprefix_param - prefix used to append to lakehouse upon creation
-
- Get Fabric Lakehouse connection details:
- Once deployment is complete, navigate to Fabric Workspace
- Find Lakehouse in workspace (ex.lakehouse_solutionprefix_param)
- Click on the
...
next to the SQL Analytics Endpoint - Click on
Copy SQL connection string
- Click Copy button in popup window.
- Wait 10-15 minutes to allow the data pipelines to finish processing then proceed to next step.
-
Open Power BI report
- Download the .pbix file from the Reports folder.
- Open Power BI report in Power BI Dashboard
- Click on
Transform Data
menu option from the Task Bar - Click
Data source settings
- Click
Change Source...
- Input the Server link (from Fabric Workspace)
- Input Database name (the lakehouse name from Fabric Workspace)
- Click
OK
- Click
Edit Permissions
- If not signed in, sign in your credentials and proceed to click OK
- Click
Close
- Report should refresh with new connection.
-
Publish Power BI
- Click
Publish
(from PBI report in Power BI Desktop application) - Select Fabric Workspace
- Click
Select
- After publish is complete, navigate to Fabric Workspace
- Click
...
next to the Semantic model for Power BI report - Click on
Settings
- Click on
Edit credentials
(under Data source credentials) - Select
OAuth2
for the Authentication method - Select option for
Privacy level setting for this data source
- Click
Sign in
- Navigate back to Fabric workspace and click on Power BI report
- Click
-
Schedule Post-Processing Notebook
It is essential to update dates daily as they advance based on the current day at the time of deployment. Since the Power BI report relies on the current date, we highly recommend scheduling or running the 03_post_processing notebook daily in the workspace. Please note that this process modifies the original date of the processed data. If you do not wish to run this, do not execute the 03_post_processing notebook.To schedule the notebook, follow these steps:
- Navigate to the Workspace
- Click on the "..." next to the 03_post_processing notebook
- Select "Schedule"
- Configure the schedule settings (we recommend running the notebook at least daily)
Currently, audio files are not processed during deployment. To manually process audio files, follow these steps:
- Open the
pipeline_notebook
- Comment out cell 2 (only if there are zero files in the
conversation_input
data folder waiting for JSON processing) - Uncomment cells 3 and 4
- Run
pipeline_notebook
All files JSON and WAV files can be uploaded in the corresponding Lakehouse in the data/Files folder:
-
Conversation (JSON files): Upload JSON files in the conversation_input folder.
-
Audio (WAV files): Upload Audio files in the audio_input folder.
- To process additional files, manually execute the pipeline_notebook after uploading new files.
- The OpenAI prompt can be modified within the Fabric notebooks.
If you'd like to customize the accelerator, here are some ways you might do that:
- Modify the Power BI report to use a custom logo
- Ingest your own JSON conversation files by uploading them into the
conversation_input
lakehouse folder and run the data pipeline - Ingest your own audio conversation files by uploading them into the
audio_input
lakehouse folder and run the data pipeline
- Microsoft Fabric documentation - Microsoft Fabric | Microsoft Learn
- Azure OpenAI Service - Documentation, quickstarts, API reference - Azure AI services | Microsoft Learn
- Speech service documentation - Tutorials, API Reference - Azure AI services - Azure AI services | Microsoft Learn
An updated version of the Conversation Knowledge Mining (CKM) solution accelerator was published on 08/15/2024. If you deployed the accelerator prior to that date, please note that CKM v2 cannot be deployed over CKM v1. Please also note that the CKM v2 .json conversation file format has been revised to include additional metadata, therefore CKM v1 files are no longer compatible. For resources related to CKM v1, please visit our archive (link-to-archive).
Customer stories coming soon.To the extent that the Software includes components or code used in or derived from Microsoft products or services, including without limitation Microsoft Azure Services (collectively, “Microsoft Products and Services”), you must also comply with the Product Terms applicable to such Microsoft Products and Services. You acknowledge and agree that the license governing the Software does not grant you a license or other right to use Microsoft Products and Services. Nothing in the license or this ReadMe file will serve to supersede, amend, terminate or modify any terms in the Product Terms for any Microsoft Products and Services.
You must also comply with all domestic and international export laws and regulations that apply to the Software, which include restrictions on destinations, end users, and end use. For further information on export restrictions, visit https://aka.ms/exporting.
You acknowledge that the Software and Microsoft Products and Services (1) are not designed, intended or made available as a medical device(s), and (2) are not designed or intended to be a substitute for professional medical advice, diagnosis, treatment, or judgment and should not be used to replace or as a substitute for professional medical advice, diagnosis, treatment, or judgment. Customer is solely responsible for displaying and/or obtaining appropriate consents, warnings, disclaimers, and acknowledgements to end users of Customer’s implementation of the Online Services.
You acknowledge the Software is not subject to SOC 1 and SOC 2 compliance audits. No Microsoft technology, nor any of its component technologies, including the Software, is intended or made available as a substitute for the professional advice, opinion, or judgement of a certified financial services professional. Do not use the Software to replace, substitute, or provide professional financial advice or judgment.
BY ACCESSING OR USING THE SOFTWARE, YOU ACKNOWLEDGE THAT THE SOFTWARE IS NOT DESIGNED OR INTENDED TO SUPPORT ANY USE IN WHICH A SERVICE INTERRUPTION, DEFECT, ERROR, OR OTHER FAILURE OF THE SOFTWARE COULD RESULT IN THE DEATH OR SERIOUS BODILY INJURY OF ANY PERSON OR IN PHYSICAL OR ENVIRONMENTAL DAMAGE (COLLECTIVELY, “HIGH-RISK USE”), AND THAT YOU WILL ENSURE THAT, IN THE EVENT OF ANY INTERRUPTION, DEFECT, ERROR, OR OTHER FAILURE OF THE SOFTWARE, THE SAFETY OF PEOPLE, PROPERTY, AND THE ENVIRONMENT ARE NOT REDUCED BELOW A LEVEL THAT IS REASONABLY, APPROPRIATE, AND LEGAL, WHETHER IN GENERAL OR IN A SPECIFIC INDUSTRY. BY ACCESSING THE SOFTWARE, YOU FURTHER ACKNOWLEDGE THAT YOUR HIGH-RISK USE OF THE SOFTWARE IS AT YOUR OWN RISK.
For Tasks:
Click tags to check more tools for each tasksFor Jobs:
Alternative AI tools for Customer-Service-Conversational-Insights-with-Azure-OpenAI-Services
Similar Open Source Tools
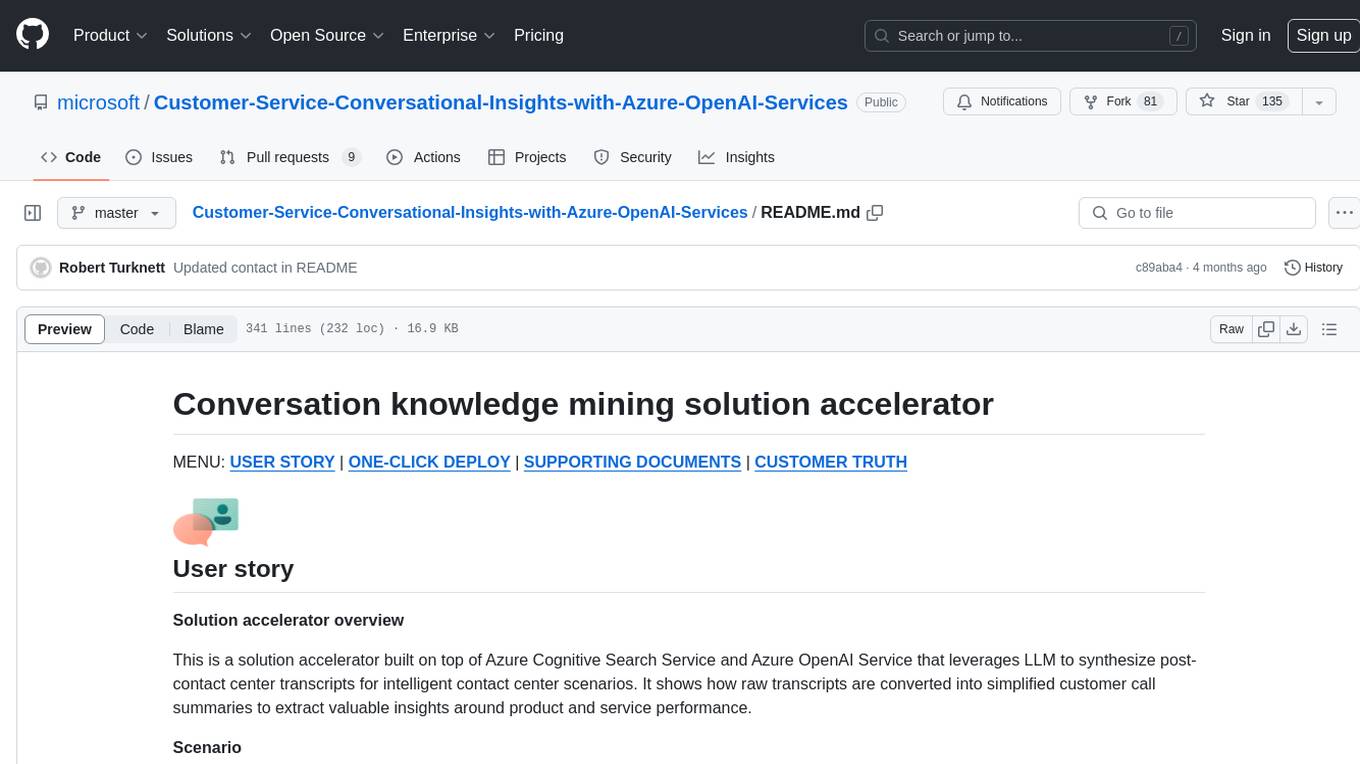
Customer-Service-Conversational-Insights-with-Azure-OpenAI-Services
This solution accelerator is built on Azure Cognitive Search Service and Azure OpenAI Service to synthesize post-contact center transcripts for intelligent contact center scenarios. It converts raw transcripts into customer call summaries to extract insights around product and service performance. Key features include conversation summarization, key phrase extraction, speech-to-text transcription, sensitive information extraction, sentiment analysis, and opinion mining. The tool enables data professionals to quickly analyze call logs for improvement in contact center operations.
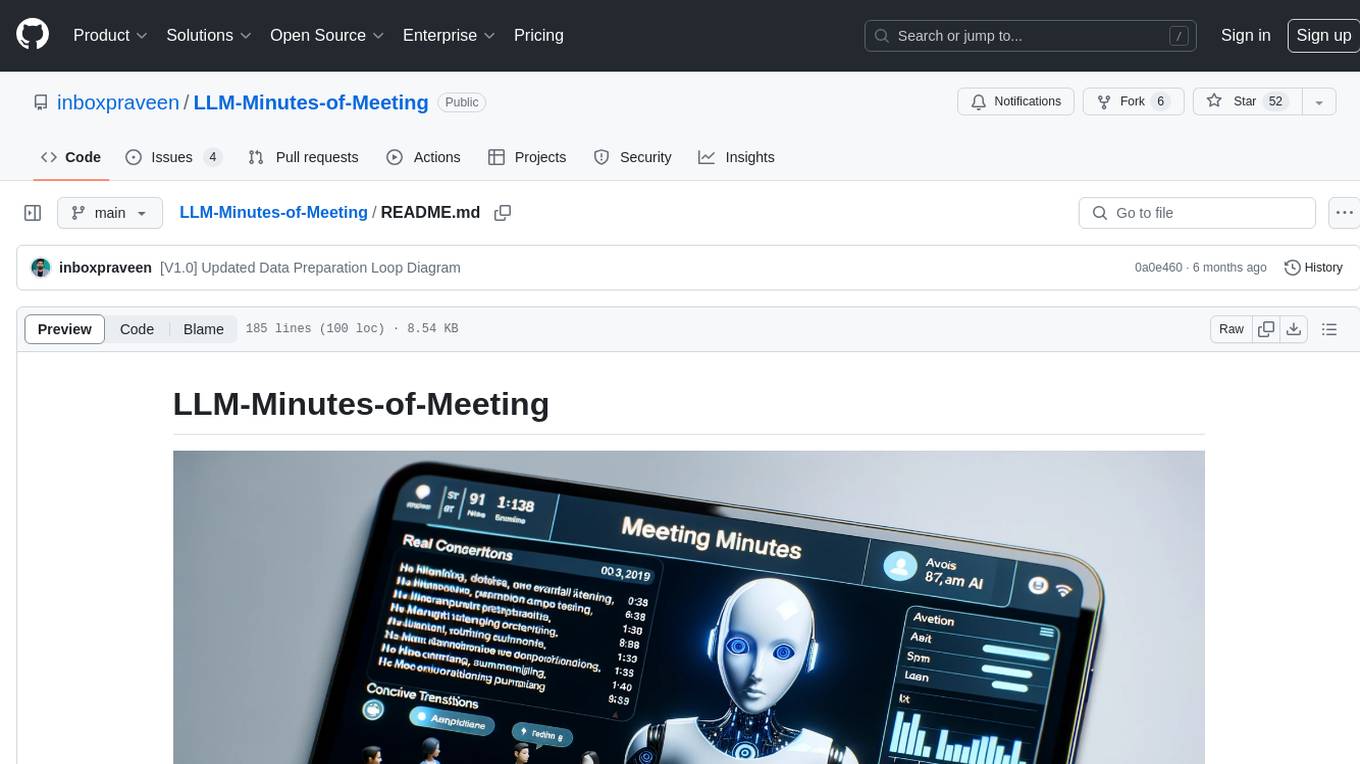
LLM-Minutes-of-Meeting
LLM-Minutes-of-Meeting is a project showcasing NLP & LLM's capability to summarize long meetings and automate the task of delegating Minutes of Meeting(MoM) emails. It converts audio/video files to text, generates editable MoM, and aims to develop a real-time python web-application for meeting automation. The tool features keyword highlighting, topic tagging, export in various formats, user-friendly interface, and uses Celery for asynchronous processing. It is designed for corporate meetings, educational institutions, legal and medical fields, accessibility, and event coverage.
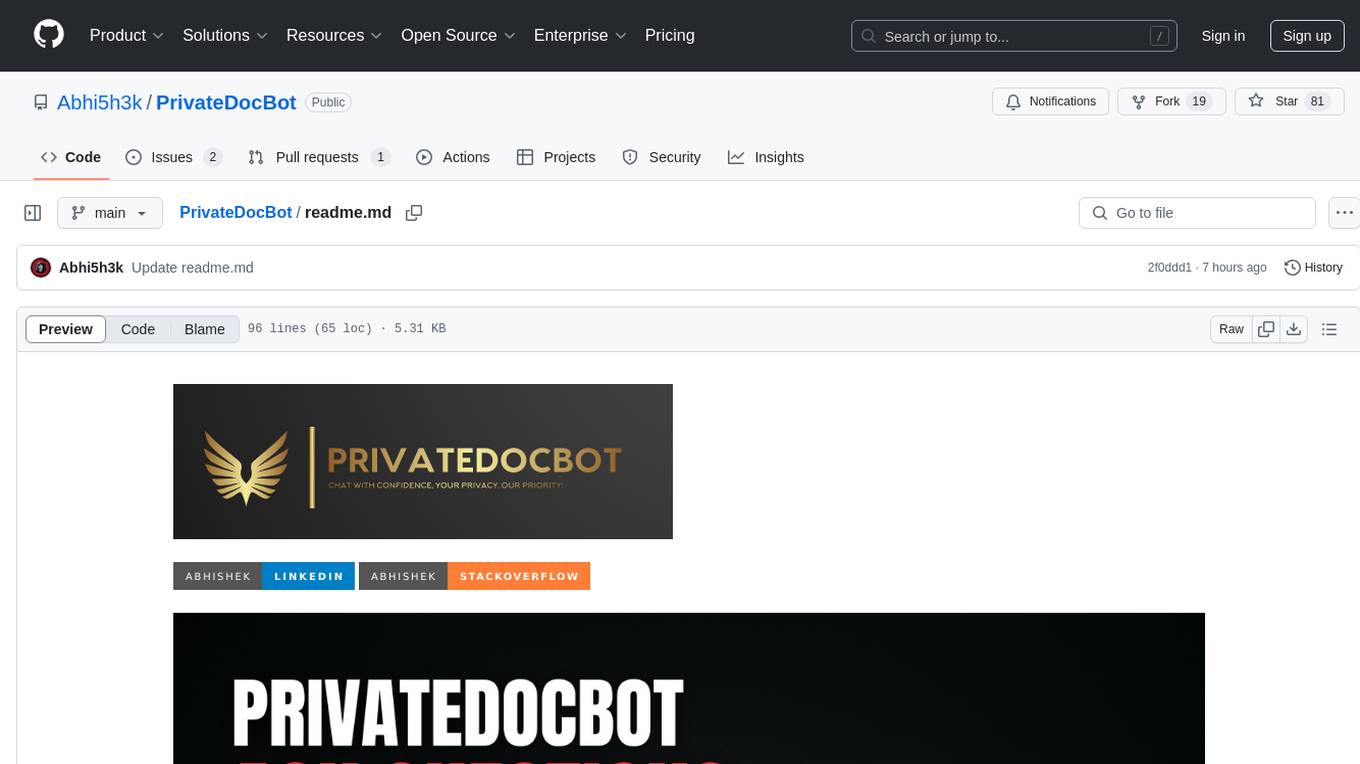
PrivateDocBot
PrivateDocBot is a local LLM-powered chatbot designed for secure document interactions. It seamlessly merges Chainlit user-friendly interface with localized language models, tailored for sensitive data. The project streamlines data access by deciphering intricate user guides and extracting vital insights from complex PDF reports. Equipped with advanced technology, it offers an engaging conversational experience, redefining data interaction and empowering users with control.
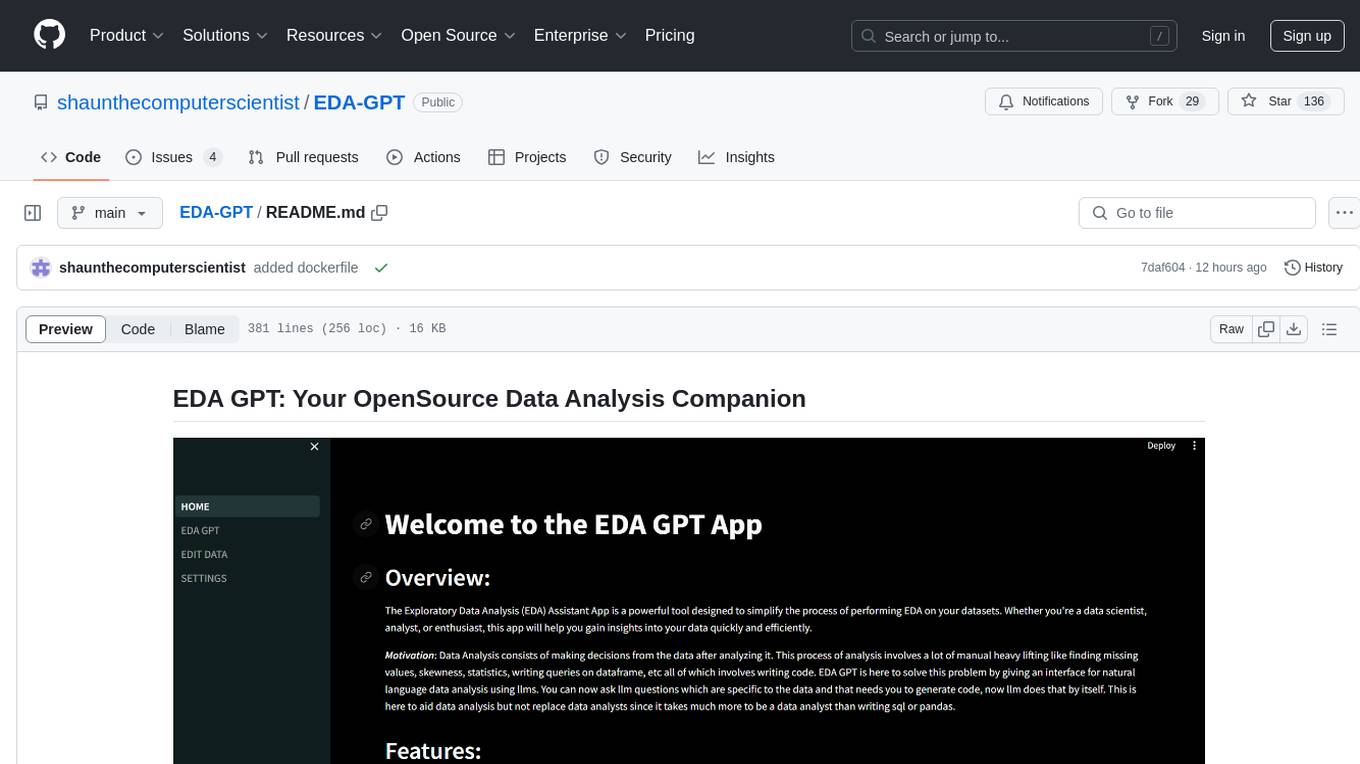
EDA-GPT
EDA GPT is an open-source data analysis companion that offers a comprehensive solution for structured and unstructured data analysis. It streamlines the data analysis process, empowering users to explore, visualize, and gain insights from their data. EDA GPT supports analyzing structured data in various formats like CSV, XLSX, and SQLite, generating graphs, and conducting in-depth analysis of unstructured data such as PDFs and images. It provides a user-friendly interface, powerful features, and capabilities like comparing performance with other tools, analyzing large language models, multimodal search, data cleaning, and editing. The tool is optimized for maximal parallel processing, searching internet and documents, and creating analysis reports from structured and unstructured data.
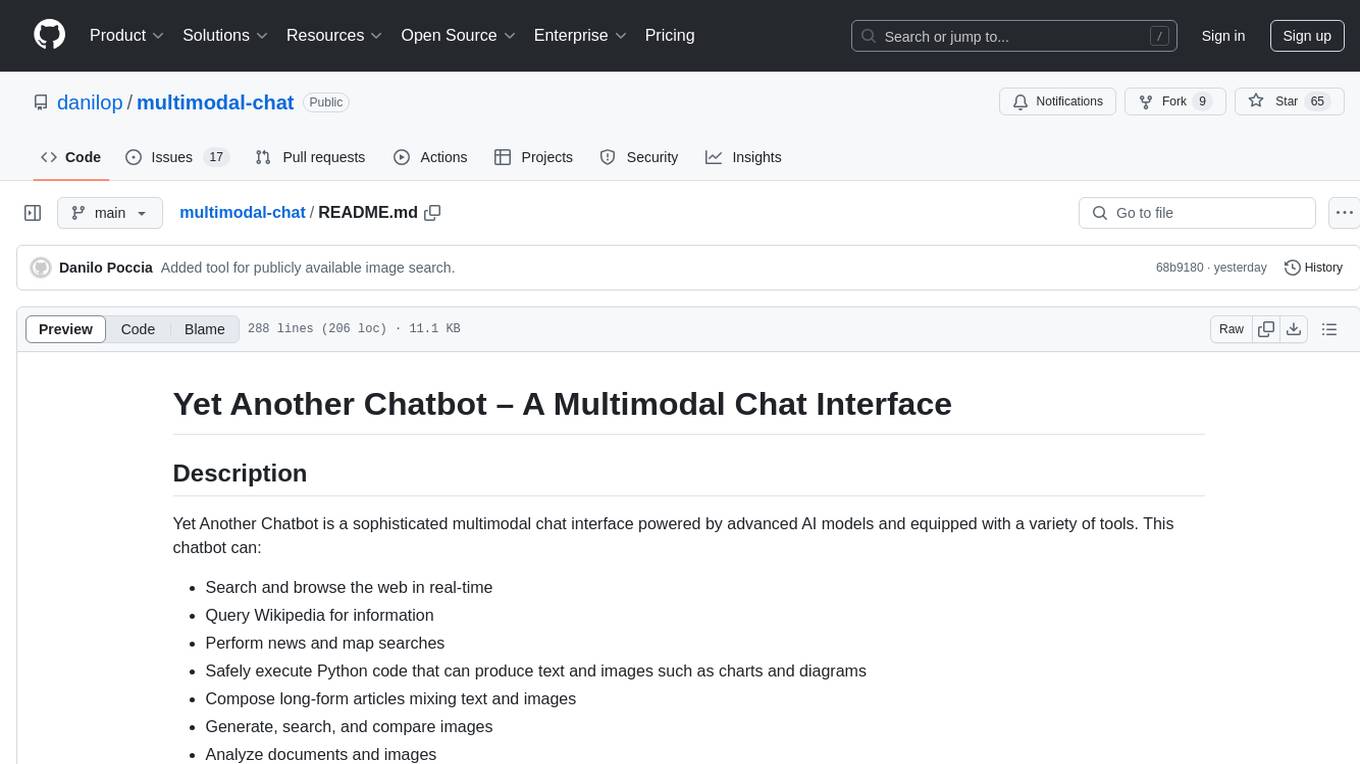
multimodal-chat
Yet Another Chatbot is a sophisticated multimodal chat interface powered by advanced AI models and equipped with a variety of tools. This chatbot can search and browse the web in real-time, query Wikipedia for information, perform news and map searches, execute Python code, compose long-form articles mixing text and images, generate, search, and compare images, analyze documents and images, search and download arXiv papers, save conversations as text and audio files, manage checklists, and track personal improvements. It offers tools for web interaction, Wikipedia search, Python scripting, content management, image handling, arXiv integration, conversation generation, file management, personal improvement, and checklist management.
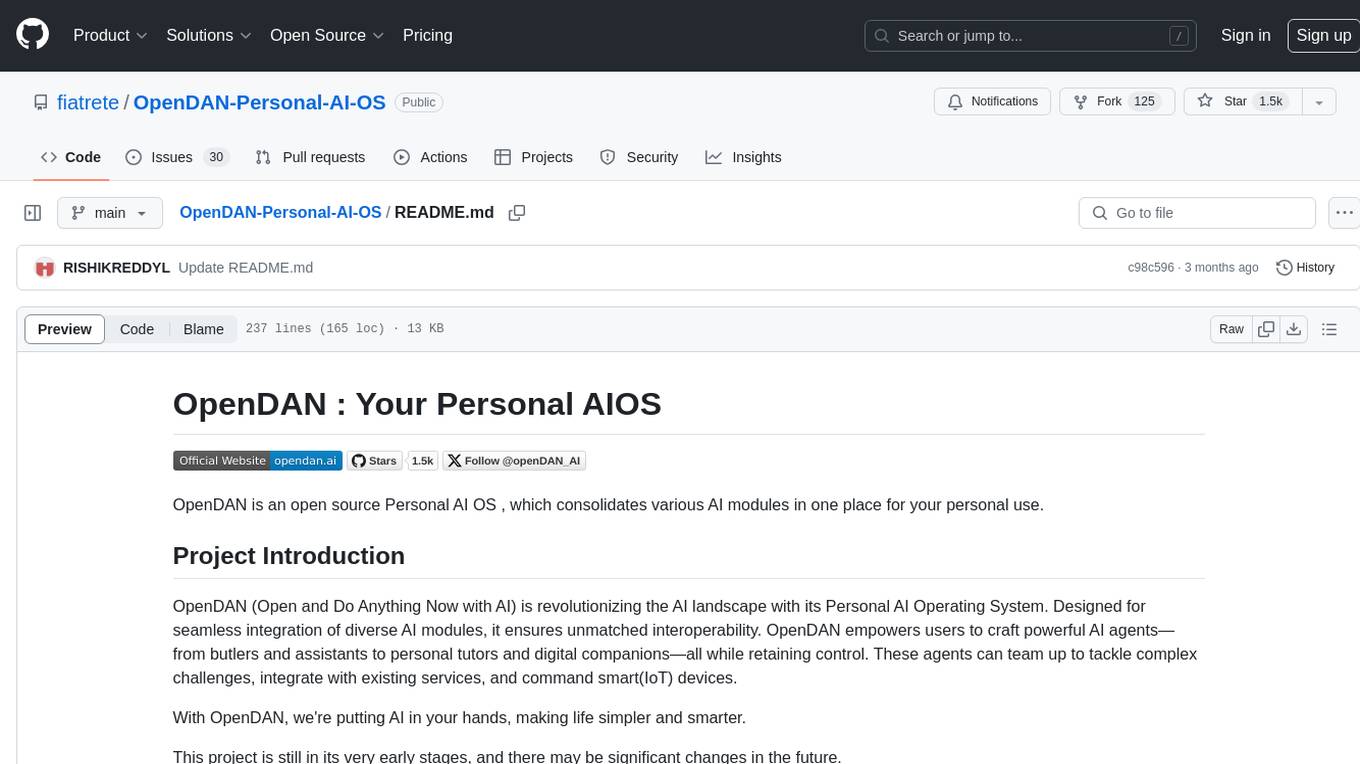
OpenDAN-Personal-AI-OS
OpenDAN is an open source Personal AI OS that consolidates various AI modules for personal use. It empowers users to create powerful AI agents like assistants, tutors, and companions. The OS allows agents to collaborate, integrate with services, and control smart devices. OpenDAN offers features like rapid installation, AI agent customization, connectivity via Telegram/Email, building a local knowledge base, distributed AI computing, and more. It aims to simplify life by putting AI in users' hands. The project is in early stages with ongoing development and future plans for user and kernel mode separation, home IoT device control, and an official OpenDAN SDK release.
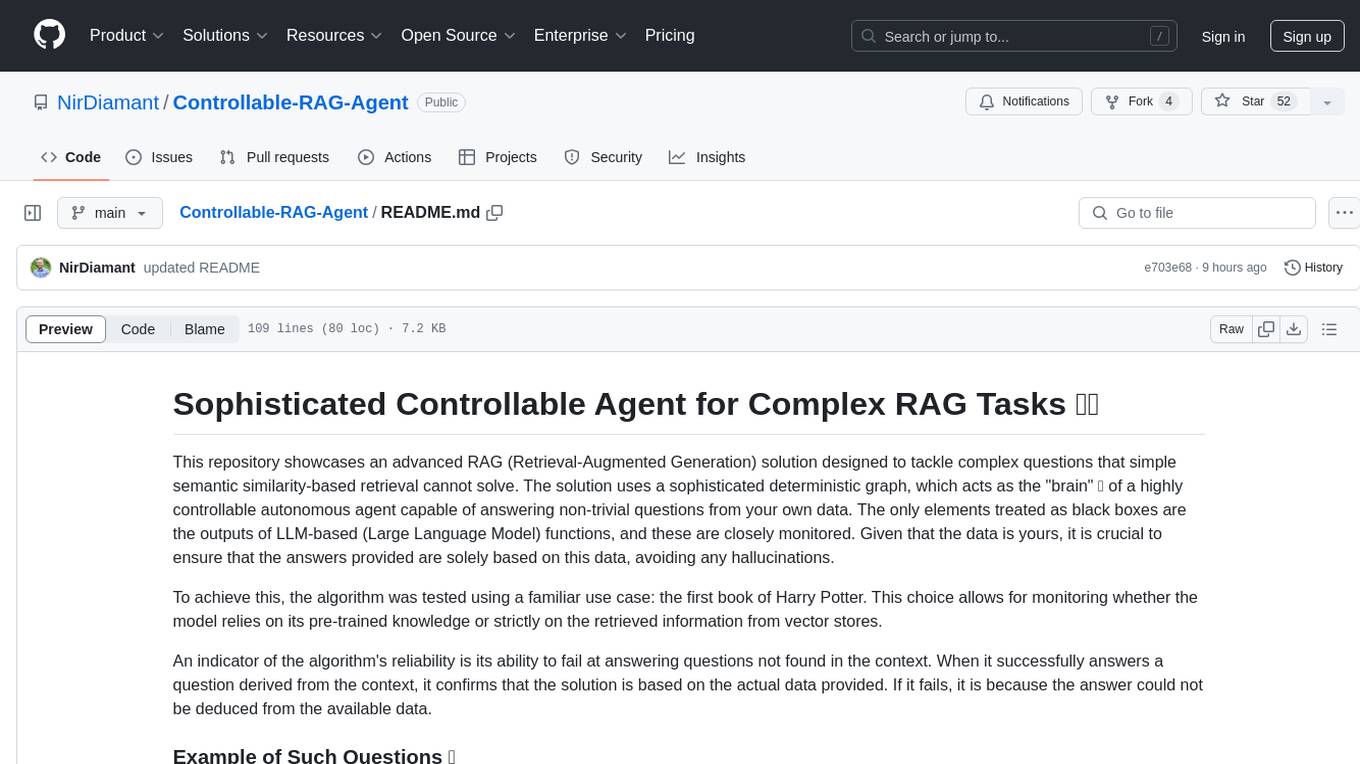
Controllable-RAG-Agent
This repository contains a sophisticated deterministic graph-based solution for answering complex questions using a controllable autonomous agent. The solution is designed to ensure that answers are solely based on the provided data, avoiding hallucinations. It involves various steps such as PDF loading, text preprocessing, summarization, database creation, encoding, and utilizing large language models. The algorithm follows a detailed workflow involving planning, retrieval, answering, replanning, content distillation, and performance evaluation. Heuristics and techniques implemented focus on content encoding, anonymizing questions, task breakdown, content distillation, chain of thought answering, verification, and model performance evaluation.
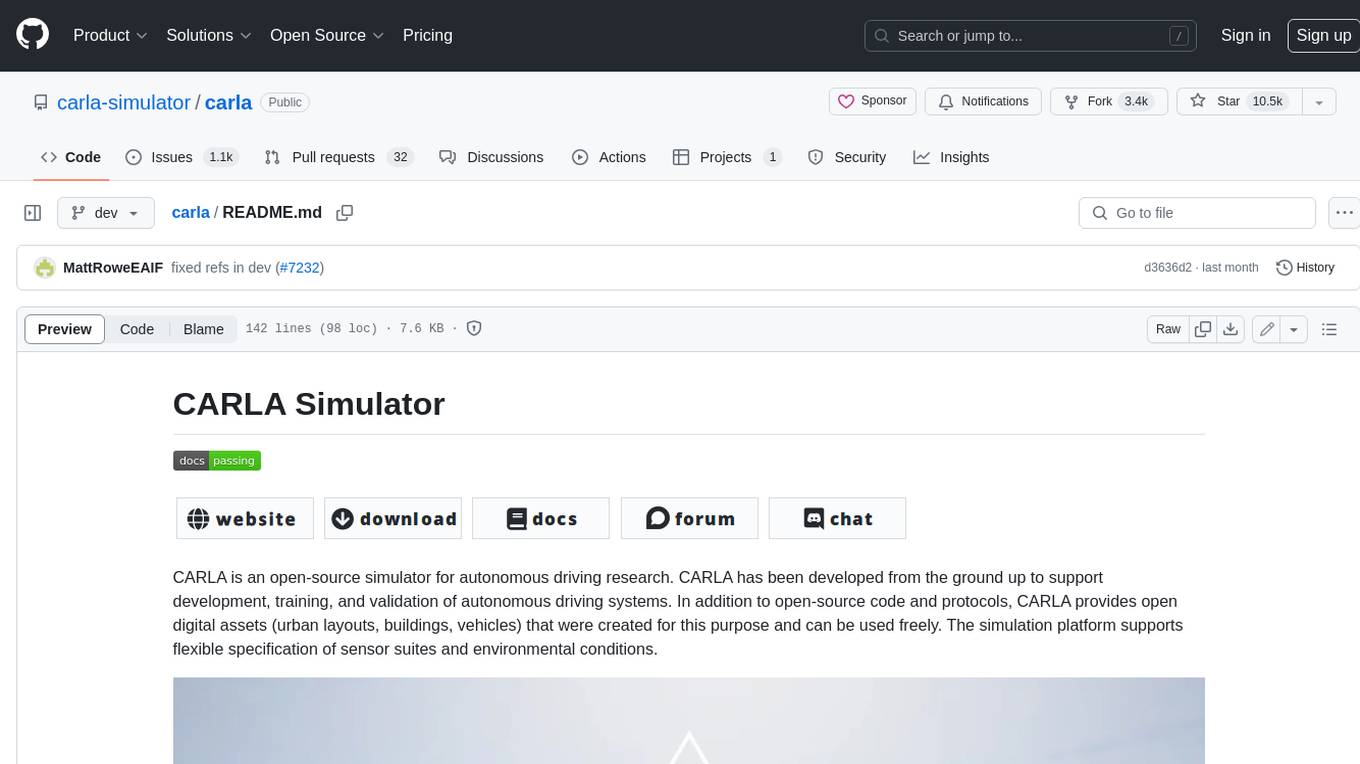
carla
CARLA is an open-source simulator for autonomous driving research. It provides open-source code, protocols, and digital assets (urban layouts, buildings, vehicles) for developing, training, and validating autonomous driving systems. CARLA supports flexible specification of sensor suites and environmental conditions.
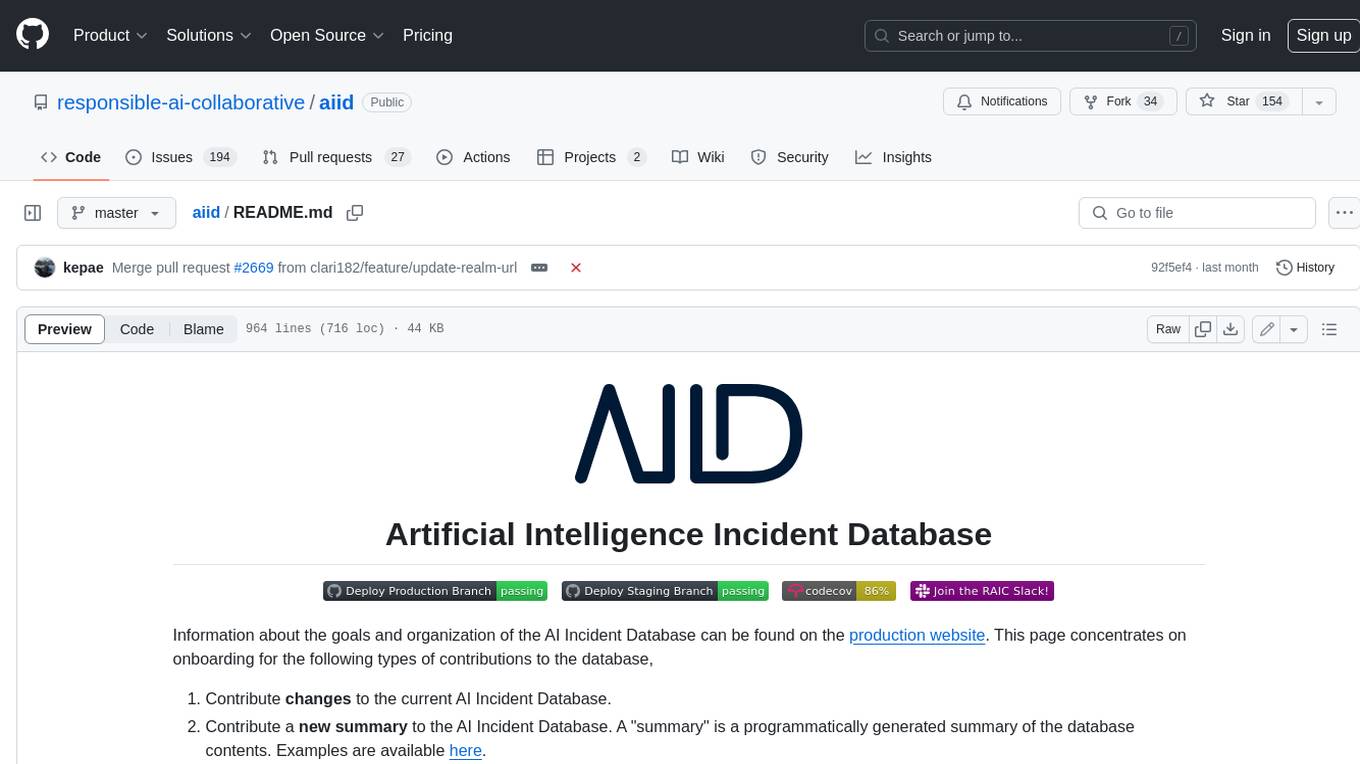
aiid
The Artificial Intelligence Incident Database (AIID) is a collection of incidents involving the development and use of artificial intelligence (AI). The database is designed to help researchers, policymakers, and the public understand the potential risks and benefits of AI, and to inform the development of policies and practices to mitigate the risks and promote the benefits of AI. The AIID is a collaborative project involving researchers from the University of California, Berkeley, the University of Washington, and the University of Toronto.
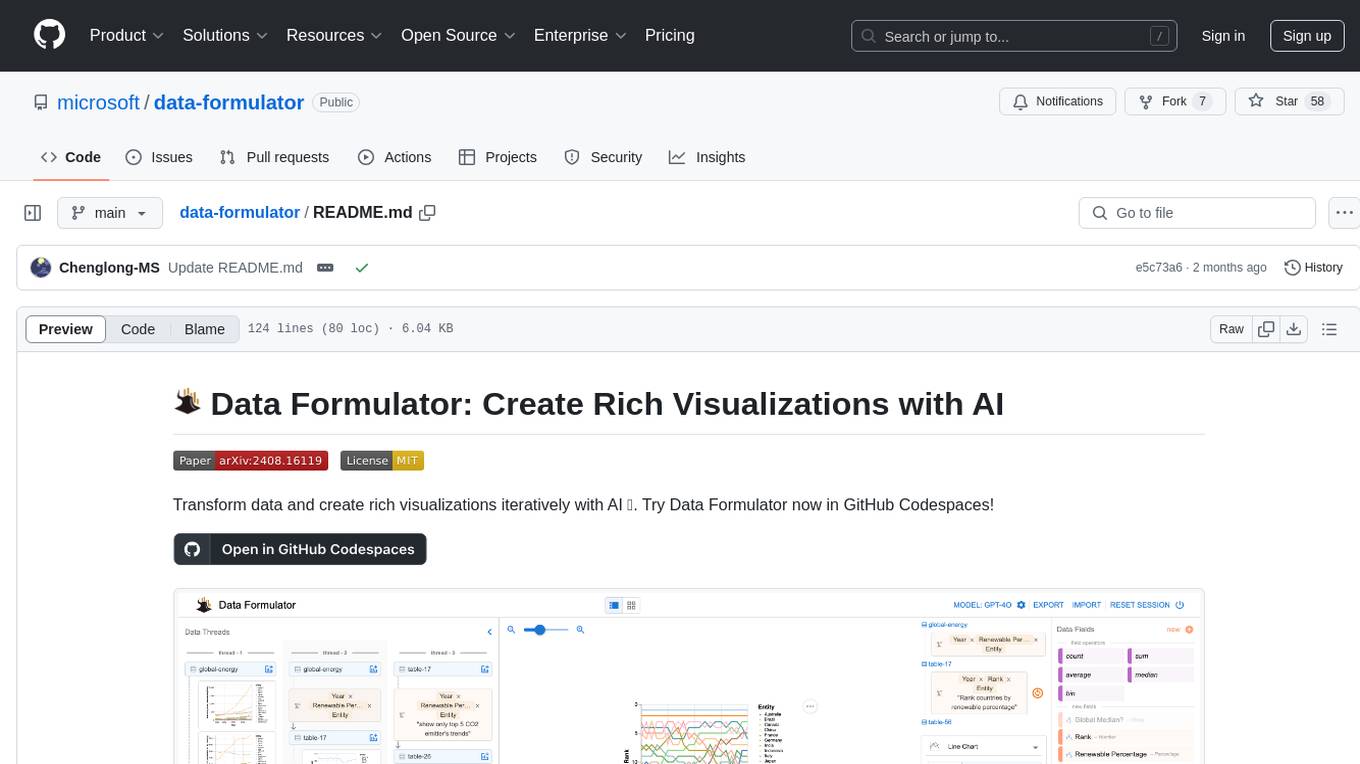
data-formulator
Data Formulator is an AI-powered tool developed by Microsoft Research to help data analysts create rich visualizations iteratively. It combines user interface interactions with natural language inputs to simplify the process of describing chart designs while delegating data transformation to AI. Users can utilize features like blended UI and NL inputs, data threads for history navigation, and code inspection to create impressive visualizations. The tool supports local installation for customization and Codespaces for quick setup. Developers can build new data analysis tools on top of Data Formulator, and research papers are available for further reading.
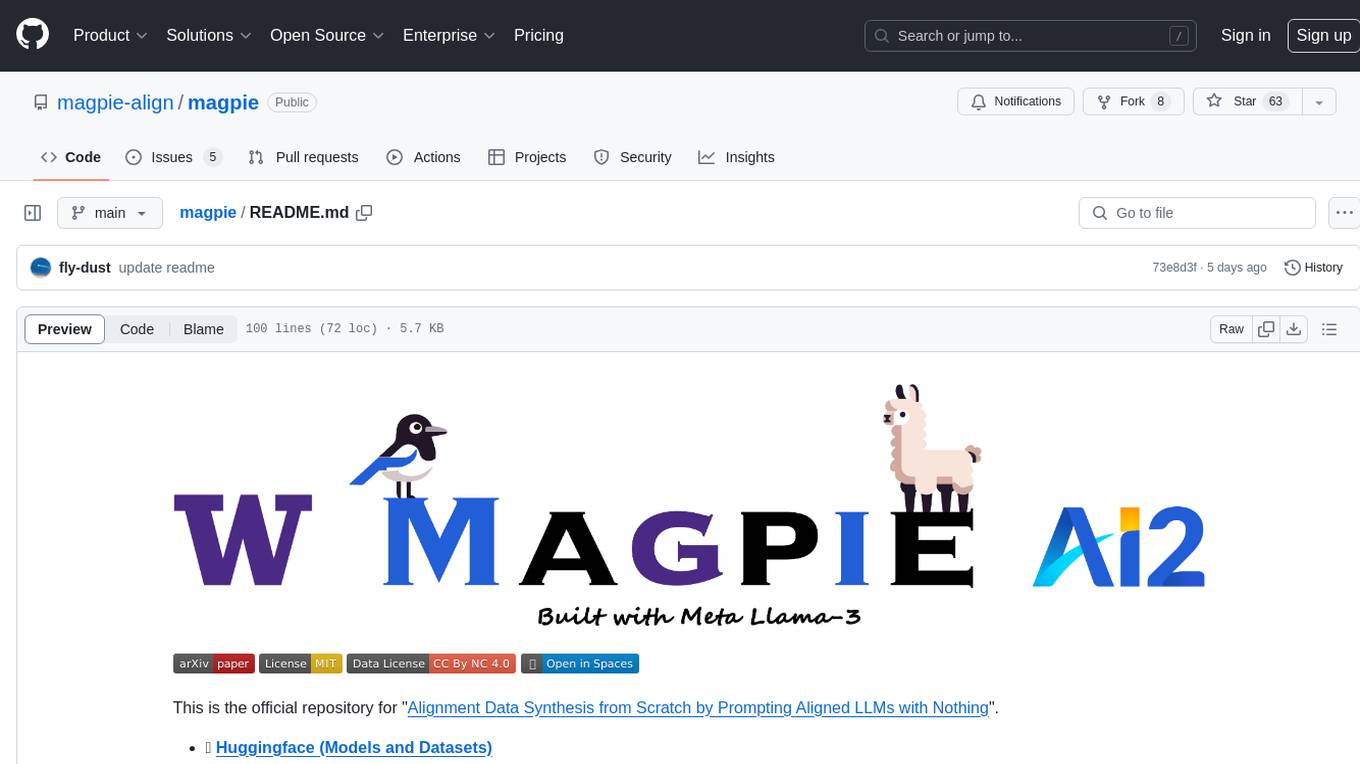
magpie
This is the official repository for 'Alignment Data Synthesis from Scratch by Prompting Aligned LLMs with Nothing'. Magpie is a tool designed to synthesize high-quality instruction data at scale by extracting it directly from an aligned Large Language Models (LLMs). It aims to democratize AI by generating large-scale alignment data and enhancing the transparency of model alignment processes. Magpie has been tested on various model families and can be used to fine-tune models for improved performance on alignment benchmarks such as AlpacaEval, ArenaHard, and WildBench.
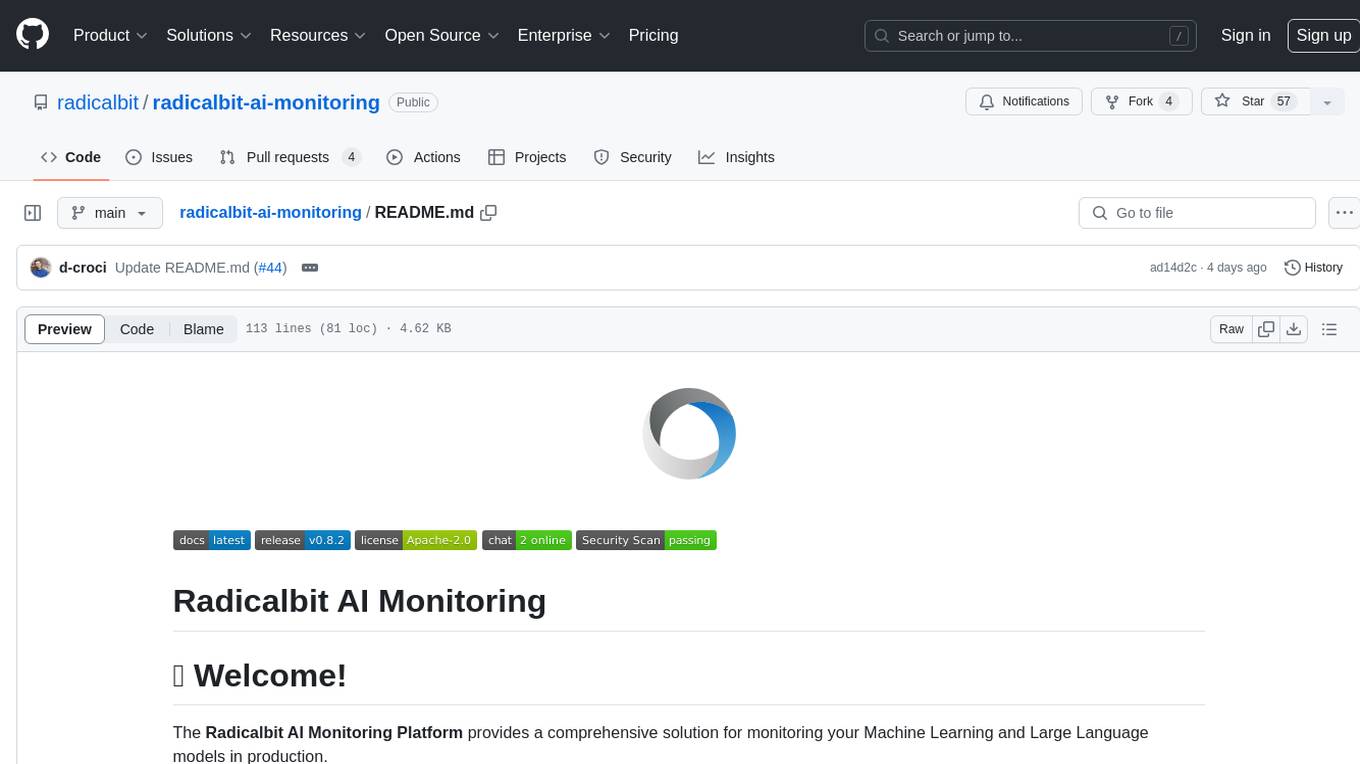
radicalbit-ai-monitoring
The Radicalbit AI Monitoring Platform provides a comprehensive solution for monitoring Machine Learning and Large Language models in production. It helps proactively identify and address potential performance issues by analyzing data quality, model quality, and model drift. The repository contains files and projects for running the platform, including UI, API, SDK, and Spark components. Installation using Docker compose is provided, allowing deployment with a K3s cluster and interaction with a k9s container. The platform documentation includes a step-by-step guide for installation and creating dashboards. Community engagement is encouraged through a Discord server. The roadmap includes adding functionalities for batch and real-time workloads, covering various model types and tasks.
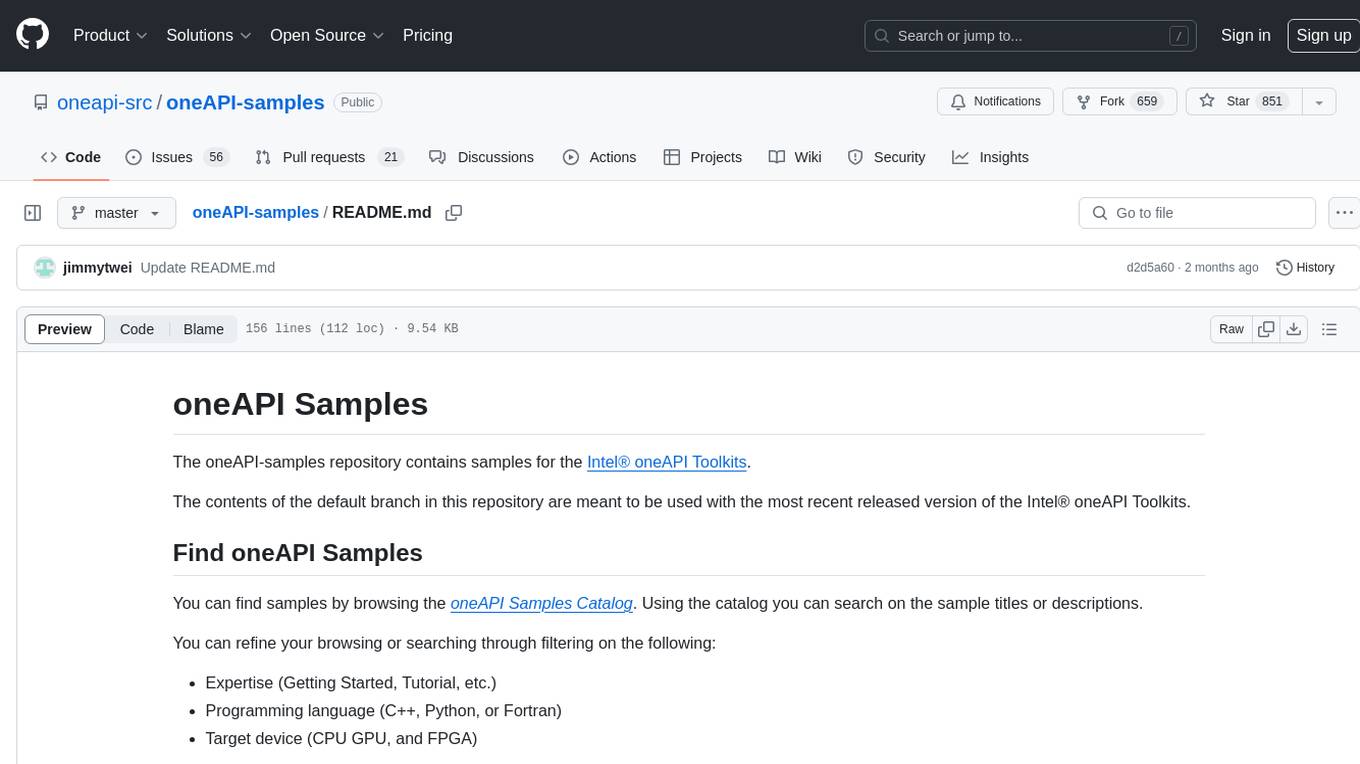
oneAPI-samples
The oneAPI-samples repository contains a collection of samples for the Intel oneAPI Toolkits. These samples cover various topics such as AI and analytics, end-to-end workloads, features and functionality, getting started samples, Jupyter notebooks, direct programming, C++, Fortran, libraries, publications, rendering toolkit, and tools. Users can find samples based on expertise, programming language, and target device. The repository structure is organized by high-level categories, and platform validation includes Ubuntu 22.04, Windows 11, and macOS. The repository provides instructions for getting samples, including cloning the repository or downloading specific tagged versions. Users can also use integrated development environments (IDEs) like Visual Studio Code. The code samples are licensed under the MIT license.
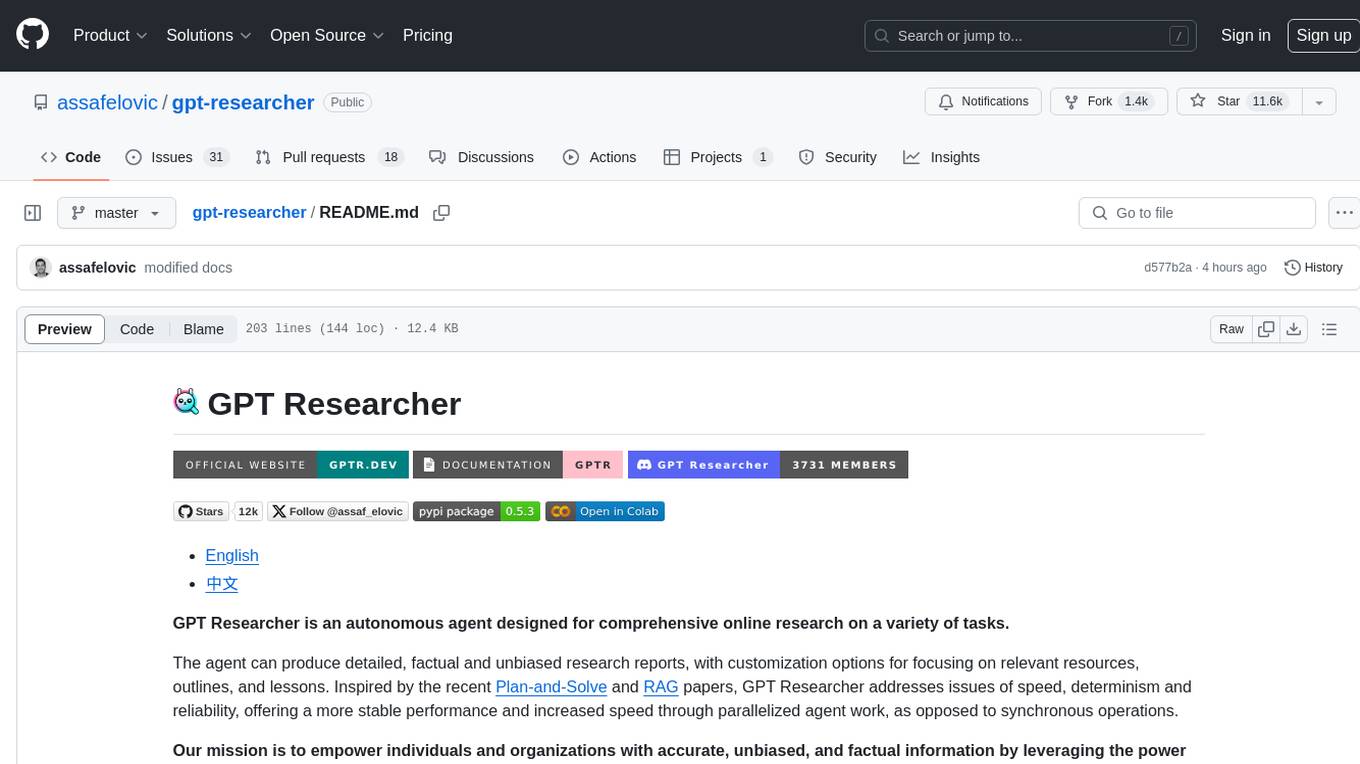
gpt-researcher
GPT Researcher is an autonomous agent designed for comprehensive online research on a variety of tasks. It can produce detailed, factual, and unbiased research reports with customization options. The tool addresses issues of speed, determinism, and reliability by leveraging parallelized agent work. The main idea involves running 'planner' and 'execution' agents to generate research questions, seek related information, and create research reports. GPT Researcher optimizes costs and completes tasks in around 3 minutes. Features include generating long research reports, aggregating web sources, an easy-to-use web interface, scraping web sources, and exporting reports to various formats.
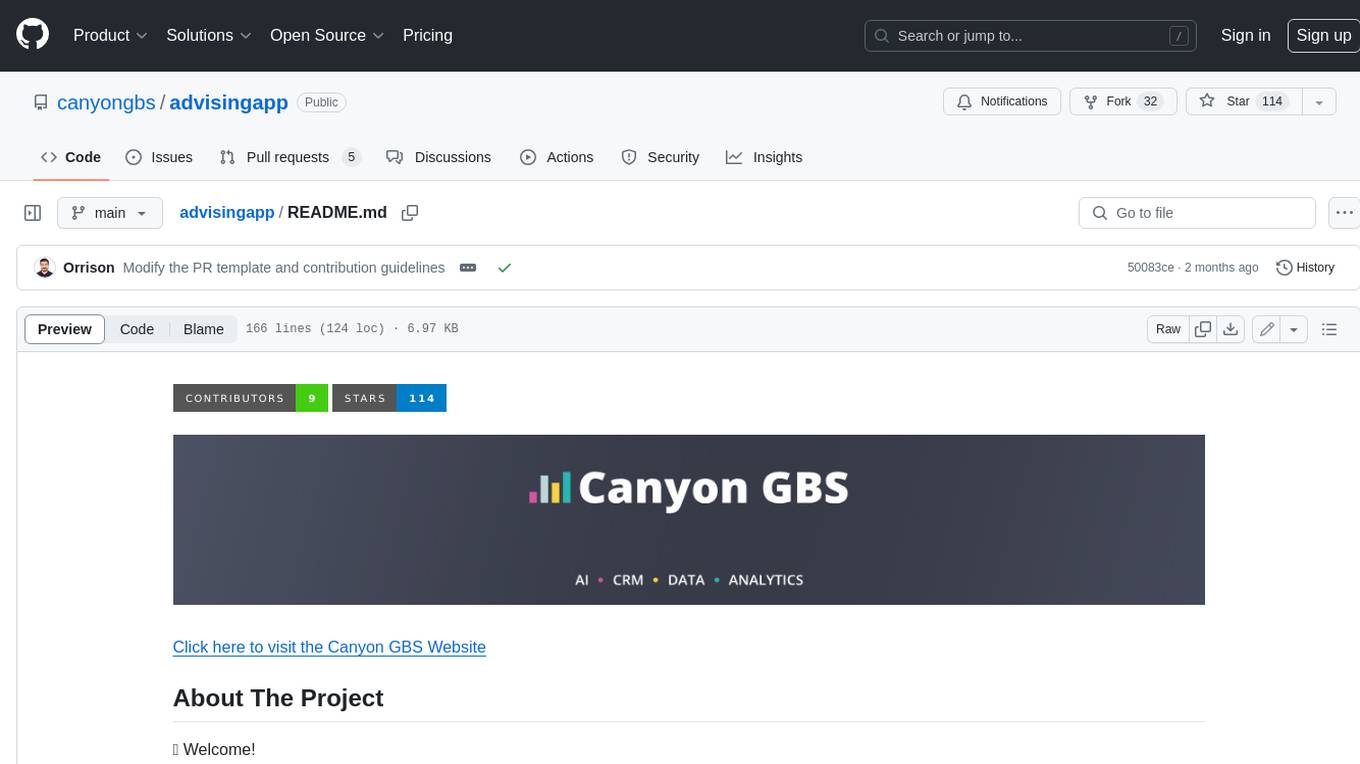
advisingapp
**Advising App™** is a software solution created by Canyon GBS™ that includes a robust personal assistant designed to support student service professionals in their day-to-day roles. The assistant can help with research tasks, draft communication, language translation, content creation, student profile analysis, project planning, ideation, and much more. The software also includes a student service CRM designed to support the management of prospective and enrolled students. Key features of the CRM include record management, email and SMS, service management, caseload management, task management, interaction tracking, files and documents, and much more.
For similar tasks
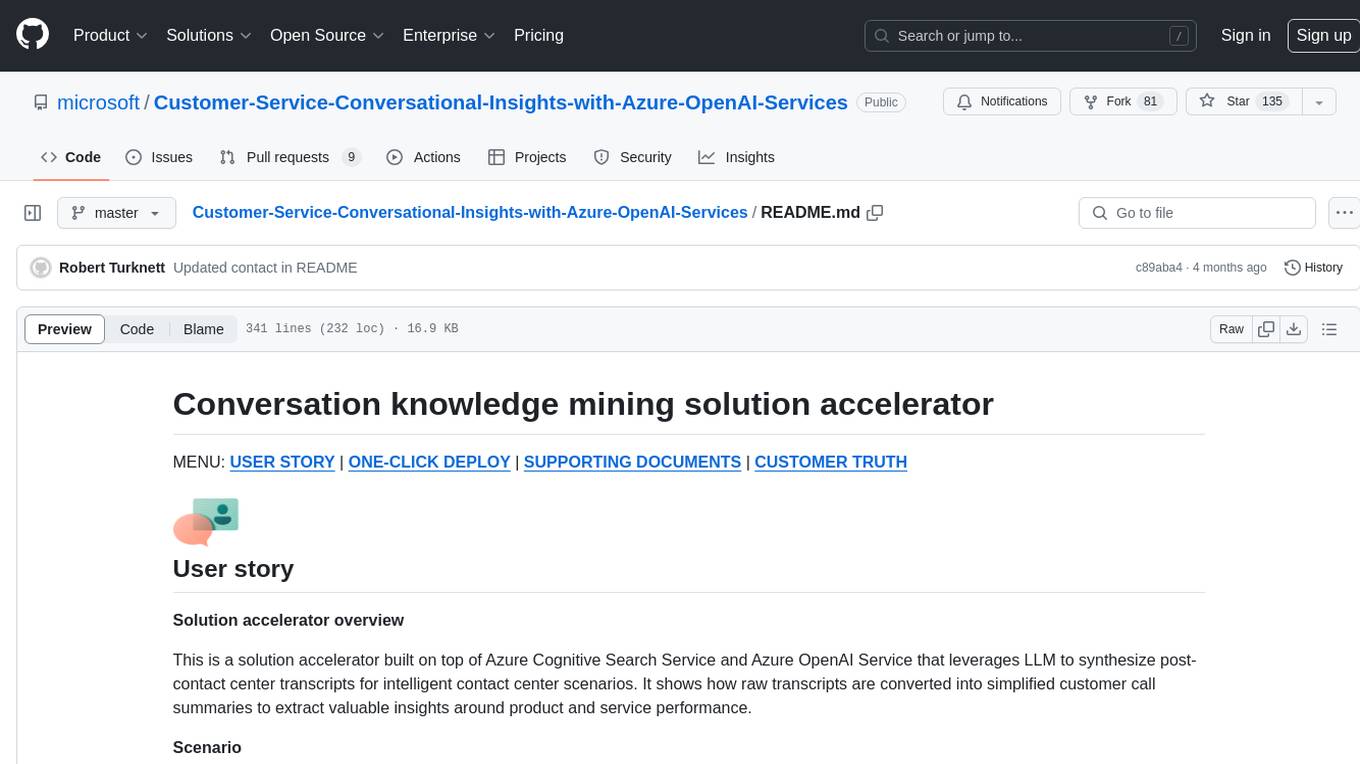
Customer-Service-Conversational-Insights-with-Azure-OpenAI-Services
This solution accelerator is built on Azure Cognitive Search Service and Azure OpenAI Service to synthesize post-contact center transcripts for intelligent contact center scenarios. It converts raw transcripts into customer call summaries to extract insights around product and service performance. Key features include conversation summarization, key phrase extraction, speech-to-text transcription, sensitive information extraction, sentiment analysis, and opinion mining. The tool enables data professionals to quickly analyze call logs for improvement in contact center operations.
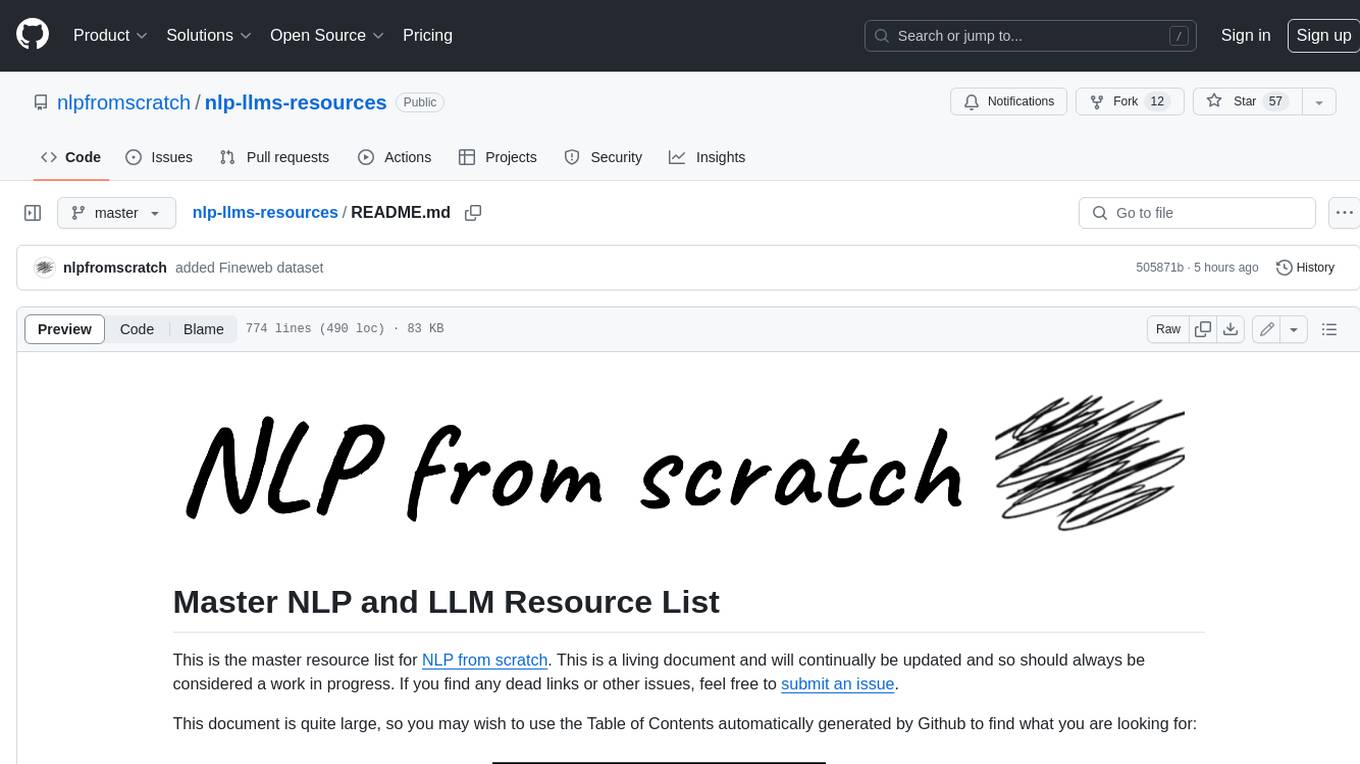
nlp-llms-resources
The 'nlp-llms-resources' repository is a comprehensive resource list for Natural Language Processing (NLP) and Large Language Models (LLMs). It covers a wide range of topics including traditional NLP datasets, data acquisition, libraries for NLP, neural networks, sentiment analysis, optical character recognition, information extraction, semantics, topic modeling, multilingual NLP, domain-specific LLMs, vector databases, ethics, costing, books, courses, surveys, aggregators, newsletters, papers, conferences, and societies. The repository provides valuable information and resources for individuals interested in NLP and LLMs.
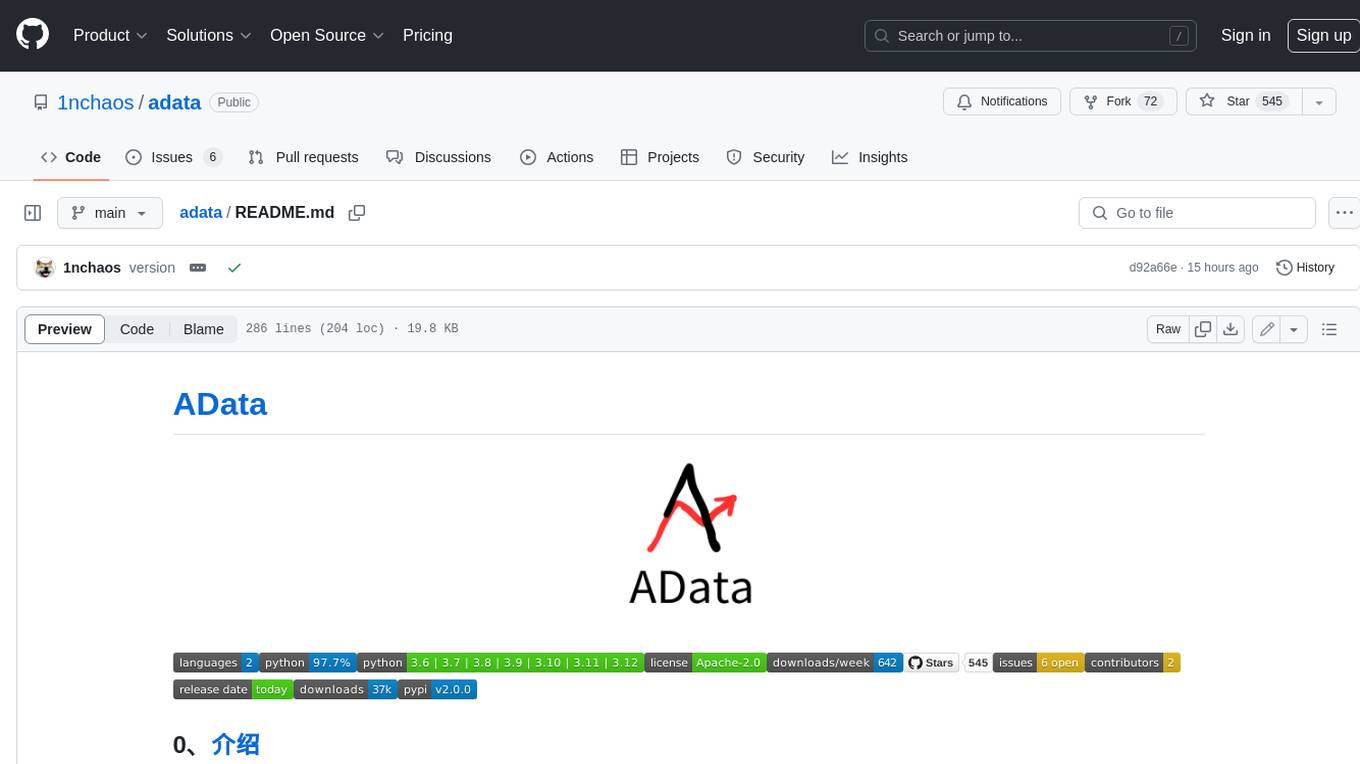
adata
AData is a free and open-source A-share database that focuses on transaction-related data. It provides comprehensive data on stocks, including basic information, market data, and sentiment analysis. AData is designed to be easy to use and integrate with other applications, making it a valuable tool for quantitative trading and AI training.
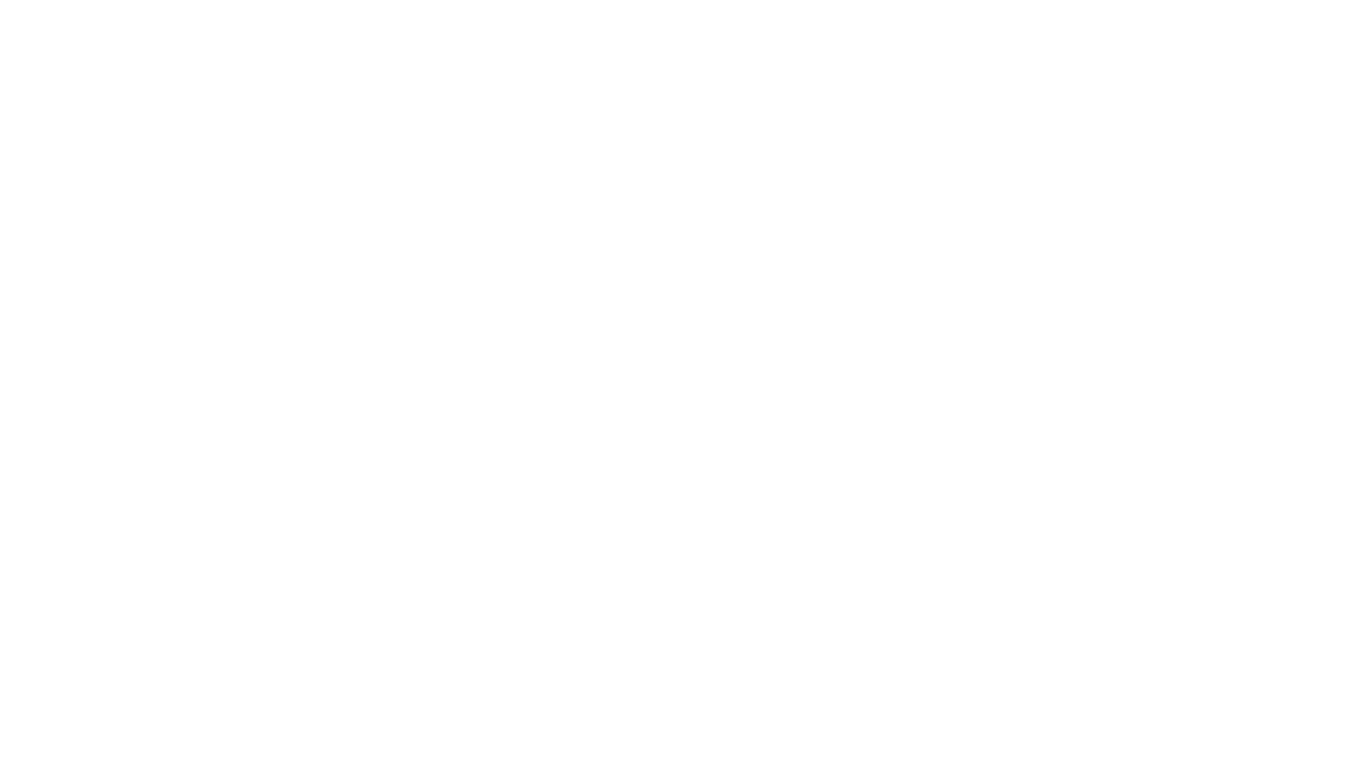
PIXIU
PIXIU is a project designed to support the development, fine-tuning, and evaluation of Large Language Models (LLMs) in the financial domain. It includes components like FinBen, a Financial Language Understanding and Prediction Evaluation Benchmark, FIT, a Financial Instruction Dataset, and FinMA, a Financial Large Language Model. The project provides open resources, multi-task and multi-modal financial data, and diverse financial tasks for training and evaluation. It aims to encourage open research and transparency in the financial NLP field.
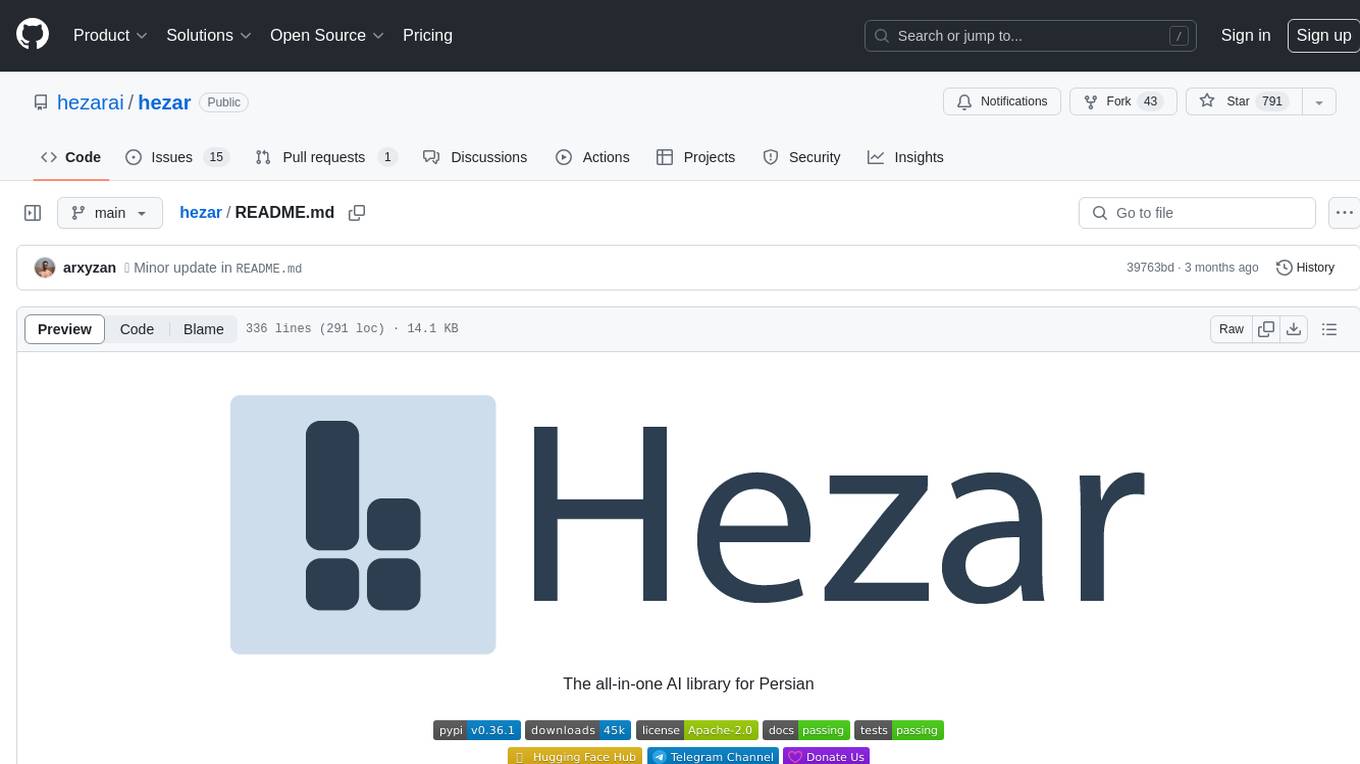
hezar
Hezar is an all-in-one AI library designed specifically for the Persian community. It brings together various AI models and tools, making it easy to use AI with just a few lines of code. The library seamlessly integrates with Hugging Face Hub, offering a developer-friendly interface and task-based model interface. In addition to models, Hezar provides tools like word embeddings, tokenizers, feature extractors, and more. It also includes supplementary ML tools for deployment, benchmarking, and optimization.
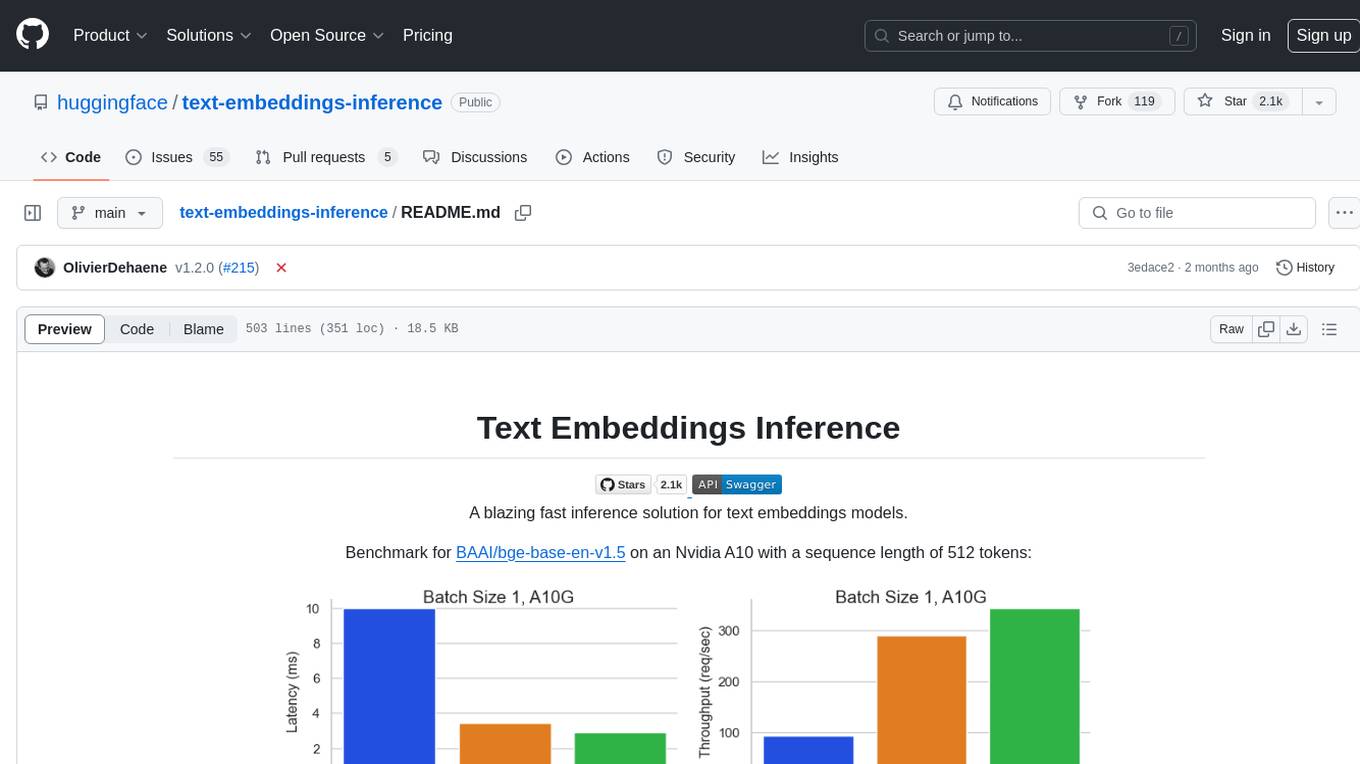
text-embeddings-inference
Text Embeddings Inference (TEI) is a toolkit for deploying and serving open source text embeddings and sequence classification models. TEI enables high-performance extraction for popular models like FlagEmbedding, Ember, GTE, and E5. It implements features such as no model graph compilation step, Metal support for local execution on Macs, small docker images with fast boot times, token-based dynamic batching, optimized transformers code for inference using Flash Attention, Candle, and cuBLASLt, Safetensors weight loading, and production-ready features like distributed tracing with Open Telemetry and Prometheus metrics.
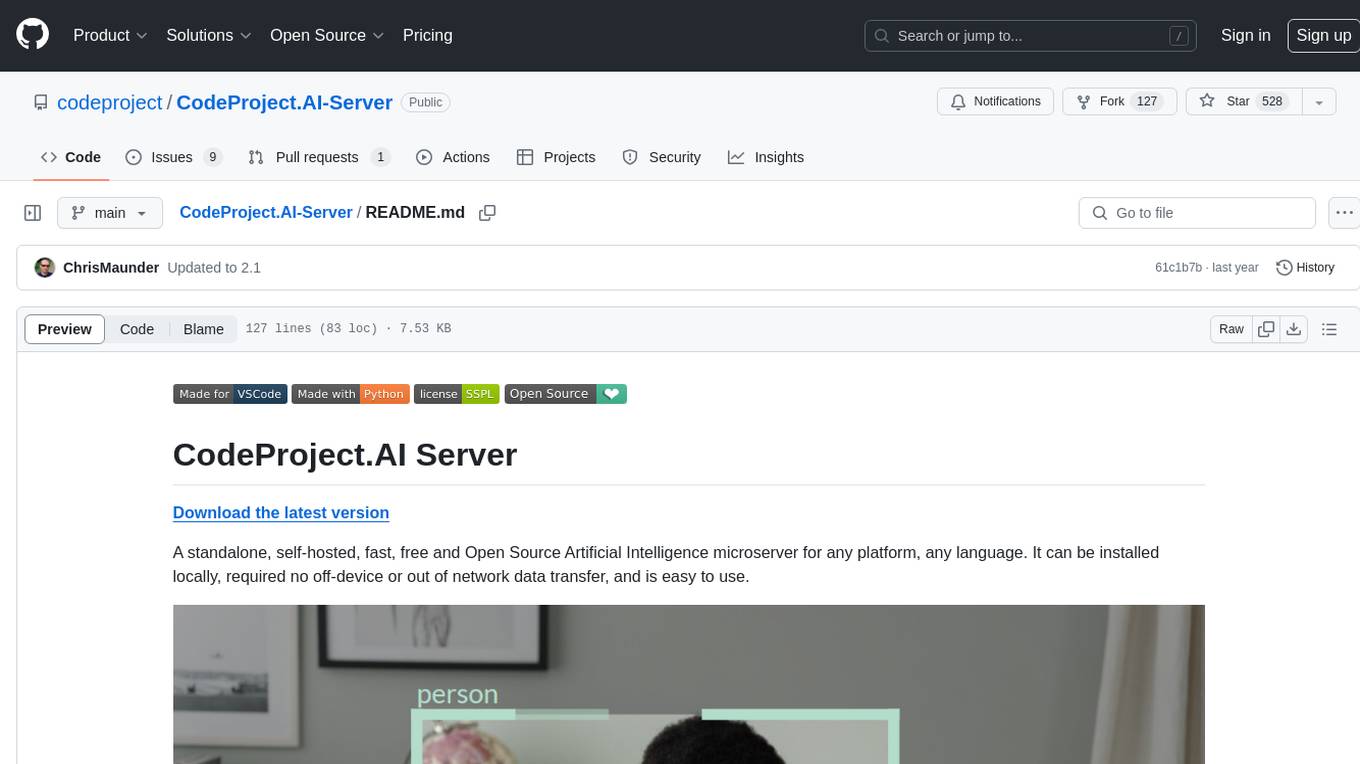
CodeProject.AI-Server
CodeProject.AI Server is a standalone, self-hosted, fast, free, and open-source Artificial Intelligence microserver designed for any platform and language. It can be installed locally without the need for off-device or out-of-network data transfer, providing an easy-to-use solution for developers interested in AI programming. The server includes a HTTP REST API server, backend analysis services, and the source code, enabling users to perform various AI tasks locally without relying on external services or cloud computing. Current capabilities include object detection, face detection, scene recognition, sentiment analysis, and more, with ongoing feature expansions planned. The project aims to promote AI development, simplify AI implementation, focus on core use-cases, and leverage the expertise of the developer community.
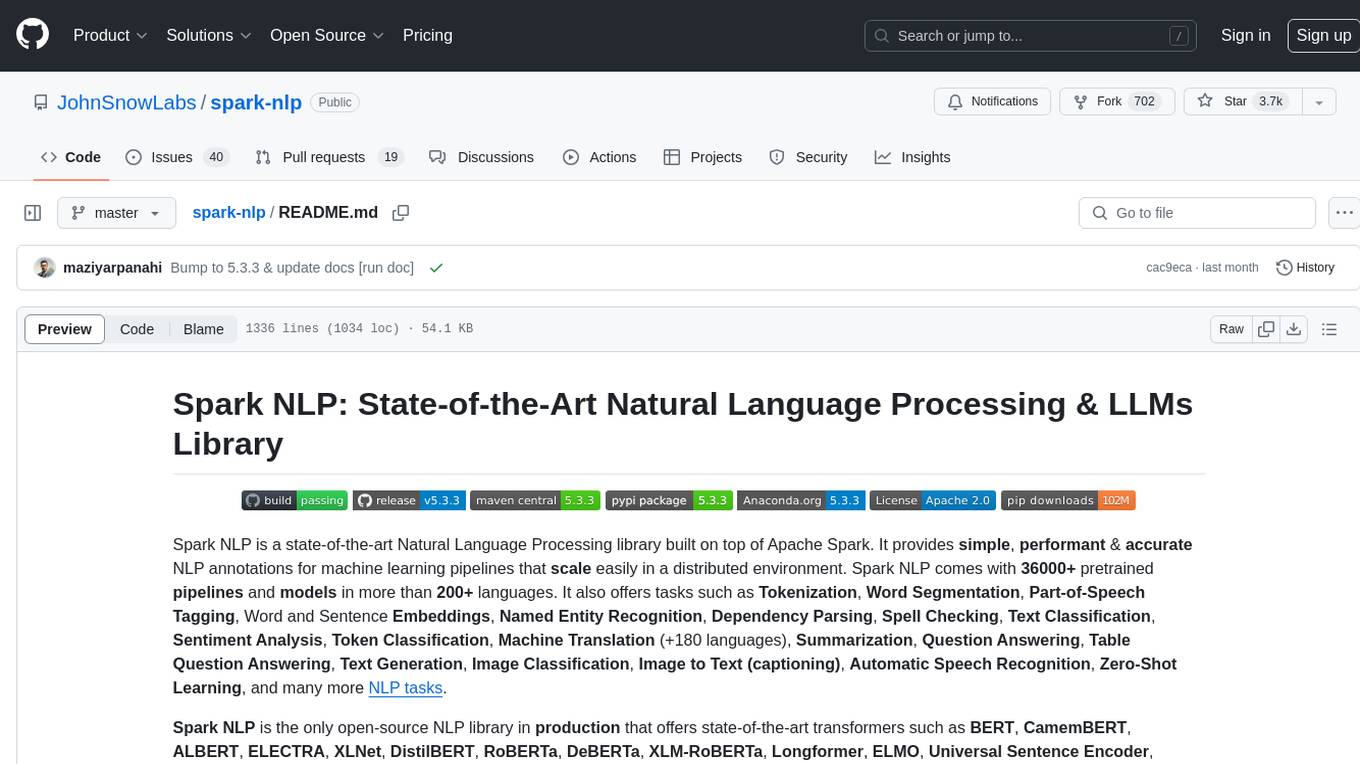
spark-nlp
Spark NLP is a state-of-the-art Natural Language Processing library built on top of Apache Spark. It provides simple, performant, and accurate NLP annotations for machine learning pipelines that scale easily in a distributed environment. Spark NLP comes with 36000+ pretrained pipelines and models in more than 200+ languages. It offers tasks such as Tokenization, Word Segmentation, Part-of-Speech Tagging, Named Entity Recognition, Dependency Parsing, Spell Checking, Text Classification, Sentiment Analysis, Token Classification, Machine Translation, Summarization, Question Answering, Table Question Answering, Text Generation, Image Classification, Image to Text (captioning), Automatic Speech Recognition, Zero-Shot Learning, and many more NLP tasks. Spark NLP is the only open-source NLP library in production that offers state-of-the-art transformers such as BERT, CamemBERT, ALBERT, ELECTRA, XLNet, DistilBERT, RoBERTa, DeBERTa, XLM-RoBERTa, Longformer, ELMO, Universal Sentence Encoder, Llama-2, M2M100, BART, Instructor, E5, Google T5, MarianMT, OpenAI GPT2, Vision Transformers (ViT), OpenAI Whisper, and many more not only to Python and R, but also to JVM ecosystem (Java, Scala, and Kotlin) at scale by extending Apache Spark natively.
For similar jobs
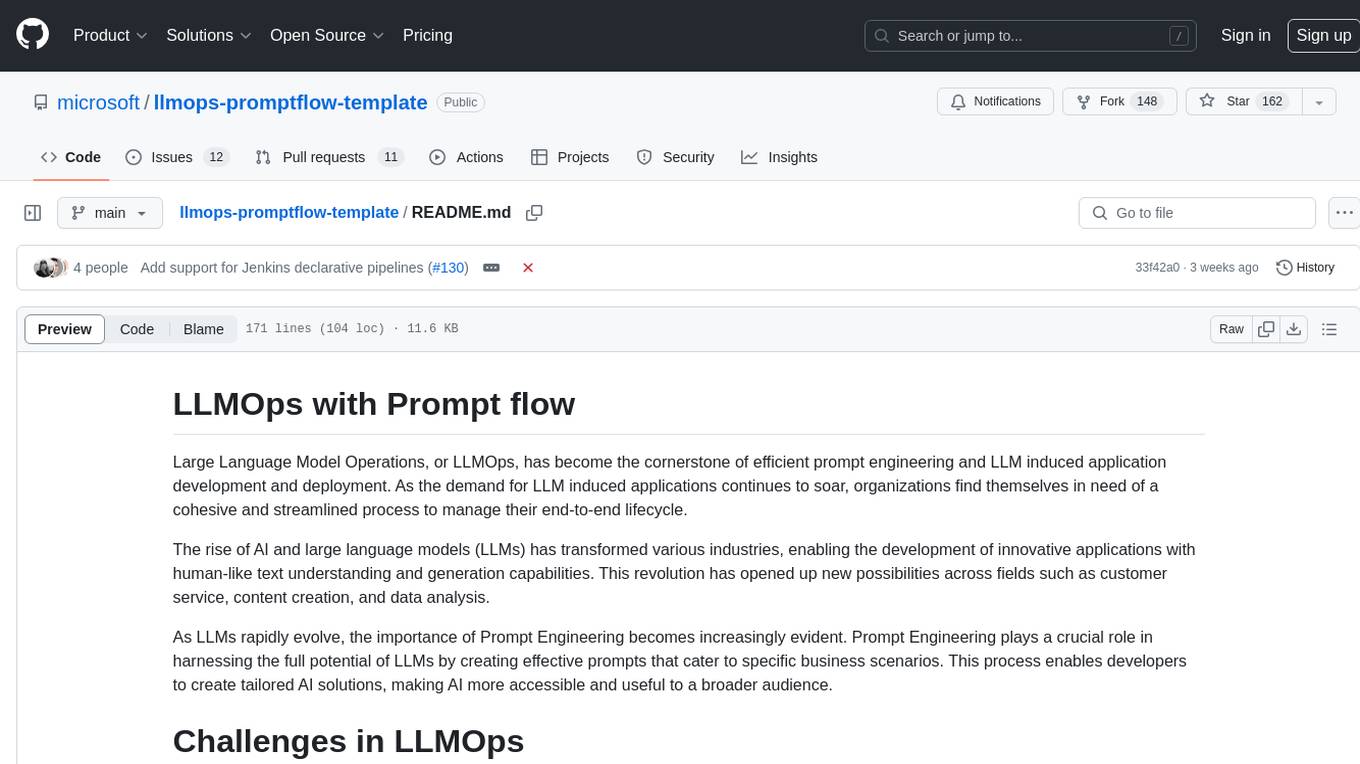
llmops-promptflow-template
LLMOps with Prompt flow is a template and guidance for building LLM-infused apps using Prompt flow. It provides centralized code hosting, lifecycle management, variant and hyperparameter experimentation, A/B deployment, many-to-many dataset/flow relationships, multiple deployment targets, comprehensive reporting, BYOF capabilities, configuration-based development, local prompt experimentation and evaluation, endpoint testing, and optional Human-in-loop validation. The tool is customizable to suit various application needs.
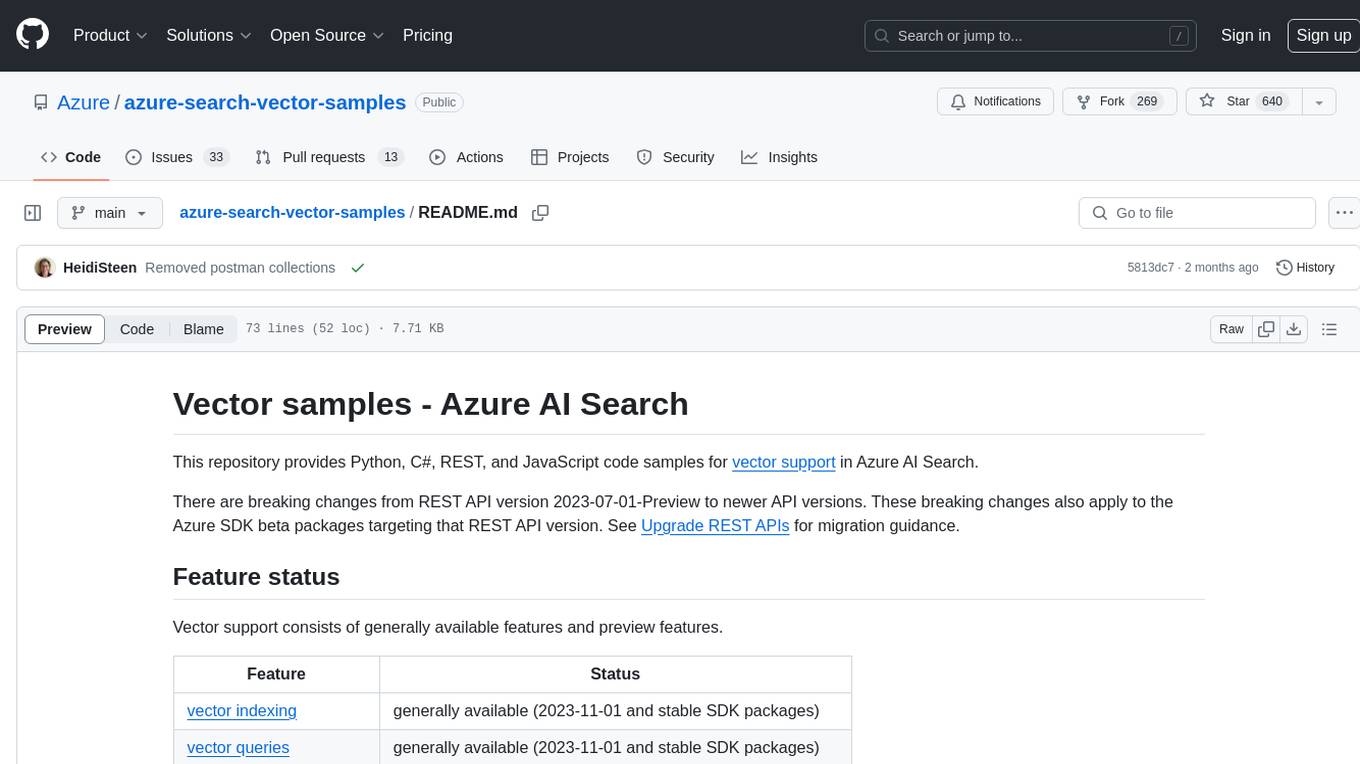
azure-search-vector-samples
This repository provides code samples in Python, C#, REST, and JavaScript for vector support in Azure AI Search. It includes demos for various languages showcasing vectorization of data, creating indexes, and querying vector data. Additionally, it offers tools like Azure AI Search Lab for experimenting with AI-enabled search scenarios in Azure and templates for deploying custom chat-with-your-data solutions. The repository also features documentation on vector search, hybrid search, creating and querying vector indexes, and REST API references for Azure AI Search and Azure OpenAI Service.
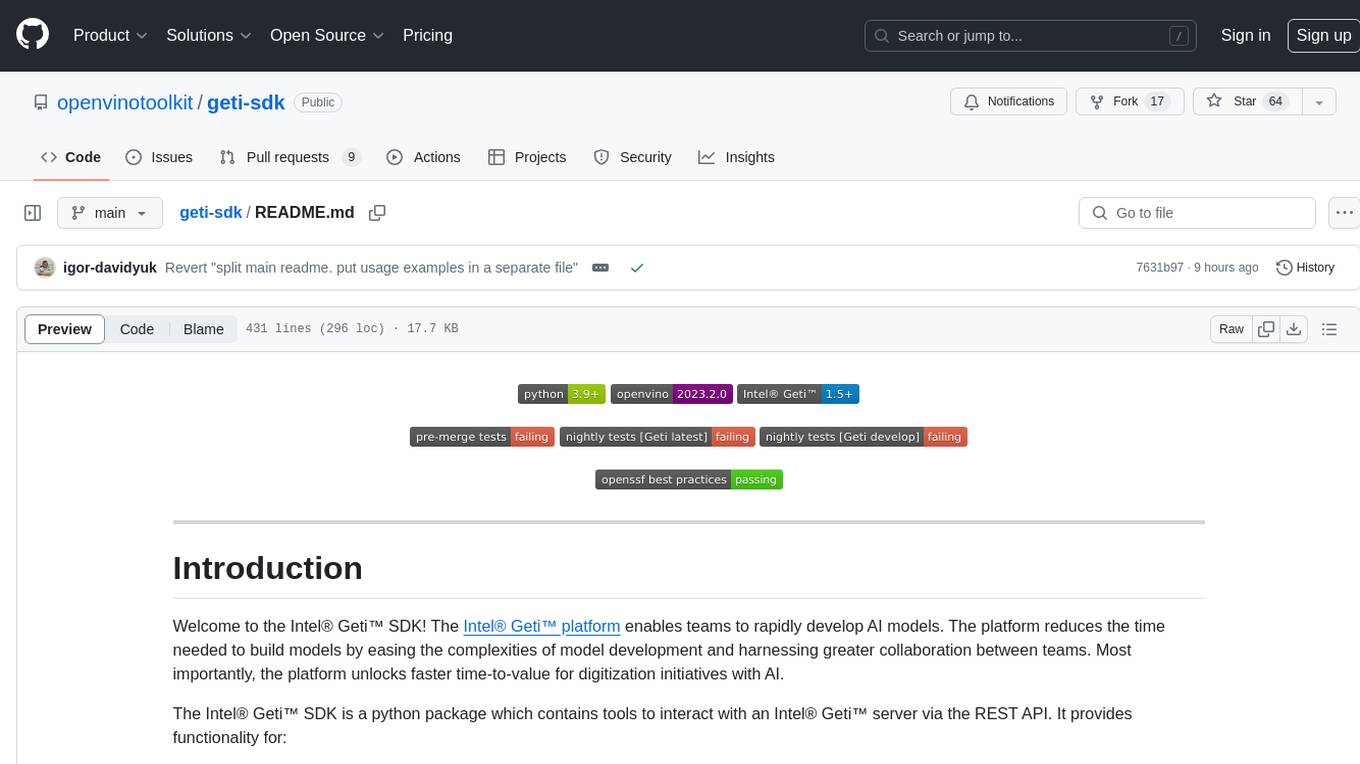
geti-sdk
The Intel® Geti™ SDK is a python package that enables teams to rapidly develop AI models by easing the complexities of model development and enhancing collaboration between teams. It provides tools to interact with an Intel® Geti™ server via the REST API, allowing for project creation, downloading, uploading, deploying for local inference with OpenVINO, setting project and model configuration, launching and monitoring training jobs, and media upload and prediction. The SDK also includes tutorial-style Jupyter notebooks demonstrating its usage.
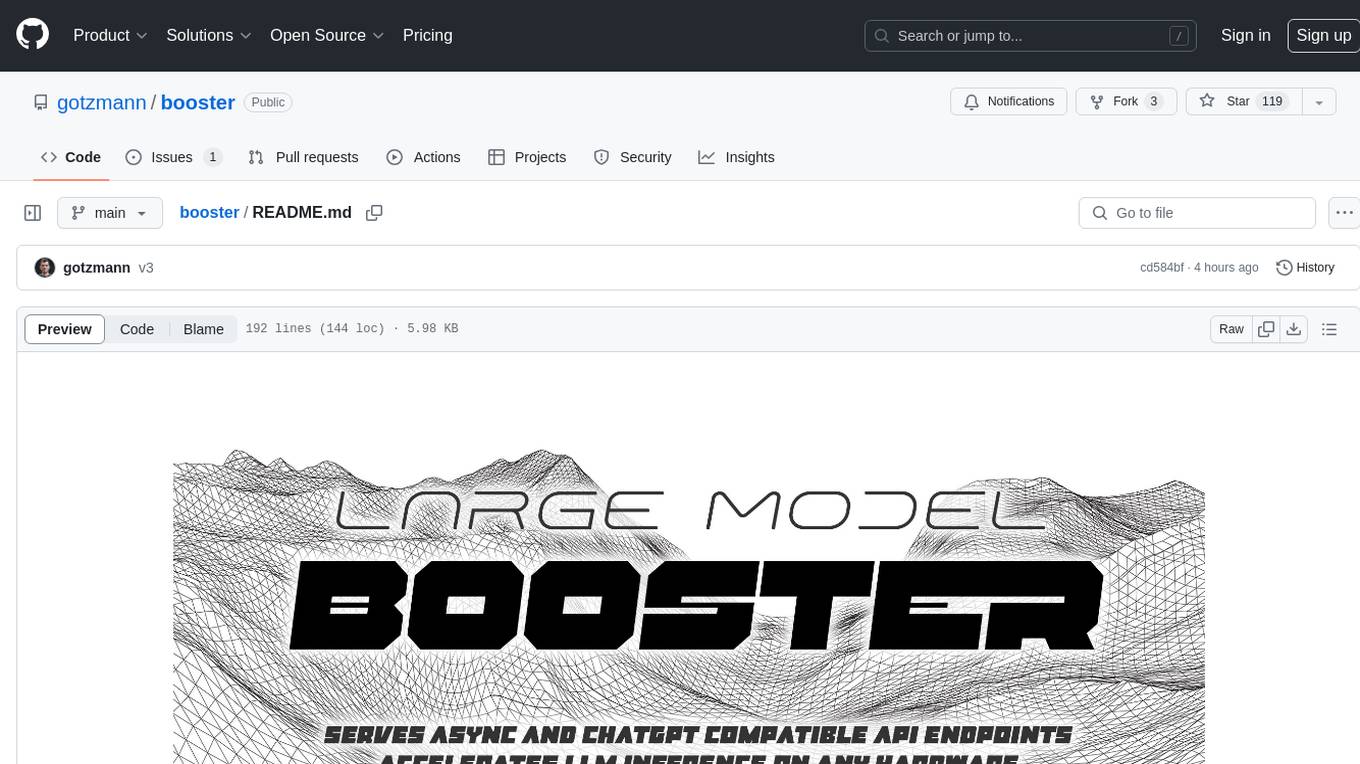
booster
Booster is a powerful inference accelerator designed for scaling large language models within production environments or for experimental purposes. It is built with performance and scaling in mind, supporting various CPUs and GPUs, including Nvidia CUDA, Apple Metal, and OpenCL cards. The tool can split large models across multiple GPUs, offering fast inference on machines with beefy GPUs. It supports both regular FP16/FP32 models and quantised versions, along with popular LLM architectures. Additionally, Booster features proprietary Janus Sampling for code generation and non-English languages.
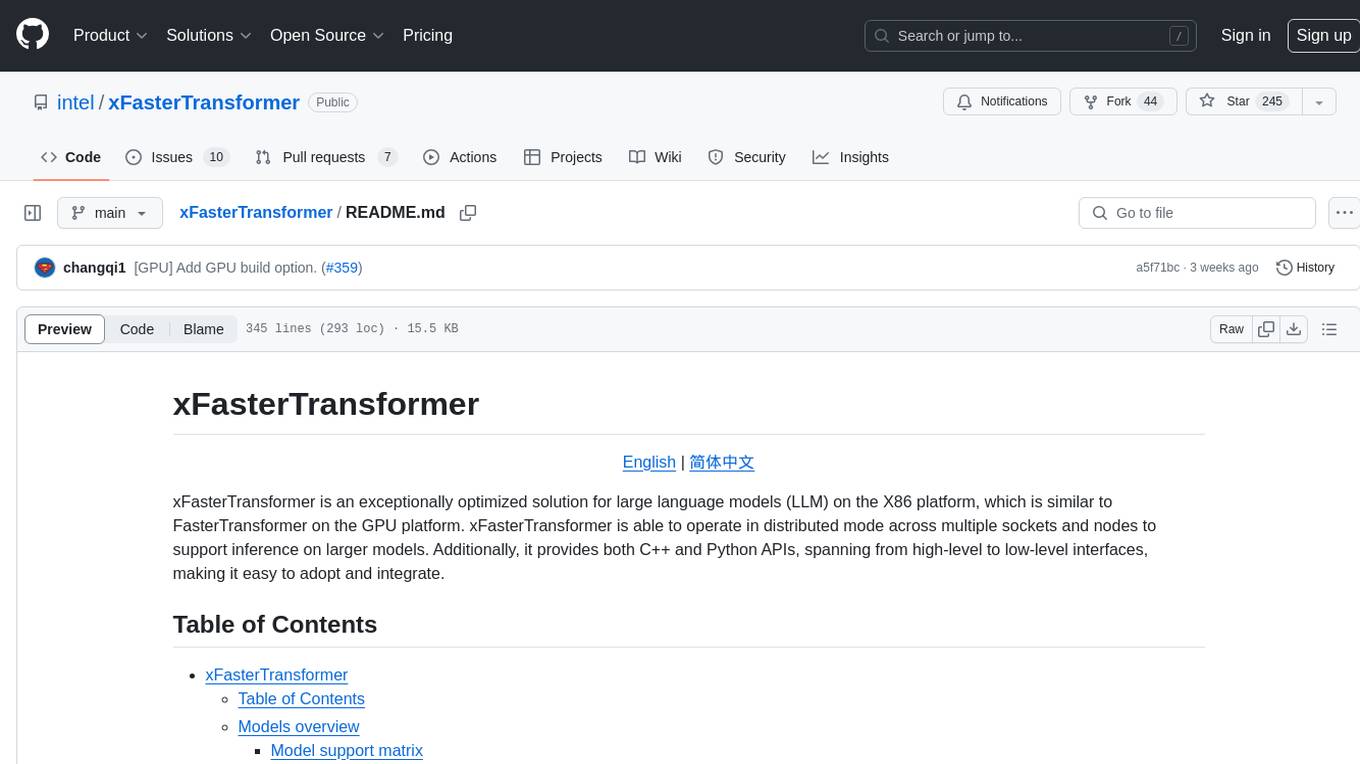
xFasterTransformer
xFasterTransformer is an optimized solution for Large Language Models (LLMs) on the X86 platform, providing high performance and scalability for inference on mainstream LLM models. It offers C++ and Python APIs for easy integration, along with example codes and benchmark scripts. Users can prepare models in a different format, convert them, and use the APIs for tasks like encoding input prompts, generating token ids, and serving inference requests. The tool supports various data types and models, and can run in single or multi-rank modes using MPI. A web demo based on Gradio is available for popular LLM models like ChatGLM and Llama2. Benchmark scripts help evaluate model inference performance quickly, and MLServer enables serving with REST and gRPC interfaces.
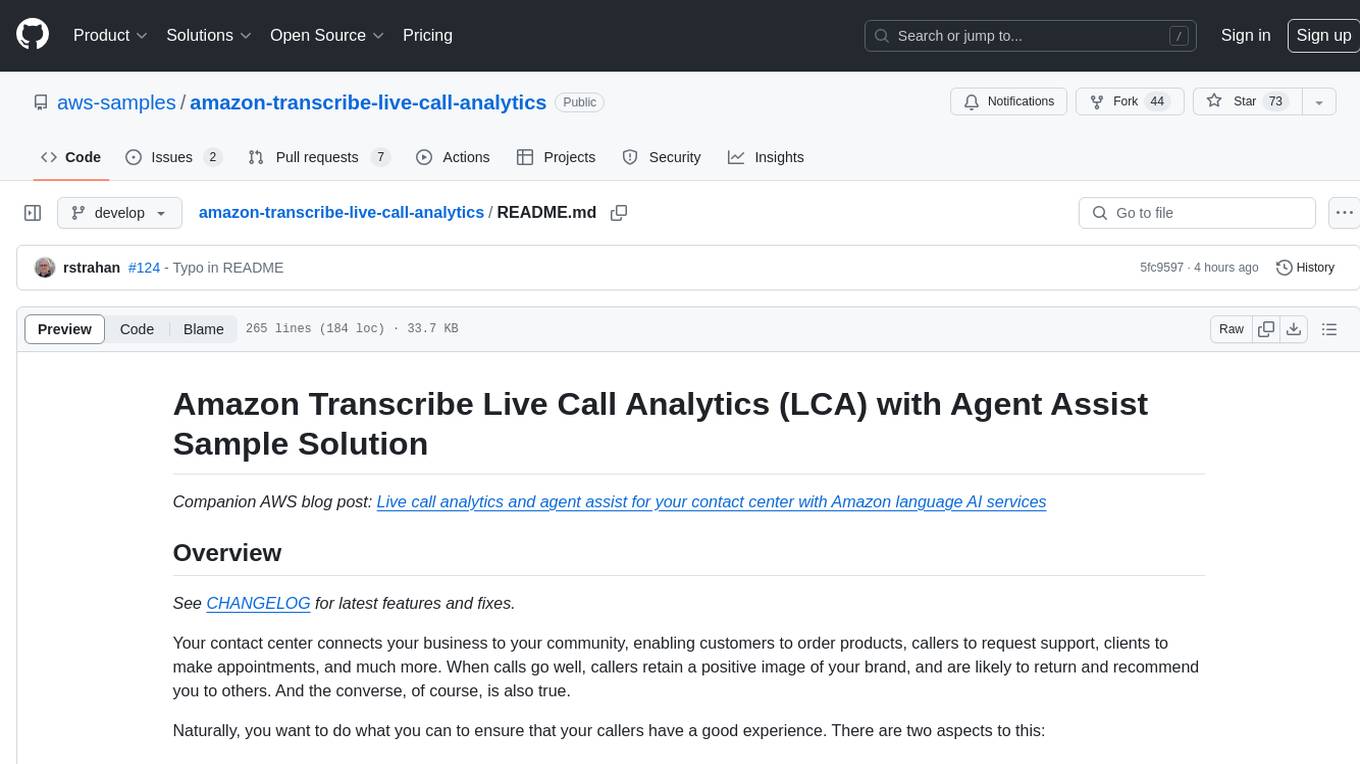
amazon-transcribe-live-call-analytics
The Amazon Transcribe Live Call Analytics (LCA) with Agent Assist Sample Solution is designed to help contact centers assess and optimize caller experiences in real time. It leverages Amazon machine learning services like Amazon Transcribe, Amazon Comprehend, and Amazon SageMaker to transcribe and extract insights from contact center audio. The solution provides real-time supervisor and agent assist features, integrates with existing contact centers, and offers a scalable, cost-effective approach to improve customer interactions. The end-to-end architecture includes features like live call transcription, call summarization, AI-powered agent assistance, and real-time analytics. The solution is event-driven, ensuring low latency and seamless processing flow from ingested speech to live webpage updates.
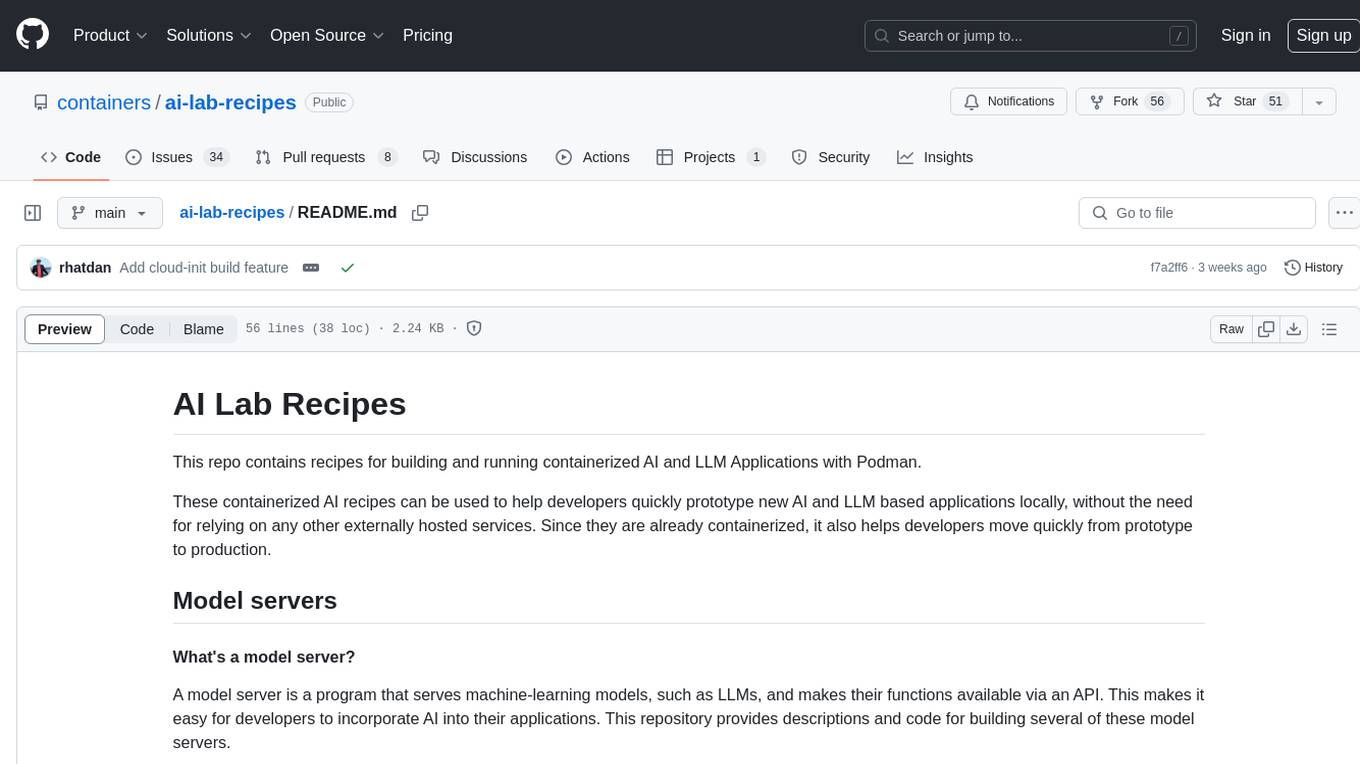
ai-lab-recipes
This repository contains recipes for building and running containerized AI and LLM applications with Podman. It provides model servers that serve machine-learning models via an API, allowing developers to quickly prototype new AI applications locally. The recipes include components like model servers and AI applications for tasks such as chat, summarization, object detection, etc. Images for sample applications and models are available in `quay.io`, and bootable containers for AI training on Linux OS are enabled.
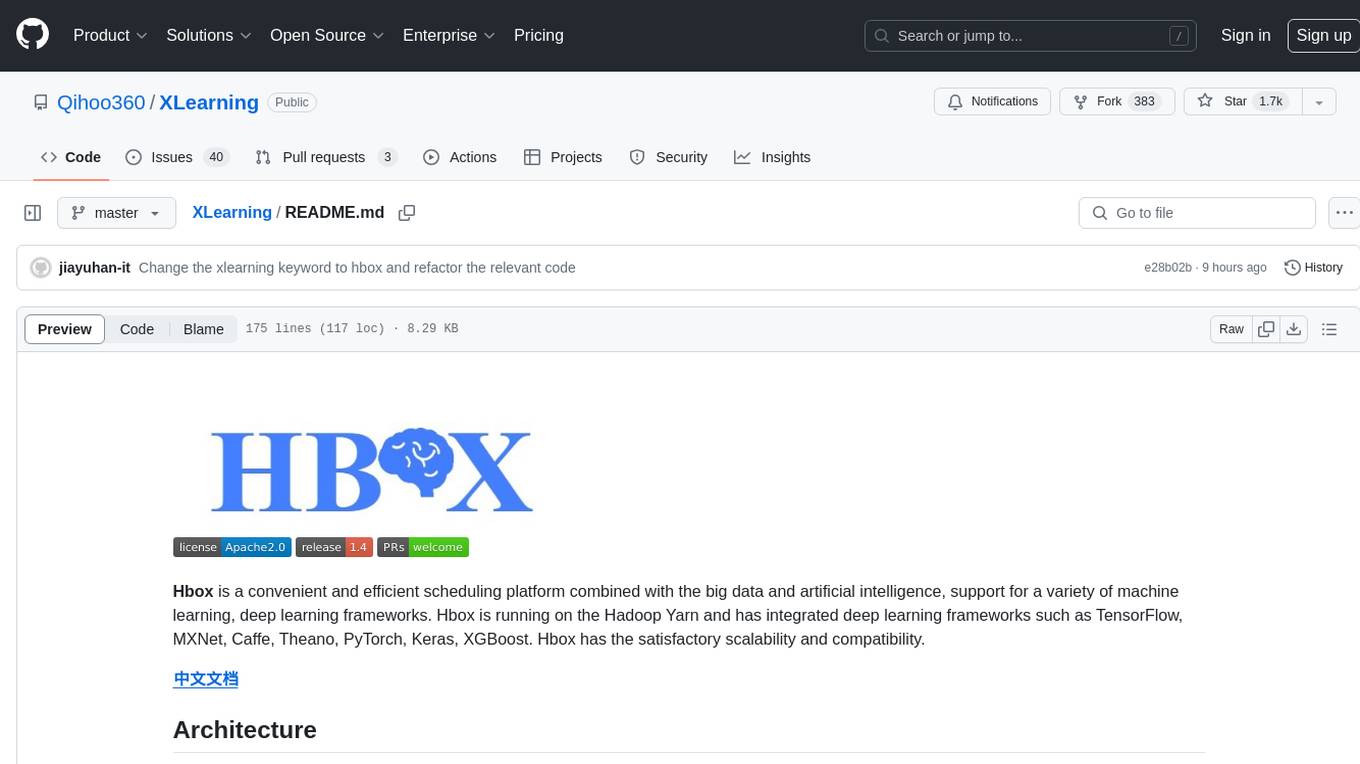
XLearning
XLearning is a scheduling platform for big data and artificial intelligence, supporting various machine learning and deep learning frameworks. It runs on Hadoop Yarn and integrates frameworks like TensorFlow, MXNet, Caffe, Theano, PyTorch, Keras, XGBoost. XLearning offers scalability, compatibility, multiple deep learning framework support, unified data management based on HDFS, visualization display, and compatibility with code at native frameworks. It provides functions for data input/output strategies, container management, TensorBoard service, and resource usage metrics display. XLearning requires JDK >= 1.7 and Maven >= 3.3 for compilation, and deployment on CentOS 7.2 with Java >= 1.7 and Hadoop 2.6, 2.7, 2.8.